User login
Severe Sepsis
Severe sepsis and septic shock are associated with excess mortality when inappropriate initial antimicrobial therapy, defined as an antimicrobial regimen that lacks in vitro activity against the isolated organism(s) responsible for the infection, is administered.14 Unfortunately, bacterial resistance to antibiotics is increasing and creates a therapeutic challenge for clinicians when treating patients with serious infections, such as severe sepsis. Increasing rates of bacterial resistance leads many clinicians to empirically treat critically ill patients with broad‐spectrum antibiotics, which can perpetuate the cycle of increasing resistance.5, 6 Conversely, inappropriate initial antimicrobial therapy can lead to treatment failures and adverse patient outcomes.7 Individuals with severe sepsis appear to be at particularly high risk of excess mortality when inappropriate initial antimicrobial therapy is administered.8, 9
The most recent Surviving Sepsis Guidelines recommend empiric combination therapy targeting Gram‐negative bacteria, particularly for patients with known or suspected Pseudomonas infections, as a means to decrease the likelihood of administering inappropriate initial antimicrobial therapy.10 However, the selection of an antimicrobial regimen that is active against the causative pathogen(s) is problematic, as the treating physician usually does not know the susceptibilities of the pathogen(s) for the selected empiric antibiotics. Therefore, we performed a study with the main goal of determining whether resistance to the initially prescribed antimicrobial regimen was associated with clinical outcome in patients with severe sepsis attributed to Gram‐negative bacteremia.
Materials and Methods
Study Location and Patients
This study was conducted at a university‐affiliated, urban teaching hospital: Barnes‐Jewish Hospital (1200 beds). During a 6‐year period (January 2002 to December 2007), all hospitalized patients with a positive blood culture for Gram‐negative bacteria, with antimicrobial susceptibility testing performed for the blood isolate(s), were eligible for this investigation. This study was approved by the Washington University School of Medicine Human Studies Committee.
Study Design and Data Collection
A retrospective cohort study design was employed. Two investigators (J.A.D., R.M.R.) identified potential study patients by the presence of a positive blood culture for Pseudomonas aeruginosa, Acinetobacter species, or Enterobacteriaceae (Escherichia coli, Klebsiella species, Enterobacter species) combined with primary or secondary International Classification of Diseases (ICD‐9‐CM) codes indicative of acute organ dysfunction, at least two criteria from the systemic inflammatory response syndrome (SIRS),10 and initial antibiotic treatment with either cefepime, piperacillin‐tazobactam, or a carbapenem (imipenem or meropenem). These antimicrobials represent the primary agents employed for the treatment of Gram‐negative infections at Barnes‐Jewish Hospital during the study period, and had to be administered within 12 hours of having the subsequently positive blood cultures drawn. Based on the initial study database construction, 3 investigators (E.C.W., J.K., M.P.) merged patient‐specific data from the automated hospital medical records, microbiology database, and pharmacy database of Barnes‐Jewish Hospital to complete the clinical database under the auspices of the definitions described below.
The baseline characteristics collected by the study investigators included: age, gender, race, the presence of congestive heart failure, chronic obstructive pulmonary disease, diabetes mellitus, chronic liver disease, underlying malignancy, and end‐stage renal disease requiring renal replacement therapy. All cause hospital mortality was evaluated as the primary outcome variable. Secondary outcomes included acquired organ dysfunction and hospital length of stay. The Acute Physiology and Chronic Health Evaluation (APACHE) II11 and Charlson co‐morbidity scores were also calculated during the 24 hours after the positive blood cultures were drawn. This was done because we included patients with community‐acquired infections who only had clinical data available after blood cultures were drawn.
Definitions
All definitions were selected prospectively as part of the original study design. Cases of Gram‐negative bacteremia were classified into mutually exclusive groups comprised of either community‐acquired or healthcare‐associated infection. Patients with healthcare‐associated bacteremia were categorized as community‐onset or hospital‐onset, as previously described.12 In brief, patients with healthcare‐associated community‐onset bacteremia had the positive culture obtained within the first 48 hours of hospital admission in combination with one or more of the following risk factors: (1) residence in a nursing home, rehabilitation hospital, or other long‐term nursing facility; (2) previous hospitalization within the immediately preceding 12 months; (3) receiving outpatient hemodialysis, peritoneal dialysis, wound care, or infusion therapy necessitating regular visits to a hospital‐based clinic; and (4) having an immune‐compromised state. Patients were classified as having healthcare‐associated hospital‐onset bacteremia when the culture was obtained 48 hours or more after admission. Community‐acquired bacteremia occurred in patients without healthcare risk factors and a positive blood culture within the first 48 hours of admission. Prior antibiotic exposure was defined as having occurred within the previous 30 days from the onset of severe sepsis.
To be included in the analysis, patients had to meet criteria for severe sepsis based on discharge ICD‐9‐CM codes for acute organ dysfunction, as previously described.13 The organs of interest included the heart, lungs, kidneys, bone marrow (hematologic), brain, and liver. Patients were classified as having septic shock if vasopressors (norepinephrine, dopamine, epinephrine, phenylephrine, or vasopressin) were initiated within 24 hours of the blood culture collection date and time. Empiric antimicrobial treatment was classified as being appropriate if the initially prescribed antibiotic regimen was active against the identified pathogen(s) based on in vitro susceptibility testing and administered within 12 hours following blood culture collection. Appropriate antimicrobial treatment also had to be prescribed for at least 24 hours. However, the total duration of antimicrobial therapy was at the discretion of the treating physicians. The Charlson co‐morbidity score was calculated using ICD‐9‐CM codes abstracted from the index hospitalization employing MS‐DRG Grouper version 26.
Antimicrobial Monitoring
From January 2002 through the present, Barnes‐Jewish Hospital utilized an antibiotic control program to help guide antimicrobial therapy. During this time, the use of cefepime and gentamicin was unrestricted. However, initiation of intravenous ciprofloxacin, imipenem/cilastatin, meropenem, or piperacillin/tazobactam was restricted and required preauthorization from either a clinical pharmacist or infectious diseases physician. Each intensive care unit (ICU) had a clinical pharmacist who reviewed all antibiotic orders to insure that dosing and interval of antibiotic administration was adequate for individual patients based on body size, renal function, and the resuscitation status of the patient. After daytime hours, the on‐call clinical pharmacist reviewed and approved the antibiotic orders. The initial antibiotic dosages for the antibiotics employed for the treatment of Gram‐negative infections at Barnes‐Jewish Hospital were as follows: cefepime, 1 to 2 grams every eight hours; pipercillin‐tazobactam, 4.5 grams every six hours; imipenem, 0.5 grams every six hours; meropenem, 1 gram every eight hours; ciprofloxacin, 400 mg every eight hours; gentamicin, 5 mg/kg once daily.
Starting in June 2005, a sepsis order set was implemented in the emergency department, general medical wards, and the intensive care units with the intent of standardizing empiric antibiotic selection for patients with sepsis based on the infection type (ie, community‐acquired pneumonia, healthcare‐associated pneumonia, intra‐abdominal infection, etc) and the hospital's antibiogram.14, 15 However, antimicrobial selection, dosing, and de‐escalation of therapy were still optimized by clinical pharmacists in these clinical areas.
Antimicrobial Susceptibility Testing
The microbiology laboratory performed antimicrobial susceptibility testing of the Gram‐negative blood isolates using the disk diffusion method according to guidelines and breakpoints established by the Clinical Laboratory and Standards Institute (CLSI) and published during the inclusive years of the study.16, 17 Zone diameters obtained by disk diffusion testing were converted to minimum inhibitory concentrations (MICs in mg/L) by linear regression analysis for each antimicrobial agent using the BIOMIC V3 antimicrobial susceptibility system (Giles Scientific, Inc., Santa Barbara, CA). Linear regression algorithms contained in the software of this system were determined by comparative studies correlating microbroth dilution‐determined MIC values with zone sizes obtained by disk diffusion testing.18
Data Analysis
Continuous variables were reported as mean the standard deviation, or median and quartiles. The Student's t test was used when comparing normally distributed data, and the MannWhitney U test was employed to analyze nonnormally distributed data. Categorical data were expressed as frequency distributions and the Chi‐squared test was used to determine if differences existed between groups. We performed multiple logistic regression analysis to identify clinical risk factors that were associated with hospital mortality (SPSS, Inc., Chicago, IL). All risk factors from Table 1, as well as the individual pathogens examined, were included in the corresponding multivariable analysis with the exception of acquired organ dysfunction (considered a secondary outcome). All tests were two‐tailed, and a P value <0.05 was determined to represent statistical significance.
Variable | Hospital Survivors (n = 302) | Hospital Nonsurvivors (n = 233) | P value |
---|---|---|---|
| |||
Age, years | 57.9 16.2 | 60.3 15.8 | 0.091 |
Male | 156 (51.7) | 132 (56.7) | 0.250 |
Infection onset source | |||
Community‐acquired | 31 (10.3) | 15 (6.4) | 0.005 |
Healthcare‐associated community‐onset | 119 (39.4) | 68 (29.2) | |
Healthcare‐associated hospital‐onset | 152 (50.3) | 150 (64.4) | |
Underlying co‐morbidities | |||
CHF | 43 (14.2) | 53 (22.7) | 0.011 |
COPD | 42 (13.9) | 56 (24.0) | 0.003 |
Chronic kidney disease | 31 (10.3) | 41 (17.6) | 0.014 |
Liver disease | 34 (11.3) | 31 (13.3) | 0.473 |
Active malignancy | 100 (33.1) | 83 (35.6) | 0.544 |
Diabetes | 68 (22.5) | 50 (21.5) | 0.770 |
Charlson co‐morbidity score | 4.5 3.5 | 5.2 3.9 | 0.041 |
APACHE II score | 21.8 6.1 | 27.1 6.2 | <0.001 |
ICU admission | 221 (73.2) | 216 (92.7) | <0.001 |
Vasopressors | 137 (45.4) | 197 (84.5) | <0.001 |
Mechanical ventilation | 124 (41.1) | 183 (78.5) | <0.001 |
Drotrecogin alfa (activated) | 6 (2.0) | 21 (9.0) | <0.001 |
Dysfunctional acquired organ systems | |||
Cardiovascular | 149 (49.3) | 204 (87.6) | <0.001 |
Respiratory | 141 (46.7) | 202 (86.7) | <0.001 |
Renal | 145 (48.0) | 136 (58.4) | 0.017 |
Hepatic | 13 (4.3) | 27 (11.6) | 0.001 |
Hematologic | 103 (34.1) | 63 (27.0) | 0.080 |
Neurologic | 11 (3.6) | 19 (8.2) | 0.024 |
2 Dysfunctional acquired organ systems | 164 (54.3) | 213 (91.4) | <0.001 |
Source of bloodstream infection | |||
Lungs | 95 (31.5) | 127 (54.5) | <0.001 |
Urinary tract | 92 (30.5) | 45 (19.3) | |
Central venous catheter | 30 (9.9) | 16 (6.9) | |
Intra‐abdominal | 63 (20.9) | 33 (14.2) | |
Unknown | 22 (7.3) | 12 (5.2) | |
Prior antibiotics* | 103 (34.1) | 110 (47.2) | 0.002 |
Results
Patient Characteristics
Included in the study were 535 consecutive patients with severe sepsis attributed to Pseudomonas aeruginosa, Acinetobacter species, or Enterobacteriaceae bacteremia, of whom 233 (43.6%) died during their hospitalization. The mean age was 58.9 16.0 years (range, 18 to 96 years) with 288 (53.8%) males and 247 (46.2%) females. The infection sources included community‐acquired (n = 46, 8.6%), healthcare‐associated community‐onset (n = 187, 35.0%), and healthcare‐associated hospital‐onset (n = 302, 56.4%). Hospital nonsurvivors were statistically more likely to have a healthcare‐associated hospital‐onset infection, congestive heart failure, chronic obstructive pulmonary disease, chronic kidney disease, ICU admission, need for mechanical ventilation and/or vasopressors, administration of drotrecogin alfa (activated), prior antibiotic administration, the lungs as the source of infection, acquired dysfunction of the cardiovascular, respiratory, renal, hepatic, and neurologic organ systems, and greater APACHE II and Charlson co‐morbidity scores compared to hospital survivors (Table 1). Hospital nonsurvivors were also statistically less likely to have a healthcare‐associated community‐onset infection and a urinary source of infection compared to hospital survivors (Table 1).
Microbiology
Among the 547 Gram‐negative bacteria isolated from blood, the most common were Enterobacteriaceae (Escherichia coli, Klebsiella species, Enterobacter species) (70.2%) followed by Pseudomonas aeruginosa (20.8%) and Acinetobacter species (9.0%) (Table 2). Nine patients had two different Enterobacteriaceae species isolated from their blood cultures, and three patients had an Enterobacteriaceae species and Pseudomonas aeruginosa isolated from their blood cultures. Hospital nonsurvivors were statistically more likely to be infected with Pseudomonas aeruginosa and less likely to be infected with Enterobacteriaceae. The pathogen‐specific hospital mortality rate was significantly greater for Pseudomonas aeruginosa and Acinetobacter species compared to Enterobacteriaceae (P < 0.001 and P = 0.008, respectively).
Bacteria | Hospital Survivors (n = 302) | Hospital Nonsurvivors (n = 233) | P value* | Percent Resistant | Pathogen‐ Specific Mortality Rate |
---|---|---|---|---|---|
| |||||
Enterobacteriaceae | 241 (79.8) | 143 (61.4) | <0.001 | 9.1 | 37.2 |
Pseudomonas aeruginosa | 47 (15.6) | 67 (28.8) | <0.001 | 16.7 | 58.8 |
Acinetobacter species | 22 (7.3) | 27 (11.6) | 0.087 | 71.4 | 55.1 |
Antimicrobial Treatment and Resistance
Among the study patients, 358 (66.9%) received cefepime, 102 (19.1%) received piperacillin‐tazobactam, and 75 (14.0%) received a carbapenem (meropenem or imipenem) as their initial antibiotic treatment. There were 169 (31.6%) patients who received initial combination therapy with either an aminoglycoside (n = 99, 58.6%) or ciprofloxacin (n = 70, 41.4%). Eighty‐two (15.3%) patients were infected with a pathogen that was resistant to the initial antibiotic treatment regimen [cefepime (n = 41; 50.0%), piperacillin‐tazobactam (n = 25; 30.5%), or imipenem/meropenem (n = 16; 19.5%), plus either an aminoglycoside or ciprofloxacin (n = 28; 34.1%)], and were classified as receiving inappropriate initial antibiotic therapy. Among the 453 (84.7%) patients infected with a pathogen that was susceptible to the initial antibiotic regimen, there was no relationship identified between minimum inhibitory concentration values and hospital mortality.
Patients infected with a pathogen resistant to the initial antibiotic regimen had significantly greater risk of hospital mortality (63.4% vs 40.0%; P < 0.001) (Figure 1). For the 82 individuals infected with a pathogen that was resistant to the initial antibiotic regimen, no difference in hospital mortality was observed among those prescribed initial combination treatment with an aminoglycoside (n = 17) (64.7% vs 61.1%; P = 0.790) or ciprofloxacin (n = 11) (72.7% vs 61.1%; P = 0.733) compared to monotherapy (n = 54). Similarly, among the patients infected with a pathogen that was susceptible to the initial antibiotic regimen, there was no difference in hospital mortality among those whose bloodstream isolate was only susceptible to the prescribed aminoglycoside (n = 12) compared to patients with isolates that were susceptible to the prescribed beta‐lactam antibiotic (n = 441) (41.7% vs 39.9%; P = 0.902).
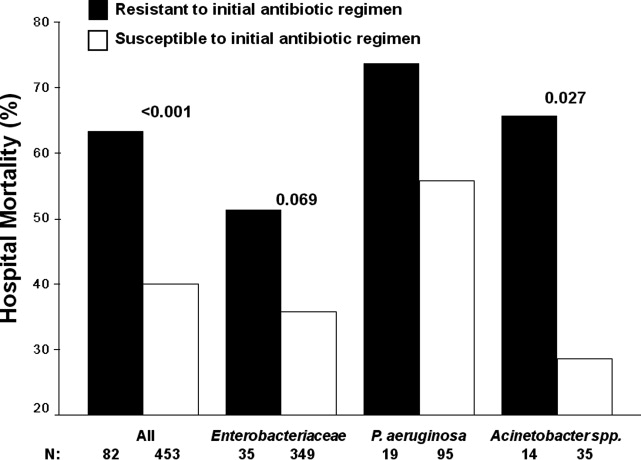
Logistic regression analysis identified infection with a pathogen resistant to the initial antibiotic regimen [adjusted odds ratio (AOR), 2.28; 95% confidence interval (CI), 1.69‐3.08; P = 0.006], increasing APACHE II scores (1‐point increments) (AOR, 1.13; 95% CI, 1.10‐1.15; P < 0.001), the need for vasopressors (AOR, 2.57; 95% CI, 2.15‐3.53; P < 0.001), the need for mechanical ventilation (AOR, 2.54; 95% CI, 2.19‐3.47; P < 0.001), healthcare‐associated hospital‐onset infection (AOR, 1.67; 95% CI, 1.32‐2.10; P =0.027), and infection with Pseudomonas aeruginosa (AOR, 2.21; 95% CI, 1.74‐2.86; P =0.002) as independent risk factors for hospital mortality (Hosmer‐Lemeshow goodness‐of‐fit test = 0.305). The model explained between 29.7% (Cox and Snell R square) and 39.8% (Nagelkerke R squared) of the variance in hospital mortality, and correctly classified 75.3% of cases.
Secondary Outcomes
Two or more acquired organ system derangements occurred significantly more often among patients with a pathogen resistant to the initial antibiotic regimen compared to those infected with susceptible isolates (84.1% vs 68.0%; P = 0.003). Hospital length of stay was significantly longer for patients infected with a pathogen resistant to the initial antibiotic regimen compared to those infected with susceptible isolates [39.9 50.6 days (median 27 days; quartiles 12 days and 45.5 days) vs 21.6 22.0 days (median 15 days; quartiles 7 days and 30 days); P < 0.001].
Discussion
Our study demonstrated that hospital nonsurvivors with severe sepsis attributed to Gram‐negative bacteremia had significantly greater rates of resistance to their initially prescribed antibiotic regimen compared to hospital survivors. This observation was confirmed in a multivariate analysis controlling for severity of illness and other potential confounding variables. Additionally, acquired organ system derangements and hospital length of stay were greater for patients infected with Gram‐negative pathogens resistant to the empiric antibiotic regimen. We also observed no survival advantage with the use of combination antimicrobial therapy for the subgroup of patients whose pathogens were resistant to the initially prescribed antibiotic regimen. Lastly, no difference in mortality was observed for patients with bacterial isolates that were susceptible only to the prescribed aminoglycoside compared to those with isolates susceptible to the prescribed beta‐lactam antibiotic.
Several previous investigators have linked antibiotic resistance and outcome in patients with serious infections attributed to Gram‐negative bacteria. Tam et al. examined 34 patients with Pseudomonas aeruginosa bacteremia having elevated MICs to piperacillin‐tazobactam (32 g/mL) that were reported as susceptible.19 In seven of these cases, piperacillin‐tazobactam was prescribed empirically, whereas other agents directed against Gram‐negative bacteria were employed in the other patients (carbapenems, aminoglycosides). Thirty‐day mortality was significantly greater for the patients treated with piperacillin‐tazobactam (85.7% vs 22.2%; P = 0.004), and a multivariate analysis found treatment with piperacillin‐tazobactam to be independently associated with 30‐day mortality. Similarly, Bhat et al. examined 204 episodes of bacteremia caused by Gram‐negative bacteria for which patients received cefepime.20 Patients infected with a Gram‐negative bacteria having an MIC to cefepime greater than, or equal to, 8 g/mL had a significantly greater 28‐day mortality compared to patients infected with isolates having an MIC to cefepime that was less than 8 g/mL (54.8% vs 24.1%; P = 0.001).
Our findings are consistent with earlier studies of patients with serious Gram‐negative infections including bacteremia and nosocomial pneumonia. Micek et al. showed that patients with Pseudomonas aeruginosa bacteremia who received inappropriate initial antimicrobial therapy had a greater risk of hospital mortality compared to patients initially treated with an antimicrobial regimen having activity for the Pseudomonas isolate based on in vitro susceptibility testing.21 Similarly, Trouillet et al.,22 Beardsley et al.,23 and Heyland et al.24 found that combination antimicrobial regimens directed against Gram‐negative bacteria in patients with nosocomial pneumonia were more likely to be appropriate based on the antimicrobial susceptibility patterns of the organisms compared to monotherapy. In a more recent study, Micek et al. demonstrated that combination antimicrobial therapy directed against severe sepsis attributed to Gram‐negative bacteria was associated with improved outcomes compared to monotherapy, especially when the combination agent was an aminoglycoside.25 However, empiric combination therapy that included an aminoglycoside was also associated with increased nephrotoxicity which makes the empiric use of aminoglycosides in all patients with suspected Gram‐negative severe sepsis problematic.25, 26 Nevertheless, the use of combination therapy represents a potential strategy to maximize the administration of appropriate treatment for serious Gram‐negative bacterial infections.
Rapid assessment of antimicrobial susceptibility is another strategy that offers the possibility of identifying the resistance pattern of Gram‐negative pathogens quickly in order to provide more appropriate treatment. Bouza et al. found that use of a rapid E‐test on the respiratory specimens of patients with ventilator‐associated pneumonia was associated with fewer days of fever, fewer days of antibiotic administration until resolution of the episode of ventilator‐associated pneumonia, decreased antibiotic consumption, less Clostridium difficile‐associated diarrhea, lower costs of antimicrobial agents, and fewer days receiving mechanical ventilation.27 Other methods for the rapid identification of resistant bacteria include real‐time polymerase chain reaction assays based on hybridization probes to identify specific resistance mechanisms in bacteria.28 Application of such methods for identification of broad categories of resistance mechanisms in Gram‐negative bacteria offer the possibility of tailoring initial antimicrobial regimens in order to provide appropriate therapy in a more timely manner.
Our study has several important limitations that should be noted. First, the study was performed at a single center and the results may not be generalizable to other institutions. However, the findings from other investigators corroborate the importance of antimicrobial resistance as a predictor of outcome for patients with serious Gram‐negative infections.19, 20 Additionally, a similar association has been observed in patients with methicillin‐resistant Staphylococcus aureus bacteremia, supporting the more general importance of antimicrobial resistance as an outcome predictor.29 Second, the method employed for determining MICs was a literature‐based linear regression method correlating disk diffusion diameters with broth dilution MIC determinations. Therefore, the lack of correlation we observed between MIC values and outcome for susceptible Gram‐negative isolates associated with severe sepsis requires further confirmation. Third, we only examined 3 antibiotics, or antibiotic classes, so our results may not be applicable to other agents. This also applies to doripenem, as we did not have that specific carbapenem available at the time this investigation took place.
Another important limitation of our study is the relatively small number of individuals infected with a pathogen that was resistant to the initial treatment regimen, or only susceptible to the aminoglycoside when combination therapy was prescribed. This limited our ability to detect meaningful associations in these subgroups of patients, to include whether or not combination therapy influenced their clinical outcome. Finally, we did not examine the exact timing of antibiotic therapy relative to the onset of severe sepsis. Instead we used a 12‐hour window from when subsequently positive blood cultures were drawn to the administration of initial antibiotic therapy. Other investigators have shown that delays in initial appropriate therapy of more than one hour for patients with septic shock increases the risk of death.9, 30 Failure to include the exact timing of therapy could have resulted in a final multivariate model that includes prediction variables that would not otherwise have been incorporated.
In summary, we demonstrated that resistance to the initial antibiotic treatment regimen was associated with a greater risk of hospital mortality in patients with severe sepsis attributed to Gram‐negative bacteremia. These findings imply that more rapid assessment of antimicrobial susceptibility could result in improved prescription of antibiotics in order to maximize initial administration of appropriate therapy. Future studies are required to address whether rapid determination of antimicrobial susceptibility can result in more effective administration of appropriate therapy, and if this can result in improved patient outcomes.
- Inadequate antimicrobial treatment of infections: a risk factor for hospital mortality among critically ill patients.Chest.1999;115:462–474. , , , .
- The clinical evaluation committee in a large multicenter phase 3 trial of drotrecogin alfa (activated) in patients with severe sepsis (PROWESS): role, methodology, and results.Crit Care Med.2003;31:2291–2301. , , , et al.
- Impact of adequate empical antibiotic therapy on the outcome of patients admitted to the intensive care unit with sepsis.Crit Care Med.2003;31:2742–2751. , , , , , .
- Inappropriate initial antimicrobial therapy and its effect on survival in a clinical trial of immunomodulating therapy for severe sepsis.Am J Med.2003;115:529–535. , , , , , .
- Antibiotic‐resistant bugs in the 21st century—a clinical super‐challenge.N Engl J Med.2009;360:439–443. , .
- Bad bugs, no drugs: no ESKAPE! An update from the Infectious Diseases Society of America.Clin Infect Dis.2009;48:1–12. , , , et al.
- Broad‐spectrum antimicrobials and the treatment of serious bacterial infections: getting it right up front.Clin Infect Dis.2008;47:S3–S13. .
- Bundled care for septic shock: an analysis of clinical trials.Crit Care Med.2010;38:668–678. , , , et al.
- Effectiveness of treatments for severe sepsis: a prospective, multicenter, observational study.Am J Respir Crit Care Med.2009;180:861–866. , , , et al.
- Surviving Sepsis Campaign: international guidelines for management of severe sepsis and septic shock: 2008.Crit Care Med.2008;36:296–327. , , , et al.
- APACHE II: a severity of disease classification system.Crit Care Med.1985;13:818–829. , , , .
- Invasive methicillin‐resistant Staphylococcus aureus infections in the United States.JAMA.2007;298:1763–1771. , , , et al.
- Epidemiology of severe sepsis in the United States: analysis of incidence, outcome, and associated costs of care.Crit Care Med.2001;29:1303–1310. , , , , , .
- Hospital‐wide impact of a standardized order set for the management of bacteremic severe sepsis.Crit Care Med.2009;37:819–824. , , , , , .
- Before‐after study of a standardized hospital order set for the management of septic shock.Crit Care Med.2007;34:2707–2713. , , , et al.
- National Committee for Clinical Laboratory Standards.Performance Standards for Antimicrobial Susceptibility Testing: Twelfth Informational Supplement. M100‐S12.Wayne, PA:National Committee for Clinical Laboratory Standards;2002.
- Clinical Laboratory Standards Institute.Performance Standards for Antimicrobial Susceptibility Testing: Seventeenth Informational Supplement. M100‐S17.Wayne, PA:Clinical Laboratory Standards Institute;2007.
- Evaluation of the BIOGRAM antimicrobial susceptibility test system.J Clin Microbiol.1985;22:793–798. , , , et al.
- Outcomes of bacteremia due to Pseudomonas aeruginosa with reduced susceptibility to piperacillin‐tazobactam: implications on the appropriateness of the resistance breakpoint.Clin Infect Dis.2008;46:862–867. , , , et al.
- Failure of current cefepime breakpoints to predict clinical outcomes of bacteremia caused by Gram‐negative organisms.Antimicrob Agents Chemother.2007;51:4390–4395. , , , et al.
- Pseudomonas aeruginosa bloodstream infection: importance of appropriate initial antimicrobial treatment.Antimicrob Agents Chemother.2005;49:1306–1311. , , , , , .
- Ventilator‐associated pneumonia caused by potentially drug‐resistant bacteria.Am J Respir Crit Care Med.1998;157:531–539. , , .
- Using local microbiologic data to develop institution‐specific guidelines for the treatment of hospital‐acquired pneumonia.Chest.2006;130:787–793. , , , , , .
- Randomized trial of combination versus monotherapy for the empiric treatment of suspected ventilator‐associated pneumonia.Crit Care Med.2008;36:737–744. , , , et al.
- Empiric combination antibiotic therapy is associated with improved outcome in Gram‐negative sepsis: a retrospective analysis.Antimicrob Agents Chemother.2010;54:1742–1748. , , , et al.
- Monotherapy versus beta‐lactam‐aminoglycoside combination treatment for Gram‐negative bacteremia: a prospective, observational study.Antimicrob Agents Chemother.1997;41:1127–1133. , , , et al.
- Direct E‐test (AB Biodisk) of respiratory samples improves antimicrobial use in ventilator‐associated pneumonia.Clin Infect Dis.2007;44:382–387. , , , et al.
- Rapid detection of CTX‐M‐producing Enterobacteriaceae in urine samples.J Antimicrob Chemother.2009;64:986–989. , , , et al.
- Influence of vancomycin minimum inhibitory concentration on the treatment of methicillin‐resistant Staphylococcus aureus bacteremia.Clin Infect Dis.2008;46:193–200. , , , et al.
- Duration of hypotension before initiation of effective antimicrobial therapy is the critical determinant of survival in human septic shock.Crit Care Med.2006;34:1589–1596. , , , et al.
Severe sepsis and septic shock are associated with excess mortality when inappropriate initial antimicrobial therapy, defined as an antimicrobial regimen that lacks in vitro activity against the isolated organism(s) responsible for the infection, is administered.14 Unfortunately, bacterial resistance to antibiotics is increasing and creates a therapeutic challenge for clinicians when treating patients with serious infections, such as severe sepsis. Increasing rates of bacterial resistance leads many clinicians to empirically treat critically ill patients with broad‐spectrum antibiotics, which can perpetuate the cycle of increasing resistance.5, 6 Conversely, inappropriate initial antimicrobial therapy can lead to treatment failures and adverse patient outcomes.7 Individuals with severe sepsis appear to be at particularly high risk of excess mortality when inappropriate initial antimicrobial therapy is administered.8, 9
The most recent Surviving Sepsis Guidelines recommend empiric combination therapy targeting Gram‐negative bacteria, particularly for patients with known or suspected Pseudomonas infections, as a means to decrease the likelihood of administering inappropriate initial antimicrobial therapy.10 However, the selection of an antimicrobial regimen that is active against the causative pathogen(s) is problematic, as the treating physician usually does not know the susceptibilities of the pathogen(s) for the selected empiric antibiotics. Therefore, we performed a study with the main goal of determining whether resistance to the initially prescribed antimicrobial regimen was associated with clinical outcome in patients with severe sepsis attributed to Gram‐negative bacteremia.
Materials and Methods
Study Location and Patients
This study was conducted at a university‐affiliated, urban teaching hospital: Barnes‐Jewish Hospital (1200 beds). During a 6‐year period (January 2002 to December 2007), all hospitalized patients with a positive blood culture for Gram‐negative bacteria, with antimicrobial susceptibility testing performed for the blood isolate(s), were eligible for this investigation. This study was approved by the Washington University School of Medicine Human Studies Committee.
Study Design and Data Collection
A retrospective cohort study design was employed. Two investigators (J.A.D., R.M.R.) identified potential study patients by the presence of a positive blood culture for Pseudomonas aeruginosa, Acinetobacter species, or Enterobacteriaceae (Escherichia coli, Klebsiella species, Enterobacter species) combined with primary or secondary International Classification of Diseases (ICD‐9‐CM) codes indicative of acute organ dysfunction, at least two criteria from the systemic inflammatory response syndrome (SIRS),10 and initial antibiotic treatment with either cefepime, piperacillin‐tazobactam, or a carbapenem (imipenem or meropenem). These antimicrobials represent the primary agents employed for the treatment of Gram‐negative infections at Barnes‐Jewish Hospital during the study period, and had to be administered within 12 hours of having the subsequently positive blood cultures drawn. Based on the initial study database construction, 3 investigators (E.C.W., J.K., M.P.) merged patient‐specific data from the automated hospital medical records, microbiology database, and pharmacy database of Barnes‐Jewish Hospital to complete the clinical database under the auspices of the definitions described below.
The baseline characteristics collected by the study investigators included: age, gender, race, the presence of congestive heart failure, chronic obstructive pulmonary disease, diabetes mellitus, chronic liver disease, underlying malignancy, and end‐stage renal disease requiring renal replacement therapy. All cause hospital mortality was evaluated as the primary outcome variable. Secondary outcomes included acquired organ dysfunction and hospital length of stay. The Acute Physiology and Chronic Health Evaluation (APACHE) II11 and Charlson co‐morbidity scores were also calculated during the 24 hours after the positive blood cultures were drawn. This was done because we included patients with community‐acquired infections who only had clinical data available after blood cultures were drawn.
Definitions
All definitions were selected prospectively as part of the original study design. Cases of Gram‐negative bacteremia were classified into mutually exclusive groups comprised of either community‐acquired or healthcare‐associated infection. Patients with healthcare‐associated bacteremia were categorized as community‐onset or hospital‐onset, as previously described.12 In brief, patients with healthcare‐associated community‐onset bacteremia had the positive culture obtained within the first 48 hours of hospital admission in combination with one or more of the following risk factors: (1) residence in a nursing home, rehabilitation hospital, or other long‐term nursing facility; (2) previous hospitalization within the immediately preceding 12 months; (3) receiving outpatient hemodialysis, peritoneal dialysis, wound care, or infusion therapy necessitating regular visits to a hospital‐based clinic; and (4) having an immune‐compromised state. Patients were classified as having healthcare‐associated hospital‐onset bacteremia when the culture was obtained 48 hours or more after admission. Community‐acquired bacteremia occurred in patients without healthcare risk factors and a positive blood culture within the first 48 hours of admission. Prior antibiotic exposure was defined as having occurred within the previous 30 days from the onset of severe sepsis.
To be included in the analysis, patients had to meet criteria for severe sepsis based on discharge ICD‐9‐CM codes for acute organ dysfunction, as previously described.13 The organs of interest included the heart, lungs, kidneys, bone marrow (hematologic), brain, and liver. Patients were classified as having septic shock if vasopressors (norepinephrine, dopamine, epinephrine, phenylephrine, or vasopressin) were initiated within 24 hours of the blood culture collection date and time. Empiric antimicrobial treatment was classified as being appropriate if the initially prescribed antibiotic regimen was active against the identified pathogen(s) based on in vitro susceptibility testing and administered within 12 hours following blood culture collection. Appropriate antimicrobial treatment also had to be prescribed for at least 24 hours. However, the total duration of antimicrobial therapy was at the discretion of the treating physicians. The Charlson co‐morbidity score was calculated using ICD‐9‐CM codes abstracted from the index hospitalization employing MS‐DRG Grouper version 26.
Antimicrobial Monitoring
From January 2002 through the present, Barnes‐Jewish Hospital utilized an antibiotic control program to help guide antimicrobial therapy. During this time, the use of cefepime and gentamicin was unrestricted. However, initiation of intravenous ciprofloxacin, imipenem/cilastatin, meropenem, or piperacillin/tazobactam was restricted and required preauthorization from either a clinical pharmacist or infectious diseases physician. Each intensive care unit (ICU) had a clinical pharmacist who reviewed all antibiotic orders to insure that dosing and interval of antibiotic administration was adequate for individual patients based on body size, renal function, and the resuscitation status of the patient. After daytime hours, the on‐call clinical pharmacist reviewed and approved the antibiotic orders. The initial antibiotic dosages for the antibiotics employed for the treatment of Gram‐negative infections at Barnes‐Jewish Hospital were as follows: cefepime, 1 to 2 grams every eight hours; pipercillin‐tazobactam, 4.5 grams every six hours; imipenem, 0.5 grams every six hours; meropenem, 1 gram every eight hours; ciprofloxacin, 400 mg every eight hours; gentamicin, 5 mg/kg once daily.
Starting in June 2005, a sepsis order set was implemented in the emergency department, general medical wards, and the intensive care units with the intent of standardizing empiric antibiotic selection for patients with sepsis based on the infection type (ie, community‐acquired pneumonia, healthcare‐associated pneumonia, intra‐abdominal infection, etc) and the hospital's antibiogram.14, 15 However, antimicrobial selection, dosing, and de‐escalation of therapy were still optimized by clinical pharmacists in these clinical areas.
Antimicrobial Susceptibility Testing
The microbiology laboratory performed antimicrobial susceptibility testing of the Gram‐negative blood isolates using the disk diffusion method according to guidelines and breakpoints established by the Clinical Laboratory and Standards Institute (CLSI) and published during the inclusive years of the study.16, 17 Zone diameters obtained by disk diffusion testing were converted to minimum inhibitory concentrations (MICs in mg/L) by linear regression analysis for each antimicrobial agent using the BIOMIC V3 antimicrobial susceptibility system (Giles Scientific, Inc., Santa Barbara, CA). Linear regression algorithms contained in the software of this system were determined by comparative studies correlating microbroth dilution‐determined MIC values with zone sizes obtained by disk diffusion testing.18
Data Analysis
Continuous variables were reported as mean the standard deviation, or median and quartiles. The Student's t test was used when comparing normally distributed data, and the MannWhitney U test was employed to analyze nonnormally distributed data. Categorical data were expressed as frequency distributions and the Chi‐squared test was used to determine if differences existed between groups. We performed multiple logistic regression analysis to identify clinical risk factors that were associated with hospital mortality (SPSS, Inc., Chicago, IL). All risk factors from Table 1, as well as the individual pathogens examined, were included in the corresponding multivariable analysis with the exception of acquired organ dysfunction (considered a secondary outcome). All tests were two‐tailed, and a P value <0.05 was determined to represent statistical significance.
Variable | Hospital Survivors (n = 302) | Hospital Nonsurvivors (n = 233) | P value |
---|---|---|---|
| |||
Age, years | 57.9 16.2 | 60.3 15.8 | 0.091 |
Male | 156 (51.7) | 132 (56.7) | 0.250 |
Infection onset source | |||
Community‐acquired | 31 (10.3) | 15 (6.4) | 0.005 |
Healthcare‐associated community‐onset | 119 (39.4) | 68 (29.2) | |
Healthcare‐associated hospital‐onset | 152 (50.3) | 150 (64.4) | |
Underlying co‐morbidities | |||
CHF | 43 (14.2) | 53 (22.7) | 0.011 |
COPD | 42 (13.9) | 56 (24.0) | 0.003 |
Chronic kidney disease | 31 (10.3) | 41 (17.6) | 0.014 |
Liver disease | 34 (11.3) | 31 (13.3) | 0.473 |
Active malignancy | 100 (33.1) | 83 (35.6) | 0.544 |
Diabetes | 68 (22.5) | 50 (21.5) | 0.770 |
Charlson co‐morbidity score | 4.5 3.5 | 5.2 3.9 | 0.041 |
APACHE II score | 21.8 6.1 | 27.1 6.2 | <0.001 |
ICU admission | 221 (73.2) | 216 (92.7) | <0.001 |
Vasopressors | 137 (45.4) | 197 (84.5) | <0.001 |
Mechanical ventilation | 124 (41.1) | 183 (78.5) | <0.001 |
Drotrecogin alfa (activated) | 6 (2.0) | 21 (9.0) | <0.001 |
Dysfunctional acquired organ systems | |||
Cardiovascular | 149 (49.3) | 204 (87.6) | <0.001 |
Respiratory | 141 (46.7) | 202 (86.7) | <0.001 |
Renal | 145 (48.0) | 136 (58.4) | 0.017 |
Hepatic | 13 (4.3) | 27 (11.6) | 0.001 |
Hematologic | 103 (34.1) | 63 (27.0) | 0.080 |
Neurologic | 11 (3.6) | 19 (8.2) | 0.024 |
2 Dysfunctional acquired organ systems | 164 (54.3) | 213 (91.4) | <0.001 |
Source of bloodstream infection | |||
Lungs | 95 (31.5) | 127 (54.5) | <0.001 |
Urinary tract | 92 (30.5) | 45 (19.3) | |
Central venous catheter | 30 (9.9) | 16 (6.9) | |
Intra‐abdominal | 63 (20.9) | 33 (14.2) | |
Unknown | 22 (7.3) | 12 (5.2) | |
Prior antibiotics* | 103 (34.1) | 110 (47.2) | 0.002 |
Results
Patient Characteristics
Included in the study were 535 consecutive patients with severe sepsis attributed to Pseudomonas aeruginosa, Acinetobacter species, or Enterobacteriaceae bacteremia, of whom 233 (43.6%) died during their hospitalization. The mean age was 58.9 16.0 years (range, 18 to 96 years) with 288 (53.8%) males and 247 (46.2%) females. The infection sources included community‐acquired (n = 46, 8.6%), healthcare‐associated community‐onset (n = 187, 35.0%), and healthcare‐associated hospital‐onset (n = 302, 56.4%). Hospital nonsurvivors were statistically more likely to have a healthcare‐associated hospital‐onset infection, congestive heart failure, chronic obstructive pulmonary disease, chronic kidney disease, ICU admission, need for mechanical ventilation and/or vasopressors, administration of drotrecogin alfa (activated), prior antibiotic administration, the lungs as the source of infection, acquired dysfunction of the cardiovascular, respiratory, renal, hepatic, and neurologic organ systems, and greater APACHE II and Charlson co‐morbidity scores compared to hospital survivors (Table 1). Hospital nonsurvivors were also statistically less likely to have a healthcare‐associated community‐onset infection and a urinary source of infection compared to hospital survivors (Table 1).
Microbiology
Among the 547 Gram‐negative bacteria isolated from blood, the most common were Enterobacteriaceae (Escherichia coli, Klebsiella species, Enterobacter species) (70.2%) followed by Pseudomonas aeruginosa (20.8%) and Acinetobacter species (9.0%) (Table 2). Nine patients had two different Enterobacteriaceae species isolated from their blood cultures, and three patients had an Enterobacteriaceae species and Pseudomonas aeruginosa isolated from their blood cultures. Hospital nonsurvivors were statistically more likely to be infected with Pseudomonas aeruginosa and less likely to be infected with Enterobacteriaceae. The pathogen‐specific hospital mortality rate was significantly greater for Pseudomonas aeruginosa and Acinetobacter species compared to Enterobacteriaceae (P < 0.001 and P = 0.008, respectively).
Bacteria | Hospital Survivors (n = 302) | Hospital Nonsurvivors (n = 233) | P value* | Percent Resistant | Pathogen‐ Specific Mortality Rate |
---|---|---|---|---|---|
| |||||
Enterobacteriaceae | 241 (79.8) | 143 (61.4) | <0.001 | 9.1 | 37.2 |
Pseudomonas aeruginosa | 47 (15.6) | 67 (28.8) | <0.001 | 16.7 | 58.8 |
Acinetobacter species | 22 (7.3) | 27 (11.6) | 0.087 | 71.4 | 55.1 |
Antimicrobial Treatment and Resistance
Among the study patients, 358 (66.9%) received cefepime, 102 (19.1%) received piperacillin‐tazobactam, and 75 (14.0%) received a carbapenem (meropenem or imipenem) as their initial antibiotic treatment. There were 169 (31.6%) patients who received initial combination therapy with either an aminoglycoside (n = 99, 58.6%) or ciprofloxacin (n = 70, 41.4%). Eighty‐two (15.3%) patients were infected with a pathogen that was resistant to the initial antibiotic treatment regimen [cefepime (n = 41; 50.0%), piperacillin‐tazobactam (n = 25; 30.5%), or imipenem/meropenem (n = 16; 19.5%), plus either an aminoglycoside or ciprofloxacin (n = 28; 34.1%)], and were classified as receiving inappropriate initial antibiotic therapy. Among the 453 (84.7%) patients infected with a pathogen that was susceptible to the initial antibiotic regimen, there was no relationship identified between minimum inhibitory concentration values and hospital mortality.
Patients infected with a pathogen resistant to the initial antibiotic regimen had significantly greater risk of hospital mortality (63.4% vs 40.0%; P < 0.001) (Figure 1). For the 82 individuals infected with a pathogen that was resistant to the initial antibiotic regimen, no difference in hospital mortality was observed among those prescribed initial combination treatment with an aminoglycoside (n = 17) (64.7% vs 61.1%; P = 0.790) or ciprofloxacin (n = 11) (72.7% vs 61.1%; P = 0.733) compared to monotherapy (n = 54). Similarly, among the patients infected with a pathogen that was susceptible to the initial antibiotic regimen, there was no difference in hospital mortality among those whose bloodstream isolate was only susceptible to the prescribed aminoglycoside (n = 12) compared to patients with isolates that were susceptible to the prescribed beta‐lactam antibiotic (n = 441) (41.7% vs 39.9%; P = 0.902).
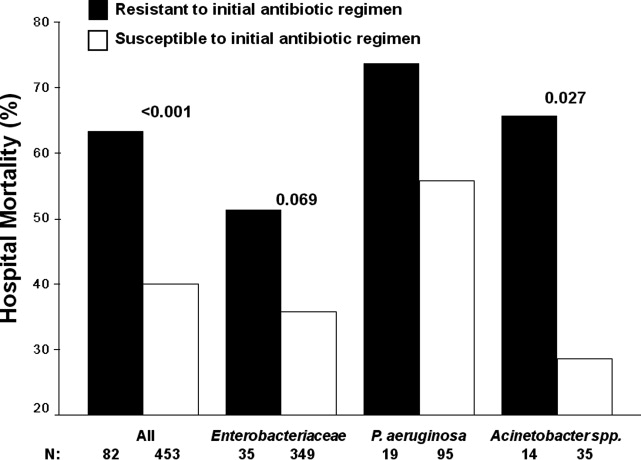
Logistic regression analysis identified infection with a pathogen resistant to the initial antibiotic regimen [adjusted odds ratio (AOR), 2.28; 95% confidence interval (CI), 1.69‐3.08; P = 0.006], increasing APACHE II scores (1‐point increments) (AOR, 1.13; 95% CI, 1.10‐1.15; P < 0.001), the need for vasopressors (AOR, 2.57; 95% CI, 2.15‐3.53; P < 0.001), the need for mechanical ventilation (AOR, 2.54; 95% CI, 2.19‐3.47; P < 0.001), healthcare‐associated hospital‐onset infection (AOR, 1.67; 95% CI, 1.32‐2.10; P =0.027), and infection with Pseudomonas aeruginosa (AOR, 2.21; 95% CI, 1.74‐2.86; P =0.002) as independent risk factors for hospital mortality (Hosmer‐Lemeshow goodness‐of‐fit test = 0.305). The model explained between 29.7% (Cox and Snell R square) and 39.8% (Nagelkerke R squared) of the variance in hospital mortality, and correctly classified 75.3% of cases.
Secondary Outcomes
Two or more acquired organ system derangements occurred significantly more often among patients with a pathogen resistant to the initial antibiotic regimen compared to those infected with susceptible isolates (84.1% vs 68.0%; P = 0.003). Hospital length of stay was significantly longer for patients infected with a pathogen resistant to the initial antibiotic regimen compared to those infected with susceptible isolates [39.9 50.6 days (median 27 days; quartiles 12 days and 45.5 days) vs 21.6 22.0 days (median 15 days; quartiles 7 days and 30 days); P < 0.001].
Discussion
Our study demonstrated that hospital nonsurvivors with severe sepsis attributed to Gram‐negative bacteremia had significantly greater rates of resistance to their initially prescribed antibiotic regimen compared to hospital survivors. This observation was confirmed in a multivariate analysis controlling for severity of illness and other potential confounding variables. Additionally, acquired organ system derangements and hospital length of stay were greater for patients infected with Gram‐negative pathogens resistant to the empiric antibiotic regimen. We also observed no survival advantage with the use of combination antimicrobial therapy for the subgroup of patients whose pathogens were resistant to the initially prescribed antibiotic regimen. Lastly, no difference in mortality was observed for patients with bacterial isolates that were susceptible only to the prescribed aminoglycoside compared to those with isolates susceptible to the prescribed beta‐lactam antibiotic.
Several previous investigators have linked antibiotic resistance and outcome in patients with serious infections attributed to Gram‐negative bacteria. Tam et al. examined 34 patients with Pseudomonas aeruginosa bacteremia having elevated MICs to piperacillin‐tazobactam (32 g/mL) that were reported as susceptible.19 In seven of these cases, piperacillin‐tazobactam was prescribed empirically, whereas other agents directed against Gram‐negative bacteria were employed in the other patients (carbapenems, aminoglycosides). Thirty‐day mortality was significantly greater for the patients treated with piperacillin‐tazobactam (85.7% vs 22.2%; P = 0.004), and a multivariate analysis found treatment with piperacillin‐tazobactam to be independently associated with 30‐day mortality. Similarly, Bhat et al. examined 204 episodes of bacteremia caused by Gram‐negative bacteria for which patients received cefepime.20 Patients infected with a Gram‐negative bacteria having an MIC to cefepime greater than, or equal to, 8 g/mL had a significantly greater 28‐day mortality compared to patients infected with isolates having an MIC to cefepime that was less than 8 g/mL (54.8% vs 24.1%; P = 0.001).
Our findings are consistent with earlier studies of patients with serious Gram‐negative infections including bacteremia and nosocomial pneumonia. Micek et al. showed that patients with Pseudomonas aeruginosa bacteremia who received inappropriate initial antimicrobial therapy had a greater risk of hospital mortality compared to patients initially treated with an antimicrobial regimen having activity for the Pseudomonas isolate based on in vitro susceptibility testing.21 Similarly, Trouillet et al.,22 Beardsley et al.,23 and Heyland et al.24 found that combination antimicrobial regimens directed against Gram‐negative bacteria in patients with nosocomial pneumonia were more likely to be appropriate based on the antimicrobial susceptibility patterns of the organisms compared to monotherapy. In a more recent study, Micek et al. demonstrated that combination antimicrobial therapy directed against severe sepsis attributed to Gram‐negative bacteria was associated with improved outcomes compared to monotherapy, especially when the combination agent was an aminoglycoside.25 However, empiric combination therapy that included an aminoglycoside was also associated with increased nephrotoxicity which makes the empiric use of aminoglycosides in all patients with suspected Gram‐negative severe sepsis problematic.25, 26 Nevertheless, the use of combination therapy represents a potential strategy to maximize the administration of appropriate treatment for serious Gram‐negative bacterial infections.
Rapid assessment of antimicrobial susceptibility is another strategy that offers the possibility of identifying the resistance pattern of Gram‐negative pathogens quickly in order to provide more appropriate treatment. Bouza et al. found that use of a rapid E‐test on the respiratory specimens of patients with ventilator‐associated pneumonia was associated with fewer days of fever, fewer days of antibiotic administration until resolution of the episode of ventilator‐associated pneumonia, decreased antibiotic consumption, less Clostridium difficile‐associated diarrhea, lower costs of antimicrobial agents, and fewer days receiving mechanical ventilation.27 Other methods for the rapid identification of resistant bacteria include real‐time polymerase chain reaction assays based on hybridization probes to identify specific resistance mechanisms in bacteria.28 Application of such methods for identification of broad categories of resistance mechanisms in Gram‐negative bacteria offer the possibility of tailoring initial antimicrobial regimens in order to provide appropriate therapy in a more timely manner.
Our study has several important limitations that should be noted. First, the study was performed at a single center and the results may not be generalizable to other institutions. However, the findings from other investigators corroborate the importance of antimicrobial resistance as a predictor of outcome for patients with serious Gram‐negative infections.19, 20 Additionally, a similar association has been observed in patients with methicillin‐resistant Staphylococcus aureus bacteremia, supporting the more general importance of antimicrobial resistance as an outcome predictor.29 Second, the method employed for determining MICs was a literature‐based linear regression method correlating disk diffusion diameters with broth dilution MIC determinations. Therefore, the lack of correlation we observed between MIC values and outcome for susceptible Gram‐negative isolates associated with severe sepsis requires further confirmation. Third, we only examined 3 antibiotics, or antibiotic classes, so our results may not be applicable to other agents. This also applies to doripenem, as we did not have that specific carbapenem available at the time this investigation took place.
Another important limitation of our study is the relatively small number of individuals infected with a pathogen that was resistant to the initial treatment regimen, or only susceptible to the aminoglycoside when combination therapy was prescribed. This limited our ability to detect meaningful associations in these subgroups of patients, to include whether or not combination therapy influenced their clinical outcome. Finally, we did not examine the exact timing of antibiotic therapy relative to the onset of severe sepsis. Instead we used a 12‐hour window from when subsequently positive blood cultures were drawn to the administration of initial antibiotic therapy. Other investigators have shown that delays in initial appropriate therapy of more than one hour for patients with septic shock increases the risk of death.9, 30 Failure to include the exact timing of therapy could have resulted in a final multivariate model that includes prediction variables that would not otherwise have been incorporated.
In summary, we demonstrated that resistance to the initial antibiotic treatment regimen was associated with a greater risk of hospital mortality in patients with severe sepsis attributed to Gram‐negative bacteremia. These findings imply that more rapid assessment of antimicrobial susceptibility could result in improved prescription of antibiotics in order to maximize initial administration of appropriate therapy. Future studies are required to address whether rapid determination of antimicrobial susceptibility can result in more effective administration of appropriate therapy, and if this can result in improved patient outcomes.
Severe sepsis and septic shock are associated with excess mortality when inappropriate initial antimicrobial therapy, defined as an antimicrobial regimen that lacks in vitro activity against the isolated organism(s) responsible for the infection, is administered.14 Unfortunately, bacterial resistance to antibiotics is increasing and creates a therapeutic challenge for clinicians when treating patients with serious infections, such as severe sepsis. Increasing rates of bacterial resistance leads many clinicians to empirically treat critically ill patients with broad‐spectrum antibiotics, which can perpetuate the cycle of increasing resistance.5, 6 Conversely, inappropriate initial antimicrobial therapy can lead to treatment failures and adverse patient outcomes.7 Individuals with severe sepsis appear to be at particularly high risk of excess mortality when inappropriate initial antimicrobial therapy is administered.8, 9
The most recent Surviving Sepsis Guidelines recommend empiric combination therapy targeting Gram‐negative bacteria, particularly for patients with known or suspected Pseudomonas infections, as a means to decrease the likelihood of administering inappropriate initial antimicrobial therapy.10 However, the selection of an antimicrobial regimen that is active against the causative pathogen(s) is problematic, as the treating physician usually does not know the susceptibilities of the pathogen(s) for the selected empiric antibiotics. Therefore, we performed a study with the main goal of determining whether resistance to the initially prescribed antimicrobial regimen was associated with clinical outcome in patients with severe sepsis attributed to Gram‐negative bacteremia.
Materials and Methods
Study Location and Patients
This study was conducted at a university‐affiliated, urban teaching hospital: Barnes‐Jewish Hospital (1200 beds). During a 6‐year period (January 2002 to December 2007), all hospitalized patients with a positive blood culture for Gram‐negative bacteria, with antimicrobial susceptibility testing performed for the blood isolate(s), were eligible for this investigation. This study was approved by the Washington University School of Medicine Human Studies Committee.
Study Design and Data Collection
A retrospective cohort study design was employed. Two investigators (J.A.D., R.M.R.) identified potential study patients by the presence of a positive blood culture for Pseudomonas aeruginosa, Acinetobacter species, or Enterobacteriaceae (Escherichia coli, Klebsiella species, Enterobacter species) combined with primary or secondary International Classification of Diseases (ICD‐9‐CM) codes indicative of acute organ dysfunction, at least two criteria from the systemic inflammatory response syndrome (SIRS),10 and initial antibiotic treatment with either cefepime, piperacillin‐tazobactam, or a carbapenem (imipenem or meropenem). These antimicrobials represent the primary agents employed for the treatment of Gram‐negative infections at Barnes‐Jewish Hospital during the study period, and had to be administered within 12 hours of having the subsequently positive blood cultures drawn. Based on the initial study database construction, 3 investigators (E.C.W., J.K., M.P.) merged patient‐specific data from the automated hospital medical records, microbiology database, and pharmacy database of Barnes‐Jewish Hospital to complete the clinical database under the auspices of the definitions described below.
The baseline characteristics collected by the study investigators included: age, gender, race, the presence of congestive heart failure, chronic obstructive pulmonary disease, diabetes mellitus, chronic liver disease, underlying malignancy, and end‐stage renal disease requiring renal replacement therapy. All cause hospital mortality was evaluated as the primary outcome variable. Secondary outcomes included acquired organ dysfunction and hospital length of stay. The Acute Physiology and Chronic Health Evaluation (APACHE) II11 and Charlson co‐morbidity scores were also calculated during the 24 hours after the positive blood cultures were drawn. This was done because we included patients with community‐acquired infections who only had clinical data available after blood cultures were drawn.
Definitions
All definitions were selected prospectively as part of the original study design. Cases of Gram‐negative bacteremia were classified into mutually exclusive groups comprised of either community‐acquired or healthcare‐associated infection. Patients with healthcare‐associated bacteremia were categorized as community‐onset or hospital‐onset, as previously described.12 In brief, patients with healthcare‐associated community‐onset bacteremia had the positive culture obtained within the first 48 hours of hospital admission in combination with one or more of the following risk factors: (1) residence in a nursing home, rehabilitation hospital, or other long‐term nursing facility; (2) previous hospitalization within the immediately preceding 12 months; (3) receiving outpatient hemodialysis, peritoneal dialysis, wound care, or infusion therapy necessitating regular visits to a hospital‐based clinic; and (4) having an immune‐compromised state. Patients were classified as having healthcare‐associated hospital‐onset bacteremia when the culture was obtained 48 hours or more after admission. Community‐acquired bacteremia occurred in patients without healthcare risk factors and a positive blood culture within the first 48 hours of admission. Prior antibiotic exposure was defined as having occurred within the previous 30 days from the onset of severe sepsis.
To be included in the analysis, patients had to meet criteria for severe sepsis based on discharge ICD‐9‐CM codes for acute organ dysfunction, as previously described.13 The organs of interest included the heart, lungs, kidneys, bone marrow (hematologic), brain, and liver. Patients were classified as having septic shock if vasopressors (norepinephrine, dopamine, epinephrine, phenylephrine, or vasopressin) were initiated within 24 hours of the blood culture collection date and time. Empiric antimicrobial treatment was classified as being appropriate if the initially prescribed antibiotic regimen was active against the identified pathogen(s) based on in vitro susceptibility testing and administered within 12 hours following blood culture collection. Appropriate antimicrobial treatment also had to be prescribed for at least 24 hours. However, the total duration of antimicrobial therapy was at the discretion of the treating physicians. The Charlson co‐morbidity score was calculated using ICD‐9‐CM codes abstracted from the index hospitalization employing MS‐DRG Grouper version 26.
Antimicrobial Monitoring
From January 2002 through the present, Barnes‐Jewish Hospital utilized an antibiotic control program to help guide antimicrobial therapy. During this time, the use of cefepime and gentamicin was unrestricted. However, initiation of intravenous ciprofloxacin, imipenem/cilastatin, meropenem, or piperacillin/tazobactam was restricted and required preauthorization from either a clinical pharmacist or infectious diseases physician. Each intensive care unit (ICU) had a clinical pharmacist who reviewed all antibiotic orders to insure that dosing and interval of antibiotic administration was adequate for individual patients based on body size, renal function, and the resuscitation status of the patient. After daytime hours, the on‐call clinical pharmacist reviewed and approved the antibiotic orders. The initial antibiotic dosages for the antibiotics employed for the treatment of Gram‐negative infections at Barnes‐Jewish Hospital were as follows: cefepime, 1 to 2 grams every eight hours; pipercillin‐tazobactam, 4.5 grams every six hours; imipenem, 0.5 grams every six hours; meropenem, 1 gram every eight hours; ciprofloxacin, 400 mg every eight hours; gentamicin, 5 mg/kg once daily.
Starting in June 2005, a sepsis order set was implemented in the emergency department, general medical wards, and the intensive care units with the intent of standardizing empiric antibiotic selection for patients with sepsis based on the infection type (ie, community‐acquired pneumonia, healthcare‐associated pneumonia, intra‐abdominal infection, etc) and the hospital's antibiogram.14, 15 However, antimicrobial selection, dosing, and de‐escalation of therapy were still optimized by clinical pharmacists in these clinical areas.
Antimicrobial Susceptibility Testing
The microbiology laboratory performed antimicrobial susceptibility testing of the Gram‐negative blood isolates using the disk diffusion method according to guidelines and breakpoints established by the Clinical Laboratory and Standards Institute (CLSI) and published during the inclusive years of the study.16, 17 Zone diameters obtained by disk diffusion testing were converted to minimum inhibitory concentrations (MICs in mg/L) by linear regression analysis for each antimicrobial agent using the BIOMIC V3 antimicrobial susceptibility system (Giles Scientific, Inc., Santa Barbara, CA). Linear regression algorithms contained in the software of this system were determined by comparative studies correlating microbroth dilution‐determined MIC values with zone sizes obtained by disk diffusion testing.18
Data Analysis
Continuous variables were reported as mean the standard deviation, or median and quartiles. The Student's t test was used when comparing normally distributed data, and the MannWhitney U test was employed to analyze nonnormally distributed data. Categorical data were expressed as frequency distributions and the Chi‐squared test was used to determine if differences existed between groups. We performed multiple logistic regression analysis to identify clinical risk factors that were associated with hospital mortality (SPSS, Inc., Chicago, IL). All risk factors from Table 1, as well as the individual pathogens examined, were included in the corresponding multivariable analysis with the exception of acquired organ dysfunction (considered a secondary outcome). All tests were two‐tailed, and a P value <0.05 was determined to represent statistical significance.
Variable | Hospital Survivors (n = 302) | Hospital Nonsurvivors (n = 233) | P value |
---|---|---|---|
| |||
Age, years | 57.9 16.2 | 60.3 15.8 | 0.091 |
Male | 156 (51.7) | 132 (56.7) | 0.250 |
Infection onset source | |||
Community‐acquired | 31 (10.3) | 15 (6.4) | 0.005 |
Healthcare‐associated community‐onset | 119 (39.4) | 68 (29.2) | |
Healthcare‐associated hospital‐onset | 152 (50.3) | 150 (64.4) | |
Underlying co‐morbidities | |||
CHF | 43 (14.2) | 53 (22.7) | 0.011 |
COPD | 42 (13.9) | 56 (24.0) | 0.003 |
Chronic kidney disease | 31 (10.3) | 41 (17.6) | 0.014 |
Liver disease | 34 (11.3) | 31 (13.3) | 0.473 |
Active malignancy | 100 (33.1) | 83 (35.6) | 0.544 |
Diabetes | 68 (22.5) | 50 (21.5) | 0.770 |
Charlson co‐morbidity score | 4.5 3.5 | 5.2 3.9 | 0.041 |
APACHE II score | 21.8 6.1 | 27.1 6.2 | <0.001 |
ICU admission | 221 (73.2) | 216 (92.7) | <0.001 |
Vasopressors | 137 (45.4) | 197 (84.5) | <0.001 |
Mechanical ventilation | 124 (41.1) | 183 (78.5) | <0.001 |
Drotrecogin alfa (activated) | 6 (2.0) | 21 (9.0) | <0.001 |
Dysfunctional acquired organ systems | |||
Cardiovascular | 149 (49.3) | 204 (87.6) | <0.001 |
Respiratory | 141 (46.7) | 202 (86.7) | <0.001 |
Renal | 145 (48.0) | 136 (58.4) | 0.017 |
Hepatic | 13 (4.3) | 27 (11.6) | 0.001 |
Hematologic | 103 (34.1) | 63 (27.0) | 0.080 |
Neurologic | 11 (3.6) | 19 (8.2) | 0.024 |
2 Dysfunctional acquired organ systems | 164 (54.3) | 213 (91.4) | <0.001 |
Source of bloodstream infection | |||
Lungs | 95 (31.5) | 127 (54.5) | <0.001 |
Urinary tract | 92 (30.5) | 45 (19.3) | |
Central venous catheter | 30 (9.9) | 16 (6.9) | |
Intra‐abdominal | 63 (20.9) | 33 (14.2) | |
Unknown | 22 (7.3) | 12 (5.2) | |
Prior antibiotics* | 103 (34.1) | 110 (47.2) | 0.002 |
Results
Patient Characteristics
Included in the study were 535 consecutive patients with severe sepsis attributed to Pseudomonas aeruginosa, Acinetobacter species, or Enterobacteriaceae bacteremia, of whom 233 (43.6%) died during their hospitalization. The mean age was 58.9 16.0 years (range, 18 to 96 years) with 288 (53.8%) males and 247 (46.2%) females. The infection sources included community‐acquired (n = 46, 8.6%), healthcare‐associated community‐onset (n = 187, 35.0%), and healthcare‐associated hospital‐onset (n = 302, 56.4%). Hospital nonsurvivors were statistically more likely to have a healthcare‐associated hospital‐onset infection, congestive heart failure, chronic obstructive pulmonary disease, chronic kidney disease, ICU admission, need for mechanical ventilation and/or vasopressors, administration of drotrecogin alfa (activated), prior antibiotic administration, the lungs as the source of infection, acquired dysfunction of the cardiovascular, respiratory, renal, hepatic, and neurologic organ systems, and greater APACHE II and Charlson co‐morbidity scores compared to hospital survivors (Table 1). Hospital nonsurvivors were also statistically less likely to have a healthcare‐associated community‐onset infection and a urinary source of infection compared to hospital survivors (Table 1).
Microbiology
Among the 547 Gram‐negative bacteria isolated from blood, the most common were Enterobacteriaceae (Escherichia coli, Klebsiella species, Enterobacter species) (70.2%) followed by Pseudomonas aeruginosa (20.8%) and Acinetobacter species (9.0%) (Table 2). Nine patients had two different Enterobacteriaceae species isolated from their blood cultures, and three patients had an Enterobacteriaceae species and Pseudomonas aeruginosa isolated from their blood cultures. Hospital nonsurvivors were statistically more likely to be infected with Pseudomonas aeruginosa and less likely to be infected with Enterobacteriaceae. The pathogen‐specific hospital mortality rate was significantly greater for Pseudomonas aeruginosa and Acinetobacter species compared to Enterobacteriaceae (P < 0.001 and P = 0.008, respectively).
Bacteria | Hospital Survivors (n = 302) | Hospital Nonsurvivors (n = 233) | P value* | Percent Resistant | Pathogen‐ Specific Mortality Rate |
---|---|---|---|---|---|
| |||||
Enterobacteriaceae | 241 (79.8) | 143 (61.4) | <0.001 | 9.1 | 37.2 |
Pseudomonas aeruginosa | 47 (15.6) | 67 (28.8) | <0.001 | 16.7 | 58.8 |
Acinetobacter species | 22 (7.3) | 27 (11.6) | 0.087 | 71.4 | 55.1 |
Antimicrobial Treatment and Resistance
Among the study patients, 358 (66.9%) received cefepime, 102 (19.1%) received piperacillin‐tazobactam, and 75 (14.0%) received a carbapenem (meropenem or imipenem) as their initial antibiotic treatment. There were 169 (31.6%) patients who received initial combination therapy with either an aminoglycoside (n = 99, 58.6%) or ciprofloxacin (n = 70, 41.4%). Eighty‐two (15.3%) patients were infected with a pathogen that was resistant to the initial antibiotic treatment regimen [cefepime (n = 41; 50.0%), piperacillin‐tazobactam (n = 25; 30.5%), or imipenem/meropenem (n = 16; 19.5%), plus either an aminoglycoside or ciprofloxacin (n = 28; 34.1%)], and were classified as receiving inappropriate initial antibiotic therapy. Among the 453 (84.7%) patients infected with a pathogen that was susceptible to the initial antibiotic regimen, there was no relationship identified between minimum inhibitory concentration values and hospital mortality.
Patients infected with a pathogen resistant to the initial antibiotic regimen had significantly greater risk of hospital mortality (63.4% vs 40.0%; P < 0.001) (Figure 1). For the 82 individuals infected with a pathogen that was resistant to the initial antibiotic regimen, no difference in hospital mortality was observed among those prescribed initial combination treatment with an aminoglycoside (n = 17) (64.7% vs 61.1%; P = 0.790) or ciprofloxacin (n = 11) (72.7% vs 61.1%; P = 0.733) compared to monotherapy (n = 54). Similarly, among the patients infected with a pathogen that was susceptible to the initial antibiotic regimen, there was no difference in hospital mortality among those whose bloodstream isolate was only susceptible to the prescribed aminoglycoside (n = 12) compared to patients with isolates that were susceptible to the prescribed beta‐lactam antibiotic (n = 441) (41.7% vs 39.9%; P = 0.902).
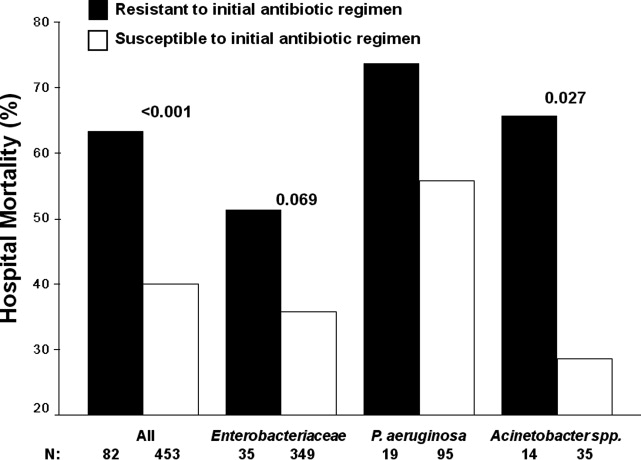
Logistic regression analysis identified infection with a pathogen resistant to the initial antibiotic regimen [adjusted odds ratio (AOR), 2.28; 95% confidence interval (CI), 1.69‐3.08; P = 0.006], increasing APACHE II scores (1‐point increments) (AOR, 1.13; 95% CI, 1.10‐1.15; P < 0.001), the need for vasopressors (AOR, 2.57; 95% CI, 2.15‐3.53; P < 0.001), the need for mechanical ventilation (AOR, 2.54; 95% CI, 2.19‐3.47; P < 0.001), healthcare‐associated hospital‐onset infection (AOR, 1.67; 95% CI, 1.32‐2.10; P =0.027), and infection with Pseudomonas aeruginosa (AOR, 2.21; 95% CI, 1.74‐2.86; P =0.002) as independent risk factors for hospital mortality (Hosmer‐Lemeshow goodness‐of‐fit test = 0.305). The model explained between 29.7% (Cox and Snell R square) and 39.8% (Nagelkerke R squared) of the variance in hospital mortality, and correctly classified 75.3% of cases.
Secondary Outcomes
Two or more acquired organ system derangements occurred significantly more often among patients with a pathogen resistant to the initial antibiotic regimen compared to those infected with susceptible isolates (84.1% vs 68.0%; P = 0.003). Hospital length of stay was significantly longer for patients infected with a pathogen resistant to the initial antibiotic regimen compared to those infected with susceptible isolates [39.9 50.6 days (median 27 days; quartiles 12 days and 45.5 days) vs 21.6 22.0 days (median 15 days; quartiles 7 days and 30 days); P < 0.001].
Discussion
Our study demonstrated that hospital nonsurvivors with severe sepsis attributed to Gram‐negative bacteremia had significantly greater rates of resistance to their initially prescribed antibiotic regimen compared to hospital survivors. This observation was confirmed in a multivariate analysis controlling for severity of illness and other potential confounding variables. Additionally, acquired organ system derangements and hospital length of stay were greater for patients infected with Gram‐negative pathogens resistant to the empiric antibiotic regimen. We also observed no survival advantage with the use of combination antimicrobial therapy for the subgroup of patients whose pathogens were resistant to the initially prescribed antibiotic regimen. Lastly, no difference in mortality was observed for patients with bacterial isolates that were susceptible only to the prescribed aminoglycoside compared to those with isolates susceptible to the prescribed beta‐lactam antibiotic.
Several previous investigators have linked antibiotic resistance and outcome in patients with serious infections attributed to Gram‐negative bacteria. Tam et al. examined 34 patients with Pseudomonas aeruginosa bacteremia having elevated MICs to piperacillin‐tazobactam (32 g/mL) that were reported as susceptible.19 In seven of these cases, piperacillin‐tazobactam was prescribed empirically, whereas other agents directed against Gram‐negative bacteria were employed in the other patients (carbapenems, aminoglycosides). Thirty‐day mortality was significantly greater for the patients treated with piperacillin‐tazobactam (85.7% vs 22.2%; P = 0.004), and a multivariate analysis found treatment with piperacillin‐tazobactam to be independently associated with 30‐day mortality. Similarly, Bhat et al. examined 204 episodes of bacteremia caused by Gram‐negative bacteria for which patients received cefepime.20 Patients infected with a Gram‐negative bacteria having an MIC to cefepime greater than, or equal to, 8 g/mL had a significantly greater 28‐day mortality compared to patients infected with isolates having an MIC to cefepime that was less than 8 g/mL (54.8% vs 24.1%; P = 0.001).
Our findings are consistent with earlier studies of patients with serious Gram‐negative infections including bacteremia and nosocomial pneumonia. Micek et al. showed that patients with Pseudomonas aeruginosa bacteremia who received inappropriate initial antimicrobial therapy had a greater risk of hospital mortality compared to patients initially treated with an antimicrobial regimen having activity for the Pseudomonas isolate based on in vitro susceptibility testing.21 Similarly, Trouillet et al.,22 Beardsley et al.,23 and Heyland et al.24 found that combination antimicrobial regimens directed against Gram‐negative bacteria in patients with nosocomial pneumonia were more likely to be appropriate based on the antimicrobial susceptibility patterns of the organisms compared to monotherapy. In a more recent study, Micek et al. demonstrated that combination antimicrobial therapy directed against severe sepsis attributed to Gram‐negative bacteria was associated with improved outcomes compared to monotherapy, especially when the combination agent was an aminoglycoside.25 However, empiric combination therapy that included an aminoglycoside was also associated with increased nephrotoxicity which makes the empiric use of aminoglycosides in all patients with suspected Gram‐negative severe sepsis problematic.25, 26 Nevertheless, the use of combination therapy represents a potential strategy to maximize the administration of appropriate treatment for serious Gram‐negative bacterial infections.
Rapid assessment of antimicrobial susceptibility is another strategy that offers the possibility of identifying the resistance pattern of Gram‐negative pathogens quickly in order to provide more appropriate treatment. Bouza et al. found that use of a rapid E‐test on the respiratory specimens of patients with ventilator‐associated pneumonia was associated with fewer days of fever, fewer days of antibiotic administration until resolution of the episode of ventilator‐associated pneumonia, decreased antibiotic consumption, less Clostridium difficile‐associated diarrhea, lower costs of antimicrobial agents, and fewer days receiving mechanical ventilation.27 Other methods for the rapid identification of resistant bacteria include real‐time polymerase chain reaction assays based on hybridization probes to identify specific resistance mechanisms in bacteria.28 Application of such methods for identification of broad categories of resistance mechanisms in Gram‐negative bacteria offer the possibility of tailoring initial antimicrobial regimens in order to provide appropriate therapy in a more timely manner.
Our study has several important limitations that should be noted. First, the study was performed at a single center and the results may not be generalizable to other institutions. However, the findings from other investigators corroborate the importance of antimicrobial resistance as a predictor of outcome for patients with serious Gram‐negative infections.19, 20 Additionally, a similar association has been observed in patients with methicillin‐resistant Staphylococcus aureus bacteremia, supporting the more general importance of antimicrobial resistance as an outcome predictor.29 Second, the method employed for determining MICs was a literature‐based linear regression method correlating disk diffusion diameters with broth dilution MIC determinations. Therefore, the lack of correlation we observed between MIC values and outcome for susceptible Gram‐negative isolates associated with severe sepsis requires further confirmation. Third, we only examined 3 antibiotics, or antibiotic classes, so our results may not be applicable to other agents. This also applies to doripenem, as we did not have that specific carbapenem available at the time this investigation took place.
Another important limitation of our study is the relatively small number of individuals infected with a pathogen that was resistant to the initial treatment regimen, or only susceptible to the aminoglycoside when combination therapy was prescribed. This limited our ability to detect meaningful associations in these subgroups of patients, to include whether or not combination therapy influenced their clinical outcome. Finally, we did not examine the exact timing of antibiotic therapy relative to the onset of severe sepsis. Instead we used a 12‐hour window from when subsequently positive blood cultures were drawn to the administration of initial antibiotic therapy. Other investigators have shown that delays in initial appropriate therapy of more than one hour for patients with septic shock increases the risk of death.9, 30 Failure to include the exact timing of therapy could have resulted in a final multivariate model that includes prediction variables that would not otherwise have been incorporated.
In summary, we demonstrated that resistance to the initial antibiotic treatment regimen was associated with a greater risk of hospital mortality in patients with severe sepsis attributed to Gram‐negative bacteremia. These findings imply that more rapid assessment of antimicrobial susceptibility could result in improved prescription of antibiotics in order to maximize initial administration of appropriate therapy. Future studies are required to address whether rapid determination of antimicrobial susceptibility can result in more effective administration of appropriate therapy, and if this can result in improved patient outcomes.
- Inadequate antimicrobial treatment of infections: a risk factor for hospital mortality among critically ill patients.Chest.1999;115:462–474. , , , .
- The clinical evaluation committee in a large multicenter phase 3 trial of drotrecogin alfa (activated) in patients with severe sepsis (PROWESS): role, methodology, and results.Crit Care Med.2003;31:2291–2301. , , , et al.
- Impact of adequate empical antibiotic therapy on the outcome of patients admitted to the intensive care unit with sepsis.Crit Care Med.2003;31:2742–2751. , , , , , .
- Inappropriate initial antimicrobial therapy and its effect on survival in a clinical trial of immunomodulating therapy for severe sepsis.Am J Med.2003;115:529–535. , , , , , .
- Antibiotic‐resistant bugs in the 21st century—a clinical super‐challenge.N Engl J Med.2009;360:439–443. , .
- Bad bugs, no drugs: no ESKAPE! An update from the Infectious Diseases Society of America.Clin Infect Dis.2009;48:1–12. , , , et al.
- Broad‐spectrum antimicrobials and the treatment of serious bacterial infections: getting it right up front.Clin Infect Dis.2008;47:S3–S13. .
- Bundled care for septic shock: an analysis of clinical trials.Crit Care Med.2010;38:668–678. , , , et al.
- Effectiveness of treatments for severe sepsis: a prospective, multicenter, observational study.Am J Respir Crit Care Med.2009;180:861–866. , , , et al.
- Surviving Sepsis Campaign: international guidelines for management of severe sepsis and septic shock: 2008.Crit Care Med.2008;36:296–327. , , , et al.
- APACHE II: a severity of disease classification system.Crit Care Med.1985;13:818–829. , , , .
- Invasive methicillin‐resistant Staphylococcus aureus infections in the United States.JAMA.2007;298:1763–1771. , , , et al.
- Epidemiology of severe sepsis in the United States: analysis of incidence, outcome, and associated costs of care.Crit Care Med.2001;29:1303–1310. , , , , , .
- Hospital‐wide impact of a standardized order set for the management of bacteremic severe sepsis.Crit Care Med.2009;37:819–824. , , , , , .
- Before‐after study of a standardized hospital order set for the management of septic shock.Crit Care Med.2007;34:2707–2713. , , , et al.
- National Committee for Clinical Laboratory Standards.Performance Standards for Antimicrobial Susceptibility Testing: Twelfth Informational Supplement. M100‐S12.Wayne, PA:National Committee for Clinical Laboratory Standards;2002.
- Clinical Laboratory Standards Institute.Performance Standards for Antimicrobial Susceptibility Testing: Seventeenth Informational Supplement. M100‐S17.Wayne, PA:Clinical Laboratory Standards Institute;2007.
- Evaluation of the BIOGRAM antimicrobial susceptibility test system.J Clin Microbiol.1985;22:793–798. , , , et al.
- Outcomes of bacteremia due to Pseudomonas aeruginosa with reduced susceptibility to piperacillin‐tazobactam: implications on the appropriateness of the resistance breakpoint.Clin Infect Dis.2008;46:862–867. , , , et al.
- Failure of current cefepime breakpoints to predict clinical outcomes of bacteremia caused by Gram‐negative organisms.Antimicrob Agents Chemother.2007;51:4390–4395. , , , et al.
- Pseudomonas aeruginosa bloodstream infection: importance of appropriate initial antimicrobial treatment.Antimicrob Agents Chemother.2005;49:1306–1311. , , , , , .
- Ventilator‐associated pneumonia caused by potentially drug‐resistant bacteria.Am J Respir Crit Care Med.1998;157:531–539. , , .
- Using local microbiologic data to develop institution‐specific guidelines for the treatment of hospital‐acquired pneumonia.Chest.2006;130:787–793. , , , , , .
- Randomized trial of combination versus monotherapy for the empiric treatment of suspected ventilator‐associated pneumonia.Crit Care Med.2008;36:737–744. , , , et al.
- Empiric combination antibiotic therapy is associated with improved outcome in Gram‐negative sepsis: a retrospective analysis.Antimicrob Agents Chemother.2010;54:1742–1748. , , , et al.
- Monotherapy versus beta‐lactam‐aminoglycoside combination treatment for Gram‐negative bacteremia: a prospective, observational study.Antimicrob Agents Chemother.1997;41:1127–1133. , , , et al.
- Direct E‐test (AB Biodisk) of respiratory samples improves antimicrobial use in ventilator‐associated pneumonia.Clin Infect Dis.2007;44:382–387. , , , et al.
- Rapid detection of CTX‐M‐producing Enterobacteriaceae in urine samples.J Antimicrob Chemother.2009;64:986–989. , , , et al.
- Influence of vancomycin minimum inhibitory concentration on the treatment of methicillin‐resistant Staphylococcus aureus bacteremia.Clin Infect Dis.2008;46:193–200. , , , et al.
- Duration of hypotension before initiation of effective antimicrobial therapy is the critical determinant of survival in human septic shock.Crit Care Med.2006;34:1589–1596. , , , et al.
- Inadequate antimicrobial treatment of infections: a risk factor for hospital mortality among critically ill patients.Chest.1999;115:462–474. , , , .
- The clinical evaluation committee in a large multicenter phase 3 trial of drotrecogin alfa (activated) in patients with severe sepsis (PROWESS): role, methodology, and results.Crit Care Med.2003;31:2291–2301. , , , et al.
- Impact of adequate empical antibiotic therapy on the outcome of patients admitted to the intensive care unit with sepsis.Crit Care Med.2003;31:2742–2751. , , , , , .
- Inappropriate initial antimicrobial therapy and its effect on survival in a clinical trial of immunomodulating therapy for severe sepsis.Am J Med.2003;115:529–535. , , , , , .
- Antibiotic‐resistant bugs in the 21st century—a clinical super‐challenge.N Engl J Med.2009;360:439–443. , .
- Bad bugs, no drugs: no ESKAPE! An update from the Infectious Diseases Society of America.Clin Infect Dis.2009;48:1–12. , , , et al.
- Broad‐spectrum antimicrobials and the treatment of serious bacterial infections: getting it right up front.Clin Infect Dis.2008;47:S3–S13. .
- Bundled care for septic shock: an analysis of clinical trials.Crit Care Med.2010;38:668–678. , , , et al.
- Effectiveness of treatments for severe sepsis: a prospective, multicenter, observational study.Am J Respir Crit Care Med.2009;180:861–866. , , , et al.
- Surviving Sepsis Campaign: international guidelines for management of severe sepsis and septic shock: 2008.Crit Care Med.2008;36:296–327. , , , et al.
- APACHE II: a severity of disease classification system.Crit Care Med.1985;13:818–829. , , , .
- Invasive methicillin‐resistant Staphylococcus aureus infections in the United States.JAMA.2007;298:1763–1771. , , , et al.
- Epidemiology of severe sepsis in the United States: analysis of incidence, outcome, and associated costs of care.Crit Care Med.2001;29:1303–1310. , , , , , .
- Hospital‐wide impact of a standardized order set for the management of bacteremic severe sepsis.Crit Care Med.2009;37:819–824. , , , , , .
- Before‐after study of a standardized hospital order set for the management of septic shock.Crit Care Med.2007;34:2707–2713. , , , et al.
- National Committee for Clinical Laboratory Standards.Performance Standards for Antimicrobial Susceptibility Testing: Twelfth Informational Supplement. M100‐S12.Wayne, PA:National Committee for Clinical Laboratory Standards;2002.
- Clinical Laboratory Standards Institute.Performance Standards for Antimicrobial Susceptibility Testing: Seventeenth Informational Supplement. M100‐S17.Wayne, PA:Clinical Laboratory Standards Institute;2007.
- Evaluation of the BIOGRAM antimicrobial susceptibility test system.J Clin Microbiol.1985;22:793–798. , , , et al.
- Outcomes of bacteremia due to Pseudomonas aeruginosa with reduced susceptibility to piperacillin‐tazobactam: implications on the appropriateness of the resistance breakpoint.Clin Infect Dis.2008;46:862–867. , , , et al.
- Failure of current cefepime breakpoints to predict clinical outcomes of bacteremia caused by Gram‐negative organisms.Antimicrob Agents Chemother.2007;51:4390–4395. , , , et al.
- Pseudomonas aeruginosa bloodstream infection: importance of appropriate initial antimicrobial treatment.Antimicrob Agents Chemother.2005;49:1306–1311. , , , , , .
- Ventilator‐associated pneumonia caused by potentially drug‐resistant bacteria.Am J Respir Crit Care Med.1998;157:531–539. , , .
- Using local microbiologic data to develop institution‐specific guidelines for the treatment of hospital‐acquired pneumonia.Chest.2006;130:787–793. , , , , , .
- Randomized trial of combination versus monotherapy for the empiric treatment of suspected ventilator‐associated pneumonia.Crit Care Med.2008;36:737–744. , , , et al.
- Empiric combination antibiotic therapy is associated with improved outcome in Gram‐negative sepsis: a retrospective analysis.Antimicrob Agents Chemother.2010;54:1742–1748. , , , et al.
- Monotherapy versus beta‐lactam‐aminoglycoside combination treatment for Gram‐negative bacteremia: a prospective, observational study.Antimicrob Agents Chemother.1997;41:1127–1133. , , , et al.
- Direct E‐test (AB Biodisk) of respiratory samples improves antimicrobial use in ventilator‐associated pneumonia.Clin Infect Dis.2007;44:382–387. , , , et al.
- Rapid detection of CTX‐M‐producing Enterobacteriaceae in urine samples.J Antimicrob Chemother.2009;64:986–989. , , , et al.
- Influence of vancomycin minimum inhibitory concentration on the treatment of methicillin‐resistant Staphylococcus aureus bacteremia.Clin Infect Dis.2008;46:193–200. , , , et al.
- Duration of hypotension before initiation of effective antimicrobial therapy is the critical determinant of survival in human septic shock.Crit Care Med.2006;34:1589–1596. , , , et al.
Copyright © 2011 Society of Hospital Medicine
Inappropriate Treatment of HCA‐cSSTI
Classically, infections have been categorized as either community‐acquired (CAI) or nosocomial in origin. Until recently, this scheme was thought adequate to capture the differences in the microbiology and outcomes in the corresponding scenarios. However, recent evidence suggests that this distinction may no longer be valid. For example, with the spread and diffusion of healthcare delivery beyond the confines of the hospital along with the increasing use of broad spectrum antibiotics both in and out of the hospital, pathogens such as methicillin‐resistant Staphylococcus aureus (MRSA) and Pseudomonas aeruginosa (PA), traditionally thought to be confined to the hospital, are now seen in patients presenting from the community to the emergency department (ED).1, 2 Reflecting this shift in epidemiology, some national guidelines now recognize healthcare‐associated infection (HCAI) as a distinct entity.3 The concept of HCAI allows the clinician to identify patients who, despite suffering a community onset infection, still may be at risk for a resistant bacterial pathogen. Recent studies in both bloodstream infection and pneumonia have clearly demonstrated that those with HCAI have distinct microbiology and outcomes relative to those with pure CAI.47
Most work focusing on establishing HCAI has not addressed skin and soft tissue infections. These infections, although not often fatal, account for an increasing number of admissions to the hospital.8, 9 In addition, they may be associated with substantial morbidity and cost.8 Given that many pathogens such as S. aureus, which may be resistant to typical antimicrobials used in the ED, are also major culprits in complicated skin and skin structure infections (cSSSI), the HCAI paradigm may apply in cSSSI. Furthermore, because of these patterns of increased resistance, HCA‐cSSSI patients, similar to other HCAI groups, may be at an increased risk of being treated with initially inappropriate antibiotic therapy.7, 10
Since in the setting of other types of infection inappropriate empiric treatment has been shown to be associated with increased mortality and costs,7, 1015 and since indirect evidence suggests a similar impact on healthcare utilization among cSSSI patients,8 we hypothesized that among a cohort of patients hospitalized with a cSSSI, the initial empiric choice of therapy is independently associated with hospital length of stay (LOS). We performed a retrospective cohort study to address this question.
Methods
Study Design
We performed a single‐center retrospective cohort study of patients with cSSSI admitted to the hospital through the ED. All consecutive patients hospitalized between April 2006 and December 2007 meeting predefined inclusion criteria (see below) were enrolled. The study was approved by the Washington University School of Medicine Human Studies Committee, and informed consent was waived. We have previously reported on the characteristics and outcomes of this cohort, including both community‐acquired and HCA‐cSSSI patients.16
Study Cohort
All consecutive patients admitted from the community through the ED between April 2006 and December 2007 at the Barnes‐Jewish Hospital, a 1200‐bed university‐affiliated, urban teaching hospital in St. Louis, MO were included if: (1) they had a diagnosis of a predefined cSSSI (see Appendix Table A1, based on reference 8) and (2) they had a positive microbiology culture obtained within 24 hours of hospital admission. Similar to the work by Edelsberg et al.8 we excluded patients if certain diagnoses and procedures were present (Appendix Table A2). Cases were also excluded if they represented a readmission for the same diagnosis within 30 days of the original hospitalization.
Definitions
HCAI was defined as any cSSSI in a patient with a history of recent hospitalization (within the previous year, consistent with the previous study16), receiving antibiotics prior to admission (previous 90 days), transferring from a nursing home, or needing chronic dialysis. We defined a polymicrobial infection as one with more than one organism, and mixed infection as an infection with both a gram‐positive and a gram‐negative organism. Inappropriate empiric therapy took place if a patient did not receive treatment within 24 hours of the time the culture was obtained with an agent exhibiting in vitro activity against the isolated pathogen(s). In mixed infections, appropriate therapy was treatment within 24 hours of culture being obtained with agent(s) active against all pathogens recovered.
Data Elements
We collected information about multiple baseline demographic and clinical factors including: age, gender, race/ethnicity, comorbidities, the presence of risk factors for HCAI, the presence of bacteremia at admission, and the location of admission (ward vs. intensive care unit [ICU]). Bacteriology data included information on specific bacterium/a recovered from culture, the site of the culture (eg, tissue, blood), susceptibility patterns, and whether the infection was monomicrobial, polymicrobial, or mixed. When blood culture was available and positive, we prioritized this over wound and other cultures and designated the corresponding organism as the culprit in the index infection. Cultures growing our coagulase‐negative S. aureus were excluded as a probable contaminant. Treatment data included information on the choice of the antimicrobial therapy and the timing of its institution relative to the timing of obtaining the culture specimen. The presence of such procedures as incision and drainage (I&D) or debridement was recorded.
Statistical Analyses
Descriptive statistics comparing HCAI patients treated appropriately to those receiving inappropriate empiric coverage based on their clinical, demographic, microbiologic and treatment characteristics were computed. Hospital LOS served as the primary and hospital mortality as the secondary outcomes, comparing patients with HCAI treated appropriately to those treated inappropriately. All continuous variables were compared using Student's t test or the Mann‐Whitney U test as appropriate. All categorical variables were compared using the chi‐square test or Fisher's exact test. To assess the attributable impact of inappropriate therapy in HCAI on the outcomes of interest, general linear models with log transformation were developed to model hospital LOS parameters; all means are presented as geometric means. All potential risk factors significant at the 0.1 level in univariate analyses were entered into the model. All calculations were performed in Stata version 9 (Statacorp, College Station, TX).
Results
Of the 717 patients with culture‐positive cSSSI admitted during the study period, 527 (73.5%) were classified as HCAI. The most common reason for classification as an HCAI was recent hospitalization. Among those with an HCA‐cSSSI, 405 (76.9%) received appropriate empiric treatment, with nearly one‐quarter receiving inappropriate initial coverage. Those receiving inappropriate antibiotic were more likely to be African American, and had a higher likelihood of having end‐stage renal disease (ESRD) than those with appropriate coverage (Table 1). While those patients treated appropriately had higher rates of both cellulitis and abscess as the presenting infection, a substantially higher proportion of those receiving inappropriate initial treatment had a decubitus ulcer (29.5% vs. 10.9%, P <0.001), a device‐associated infection (42.6% vs. 28.6%, P = 0.004), and had evidence of bacteremia (68.9% vs. 57.8%, P = 0.028) than those receiving appropriate empiric coverage (Table 2).
Inappropriate (n = 122), n (%) | Appropriate (n = 405), n (%) | P Value | |
---|---|---|---|
| |||
Age, years | 56.3 18.0 | 53.6 16.7 | 0.147 |
Gender (F) | 62 (50.8) | 190 (46.9) | 0.449 |
Race | |||
Caucasian | 51 (41.8) | 219 (54.1) | 0.048 |
African American | 68 (55.7) | 178 (43.9) | |
Other | 3 (2.5) | 8 (2.0) | |
HCAI risk factors | |||
Recent hospitalization* | 110 (90.2) | 373 (92.1) | 0.498 |
Within 90 days | 98 (80.3) | 274 (67.7) | 0.007 |
>90 and 180 days | 52 (42.6) | 170 (42.0) | 0.899 |
>180 days and 1 year | 46 (37.7) | 164 (40.5) | 0.581 |
Prior antibiotics | 26 (21.3) | 90 (22.2) | 0.831 |
Nursing home resident | 29 (23.8) | 54 (13.3) | 0.006 |
Hemodialysis | 19 (15.6) | 39 (9.7) | 0.067 |
Comorbidities | |||
DM | 40 (37.8) | 128 (31.6) | 0.806 |
PVD | 5 (4.1) | 15 (3.7) | 0.841 |
Liver disease | 6 (4.9) | 33 (8.2) | 0.232 |
Cancer | 21 (17.2) | 85 (21.0) | 0.362 |
HIV | 1 (0.8) | 12 (3.0) | 0.316 |
Organ transplant | 2 (1.6) | 8 (2.0) | 1.000 |
Autoimmune disease | 5 (4.1) | 8 (2.0) | 0.185 |
ESRD | 22 (18.0) | 46 (11.4) | 0.054 |
Inappropriate (n = 122), n (%) | Appropriate (n = 405), n (%) | P Value | |
---|---|---|---|
| |||
Cellulitis | 28 (23.0) | 171 (42.2) | <0.001 |
Decubitus ulcer | 36 (29.5) | 44 (10.9) | <0.001 |
Post‐op wound | 25 (20.5) | 75 (18.5) | 0.626 |
Device‐associated infection | 52 (42.6) | 116 (28.6) | 0.004 |
Diabetic foot ulcer | 9 (7.4) | 24 (5.9) | 0.562 |
Abscess | 22 (18.0) | 108 (26.7) | 0.052 |
Other* | 2 (1.6) | 17 (4.2) | 0.269 |
Presence of bacteremia | 84 (68.9) | 234 (57.8) | 0.028 |
The pathogens recovered from the appropriately and inappropriately treated groups are listed in Figure 1. While S. aureus overall was more common among those treated appropriately, the frequency of MRSA did not differ between the groups. Both E. faecalis and E. faecium were recovered more frequently in the inappropriate group, resulting in a similar pattern among the vancomycin‐resistant enterococcal species. Likewise, P. aeruginosa, P. mirabilis, and A. baumannii were all more frequently seen in the group treated inappropriately than in the group getting appropriate empiric coverage. A mixed infection was also more likely to be present among those not exposed (16.5%) than among those exposed (7.5%) to appropriate early therapy (P = 0.001) (Figure 1).
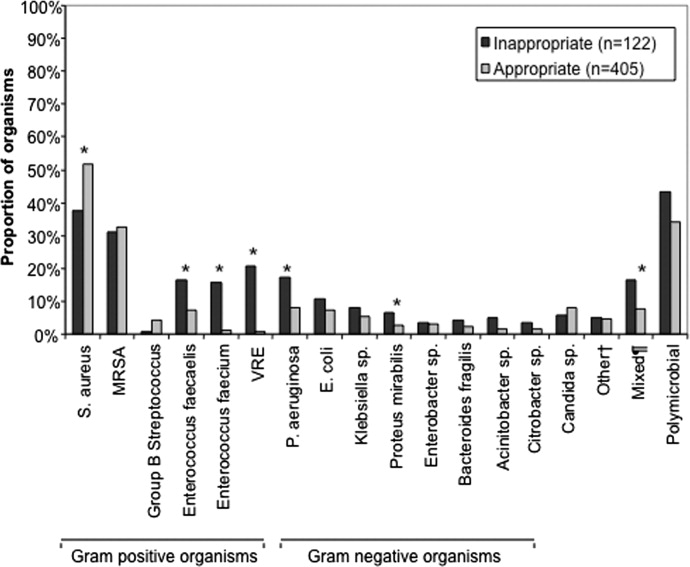
In terms of processes of care and outcomes (Table 3), commensurate with the higher prevalence of abscess in the appropriately treated group, the rate of I&D was significantly higher in this cohort (36.8%) than in the inappropriately treated (23.0%) group (P = 0.005). Need for initial ICU care did not differ as a function of appropriateness of therapy (P = 0.635).
Inappropriate (n = 122) | Appropriate (n = 405) | P Value | |
---|---|---|---|
| |||
I&D/debridement | 28 (23.0%) | 149 (36.8%) | 0.005 |
I&D in ED | 0 | 7 (1.7) | 0.361 |
ICU | 9 (7.4%) | 25 (6.2%) | 0.635 |
Hospital LOS, days | |||
Median (IQR 25, 75) [Range] | 7.0 (4.2, 13.6) [0.686.6] | 6 (3.3, 10.1) [0.748.3] | 0.026 |
Hospital mortality | 9 (7.4%) | 26 (6.4%) | 0.710 |
The unadjusted mortality rate was low overall and did not vary based on initial treatment (Table 3). In a generalized linear model with the log‐transformed LOS as the dependent variable, adjusting for multiple potential confounders, initial inappropriate antibiotic therapy had an attributable incremental increase in the hospital LOS of 1.8 days (95% CI, 1.42.3) (Table 4).
Factor | Attributable LOS (days) | 95% CI | P Value |
---|---|---|---|
| |||
Infection type: device | 3.6 | 2.74.8 | <0.001 |
Infection type: decubitus ulcer | 3.3 | 2.64.2 | <0.001 |
Infection type: abscess | 2.5 | 1.64.0 | <0.001 |
Organism: P. mirabilis | 2.2 | 1.43.4 | <0.001 |
Organism: E. faecalis | 2.1 | 1.72.6 | <0.001 |
Nursing home resident | 2.1 | 1.62.6 | <0.001 |
Inappropriate antibiotic | 1.8 | 1.42.3 | <0.001 |
Race: Non‐Caucasian | 0.31 | 0.240.41 | <0.001 |
Organism: E. faecium | 0.23 | 0.150.35 | <0.001 |
Because bacteremia is known to be an effect modifier of the relationship between the empiric choice of antibiotic and infection outcomes, we further explored its role in the HCAI cSSSI on the outcomes of interest (Table 5). Similar to the effect detected in the overall cohort, treatment with inappropriate therapy was associated with an increase in the hospital LOS, but not hospital mortality in those with bacteremia, though this phenomenon was observed only among patients with secondary bacteremia, and not among those without (Table 5).
Bacteremia Present (n = 318) | Bacteremia Absent (n = 209) | |||||
---|---|---|---|---|---|---|
I (n = 84) | A (n = 234) | P Value | I (n = 38) | A (n = 171) | P Value | |
| ||||||
Hospital LOS, days | ||||||
Mean SD | 14.4 27.5 | 9.8 9.7 | 0.041 | 6.6 6.8 | 6.9 8.2 | 0.761 |
Median (IQR 25, 75) | 8.8 (5.4, 13.9) | 7.0 (4.3, 11.7) | 4.4 (2.4, 7.7) | 3.9 (2.0, 8.2) | ||
Hospital mortality | 8 (9.5%) | 24 (10.3%) | 0.848 | 1 (2.6%) | 2 (1.2%) | 0.454 |
Discussion
This retrospective analysis provides evidence that inappropriate empiric antibiotic therapy for HCA‐cSSSI independently prolongs hospital LOS. The impact of inappropriate initial treatment on LOS is independent of many important confounders. In addition, we observed that this effect, while present among patients with secondary bacteremia, is absent among those without a blood stream infection.
To the best of our knowledge, ours is the first cohort study to examine the outcomes associated with inappropriate treatment of a HCAI cSSSI within the context of available microbiology data. Edelsberg et al.8 examined clinical and economic outcomes associated with the failure of the initial treatment of cSSSI. While not specifically focusing on HCAI patients, these authors noted an overall 23% initial therapy failure rate. Among those patients who failed initial therapy, the risk of hospital death was nearly 3‐fold higher (adjusted odds ratio [OR], 2.91; 95% CI, 2.343.62), and they incurred the mean of 5.4 additional hospital days, compared to patients treated successfully with the initial regimen.8 Our study confirms Edelsberg et al.'s8 observation of prolonged hospital LOS in association with treatment failure, and builds upon it by defining the actual LOS increment attributable to inappropriate empiric therapy. It is worth noting that the study by Edelsberg et al.,8 however, lacked explicit definition of the HCAI population and microbiology data, and used treatment failure as a surrogate marker for inappropriate treatment. It is likely these differences between our two studies in the underlying population and exposure definitions that account for the differences in the mortality data between that study and ours.
It is not fundamentally surprising that early exposure to inappropriate empiric therapy alters healthcare resource utilization outcomes for the worse. Others have demonstrated that infection with a resistant organism results in prolongation of hospital LOS and costs. For example, in a large cohort of over 600 surgical hospitalizations requiring treatment for a gram‐negative infection, antibiotic resistance was an independent predictor of increased LOS and costs.15 These authors quantified the incremental burden of early gram‐negative resistance at over $11,000 in hospital costs.15 Unfortunately, the treatment differences for resistant and sensitive organisms were not examined.15 Similarly, Shorr et al. examined risk factors for prolonged hospital LOS and increased costs in a cohort of 291 patients with MRSA sterile site infection.17 Because in this study 23% of the patients received inappropriate empiric therapy, the authors were able to examine the impact of this exposure on utilization outcomes.17 In an adjusted analysis, inappropriate initial treatment was associated with an incremental increase in the LOS of 2.5 days, corresponding to the unadjusted cost differential of nearly $6,000.17 Although focusing on a different population, our results are consistent with these previous observations that antibiotic resistance and early inappropriate therapy affect hospital utilization parameters, in our case by adding nearly 2 days to the hospital LOS.
Our study has a number of limitations. First, as a retrospective cohort study it is prone to various forms of bias, most notably selection bias. To minimize the possibility of such, we established a priori case definitions and enrolled consecutive patients over a specific period of time. Second, as in any observational study, confounding is an issue. We dealt with this statistically by constructing a multivariable regression model; however, the possibility of residual confounding remains. Third, because some of the wound and ulcer cultures likely were obtained with a swab and thus represented colonization, rather than infection, we may have over‐estimated the rate of inappropriate therapy, and this needs to be followed up in future prospective studies. Similarly, we may have over‐estimated the likelihood of inappropriate therapy among polymicrobial and mixed infections as well, given that, for example, a gram‐negative organism may carry a different clinical significance when cultured from blood (infection) than when it is detected in a decubitus ulcer (potential colonization). Fourth, because we limited our cohort to patients without deep‐seated infections, such as necrotizing fasciitis, other procedures were not collected. This omission may have led to either over‐estimation or under‐estimation of the impact of inappropriate therapy on the outcomes of interest.
The fact that our cohort represents a single large urban academic tertiary care medical center may limit the generalizability of our results only to centers that share similar characteristics. Finally, similar to most other studies of this type, ours lacks data on posthospitalization outcomes and for this reason limits itself to hospital outcomes only.
In summary, we have shown that, similar to other populations with HCAI, a substantial proportion (nearly 1/4) of cSSSI patients with HCAI receive inappropriate empiric therapy for their infection, and this early exposure, though not affecting hospital mortality, is associated with a significant prolongation of the hospitalization by as much as 2 days. Studies are needed to refine decision rules for risk‐stratifying patients with cSSSI HCAI in order to determine the probability of infection with a resistant organism. In turn, such instruments at the bedside may assure improved utilization of appropriately targeted empiric therapy that will both optimize individual patient outcomes and reduce the risk of emergence of antimicrobial resistance.
Appendix
Principal diagnosis code | Description |
---|---|
680 | Carbuncle and furuncle |
681 | Cellulitis and abscess of finger and toe |
682 | Other cellulitis and abscess |
683 | Acute lymphadenitis |
685 | Pilonidal cyst with abscess |
686 | Other local infections of skin and subcutaneous tissue |
707 | Decubitus ulcer |
707.1 | Ulcers of lower limbs, except decubitus |
707.8 | Chronic ulcer of other specified sites |
707.9 | Chronic ulcer of unspecified site |
958.3 | Posttraumatic wound infection, not elsewhere classified |
996.62 | Infection due to other vascular device, implant, and graft |
997.62 | Infection (chronic) of amputation stump |
998.5 | Postoperative wound infection |
Diagnosis code | Description |
---|---|
728.86 | Necrotizing fasciitis |
785.4 | Gangrene |
686.09 | Ecthyma gangrenosum |
730.00730.2 | Osteomyelitis |
630677 | Complications of pregnancy, childbirth and puerperium |
288.0 | Neutropenia |
684 | Impetigo |
Procedure code | |
39.95 | Plasmapheresis |
99.71 | Hemoperfusion |
- Invasive methicillin‐resistant Staphylococcus aureus infections in the United States.JAMA.2007;298:1762–1771. , , , et al.
- Methicillin‐resistant S. aureus infections among patients in the emergency department.N Engl J Med.2006;17;355:666–674. , , , et al.
- Hospital‐Acquired Pneumonia Guideline Committee of the American Thoracic Society and Infectious Diseases Society of America.Guidelines for the management of adults with hospital‐acquired pneumonia, ventilator‐associated pneumonia, and healthcare‐associated pneumonia.Am J Respir Crit Care Med.2005;171:388–416.
- Epidemiology and outcomes of health‐care‐associated pneumonia: Results from a large US database of culture‐positive pneumonia.Chest.2005;128:3854–3862. , , , et al.
- Health care‐associated bloodstream infections in adults: A reason to change the accepted definition of community‐acquired infections.Ann Intern Med.2002;137:791–797. , , , et al.
- Healthcare‐associated bloodstream infection: A distinct entity? Insights from a large U.S. database.Crit Care Med.2006;34:2588–2595. , , , , , .
- Health care‐associated pneumonia and community‐acquired pneumonia: a single‐center experience.Antimicrob Agents Chemother.2007;51:3568–3573. , , , et al.
- Clinical and economic consequences of failure of initial antibiotic therapy for hospitalized patients with complicated skin and skin‐structure infections.Infect Control Hosp Epidemiol.2008;29:160–169. , , , , , .
- Skin, soft tissue, bone, and joint infections in hospitalized patients: Epidemiology and microbiological, clinical, and economic outcomes.Infect Control Hosp Epidemiol.2007;28:1290–1298. , , , et al.
- Methicillin‐resistant Staphylococcus aureus sterile‐site infection: The importance of appropriate initial antimicrobial treatment.Crit Care Med.2006;34:2069–2074. , , , et al.
- The influence of inadequate antimicrobial treatment of bloodstream infections on patient outcomes in the ICU setting.Chest.2000;118:146–155. , , , et al.
- Modification of empiric antibiotic treatment in patients with pneumonia acquired in the intensive care unit.Intensive Care Med.1996;22:387–394. , .
- Clinical importance of delays in the initiation of appropriate antibiotic treatment for ventilator‐associated pneumonia.Chest.2002;122:262–268. , , , et al.
- Antimicrobial therapy escalation and hospital mortality among patients with HCAP: A single center experience.Chest.2008;134:963–968. , , , , .
- Cost of gram‐negative resistance.Crit Care Med.2007;35:89–95. , , , et al.
- Epidemiology and outcomes of hospitalizations with complicated skin and skin‐structure infections: implications of healthcare‐associated infection risk factors.Infect Control Hosp Epidemiol.2009;30:1203–1210. , , , et al.
- Inappropriate therapy for methicillin‐resistant Staphylococcus aureus: resource utilization and cost implications.Crit Care Med.2008;36:2335–2340. , , .
Classically, infections have been categorized as either community‐acquired (CAI) or nosocomial in origin. Until recently, this scheme was thought adequate to capture the differences in the microbiology and outcomes in the corresponding scenarios. However, recent evidence suggests that this distinction may no longer be valid. For example, with the spread and diffusion of healthcare delivery beyond the confines of the hospital along with the increasing use of broad spectrum antibiotics both in and out of the hospital, pathogens such as methicillin‐resistant Staphylococcus aureus (MRSA) and Pseudomonas aeruginosa (PA), traditionally thought to be confined to the hospital, are now seen in patients presenting from the community to the emergency department (ED).1, 2 Reflecting this shift in epidemiology, some national guidelines now recognize healthcare‐associated infection (HCAI) as a distinct entity.3 The concept of HCAI allows the clinician to identify patients who, despite suffering a community onset infection, still may be at risk for a resistant bacterial pathogen. Recent studies in both bloodstream infection and pneumonia have clearly demonstrated that those with HCAI have distinct microbiology and outcomes relative to those with pure CAI.47
Most work focusing on establishing HCAI has not addressed skin and soft tissue infections. These infections, although not often fatal, account for an increasing number of admissions to the hospital.8, 9 In addition, they may be associated with substantial morbidity and cost.8 Given that many pathogens such as S. aureus, which may be resistant to typical antimicrobials used in the ED, are also major culprits in complicated skin and skin structure infections (cSSSI), the HCAI paradigm may apply in cSSSI. Furthermore, because of these patterns of increased resistance, HCA‐cSSSI patients, similar to other HCAI groups, may be at an increased risk of being treated with initially inappropriate antibiotic therapy.7, 10
Since in the setting of other types of infection inappropriate empiric treatment has been shown to be associated with increased mortality and costs,7, 1015 and since indirect evidence suggests a similar impact on healthcare utilization among cSSSI patients,8 we hypothesized that among a cohort of patients hospitalized with a cSSSI, the initial empiric choice of therapy is independently associated with hospital length of stay (LOS). We performed a retrospective cohort study to address this question.
Methods
Study Design
We performed a single‐center retrospective cohort study of patients with cSSSI admitted to the hospital through the ED. All consecutive patients hospitalized between April 2006 and December 2007 meeting predefined inclusion criteria (see below) were enrolled. The study was approved by the Washington University School of Medicine Human Studies Committee, and informed consent was waived. We have previously reported on the characteristics and outcomes of this cohort, including both community‐acquired and HCA‐cSSSI patients.16
Study Cohort
All consecutive patients admitted from the community through the ED between April 2006 and December 2007 at the Barnes‐Jewish Hospital, a 1200‐bed university‐affiliated, urban teaching hospital in St. Louis, MO were included if: (1) they had a diagnosis of a predefined cSSSI (see Appendix Table A1, based on reference 8) and (2) they had a positive microbiology culture obtained within 24 hours of hospital admission. Similar to the work by Edelsberg et al.8 we excluded patients if certain diagnoses and procedures were present (Appendix Table A2). Cases were also excluded if they represented a readmission for the same diagnosis within 30 days of the original hospitalization.
Definitions
HCAI was defined as any cSSSI in a patient with a history of recent hospitalization (within the previous year, consistent with the previous study16), receiving antibiotics prior to admission (previous 90 days), transferring from a nursing home, or needing chronic dialysis. We defined a polymicrobial infection as one with more than one organism, and mixed infection as an infection with both a gram‐positive and a gram‐negative organism. Inappropriate empiric therapy took place if a patient did not receive treatment within 24 hours of the time the culture was obtained with an agent exhibiting in vitro activity against the isolated pathogen(s). In mixed infections, appropriate therapy was treatment within 24 hours of culture being obtained with agent(s) active against all pathogens recovered.
Data Elements
We collected information about multiple baseline demographic and clinical factors including: age, gender, race/ethnicity, comorbidities, the presence of risk factors for HCAI, the presence of bacteremia at admission, and the location of admission (ward vs. intensive care unit [ICU]). Bacteriology data included information on specific bacterium/a recovered from culture, the site of the culture (eg, tissue, blood), susceptibility patterns, and whether the infection was monomicrobial, polymicrobial, or mixed. When blood culture was available and positive, we prioritized this over wound and other cultures and designated the corresponding organism as the culprit in the index infection. Cultures growing our coagulase‐negative S. aureus were excluded as a probable contaminant. Treatment data included information on the choice of the antimicrobial therapy and the timing of its institution relative to the timing of obtaining the culture specimen. The presence of such procedures as incision and drainage (I&D) or debridement was recorded.
Statistical Analyses
Descriptive statistics comparing HCAI patients treated appropriately to those receiving inappropriate empiric coverage based on their clinical, demographic, microbiologic and treatment characteristics were computed. Hospital LOS served as the primary and hospital mortality as the secondary outcomes, comparing patients with HCAI treated appropriately to those treated inappropriately. All continuous variables were compared using Student's t test or the Mann‐Whitney U test as appropriate. All categorical variables were compared using the chi‐square test or Fisher's exact test. To assess the attributable impact of inappropriate therapy in HCAI on the outcomes of interest, general linear models with log transformation were developed to model hospital LOS parameters; all means are presented as geometric means. All potential risk factors significant at the 0.1 level in univariate analyses were entered into the model. All calculations were performed in Stata version 9 (Statacorp, College Station, TX).
Results
Of the 717 patients with culture‐positive cSSSI admitted during the study period, 527 (73.5%) were classified as HCAI. The most common reason for classification as an HCAI was recent hospitalization. Among those with an HCA‐cSSSI, 405 (76.9%) received appropriate empiric treatment, with nearly one‐quarter receiving inappropriate initial coverage. Those receiving inappropriate antibiotic were more likely to be African American, and had a higher likelihood of having end‐stage renal disease (ESRD) than those with appropriate coverage (Table 1). While those patients treated appropriately had higher rates of both cellulitis and abscess as the presenting infection, a substantially higher proportion of those receiving inappropriate initial treatment had a decubitus ulcer (29.5% vs. 10.9%, P <0.001), a device‐associated infection (42.6% vs. 28.6%, P = 0.004), and had evidence of bacteremia (68.9% vs. 57.8%, P = 0.028) than those receiving appropriate empiric coverage (Table 2).
Inappropriate (n = 122), n (%) | Appropriate (n = 405), n (%) | P Value | |
---|---|---|---|
| |||
Age, years | 56.3 18.0 | 53.6 16.7 | 0.147 |
Gender (F) | 62 (50.8) | 190 (46.9) | 0.449 |
Race | |||
Caucasian | 51 (41.8) | 219 (54.1) | 0.048 |
African American | 68 (55.7) | 178 (43.9) | |
Other | 3 (2.5) | 8 (2.0) | |
HCAI risk factors | |||
Recent hospitalization* | 110 (90.2) | 373 (92.1) | 0.498 |
Within 90 days | 98 (80.3) | 274 (67.7) | 0.007 |
>90 and 180 days | 52 (42.6) | 170 (42.0) | 0.899 |
>180 days and 1 year | 46 (37.7) | 164 (40.5) | 0.581 |
Prior antibiotics | 26 (21.3) | 90 (22.2) | 0.831 |
Nursing home resident | 29 (23.8) | 54 (13.3) | 0.006 |
Hemodialysis | 19 (15.6) | 39 (9.7) | 0.067 |
Comorbidities | |||
DM | 40 (37.8) | 128 (31.6) | 0.806 |
PVD | 5 (4.1) | 15 (3.7) | 0.841 |
Liver disease | 6 (4.9) | 33 (8.2) | 0.232 |
Cancer | 21 (17.2) | 85 (21.0) | 0.362 |
HIV | 1 (0.8) | 12 (3.0) | 0.316 |
Organ transplant | 2 (1.6) | 8 (2.0) | 1.000 |
Autoimmune disease | 5 (4.1) | 8 (2.0) | 0.185 |
ESRD | 22 (18.0) | 46 (11.4) | 0.054 |
Inappropriate (n = 122), n (%) | Appropriate (n = 405), n (%) | P Value | |
---|---|---|---|
| |||
Cellulitis | 28 (23.0) | 171 (42.2) | <0.001 |
Decubitus ulcer | 36 (29.5) | 44 (10.9) | <0.001 |
Post‐op wound | 25 (20.5) | 75 (18.5) | 0.626 |
Device‐associated infection | 52 (42.6) | 116 (28.6) | 0.004 |
Diabetic foot ulcer | 9 (7.4) | 24 (5.9) | 0.562 |
Abscess | 22 (18.0) | 108 (26.7) | 0.052 |
Other* | 2 (1.6) | 17 (4.2) | 0.269 |
Presence of bacteremia | 84 (68.9) | 234 (57.8) | 0.028 |
The pathogens recovered from the appropriately and inappropriately treated groups are listed in Figure 1. While S. aureus overall was more common among those treated appropriately, the frequency of MRSA did not differ between the groups. Both E. faecalis and E. faecium were recovered more frequently in the inappropriate group, resulting in a similar pattern among the vancomycin‐resistant enterococcal species. Likewise, P. aeruginosa, P. mirabilis, and A. baumannii were all more frequently seen in the group treated inappropriately than in the group getting appropriate empiric coverage. A mixed infection was also more likely to be present among those not exposed (16.5%) than among those exposed (7.5%) to appropriate early therapy (P = 0.001) (Figure 1).
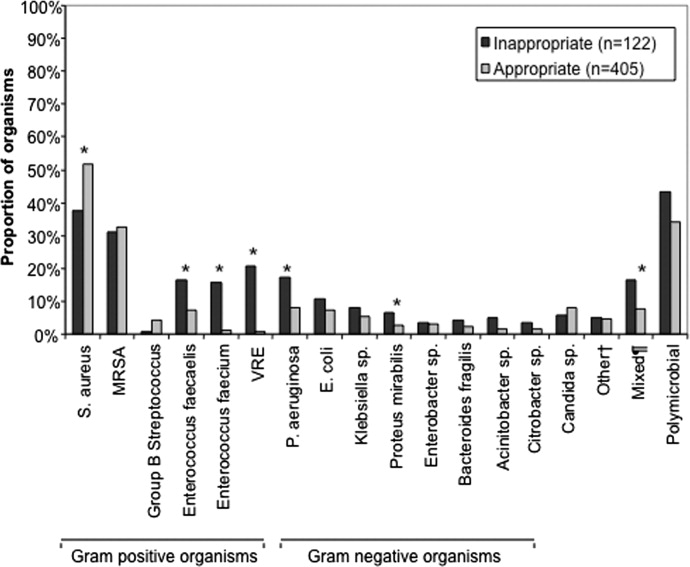
In terms of processes of care and outcomes (Table 3), commensurate with the higher prevalence of abscess in the appropriately treated group, the rate of I&D was significantly higher in this cohort (36.8%) than in the inappropriately treated (23.0%) group (P = 0.005). Need for initial ICU care did not differ as a function of appropriateness of therapy (P = 0.635).
Inappropriate (n = 122) | Appropriate (n = 405) | P Value | |
---|---|---|---|
| |||
I&D/debridement | 28 (23.0%) | 149 (36.8%) | 0.005 |
I&D in ED | 0 | 7 (1.7) | 0.361 |
ICU | 9 (7.4%) | 25 (6.2%) | 0.635 |
Hospital LOS, days | |||
Median (IQR 25, 75) [Range] | 7.0 (4.2, 13.6) [0.686.6] | 6 (3.3, 10.1) [0.748.3] | 0.026 |
Hospital mortality | 9 (7.4%) | 26 (6.4%) | 0.710 |
The unadjusted mortality rate was low overall and did not vary based on initial treatment (Table 3). In a generalized linear model with the log‐transformed LOS as the dependent variable, adjusting for multiple potential confounders, initial inappropriate antibiotic therapy had an attributable incremental increase in the hospital LOS of 1.8 days (95% CI, 1.42.3) (Table 4).
Factor | Attributable LOS (days) | 95% CI | P Value |
---|---|---|---|
| |||
Infection type: device | 3.6 | 2.74.8 | <0.001 |
Infection type: decubitus ulcer | 3.3 | 2.64.2 | <0.001 |
Infection type: abscess | 2.5 | 1.64.0 | <0.001 |
Organism: P. mirabilis | 2.2 | 1.43.4 | <0.001 |
Organism: E. faecalis | 2.1 | 1.72.6 | <0.001 |
Nursing home resident | 2.1 | 1.62.6 | <0.001 |
Inappropriate antibiotic | 1.8 | 1.42.3 | <0.001 |
Race: Non‐Caucasian | 0.31 | 0.240.41 | <0.001 |
Organism: E. faecium | 0.23 | 0.150.35 | <0.001 |
Because bacteremia is known to be an effect modifier of the relationship between the empiric choice of antibiotic and infection outcomes, we further explored its role in the HCAI cSSSI on the outcomes of interest (Table 5). Similar to the effect detected in the overall cohort, treatment with inappropriate therapy was associated with an increase in the hospital LOS, but not hospital mortality in those with bacteremia, though this phenomenon was observed only among patients with secondary bacteremia, and not among those without (Table 5).
Bacteremia Present (n = 318) | Bacteremia Absent (n = 209) | |||||
---|---|---|---|---|---|---|
I (n = 84) | A (n = 234) | P Value | I (n = 38) | A (n = 171) | P Value | |
| ||||||
Hospital LOS, days | ||||||
Mean SD | 14.4 27.5 | 9.8 9.7 | 0.041 | 6.6 6.8 | 6.9 8.2 | 0.761 |
Median (IQR 25, 75) | 8.8 (5.4, 13.9) | 7.0 (4.3, 11.7) | 4.4 (2.4, 7.7) | 3.9 (2.0, 8.2) | ||
Hospital mortality | 8 (9.5%) | 24 (10.3%) | 0.848 | 1 (2.6%) | 2 (1.2%) | 0.454 |
Discussion
This retrospective analysis provides evidence that inappropriate empiric antibiotic therapy for HCA‐cSSSI independently prolongs hospital LOS. The impact of inappropriate initial treatment on LOS is independent of many important confounders. In addition, we observed that this effect, while present among patients with secondary bacteremia, is absent among those without a blood stream infection.
To the best of our knowledge, ours is the first cohort study to examine the outcomes associated with inappropriate treatment of a HCAI cSSSI within the context of available microbiology data. Edelsberg et al.8 examined clinical and economic outcomes associated with the failure of the initial treatment of cSSSI. While not specifically focusing on HCAI patients, these authors noted an overall 23% initial therapy failure rate. Among those patients who failed initial therapy, the risk of hospital death was nearly 3‐fold higher (adjusted odds ratio [OR], 2.91; 95% CI, 2.343.62), and they incurred the mean of 5.4 additional hospital days, compared to patients treated successfully with the initial regimen.8 Our study confirms Edelsberg et al.'s8 observation of prolonged hospital LOS in association with treatment failure, and builds upon it by defining the actual LOS increment attributable to inappropriate empiric therapy. It is worth noting that the study by Edelsberg et al.,8 however, lacked explicit definition of the HCAI population and microbiology data, and used treatment failure as a surrogate marker for inappropriate treatment. It is likely these differences between our two studies in the underlying population and exposure definitions that account for the differences in the mortality data between that study and ours.
It is not fundamentally surprising that early exposure to inappropriate empiric therapy alters healthcare resource utilization outcomes for the worse. Others have demonstrated that infection with a resistant organism results in prolongation of hospital LOS and costs. For example, in a large cohort of over 600 surgical hospitalizations requiring treatment for a gram‐negative infection, antibiotic resistance was an independent predictor of increased LOS and costs.15 These authors quantified the incremental burden of early gram‐negative resistance at over $11,000 in hospital costs.15 Unfortunately, the treatment differences for resistant and sensitive organisms were not examined.15 Similarly, Shorr et al. examined risk factors for prolonged hospital LOS and increased costs in a cohort of 291 patients with MRSA sterile site infection.17 Because in this study 23% of the patients received inappropriate empiric therapy, the authors were able to examine the impact of this exposure on utilization outcomes.17 In an adjusted analysis, inappropriate initial treatment was associated with an incremental increase in the LOS of 2.5 days, corresponding to the unadjusted cost differential of nearly $6,000.17 Although focusing on a different population, our results are consistent with these previous observations that antibiotic resistance and early inappropriate therapy affect hospital utilization parameters, in our case by adding nearly 2 days to the hospital LOS.
Our study has a number of limitations. First, as a retrospective cohort study it is prone to various forms of bias, most notably selection bias. To minimize the possibility of such, we established a priori case definitions and enrolled consecutive patients over a specific period of time. Second, as in any observational study, confounding is an issue. We dealt with this statistically by constructing a multivariable regression model; however, the possibility of residual confounding remains. Third, because some of the wound and ulcer cultures likely were obtained with a swab and thus represented colonization, rather than infection, we may have over‐estimated the rate of inappropriate therapy, and this needs to be followed up in future prospective studies. Similarly, we may have over‐estimated the likelihood of inappropriate therapy among polymicrobial and mixed infections as well, given that, for example, a gram‐negative organism may carry a different clinical significance when cultured from blood (infection) than when it is detected in a decubitus ulcer (potential colonization). Fourth, because we limited our cohort to patients without deep‐seated infections, such as necrotizing fasciitis, other procedures were not collected. This omission may have led to either over‐estimation or under‐estimation of the impact of inappropriate therapy on the outcomes of interest.
The fact that our cohort represents a single large urban academic tertiary care medical center may limit the generalizability of our results only to centers that share similar characteristics. Finally, similar to most other studies of this type, ours lacks data on posthospitalization outcomes and for this reason limits itself to hospital outcomes only.
In summary, we have shown that, similar to other populations with HCAI, a substantial proportion (nearly 1/4) of cSSSI patients with HCAI receive inappropriate empiric therapy for their infection, and this early exposure, though not affecting hospital mortality, is associated with a significant prolongation of the hospitalization by as much as 2 days. Studies are needed to refine decision rules for risk‐stratifying patients with cSSSI HCAI in order to determine the probability of infection with a resistant organism. In turn, such instruments at the bedside may assure improved utilization of appropriately targeted empiric therapy that will both optimize individual patient outcomes and reduce the risk of emergence of antimicrobial resistance.
Appendix
Principal diagnosis code | Description |
---|---|
680 | Carbuncle and furuncle |
681 | Cellulitis and abscess of finger and toe |
682 | Other cellulitis and abscess |
683 | Acute lymphadenitis |
685 | Pilonidal cyst with abscess |
686 | Other local infections of skin and subcutaneous tissue |
707 | Decubitus ulcer |
707.1 | Ulcers of lower limbs, except decubitus |
707.8 | Chronic ulcer of other specified sites |
707.9 | Chronic ulcer of unspecified site |
958.3 | Posttraumatic wound infection, not elsewhere classified |
996.62 | Infection due to other vascular device, implant, and graft |
997.62 | Infection (chronic) of amputation stump |
998.5 | Postoperative wound infection |
Diagnosis code | Description |
---|---|
728.86 | Necrotizing fasciitis |
785.4 | Gangrene |
686.09 | Ecthyma gangrenosum |
730.00730.2 | Osteomyelitis |
630677 | Complications of pregnancy, childbirth and puerperium |
288.0 | Neutropenia |
684 | Impetigo |
Procedure code | |
39.95 | Plasmapheresis |
99.71 | Hemoperfusion |
Classically, infections have been categorized as either community‐acquired (CAI) or nosocomial in origin. Until recently, this scheme was thought adequate to capture the differences in the microbiology and outcomes in the corresponding scenarios. However, recent evidence suggests that this distinction may no longer be valid. For example, with the spread and diffusion of healthcare delivery beyond the confines of the hospital along with the increasing use of broad spectrum antibiotics both in and out of the hospital, pathogens such as methicillin‐resistant Staphylococcus aureus (MRSA) and Pseudomonas aeruginosa (PA), traditionally thought to be confined to the hospital, are now seen in patients presenting from the community to the emergency department (ED).1, 2 Reflecting this shift in epidemiology, some national guidelines now recognize healthcare‐associated infection (HCAI) as a distinct entity.3 The concept of HCAI allows the clinician to identify patients who, despite suffering a community onset infection, still may be at risk for a resistant bacterial pathogen. Recent studies in both bloodstream infection and pneumonia have clearly demonstrated that those with HCAI have distinct microbiology and outcomes relative to those with pure CAI.47
Most work focusing on establishing HCAI has not addressed skin and soft tissue infections. These infections, although not often fatal, account for an increasing number of admissions to the hospital.8, 9 In addition, they may be associated with substantial morbidity and cost.8 Given that many pathogens such as S. aureus, which may be resistant to typical antimicrobials used in the ED, are also major culprits in complicated skin and skin structure infections (cSSSI), the HCAI paradigm may apply in cSSSI. Furthermore, because of these patterns of increased resistance, HCA‐cSSSI patients, similar to other HCAI groups, may be at an increased risk of being treated with initially inappropriate antibiotic therapy.7, 10
Since in the setting of other types of infection inappropriate empiric treatment has been shown to be associated with increased mortality and costs,7, 1015 and since indirect evidence suggests a similar impact on healthcare utilization among cSSSI patients,8 we hypothesized that among a cohort of patients hospitalized with a cSSSI, the initial empiric choice of therapy is independently associated with hospital length of stay (LOS). We performed a retrospective cohort study to address this question.
Methods
Study Design
We performed a single‐center retrospective cohort study of patients with cSSSI admitted to the hospital through the ED. All consecutive patients hospitalized between April 2006 and December 2007 meeting predefined inclusion criteria (see below) were enrolled. The study was approved by the Washington University School of Medicine Human Studies Committee, and informed consent was waived. We have previously reported on the characteristics and outcomes of this cohort, including both community‐acquired and HCA‐cSSSI patients.16
Study Cohort
All consecutive patients admitted from the community through the ED between April 2006 and December 2007 at the Barnes‐Jewish Hospital, a 1200‐bed university‐affiliated, urban teaching hospital in St. Louis, MO were included if: (1) they had a diagnosis of a predefined cSSSI (see Appendix Table A1, based on reference 8) and (2) they had a positive microbiology culture obtained within 24 hours of hospital admission. Similar to the work by Edelsberg et al.8 we excluded patients if certain diagnoses and procedures were present (Appendix Table A2). Cases were also excluded if they represented a readmission for the same diagnosis within 30 days of the original hospitalization.
Definitions
HCAI was defined as any cSSSI in a patient with a history of recent hospitalization (within the previous year, consistent with the previous study16), receiving antibiotics prior to admission (previous 90 days), transferring from a nursing home, or needing chronic dialysis. We defined a polymicrobial infection as one with more than one organism, and mixed infection as an infection with both a gram‐positive and a gram‐negative organism. Inappropriate empiric therapy took place if a patient did not receive treatment within 24 hours of the time the culture was obtained with an agent exhibiting in vitro activity against the isolated pathogen(s). In mixed infections, appropriate therapy was treatment within 24 hours of culture being obtained with agent(s) active against all pathogens recovered.
Data Elements
We collected information about multiple baseline demographic and clinical factors including: age, gender, race/ethnicity, comorbidities, the presence of risk factors for HCAI, the presence of bacteremia at admission, and the location of admission (ward vs. intensive care unit [ICU]). Bacteriology data included information on specific bacterium/a recovered from culture, the site of the culture (eg, tissue, blood), susceptibility patterns, and whether the infection was monomicrobial, polymicrobial, or mixed. When blood culture was available and positive, we prioritized this over wound and other cultures and designated the corresponding organism as the culprit in the index infection. Cultures growing our coagulase‐negative S. aureus were excluded as a probable contaminant. Treatment data included information on the choice of the antimicrobial therapy and the timing of its institution relative to the timing of obtaining the culture specimen. The presence of such procedures as incision and drainage (I&D) or debridement was recorded.
Statistical Analyses
Descriptive statistics comparing HCAI patients treated appropriately to those receiving inappropriate empiric coverage based on their clinical, demographic, microbiologic and treatment characteristics were computed. Hospital LOS served as the primary and hospital mortality as the secondary outcomes, comparing patients with HCAI treated appropriately to those treated inappropriately. All continuous variables were compared using Student's t test or the Mann‐Whitney U test as appropriate. All categorical variables were compared using the chi‐square test or Fisher's exact test. To assess the attributable impact of inappropriate therapy in HCAI on the outcomes of interest, general linear models with log transformation were developed to model hospital LOS parameters; all means are presented as geometric means. All potential risk factors significant at the 0.1 level in univariate analyses were entered into the model. All calculations were performed in Stata version 9 (Statacorp, College Station, TX).
Results
Of the 717 patients with culture‐positive cSSSI admitted during the study period, 527 (73.5%) were classified as HCAI. The most common reason for classification as an HCAI was recent hospitalization. Among those with an HCA‐cSSSI, 405 (76.9%) received appropriate empiric treatment, with nearly one‐quarter receiving inappropriate initial coverage. Those receiving inappropriate antibiotic were more likely to be African American, and had a higher likelihood of having end‐stage renal disease (ESRD) than those with appropriate coverage (Table 1). While those patients treated appropriately had higher rates of both cellulitis and abscess as the presenting infection, a substantially higher proportion of those receiving inappropriate initial treatment had a decubitus ulcer (29.5% vs. 10.9%, P <0.001), a device‐associated infection (42.6% vs. 28.6%, P = 0.004), and had evidence of bacteremia (68.9% vs. 57.8%, P = 0.028) than those receiving appropriate empiric coverage (Table 2).
Inappropriate (n = 122), n (%) | Appropriate (n = 405), n (%) | P Value | |
---|---|---|---|
| |||
Age, years | 56.3 18.0 | 53.6 16.7 | 0.147 |
Gender (F) | 62 (50.8) | 190 (46.9) | 0.449 |
Race | |||
Caucasian | 51 (41.8) | 219 (54.1) | 0.048 |
African American | 68 (55.7) | 178 (43.9) | |
Other | 3 (2.5) | 8 (2.0) | |
HCAI risk factors | |||
Recent hospitalization* | 110 (90.2) | 373 (92.1) | 0.498 |
Within 90 days | 98 (80.3) | 274 (67.7) | 0.007 |
>90 and 180 days | 52 (42.6) | 170 (42.0) | 0.899 |
>180 days and 1 year | 46 (37.7) | 164 (40.5) | 0.581 |
Prior antibiotics | 26 (21.3) | 90 (22.2) | 0.831 |
Nursing home resident | 29 (23.8) | 54 (13.3) | 0.006 |
Hemodialysis | 19 (15.6) | 39 (9.7) | 0.067 |
Comorbidities | |||
DM | 40 (37.8) | 128 (31.6) | 0.806 |
PVD | 5 (4.1) | 15 (3.7) | 0.841 |
Liver disease | 6 (4.9) | 33 (8.2) | 0.232 |
Cancer | 21 (17.2) | 85 (21.0) | 0.362 |
HIV | 1 (0.8) | 12 (3.0) | 0.316 |
Organ transplant | 2 (1.6) | 8 (2.0) | 1.000 |
Autoimmune disease | 5 (4.1) | 8 (2.0) | 0.185 |
ESRD | 22 (18.0) | 46 (11.4) | 0.054 |
Inappropriate (n = 122), n (%) | Appropriate (n = 405), n (%) | P Value | |
---|---|---|---|
| |||
Cellulitis | 28 (23.0) | 171 (42.2) | <0.001 |
Decubitus ulcer | 36 (29.5) | 44 (10.9) | <0.001 |
Post‐op wound | 25 (20.5) | 75 (18.5) | 0.626 |
Device‐associated infection | 52 (42.6) | 116 (28.6) | 0.004 |
Diabetic foot ulcer | 9 (7.4) | 24 (5.9) | 0.562 |
Abscess | 22 (18.0) | 108 (26.7) | 0.052 |
Other* | 2 (1.6) | 17 (4.2) | 0.269 |
Presence of bacteremia | 84 (68.9) | 234 (57.8) | 0.028 |
The pathogens recovered from the appropriately and inappropriately treated groups are listed in Figure 1. While S. aureus overall was more common among those treated appropriately, the frequency of MRSA did not differ between the groups. Both E. faecalis and E. faecium were recovered more frequently in the inappropriate group, resulting in a similar pattern among the vancomycin‐resistant enterococcal species. Likewise, P. aeruginosa, P. mirabilis, and A. baumannii were all more frequently seen in the group treated inappropriately than in the group getting appropriate empiric coverage. A mixed infection was also more likely to be present among those not exposed (16.5%) than among those exposed (7.5%) to appropriate early therapy (P = 0.001) (Figure 1).
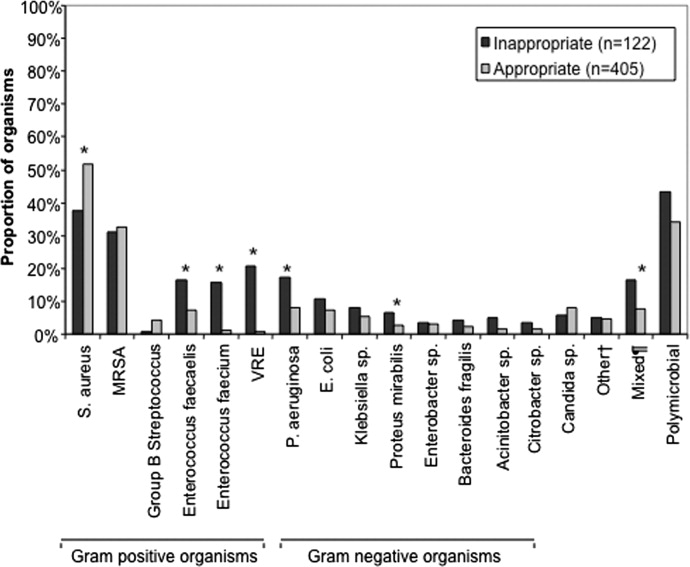
In terms of processes of care and outcomes (Table 3), commensurate with the higher prevalence of abscess in the appropriately treated group, the rate of I&D was significantly higher in this cohort (36.8%) than in the inappropriately treated (23.0%) group (P = 0.005). Need for initial ICU care did not differ as a function of appropriateness of therapy (P = 0.635).
Inappropriate (n = 122) | Appropriate (n = 405) | P Value | |
---|---|---|---|
| |||
I&D/debridement | 28 (23.0%) | 149 (36.8%) | 0.005 |
I&D in ED | 0 | 7 (1.7) | 0.361 |
ICU | 9 (7.4%) | 25 (6.2%) | 0.635 |
Hospital LOS, days | |||
Median (IQR 25, 75) [Range] | 7.0 (4.2, 13.6) [0.686.6] | 6 (3.3, 10.1) [0.748.3] | 0.026 |
Hospital mortality | 9 (7.4%) | 26 (6.4%) | 0.710 |
The unadjusted mortality rate was low overall and did not vary based on initial treatment (Table 3). In a generalized linear model with the log‐transformed LOS as the dependent variable, adjusting for multiple potential confounders, initial inappropriate antibiotic therapy had an attributable incremental increase in the hospital LOS of 1.8 days (95% CI, 1.42.3) (Table 4).
Factor | Attributable LOS (days) | 95% CI | P Value |
---|---|---|---|
| |||
Infection type: device | 3.6 | 2.74.8 | <0.001 |
Infection type: decubitus ulcer | 3.3 | 2.64.2 | <0.001 |
Infection type: abscess | 2.5 | 1.64.0 | <0.001 |
Organism: P. mirabilis | 2.2 | 1.43.4 | <0.001 |
Organism: E. faecalis | 2.1 | 1.72.6 | <0.001 |
Nursing home resident | 2.1 | 1.62.6 | <0.001 |
Inappropriate antibiotic | 1.8 | 1.42.3 | <0.001 |
Race: Non‐Caucasian | 0.31 | 0.240.41 | <0.001 |
Organism: E. faecium | 0.23 | 0.150.35 | <0.001 |
Because bacteremia is known to be an effect modifier of the relationship between the empiric choice of antibiotic and infection outcomes, we further explored its role in the HCAI cSSSI on the outcomes of interest (Table 5). Similar to the effect detected in the overall cohort, treatment with inappropriate therapy was associated with an increase in the hospital LOS, but not hospital mortality in those with bacteremia, though this phenomenon was observed only among patients with secondary bacteremia, and not among those without (Table 5).
Bacteremia Present (n = 318) | Bacteremia Absent (n = 209) | |||||
---|---|---|---|---|---|---|
I (n = 84) | A (n = 234) | P Value | I (n = 38) | A (n = 171) | P Value | |
| ||||||
Hospital LOS, days | ||||||
Mean SD | 14.4 27.5 | 9.8 9.7 | 0.041 | 6.6 6.8 | 6.9 8.2 | 0.761 |
Median (IQR 25, 75) | 8.8 (5.4, 13.9) | 7.0 (4.3, 11.7) | 4.4 (2.4, 7.7) | 3.9 (2.0, 8.2) | ||
Hospital mortality | 8 (9.5%) | 24 (10.3%) | 0.848 | 1 (2.6%) | 2 (1.2%) | 0.454 |
Discussion
This retrospective analysis provides evidence that inappropriate empiric antibiotic therapy for HCA‐cSSSI independently prolongs hospital LOS. The impact of inappropriate initial treatment on LOS is independent of many important confounders. In addition, we observed that this effect, while present among patients with secondary bacteremia, is absent among those without a blood stream infection.
To the best of our knowledge, ours is the first cohort study to examine the outcomes associated with inappropriate treatment of a HCAI cSSSI within the context of available microbiology data. Edelsberg et al.8 examined clinical and economic outcomes associated with the failure of the initial treatment of cSSSI. While not specifically focusing on HCAI patients, these authors noted an overall 23% initial therapy failure rate. Among those patients who failed initial therapy, the risk of hospital death was nearly 3‐fold higher (adjusted odds ratio [OR], 2.91; 95% CI, 2.343.62), and they incurred the mean of 5.4 additional hospital days, compared to patients treated successfully with the initial regimen.8 Our study confirms Edelsberg et al.'s8 observation of prolonged hospital LOS in association with treatment failure, and builds upon it by defining the actual LOS increment attributable to inappropriate empiric therapy. It is worth noting that the study by Edelsberg et al.,8 however, lacked explicit definition of the HCAI population and microbiology data, and used treatment failure as a surrogate marker for inappropriate treatment. It is likely these differences between our two studies in the underlying population and exposure definitions that account for the differences in the mortality data between that study and ours.
It is not fundamentally surprising that early exposure to inappropriate empiric therapy alters healthcare resource utilization outcomes for the worse. Others have demonstrated that infection with a resistant organism results in prolongation of hospital LOS and costs. For example, in a large cohort of over 600 surgical hospitalizations requiring treatment for a gram‐negative infection, antibiotic resistance was an independent predictor of increased LOS and costs.15 These authors quantified the incremental burden of early gram‐negative resistance at over $11,000 in hospital costs.15 Unfortunately, the treatment differences for resistant and sensitive organisms were not examined.15 Similarly, Shorr et al. examined risk factors for prolonged hospital LOS and increased costs in a cohort of 291 patients with MRSA sterile site infection.17 Because in this study 23% of the patients received inappropriate empiric therapy, the authors were able to examine the impact of this exposure on utilization outcomes.17 In an adjusted analysis, inappropriate initial treatment was associated with an incremental increase in the LOS of 2.5 days, corresponding to the unadjusted cost differential of nearly $6,000.17 Although focusing on a different population, our results are consistent with these previous observations that antibiotic resistance and early inappropriate therapy affect hospital utilization parameters, in our case by adding nearly 2 days to the hospital LOS.
Our study has a number of limitations. First, as a retrospective cohort study it is prone to various forms of bias, most notably selection bias. To minimize the possibility of such, we established a priori case definitions and enrolled consecutive patients over a specific period of time. Second, as in any observational study, confounding is an issue. We dealt with this statistically by constructing a multivariable regression model; however, the possibility of residual confounding remains. Third, because some of the wound and ulcer cultures likely were obtained with a swab and thus represented colonization, rather than infection, we may have over‐estimated the rate of inappropriate therapy, and this needs to be followed up in future prospective studies. Similarly, we may have over‐estimated the likelihood of inappropriate therapy among polymicrobial and mixed infections as well, given that, for example, a gram‐negative organism may carry a different clinical significance when cultured from blood (infection) than when it is detected in a decubitus ulcer (potential colonization). Fourth, because we limited our cohort to patients without deep‐seated infections, such as necrotizing fasciitis, other procedures were not collected. This omission may have led to either over‐estimation or under‐estimation of the impact of inappropriate therapy on the outcomes of interest.
The fact that our cohort represents a single large urban academic tertiary care medical center may limit the generalizability of our results only to centers that share similar characteristics. Finally, similar to most other studies of this type, ours lacks data on posthospitalization outcomes and for this reason limits itself to hospital outcomes only.
In summary, we have shown that, similar to other populations with HCAI, a substantial proportion (nearly 1/4) of cSSSI patients with HCAI receive inappropriate empiric therapy for their infection, and this early exposure, though not affecting hospital mortality, is associated with a significant prolongation of the hospitalization by as much as 2 days. Studies are needed to refine decision rules for risk‐stratifying patients with cSSSI HCAI in order to determine the probability of infection with a resistant organism. In turn, such instruments at the bedside may assure improved utilization of appropriately targeted empiric therapy that will both optimize individual patient outcomes and reduce the risk of emergence of antimicrobial resistance.
Appendix
Principal diagnosis code | Description |
---|---|
680 | Carbuncle and furuncle |
681 | Cellulitis and abscess of finger and toe |
682 | Other cellulitis and abscess |
683 | Acute lymphadenitis |
685 | Pilonidal cyst with abscess |
686 | Other local infections of skin and subcutaneous tissue |
707 | Decubitus ulcer |
707.1 | Ulcers of lower limbs, except decubitus |
707.8 | Chronic ulcer of other specified sites |
707.9 | Chronic ulcer of unspecified site |
958.3 | Posttraumatic wound infection, not elsewhere classified |
996.62 | Infection due to other vascular device, implant, and graft |
997.62 | Infection (chronic) of amputation stump |
998.5 | Postoperative wound infection |
Diagnosis code | Description |
---|---|
728.86 | Necrotizing fasciitis |
785.4 | Gangrene |
686.09 | Ecthyma gangrenosum |
730.00730.2 | Osteomyelitis |
630677 | Complications of pregnancy, childbirth and puerperium |
288.0 | Neutropenia |
684 | Impetigo |
Procedure code | |
39.95 | Plasmapheresis |
99.71 | Hemoperfusion |
- Invasive methicillin‐resistant Staphylococcus aureus infections in the United States.JAMA.2007;298:1762–1771. , , , et al.
- Methicillin‐resistant S. aureus infections among patients in the emergency department.N Engl J Med.2006;17;355:666–674. , , , et al.
- Hospital‐Acquired Pneumonia Guideline Committee of the American Thoracic Society and Infectious Diseases Society of America.Guidelines for the management of adults with hospital‐acquired pneumonia, ventilator‐associated pneumonia, and healthcare‐associated pneumonia.Am J Respir Crit Care Med.2005;171:388–416.
- Epidemiology and outcomes of health‐care‐associated pneumonia: Results from a large US database of culture‐positive pneumonia.Chest.2005;128:3854–3862. , , , et al.
- Health care‐associated bloodstream infections in adults: A reason to change the accepted definition of community‐acquired infections.Ann Intern Med.2002;137:791–797. , , , et al.
- Healthcare‐associated bloodstream infection: A distinct entity? Insights from a large U.S. database.Crit Care Med.2006;34:2588–2595. , , , , , .
- Health care‐associated pneumonia and community‐acquired pneumonia: a single‐center experience.Antimicrob Agents Chemother.2007;51:3568–3573. , , , et al.
- Clinical and economic consequences of failure of initial antibiotic therapy for hospitalized patients with complicated skin and skin‐structure infections.Infect Control Hosp Epidemiol.2008;29:160–169. , , , , , .
- Skin, soft tissue, bone, and joint infections in hospitalized patients: Epidemiology and microbiological, clinical, and economic outcomes.Infect Control Hosp Epidemiol.2007;28:1290–1298. , , , et al.
- Methicillin‐resistant Staphylococcus aureus sterile‐site infection: The importance of appropriate initial antimicrobial treatment.Crit Care Med.2006;34:2069–2074. , , , et al.
- The influence of inadequate antimicrobial treatment of bloodstream infections on patient outcomes in the ICU setting.Chest.2000;118:146–155. , , , et al.
- Modification of empiric antibiotic treatment in patients with pneumonia acquired in the intensive care unit.Intensive Care Med.1996;22:387–394. , .
- Clinical importance of delays in the initiation of appropriate antibiotic treatment for ventilator‐associated pneumonia.Chest.2002;122:262–268. , , , et al.
- Antimicrobial therapy escalation and hospital mortality among patients with HCAP: A single center experience.Chest.2008;134:963–968. , , , , .
- Cost of gram‐negative resistance.Crit Care Med.2007;35:89–95. , , , et al.
- Epidemiology and outcomes of hospitalizations with complicated skin and skin‐structure infections: implications of healthcare‐associated infection risk factors.Infect Control Hosp Epidemiol.2009;30:1203–1210. , , , et al.
- Inappropriate therapy for methicillin‐resistant Staphylococcus aureus: resource utilization and cost implications.Crit Care Med.2008;36:2335–2340. , , .
- Invasive methicillin‐resistant Staphylococcus aureus infections in the United States.JAMA.2007;298:1762–1771. , , , et al.
- Methicillin‐resistant S. aureus infections among patients in the emergency department.N Engl J Med.2006;17;355:666–674. , , , et al.
- Hospital‐Acquired Pneumonia Guideline Committee of the American Thoracic Society and Infectious Diseases Society of America.Guidelines for the management of adults with hospital‐acquired pneumonia, ventilator‐associated pneumonia, and healthcare‐associated pneumonia.Am J Respir Crit Care Med.2005;171:388–416.
- Epidemiology and outcomes of health‐care‐associated pneumonia: Results from a large US database of culture‐positive pneumonia.Chest.2005;128:3854–3862. , , , et al.
- Health care‐associated bloodstream infections in adults: A reason to change the accepted definition of community‐acquired infections.Ann Intern Med.2002;137:791–797. , , , et al.
- Healthcare‐associated bloodstream infection: A distinct entity? Insights from a large U.S. database.Crit Care Med.2006;34:2588–2595. , , , , , .
- Health care‐associated pneumonia and community‐acquired pneumonia: a single‐center experience.Antimicrob Agents Chemother.2007;51:3568–3573. , , , et al.
- Clinical and economic consequences of failure of initial antibiotic therapy for hospitalized patients with complicated skin and skin‐structure infections.Infect Control Hosp Epidemiol.2008;29:160–169. , , , , , .
- Skin, soft tissue, bone, and joint infections in hospitalized patients: Epidemiology and microbiological, clinical, and economic outcomes.Infect Control Hosp Epidemiol.2007;28:1290–1298. , , , et al.
- Methicillin‐resistant Staphylococcus aureus sterile‐site infection: The importance of appropriate initial antimicrobial treatment.Crit Care Med.2006;34:2069–2074. , , , et al.
- The influence of inadequate antimicrobial treatment of bloodstream infections on patient outcomes in the ICU setting.Chest.2000;118:146–155. , , , et al.
- Modification of empiric antibiotic treatment in patients with pneumonia acquired in the intensive care unit.Intensive Care Med.1996;22:387–394. , .
- Clinical importance of delays in the initiation of appropriate antibiotic treatment for ventilator‐associated pneumonia.Chest.2002;122:262–268. , , , et al.
- Antimicrobial therapy escalation and hospital mortality among patients with HCAP: A single center experience.Chest.2008;134:963–968. , , , , .
- Cost of gram‐negative resistance.Crit Care Med.2007;35:89–95. , , , et al.
- Epidemiology and outcomes of hospitalizations with complicated skin and skin‐structure infections: implications of healthcare‐associated infection risk factors.Infect Control Hosp Epidemiol.2009;30:1203–1210. , , , et al.
- Inappropriate therapy for methicillin‐resistant Staphylococcus aureus: resource utilization and cost implications.Crit Care Med.2008;36:2335–2340. , , .
Copyright © 2010 Society of Hospital Medicine
Early Prediction of Septic Shock
Severe sepsis is responsible for significant morbidity and mortality. In the United States, approximately 750,000 cases occur each year with an estimated mortality of 30% to 50%.1 Early goal‐directed therapy has been shown to decrease mortality in patients with severe sepsis and septic shock.2, 3 As a result, efforts have been focused toward providing early and aggressive intervention once sepsis has been established. In many cases this has been accomplished through the implementation of a protocol with guidelines for fluid management, antibiotic and vasopressor administration, and other interventions.410 Prior studies have demonstrated that care of hospitalized patients before intensive care unit (ICU) admission is often suboptimal,1113 and have suggested that patients with clear indicators of acute deterioration may go unrecognized on the ward. We previously reported the effects of implementing a hospital‐wide protocol for the management of severe sepsis,14 finding that although there was a significant reduction in overall mortality there was no difference for patients who developed severe sepsis on the hospital ward. This finding also suggests that the initial care of patients with severe sepsis on hospital wards may differ in intensity compared to emergency departments and ICUs. Failure on the part of the clinician to recognize the harbingers of impending sepsis before the onset of organ dysfunction or hypotension may contribute to a delay in aggressive therapy.
Previous efforts at early recognition of sepsis have relied on diagnostic studies or specific biomarkers to screen at‐risk patients. These have included such studies as messenger RNA (mRNA) expression,15 C‐reactive protein,16 procalcitonin in newborns,17 immunocompetence measures in burn patients,18 protein C concentration in neutropenic patients,19 and several immune markers (eg, tumor necrosis factor‐alpha, interleukin [IL]‐1 beta, IL‐6, IL‐8, and IL‐10).20 However, these biomarkers have been studied only in specific patient populations, require suspicion on the part of the clinician and the measurement of diagnostic or laboratory values that would otherwise not have been obtained. The ideal tool for predicting the onset of sepsis would be applicable to a broad patient population, not require specific suspicion on the part of the clinician, and use only routinely obtained clinical measurements and laboratory values.
Prediction models and scoring systems that use routine hemodynamic and laboratory values for several endpoints related to sepsis and septic shock have been developed. Many such tools are used to define severity of illness and predict outcome, while others have been developed to predict such events as bacteremia in patients presenting with fever,21 the probability of infection in the critically ill,22 and end‐organ dysfunction in severe sepsis.23 Little work has been done to develop such a model capable of predicting the onset of sepsis,24 and there have been no attempts to deploy a model as a large‐scale screening tool.
Our objective was to develop a simple algorithm that can be used in an automated fashion to screen hospitalized patients for impending septic shock. Such a model would be derived from routine hemodynamic and laboratory values, and take advantage of a computerized medical record system for data collection.
Patients and Methods
Patient Enrollment and Data Collection
This study was conducted at Barnes‐Jewish Hospital, St. Louis, MO, a university‐affiliated, urban teaching hospital. The study was approved by the Washington University (St. Louis, MO) School of Medicine Human Studies Committee. Patients included in the study where those hospitalized during 2005, 2006, and 2007, and who had at least 1 International Statistical Classification of Diseases and Related Health Problems, 9th edition (ICD9) discharge diagnosis code for the medical/nonsurgical diagnoses listed in Appendix 1. From this pool of patients, septic shock patients were identified as those who were admitted to the hospital ward and later developed septic shock requiring transfer to an ICU for vasopressor support and hemodynamic monitoring. This was accomplished by using discharge ICD9 codes for acute infection matched to codes for acute organ dysfunction and the need for vasopressors within 24 hours of ICU transfer (Appendix 1). The patients used as controls were then all those remaining in the pool once the septic shock patients were identified and separated.
Case patients were excluded from the analysis if they were transferred to the ICU within 2 hours of hospital admission, as these patients are unlikely to have an adequate amount of pretransfer clinical data available for analysis. Both case and control patients were excluded if they lacked any value for basic, routine laboratory data (serum sodium, chloride, total bicarbonate, urea nitrogen, creatinine, glucose, white blood cell count, neutrophil count, hemoglobin, hematocrit, and platelet count) and certain vital signs (blood pressure, heart rate, temperature). Patient data from 2005 were used in the derivation of the prediction model, and 2006 and 2007 patient data were used to prospectively validate the model. Clinical variables used in the analysis were selected based on both ease of access from the electronic medical record and clinical relevance, and are shown in Table 1.
|
Age (years) |
Albumin (g/dL) |
Arterial blood gas (pH, PaCO2, PaO2) |
Anion gap |
Bilirubin (mg/dL) |
BP, systolic and diastolic (mm of Hg) |
Blood urea nitrogen (mg/dL) |
Chloride (mmol/L) |
Creatinine (mg/dL) |
Glucose (mg/dL) |
Hemoglobin (g/dL) |
International normalized ratio |
Neutrophil count, absolute (1 103/L) |
Platelet count (1 103/L) |
Pulse (beats/minute) |
Pulse pressure (mm of Hg) |
Shock index (pulse divided by systolic BP) |
Sodium (mmol/L) |
Total bicarbonate (mmol/L) |
Temperature (degrees Celsius) |
White blood cell count (1 103/L) |
In performing the Recursive Partitioning And Regression Tree (RPART) analysis to generate a prediction model, data for case patients were extracted in a window from 24 hours to 2 hours before ICU admission. The data collection window excluded the 2 hours prior to ICU transfer in order to minimize the effect of acute hemodynamic or laboratory changes that may have prompted the transfer; the purpose of the model is to identify hemodynamic and laboratory patterns in the several hours before the onset of clinically evident shock, so data from a time during which impending shock was clinically apparent were excluded. For the control patients, data from the first 48 hours of their hospitalization were included in the analysis.
Statistical Analysis
RPART analysis was performed on the 2005 patient data set to generate a prediction algorithm. This method of analysis results in a classification tree that contains a series of binary splits designed to separate patients into mutually exclusive subgroups.25 Each split in the tree is selected based on its ability to produce a partition with the greatest purity. Initially, a large tree that contains splits for all input variables is generated. This initial tree is generally too large to be useful as the final subgroups are too small to make sensible statistical inference.25 A pruning process is then applied to the initial tree with the goal of finding the subtree that is most predictive of the outcome of interest. The analysis was done using the RPART package of the R statistical analysis program, version 2.7.0 (R: A Language and Environment for Statistical Computing, R Development Core Team, Foundation for Statistical Computing, Vienna, Austria). The resulting classification tree was then used as a prediction algorithm and applied in a prospective fashion to the 2006 and 2007 patient data sets.
For the purpose of performing the RPART analysis, each set of case data entered into the analysis consisted of a random extraction of the desired clinical data within the specified extraction window from a single case patient. Thus, if a case patient had more than 1 value available for any variable of interest, 1 value was randomly selected to be entered in combination with the other available clinical data. Furthermore, in order to ensure that the majority of case patient data were included in the analysis, this process was iterated 10 times for each case patient. This resulted in 10 sets of case patient data being entered into the analysis for each case patient in the database, with each set containing a value for all variables of interest randomly extracted from those available for that patient. In addition to ensuring that the majority of case patient data were included, this technique also functionally expands the number of case patients present in the analysis. As there were far more control patients than case patients in the database, this in turn results in a classification tree that does not simply identify controls without regard to the relatively small number of case patients.
Data for the control patients entered into the analysis were extracted in a similar fashion, though only 1 set of data were included in the analysis for each control patient present in the database. As a result, only 1 randomly selected value per variable was included in the analysis.
Results
Patients
During 2005, 562 septic patients and 13,223 control patients were identified. For 2006 and 2007 there were 635 and 667 case patients, and 13,102 and 13,270 control patients, respectively.
Predictors of Sepsis
RPART analysis of the 2005 patient data set demonstrated that the most significant predictors of sepsis in the 24 hours preceding transfer to the medical ICU were the partial pressure of arterial oxygen (PaO2), systolic blood pressure, absolute neutrophil count, blood urea nitrogen (BUN), pH, bicarbonate, chloride, and albumin. This resulted in a simple algorithm with nine classification splits (Figure 1), which was then prospectively applied to the 2006 and 2007 patient data sets. These results are summarized in Table 2.
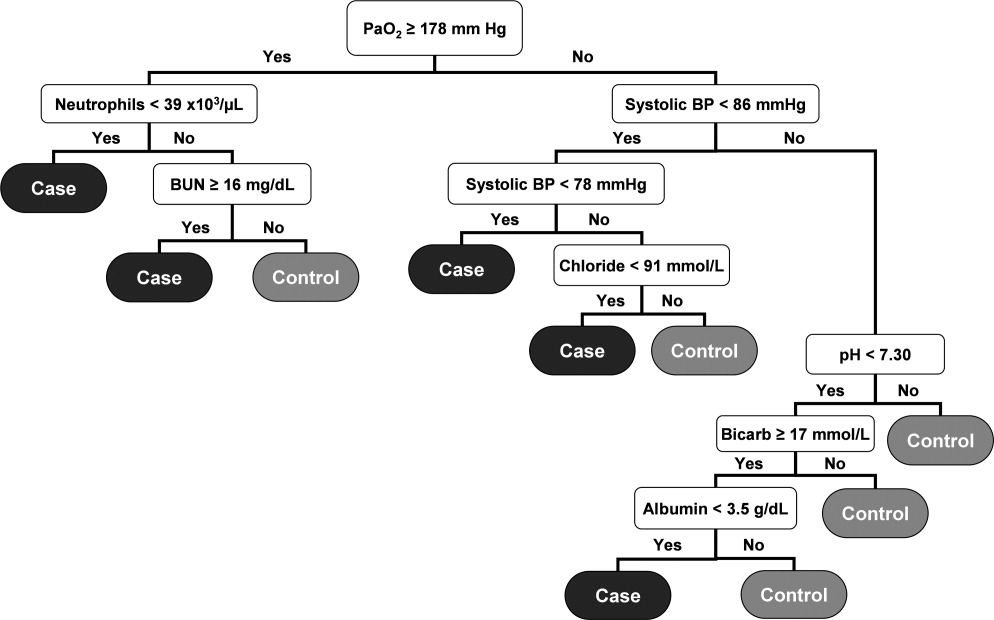
Total Number | Number Correctly Classified (%) | Case Identification Time Before ICU Admission (minutes) | PPV (%) | NPV (%) | MCR (%) | |
---|---|---|---|---|---|---|
| ||||||
2005 | 27.9 | 98.1 | 7.8 | |||
Cases | 562 | 320 (56.9) | ||||
Controls | 13,223 | 12,394 (93.7) | ||||
2006 | 179 230 | 28.7 | 97.7 | 8.4 | ||
Cases | 635 | 347 (54.7) | ||||
Controls | 13,102 | 12,241 (93.4) | ||||
2007 | 192 210 | 28.3 | 97.6 | 8.8 | ||
Cases | 667 | 367 (55.0) | ||||
Controls | 13,270 | 12,341 (93.0) |
The resulting classification model had a low total misclassification rate for the 2005 data. Of the 562 septic patients, 320 (56.9%) were correctly classified, and 12,394 (93.7%) of the control patients were appropriately identified. The number of septic and control patients misclassified was 242 and 829, respectively, yielding a total misclassification rate of 7.8%. When applied to the 2006 patient data set, 347 (54.7%) of the 635 septic shock patients were correctly identified, while 12,241 (93.4%) of the 13,102 control patients were correctly classified. The total misclassification rate for the 2006 patient set was 8.4%. For the 2007 patient data, 367 (55.0%) of the 667 case patients were correctly identified, and 12,341 (93.0%) of the 13,270 control patients were correctly identified. This resulted in a total misclassification rate of 8.8%.
The 2006 and 2007 case patients were identified 179 230 minutes and 192 210 minutes before ICU transfer, respectively (Figure 2). The algorithm demonstrated positive and negative predictive values of 28.7% and 97.7% for the 2006 patient set, respectively, and 28.3% and 97.6% for the 2007 patient set, respectively.
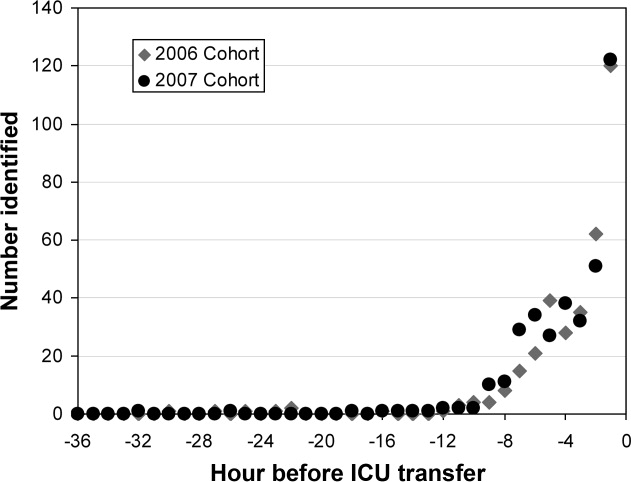
Although the prediction algorithm shown in Figure 1 identified the majority of the case patients with ample time for clinical intervention prior to ICU transfer, the analysis used to derive this model included values for the arterial blood gas (ABG). As this is not a routinely obtained study for hospitalized patients outside of an ICU, it is possible that the performance of this model can in part be attributed to clinical acumen rather than changes in patient physiology. The ABG would likely only be obtained in patients with a more concerning or deteriorating clinical course, and thus more likely to develop shock. To address this possibility, a second analysis was performed that did not include the values for the ABG. The result was an algorithm with 13 classification splits, as shown in Figure 3.
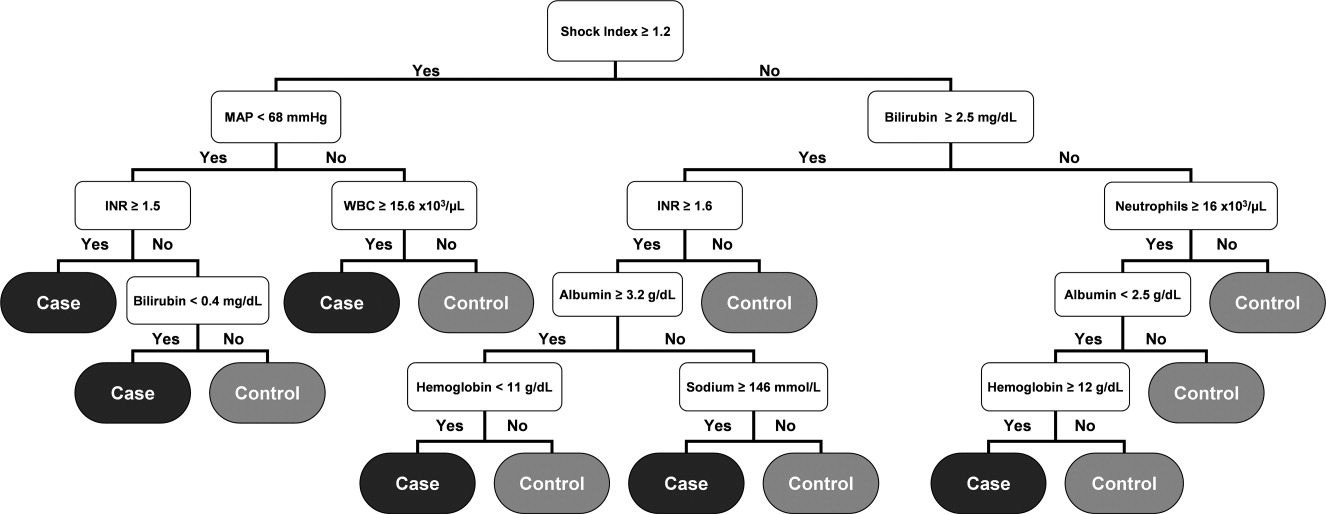
The most predictive clinical variables in this analysis included the shock index (heart rate divided by systolic blood pressure), mean arterial pressure, total bilirubin, international normalized ratio (INR), total white blood cell count, absolute neutrophil count, albumin, hemoglobin, and sodium. This model was again applied to the 2006 and 2007 patient data sets (Table 3).
Total Number | Number Correctly Classified (%) | Case Identification Time Before ICU Admission (minutes) | PPV (%) | NPV (%) | MCR (%) | |
---|---|---|---|---|---|---|
| ||||||
2005 | 20.5 | 96.7 | 6.7 | |||
Cases | 562 | 126 (22.4) | ||||
Controls | 13,223 | 12,735 (96.3) | ||||
2006 | 508 536 | 21.4 | 96.1 | 7.0 | ||
Cases | 635 | 121 (19.1) | ||||
Controls | 13,102 | 12,657 (96.6) | ||||
2007 | 496 512 | 19.5 | 95.8 | 7.1 | ||
Cases | 667 | 102 (15.3) | ||||
Controls | 13,270 | 12,850 (96.8) |
The overall misclassification rates for 2006 and 2007 were 7.0% and 7.1%, respectively. The model correctly identified 121 (19.1%) of the 635 cases and 12,657 (96.6%) of the 13,102 control patients from 2006, and 102 (15.3%) of the 667 cases and 12,850 (96.8%) of the 13,270 control patients from 2007. The respective positive and negative predictive values were 21.4% and 96.1% for 2006, respectively, and 19.5% and 95.8% for 2007, respectively.
Although the overall performance of the model derived without the ABG data was not as good, the identification times prior to ICU transfer were significantly improved. For the 2006 data, patients were identified 508 536 minutes before transfer (Figure 4), compared to 179 230 minutes for the model that included the ABG data (P < 0.01). For the 2007 data, patients were identified 496 512 minutes prior to ICU admission (Figure 4), compared to 192 210 minutes for the previous model (P < 0.01).
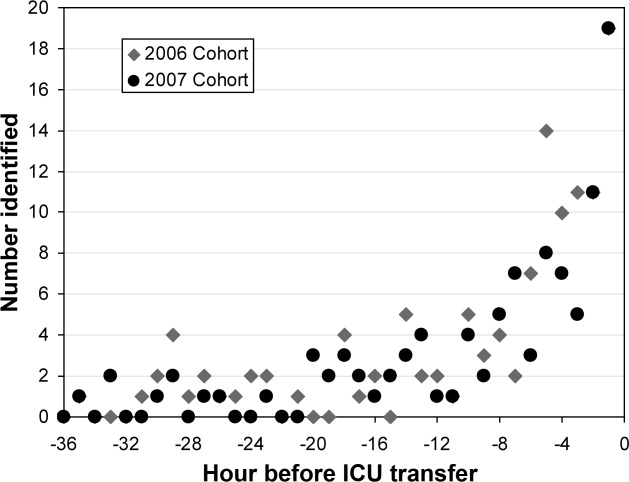
Discussion
We have demonstrated a simple method for generating an algorithm derived from routine laboratory and hemodynamic values that is capable of predicting the onset of sepsis in a significant proportion of non‐ICU patients. Two prediction models were generated, 1 with and 1 without ABG data included in the analysis. In the 2006 and 2007 validation cohorts, the model including these data correctly classified 54.7% and 55.0% of the patients who developed septic shock and 93.4% and 93.0% of control patients, respectively. The second model identified 19.1% and 15.3% of the septic shock patients and 96.6% and 96.8% of the control patients for 2006 and 2007, respectively. The methods used in generating this model are relatively simple and can be executed with the use of an electronic medical record system.
Early, goal‐directed cardiovascular resuscitation and adequate initial antibiotic therapy have been shown to decrease mortality in patients with severe sepsis and septic shock.2, 26 Prior studies employing early, targeted resuscitation strategies have demonstrated decreased use of vasopressors10 and decreased mortality.510 In addition, we previously demonstrated that a standardized order set for the management of severe sepsis in the emergency department that focused on early and aggressive intervention was associated with decreased 28‐day mortality.1 These studies suggest that early, aggressive management of septic shock can improve outcomes. Identification of patients prior to overt clinical deterioration may allow for early intervention aimed at preventing shock or improving its outcome.
The purpose of this method is to develop a model capable of recognizing patterns in clinical data that herald a patient's otherwise unidentified clinical deterioration. It is not intended to replace existing outcome prediction tools or severity of illness scoring systems, where a high degree of accuracy would be required. Rather, it would be best implemented as an automated screening tool incorporated into an electronic medical record system. When a hospitalized patient is identified as a possible septic shock patient by the classification tree, a notification is then issued to the clinicians caring for the patient. The primary goal of this method is to notify clinicians of potential clinical deterioration. Any action taken as a result of this notification is at the discretion of the clinician. This method could be employed for any population of hospitalized patients, though because of variations in clinical practice and patient physiology, different models would need to be generated for differing patient populations.
This method has limitations, the foremost of which is the possible instability of the resulting classification model. This type of analysis results in an algorithm that depends on binary splits to classify patients. In generating the algorithm, the recursive partitioning analysis selects the variables and cutoff values that result in the strongest decision tree with the most pure classifications at the end nodes. These variables and cutoff values may not immediately seem logical from a clinical standpoint, and may vary with changes in practice and even possibly between divisions within a hospital. As a result, the algorithm would likely require intermittent updating to remain effective and a model derived from 1 hospital or patient population would not necessarily be applicable to patients at another institution or from a different population. However, once the method has been developed at an institution, the process of revising the algorithm could be essentially automated and uses few resources.
Another shortcoming of this method is the relatively low sensitivity of the resulting algorithm. In a role as an automated alert system, a low false‐positive rate is particularly desirable to avoid unnecessary frequent distraction of clinicians. The sensitivity of the model can be improved through manipulation of how the analysis is performed, but this would be at the expense of a higher false‐positive rate, which is not acceptable. Finally, prior studies examining treatment for sepsis have demonstrated an advantage to early and aggressive therapy. It is not clear, however, if identifying these patients prior to the onset of clinically evident sepsis would result in improved outcomes. Further work is required to determine if this is the case. We are currently conducting a prospective study that employs the method described here in conjunction with an automated alert system to ascertain if it impacts outcomes on patients admitted to the medicine wards of Barnes‐Jewish Hospital.
In conclusion, the method presented here represents a technique that consumes few resources and is capable of identifying some patients before septic shock becomes clinically evident. When applied in an automated fashion with the capability to alert clinicians caring for a patient, the method demonstrated here may allow for earlier diagnosis and possibly intervention for septic shock patients.
- Before–after study of a standardized hospital order set for the management of septic shock.Crit Care Med.2006;34(11):2707–2713. , , , et al.
- Early goal‐directed therapy in the treatment of severe sepsis and septic shock.N Engl J Med.2001;345:1368–1377. , , , et al.
- Early goal‐directed therapy in severe sepsis and septic shock revisited; concepts, controversies, and contemporary findings.Chest.2006;130(5):1579–1595. , , , et al.
- Implementing the severe sepsis care bundles outside the ICU by outreach.Nurs Crit Care.2007;12:225–229. .
- The impact of compliance with 6‐hour and 24‐hour sepsis bundles on hospital mortality in patients with severe sepsis: a prospective observational study.Crit Care.2005;9:R764–R770. , , , et al.
- Translating research to clinical practice, a 1‐year experience with implementing early goal‐directed therapy for septic shock in the emergency department.Chest.2006;129:225–232. , , , et al.
- Prospective external validation of the clinical effectiveness of an emergency department‐based early goal‐directed therapy protocol for severe sepsis and septic shock.Chest.2007;132:425–432. , , , et al.
- Implementation of a bundle of quality indicators for the early management of severe sepsis and septic shock is associated with decreased mortality.Crit Care Med.2007;35:1105–1112. , , , et al.
- Implementation of an evidence‐based “standard operating procedure” and outcome in septic shock.Crit Care Med.2006;34:943–949. , , , et al.
- Outcome of septic shock in older adults after implementation of the sepsis “bundle”.J Am Geriatr Soc.2008;56:272–278. , , , et al.
- Confidential inquiry into quality of care before admission to intensive care.BMJ.1998;316:1853–1858. , , , et al.
- Unexpected deaths and referrals to intensive care of patients on general wards. Are some cases potentially avoidable?J R Coll Physicians Lond.1999;33(3):255–259. , , .
- Septic shock: an analysis of outcomes for patients with onset on hospital wards versus intensive care units.Crit Care Med.1998;26(6):1020–1024. , , , et al.
- Hospital‐wide impact of a standardized order set for the management of bacteremic severe sepsis.Crit Care Med.2009;37(3):819–824. , , , , , .
- The mRNA expression of fatty acid amide hydrolase in human whole blood correlates with sepsis.J Endotoxin Res.2007;13(1):35–38. , , , , , .
- C‐reactive protein use as an early indicator of infection in patients with systemic inflammatory response syndrome.Intensive Care Med.2004;30(11):2038–2045. , , , et al.
- Procalcitonin as a screening test of late‐onset sepsis in preterm very low birth weight infants.J Perinatol.2005;25(6):397–402. , , , , .
- A simple method for predicting severe sepsis in burn patients.Am J Surg.1980;139(4):513–517. , , .
- Prognostic value of protein C concentrations in neutropenic patients at high risk of severe septic complications.Crit Care Med.2000;28(7):2209–2216. , , , et al.
- Circulating immune parameters predicting the progression from hospital‐acquired pneumonia to septic shock in surgical patients.Crit Care Med.2005;9(6):R662–R669. , , , , .
- A simple prediction algorithm for bacteremia in patients with acute febrile illness.Q J Med.2005;98:813–820. , , .
- Infection probability score (IPS): a method to help assess the probability of infection in critically ill patients.Crit Care Med.2003;31(11):2579–2584. , , , .
- Multivariate regression modeling for the prediction of inflammation, systemic pressure, and end‐organ function in severe sepsis.Shock.1997;8(3):225–231. , .
- Abnormal heart rate characteristics preceding neonatal sepsis and sepsis‐like illness.Pediatr Res.2003;53:920–926. , , , , , .
- Statistics for Biology and Health.New York:Springer‐Verlag;1999. , .
- Impact of adequate empiric antibiotic therapy on the outcome of patients admitted to the intensive care unit with sepsis.Crit Care Med.2003;31:2742–2751. , , , et al.
Severe sepsis is responsible for significant morbidity and mortality. In the United States, approximately 750,000 cases occur each year with an estimated mortality of 30% to 50%.1 Early goal‐directed therapy has been shown to decrease mortality in patients with severe sepsis and septic shock.2, 3 As a result, efforts have been focused toward providing early and aggressive intervention once sepsis has been established. In many cases this has been accomplished through the implementation of a protocol with guidelines for fluid management, antibiotic and vasopressor administration, and other interventions.410 Prior studies have demonstrated that care of hospitalized patients before intensive care unit (ICU) admission is often suboptimal,1113 and have suggested that patients with clear indicators of acute deterioration may go unrecognized on the ward. We previously reported the effects of implementing a hospital‐wide protocol for the management of severe sepsis,14 finding that although there was a significant reduction in overall mortality there was no difference for patients who developed severe sepsis on the hospital ward. This finding also suggests that the initial care of patients with severe sepsis on hospital wards may differ in intensity compared to emergency departments and ICUs. Failure on the part of the clinician to recognize the harbingers of impending sepsis before the onset of organ dysfunction or hypotension may contribute to a delay in aggressive therapy.
Previous efforts at early recognition of sepsis have relied on diagnostic studies or specific biomarkers to screen at‐risk patients. These have included such studies as messenger RNA (mRNA) expression,15 C‐reactive protein,16 procalcitonin in newborns,17 immunocompetence measures in burn patients,18 protein C concentration in neutropenic patients,19 and several immune markers (eg, tumor necrosis factor‐alpha, interleukin [IL]‐1 beta, IL‐6, IL‐8, and IL‐10).20 However, these biomarkers have been studied only in specific patient populations, require suspicion on the part of the clinician and the measurement of diagnostic or laboratory values that would otherwise not have been obtained. The ideal tool for predicting the onset of sepsis would be applicable to a broad patient population, not require specific suspicion on the part of the clinician, and use only routinely obtained clinical measurements and laboratory values.
Prediction models and scoring systems that use routine hemodynamic and laboratory values for several endpoints related to sepsis and septic shock have been developed. Many such tools are used to define severity of illness and predict outcome, while others have been developed to predict such events as bacteremia in patients presenting with fever,21 the probability of infection in the critically ill,22 and end‐organ dysfunction in severe sepsis.23 Little work has been done to develop such a model capable of predicting the onset of sepsis,24 and there have been no attempts to deploy a model as a large‐scale screening tool.
Our objective was to develop a simple algorithm that can be used in an automated fashion to screen hospitalized patients for impending septic shock. Such a model would be derived from routine hemodynamic and laboratory values, and take advantage of a computerized medical record system for data collection.
Patients and Methods
Patient Enrollment and Data Collection
This study was conducted at Barnes‐Jewish Hospital, St. Louis, MO, a university‐affiliated, urban teaching hospital. The study was approved by the Washington University (St. Louis, MO) School of Medicine Human Studies Committee. Patients included in the study where those hospitalized during 2005, 2006, and 2007, and who had at least 1 International Statistical Classification of Diseases and Related Health Problems, 9th edition (ICD9) discharge diagnosis code for the medical/nonsurgical diagnoses listed in Appendix 1. From this pool of patients, septic shock patients were identified as those who were admitted to the hospital ward and later developed septic shock requiring transfer to an ICU for vasopressor support and hemodynamic monitoring. This was accomplished by using discharge ICD9 codes for acute infection matched to codes for acute organ dysfunction and the need for vasopressors within 24 hours of ICU transfer (Appendix 1). The patients used as controls were then all those remaining in the pool once the septic shock patients were identified and separated.
Case patients were excluded from the analysis if they were transferred to the ICU within 2 hours of hospital admission, as these patients are unlikely to have an adequate amount of pretransfer clinical data available for analysis. Both case and control patients were excluded if they lacked any value for basic, routine laboratory data (serum sodium, chloride, total bicarbonate, urea nitrogen, creatinine, glucose, white blood cell count, neutrophil count, hemoglobin, hematocrit, and platelet count) and certain vital signs (blood pressure, heart rate, temperature). Patient data from 2005 were used in the derivation of the prediction model, and 2006 and 2007 patient data were used to prospectively validate the model. Clinical variables used in the analysis were selected based on both ease of access from the electronic medical record and clinical relevance, and are shown in Table 1.
|
Age (years) |
Albumin (g/dL) |
Arterial blood gas (pH, PaCO2, PaO2) |
Anion gap |
Bilirubin (mg/dL) |
BP, systolic and diastolic (mm of Hg) |
Blood urea nitrogen (mg/dL) |
Chloride (mmol/L) |
Creatinine (mg/dL) |
Glucose (mg/dL) |
Hemoglobin (g/dL) |
International normalized ratio |
Neutrophil count, absolute (1 103/L) |
Platelet count (1 103/L) |
Pulse (beats/minute) |
Pulse pressure (mm of Hg) |
Shock index (pulse divided by systolic BP) |
Sodium (mmol/L) |
Total bicarbonate (mmol/L) |
Temperature (degrees Celsius) |
White blood cell count (1 103/L) |
In performing the Recursive Partitioning And Regression Tree (RPART) analysis to generate a prediction model, data for case patients were extracted in a window from 24 hours to 2 hours before ICU admission. The data collection window excluded the 2 hours prior to ICU transfer in order to minimize the effect of acute hemodynamic or laboratory changes that may have prompted the transfer; the purpose of the model is to identify hemodynamic and laboratory patterns in the several hours before the onset of clinically evident shock, so data from a time during which impending shock was clinically apparent were excluded. For the control patients, data from the first 48 hours of their hospitalization were included in the analysis.
Statistical Analysis
RPART analysis was performed on the 2005 patient data set to generate a prediction algorithm. This method of analysis results in a classification tree that contains a series of binary splits designed to separate patients into mutually exclusive subgroups.25 Each split in the tree is selected based on its ability to produce a partition with the greatest purity. Initially, a large tree that contains splits for all input variables is generated. This initial tree is generally too large to be useful as the final subgroups are too small to make sensible statistical inference.25 A pruning process is then applied to the initial tree with the goal of finding the subtree that is most predictive of the outcome of interest. The analysis was done using the RPART package of the R statistical analysis program, version 2.7.0 (R: A Language and Environment for Statistical Computing, R Development Core Team, Foundation for Statistical Computing, Vienna, Austria). The resulting classification tree was then used as a prediction algorithm and applied in a prospective fashion to the 2006 and 2007 patient data sets.
For the purpose of performing the RPART analysis, each set of case data entered into the analysis consisted of a random extraction of the desired clinical data within the specified extraction window from a single case patient. Thus, if a case patient had more than 1 value available for any variable of interest, 1 value was randomly selected to be entered in combination with the other available clinical data. Furthermore, in order to ensure that the majority of case patient data were included in the analysis, this process was iterated 10 times for each case patient. This resulted in 10 sets of case patient data being entered into the analysis for each case patient in the database, with each set containing a value for all variables of interest randomly extracted from those available for that patient. In addition to ensuring that the majority of case patient data were included, this technique also functionally expands the number of case patients present in the analysis. As there were far more control patients than case patients in the database, this in turn results in a classification tree that does not simply identify controls without regard to the relatively small number of case patients.
Data for the control patients entered into the analysis were extracted in a similar fashion, though only 1 set of data were included in the analysis for each control patient present in the database. As a result, only 1 randomly selected value per variable was included in the analysis.
Results
Patients
During 2005, 562 septic patients and 13,223 control patients were identified. For 2006 and 2007 there were 635 and 667 case patients, and 13,102 and 13,270 control patients, respectively.
Predictors of Sepsis
RPART analysis of the 2005 patient data set demonstrated that the most significant predictors of sepsis in the 24 hours preceding transfer to the medical ICU were the partial pressure of arterial oxygen (PaO2), systolic blood pressure, absolute neutrophil count, blood urea nitrogen (BUN), pH, bicarbonate, chloride, and albumin. This resulted in a simple algorithm with nine classification splits (Figure 1), which was then prospectively applied to the 2006 and 2007 patient data sets. These results are summarized in Table 2.
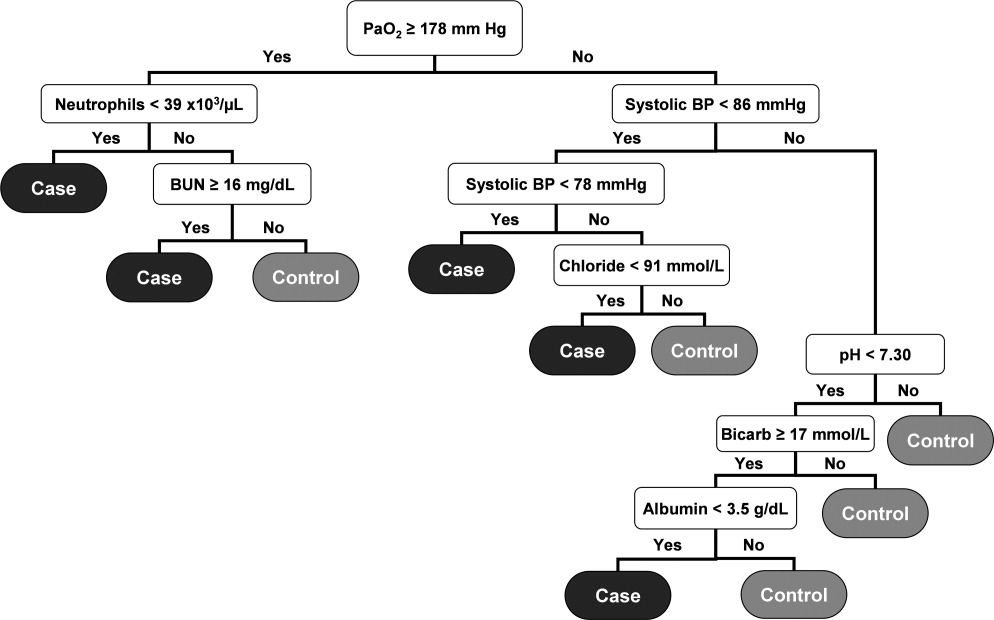
Total Number | Number Correctly Classified (%) | Case Identification Time Before ICU Admission (minutes) | PPV (%) | NPV (%) | MCR (%) | |
---|---|---|---|---|---|---|
| ||||||
2005 | 27.9 | 98.1 | 7.8 | |||
Cases | 562 | 320 (56.9) | ||||
Controls | 13,223 | 12,394 (93.7) | ||||
2006 | 179 230 | 28.7 | 97.7 | 8.4 | ||
Cases | 635 | 347 (54.7) | ||||
Controls | 13,102 | 12,241 (93.4) | ||||
2007 | 192 210 | 28.3 | 97.6 | 8.8 | ||
Cases | 667 | 367 (55.0) | ||||
Controls | 13,270 | 12,341 (93.0) |
The resulting classification model had a low total misclassification rate for the 2005 data. Of the 562 septic patients, 320 (56.9%) were correctly classified, and 12,394 (93.7%) of the control patients were appropriately identified. The number of septic and control patients misclassified was 242 and 829, respectively, yielding a total misclassification rate of 7.8%. When applied to the 2006 patient data set, 347 (54.7%) of the 635 septic shock patients were correctly identified, while 12,241 (93.4%) of the 13,102 control patients were correctly classified. The total misclassification rate for the 2006 patient set was 8.4%. For the 2007 patient data, 367 (55.0%) of the 667 case patients were correctly identified, and 12,341 (93.0%) of the 13,270 control patients were correctly identified. This resulted in a total misclassification rate of 8.8%.
The 2006 and 2007 case patients were identified 179 230 minutes and 192 210 minutes before ICU transfer, respectively (Figure 2). The algorithm demonstrated positive and negative predictive values of 28.7% and 97.7% for the 2006 patient set, respectively, and 28.3% and 97.6% for the 2007 patient set, respectively.
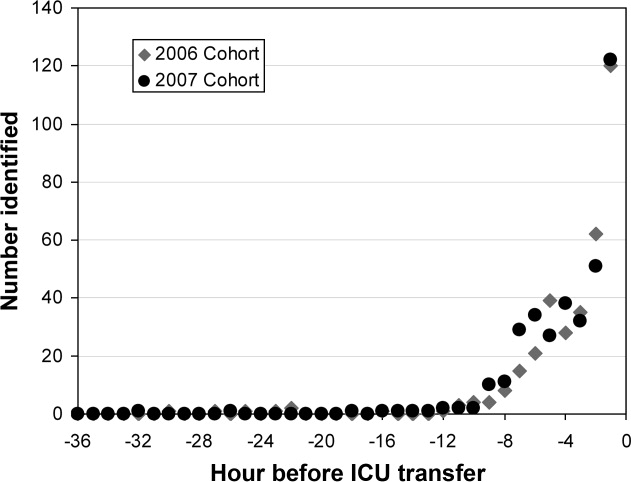
Although the prediction algorithm shown in Figure 1 identified the majority of the case patients with ample time for clinical intervention prior to ICU transfer, the analysis used to derive this model included values for the arterial blood gas (ABG). As this is not a routinely obtained study for hospitalized patients outside of an ICU, it is possible that the performance of this model can in part be attributed to clinical acumen rather than changes in patient physiology. The ABG would likely only be obtained in patients with a more concerning or deteriorating clinical course, and thus more likely to develop shock. To address this possibility, a second analysis was performed that did not include the values for the ABG. The result was an algorithm with 13 classification splits, as shown in Figure 3.
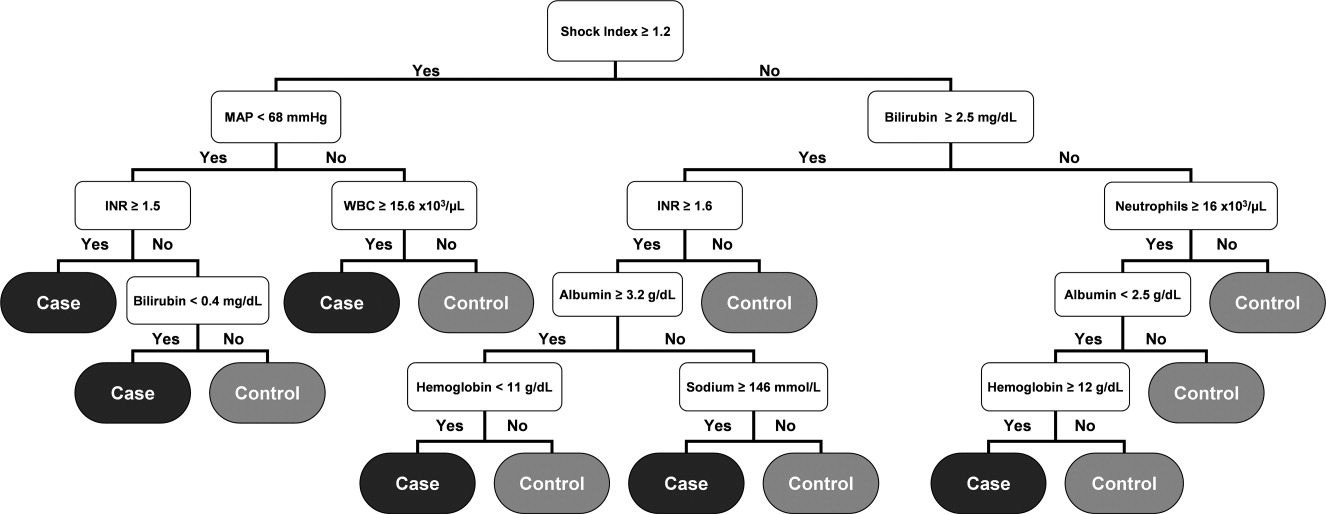
The most predictive clinical variables in this analysis included the shock index (heart rate divided by systolic blood pressure), mean arterial pressure, total bilirubin, international normalized ratio (INR), total white blood cell count, absolute neutrophil count, albumin, hemoglobin, and sodium. This model was again applied to the 2006 and 2007 patient data sets (Table 3).
Total Number | Number Correctly Classified (%) | Case Identification Time Before ICU Admission (minutes) | PPV (%) | NPV (%) | MCR (%) | |
---|---|---|---|---|---|---|
| ||||||
2005 | 20.5 | 96.7 | 6.7 | |||
Cases | 562 | 126 (22.4) | ||||
Controls | 13,223 | 12,735 (96.3) | ||||
2006 | 508 536 | 21.4 | 96.1 | 7.0 | ||
Cases | 635 | 121 (19.1) | ||||
Controls | 13,102 | 12,657 (96.6) | ||||
2007 | 496 512 | 19.5 | 95.8 | 7.1 | ||
Cases | 667 | 102 (15.3) | ||||
Controls | 13,270 | 12,850 (96.8) |
The overall misclassification rates for 2006 and 2007 were 7.0% and 7.1%, respectively. The model correctly identified 121 (19.1%) of the 635 cases and 12,657 (96.6%) of the 13,102 control patients from 2006, and 102 (15.3%) of the 667 cases and 12,850 (96.8%) of the 13,270 control patients from 2007. The respective positive and negative predictive values were 21.4% and 96.1% for 2006, respectively, and 19.5% and 95.8% for 2007, respectively.
Although the overall performance of the model derived without the ABG data was not as good, the identification times prior to ICU transfer were significantly improved. For the 2006 data, patients were identified 508 536 minutes before transfer (Figure 4), compared to 179 230 minutes for the model that included the ABG data (P < 0.01). For the 2007 data, patients were identified 496 512 minutes prior to ICU admission (Figure 4), compared to 192 210 minutes for the previous model (P < 0.01).
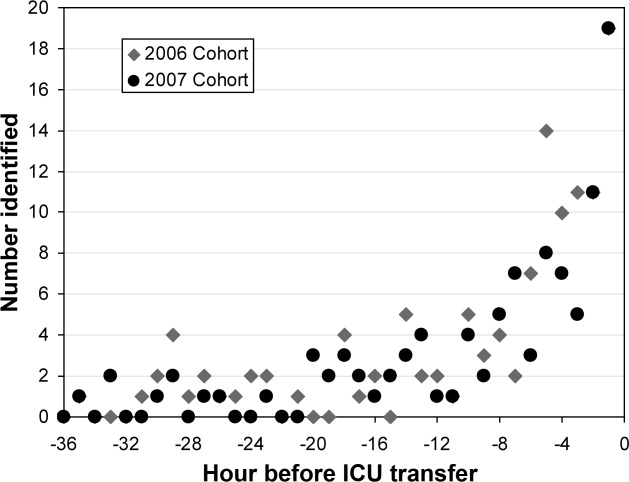
Discussion
We have demonstrated a simple method for generating an algorithm derived from routine laboratory and hemodynamic values that is capable of predicting the onset of sepsis in a significant proportion of non‐ICU patients. Two prediction models were generated, 1 with and 1 without ABG data included in the analysis. In the 2006 and 2007 validation cohorts, the model including these data correctly classified 54.7% and 55.0% of the patients who developed septic shock and 93.4% and 93.0% of control patients, respectively. The second model identified 19.1% and 15.3% of the septic shock patients and 96.6% and 96.8% of the control patients for 2006 and 2007, respectively. The methods used in generating this model are relatively simple and can be executed with the use of an electronic medical record system.
Early, goal‐directed cardiovascular resuscitation and adequate initial antibiotic therapy have been shown to decrease mortality in patients with severe sepsis and septic shock.2, 26 Prior studies employing early, targeted resuscitation strategies have demonstrated decreased use of vasopressors10 and decreased mortality.510 In addition, we previously demonstrated that a standardized order set for the management of severe sepsis in the emergency department that focused on early and aggressive intervention was associated with decreased 28‐day mortality.1 These studies suggest that early, aggressive management of septic shock can improve outcomes. Identification of patients prior to overt clinical deterioration may allow for early intervention aimed at preventing shock or improving its outcome.
The purpose of this method is to develop a model capable of recognizing patterns in clinical data that herald a patient's otherwise unidentified clinical deterioration. It is not intended to replace existing outcome prediction tools or severity of illness scoring systems, where a high degree of accuracy would be required. Rather, it would be best implemented as an automated screening tool incorporated into an electronic medical record system. When a hospitalized patient is identified as a possible septic shock patient by the classification tree, a notification is then issued to the clinicians caring for the patient. The primary goal of this method is to notify clinicians of potential clinical deterioration. Any action taken as a result of this notification is at the discretion of the clinician. This method could be employed for any population of hospitalized patients, though because of variations in clinical practice and patient physiology, different models would need to be generated for differing patient populations.
This method has limitations, the foremost of which is the possible instability of the resulting classification model. This type of analysis results in an algorithm that depends on binary splits to classify patients. In generating the algorithm, the recursive partitioning analysis selects the variables and cutoff values that result in the strongest decision tree with the most pure classifications at the end nodes. These variables and cutoff values may not immediately seem logical from a clinical standpoint, and may vary with changes in practice and even possibly between divisions within a hospital. As a result, the algorithm would likely require intermittent updating to remain effective and a model derived from 1 hospital or patient population would not necessarily be applicable to patients at another institution or from a different population. However, once the method has been developed at an institution, the process of revising the algorithm could be essentially automated and uses few resources.
Another shortcoming of this method is the relatively low sensitivity of the resulting algorithm. In a role as an automated alert system, a low false‐positive rate is particularly desirable to avoid unnecessary frequent distraction of clinicians. The sensitivity of the model can be improved through manipulation of how the analysis is performed, but this would be at the expense of a higher false‐positive rate, which is not acceptable. Finally, prior studies examining treatment for sepsis have demonstrated an advantage to early and aggressive therapy. It is not clear, however, if identifying these patients prior to the onset of clinically evident sepsis would result in improved outcomes. Further work is required to determine if this is the case. We are currently conducting a prospective study that employs the method described here in conjunction with an automated alert system to ascertain if it impacts outcomes on patients admitted to the medicine wards of Barnes‐Jewish Hospital.
In conclusion, the method presented here represents a technique that consumes few resources and is capable of identifying some patients before septic shock becomes clinically evident. When applied in an automated fashion with the capability to alert clinicians caring for a patient, the method demonstrated here may allow for earlier diagnosis and possibly intervention for septic shock patients.
Severe sepsis is responsible for significant morbidity and mortality. In the United States, approximately 750,000 cases occur each year with an estimated mortality of 30% to 50%.1 Early goal‐directed therapy has been shown to decrease mortality in patients with severe sepsis and septic shock.2, 3 As a result, efforts have been focused toward providing early and aggressive intervention once sepsis has been established. In many cases this has been accomplished through the implementation of a protocol with guidelines for fluid management, antibiotic and vasopressor administration, and other interventions.410 Prior studies have demonstrated that care of hospitalized patients before intensive care unit (ICU) admission is often suboptimal,1113 and have suggested that patients with clear indicators of acute deterioration may go unrecognized on the ward. We previously reported the effects of implementing a hospital‐wide protocol for the management of severe sepsis,14 finding that although there was a significant reduction in overall mortality there was no difference for patients who developed severe sepsis on the hospital ward. This finding also suggests that the initial care of patients with severe sepsis on hospital wards may differ in intensity compared to emergency departments and ICUs. Failure on the part of the clinician to recognize the harbingers of impending sepsis before the onset of organ dysfunction or hypotension may contribute to a delay in aggressive therapy.
Previous efforts at early recognition of sepsis have relied on diagnostic studies or specific biomarkers to screen at‐risk patients. These have included such studies as messenger RNA (mRNA) expression,15 C‐reactive protein,16 procalcitonin in newborns,17 immunocompetence measures in burn patients,18 protein C concentration in neutropenic patients,19 and several immune markers (eg, tumor necrosis factor‐alpha, interleukin [IL]‐1 beta, IL‐6, IL‐8, and IL‐10).20 However, these biomarkers have been studied only in specific patient populations, require suspicion on the part of the clinician and the measurement of diagnostic or laboratory values that would otherwise not have been obtained. The ideal tool for predicting the onset of sepsis would be applicable to a broad patient population, not require specific suspicion on the part of the clinician, and use only routinely obtained clinical measurements and laboratory values.
Prediction models and scoring systems that use routine hemodynamic and laboratory values for several endpoints related to sepsis and septic shock have been developed. Many such tools are used to define severity of illness and predict outcome, while others have been developed to predict such events as bacteremia in patients presenting with fever,21 the probability of infection in the critically ill,22 and end‐organ dysfunction in severe sepsis.23 Little work has been done to develop such a model capable of predicting the onset of sepsis,24 and there have been no attempts to deploy a model as a large‐scale screening tool.
Our objective was to develop a simple algorithm that can be used in an automated fashion to screen hospitalized patients for impending septic shock. Such a model would be derived from routine hemodynamic and laboratory values, and take advantage of a computerized medical record system for data collection.
Patients and Methods
Patient Enrollment and Data Collection
This study was conducted at Barnes‐Jewish Hospital, St. Louis, MO, a university‐affiliated, urban teaching hospital. The study was approved by the Washington University (St. Louis, MO) School of Medicine Human Studies Committee. Patients included in the study where those hospitalized during 2005, 2006, and 2007, and who had at least 1 International Statistical Classification of Diseases and Related Health Problems, 9th edition (ICD9) discharge diagnosis code for the medical/nonsurgical diagnoses listed in Appendix 1. From this pool of patients, septic shock patients were identified as those who were admitted to the hospital ward and later developed septic shock requiring transfer to an ICU for vasopressor support and hemodynamic monitoring. This was accomplished by using discharge ICD9 codes for acute infection matched to codes for acute organ dysfunction and the need for vasopressors within 24 hours of ICU transfer (Appendix 1). The patients used as controls were then all those remaining in the pool once the septic shock patients were identified and separated.
Case patients were excluded from the analysis if they were transferred to the ICU within 2 hours of hospital admission, as these patients are unlikely to have an adequate amount of pretransfer clinical data available for analysis. Both case and control patients were excluded if they lacked any value for basic, routine laboratory data (serum sodium, chloride, total bicarbonate, urea nitrogen, creatinine, glucose, white blood cell count, neutrophil count, hemoglobin, hematocrit, and platelet count) and certain vital signs (blood pressure, heart rate, temperature). Patient data from 2005 were used in the derivation of the prediction model, and 2006 and 2007 patient data were used to prospectively validate the model. Clinical variables used in the analysis were selected based on both ease of access from the electronic medical record and clinical relevance, and are shown in Table 1.
|
Age (years) |
Albumin (g/dL) |
Arterial blood gas (pH, PaCO2, PaO2) |
Anion gap |
Bilirubin (mg/dL) |
BP, systolic and diastolic (mm of Hg) |
Blood urea nitrogen (mg/dL) |
Chloride (mmol/L) |
Creatinine (mg/dL) |
Glucose (mg/dL) |
Hemoglobin (g/dL) |
International normalized ratio |
Neutrophil count, absolute (1 103/L) |
Platelet count (1 103/L) |
Pulse (beats/minute) |
Pulse pressure (mm of Hg) |
Shock index (pulse divided by systolic BP) |
Sodium (mmol/L) |
Total bicarbonate (mmol/L) |
Temperature (degrees Celsius) |
White blood cell count (1 103/L) |
In performing the Recursive Partitioning And Regression Tree (RPART) analysis to generate a prediction model, data for case patients were extracted in a window from 24 hours to 2 hours before ICU admission. The data collection window excluded the 2 hours prior to ICU transfer in order to minimize the effect of acute hemodynamic or laboratory changes that may have prompted the transfer; the purpose of the model is to identify hemodynamic and laboratory patterns in the several hours before the onset of clinically evident shock, so data from a time during which impending shock was clinically apparent were excluded. For the control patients, data from the first 48 hours of their hospitalization were included in the analysis.
Statistical Analysis
RPART analysis was performed on the 2005 patient data set to generate a prediction algorithm. This method of analysis results in a classification tree that contains a series of binary splits designed to separate patients into mutually exclusive subgroups.25 Each split in the tree is selected based on its ability to produce a partition with the greatest purity. Initially, a large tree that contains splits for all input variables is generated. This initial tree is generally too large to be useful as the final subgroups are too small to make sensible statistical inference.25 A pruning process is then applied to the initial tree with the goal of finding the subtree that is most predictive of the outcome of interest. The analysis was done using the RPART package of the R statistical analysis program, version 2.7.0 (R: A Language and Environment for Statistical Computing, R Development Core Team, Foundation for Statistical Computing, Vienna, Austria). The resulting classification tree was then used as a prediction algorithm and applied in a prospective fashion to the 2006 and 2007 patient data sets.
For the purpose of performing the RPART analysis, each set of case data entered into the analysis consisted of a random extraction of the desired clinical data within the specified extraction window from a single case patient. Thus, if a case patient had more than 1 value available for any variable of interest, 1 value was randomly selected to be entered in combination with the other available clinical data. Furthermore, in order to ensure that the majority of case patient data were included in the analysis, this process was iterated 10 times for each case patient. This resulted in 10 sets of case patient data being entered into the analysis for each case patient in the database, with each set containing a value for all variables of interest randomly extracted from those available for that patient. In addition to ensuring that the majority of case patient data were included, this technique also functionally expands the number of case patients present in the analysis. As there were far more control patients than case patients in the database, this in turn results in a classification tree that does not simply identify controls without regard to the relatively small number of case patients.
Data for the control patients entered into the analysis were extracted in a similar fashion, though only 1 set of data were included in the analysis for each control patient present in the database. As a result, only 1 randomly selected value per variable was included in the analysis.
Results
Patients
During 2005, 562 septic patients and 13,223 control patients were identified. For 2006 and 2007 there were 635 and 667 case patients, and 13,102 and 13,270 control patients, respectively.
Predictors of Sepsis
RPART analysis of the 2005 patient data set demonstrated that the most significant predictors of sepsis in the 24 hours preceding transfer to the medical ICU were the partial pressure of arterial oxygen (PaO2), systolic blood pressure, absolute neutrophil count, blood urea nitrogen (BUN), pH, bicarbonate, chloride, and albumin. This resulted in a simple algorithm with nine classification splits (Figure 1), which was then prospectively applied to the 2006 and 2007 patient data sets. These results are summarized in Table 2.
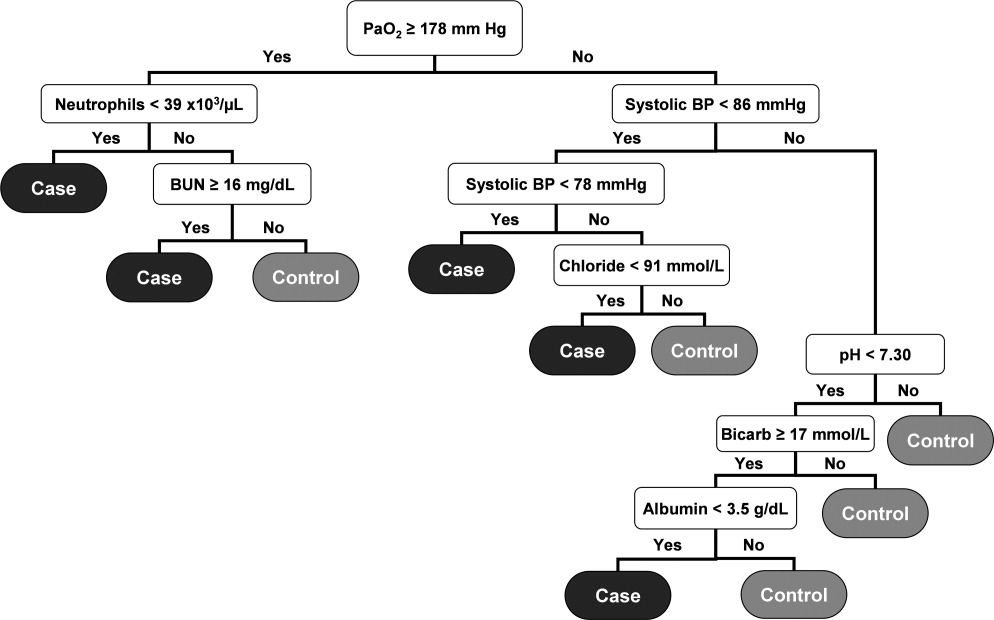
Total Number | Number Correctly Classified (%) | Case Identification Time Before ICU Admission (minutes) | PPV (%) | NPV (%) | MCR (%) | |
---|---|---|---|---|---|---|
| ||||||
2005 | 27.9 | 98.1 | 7.8 | |||
Cases | 562 | 320 (56.9) | ||||
Controls | 13,223 | 12,394 (93.7) | ||||
2006 | 179 230 | 28.7 | 97.7 | 8.4 | ||
Cases | 635 | 347 (54.7) | ||||
Controls | 13,102 | 12,241 (93.4) | ||||
2007 | 192 210 | 28.3 | 97.6 | 8.8 | ||
Cases | 667 | 367 (55.0) | ||||
Controls | 13,270 | 12,341 (93.0) |
The resulting classification model had a low total misclassification rate for the 2005 data. Of the 562 septic patients, 320 (56.9%) were correctly classified, and 12,394 (93.7%) of the control patients were appropriately identified. The number of septic and control patients misclassified was 242 and 829, respectively, yielding a total misclassification rate of 7.8%. When applied to the 2006 patient data set, 347 (54.7%) of the 635 septic shock patients were correctly identified, while 12,241 (93.4%) of the 13,102 control patients were correctly classified. The total misclassification rate for the 2006 patient set was 8.4%. For the 2007 patient data, 367 (55.0%) of the 667 case patients were correctly identified, and 12,341 (93.0%) of the 13,270 control patients were correctly identified. This resulted in a total misclassification rate of 8.8%.
The 2006 and 2007 case patients were identified 179 230 minutes and 192 210 minutes before ICU transfer, respectively (Figure 2). The algorithm demonstrated positive and negative predictive values of 28.7% and 97.7% for the 2006 patient set, respectively, and 28.3% and 97.6% for the 2007 patient set, respectively.
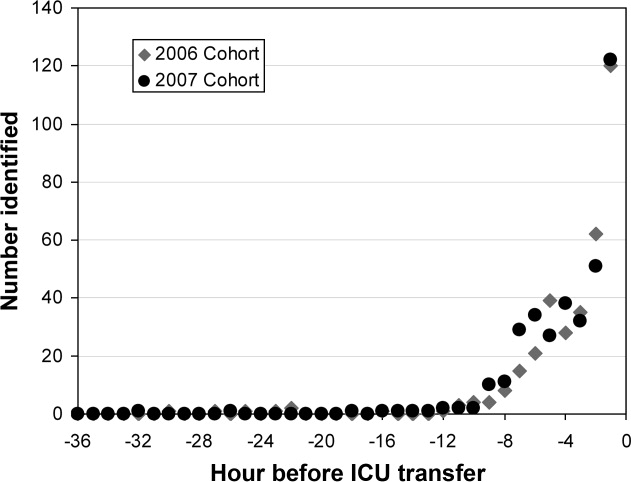
Although the prediction algorithm shown in Figure 1 identified the majority of the case patients with ample time for clinical intervention prior to ICU transfer, the analysis used to derive this model included values for the arterial blood gas (ABG). As this is not a routinely obtained study for hospitalized patients outside of an ICU, it is possible that the performance of this model can in part be attributed to clinical acumen rather than changes in patient physiology. The ABG would likely only be obtained in patients with a more concerning or deteriorating clinical course, and thus more likely to develop shock. To address this possibility, a second analysis was performed that did not include the values for the ABG. The result was an algorithm with 13 classification splits, as shown in Figure 3.
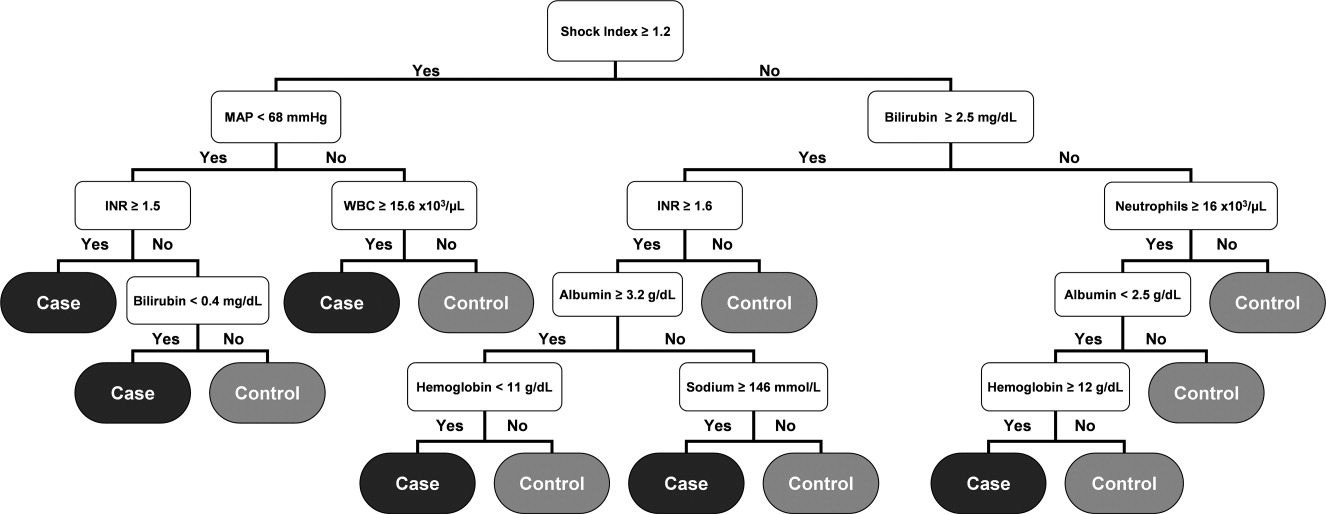
The most predictive clinical variables in this analysis included the shock index (heart rate divided by systolic blood pressure), mean arterial pressure, total bilirubin, international normalized ratio (INR), total white blood cell count, absolute neutrophil count, albumin, hemoglobin, and sodium. This model was again applied to the 2006 and 2007 patient data sets (Table 3).
Total Number | Number Correctly Classified (%) | Case Identification Time Before ICU Admission (minutes) | PPV (%) | NPV (%) | MCR (%) | |
---|---|---|---|---|---|---|
| ||||||
2005 | 20.5 | 96.7 | 6.7 | |||
Cases | 562 | 126 (22.4) | ||||
Controls | 13,223 | 12,735 (96.3) | ||||
2006 | 508 536 | 21.4 | 96.1 | 7.0 | ||
Cases | 635 | 121 (19.1) | ||||
Controls | 13,102 | 12,657 (96.6) | ||||
2007 | 496 512 | 19.5 | 95.8 | 7.1 | ||
Cases | 667 | 102 (15.3) | ||||
Controls | 13,270 | 12,850 (96.8) |
The overall misclassification rates for 2006 and 2007 were 7.0% and 7.1%, respectively. The model correctly identified 121 (19.1%) of the 635 cases and 12,657 (96.6%) of the 13,102 control patients from 2006, and 102 (15.3%) of the 667 cases and 12,850 (96.8%) of the 13,270 control patients from 2007. The respective positive and negative predictive values were 21.4% and 96.1% for 2006, respectively, and 19.5% and 95.8% for 2007, respectively.
Although the overall performance of the model derived without the ABG data was not as good, the identification times prior to ICU transfer were significantly improved. For the 2006 data, patients were identified 508 536 minutes before transfer (Figure 4), compared to 179 230 minutes for the model that included the ABG data (P < 0.01). For the 2007 data, patients were identified 496 512 minutes prior to ICU admission (Figure 4), compared to 192 210 minutes for the previous model (P < 0.01).
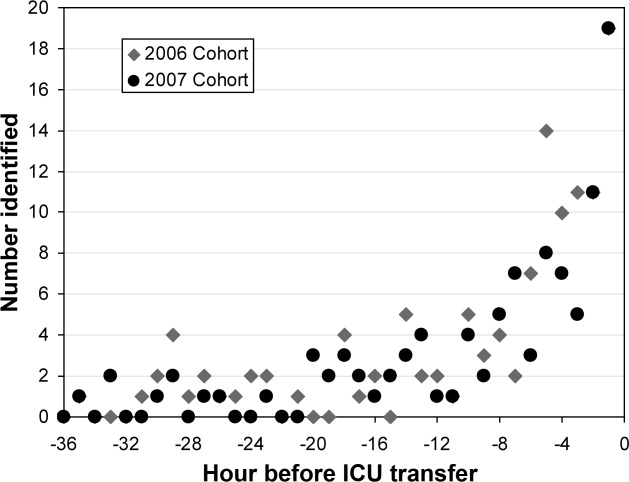
Discussion
We have demonstrated a simple method for generating an algorithm derived from routine laboratory and hemodynamic values that is capable of predicting the onset of sepsis in a significant proportion of non‐ICU patients. Two prediction models were generated, 1 with and 1 without ABG data included in the analysis. In the 2006 and 2007 validation cohorts, the model including these data correctly classified 54.7% and 55.0% of the patients who developed septic shock and 93.4% and 93.0% of control patients, respectively. The second model identified 19.1% and 15.3% of the septic shock patients and 96.6% and 96.8% of the control patients for 2006 and 2007, respectively. The methods used in generating this model are relatively simple and can be executed with the use of an electronic medical record system.
Early, goal‐directed cardiovascular resuscitation and adequate initial antibiotic therapy have been shown to decrease mortality in patients with severe sepsis and septic shock.2, 26 Prior studies employing early, targeted resuscitation strategies have demonstrated decreased use of vasopressors10 and decreased mortality.510 In addition, we previously demonstrated that a standardized order set for the management of severe sepsis in the emergency department that focused on early and aggressive intervention was associated with decreased 28‐day mortality.1 These studies suggest that early, aggressive management of septic shock can improve outcomes. Identification of patients prior to overt clinical deterioration may allow for early intervention aimed at preventing shock or improving its outcome.
The purpose of this method is to develop a model capable of recognizing patterns in clinical data that herald a patient's otherwise unidentified clinical deterioration. It is not intended to replace existing outcome prediction tools or severity of illness scoring systems, where a high degree of accuracy would be required. Rather, it would be best implemented as an automated screening tool incorporated into an electronic medical record system. When a hospitalized patient is identified as a possible septic shock patient by the classification tree, a notification is then issued to the clinicians caring for the patient. The primary goal of this method is to notify clinicians of potential clinical deterioration. Any action taken as a result of this notification is at the discretion of the clinician. This method could be employed for any population of hospitalized patients, though because of variations in clinical practice and patient physiology, different models would need to be generated for differing patient populations.
This method has limitations, the foremost of which is the possible instability of the resulting classification model. This type of analysis results in an algorithm that depends on binary splits to classify patients. In generating the algorithm, the recursive partitioning analysis selects the variables and cutoff values that result in the strongest decision tree with the most pure classifications at the end nodes. These variables and cutoff values may not immediately seem logical from a clinical standpoint, and may vary with changes in practice and even possibly between divisions within a hospital. As a result, the algorithm would likely require intermittent updating to remain effective and a model derived from 1 hospital or patient population would not necessarily be applicable to patients at another institution or from a different population. However, once the method has been developed at an institution, the process of revising the algorithm could be essentially automated and uses few resources.
Another shortcoming of this method is the relatively low sensitivity of the resulting algorithm. In a role as an automated alert system, a low false‐positive rate is particularly desirable to avoid unnecessary frequent distraction of clinicians. The sensitivity of the model can be improved through manipulation of how the analysis is performed, but this would be at the expense of a higher false‐positive rate, which is not acceptable. Finally, prior studies examining treatment for sepsis have demonstrated an advantage to early and aggressive therapy. It is not clear, however, if identifying these patients prior to the onset of clinically evident sepsis would result in improved outcomes. Further work is required to determine if this is the case. We are currently conducting a prospective study that employs the method described here in conjunction with an automated alert system to ascertain if it impacts outcomes on patients admitted to the medicine wards of Barnes‐Jewish Hospital.
In conclusion, the method presented here represents a technique that consumes few resources and is capable of identifying some patients before septic shock becomes clinically evident. When applied in an automated fashion with the capability to alert clinicians caring for a patient, the method demonstrated here may allow for earlier diagnosis and possibly intervention for septic shock patients.
- Before–after study of a standardized hospital order set for the management of septic shock.Crit Care Med.2006;34(11):2707–2713. , , , et al.
- Early goal‐directed therapy in the treatment of severe sepsis and septic shock.N Engl J Med.2001;345:1368–1377. , , , et al.
- Early goal‐directed therapy in severe sepsis and septic shock revisited; concepts, controversies, and contemporary findings.Chest.2006;130(5):1579–1595. , , , et al.
- Implementing the severe sepsis care bundles outside the ICU by outreach.Nurs Crit Care.2007;12:225–229. .
- The impact of compliance with 6‐hour and 24‐hour sepsis bundles on hospital mortality in patients with severe sepsis: a prospective observational study.Crit Care.2005;9:R764–R770. , , , et al.
- Translating research to clinical practice, a 1‐year experience with implementing early goal‐directed therapy for septic shock in the emergency department.Chest.2006;129:225–232. , , , et al.
- Prospective external validation of the clinical effectiveness of an emergency department‐based early goal‐directed therapy protocol for severe sepsis and septic shock.Chest.2007;132:425–432. , , , et al.
- Implementation of a bundle of quality indicators for the early management of severe sepsis and septic shock is associated with decreased mortality.Crit Care Med.2007;35:1105–1112. , , , et al.
- Implementation of an evidence‐based “standard operating procedure” and outcome in septic shock.Crit Care Med.2006;34:943–949. , , , et al.
- Outcome of septic shock in older adults after implementation of the sepsis “bundle”.J Am Geriatr Soc.2008;56:272–278. , , , et al.
- Confidential inquiry into quality of care before admission to intensive care.BMJ.1998;316:1853–1858. , , , et al.
- Unexpected deaths and referrals to intensive care of patients on general wards. Are some cases potentially avoidable?J R Coll Physicians Lond.1999;33(3):255–259. , , .
- Septic shock: an analysis of outcomes for patients with onset on hospital wards versus intensive care units.Crit Care Med.1998;26(6):1020–1024. , , , et al.
- Hospital‐wide impact of a standardized order set for the management of bacteremic severe sepsis.Crit Care Med.2009;37(3):819–824. , , , , , .
- The mRNA expression of fatty acid amide hydrolase in human whole blood correlates with sepsis.J Endotoxin Res.2007;13(1):35–38. , , , , , .
- C‐reactive protein use as an early indicator of infection in patients with systemic inflammatory response syndrome.Intensive Care Med.2004;30(11):2038–2045. , , , et al.
- Procalcitonin as a screening test of late‐onset sepsis in preterm very low birth weight infants.J Perinatol.2005;25(6):397–402. , , , , .
- A simple method for predicting severe sepsis in burn patients.Am J Surg.1980;139(4):513–517. , , .
- Prognostic value of protein C concentrations in neutropenic patients at high risk of severe septic complications.Crit Care Med.2000;28(7):2209–2216. , , , et al.
- Circulating immune parameters predicting the progression from hospital‐acquired pneumonia to septic shock in surgical patients.Crit Care Med.2005;9(6):R662–R669. , , , , .
- A simple prediction algorithm for bacteremia in patients with acute febrile illness.Q J Med.2005;98:813–820. , , .
- Infection probability score (IPS): a method to help assess the probability of infection in critically ill patients.Crit Care Med.2003;31(11):2579–2584. , , , .
- Multivariate regression modeling for the prediction of inflammation, systemic pressure, and end‐organ function in severe sepsis.Shock.1997;8(3):225–231. , .
- Abnormal heart rate characteristics preceding neonatal sepsis and sepsis‐like illness.Pediatr Res.2003;53:920–926. , , , , , .
- Statistics for Biology and Health.New York:Springer‐Verlag;1999. , .
- Impact of adequate empiric antibiotic therapy on the outcome of patients admitted to the intensive care unit with sepsis.Crit Care Med.2003;31:2742–2751. , , , et al.
- Before–after study of a standardized hospital order set for the management of septic shock.Crit Care Med.2006;34(11):2707–2713. , , , et al.
- Early goal‐directed therapy in the treatment of severe sepsis and septic shock.N Engl J Med.2001;345:1368–1377. , , , et al.
- Early goal‐directed therapy in severe sepsis and septic shock revisited; concepts, controversies, and contemporary findings.Chest.2006;130(5):1579–1595. , , , et al.
- Implementing the severe sepsis care bundles outside the ICU by outreach.Nurs Crit Care.2007;12:225–229. .
- The impact of compliance with 6‐hour and 24‐hour sepsis bundles on hospital mortality in patients with severe sepsis: a prospective observational study.Crit Care.2005;9:R764–R770. , , , et al.
- Translating research to clinical practice, a 1‐year experience with implementing early goal‐directed therapy for septic shock in the emergency department.Chest.2006;129:225–232. , , , et al.
- Prospective external validation of the clinical effectiveness of an emergency department‐based early goal‐directed therapy protocol for severe sepsis and septic shock.Chest.2007;132:425–432. , , , et al.
- Implementation of a bundle of quality indicators for the early management of severe sepsis and septic shock is associated with decreased mortality.Crit Care Med.2007;35:1105–1112. , , , et al.
- Implementation of an evidence‐based “standard operating procedure” and outcome in septic shock.Crit Care Med.2006;34:943–949. , , , et al.
- Outcome of septic shock in older adults after implementation of the sepsis “bundle”.J Am Geriatr Soc.2008;56:272–278. , , , et al.
- Confidential inquiry into quality of care before admission to intensive care.BMJ.1998;316:1853–1858. , , , et al.
- Unexpected deaths and referrals to intensive care of patients on general wards. Are some cases potentially avoidable?J R Coll Physicians Lond.1999;33(3):255–259. , , .
- Septic shock: an analysis of outcomes for patients with onset on hospital wards versus intensive care units.Crit Care Med.1998;26(6):1020–1024. , , , et al.
- Hospital‐wide impact of a standardized order set for the management of bacteremic severe sepsis.Crit Care Med.2009;37(3):819–824. , , , , , .
- The mRNA expression of fatty acid amide hydrolase in human whole blood correlates with sepsis.J Endotoxin Res.2007;13(1):35–38. , , , , , .
- C‐reactive protein use as an early indicator of infection in patients with systemic inflammatory response syndrome.Intensive Care Med.2004;30(11):2038–2045. , , , et al.
- Procalcitonin as a screening test of late‐onset sepsis in preterm very low birth weight infants.J Perinatol.2005;25(6):397–402. , , , , .
- A simple method for predicting severe sepsis in burn patients.Am J Surg.1980;139(4):513–517. , , .
- Prognostic value of protein C concentrations in neutropenic patients at high risk of severe septic complications.Crit Care Med.2000;28(7):2209–2216. , , , et al.
- Circulating immune parameters predicting the progression from hospital‐acquired pneumonia to septic shock in surgical patients.Crit Care Med.2005;9(6):R662–R669. , , , , .
- A simple prediction algorithm for bacteremia in patients with acute febrile illness.Q J Med.2005;98:813–820. , , .
- Infection probability score (IPS): a method to help assess the probability of infection in critically ill patients.Crit Care Med.2003;31(11):2579–2584. , , , .
- Multivariate regression modeling for the prediction of inflammation, systemic pressure, and end‐organ function in severe sepsis.Shock.1997;8(3):225–231. , .
- Abnormal heart rate characteristics preceding neonatal sepsis and sepsis‐like illness.Pediatr Res.2003;53:920–926. , , , , , .
- Statistics for Biology and Health.New York:Springer‐Verlag;1999. , .
- Impact of adequate empiric antibiotic therapy on the outcome of patients admitted to the intensive care unit with sepsis.Crit Care Med.2003;31:2742–2751. , , , et al.
Copyright © 2010 Society of Hospital Medicine