User login
Readmissions in Primary Care Clinics
Reducing hospital readmissions is a national healthcare priority. In October 2012, the Centers for Medicare and Medicaid Services (CMS) enacted financial penalties on hospitals with higher than average risk‐adjusted readmissions, offering an incentive pool of $850 million in the first year.[1] As a result, a wide range of activities to understand and reduce readmissions among patients with congestive heart failure (CHF), acute myocardial infarction (AMI), and pneumonia have emerged.[2, 3, 4, 5] Some have proposed that to effectively reduce hospital readmissions, a community of inpatient and outpatient providers and local support organizations must coordinate efforts.[6, 7] In fact, CMS has recognized the important role of community support groups and outpatient providers in safe discharge transitions in 2 ways. First, in 2011 CMS launched the Community‐based Care Transitions Program to fund community‐based organizations to assist Medicare patients with care transitions.[8] Second, CMS introduced 2 new reimbursement codes for primary care providers (PCPs) to perform care coordination immediately after hospital discharge.[9] Both of these new payment programs represent an evolving perspective that reducing hospital readmissions requires active participation among outpatient partners.
As leaders of the outpatient care team, PCPs play a significant role in reducing hospital readmissions. One way in which PCPs can begin to understand the magnitude of the issue within their clinic is to evaluate the clinic's 30‐day readmission rates. Currently, CMS calculates readmission rates at the hospital level. However, understanding these rates at the clinic level is critical for developing strategies for improvement across the care continuum. Our current understanding of effective outpatient interventions to reduce hospital readmission is limited.[3] As clinics introduce and refine strategies to reduce readmissions, tracking the impact of these strategies on readmission rates will be critical for identifying effective outpatient interventions. Clinics with similar patient case‐mix can also benchmark readmission rates, sharing best practices from clinics with lower‐than‐expected rates.
There are no available studies or proposed methodologies to guide primary care clinics in calculating their 30‐day readmission rates. A particularly difficult challenge is obtaining the admission information when patients may be admitted to 1 of several area hospitals. For large integrated delivery networks where primary care patients are relatively loyal to the network of physicians and hospitals, an opportunity exists to explore the data. Furthermore, variations in readmission rates across primary care specialties (such as internal medicine and family practice) are not well understood. In this study, we set out to develop a methodology for calculating all‐cause 30‐day hospital readmission rates at the level of individual primary care practices and to identify factors associated with variations in these rates.
METHODS
Study Design
We conducted a retrospective observational study of adult primary care patients at the University of California, San Francisco (UCSF) who were hospitalized at UCSF Medical Center between July 1, 2009 and June 30, 2012. UCSF Medical Center is comprised of Moffitt‐Long Hospital (a 600‐bed facility) and UCSF‐Mount Zion Hospital (a 90‐bed facility) located in San Francisco, CA. The patient population was limited to adults ages 18 and over with a PCP at UCSF. UCSF has 7 adult primary care clinics: General Internal Medicine (IM), Family Practice (FP), Women's Health, Geriatrics, a combined IM/FP clinic, Human Immunodeficiency Virus (HIV) Primary Care, and a Concierge Internal Medicine clinic staffed by IM physicians. Between 2009 and 2011, all clinics completed the process of enpanelment, or defining the population of patients for which each PCP and the clinic is responsible. We obtained the list of patient and PCP assignments at each of the 7 clinics across the time period of study. We then obtained UCSF Medical Center hospital claims data for this group, including dates of admission and discharge, patient age, sex, race/ethnicity, language, insurance, admitting service, diagnosis codes, information on intensive care unit stay, and discharge disposition. Hospital claims data are housed in Transition Systems International (Boston, MA) administrative databases, a cost‐accounting system that collects data abstracted from patient charts upon discharge from UCSF Medical Center.
All‐cause 30‐day hospital readmission rates were calculated for each primary care clinic, using an adaptation of the CMS definition. First, we defined index discharges as the first discharge for an individual patient in any given 30‐day interval. Only 1 index discharge is flagged for each 30‐day interval. The first admission within 30 days after the index discharge was flagged as the readmission. Consistent with CMS methodology, only the first readmission in the 30‐day period was counted. We included all inpatient and observation status admissions and excluded patients who died during the index encounter, left against medical advice, or transferred to another acute care hospital after the index encounter.
We used secondary diagnosis codes in the administrative data to classify comorbidities by the Elixhauser methodology.[10] All but 1 adult primary care clinic at UCSF are faculty‐only clinics, whereby the assigned PCP is an attending physician. In the general internal medicine clinic, an attending or a resident can serve as the PCP, and approximately 20% of IM clinic patients have a resident as their PCP. For the IM clinic, we classified patients' PCP as attending, resident, or departed. The latter category refers to patients whose PCPs were residents who had graduated or faculty who had departed and had not been assigned to a new PCP prior to the index admission or readmission.
Statistical Analysis
We built a model to predict readmissions using the demographic and clinical variables with [2] P<0.20 in an initial bivariate analysis, and then removed, with backward selection, the least significant variables until only those with P0.05 remained. Age, log‐length of stay (LOS), and intensive care unit stay were forced in the model, as studies evaluating factors related to readmissions have often included these as important covariates.[11, 12, 13, 14] Results were expressed as adjusted odds ratio (OR) with 95% confidence interval (CI). All analyses were carried out using SAS version 9.2 (SAS Institute, Inc., Cary, NC).
This study was exempt from review by the institutional review board of UCSF.
RESULTS
During the study period, there were 12,564 discharges from UCSF Medical Center for primary care patients belonging to the 7 clinics. Of these, 8685 were index discharges and 1032 were readmissions within 30 days. Table 1 shows the characteristics of the patients who had at least 1 admission during the study period. In all but 2 clinics (HIV Primary Care and Concierge Internal Medicine), there were more women hospitalized than men. Age and gender differences across clinics are consistent with the patient populations served by these clinics, with Women's Health having more female patients and younger patients hospitalized compared to Geriatrics.
General Internal Medicine | Family Practice | Women's Health | Geriatrics | Combined IM/FP | HIV Primary Care | Concierge IM | |
---|---|---|---|---|---|---|---|
| |||||||
Panel size | 26,521 | 10,495 | 8,526 | 190 | 4,109 | 1,039 | 706 |
FTE attending MD and NP providers | 18.8 | 7.3 | 4.5 | 1.7 | 5.0 | 2.3 | 2.1 |
Mean panel size per FTE | 1,311 | 1,448 | 1,895 | 112 | 822 | 452 | 336 |
Clinic visits in FY 2012 | 50,362 | 20,647 | 11,014 | 4,425 | 8,120 | 4,182 | 1,713 |
No. of index discharges | 5,388 | 1,204 | 983 | 409 | 339 | 249 | 113 |
No. of readmissions | 718 | 104 | 76 | 56 | 30 | 37 | 11 |
No. of patients discharged during study period | 4,063 | 1,003 | 818 | 289* | 290 | 185 | 84 |
% Male | 40.1% | 33.9% | 4.3% | 35.3% | 33.8% | 69.7% | 52.4% |
Average age (SD), y | 60.4 (18.6) | 52.0 (19.0) | 47.0 (15.7) | 83.2 (6.9) | 46.3 (16.9) | 49.5 (9.9) | 64.5 (15.6) |
Age range, y | 19104 | 1896 | 1997 | 6399 | 1890 | 2273 | 2092 |
Race | |||||||
% White | 42.2% | 41.3% | 58.8% | 61.9% | 49.3% | 61.0% | 91.7% |
% Black | 16.3% | 8.5% | 7.5% | 7.3% | 10.0% | 27.6% | 0.0% |
% Asian | 23.6% | 31.4% | 19.0% | 17.0% | 16.6% | 2.2% | 4.8% |
% Native America/ Alaskan Native | 0.7% | 1.6% | 0.7% | 0.7% | 0.7% | 0.5% | 0.0% |
% Other | 16.3% | 15.7% | 13.1% | 12.5% | 18.3% | 8.1% | 2.4% |
Not available | 1.0% | 1.6% | 1.0% | 0.7% | 5.2% | 0.5% | 1.2% |
Ethnicity | |||||||
% Hispanic | 8.8% | 10.6% | 5.0% | 6.2% | 8.3% | 4.9% | 0.0% |
% Non‐Hispanic | 73.8% | 74.5% | 81.8% | 73.0% | 74.5% | 84.9% | 75.0% |
Not available | 17.4% | 15.0% | 13.2% | 20.8% | 17.2% | 10.3% | 25.0% |
Language | |||||||
% English | 69.7% | 75.6% | 88.8% | 69.6% | 85.9% | 89.7% | 89.3% |
% Spanish | 3.8% | 1.9% | 0.6% | 4.2% | 1.0% | 0.5% | 0.0% |
% Chinese (Mandarin or Cantonese) | 8.0% | 6.7% | 1.2% | 4.8% | 2.1% | 0.0% | 0.0% |
% Russian | 1.7% | 0.6% | 0.1% | 0.4% | 1.0% | 0.0% | 0.0% |
% Vietnamese | 1.0% | 1.4% | 0.0% | 0.4% | 0.0% | 0.0% | 0.0% |
% Other | 11.7% | 9.3% | 5.6% | 18.0% | 6.9% | 8.1% | 4.8% |
Not available | 4.1% | 4.6% | 3.7% | 2.8% | 3.1% | 1.6% | 6.0% |
Insurance type | |||||||
% Private | 35.4% | 58.7% | 77.4% | 10.7% | 63.8% | 36.8% | 66.7% |
% Medicare | 22.0% | 17.2% | 12.0% | 67.8% | 14.8% | 8.7% | 33.3% |
% Medicaid | 15.5% | 12.5% | 3.1% | 1.4% | 12.8% | 24.3% | 0.0% |
% Dual eligible | 24.4% | 8.8% | 5.4% | 19.4% | 6.6% | 27.6% | 0.0% |
% Self‐pay/other | 2.6% | 2.9% | 2.2% | 0.7% | 2.1% | 2.7% | 0.0% |
All‐cause 30‐day readmission rates varied across practices, with HIV Primary Care being the highest at 14.9%, followed by Geriatrics at 13.7%, General Internal Medicine at 13.3%, Concierge Internal Medicine at 9.7%, combined IM/FP at 8.9%, Family Practice at 8.6%, and Women's Health at 7.7% (Figure 1). Despite HIV Primary Care having the highest readmission rate, the number of index discharges during the 3‐year period was relatively low (249) compared to General Internal Medicine (5388). For the index admission, the top 5 admitting services were medicine, obstetrics, cardiology, orthopedic surgery, and general surgery (Table 2). Medicine was the primary admitting service for patients in the following clinics: General Internal Medicine, Geriatrics, HIV Primary Care, and Concierge Internal Medicine. Obstetrics was the primary admitting service for patients in the Family Practice, Women's Health, and combined IM/FP clinics. Vaginal delivery was the top discharge diagnosis‐related group (DRG) for patients in the General Internal Medicine, Family Practice, Women's Health, and combined IM/FP clinics. The top discharge DRG was urinary tract infection for Geriatrics, HIV for the HIV clinic (13.6%), and chemotherapy (8.0%) for Concierge Internal Medicine. Average LOS varied from 4.7 days for patients in the HIV Primary Care clinic to 2.8 days for patients in the Concierge Internal Medicine clinic. Average LOS was 3.4, 3.1, and 3.2 days in the Family Practice, Women's Health, and Geriatrics clinics, respectively, and 3.8 days in the General Internal Medicine and combined IM/FP clinics. For all clinics except Geriatrics, the majority of patients were discharged home without home health. A larger proportion of patients in the Geriatrics clinic were discharged home with home health or discharged to a skilled nursing facility.
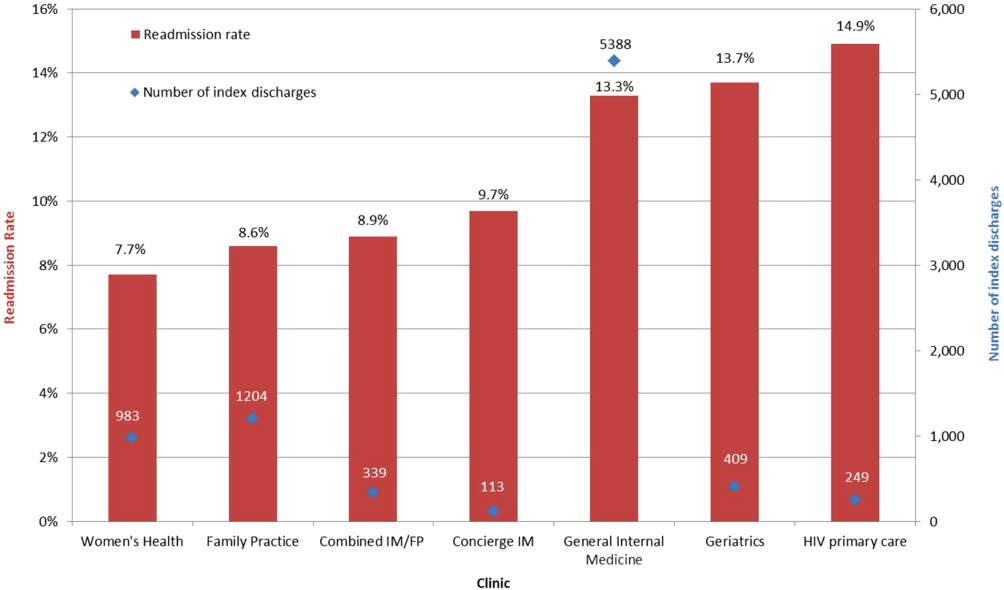
General Internal Medicine, N=5,388 | Family Practice, N=1,204 | Women's Health, N=983 | Geriatrics, N=409 | Combined IM/FP, N=339 | HIV Primary Care, N=249 | Concierge IM, N=113 | |
---|---|---|---|---|---|---|---|
| |||||||
Top 5 admitting services | |||||||
Medicine | 41.8% | 25.0% | 15.2% | 56.4% | 23.5% | 59.7% | 19.0% |
Obstetrics | 7.5% | 24.7% | 38.7% | 0.0% | 35.6% | 2.1% | 9.0% |
Cardiology | 15.1% | 10.9% | 6.2% | 16.5% | 10.3% | 6.5% | 17.0% |
Orthopedic surgery | 8.3% | 8.3% | 8.2% | 7.3% | 5.3% | 4.8% | 12.0% |
Adult general surgery | 7.2% | 9.8% | 10.0% | 6.0% | 5.0% | 5.8% | 7.0% |
Top 5 discharge diagnoses | |||||||
Vaginal delivery | 3.5% | 13.6% | 20.6% | 0.0% | 17.9% | 0.3% | 3.0% |
Major joint replacement | 3.5% | 3.4% | 4.8% | 4.3% | 3.8% | 0.7% | 5.0% |
Vaginal delivery with complications | 1.3% | 5.0% | 6.9% | 0.0% | 4.1% | 1.0% | 2.0% |
Simple pneumonia and pleurisy | 2.8% | 1.2% | 0.6% | 3.8% | 0.3% | 2.1% | 0.0% |
Urinary tract infection | 2.0% | 1.5% | 1.4% | 6.2% | 0.9% | 2.4% | 1.0% |
Discharge disposition | |||||||
% Home | 69.1% | 74.6% | 76.6% | 47.8% | 69.9% | 78.6% | 68.4% |
% Home with home health | 19.4% | 16.5% | 17.8% | 24.1% | 21.7% | 11.8% | 18.8% |
% Skilled nursing facility | 7.2% | 4.9% | 3.0% | 18.9% | 3.3% | 5.0% | 0.8% |
% Other | 4.3% | 4.0% | 2.6% | 9.2% | 5.1% | 4.6% | 12.0% |
Average length of stay (SD) | 3.8 (5.6) | 3.4 (7.5) | 3.1 (3.7) | 3.2 (3.7) | 3.8 (5.2) | 4.7 (6.5) | 2.8 (2.8) |
% Discharges with ICU stay | 11.0% | 10.6% | 5.9% | 8.5% | 9.2% | 11.1% | 15.8% |
Factors associated with variation in readmission rates included: male gender (OR: 1.21, 95% CI: 1.051.40), Medicare (OR: 1.31, 95% CI: 1.051.64; Ref=private) and dual‐eligible Medicare‐Medicaid insurance (OR: 1.26, 95% CI: 1.011.56), unknown primary language (OR: 0.06, 95% CI: 0.020.25; Ref=English), and the following comorbidities: peripheral vascular disease, renal failure, lymphoma, fluid and electrolyte disorders, and anemia. Multivariable logistic regression modeling results are listed in Table 3. Patients having a resident PCP showed no increased odds of readmission (OR: 1.13, 95% CI: 0.931.37; Ref=attending PCP). However, patients with a graduated resident PCP or departed faculty PCP awaiting transfer to a new PCP had an OR of 1.59 (95% CI: 1.162.17) compared with having a current faculty PCP. The C‐statistic for this model was 0.67.
Adjusted Odds Ratio (95% CI) | |
---|---|
| |
Age | |
<65 years | Ref |
>65 years | 0.91 (0.751.10) |
Clinic | |
General Internal Medicine | 1.24 (0.991.56) |
Family Practice | Ref |
Women's Health | 1.13 (0.821.56) |
Geriatrics | 1.20 (0.841.74) |
Combined Internal Medicine/Family Practice | 1.13 (0.731.74) |
HIV Primary Care | 1.24 (0.811.89) |
Concierge Internal Medicine | 1.06 (0.542.07) |
Sex | |
Female | Ref |
Male | 1.21 (1.051.40) |
Insurance | |
Private | Ref |
Medicaid | 1.09 (0.871.37) |
Medicare | 1.31 (1.051.64) |
Dual eligible MedicareMedicaid | 1.26 (1.011.56) |
Self/other | 1.20 (0.741.94) |
Language | |
English | Ref |
Spanish | 1.02 (0.701.47) |
Chinese | 1.13 (0.871.47) |
Other | 0.87 (0.701.08) |
Unknown | 0.06 (0.020.25) |
Comorbidities | |
Pulmonary circulation disease | 1.51 (0.982.32) |
Peripheral vascular disease | 1.50 (1.141.98) |
Renal failure | 1.31 (1.091.58) |
Lymphoma | 2.50 (1.434.36) |
Fluid and electrolyte disorders | 1.27 (1.071.52) |
Deficiency anemia | 1.25 (1.041.51) |
Physician | |
Attending | Ref |
Resident | 1.13 (0.931.37) |
Departed PCP (internal medicine only) | 1.59 (1.162.17) |
ICU stay | 1.03 (0.831.28) |
LOS (log) | 1.02 (0.981.06) |
Admitting service | |
Medicine | Ref |
Obstetrics | 0.41 (0.300.56) |
Cardiology | 1.12 (0.911.37) |
Orthopedic surgery | 0.36 (0.250.51) |
Adult general surgery | 0.64 (0.470.87) |
Other services | 0.76 (0.630.92) |
DISCUSSION
In this study we introduce a complementary way to view hospital readmissions, from the perspective of primary care practices. We found variation in readmission rates across primary care practices. After controlling for admitting service, clinic, provider, and patient factors, the specific characteristics of male gender, patients with Medicare or Medicare with Medicaid, and patients in our General Internal Medicine clinic with a departed PCP were independent risk factors for hospital readmission. Patients with specific comorbidities were also at increased risk for admissions, including those with peripheral vascular disease, renal failure, lymphoma, fluid and electrolyte disorders, and anemia. Because this is the first study viewing readmissions from the perspective of primary care practices, our findings are unique in the literature. However, hospital‐based studies have found similar relationships between readmission rates and these specific comorbidities.[11, 12, 15] Unlike other studies, our study cohort did not show CHF as an independent risk factor. We hypothesize this is because in 2008, UCSF Medical Center introduced an inpatient‐based intervention to reduce readmissions in patients with heart failure. By 2011, the readmission rates of patients with heart failure (primary or secondary diagnoses) had dropped by 30%. The success of this program, focused only on patients with heart failure, likely affected our analysis of comorbidities as risk factors for readmissions.
Models developed to predict hospital readmissions, overall and for specific disease conditions, have inconsistently identified predictive factors, and there is not a specific set of variables that dominate.[16, 17, 18, 19] A recent review of readmission risk prediction models suggested that models that take into account psychosocial factors such as social support, substance abuse, and functional status improved model performance.[16] We hypothesize that the reason why male gender was significant in our model may be related to lack of social support, especially among those who may be single or widowed. Other studies have also showed male gender as a predictor for hospital readmissions.[14, 20]
People with Medicare as the primary payor or Medicare with Medicaid also tended to have higher risk of readmission. We believe that this may be a proxy for the combined effect of age, multiple comorbidities, and psychosocial factors. In a multicenter study of general medicine inpatients, Medicare, but not age, was also found to be a predictive variable.[21] Unlike other studies, our study did not find Medicaid alone as a significant predictive variable for readmissions.[15, 21] One explanation may be that in hospital‐based studies, people with Medicaid who are at high risk for readmission may be high‐risk because they do not have a PCP or good access to outpatient care. In our study, all patients have a PCP in 1 of the UCSF clinics, and access to care is improved with this established relationship. These other studies did not examine Medicare‐Medicaid dual eligibility status. Our results are consistent with a national study on avoidable hospital admissions that showed the dual eligibility population experiencing 60% higher avoidable admission rates compared to the Medicaid‐only population.[22]
An interesting finding was that there were no statistically significant differences in readmissions among patients who report a language other than English as their primary language. Although language barriers and health literacy can affect a patient's ability to understand discharge instructions, the use of translators at UCSF Medical Center may have decreased the risk of readmissions. For a small number of patients whose primary language was not recorded (unknown language in our model), they appeared to have a lower risk of readmissions. Language preferences are recorded by our admitting staff during the process of admission. This step may have been skipped after hours or if the patient was not able to answer the question. However, we do not have a good hypothesis as to why these patients may have a reduced risk of readmissions.
Having a departed PCP in the General Internal Medicine clinic was an independent predictor of readmissions. These patients have access to primary care; they can schedule acute appointments with covering providers for new medical issues or follow‐up of chronic conditions. However, until they are transferred to a new PCP, they do not have a provider who is proactively managing their preventive and chronic disease care, including follow‐up and coordinating care after hospital discharge. Our study is not the first to suggest adverse outcomes for patients who are in transition from 1 primary care provider to the next.[23] Studies conducted in General Internal Medicine clinics have shown missed opportunities for cancer screening and overlooked test results during the transition period,[24] and as many as one‐fifth of patients whom residents identified as high‐risk were lost to follow‐up.[25] However, our study is the first to show the link between PCP transition in a teaching clinic and hospital readmissions. This finding underscores the importance of continuity of care in the optimal management of patients following hospital discharge.
There are several limitations to this study. First, our study only considers hospitalizations to UCSF Medical Center, potentially undercounting readmissions to area hospitals. Because our study population are patients with PCPs at UCSF, these patients tend to seek specialty and acute care at UCSF Medical Center as well. We obtained payor data from our medical group (Hill Physicians), which covers our largest private payors, to understand whether our results can be applied on a global basis. We found that in 87.4% of index admissions with readmissions from January 1, 2010 through May 30, 2012, the readmission occurred at UCSF Medical Center. We conducted a similar analysis with CMS data from October 1, 2008 to June 30, 2011, the latest data we have from CMS. For patients with UCSF PCPs and a diagnosis of AMI or CHF, 100% were readmitted back to UCSF Medical Center. For patients with UCSF PCPs and a diagnosis of pneumonia, 89% were readmitted back to UCSF Medical Center. Given that only a small percentage of patients with PCPs at UCSF present for readmission at other area hospitals, we believe that limiting our analysis to UCSF Medical Center is reasonable.
In our study, we did not remove vaginal deliveries or Caesarian sections prior to building the model. Primary care physicians and their clinic leadership are accustomed to taking a population‐health perspective. We anticipate they would be interested in designing interventions and addressing readmission for the entire primary care panel. Although readmissions after delivery are not frequent, they can still occur, and interventions should not necessarily exclude this population. We did run a sensitivity analysis by removing vaginal delivery and Caesarian sections from the analysis. As expected, readmission rates for all practices increased except for Geriatrics. However, the independent predictors of readmissions did not change.
Our study is based on a population of patients at 1 urban academic medical center and may not be generalizable across all delivery systems. Our population is racially and ethnically diverse, and many do not speak English as their primary language. The study also spans different types of primary care clinics, capturing a wide range of ages and case mix. As PCP assignments fluctuate over time, there may be errors with PCP attribution in the UCSF Medical Center data systems. We do not believe errors in PCP attribution would differ across primary care practices. Because the primary care practices' performance reports on quality measures are based on PCP assignment, each clinic regularly updates their clinic panels and has specific protocols to address errors with PCP attribution.
Finally, our study includes only the variables that we were able to extract from administrative claims. Other explanatory variables that have been suggested as important for evaluation, such as social support, functional assessment, access to care, hospital discharge process, and posthospitalization follow‐up, were not included. Each of these could be explored in future studies.
This study offers a unique perspective of hospital readmission by introducing a new methodology for primary care clinics to calculate and evaluate their all‐cause 30‐day readmission rates. Although this methodology is not intended to provide real‐time feedback to clinics on readmitted patients, it opens the door for benchmarking based on specific case‐mix indices. Another direction for future research is to design robust evaluations of the impact of interventions, both inpatient and outpatient, on primary care clinic readmission rates. Finally, future research should replicate this analysis across teaching clinics to identify whether provider turnover is a consistent independent predictor of hospital readmissions.
This study also has implications for inpatient interventions. For example, discharging physicians may consider proactively identifying whether the patient has a continuous primary care provider. Patients who are in between PCPs may need closer follow‐up after discharge, until they re‐establish with a new PCP. This can be accomplished through a postdischarge clinic visit, either run by inpatient providers or covering physicians in the primary care clinic. In addition, the discharging physician may work with the case manager to increase the level of care coordination. The case manager could contact the primary care clinic and proactively ask for immediate PCP re‐assignment. Once a new PCP has been identified, the discharging physician could consider a warm hand‐off. With a warm hand‐off, the new PCP may feel more comfortable managing problems that may arise after hospital discharge, and especially before the first outpatient visit with the new PCP. Future research can test whether these interventions could effectively reduce hospital readmissions across a broad primary care population.
CONCLUSION
Primary care providers and their clinics play an important role in managing population health, decreasing healthcare spending, and keeping patients out of the hospital. In this study, we introduce a tool in which primary care clinics can begin to understand their hospital readmission rates. This may be particularly valuable as primary care providers enter global payment arrangements such as accountable care organizations or bundled payments and are responsible for a population of patients across the continuum of care. We found significant variation in readmission rates between different primary care practices, but much of this variation appears to be due to differences between practices in patient demographics, comorbidities, and hospitalization factors. Our study is the first to show the association between provider transitions and higher hospital readmissions. Continuity of care is critical for the optimal management of patients following hospital discharge. More attention will need to be focused on providing good continuity outpatient care for patients at high risk for readmissions.
Acknowledgements
The authors acknowledge Janelle Lee, MBA, MHA, DrPH, for her assistance with extracting the hospital claims data at UCSF Medical Center.
Disclosure: Dr. Tang and Ms. Maselli were supported by a University of California, Center for Health Quality and Innovation grant. The authors report no conflicts of interest.
- Medicare's new hospital value‐based purchasing program is likely to have only a small impact on hospital payments. Health Aff. 2012;31:1932–1940. , .
- Contemporary evidence about hospital strategies for reducing 30‐day readmissions. J Am Coll Cardiol. 2012;60:607–614. , , , et al.
- Interventions to reduce 30‐day rehospitalization: a systematic review. Ann Intern Med. 2011;155:520–528. , , , , .
- Reducing hospital readmissions: lessons from top‐performing hospitals. The Commonwealth Fund Synthesis Report. Available at: http://www.commonwealthfund.org/publications/case‐studies/2011/apr/reducing‐hospital‐readmissions. Published April 6, 2011. Accessed February 8, 2013. , , .
- Effective Interventions to Reduce Rehospitalizations: A Survey of the Published Evidence. Cambridge, MA: Institute for Healthcare Improvement; 2009. , .
- Recasting readmissions by placing the hospital role in community context. JAMA. 2013;309:351–352. , , .
- Hospitalists and care transitions: the divorce of inpatient and outpatient care. Health Affairs. 2008;27:1315–1327. , , , .
- Center for Medicare and Medicaid Innovation. Community‐based Care Transitions Program. Available at: http://innovation.cms.gov/initiatives/CCTP/#collapse‐tableDetails. Accessed February 8, 2013.
- Medicare's transitional care payment—a step toward the medical home. N Engl J Med. 2013;368:692–694. , , .
- Volume thresholds and hospital characteristics in the United States. Health Aff (Millwood). 2003;22:167–177. , , .
- Thirty‐day rehospitalizations after acute myocardial infarction: a cohort study. Ann Intern Med. 2012;157:11–18. , , , , , .
- Rehospitalizations among patients in the Medicare fee‐for‐service program. N Engl J Med. 2009;360:1418–1428. , , .
- Associations between reduced hospital length of stay and 30‐day readmission rate and mortality: 14‐year experience in 129 Veterans Affairs hospitals. Ann Intern Med. 2012;157:837–845. , , , et al.
- Readmission after hospitalization for congestive heart failure among Medicare beneficiaries. Arch Intern Med. 1997;157:99–104. , , , et al.
- Redefining readmission risk factors for general medicine patients. J Hosp Med. 2011;6:54–60. , , , .
- Risk prediction models for hospital readmission: a systematic review. JAMA. 2011;206:1688–1698. , , , et al.
- Statistical models and patient predictors of readmission for heart failure: a systematic review. Arch Intern Med. 2008;168:1371–1386. , , , et al.
- Statistical models and patient predictors of readmission for acute myocardial infarction: a systematic review. Circ Cardiovasc Qual Outcomes. 2009;2:500–507. , , , .
- Predictors of hospital readmission after stroke: a systematic review. Stroke. 2010;41:2525–2533. , , , et al.
- Risk factors for 30‐day hospital readmission in patients ≥65 years of age. Proc (Bayl Univ Med Cent). 2008;21:363–372. , , , , .
- Hospital readmission in general medicine patients: a prediction model. J Gen Intern Med. 2010;25:211–219. , , , et al.
- Users of Medicaid home and community‐based services are especially vulnerable to costly avoidable hospital admissions. Health Affairs. 2012;31:1167–1175. , , .
- Academic year‐end transfers of outpatients from outgoing to incoming residents: an unaddressed patient safety issue. JAMA. 2009;302:1327–1329. , .
- The revolving door of resident continuity practice: identifying gaps in transitions of care. J Gen Intern Med. 2011;26:995–998. , , , , , .
- Outcomes for resident‐identified high‐risk patients and resident perspectives of year‐end continuity clinic handoffs. J Gen Intern Med. 2012;27:1438–1444. , , , et al.
Reducing hospital readmissions is a national healthcare priority. In October 2012, the Centers for Medicare and Medicaid Services (CMS) enacted financial penalties on hospitals with higher than average risk‐adjusted readmissions, offering an incentive pool of $850 million in the first year.[1] As a result, a wide range of activities to understand and reduce readmissions among patients with congestive heart failure (CHF), acute myocardial infarction (AMI), and pneumonia have emerged.[2, 3, 4, 5] Some have proposed that to effectively reduce hospital readmissions, a community of inpatient and outpatient providers and local support organizations must coordinate efforts.[6, 7] In fact, CMS has recognized the important role of community support groups and outpatient providers in safe discharge transitions in 2 ways. First, in 2011 CMS launched the Community‐based Care Transitions Program to fund community‐based organizations to assist Medicare patients with care transitions.[8] Second, CMS introduced 2 new reimbursement codes for primary care providers (PCPs) to perform care coordination immediately after hospital discharge.[9] Both of these new payment programs represent an evolving perspective that reducing hospital readmissions requires active participation among outpatient partners.
As leaders of the outpatient care team, PCPs play a significant role in reducing hospital readmissions. One way in which PCPs can begin to understand the magnitude of the issue within their clinic is to evaluate the clinic's 30‐day readmission rates. Currently, CMS calculates readmission rates at the hospital level. However, understanding these rates at the clinic level is critical for developing strategies for improvement across the care continuum. Our current understanding of effective outpatient interventions to reduce hospital readmission is limited.[3] As clinics introduce and refine strategies to reduce readmissions, tracking the impact of these strategies on readmission rates will be critical for identifying effective outpatient interventions. Clinics with similar patient case‐mix can also benchmark readmission rates, sharing best practices from clinics with lower‐than‐expected rates.
There are no available studies or proposed methodologies to guide primary care clinics in calculating their 30‐day readmission rates. A particularly difficult challenge is obtaining the admission information when patients may be admitted to 1 of several area hospitals. For large integrated delivery networks where primary care patients are relatively loyal to the network of physicians and hospitals, an opportunity exists to explore the data. Furthermore, variations in readmission rates across primary care specialties (such as internal medicine and family practice) are not well understood. In this study, we set out to develop a methodology for calculating all‐cause 30‐day hospital readmission rates at the level of individual primary care practices and to identify factors associated with variations in these rates.
METHODS
Study Design
We conducted a retrospective observational study of adult primary care patients at the University of California, San Francisco (UCSF) who were hospitalized at UCSF Medical Center between July 1, 2009 and June 30, 2012. UCSF Medical Center is comprised of Moffitt‐Long Hospital (a 600‐bed facility) and UCSF‐Mount Zion Hospital (a 90‐bed facility) located in San Francisco, CA. The patient population was limited to adults ages 18 and over with a PCP at UCSF. UCSF has 7 adult primary care clinics: General Internal Medicine (IM), Family Practice (FP), Women's Health, Geriatrics, a combined IM/FP clinic, Human Immunodeficiency Virus (HIV) Primary Care, and a Concierge Internal Medicine clinic staffed by IM physicians. Between 2009 and 2011, all clinics completed the process of enpanelment, or defining the population of patients for which each PCP and the clinic is responsible. We obtained the list of patient and PCP assignments at each of the 7 clinics across the time period of study. We then obtained UCSF Medical Center hospital claims data for this group, including dates of admission and discharge, patient age, sex, race/ethnicity, language, insurance, admitting service, diagnosis codes, information on intensive care unit stay, and discharge disposition. Hospital claims data are housed in Transition Systems International (Boston, MA) administrative databases, a cost‐accounting system that collects data abstracted from patient charts upon discharge from UCSF Medical Center.
All‐cause 30‐day hospital readmission rates were calculated for each primary care clinic, using an adaptation of the CMS definition. First, we defined index discharges as the first discharge for an individual patient in any given 30‐day interval. Only 1 index discharge is flagged for each 30‐day interval. The first admission within 30 days after the index discharge was flagged as the readmission. Consistent with CMS methodology, only the first readmission in the 30‐day period was counted. We included all inpatient and observation status admissions and excluded patients who died during the index encounter, left against medical advice, or transferred to another acute care hospital after the index encounter.
We used secondary diagnosis codes in the administrative data to classify comorbidities by the Elixhauser methodology.[10] All but 1 adult primary care clinic at UCSF are faculty‐only clinics, whereby the assigned PCP is an attending physician. In the general internal medicine clinic, an attending or a resident can serve as the PCP, and approximately 20% of IM clinic patients have a resident as their PCP. For the IM clinic, we classified patients' PCP as attending, resident, or departed. The latter category refers to patients whose PCPs were residents who had graduated or faculty who had departed and had not been assigned to a new PCP prior to the index admission or readmission.
Statistical Analysis
We built a model to predict readmissions using the demographic and clinical variables with [2] P<0.20 in an initial bivariate analysis, and then removed, with backward selection, the least significant variables until only those with P0.05 remained. Age, log‐length of stay (LOS), and intensive care unit stay were forced in the model, as studies evaluating factors related to readmissions have often included these as important covariates.[11, 12, 13, 14] Results were expressed as adjusted odds ratio (OR) with 95% confidence interval (CI). All analyses were carried out using SAS version 9.2 (SAS Institute, Inc., Cary, NC).
This study was exempt from review by the institutional review board of UCSF.
RESULTS
During the study period, there were 12,564 discharges from UCSF Medical Center for primary care patients belonging to the 7 clinics. Of these, 8685 were index discharges and 1032 were readmissions within 30 days. Table 1 shows the characteristics of the patients who had at least 1 admission during the study period. In all but 2 clinics (HIV Primary Care and Concierge Internal Medicine), there were more women hospitalized than men. Age and gender differences across clinics are consistent with the patient populations served by these clinics, with Women's Health having more female patients and younger patients hospitalized compared to Geriatrics.
General Internal Medicine | Family Practice | Women's Health | Geriatrics | Combined IM/FP | HIV Primary Care | Concierge IM | |
---|---|---|---|---|---|---|---|
| |||||||
Panel size | 26,521 | 10,495 | 8,526 | 190 | 4,109 | 1,039 | 706 |
FTE attending MD and NP providers | 18.8 | 7.3 | 4.5 | 1.7 | 5.0 | 2.3 | 2.1 |
Mean panel size per FTE | 1,311 | 1,448 | 1,895 | 112 | 822 | 452 | 336 |
Clinic visits in FY 2012 | 50,362 | 20,647 | 11,014 | 4,425 | 8,120 | 4,182 | 1,713 |
No. of index discharges | 5,388 | 1,204 | 983 | 409 | 339 | 249 | 113 |
No. of readmissions | 718 | 104 | 76 | 56 | 30 | 37 | 11 |
No. of patients discharged during study period | 4,063 | 1,003 | 818 | 289* | 290 | 185 | 84 |
% Male | 40.1% | 33.9% | 4.3% | 35.3% | 33.8% | 69.7% | 52.4% |
Average age (SD), y | 60.4 (18.6) | 52.0 (19.0) | 47.0 (15.7) | 83.2 (6.9) | 46.3 (16.9) | 49.5 (9.9) | 64.5 (15.6) |
Age range, y | 19104 | 1896 | 1997 | 6399 | 1890 | 2273 | 2092 |
Race | |||||||
% White | 42.2% | 41.3% | 58.8% | 61.9% | 49.3% | 61.0% | 91.7% |
% Black | 16.3% | 8.5% | 7.5% | 7.3% | 10.0% | 27.6% | 0.0% |
% Asian | 23.6% | 31.4% | 19.0% | 17.0% | 16.6% | 2.2% | 4.8% |
% Native America/ Alaskan Native | 0.7% | 1.6% | 0.7% | 0.7% | 0.7% | 0.5% | 0.0% |
% Other | 16.3% | 15.7% | 13.1% | 12.5% | 18.3% | 8.1% | 2.4% |
Not available | 1.0% | 1.6% | 1.0% | 0.7% | 5.2% | 0.5% | 1.2% |
Ethnicity | |||||||
% Hispanic | 8.8% | 10.6% | 5.0% | 6.2% | 8.3% | 4.9% | 0.0% |
% Non‐Hispanic | 73.8% | 74.5% | 81.8% | 73.0% | 74.5% | 84.9% | 75.0% |
Not available | 17.4% | 15.0% | 13.2% | 20.8% | 17.2% | 10.3% | 25.0% |
Language | |||||||
% English | 69.7% | 75.6% | 88.8% | 69.6% | 85.9% | 89.7% | 89.3% |
% Spanish | 3.8% | 1.9% | 0.6% | 4.2% | 1.0% | 0.5% | 0.0% |
% Chinese (Mandarin or Cantonese) | 8.0% | 6.7% | 1.2% | 4.8% | 2.1% | 0.0% | 0.0% |
% Russian | 1.7% | 0.6% | 0.1% | 0.4% | 1.0% | 0.0% | 0.0% |
% Vietnamese | 1.0% | 1.4% | 0.0% | 0.4% | 0.0% | 0.0% | 0.0% |
% Other | 11.7% | 9.3% | 5.6% | 18.0% | 6.9% | 8.1% | 4.8% |
Not available | 4.1% | 4.6% | 3.7% | 2.8% | 3.1% | 1.6% | 6.0% |
Insurance type | |||||||
% Private | 35.4% | 58.7% | 77.4% | 10.7% | 63.8% | 36.8% | 66.7% |
% Medicare | 22.0% | 17.2% | 12.0% | 67.8% | 14.8% | 8.7% | 33.3% |
% Medicaid | 15.5% | 12.5% | 3.1% | 1.4% | 12.8% | 24.3% | 0.0% |
% Dual eligible | 24.4% | 8.8% | 5.4% | 19.4% | 6.6% | 27.6% | 0.0% |
% Self‐pay/other | 2.6% | 2.9% | 2.2% | 0.7% | 2.1% | 2.7% | 0.0% |
All‐cause 30‐day readmission rates varied across practices, with HIV Primary Care being the highest at 14.9%, followed by Geriatrics at 13.7%, General Internal Medicine at 13.3%, Concierge Internal Medicine at 9.7%, combined IM/FP at 8.9%, Family Practice at 8.6%, and Women's Health at 7.7% (Figure 1). Despite HIV Primary Care having the highest readmission rate, the number of index discharges during the 3‐year period was relatively low (249) compared to General Internal Medicine (5388). For the index admission, the top 5 admitting services were medicine, obstetrics, cardiology, orthopedic surgery, and general surgery (Table 2). Medicine was the primary admitting service for patients in the following clinics: General Internal Medicine, Geriatrics, HIV Primary Care, and Concierge Internal Medicine. Obstetrics was the primary admitting service for patients in the Family Practice, Women's Health, and combined IM/FP clinics. Vaginal delivery was the top discharge diagnosis‐related group (DRG) for patients in the General Internal Medicine, Family Practice, Women's Health, and combined IM/FP clinics. The top discharge DRG was urinary tract infection for Geriatrics, HIV for the HIV clinic (13.6%), and chemotherapy (8.0%) for Concierge Internal Medicine. Average LOS varied from 4.7 days for patients in the HIV Primary Care clinic to 2.8 days for patients in the Concierge Internal Medicine clinic. Average LOS was 3.4, 3.1, and 3.2 days in the Family Practice, Women's Health, and Geriatrics clinics, respectively, and 3.8 days in the General Internal Medicine and combined IM/FP clinics. For all clinics except Geriatrics, the majority of patients were discharged home without home health. A larger proportion of patients in the Geriatrics clinic were discharged home with home health or discharged to a skilled nursing facility.
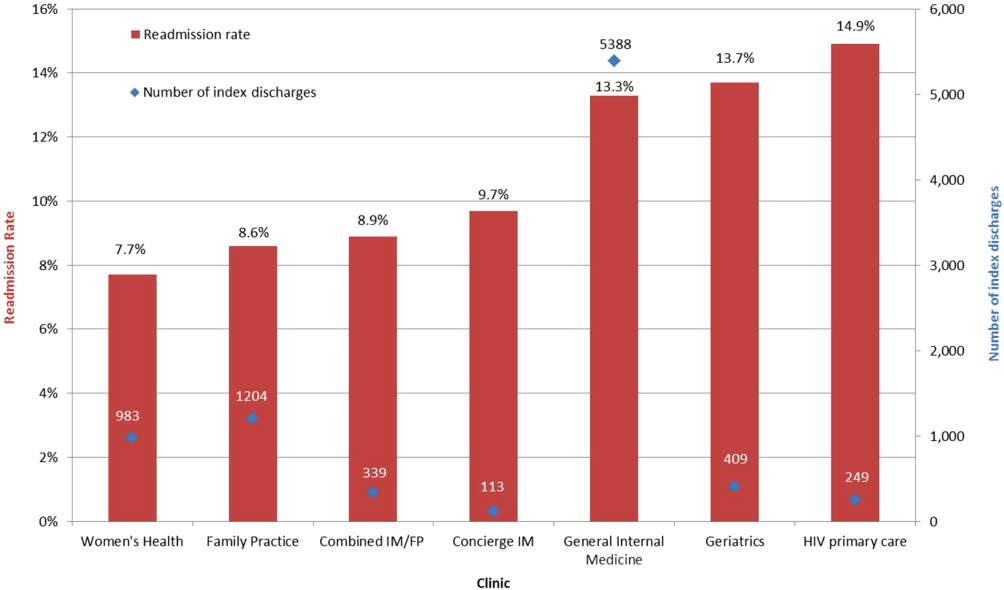
General Internal Medicine, N=5,388 | Family Practice, N=1,204 | Women's Health, N=983 | Geriatrics, N=409 | Combined IM/FP, N=339 | HIV Primary Care, N=249 | Concierge IM, N=113 | |
---|---|---|---|---|---|---|---|
| |||||||
Top 5 admitting services | |||||||
Medicine | 41.8% | 25.0% | 15.2% | 56.4% | 23.5% | 59.7% | 19.0% |
Obstetrics | 7.5% | 24.7% | 38.7% | 0.0% | 35.6% | 2.1% | 9.0% |
Cardiology | 15.1% | 10.9% | 6.2% | 16.5% | 10.3% | 6.5% | 17.0% |
Orthopedic surgery | 8.3% | 8.3% | 8.2% | 7.3% | 5.3% | 4.8% | 12.0% |
Adult general surgery | 7.2% | 9.8% | 10.0% | 6.0% | 5.0% | 5.8% | 7.0% |
Top 5 discharge diagnoses | |||||||
Vaginal delivery | 3.5% | 13.6% | 20.6% | 0.0% | 17.9% | 0.3% | 3.0% |
Major joint replacement | 3.5% | 3.4% | 4.8% | 4.3% | 3.8% | 0.7% | 5.0% |
Vaginal delivery with complications | 1.3% | 5.0% | 6.9% | 0.0% | 4.1% | 1.0% | 2.0% |
Simple pneumonia and pleurisy | 2.8% | 1.2% | 0.6% | 3.8% | 0.3% | 2.1% | 0.0% |
Urinary tract infection | 2.0% | 1.5% | 1.4% | 6.2% | 0.9% | 2.4% | 1.0% |
Discharge disposition | |||||||
% Home | 69.1% | 74.6% | 76.6% | 47.8% | 69.9% | 78.6% | 68.4% |
% Home with home health | 19.4% | 16.5% | 17.8% | 24.1% | 21.7% | 11.8% | 18.8% |
% Skilled nursing facility | 7.2% | 4.9% | 3.0% | 18.9% | 3.3% | 5.0% | 0.8% |
% Other | 4.3% | 4.0% | 2.6% | 9.2% | 5.1% | 4.6% | 12.0% |
Average length of stay (SD) | 3.8 (5.6) | 3.4 (7.5) | 3.1 (3.7) | 3.2 (3.7) | 3.8 (5.2) | 4.7 (6.5) | 2.8 (2.8) |
% Discharges with ICU stay | 11.0% | 10.6% | 5.9% | 8.5% | 9.2% | 11.1% | 15.8% |
Factors associated with variation in readmission rates included: male gender (OR: 1.21, 95% CI: 1.051.40), Medicare (OR: 1.31, 95% CI: 1.051.64; Ref=private) and dual‐eligible Medicare‐Medicaid insurance (OR: 1.26, 95% CI: 1.011.56), unknown primary language (OR: 0.06, 95% CI: 0.020.25; Ref=English), and the following comorbidities: peripheral vascular disease, renal failure, lymphoma, fluid and electrolyte disorders, and anemia. Multivariable logistic regression modeling results are listed in Table 3. Patients having a resident PCP showed no increased odds of readmission (OR: 1.13, 95% CI: 0.931.37; Ref=attending PCP). However, patients with a graduated resident PCP or departed faculty PCP awaiting transfer to a new PCP had an OR of 1.59 (95% CI: 1.162.17) compared with having a current faculty PCP. The C‐statistic for this model was 0.67.
Adjusted Odds Ratio (95% CI) | |
---|---|
| |
Age | |
<65 years | Ref |
>65 years | 0.91 (0.751.10) |
Clinic | |
General Internal Medicine | 1.24 (0.991.56) |
Family Practice | Ref |
Women's Health | 1.13 (0.821.56) |
Geriatrics | 1.20 (0.841.74) |
Combined Internal Medicine/Family Practice | 1.13 (0.731.74) |
HIV Primary Care | 1.24 (0.811.89) |
Concierge Internal Medicine | 1.06 (0.542.07) |
Sex | |
Female | Ref |
Male | 1.21 (1.051.40) |
Insurance | |
Private | Ref |
Medicaid | 1.09 (0.871.37) |
Medicare | 1.31 (1.051.64) |
Dual eligible MedicareMedicaid | 1.26 (1.011.56) |
Self/other | 1.20 (0.741.94) |
Language | |
English | Ref |
Spanish | 1.02 (0.701.47) |
Chinese | 1.13 (0.871.47) |
Other | 0.87 (0.701.08) |
Unknown | 0.06 (0.020.25) |
Comorbidities | |
Pulmonary circulation disease | 1.51 (0.982.32) |
Peripheral vascular disease | 1.50 (1.141.98) |
Renal failure | 1.31 (1.091.58) |
Lymphoma | 2.50 (1.434.36) |
Fluid and electrolyte disorders | 1.27 (1.071.52) |
Deficiency anemia | 1.25 (1.041.51) |
Physician | |
Attending | Ref |
Resident | 1.13 (0.931.37) |
Departed PCP (internal medicine only) | 1.59 (1.162.17) |
ICU stay | 1.03 (0.831.28) |
LOS (log) | 1.02 (0.981.06) |
Admitting service | |
Medicine | Ref |
Obstetrics | 0.41 (0.300.56) |
Cardiology | 1.12 (0.911.37) |
Orthopedic surgery | 0.36 (0.250.51) |
Adult general surgery | 0.64 (0.470.87) |
Other services | 0.76 (0.630.92) |
DISCUSSION
In this study we introduce a complementary way to view hospital readmissions, from the perspective of primary care practices. We found variation in readmission rates across primary care practices. After controlling for admitting service, clinic, provider, and patient factors, the specific characteristics of male gender, patients with Medicare or Medicare with Medicaid, and patients in our General Internal Medicine clinic with a departed PCP were independent risk factors for hospital readmission. Patients with specific comorbidities were also at increased risk for admissions, including those with peripheral vascular disease, renal failure, lymphoma, fluid and electrolyte disorders, and anemia. Because this is the first study viewing readmissions from the perspective of primary care practices, our findings are unique in the literature. However, hospital‐based studies have found similar relationships between readmission rates and these specific comorbidities.[11, 12, 15] Unlike other studies, our study cohort did not show CHF as an independent risk factor. We hypothesize this is because in 2008, UCSF Medical Center introduced an inpatient‐based intervention to reduce readmissions in patients with heart failure. By 2011, the readmission rates of patients with heart failure (primary or secondary diagnoses) had dropped by 30%. The success of this program, focused only on patients with heart failure, likely affected our analysis of comorbidities as risk factors for readmissions.
Models developed to predict hospital readmissions, overall and for specific disease conditions, have inconsistently identified predictive factors, and there is not a specific set of variables that dominate.[16, 17, 18, 19] A recent review of readmission risk prediction models suggested that models that take into account psychosocial factors such as social support, substance abuse, and functional status improved model performance.[16] We hypothesize that the reason why male gender was significant in our model may be related to lack of social support, especially among those who may be single or widowed. Other studies have also showed male gender as a predictor for hospital readmissions.[14, 20]
People with Medicare as the primary payor or Medicare with Medicaid also tended to have higher risk of readmission. We believe that this may be a proxy for the combined effect of age, multiple comorbidities, and psychosocial factors. In a multicenter study of general medicine inpatients, Medicare, but not age, was also found to be a predictive variable.[21] Unlike other studies, our study did not find Medicaid alone as a significant predictive variable for readmissions.[15, 21] One explanation may be that in hospital‐based studies, people with Medicaid who are at high risk for readmission may be high‐risk because they do not have a PCP or good access to outpatient care. In our study, all patients have a PCP in 1 of the UCSF clinics, and access to care is improved with this established relationship. These other studies did not examine Medicare‐Medicaid dual eligibility status. Our results are consistent with a national study on avoidable hospital admissions that showed the dual eligibility population experiencing 60% higher avoidable admission rates compared to the Medicaid‐only population.[22]
An interesting finding was that there were no statistically significant differences in readmissions among patients who report a language other than English as their primary language. Although language barriers and health literacy can affect a patient's ability to understand discharge instructions, the use of translators at UCSF Medical Center may have decreased the risk of readmissions. For a small number of patients whose primary language was not recorded (unknown language in our model), they appeared to have a lower risk of readmissions. Language preferences are recorded by our admitting staff during the process of admission. This step may have been skipped after hours or if the patient was not able to answer the question. However, we do not have a good hypothesis as to why these patients may have a reduced risk of readmissions.
Having a departed PCP in the General Internal Medicine clinic was an independent predictor of readmissions. These patients have access to primary care; they can schedule acute appointments with covering providers for new medical issues or follow‐up of chronic conditions. However, until they are transferred to a new PCP, they do not have a provider who is proactively managing their preventive and chronic disease care, including follow‐up and coordinating care after hospital discharge. Our study is not the first to suggest adverse outcomes for patients who are in transition from 1 primary care provider to the next.[23] Studies conducted in General Internal Medicine clinics have shown missed opportunities for cancer screening and overlooked test results during the transition period,[24] and as many as one‐fifth of patients whom residents identified as high‐risk were lost to follow‐up.[25] However, our study is the first to show the link between PCP transition in a teaching clinic and hospital readmissions. This finding underscores the importance of continuity of care in the optimal management of patients following hospital discharge.
There are several limitations to this study. First, our study only considers hospitalizations to UCSF Medical Center, potentially undercounting readmissions to area hospitals. Because our study population are patients with PCPs at UCSF, these patients tend to seek specialty and acute care at UCSF Medical Center as well. We obtained payor data from our medical group (Hill Physicians), which covers our largest private payors, to understand whether our results can be applied on a global basis. We found that in 87.4% of index admissions with readmissions from January 1, 2010 through May 30, 2012, the readmission occurred at UCSF Medical Center. We conducted a similar analysis with CMS data from October 1, 2008 to June 30, 2011, the latest data we have from CMS. For patients with UCSF PCPs and a diagnosis of AMI or CHF, 100% were readmitted back to UCSF Medical Center. For patients with UCSF PCPs and a diagnosis of pneumonia, 89% were readmitted back to UCSF Medical Center. Given that only a small percentage of patients with PCPs at UCSF present for readmission at other area hospitals, we believe that limiting our analysis to UCSF Medical Center is reasonable.
In our study, we did not remove vaginal deliveries or Caesarian sections prior to building the model. Primary care physicians and their clinic leadership are accustomed to taking a population‐health perspective. We anticipate they would be interested in designing interventions and addressing readmission for the entire primary care panel. Although readmissions after delivery are not frequent, they can still occur, and interventions should not necessarily exclude this population. We did run a sensitivity analysis by removing vaginal delivery and Caesarian sections from the analysis. As expected, readmission rates for all practices increased except for Geriatrics. However, the independent predictors of readmissions did not change.
Our study is based on a population of patients at 1 urban academic medical center and may not be generalizable across all delivery systems. Our population is racially and ethnically diverse, and many do not speak English as their primary language. The study also spans different types of primary care clinics, capturing a wide range of ages and case mix. As PCP assignments fluctuate over time, there may be errors with PCP attribution in the UCSF Medical Center data systems. We do not believe errors in PCP attribution would differ across primary care practices. Because the primary care practices' performance reports on quality measures are based on PCP assignment, each clinic regularly updates their clinic panels and has specific protocols to address errors with PCP attribution.
Finally, our study includes only the variables that we were able to extract from administrative claims. Other explanatory variables that have been suggested as important for evaluation, such as social support, functional assessment, access to care, hospital discharge process, and posthospitalization follow‐up, were not included. Each of these could be explored in future studies.
This study offers a unique perspective of hospital readmission by introducing a new methodology for primary care clinics to calculate and evaluate their all‐cause 30‐day readmission rates. Although this methodology is not intended to provide real‐time feedback to clinics on readmitted patients, it opens the door for benchmarking based on specific case‐mix indices. Another direction for future research is to design robust evaluations of the impact of interventions, both inpatient and outpatient, on primary care clinic readmission rates. Finally, future research should replicate this analysis across teaching clinics to identify whether provider turnover is a consistent independent predictor of hospital readmissions.
This study also has implications for inpatient interventions. For example, discharging physicians may consider proactively identifying whether the patient has a continuous primary care provider. Patients who are in between PCPs may need closer follow‐up after discharge, until they re‐establish with a new PCP. This can be accomplished through a postdischarge clinic visit, either run by inpatient providers or covering physicians in the primary care clinic. In addition, the discharging physician may work with the case manager to increase the level of care coordination. The case manager could contact the primary care clinic and proactively ask for immediate PCP re‐assignment. Once a new PCP has been identified, the discharging physician could consider a warm hand‐off. With a warm hand‐off, the new PCP may feel more comfortable managing problems that may arise after hospital discharge, and especially before the first outpatient visit with the new PCP. Future research can test whether these interventions could effectively reduce hospital readmissions across a broad primary care population.
CONCLUSION
Primary care providers and their clinics play an important role in managing population health, decreasing healthcare spending, and keeping patients out of the hospital. In this study, we introduce a tool in which primary care clinics can begin to understand their hospital readmission rates. This may be particularly valuable as primary care providers enter global payment arrangements such as accountable care organizations or bundled payments and are responsible for a population of patients across the continuum of care. We found significant variation in readmission rates between different primary care practices, but much of this variation appears to be due to differences between practices in patient demographics, comorbidities, and hospitalization factors. Our study is the first to show the association between provider transitions and higher hospital readmissions. Continuity of care is critical for the optimal management of patients following hospital discharge. More attention will need to be focused on providing good continuity outpatient care for patients at high risk for readmissions.
Acknowledgements
The authors acknowledge Janelle Lee, MBA, MHA, DrPH, for her assistance with extracting the hospital claims data at UCSF Medical Center.
Disclosure: Dr. Tang and Ms. Maselli were supported by a University of California, Center for Health Quality and Innovation grant. The authors report no conflicts of interest.
Reducing hospital readmissions is a national healthcare priority. In October 2012, the Centers for Medicare and Medicaid Services (CMS) enacted financial penalties on hospitals with higher than average risk‐adjusted readmissions, offering an incentive pool of $850 million in the first year.[1] As a result, a wide range of activities to understand and reduce readmissions among patients with congestive heart failure (CHF), acute myocardial infarction (AMI), and pneumonia have emerged.[2, 3, 4, 5] Some have proposed that to effectively reduce hospital readmissions, a community of inpatient and outpatient providers and local support organizations must coordinate efforts.[6, 7] In fact, CMS has recognized the important role of community support groups and outpatient providers in safe discharge transitions in 2 ways. First, in 2011 CMS launched the Community‐based Care Transitions Program to fund community‐based organizations to assist Medicare patients with care transitions.[8] Second, CMS introduced 2 new reimbursement codes for primary care providers (PCPs) to perform care coordination immediately after hospital discharge.[9] Both of these new payment programs represent an evolving perspective that reducing hospital readmissions requires active participation among outpatient partners.
As leaders of the outpatient care team, PCPs play a significant role in reducing hospital readmissions. One way in which PCPs can begin to understand the magnitude of the issue within their clinic is to evaluate the clinic's 30‐day readmission rates. Currently, CMS calculates readmission rates at the hospital level. However, understanding these rates at the clinic level is critical for developing strategies for improvement across the care continuum. Our current understanding of effective outpatient interventions to reduce hospital readmission is limited.[3] As clinics introduce and refine strategies to reduce readmissions, tracking the impact of these strategies on readmission rates will be critical for identifying effective outpatient interventions. Clinics with similar patient case‐mix can also benchmark readmission rates, sharing best practices from clinics with lower‐than‐expected rates.
There are no available studies or proposed methodologies to guide primary care clinics in calculating their 30‐day readmission rates. A particularly difficult challenge is obtaining the admission information when patients may be admitted to 1 of several area hospitals. For large integrated delivery networks where primary care patients are relatively loyal to the network of physicians and hospitals, an opportunity exists to explore the data. Furthermore, variations in readmission rates across primary care specialties (such as internal medicine and family practice) are not well understood. In this study, we set out to develop a methodology for calculating all‐cause 30‐day hospital readmission rates at the level of individual primary care practices and to identify factors associated with variations in these rates.
METHODS
Study Design
We conducted a retrospective observational study of adult primary care patients at the University of California, San Francisco (UCSF) who were hospitalized at UCSF Medical Center between July 1, 2009 and June 30, 2012. UCSF Medical Center is comprised of Moffitt‐Long Hospital (a 600‐bed facility) and UCSF‐Mount Zion Hospital (a 90‐bed facility) located in San Francisco, CA. The patient population was limited to adults ages 18 and over with a PCP at UCSF. UCSF has 7 adult primary care clinics: General Internal Medicine (IM), Family Practice (FP), Women's Health, Geriatrics, a combined IM/FP clinic, Human Immunodeficiency Virus (HIV) Primary Care, and a Concierge Internal Medicine clinic staffed by IM physicians. Between 2009 and 2011, all clinics completed the process of enpanelment, or defining the population of patients for which each PCP and the clinic is responsible. We obtained the list of patient and PCP assignments at each of the 7 clinics across the time period of study. We then obtained UCSF Medical Center hospital claims data for this group, including dates of admission and discharge, patient age, sex, race/ethnicity, language, insurance, admitting service, diagnosis codes, information on intensive care unit stay, and discharge disposition. Hospital claims data are housed in Transition Systems International (Boston, MA) administrative databases, a cost‐accounting system that collects data abstracted from patient charts upon discharge from UCSF Medical Center.
All‐cause 30‐day hospital readmission rates were calculated for each primary care clinic, using an adaptation of the CMS definition. First, we defined index discharges as the first discharge for an individual patient in any given 30‐day interval. Only 1 index discharge is flagged for each 30‐day interval. The first admission within 30 days after the index discharge was flagged as the readmission. Consistent with CMS methodology, only the first readmission in the 30‐day period was counted. We included all inpatient and observation status admissions and excluded patients who died during the index encounter, left against medical advice, or transferred to another acute care hospital after the index encounter.
We used secondary diagnosis codes in the administrative data to classify comorbidities by the Elixhauser methodology.[10] All but 1 adult primary care clinic at UCSF are faculty‐only clinics, whereby the assigned PCP is an attending physician. In the general internal medicine clinic, an attending or a resident can serve as the PCP, and approximately 20% of IM clinic patients have a resident as their PCP. For the IM clinic, we classified patients' PCP as attending, resident, or departed. The latter category refers to patients whose PCPs were residents who had graduated or faculty who had departed and had not been assigned to a new PCP prior to the index admission or readmission.
Statistical Analysis
We built a model to predict readmissions using the demographic and clinical variables with [2] P<0.20 in an initial bivariate analysis, and then removed, with backward selection, the least significant variables until only those with P0.05 remained. Age, log‐length of stay (LOS), and intensive care unit stay were forced in the model, as studies evaluating factors related to readmissions have often included these as important covariates.[11, 12, 13, 14] Results were expressed as adjusted odds ratio (OR) with 95% confidence interval (CI). All analyses were carried out using SAS version 9.2 (SAS Institute, Inc., Cary, NC).
This study was exempt from review by the institutional review board of UCSF.
RESULTS
During the study period, there were 12,564 discharges from UCSF Medical Center for primary care patients belonging to the 7 clinics. Of these, 8685 were index discharges and 1032 were readmissions within 30 days. Table 1 shows the characteristics of the patients who had at least 1 admission during the study period. In all but 2 clinics (HIV Primary Care and Concierge Internal Medicine), there were more women hospitalized than men. Age and gender differences across clinics are consistent with the patient populations served by these clinics, with Women's Health having more female patients and younger patients hospitalized compared to Geriatrics.
General Internal Medicine | Family Practice | Women's Health | Geriatrics | Combined IM/FP | HIV Primary Care | Concierge IM | |
---|---|---|---|---|---|---|---|
| |||||||
Panel size | 26,521 | 10,495 | 8,526 | 190 | 4,109 | 1,039 | 706 |
FTE attending MD and NP providers | 18.8 | 7.3 | 4.5 | 1.7 | 5.0 | 2.3 | 2.1 |
Mean panel size per FTE | 1,311 | 1,448 | 1,895 | 112 | 822 | 452 | 336 |
Clinic visits in FY 2012 | 50,362 | 20,647 | 11,014 | 4,425 | 8,120 | 4,182 | 1,713 |
No. of index discharges | 5,388 | 1,204 | 983 | 409 | 339 | 249 | 113 |
No. of readmissions | 718 | 104 | 76 | 56 | 30 | 37 | 11 |
No. of patients discharged during study period | 4,063 | 1,003 | 818 | 289* | 290 | 185 | 84 |
% Male | 40.1% | 33.9% | 4.3% | 35.3% | 33.8% | 69.7% | 52.4% |
Average age (SD), y | 60.4 (18.6) | 52.0 (19.0) | 47.0 (15.7) | 83.2 (6.9) | 46.3 (16.9) | 49.5 (9.9) | 64.5 (15.6) |
Age range, y | 19104 | 1896 | 1997 | 6399 | 1890 | 2273 | 2092 |
Race | |||||||
% White | 42.2% | 41.3% | 58.8% | 61.9% | 49.3% | 61.0% | 91.7% |
% Black | 16.3% | 8.5% | 7.5% | 7.3% | 10.0% | 27.6% | 0.0% |
% Asian | 23.6% | 31.4% | 19.0% | 17.0% | 16.6% | 2.2% | 4.8% |
% Native America/ Alaskan Native | 0.7% | 1.6% | 0.7% | 0.7% | 0.7% | 0.5% | 0.0% |
% Other | 16.3% | 15.7% | 13.1% | 12.5% | 18.3% | 8.1% | 2.4% |
Not available | 1.0% | 1.6% | 1.0% | 0.7% | 5.2% | 0.5% | 1.2% |
Ethnicity | |||||||
% Hispanic | 8.8% | 10.6% | 5.0% | 6.2% | 8.3% | 4.9% | 0.0% |
% Non‐Hispanic | 73.8% | 74.5% | 81.8% | 73.0% | 74.5% | 84.9% | 75.0% |
Not available | 17.4% | 15.0% | 13.2% | 20.8% | 17.2% | 10.3% | 25.0% |
Language | |||||||
% English | 69.7% | 75.6% | 88.8% | 69.6% | 85.9% | 89.7% | 89.3% |
% Spanish | 3.8% | 1.9% | 0.6% | 4.2% | 1.0% | 0.5% | 0.0% |
% Chinese (Mandarin or Cantonese) | 8.0% | 6.7% | 1.2% | 4.8% | 2.1% | 0.0% | 0.0% |
% Russian | 1.7% | 0.6% | 0.1% | 0.4% | 1.0% | 0.0% | 0.0% |
% Vietnamese | 1.0% | 1.4% | 0.0% | 0.4% | 0.0% | 0.0% | 0.0% |
% Other | 11.7% | 9.3% | 5.6% | 18.0% | 6.9% | 8.1% | 4.8% |
Not available | 4.1% | 4.6% | 3.7% | 2.8% | 3.1% | 1.6% | 6.0% |
Insurance type | |||||||
% Private | 35.4% | 58.7% | 77.4% | 10.7% | 63.8% | 36.8% | 66.7% |
% Medicare | 22.0% | 17.2% | 12.0% | 67.8% | 14.8% | 8.7% | 33.3% |
% Medicaid | 15.5% | 12.5% | 3.1% | 1.4% | 12.8% | 24.3% | 0.0% |
% Dual eligible | 24.4% | 8.8% | 5.4% | 19.4% | 6.6% | 27.6% | 0.0% |
% Self‐pay/other | 2.6% | 2.9% | 2.2% | 0.7% | 2.1% | 2.7% | 0.0% |
All‐cause 30‐day readmission rates varied across practices, with HIV Primary Care being the highest at 14.9%, followed by Geriatrics at 13.7%, General Internal Medicine at 13.3%, Concierge Internal Medicine at 9.7%, combined IM/FP at 8.9%, Family Practice at 8.6%, and Women's Health at 7.7% (Figure 1). Despite HIV Primary Care having the highest readmission rate, the number of index discharges during the 3‐year period was relatively low (249) compared to General Internal Medicine (5388). For the index admission, the top 5 admitting services were medicine, obstetrics, cardiology, orthopedic surgery, and general surgery (Table 2). Medicine was the primary admitting service for patients in the following clinics: General Internal Medicine, Geriatrics, HIV Primary Care, and Concierge Internal Medicine. Obstetrics was the primary admitting service for patients in the Family Practice, Women's Health, and combined IM/FP clinics. Vaginal delivery was the top discharge diagnosis‐related group (DRG) for patients in the General Internal Medicine, Family Practice, Women's Health, and combined IM/FP clinics. The top discharge DRG was urinary tract infection for Geriatrics, HIV for the HIV clinic (13.6%), and chemotherapy (8.0%) for Concierge Internal Medicine. Average LOS varied from 4.7 days for patients in the HIV Primary Care clinic to 2.8 days for patients in the Concierge Internal Medicine clinic. Average LOS was 3.4, 3.1, and 3.2 days in the Family Practice, Women's Health, and Geriatrics clinics, respectively, and 3.8 days in the General Internal Medicine and combined IM/FP clinics. For all clinics except Geriatrics, the majority of patients were discharged home without home health. A larger proportion of patients in the Geriatrics clinic were discharged home with home health or discharged to a skilled nursing facility.
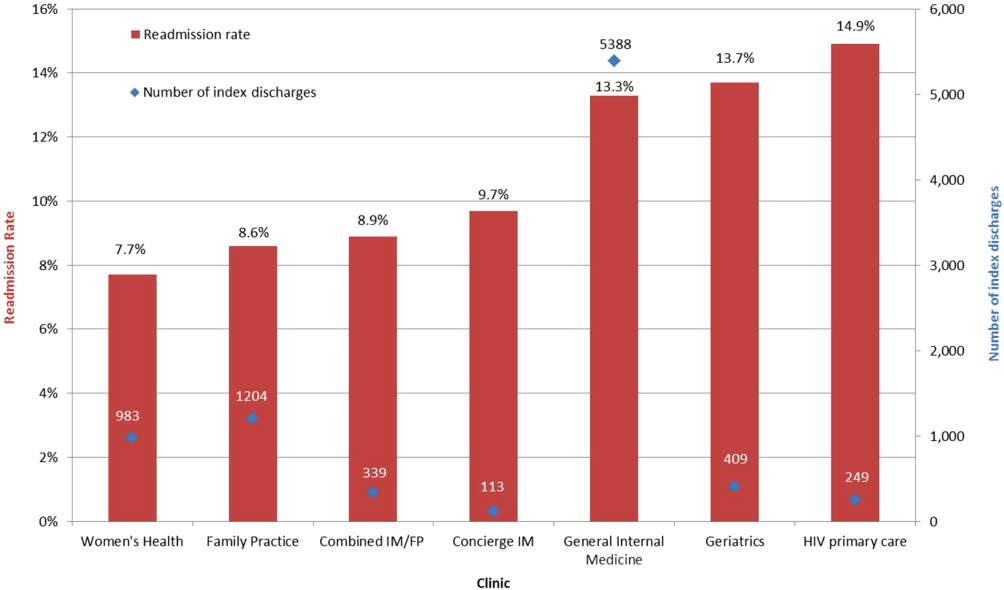
General Internal Medicine, N=5,388 | Family Practice, N=1,204 | Women's Health, N=983 | Geriatrics, N=409 | Combined IM/FP, N=339 | HIV Primary Care, N=249 | Concierge IM, N=113 | |
---|---|---|---|---|---|---|---|
| |||||||
Top 5 admitting services | |||||||
Medicine | 41.8% | 25.0% | 15.2% | 56.4% | 23.5% | 59.7% | 19.0% |
Obstetrics | 7.5% | 24.7% | 38.7% | 0.0% | 35.6% | 2.1% | 9.0% |
Cardiology | 15.1% | 10.9% | 6.2% | 16.5% | 10.3% | 6.5% | 17.0% |
Orthopedic surgery | 8.3% | 8.3% | 8.2% | 7.3% | 5.3% | 4.8% | 12.0% |
Adult general surgery | 7.2% | 9.8% | 10.0% | 6.0% | 5.0% | 5.8% | 7.0% |
Top 5 discharge diagnoses | |||||||
Vaginal delivery | 3.5% | 13.6% | 20.6% | 0.0% | 17.9% | 0.3% | 3.0% |
Major joint replacement | 3.5% | 3.4% | 4.8% | 4.3% | 3.8% | 0.7% | 5.0% |
Vaginal delivery with complications | 1.3% | 5.0% | 6.9% | 0.0% | 4.1% | 1.0% | 2.0% |
Simple pneumonia and pleurisy | 2.8% | 1.2% | 0.6% | 3.8% | 0.3% | 2.1% | 0.0% |
Urinary tract infection | 2.0% | 1.5% | 1.4% | 6.2% | 0.9% | 2.4% | 1.0% |
Discharge disposition | |||||||
% Home | 69.1% | 74.6% | 76.6% | 47.8% | 69.9% | 78.6% | 68.4% |
% Home with home health | 19.4% | 16.5% | 17.8% | 24.1% | 21.7% | 11.8% | 18.8% |
% Skilled nursing facility | 7.2% | 4.9% | 3.0% | 18.9% | 3.3% | 5.0% | 0.8% |
% Other | 4.3% | 4.0% | 2.6% | 9.2% | 5.1% | 4.6% | 12.0% |
Average length of stay (SD) | 3.8 (5.6) | 3.4 (7.5) | 3.1 (3.7) | 3.2 (3.7) | 3.8 (5.2) | 4.7 (6.5) | 2.8 (2.8) |
% Discharges with ICU stay | 11.0% | 10.6% | 5.9% | 8.5% | 9.2% | 11.1% | 15.8% |
Factors associated with variation in readmission rates included: male gender (OR: 1.21, 95% CI: 1.051.40), Medicare (OR: 1.31, 95% CI: 1.051.64; Ref=private) and dual‐eligible Medicare‐Medicaid insurance (OR: 1.26, 95% CI: 1.011.56), unknown primary language (OR: 0.06, 95% CI: 0.020.25; Ref=English), and the following comorbidities: peripheral vascular disease, renal failure, lymphoma, fluid and electrolyte disorders, and anemia. Multivariable logistic regression modeling results are listed in Table 3. Patients having a resident PCP showed no increased odds of readmission (OR: 1.13, 95% CI: 0.931.37; Ref=attending PCP). However, patients with a graduated resident PCP or departed faculty PCP awaiting transfer to a new PCP had an OR of 1.59 (95% CI: 1.162.17) compared with having a current faculty PCP. The C‐statistic for this model was 0.67.
Adjusted Odds Ratio (95% CI) | |
---|---|
| |
Age | |
<65 years | Ref |
>65 years | 0.91 (0.751.10) |
Clinic | |
General Internal Medicine | 1.24 (0.991.56) |
Family Practice | Ref |
Women's Health | 1.13 (0.821.56) |
Geriatrics | 1.20 (0.841.74) |
Combined Internal Medicine/Family Practice | 1.13 (0.731.74) |
HIV Primary Care | 1.24 (0.811.89) |
Concierge Internal Medicine | 1.06 (0.542.07) |
Sex | |
Female | Ref |
Male | 1.21 (1.051.40) |
Insurance | |
Private | Ref |
Medicaid | 1.09 (0.871.37) |
Medicare | 1.31 (1.051.64) |
Dual eligible MedicareMedicaid | 1.26 (1.011.56) |
Self/other | 1.20 (0.741.94) |
Language | |
English | Ref |
Spanish | 1.02 (0.701.47) |
Chinese | 1.13 (0.871.47) |
Other | 0.87 (0.701.08) |
Unknown | 0.06 (0.020.25) |
Comorbidities | |
Pulmonary circulation disease | 1.51 (0.982.32) |
Peripheral vascular disease | 1.50 (1.141.98) |
Renal failure | 1.31 (1.091.58) |
Lymphoma | 2.50 (1.434.36) |
Fluid and electrolyte disorders | 1.27 (1.071.52) |
Deficiency anemia | 1.25 (1.041.51) |
Physician | |
Attending | Ref |
Resident | 1.13 (0.931.37) |
Departed PCP (internal medicine only) | 1.59 (1.162.17) |
ICU stay | 1.03 (0.831.28) |
LOS (log) | 1.02 (0.981.06) |
Admitting service | |
Medicine | Ref |
Obstetrics | 0.41 (0.300.56) |
Cardiology | 1.12 (0.911.37) |
Orthopedic surgery | 0.36 (0.250.51) |
Adult general surgery | 0.64 (0.470.87) |
Other services | 0.76 (0.630.92) |
DISCUSSION
In this study we introduce a complementary way to view hospital readmissions, from the perspective of primary care practices. We found variation in readmission rates across primary care practices. After controlling for admitting service, clinic, provider, and patient factors, the specific characteristics of male gender, patients with Medicare or Medicare with Medicaid, and patients in our General Internal Medicine clinic with a departed PCP were independent risk factors for hospital readmission. Patients with specific comorbidities were also at increased risk for admissions, including those with peripheral vascular disease, renal failure, lymphoma, fluid and electrolyte disorders, and anemia. Because this is the first study viewing readmissions from the perspective of primary care practices, our findings are unique in the literature. However, hospital‐based studies have found similar relationships between readmission rates and these specific comorbidities.[11, 12, 15] Unlike other studies, our study cohort did not show CHF as an independent risk factor. We hypothesize this is because in 2008, UCSF Medical Center introduced an inpatient‐based intervention to reduce readmissions in patients with heart failure. By 2011, the readmission rates of patients with heart failure (primary or secondary diagnoses) had dropped by 30%. The success of this program, focused only on patients with heart failure, likely affected our analysis of comorbidities as risk factors for readmissions.
Models developed to predict hospital readmissions, overall and for specific disease conditions, have inconsistently identified predictive factors, and there is not a specific set of variables that dominate.[16, 17, 18, 19] A recent review of readmission risk prediction models suggested that models that take into account psychosocial factors such as social support, substance abuse, and functional status improved model performance.[16] We hypothesize that the reason why male gender was significant in our model may be related to lack of social support, especially among those who may be single or widowed. Other studies have also showed male gender as a predictor for hospital readmissions.[14, 20]
People with Medicare as the primary payor or Medicare with Medicaid also tended to have higher risk of readmission. We believe that this may be a proxy for the combined effect of age, multiple comorbidities, and psychosocial factors. In a multicenter study of general medicine inpatients, Medicare, but not age, was also found to be a predictive variable.[21] Unlike other studies, our study did not find Medicaid alone as a significant predictive variable for readmissions.[15, 21] One explanation may be that in hospital‐based studies, people with Medicaid who are at high risk for readmission may be high‐risk because they do not have a PCP or good access to outpatient care. In our study, all patients have a PCP in 1 of the UCSF clinics, and access to care is improved with this established relationship. These other studies did not examine Medicare‐Medicaid dual eligibility status. Our results are consistent with a national study on avoidable hospital admissions that showed the dual eligibility population experiencing 60% higher avoidable admission rates compared to the Medicaid‐only population.[22]
An interesting finding was that there were no statistically significant differences in readmissions among patients who report a language other than English as their primary language. Although language barriers and health literacy can affect a patient's ability to understand discharge instructions, the use of translators at UCSF Medical Center may have decreased the risk of readmissions. For a small number of patients whose primary language was not recorded (unknown language in our model), they appeared to have a lower risk of readmissions. Language preferences are recorded by our admitting staff during the process of admission. This step may have been skipped after hours or if the patient was not able to answer the question. However, we do not have a good hypothesis as to why these patients may have a reduced risk of readmissions.
Having a departed PCP in the General Internal Medicine clinic was an independent predictor of readmissions. These patients have access to primary care; they can schedule acute appointments with covering providers for new medical issues or follow‐up of chronic conditions. However, until they are transferred to a new PCP, they do not have a provider who is proactively managing their preventive and chronic disease care, including follow‐up and coordinating care after hospital discharge. Our study is not the first to suggest adverse outcomes for patients who are in transition from 1 primary care provider to the next.[23] Studies conducted in General Internal Medicine clinics have shown missed opportunities for cancer screening and overlooked test results during the transition period,[24] and as many as one‐fifth of patients whom residents identified as high‐risk were lost to follow‐up.[25] However, our study is the first to show the link between PCP transition in a teaching clinic and hospital readmissions. This finding underscores the importance of continuity of care in the optimal management of patients following hospital discharge.
There are several limitations to this study. First, our study only considers hospitalizations to UCSF Medical Center, potentially undercounting readmissions to area hospitals. Because our study population are patients with PCPs at UCSF, these patients tend to seek specialty and acute care at UCSF Medical Center as well. We obtained payor data from our medical group (Hill Physicians), which covers our largest private payors, to understand whether our results can be applied on a global basis. We found that in 87.4% of index admissions with readmissions from January 1, 2010 through May 30, 2012, the readmission occurred at UCSF Medical Center. We conducted a similar analysis with CMS data from October 1, 2008 to June 30, 2011, the latest data we have from CMS. For patients with UCSF PCPs and a diagnosis of AMI or CHF, 100% were readmitted back to UCSF Medical Center. For patients with UCSF PCPs and a diagnosis of pneumonia, 89% were readmitted back to UCSF Medical Center. Given that only a small percentage of patients with PCPs at UCSF present for readmission at other area hospitals, we believe that limiting our analysis to UCSF Medical Center is reasonable.
In our study, we did not remove vaginal deliveries or Caesarian sections prior to building the model. Primary care physicians and their clinic leadership are accustomed to taking a population‐health perspective. We anticipate they would be interested in designing interventions and addressing readmission for the entire primary care panel. Although readmissions after delivery are not frequent, they can still occur, and interventions should not necessarily exclude this population. We did run a sensitivity analysis by removing vaginal delivery and Caesarian sections from the analysis. As expected, readmission rates for all practices increased except for Geriatrics. However, the independent predictors of readmissions did not change.
Our study is based on a population of patients at 1 urban academic medical center and may not be generalizable across all delivery systems. Our population is racially and ethnically diverse, and many do not speak English as their primary language. The study also spans different types of primary care clinics, capturing a wide range of ages and case mix. As PCP assignments fluctuate over time, there may be errors with PCP attribution in the UCSF Medical Center data systems. We do not believe errors in PCP attribution would differ across primary care practices. Because the primary care practices' performance reports on quality measures are based on PCP assignment, each clinic regularly updates their clinic panels and has specific protocols to address errors with PCP attribution.
Finally, our study includes only the variables that we were able to extract from administrative claims. Other explanatory variables that have been suggested as important for evaluation, such as social support, functional assessment, access to care, hospital discharge process, and posthospitalization follow‐up, were not included. Each of these could be explored in future studies.
This study offers a unique perspective of hospital readmission by introducing a new methodology for primary care clinics to calculate and evaluate their all‐cause 30‐day readmission rates. Although this methodology is not intended to provide real‐time feedback to clinics on readmitted patients, it opens the door for benchmarking based on specific case‐mix indices. Another direction for future research is to design robust evaluations of the impact of interventions, both inpatient and outpatient, on primary care clinic readmission rates. Finally, future research should replicate this analysis across teaching clinics to identify whether provider turnover is a consistent independent predictor of hospital readmissions.
This study also has implications for inpatient interventions. For example, discharging physicians may consider proactively identifying whether the patient has a continuous primary care provider. Patients who are in between PCPs may need closer follow‐up after discharge, until they re‐establish with a new PCP. This can be accomplished through a postdischarge clinic visit, either run by inpatient providers or covering physicians in the primary care clinic. In addition, the discharging physician may work with the case manager to increase the level of care coordination. The case manager could contact the primary care clinic and proactively ask for immediate PCP re‐assignment. Once a new PCP has been identified, the discharging physician could consider a warm hand‐off. With a warm hand‐off, the new PCP may feel more comfortable managing problems that may arise after hospital discharge, and especially before the first outpatient visit with the new PCP. Future research can test whether these interventions could effectively reduce hospital readmissions across a broad primary care population.
CONCLUSION
Primary care providers and their clinics play an important role in managing population health, decreasing healthcare spending, and keeping patients out of the hospital. In this study, we introduce a tool in which primary care clinics can begin to understand their hospital readmission rates. This may be particularly valuable as primary care providers enter global payment arrangements such as accountable care organizations or bundled payments and are responsible for a population of patients across the continuum of care. We found significant variation in readmission rates between different primary care practices, but much of this variation appears to be due to differences between practices in patient demographics, comorbidities, and hospitalization factors. Our study is the first to show the association between provider transitions and higher hospital readmissions. Continuity of care is critical for the optimal management of patients following hospital discharge. More attention will need to be focused on providing good continuity outpatient care for patients at high risk for readmissions.
Acknowledgements
The authors acknowledge Janelle Lee, MBA, MHA, DrPH, for her assistance with extracting the hospital claims data at UCSF Medical Center.
Disclosure: Dr. Tang and Ms. Maselli were supported by a University of California, Center for Health Quality and Innovation grant. The authors report no conflicts of interest.
- Medicare's new hospital value‐based purchasing program is likely to have only a small impact on hospital payments. Health Aff. 2012;31:1932–1940. , .
- Contemporary evidence about hospital strategies for reducing 30‐day readmissions. J Am Coll Cardiol. 2012;60:607–614. , , , et al.
- Interventions to reduce 30‐day rehospitalization: a systematic review. Ann Intern Med. 2011;155:520–528. , , , , .
- Reducing hospital readmissions: lessons from top‐performing hospitals. The Commonwealth Fund Synthesis Report. Available at: http://www.commonwealthfund.org/publications/case‐studies/2011/apr/reducing‐hospital‐readmissions. Published April 6, 2011. Accessed February 8, 2013. , , .
- Effective Interventions to Reduce Rehospitalizations: A Survey of the Published Evidence. Cambridge, MA: Institute for Healthcare Improvement; 2009. , .
- Recasting readmissions by placing the hospital role in community context. JAMA. 2013;309:351–352. , , .
- Hospitalists and care transitions: the divorce of inpatient and outpatient care. Health Affairs. 2008;27:1315–1327. , , , .
- Center for Medicare and Medicaid Innovation. Community‐based Care Transitions Program. Available at: http://innovation.cms.gov/initiatives/CCTP/#collapse‐tableDetails. Accessed February 8, 2013.
- Medicare's transitional care payment—a step toward the medical home. N Engl J Med. 2013;368:692–694. , , .
- Volume thresholds and hospital characteristics in the United States. Health Aff (Millwood). 2003;22:167–177. , , .
- Thirty‐day rehospitalizations after acute myocardial infarction: a cohort study. Ann Intern Med. 2012;157:11–18. , , , , , .
- Rehospitalizations among patients in the Medicare fee‐for‐service program. N Engl J Med. 2009;360:1418–1428. , , .
- Associations between reduced hospital length of stay and 30‐day readmission rate and mortality: 14‐year experience in 129 Veterans Affairs hospitals. Ann Intern Med. 2012;157:837–845. , , , et al.
- Readmission after hospitalization for congestive heart failure among Medicare beneficiaries. Arch Intern Med. 1997;157:99–104. , , , et al.
- Redefining readmission risk factors for general medicine patients. J Hosp Med. 2011;6:54–60. , , , .
- Risk prediction models for hospital readmission: a systematic review. JAMA. 2011;206:1688–1698. , , , et al.
- Statistical models and patient predictors of readmission for heart failure: a systematic review. Arch Intern Med. 2008;168:1371–1386. , , , et al.
- Statistical models and patient predictors of readmission for acute myocardial infarction: a systematic review. Circ Cardiovasc Qual Outcomes. 2009;2:500–507. , , , .
- Predictors of hospital readmission after stroke: a systematic review. Stroke. 2010;41:2525–2533. , , , et al.
- Risk factors for 30‐day hospital readmission in patients ≥65 years of age. Proc (Bayl Univ Med Cent). 2008;21:363–372. , , , , .
- Hospital readmission in general medicine patients: a prediction model. J Gen Intern Med. 2010;25:211–219. , , , et al.
- Users of Medicaid home and community‐based services are especially vulnerable to costly avoidable hospital admissions. Health Affairs. 2012;31:1167–1175. , , .
- Academic year‐end transfers of outpatients from outgoing to incoming residents: an unaddressed patient safety issue. JAMA. 2009;302:1327–1329. , .
- The revolving door of resident continuity practice: identifying gaps in transitions of care. J Gen Intern Med. 2011;26:995–998. , , , , , .
- Outcomes for resident‐identified high‐risk patients and resident perspectives of year‐end continuity clinic handoffs. J Gen Intern Med. 2012;27:1438–1444. , , , et al.
- Medicare's new hospital value‐based purchasing program is likely to have only a small impact on hospital payments. Health Aff. 2012;31:1932–1940. , .
- Contemporary evidence about hospital strategies for reducing 30‐day readmissions. J Am Coll Cardiol. 2012;60:607–614. , , , et al.
- Interventions to reduce 30‐day rehospitalization: a systematic review. Ann Intern Med. 2011;155:520–528. , , , , .
- Reducing hospital readmissions: lessons from top‐performing hospitals. The Commonwealth Fund Synthesis Report. Available at: http://www.commonwealthfund.org/publications/case‐studies/2011/apr/reducing‐hospital‐readmissions. Published April 6, 2011. Accessed February 8, 2013. , , .
- Effective Interventions to Reduce Rehospitalizations: A Survey of the Published Evidence. Cambridge, MA: Institute for Healthcare Improvement; 2009. , .
- Recasting readmissions by placing the hospital role in community context. JAMA. 2013;309:351–352. , , .
- Hospitalists and care transitions: the divorce of inpatient and outpatient care. Health Affairs. 2008;27:1315–1327. , , , .
- Center for Medicare and Medicaid Innovation. Community‐based Care Transitions Program. Available at: http://innovation.cms.gov/initiatives/CCTP/#collapse‐tableDetails. Accessed February 8, 2013.
- Medicare's transitional care payment—a step toward the medical home. N Engl J Med. 2013;368:692–694. , , .
- Volume thresholds and hospital characteristics in the United States. Health Aff (Millwood). 2003;22:167–177. , , .
- Thirty‐day rehospitalizations after acute myocardial infarction: a cohort study. Ann Intern Med. 2012;157:11–18. , , , , , .
- Rehospitalizations among patients in the Medicare fee‐for‐service program. N Engl J Med. 2009;360:1418–1428. , , .
- Associations between reduced hospital length of stay and 30‐day readmission rate and mortality: 14‐year experience in 129 Veterans Affairs hospitals. Ann Intern Med. 2012;157:837–845. , , , et al.
- Readmission after hospitalization for congestive heart failure among Medicare beneficiaries. Arch Intern Med. 1997;157:99–104. , , , et al.
- Redefining readmission risk factors for general medicine patients. J Hosp Med. 2011;6:54–60. , , , .
- Risk prediction models for hospital readmission: a systematic review. JAMA. 2011;206:1688–1698. , , , et al.
- Statistical models and patient predictors of readmission for heart failure: a systematic review. Arch Intern Med. 2008;168:1371–1386. , , , et al.
- Statistical models and patient predictors of readmission for acute myocardial infarction: a systematic review. Circ Cardiovasc Qual Outcomes. 2009;2:500–507. , , , .
- Predictors of hospital readmission after stroke: a systematic review. Stroke. 2010;41:2525–2533. , , , et al.
- Risk factors for 30‐day hospital readmission in patients ≥65 years of age. Proc (Bayl Univ Med Cent). 2008;21:363–372. , , , , .
- Hospital readmission in general medicine patients: a prediction model. J Gen Intern Med. 2010;25:211–219. , , , et al.
- Users of Medicaid home and community‐based services are especially vulnerable to costly avoidable hospital admissions. Health Affairs. 2012;31:1167–1175. , , .
- Academic year‐end transfers of outpatients from outgoing to incoming residents: an unaddressed patient safety issue. JAMA. 2009;302:1327–1329. , .
- The revolving door of resident continuity practice: identifying gaps in transitions of care. J Gen Intern Med. 2011;26:995–998. , , , , , .
- Outcomes for resident‐identified high‐risk patients and resident perspectives of year‐end continuity clinic handoffs. J Gen Intern Med. 2012;27:1438–1444. , , , et al.
© 2014 Society of Hospital Medicine