User login
Inpatient Pediatric Service Redesign
Given its positive effects on improving effectiveness and efficiency, Lean Six Sigma (LSS) is a business approach that is receiving a great deal of attention in the healthcare industry.[1, 2, 3, 4, 5, 6, 7] Although there are differences between Lean and Six Sigma, at their core they are both customer‐centered, quality methodologies designed to improve process efficiency and product quality through waste elimination, creating standardized work and reducing variation.[8]
Six Sigma is a rigorous problem‐focused process improvement method that focuses on defect removal, variation reduction, and customer satisfaction that relies heavily on statistical analysis. It includes 5 steps: define, measure, analyze, improvement, and control.[7, 8] Six Sigma assumes through variation reduction, defect removal, and meeting customer specifications, the performance of the organization can be improved and also meet the requirements of the customer.[8]
Lean is more process‐focused. It places emphasis on creating flow by removing waste and getting the steps of any given process in the right sequence.[8] In Lean terms, waste is defined as anything that the customer does not value and anything that is not done right the first time.[9] This category of waste is termed nonvalue adding and unnecessary. It is estimated that 30% to 50% of all steps of hospital processes are nonvalue adding and unnecessary, and therefore can be defined as waste.[10] Lean identifies 8 different types of nonvalue adding and unnecessary wastes. They are defects and rework, overproduction, waiting, nonutilization of resources, transport, inventory, motion, and extra processing. Waste creates delays that negatively impact patient care and reduce healthcare productivity.[10] Therefore, it makes sense to apply Lean concepts of waste identification and elimination to improve process efficiency. For example, when a facility is at or exceeds its bed capacity, any delay in discharge creates throughput delays throughout the hospital.[5] Discharge delays often result in emergency department (ED) overcrowding, and also affects a hospital's ability to accommodate internal downgrades and outside referrals in a timely fashion.[11, 12] However, because the sequence of steps of the discharge process is variable and not standardized, the goal to achieve early discharges remains elusive.[13]
There are emerging data to support that current rounding censuses exceed most hospitalist's abilities to deliver safe and efficient care.[12, 14, 15, 16] It is unclear what that threshold should be, but the current industry standard has nonacademic hospitalists seeing 15 patients per day. Therefore, high patient censuses could be contributing to delays in patient discharge times that effect hospital throughput. We speculated that by implementing a lean, quick‐strike approach[17] designed to improve the sequencing of housestaff, attending, and nursing work by eliminating the wastes of rework, waiting, extra processing, and nonutilization of physician resources by restaffing, we could improve patient discharge times. We augmented the intervention by creating standardized workflow expectations, a discharge checklist, and implemented daily interdisciplinary discharge planning huddles.
We hypothesized these interventions would improve the median time of discharge order entry and time of patient discharge. Primary outcome measures were: (1) the change in time of discharge order and discharge time and (2) the proportion of patients discharged before noon and 2 pm. Secondary outcomes that were used as balance measures were length of stay (LOS) and 7‐day, 14‐day, and 30‐day readmission rates.
METHODS
Study Design
This was a prospective quality improvement intervention with concurrent controls aimed to determine if discharge efficiency could be improved by load‐balancing our service line with existing faculty and residents, creating daily standard work using a discharge checklist and interdisciplinary huddles (see Supporting Figure 1 through Supporting Figure 3 in the online version of this article). All discharge data were collected as part of our medical center's Department of Logistics standard data collection procedures using solutions from TeleTracking Technologies, Inc. (Pittsburgh, PA). All patients discharged Monday through Friday from the pediatric hospitalist service prior to the 6‐month high‐census period (before intervention) and the 6‐month high‐census period (intervention period) were included in the study. To serve as our control, we collected the same discharge data during the same time periods for the remaining services of the children's hospital. This study was approved by Penn State Hershey Medical Center's institutional review board.
Study Setting
The study was conducted at the Penn State Hershey Children's Hospital (PSHCH), which is a physically free‐standing 133‐bed university‐based tertiary care hospital located in central Pennsylvania. The hospital has 36 pediatric medical/surgical beds located in 2 units (1 general and 1 intermediate care). PSHCH performs approximately 4100 admissions per year, of which approximately 1100 are performed by the Division of Pediatric Hospital Medicine. Our division is composed of 8 academic hospitalists with 1 to 20+ years' experience. Historically, the months of October through April are months when our service‐line has average daily censuses (ADC) that routinely exceed 12 patients per hospitalist. During these months, the median times patient discharge orders are placed and patient discharges occur historically approach 2 pm and 4 pm, respectively, and exceed our internal benchmark by 2 hours. Discharges from the remaining medical and surgical service lines at PSHCH that occurred Monday through Friday during the concurrent pre‐ and postintervention time periods served as the control group.
Needs Assessment and LSS
Traditionally, morning patient rounds are allotted approximately 180 minutes. Therefore, a rounding team can only be expected to spend 13 minutes or less per patient when the census exceeds 12 patients. The cycle time to perform 1 discharge using our electronic medical record is approximately 20 minutes, which is almost 10 minutes longer than the allotted time per patient. During high‐census months, our service averages 4 to 5 discharges per day. To accommodate performing discharges during rounds would require spending 80 to 100 minutes of the 180 available minutes. This would leave only 80 to 100 minutes to see the remaining 8 to 10 patients. As a result of these constraints, discharges are typically completed by the residents in unsupervised batches each afternoon following the noon conference (Figure 1A).
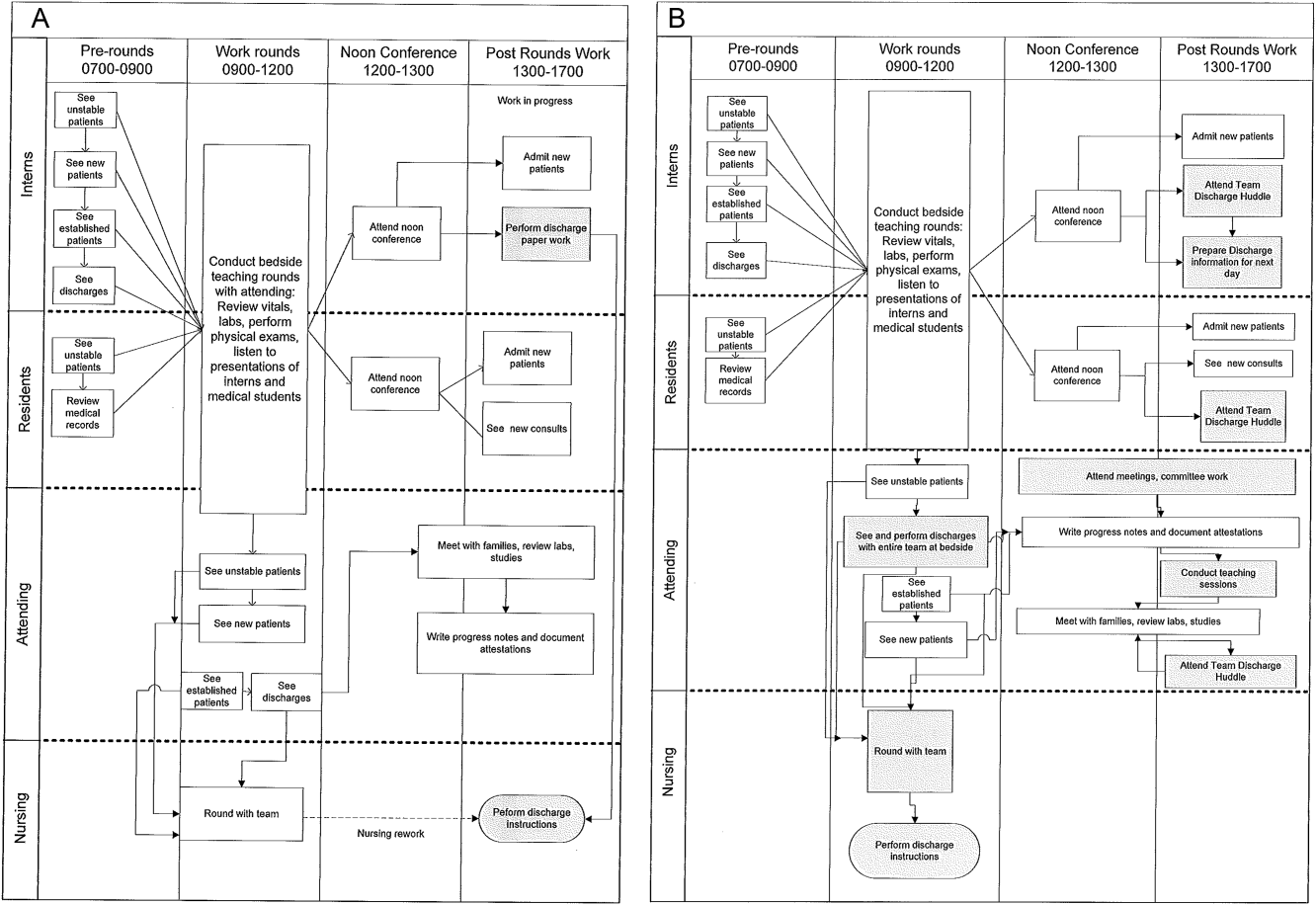
Because LSS focuses on eliminating nonvalue adding and unnecessary waste by load balancing processes and minimizing batching tasks,[8] this approach should lead one to question whether the current rounding model that requires 1 attending to see >12 high‐acuity patients with a maximum of 13 minutes per patient is system design flaw that leads to errors and inefficiency,[16] Theoretically, having an additional attending present would allow teams to resequence the work on smaller batches of patients and double the time to spend on each patient. This could create the opportunity to do value‐added work at the bedside in the presence of the family and nurse and eliminate the amount of nursing rework and time spent as work in progress on dischargeable patients (Figure 1).
Additionally, improving discharge efficiency creates virtual beds. Virtual beds permit hospitals to accommodate additional admissions despite operating with a fixed‐bed capacity. A way to calculate virtual beds is to calculate the reduction in LOS, and multiply that by the number of admissions per year divided by 36518 (see Supporting Figure 4 in the online version of this article). Our study was intended to determine the impact of discharge efficiency on this metric.
Intervention
We re‐structured our service line in a way that would balance both physician workload needs and patient expectations. To accomplish this, off service attendings were reallocated to round with a smaller resident team on fewer patients for the duration of the 6‐month study. Each member of the division agreed to work an average of 3 more weeks per year. One work day was estimated to be approximately 10 hours and 1 work week equaled 5 days, which asked for 150 hours of additional work per year. Because there increases in functional FTEs, the 2 teams consolidated into 1 team each weekend, to meet the group requirement that this model not result in additional weekend coverage. A balanced workload also theoretically allows the physician to spend more time at the bedside in direct patient care and resident education activities/observations.
In addition to reallocating physician and resident resources, our model created standard work expectations to reduce the variations in physician work sequences that can account for delayed discharge orders and delayed discharges, which is also an LSS principle. The intervention consisted of 3 changes: (1) fundamentally altering the composition of the rounding teams to optimize the provider: patient ratio; (2) defining rounding standards to expedite discharges; and (3) establishing a daily predischarge planning process.
The preintervention team typically had 1 attending, 1 to 2 senior residents, and 2 to 3 interns. The intervention period required creating 2 independently functioning teams, each composed of 1 hospitalist attending, and a minimum of 1 senior and 1 intern. The intervention occurred November through April, when the censuses predictably exceed 12 patients for the rounding attending. Because both teams functioned independently, all of the patients were divided equally between the 2 teams. Each team carried a panel of patients that included new, established, and dischargeable patients (Figure 1). We did not compare the number of provider handoffs before and during the intervention or time spent per patient.
Because the intervention required increasing the number of weeks on‐service by 2 to 3weeks per physician to reduce clinical work time, it meant redeploying previously off‐service attendings to coincide with peak demands. This aspect of the intervention made group buy‐in mandatory. The group agreed to distribute the predictably heavy workload that usually falls on 1 attending by adding a second attending for the busiest 6 months of the year. Our division voted unanimously to adopt this model despite the increase in service time, as long as weekend coverage was not increased.
As part of the intervention, we created standard work expectations within our division to (1) start rounds on dischargeable patients who were identified the prior evening during the (2) interdisciplinary huddle, and (3) have the entire departure process completed at the bedside using a discharge checklist (see Supporting Figure 1 through Figure 3 in the online version of this article). The expectations included a standard script for beginning rounds, selecting patients who could be discharged first, and completing all necessary discharge computer work at the bedside, before proceeding to the next patient. The daily predischarge huddle was instituted each afternoon to prepare discharges that were expected to occur the following day. The huddles were attended by care coordinators, social workers, and both medical teams. During the huddle, the team discussed anticipated discharges, scheduled follow‐up appointments and testing, faxed necessary prescriptions, and arranged any needed home services.
Inclusion and Exclusion Criteria for Patients
All patients discharged from the pediatric hospitalist inpatient service between Monday and Friday from April 8, 2013 to October 25, 2013 (preimplementation cohort) and October 28, 2013 to April 18, 2014 (postimplementation cohort) were eligible for inclusion. This included admitted patients and observation status patients. Patients discharged from the remaining PSHCH medical and surgical service lines were included in the control group analysis using the above criteria.
OUTCOME MEASURES
Throughput and Patient‐Level Outcomes
Primary outcomes included (1) time of electronic discharge order placement, (2) actual patient discharge time, (3) proportion of patients discharged before noon and 2 pm, (4) 7‐day, 14‐day, and 30‐day readmission rates, (5) length of stay (LOS), and (6) average daily census (ADC).
Statistical Analysis
The null hypothesis was that there would be no difference in discharge order time, discharge time, LOS, readmission rates, and daily discharges in the preintervention group compared to the intervention group. For time of order placement and actual patient discharge, the significance was assessed using Wilcoxon rank sum test and expressed as median time among the groups. Patient discharge before noon/2 pm was assessed by a logistic regression model. The predictor being the intervention group with the results expressed as odds ratios of discharge before noon/2 pm comparing the intervention group to the preintervention group. Readmission rates were assessed using a [2] test to see if there was a significant difference from what would be expected. Last, LOS and ADC were assessed by a Student t test and expressed as the means. The data were analyzed using SAS version 9.3 (SAS Institute, Cary, NC).
RESULTS
For our division's service line, both the ADC and number of patients discharged per day were significantly higher during the intervention months (Table 1). By comparison, the control group had a significantly lower ADC and lower average of discharges per day in the intervention time period. The new model permitted the teams to enter discharge orders earlier in the day, which ultimately lead to earlier patient discharges. The additive effect of the 3 interventions had a statistically significant effect on process efficiency metrics (Table 1). The median discharge order entry time decreased by 200 minutes from 14:05 to 10:45, and the median time of patient discharge decreased by 93 minutes from 15:48 to 14:15. By comparison, the median time of discharge order entry decreased 13:13 to 12:56 pm, but the median time of discharge increased 5 minutes 14:45 versus 14:50 in the control group. A significantly higher proportion of patients were discharged by noon (27% vs 14%; P<0.0001; odds ratio [OR]:2.2; 95% confidence interval [CI]: 1.6‐3.1) and by 2 pm during the intervention period (47% vs 30%; P<0.0001; OR: 2.1; 95% CI: 1.6‐2.7). There was no observed difference in the proportion of patients who were discharged by noon or 2 pm in the control group. Finally, in the intervention group, approximately 50% of patients had discharge orders entered before noon compared to 23% in the control group (Figure 2). The intervention demonstrated statistical significance in shifting the time of discharge order entry and the time of patient discharge when compared to the relatively less burdened PSHCH control group (Figures 2 and 3). As seen in Figure 4, the results were sustained for the duration of the study and appeared to improve throughout intervention. Finally, readmission rates at 7, 14, and 30 days postdischarge and LOS were not negatively affected (Table 1) in either the intervention or control group.
Outcomes | Experimental Model | Control Group | ||||
---|---|---|---|---|---|---|
Preintervention, n=421 | Intervention, n=552 | P Value | Preintervention, n=1,390 | Intervention, n=1,146 | P Value | |
Average daily census | 9.7 | 12.4 | <0.0001 | 45.9 | 43.4 | 0.002 |
Discharges per day | 3.1 | 4.5 | <0.00001 | 9.5 | 9.2 | 0.419 |
Average length of stay | 3.1 | 3.0 | 0.864 | 6.3 | 5.9 | 0.714 |
Discharge order time, median | 14:05 | 10:45 | <0.0001 | 13:13 | 12:56 | 0.053 |
Discharge from hospital, median | 15:48 | 14:15 | <0.0001 | 14:45 | 14:50 | 0.113 |
Patients discharged before noon | 59 (14%) | 147 (27%) | <0.0001 | 176 (13%) | 170 (15%) | 0.138 |
Patients discharged before 2 pm | 128 (30%) | 261 (47%) | <0.0001 | 519 (38%) | 447 (39%) | 0.512 |
7‐day readmission rates | 3.1% | 3.5% | 0.965 | 6.7% | 6.8% | 0.970 |
14‐day readmission rates | 5.8% | 5.8% | 0.981 | 12.0% | 13.5% | 0.301 |
30‐day readmission rates | 9.4% | 9.1% | 0.703 | 20.0% | 20.6% | 0.705 |
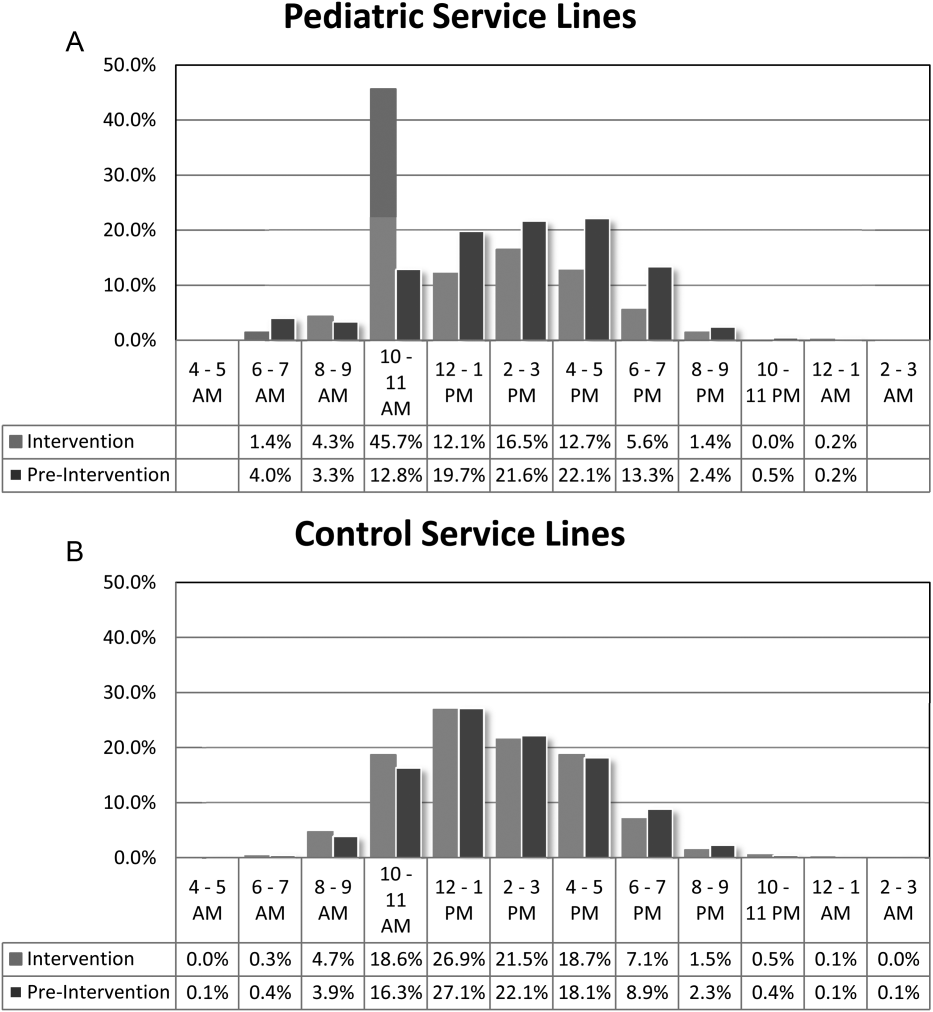
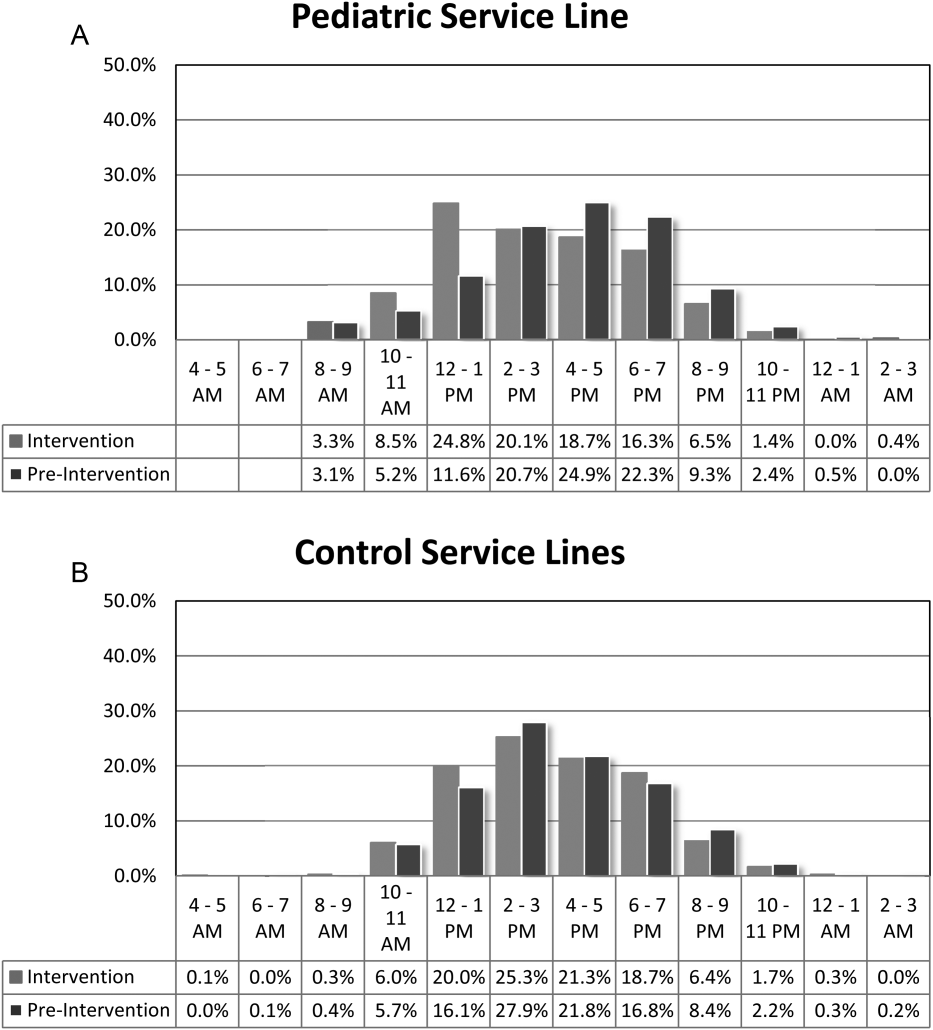
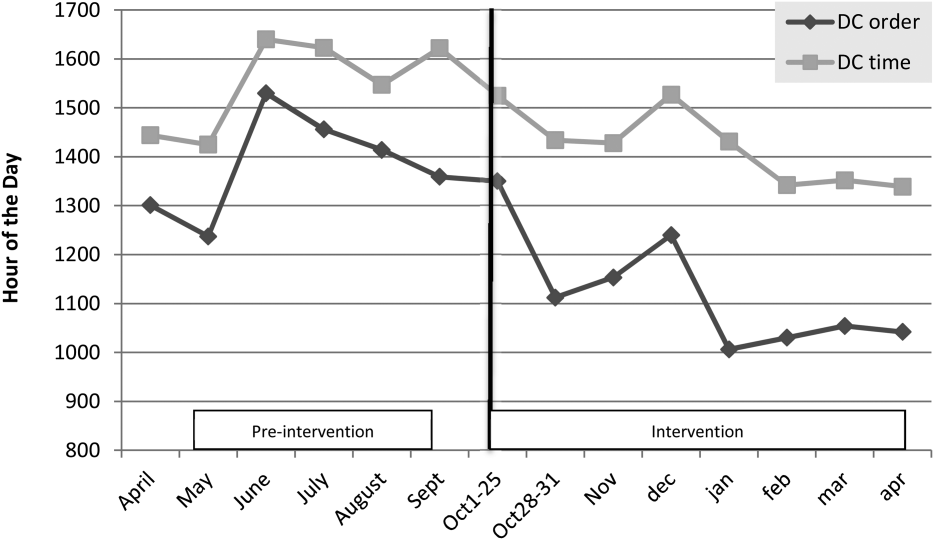
DISCUSSION
We demonstrated a statistically significant and what appears to be a sustainable improvement in median discharge order times, discharge times, and proportion of discharges by noon and 2 pm. Ours was the only service line in our medical center to achieve a median time discharge before our institution's internal metric of 2 pm and maintain it for 3 consecutive months. Additionally, the process demonstrated consistent performance independent of the varying styles and experience of the rounding attending during the busiest months of the year without incurring a negative impact on LOS or readmission rates.
Although our intervention demonstrated statistical significance in shifting the discharge distribution curves by almost 2 hours, more relevant is its potential clinical and financial impact. First, it puts our hospital in compliance with the Joint Commission's recommendations standard LD.04.03.1, stipulating that hospitals measure and set goals for mitigating and managing the flow of patients though the hospital. Second, our findings confirm the results of earlier studies suggesting that shifting discharge times could likely be achieved without the additional staff, but with alterations in staff shift scheduling.[11] Third, by doing required discharge work at the bedside and making it available earlier in the day, every day, we consistently reduced patient waiting along the entire supply chain.
Advancing the discharge time creates virtual beds that allow our facility to theoretically accommodate new patients. Using the calculation in the Methods section (see Supporting Figure 4 in the online version of this article) on how to calculate virtual beds, we determined that our intervention created between 0.30 and 0.38 virtual beds in a hospital with only 72 beds. We calculated that this would create 6.8 more open bed hours per day, 74 additional patient days per year, and assuming patients were waiting for the beds and rapid bed turnover, our intervention theoretically created the capacity to accommodate approximately 25 additional admissions per year (see Supporting Figure 4 in the online version of this article). As the only children's hospital in the region, this intervention will enable our organization to provide timelier access and possibly reduce time sensitive medical errors.
Timelier evaluations also have revenue potential by eliminating lost referrals, thus turning waste into value. When comparing the previous year's high‐census monthsOctober through Marchthere were 20 lost referrals due to lack of bed capacity, as compared to zero lost referrals during our intervention period. By accommodating these 20 additional admissions, we estimated this generated between $275,000 and $412,000 dollars in additional revenue without additional resources but simply staffing to demand.
Finally, when we looked at patient satisfaction metrics obtained through Press Ganey (PG), comparing the time periods we observed that overall satisfaction increased from the 91 percentile to the 94 percentile, trust in doctor increased from the 20 percentile to the 70 percentile, and would recommend this hospital to others increased from the 53 percentile to the 75 percentile. Interestingly, despite being a study that improved discharge efficiency, none of the discharge metrics gathered by PG improved. It is possible that this is a limitation of the PG survey, or could reflect the possibility that our new process exposed that our discharge order entry and discharge processes are misaligned.
When we surveyed the nursing staff and members of the division regarding whether or not to continue the intervention rounding model, 75% and 100%, respectively, voted in favor of continuing with the intervention model. Unfortunately, housestaff satisfaction was not measured for this study.
Despite more weeks in the hospital, but because there was better process sequencing, our providers indicated that because the workload of the primary attending was reduced and the workload for the additional attending was light, there was ample time to engage in afternoon nonclinical activities (Figure 1B). In fact, several division members assumed departmental and educational leadership positions, and others volunteered to facilitate highly valued, but unsubsidized, afternoon medical student and resident teaching sessions that occurred solely as a result of the resequenced and redistributed clinical load.
There are limitations to this study. First, because 3 interventions were implemented simultaneously, it is difficult to identify which component of the intervention was the primary driver for the measured differences. It is conceivable the proactive discharge planning that occurred during the afternoon predischarge planning huddles allowed the teams to complete discharge requirements the night before anticipated discharge therefore expediting the next morning's discharge. A second limitation was not simultaneously comparing the traditional rounding structure with the experimental model. One could argue that the improved efficiency we observed was not due to any of the interventions and represents secular trends that all residents' teams experience through the course of the year as they get more adept at performing patient discharges. However, when we compare our performance to the control group performance, this efficiency trend was not present. Additionally, it was possible that attendings were so result focused that they delayed discharges if the 2 pm discharge goal was missed for that day and planned for early discharges the following morning. If this behavior occurred, this would likely have been reflected as increases in our LOS data, however this was not observed. Third, because our preintervention data reflected discharge behaviors during a low‐census period, it is possible that there was less urgency to discharge patients when bed capacity issues did not exist. Comparing the intervention period to a period when censuses are similar would better address this issue. Finally, although we assert that attending workload is a fundamental waste‐producing constraint in the discharge process, this study did not determine what the optimal patient census should be.
Most hospitalists struggle with finding a balance of meeting patient quality and administrative productivity demands.[16] Hospitalists at academic medical centers have the added demand of maintaining their educational mission. Since 2001, the Institute of Medicine[19] has advocated process re‐engineering using more patient‐centered approaches. A recent study found that when hospitals reach capacity, the excess workload placed on internal medicine hospitalists reduces efficiency and increases costs.[16] Interestingly, in a study conducted by McMahon et al., they found that reducing team censuses by 50%, resident educational outcomes can be improved.[20] Similar to this study, our study reduced attending workload by 50% with the goal to assess the impact on discharge efficiency rather than educational outcomes. Also similar to that study, we radically altered the operational model in which physicians historically had functioned.[14, 20] Because the rounding structures in both studies reduced patient:provider ratios we believe that our model will successfully balance education, patient quality, and productivity.
LSS, when thoughtfully applied to the problems we face, could be part of the solution. It delivers quick results without large capital investments, by identifying and implementing high‐leverage changes that value a creative solution before a capital investment. One of the strengths of this model is that it does not require substantial financial investment to produce these outcomes. Because the morning clinical loads were more evenly distributed during the busiest months of the year, our division members were able to engage in nonclinical duties and teaching sessions, both of which often required afternoon commitments, but permitted us to balance work and professional achievement (Figure 1B). Finally, as part of any new process, one must consider the factors that influence its sustainability: provider level satisfaction, impact of the process change, and remuneration. Because the intervention reduced lost referrals, the departmental and institutional leadership agreed to financially incentivize the value‐generating potential this intervention had on increasing patient access by facilitating organizational throughput. Therefore, having met the three aforementioned elements, we believe this model is sustainable.
Although many studies remain results focused with aims at documenting how hospital processes fail when overburdened, our study takes a novel process‐focused approach to look at how processes can excel during periods of high demands, simply by reallocating existing resources.
Medicine is in the midst of multiple paradigm shifts involving resident work hour reduction, public safety reporting, reimbursement constraints, and value‐driven care, to name a few. Whether we take a resident or patient‐centered approach, it seems highly unlikely that the current approaches will meet these demands without making significant changes in how we deliver care. Next steps should include construction of a value stream map (VSM), with the input of all of the process stakeholders, that diagrams the entire discharge process. The VSM should highlight all nonvalue adding steps and eliminate them. They are likely a contributing cause to the disproportionate reduction in time of discharge order entry (200 minutes) versus actual discharge (93 minutes) seen in our study. Future work needs to establish the generalizability and sustainability of this model across other hospital service lines. Future studies should establish if this model has sustained impact on patient, provider, and resident satisfaction and overall system efficiency (ED boarding), with aims to quantify the revenue generating potential that occurs through waste elimination.
We close with the following thought: [T]o ask people to make different decisions without fundamentally changing the equation presented to them is wrong. If we wish to change the types of decisions our people make, we owe it to them to design and build processes that will put them in a position to succeed.[21]
Acknowledgements
The authors recognize the contributions made by members of the Division of Pediatric Hospital Medicine at PSHCH, without which this project would not have been possible. They are: Drs. Marta Biderman, Anika Kumar, Margaret Mikula, Chris O'Hara, Brandon Smith, and Ron Williams, and Heidi Wolf, and Lyndsay Gardener, CRNP. This project would not have been possible without the help from Brenda Ruhl, Manjula Narasimhan, and Heather Boyle from the Department of Logistics. Finally, the authors would like to thank the following for their thoughtful reviews of this manuscript: Drs. Lisa Scalzi, Barbara Ostrov, Jed Gonzalo, Chris Sciamanna, and Matt Wain.
Disclosures: Nothing to report.
- Theory of constraints—a review of the philosophy and its applications. Int J Oper Prod Man. 1998;18(4):336–355. .
- Use of lean and six sigma methodology to improve operating room efficiency in a high‐volume tertiary‐care academic medical center. J Am Coll Surg. 2011;213(1):83–92; discussion 93–84. , , , et al.
- The promise of Lean in health care. Mayo Clin Proc. 2013;88(1):74–82. , .
- Assessing the evidence of Six Sigma and Lean in the health care industry. Qual Manag Health Care. 2010;19(3):211–225. , , .
- Redesigning care at the Flinders Medical Centre: clinical process redesign using “lean thinking”. Med J Aust. 2008;188(6 suppl):S27–S31. , , , et al.
- Impact of 5 years of lean six sigma in a University Medical Center. Qual Manag Health Care. 2012;21(4):262–268. , , , , .
- A Lean Six Sigma quality improvement project to increase discharge paperwork completeness for admission to a comprehensive integrated inpatient rehabilitation program. Am J Med Qual. 2013;28(4):301–307. , , , et al.
- How to compare Six Sigma, lean and the theory of constraints—a framework for choosing what's best for your organization. Qual Prog. 2002;35(3):73–78. .
- Application of lean manufacturing techniques in the Emergency Department. J Emerg Med. 2009;37(2):177–182. , , , , .
- Lean Hospitals: Improving Quality, Patient Safety, and Employee Engagement. 2nd ed. New York, NY: Productivity Press/Taylor 2012. .
- The relationship between inpatient discharge timing and emergency department boarding. J Emerg Med. 2012;42(2):186–196. , , , , , .
- Unravelling relationships: Hospital occupancy levels, discharge timing and emergency department access block. Emerg Med Aust. 2012;24(5):510–517. , , , .
- Today's Hospitalist. 2013. Accessed November 2014. Available at: http://www.todayshospitalist.com/index.php?b=articles_read3(2):144–150. . Need to speed up discharges? The pros and cons of putting discharges on the clock.
- Hospitalist staffing requirements. Eff Clin Pract. 1999;2(3):126–130. , .
- Effect of hospitalist workload on the quality and efficiency of care. JAMA Intern Med. 2014;174(5):786–793. , , , , .
- Accelerating Lean Six Sigma Results: How to Achieve Improvement Excellence in the New Economy. Plantation, FL: J. Ross Publishing; 2011. .
- Healthcare Finance. Accessed October 2014. Available at: http://www.healthcarefinancenews.com/blog/virtual‐bed‐capacity‐may‐offer‐revenue‐boost‐hospitals. Published November 30, 2011. . “Virtual bed capacity” may offer revenue boost for hospitals.
- Institute of Medicine. Committee on Quality of Health Care in America. Crossing the Quality Chasm: A New Health System for the 21st Century. Washington, DC: National Academy Press; 2001.
- Evaluation of a redesign initiative in an internal‐medicine residency. N Engl J Med. 2010;362(14):1304–1311. , , , , .
- John Kim and Associates website. Available at: http://www.johnkimconsulting.com. Accessed June 2014.
Given its positive effects on improving effectiveness and efficiency, Lean Six Sigma (LSS) is a business approach that is receiving a great deal of attention in the healthcare industry.[1, 2, 3, 4, 5, 6, 7] Although there are differences between Lean and Six Sigma, at their core they are both customer‐centered, quality methodologies designed to improve process efficiency and product quality through waste elimination, creating standardized work and reducing variation.[8]
Six Sigma is a rigorous problem‐focused process improvement method that focuses on defect removal, variation reduction, and customer satisfaction that relies heavily on statistical analysis. It includes 5 steps: define, measure, analyze, improvement, and control.[7, 8] Six Sigma assumes through variation reduction, defect removal, and meeting customer specifications, the performance of the organization can be improved and also meet the requirements of the customer.[8]
Lean is more process‐focused. It places emphasis on creating flow by removing waste and getting the steps of any given process in the right sequence.[8] In Lean terms, waste is defined as anything that the customer does not value and anything that is not done right the first time.[9] This category of waste is termed nonvalue adding and unnecessary. It is estimated that 30% to 50% of all steps of hospital processes are nonvalue adding and unnecessary, and therefore can be defined as waste.[10] Lean identifies 8 different types of nonvalue adding and unnecessary wastes. They are defects and rework, overproduction, waiting, nonutilization of resources, transport, inventory, motion, and extra processing. Waste creates delays that negatively impact patient care and reduce healthcare productivity.[10] Therefore, it makes sense to apply Lean concepts of waste identification and elimination to improve process efficiency. For example, when a facility is at or exceeds its bed capacity, any delay in discharge creates throughput delays throughout the hospital.[5] Discharge delays often result in emergency department (ED) overcrowding, and also affects a hospital's ability to accommodate internal downgrades and outside referrals in a timely fashion.[11, 12] However, because the sequence of steps of the discharge process is variable and not standardized, the goal to achieve early discharges remains elusive.[13]
There are emerging data to support that current rounding censuses exceed most hospitalist's abilities to deliver safe and efficient care.[12, 14, 15, 16] It is unclear what that threshold should be, but the current industry standard has nonacademic hospitalists seeing 15 patients per day. Therefore, high patient censuses could be contributing to delays in patient discharge times that effect hospital throughput. We speculated that by implementing a lean, quick‐strike approach[17] designed to improve the sequencing of housestaff, attending, and nursing work by eliminating the wastes of rework, waiting, extra processing, and nonutilization of physician resources by restaffing, we could improve patient discharge times. We augmented the intervention by creating standardized workflow expectations, a discharge checklist, and implemented daily interdisciplinary discharge planning huddles.
We hypothesized these interventions would improve the median time of discharge order entry and time of patient discharge. Primary outcome measures were: (1) the change in time of discharge order and discharge time and (2) the proportion of patients discharged before noon and 2 pm. Secondary outcomes that were used as balance measures were length of stay (LOS) and 7‐day, 14‐day, and 30‐day readmission rates.
METHODS
Study Design
This was a prospective quality improvement intervention with concurrent controls aimed to determine if discharge efficiency could be improved by load‐balancing our service line with existing faculty and residents, creating daily standard work using a discharge checklist and interdisciplinary huddles (see Supporting Figure 1 through Supporting Figure 3 in the online version of this article). All discharge data were collected as part of our medical center's Department of Logistics standard data collection procedures using solutions from TeleTracking Technologies, Inc. (Pittsburgh, PA). All patients discharged Monday through Friday from the pediatric hospitalist service prior to the 6‐month high‐census period (before intervention) and the 6‐month high‐census period (intervention period) were included in the study. To serve as our control, we collected the same discharge data during the same time periods for the remaining services of the children's hospital. This study was approved by Penn State Hershey Medical Center's institutional review board.
Study Setting
The study was conducted at the Penn State Hershey Children's Hospital (PSHCH), which is a physically free‐standing 133‐bed university‐based tertiary care hospital located in central Pennsylvania. The hospital has 36 pediatric medical/surgical beds located in 2 units (1 general and 1 intermediate care). PSHCH performs approximately 4100 admissions per year, of which approximately 1100 are performed by the Division of Pediatric Hospital Medicine. Our division is composed of 8 academic hospitalists with 1 to 20+ years' experience. Historically, the months of October through April are months when our service‐line has average daily censuses (ADC) that routinely exceed 12 patients per hospitalist. During these months, the median times patient discharge orders are placed and patient discharges occur historically approach 2 pm and 4 pm, respectively, and exceed our internal benchmark by 2 hours. Discharges from the remaining medical and surgical service lines at PSHCH that occurred Monday through Friday during the concurrent pre‐ and postintervention time periods served as the control group.
Needs Assessment and LSS
Traditionally, morning patient rounds are allotted approximately 180 minutes. Therefore, a rounding team can only be expected to spend 13 minutes or less per patient when the census exceeds 12 patients. The cycle time to perform 1 discharge using our electronic medical record is approximately 20 minutes, which is almost 10 minutes longer than the allotted time per patient. During high‐census months, our service averages 4 to 5 discharges per day. To accommodate performing discharges during rounds would require spending 80 to 100 minutes of the 180 available minutes. This would leave only 80 to 100 minutes to see the remaining 8 to 10 patients. As a result of these constraints, discharges are typically completed by the residents in unsupervised batches each afternoon following the noon conference (Figure 1A).
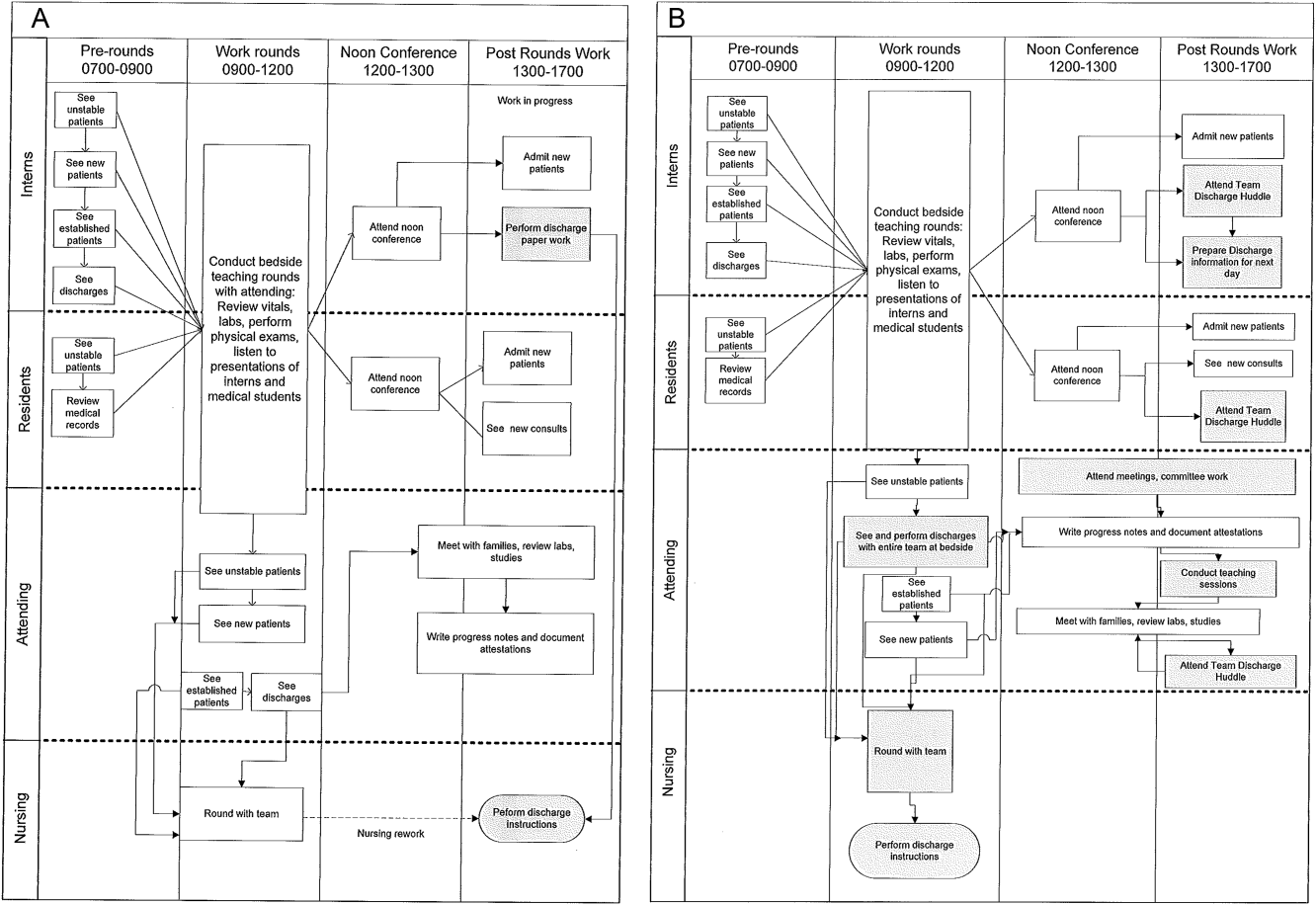
Because LSS focuses on eliminating nonvalue adding and unnecessary waste by load balancing processes and minimizing batching tasks,[8] this approach should lead one to question whether the current rounding model that requires 1 attending to see >12 high‐acuity patients with a maximum of 13 minutes per patient is system design flaw that leads to errors and inefficiency,[16] Theoretically, having an additional attending present would allow teams to resequence the work on smaller batches of patients and double the time to spend on each patient. This could create the opportunity to do value‐added work at the bedside in the presence of the family and nurse and eliminate the amount of nursing rework and time spent as work in progress on dischargeable patients (Figure 1).
Additionally, improving discharge efficiency creates virtual beds. Virtual beds permit hospitals to accommodate additional admissions despite operating with a fixed‐bed capacity. A way to calculate virtual beds is to calculate the reduction in LOS, and multiply that by the number of admissions per year divided by 36518 (see Supporting Figure 4 in the online version of this article). Our study was intended to determine the impact of discharge efficiency on this metric.
Intervention
We re‐structured our service line in a way that would balance both physician workload needs and patient expectations. To accomplish this, off service attendings were reallocated to round with a smaller resident team on fewer patients for the duration of the 6‐month study. Each member of the division agreed to work an average of 3 more weeks per year. One work day was estimated to be approximately 10 hours and 1 work week equaled 5 days, which asked for 150 hours of additional work per year. Because there increases in functional FTEs, the 2 teams consolidated into 1 team each weekend, to meet the group requirement that this model not result in additional weekend coverage. A balanced workload also theoretically allows the physician to spend more time at the bedside in direct patient care and resident education activities/observations.
In addition to reallocating physician and resident resources, our model created standard work expectations to reduce the variations in physician work sequences that can account for delayed discharge orders and delayed discharges, which is also an LSS principle. The intervention consisted of 3 changes: (1) fundamentally altering the composition of the rounding teams to optimize the provider: patient ratio; (2) defining rounding standards to expedite discharges; and (3) establishing a daily predischarge planning process.
The preintervention team typically had 1 attending, 1 to 2 senior residents, and 2 to 3 interns. The intervention period required creating 2 independently functioning teams, each composed of 1 hospitalist attending, and a minimum of 1 senior and 1 intern. The intervention occurred November through April, when the censuses predictably exceed 12 patients for the rounding attending. Because both teams functioned independently, all of the patients were divided equally between the 2 teams. Each team carried a panel of patients that included new, established, and dischargeable patients (Figure 1). We did not compare the number of provider handoffs before and during the intervention or time spent per patient.
Because the intervention required increasing the number of weeks on‐service by 2 to 3weeks per physician to reduce clinical work time, it meant redeploying previously off‐service attendings to coincide with peak demands. This aspect of the intervention made group buy‐in mandatory. The group agreed to distribute the predictably heavy workload that usually falls on 1 attending by adding a second attending for the busiest 6 months of the year. Our division voted unanimously to adopt this model despite the increase in service time, as long as weekend coverage was not increased.
As part of the intervention, we created standard work expectations within our division to (1) start rounds on dischargeable patients who were identified the prior evening during the (2) interdisciplinary huddle, and (3) have the entire departure process completed at the bedside using a discharge checklist (see Supporting Figure 1 through Figure 3 in the online version of this article). The expectations included a standard script for beginning rounds, selecting patients who could be discharged first, and completing all necessary discharge computer work at the bedside, before proceeding to the next patient. The daily predischarge huddle was instituted each afternoon to prepare discharges that were expected to occur the following day. The huddles were attended by care coordinators, social workers, and both medical teams. During the huddle, the team discussed anticipated discharges, scheduled follow‐up appointments and testing, faxed necessary prescriptions, and arranged any needed home services.
Inclusion and Exclusion Criteria for Patients
All patients discharged from the pediatric hospitalist inpatient service between Monday and Friday from April 8, 2013 to October 25, 2013 (preimplementation cohort) and October 28, 2013 to April 18, 2014 (postimplementation cohort) were eligible for inclusion. This included admitted patients and observation status patients. Patients discharged from the remaining PSHCH medical and surgical service lines were included in the control group analysis using the above criteria.
OUTCOME MEASURES
Throughput and Patient‐Level Outcomes
Primary outcomes included (1) time of electronic discharge order placement, (2) actual patient discharge time, (3) proportion of patients discharged before noon and 2 pm, (4) 7‐day, 14‐day, and 30‐day readmission rates, (5) length of stay (LOS), and (6) average daily census (ADC).
Statistical Analysis
The null hypothesis was that there would be no difference in discharge order time, discharge time, LOS, readmission rates, and daily discharges in the preintervention group compared to the intervention group. For time of order placement and actual patient discharge, the significance was assessed using Wilcoxon rank sum test and expressed as median time among the groups. Patient discharge before noon/2 pm was assessed by a logistic regression model. The predictor being the intervention group with the results expressed as odds ratios of discharge before noon/2 pm comparing the intervention group to the preintervention group. Readmission rates were assessed using a [2] test to see if there was a significant difference from what would be expected. Last, LOS and ADC were assessed by a Student t test and expressed as the means. The data were analyzed using SAS version 9.3 (SAS Institute, Cary, NC).
RESULTS
For our division's service line, both the ADC and number of patients discharged per day were significantly higher during the intervention months (Table 1). By comparison, the control group had a significantly lower ADC and lower average of discharges per day in the intervention time period. The new model permitted the teams to enter discharge orders earlier in the day, which ultimately lead to earlier patient discharges. The additive effect of the 3 interventions had a statistically significant effect on process efficiency metrics (Table 1). The median discharge order entry time decreased by 200 minutes from 14:05 to 10:45, and the median time of patient discharge decreased by 93 minutes from 15:48 to 14:15. By comparison, the median time of discharge order entry decreased 13:13 to 12:56 pm, but the median time of discharge increased 5 minutes 14:45 versus 14:50 in the control group. A significantly higher proportion of patients were discharged by noon (27% vs 14%; P<0.0001; odds ratio [OR]:2.2; 95% confidence interval [CI]: 1.6‐3.1) and by 2 pm during the intervention period (47% vs 30%; P<0.0001; OR: 2.1; 95% CI: 1.6‐2.7). There was no observed difference in the proportion of patients who were discharged by noon or 2 pm in the control group. Finally, in the intervention group, approximately 50% of patients had discharge orders entered before noon compared to 23% in the control group (Figure 2). The intervention demonstrated statistical significance in shifting the time of discharge order entry and the time of patient discharge when compared to the relatively less burdened PSHCH control group (Figures 2 and 3). As seen in Figure 4, the results were sustained for the duration of the study and appeared to improve throughout intervention. Finally, readmission rates at 7, 14, and 30 days postdischarge and LOS were not negatively affected (Table 1) in either the intervention or control group.
Outcomes | Experimental Model | Control Group | ||||
---|---|---|---|---|---|---|
Preintervention, n=421 | Intervention, n=552 | P Value | Preintervention, n=1,390 | Intervention, n=1,146 | P Value | |
Average daily census | 9.7 | 12.4 | <0.0001 | 45.9 | 43.4 | 0.002 |
Discharges per day | 3.1 | 4.5 | <0.00001 | 9.5 | 9.2 | 0.419 |
Average length of stay | 3.1 | 3.0 | 0.864 | 6.3 | 5.9 | 0.714 |
Discharge order time, median | 14:05 | 10:45 | <0.0001 | 13:13 | 12:56 | 0.053 |
Discharge from hospital, median | 15:48 | 14:15 | <0.0001 | 14:45 | 14:50 | 0.113 |
Patients discharged before noon | 59 (14%) | 147 (27%) | <0.0001 | 176 (13%) | 170 (15%) | 0.138 |
Patients discharged before 2 pm | 128 (30%) | 261 (47%) | <0.0001 | 519 (38%) | 447 (39%) | 0.512 |
7‐day readmission rates | 3.1% | 3.5% | 0.965 | 6.7% | 6.8% | 0.970 |
14‐day readmission rates | 5.8% | 5.8% | 0.981 | 12.0% | 13.5% | 0.301 |
30‐day readmission rates | 9.4% | 9.1% | 0.703 | 20.0% | 20.6% | 0.705 |
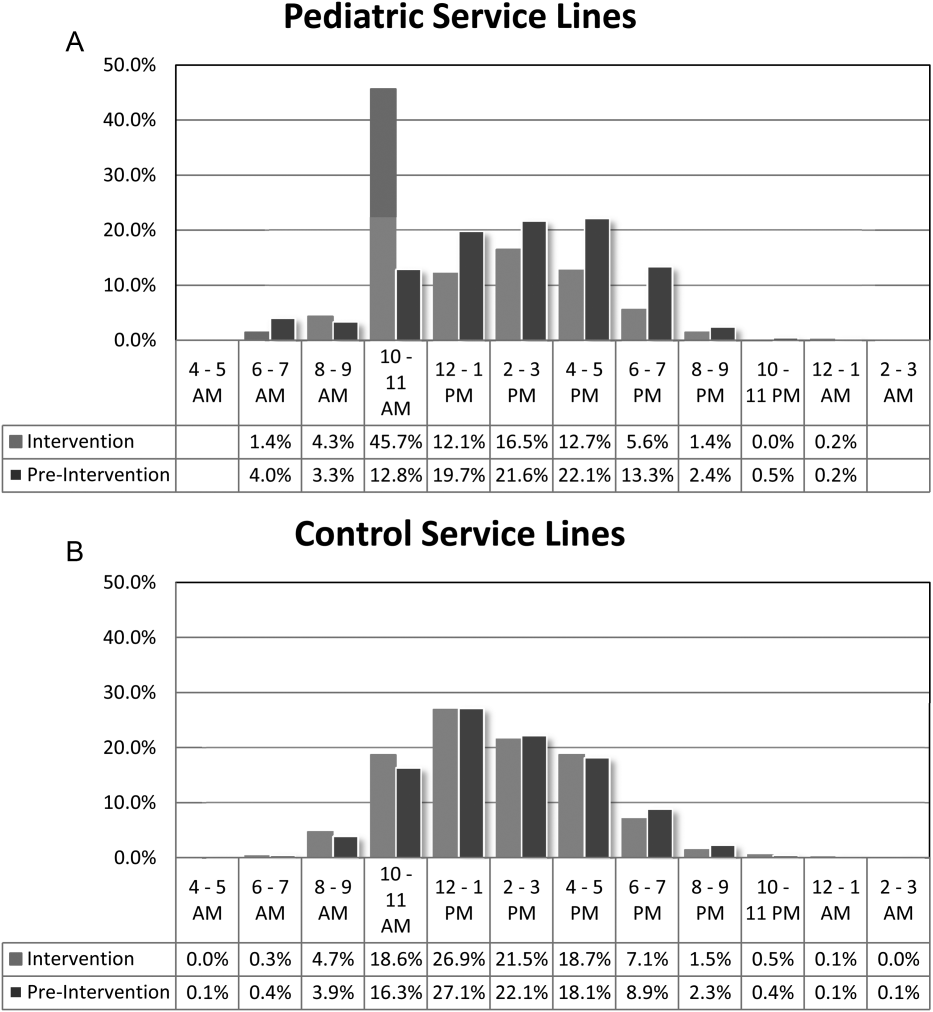
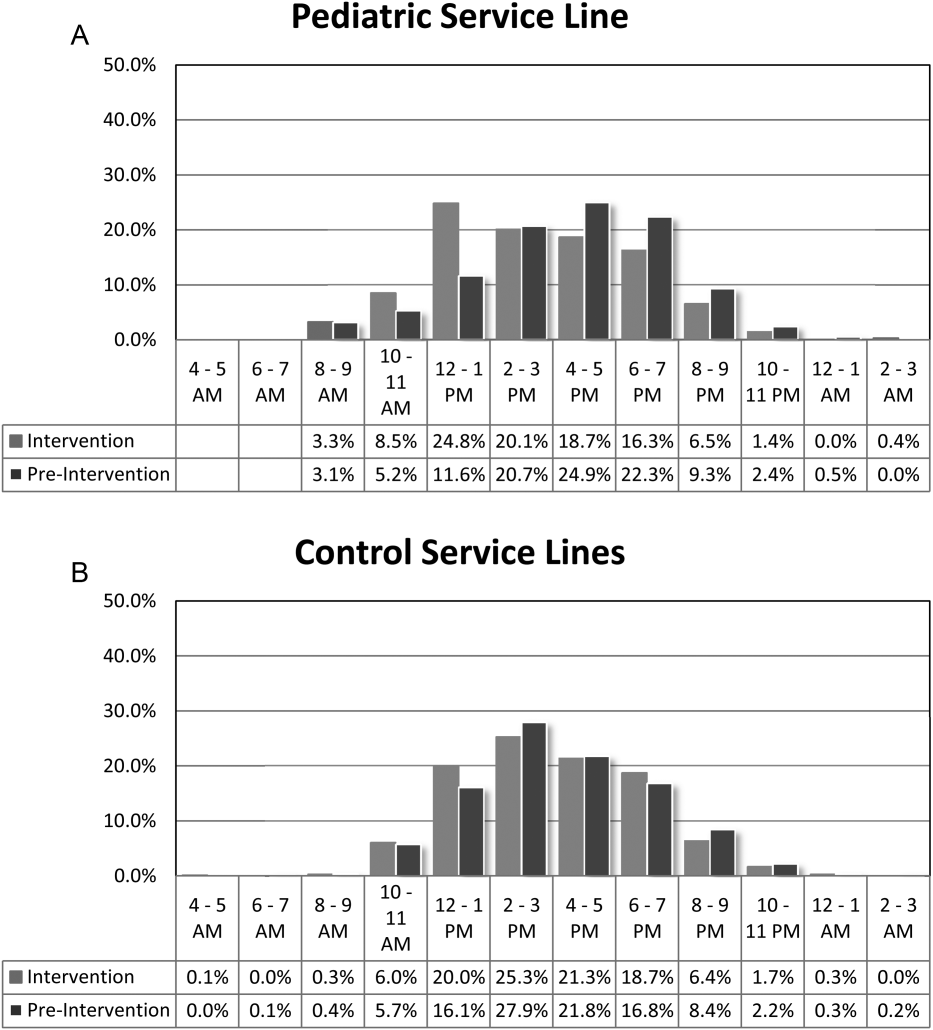
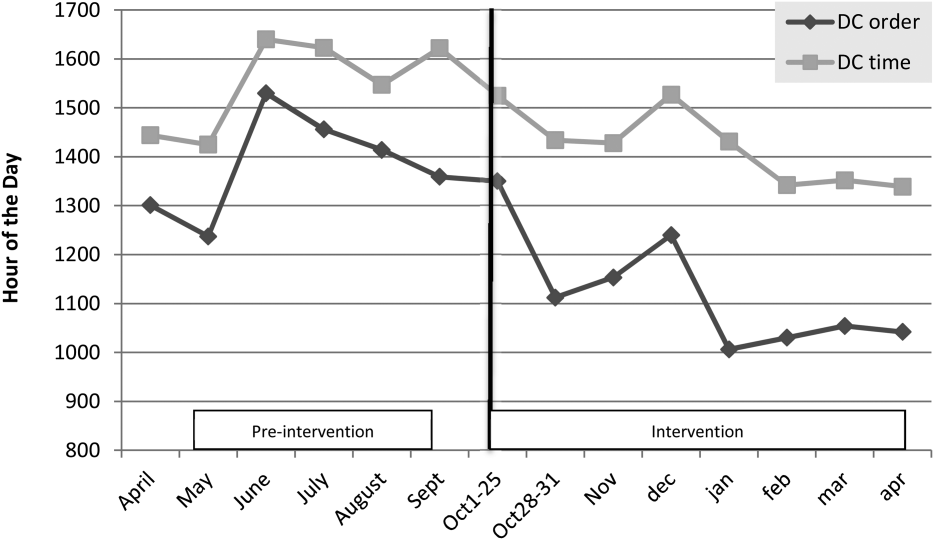
DISCUSSION
We demonstrated a statistically significant and what appears to be a sustainable improvement in median discharge order times, discharge times, and proportion of discharges by noon and 2 pm. Ours was the only service line in our medical center to achieve a median time discharge before our institution's internal metric of 2 pm and maintain it for 3 consecutive months. Additionally, the process demonstrated consistent performance independent of the varying styles and experience of the rounding attending during the busiest months of the year without incurring a negative impact on LOS or readmission rates.
Although our intervention demonstrated statistical significance in shifting the discharge distribution curves by almost 2 hours, more relevant is its potential clinical and financial impact. First, it puts our hospital in compliance with the Joint Commission's recommendations standard LD.04.03.1, stipulating that hospitals measure and set goals for mitigating and managing the flow of patients though the hospital. Second, our findings confirm the results of earlier studies suggesting that shifting discharge times could likely be achieved without the additional staff, but with alterations in staff shift scheduling.[11] Third, by doing required discharge work at the bedside and making it available earlier in the day, every day, we consistently reduced patient waiting along the entire supply chain.
Advancing the discharge time creates virtual beds that allow our facility to theoretically accommodate new patients. Using the calculation in the Methods section (see Supporting Figure 4 in the online version of this article) on how to calculate virtual beds, we determined that our intervention created between 0.30 and 0.38 virtual beds in a hospital with only 72 beds. We calculated that this would create 6.8 more open bed hours per day, 74 additional patient days per year, and assuming patients were waiting for the beds and rapid bed turnover, our intervention theoretically created the capacity to accommodate approximately 25 additional admissions per year (see Supporting Figure 4 in the online version of this article). As the only children's hospital in the region, this intervention will enable our organization to provide timelier access and possibly reduce time sensitive medical errors.
Timelier evaluations also have revenue potential by eliminating lost referrals, thus turning waste into value. When comparing the previous year's high‐census monthsOctober through Marchthere were 20 lost referrals due to lack of bed capacity, as compared to zero lost referrals during our intervention period. By accommodating these 20 additional admissions, we estimated this generated between $275,000 and $412,000 dollars in additional revenue without additional resources but simply staffing to demand.
Finally, when we looked at patient satisfaction metrics obtained through Press Ganey (PG), comparing the time periods we observed that overall satisfaction increased from the 91 percentile to the 94 percentile, trust in doctor increased from the 20 percentile to the 70 percentile, and would recommend this hospital to others increased from the 53 percentile to the 75 percentile. Interestingly, despite being a study that improved discharge efficiency, none of the discharge metrics gathered by PG improved. It is possible that this is a limitation of the PG survey, or could reflect the possibility that our new process exposed that our discharge order entry and discharge processes are misaligned.
When we surveyed the nursing staff and members of the division regarding whether or not to continue the intervention rounding model, 75% and 100%, respectively, voted in favor of continuing with the intervention model. Unfortunately, housestaff satisfaction was not measured for this study.
Despite more weeks in the hospital, but because there was better process sequencing, our providers indicated that because the workload of the primary attending was reduced and the workload for the additional attending was light, there was ample time to engage in afternoon nonclinical activities (Figure 1B). In fact, several division members assumed departmental and educational leadership positions, and others volunteered to facilitate highly valued, but unsubsidized, afternoon medical student and resident teaching sessions that occurred solely as a result of the resequenced and redistributed clinical load.
There are limitations to this study. First, because 3 interventions were implemented simultaneously, it is difficult to identify which component of the intervention was the primary driver for the measured differences. It is conceivable the proactive discharge planning that occurred during the afternoon predischarge planning huddles allowed the teams to complete discharge requirements the night before anticipated discharge therefore expediting the next morning's discharge. A second limitation was not simultaneously comparing the traditional rounding structure with the experimental model. One could argue that the improved efficiency we observed was not due to any of the interventions and represents secular trends that all residents' teams experience through the course of the year as they get more adept at performing patient discharges. However, when we compare our performance to the control group performance, this efficiency trend was not present. Additionally, it was possible that attendings were so result focused that they delayed discharges if the 2 pm discharge goal was missed for that day and planned for early discharges the following morning. If this behavior occurred, this would likely have been reflected as increases in our LOS data, however this was not observed. Third, because our preintervention data reflected discharge behaviors during a low‐census period, it is possible that there was less urgency to discharge patients when bed capacity issues did not exist. Comparing the intervention period to a period when censuses are similar would better address this issue. Finally, although we assert that attending workload is a fundamental waste‐producing constraint in the discharge process, this study did not determine what the optimal patient census should be.
Most hospitalists struggle with finding a balance of meeting patient quality and administrative productivity demands.[16] Hospitalists at academic medical centers have the added demand of maintaining their educational mission. Since 2001, the Institute of Medicine[19] has advocated process re‐engineering using more patient‐centered approaches. A recent study found that when hospitals reach capacity, the excess workload placed on internal medicine hospitalists reduces efficiency and increases costs.[16] Interestingly, in a study conducted by McMahon et al., they found that reducing team censuses by 50%, resident educational outcomes can be improved.[20] Similar to this study, our study reduced attending workload by 50% with the goal to assess the impact on discharge efficiency rather than educational outcomes. Also similar to that study, we radically altered the operational model in which physicians historically had functioned.[14, 20] Because the rounding structures in both studies reduced patient:provider ratios we believe that our model will successfully balance education, patient quality, and productivity.
LSS, when thoughtfully applied to the problems we face, could be part of the solution. It delivers quick results without large capital investments, by identifying and implementing high‐leverage changes that value a creative solution before a capital investment. One of the strengths of this model is that it does not require substantial financial investment to produce these outcomes. Because the morning clinical loads were more evenly distributed during the busiest months of the year, our division members were able to engage in nonclinical duties and teaching sessions, both of which often required afternoon commitments, but permitted us to balance work and professional achievement (Figure 1B). Finally, as part of any new process, one must consider the factors that influence its sustainability: provider level satisfaction, impact of the process change, and remuneration. Because the intervention reduced lost referrals, the departmental and institutional leadership agreed to financially incentivize the value‐generating potential this intervention had on increasing patient access by facilitating organizational throughput. Therefore, having met the three aforementioned elements, we believe this model is sustainable.
Although many studies remain results focused with aims at documenting how hospital processes fail when overburdened, our study takes a novel process‐focused approach to look at how processes can excel during periods of high demands, simply by reallocating existing resources.
Medicine is in the midst of multiple paradigm shifts involving resident work hour reduction, public safety reporting, reimbursement constraints, and value‐driven care, to name a few. Whether we take a resident or patient‐centered approach, it seems highly unlikely that the current approaches will meet these demands without making significant changes in how we deliver care. Next steps should include construction of a value stream map (VSM), with the input of all of the process stakeholders, that diagrams the entire discharge process. The VSM should highlight all nonvalue adding steps and eliminate them. They are likely a contributing cause to the disproportionate reduction in time of discharge order entry (200 minutes) versus actual discharge (93 minutes) seen in our study. Future work needs to establish the generalizability and sustainability of this model across other hospital service lines. Future studies should establish if this model has sustained impact on patient, provider, and resident satisfaction and overall system efficiency (ED boarding), with aims to quantify the revenue generating potential that occurs through waste elimination.
We close with the following thought: [T]o ask people to make different decisions without fundamentally changing the equation presented to them is wrong. If we wish to change the types of decisions our people make, we owe it to them to design and build processes that will put them in a position to succeed.[21]
Acknowledgements
The authors recognize the contributions made by members of the Division of Pediatric Hospital Medicine at PSHCH, without which this project would not have been possible. They are: Drs. Marta Biderman, Anika Kumar, Margaret Mikula, Chris O'Hara, Brandon Smith, and Ron Williams, and Heidi Wolf, and Lyndsay Gardener, CRNP. This project would not have been possible without the help from Brenda Ruhl, Manjula Narasimhan, and Heather Boyle from the Department of Logistics. Finally, the authors would like to thank the following for their thoughtful reviews of this manuscript: Drs. Lisa Scalzi, Barbara Ostrov, Jed Gonzalo, Chris Sciamanna, and Matt Wain.
Disclosures: Nothing to report.
Given its positive effects on improving effectiveness and efficiency, Lean Six Sigma (LSS) is a business approach that is receiving a great deal of attention in the healthcare industry.[1, 2, 3, 4, 5, 6, 7] Although there are differences between Lean and Six Sigma, at their core they are both customer‐centered, quality methodologies designed to improve process efficiency and product quality through waste elimination, creating standardized work and reducing variation.[8]
Six Sigma is a rigorous problem‐focused process improvement method that focuses on defect removal, variation reduction, and customer satisfaction that relies heavily on statistical analysis. It includes 5 steps: define, measure, analyze, improvement, and control.[7, 8] Six Sigma assumes through variation reduction, defect removal, and meeting customer specifications, the performance of the organization can be improved and also meet the requirements of the customer.[8]
Lean is more process‐focused. It places emphasis on creating flow by removing waste and getting the steps of any given process in the right sequence.[8] In Lean terms, waste is defined as anything that the customer does not value and anything that is not done right the first time.[9] This category of waste is termed nonvalue adding and unnecessary. It is estimated that 30% to 50% of all steps of hospital processes are nonvalue adding and unnecessary, and therefore can be defined as waste.[10] Lean identifies 8 different types of nonvalue adding and unnecessary wastes. They are defects and rework, overproduction, waiting, nonutilization of resources, transport, inventory, motion, and extra processing. Waste creates delays that negatively impact patient care and reduce healthcare productivity.[10] Therefore, it makes sense to apply Lean concepts of waste identification and elimination to improve process efficiency. For example, when a facility is at or exceeds its bed capacity, any delay in discharge creates throughput delays throughout the hospital.[5] Discharge delays often result in emergency department (ED) overcrowding, and also affects a hospital's ability to accommodate internal downgrades and outside referrals in a timely fashion.[11, 12] However, because the sequence of steps of the discharge process is variable and not standardized, the goal to achieve early discharges remains elusive.[13]
There are emerging data to support that current rounding censuses exceed most hospitalist's abilities to deliver safe and efficient care.[12, 14, 15, 16] It is unclear what that threshold should be, but the current industry standard has nonacademic hospitalists seeing 15 patients per day. Therefore, high patient censuses could be contributing to delays in patient discharge times that effect hospital throughput. We speculated that by implementing a lean, quick‐strike approach[17] designed to improve the sequencing of housestaff, attending, and nursing work by eliminating the wastes of rework, waiting, extra processing, and nonutilization of physician resources by restaffing, we could improve patient discharge times. We augmented the intervention by creating standardized workflow expectations, a discharge checklist, and implemented daily interdisciplinary discharge planning huddles.
We hypothesized these interventions would improve the median time of discharge order entry and time of patient discharge. Primary outcome measures were: (1) the change in time of discharge order and discharge time and (2) the proportion of patients discharged before noon and 2 pm. Secondary outcomes that were used as balance measures were length of stay (LOS) and 7‐day, 14‐day, and 30‐day readmission rates.
METHODS
Study Design
This was a prospective quality improvement intervention with concurrent controls aimed to determine if discharge efficiency could be improved by load‐balancing our service line with existing faculty and residents, creating daily standard work using a discharge checklist and interdisciplinary huddles (see Supporting Figure 1 through Supporting Figure 3 in the online version of this article). All discharge data were collected as part of our medical center's Department of Logistics standard data collection procedures using solutions from TeleTracking Technologies, Inc. (Pittsburgh, PA). All patients discharged Monday through Friday from the pediatric hospitalist service prior to the 6‐month high‐census period (before intervention) and the 6‐month high‐census period (intervention period) were included in the study. To serve as our control, we collected the same discharge data during the same time periods for the remaining services of the children's hospital. This study was approved by Penn State Hershey Medical Center's institutional review board.
Study Setting
The study was conducted at the Penn State Hershey Children's Hospital (PSHCH), which is a physically free‐standing 133‐bed university‐based tertiary care hospital located in central Pennsylvania. The hospital has 36 pediatric medical/surgical beds located in 2 units (1 general and 1 intermediate care). PSHCH performs approximately 4100 admissions per year, of which approximately 1100 are performed by the Division of Pediatric Hospital Medicine. Our division is composed of 8 academic hospitalists with 1 to 20+ years' experience. Historically, the months of October through April are months when our service‐line has average daily censuses (ADC) that routinely exceed 12 patients per hospitalist. During these months, the median times patient discharge orders are placed and patient discharges occur historically approach 2 pm and 4 pm, respectively, and exceed our internal benchmark by 2 hours. Discharges from the remaining medical and surgical service lines at PSHCH that occurred Monday through Friday during the concurrent pre‐ and postintervention time periods served as the control group.
Needs Assessment and LSS
Traditionally, morning patient rounds are allotted approximately 180 minutes. Therefore, a rounding team can only be expected to spend 13 minutes or less per patient when the census exceeds 12 patients. The cycle time to perform 1 discharge using our electronic medical record is approximately 20 minutes, which is almost 10 minutes longer than the allotted time per patient. During high‐census months, our service averages 4 to 5 discharges per day. To accommodate performing discharges during rounds would require spending 80 to 100 minutes of the 180 available minutes. This would leave only 80 to 100 minutes to see the remaining 8 to 10 patients. As a result of these constraints, discharges are typically completed by the residents in unsupervised batches each afternoon following the noon conference (Figure 1A).
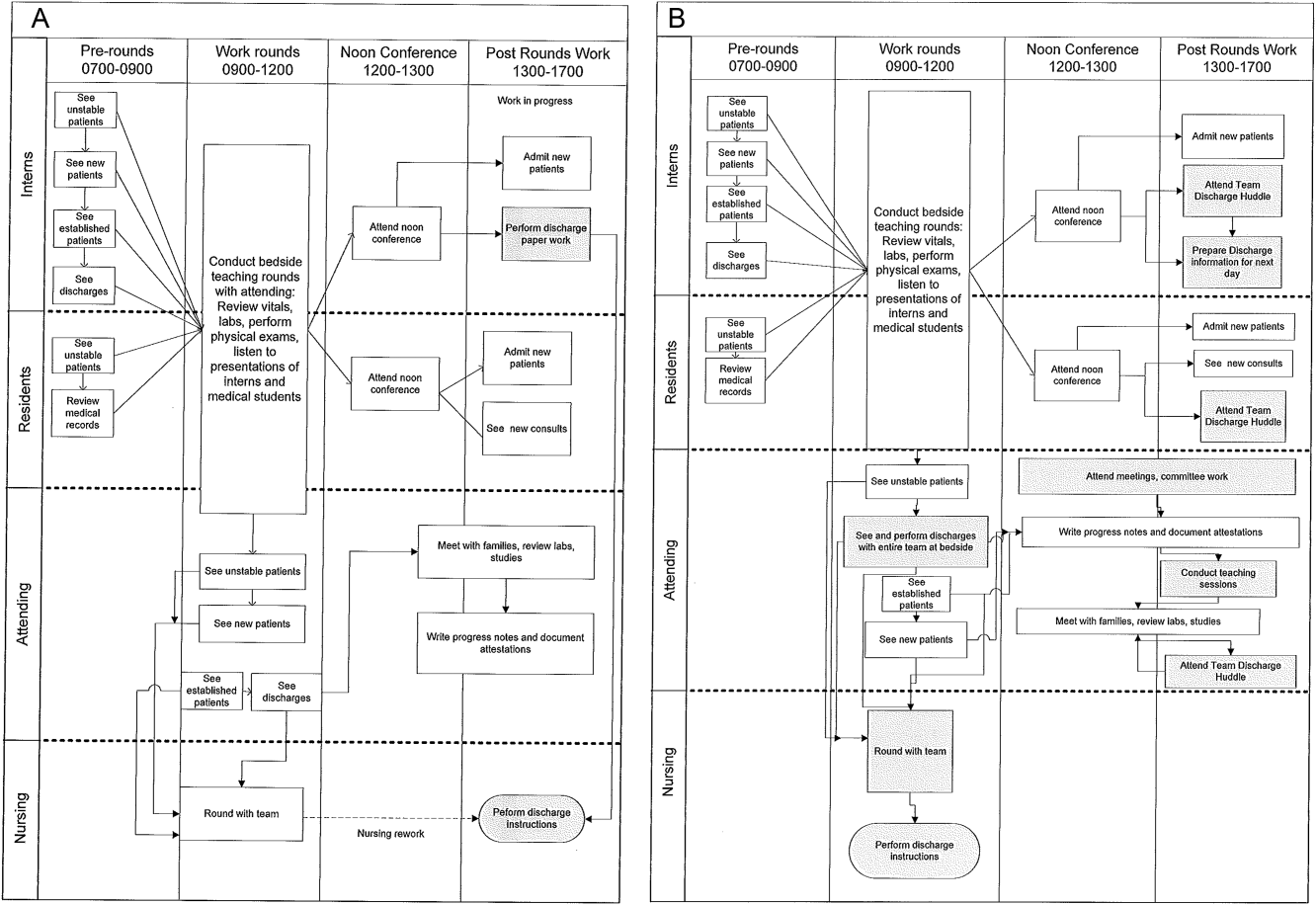
Because LSS focuses on eliminating nonvalue adding and unnecessary waste by load balancing processes and minimizing batching tasks,[8] this approach should lead one to question whether the current rounding model that requires 1 attending to see >12 high‐acuity patients with a maximum of 13 minutes per patient is system design flaw that leads to errors and inefficiency,[16] Theoretically, having an additional attending present would allow teams to resequence the work on smaller batches of patients and double the time to spend on each patient. This could create the opportunity to do value‐added work at the bedside in the presence of the family and nurse and eliminate the amount of nursing rework and time spent as work in progress on dischargeable patients (Figure 1).
Additionally, improving discharge efficiency creates virtual beds. Virtual beds permit hospitals to accommodate additional admissions despite operating with a fixed‐bed capacity. A way to calculate virtual beds is to calculate the reduction in LOS, and multiply that by the number of admissions per year divided by 36518 (see Supporting Figure 4 in the online version of this article). Our study was intended to determine the impact of discharge efficiency on this metric.
Intervention
We re‐structured our service line in a way that would balance both physician workload needs and patient expectations. To accomplish this, off service attendings were reallocated to round with a smaller resident team on fewer patients for the duration of the 6‐month study. Each member of the division agreed to work an average of 3 more weeks per year. One work day was estimated to be approximately 10 hours and 1 work week equaled 5 days, which asked for 150 hours of additional work per year. Because there increases in functional FTEs, the 2 teams consolidated into 1 team each weekend, to meet the group requirement that this model not result in additional weekend coverage. A balanced workload also theoretically allows the physician to spend more time at the bedside in direct patient care and resident education activities/observations.
In addition to reallocating physician and resident resources, our model created standard work expectations to reduce the variations in physician work sequences that can account for delayed discharge orders and delayed discharges, which is also an LSS principle. The intervention consisted of 3 changes: (1) fundamentally altering the composition of the rounding teams to optimize the provider: patient ratio; (2) defining rounding standards to expedite discharges; and (3) establishing a daily predischarge planning process.
The preintervention team typically had 1 attending, 1 to 2 senior residents, and 2 to 3 interns. The intervention period required creating 2 independently functioning teams, each composed of 1 hospitalist attending, and a minimum of 1 senior and 1 intern. The intervention occurred November through April, when the censuses predictably exceed 12 patients for the rounding attending. Because both teams functioned independently, all of the patients were divided equally between the 2 teams. Each team carried a panel of patients that included new, established, and dischargeable patients (Figure 1). We did not compare the number of provider handoffs before and during the intervention or time spent per patient.
Because the intervention required increasing the number of weeks on‐service by 2 to 3weeks per physician to reduce clinical work time, it meant redeploying previously off‐service attendings to coincide with peak demands. This aspect of the intervention made group buy‐in mandatory. The group agreed to distribute the predictably heavy workload that usually falls on 1 attending by adding a second attending for the busiest 6 months of the year. Our division voted unanimously to adopt this model despite the increase in service time, as long as weekend coverage was not increased.
As part of the intervention, we created standard work expectations within our division to (1) start rounds on dischargeable patients who were identified the prior evening during the (2) interdisciplinary huddle, and (3) have the entire departure process completed at the bedside using a discharge checklist (see Supporting Figure 1 through Figure 3 in the online version of this article). The expectations included a standard script for beginning rounds, selecting patients who could be discharged first, and completing all necessary discharge computer work at the bedside, before proceeding to the next patient. The daily predischarge huddle was instituted each afternoon to prepare discharges that were expected to occur the following day. The huddles were attended by care coordinators, social workers, and both medical teams. During the huddle, the team discussed anticipated discharges, scheduled follow‐up appointments and testing, faxed necessary prescriptions, and arranged any needed home services.
Inclusion and Exclusion Criteria for Patients
All patients discharged from the pediatric hospitalist inpatient service between Monday and Friday from April 8, 2013 to October 25, 2013 (preimplementation cohort) and October 28, 2013 to April 18, 2014 (postimplementation cohort) were eligible for inclusion. This included admitted patients and observation status patients. Patients discharged from the remaining PSHCH medical and surgical service lines were included in the control group analysis using the above criteria.
OUTCOME MEASURES
Throughput and Patient‐Level Outcomes
Primary outcomes included (1) time of electronic discharge order placement, (2) actual patient discharge time, (3) proportion of patients discharged before noon and 2 pm, (4) 7‐day, 14‐day, and 30‐day readmission rates, (5) length of stay (LOS), and (6) average daily census (ADC).
Statistical Analysis
The null hypothesis was that there would be no difference in discharge order time, discharge time, LOS, readmission rates, and daily discharges in the preintervention group compared to the intervention group. For time of order placement and actual patient discharge, the significance was assessed using Wilcoxon rank sum test and expressed as median time among the groups. Patient discharge before noon/2 pm was assessed by a logistic regression model. The predictor being the intervention group with the results expressed as odds ratios of discharge before noon/2 pm comparing the intervention group to the preintervention group. Readmission rates were assessed using a [2] test to see if there was a significant difference from what would be expected. Last, LOS and ADC were assessed by a Student t test and expressed as the means. The data were analyzed using SAS version 9.3 (SAS Institute, Cary, NC).
RESULTS
For our division's service line, both the ADC and number of patients discharged per day were significantly higher during the intervention months (Table 1). By comparison, the control group had a significantly lower ADC and lower average of discharges per day in the intervention time period. The new model permitted the teams to enter discharge orders earlier in the day, which ultimately lead to earlier patient discharges. The additive effect of the 3 interventions had a statistically significant effect on process efficiency metrics (Table 1). The median discharge order entry time decreased by 200 minutes from 14:05 to 10:45, and the median time of patient discharge decreased by 93 minutes from 15:48 to 14:15. By comparison, the median time of discharge order entry decreased 13:13 to 12:56 pm, but the median time of discharge increased 5 minutes 14:45 versus 14:50 in the control group. A significantly higher proportion of patients were discharged by noon (27% vs 14%; P<0.0001; odds ratio [OR]:2.2; 95% confidence interval [CI]: 1.6‐3.1) and by 2 pm during the intervention period (47% vs 30%; P<0.0001; OR: 2.1; 95% CI: 1.6‐2.7). There was no observed difference in the proportion of patients who were discharged by noon or 2 pm in the control group. Finally, in the intervention group, approximately 50% of patients had discharge orders entered before noon compared to 23% in the control group (Figure 2). The intervention demonstrated statistical significance in shifting the time of discharge order entry and the time of patient discharge when compared to the relatively less burdened PSHCH control group (Figures 2 and 3). As seen in Figure 4, the results were sustained for the duration of the study and appeared to improve throughout intervention. Finally, readmission rates at 7, 14, and 30 days postdischarge and LOS were not negatively affected (Table 1) in either the intervention or control group.
Outcomes | Experimental Model | Control Group | ||||
---|---|---|---|---|---|---|
Preintervention, n=421 | Intervention, n=552 | P Value | Preintervention, n=1,390 | Intervention, n=1,146 | P Value | |
Average daily census | 9.7 | 12.4 | <0.0001 | 45.9 | 43.4 | 0.002 |
Discharges per day | 3.1 | 4.5 | <0.00001 | 9.5 | 9.2 | 0.419 |
Average length of stay | 3.1 | 3.0 | 0.864 | 6.3 | 5.9 | 0.714 |
Discharge order time, median | 14:05 | 10:45 | <0.0001 | 13:13 | 12:56 | 0.053 |
Discharge from hospital, median | 15:48 | 14:15 | <0.0001 | 14:45 | 14:50 | 0.113 |
Patients discharged before noon | 59 (14%) | 147 (27%) | <0.0001 | 176 (13%) | 170 (15%) | 0.138 |
Patients discharged before 2 pm | 128 (30%) | 261 (47%) | <0.0001 | 519 (38%) | 447 (39%) | 0.512 |
7‐day readmission rates | 3.1% | 3.5% | 0.965 | 6.7% | 6.8% | 0.970 |
14‐day readmission rates | 5.8% | 5.8% | 0.981 | 12.0% | 13.5% | 0.301 |
30‐day readmission rates | 9.4% | 9.1% | 0.703 | 20.0% | 20.6% | 0.705 |
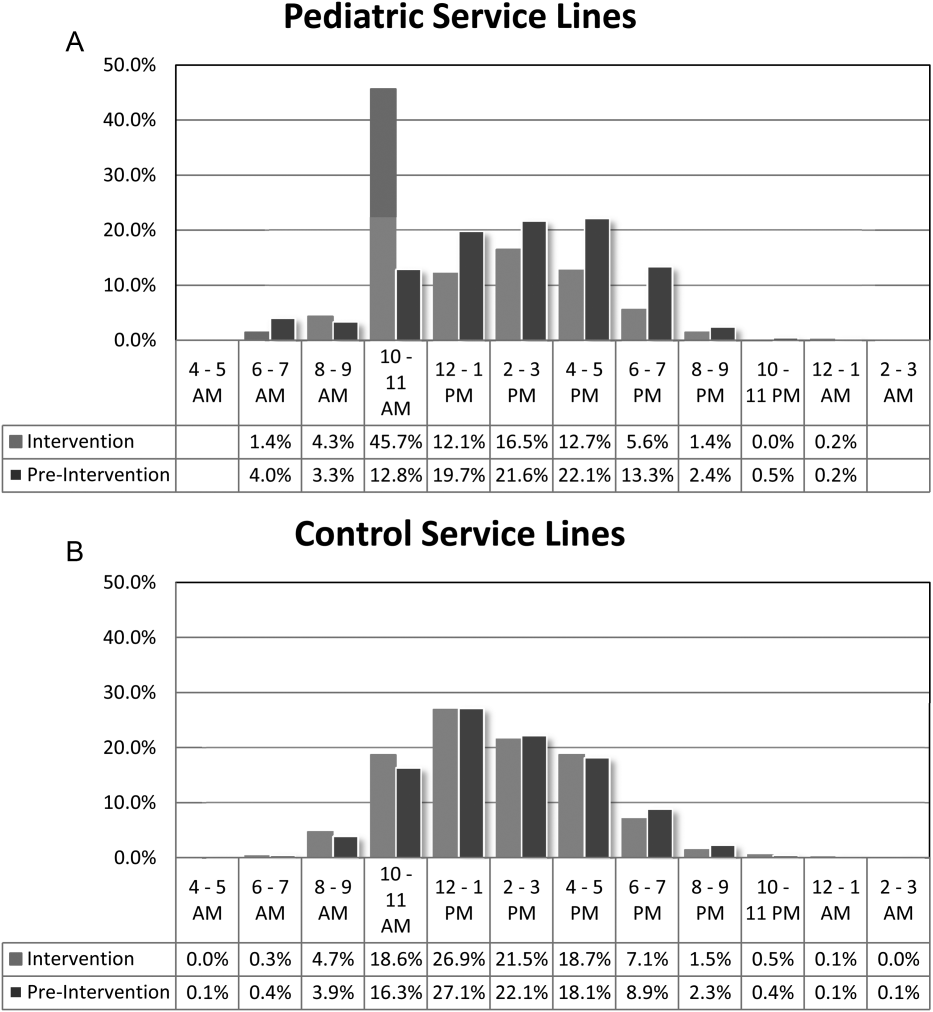
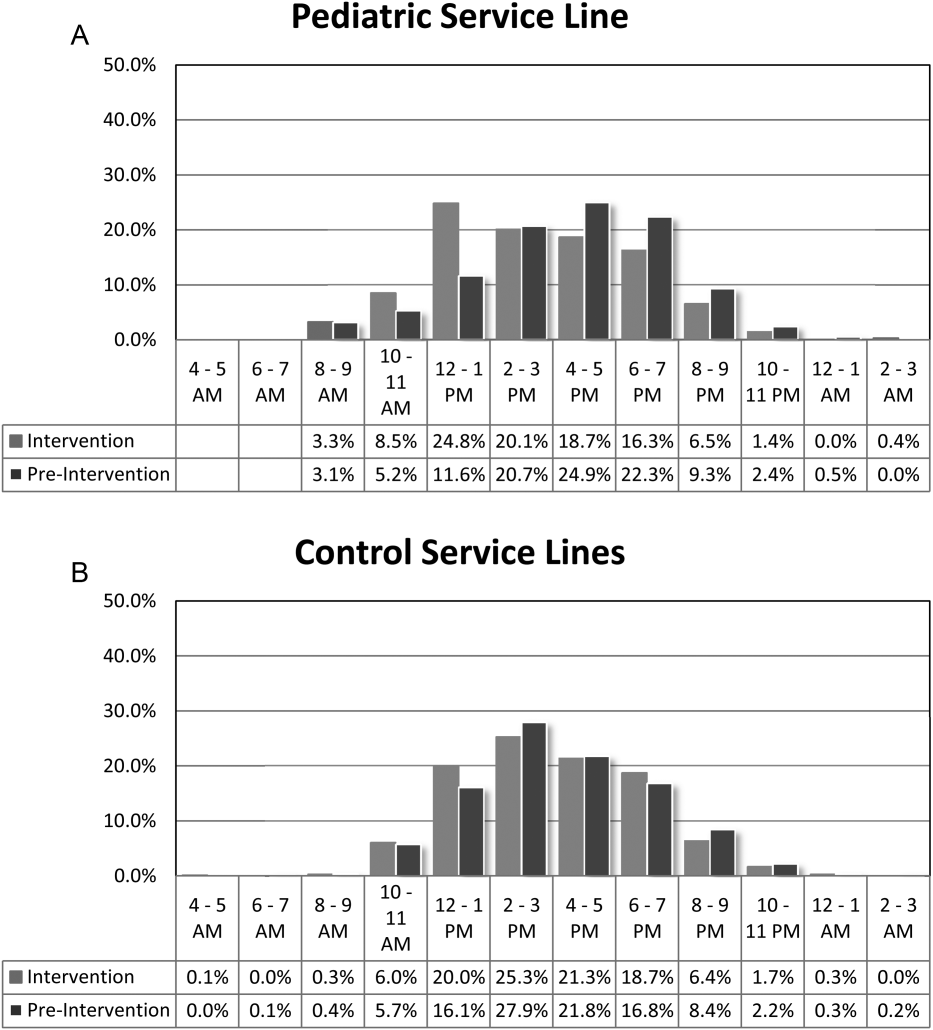
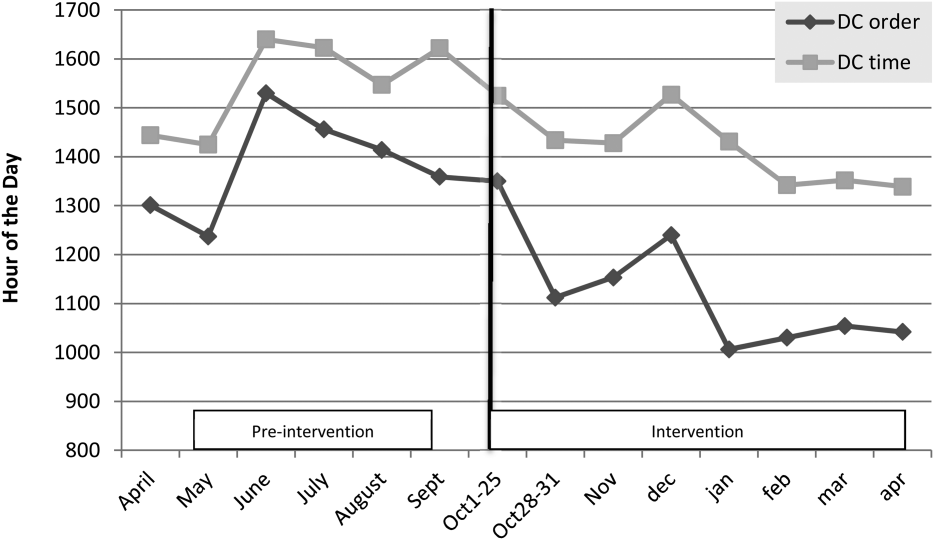
DISCUSSION
We demonstrated a statistically significant and what appears to be a sustainable improvement in median discharge order times, discharge times, and proportion of discharges by noon and 2 pm. Ours was the only service line in our medical center to achieve a median time discharge before our institution's internal metric of 2 pm and maintain it for 3 consecutive months. Additionally, the process demonstrated consistent performance independent of the varying styles and experience of the rounding attending during the busiest months of the year without incurring a negative impact on LOS or readmission rates.
Although our intervention demonstrated statistical significance in shifting the discharge distribution curves by almost 2 hours, more relevant is its potential clinical and financial impact. First, it puts our hospital in compliance with the Joint Commission's recommendations standard LD.04.03.1, stipulating that hospitals measure and set goals for mitigating and managing the flow of patients though the hospital. Second, our findings confirm the results of earlier studies suggesting that shifting discharge times could likely be achieved without the additional staff, but with alterations in staff shift scheduling.[11] Third, by doing required discharge work at the bedside and making it available earlier in the day, every day, we consistently reduced patient waiting along the entire supply chain.
Advancing the discharge time creates virtual beds that allow our facility to theoretically accommodate new patients. Using the calculation in the Methods section (see Supporting Figure 4 in the online version of this article) on how to calculate virtual beds, we determined that our intervention created between 0.30 and 0.38 virtual beds in a hospital with only 72 beds. We calculated that this would create 6.8 more open bed hours per day, 74 additional patient days per year, and assuming patients were waiting for the beds and rapid bed turnover, our intervention theoretically created the capacity to accommodate approximately 25 additional admissions per year (see Supporting Figure 4 in the online version of this article). As the only children's hospital in the region, this intervention will enable our organization to provide timelier access and possibly reduce time sensitive medical errors.
Timelier evaluations also have revenue potential by eliminating lost referrals, thus turning waste into value. When comparing the previous year's high‐census monthsOctober through Marchthere were 20 lost referrals due to lack of bed capacity, as compared to zero lost referrals during our intervention period. By accommodating these 20 additional admissions, we estimated this generated between $275,000 and $412,000 dollars in additional revenue without additional resources but simply staffing to demand.
Finally, when we looked at patient satisfaction metrics obtained through Press Ganey (PG), comparing the time periods we observed that overall satisfaction increased from the 91 percentile to the 94 percentile, trust in doctor increased from the 20 percentile to the 70 percentile, and would recommend this hospital to others increased from the 53 percentile to the 75 percentile. Interestingly, despite being a study that improved discharge efficiency, none of the discharge metrics gathered by PG improved. It is possible that this is a limitation of the PG survey, or could reflect the possibility that our new process exposed that our discharge order entry and discharge processes are misaligned.
When we surveyed the nursing staff and members of the division regarding whether or not to continue the intervention rounding model, 75% and 100%, respectively, voted in favor of continuing with the intervention model. Unfortunately, housestaff satisfaction was not measured for this study.
Despite more weeks in the hospital, but because there was better process sequencing, our providers indicated that because the workload of the primary attending was reduced and the workload for the additional attending was light, there was ample time to engage in afternoon nonclinical activities (Figure 1B). In fact, several division members assumed departmental and educational leadership positions, and others volunteered to facilitate highly valued, but unsubsidized, afternoon medical student and resident teaching sessions that occurred solely as a result of the resequenced and redistributed clinical load.
There are limitations to this study. First, because 3 interventions were implemented simultaneously, it is difficult to identify which component of the intervention was the primary driver for the measured differences. It is conceivable the proactive discharge planning that occurred during the afternoon predischarge planning huddles allowed the teams to complete discharge requirements the night before anticipated discharge therefore expediting the next morning's discharge. A second limitation was not simultaneously comparing the traditional rounding structure with the experimental model. One could argue that the improved efficiency we observed was not due to any of the interventions and represents secular trends that all residents' teams experience through the course of the year as they get more adept at performing patient discharges. However, when we compare our performance to the control group performance, this efficiency trend was not present. Additionally, it was possible that attendings were so result focused that they delayed discharges if the 2 pm discharge goal was missed for that day and planned for early discharges the following morning. If this behavior occurred, this would likely have been reflected as increases in our LOS data, however this was not observed. Third, because our preintervention data reflected discharge behaviors during a low‐census period, it is possible that there was less urgency to discharge patients when bed capacity issues did not exist. Comparing the intervention period to a period when censuses are similar would better address this issue. Finally, although we assert that attending workload is a fundamental waste‐producing constraint in the discharge process, this study did not determine what the optimal patient census should be.
Most hospitalists struggle with finding a balance of meeting patient quality and administrative productivity demands.[16] Hospitalists at academic medical centers have the added demand of maintaining their educational mission. Since 2001, the Institute of Medicine[19] has advocated process re‐engineering using more patient‐centered approaches. A recent study found that when hospitals reach capacity, the excess workload placed on internal medicine hospitalists reduces efficiency and increases costs.[16] Interestingly, in a study conducted by McMahon et al., they found that reducing team censuses by 50%, resident educational outcomes can be improved.[20] Similar to this study, our study reduced attending workload by 50% with the goal to assess the impact on discharge efficiency rather than educational outcomes. Also similar to that study, we radically altered the operational model in which physicians historically had functioned.[14, 20] Because the rounding structures in both studies reduced patient:provider ratios we believe that our model will successfully balance education, patient quality, and productivity.
LSS, when thoughtfully applied to the problems we face, could be part of the solution. It delivers quick results without large capital investments, by identifying and implementing high‐leverage changes that value a creative solution before a capital investment. One of the strengths of this model is that it does not require substantial financial investment to produce these outcomes. Because the morning clinical loads were more evenly distributed during the busiest months of the year, our division members were able to engage in nonclinical duties and teaching sessions, both of which often required afternoon commitments, but permitted us to balance work and professional achievement (Figure 1B). Finally, as part of any new process, one must consider the factors that influence its sustainability: provider level satisfaction, impact of the process change, and remuneration. Because the intervention reduced lost referrals, the departmental and institutional leadership agreed to financially incentivize the value‐generating potential this intervention had on increasing patient access by facilitating organizational throughput. Therefore, having met the three aforementioned elements, we believe this model is sustainable.
Although many studies remain results focused with aims at documenting how hospital processes fail when overburdened, our study takes a novel process‐focused approach to look at how processes can excel during periods of high demands, simply by reallocating existing resources.
Medicine is in the midst of multiple paradigm shifts involving resident work hour reduction, public safety reporting, reimbursement constraints, and value‐driven care, to name a few. Whether we take a resident or patient‐centered approach, it seems highly unlikely that the current approaches will meet these demands without making significant changes in how we deliver care. Next steps should include construction of a value stream map (VSM), with the input of all of the process stakeholders, that diagrams the entire discharge process. The VSM should highlight all nonvalue adding steps and eliminate them. They are likely a contributing cause to the disproportionate reduction in time of discharge order entry (200 minutes) versus actual discharge (93 minutes) seen in our study. Future work needs to establish the generalizability and sustainability of this model across other hospital service lines. Future studies should establish if this model has sustained impact on patient, provider, and resident satisfaction and overall system efficiency (ED boarding), with aims to quantify the revenue generating potential that occurs through waste elimination.
We close with the following thought: [T]o ask people to make different decisions without fundamentally changing the equation presented to them is wrong. If we wish to change the types of decisions our people make, we owe it to them to design and build processes that will put them in a position to succeed.[21]
Acknowledgements
The authors recognize the contributions made by members of the Division of Pediatric Hospital Medicine at PSHCH, without which this project would not have been possible. They are: Drs. Marta Biderman, Anika Kumar, Margaret Mikula, Chris O'Hara, Brandon Smith, and Ron Williams, and Heidi Wolf, and Lyndsay Gardener, CRNP. This project would not have been possible without the help from Brenda Ruhl, Manjula Narasimhan, and Heather Boyle from the Department of Logistics. Finally, the authors would like to thank the following for their thoughtful reviews of this manuscript: Drs. Lisa Scalzi, Barbara Ostrov, Jed Gonzalo, Chris Sciamanna, and Matt Wain.
Disclosures: Nothing to report.
- Theory of constraints—a review of the philosophy and its applications. Int J Oper Prod Man. 1998;18(4):336–355. .
- Use of lean and six sigma methodology to improve operating room efficiency in a high‐volume tertiary‐care academic medical center. J Am Coll Surg. 2011;213(1):83–92; discussion 93–84. , , , et al.
- The promise of Lean in health care. Mayo Clin Proc. 2013;88(1):74–82. , .
- Assessing the evidence of Six Sigma and Lean in the health care industry. Qual Manag Health Care. 2010;19(3):211–225. , , .
- Redesigning care at the Flinders Medical Centre: clinical process redesign using “lean thinking”. Med J Aust. 2008;188(6 suppl):S27–S31. , , , et al.
- Impact of 5 years of lean six sigma in a University Medical Center. Qual Manag Health Care. 2012;21(4):262–268. , , , , .
- A Lean Six Sigma quality improvement project to increase discharge paperwork completeness for admission to a comprehensive integrated inpatient rehabilitation program. Am J Med Qual. 2013;28(4):301–307. , , , et al.
- How to compare Six Sigma, lean and the theory of constraints—a framework for choosing what's best for your organization. Qual Prog. 2002;35(3):73–78. .
- Application of lean manufacturing techniques in the Emergency Department. J Emerg Med. 2009;37(2):177–182. , , , , .
- Lean Hospitals: Improving Quality, Patient Safety, and Employee Engagement. 2nd ed. New York, NY: Productivity Press/Taylor 2012. .
- The relationship between inpatient discharge timing and emergency department boarding. J Emerg Med. 2012;42(2):186–196. , , , , , .
- Unravelling relationships: Hospital occupancy levels, discharge timing and emergency department access block. Emerg Med Aust. 2012;24(5):510–517. , , , .
- Today's Hospitalist. 2013. Accessed November 2014. Available at: http://www.todayshospitalist.com/index.php?b=articles_read3(2):144–150. . Need to speed up discharges? The pros and cons of putting discharges on the clock.
- Hospitalist staffing requirements. Eff Clin Pract. 1999;2(3):126–130. , .
- Effect of hospitalist workload on the quality and efficiency of care. JAMA Intern Med. 2014;174(5):786–793. , , , , .
- Accelerating Lean Six Sigma Results: How to Achieve Improvement Excellence in the New Economy. Plantation, FL: J. Ross Publishing; 2011. .
- Healthcare Finance. Accessed October 2014. Available at: http://www.healthcarefinancenews.com/blog/virtual‐bed‐capacity‐may‐offer‐revenue‐boost‐hospitals. Published November 30, 2011. . “Virtual bed capacity” may offer revenue boost for hospitals.
- Institute of Medicine. Committee on Quality of Health Care in America. Crossing the Quality Chasm: A New Health System for the 21st Century. Washington, DC: National Academy Press; 2001.
- Evaluation of a redesign initiative in an internal‐medicine residency. N Engl J Med. 2010;362(14):1304–1311. , , , , .
- John Kim and Associates website. Available at: http://www.johnkimconsulting.com. Accessed June 2014.
- Theory of constraints—a review of the philosophy and its applications. Int J Oper Prod Man. 1998;18(4):336–355. .
- Use of lean and six sigma methodology to improve operating room efficiency in a high‐volume tertiary‐care academic medical center. J Am Coll Surg. 2011;213(1):83–92; discussion 93–84. , , , et al.
- The promise of Lean in health care. Mayo Clin Proc. 2013;88(1):74–82. , .
- Assessing the evidence of Six Sigma and Lean in the health care industry. Qual Manag Health Care. 2010;19(3):211–225. , , .
- Redesigning care at the Flinders Medical Centre: clinical process redesign using “lean thinking”. Med J Aust. 2008;188(6 suppl):S27–S31. , , , et al.
- Impact of 5 years of lean six sigma in a University Medical Center. Qual Manag Health Care. 2012;21(4):262–268. , , , , .
- A Lean Six Sigma quality improvement project to increase discharge paperwork completeness for admission to a comprehensive integrated inpatient rehabilitation program. Am J Med Qual. 2013;28(4):301–307. , , , et al.
- How to compare Six Sigma, lean and the theory of constraints—a framework for choosing what's best for your organization. Qual Prog. 2002;35(3):73–78. .
- Application of lean manufacturing techniques in the Emergency Department. J Emerg Med. 2009;37(2):177–182. , , , , .
- Lean Hospitals: Improving Quality, Patient Safety, and Employee Engagement. 2nd ed. New York, NY: Productivity Press/Taylor 2012. .
- The relationship between inpatient discharge timing and emergency department boarding. J Emerg Med. 2012;42(2):186–196. , , , , , .
- Unravelling relationships: Hospital occupancy levels, discharge timing and emergency department access block. Emerg Med Aust. 2012;24(5):510–517. , , , .
- Today's Hospitalist. 2013. Accessed November 2014. Available at: http://www.todayshospitalist.com/index.php?b=articles_read3(2):144–150. . Need to speed up discharges? The pros and cons of putting discharges on the clock.
- Hospitalist staffing requirements. Eff Clin Pract. 1999;2(3):126–130. , .
- Effect of hospitalist workload on the quality and efficiency of care. JAMA Intern Med. 2014;174(5):786–793. , , , , .
- Accelerating Lean Six Sigma Results: How to Achieve Improvement Excellence in the New Economy. Plantation, FL: J. Ross Publishing; 2011. .
- Healthcare Finance. Accessed October 2014. Available at: http://www.healthcarefinancenews.com/blog/virtual‐bed‐capacity‐may‐offer‐revenue‐boost‐hospitals. Published November 30, 2011. . “Virtual bed capacity” may offer revenue boost for hospitals.
- Institute of Medicine. Committee on Quality of Health Care in America. Crossing the Quality Chasm: A New Health System for the 21st Century. Washington, DC: National Academy Press; 2001.
- Evaluation of a redesign initiative in an internal‐medicine residency. N Engl J Med. 2010;362(14):1304–1311. , , , , .
- John Kim and Associates website. Available at: http://www.johnkimconsulting.com. Accessed June 2014.
© 2014 Society of Hospital Medicine