User login
Patients in general medicalsurgical wards who experience unplanned transfer to the intensive care unit (ICU) have increased mortality and morbidity.13 Using an externally validated methodology permitting assessment of illness severity and mortality risk among all hospitalized patients,4, 5 we recently documented observed‐to‐expected mortality ratios >3.0 and excess length of stay of 10 days among patients who experienced such transfers.6
It is possible to predict adverse outcomes among monitored patients (eg, patients in the ICU or undergoing continuous electronic monitoring).7, 8 However, prediction of unplanned transfers among medicalsurgical ward patients presents challenges. Data collection (vital signs and laboratory tests) is relatively infrequent. The event rate (3% of hospital admissions) is low, and the rate in narrow time periods (eg, 12 hours) is extremely low: a hospital with 4000 admissions per year might experience 1 unplanned transfer to the ICU every 3 days. Not surprisingly, performance of models suitable for predicting ward patients' need for intensive care within narrow time frames have been disappointing.9 The Modified Early Warning Score (MEWS), has a c‐statistic, or area under the receiver operator characteristic of 0.67,1012 and our own model incorporating 14 laboratory tests, but no vital signs, has excellent performance with respect to predicting inpatient mortality, but poor performance with respect to unplanned transfer.6
In this report, we describe the development and validation of a complex predictive model suitable for use with ward patients. Our objective for this work was to develop a predictive model based on clinical and physiologic data available in real time from a comprehensive electronic medical record (EMR), not a clinically intuitive, manually assigned tool. The outcome of interest was unplanned transfer from the ward to the ICU, or death on the ward in a patient who was full code. This model has been developed as part of a regional effort to decrease preventable mortality in the Northern California Kaiser Permanente Medical Care Program (KPMCP), an integrated healthcare delivery system with 22 hospitals.
MATERIALS AND METHODS
For additional details, see the Supporting Information, Appendices 112, in the online version of this article.
This project was approved by the KPMCP Institutional Board for the Protection of Human Subjects.
The Northern California KPMCP serves a total population of approximately 3.3 million members. All Northern California KPMCP hospitals and clinics employ the same information systems with a common medical record number and can track care covered by the plan but delivered elsewhere. Databases maintained by the KPMCP capture admission and discharge times, admission and discharge diagnoses and procedures (assigned by professional coders), bed histories permitting quantification of intra‐hospital transfers, inter‐hospital transfers, as well as the results of all inpatient and outpatient laboratory tests. In July 2006, the KPMCP began deployment of the EMR developed by Epic Systems Corporation (
Our setting consisted of 14 hospitals in which the KPHC inpatient EMR had been running for at least 3 months (the KPMCP Antioch, Fremont, Hayward, Manteca, Modesto, Roseville, Sacramento, Santa Clara, San Francisco, Santa Rosa, South Sacramento, South San Francisco, Santa Teresa, and Walnut Creek hospitals). We have described the general characteristics of KPMCP hospitals elsewhere.4, 6 Our initial study population consisted of all patients admitted to these hospitals who met the following criteria: hospitalization began from November 1, 2006 through December 31, 2009; initial hospitalization occurred at a Northern California KPMCP hospital (ie, for inter‐hospital transfers, the first hospital stay occurred within the KPMCP); age 18 years; hospitalization was not for childbirth; and KPHC had been operational at the hospital for at least 3 months.
Analytic Approach
The primary outcome for this study was transfer to the ICU after admission to the hospital among patients residing either in a general medicalsurgical ward (ward) or transitional care unit (TCU), or death in the ward or TCU in a patient who was full code at the time of death (ie, had the patient survived, s/he would have been transferred to the ICU). The unit of analysis for this study was a 12‐hour patient shift, which could begin with a 7 AM T0 (henceforth, day shift) or a 7 PM T0 (night shift); in other words, we aimed to predict the occurrence of an event within 12 hours of T0 using only data available prior to T0. A shift in which a patient experienced the primary study outcome is an event shift, while one in which a patient did not experience the primary outcome is a comparison shift. Using this approach, an individual patient record could consist of both event and comparison shifts, since some patients might have multiple unplanned transfers and some patients might have none. Our basic analytic approach consisted of creating a cohort of event and comparison shifts (10 comparison shifts were randomly selected for each event shift), splitting the cohort into a derivation dataset (50%) and validation dataset (50%), developing a model using the derivation dataset, then applying the coefficients of the derivation dataset to the validation dataset. Because some event shifts were excluded due to the minimum 4‐hour length‐of‐stay requirement, we also applied model coefficients to these excluded shifts and a set of randomly selected comparison shifts.
Since the purpose of these analyses was to develop models with maximal signal extraction from sparsely collected predictors, we did not block a time period after the T0 to allow for a reaction time to the alarm. Thus, since some events could occur immediately after the T0 (as can be seen in the Supporting Information, Appendices, in the online version of this article), our models would need to be run at intervals that are more frequent than 2 times a day.
Independent Variables
In addition to patients' age and sex, we tested the following candidate independent variables. Some of these variables are part of the KPMCP risk adjustment model4, 5 and were available electronically for all patients in the cohort. We grouped admission diagnoses into 44 broad diagnostic categories (primary conditions), and admission types into 4 groups (emergency medical, emergency surgical, elective medical, and elective surgical). We quantified patients' degree of physiologic derangement in the 72 hours preceding hospitalization with a Laboratory‐based Acute Physiology Score (LAPS) using 14 laboratory test results prior to hospitalization; we also tested individual laboratory test results obtained after admission to the hospital. We quantified patients' comorbid illness burden using a COmorbidity Point Score (COPS) based on patients' preexisting diagnoses over the 12‐month period preceding hospitalization.4 We extracted temperature, heart rate, respiratory rate, systolic blood pressure, diastolic blood pressure, oxygen saturation, and neurological status from the EMR. We also tested the following variables based on specific information extracted from the EMR: shock index (heart rate divided by systolic blood pressure)13; care directive status (patients were placed into 4 groups: full code, partial code, do not resuscitate [DNR], and no care directive in place); and a proxy for measured lactate (PML; anion gap/serum bicarbonate 100).1416 For comparison purposes, we also created a retrospective electronically assigned MEWS, which we refer to as the MEWS(re), and we assigned this score to patient records electronically using data from KP HealthConnect.
Statistical Methods
Analyses were performed in SAS 9.1, Stata 10, and R 2.12. Final validation was performed using SAS (SAS Institute Inc., Carey, North Carolina). Since we did not limit ourselves to traditional severity‐scoring approaches (eg, selecting the worst heart rate in a given time interval), but also included trend terms (eg, change in heart rate over the 24 hours preceding T0), the number of potential variables to test was very large. Detailed description of the statistical strategies employed for variable selection is provided in the Supporting Information, Appendices, in the online version of this article. Once variables were selected, our basic approach was to test a series of diagnosis‐specific logistic regression submodels using a variety of predictors that included vital signs, vital signs trends (eg, most recent heart rate minus earliest heart rate, heart rate over preceding 24 hours), and other above‐mentioned variables.
We assessed the ability of a submodel to correctly distinguish patients who died, from survivors, using the c‐statistic, as well as other metrics recommended by Cook.17 At the end of the modeling process, we pooled the results across all submodels. For vital signs, where the rate of missing data was <3%, we tested submodels in which we dropped shifts with missing data, as well as submodels in which we imputed missing vital signs to a normal value. For laboratory data, where the rate of missing data for a given shift was much greater, we employed a probabilistic imputation method that included consideration of when a laboratory test result became available.
RESULTS
During the study period, a total of 102,488 patients experienced 145,335 hospitalizations at the study hospitals. We removed 66 patients with 138 hospitalizations for data quality reasons, leaving us with our initial study sample of 102,422 patients whose characteristics are summarized in Table 1. Table 1, in which the unit of analysis is an individual patient, shows that patients who experienced the primary outcome were similar to those patients described in our previous report, in terms of their characteristics on admission as well as in experiencing excess morbidity and mortality.6
Never Admitted to ICU | Direct Admit to ICU From ED | Unplanned Transfer to ICU* | Other ICU Admission | |
---|---|---|---|---|
| ||||
N | 89,269 | 5963 | 2880 | 4310 |
Age (mean SD) | 61.26 18.62 | 62.25 18.13 | 66.12 16.20 | 64.45 15.91 |
Male (n, %) | 37,228 (41.70%) | 3091 (51.84%) | 1416 (49.17%) | 2378 (55.17%) |
LAPS (mean SD) | 13.02 15.79 | 32.72 24.85 | 24.83 21.53 | 11.79 18.16 |
COPS(mean SD) | 67.25 51.42 | 73.88 57.42 | 86.33 59.33 | 78.44 52.49 |
% Predicted mortality risk (mean SD) | 1.93% 3.98% | 7.69% 12.59% | 5.23% 7.70% | 3.66% 6.81% |
Survived first hospitalization to discharge∥ | 88,479 (99.12%) | 5336 (89.49%) | 2316 (80.42%) | 4063 (94.27%) |
Care order on admission | ||||
Full code | 78,877 (88.36%) | 5198 (87.17%) | 2598 (90.21%) | 4097 (95.06%) |
Partial code | 664 (0.74%) | 156 (2.62%) | 50 (1.74%) | 27 (0.63%) |
Comfort care | 21 (0.02%) | 2 (0.03%) | 0 (0%) | 0 (0%) |
DNR | 8227 (9.22%) | 539 (9.04%) | 219 (7.60%) | 161 (3.74%) |
Comfort care and DNR | 229 (0.26%) | 9 (0.15%) | 2 (0.07%) | 2 (0.05%) |
No order | 1251 (1.40%) | 59 (0.99%) | 11 (0.38%) | 23 (0.53%) |
Admission diagnosis (n, %) | ||||
Pneumonia | 2385 (2.67%) | 258 (4.33%) | 242 (8.40%) | 68 (1.58%) |
Sepsis | 5822 (6.52%) | 503 (8.44%) | 279 (9.69%) | 169 (3.92%) |
GI bleeding | 9938 (11.13%) | 616 (10.33%) | 333 (11.56%) | 290 (6.73%) |
Cancer | 2845 (3.19%) | 14 (0.23%) | 95 (3.30%) | 492 (11.42%) |
Total hospital length of stay (days SD) | 3.08 3.29 | 5.37 7.50 | 12.16 13.12 | 8.06 9.53 |
Figure 1shows how we developed the analysis cohort, by removing patients with a comfort‐care‐only order placed within 4 hours after admission (369 patients/744 hospitalizations) and patients who were never admitted to the ward or TCU (7,220/10,574). This left a cohort consisting of 94,833 patients who experienced 133,879 hospitalizations spanning a total of 1,079,062 shifts. We then removed shifts where: 1) a patient was not on the ward at the start of a shift, or was on the ward for <4 hours of a shift; 2) the patient had a comfort‐care order in place at the start of the shift; and 3) the patient died and was ineligible to be a case (the patient had a DNR order in place or died in the ICU). The final cohort eligible for sampling consisted of 846,907 shifts, which involved a total of 92,797 patients and 130,627 hospitalizations. There were a total of 4,036 event shifts, which included 3,224 where a patient was transferred from the ward to the ICU, 717 from the TCU to the ICU, and 95 where a patient died on the ward or TCU without a DNR order in place. We then randomly selected 39,782 comparison shifts. Thus, our final cohort for analysis included 4,036 event shifts (1,979 derivation/2,057 validation and 39,782 comparison shifts (19,509/20,273). As a secondary validation, we also applied model coefficients to the 429 event shifts excluded due to the <4‐hour length‐of‐stay requirement.
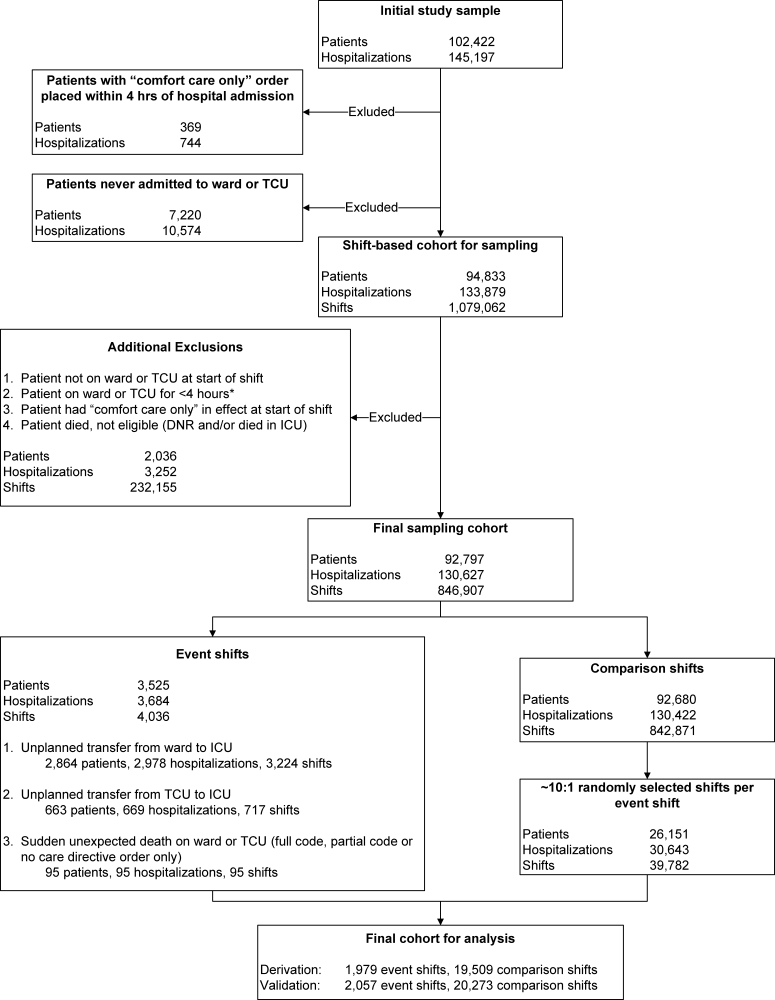
Table 2 compares event shifts with comparison shifts. In the 24 hours preceding ICU transfer, patients who were subsequently transferred had statistically significant, but not necessarily clinically significant, differences in terms of these variables. However, missing laboratory data were more common, ranging from 18% to 31% of all shifts (we did not incorporate laboratory tests where 35% of the shifts had missing data for that test).
Predictor | Event Shifts | Comparison Shifts | P |
---|---|---|---|
| |||
Number | 4036 | 39,782 | |
Age (mean SD) | 67.19 15.25 | 65.41 17.40 | <0.001 |
Male (n, %) | 2007 (49.73%) | 17,709 (44.52%) | <0.001 |
Day shift | 1364 (33.80%) | 17,714 (44.53%) | <0.001 |
LAPS* | 27.89 22.10 | 20.49 20.16 | <0.001 |
COPS | 116.33 72.31 | 100.81 68.44 | <0.001 |
Full code (n, %) | 3496 (86.2%) | 32,156 (80.8%) | <0.001 |
ICU shift during hospitalization | 3964 (98.22%) | 7197 (18.09%) | <0.001 |
Unplanned transfer to ICU during hospitalization∥ | 353 (8.8%) | 1466 (3.7%) | <0.001 |
Temperature (mean SD) | 98.15 (1.13) | 98.10 (0.85) | 0.009 |
Heart rate (mean SD) | 90.30 (20.48) | 79.86 (5.27) | <0.001 |
Respiratory rate (mean SD) | 20.36 (3.70) | 18.87 (1.79) | <0.001 |
Systolic blood pressure (mean SD) | 123.65 (23.26) | 126.21 (19.88) | <0.001 |
Diastolic blood pressure (mean SD) | 68.38 (14.49) | 69.46 (11.95) | <0.001 |
Oxygen saturation (mean SD) | 95.72% (3.00) | 96.47 % (2.26) | <0.001 |
MEWS(re) (mean SD) | 3.64 (2.02) | 2.34 (1.61) | <0.001 |
% <5 | 74.86% | 92.79% | |
% 5 | 25.14% | 7.21% | <0.001 |
Proxy for measured lactate# (mean SD) | 36.85 (28.24) | 28.73 (16.74) | <0.001 |
% Missing in 24 hr before start of shift** | 17.91% | 28.78% | <0.001 |
Blood urea nitrogen (mean SD) | 32.03 (25.39) | 22.72 (18.9) | <0.001 |
% Missing in 24 hr before start of shift | 19.67% | 20.90% | <0.001 |
White blood cell count 1000 (mean SD) | 12.33 (11.42) | 9.83 (6.58) | <0.001 |
% Missing in 24 hr before start of shift | 21.43% | 30.98% | <0.001 |
Hematocrit (mean SD) | 33.08 (6.28) | 33.07 (5.25) | 0.978 |
% Missing in 24 hr before start of shift | 19.87% | 29.55% | <0.001 |
After conducting multiple analyses using the derivation dataset, we developed 24 submodels, a compromise between our finding that primary‐condition‐specific models showed better performance and the fact that we had very few events among patients with certain primary conditions (eg, pericarditis/valvular heart disease), which forced us to create composite categories (eg, a category pooling patients with pericarditis, atherosclerosis, and peripheral vascular disease). Table 3 lists variables included in our final submodels.
Variable | Description |
---|---|
| |
Directive status | Full code or not full code |
LAPS* | Admission physiologic severity of illness score (continuous variable ranging from 0 to 256). Standardized and included as LAPS and LAPS squared |
COPS | Comorbidity burden score (continuous variable ranging from 0 to 701). Standardized and included as COPS and COPS squared. |
COPS status | Indicator for absent comorbidity data |
LOS at T0 | Length of stay in the hospital (total time in hours) at the T0; standardized. |
T0 time of day | 7 AM or 7 PM |
Temperature | Worst (highest) temperature in 24 hr preceding T0; variability in temperature in 24 hr preceding T0. |
Heart rate | Most recent heart rate in 24 hr preceding T0; variability in heart rate in 24 hr preceding T0. |
Respiratory rate | Most recent respiratory rate in 24 hr preceding T0; worst (highest) respiratory rate in 24 hr preceding T0; variability in respiratory rate in 24 hr preceding T0. |
Diastolic blood pressure | Most recent diastolic blood pressure in 24 hr preceding T0 transformed by subtracting 70 from the actual value and squaring the result. Any value above 2000 is subsequently then set to 2000, yielding a continuous variable ranging from 0 to 2000. |
Systolic pressure | Variability in systolic blood pressure in 24 hr preceding T0. |
Pulse oximetry | Worst (lowest) oxygen saturation in 24 hr preceding T0; variability in oxygen saturation in 24 hr preceding T0. |
Neurological status | Most recent neurological status check in 24 hr preceding T0. |
Laboratory tests | Blood urea nitrogen |
Proxy for measured lactate = (anion gap serum bicarbonate) 100 | |
Hematocrit | |
Total white blood cell count |
Table 4 summarizes key results in the validation dataset. Across all diagnoses, the MEWS(re) had c‐statistic of 0.709 (95% confidence interval, 0.6970.721) in the derivation dataset and 0.698 (0.6860.710) in the validation dataset. In the validation dataset, the MEWS(re) performed best among patients with a set of gastrointestinal diagnoses (c = 0.792; 0.7260.857) and worst among patients with congestive heart failure (0.541; 0.5000.620). In contrast, across all primary conditions, the EMR‐based models had a c‐statistic of 0.845 (0.8260.863) in the derivation dataset and 0.775 (0.7530.797) in the validation dataset. In the validation dataset, the EMR‐based models also performed best among patients with a set of gastrointestinal diagnoses (0.841; 0.7830.897) and worst among patients with congestive heart failure (0.683; 0.6100.755). A negative correlation (R = 0.63) was evident between the number of event shifts in a submodel and the drop in the c‐statistic seen in the validation dataset.
No. of Shifts in Validation Dataset | c‐Statistic | |||
---|---|---|---|---|
Diagnoses Group* | Event | Comparison | MEWS(re) | EMR Model |
| ||||
Acute myocardial infarction | 36 | 169 | 0.541 | 0.572 |
Diseases of pulmonary circulation and cardiac dysrhythmias | 40 | 329 | 0.565 | 0.645 |
Seizure disorders | 45 | 497 | 0.594 | 0.647 |
Rule out myocardial infarction | 77 | 727 | 0.602 | 0.648 |
Pneumonia | 163 | 847 | 0.741 | 0.801 |
GI diagnoses, set A | 58 | 942 | 0.755 | 0.803 |
GI diagnoses, set B∥ | 256 | 2,610 | 0.772 | 0.806 |
GI diagnoses, set C | 46 | 520 | 0.792 | 0.841 |
All diagnosis | 2,032 | 20,106 | 0.698 | 0.775 |
We also compared model performance when our datasets were restricted to 1 randomly selected observation per patient; in these analyses, the total number of event shifts was 3,647 and the number of comparison shifts was 29,052. The c‐statistic for the MEWS(re) in the derivation dataset was 0.709 (0.6940.725); in the validation dataset, it was 0.698 (0.6920.714). The corresponding values for the EMR‐based models were 0.856 (0.8350.877) and 0.780 (0.7560.804). We also tested models in which, instead of dropping shifts with missing vital signs, we imputed missing vital signs to their normal value. The c‐statistic for the EMR‐based model with imputed vital sign values was 0.842 (0.8230.861) in the derivation dataset and 0.773 (0.7520.794) in the validation dataset. Lastly, we applied model coefficients to a dataset consisting of 4,290 randomly selected comparison shifts plus the 429 shifts excluded because of the 4‐hour length‐of‐stay criterion. The c‐statistic for this analysis was 0.756 (0.7030.809).
As a general rule, the EMR‐based models were more than twice as efficient as the MEWS(re). For example, a MEWS(re) threshold of 6 as the trigger for an alarm would identify 15% of all transfers to the ICU, with 34.4 false alarms for each transfer; in contrast, using the EMR‐based approach to identify 15% of all transfers, there were 14.5 false alarms for each transfer. Applied to the entire KPMCP Northern California Region, using the MEWS(re), a total of 52 patients per day would need to be evaluated, but only 22 per day using the EMR‐based approach. If one employed a MEWS(re) threshold of 4, this would lead to identification of 44% of all transfers, with a ratio of 69 false alarms for each transfer; using the EMR, the ratio would be 34 to 1. Across the entire KPMCP, a total of 276 patients per day (or about 19.5 a day per hospital) would need to be evaluated using the MEWS(re), but only 136 (or about 9.5 per hospital per day) using the EMR.
DISCUSSION
Using data from a large hospital cohort, we have developed a predictive model suitable for use in non‐ICU populations cared for in integrated healthcare settings with fully automated EMRs. The overall performance of our model, which incorporates acute physiology, diagnosis, and longitudinal data, is superior to the predictive ability of a model that can be assigned manually. This is not surprising, given that scoring systems such as the MEWS make an explicit tradeoff losing information found in multiple variables in exchange for ease of manual assignment. Currently, the model described in this report is being implemented in a simulated environment, a final safety test prior to piloting real‐time provision of probability estimates to clinicians and nurses. Though not yet ready for real‐time use, it is reasonable for our model to be tested using the KPHC shadow server, since evaluation in a simulated environment constitutes a critical evaluation step prior to deployment for clinical use. We also anticipate further refinement and revalidation to occur as more inpatient data become available in the KPMCP and elsewhere.
A number of limitations to our approach must be emphasized. In developing our models, we determined that, while modeling by clinical condition was important, the study outcome was rare for some primary conditions. In these diagnostic groups, which accounted for 12.5% of the event shifts and 10.6% of the comparison shifts, the c‐statistic in the validation dataset was <0.70. Since all 22 KPMCP hospitals are now online and will generate an additional 150,000 adult hospitalizations per year, we expect to be able to correct this problem prior to deployment of these models for clinical use. Having additional data will permit us to improve model discrimination and thus decrease the evaluation‐to‐detection ratio. In future iterations of these models, more experimentation with grouping of International Classification of Diseases (ICD) codes may be required. The problem of grouping ICD codes is not an easy one to resolve, in that diagnoses in the grouping must share common pathophysiology while having a grouping with a sufficient number of adverse events for stable statistical models.
Ideally, it would have been desirable to employ a more objective measure of deterioration, since the decision to transfer a patient to the ICU is discretionary. However, we have found that key data points needed to define such a measure (eg, vital signs) are not consistently charted when a patient deterioratesthis is not surprising outside the research setting, given that nurses and physicians involved in a transfer may be focusing on caring for the patient rather than immediately charting. Given the complexities of end‐of‐life‐care decision‐making, we could not employ death as the outcome of interest. A related issue is that our model does not differentiate between reasons for needing transfer to the ICU, an issue recently discussed by Bapoje et al.18
Our model does not address an important issue raised by Bapoje et al18 and Litvak, Pronovost, and others,19, 20 namely, whether a patient should have been admitted to a non‐ICU setting in the first place. Our team is currently developing a model for doing exactly this (providing decision support for triage in the emergency department), but discussion of this methodology is outside the scope of this article.
Because of resource and data limitations, our model also does not include newborns, children, women admitted for childbirth, or patients transferred from non‐KPMCP hospitals. However, the approach described here could serve as a starting point for developing models for these other populations.
The generalizability of our model must also be considered. The Northern California KPMCP is unusual in having large electronic databases that include physiologic as well as longitudinal patient data. Many hospitals cannot take advantage of all the methods described here. However, the methods we employed could be modified for use by hospital systems in countries such as Great Britain and Canada, and entities such as the Veterans Administration Hospital System in the United States. The KPMCP population, an insured population with few barriers to access, is healthier than the general population, and some population subsets are underrepresented in our cohort. Practice patterns may also vary. Nonetheless, the model described here could serve as a good starting point for future collaborative studies, and it would be possible to develop models suitable for use by stand‐alone hospitals (eg, recalibrating so that one used a Charlson comorbidity21 score based on present on‐admission codes rather than the COPS).
The need for early detection of patient deterioration has played a major role in the development of rapid response teams, as well as scores such as the MEWS. In particular, entities such as the Institute for Healthcare Improvement have advocated the use of early warning systems.22 However, having a statistically robust model to support an early warning system is only part of the solution, and a number of new challenges must then be addressed. The first is actual electronic deployment. Existing inpatient EMRs were not designed with complex calculations in mind, and we anticipate that some degradation in performance will occur when we test our models using real‐time data capture. As Bapoje et al point out, simply having an alert may be insufficient, since not all transfers are preventable.18 Early warning systems also raise ethical issues (for example, what should be done if an alert leads a clinician to confront the fact that an end‐of‐life‐care discussion needs to occur?). From a research perspective, if one were to formally test the benefits of such models, it would be critical to define outcome measures other than death (which is strongly affected by end‐of‐life‐care decisions) or ICU transfer (which is often desirable).
In conclusion, we have developed an approach for predicting impending physiologic deterioration of hospitalized adults outside the ICU. Our approach illustrates how organizations can take maximal advantage of EMRs in a manner that exceeds meaningful use specifications.23, 24 Our study highlights the possibility of using fully automated EMR data for building and applying sophisticated statistical models in settings other than the highly monitored ICU without the need for additional equipment. It also expands the universe of severity scoring to one in which probability estimates are provided in real time and throughout an entire hospitalization. Model performance will undoubtedly improve over time, as more patient data become available. Although our approach has important limitations, it is suitable for testing using real‐time data in a simulated environment. Such testing would permit identification of unanticipated problems and quantification of the degradation of model performance due to real life factors, such as delays in vital signs charting or EMR system brownouts. It could also serve as the springboard for future collaborative studies, with a broader population base, in which the EMR becomes a tool for care, not just documentation.
Acknowledgements
We thank Ms Marla Gardner and Mr John Greene for their work in the development phase of this project. We are grateful to Brian Hoberman, Andrew Hwang, and Marc Flagg from the RIMS group; to Colin Stobbs, Sriram Thiruvenkatachari, and Sundeep Sood from KP IT, Inc; and to Dennis Andaya, Linda Gliner, and Cyndi Vasallo for their assistance with data‐quality audits. We are also grateful to Dr Philip Madvig, Dr Paul Feigenbaum, Dr Alan Whippy, Mr Gregory Adams, Ms Barbara Crawford, and Dr Marybeth Sharpe for their administrative support and encouragement; and to Dr Alan S. Go, Acting Director of the Kaiser Permanente Division of Research, for reviewing the manuscript.
- Day of the week of intensive care admission and patient outcomes: a multisite regional evaluation.Med Care.2002;40(6):530–539. , , , .
- The hospital mortality of patients admitted to the ICU on weekends.Chest.2004;126(4):1292–1298. , , , et al.
- Mortality among patients admitted to intensive care units during weekday day shifts compared with “off” hours.Crit Care Med.2007;35(1):3–11. , , , et al.
- Risk adjusting hospital inpatient mortality using automated inpatient, outpatient, and laboratory databases.Med Care.2008;46(3):232–239. , , , , , .
- The Kaiser Permanente inpatient risk adjustment methodology was valid in an external patient population.J Clin Epidemiol.2010;63(7):798–803. , , , .
- Intra‐hospital transfers to a higher level of care: contribution to total hospital and intensive care unit (ICU) mortality and length of stay (LOS).J Hosp Med.2011;6(2):74–80. , , , , , .
- Multicentric study of monitoring alarms in the adult intensive care unit (ICU): a descriptive analysis.Intensive Care Med.1999;25(12):1360–1366. , , , , , .
- Integration of early physiological responses predicts later illness severity in preterm infants.Sci Transl Med.2010;2(48):48ra65. , , , , .
- Reproducibility of physiological track‐and‐trigger warning systems for identifying at‐risk patients on the ward.Intensive Care Med.2007;33(4):619–624. , , .
- Validation of a Modified Early Warning Score in medical admissions.Q J Med.2001;94:521–526. , , , .
- Effect of introducing the Modified Early Warning score on clinical outcomes, cardio‐pulmonary arrests and intensive care utilisation in acute medical admissions.Anaesthesia.2003;58(8):797–802. , , , , .
- MERIT Study Investigators.Introduction of the medical emergency team (MET) system: a cluster‐randomized controlled trial.Lancet.2005;365(9477):2091–2097.
- Unplanned transfers to the intensive care unit: the role of the shock index.J Hosp Med.2010;5(8):460–465. , , , , , .
- The delta (delta) gap: an approach to mixed acid‐base disorders.Ann Emerg Med.1990;19(11):1310–1313. .
- Acid‐base disorders: classification and management strategies.Am Fam Physician.1995;52(2):584–590. .
- Unmeasured anions in critically ill patients: can they predict mortality?Crit Care Med.2003;31(8):2131–2136. , , , .
- Use and misuse of the receiver operating characteristic curve in risk prediction.Circulation.2007;115(7):928–935. .
- Unplanned transfers to a medical intensive care unit: causes and relationship to preventable errors in care.J Hosp Med.2011;6(2):68–72. , , , .
- Rethinking rapid response teams.JAMA.2010;304(12):1375–1376. , .
- Rapid response teams—walk, don't run.JAMA.2006;296(13):1645–1647. , , .
- A new method of classifying prognostic comorbidity in longitudinal populations: development and validation.J Chronic Dis.1987;40:373–383. , , , .
- Institute for Healthcare Improvement.Early Warning Systems:The Next Level of Rapid Response.2011. http://www.ihi.org/IHI/Programs/AudioAndWebPrograms/ExpeditionEarlyWarningSystemsTheNextLevelofRapidResponse.htm?player=wmp. Accessed 4/6/11.
- Assessing readiness for meeting meaningful use: identifying electronic health record functionality and measuring levels of adoption.AMIA Annu Symp Proc.2010;2010:66–70. .
- Medicare and Medicaid Programs;Electronic Health Record Incentive Program. Final Rule.Fed Reg.2010;75(144):44313–44588.
Patients in general medicalsurgical wards who experience unplanned transfer to the intensive care unit (ICU) have increased mortality and morbidity.13 Using an externally validated methodology permitting assessment of illness severity and mortality risk among all hospitalized patients,4, 5 we recently documented observed‐to‐expected mortality ratios >3.0 and excess length of stay of 10 days among patients who experienced such transfers.6
It is possible to predict adverse outcomes among monitored patients (eg, patients in the ICU or undergoing continuous electronic monitoring).7, 8 However, prediction of unplanned transfers among medicalsurgical ward patients presents challenges. Data collection (vital signs and laboratory tests) is relatively infrequent. The event rate (3% of hospital admissions) is low, and the rate in narrow time periods (eg, 12 hours) is extremely low: a hospital with 4000 admissions per year might experience 1 unplanned transfer to the ICU every 3 days. Not surprisingly, performance of models suitable for predicting ward patients' need for intensive care within narrow time frames have been disappointing.9 The Modified Early Warning Score (MEWS), has a c‐statistic, or area under the receiver operator characteristic of 0.67,1012 and our own model incorporating 14 laboratory tests, but no vital signs, has excellent performance with respect to predicting inpatient mortality, but poor performance with respect to unplanned transfer.6
In this report, we describe the development and validation of a complex predictive model suitable for use with ward patients. Our objective for this work was to develop a predictive model based on clinical and physiologic data available in real time from a comprehensive electronic medical record (EMR), not a clinically intuitive, manually assigned tool. The outcome of interest was unplanned transfer from the ward to the ICU, or death on the ward in a patient who was full code. This model has been developed as part of a regional effort to decrease preventable mortality in the Northern California Kaiser Permanente Medical Care Program (KPMCP), an integrated healthcare delivery system with 22 hospitals.
MATERIALS AND METHODS
For additional details, see the Supporting Information, Appendices 112, in the online version of this article.
This project was approved by the KPMCP Institutional Board for the Protection of Human Subjects.
The Northern California KPMCP serves a total population of approximately 3.3 million members. All Northern California KPMCP hospitals and clinics employ the same information systems with a common medical record number and can track care covered by the plan but delivered elsewhere. Databases maintained by the KPMCP capture admission and discharge times, admission and discharge diagnoses and procedures (assigned by professional coders), bed histories permitting quantification of intra‐hospital transfers, inter‐hospital transfers, as well as the results of all inpatient and outpatient laboratory tests. In July 2006, the KPMCP began deployment of the EMR developed by Epic Systems Corporation (
Our setting consisted of 14 hospitals in which the KPHC inpatient EMR had been running for at least 3 months (the KPMCP Antioch, Fremont, Hayward, Manteca, Modesto, Roseville, Sacramento, Santa Clara, San Francisco, Santa Rosa, South Sacramento, South San Francisco, Santa Teresa, and Walnut Creek hospitals). We have described the general characteristics of KPMCP hospitals elsewhere.4, 6 Our initial study population consisted of all patients admitted to these hospitals who met the following criteria: hospitalization began from November 1, 2006 through December 31, 2009; initial hospitalization occurred at a Northern California KPMCP hospital (ie, for inter‐hospital transfers, the first hospital stay occurred within the KPMCP); age 18 years; hospitalization was not for childbirth; and KPHC had been operational at the hospital for at least 3 months.
Analytic Approach
The primary outcome for this study was transfer to the ICU after admission to the hospital among patients residing either in a general medicalsurgical ward (ward) or transitional care unit (TCU), or death in the ward or TCU in a patient who was full code at the time of death (ie, had the patient survived, s/he would have been transferred to the ICU). The unit of analysis for this study was a 12‐hour patient shift, which could begin with a 7 AM T0 (henceforth, day shift) or a 7 PM T0 (night shift); in other words, we aimed to predict the occurrence of an event within 12 hours of T0 using only data available prior to T0. A shift in which a patient experienced the primary study outcome is an event shift, while one in which a patient did not experience the primary outcome is a comparison shift. Using this approach, an individual patient record could consist of both event and comparison shifts, since some patients might have multiple unplanned transfers and some patients might have none. Our basic analytic approach consisted of creating a cohort of event and comparison shifts (10 comparison shifts were randomly selected for each event shift), splitting the cohort into a derivation dataset (50%) and validation dataset (50%), developing a model using the derivation dataset, then applying the coefficients of the derivation dataset to the validation dataset. Because some event shifts were excluded due to the minimum 4‐hour length‐of‐stay requirement, we also applied model coefficients to these excluded shifts and a set of randomly selected comparison shifts.
Since the purpose of these analyses was to develop models with maximal signal extraction from sparsely collected predictors, we did not block a time period after the T0 to allow for a reaction time to the alarm. Thus, since some events could occur immediately after the T0 (as can be seen in the Supporting Information, Appendices, in the online version of this article), our models would need to be run at intervals that are more frequent than 2 times a day.
Independent Variables
In addition to patients' age and sex, we tested the following candidate independent variables. Some of these variables are part of the KPMCP risk adjustment model4, 5 and were available electronically for all patients in the cohort. We grouped admission diagnoses into 44 broad diagnostic categories (primary conditions), and admission types into 4 groups (emergency medical, emergency surgical, elective medical, and elective surgical). We quantified patients' degree of physiologic derangement in the 72 hours preceding hospitalization with a Laboratory‐based Acute Physiology Score (LAPS) using 14 laboratory test results prior to hospitalization; we also tested individual laboratory test results obtained after admission to the hospital. We quantified patients' comorbid illness burden using a COmorbidity Point Score (COPS) based on patients' preexisting diagnoses over the 12‐month period preceding hospitalization.4 We extracted temperature, heart rate, respiratory rate, systolic blood pressure, diastolic blood pressure, oxygen saturation, and neurological status from the EMR. We also tested the following variables based on specific information extracted from the EMR: shock index (heart rate divided by systolic blood pressure)13; care directive status (patients were placed into 4 groups: full code, partial code, do not resuscitate [DNR], and no care directive in place); and a proxy for measured lactate (PML; anion gap/serum bicarbonate 100).1416 For comparison purposes, we also created a retrospective electronically assigned MEWS, which we refer to as the MEWS(re), and we assigned this score to patient records electronically using data from KP HealthConnect.
Statistical Methods
Analyses were performed in SAS 9.1, Stata 10, and R 2.12. Final validation was performed using SAS (SAS Institute Inc., Carey, North Carolina). Since we did not limit ourselves to traditional severity‐scoring approaches (eg, selecting the worst heart rate in a given time interval), but also included trend terms (eg, change in heart rate over the 24 hours preceding T0), the number of potential variables to test was very large. Detailed description of the statistical strategies employed for variable selection is provided in the Supporting Information, Appendices, in the online version of this article. Once variables were selected, our basic approach was to test a series of diagnosis‐specific logistic regression submodels using a variety of predictors that included vital signs, vital signs trends (eg, most recent heart rate minus earliest heart rate, heart rate over preceding 24 hours), and other above‐mentioned variables.
We assessed the ability of a submodel to correctly distinguish patients who died, from survivors, using the c‐statistic, as well as other metrics recommended by Cook.17 At the end of the modeling process, we pooled the results across all submodels. For vital signs, where the rate of missing data was <3%, we tested submodels in which we dropped shifts with missing data, as well as submodels in which we imputed missing vital signs to a normal value. For laboratory data, where the rate of missing data for a given shift was much greater, we employed a probabilistic imputation method that included consideration of when a laboratory test result became available.
RESULTS
During the study period, a total of 102,488 patients experienced 145,335 hospitalizations at the study hospitals. We removed 66 patients with 138 hospitalizations for data quality reasons, leaving us with our initial study sample of 102,422 patients whose characteristics are summarized in Table 1. Table 1, in which the unit of analysis is an individual patient, shows that patients who experienced the primary outcome were similar to those patients described in our previous report, in terms of their characteristics on admission as well as in experiencing excess morbidity and mortality.6
Never Admitted to ICU | Direct Admit to ICU From ED | Unplanned Transfer to ICU* | Other ICU Admission | |
---|---|---|---|---|
| ||||
N | 89,269 | 5963 | 2880 | 4310 |
Age (mean SD) | 61.26 18.62 | 62.25 18.13 | 66.12 16.20 | 64.45 15.91 |
Male (n, %) | 37,228 (41.70%) | 3091 (51.84%) | 1416 (49.17%) | 2378 (55.17%) |
LAPS (mean SD) | 13.02 15.79 | 32.72 24.85 | 24.83 21.53 | 11.79 18.16 |
COPS(mean SD) | 67.25 51.42 | 73.88 57.42 | 86.33 59.33 | 78.44 52.49 |
% Predicted mortality risk (mean SD) | 1.93% 3.98% | 7.69% 12.59% | 5.23% 7.70% | 3.66% 6.81% |
Survived first hospitalization to discharge∥ | 88,479 (99.12%) | 5336 (89.49%) | 2316 (80.42%) | 4063 (94.27%) |
Care order on admission | ||||
Full code | 78,877 (88.36%) | 5198 (87.17%) | 2598 (90.21%) | 4097 (95.06%) |
Partial code | 664 (0.74%) | 156 (2.62%) | 50 (1.74%) | 27 (0.63%) |
Comfort care | 21 (0.02%) | 2 (0.03%) | 0 (0%) | 0 (0%) |
DNR | 8227 (9.22%) | 539 (9.04%) | 219 (7.60%) | 161 (3.74%) |
Comfort care and DNR | 229 (0.26%) | 9 (0.15%) | 2 (0.07%) | 2 (0.05%) |
No order | 1251 (1.40%) | 59 (0.99%) | 11 (0.38%) | 23 (0.53%) |
Admission diagnosis (n, %) | ||||
Pneumonia | 2385 (2.67%) | 258 (4.33%) | 242 (8.40%) | 68 (1.58%) |
Sepsis | 5822 (6.52%) | 503 (8.44%) | 279 (9.69%) | 169 (3.92%) |
GI bleeding | 9938 (11.13%) | 616 (10.33%) | 333 (11.56%) | 290 (6.73%) |
Cancer | 2845 (3.19%) | 14 (0.23%) | 95 (3.30%) | 492 (11.42%) |
Total hospital length of stay (days SD) | 3.08 3.29 | 5.37 7.50 | 12.16 13.12 | 8.06 9.53 |
Figure 1shows how we developed the analysis cohort, by removing patients with a comfort‐care‐only order placed within 4 hours after admission (369 patients/744 hospitalizations) and patients who were never admitted to the ward or TCU (7,220/10,574). This left a cohort consisting of 94,833 patients who experienced 133,879 hospitalizations spanning a total of 1,079,062 shifts. We then removed shifts where: 1) a patient was not on the ward at the start of a shift, or was on the ward for <4 hours of a shift; 2) the patient had a comfort‐care order in place at the start of the shift; and 3) the patient died and was ineligible to be a case (the patient had a DNR order in place or died in the ICU). The final cohort eligible for sampling consisted of 846,907 shifts, which involved a total of 92,797 patients and 130,627 hospitalizations. There were a total of 4,036 event shifts, which included 3,224 where a patient was transferred from the ward to the ICU, 717 from the TCU to the ICU, and 95 where a patient died on the ward or TCU without a DNR order in place. We then randomly selected 39,782 comparison shifts. Thus, our final cohort for analysis included 4,036 event shifts (1,979 derivation/2,057 validation and 39,782 comparison shifts (19,509/20,273). As a secondary validation, we also applied model coefficients to the 429 event shifts excluded due to the <4‐hour length‐of‐stay requirement.
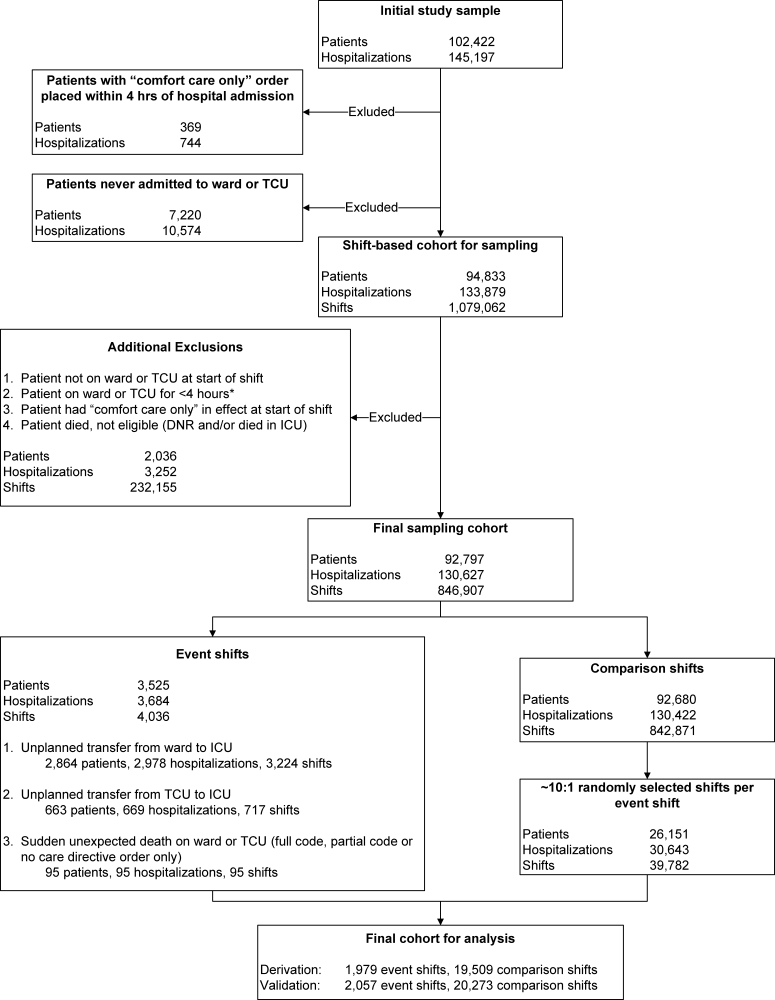
Table 2 compares event shifts with comparison shifts. In the 24 hours preceding ICU transfer, patients who were subsequently transferred had statistically significant, but not necessarily clinically significant, differences in terms of these variables. However, missing laboratory data were more common, ranging from 18% to 31% of all shifts (we did not incorporate laboratory tests where 35% of the shifts had missing data for that test).
Predictor | Event Shifts | Comparison Shifts | P |
---|---|---|---|
| |||
Number | 4036 | 39,782 | |
Age (mean SD) | 67.19 15.25 | 65.41 17.40 | <0.001 |
Male (n, %) | 2007 (49.73%) | 17,709 (44.52%) | <0.001 |
Day shift | 1364 (33.80%) | 17,714 (44.53%) | <0.001 |
LAPS* | 27.89 22.10 | 20.49 20.16 | <0.001 |
COPS | 116.33 72.31 | 100.81 68.44 | <0.001 |
Full code (n, %) | 3496 (86.2%) | 32,156 (80.8%) | <0.001 |
ICU shift during hospitalization | 3964 (98.22%) | 7197 (18.09%) | <0.001 |
Unplanned transfer to ICU during hospitalization∥ | 353 (8.8%) | 1466 (3.7%) | <0.001 |
Temperature (mean SD) | 98.15 (1.13) | 98.10 (0.85) | 0.009 |
Heart rate (mean SD) | 90.30 (20.48) | 79.86 (5.27) | <0.001 |
Respiratory rate (mean SD) | 20.36 (3.70) | 18.87 (1.79) | <0.001 |
Systolic blood pressure (mean SD) | 123.65 (23.26) | 126.21 (19.88) | <0.001 |
Diastolic blood pressure (mean SD) | 68.38 (14.49) | 69.46 (11.95) | <0.001 |
Oxygen saturation (mean SD) | 95.72% (3.00) | 96.47 % (2.26) | <0.001 |
MEWS(re) (mean SD) | 3.64 (2.02) | 2.34 (1.61) | <0.001 |
% <5 | 74.86% | 92.79% | |
% 5 | 25.14% | 7.21% | <0.001 |
Proxy for measured lactate# (mean SD) | 36.85 (28.24) | 28.73 (16.74) | <0.001 |
% Missing in 24 hr before start of shift** | 17.91% | 28.78% | <0.001 |
Blood urea nitrogen (mean SD) | 32.03 (25.39) | 22.72 (18.9) | <0.001 |
% Missing in 24 hr before start of shift | 19.67% | 20.90% | <0.001 |
White blood cell count 1000 (mean SD) | 12.33 (11.42) | 9.83 (6.58) | <0.001 |
% Missing in 24 hr before start of shift | 21.43% | 30.98% | <0.001 |
Hematocrit (mean SD) | 33.08 (6.28) | 33.07 (5.25) | 0.978 |
% Missing in 24 hr before start of shift | 19.87% | 29.55% | <0.001 |
After conducting multiple analyses using the derivation dataset, we developed 24 submodels, a compromise between our finding that primary‐condition‐specific models showed better performance and the fact that we had very few events among patients with certain primary conditions (eg, pericarditis/valvular heart disease), which forced us to create composite categories (eg, a category pooling patients with pericarditis, atherosclerosis, and peripheral vascular disease). Table 3 lists variables included in our final submodels.
Variable | Description |
---|---|
| |
Directive status | Full code or not full code |
LAPS* | Admission physiologic severity of illness score (continuous variable ranging from 0 to 256). Standardized and included as LAPS and LAPS squared |
COPS | Comorbidity burden score (continuous variable ranging from 0 to 701). Standardized and included as COPS and COPS squared. |
COPS status | Indicator for absent comorbidity data |
LOS at T0 | Length of stay in the hospital (total time in hours) at the T0; standardized. |
T0 time of day | 7 AM or 7 PM |
Temperature | Worst (highest) temperature in 24 hr preceding T0; variability in temperature in 24 hr preceding T0. |
Heart rate | Most recent heart rate in 24 hr preceding T0; variability in heart rate in 24 hr preceding T0. |
Respiratory rate | Most recent respiratory rate in 24 hr preceding T0; worst (highest) respiratory rate in 24 hr preceding T0; variability in respiratory rate in 24 hr preceding T0. |
Diastolic blood pressure | Most recent diastolic blood pressure in 24 hr preceding T0 transformed by subtracting 70 from the actual value and squaring the result. Any value above 2000 is subsequently then set to 2000, yielding a continuous variable ranging from 0 to 2000. |
Systolic pressure | Variability in systolic blood pressure in 24 hr preceding T0. |
Pulse oximetry | Worst (lowest) oxygen saturation in 24 hr preceding T0; variability in oxygen saturation in 24 hr preceding T0. |
Neurological status | Most recent neurological status check in 24 hr preceding T0. |
Laboratory tests | Blood urea nitrogen |
Proxy for measured lactate = (anion gap serum bicarbonate) 100 | |
Hematocrit | |
Total white blood cell count |
Table 4 summarizes key results in the validation dataset. Across all diagnoses, the MEWS(re) had c‐statistic of 0.709 (95% confidence interval, 0.6970.721) in the derivation dataset and 0.698 (0.6860.710) in the validation dataset. In the validation dataset, the MEWS(re) performed best among patients with a set of gastrointestinal diagnoses (c = 0.792; 0.7260.857) and worst among patients with congestive heart failure (0.541; 0.5000.620). In contrast, across all primary conditions, the EMR‐based models had a c‐statistic of 0.845 (0.8260.863) in the derivation dataset and 0.775 (0.7530.797) in the validation dataset. In the validation dataset, the EMR‐based models also performed best among patients with a set of gastrointestinal diagnoses (0.841; 0.7830.897) and worst among patients with congestive heart failure (0.683; 0.6100.755). A negative correlation (R = 0.63) was evident between the number of event shifts in a submodel and the drop in the c‐statistic seen in the validation dataset.
No. of Shifts in Validation Dataset | c‐Statistic | |||
---|---|---|---|---|
Diagnoses Group* | Event | Comparison | MEWS(re) | EMR Model |
| ||||
Acute myocardial infarction | 36 | 169 | 0.541 | 0.572 |
Diseases of pulmonary circulation and cardiac dysrhythmias | 40 | 329 | 0.565 | 0.645 |
Seizure disorders | 45 | 497 | 0.594 | 0.647 |
Rule out myocardial infarction | 77 | 727 | 0.602 | 0.648 |
Pneumonia | 163 | 847 | 0.741 | 0.801 |
GI diagnoses, set A | 58 | 942 | 0.755 | 0.803 |
GI diagnoses, set B∥ | 256 | 2,610 | 0.772 | 0.806 |
GI diagnoses, set C | 46 | 520 | 0.792 | 0.841 |
All diagnosis | 2,032 | 20,106 | 0.698 | 0.775 |
We also compared model performance when our datasets were restricted to 1 randomly selected observation per patient; in these analyses, the total number of event shifts was 3,647 and the number of comparison shifts was 29,052. The c‐statistic for the MEWS(re) in the derivation dataset was 0.709 (0.6940.725); in the validation dataset, it was 0.698 (0.6920.714). The corresponding values for the EMR‐based models were 0.856 (0.8350.877) and 0.780 (0.7560.804). We also tested models in which, instead of dropping shifts with missing vital signs, we imputed missing vital signs to their normal value. The c‐statistic for the EMR‐based model with imputed vital sign values was 0.842 (0.8230.861) in the derivation dataset and 0.773 (0.7520.794) in the validation dataset. Lastly, we applied model coefficients to a dataset consisting of 4,290 randomly selected comparison shifts plus the 429 shifts excluded because of the 4‐hour length‐of‐stay criterion. The c‐statistic for this analysis was 0.756 (0.7030.809).
As a general rule, the EMR‐based models were more than twice as efficient as the MEWS(re). For example, a MEWS(re) threshold of 6 as the trigger for an alarm would identify 15% of all transfers to the ICU, with 34.4 false alarms for each transfer; in contrast, using the EMR‐based approach to identify 15% of all transfers, there were 14.5 false alarms for each transfer. Applied to the entire KPMCP Northern California Region, using the MEWS(re), a total of 52 patients per day would need to be evaluated, but only 22 per day using the EMR‐based approach. If one employed a MEWS(re) threshold of 4, this would lead to identification of 44% of all transfers, with a ratio of 69 false alarms for each transfer; using the EMR, the ratio would be 34 to 1. Across the entire KPMCP, a total of 276 patients per day (or about 19.5 a day per hospital) would need to be evaluated using the MEWS(re), but only 136 (or about 9.5 per hospital per day) using the EMR.
DISCUSSION
Using data from a large hospital cohort, we have developed a predictive model suitable for use in non‐ICU populations cared for in integrated healthcare settings with fully automated EMRs. The overall performance of our model, which incorporates acute physiology, diagnosis, and longitudinal data, is superior to the predictive ability of a model that can be assigned manually. This is not surprising, given that scoring systems such as the MEWS make an explicit tradeoff losing information found in multiple variables in exchange for ease of manual assignment. Currently, the model described in this report is being implemented in a simulated environment, a final safety test prior to piloting real‐time provision of probability estimates to clinicians and nurses. Though not yet ready for real‐time use, it is reasonable for our model to be tested using the KPHC shadow server, since evaluation in a simulated environment constitutes a critical evaluation step prior to deployment for clinical use. We also anticipate further refinement and revalidation to occur as more inpatient data become available in the KPMCP and elsewhere.
A number of limitations to our approach must be emphasized. In developing our models, we determined that, while modeling by clinical condition was important, the study outcome was rare for some primary conditions. In these diagnostic groups, which accounted for 12.5% of the event shifts and 10.6% of the comparison shifts, the c‐statistic in the validation dataset was <0.70. Since all 22 KPMCP hospitals are now online and will generate an additional 150,000 adult hospitalizations per year, we expect to be able to correct this problem prior to deployment of these models for clinical use. Having additional data will permit us to improve model discrimination and thus decrease the evaluation‐to‐detection ratio. In future iterations of these models, more experimentation with grouping of International Classification of Diseases (ICD) codes may be required. The problem of grouping ICD codes is not an easy one to resolve, in that diagnoses in the grouping must share common pathophysiology while having a grouping with a sufficient number of adverse events for stable statistical models.
Ideally, it would have been desirable to employ a more objective measure of deterioration, since the decision to transfer a patient to the ICU is discretionary. However, we have found that key data points needed to define such a measure (eg, vital signs) are not consistently charted when a patient deterioratesthis is not surprising outside the research setting, given that nurses and physicians involved in a transfer may be focusing on caring for the patient rather than immediately charting. Given the complexities of end‐of‐life‐care decision‐making, we could not employ death as the outcome of interest. A related issue is that our model does not differentiate between reasons for needing transfer to the ICU, an issue recently discussed by Bapoje et al.18
Our model does not address an important issue raised by Bapoje et al18 and Litvak, Pronovost, and others,19, 20 namely, whether a patient should have been admitted to a non‐ICU setting in the first place. Our team is currently developing a model for doing exactly this (providing decision support for triage in the emergency department), but discussion of this methodology is outside the scope of this article.
Because of resource and data limitations, our model also does not include newborns, children, women admitted for childbirth, or patients transferred from non‐KPMCP hospitals. However, the approach described here could serve as a starting point for developing models for these other populations.
The generalizability of our model must also be considered. The Northern California KPMCP is unusual in having large electronic databases that include physiologic as well as longitudinal patient data. Many hospitals cannot take advantage of all the methods described here. However, the methods we employed could be modified for use by hospital systems in countries such as Great Britain and Canada, and entities such as the Veterans Administration Hospital System in the United States. The KPMCP population, an insured population with few barriers to access, is healthier than the general population, and some population subsets are underrepresented in our cohort. Practice patterns may also vary. Nonetheless, the model described here could serve as a good starting point for future collaborative studies, and it would be possible to develop models suitable for use by stand‐alone hospitals (eg, recalibrating so that one used a Charlson comorbidity21 score based on present on‐admission codes rather than the COPS).
The need for early detection of patient deterioration has played a major role in the development of rapid response teams, as well as scores such as the MEWS. In particular, entities such as the Institute for Healthcare Improvement have advocated the use of early warning systems.22 However, having a statistically robust model to support an early warning system is only part of the solution, and a number of new challenges must then be addressed. The first is actual electronic deployment. Existing inpatient EMRs were not designed with complex calculations in mind, and we anticipate that some degradation in performance will occur when we test our models using real‐time data capture. As Bapoje et al point out, simply having an alert may be insufficient, since not all transfers are preventable.18 Early warning systems also raise ethical issues (for example, what should be done if an alert leads a clinician to confront the fact that an end‐of‐life‐care discussion needs to occur?). From a research perspective, if one were to formally test the benefits of such models, it would be critical to define outcome measures other than death (which is strongly affected by end‐of‐life‐care decisions) or ICU transfer (which is often desirable).
In conclusion, we have developed an approach for predicting impending physiologic deterioration of hospitalized adults outside the ICU. Our approach illustrates how organizations can take maximal advantage of EMRs in a manner that exceeds meaningful use specifications.23, 24 Our study highlights the possibility of using fully automated EMR data for building and applying sophisticated statistical models in settings other than the highly monitored ICU without the need for additional equipment. It also expands the universe of severity scoring to one in which probability estimates are provided in real time and throughout an entire hospitalization. Model performance will undoubtedly improve over time, as more patient data become available. Although our approach has important limitations, it is suitable for testing using real‐time data in a simulated environment. Such testing would permit identification of unanticipated problems and quantification of the degradation of model performance due to real life factors, such as delays in vital signs charting or EMR system brownouts. It could also serve as the springboard for future collaborative studies, with a broader population base, in which the EMR becomes a tool for care, not just documentation.
Acknowledgements
We thank Ms Marla Gardner and Mr John Greene for their work in the development phase of this project. We are grateful to Brian Hoberman, Andrew Hwang, and Marc Flagg from the RIMS group; to Colin Stobbs, Sriram Thiruvenkatachari, and Sundeep Sood from KP IT, Inc; and to Dennis Andaya, Linda Gliner, and Cyndi Vasallo for their assistance with data‐quality audits. We are also grateful to Dr Philip Madvig, Dr Paul Feigenbaum, Dr Alan Whippy, Mr Gregory Adams, Ms Barbara Crawford, and Dr Marybeth Sharpe for their administrative support and encouragement; and to Dr Alan S. Go, Acting Director of the Kaiser Permanente Division of Research, for reviewing the manuscript.
Patients in general medicalsurgical wards who experience unplanned transfer to the intensive care unit (ICU) have increased mortality and morbidity.13 Using an externally validated methodology permitting assessment of illness severity and mortality risk among all hospitalized patients,4, 5 we recently documented observed‐to‐expected mortality ratios >3.0 and excess length of stay of 10 days among patients who experienced such transfers.6
It is possible to predict adverse outcomes among monitored patients (eg, patients in the ICU or undergoing continuous electronic monitoring).7, 8 However, prediction of unplanned transfers among medicalsurgical ward patients presents challenges. Data collection (vital signs and laboratory tests) is relatively infrequent. The event rate (3% of hospital admissions) is low, and the rate in narrow time periods (eg, 12 hours) is extremely low: a hospital with 4000 admissions per year might experience 1 unplanned transfer to the ICU every 3 days. Not surprisingly, performance of models suitable for predicting ward patients' need for intensive care within narrow time frames have been disappointing.9 The Modified Early Warning Score (MEWS), has a c‐statistic, or area under the receiver operator characteristic of 0.67,1012 and our own model incorporating 14 laboratory tests, but no vital signs, has excellent performance with respect to predicting inpatient mortality, but poor performance with respect to unplanned transfer.6
In this report, we describe the development and validation of a complex predictive model suitable for use with ward patients. Our objective for this work was to develop a predictive model based on clinical and physiologic data available in real time from a comprehensive electronic medical record (EMR), not a clinically intuitive, manually assigned tool. The outcome of interest was unplanned transfer from the ward to the ICU, or death on the ward in a patient who was full code. This model has been developed as part of a regional effort to decrease preventable mortality in the Northern California Kaiser Permanente Medical Care Program (KPMCP), an integrated healthcare delivery system with 22 hospitals.
MATERIALS AND METHODS
For additional details, see the Supporting Information, Appendices 112, in the online version of this article.
This project was approved by the KPMCP Institutional Board for the Protection of Human Subjects.
The Northern California KPMCP serves a total population of approximately 3.3 million members. All Northern California KPMCP hospitals and clinics employ the same information systems with a common medical record number and can track care covered by the plan but delivered elsewhere. Databases maintained by the KPMCP capture admission and discharge times, admission and discharge diagnoses and procedures (assigned by professional coders), bed histories permitting quantification of intra‐hospital transfers, inter‐hospital transfers, as well as the results of all inpatient and outpatient laboratory tests. In July 2006, the KPMCP began deployment of the EMR developed by Epic Systems Corporation (
Our setting consisted of 14 hospitals in which the KPHC inpatient EMR had been running for at least 3 months (the KPMCP Antioch, Fremont, Hayward, Manteca, Modesto, Roseville, Sacramento, Santa Clara, San Francisco, Santa Rosa, South Sacramento, South San Francisco, Santa Teresa, and Walnut Creek hospitals). We have described the general characteristics of KPMCP hospitals elsewhere.4, 6 Our initial study population consisted of all patients admitted to these hospitals who met the following criteria: hospitalization began from November 1, 2006 through December 31, 2009; initial hospitalization occurred at a Northern California KPMCP hospital (ie, for inter‐hospital transfers, the first hospital stay occurred within the KPMCP); age 18 years; hospitalization was not for childbirth; and KPHC had been operational at the hospital for at least 3 months.
Analytic Approach
The primary outcome for this study was transfer to the ICU after admission to the hospital among patients residing either in a general medicalsurgical ward (ward) or transitional care unit (TCU), or death in the ward or TCU in a patient who was full code at the time of death (ie, had the patient survived, s/he would have been transferred to the ICU). The unit of analysis for this study was a 12‐hour patient shift, which could begin with a 7 AM T0 (henceforth, day shift) or a 7 PM T0 (night shift); in other words, we aimed to predict the occurrence of an event within 12 hours of T0 using only data available prior to T0. A shift in which a patient experienced the primary study outcome is an event shift, while one in which a patient did not experience the primary outcome is a comparison shift. Using this approach, an individual patient record could consist of both event and comparison shifts, since some patients might have multiple unplanned transfers and some patients might have none. Our basic analytic approach consisted of creating a cohort of event and comparison shifts (10 comparison shifts were randomly selected for each event shift), splitting the cohort into a derivation dataset (50%) and validation dataset (50%), developing a model using the derivation dataset, then applying the coefficients of the derivation dataset to the validation dataset. Because some event shifts were excluded due to the minimum 4‐hour length‐of‐stay requirement, we also applied model coefficients to these excluded shifts and a set of randomly selected comparison shifts.
Since the purpose of these analyses was to develop models with maximal signal extraction from sparsely collected predictors, we did not block a time period after the T0 to allow for a reaction time to the alarm. Thus, since some events could occur immediately after the T0 (as can be seen in the Supporting Information, Appendices, in the online version of this article), our models would need to be run at intervals that are more frequent than 2 times a day.
Independent Variables
In addition to patients' age and sex, we tested the following candidate independent variables. Some of these variables are part of the KPMCP risk adjustment model4, 5 and were available electronically for all patients in the cohort. We grouped admission diagnoses into 44 broad diagnostic categories (primary conditions), and admission types into 4 groups (emergency medical, emergency surgical, elective medical, and elective surgical). We quantified patients' degree of physiologic derangement in the 72 hours preceding hospitalization with a Laboratory‐based Acute Physiology Score (LAPS) using 14 laboratory test results prior to hospitalization; we also tested individual laboratory test results obtained after admission to the hospital. We quantified patients' comorbid illness burden using a COmorbidity Point Score (COPS) based on patients' preexisting diagnoses over the 12‐month period preceding hospitalization.4 We extracted temperature, heart rate, respiratory rate, systolic blood pressure, diastolic blood pressure, oxygen saturation, and neurological status from the EMR. We also tested the following variables based on specific information extracted from the EMR: shock index (heart rate divided by systolic blood pressure)13; care directive status (patients were placed into 4 groups: full code, partial code, do not resuscitate [DNR], and no care directive in place); and a proxy for measured lactate (PML; anion gap/serum bicarbonate 100).1416 For comparison purposes, we also created a retrospective electronically assigned MEWS, which we refer to as the MEWS(re), and we assigned this score to patient records electronically using data from KP HealthConnect.
Statistical Methods
Analyses were performed in SAS 9.1, Stata 10, and R 2.12. Final validation was performed using SAS (SAS Institute Inc., Carey, North Carolina). Since we did not limit ourselves to traditional severity‐scoring approaches (eg, selecting the worst heart rate in a given time interval), but also included trend terms (eg, change in heart rate over the 24 hours preceding T0), the number of potential variables to test was very large. Detailed description of the statistical strategies employed for variable selection is provided in the Supporting Information, Appendices, in the online version of this article. Once variables were selected, our basic approach was to test a series of diagnosis‐specific logistic regression submodels using a variety of predictors that included vital signs, vital signs trends (eg, most recent heart rate minus earliest heart rate, heart rate over preceding 24 hours), and other above‐mentioned variables.
We assessed the ability of a submodel to correctly distinguish patients who died, from survivors, using the c‐statistic, as well as other metrics recommended by Cook.17 At the end of the modeling process, we pooled the results across all submodels. For vital signs, where the rate of missing data was <3%, we tested submodels in which we dropped shifts with missing data, as well as submodels in which we imputed missing vital signs to a normal value. For laboratory data, where the rate of missing data for a given shift was much greater, we employed a probabilistic imputation method that included consideration of when a laboratory test result became available.
RESULTS
During the study period, a total of 102,488 patients experienced 145,335 hospitalizations at the study hospitals. We removed 66 patients with 138 hospitalizations for data quality reasons, leaving us with our initial study sample of 102,422 patients whose characteristics are summarized in Table 1. Table 1, in which the unit of analysis is an individual patient, shows that patients who experienced the primary outcome were similar to those patients described in our previous report, in terms of their characteristics on admission as well as in experiencing excess morbidity and mortality.6
Never Admitted to ICU | Direct Admit to ICU From ED | Unplanned Transfer to ICU* | Other ICU Admission | |
---|---|---|---|---|
| ||||
N | 89,269 | 5963 | 2880 | 4310 |
Age (mean SD) | 61.26 18.62 | 62.25 18.13 | 66.12 16.20 | 64.45 15.91 |
Male (n, %) | 37,228 (41.70%) | 3091 (51.84%) | 1416 (49.17%) | 2378 (55.17%) |
LAPS (mean SD) | 13.02 15.79 | 32.72 24.85 | 24.83 21.53 | 11.79 18.16 |
COPS(mean SD) | 67.25 51.42 | 73.88 57.42 | 86.33 59.33 | 78.44 52.49 |
% Predicted mortality risk (mean SD) | 1.93% 3.98% | 7.69% 12.59% | 5.23% 7.70% | 3.66% 6.81% |
Survived first hospitalization to discharge∥ | 88,479 (99.12%) | 5336 (89.49%) | 2316 (80.42%) | 4063 (94.27%) |
Care order on admission | ||||
Full code | 78,877 (88.36%) | 5198 (87.17%) | 2598 (90.21%) | 4097 (95.06%) |
Partial code | 664 (0.74%) | 156 (2.62%) | 50 (1.74%) | 27 (0.63%) |
Comfort care | 21 (0.02%) | 2 (0.03%) | 0 (0%) | 0 (0%) |
DNR | 8227 (9.22%) | 539 (9.04%) | 219 (7.60%) | 161 (3.74%) |
Comfort care and DNR | 229 (0.26%) | 9 (0.15%) | 2 (0.07%) | 2 (0.05%) |
No order | 1251 (1.40%) | 59 (0.99%) | 11 (0.38%) | 23 (0.53%) |
Admission diagnosis (n, %) | ||||
Pneumonia | 2385 (2.67%) | 258 (4.33%) | 242 (8.40%) | 68 (1.58%) |
Sepsis | 5822 (6.52%) | 503 (8.44%) | 279 (9.69%) | 169 (3.92%) |
GI bleeding | 9938 (11.13%) | 616 (10.33%) | 333 (11.56%) | 290 (6.73%) |
Cancer | 2845 (3.19%) | 14 (0.23%) | 95 (3.30%) | 492 (11.42%) |
Total hospital length of stay (days SD) | 3.08 3.29 | 5.37 7.50 | 12.16 13.12 | 8.06 9.53 |
Figure 1shows how we developed the analysis cohort, by removing patients with a comfort‐care‐only order placed within 4 hours after admission (369 patients/744 hospitalizations) and patients who were never admitted to the ward or TCU (7,220/10,574). This left a cohort consisting of 94,833 patients who experienced 133,879 hospitalizations spanning a total of 1,079,062 shifts. We then removed shifts where: 1) a patient was not on the ward at the start of a shift, or was on the ward for <4 hours of a shift; 2) the patient had a comfort‐care order in place at the start of the shift; and 3) the patient died and was ineligible to be a case (the patient had a DNR order in place or died in the ICU). The final cohort eligible for sampling consisted of 846,907 shifts, which involved a total of 92,797 patients and 130,627 hospitalizations. There were a total of 4,036 event shifts, which included 3,224 where a patient was transferred from the ward to the ICU, 717 from the TCU to the ICU, and 95 where a patient died on the ward or TCU without a DNR order in place. We then randomly selected 39,782 comparison shifts. Thus, our final cohort for analysis included 4,036 event shifts (1,979 derivation/2,057 validation and 39,782 comparison shifts (19,509/20,273). As a secondary validation, we also applied model coefficients to the 429 event shifts excluded due to the <4‐hour length‐of‐stay requirement.
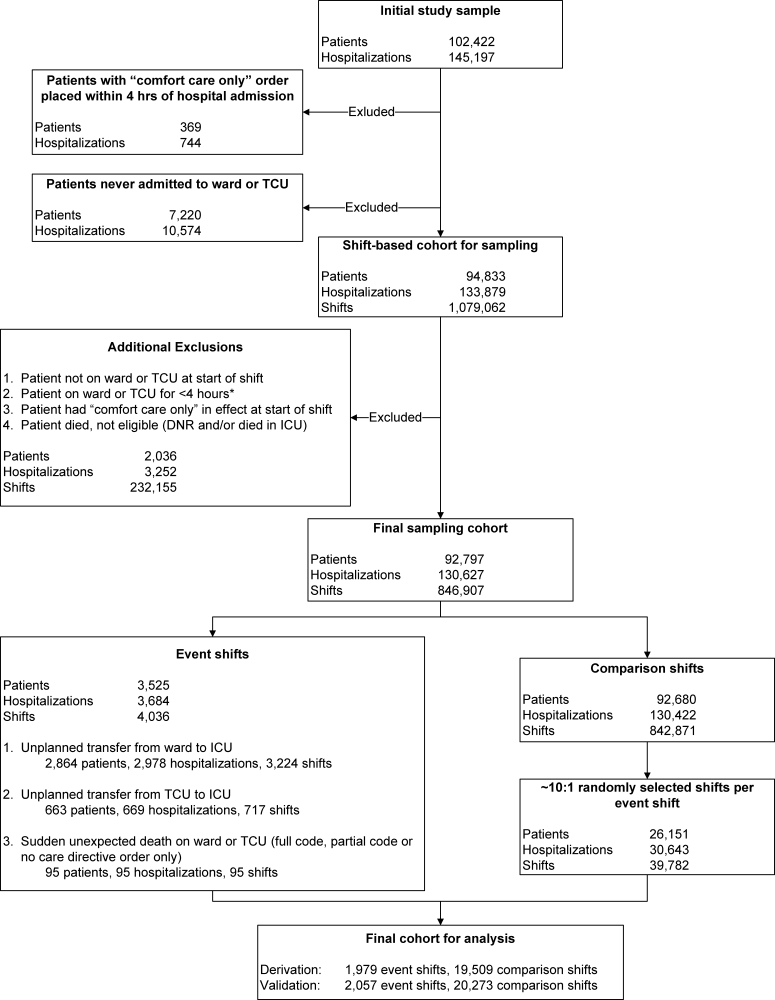
Table 2 compares event shifts with comparison shifts. In the 24 hours preceding ICU transfer, patients who were subsequently transferred had statistically significant, but not necessarily clinically significant, differences in terms of these variables. However, missing laboratory data were more common, ranging from 18% to 31% of all shifts (we did not incorporate laboratory tests where 35% of the shifts had missing data for that test).
Predictor | Event Shifts | Comparison Shifts | P |
---|---|---|---|
| |||
Number | 4036 | 39,782 | |
Age (mean SD) | 67.19 15.25 | 65.41 17.40 | <0.001 |
Male (n, %) | 2007 (49.73%) | 17,709 (44.52%) | <0.001 |
Day shift | 1364 (33.80%) | 17,714 (44.53%) | <0.001 |
LAPS* | 27.89 22.10 | 20.49 20.16 | <0.001 |
COPS | 116.33 72.31 | 100.81 68.44 | <0.001 |
Full code (n, %) | 3496 (86.2%) | 32,156 (80.8%) | <0.001 |
ICU shift during hospitalization | 3964 (98.22%) | 7197 (18.09%) | <0.001 |
Unplanned transfer to ICU during hospitalization∥ | 353 (8.8%) | 1466 (3.7%) | <0.001 |
Temperature (mean SD) | 98.15 (1.13) | 98.10 (0.85) | 0.009 |
Heart rate (mean SD) | 90.30 (20.48) | 79.86 (5.27) | <0.001 |
Respiratory rate (mean SD) | 20.36 (3.70) | 18.87 (1.79) | <0.001 |
Systolic blood pressure (mean SD) | 123.65 (23.26) | 126.21 (19.88) | <0.001 |
Diastolic blood pressure (mean SD) | 68.38 (14.49) | 69.46 (11.95) | <0.001 |
Oxygen saturation (mean SD) | 95.72% (3.00) | 96.47 % (2.26) | <0.001 |
MEWS(re) (mean SD) | 3.64 (2.02) | 2.34 (1.61) | <0.001 |
% <5 | 74.86% | 92.79% | |
% 5 | 25.14% | 7.21% | <0.001 |
Proxy for measured lactate# (mean SD) | 36.85 (28.24) | 28.73 (16.74) | <0.001 |
% Missing in 24 hr before start of shift** | 17.91% | 28.78% | <0.001 |
Blood urea nitrogen (mean SD) | 32.03 (25.39) | 22.72 (18.9) | <0.001 |
% Missing in 24 hr before start of shift | 19.67% | 20.90% | <0.001 |
White blood cell count 1000 (mean SD) | 12.33 (11.42) | 9.83 (6.58) | <0.001 |
% Missing in 24 hr before start of shift | 21.43% | 30.98% | <0.001 |
Hematocrit (mean SD) | 33.08 (6.28) | 33.07 (5.25) | 0.978 |
% Missing in 24 hr before start of shift | 19.87% | 29.55% | <0.001 |
After conducting multiple analyses using the derivation dataset, we developed 24 submodels, a compromise between our finding that primary‐condition‐specific models showed better performance and the fact that we had very few events among patients with certain primary conditions (eg, pericarditis/valvular heart disease), which forced us to create composite categories (eg, a category pooling patients with pericarditis, atherosclerosis, and peripheral vascular disease). Table 3 lists variables included in our final submodels.
Variable | Description |
---|---|
| |
Directive status | Full code or not full code |
LAPS* | Admission physiologic severity of illness score (continuous variable ranging from 0 to 256). Standardized and included as LAPS and LAPS squared |
COPS | Comorbidity burden score (continuous variable ranging from 0 to 701). Standardized and included as COPS and COPS squared. |
COPS status | Indicator for absent comorbidity data |
LOS at T0 | Length of stay in the hospital (total time in hours) at the T0; standardized. |
T0 time of day | 7 AM or 7 PM |
Temperature | Worst (highest) temperature in 24 hr preceding T0; variability in temperature in 24 hr preceding T0. |
Heart rate | Most recent heart rate in 24 hr preceding T0; variability in heart rate in 24 hr preceding T0. |
Respiratory rate | Most recent respiratory rate in 24 hr preceding T0; worst (highest) respiratory rate in 24 hr preceding T0; variability in respiratory rate in 24 hr preceding T0. |
Diastolic blood pressure | Most recent diastolic blood pressure in 24 hr preceding T0 transformed by subtracting 70 from the actual value and squaring the result. Any value above 2000 is subsequently then set to 2000, yielding a continuous variable ranging from 0 to 2000. |
Systolic pressure | Variability in systolic blood pressure in 24 hr preceding T0. |
Pulse oximetry | Worst (lowest) oxygen saturation in 24 hr preceding T0; variability in oxygen saturation in 24 hr preceding T0. |
Neurological status | Most recent neurological status check in 24 hr preceding T0. |
Laboratory tests | Blood urea nitrogen |
Proxy for measured lactate = (anion gap serum bicarbonate) 100 | |
Hematocrit | |
Total white blood cell count |
Table 4 summarizes key results in the validation dataset. Across all diagnoses, the MEWS(re) had c‐statistic of 0.709 (95% confidence interval, 0.6970.721) in the derivation dataset and 0.698 (0.6860.710) in the validation dataset. In the validation dataset, the MEWS(re) performed best among patients with a set of gastrointestinal diagnoses (c = 0.792; 0.7260.857) and worst among patients with congestive heart failure (0.541; 0.5000.620). In contrast, across all primary conditions, the EMR‐based models had a c‐statistic of 0.845 (0.8260.863) in the derivation dataset and 0.775 (0.7530.797) in the validation dataset. In the validation dataset, the EMR‐based models also performed best among patients with a set of gastrointestinal diagnoses (0.841; 0.7830.897) and worst among patients with congestive heart failure (0.683; 0.6100.755). A negative correlation (R = 0.63) was evident between the number of event shifts in a submodel and the drop in the c‐statistic seen in the validation dataset.
No. of Shifts in Validation Dataset | c‐Statistic | |||
---|---|---|---|---|
Diagnoses Group* | Event | Comparison | MEWS(re) | EMR Model |
| ||||
Acute myocardial infarction | 36 | 169 | 0.541 | 0.572 |
Diseases of pulmonary circulation and cardiac dysrhythmias | 40 | 329 | 0.565 | 0.645 |
Seizure disorders | 45 | 497 | 0.594 | 0.647 |
Rule out myocardial infarction | 77 | 727 | 0.602 | 0.648 |
Pneumonia | 163 | 847 | 0.741 | 0.801 |
GI diagnoses, set A | 58 | 942 | 0.755 | 0.803 |
GI diagnoses, set B∥ | 256 | 2,610 | 0.772 | 0.806 |
GI diagnoses, set C | 46 | 520 | 0.792 | 0.841 |
All diagnosis | 2,032 | 20,106 | 0.698 | 0.775 |
We also compared model performance when our datasets were restricted to 1 randomly selected observation per patient; in these analyses, the total number of event shifts was 3,647 and the number of comparison shifts was 29,052. The c‐statistic for the MEWS(re) in the derivation dataset was 0.709 (0.6940.725); in the validation dataset, it was 0.698 (0.6920.714). The corresponding values for the EMR‐based models were 0.856 (0.8350.877) and 0.780 (0.7560.804). We also tested models in which, instead of dropping shifts with missing vital signs, we imputed missing vital signs to their normal value. The c‐statistic for the EMR‐based model with imputed vital sign values was 0.842 (0.8230.861) in the derivation dataset and 0.773 (0.7520.794) in the validation dataset. Lastly, we applied model coefficients to a dataset consisting of 4,290 randomly selected comparison shifts plus the 429 shifts excluded because of the 4‐hour length‐of‐stay criterion. The c‐statistic for this analysis was 0.756 (0.7030.809).
As a general rule, the EMR‐based models were more than twice as efficient as the MEWS(re). For example, a MEWS(re) threshold of 6 as the trigger for an alarm would identify 15% of all transfers to the ICU, with 34.4 false alarms for each transfer; in contrast, using the EMR‐based approach to identify 15% of all transfers, there were 14.5 false alarms for each transfer. Applied to the entire KPMCP Northern California Region, using the MEWS(re), a total of 52 patients per day would need to be evaluated, but only 22 per day using the EMR‐based approach. If one employed a MEWS(re) threshold of 4, this would lead to identification of 44% of all transfers, with a ratio of 69 false alarms for each transfer; using the EMR, the ratio would be 34 to 1. Across the entire KPMCP, a total of 276 patients per day (or about 19.5 a day per hospital) would need to be evaluated using the MEWS(re), but only 136 (or about 9.5 per hospital per day) using the EMR.
DISCUSSION
Using data from a large hospital cohort, we have developed a predictive model suitable for use in non‐ICU populations cared for in integrated healthcare settings with fully automated EMRs. The overall performance of our model, which incorporates acute physiology, diagnosis, and longitudinal data, is superior to the predictive ability of a model that can be assigned manually. This is not surprising, given that scoring systems such as the MEWS make an explicit tradeoff losing information found in multiple variables in exchange for ease of manual assignment. Currently, the model described in this report is being implemented in a simulated environment, a final safety test prior to piloting real‐time provision of probability estimates to clinicians and nurses. Though not yet ready for real‐time use, it is reasonable for our model to be tested using the KPHC shadow server, since evaluation in a simulated environment constitutes a critical evaluation step prior to deployment for clinical use. We also anticipate further refinement and revalidation to occur as more inpatient data become available in the KPMCP and elsewhere.
A number of limitations to our approach must be emphasized. In developing our models, we determined that, while modeling by clinical condition was important, the study outcome was rare for some primary conditions. In these diagnostic groups, which accounted for 12.5% of the event shifts and 10.6% of the comparison shifts, the c‐statistic in the validation dataset was <0.70. Since all 22 KPMCP hospitals are now online and will generate an additional 150,000 adult hospitalizations per year, we expect to be able to correct this problem prior to deployment of these models for clinical use. Having additional data will permit us to improve model discrimination and thus decrease the evaluation‐to‐detection ratio. In future iterations of these models, more experimentation with grouping of International Classification of Diseases (ICD) codes may be required. The problem of grouping ICD codes is not an easy one to resolve, in that diagnoses in the grouping must share common pathophysiology while having a grouping with a sufficient number of adverse events for stable statistical models.
Ideally, it would have been desirable to employ a more objective measure of deterioration, since the decision to transfer a patient to the ICU is discretionary. However, we have found that key data points needed to define such a measure (eg, vital signs) are not consistently charted when a patient deterioratesthis is not surprising outside the research setting, given that nurses and physicians involved in a transfer may be focusing on caring for the patient rather than immediately charting. Given the complexities of end‐of‐life‐care decision‐making, we could not employ death as the outcome of interest. A related issue is that our model does not differentiate between reasons for needing transfer to the ICU, an issue recently discussed by Bapoje et al.18
Our model does not address an important issue raised by Bapoje et al18 and Litvak, Pronovost, and others,19, 20 namely, whether a patient should have been admitted to a non‐ICU setting in the first place. Our team is currently developing a model for doing exactly this (providing decision support for triage in the emergency department), but discussion of this methodology is outside the scope of this article.
Because of resource and data limitations, our model also does not include newborns, children, women admitted for childbirth, or patients transferred from non‐KPMCP hospitals. However, the approach described here could serve as a starting point for developing models for these other populations.
The generalizability of our model must also be considered. The Northern California KPMCP is unusual in having large electronic databases that include physiologic as well as longitudinal patient data. Many hospitals cannot take advantage of all the methods described here. However, the methods we employed could be modified for use by hospital systems in countries such as Great Britain and Canada, and entities such as the Veterans Administration Hospital System in the United States. The KPMCP population, an insured population with few barriers to access, is healthier than the general population, and some population subsets are underrepresented in our cohort. Practice patterns may also vary. Nonetheless, the model described here could serve as a good starting point for future collaborative studies, and it would be possible to develop models suitable for use by stand‐alone hospitals (eg, recalibrating so that one used a Charlson comorbidity21 score based on present on‐admission codes rather than the COPS).
The need for early detection of patient deterioration has played a major role in the development of rapid response teams, as well as scores such as the MEWS. In particular, entities such as the Institute for Healthcare Improvement have advocated the use of early warning systems.22 However, having a statistically robust model to support an early warning system is only part of the solution, and a number of new challenges must then be addressed. The first is actual electronic deployment. Existing inpatient EMRs were not designed with complex calculations in mind, and we anticipate that some degradation in performance will occur when we test our models using real‐time data capture. As Bapoje et al point out, simply having an alert may be insufficient, since not all transfers are preventable.18 Early warning systems also raise ethical issues (for example, what should be done if an alert leads a clinician to confront the fact that an end‐of‐life‐care discussion needs to occur?). From a research perspective, if one were to formally test the benefits of such models, it would be critical to define outcome measures other than death (which is strongly affected by end‐of‐life‐care decisions) or ICU transfer (which is often desirable).
In conclusion, we have developed an approach for predicting impending physiologic deterioration of hospitalized adults outside the ICU. Our approach illustrates how organizations can take maximal advantage of EMRs in a manner that exceeds meaningful use specifications.23, 24 Our study highlights the possibility of using fully automated EMR data for building and applying sophisticated statistical models in settings other than the highly monitored ICU without the need for additional equipment. It also expands the universe of severity scoring to one in which probability estimates are provided in real time and throughout an entire hospitalization. Model performance will undoubtedly improve over time, as more patient data become available. Although our approach has important limitations, it is suitable for testing using real‐time data in a simulated environment. Such testing would permit identification of unanticipated problems and quantification of the degradation of model performance due to real life factors, such as delays in vital signs charting or EMR system brownouts. It could also serve as the springboard for future collaborative studies, with a broader population base, in which the EMR becomes a tool for care, not just documentation.
Acknowledgements
We thank Ms Marla Gardner and Mr John Greene for their work in the development phase of this project. We are grateful to Brian Hoberman, Andrew Hwang, and Marc Flagg from the RIMS group; to Colin Stobbs, Sriram Thiruvenkatachari, and Sundeep Sood from KP IT, Inc; and to Dennis Andaya, Linda Gliner, and Cyndi Vasallo for their assistance with data‐quality audits. We are also grateful to Dr Philip Madvig, Dr Paul Feigenbaum, Dr Alan Whippy, Mr Gregory Adams, Ms Barbara Crawford, and Dr Marybeth Sharpe for their administrative support and encouragement; and to Dr Alan S. Go, Acting Director of the Kaiser Permanente Division of Research, for reviewing the manuscript.
- Day of the week of intensive care admission and patient outcomes: a multisite regional evaluation.Med Care.2002;40(6):530–539. , , , .
- The hospital mortality of patients admitted to the ICU on weekends.Chest.2004;126(4):1292–1298. , , , et al.
- Mortality among patients admitted to intensive care units during weekday day shifts compared with “off” hours.Crit Care Med.2007;35(1):3–11. , , , et al.
- Risk adjusting hospital inpatient mortality using automated inpatient, outpatient, and laboratory databases.Med Care.2008;46(3):232–239. , , , , , .
- The Kaiser Permanente inpatient risk adjustment methodology was valid in an external patient population.J Clin Epidemiol.2010;63(7):798–803. , , , .
- Intra‐hospital transfers to a higher level of care: contribution to total hospital and intensive care unit (ICU) mortality and length of stay (LOS).J Hosp Med.2011;6(2):74–80. , , , , , .
- Multicentric study of monitoring alarms in the adult intensive care unit (ICU): a descriptive analysis.Intensive Care Med.1999;25(12):1360–1366. , , , , , .
- Integration of early physiological responses predicts later illness severity in preterm infants.Sci Transl Med.2010;2(48):48ra65. , , , , .
- Reproducibility of physiological track‐and‐trigger warning systems for identifying at‐risk patients on the ward.Intensive Care Med.2007;33(4):619–624. , , .
- Validation of a Modified Early Warning Score in medical admissions.Q J Med.2001;94:521–526. , , , .
- Effect of introducing the Modified Early Warning score on clinical outcomes, cardio‐pulmonary arrests and intensive care utilisation in acute medical admissions.Anaesthesia.2003;58(8):797–802. , , , , .
- MERIT Study Investigators.Introduction of the medical emergency team (MET) system: a cluster‐randomized controlled trial.Lancet.2005;365(9477):2091–2097.
- Unplanned transfers to the intensive care unit: the role of the shock index.J Hosp Med.2010;5(8):460–465. , , , , , .
- The delta (delta) gap: an approach to mixed acid‐base disorders.Ann Emerg Med.1990;19(11):1310–1313. .
- Acid‐base disorders: classification and management strategies.Am Fam Physician.1995;52(2):584–590. .
- Unmeasured anions in critically ill patients: can they predict mortality?Crit Care Med.2003;31(8):2131–2136. , , , .
- Use and misuse of the receiver operating characteristic curve in risk prediction.Circulation.2007;115(7):928–935. .
- Unplanned transfers to a medical intensive care unit: causes and relationship to preventable errors in care.J Hosp Med.2011;6(2):68–72. , , , .
- Rethinking rapid response teams.JAMA.2010;304(12):1375–1376. , .
- Rapid response teams—walk, don't run.JAMA.2006;296(13):1645–1647. , , .
- A new method of classifying prognostic comorbidity in longitudinal populations: development and validation.J Chronic Dis.1987;40:373–383. , , , .
- Institute for Healthcare Improvement.Early Warning Systems:The Next Level of Rapid Response.2011. http://www.ihi.org/IHI/Programs/AudioAndWebPrograms/ExpeditionEarlyWarningSystemsTheNextLevelofRapidResponse.htm?player=wmp. Accessed 4/6/11.
- Assessing readiness for meeting meaningful use: identifying electronic health record functionality and measuring levels of adoption.AMIA Annu Symp Proc.2010;2010:66–70. .
- Medicare and Medicaid Programs;Electronic Health Record Incentive Program. Final Rule.Fed Reg.2010;75(144):44313–44588.
- Day of the week of intensive care admission and patient outcomes: a multisite regional evaluation.Med Care.2002;40(6):530–539. , , , .
- The hospital mortality of patients admitted to the ICU on weekends.Chest.2004;126(4):1292–1298. , , , et al.
- Mortality among patients admitted to intensive care units during weekday day shifts compared with “off” hours.Crit Care Med.2007;35(1):3–11. , , , et al.
- Risk adjusting hospital inpatient mortality using automated inpatient, outpatient, and laboratory databases.Med Care.2008;46(3):232–239. , , , , , .
- The Kaiser Permanente inpatient risk adjustment methodology was valid in an external patient population.J Clin Epidemiol.2010;63(7):798–803. , , , .
- Intra‐hospital transfers to a higher level of care: contribution to total hospital and intensive care unit (ICU) mortality and length of stay (LOS).J Hosp Med.2011;6(2):74–80. , , , , , .
- Multicentric study of monitoring alarms in the adult intensive care unit (ICU): a descriptive analysis.Intensive Care Med.1999;25(12):1360–1366. , , , , , .
- Integration of early physiological responses predicts later illness severity in preterm infants.Sci Transl Med.2010;2(48):48ra65. , , , , .
- Reproducibility of physiological track‐and‐trigger warning systems for identifying at‐risk patients on the ward.Intensive Care Med.2007;33(4):619–624. , , .
- Validation of a Modified Early Warning Score in medical admissions.Q J Med.2001;94:521–526. , , , .
- Effect of introducing the Modified Early Warning score on clinical outcomes, cardio‐pulmonary arrests and intensive care utilisation in acute medical admissions.Anaesthesia.2003;58(8):797–802. , , , , .
- MERIT Study Investigators.Introduction of the medical emergency team (MET) system: a cluster‐randomized controlled trial.Lancet.2005;365(9477):2091–2097.
- Unplanned transfers to the intensive care unit: the role of the shock index.J Hosp Med.2010;5(8):460–465. , , , , , .
- The delta (delta) gap: an approach to mixed acid‐base disorders.Ann Emerg Med.1990;19(11):1310–1313. .
- Acid‐base disorders: classification and management strategies.Am Fam Physician.1995;52(2):584–590. .
- Unmeasured anions in critically ill patients: can they predict mortality?Crit Care Med.2003;31(8):2131–2136. , , , .
- Use and misuse of the receiver operating characteristic curve in risk prediction.Circulation.2007;115(7):928–935. .
- Unplanned transfers to a medical intensive care unit: causes and relationship to preventable errors in care.J Hosp Med.2011;6(2):68–72. , , , .
- Rethinking rapid response teams.JAMA.2010;304(12):1375–1376. , .
- Rapid response teams—walk, don't run.JAMA.2006;296(13):1645–1647. , , .
- A new method of classifying prognostic comorbidity in longitudinal populations: development and validation.J Chronic Dis.1987;40:373–383. , , , .
- Institute for Healthcare Improvement.Early Warning Systems:The Next Level of Rapid Response.2011. http://www.ihi.org/IHI/Programs/AudioAndWebPrograms/ExpeditionEarlyWarningSystemsTheNextLevelofRapidResponse.htm?player=wmp. Accessed 4/6/11.
- Assessing readiness for meeting meaningful use: identifying electronic health record functionality and measuring levels of adoption.AMIA Annu Symp Proc.2010;2010:66–70. .
- Medicare and Medicaid Programs;Electronic Health Record Incentive Program. Final Rule.Fed Reg.2010;75(144):44313–44588.
Copyright © 2012 Society of Hospital Medicine