User login
Evidence‐Based Care for Cellulitis
Cellulitis is a common infection causing inflammation of the skin and subcutaneous tissues. Cellulitis has been attributed to gram‐positive organisms through historical evaluations including fine‐needle aspirates and punch biopsies of the infected tissue.[1] Neither of these diagnostic tests is currently used due to their invasiveness, poor diagnostic yield, and availability. Similarly, readily available tests such as blood cultures provide an etiology <5% of the time[1] and are not cost‐effective for most patients for diagnosing cellulitis.[2] In addition, the prevalence of methicillin‐resistant Staphylococcus aureus (MRSA) has steadily increased, complicating decisions about antibiotic selection.[3] The result of this uncertainty is a large variation in practice with respect to antibiotic and imaging selection for patients with a diagnosis of cellulitis.
University of Utah Health Care (UUHC) performed benchmarking for the management of cellulitis using the University HealthSystem Consortium (UHC) database and associated CareFx analytics tool. Benchmarking demonstrated that UUHC had a greater percentage of broad‐spectrum antibiotic use (defined as vancomycin, piperacillin/tazobactam, or carbapenems) than the top 5 performing UHC facilities for International Classification of Diseases, Ninth Revision, Clinical Modification (ICD‐9‐CM) diagnoses of cellulitis (vancomycin 83% vs 58% and carbapenem or piperacillin/tazobactam 44% vs 16%). Advanced imaging (computed tomography [CT] or magnetic resonance imaging [MRI]) for the diagnosis of cellulitis was also found to be an opportunity for improvement (CT 27% vs 20% and MRI 8% vs 5%). The hospitalist group (most patients admitted with cellulitis were on this service) believed these data reflected current practice, as there was no standard of treatment for cellulitis despite an active order set. Therefore, cellulitis was considered an opportunity to improve value to our patients. A standardized clinical care pathway was created, as such pathways have demonstrated a reduction in variation in practice and improved efficiency and effectiveness of care for multiple disease states including cellulitis.[4, 5] We hypothesized that implementation of an evidence‐based care pathway would decrease broad‐spectrum antibiotic use, cost, and use of advanced imaging without having any adverse effects on clinical outcomes such as length of stay (LOS) or readmission.
METHODS
Study Setting and Population
UUHC is a 500‐bed academic medical center in Salt Lake City, Utah. All patients admitted to the emergency department observation unit (EDOU) or the hospital with a primary ICD‐9‐CM diagnosis of cellulitis between July 1, 2011 and December 31, 2013 were evaluated.
Intervention
Initial steps involved the formation of a multidisciplinary team including key stakeholders from the hospitalist group, infectious diseases, the emergency department (ED), and nursing. This multidisciplinary team was charged with developing a clinical care pathway appropriate for local implementation. National guidance for the care pathway was mainly obtained from the Infectious Disease Society of America (IDSA) guidelines on skin and soft tissue infections (SSTIs)[6] and MRSA.[7] Specific attention was paid to recommendations on blood cultures (only when systemically ill), imaging (rarely needed), antibiotic selection (rarely gram‐negative coverage and consideration of MRSA coverage), and patient‐care principles that are often overlooked (elevation of the affected extremity). A distinction of purulent versus nonpurulent cellulitis was adopted based on the guidelines and a prospective evaluation of the care of patients with nonpurulent cellulitis.[8] The 2014 IDSA update on SSTIs incorporates this distinction more clearly in hopes of determining staphylococcal versus streptococcal infections.[9] After multiple iterations, an agreed‐upon care pathway was created that excluded patients with neutropenia, osteomyelitis, diabetic foot ulcerations; hand, perineal, periorbital, or surgical site infections; and human or animal bites (Figure 1). After the care pathway was determined, interventions were performed to implement this change.
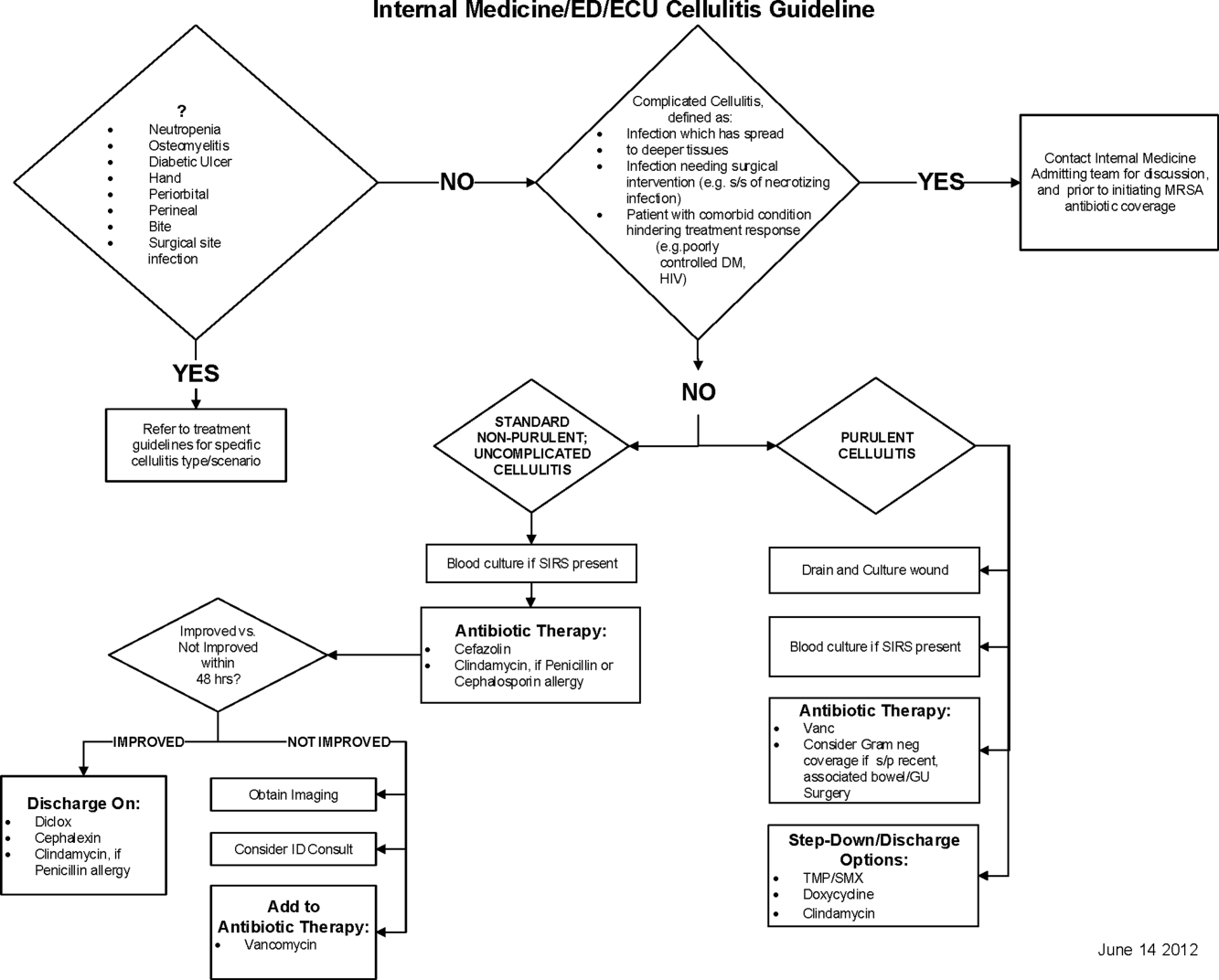
Education of all providers involved included discussion of cellulitis as a disease process, presentation of benchmarking data, dissemination of the care pathway to hospitalist and ED physicians, teaching conferences for internal medicine residents and ED residents, and reinforcement of these concepts at the beginning of resident rotations.
Incorporation of the care pathway into the existing electronic order sets for cellulitis care in the inpatient and ED settings, with links to the care pathway, links to excluded disease processes (eg, hand cellulitis), preselection of commonly needed items (eg, elevate leg), and recommendations for antibiotic selection based on categories of purulent or nonpurulent cellulitis. The electronic health record (EHR) did not allow for forced order set usage, so the order set required selection by the admitting physician if indicated. Additionally, an embedded 48‐hour order set could be accessed at any time by the ordering physician and included vancomycin dosing. Specific changes to the preexisting order set included the development of sections for purulent and nonpurulent cellulitis as well as recommended antibiotics. Piperacillin/tazobactam and nafcillin were both removed and vancomycin was limited to the purulent subheading. Additionally, elevation of the extremity was preselected, and orderables for imaging (chest x‐ray and duplex ultrasound), antiulcer prophylaxis, telemetry, and electrocardiograph were all removed.
Audit and feedback of cases of cellulitis and broad‐spectrum antibiotic usage was performed by a senior hospitalist.
Study Design
A retrospective before/after study was performed to assess overall impact of the intervention on the patient population. Additionally, a retrospective controlled pre‐/postintervention study was performed to compare changes in cellulitis management for visits where order sets were used with visits where order sets were not used. The intervention initiation date was July 9, 2012. The institutional review board classified this project as quality improvement and did not require review and oversight.
Study Population
We analyzed 2278 ED and inpatient visits for cellulitis, of which 677 met inclusion criteria. We partitioned visits into 2 groups: (1) those for which order sets were used (n = 370) and (2) control visits for which order sets were not used (n = 307). We analyzed outcomes for 2 subpopulations: hospitalized patients for whom the EDOU or admission order sets were used (n = 149) and patients not admitted and only seen in the EDOU for whom the EDOU order set was used (n = 262).
Inclusion Criteria
Inclusion criteria included hospital admission or admission to the EDOU between July 1, 2011 and December 31, 2013, age greater or equal to 18 years, and primary diagnosis of cellulitis as determined by ICD‐9‐CM billing codes 035, 457.2, 681, 681.0, 681.00, 681.01, 681.02, 681.1, 681.10, 681.11, 681.9, 680, 680.0‐9, 682.0‐9, 684, 685.0, 685.1, 686.00, 686.01, 686.09, 686.1, 686.8, 686.9, 910.1, 910.5, 910.7, 910.9, 911.1, 911.3, 911.5, 911.7, 911.9, 912.1, 912.3, 912.5, 912.7, 912.9, 913.1, 913.3, 913.5, 913.7, 913.9, 914.1, 914.3, 914.5, 914.7, 914.9, 915.1, 915.3, 915.5, 915.7, 915.9, 916.1, 916.3, 916.5, 916.7, 916.9, 917.1, 917.3, 917.5, 917.7, 917.9, 919.1, 919.3, 919.5, 919.7, or 919.9.
Data Collection and Preparation
Clinical data were collected in the inpatient EHR (Cerner Corp., Kansas City, MO) and later imported into the enterprise data warehouse (EDW) as part of the normal data flow. Billing data were imported into the EDW from the billing system. Cost data were estimated using the value‐driven outcomes (VDO) tool developed by the University of Utah to identify clinical costs to the UUHC system.[10] All data were extracted from the EDW on September 10, 2014.
Process Metrics, Clinical, and Cost Outcomes
We defined 1 primary outcome (use of broad‐spectrum antibiotics) and 8 secondary outcomes, including process metrics (MRI and CT orders), clinical outcomes (LOS and 30‐day readmissions), and cost outcomes (pharmacy, lab, imaging cost from radiology department, and total facility cost). Broad‐spectrum antibiotics were defined as any use of meropenem (UUHC's carbapenem), piperacillin/tazobactam, or vancomycin and were determined by orders. Thirty‐day readmissions included only inpatient encounters with the primary diagnosis of cellulitis.
Covariates
To control for patient demographics we included age at admission in years and gender into the statistical model. To control for background health state as well as cellulitis severity, we included Charlson Comorbidity Index (CCI) and hospitalization status. CCI was calculated according to the algorithm specified by Quan et al.[11]
Study Hypotheses
First, for all patients, we hypothesized that process metrics as well as clinical and cost outcomes would improve following the implementation of the care pathway. To evaluate this hypothesis, we estimated impact of the time interval (pre‐/postintervention) on all outcomes. Second, we hypothesized that among patients for whom order sets were used (which we deemed to be a proxy for following the agreed‐upon care pathway), there would be a greater improvement than in patients for whom order sets were not used. To evaluate this hypothesis, we estimated interactions between order set use and time period (pre‐/postintervention) for all outcomes.
Statistical Analysis
The variable time period was created to represent the time period before and after the intervention.
We provided unadjusted descriptive statistics for study outcomes and visit characteristics for all patients before and after intervention. Descriptive statistics were expressed as n (%) and mean standard deviation. Simple comparisons were performed based on 2 test of homogeneity for categorical variables and t test or Wilcoxon test for continuous variables.
For before/after analysis, we fitted generalized linear regression models to estimate the change in outcomes of interest before and after intervention for all patients simultaneously. Generalized linear model defined by a binomial distributional assumption and logit link function was used to estimate the effect of the intervention on antibiotic use, imaging orders, and readmission adjusting for effects of age, gender, CCI, and hospitalization status. A generalized linear model defined by a gamma distributional assumption and log link function was used to estimate effect of the intervention on clinical LOS and cost outcomes adjusting for the effects of the same covariates. Generalized linear models with gamma distributional assumptions were used because they are known to perform well even for zero‐inflated semicontinuous cost variables and are easier to interpret than 2‐part models.
For the controlled before/after analysis, the variable order set used was created to represent groups where order sets were used or not used. Similarly, generalized linear models were used to estimate differential effect of the intervention at 2 different order set use levels using an interaction term between order set use and the time period.
P values <0.05 were considered significant. We used SAS version 9.3 statistical software (SAS Institute Inc., Cary, NC) for data analysis.
RESULTS
Descriptive Characteristics
Patient characteristics before and after intervention for 677 EDOU and inpatient visits for cellulitis by 618 patients are summarized in the first 4 columns of Table 1. Patient age at admission ranged from 18 to 98 years. Thirty‐eight percent of visits were by female patients. There were 274 visits before the intervention and 403 visits after. Four hundred thirty‐two (64%) were admitted, and 295 (44%) were seen in the EDOU. The admission order set alone was used for 104 visits, the EDOU order set alone was used for 242 visits, and both order sets were used for 24 visits.
Characteristic | Overall | Order Sets Not Used | Order Sets Used | ||||||
---|---|---|---|---|---|---|---|---|---|
Baseline, N = 274 | Intervention, N = 403 | P* | Baseline, N = 127 | Intervention, N = 180 | P* | Baseline, N = 147 | Intervention, N = 223 | P* | |
| |||||||||
Patient Characteristics | |||||||||
Age, y | 46.8 16.0 | 48.9 17.1 | 0.097 | 49.8 16.0 | 5.1 16.3 | 0.88 | 44.2 15.5 | 48.0 17.6 | 0.032 |
Female gender | 105 (38%) | 155 (39%) | 0.93 | 50 (39%) | 74 (41%) | 0.73 | 55 (37%) | 81 (36%) | 0.86 |
CCI | 2.6 3.2 | 2.6 3.0 | 0.69 | 3.2 3.5 | 3.2 3.2 | 0.82 | 2.0 2.8 | 2.1 2.7 | 0.68 |
Clinical process characteristics | |||||||||
EDOU admission | 122 (45%) | 173 (43%) | 0.68 | 12 (9%) | 19 (11%) | 0.75 | 110 (75%) | 154 (69%) | 0.23 |
Hospital admission | 173 (63%) | 259 (64%) | 0.76 | 117 (92%) | 166 (92%) | 0.98 | 56 (38%) | 93 (42%) | 0.49 |
EDOU order set used | 111 (41%) | 155 (38%) | 0.59 | NA | NA | NA | 111 (76%) | 155 (70%) | 0.21 |
ADM order set used | 47 (17%) | 81 (20%) | 0.34 | NA | NA | NA | 47 (32%) | 81 (36%) | 0.39 |
Process outcomes | |||||||||
Broad‐spectrum antibiotics used | 205 (75%) | 230 (57%) | <0.001 | 90 (71%) | 121 (67%) | 0.50 | 115 (78%) | 109 (49%) | <0.001 |
MRI done | 27 (10%) | 32 (8%) | 0.39 | 13 (10%) | 20 (11%) | 0.81 | 14 (10%) | 12 (5%) | 0.13 |
CT done | 56 (20%) | 76 (19%) | 0.61 | 32 (25%) | 43 (24%) | 0.79 | 24 (16%) | 33 (15%) | 0.69 |
Clinical outcomes | |||||||||
Length of stay, d | 2.7 2.6 | 2.6 2.8 | 0.35 | 3.6 2.8 | 3.8 3.4 | 0.62 | 2.0 2.1 | 1.7 1.6 | 0.48 |
30‐day readmission | 14 (5%) | 17 (4%) | 0.59 | 7 (6%) | 9 (5%) | 0.84 | 7 (5%) | 8 (4%) | 0.58 |
Cost outcomes | |||||||||
Pharmacy cost ($) | 1 | 0.76 | 0.002 | 1 | 0.89 | 0.13 | 1 | 0.56 | 0.004 |
Lab cost ($) | 1 | 0.52 | <0.001 | 1 | 0.53 | 0.001 | 1 | 0.51 | 0.055 |
Imaging cost ($) | 1 | 0.82 | 0.11 | 1 | 0.95 | 0.52 | 1 | 0.67 | 0.13 |
Total facility cost ($) | 1 | 0.85 | 0.027 | 1 | 0.91 | 0.042 | 1 | 0.77 | 0.26 |
Before/After Analysis
Among all patients, use of broad‐spectrum antibiotics decreased from 75% to 57% (Table 1). Analysis adjusted for gender, age at admission, CCI, and hospital admission status is provided in Table 2. Overall, there was a 59% decrease in the odds of ordering broad‐spectrum antibiotics (P < 0.001), a 23% decrease in pharmacy cost (P = 0.002), a 44% decrease in laboratory cost (P < 0.001), and a 13% decrease in total facility cost (P = 0.006).
Logistic Regression | ||||
---|---|---|---|---|
Outcome Variables | Selected Predictor Variables | Odds* | Percent Change | P |
Gamma Regression | ||||
Outcome Variables | Selected Predictor Variables | Fold Change* | Percent Change | P |
| ||||
Antibiotics used | Time period | 0.41 (0.29, 0.59) | 59% (71% to 41%) | <0.001 |
MRI done | Time period | 0.74 (0.43, 1.30) | 26% (57% to 30%) | 0.29 |
CT done | Time period | 0.92 (0.62, 1.36) | 8% (38% to 36%) | 0.67 |
30‐day readmission | Time period | 0.86 (0.41, 1.80) | 14% (59% to 80%) | 0.69 |
Length of stay, d | Time period | 0.97 (0.91, 1.03) | 3% (9% to 3%) | 0.34 |
Pharmacy cost ($) | Time period | 0.77 (0.65, 0.91) | 23% (35% to 9%) | 0.002 |
Lab cost ($) | Time period | 0.56 (0.48, 0.65) | 44% (52% to 35%) | <0.001 |
Imaging cost($) | Time period | 0.90 (0.71, 1.14) | 10% (29% to 14%) | 0.38 |
Total facility cost ($) | Time Period | 0.87 (0.79, 0.96) | 13% (21% to 4%) | 0.006 |
Order Set Use Groups Analysis
Descriptive statistics and simple comparison before/after the intervention for the 2 study groups are shown in the last 6 columns of Table 1. Among patients for whom order sets were used, broad‐spectrum antibiotic usage significantly decreased from 78% before the intervention to 49% after the intervention (P < 0.001). In contrast, among patients for whom order sets were not used, broad‐spectrum antibiotic usage remained relatively constant71% before the intervention versus 67% after the intervention (P = 0.50). Figure 2 shows semiannual changes in the prescription of broad‐spectrum antibiotics. There is a noticeable drop after the intervention among patients for whom order sets were used.
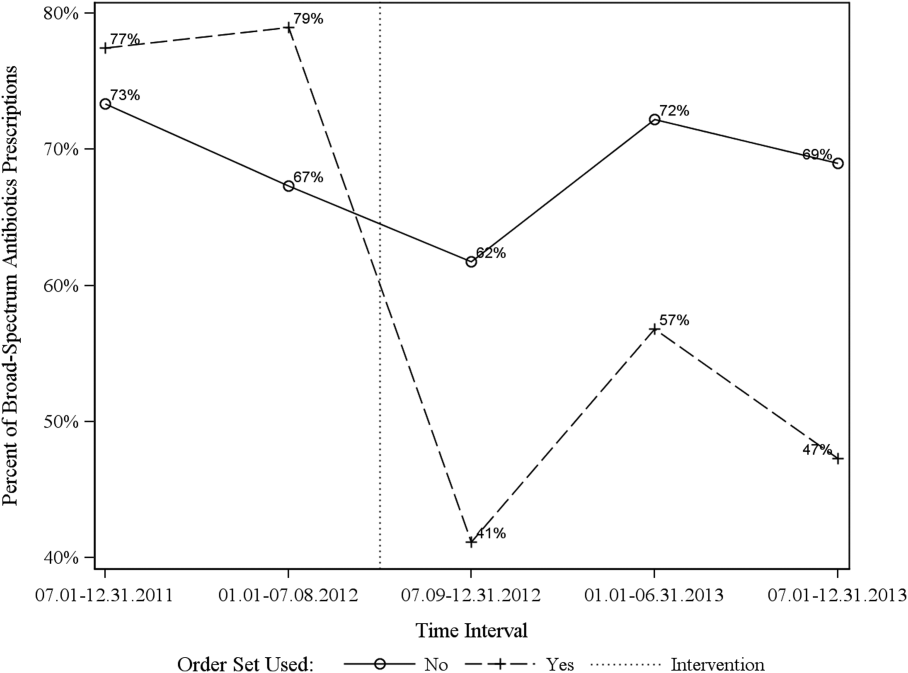
Analysis of the interaction between time period and order set usage is provided in Table 3. After the intervention, patients for whom the order sets were used had greater improvement in broad‐spectrum antibiotic selection (75% decrease, P < 0.001) and LOS (25% decrease, P = 0.041) than patients for whom order sets were not used. Pharmacy costs also decreased by 13% more among patients for whom the order sets were used, although the interaction was not statistically significant (P = 0.074). Laboratory costs decreased in both groups, but order set use did not demonstrate an interaction (P = 0.5). Similar results were found for the subgroups of admitted patients and patients seen in the EDOU.
Logistic Regression | ||||
---|---|---|---|---|
Outcome Variables | Selected Predictor Variables | Odds* | Percent Change | P |
Gamma Regression | ||||
Outcome Variables | Selected Predictor Variables | Fold Change* | Percent Change | P |
| ||||
Broad spectrum antibiotics | Time period | 0.84 (0.50, 1.40) | 16% (50% to 40%) | 0.50 |
Time periodorder set | 0.25 (0.12, 0.52) | 75% (88% to 48%) | <0.001 | |
MRI done | Time period | 1.04 (0.49, 2.20) | 4% (51% to 120%) | 0.92 |
Time periodorder set | 0.44 (0.14, 1.38) | 56% (86% to 38%) | 0.16 | |
CT done | Time period | 0.94 (0.55, 1.60) | 6% (45% to 60%) | 0.81 |
Time periodorder set | 0.96 (0.44, 2.12) | 4% (56% to 112%) | 0.93 | |
30‐day readmission | Time period | 0.91 (0.33, 2.53) | 9% (67% to 153%) | 0.86 |
Time periodorder set | 0.88 (0.20, 3.93) | 12% (80% to 293%) | 0.87 | |
Clinical length of stay | Time period | 1.04 (0.95, 1.14) | 4% (5% to 14%) | 0.41 |
Time periodorder set | 0.87 (0.77, 0.99) | 13% (23% to 1%) | 0.041 | |
Pharmacy cost ($) | Time period | 0.88 (0.70, 1.12) | 12% (30% to 12%) | 0.31 |
Time periodorder set | 0.75 (0.54, 1.03) | 25% (46% to 3%) | 0.074 | |
Lab cost ($) | Time period | 0.53 (0.42, 0.66) | 47% (58% to 34%) | <0.001 |
Time periodorder set | 1.11 (0.82, 1.50) | 11% (18% to 50%) | 0.50 | |
Imaging cost ($) | Time period | 1.00 (0.71, 1.40) | 0% (29% to 40%) | 0.98 |
Time periodorder set | 0.82 (0.51, 1.30) | 18% (49% to 30%) | 0.39 | |
Facility cost ($) | Time period | 0.92 (0.80, 1.05) | 8% (20% to 5%) | 0.22 |
Time periodorder set | 0.90 (0.75, 1.09) | 10% (25% to 9%) | 0.29 |
Audit and feedback was initially performed for cases of cellulitis using broad‐spectrum antibiotics. However, given the complexity of cellulitis as a disease process and the frequency of broad‐spectrum antibiotic usage, in all cases of review, it was deemed reasonable to use broad‐spectrum antibiotics. Therefore, the audit was not continued.
DISCUSSION
Care pathways have demonstrated improvement across multiple different disease states including cellulitis.[4, 5] They have been noted to reduce variation in practice and improve physician agreement about treatment options.[4] The best method for implementation is not clearly understood,[12] and there remains concern about maintaining flexibility for patient care.[13] Additionally, although implementation of pathways is often well described, evaluations of the processes are noted to frequently be weak.[12] UUHC felt that the literature supported implementing a care pathway for the diagnosis of cellulitis, but that a thorough evaluation was also needed to understand any resulting benefits or harms. Through this study, we found that the implementation of this pathway resulted in a significant decrease in broad‐spectrum antibiotic use, pharmacy costs, and total facility costs. There was also a trend to decrease in imaging cost, and there were no adverse effects on LOS or 30‐day readmissions. Our findings demonstrate that care‐pathway implementation accompanied by education, pathway‐compliant electronic order sets, and audit and feedback can help drive improvements in quality while reducing costs. This finding furthers the evidence supporting standard work through the creation of clinical care pathways for cellulitis as an effective intervention.[4] Additionally, although not measured in this study, reduction of antibiotic use is supported as a measure to help reduce Clostridium difficile infections, a further potential benefit.[14]
This study has several important strengths. First, we included accurate cost analyses using the VDO tool. Given the increasing importance of improving care value, we feel the inclusion of such cost analysis is an increasingly important aspect of health service intervention evaluations. Second, we used a formal benchmarking approach to identify a priority care improvement area and to monitor changes in practice following the rollout of the intervention. We feel this approach provides a useful example on how to systematically improve care quality and value in a broader health system context. Third, we evaluated not order set implementation per se, but rather changing an existing order set. Because studies in this area generally focus on initial order set implementation, our study contributes insights on what can be expected through modifications of existing order sets based on care pathways. Fourth, the analysis accounted for a variety of variables including the CCI. Of interest, our study found that the intervention group (patients for whom order sets were used) had a lower CCI, confirming Allen et al.'s findings that diseases with predictable trajectories are the most likely to benefit from care pathways.[4] As a final strength, the narrative‐based order set intervention was relatively simple, and the inclusion criteria were broad, making the process generalizable.
Limitations of this study include that it was a single center pre‐/postintervention study and not a randomized controlled trial. Related to this limitation, the control group for which order sets were not used reflected a different patient population compared to the intervention group for which order sets were used. Specifically, it was more common for order sets to be used in the EDOU than upon admission, resulting in the order set group consisting of patients with less comorbidities than patients in the nonorder set group. Additionally, patients in the order set intervention group were older than in the baseline group (48.0 vs 44.2 years). However, these differences in population remained relatively stable before and after the intervention, and relevant variables including demographic factors and CCI were accounted for in the regression models. Nevertheless, it remains possible that secular trends existed that we did not capture that affected the 2 populations differently. For example, there was a separate project that overlapped with the intervention period to reduce unnecessary laboratory usage at UUHC. This intervention could have influenced the trend to decreased laboratory utilization in the postintervention period. However, there were no concurrent initiatives to reduce antibiotic use during the study period. As a final limitation, the statistical analyses have not corrected for multiple testing for the secondary outcomes.
CONCLUSION
Using benchmark data from UHC, an academic medical center was able to identify an opportunity for improving the care of patients with cellulitis and subsequently develop an evidence‐based care pathway. The implementation of this pathway correlated with a significant reduction of broad‐spectrum antibiotic use, pharmacy costs, and total facility costs without adverse clinical affects. An important factor in the success of the intervention was the use of electronic order sets for cellulitis, which provided support for the implementation of the care pathway. This study demonstrates that the intervention was not only effective overall, but that it was more effective for those patients for whom the order set was used. This study adds to the growing body of literature suggesting that a well‐defined care pathway can improve outcomes and reduce costs for patients and institutions.
Acknowledgements
The authors thank Ms. Pam Proctor for her assistance in implementation of the care pathway and Ms. Selma Lopez for her editorial assistance.
Disclosures: K.K. is or has been a consultant on clinical decision support (CDS) or electronic clinical quality measurement to the US Office of the National Coordinator for Health Information Technology, ARUP Laboratories, McKesson InterQual, ESAC, Inc., JBS International, Inc., Inflexxion, Inc., Intelligent Automation, Inc., Partners HealthCare, Mayo Clinic, and the RAND Corporation. K.K. receives royalties for a Duke Universityowned CDS technology for infectious disease management known as CustomID that he helped develop. K.K. was formerly a consultant for Religent, Inc. and a co‐owner and consultant for Clinica Software, Inc., both of which provide commercial CDS services, including through use of a CDS technology known as SEBASTIAN that K.K. developed. K.K. no longer has a financial relationship with either Religent or Clinica Software. K.K. has no competing interest with any specific product or intervention evaluated in this manuscript. All other authors declare no competing interests.
- Cellulitis. N Engl J Med. 2004;350(9):904–912. .
- Cost‐effectiveness of blood cultures for adult patients with cellulitis. Clin Infect Dis. 1999;29(6):1483–1488. , , , , , .
- Methicillin‐resistant s. aureus infectious among patients in the emergency department. N Engl J Med. 2006;355:666–674. , , , et al.
- Systematic review of the effectiveness of integrated care pathways: what works, for whom, in which circumstances? Int J Evid Based Healthc. 2009;7:61–74. , , .
- Decreased antibiotic utilization after implementation of a guideline for inpatient cellulitis and cutaneous abscess. Arch Intern Med. 2011;171(12):1072–1079. .
- Practice guidelines for the diagnosis and management of skin and soft‐tissue infections. Clin Infect Dis. 2005;41:1373–1406. , , , et al.
- Clinical practice guidelines by the Infectious Disease Society of American for the treatment of methicillin‐resistant Staphylococcus aureus infectious in adults and children. Clin Infect Dis. 2011;42:1–38. , , , et al.
- The role of b‐hemolytic streptococci in causing diffuse, nonculturable cellulitis. Medicine. 2010;89:217–226. , , , .
- Practice guidelines for the diagnosis and management of skin and soft tissue infections: 2014 update by the Infectious Disease Society of America. Clin Infect Dis. 2014;59(2):147–159. , , , et al.
- Value driven outcomes (VDO): a pragmatic, modular, and extensible software framework for understanding and improving health care costs and outcomes. J Am Med Inform Assoc. 2015;22(1):223–235. , , , et al.
- Coding algorithms for defining comorbidities in ICD‐9‐CM and ICD‐10 administrative data. Med Care. 2005;43:113–1139. , , , et al.
- Computerization of workflows, guidelines, and care pathways: a review of implementation challenges for process‐oriented health information systems. J Am Med Inform Assoc. 2011;18:738–748. , .
- Standardized clinical assessment and management plans (SCAMPs) provide a better alternative to clinical practice guidelines. Health Aff (Millwood) 2013;32(5):911–920. , , , et al.
- Clinical practice guidelines for clostridium difficile infection in adults: 2010 update by the Society for Healthcare Epidemiology of America (SHEA) and Infectious Diseases Society of America (IDSA). Infect Control Hosp Epidemiol. 2010;31:431–455. , , , et al.
Cellulitis is a common infection causing inflammation of the skin and subcutaneous tissues. Cellulitis has been attributed to gram‐positive organisms through historical evaluations including fine‐needle aspirates and punch biopsies of the infected tissue.[1] Neither of these diagnostic tests is currently used due to their invasiveness, poor diagnostic yield, and availability. Similarly, readily available tests such as blood cultures provide an etiology <5% of the time[1] and are not cost‐effective for most patients for diagnosing cellulitis.[2] In addition, the prevalence of methicillin‐resistant Staphylococcus aureus (MRSA) has steadily increased, complicating decisions about antibiotic selection.[3] The result of this uncertainty is a large variation in practice with respect to antibiotic and imaging selection for patients with a diagnosis of cellulitis.
University of Utah Health Care (UUHC) performed benchmarking for the management of cellulitis using the University HealthSystem Consortium (UHC) database and associated CareFx analytics tool. Benchmarking demonstrated that UUHC had a greater percentage of broad‐spectrum antibiotic use (defined as vancomycin, piperacillin/tazobactam, or carbapenems) than the top 5 performing UHC facilities for International Classification of Diseases, Ninth Revision, Clinical Modification (ICD‐9‐CM) diagnoses of cellulitis (vancomycin 83% vs 58% and carbapenem or piperacillin/tazobactam 44% vs 16%). Advanced imaging (computed tomography [CT] or magnetic resonance imaging [MRI]) for the diagnosis of cellulitis was also found to be an opportunity for improvement (CT 27% vs 20% and MRI 8% vs 5%). The hospitalist group (most patients admitted with cellulitis were on this service) believed these data reflected current practice, as there was no standard of treatment for cellulitis despite an active order set. Therefore, cellulitis was considered an opportunity to improve value to our patients. A standardized clinical care pathway was created, as such pathways have demonstrated a reduction in variation in practice and improved efficiency and effectiveness of care for multiple disease states including cellulitis.[4, 5] We hypothesized that implementation of an evidence‐based care pathway would decrease broad‐spectrum antibiotic use, cost, and use of advanced imaging without having any adverse effects on clinical outcomes such as length of stay (LOS) or readmission.
METHODS
Study Setting and Population
UUHC is a 500‐bed academic medical center in Salt Lake City, Utah. All patients admitted to the emergency department observation unit (EDOU) or the hospital with a primary ICD‐9‐CM diagnosis of cellulitis between July 1, 2011 and December 31, 2013 were evaluated.
Intervention
Initial steps involved the formation of a multidisciplinary team including key stakeholders from the hospitalist group, infectious diseases, the emergency department (ED), and nursing. This multidisciplinary team was charged with developing a clinical care pathway appropriate for local implementation. National guidance for the care pathway was mainly obtained from the Infectious Disease Society of America (IDSA) guidelines on skin and soft tissue infections (SSTIs)[6] and MRSA.[7] Specific attention was paid to recommendations on blood cultures (only when systemically ill), imaging (rarely needed), antibiotic selection (rarely gram‐negative coverage and consideration of MRSA coverage), and patient‐care principles that are often overlooked (elevation of the affected extremity). A distinction of purulent versus nonpurulent cellulitis was adopted based on the guidelines and a prospective evaluation of the care of patients with nonpurulent cellulitis.[8] The 2014 IDSA update on SSTIs incorporates this distinction more clearly in hopes of determining staphylococcal versus streptococcal infections.[9] After multiple iterations, an agreed‐upon care pathway was created that excluded patients with neutropenia, osteomyelitis, diabetic foot ulcerations; hand, perineal, periorbital, or surgical site infections; and human or animal bites (Figure 1). After the care pathway was determined, interventions were performed to implement this change.
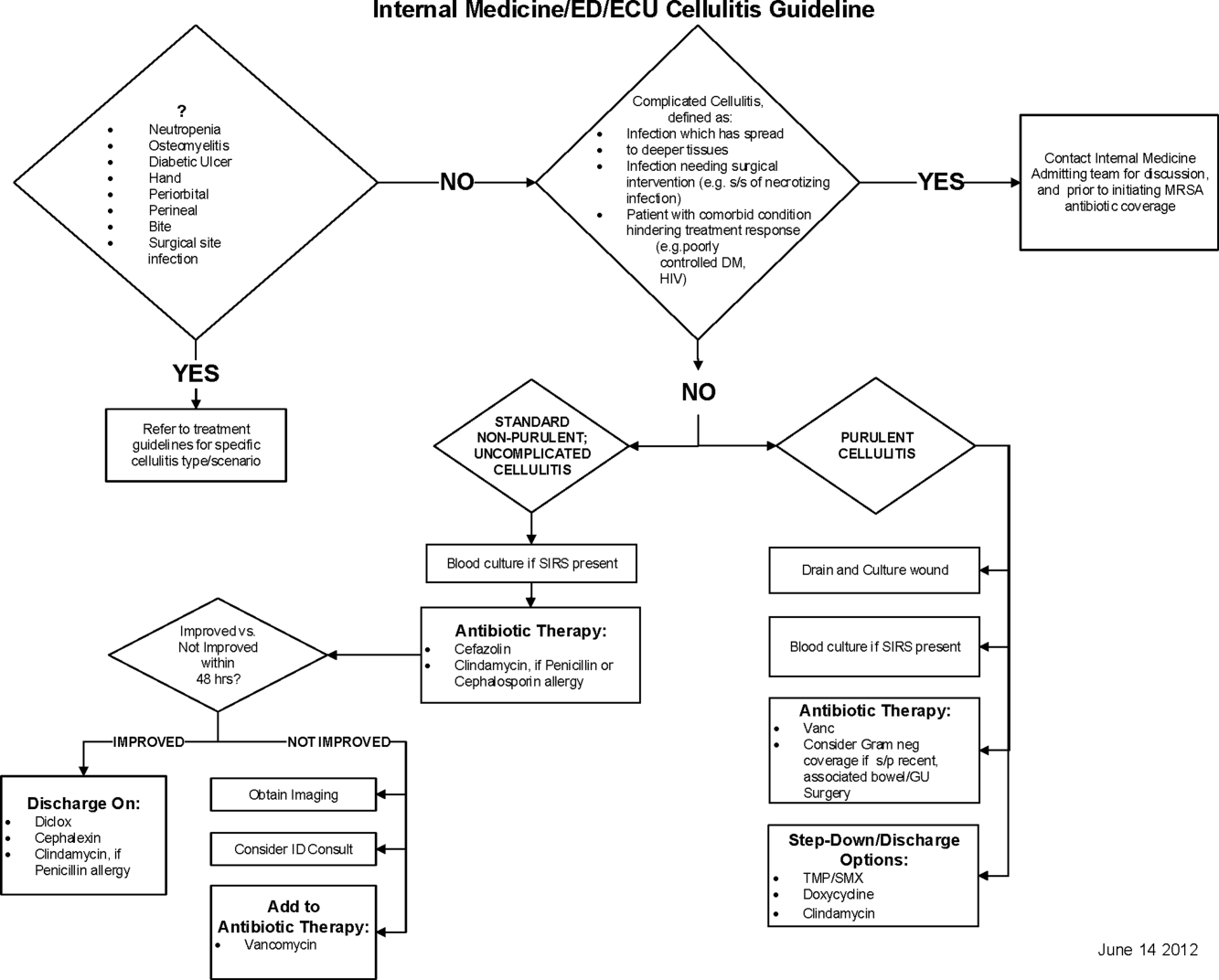
Education of all providers involved included discussion of cellulitis as a disease process, presentation of benchmarking data, dissemination of the care pathway to hospitalist and ED physicians, teaching conferences for internal medicine residents and ED residents, and reinforcement of these concepts at the beginning of resident rotations.
Incorporation of the care pathway into the existing electronic order sets for cellulitis care in the inpatient and ED settings, with links to the care pathway, links to excluded disease processes (eg, hand cellulitis), preselection of commonly needed items (eg, elevate leg), and recommendations for antibiotic selection based on categories of purulent or nonpurulent cellulitis. The electronic health record (EHR) did not allow for forced order set usage, so the order set required selection by the admitting physician if indicated. Additionally, an embedded 48‐hour order set could be accessed at any time by the ordering physician and included vancomycin dosing. Specific changes to the preexisting order set included the development of sections for purulent and nonpurulent cellulitis as well as recommended antibiotics. Piperacillin/tazobactam and nafcillin were both removed and vancomycin was limited to the purulent subheading. Additionally, elevation of the extremity was preselected, and orderables for imaging (chest x‐ray and duplex ultrasound), antiulcer prophylaxis, telemetry, and electrocardiograph were all removed.
Audit and feedback of cases of cellulitis and broad‐spectrum antibiotic usage was performed by a senior hospitalist.
Study Design
A retrospective before/after study was performed to assess overall impact of the intervention on the patient population. Additionally, a retrospective controlled pre‐/postintervention study was performed to compare changes in cellulitis management for visits where order sets were used with visits where order sets were not used. The intervention initiation date was July 9, 2012. The institutional review board classified this project as quality improvement and did not require review and oversight.
Study Population
We analyzed 2278 ED and inpatient visits for cellulitis, of which 677 met inclusion criteria. We partitioned visits into 2 groups: (1) those for which order sets were used (n = 370) and (2) control visits for which order sets were not used (n = 307). We analyzed outcomes for 2 subpopulations: hospitalized patients for whom the EDOU or admission order sets were used (n = 149) and patients not admitted and only seen in the EDOU for whom the EDOU order set was used (n = 262).
Inclusion Criteria
Inclusion criteria included hospital admission or admission to the EDOU between July 1, 2011 and December 31, 2013, age greater or equal to 18 years, and primary diagnosis of cellulitis as determined by ICD‐9‐CM billing codes 035, 457.2, 681, 681.0, 681.00, 681.01, 681.02, 681.1, 681.10, 681.11, 681.9, 680, 680.0‐9, 682.0‐9, 684, 685.0, 685.1, 686.00, 686.01, 686.09, 686.1, 686.8, 686.9, 910.1, 910.5, 910.7, 910.9, 911.1, 911.3, 911.5, 911.7, 911.9, 912.1, 912.3, 912.5, 912.7, 912.9, 913.1, 913.3, 913.5, 913.7, 913.9, 914.1, 914.3, 914.5, 914.7, 914.9, 915.1, 915.3, 915.5, 915.7, 915.9, 916.1, 916.3, 916.5, 916.7, 916.9, 917.1, 917.3, 917.5, 917.7, 917.9, 919.1, 919.3, 919.5, 919.7, or 919.9.
Data Collection and Preparation
Clinical data were collected in the inpatient EHR (Cerner Corp., Kansas City, MO) and later imported into the enterprise data warehouse (EDW) as part of the normal data flow. Billing data were imported into the EDW from the billing system. Cost data were estimated using the value‐driven outcomes (VDO) tool developed by the University of Utah to identify clinical costs to the UUHC system.[10] All data were extracted from the EDW on September 10, 2014.
Process Metrics, Clinical, and Cost Outcomes
We defined 1 primary outcome (use of broad‐spectrum antibiotics) and 8 secondary outcomes, including process metrics (MRI and CT orders), clinical outcomes (LOS and 30‐day readmissions), and cost outcomes (pharmacy, lab, imaging cost from radiology department, and total facility cost). Broad‐spectrum antibiotics were defined as any use of meropenem (UUHC's carbapenem), piperacillin/tazobactam, or vancomycin and were determined by orders. Thirty‐day readmissions included only inpatient encounters with the primary diagnosis of cellulitis.
Covariates
To control for patient demographics we included age at admission in years and gender into the statistical model. To control for background health state as well as cellulitis severity, we included Charlson Comorbidity Index (CCI) and hospitalization status. CCI was calculated according to the algorithm specified by Quan et al.[11]
Study Hypotheses
First, for all patients, we hypothesized that process metrics as well as clinical and cost outcomes would improve following the implementation of the care pathway. To evaluate this hypothesis, we estimated impact of the time interval (pre‐/postintervention) on all outcomes. Second, we hypothesized that among patients for whom order sets were used (which we deemed to be a proxy for following the agreed‐upon care pathway), there would be a greater improvement than in patients for whom order sets were not used. To evaluate this hypothesis, we estimated interactions between order set use and time period (pre‐/postintervention) for all outcomes.
Statistical Analysis
The variable time period was created to represent the time period before and after the intervention.
We provided unadjusted descriptive statistics for study outcomes and visit characteristics for all patients before and after intervention. Descriptive statistics were expressed as n (%) and mean standard deviation. Simple comparisons were performed based on 2 test of homogeneity for categorical variables and t test or Wilcoxon test for continuous variables.
For before/after analysis, we fitted generalized linear regression models to estimate the change in outcomes of interest before and after intervention for all patients simultaneously. Generalized linear model defined by a binomial distributional assumption and logit link function was used to estimate the effect of the intervention on antibiotic use, imaging orders, and readmission adjusting for effects of age, gender, CCI, and hospitalization status. A generalized linear model defined by a gamma distributional assumption and log link function was used to estimate effect of the intervention on clinical LOS and cost outcomes adjusting for the effects of the same covariates. Generalized linear models with gamma distributional assumptions were used because they are known to perform well even for zero‐inflated semicontinuous cost variables and are easier to interpret than 2‐part models.
For the controlled before/after analysis, the variable order set used was created to represent groups where order sets were used or not used. Similarly, generalized linear models were used to estimate differential effect of the intervention at 2 different order set use levels using an interaction term between order set use and the time period.
P values <0.05 were considered significant. We used SAS version 9.3 statistical software (SAS Institute Inc., Cary, NC) for data analysis.
RESULTS
Descriptive Characteristics
Patient characteristics before and after intervention for 677 EDOU and inpatient visits for cellulitis by 618 patients are summarized in the first 4 columns of Table 1. Patient age at admission ranged from 18 to 98 years. Thirty‐eight percent of visits were by female patients. There were 274 visits before the intervention and 403 visits after. Four hundred thirty‐two (64%) were admitted, and 295 (44%) were seen in the EDOU. The admission order set alone was used for 104 visits, the EDOU order set alone was used for 242 visits, and both order sets were used for 24 visits.
Characteristic | Overall | Order Sets Not Used | Order Sets Used | ||||||
---|---|---|---|---|---|---|---|---|---|
Baseline, N = 274 | Intervention, N = 403 | P* | Baseline, N = 127 | Intervention, N = 180 | P* | Baseline, N = 147 | Intervention, N = 223 | P* | |
| |||||||||
Patient Characteristics | |||||||||
Age, y | 46.8 16.0 | 48.9 17.1 | 0.097 | 49.8 16.0 | 5.1 16.3 | 0.88 | 44.2 15.5 | 48.0 17.6 | 0.032 |
Female gender | 105 (38%) | 155 (39%) | 0.93 | 50 (39%) | 74 (41%) | 0.73 | 55 (37%) | 81 (36%) | 0.86 |
CCI | 2.6 3.2 | 2.6 3.0 | 0.69 | 3.2 3.5 | 3.2 3.2 | 0.82 | 2.0 2.8 | 2.1 2.7 | 0.68 |
Clinical process characteristics | |||||||||
EDOU admission | 122 (45%) | 173 (43%) | 0.68 | 12 (9%) | 19 (11%) | 0.75 | 110 (75%) | 154 (69%) | 0.23 |
Hospital admission | 173 (63%) | 259 (64%) | 0.76 | 117 (92%) | 166 (92%) | 0.98 | 56 (38%) | 93 (42%) | 0.49 |
EDOU order set used | 111 (41%) | 155 (38%) | 0.59 | NA | NA | NA | 111 (76%) | 155 (70%) | 0.21 |
ADM order set used | 47 (17%) | 81 (20%) | 0.34 | NA | NA | NA | 47 (32%) | 81 (36%) | 0.39 |
Process outcomes | |||||||||
Broad‐spectrum antibiotics used | 205 (75%) | 230 (57%) | <0.001 | 90 (71%) | 121 (67%) | 0.50 | 115 (78%) | 109 (49%) | <0.001 |
MRI done | 27 (10%) | 32 (8%) | 0.39 | 13 (10%) | 20 (11%) | 0.81 | 14 (10%) | 12 (5%) | 0.13 |
CT done | 56 (20%) | 76 (19%) | 0.61 | 32 (25%) | 43 (24%) | 0.79 | 24 (16%) | 33 (15%) | 0.69 |
Clinical outcomes | |||||||||
Length of stay, d | 2.7 2.6 | 2.6 2.8 | 0.35 | 3.6 2.8 | 3.8 3.4 | 0.62 | 2.0 2.1 | 1.7 1.6 | 0.48 |
30‐day readmission | 14 (5%) | 17 (4%) | 0.59 | 7 (6%) | 9 (5%) | 0.84 | 7 (5%) | 8 (4%) | 0.58 |
Cost outcomes | |||||||||
Pharmacy cost ($) | 1 | 0.76 | 0.002 | 1 | 0.89 | 0.13 | 1 | 0.56 | 0.004 |
Lab cost ($) | 1 | 0.52 | <0.001 | 1 | 0.53 | 0.001 | 1 | 0.51 | 0.055 |
Imaging cost ($) | 1 | 0.82 | 0.11 | 1 | 0.95 | 0.52 | 1 | 0.67 | 0.13 |
Total facility cost ($) | 1 | 0.85 | 0.027 | 1 | 0.91 | 0.042 | 1 | 0.77 | 0.26 |
Before/After Analysis
Among all patients, use of broad‐spectrum antibiotics decreased from 75% to 57% (Table 1). Analysis adjusted for gender, age at admission, CCI, and hospital admission status is provided in Table 2. Overall, there was a 59% decrease in the odds of ordering broad‐spectrum antibiotics (P < 0.001), a 23% decrease in pharmacy cost (P = 0.002), a 44% decrease in laboratory cost (P < 0.001), and a 13% decrease in total facility cost (P = 0.006).
Logistic Regression | ||||
---|---|---|---|---|
Outcome Variables | Selected Predictor Variables | Odds* | Percent Change | P |
Gamma Regression | ||||
Outcome Variables | Selected Predictor Variables | Fold Change* | Percent Change | P |
| ||||
Antibiotics used | Time period | 0.41 (0.29, 0.59) | 59% (71% to 41%) | <0.001 |
MRI done | Time period | 0.74 (0.43, 1.30) | 26% (57% to 30%) | 0.29 |
CT done | Time period | 0.92 (0.62, 1.36) | 8% (38% to 36%) | 0.67 |
30‐day readmission | Time period | 0.86 (0.41, 1.80) | 14% (59% to 80%) | 0.69 |
Length of stay, d | Time period | 0.97 (0.91, 1.03) | 3% (9% to 3%) | 0.34 |
Pharmacy cost ($) | Time period | 0.77 (0.65, 0.91) | 23% (35% to 9%) | 0.002 |
Lab cost ($) | Time period | 0.56 (0.48, 0.65) | 44% (52% to 35%) | <0.001 |
Imaging cost($) | Time period | 0.90 (0.71, 1.14) | 10% (29% to 14%) | 0.38 |
Total facility cost ($) | Time Period | 0.87 (0.79, 0.96) | 13% (21% to 4%) | 0.006 |
Order Set Use Groups Analysis
Descriptive statistics and simple comparison before/after the intervention for the 2 study groups are shown in the last 6 columns of Table 1. Among patients for whom order sets were used, broad‐spectrum antibiotic usage significantly decreased from 78% before the intervention to 49% after the intervention (P < 0.001). In contrast, among patients for whom order sets were not used, broad‐spectrum antibiotic usage remained relatively constant71% before the intervention versus 67% after the intervention (P = 0.50). Figure 2 shows semiannual changes in the prescription of broad‐spectrum antibiotics. There is a noticeable drop after the intervention among patients for whom order sets were used.
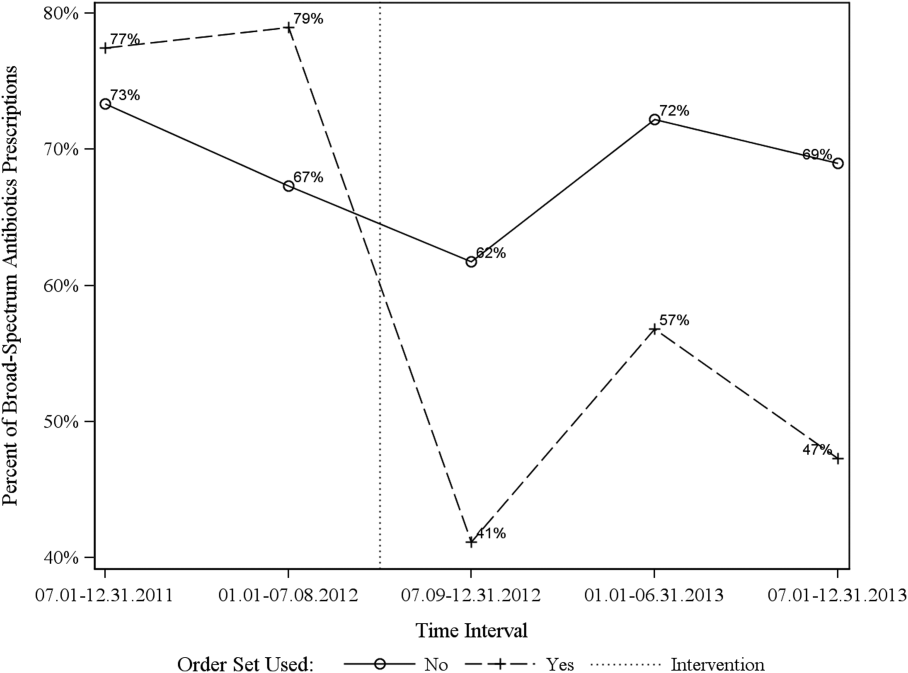
Analysis of the interaction between time period and order set usage is provided in Table 3. After the intervention, patients for whom the order sets were used had greater improvement in broad‐spectrum antibiotic selection (75% decrease, P < 0.001) and LOS (25% decrease, P = 0.041) than patients for whom order sets were not used. Pharmacy costs also decreased by 13% more among patients for whom the order sets were used, although the interaction was not statistically significant (P = 0.074). Laboratory costs decreased in both groups, but order set use did not demonstrate an interaction (P = 0.5). Similar results were found for the subgroups of admitted patients and patients seen in the EDOU.
Logistic Regression | ||||
---|---|---|---|---|
Outcome Variables | Selected Predictor Variables | Odds* | Percent Change | P |
Gamma Regression | ||||
Outcome Variables | Selected Predictor Variables | Fold Change* | Percent Change | P |
| ||||
Broad spectrum antibiotics | Time period | 0.84 (0.50, 1.40) | 16% (50% to 40%) | 0.50 |
Time periodorder set | 0.25 (0.12, 0.52) | 75% (88% to 48%) | <0.001 | |
MRI done | Time period | 1.04 (0.49, 2.20) | 4% (51% to 120%) | 0.92 |
Time periodorder set | 0.44 (0.14, 1.38) | 56% (86% to 38%) | 0.16 | |
CT done | Time period | 0.94 (0.55, 1.60) | 6% (45% to 60%) | 0.81 |
Time periodorder set | 0.96 (0.44, 2.12) | 4% (56% to 112%) | 0.93 | |
30‐day readmission | Time period | 0.91 (0.33, 2.53) | 9% (67% to 153%) | 0.86 |
Time periodorder set | 0.88 (0.20, 3.93) | 12% (80% to 293%) | 0.87 | |
Clinical length of stay | Time period | 1.04 (0.95, 1.14) | 4% (5% to 14%) | 0.41 |
Time periodorder set | 0.87 (0.77, 0.99) | 13% (23% to 1%) | 0.041 | |
Pharmacy cost ($) | Time period | 0.88 (0.70, 1.12) | 12% (30% to 12%) | 0.31 |
Time periodorder set | 0.75 (0.54, 1.03) | 25% (46% to 3%) | 0.074 | |
Lab cost ($) | Time period | 0.53 (0.42, 0.66) | 47% (58% to 34%) | <0.001 |
Time periodorder set | 1.11 (0.82, 1.50) | 11% (18% to 50%) | 0.50 | |
Imaging cost ($) | Time period | 1.00 (0.71, 1.40) | 0% (29% to 40%) | 0.98 |
Time periodorder set | 0.82 (0.51, 1.30) | 18% (49% to 30%) | 0.39 | |
Facility cost ($) | Time period | 0.92 (0.80, 1.05) | 8% (20% to 5%) | 0.22 |
Time periodorder set | 0.90 (0.75, 1.09) | 10% (25% to 9%) | 0.29 |
Audit and feedback was initially performed for cases of cellulitis using broad‐spectrum antibiotics. However, given the complexity of cellulitis as a disease process and the frequency of broad‐spectrum antibiotic usage, in all cases of review, it was deemed reasonable to use broad‐spectrum antibiotics. Therefore, the audit was not continued.
DISCUSSION
Care pathways have demonstrated improvement across multiple different disease states including cellulitis.[4, 5] They have been noted to reduce variation in practice and improve physician agreement about treatment options.[4] The best method for implementation is not clearly understood,[12] and there remains concern about maintaining flexibility for patient care.[13] Additionally, although implementation of pathways is often well described, evaluations of the processes are noted to frequently be weak.[12] UUHC felt that the literature supported implementing a care pathway for the diagnosis of cellulitis, but that a thorough evaluation was also needed to understand any resulting benefits or harms. Through this study, we found that the implementation of this pathway resulted in a significant decrease in broad‐spectrum antibiotic use, pharmacy costs, and total facility costs. There was also a trend to decrease in imaging cost, and there were no adverse effects on LOS or 30‐day readmissions. Our findings demonstrate that care‐pathway implementation accompanied by education, pathway‐compliant electronic order sets, and audit and feedback can help drive improvements in quality while reducing costs. This finding furthers the evidence supporting standard work through the creation of clinical care pathways for cellulitis as an effective intervention.[4] Additionally, although not measured in this study, reduction of antibiotic use is supported as a measure to help reduce Clostridium difficile infections, a further potential benefit.[14]
This study has several important strengths. First, we included accurate cost analyses using the VDO tool. Given the increasing importance of improving care value, we feel the inclusion of such cost analysis is an increasingly important aspect of health service intervention evaluations. Second, we used a formal benchmarking approach to identify a priority care improvement area and to monitor changes in practice following the rollout of the intervention. We feel this approach provides a useful example on how to systematically improve care quality and value in a broader health system context. Third, we evaluated not order set implementation per se, but rather changing an existing order set. Because studies in this area generally focus on initial order set implementation, our study contributes insights on what can be expected through modifications of existing order sets based on care pathways. Fourth, the analysis accounted for a variety of variables including the CCI. Of interest, our study found that the intervention group (patients for whom order sets were used) had a lower CCI, confirming Allen et al.'s findings that diseases with predictable trajectories are the most likely to benefit from care pathways.[4] As a final strength, the narrative‐based order set intervention was relatively simple, and the inclusion criteria were broad, making the process generalizable.
Limitations of this study include that it was a single center pre‐/postintervention study and not a randomized controlled trial. Related to this limitation, the control group for which order sets were not used reflected a different patient population compared to the intervention group for which order sets were used. Specifically, it was more common for order sets to be used in the EDOU than upon admission, resulting in the order set group consisting of patients with less comorbidities than patients in the nonorder set group. Additionally, patients in the order set intervention group were older than in the baseline group (48.0 vs 44.2 years). However, these differences in population remained relatively stable before and after the intervention, and relevant variables including demographic factors and CCI were accounted for in the regression models. Nevertheless, it remains possible that secular trends existed that we did not capture that affected the 2 populations differently. For example, there was a separate project that overlapped with the intervention period to reduce unnecessary laboratory usage at UUHC. This intervention could have influenced the trend to decreased laboratory utilization in the postintervention period. However, there were no concurrent initiatives to reduce antibiotic use during the study period. As a final limitation, the statistical analyses have not corrected for multiple testing for the secondary outcomes.
CONCLUSION
Using benchmark data from UHC, an academic medical center was able to identify an opportunity for improving the care of patients with cellulitis and subsequently develop an evidence‐based care pathway. The implementation of this pathway correlated with a significant reduction of broad‐spectrum antibiotic use, pharmacy costs, and total facility costs without adverse clinical affects. An important factor in the success of the intervention was the use of electronic order sets for cellulitis, which provided support for the implementation of the care pathway. This study demonstrates that the intervention was not only effective overall, but that it was more effective for those patients for whom the order set was used. This study adds to the growing body of literature suggesting that a well‐defined care pathway can improve outcomes and reduce costs for patients and institutions.
Acknowledgements
The authors thank Ms. Pam Proctor for her assistance in implementation of the care pathway and Ms. Selma Lopez for her editorial assistance.
Disclosures: K.K. is or has been a consultant on clinical decision support (CDS) or electronic clinical quality measurement to the US Office of the National Coordinator for Health Information Technology, ARUP Laboratories, McKesson InterQual, ESAC, Inc., JBS International, Inc., Inflexxion, Inc., Intelligent Automation, Inc., Partners HealthCare, Mayo Clinic, and the RAND Corporation. K.K. receives royalties for a Duke Universityowned CDS technology for infectious disease management known as CustomID that he helped develop. K.K. was formerly a consultant for Religent, Inc. and a co‐owner and consultant for Clinica Software, Inc., both of which provide commercial CDS services, including through use of a CDS technology known as SEBASTIAN that K.K. developed. K.K. no longer has a financial relationship with either Religent or Clinica Software. K.K. has no competing interest with any specific product or intervention evaluated in this manuscript. All other authors declare no competing interests.
Cellulitis is a common infection causing inflammation of the skin and subcutaneous tissues. Cellulitis has been attributed to gram‐positive organisms through historical evaluations including fine‐needle aspirates and punch biopsies of the infected tissue.[1] Neither of these diagnostic tests is currently used due to their invasiveness, poor diagnostic yield, and availability. Similarly, readily available tests such as blood cultures provide an etiology <5% of the time[1] and are not cost‐effective for most patients for diagnosing cellulitis.[2] In addition, the prevalence of methicillin‐resistant Staphylococcus aureus (MRSA) has steadily increased, complicating decisions about antibiotic selection.[3] The result of this uncertainty is a large variation in practice with respect to antibiotic and imaging selection for patients with a diagnosis of cellulitis.
University of Utah Health Care (UUHC) performed benchmarking for the management of cellulitis using the University HealthSystem Consortium (UHC) database and associated CareFx analytics tool. Benchmarking demonstrated that UUHC had a greater percentage of broad‐spectrum antibiotic use (defined as vancomycin, piperacillin/tazobactam, or carbapenems) than the top 5 performing UHC facilities for International Classification of Diseases, Ninth Revision, Clinical Modification (ICD‐9‐CM) diagnoses of cellulitis (vancomycin 83% vs 58% and carbapenem or piperacillin/tazobactam 44% vs 16%). Advanced imaging (computed tomography [CT] or magnetic resonance imaging [MRI]) for the diagnosis of cellulitis was also found to be an opportunity for improvement (CT 27% vs 20% and MRI 8% vs 5%). The hospitalist group (most patients admitted with cellulitis were on this service) believed these data reflected current practice, as there was no standard of treatment for cellulitis despite an active order set. Therefore, cellulitis was considered an opportunity to improve value to our patients. A standardized clinical care pathway was created, as such pathways have demonstrated a reduction in variation in practice and improved efficiency and effectiveness of care for multiple disease states including cellulitis.[4, 5] We hypothesized that implementation of an evidence‐based care pathway would decrease broad‐spectrum antibiotic use, cost, and use of advanced imaging without having any adverse effects on clinical outcomes such as length of stay (LOS) or readmission.
METHODS
Study Setting and Population
UUHC is a 500‐bed academic medical center in Salt Lake City, Utah. All patients admitted to the emergency department observation unit (EDOU) or the hospital with a primary ICD‐9‐CM diagnosis of cellulitis between July 1, 2011 and December 31, 2013 were evaluated.
Intervention
Initial steps involved the formation of a multidisciplinary team including key stakeholders from the hospitalist group, infectious diseases, the emergency department (ED), and nursing. This multidisciplinary team was charged with developing a clinical care pathway appropriate for local implementation. National guidance for the care pathway was mainly obtained from the Infectious Disease Society of America (IDSA) guidelines on skin and soft tissue infections (SSTIs)[6] and MRSA.[7] Specific attention was paid to recommendations on blood cultures (only when systemically ill), imaging (rarely needed), antibiotic selection (rarely gram‐negative coverage and consideration of MRSA coverage), and patient‐care principles that are often overlooked (elevation of the affected extremity). A distinction of purulent versus nonpurulent cellulitis was adopted based on the guidelines and a prospective evaluation of the care of patients with nonpurulent cellulitis.[8] The 2014 IDSA update on SSTIs incorporates this distinction more clearly in hopes of determining staphylococcal versus streptococcal infections.[9] After multiple iterations, an agreed‐upon care pathway was created that excluded patients with neutropenia, osteomyelitis, diabetic foot ulcerations; hand, perineal, periorbital, or surgical site infections; and human or animal bites (Figure 1). After the care pathway was determined, interventions were performed to implement this change.
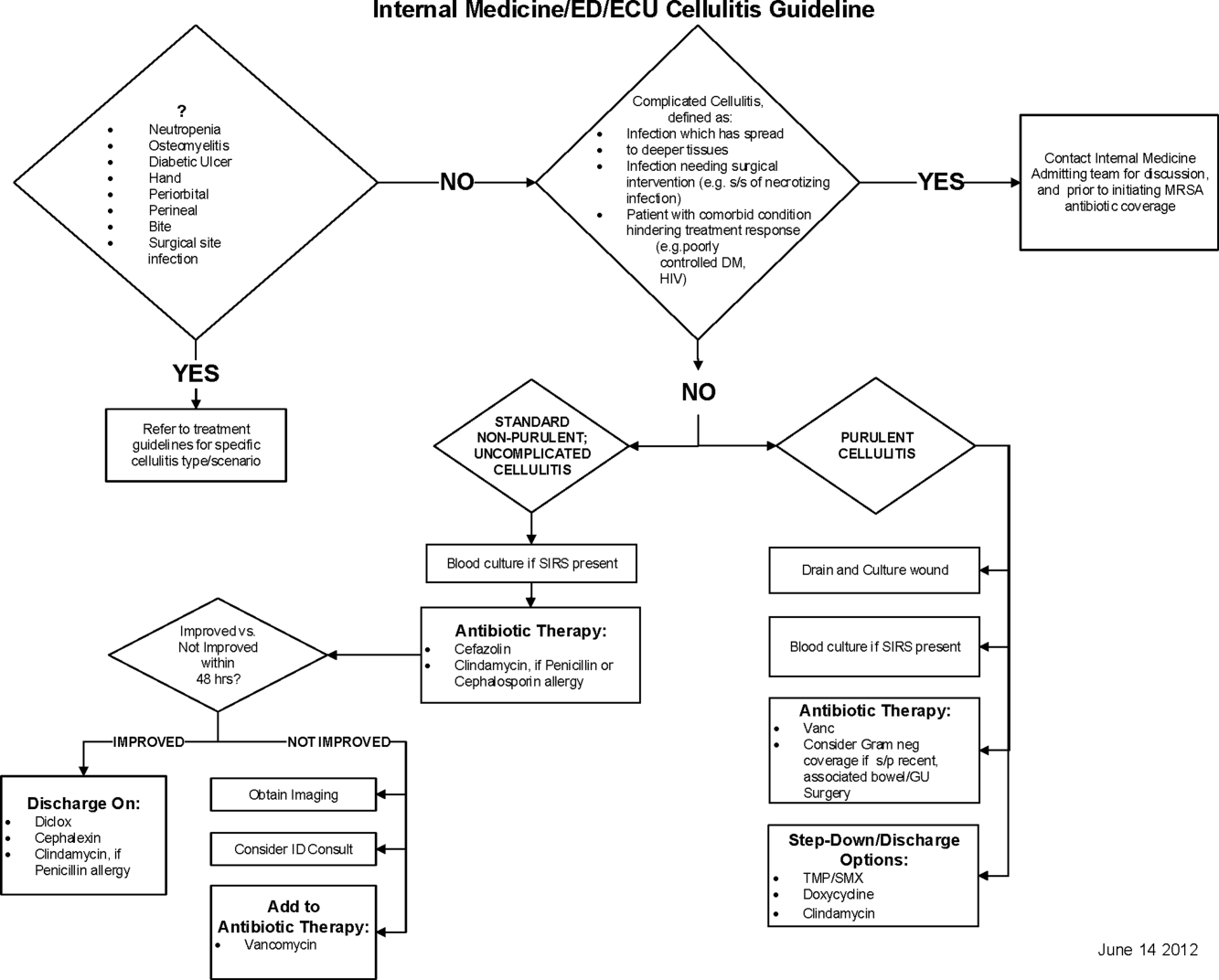
Education of all providers involved included discussion of cellulitis as a disease process, presentation of benchmarking data, dissemination of the care pathway to hospitalist and ED physicians, teaching conferences for internal medicine residents and ED residents, and reinforcement of these concepts at the beginning of resident rotations.
Incorporation of the care pathway into the existing electronic order sets for cellulitis care in the inpatient and ED settings, with links to the care pathway, links to excluded disease processes (eg, hand cellulitis), preselection of commonly needed items (eg, elevate leg), and recommendations for antibiotic selection based on categories of purulent or nonpurulent cellulitis. The electronic health record (EHR) did not allow for forced order set usage, so the order set required selection by the admitting physician if indicated. Additionally, an embedded 48‐hour order set could be accessed at any time by the ordering physician and included vancomycin dosing. Specific changes to the preexisting order set included the development of sections for purulent and nonpurulent cellulitis as well as recommended antibiotics. Piperacillin/tazobactam and nafcillin were both removed and vancomycin was limited to the purulent subheading. Additionally, elevation of the extremity was preselected, and orderables for imaging (chest x‐ray and duplex ultrasound), antiulcer prophylaxis, telemetry, and electrocardiograph were all removed.
Audit and feedback of cases of cellulitis and broad‐spectrum antibiotic usage was performed by a senior hospitalist.
Study Design
A retrospective before/after study was performed to assess overall impact of the intervention on the patient population. Additionally, a retrospective controlled pre‐/postintervention study was performed to compare changes in cellulitis management for visits where order sets were used with visits where order sets were not used. The intervention initiation date was July 9, 2012. The institutional review board classified this project as quality improvement and did not require review and oversight.
Study Population
We analyzed 2278 ED and inpatient visits for cellulitis, of which 677 met inclusion criteria. We partitioned visits into 2 groups: (1) those for which order sets were used (n = 370) and (2) control visits for which order sets were not used (n = 307). We analyzed outcomes for 2 subpopulations: hospitalized patients for whom the EDOU or admission order sets were used (n = 149) and patients not admitted and only seen in the EDOU for whom the EDOU order set was used (n = 262).
Inclusion Criteria
Inclusion criteria included hospital admission or admission to the EDOU between July 1, 2011 and December 31, 2013, age greater or equal to 18 years, and primary diagnosis of cellulitis as determined by ICD‐9‐CM billing codes 035, 457.2, 681, 681.0, 681.00, 681.01, 681.02, 681.1, 681.10, 681.11, 681.9, 680, 680.0‐9, 682.0‐9, 684, 685.0, 685.1, 686.00, 686.01, 686.09, 686.1, 686.8, 686.9, 910.1, 910.5, 910.7, 910.9, 911.1, 911.3, 911.5, 911.7, 911.9, 912.1, 912.3, 912.5, 912.7, 912.9, 913.1, 913.3, 913.5, 913.7, 913.9, 914.1, 914.3, 914.5, 914.7, 914.9, 915.1, 915.3, 915.5, 915.7, 915.9, 916.1, 916.3, 916.5, 916.7, 916.9, 917.1, 917.3, 917.5, 917.7, 917.9, 919.1, 919.3, 919.5, 919.7, or 919.9.
Data Collection and Preparation
Clinical data were collected in the inpatient EHR (Cerner Corp., Kansas City, MO) and later imported into the enterprise data warehouse (EDW) as part of the normal data flow. Billing data were imported into the EDW from the billing system. Cost data were estimated using the value‐driven outcomes (VDO) tool developed by the University of Utah to identify clinical costs to the UUHC system.[10] All data were extracted from the EDW on September 10, 2014.
Process Metrics, Clinical, and Cost Outcomes
We defined 1 primary outcome (use of broad‐spectrum antibiotics) and 8 secondary outcomes, including process metrics (MRI and CT orders), clinical outcomes (LOS and 30‐day readmissions), and cost outcomes (pharmacy, lab, imaging cost from radiology department, and total facility cost). Broad‐spectrum antibiotics were defined as any use of meropenem (UUHC's carbapenem), piperacillin/tazobactam, or vancomycin and were determined by orders. Thirty‐day readmissions included only inpatient encounters with the primary diagnosis of cellulitis.
Covariates
To control for patient demographics we included age at admission in years and gender into the statistical model. To control for background health state as well as cellulitis severity, we included Charlson Comorbidity Index (CCI) and hospitalization status. CCI was calculated according to the algorithm specified by Quan et al.[11]
Study Hypotheses
First, for all patients, we hypothesized that process metrics as well as clinical and cost outcomes would improve following the implementation of the care pathway. To evaluate this hypothesis, we estimated impact of the time interval (pre‐/postintervention) on all outcomes. Second, we hypothesized that among patients for whom order sets were used (which we deemed to be a proxy for following the agreed‐upon care pathway), there would be a greater improvement than in patients for whom order sets were not used. To evaluate this hypothesis, we estimated interactions between order set use and time period (pre‐/postintervention) for all outcomes.
Statistical Analysis
The variable time period was created to represent the time period before and after the intervention.
We provided unadjusted descriptive statistics for study outcomes and visit characteristics for all patients before and after intervention. Descriptive statistics were expressed as n (%) and mean standard deviation. Simple comparisons were performed based on 2 test of homogeneity for categorical variables and t test or Wilcoxon test for continuous variables.
For before/after analysis, we fitted generalized linear regression models to estimate the change in outcomes of interest before and after intervention for all patients simultaneously. Generalized linear model defined by a binomial distributional assumption and logit link function was used to estimate the effect of the intervention on antibiotic use, imaging orders, and readmission adjusting for effects of age, gender, CCI, and hospitalization status. A generalized linear model defined by a gamma distributional assumption and log link function was used to estimate effect of the intervention on clinical LOS and cost outcomes adjusting for the effects of the same covariates. Generalized linear models with gamma distributional assumptions were used because they are known to perform well even for zero‐inflated semicontinuous cost variables and are easier to interpret than 2‐part models.
For the controlled before/after analysis, the variable order set used was created to represent groups where order sets were used or not used. Similarly, generalized linear models were used to estimate differential effect of the intervention at 2 different order set use levels using an interaction term between order set use and the time period.
P values <0.05 were considered significant. We used SAS version 9.3 statistical software (SAS Institute Inc., Cary, NC) for data analysis.
RESULTS
Descriptive Characteristics
Patient characteristics before and after intervention for 677 EDOU and inpatient visits for cellulitis by 618 patients are summarized in the first 4 columns of Table 1. Patient age at admission ranged from 18 to 98 years. Thirty‐eight percent of visits were by female patients. There were 274 visits before the intervention and 403 visits after. Four hundred thirty‐two (64%) were admitted, and 295 (44%) were seen in the EDOU. The admission order set alone was used for 104 visits, the EDOU order set alone was used for 242 visits, and both order sets were used for 24 visits.
Characteristic | Overall | Order Sets Not Used | Order Sets Used | ||||||
---|---|---|---|---|---|---|---|---|---|
Baseline, N = 274 | Intervention, N = 403 | P* | Baseline, N = 127 | Intervention, N = 180 | P* | Baseline, N = 147 | Intervention, N = 223 | P* | |
| |||||||||
Patient Characteristics | |||||||||
Age, y | 46.8 16.0 | 48.9 17.1 | 0.097 | 49.8 16.0 | 5.1 16.3 | 0.88 | 44.2 15.5 | 48.0 17.6 | 0.032 |
Female gender | 105 (38%) | 155 (39%) | 0.93 | 50 (39%) | 74 (41%) | 0.73 | 55 (37%) | 81 (36%) | 0.86 |
CCI | 2.6 3.2 | 2.6 3.0 | 0.69 | 3.2 3.5 | 3.2 3.2 | 0.82 | 2.0 2.8 | 2.1 2.7 | 0.68 |
Clinical process characteristics | |||||||||
EDOU admission | 122 (45%) | 173 (43%) | 0.68 | 12 (9%) | 19 (11%) | 0.75 | 110 (75%) | 154 (69%) | 0.23 |
Hospital admission | 173 (63%) | 259 (64%) | 0.76 | 117 (92%) | 166 (92%) | 0.98 | 56 (38%) | 93 (42%) | 0.49 |
EDOU order set used | 111 (41%) | 155 (38%) | 0.59 | NA | NA | NA | 111 (76%) | 155 (70%) | 0.21 |
ADM order set used | 47 (17%) | 81 (20%) | 0.34 | NA | NA | NA | 47 (32%) | 81 (36%) | 0.39 |
Process outcomes | |||||||||
Broad‐spectrum antibiotics used | 205 (75%) | 230 (57%) | <0.001 | 90 (71%) | 121 (67%) | 0.50 | 115 (78%) | 109 (49%) | <0.001 |
MRI done | 27 (10%) | 32 (8%) | 0.39 | 13 (10%) | 20 (11%) | 0.81 | 14 (10%) | 12 (5%) | 0.13 |
CT done | 56 (20%) | 76 (19%) | 0.61 | 32 (25%) | 43 (24%) | 0.79 | 24 (16%) | 33 (15%) | 0.69 |
Clinical outcomes | |||||||||
Length of stay, d | 2.7 2.6 | 2.6 2.8 | 0.35 | 3.6 2.8 | 3.8 3.4 | 0.62 | 2.0 2.1 | 1.7 1.6 | 0.48 |
30‐day readmission | 14 (5%) | 17 (4%) | 0.59 | 7 (6%) | 9 (5%) | 0.84 | 7 (5%) | 8 (4%) | 0.58 |
Cost outcomes | |||||||||
Pharmacy cost ($) | 1 | 0.76 | 0.002 | 1 | 0.89 | 0.13 | 1 | 0.56 | 0.004 |
Lab cost ($) | 1 | 0.52 | <0.001 | 1 | 0.53 | 0.001 | 1 | 0.51 | 0.055 |
Imaging cost ($) | 1 | 0.82 | 0.11 | 1 | 0.95 | 0.52 | 1 | 0.67 | 0.13 |
Total facility cost ($) | 1 | 0.85 | 0.027 | 1 | 0.91 | 0.042 | 1 | 0.77 | 0.26 |
Before/After Analysis
Among all patients, use of broad‐spectrum antibiotics decreased from 75% to 57% (Table 1). Analysis adjusted for gender, age at admission, CCI, and hospital admission status is provided in Table 2. Overall, there was a 59% decrease in the odds of ordering broad‐spectrum antibiotics (P < 0.001), a 23% decrease in pharmacy cost (P = 0.002), a 44% decrease in laboratory cost (P < 0.001), and a 13% decrease in total facility cost (P = 0.006).
Logistic Regression | ||||
---|---|---|---|---|
Outcome Variables | Selected Predictor Variables | Odds* | Percent Change | P |
Gamma Regression | ||||
Outcome Variables | Selected Predictor Variables | Fold Change* | Percent Change | P |
| ||||
Antibiotics used | Time period | 0.41 (0.29, 0.59) | 59% (71% to 41%) | <0.001 |
MRI done | Time period | 0.74 (0.43, 1.30) | 26% (57% to 30%) | 0.29 |
CT done | Time period | 0.92 (0.62, 1.36) | 8% (38% to 36%) | 0.67 |
30‐day readmission | Time period | 0.86 (0.41, 1.80) | 14% (59% to 80%) | 0.69 |
Length of stay, d | Time period | 0.97 (0.91, 1.03) | 3% (9% to 3%) | 0.34 |
Pharmacy cost ($) | Time period | 0.77 (0.65, 0.91) | 23% (35% to 9%) | 0.002 |
Lab cost ($) | Time period | 0.56 (0.48, 0.65) | 44% (52% to 35%) | <0.001 |
Imaging cost($) | Time period | 0.90 (0.71, 1.14) | 10% (29% to 14%) | 0.38 |
Total facility cost ($) | Time Period | 0.87 (0.79, 0.96) | 13% (21% to 4%) | 0.006 |
Order Set Use Groups Analysis
Descriptive statistics and simple comparison before/after the intervention for the 2 study groups are shown in the last 6 columns of Table 1. Among patients for whom order sets were used, broad‐spectrum antibiotic usage significantly decreased from 78% before the intervention to 49% after the intervention (P < 0.001). In contrast, among patients for whom order sets were not used, broad‐spectrum antibiotic usage remained relatively constant71% before the intervention versus 67% after the intervention (P = 0.50). Figure 2 shows semiannual changes in the prescription of broad‐spectrum antibiotics. There is a noticeable drop after the intervention among patients for whom order sets were used.
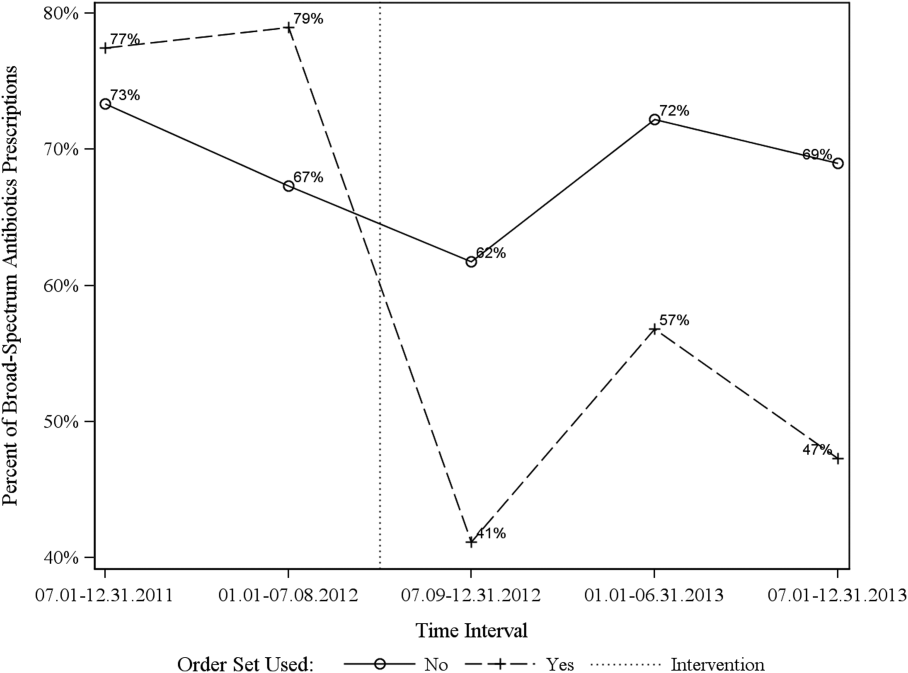
Analysis of the interaction between time period and order set usage is provided in Table 3. After the intervention, patients for whom the order sets were used had greater improvement in broad‐spectrum antibiotic selection (75% decrease, P < 0.001) and LOS (25% decrease, P = 0.041) than patients for whom order sets were not used. Pharmacy costs also decreased by 13% more among patients for whom the order sets were used, although the interaction was not statistically significant (P = 0.074). Laboratory costs decreased in both groups, but order set use did not demonstrate an interaction (P = 0.5). Similar results were found for the subgroups of admitted patients and patients seen in the EDOU.
Logistic Regression | ||||
---|---|---|---|---|
Outcome Variables | Selected Predictor Variables | Odds* | Percent Change | P |
Gamma Regression | ||||
Outcome Variables | Selected Predictor Variables | Fold Change* | Percent Change | P |
| ||||
Broad spectrum antibiotics | Time period | 0.84 (0.50, 1.40) | 16% (50% to 40%) | 0.50 |
Time periodorder set | 0.25 (0.12, 0.52) | 75% (88% to 48%) | <0.001 | |
MRI done | Time period | 1.04 (0.49, 2.20) | 4% (51% to 120%) | 0.92 |
Time periodorder set | 0.44 (0.14, 1.38) | 56% (86% to 38%) | 0.16 | |
CT done | Time period | 0.94 (0.55, 1.60) | 6% (45% to 60%) | 0.81 |
Time periodorder set | 0.96 (0.44, 2.12) | 4% (56% to 112%) | 0.93 | |
30‐day readmission | Time period | 0.91 (0.33, 2.53) | 9% (67% to 153%) | 0.86 |
Time periodorder set | 0.88 (0.20, 3.93) | 12% (80% to 293%) | 0.87 | |
Clinical length of stay | Time period | 1.04 (0.95, 1.14) | 4% (5% to 14%) | 0.41 |
Time periodorder set | 0.87 (0.77, 0.99) | 13% (23% to 1%) | 0.041 | |
Pharmacy cost ($) | Time period | 0.88 (0.70, 1.12) | 12% (30% to 12%) | 0.31 |
Time periodorder set | 0.75 (0.54, 1.03) | 25% (46% to 3%) | 0.074 | |
Lab cost ($) | Time period | 0.53 (0.42, 0.66) | 47% (58% to 34%) | <0.001 |
Time periodorder set | 1.11 (0.82, 1.50) | 11% (18% to 50%) | 0.50 | |
Imaging cost ($) | Time period | 1.00 (0.71, 1.40) | 0% (29% to 40%) | 0.98 |
Time periodorder set | 0.82 (0.51, 1.30) | 18% (49% to 30%) | 0.39 | |
Facility cost ($) | Time period | 0.92 (0.80, 1.05) | 8% (20% to 5%) | 0.22 |
Time periodorder set | 0.90 (0.75, 1.09) | 10% (25% to 9%) | 0.29 |
Audit and feedback was initially performed for cases of cellulitis using broad‐spectrum antibiotics. However, given the complexity of cellulitis as a disease process and the frequency of broad‐spectrum antibiotic usage, in all cases of review, it was deemed reasonable to use broad‐spectrum antibiotics. Therefore, the audit was not continued.
DISCUSSION
Care pathways have demonstrated improvement across multiple different disease states including cellulitis.[4, 5] They have been noted to reduce variation in practice and improve physician agreement about treatment options.[4] The best method for implementation is not clearly understood,[12] and there remains concern about maintaining flexibility for patient care.[13] Additionally, although implementation of pathways is often well described, evaluations of the processes are noted to frequently be weak.[12] UUHC felt that the literature supported implementing a care pathway for the diagnosis of cellulitis, but that a thorough evaluation was also needed to understand any resulting benefits or harms. Through this study, we found that the implementation of this pathway resulted in a significant decrease in broad‐spectrum antibiotic use, pharmacy costs, and total facility costs. There was also a trend to decrease in imaging cost, and there were no adverse effects on LOS or 30‐day readmissions. Our findings demonstrate that care‐pathway implementation accompanied by education, pathway‐compliant electronic order sets, and audit and feedback can help drive improvements in quality while reducing costs. This finding furthers the evidence supporting standard work through the creation of clinical care pathways for cellulitis as an effective intervention.[4] Additionally, although not measured in this study, reduction of antibiotic use is supported as a measure to help reduce Clostridium difficile infections, a further potential benefit.[14]
This study has several important strengths. First, we included accurate cost analyses using the VDO tool. Given the increasing importance of improving care value, we feel the inclusion of such cost analysis is an increasingly important aspect of health service intervention evaluations. Second, we used a formal benchmarking approach to identify a priority care improvement area and to monitor changes in practice following the rollout of the intervention. We feel this approach provides a useful example on how to systematically improve care quality and value in a broader health system context. Third, we evaluated not order set implementation per se, but rather changing an existing order set. Because studies in this area generally focus on initial order set implementation, our study contributes insights on what can be expected through modifications of existing order sets based on care pathways. Fourth, the analysis accounted for a variety of variables including the CCI. Of interest, our study found that the intervention group (patients for whom order sets were used) had a lower CCI, confirming Allen et al.'s findings that diseases with predictable trajectories are the most likely to benefit from care pathways.[4] As a final strength, the narrative‐based order set intervention was relatively simple, and the inclusion criteria were broad, making the process generalizable.
Limitations of this study include that it was a single center pre‐/postintervention study and not a randomized controlled trial. Related to this limitation, the control group for which order sets were not used reflected a different patient population compared to the intervention group for which order sets were used. Specifically, it was more common for order sets to be used in the EDOU than upon admission, resulting in the order set group consisting of patients with less comorbidities than patients in the nonorder set group. Additionally, patients in the order set intervention group were older than in the baseline group (48.0 vs 44.2 years). However, these differences in population remained relatively stable before and after the intervention, and relevant variables including demographic factors and CCI were accounted for in the regression models. Nevertheless, it remains possible that secular trends existed that we did not capture that affected the 2 populations differently. For example, there was a separate project that overlapped with the intervention period to reduce unnecessary laboratory usage at UUHC. This intervention could have influenced the trend to decreased laboratory utilization in the postintervention period. However, there were no concurrent initiatives to reduce antibiotic use during the study period. As a final limitation, the statistical analyses have not corrected for multiple testing for the secondary outcomes.
CONCLUSION
Using benchmark data from UHC, an academic medical center was able to identify an opportunity for improving the care of patients with cellulitis and subsequently develop an evidence‐based care pathway. The implementation of this pathway correlated with a significant reduction of broad‐spectrum antibiotic use, pharmacy costs, and total facility costs without adverse clinical affects. An important factor in the success of the intervention was the use of electronic order sets for cellulitis, which provided support for the implementation of the care pathway. This study demonstrates that the intervention was not only effective overall, but that it was more effective for those patients for whom the order set was used. This study adds to the growing body of literature suggesting that a well‐defined care pathway can improve outcomes and reduce costs for patients and institutions.
Acknowledgements
The authors thank Ms. Pam Proctor for her assistance in implementation of the care pathway and Ms. Selma Lopez for her editorial assistance.
Disclosures: K.K. is or has been a consultant on clinical decision support (CDS) or electronic clinical quality measurement to the US Office of the National Coordinator for Health Information Technology, ARUP Laboratories, McKesson InterQual, ESAC, Inc., JBS International, Inc., Inflexxion, Inc., Intelligent Automation, Inc., Partners HealthCare, Mayo Clinic, and the RAND Corporation. K.K. receives royalties for a Duke Universityowned CDS technology for infectious disease management known as CustomID that he helped develop. K.K. was formerly a consultant for Religent, Inc. and a co‐owner and consultant for Clinica Software, Inc., both of which provide commercial CDS services, including through use of a CDS technology known as SEBASTIAN that K.K. developed. K.K. no longer has a financial relationship with either Religent or Clinica Software. K.K. has no competing interest with any specific product or intervention evaluated in this manuscript. All other authors declare no competing interests.
- Cellulitis. N Engl J Med. 2004;350(9):904–912. .
- Cost‐effectiveness of blood cultures for adult patients with cellulitis. Clin Infect Dis. 1999;29(6):1483–1488. , , , , , .
- Methicillin‐resistant s. aureus infectious among patients in the emergency department. N Engl J Med. 2006;355:666–674. , , , et al.
- Systematic review of the effectiveness of integrated care pathways: what works, for whom, in which circumstances? Int J Evid Based Healthc. 2009;7:61–74. , , .
- Decreased antibiotic utilization after implementation of a guideline for inpatient cellulitis and cutaneous abscess. Arch Intern Med. 2011;171(12):1072–1079. .
- Practice guidelines for the diagnosis and management of skin and soft‐tissue infections. Clin Infect Dis. 2005;41:1373–1406. , , , et al.
- Clinical practice guidelines by the Infectious Disease Society of American for the treatment of methicillin‐resistant Staphylococcus aureus infectious in adults and children. Clin Infect Dis. 2011;42:1–38. , , , et al.
- The role of b‐hemolytic streptococci in causing diffuse, nonculturable cellulitis. Medicine. 2010;89:217–226. , , , .
- Practice guidelines for the diagnosis and management of skin and soft tissue infections: 2014 update by the Infectious Disease Society of America. Clin Infect Dis. 2014;59(2):147–159. , , , et al.
- Value driven outcomes (VDO): a pragmatic, modular, and extensible software framework for understanding and improving health care costs and outcomes. J Am Med Inform Assoc. 2015;22(1):223–235. , , , et al.
- Coding algorithms for defining comorbidities in ICD‐9‐CM and ICD‐10 administrative data. Med Care. 2005;43:113–1139. , , , et al.
- Computerization of workflows, guidelines, and care pathways: a review of implementation challenges for process‐oriented health information systems. J Am Med Inform Assoc. 2011;18:738–748. , .
- Standardized clinical assessment and management plans (SCAMPs) provide a better alternative to clinical practice guidelines. Health Aff (Millwood) 2013;32(5):911–920. , , , et al.
- Clinical practice guidelines for clostridium difficile infection in adults: 2010 update by the Society for Healthcare Epidemiology of America (SHEA) and Infectious Diseases Society of America (IDSA). Infect Control Hosp Epidemiol. 2010;31:431–455. , , , et al.
- Cellulitis. N Engl J Med. 2004;350(9):904–912. .
- Cost‐effectiveness of blood cultures for adult patients with cellulitis. Clin Infect Dis. 1999;29(6):1483–1488. , , , , , .
- Methicillin‐resistant s. aureus infectious among patients in the emergency department. N Engl J Med. 2006;355:666–674. , , , et al.
- Systematic review of the effectiveness of integrated care pathways: what works, for whom, in which circumstances? Int J Evid Based Healthc. 2009;7:61–74. , , .
- Decreased antibiotic utilization after implementation of a guideline for inpatient cellulitis and cutaneous abscess. Arch Intern Med. 2011;171(12):1072–1079. .
- Practice guidelines for the diagnosis and management of skin and soft‐tissue infections. Clin Infect Dis. 2005;41:1373–1406. , , , et al.
- Clinical practice guidelines by the Infectious Disease Society of American for the treatment of methicillin‐resistant Staphylococcus aureus infectious in adults and children. Clin Infect Dis. 2011;42:1–38. , , , et al.
- The role of b‐hemolytic streptococci in causing diffuse, nonculturable cellulitis. Medicine. 2010;89:217–226. , , , .
- Practice guidelines for the diagnosis and management of skin and soft tissue infections: 2014 update by the Infectious Disease Society of America. Clin Infect Dis. 2014;59(2):147–159. , , , et al.
- Value driven outcomes (VDO): a pragmatic, modular, and extensible software framework for understanding and improving health care costs and outcomes. J Am Med Inform Assoc. 2015;22(1):223–235. , , , et al.
- Coding algorithms for defining comorbidities in ICD‐9‐CM and ICD‐10 administrative data. Med Care. 2005;43:113–1139. , , , et al.
- Computerization of workflows, guidelines, and care pathways: a review of implementation challenges for process‐oriented health information systems. J Am Med Inform Assoc. 2011;18:738–748. , .
- Standardized clinical assessment and management plans (SCAMPs) provide a better alternative to clinical practice guidelines. Health Aff (Millwood) 2013;32(5):911–920. , , , et al.
- Clinical practice guidelines for clostridium difficile infection in adults: 2010 update by the Society for Healthcare Epidemiology of America (SHEA) and Infectious Diseases Society of America (IDSA). Infect Control Hosp Epidemiol. 2010;31:431–455. , , , et al.
© 2015 Society of Hospital Medicine