User login
Relationship between Hospital 30-Day Mortality Rates for Heart Failure and Patterns of Early Inpatient Comfort Care
In an effort to improve the quality of care delivered to heart failure (HF) patients, the Centers for Medicare & Medicaid Services (CMS) publish hospitals’ 30-day risk-standardized mortality rates (RSMRs) for HF.1 These mortality rates are also used by CMS to determine the financial penalties and bonuses that hospitals receive as part of the national Hospital Value-based Purchasing program.2 Whether or not these efforts effectively direct patients towards high-quality providers or motivate hospitals to provide better care, few would disagree with the overarching goal of decreasing the number of patients who die from HF.
However, for some patients with chronic disease at the end of life, goals of care may change. The quality of days lived may become more important than the quantity of days lived. As a consequence, high-quality care for some patients at the end of life is associated with withdrawing life-sustaining or life-extending therapies. Over time, this therapeutic perspective has become more common, with use of hospice care doubling from 23% to 47% between 2000 and 2012 among Medicare beneficiaries who died.3 For a national cohort of older patients admitted with HF—not just those patients who died in that same year—hospitals’ rates of referral to hospice are considerably lower, averaging 2.9% in 2010 in a national study.4 Nevertheless, it is possible that hospitals that more faithfully follow their dying patients’ wishes and withdraw life-prolonging interventions and provide comfort-focused care at the end of life might be unfairly penalized if such efforts resulted in higher mortality rates than other hospitals.
Therefore, we used Medicare data linked to a national HF registry with information about end-of-life care, to address 3 questions: (1) How much do hospitals vary in their rates of early comfort care and how has this changed over time; (2) What hospital and patient factors are associated with higher early comfort care rates; and (3) Is there a correlation between 30-day risk-adjusted mortality rates for HF with hospital rates of early comfort care?
METHODS
Data Sources
We used data from the American Heart Association’s Get With The Guidelines-Heart Failure (GWTG-HF) registry. GWTG-HF is a voluntary, inpatient, quality improvement registry5-7 that uses web-based tools and standard questionnaires to collect data on patients with HF admitted to participating hospitals nationwide. The data include information from admission (eg, sociodemographic characteristics, symptoms, medical history, and initial laboratory and test results), the inpatient stay (eg, therapies), and discharge (eg, discharge destination, whether and when comfort care was initiated). We linked the GWTG-HF registry data to Medicare claims data in order to obtain information about Medicare eligibility and patient comorbidities. Additionally, we used data from the American Hospital Association (2008) for hospital characteristics. Quintiles Real-World & Late Phase Research (Cambridge, MA) serves as the data coordinating center for GWTG-HF and the Duke Clinical Research Institute (Durham, NC) serves as the statistical analytic center. GWTG-HF participating sites have a waiver of informed consent because the data are de-identified and primarily used for quality improvement. All analyses performed on this data have been approved by the Duke Medical Center Institutional Review Board.
Study Population
Study Outcomes
Our outcome of interest was the correlation between a hospital’s rate of initiating early CMO for admitted HF patients and a hospital’s 30-day RSMR for HF. The GWTG-HF questionnaire8 asks “When is the earliest physician/advanced practice nurse/physician assistant documentation of comfort measures only?” and permits 4 responses: day 0 or 1, day 2 or after, timing unclear, or not documented/unable to determine. We defined early CMO as CMO on day 0 or 1, and late/no CMO as any other response. We chose to examine early comfort care because many hospitalized patients transition to comfort care before they die if the death is in any way predictable. Thus, if comfort care is measured at any time during the hospitalization, hospitals that have high mortality rates are likely to have high comfort care rates. Therefore, we chose to use the more precise measure of early comfort care. We created hospital-level, risk-standardized early comfort care rates using the same risk-adjustment model used for RSMRs but with the outcome of early comfort care instead of mortality.9,10
RSMRs were calculated using a validated GWTG-HF 30-day risk-standardized mortality model9 with additional variables identified from other GWTG-HF analyses.10 The 30 days are measured as the 30 days after the index admission date.
Statistical Analyses
We described trends in early comfort care rates over time, from February 17, 2008, to February 17, 2014, using the Cochran-Armitage test for trend. We then grouped hospitals into quintiles based on their unadjusted early comfort care rates. We described patient and hospital characteristics for each quintile, using χ2 tests to test for differences across quintiles for categorical variables and Wilcoxon rank sum tests to assess for differences across quintiles for continuous variables. We then examined the Spearman’s rank correlation between hospitals’ RSMR and risk-adjusted comfort care rates. Finally, we compared hospital-level RSMRs before and after adjusting for early comfort care.
We performed risk-adjustment for these last 2 analyses as follows. For each patient, covariates were obtained from the GWTG-HF registry. Clinical data captured for the index admission were utilized in the risk-adjustment model (for both RSMRs and risk-adjusted comfort care rates). Included covariates were as follows: age (per 10 years); race (black vs non-black); systolic blood pressure at admission ≤170 (per 10 mm Hg); respiratory rate (per 5 respirations/min); heart rate ≤105 (per 10 beats/min); weight ≤100 (per 5 kg); weight >100 (per 5 kg); blood urea nitrogen (per 10 mg/dl); brain natriuretic peptide ≤2000 (per 500 pg/ml); hemoglobin 10-14 (per 1 g/dl); troponin abnormal (vs normal); creatinine ≤1 (per 1 mg/dl); sodium 130-140 (per 5 mEq/l); and chronic obstructive pulmonary disease or asthma.
Hierarchical logistic regression modeling was used to calculate the hospital-specific RSMR. A predicted/expected ratio similar to an observed/expected (O/E) ratio was calculated using the following modifications: (1) instead of the observed (crude) number of deaths, the numerator is the number of deaths predicted by the hierarchical model among a hospital’s patients given the patients’ risk factors and the hospital-specific effect; (2) the denominator is the expected number of deaths among the hospital’s patients given the patients’ risk factors and the average of all hospital-specific effects overall; and (3) the ratio of the numerator and denominator are then multiplied by the observed overall mortality rate (same as O/E). This calculation is the method used by CMS to derive RSMRs.11 Multiple imputation was used to handle missing data in the models; 25 imputed datasets using the fully conditional specification method were created. Patients with missing prior comorbidities were assumed to not have those conditions. Hospital characteristics were not imputed; therefore, for analyses that required construction of risk-adjusted comfort care rates or RSMRs, we excluded 18,867 patients cared for at 82 hospitals missing hospital characteristics. We ran 2 sets of models for risk-adjusted comfort care rates and RSMRs: the first adjusted only for patient characteristics, and the second adjusted for both patient and hospital characteristics. Results from the 2 models were similar, so we present only results from the latter. Variance inflation factors were all <2, indicating the collinearity between covariates was not an issue.
All statistical analyses were performed by using SAS version 9.4 (SAS Institute, Cary, NC). We tested for statistical significance by using 2-tailed tests and considered P values <.05 to be statistically significant.
RESULTS
Of the 272 hospitals included in our final study cohort, the observed median overall rate of early comfort care in this study was 1.9% (25th to 75th percentile: 0.9% to 4.0%); hospitals varied widely in unadjusted early comfort care rates (0.00% to 0.46% in the lowest quintile, and 4.60% to 39.91% in the highest quintile; Table 1).
DISCUSSION
Among a national sample of US hospitals, we found wide variation in how frequently health care providers deliver comfort care within the first 2 days of admission for HF. A minority of hospitals reported no early comfort care on any patients throughout the 6-year study period, but hospitals in the highest quintile initiated early comfort care rates for at least 1 in 20 HF patients. Hospitals that were more likely to initiate early comfort care had a higher proportion of female and white patients and were less likely to have the capacity to deliver aggressive surgical interventions such as heart transplants. Hospital-level 30-day RSMRs were not correlated with rates of early comfort care.
While the appropriate rate of early comfort care for patients hospitalized with HF is unknown, given that the average hospital RSMR is approximately 12% for fee-for-service Medicare patients hospitalized with HF,12 it is surprising that some hospitals initiated early comfort care on none or very few of their HF patients. It is quite possible that many of these hospitals initiated comfort care for some of their patients after 48 hours of hospitalization. We were unable to estimate the average period of time patients received comfort care prior to dying, the degree to which this varies across hospitals or why it might vary, and whether the length of time between comfort care initiation and death is related to satisfaction with end-of-life care. Future research on these topics would help inform providers seeking to deliver better end-of-life care. In this study, we also were unable to estimate how often early comfort care was not initiated because patients had a good prognosis. However, prior studies have suggested low rates of comfort care or hospice referral even among patients at very high estimated mortality risk.4 It is also possible that providers and families had concerns about the ability to accurately prognosticate, although several models have been shown to perform acceptably for patients hospitalized with HF.13
We found that comfort care rates did not increase over time, even though use of hospice care doubled among Medicare beneficiaries between 2000 and 2012. By way of context, cancer—the second leading cause of death in the US—was responsible for 38% of hospice admissions in 2013, whereas heart disease (including but not limited to HF)—the leading cause of death— was responsible for 13% of hospice admissions.14 The 2013 American College of Cardiology Foundation and the American Heart Association guidelines for HF recommend consideration of hospice or palliative care for inpatient and transitional care.15 In future work, it would be important to better understand the drivers behind decisions around comfort care for patients hospitalized with HF.
With regards to the policy implications of our study, we found that on average, adjusting 30-day mortality rates for early comfort care was not associated with a change in hospital mortality rankings. For those hospitals with high comfort care rates, adjusting for comfort care did lower mortality rates, but the change was so small as to be clinically insignificant. CMS’ RSMR for HF excludes patients enrolled in hospice during the 12 months prior to index admission, including the first day of the index admission, acknowledging that death may not be an untoward outcome for such patients.16 Fee-for-service Medicare beneficiaries excluded for hospice enrollment comprised 1.29% of HF admissions from July 2012 to June 201516 and are likely a subset of early comfort care patients in our sample, both because of the inclusiveness of chart review (vs claims-based identification) and because we defined early comfort care as comfort care initiated on day 0 or 1 of hospitalization. Nevertheless, with our data we cannot assess to what degree our findings were due solely to hospice patients excluded from CMS’ current estimates.
Prior research has described the underuse of palliative care among patients with HF17 and the association of palliative care with better patient and family experiences at the end of life.18-20 We add to this literature by describing the epidemiology—prevalence, changes over time, and associated factors—of early comfort care for HF in a national sample of hospitals. This serves as a baseline for future work on end-of-life care among patients hospitalized for HF. Our findings also contribute to ongoing discussion about how best to risk-adjust mortality metrics used to assess hospital quality in pay-for-performance programs. Recent research on stroke and pneumonia based on California data suggests that not accounting for do-not-resuscitate (DNR) status biases hospital mortality rates.21,22 Earlier research examined the impact of adjusting hospital mortality rates for DNR for a broader range of conditions.23,24 We expand this line of inquiry by examining the hospital-level association of early comfort care with mortality rates for HF, utilizing a national, contemporary cohort of inpatient stays. In addition, while studies have found that DNR rates within the first 24 hours of admission are relatively high (median 15.8% for pneumonia; 13.3% for stroke),21,22 comfort care is distinct from DNR.
Our findings should be interpreted in the context of several potential limitations. First, we did not have any information about patient or family wishes regarding end-of-life care, or the exact timing of early comfort care (eg, day 0 or day 1). The initiation of comfort care usually follows conversations about end-of-life care involving a patient, his or her family, and the medical team. Thus, we do not know if low early comfort care rates represent the lack of such a conversation (and thus poor-quality care) or the desire by most patients not to initiate early comfort care (and thus high-quality care). This would be an important area for future research. Second, we included only patients admitted to hospitals that participate in GWTG-HF, a voluntary quality improvement initiative. This may limit the generalizability of our findings, but it is unclear how our sample might bias our findings. Hospitals engaged in quality improvement may be more likely to initiate early comfort care aligned with patients’ wishes; on the other hand, hospitals with advanced surgical capabilities are over-represented in our sample and these hospitals are less likely to initiate early comfort care. Third, we examined associations and cannot make conclusions about causality. Residual measured and unmeasured confounding may influence these findings.
In summary, we found that early comfort care rates for fee-for-service Medicare beneficiaries admitted for HF varies widely among hospitals, but median rates of early comfort care have not changed over time. On average, there was no correlation between hospital-level, 30-day, RSMRs and rates of early comfort care. This suggests that current efforts to lower mortality rates have not had unintended consequences for hospitals that institute early comfort care more commonly than their peers.
Acknowledgments
Dr. Chen and Ms. Cox take responsibility for the integrity of the data and the accuracy of the data analysis. Drs. Chen, Levine, and Hayward are responsible for the study concept and design. Drs. Chen and Fonarow acquired the data. Dr. Chen drafted the manuscript. Drs. Chen, Levin, Hayward, Cox, Fonarow, DeVore, Hernandez, Heidenreich, and Yancy revised the manuscript for important intellectual content. Drs. Chen, Hayward, Cox, and Schulte performed the statistical analysis. Drs. Chen and Fonarow obtained funding for the study. Drs. Hayward and Fonarow supervised the study. The authors thank Bailey Green, MPH, for the research assistance she provided. She was compensated for her work.
Disclosure
Dr. Fonarow reports research support from the National Institutes of Health, and consulting for Amgen, Janssen, Novartis, Medtronic, and St Jude Medical. Dr. DeVore reports research support from the American Heart Association, Amgen, and Novartis, and consulting for Amgen. The other authors have no relevant conflicts of interest. Dr. Chen was supported by a Career Development Grant Award (K08HS020671) from the Agency for Healthcare Research and Quality when the manuscript was being prepared. She currently receives support from the Department of Health and Human Services Office of the Assistant Secretary for Planning and Evaluation for her work there. She also receives support from the Blue Cross Blue Shield of Michigan Foundation’s Investigator Initiated Research Program, the Agency for Healthcare Research and Quality (R01 HS024698), and the National Institute on Aging (P01 AG019783). These funding sources had no role in the preparation, review, or approval of the manuscript. The GWTG-HF program is provided by the American Heart Association. GWTG-HF has been funded in the past through support from Amgen, Medtronic, GlaxoSmithKline, Ortho-McNeil, and the American Heart Association Pharmaceutical Roundtable. These sponsors had no role in the study design, data analysis or manuscript preparation and revision.
1. Centers for Medicare & Medicaid Services. Hospital Compare. https://www.medicare.gov/hospitalcompare/. Accessed on November 27, 2016.
2. Centers for Medicare & Medicaid Services. Hospital Value-based Purchasing. https://www.medicare.gov/hospitalcompare/data/hospital-vbp.html. Accessed August 30, 2017.
3. Medicare Payment Advisory Comission. Report to the Congress: Medicare payment policy. 2014. http://www.medpac.gov/docs/default-source/reports/mar14_entirereport.pdf. Accessed August 31, 2017.
4. Whellan DJ, Cox M, Hernandez AF, et al. Utilization of hospice and predicted mortality risk among older patients hospitalized with heart failure: findings from GWTG-HF. J Card Fail. 2012;18(6):471-477. PubMed
5. Hong Y, LaBresh KA. Overview of the American Heart Association “Get with the Guidelines” programs: coronary heart disease, stroke, and heart failure. Crit Pathw Cardiol. 2006;5(4):179-186. PubMed
6. LaBresh KA, Gliklich R, Liljestrand J, Peto R, Ellrodt AG. Using “get with the guidelines” to improve cardiovascular secondary prevention. Jt Comm J Qual Saf. 2003;29(10):539-550. PubMed
7. Hernandez AF, Fonarow GC, Liang L, et al. Sex and racial differences in the use of implantable cardioverter-defibrillators among patients hospitalized with heart failure. JAMA. 2007;298(13):1525-1532. PubMed
8. Get With The Guidelines-Heart Failure. HF Patient Management Tool, October 2016.
9. Eapen ZJ, Liang L, Fonarow GC, et al. Validated, electronic health record deployable prediction models for assessing patient risk of 30-day rehospitalization and mortality in older heart failure patients. JACC Heart Fail. 2013;1(3):245-251. PubMed
10. Peterson PN, Rumsfeld JS, Liang L, et al. A validated risk score for in-hospital mortality in patients with heart failure from the American Heart Association get with the guidelines program. Circ Cardiovasc Qual Outcomes. 2010;3(1):25-32. PubMed
11. Frequently Asked Questions (FAQs): Implementation and Maintenance of CMS Mortality Measures for AMI & HF. 2007. https://www.cms.gov/Medicare/Quality-Initiatives-Patient-Assessment-Instruments/HospitalQualityInits/downloads/HospitalMortalityAboutAMI_HF.pdf. Accessed August 30, 2017.
12. Suter LG, Li SX, Grady JN, et al. National patterns of risk-standardized mortality and readmission after hospitalization for acute myocardial infarction, heart failure, and pneumonia: update on publicly reported outcomes measures based on the 2013 release. J Gen Intern Med. 2014;29(10):1333-1340. PubMed
13. Lagu T, Pekow PS, Shieh MS, et al. Validation and comparison of seven mortality prediction models for hospitalized patients with acute decompensated heart failure. Circ Heart Fail. Aug 2016;9(8):e002912. PubMed
14. National Hospice and Palliative Care Organization. NHPCO’s facts and figures: hospice care in america. 2015. https://www.nhpco.org/sites/default/files/public/Statistics_Research/2015_Facts_Figures.pdf. Accessed August 30, 2017.
15. Yancy CW, Jessup M, Bozkurt B, et al. 2013 ACCF/AHA guideline for the management of heart failure: executive summary: a report of the American College of Cardiology Foundation/American Heart Association Task Force on practice guidelines. Circulation. 2013;128(16):1810-1852. PubMed
16. Centers for Medicare & Medicaid Services. 2016 Condition-Specific Measures Updates and Specifications Report Hospital-Level 30-Day Risk-Standardized Mortality Measures. https://www.qualitynet.org/dcs/ContentServer?c=Page&pagename=QnetPublic%2FPage%2FQnetTier3&cid=1228774398696. Accessed August 30, 2017.
17. Bakitas M, Macmartin M, Trzepkowski K, et al. Palliative care consultations for heart failure patients: how many, when, and why? J Card Fail. 2013;19(3):193-201. PubMed
18. Wachterman MW, Pilver C, Smith D, Ersek M, Lipsitz SR, Keating NL. Quality of End-of-Life Care Provided to Patients With Different Serious Illnesses. JAMA Intern Med. 2016;176(8):1095-1102. PubMed
19. Wright AA, Zhang B, Ray A, et al. Associations between end-of-life discussions, patient mental health, medical care near death, and caregiver bereavement adjustment. JAMA. 2008;300(14):1665-1673. PubMed
20. Rogers JG, Patel CB, Mentz RJ, et al. Palliative care in heart failure: results of a randomized, controlled clinical trial. J Card Fail. 2016;22(11):940. PubMed
21. Kelly AG, Zahuranec DB, Holloway RG, Morgenstern LB, Burke JF. Variation in do-not-resuscitate orders for patients with ischemic stroke: implications for national hospital comparisons. Stroke. 2014;45(3):822-827. PubMed
22. Walkey AJ, Weinberg J, Wiener RS, Cooke CR, Lindenauer PK. Association of Do-Not-Resuscitate Orders and Hospital Mortality Rate Among Patients With Pneumonia. JAMA Intern Med. 2016;176(1):97-104. PubMed
23. Bardach N, Zhao S, Pantilat S, Johnston SC. Adjustment for do-not-resuscitate orders reverses the apparent in-hospital mortality advantage for minorities. Am J Med. 2005;118(4):400-408. PubMed
24. Tabak YP, Johannes RS, Silber JH, Kurtz SG. Should Do-Not-Resuscitate status be included as a mortality risk adjustor? The impact of DNR variations on performance reporting. Med Care. 2005;43(7):658-666. PubMed
In an effort to improve the quality of care delivered to heart failure (HF) patients, the Centers for Medicare & Medicaid Services (CMS) publish hospitals’ 30-day risk-standardized mortality rates (RSMRs) for HF.1 These mortality rates are also used by CMS to determine the financial penalties and bonuses that hospitals receive as part of the national Hospital Value-based Purchasing program.2 Whether or not these efforts effectively direct patients towards high-quality providers or motivate hospitals to provide better care, few would disagree with the overarching goal of decreasing the number of patients who die from HF.
However, for some patients with chronic disease at the end of life, goals of care may change. The quality of days lived may become more important than the quantity of days lived. As a consequence, high-quality care for some patients at the end of life is associated with withdrawing life-sustaining or life-extending therapies. Over time, this therapeutic perspective has become more common, with use of hospice care doubling from 23% to 47% between 2000 and 2012 among Medicare beneficiaries who died.3 For a national cohort of older patients admitted with HF—not just those patients who died in that same year—hospitals’ rates of referral to hospice are considerably lower, averaging 2.9% in 2010 in a national study.4 Nevertheless, it is possible that hospitals that more faithfully follow their dying patients’ wishes and withdraw life-prolonging interventions and provide comfort-focused care at the end of life might be unfairly penalized if such efforts resulted in higher mortality rates than other hospitals.
Therefore, we used Medicare data linked to a national HF registry with information about end-of-life care, to address 3 questions: (1) How much do hospitals vary in their rates of early comfort care and how has this changed over time; (2) What hospital and patient factors are associated with higher early comfort care rates; and (3) Is there a correlation between 30-day risk-adjusted mortality rates for HF with hospital rates of early comfort care?
METHODS
Data Sources
We used data from the American Heart Association’s Get With The Guidelines-Heart Failure (GWTG-HF) registry. GWTG-HF is a voluntary, inpatient, quality improvement registry5-7 that uses web-based tools and standard questionnaires to collect data on patients with HF admitted to participating hospitals nationwide. The data include information from admission (eg, sociodemographic characteristics, symptoms, medical history, and initial laboratory and test results), the inpatient stay (eg, therapies), and discharge (eg, discharge destination, whether and when comfort care was initiated). We linked the GWTG-HF registry data to Medicare claims data in order to obtain information about Medicare eligibility and patient comorbidities. Additionally, we used data from the American Hospital Association (2008) for hospital characteristics. Quintiles Real-World & Late Phase Research (Cambridge, MA) serves as the data coordinating center for GWTG-HF and the Duke Clinical Research Institute (Durham, NC) serves as the statistical analytic center. GWTG-HF participating sites have a waiver of informed consent because the data are de-identified and primarily used for quality improvement. All analyses performed on this data have been approved by the Duke Medical Center Institutional Review Board.
Study Population
Study Outcomes
Our outcome of interest was the correlation between a hospital’s rate of initiating early CMO for admitted HF patients and a hospital’s 30-day RSMR for HF. The GWTG-HF questionnaire8 asks “When is the earliest physician/advanced practice nurse/physician assistant documentation of comfort measures only?” and permits 4 responses: day 0 or 1, day 2 or after, timing unclear, or not documented/unable to determine. We defined early CMO as CMO on day 0 or 1, and late/no CMO as any other response. We chose to examine early comfort care because many hospitalized patients transition to comfort care before they die if the death is in any way predictable. Thus, if comfort care is measured at any time during the hospitalization, hospitals that have high mortality rates are likely to have high comfort care rates. Therefore, we chose to use the more precise measure of early comfort care. We created hospital-level, risk-standardized early comfort care rates using the same risk-adjustment model used for RSMRs but with the outcome of early comfort care instead of mortality.9,10
RSMRs were calculated using a validated GWTG-HF 30-day risk-standardized mortality model9 with additional variables identified from other GWTG-HF analyses.10 The 30 days are measured as the 30 days after the index admission date.
Statistical Analyses
We described trends in early comfort care rates over time, from February 17, 2008, to February 17, 2014, using the Cochran-Armitage test for trend. We then grouped hospitals into quintiles based on their unadjusted early comfort care rates. We described patient and hospital characteristics for each quintile, using χ2 tests to test for differences across quintiles for categorical variables and Wilcoxon rank sum tests to assess for differences across quintiles for continuous variables. We then examined the Spearman’s rank correlation between hospitals’ RSMR and risk-adjusted comfort care rates. Finally, we compared hospital-level RSMRs before and after adjusting for early comfort care.
We performed risk-adjustment for these last 2 analyses as follows. For each patient, covariates were obtained from the GWTG-HF registry. Clinical data captured for the index admission were utilized in the risk-adjustment model (for both RSMRs and risk-adjusted comfort care rates). Included covariates were as follows: age (per 10 years); race (black vs non-black); systolic blood pressure at admission ≤170 (per 10 mm Hg); respiratory rate (per 5 respirations/min); heart rate ≤105 (per 10 beats/min); weight ≤100 (per 5 kg); weight >100 (per 5 kg); blood urea nitrogen (per 10 mg/dl); brain natriuretic peptide ≤2000 (per 500 pg/ml); hemoglobin 10-14 (per 1 g/dl); troponin abnormal (vs normal); creatinine ≤1 (per 1 mg/dl); sodium 130-140 (per 5 mEq/l); and chronic obstructive pulmonary disease or asthma.
Hierarchical logistic regression modeling was used to calculate the hospital-specific RSMR. A predicted/expected ratio similar to an observed/expected (O/E) ratio was calculated using the following modifications: (1) instead of the observed (crude) number of deaths, the numerator is the number of deaths predicted by the hierarchical model among a hospital’s patients given the patients’ risk factors and the hospital-specific effect; (2) the denominator is the expected number of deaths among the hospital’s patients given the patients’ risk factors and the average of all hospital-specific effects overall; and (3) the ratio of the numerator and denominator are then multiplied by the observed overall mortality rate (same as O/E). This calculation is the method used by CMS to derive RSMRs.11 Multiple imputation was used to handle missing data in the models; 25 imputed datasets using the fully conditional specification method were created. Patients with missing prior comorbidities were assumed to not have those conditions. Hospital characteristics were not imputed; therefore, for analyses that required construction of risk-adjusted comfort care rates or RSMRs, we excluded 18,867 patients cared for at 82 hospitals missing hospital characteristics. We ran 2 sets of models for risk-adjusted comfort care rates and RSMRs: the first adjusted only for patient characteristics, and the second adjusted for both patient and hospital characteristics. Results from the 2 models were similar, so we present only results from the latter. Variance inflation factors were all <2, indicating the collinearity between covariates was not an issue.
All statistical analyses were performed by using SAS version 9.4 (SAS Institute, Cary, NC). We tested for statistical significance by using 2-tailed tests and considered P values <.05 to be statistically significant.
RESULTS
Of the 272 hospitals included in our final study cohort, the observed median overall rate of early comfort care in this study was 1.9% (25th to 75th percentile: 0.9% to 4.0%); hospitals varied widely in unadjusted early comfort care rates (0.00% to 0.46% in the lowest quintile, and 4.60% to 39.91% in the highest quintile; Table 1).
DISCUSSION
Among a national sample of US hospitals, we found wide variation in how frequently health care providers deliver comfort care within the first 2 days of admission for HF. A minority of hospitals reported no early comfort care on any patients throughout the 6-year study period, but hospitals in the highest quintile initiated early comfort care rates for at least 1 in 20 HF patients. Hospitals that were more likely to initiate early comfort care had a higher proportion of female and white patients and were less likely to have the capacity to deliver aggressive surgical interventions such as heart transplants. Hospital-level 30-day RSMRs were not correlated with rates of early comfort care.
While the appropriate rate of early comfort care for patients hospitalized with HF is unknown, given that the average hospital RSMR is approximately 12% for fee-for-service Medicare patients hospitalized with HF,12 it is surprising that some hospitals initiated early comfort care on none or very few of their HF patients. It is quite possible that many of these hospitals initiated comfort care for some of their patients after 48 hours of hospitalization. We were unable to estimate the average period of time patients received comfort care prior to dying, the degree to which this varies across hospitals or why it might vary, and whether the length of time between comfort care initiation and death is related to satisfaction with end-of-life care. Future research on these topics would help inform providers seeking to deliver better end-of-life care. In this study, we also were unable to estimate how often early comfort care was not initiated because patients had a good prognosis. However, prior studies have suggested low rates of comfort care or hospice referral even among patients at very high estimated mortality risk.4 It is also possible that providers and families had concerns about the ability to accurately prognosticate, although several models have been shown to perform acceptably for patients hospitalized with HF.13
We found that comfort care rates did not increase over time, even though use of hospice care doubled among Medicare beneficiaries between 2000 and 2012. By way of context, cancer—the second leading cause of death in the US—was responsible for 38% of hospice admissions in 2013, whereas heart disease (including but not limited to HF)—the leading cause of death— was responsible for 13% of hospice admissions.14 The 2013 American College of Cardiology Foundation and the American Heart Association guidelines for HF recommend consideration of hospice or palliative care for inpatient and transitional care.15 In future work, it would be important to better understand the drivers behind decisions around comfort care for patients hospitalized with HF.
With regards to the policy implications of our study, we found that on average, adjusting 30-day mortality rates for early comfort care was not associated with a change in hospital mortality rankings. For those hospitals with high comfort care rates, adjusting for comfort care did lower mortality rates, but the change was so small as to be clinically insignificant. CMS’ RSMR for HF excludes patients enrolled in hospice during the 12 months prior to index admission, including the first day of the index admission, acknowledging that death may not be an untoward outcome for such patients.16 Fee-for-service Medicare beneficiaries excluded for hospice enrollment comprised 1.29% of HF admissions from July 2012 to June 201516 and are likely a subset of early comfort care patients in our sample, both because of the inclusiveness of chart review (vs claims-based identification) and because we defined early comfort care as comfort care initiated on day 0 or 1 of hospitalization. Nevertheless, with our data we cannot assess to what degree our findings were due solely to hospice patients excluded from CMS’ current estimates.
Prior research has described the underuse of palliative care among patients with HF17 and the association of palliative care with better patient and family experiences at the end of life.18-20 We add to this literature by describing the epidemiology—prevalence, changes over time, and associated factors—of early comfort care for HF in a national sample of hospitals. This serves as a baseline for future work on end-of-life care among patients hospitalized for HF. Our findings also contribute to ongoing discussion about how best to risk-adjust mortality metrics used to assess hospital quality in pay-for-performance programs. Recent research on stroke and pneumonia based on California data suggests that not accounting for do-not-resuscitate (DNR) status biases hospital mortality rates.21,22 Earlier research examined the impact of adjusting hospital mortality rates for DNR for a broader range of conditions.23,24 We expand this line of inquiry by examining the hospital-level association of early comfort care with mortality rates for HF, utilizing a national, contemporary cohort of inpatient stays. In addition, while studies have found that DNR rates within the first 24 hours of admission are relatively high (median 15.8% for pneumonia; 13.3% for stroke),21,22 comfort care is distinct from DNR.
Our findings should be interpreted in the context of several potential limitations. First, we did not have any information about patient or family wishes regarding end-of-life care, or the exact timing of early comfort care (eg, day 0 or day 1). The initiation of comfort care usually follows conversations about end-of-life care involving a patient, his or her family, and the medical team. Thus, we do not know if low early comfort care rates represent the lack of such a conversation (and thus poor-quality care) or the desire by most patients not to initiate early comfort care (and thus high-quality care). This would be an important area for future research. Second, we included only patients admitted to hospitals that participate in GWTG-HF, a voluntary quality improvement initiative. This may limit the generalizability of our findings, but it is unclear how our sample might bias our findings. Hospitals engaged in quality improvement may be more likely to initiate early comfort care aligned with patients’ wishes; on the other hand, hospitals with advanced surgical capabilities are over-represented in our sample and these hospitals are less likely to initiate early comfort care. Third, we examined associations and cannot make conclusions about causality. Residual measured and unmeasured confounding may influence these findings.
In summary, we found that early comfort care rates for fee-for-service Medicare beneficiaries admitted for HF varies widely among hospitals, but median rates of early comfort care have not changed over time. On average, there was no correlation between hospital-level, 30-day, RSMRs and rates of early comfort care. This suggests that current efforts to lower mortality rates have not had unintended consequences for hospitals that institute early comfort care more commonly than their peers.
Acknowledgments
Dr. Chen and Ms. Cox take responsibility for the integrity of the data and the accuracy of the data analysis. Drs. Chen, Levine, and Hayward are responsible for the study concept and design. Drs. Chen and Fonarow acquired the data. Dr. Chen drafted the manuscript. Drs. Chen, Levin, Hayward, Cox, Fonarow, DeVore, Hernandez, Heidenreich, and Yancy revised the manuscript for important intellectual content. Drs. Chen, Hayward, Cox, and Schulte performed the statistical analysis. Drs. Chen and Fonarow obtained funding for the study. Drs. Hayward and Fonarow supervised the study. The authors thank Bailey Green, MPH, for the research assistance she provided. She was compensated for her work.
Disclosure
Dr. Fonarow reports research support from the National Institutes of Health, and consulting for Amgen, Janssen, Novartis, Medtronic, and St Jude Medical. Dr. DeVore reports research support from the American Heart Association, Amgen, and Novartis, and consulting for Amgen. The other authors have no relevant conflicts of interest. Dr. Chen was supported by a Career Development Grant Award (K08HS020671) from the Agency for Healthcare Research and Quality when the manuscript was being prepared. She currently receives support from the Department of Health and Human Services Office of the Assistant Secretary for Planning and Evaluation for her work there. She also receives support from the Blue Cross Blue Shield of Michigan Foundation’s Investigator Initiated Research Program, the Agency for Healthcare Research and Quality (R01 HS024698), and the National Institute on Aging (P01 AG019783). These funding sources had no role in the preparation, review, or approval of the manuscript. The GWTG-HF program is provided by the American Heart Association. GWTG-HF has been funded in the past through support from Amgen, Medtronic, GlaxoSmithKline, Ortho-McNeil, and the American Heart Association Pharmaceutical Roundtable. These sponsors had no role in the study design, data analysis or manuscript preparation and revision.
In an effort to improve the quality of care delivered to heart failure (HF) patients, the Centers for Medicare & Medicaid Services (CMS) publish hospitals’ 30-day risk-standardized mortality rates (RSMRs) for HF.1 These mortality rates are also used by CMS to determine the financial penalties and bonuses that hospitals receive as part of the national Hospital Value-based Purchasing program.2 Whether or not these efforts effectively direct patients towards high-quality providers or motivate hospitals to provide better care, few would disagree with the overarching goal of decreasing the number of patients who die from HF.
However, for some patients with chronic disease at the end of life, goals of care may change. The quality of days lived may become more important than the quantity of days lived. As a consequence, high-quality care for some patients at the end of life is associated with withdrawing life-sustaining or life-extending therapies. Over time, this therapeutic perspective has become more common, with use of hospice care doubling from 23% to 47% between 2000 and 2012 among Medicare beneficiaries who died.3 For a national cohort of older patients admitted with HF—not just those patients who died in that same year—hospitals’ rates of referral to hospice are considerably lower, averaging 2.9% in 2010 in a national study.4 Nevertheless, it is possible that hospitals that more faithfully follow their dying patients’ wishes and withdraw life-prolonging interventions and provide comfort-focused care at the end of life might be unfairly penalized if such efforts resulted in higher mortality rates than other hospitals.
Therefore, we used Medicare data linked to a national HF registry with information about end-of-life care, to address 3 questions: (1) How much do hospitals vary in their rates of early comfort care and how has this changed over time; (2) What hospital and patient factors are associated with higher early comfort care rates; and (3) Is there a correlation between 30-day risk-adjusted mortality rates for HF with hospital rates of early comfort care?
METHODS
Data Sources
We used data from the American Heart Association’s Get With The Guidelines-Heart Failure (GWTG-HF) registry. GWTG-HF is a voluntary, inpatient, quality improvement registry5-7 that uses web-based tools and standard questionnaires to collect data on patients with HF admitted to participating hospitals nationwide. The data include information from admission (eg, sociodemographic characteristics, symptoms, medical history, and initial laboratory and test results), the inpatient stay (eg, therapies), and discharge (eg, discharge destination, whether and when comfort care was initiated). We linked the GWTG-HF registry data to Medicare claims data in order to obtain information about Medicare eligibility and patient comorbidities. Additionally, we used data from the American Hospital Association (2008) for hospital characteristics. Quintiles Real-World & Late Phase Research (Cambridge, MA) serves as the data coordinating center for GWTG-HF and the Duke Clinical Research Institute (Durham, NC) serves as the statistical analytic center. GWTG-HF participating sites have a waiver of informed consent because the data are de-identified and primarily used for quality improvement. All analyses performed on this data have been approved by the Duke Medical Center Institutional Review Board.
Study Population
Study Outcomes
Our outcome of interest was the correlation between a hospital’s rate of initiating early CMO for admitted HF patients and a hospital’s 30-day RSMR for HF. The GWTG-HF questionnaire8 asks “When is the earliest physician/advanced practice nurse/physician assistant documentation of comfort measures only?” and permits 4 responses: day 0 or 1, day 2 or after, timing unclear, or not documented/unable to determine. We defined early CMO as CMO on day 0 or 1, and late/no CMO as any other response. We chose to examine early comfort care because many hospitalized patients transition to comfort care before they die if the death is in any way predictable. Thus, if comfort care is measured at any time during the hospitalization, hospitals that have high mortality rates are likely to have high comfort care rates. Therefore, we chose to use the more precise measure of early comfort care. We created hospital-level, risk-standardized early comfort care rates using the same risk-adjustment model used for RSMRs but with the outcome of early comfort care instead of mortality.9,10
RSMRs were calculated using a validated GWTG-HF 30-day risk-standardized mortality model9 with additional variables identified from other GWTG-HF analyses.10 The 30 days are measured as the 30 days after the index admission date.
Statistical Analyses
We described trends in early comfort care rates over time, from February 17, 2008, to February 17, 2014, using the Cochran-Armitage test for trend. We then grouped hospitals into quintiles based on their unadjusted early comfort care rates. We described patient and hospital characteristics for each quintile, using χ2 tests to test for differences across quintiles for categorical variables and Wilcoxon rank sum tests to assess for differences across quintiles for continuous variables. We then examined the Spearman’s rank correlation between hospitals’ RSMR and risk-adjusted comfort care rates. Finally, we compared hospital-level RSMRs before and after adjusting for early comfort care.
We performed risk-adjustment for these last 2 analyses as follows. For each patient, covariates were obtained from the GWTG-HF registry. Clinical data captured for the index admission were utilized in the risk-adjustment model (for both RSMRs and risk-adjusted comfort care rates). Included covariates were as follows: age (per 10 years); race (black vs non-black); systolic blood pressure at admission ≤170 (per 10 mm Hg); respiratory rate (per 5 respirations/min); heart rate ≤105 (per 10 beats/min); weight ≤100 (per 5 kg); weight >100 (per 5 kg); blood urea nitrogen (per 10 mg/dl); brain natriuretic peptide ≤2000 (per 500 pg/ml); hemoglobin 10-14 (per 1 g/dl); troponin abnormal (vs normal); creatinine ≤1 (per 1 mg/dl); sodium 130-140 (per 5 mEq/l); and chronic obstructive pulmonary disease or asthma.
Hierarchical logistic regression modeling was used to calculate the hospital-specific RSMR. A predicted/expected ratio similar to an observed/expected (O/E) ratio was calculated using the following modifications: (1) instead of the observed (crude) number of deaths, the numerator is the number of deaths predicted by the hierarchical model among a hospital’s patients given the patients’ risk factors and the hospital-specific effect; (2) the denominator is the expected number of deaths among the hospital’s patients given the patients’ risk factors and the average of all hospital-specific effects overall; and (3) the ratio of the numerator and denominator are then multiplied by the observed overall mortality rate (same as O/E). This calculation is the method used by CMS to derive RSMRs.11 Multiple imputation was used to handle missing data in the models; 25 imputed datasets using the fully conditional specification method were created. Patients with missing prior comorbidities were assumed to not have those conditions. Hospital characteristics were not imputed; therefore, for analyses that required construction of risk-adjusted comfort care rates or RSMRs, we excluded 18,867 patients cared for at 82 hospitals missing hospital characteristics. We ran 2 sets of models for risk-adjusted comfort care rates and RSMRs: the first adjusted only for patient characteristics, and the second adjusted for both patient and hospital characteristics. Results from the 2 models were similar, so we present only results from the latter. Variance inflation factors were all <2, indicating the collinearity between covariates was not an issue.
All statistical analyses were performed by using SAS version 9.4 (SAS Institute, Cary, NC). We tested for statistical significance by using 2-tailed tests and considered P values <.05 to be statistically significant.
RESULTS
Of the 272 hospitals included in our final study cohort, the observed median overall rate of early comfort care in this study was 1.9% (25th to 75th percentile: 0.9% to 4.0%); hospitals varied widely in unadjusted early comfort care rates (0.00% to 0.46% in the lowest quintile, and 4.60% to 39.91% in the highest quintile; Table 1).
DISCUSSION
Among a national sample of US hospitals, we found wide variation in how frequently health care providers deliver comfort care within the first 2 days of admission for HF. A minority of hospitals reported no early comfort care on any patients throughout the 6-year study period, but hospitals in the highest quintile initiated early comfort care rates for at least 1 in 20 HF patients. Hospitals that were more likely to initiate early comfort care had a higher proportion of female and white patients and were less likely to have the capacity to deliver aggressive surgical interventions such as heart transplants. Hospital-level 30-day RSMRs were not correlated with rates of early comfort care.
While the appropriate rate of early comfort care for patients hospitalized with HF is unknown, given that the average hospital RSMR is approximately 12% for fee-for-service Medicare patients hospitalized with HF,12 it is surprising that some hospitals initiated early comfort care on none or very few of their HF patients. It is quite possible that many of these hospitals initiated comfort care for some of their patients after 48 hours of hospitalization. We were unable to estimate the average period of time patients received comfort care prior to dying, the degree to which this varies across hospitals or why it might vary, and whether the length of time between comfort care initiation and death is related to satisfaction with end-of-life care. Future research on these topics would help inform providers seeking to deliver better end-of-life care. In this study, we also were unable to estimate how often early comfort care was not initiated because patients had a good prognosis. However, prior studies have suggested low rates of comfort care or hospice referral even among patients at very high estimated mortality risk.4 It is also possible that providers and families had concerns about the ability to accurately prognosticate, although several models have been shown to perform acceptably for patients hospitalized with HF.13
We found that comfort care rates did not increase over time, even though use of hospice care doubled among Medicare beneficiaries between 2000 and 2012. By way of context, cancer—the second leading cause of death in the US—was responsible for 38% of hospice admissions in 2013, whereas heart disease (including but not limited to HF)—the leading cause of death— was responsible for 13% of hospice admissions.14 The 2013 American College of Cardiology Foundation and the American Heart Association guidelines for HF recommend consideration of hospice or palliative care for inpatient and transitional care.15 In future work, it would be important to better understand the drivers behind decisions around comfort care for patients hospitalized with HF.
With regards to the policy implications of our study, we found that on average, adjusting 30-day mortality rates for early comfort care was not associated with a change in hospital mortality rankings. For those hospitals with high comfort care rates, adjusting for comfort care did lower mortality rates, but the change was so small as to be clinically insignificant. CMS’ RSMR for HF excludes patients enrolled in hospice during the 12 months prior to index admission, including the first day of the index admission, acknowledging that death may not be an untoward outcome for such patients.16 Fee-for-service Medicare beneficiaries excluded for hospice enrollment comprised 1.29% of HF admissions from July 2012 to June 201516 and are likely a subset of early comfort care patients in our sample, both because of the inclusiveness of chart review (vs claims-based identification) and because we defined early comfort care as comfort care initiated on day 0 or 1 of hospitalization. Nevertheless, with our data we cannot assess to what degree our findings were due solely to hospice patients excluded from CMS’ current estimates.
Prior research has described the underuse of palliative care among patients with HF17 and the association of palliative care with better patient and family experiences at the end of life.18-20 We add to this literature by describing the epidemiology—prevalence, changes over time, and associated factors—of early comfort care for HF in a national sample of hospitals. This serves as a baseline for future work on end-of-life care among patients hospitalized for HF. Our findings also contribute to ongoing discussion about how best to risk-adjust mortality metrics used to assess hospital quality in pay-for-performance programs. Recent research on stroke and pneumonia based on California data suggests that not accounting for do-not-resuscitate (DNR) status biases hospital mortality rates.21,22 Earlier research examined the impact of adjusting hospital mortality rates for DNR for a broader range of conditions.23,24 We expand this line of inquiry by examining the hospital-level association of early comfort care with mortality rates for HF, utilizing a national, contemporary cohort of inpatient stays. In addition, while studies have found that DNR rates within the first 24 hours of admission are relatively high (median 15.8% for pneumonia; 13.3% for stroke),21,22 comfort care is distinct from DNR.
Our findings should be interpreted in the context of several potential limitations. First, we did not have any information about patient or family wishes regarding end-of-life care, or the exact timing of early comfort care (eg, day 0 or day 1). The initiation of comfort care usually follows conversations about end-of-life care involving a patient, his or her family, and the medical team. Thus, we do not know if low early comfort care rates represent the lack of such a conversation (and thus poor-quality care) or the desire by most patients not to initiate early comfort care (and thus high-quality care). This would be an important area for future research. Second, we included only patients admitted to hospitals that participate in GWTG-HF, a voluntary quality improvement initiative. This may limit the generalizability of our findings, but it is unclear how our sample might bias our findings. Hospitals engaged in quality improvement may be more likely to initiate early comfort care aligned with patients’ wishes; on the other hand, hospitals with advanced surgical capabilities are over-represented in our sample and these hospitals are less likely to initiate early comfort care. Third, we examined associations and cannot make conclusions about causality. Residual measured and unmeasured confounding may influence these findings.
In summary, we found that early comfort care rates for fee-for-service Medicare beneficiaries admitted for HF varies widely among hospitals, but median rates of early comfort care have not changed over time. On average, there was no correlation between hospital-level, 30-day, RSMRs and rates of early comfort care. This suggests that current efforts to lower mortality rates have not had unintended consequences for hospitals that institute early comfort care more commonly than their peers.
Acknowledgments
Dr. Chen and Ms. Cox take responsibility for the integrity of the data and the accuracy of the data analysis. Drs. Chen, Levine, and Hayward are responsible for the study concept and design. Drs. Chen and Fonarow acquired the data. Dr. Chen drafted the manuscript. Drs. Chen, Levin, Hayward, Cox, Fonarow, DeVore, Hernandez, Heidenreich, and Yancy revised the manuscript for important intellectual content. Drs. Chen, Hayward, Cox, and Schulte performed the statistical analysis. Drs. Chen and Fonarow obtained funding for the study. Drs. Hayward and Fonarow supervised the study. The authors thank Bailey Green, MPH, for the research assistance she provided. She was compensated for her work.
Disclosure
Dr. Fonarow reports research support from the National Institutes of Health, and consulting for Amgen, Janssen, Novartis, Medtronic, and St Jude Medical. Dr. DeVore reports research support from the American Heart Association, Amgen, and Novartis, and consulting for Amgen. The other authors have no relevant conflicts of interest. Dr. Chen was supported by a Career Development Grant Award (K08HS020671) from the Agency for Healthcare Research and Quality when the manuscript was being prepared. She currently receives support from the Department of Health and Human Services Office of the Assistant Secretary for Planning and Evaluation for her work there. She also receives support from the Blue Cross Blue Shield of Michigan Foundation’s Investigator Initiated Research Program, the Agency for Healthcare Research and Quality (R01 HS024698), and the National Institute on Aging (P01 AG019783). These funding sources had no role in the preparation, review, or approval of the manuscript. The GWTG-HF program is provided by the American Heart Association. GWTG-HF has been funded in the past through support from Amgen, Medtronic, GlaxoSmithKline, Ortho-McNeil, and the American Heart Association Pharmaceutical Roundtable. These sponsors had no role in the study design, data analysis or manuscript preparation and revision.
1. Centers for Medicare & Medicaid Services. Hospital Compare. https://www.medicare.gov/hospitalcompare/. Accessed on November 27, 2016.
2. Centers for Medicare & Medicaid Services. Hospital Value-based Purchasing. https://www.medicare.gov/hospitalcompare/data/hospital-vbp.html. Accessed August 30, 2017.
3. Medicare Payment Advisory Comission. Report to the Congress: Medicare payment policy. 2014. http://www.medpac.gov/docs/default-source/reports/mar14_entirereport.pdf. Accessed August 31, 2017.
4. Whellan DJ, Cox M, Hernandez AF, et al. Utilization of hospice and predicted mortality risk among older patients hospitalized with heart failure: findings from GWTG-HF. J Card Fail. 2012;18(6):471-477. PubMed
5. Hong Y, LaBresh KA. Overview of the American Heart Association “Get with the Guidelines” programs: coronary heart disease, stroke, and heart failure. Crit Pathw Cardiol. 2006;5(4):179-186. PubMed
6. LaBresh KA, Gliklich R, Liljestrand J, Peto R, Ellrodt AG. Using “get with the guidelines” to improve cardiovascular secondary prevention. Jt Comm J Qual Saf. 2003;29(10):539-550. PubMed
7. Hernandez AF, Fonarow GC, Liang L, et al. Sex and racial differences in the use of implantable cardioverter-defibrillators among patients hospitalized with heart failure. JAMA. 2007;298(13):1525-1532. PubMed
8. Get With The Guidelines-Heart Failure. HF Patient Management Tool, October 2016.
9. Eapen ZJ, Liang L, Fonarow GC, et al. Validated, electronic health record deployable prediction models for assessing patient risk of 30-day rehospitalization and mortality in older heart failure patients. JACC Heart Fail. 2013;1(3):245-251. PubMed
10. Peterson PN, Rumsfeld JS, Liang L, et al. A validated risk score for in-hospital mortality in patients with heart failure from the American Heart Association get with the guidelines program. Circ Cardiovasc Qual Outcomes. 2010;3(1):25-32. PubMed
11. Frequently Asked Questions (FAQs): Implementation and Maintenance of CMS Mortality Measures for AMI & HF. 2007. https://www.cms.gov/Medicare/Quality-Initiatives-Patient-Assessment-Instruments/HospitalQualityInits/downloads/HospitalMortalityAboutAMI_HF.pdf. Accessed August 30, 2017.
12. Suter LG, Li SX, Grady JN, et al. National patterns of risk-standardized mortality and readmission after hospitalization for acute myocardial infarction, heart failure, and pneumonia: update on publicly reported outcomes measures based on the 2013 release. J Gen Intern Med. 2014;29(10):1333-1340. PubMed
13. Lagu T, Pekow PS, Shieh MS, et al. Validation and comparison of seven mortality prediction models for hospitalized patients with acute decompensated heart failure. Circ Heart Fail. Aug 2016;9(8):e002912. PubMed
14. National Hospice and Palliative Care Organization. NHPCO’s facts and figures: hospice care in america. 2015. https://www.nhpco.org/sites/default/files/public/Statistics_Research/2015_Facts_Figures.pdf. Accessed August 30, 2017.
15. Yancy CW, Jessup M, Bozkurt B, et al. 2013 ACCF/AHA guideline for the management of heart failure: executive summary: a report of the American College of Cardiology Foundation/American Heart Association Task Force on practice guidelines. Circulation. 2013;128(16):1810-1852. PubMed
16. Centers for Medicare & Medicaid Services. 2016 Condition-Specific Measures Updates and Specifications Report Hospital-Level 30-Day Risk-Standardized Mortality Measures. https://www.qualitynet.org/dcs/ContentServer?c=Page&pagename=QnetPublic%2FPage%2FQnetTier3&cid=1228774398696. Accessed August 30, 2017.
17. Bakitas M, Macmartin M, Trzepkowski K, et al. Palliative care consultations for heart failure patients: how many, when, and why? J Card Fail. 2013;19(3):193-201. PubMed
18. Wachterman MW, Pilver C, Smith D, Ersek M, Lipsitz SR, Keating NL. Quality of End-of-Life Care Provided to Patients With Different Serious Illnesses. JAMA Intern Med. 2016;176(8):1095-1102. PubMed
19. Wright AA, Zhang B, Ray A, et al. Associations between end-of-life discussions, patient mental health, medical care near death, and caregiver bereavement adjustment. JAMA. 2008;300(14):1665-1673. PubMed
20. Rogers JG, Patel CB, Mentz RJ, et al. Palliative care in heart failure: results of a randomized, controlled clinical trial. J Card Fail. 2016;22(11):940. PubMed
21. Kelly AG, Zahuranec DB, Holloway RG, Morgenstern LB, Burke JF. Variation in do-not-resuscitate orders for patients with ischemic stroke: implications for national hospital comparisons. Stroke. 2014;45(3):822-827. PubMed
22. Walkey AJ, Weinberg J, Wiener RS, Cooke CR, Lindenauer PK. Association of Do-Not-Resuscitate Orders and Hospital Mortality Rate Among Patients With Pneumonia. JAMA Intern Med. 2016;176(1):97-104. PubMed
23. Bardach N, Zhao S, Pantilat S, Johnston SC. Adjustment for do-not-resuscitate orders reverses the apparent in-hospital mortality advantage for minorities. Am J Med. 2005;118(4):400-408. PubMed
24. Tabak YP, Johannes RS, Silber JH, Kurtz SG. Should Do-Not-Resuscitate status be included as a mortality risk adjustor? The impact of DNR variations on performance reporting. Med Care. 2005;43(7):658-666. PubMed
1. Centers for Medicare & Medicaid Services. Hospital Compare. https://www.medicare.gov/hospitalcompare/. Accessed on November 27, 2016.
2. Centers for Medicare & Medicaid Services. Hospital Value-based Purchasing. https://www.medicare.gov/hospitalcompare/data/hospital-vbp.html. Accessed August 30, 2017.
3. Medicare Payment Advisory Comission. Report to the Congress: Medicare payment policy. 2014. http://www.medpac.gov/docs/default-source/reports/mar14_entirereport.pdf. Accessed August 31, 2017.
4. Whellan DJ, Cox M, Hernandez AF, et al. Utilization of hospice and predicted mortality risk among older patients hospitalized with heart failure: findings from GWTG-HF. J Card Fail. 2012;18(6):471-477. PubMed
5. Hong Y, LaBresh KA. Overview of the American Heart Association “Get with the Guidelines” programs: coronary heart disease, stroke, and heart failure. Crit Pathw Cardiol. 2006;5(4):179-186. PubMed
6. LaBresh KA, Gliklich R, Liljestrand J, Peto R, Ellrodt AG. Using “get with the guidelines” to improve cardiovascular secondary prevention. Jt Comm J Qual Saf. 2003;29(10):539-550. PubMed
7. Hernandez AF, Fonarow GC, Liang L, et al. Sex and racial differences in the use of implantable cardioverter-defibrillators among patients hospitalized with heart failure. JAMA. 2007;298(13):1525-1532. PubMed
8. Get With The Guidelines-Heart Failure. HF Patient Management Tool, October 2016.
9. Eapen ZJ, Liang L, Fonarow GC, et al. Validated, electronic health record deployable prediction models for assessing patient risk of 30-day rehospitalization and mortality in older heart failure patients. JACC Heart Fail. 2013;1(3):245-251. PubMed
10. Peterson PN, Rumsfeld JS, Liang L, et al. A validated risk score for in-hospital mortality in patients with heart failure from the American Heart Association get with the guidelines program. Circ Cardiovasc Qual Outcomes. 2010;3(1):25-32. PubMed
11. Frequently Asked Questions (FAQs): Implementation and Maintenance of CMS Mortality Measures for AMI & HF. 2007. https://www.cms.gov/Medicare/Quality-Initiatives-Patient-Assessment-Instruments/HospitalQualityInits/downloads/HospitalMortalityAboutAMI_HF.pdf. Accessed August 30, 2017.
12. Suter LG, Li SX, Grady JN, et al. National patterns of risk-standardized mortality and readmission after hospitalization for acute myocardial infarction, heart failure, and pneumonia: update on publicly reported outcomes measures based on the 2013 release. J Gen Intern Med. 2014;29(10):1333-1340. PubMed
13. Lagu T, Pekow PS, Shieh MS, et al. Validation and comparison of seven mortality prediction models for hospitalized patients with acute decompensated heart failure. Circ Heart Fail. Aug 2016;9(8):e002912. PubMed
14. National Hospice and Palliative Care Organization. NHPCO’s facts and figures: hospice care in america. 2015. https://www.nhpco.org/sites/default/files/public/Statistics_Research/2015_Facts_Figures.pdf. Accessed August 30, 2017.
15. Yancy CW, Jessup M, Bozkurt B, et al. 2013 ACCF/AHA guideline for the management of heart failure: executive summary: a report of the American College of Cardiology Foundation/American Heart Association Task Force on practice guidelines. Circulation. 2013;128(16):1810-1852. PubMed
16. Centers for Medicare & Medicaid Services. 2016 Condition-Specific Measures Updates and Specifications Report Hospital-Level 30-Day Risk-Standardized Mortality Measures. https://www.qualitynet.org/dcs/ContentServer?c=Page&pagename=QnetPublic%2FPage%2FQnetTier3&cid=1228774398696. Accessed August 30, 2017.
17. Bakitas M, Macmartin M, Trzepkowski K, et al. Palliative care consultations for heart failure patients: how many, when, and why? J Card Fail. 2013;19(3):193-201. PubMed
18. Wachterman MW, Pilver C, Smith D, Ersek M, Lipsitz SR, Keating NL. Quality of End-of-Life Care Provided to Patients With Different Serious Illnesses. JAMA Intern Med. 2016;176(8):1095-1102. PubMed
19. Wright AA, Zhang B, Ray A, et al. Associations between end-of-life discussions, patient mental health, medical care near death, and caregiver bereavement adjustment. JAMA. 2008;300(14):1665-1673. PubMed
20. Rogers JG, Patel CB, Mentz RJ, et al. Palliative care in heart failure: results of a randomized, controlled clinical trial. J Card Fail. 2016;22(11):940. PubMed
21. Kelly AG, Zahuranec DB, Holloway RG, Morgenstern LB, Burke JF. Variation in do-not-resuscitate orders for patients with ischemic stroke: implications for national hospital comparisons. Stroke. 2014;45(3):822-827. PubMed
22. Walkey AJ, Weinberg J, Wiener RS, Cooke CR, Lindenauer PK. Association of Do-Not-Resuscitate Orders and Hospital Mortality Rate Among Patients With Pneumonia. JAMA Intern Med. 2016;176(1):97-104. PubMed
23. Bardach N, Zhao S, Pantilat S, Johnston SC. Adjustment for do-not-resuscitate orders reverses the apparent in-hospital mortality advantage for minorities. Am J Med. 2005;118(4):400-408. PubMed
24. Tabak YP, Johannes RS, Silber JH, Kurtz SG. Should Do-Not-Resuscitate status be included as a mortality risk adjustor? The impact of DNR variations on performance reporting. Med Care. 2005;43(7):658-666. PubMed
© 2018 Society of Hospital Medicine
Controlling Medicare Spending
Payers, providers, and policymakers are testing several major approaches to reducing US healthcare spending without harming quality. One strategy is to bundle payments for a longitudinal episode of care, as in Medicare's popular Bundled Payments for Care Improvement initiative.[1] A second approach is to decrease rates of inappropriate care, through programs such as Choosing Wisely, that discourage use of low‐value services.[2] Finally, a third approach adopted by the Medicare Shared Savings Program strives to reduce both episode costs and rates of inappropriate care, by incorporating annual per capita Medicare spending into performance benchmarks.[3] Given these ongoing efforts, it would be important to compare the potential impact of reducing episode payments versus rates of care on total costs of care.
METHODS
For 3 common surgical procedures, we compared the relative influence of procedure rates versus episode payments (among those with procedures) on total Medicare expenditures.
We used complete Part A and B Medicare claims data for: coronary artery bypass grafting (CABG), prostatectomy, and hip replacement. We used International Classification of Diseases, Ninth Revision codes to identify the procedures (CABG: 361.0, 361.1, 361.2, 361.3, 361.4, 361.5, 361.6, 361.7, 361.9, 36.2; prostatectomy: 60.4, 60.5, 60.62 with a prostate cancer diagnosis code of 185 or 233.4; and hip replacement: 81.51, 81.52 excluding hip fracture codes 820.0, 820.1, 820.2, 820.3, 820.8, 820.9).
For each procedure, we estimated age‐ and sex‐adjusted episode rates for each hospital referral region (HRR). The numerator was the number of admissions to an acute care hospital for CABG (total n = 118,185), prostatectomy (total n = 18,328), or hip replacement (total n = 178,982) from January 2009 to June 2010. The denominator was fee‐for‐service Medicare beneficiaries age 65 years or older. We excluded those without continuous Part A and B enrollment (total denominator n = 23,403,051). Females were also excluded from the prostatectomy cohort.
For each of the 306 HRRs, we next calculated average HRR‐level episode payments. Using CABG as an example, we aggregated up the risk‐adjusted (age, sex, race, admission type, Elixhauser[4] comorbidities), price‐standardized[5] episode payments for all CABG patients residing in an HRR, and divided this by the number of CABG patients living in that HRR.
Finally, we obtained baseline per capita spending by multiplying the age‐ and sex‐adjusted CABG episode rate by the average CABG episode payment in that HRR. All payments were standardized to 2010 dollars using the Consumer Price Index.
We simulated changes in per capita Medicare spending for CABG across all HRRs under 2 scenarios: (1) reducing HRR‐level rates to the median versus (2) reducing HRR‐level episode payments to the median. We repeated this for prostatectomy and hip replacement.
RESULTS
Age‐ and sex‐adjusted rates of CABG varied more than risk‐adjusted, price‐standardized episode payments (90th:10th percentile of 2.0 for rates vs 1.2 for payments) (see Supporting Information, Appendix, in the online version of this article). Reducing rates of CABG to the 50th percentile decreased per capita episode payments by 11.1%. In contrast, reducing CABG episode payments to the 50th percentile decreased per capita episode payments by 3.6%. The absolute difference between the 2 simulations was 7.5% (95% confidence interval [CI]: 5.6%‐9.4%) (Figure 1). Results were similar for prostatectomy and hip replacement. In sensitivity analyses, reducing hospital‐level episode payments (rather than HRR‐level episode payments) produced similar findings. Employing the 90th percentile as a cutoff (instead of the median) also produced qualitatively similar results.
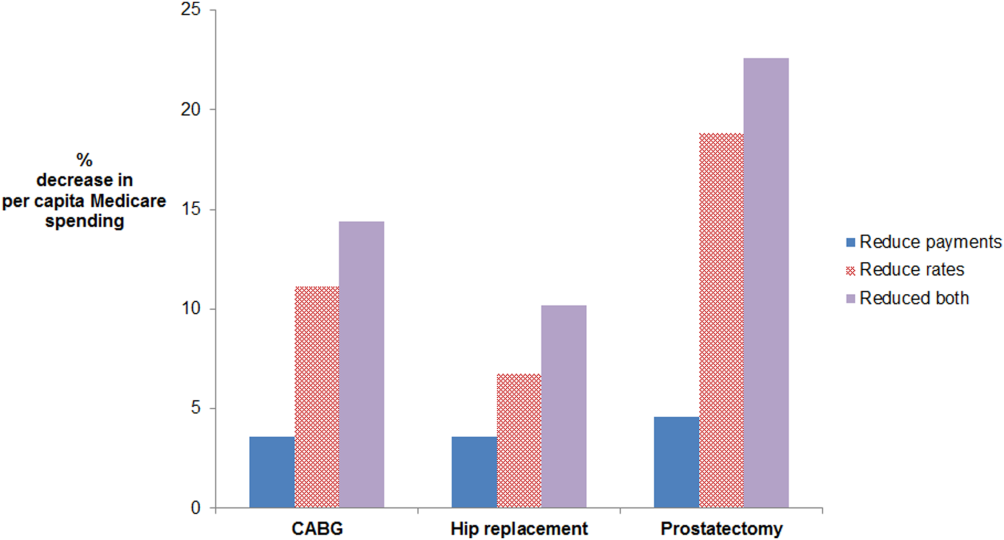
For CABG, the absolute difference between the simulated decrease in per capita Medicare spending due to reducing rates versus reducing payments was 7.5% (95% confidence interval [CI]: 5.6%‐9.4%). For hip replacement, the absolute difference was 3.2% (95% CI: 1.9%‐4.4%). For prostatectomy, the absolute difference was 14.3% (95% CI: 11.0%‐17.6%). The effect of doing both is additive in this simulation, as we did not model possible complex interdependencies between reducing payments and rates. Abbreviations: CABG, coronary artery bypass grafting.
DISCUSSION
For 3 common surgical procedures, reducing procedure rates lowers total Medicare spending substantially more than reducing episode payments. These findings are attributable to a much greater variation in procedure rates compared to episode‐based payments. Prior research has documented wide variation in rates of surgical procedures.[6] This may be due to a number of factors, including physician beliefs about indications for surgery, as well as the degree to which patient preferences are incorporated into decision making.[6]
Our findings suggest that it would be important to incorporate population‐based episode rates into efforts aimed at incentivizing higher value care. Incentives tied to population‐based episode rates are difficult to design well. They may need to be paired with appropriateness criteria to avoid stinting on care. Attribution of a population to a hospital (including those who are not admitted to a hospital) is also complex.[7] Finally, hospitals are not solely responsible for rates of care, because the decision to admit a patient is sometimes made in the emergency department (eg, for chronic medical conditions), but at other times is made in the outpatient arena (eg, for elective surgery). Nevertheless, a narrow focus on per episode spending limits the potential impact of efforts to control Medicare spending.
Acknowledgements
The authors thank Mary Oerline, MS, Yubraj Acharya, MPA, and Haiyin Liu, MA, for the analytic support they provided. They were compensated for their work.
Disclosures: Dr. John Birkmeyer has equity interest in ArborMetrix, a company that profiles hospital quality and episode cost efficiency. The company played no role in the preparation of this article. This work was supported by funding from the National Institute of Aging (grant no. P01AG019783). Dr. Lena Chen is supported by a Career Development Grant Award (K08HS020671) from the Agency for Healthcare Research and Quality. The funders had no role in the design and conduct of the study, in the collection, analysis, and interpretation of the data, and in the preparation, review, or approval of the manuscript.
- Centers for Medicare 370(7):589–592.
- Centers for Medicare 36(1):8–27.
- Prices don't drive regional Medicare spending variations. Health Aff (Millwood). 2010;29(3):537–543. , , , , , .
- Understanding of regional variation in the use of surgery. Lancet. 2013;382(9898):1121–1129. , , , , , .
- Assigning ambulatory patients and their physicians to hospitals: a method for obtaining population‐based provider performance measurements. Health Serv Res. 2007;42(1 pt 1):45–62. , , , .
Payers, providers, and policymakers are testing several major approaches to reducing US healthcare spending without harming quality. One strategy is to bundle payments for a longitudinal episode of care, as in Medicare's popular Bundled Payments for Care Improvement initiative.[1] A second approach is to decrease rates of inappropriate care, through programs such as Choosing Wisely, that discourage use of low‐value services.[2] Finally, a third approach adopted by the Medicare Shared Savings Program strives to reduce both episode costs and rates of inappropriate care, by incorporating annual per capita Medicare spending into performance benchmarks.[3] Given these ongoing efforts, it would be important to compare the potential impact of reducing episode payments versus rates of care on total costs of care.
METHODS
For 3 common surgical procedures, we compared the relative influence of procedure rates versus episode payments (among those with procedures) on total Medicare expenditures.
We used complete Part A and B Medicare claims data for: coronary artery bypass grafting (CABG), prostatectomy, and hip replacement. We used International Classification of Diseases, Ninth Revision codes to identify the procedures (CABG: 361.0, 361.1, 361.2, 361.3, 361.4, 361.5, 361.6, 361.7, 361.9, 36.2; prostatectomy: 60.4, 60.5, 60.62 with a prostate cancer diagnosis code of 185 or 233.4; and hip replacement: 81.51, 81.52 excluding hip fracture codes 820.0, 820.1, 820.2, 820.3, 820.8, 820.9).
For each procedure, we estimated age‐ and sex‐adjusted episode rates for each hospital referral region (HRR). The numerator was the number of admissions to an acute care hospital for CABG (total n = 118,185), prostatectomy (total n = 18,328), or hip replacement (total n = 178,982) from January 2009 to June 2010. The denominator was fee‐for‐service Medicare beneficiaries age 65 years or older. We excluded those without continuous Part A and B enrollment (total denominator n = 23,403,051). Females were also excluded from the prostatectomy cohort.
For each of the 306 HRRs, we next calculated average HRR‐level episode payments. Using CABG as an example, we aggregated up the risk‐adjusted (age, sex, race, admission type, Elixhauser[4] comorbidities), price‐standardized[5] episode payments for all CABG patients residing in an HRR, and divided this by the number of CABG patients living in that HRR.
Finally, we obtained baseline per capita spending by multiplying the age‐ and sex‐adjusted CABG episode rate by the average CABG episode payment in that HRR. All payments were standardized to 2010 dollars using the Consumer Price Index.
We simulated changes in per capita Medicare spending for CABG across all HRRs under 2 scenarios: (1) reducing HRR‐level rates to the median versus (2) reducing HRR‐level episode payments to the median. We repeated this for prostatectomy and hip replacement.
RESULTS
Age‐ and sex‐adjusted rates of CABG varied more than risk‐adjusted, price‐standardized episode payments (90th:10th percentile of 2.0 for rates vs 1.2 for payments) (see Supporting Information, Appendix, in the online version of this article). Reducing rates of CABG to the 50th percentile decreased per capita episode payments by 11.1%. In contrast, reducing CABG episode payments to the 50th percentile decreased per capita episode payments by 3.6%. The absolute difference between the 2 simulations was 7.5% (95% confidence interval [CI]: 5.6%‐9.4%) (Figure 1). Results were similar for prostatectomy and hip replacement. In sensitivity analyses, reducing hospital‐level episode payments (rather than HRR‐level episode payments) produced similar findings. Employing the 90th percentile as a cutoff (instead of the median) also produced qualitatively similar results.
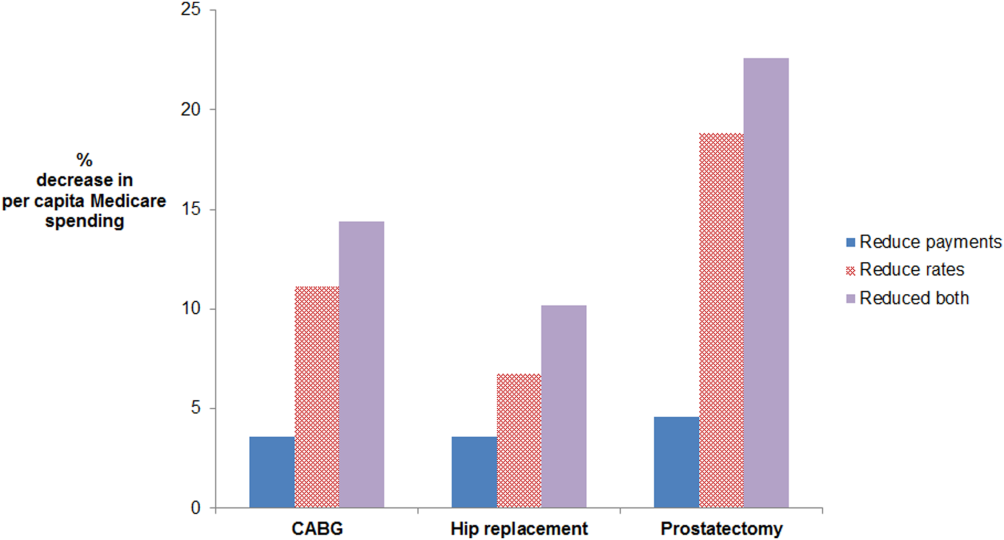
For CABG, the absolute difference between the simulated decrease in per capita Medicare spending due to reducing rates versus reducing payments was 7.5% (95% confidence interval [CI]: 5.6%‐9.4%). For hip replacement, the absolute difference was 3.2% (95% CI: 1.9%‐4.4%). For prostatectomy, the absolute difference was 14.3% (95% CI: 11.0%‐17.6%). The effect of doing both is additive in this simulation, as we did not model possible complex interdependencies between reducing payments and rates. Abbreviations: CABG, coronary artery bypass grafting.
DISCUSSION
For 3 common surgical procedures, reducing procedure rates lowers total Medicare spending substantially more than reducing episode payments. These findings are attributable to a much greater variation in procedure rates compared to episode‐based payments. Prior research has documented wide variation in rates of surgical procedures.[6] This may be due to a number of factors, including physician beliefs about indications for surgery, as well as the degree to which patient preferences are incorporated into decision making.[6]
Our findings suggest that it would be important to incorporate population‐based episode rates into efforts aimed at incentivizing higher value care. Incentives tied to population‐based episode rates are difficult to design well. They may need to be paired with appropriateness criteria to avoid stinting on care. Attribution of a population to a hospital (including those who are not admitted to a hospital) is also complex.[7] Finally, hospitals are not solely responsible for rates of care, because the decision to admit a patient is sometimes made in the emergency department (eg, for chronic medical conditions), but at other times is made in the outpatient arena (eg, for elective surgery). Nevertheless, a narrow focus on per episode spending limits the potential impact of efforts to control Medicare spending.
Acknowledgements
The authors thank Mary Oerline, MS, Yubraj Acharya, MPA, and Haiyin Liu, MA, for the analytic support they provided. They were compensated for their work.
Disclosures: Dr. John Birkmeyer has equity interest in ArborMetrix, a company that profiles hospital quality and episode cost efficiency. The company played no role in the preparation of this article. This work was supported by funding from the National Institute of Aging (grant no. P01AG019783). Dr. Lena Chen is supported by a Career Development Grant Award (K08HS020671) from the Agency for Healthcare Research and Quality. The funders had no role in the design and conduct of the study, in the collection, analysis, and interpretation of the data, and in the preparation, review, or approval of the manuscript.
Payers, providers, and policymakers are testing several major approaches to reducing US healthcare spending without harming quality. One strategy is to bundle payments for a longitudinal episode of care, as in Medicare's popular Bundled Payments for Care Improvement initiative.[1] A second approach is to decrease rates of inappropriate care, through programs such as Choosing Wisely, that discourage use of low‐value services.[2] Finally, a third approach adopted by the Medicare Shared Savings Program strives to reduce both episode costs and rates of inappropriate care, by incorporating annual per capita Medicare spending into performance benchmarks.[3] Given these ongoing efforts, it would be important to compare the potential impact of reducing episode payments versus rates of care on total costs of care.
METHODS
For 3 common surgical procedures, we compared the relative influence of procedure rates versus episode payments (among those with procedures) on total Medicare expenditures.
We used complete Part A and B Medicare claims data for: coronary artery bypass grafting (CABG), prostatectomy, and hip replacement. We used International Classification of Diseases, Ninth Revision codes to identify the procedures (CABG: 361.0, 361.1, 361.2, 361.3, 361.4, 361.5, 361.6, 361.7, 361.9, 36.2; prostatectomy: 60.4, 60.5, 60.62 with a prostate cancer diagnosis code of 185 or 233.4; and hip replacement: 81.51, 81.52 excluding hip fracture codes 820.0, 820.1, 820.2, 820.3, 820.8, 820.9).
For each procedure, we estimated age‐ and sex‐adjusted episode rates for each hospital referral region (HRR). The numerator was the number of admissions to an acute care hospital for CABG (total n = 118,185), prostatectomy (total n = 18,328), or hip replacement (total n = 178,982) from January 2009 to June 2010. The denominator was fee‐for‐service Medicare beneficiaries age 65 years or older. We excluded those without continuous Part A and B enrollment (total denominator n = 23,403,051). Females were also excluded from the prostatectomy cohort.
For each of the 306 HRRs, we next calculated average HRR‐level episode payments. Using CABG as an example, we aggregated up the risk‐adjusted (age, sex, race, admission type, Elixhauser[4] comorbidities), price‐standardized[5] episode payments for all CABG patients residing in an HRR, and divided this by the number of CABG patients living in that HRR.
Finally, we obtained baseline per capita spending by multiplying the age‐ and sex‐adjusted CABG episode rate by the average CABG episode payment in that HRR. All payments were standardized to 2010 dollars using the Consumer Price Index.
We simulated changes in per capita Medicare spending for CABG across all HRRs under 2 scenarios: (1) reducing HRR‐level rates to the median versus (2) reducing HRR‐level episode payments to the median. We repeated this for prostatectomy and hip replacement.
RESULTS
Age‐ and sex‐adjusted rates of CABG varied more than risk‐adjusted, price‐standardized episode payments (90th:10th percentile of 2.0 for rates vs 1.2 for payments) (see Supporting Information, Appendix, in the online version of this article). Reducing rates of CABG to the 50th percentile decreased per capita episode payments by 11.1%. In contrast, reducing CABG episode payments to the 50th percentile decreased per capita episode payments by 3.6%. The absolute difference between the 2 simulations was 7.5% (95% confidence interval [CI]: 5.6%‐9.4%) (Figure 1). Results were similar for prostatectomy and hip replacement. In sensitivity analyses, reducing hospital‐level episode payments (rather than HRR‐level episode payments) produced similar findings. Employing the 90th percentile as a cutoff (instead of the median) also produced qualitatively similar results.
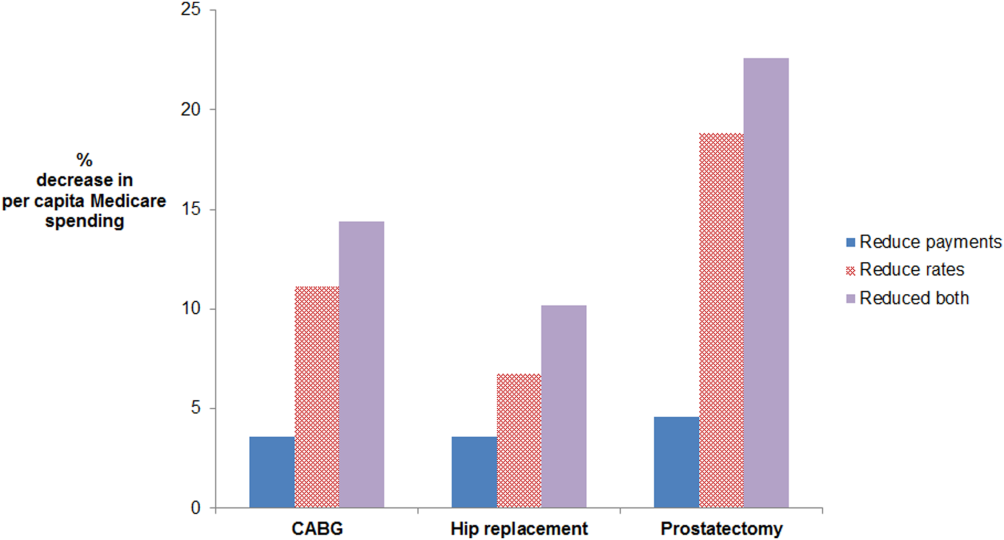
For CABG, the absolute difference between the simulated decrease in per capita Medicare spending due to reducing rates versus reducing payments was 7.5% (95% confidence interval [CI]: 5.6%‐9.4%). For hip replacement, the absolute difference was 3.2% (95% CI: 1.9%‐4.4%). For prostatectomy, the absolute difference was 14.3% (95% CI: 11.0%‐17.6%). The effect of doing both is additive in this simulation, as we did not model possible complex interdependencies between reducing payments and rates. Abbreviations: CABG, coronary artery bypass grafting.
DISCUSSION
For 3 common surgical procedures, reducing procedure rates lowers total Medicare spending substantially more than reducing episode payments. These findings are attributable to a much greater variation in procedure rates compared to episode‐based payments. Prior research has documented wide variation in rates of surgical procedures.[6] This may be due to a number of factors, including physician beliefs about indications for surgery, as well as the degree to which patient preferences are incorporated into decision making.[6]
Our findings suggest that it would be important to incorporate population‐based episode rates into efforts aimed at incentivizing higher value care. Incentives tied to population‐based episode rates are difficult to design well. They may need to be paired with appropriateness criteria to avoid stinting on care. Attribution of a population to a hospital (including those who are not admitted to a hospital) is also complex.[7] Finally, hospitals are not solely responsible for rates of care, because the decision to admit a patient is sometimes made in the emergency department (eg, for chronic medical conditions), but at other times is made in the outpatient arena (eg, for elective surgery). Nevertheless, a narrow focus on per episode spending limits the potential impact of efforts to control Medicare spending.
Acknowledgements
The authors thank Mary Oerline, MS, Yubraj Acharya, MPA, and Haiyin Liu, MA, for the analytic support they provided. They were compensated for their work.
Disclosures: Dr. John Birkmeyer has equity interest in ArborMetrix, a company that profiles hospital quality and episode cost efficiency. The company played no role in the preparation of this article. This work was supported by funding from the National Institute of Aging (grant no. P01AG019783). Dr. Lena Chen is supported by a Career Development Grant Award (K08HS020671) from the Agency for Healthcare Research and Quality. The funders had no role in the design and conduct of the study, in the collection, analysis, and interpretation of the data, and in the preparation, review, or approval of the manuscript.
- Centers for Medicare 370(7):589–592.
- Centers for Medicare 36(1):8–27.
- Prices don't drive regional Medicare spending variations. Health Aff (Millwood). 2010;29(3):537–543. , , , , , .
- Understanding of regional variation in the use of surgery. Lancet. 2013;382(9898):1121–1129. , , , , , .
- Assigning ambulatory patients and their physicians to hospitals: a method for obtaining population‐based provider performance measurements. Health Serv Res. 2007;42(1 pt 1):45–62. , , , .
- Centers for Medicare 370(7):589–592.
- Centers for Medicare 36(1):8–27.
- Prices don't drive regional Medicare spending variations. Health Aff (Millwood). 2010;29(3):537–543. , , , , , .
- Understanding of regional variation in the use of surgery. Lancet. 2013;382(9898):1121–1129. , , , , , .
- Assigning ambulatory patients and their physicians to hospitals: a method for obtaining population‐based provider performance measurements. Health Serv Res. 2007;42(1 pt 1):45–62. , , , .
Epidemiology of Organ System Dysfunction
The International Consensus Conference (ICC) for sepsis defines severe sepsis as an infection leading to acute organ dysfunction.[1, 2] Severe sepsis afflicts over 1 million patients each year in Medicare alone, and is substantially more common among older Americans than acute myocardial infarction.[3, 4, 5] Recently, the Agency for Healthcare Research and Quality identified severe sepsis as the single most expensive cause of hospitalization in the United States.[6] The incidence of severe sepsis continues to rise.[4, 5]
Severe sepsis is often mischaracterized as a diagnosis cared for primarily in the intensive care unit (ICU). Yet, studies indicate that only 32% to 50% of patients with severe sepsis require ICU care, leaving the majority on the general care wards.[7, 8] These studies also reveal mortality rates of 26% to 30% among patients with severe sepsis who are not admitted to an ICU compared to 11% to 33% in the ICU.[7, 8]
Although a number of epidemiologic and interventional studies have focused on severe sepsis in the ICU,[3, 9, 10] much less is known about patients cared for on the general medicine wards. Without this information, clinicians cannot make informed choices about important management decisions such as targeted diagnostic testing, empirical antimicrobials, and other therapies. To this end, we sought to further characterize the infectious etiologies and resultant organ system dysfunctions in the subset of patients with severe sepsis admitted to non‐ICU medical services at a tertiary academic medical center.
METHODS
Population/Setting
All hospitalizations of adult patients (18 years old) who were initially admitted to non‐ICU medical services at the University of Michigan Hospital during 2009 through 2010 were included. The University of Michigan Hospital has 610 general medical‐surgical beds, including telemetry beds, with closed ICUs comprised of 179 beds staffed by intensivists. Patients transferred from other hospitals and those admitted to non‐medical services were excluded.
Data Abstraction and Definitions
All International Classification of Diseases, 9th Revision, Clinical Modification (ICD‐9‐CM) diagnosis codes for hospitalizations were screened using a previously published and validated algorithm for severe sepsis.[11] Following this screening, 3 randomly selected round‐numbered batches of hospitalizations were sampled with subsequent application of the exclusion criteria. Medical records including physicians' notes, consultants' notes, nurses' notes, physical therapy notes, discharge coordinators' notes, emergency room flow sheets, as well as ward flow sheets were reviewed in detail by 3 practicing hospitalists using a structured instrument closely aligned with the ICC definition of severe sepsis.[2] We also sampled a smaller number of patients whose ICD‐9‐CM diagnoses screened negative for severe sepsis. Sample size was selected as part of a project with multiple objectives, and reflected a pragmatic balance between the anticipated precision of the results and the resources available to conduct chart review.[11] All discrepancies were reconciled among the 3 reviewers.
Reviewers first assessed whether infection was present, then evaluated for evidence of each organ system dysfunction, and finally determined the extent to which those organ dysfunctions were a response to the infection. Infection was defined either as a patient with a microbiologic culture growing a pathologic organism in a normally sterile site or documentation of a suspected infection with other confirmatory evidence (radiological, physical exam finding) with resultant systemic inflammatory response and administration of antimicrobials. Community‐acquired and healthcare‐associated infections were not differentiated. Microbiologic data, confirmatory tests, and site of infection were abstracted in detail.
Organ dysfunction was defined as per the 2001 ICC criteria,[2] and was assessed for neurological, pulmonary, cardiovascular, renal, gastrointestinal, hematological, and hepatic system involvement in all patients. A summary of these clinical definitions is included in Table 1. Data on important comorbidities were also abstracted. Immunosuppression was defined as having any of the following: solid organ transplant, bone marrow/stem cell transplant, human immunodeficiency virus/acquired immunodeficiency syndrome, neutropenia (absolute neutrophil count <1000), hematologic malignancy, solid organ malignancy with chemotherapy within the past 12 months, or pharmacologic immunosuppression (prednisone >20 mg daily for >4 weeks, calcineurin inhibitor, methotrexate, tumor necrosis factor inhibitors, azathioprine, sulfasalazine, hydroxychloroquine). Last, each chart was evaluated for the presence of explicit documentation with the presence of the words or phrases: sepsis, septic shock, or severe sepsis, indicating that the clinical service recognized and fully documented that a patient had severe sepsis.
Organ System | Parameters to Indicate Dysfunction |
---|---|
| |
Cardiovascular | Systolic BP <90, elevated lactate, MAP <70, requiring pressors >2 hours, decrease in systolic BP of >40 |
Renal | Creatinine increase >0.5 mg/dL, oliguria |
Neurological | Acute mental status changes |
Pulmonary | Intubation, BiPAP, supplemental oxygen >6 LPM or 40% face mask, PaO2/FiO2 <300 |
Hematologic | INR >1.5 or PTT >60 not on anticoagulation, platelets <100 or 50% of baseline |
Ileus | Decreased bowel motility requiring a change in diet |
Hepatic | Bilirubin >4 mg/dL and >1.5 baseline |
Data Analysis
Methods for assessment of reviewer concordance have been previously described and were summarized using the kappa statistic.[11] Initial data extraction was performed in SAS 9.1 (SAS Institute, Cary, NC) and all analyses were conducted in Stata 12 (StataCorp LP, College Station, TX). Binomial 95% confidence intervals (CIs) are presented. This project was approved by the University of Michigan Institutional Review Board.
RESULTS
Of 23,288 hospitalizations examined from 2009 through 2010, the ICD‐9based automated screen for severe sepsis was positive for 3,146 (14 %). A random sample of 111 medical records, of which 92 had screened positive for severe sepsis and 19 had screened negative, was reviewed in detail. After review by the hospitalists, 64 of these 111 hospitalizations were judged to have severe sepsis, 61 of the 92 screened positive cases (66%), and 3 of the 19 screened negative cases (16%). The 3 reviewers had a kappa of 0.70, indicating good agreement.
Characteristics of the 64 patients with severe sepsis are shown in Table 2. The mean age was 63 years old (standard deviation [SD]=17.7), and 41% were male. The mean length of stay was 13.7 days (SD=20.8). Thirty‐nine percent (95% CI, 27%‐52%) of patients (25/64) were immunosuppressed. Of patients initially admitted to the general medical ward, 25% (16/64; 95% CI, 15%‐37%) ultimately required ICU care during their admission. The overall in‐hospital mortality rate was 13% (8/64; 95% CI, 6%‐23%). Immunosuppressed patients had a mortality rate of 20% and nonimmunosuppressed patients had a mortality rate of 8%. Only 47% (30/64; 95% CI, 34%‐60%) of the medical records had explicit clinician documentation of severe sepsis.
Age, mean (SD), y | 63 (18) |
---|---|
| |
Male sex, no. (%) | 26 (41) |
Preexisting conditions, no. (%) | |
History of diabetes | 20 (31) |
End stage renal disease on chronic dialysis | 2 (3) |
Chronic obstructive pulmonary disease on oxygen | 3 (5) |
History of cancer | 15 (23) |
Liver cirrhosis | 5 (8) |
Immunosuppression | 25 (39) |
Median length of stay (days) | 7.5 |
Mean length of stay (SD) | 13.7 (20.8) |
The most common site of infection was found to be the genitourinary system, occurring in 41% (26/64; 95% CI, 29%‐54%) of patients (Table 3). Pulmonary and intra‐abdominal sites were also common, accounting for 14% (95% CI, 6.6%‐25%) and 13% (95% CI, 5.6%‐23%) of sites, respectively. An infecting organism was identified by culture in 66% (42/64; 95% CI, 53%‐77%) of case patients with specific pathogens listed in Table 4. Among patients with positive culture results, the majority grew Gram‐negative organisms (57%; 95% CI, 41%‐72%). Non‐Clostridium difficile Gram‐positive organisms were also prominent and identified in 48% (95% CI, 32%‐64%) of positive cultures. Candida was less common (12%, 95% CI, 4.0%‐26%). Fourteen cases (22%, 95% CI, 10%‐30%) had 2 or more concomitant infectious pathogens.
Site | No. (%) |
---|---|
| |
Genitourinary | 26 (41) |
Pulmonary | 9 (14) |
Intra‐abdominal (not intraluminal) | 8 (13) |
Bloodstream/cardiac | 5 (8) |
Skin and soft tissue | 4 (6) |
GI lumen | 4 (6) |
Joint | 2 (3) |
Multiple sites | 4 (6) |
Unknown | 2 (2) |
Absolute Frequency, Total Positive Culture Results, N=64, No. (%)*?>a | Patients With Cultures Growing at Least One of the Pathogens, N=42, No. (%)*?>a | |
---|---|---|
| ||
Gram‐negative pathogens | 30 (47) | 24 (57) |
Escherichia coli | 12 (19) | 12 (29) |
Escherichia coli (multidrug resistant) | 2 (3) | 2 (5) |
Klebsiella | 6 (9) | 5 (12) |
Pseudomonas aeruginosa | 6 (9) | 4 (10) |
Pseudomonas aeruginosa (multidrug resistant) | 2 (3) | 2 (5) |
Otherb | 6 (9) | 6 (14) |
Gram‐positive pathogens | 29 (45) | 25 (59) |
Enterococcus | 14 (22) | 13 (31) |
Vancomycin‐resistant Enterococcus species | 5 (8) | 4 (10) |
Staphylococcus aureus | 7 (11) | 7 (17) |
Methicillin‐resistant Staphylococcus aureus | 3 (5) | 3 (7) |
Streptococcus pneumoniae | 2 (3) | 2 (5) |
Coagulase‐negative staphylococci | 1 (2) | 1 (2) |
Clostridium difficile | 5 (8) | 5 (12) |
Fungi | ||
Candida species | 5 (8) | 5 (12) |
Mycobacterium avium | 1 (2) | 1 (2) |
Two organisms | 9 (21) | |
Three or more organisms | 5 (12) |
All 64 patients had at least 1 organ dysfunction, as required by the ICC definition of severe sepsis. Organ dysfunction in 2 or more organ systems occurred in 77% (95% CI, 64%‐86%) of the cases (49/64). The incidence for each organ system dysfunction is presented in Table 5, as well as its relationship to both mortality and ICU admission. The most common organ system dysfunctions were found to be cardiovascular (hypotension) and renal dysfunction occurring in 66% and 64% of the cases, respectively. In this non‐ICU population, pulmonary dysfunction occurred in 30% of cases, but was frequently associated with transfer to the ICU, as 63% of the patients with pulmonary failure required ICU care. Patients with more organ systems affected were more likely to be transferred to the ICU and to die.
No. (%) | ICU Transfer, No. (%) | Mortality, No. (%) | |
---|---|---|---|
| |||
Number of failed organs, N = 64 | |||
1 | 15 (23%) | 0 (0%) | 0 (0%) |
2 | 25 (39%) | 2 (8%) | 0 (0%) |
3 | 7 (11%) | 2 (29%) | 1 (14%) |
4 | 10 (16%) | 6 (60%) | 3 (30%) |
>4 | 7 (11%) | 6 (86%) | 4 (57%) |
Types of organ system dysfunction, all patients, N = 64*?>a | |||
Cardiovascular | 42 (66%) | 16 (38%)b | 8 (19%)c |
Renal | 41 (64%) | 10 (24%)b | 5 (12%)c |
Central nervous system | 35 (54%) | 14 (40%)b | 7 (18%)c |
Pulmonary | 19 (30%) | 12 (63%)b | 8 (42%)c |
Hematologic | 15 (23%) | 6 (40%)b | 6 (40%)c |
GI (ileus) | 8 (13%) | 5 (63%)b | 1 (13%)c |
Hepatic | 5 (8%) | 4 (80%)b | 2 (40%)c |
DISCUSSION
Severe sepsis was common among patients admitted to the general medical ward in this tertiary care center. Our patient cohort differed in important ways from previously described typical cases of severe sepsis among ICU populations. Severe sepsis on the general medical wards was more commonly associated with Gram‐negative pathogens in the setting of genitourinary tract infections. This is in contrast to Gram‐positive organisms and respiratory tract infections, which are more common in the ICU.[3, 10] Renal and cardiac dysfunction were commonly observed organ failures, whereas in the ICU, severe sepsis has been reported to more likely involve respiratory failure. These results suggest that hospitalists seeking to provide evidence‐based care to prevent postsepsis morbidity and mortality for their non‐ICU patients need to heighten their index of suspicion when caring for an infected patient and appreciate that many severe sepsis patients may not fit neatly into traditional sepsis treatment algorithms.
Studies characterizing severe sepsis in the ICU setting indicate a predominance of pulmonary infections and respiratory failure with occurrence rates of 74% to 95% and 54% to 61%, respectively.[3, 12, 13] Given that either shock or pulmonary dysfunction is often required for admission to many ICUs, it is perhaps not surprising that these rates are dramatically different on the general medicine ward, with a relative scarcity of pulmonary infections (14%) and respiratory dysfunction (30%). Instead, genitourinary infections were noted in 41% (95% CI, 29%‐54%) of the cases, in contrast to the rates of genitourinary infections in ICU patients with severe sepsis, which have rates of 5.4% to 9.1%.[3, 10] Likely as a result of this, a Gram‐negative predominance is noted in the associated microbiology. Furthermore, our study indicates that C difficile and vancomycin‐resistant Enterococcus (VRE) species appear to represent an emerging cause of severe sepsis on the general medicine wards, as they have not been noted to be causative micro‐organisms in previous studies of sepsis. This is concordant with other studies showing increases in incidence and severity of disease for C difficile as well as VRE.[14, 15]
Previous epidemiologic studies of severe sepsis originating outside the ICU are lacking, but some work has been done. One study on the epidemiology of sepsis both with and without organ dysfunction aggregated all hospitalized patients and included those both admitted to the general medicine wards and directly to the ICU.[7] Similar to our study, this study also found a predominance of Gram‐negative causative organisms, as well as comparable in‐hospital mortality rates (12.8% vs 13%). Additionally, genitourinary infections were noted in 20% of the patients, notably higher than rates reported to have been found in patients with severe sepsis in the ICU, but not the magnitude found in our study, perhaps as a result of the combined ICU‐ward population studied. A similar high prevalence of genitourinary infections was also noted in a recent administrative data‐based study of emergency medical services‐transported patients with severe sepsis, half of whom required intensive care during their hospitalization.[16]
Our study is unique in that it focuses on severe sepsis in patients, commonly cared for by hospitalists, who were admitted to the general medical ward, and uses patient level data to elucidate more characteristics of the defining organ dysfunction. Furthermore, our results suggest that severe sepsis was poorly documented in this setting, indicating a potential impact on billing, coding, case mix index, and hospital mortality statistics that rely on very specific wording, as well as a possible need for increased awareness among hospitalists. Without this awareness, an opportunity may be missed for improved patient care via specific sepsis‐targeted measures,[13, 17, 18] including more aggressive resuscitative measures[19] or intensive physical and occupational therapy interventions aimed at impacting the cognitive and functional debilities[20] that result from severe sepsis. Highlighting this growing need to better assist clinicians assess the severity of septic patients and recognize these complex cases on the general medicine wards, 1 recent study evaluated the fitness of several clinical disease‐severity scoring systems for patients with sepsis in general internal medicine departments.[21] Perhaps with the help of tools such as these, which are being piloted in some hospitals, the care of this growing population can be enhanced.
Our study has a number of limitations that should be kept in mind. First, this is a single center study performed at an academic tertiary care center with a relatively high incidence of immunosuppression, which may influence the spectrum of infecting organisms. Our center also has a relatively large, closed‐model ICU, which often operates at near capacity, potentially affecting the severity of our non‐ICU population. Second, although we screened a large number of patients, as necessitated by our intensive and detailed review of clinical information, our sample size with hospitalist‐validated severe sepsis is relatively small. With this small sample size, less prevalent infections, patient characteristics, and organ dysfunctions may by chance have been under or over‐represented, and one could expect some variance in the occurrence rates of organ system dysfunction and infection rates by sampling error alone. Further larger scale studies are warranted to confirm these data and their generalizability. Third, the data necessary to calculate sequential organ failure assessment or multiple organ dysfunction score were not collected. This may limit the ability to directly compare the organ dysfunction noted in this study with others. Additionally, given the ICC definitions of organ dysfunction, some of the organ dysfunction noted, particularly for neurological dysfunction, was reliant on subjective clinical findings documented in the record. Finally, we relied on the lack of specific terminology to indicate a lack of documentation of sepsis, which does not necessarily indicate a lack of recognition or undertreatment of this condition. However, these limitations are offset by the strengths of this study, including the patient‐level medical record validation of severe sepsis by trained hospitalist physicians, high kappa statistic, and strict application of guideline‐based definitions.
This work has important implications for both clinicians and for future research on severe sepsis. The results suggest that severe sepsis may be quite common outside the ICU, and that patients presenting with this condition who are admitted to general medical wards are not routinely characterized by the profound hypoxemia and refractory shock of iconic cases. Certainly, further study looking at larger numbers of cases is needed to better understand the specifics and nuances of this important topic as well as to further evaluate clinicians' ability to recognize and treat such patients in this setting. Furthermore, future research on the treatment of severe sepsis, including both antimicrobials and disease‐modifying agents (eg, anti‐inflammatories) must continue to include and even focus on this large population of non‐ICU patients with severe sepsis, as the risk/benefit ratios of such potential treatments may vary with severity of illness.
In conclusion, severe sepsis was commonly found in patients admitted on the general medicine wards. The epidemiology of the infections and resultant organ dysfunction appears to differ from that found in the ICU. More studies are needed to provide a deeper understanding of this disease process, as this will enable clinicians to better recognize and treat patients thus afflicted, no matter the setting.
Acknowledgments
The authors thank Laetitia Shapiro, AM, for her programming assistance.
Disclosures: This work was supported in part by the US National Institutes of HealthK08, HL091249 (TJI) and the University of Michigan SpecialistHospitalist Allied Research Program (SHARP). This work was also supported in part by VA Ann Arbor Healthcare System, Geriatric Research Education and Clinical Center (GRECC).
- Definitions for sepsis and organ failure and guidelines for the use of innovative therapies in sepsis. The ACCP/SCCM Consensus Conference Committee. American College of Chest Physicians/Society of Critical Care Medicine. Chest. 1992;101(6):1644–1655. , , , et al.
- 2001 SCCM/ESICM/ACCP/ATS/SIS International Sepsis Definitions Conference. Crit Care Med. 2003;31(4):1250–1256. , , , et al.
- Epidemiology of severe sepsis in the United States: analysis of incidence, outcome, and associated costs of care. Crit Care Med. 2001;29(7):1303–1310. , , , , , .
- Population burden of long‐term survivorship after severe sepsis in older americans. J Am Geriatr Soc. 2012;60(6):1070–1077. , , , .
- The epidemiology of sepsis in the United States from 1979 through 2000. N Engl J Med. 2003;348(16):1546–1554. , , , .
- Septicemia in U.S. hospitals, 2009: statistical brief #122. October 2011. In: Healthcare Cost and Utilization Project Statistical Briefs. Rockville, MD: Agency for Health Care Policy and Research; 2006. Available from: http://www.ncbi.nlm.nih.gov/books/NBK65391. Accessed June 2, 2012. , , .
- Sepsis incidence and outcome: contrasting the intensive care unit with the hospital ward. Crit Care Med. 2007;35(5):1284–1289. , , , et al.
- Epidemiology of sepsis in Victoria, Australia. Crit Care Med. 2005;33(1):71–80. , , , , .
- Effect of empirical treatment with moxifloxacin and meropenem vs meropenem on sepsis‐related organ dysfunction in patients with severe sepsis: a randomized trial. JAMA. 2012;307(22):2390–2399. , , , et al.
- Incidence and impact of organ dysfunctions associated with sepsis. Chest. 2005;127(3):942–951. , , , , .
- Identifying patients with severe sepsis using administrative claims: patient‐level validation of the Angus Implementation of the International Consensus Conference definition of severe sepsis [published online ahead of print September 18, 2012]. Medical Care. doi: 10.1097/MLR.0b013e318268ac86. , , , et al.
- Current epidemiology of septic shock: the CUB‐Rea Network. Am J Respir Crit Care Med. 2003;168(2):165–172. , , , .
- Management of sepsis. N Engl J Med. 2006;355(16):1699–1713. .
- Current status of Clostridium difficile infection ipidemiology. Clin Infect Dis. 2012;55(suppl 2):S65–S70. , , .
- Vancomycin‐resistant enterococci. Semin Respir Infect. 2000;15(4):314–326. , .
- Severe sepsis in prehospital emergency care: analysis of incidence, care, and outcome. Am J Respir Crit Care Med. 2012;186(12):1264–1271. , , , , , .
- Novel Therapies for Septic Shock Over the Past 4 Decades. JAMA. 2011;306(2):194–199. , .
- Impact of the Surviving Sepsis Campaign protocols on hospital length of stay and mortality in septic shock patients: results of a three‐year follow‐up quasi‐experimental study. Crit Care Med. 2010;38(4):1036–1043. , , , et al.
- Diagnosis and treatment of severe sepsis. Crit Care. 2007;11(suppl 5):S2. , .
- Long‐term cognitive impairment and functional disability among survivors of severe sepsis. JAMA. 2010;304(16):1787–1794. , , , .
- Assessment of disease‐severity scoring systems for patients with sepsis in general internal medicine departments. Crit Care. 2011;15:R95. , , , , .
The International Consensus Conference (ICC) for sepsis defines severe sepsis as an infection leading to acute organ dysfunction.[1, 2] Severe sepsis afflicts over 1 million patients each year in Medicare alone, and is substantially more common among older Americans than acute myocardial infarction.[3, 4, 5] Recently, the Agency for Healthcare Research and Quality identified severe sepsis as the single most expensive cause of hospitalization in the United States.[6] The incidence of severe sepsis continues to rise.[4, 5]
Severe sepsis is often mischaracterized as a diagnosis cared for primarily in the intensive care unit (ICU). Yet, studies indicate that only 32% to 50% of patients with severe sepsis require ICU care, leaving the majority on the general care wards.[7, 8] These studies also reveal mortality rates of 26% to 30% among patients with severe sepsis who are not admitted to an ICU compared to 11% to 33% in the ICU.[7, 8]
Although a number of epidemiologic and interventional studies have focused on severe sepsis in the ICU,[3, 9, 10] much less is known about patients cared for on the general medicine wards. Without this information, clinicians cannot make informed choices about important management decisions such as targeted diagnostic testing, empirical antimicrobials, and other therapies. To this end, we sought to further characterize the infectious etiologies and resultant organ system dysfunctions in the subset of patients with severe sepsis admitted to non‐ICU medical services at a tertiary academic medical center.
METHODS
Population/Setting
All hospitalizations of adult patients (18 years old) who were initially admitted to non‐ICU medical services at the University of Michigan Hospital during 2009 through 2010 were included. The University of Michigan Hospital has 610 general medical‐surgical beds, including telemetry beds, with closed ICUs comprised of 179 beds staffed by intensivists. Patients transferred from other hospitals and those admitted to non‐medical services were excluded.
Data Abstraction and Definitions
All International Classification of Diseases, 9th Revision, Clinical Modification (ICD‐9‐CM) diagnosis codes for hospitalizations were screened using a previously published and validated algorithm for severe sepsis.[11] Following this screening, 3 randomly selected round‐numbered batches of hospitalizations were sampled with subsequent application of the exclusion criteria. Medical records including physicians' notes, consultants' notes, nurses' notes, physical therapy notes, discharge coordinators' notes, emergency room flow sheets, as well as ward flow sheets were reviewed in detail by 3 practicing hospitalists using a structured instrument closely aligned with the ICC definition of severe sepsis.[2] We also sampled a smaller number of patients whose ICD‐9‐CM diagnoses screened negative for severe sepsis. Sample size was selected as part of a project with multiple objectives, and reflected a pragmatic balance between the anticipated precision of the results and the resources available to conduct chart review.[11] All discrepancies were reconciled among the 3 reviewers.
Reviewers first assessed whether infection was present, then evaluated for evidence of each organ system dysfunction, and finally determined the extent to which those organ dysfunctions were a response to the infection. Infection was defined either as a patient with a microbiologic culture growing a pathologic organism in a normally sterile site or documentation of a suspected infection with other confirmatory evidence (radiological, physical exam finding) with resultant systemic inflammatory response and administration of antimicrobials. Community‐acquired and healthcare‐associated infections were not differentiated. Microbiologic data, confirmatory tests, and site of infection were abstracted in detail.
Organ dysfunction was defined as per the 2001 ICC criteria,[2] and was assessed for neurological, pulmonary, cardiovascular, renal, gastrointestinal, hematological, and hepatic system involvement in all patients. A summary of these clinical definitions is included in Table 1. Data on important comorbidities were also abstracted. Immunosuppression was defined as having any of the following: solid organ transplant, bone marrow/stem cell transplant, human immunodeficiency virus/acquired immunodeficiency syndrome, neutropenia (absolute neutrophil count <1000), hematologic malignancy, solid organ malignancy with chemotherapy within the past 12 months, or pharmacologic immunosuppression (prednisone >20 mg daily for >4 weeks, calcineurin inhibitor, methotrexate, tumor necrosis factor inhibitors, azathioprine, sulfasalazine, hydroxychloroquine). Last, each chart was evaluated for the presence of explicit documentation with the presence of the words or phrases: sepsis, septic shock, or severe sepsis, indicating that the clinical service recognized and fully documented that a patient had severe sepsis.
Organ System | Parameters to Indicate Dysfunction |
---|---|
| |
Cardiovascular | Systolic BP <90, elevated lactate, MAP <70, requiring pressors >2 hours, decrease in systolic BP of >40 |
Renal | Creatinine increase >0.5 mg/dL, oliguria |
Neurological | Acute mental status changes |
Pulmonary | Intubation, BiPAP, supplemental oxygen >6 LPM or 40% face mask, PaO2/FiO2 <300 |
Hematologic | INR >1.5 or PTT >60 not on anticoagulation, platelets <100 or 50% of baseline |
Ileus | Decreased bowel motility requiring a change in diet |
Hepatic | Bilirubin >4 mg/dL and >1.5 baseline |
Data Analysis
Methods for assessment of reviewer concordance have been previously described and were summarized using the kappa statistic.[11] Initial data extraction was performed in SAS 9.1 (SAS Institute, Cary, NC) and all analyses were conducted in Stata 12 (StataCorp LP, College Station, TX). Binomial 95% confidence intervals (CIs) are presented. This project was approved by the University of Michigan Institutional Review Board.
RESULTS
Of 23,288 hospitalizations examined from 2009 through 2010, the ICD‐9based automated screen for severe sepsis was positive for 3,146 (14 %). A random sample of 111 medical records, of which 92 had screened positive for severe sepsis and 19 had screened negative, was reviewed in detail. After review by the hospitalists, 64 of these 111 hospitalizations were judged to have severe sepsis, 61 of the 92 screened positive cases (66%), and 3 of the 19 screened negative cases (16%). The 3 reviewers had a kappa of 0.70, indicating good agreement.
Characteristics of the 64 patients with severe sepsis are shown in Table 2. The mean age was 63 years old (standard deviation [SD]=17.7), and 41% were male. The mean length of stay was 13.7 days (SD=20.8). Thirty‐nine percent (95% CI, 27%‐52%) of patients (25/64) were immunosuppressed. Of patients initially admitted to the general medical ward, 25% (16/64; 95% CI, 15%‐37%) ultimately required ICU care during their admission. The overall in‐hospital mortality rate was 13% (8/64; 95% CI, 6%‐23%). Immunosuppressed patients had a mortality rate of 20% and nonimmunosuppressed patients had a mortality rate of 8%. Only 47% (30/64; 95% CI, 34%‐60%) of the medical records had explicit clinician documentation of severe sepsis.
Age, mean (SD), y | 63 (18) |
---|---|
| |
Male sex, no. (%) | 26 (41) |
Preexisting conditions, no. (%) | |
History of diabetes | 20 (31) |
End stage renal disease on chronic dialysis | 2 (3) |
Chronic obstructive pulmonary disease on oxygen | 3 (5) |
History of cancer | 15 (23) |
Liver cirrhosis | 5 (8) |
Immunosuppression | 25 (39) |
Median length of stay (days) | 7.5 |
Mean length of stay (SD) | 13.7 (20.8) |
The most common site of infection was found to be the genitourinary system, occurring in 41% (26/64; 95% CI, 29%‐54%) of patients (Table 3). Pulmonary and intra‐abdominal sites were also common, accounting for 14% (95% CI, 6.6%‐25%) and 13% (95% CI, 5.6%‐23%) of sites, respectively. An infecting organism was identified by culture in 66% (42/64; 95% CI, 53%‐77%) of case patients with specific pathogens listed in Table 4. Among patients with positive culture results, the majority grew Gram‐negative organisms (57%; 95% CI, 41%‐72%). Non‐Clostridium difficile Gram‐positive organisms were also prominent and identified in 48% (95% CI, 32%‐64%) of positive cultures. Candida was less common (12%, 95% CI, 4.0%‐26%). Fourteen cases (22%, 95% CI, 10%‐30%) had 2 or more concomitant infectious pathogens.
Site | No. (%) |
---|---|
| |
Genitourinary | 26 (41) |
Pulmonary | 9 (14) |
Intra‐abdominal (not intraluminal) | 8 (13) |
Bloodstream/cardiac | 5 (8) |
Skin and soft tissue | 4 (6) |
GI lumen | 4 (6) |
Joint | 2 (3) |
Multiple sites | 4 (6) |
Unknown | 2 (2) |
Absolute Frequency, Total Positive Culture Results, N=64, No. (%)*?>a | Patients With Cultures Growing at Least One of the Pathogens, N=42, No. (%)*?>a | |
---|---|---|
| ||
Gram‐negative pathogens | 30 (47) | 24 (57) |
Escherichia coli | 12 (19) | 12 (29) |
Escherichia coli (multidrug resistant) | 2 (3) | 2 (5) |
Klebsiella | 6 (9) | 5 (12) |
Pseudomonas aeruginosa | 6 (9) | 4 (10) |
Pseudomonas aeruginosa (multidrug resistant) | 2 (3) | 2 (5) |
Otherb | 6 (9) | 6 (14) |
Gram‐positive pathogens | 29 (45) | 25 (59) |
Enterococcus | 14 (22) | 13 (31) |
Vancomycin‐resistant Enterococcus species | 5 (8) | 4 (10) |
Staphylococcus aureus | 7 (11) | 7 (17) |
Methicillin‐resistant Staphylococcus aureus | 3 (5) | 3 (7) |
Streptococcus pneumoniae | 2 (3) | 2 (5) |
Coagulase‐negative staphylococci | 1 (2) | 1 (2) |
Clostridium difficile | 5 (8) | 5 (12) |
Fungi | ||
Candida species | 5 (8) | 5 (12) |
Mycobacterium avium | 1 (2) | 1 (2) |
Two organisms | 9 (21) | |
Three or more organisms | 5 (12) |
All 64 patients had at least 1 organ dysfunction, as required by the ICC definition of severe sepsis. Organ dysfunction in 2 or more organ systems occurred in 77% (95% CI, 64%‐86%) of the cases (49/64). The incidence for each organ system dysfunction is presented in Table 5, as well as its relationship to both mortality and ICU admission. The most common organ system dysfunctions were found to be cardiovascular (hypotension) and renal dysfunction occurring in 66% and 64% of the cases, respectively. In this non‐ICU population, pulmonary dysfunction occurred in 30% of cases, but was frequently associated with transfer to the ICU, as 63% of the patients with pulmonary failure required ICU care. Patients with more organ systems affected were more likely to be transferred to the ICU and to die.
No. (%) | ICU Transfer, No. (%) | Mortality, No. (%) | |
---|---|---|---|
| |||
Number of failed organs, N = 64 | |||
1 | 15 (23%) | 0 (0%) | 0 (0%) |
2 | 25 (39%) | 2 (8%) | 0 (0%) |
3 | 7 (11%) | 2 (29%) | 1 (14%) |
4 | 10 (16%) | 6 (60%) | 3 (30%) |
>4 | 7 (11%) | 6 (86%) | 4 (57%) |
Types of organ system dysfunction, all patients, N = 64*?>a | |||
Cardiovascular | 42 (66%) | 16 (38%)b | 8 (19%)c |
Renal | 41 (64%) | 10 (24%)b | 5 (12%)c |
Central nervous system | 35 (54%) | 14 (40%)b | 7 (18%)c |
Pulmonary | 19 (30%) | 12 (63%)b | 8 (42%)c |
Hematologic | 15 (23%) | 6 (40%)b | 6 (40%)c |
GI (ileus) | 8 (13%) | 5 (63%)b | 1 (13%)c |
Hepatic | 5 (8%) | 4 (80%)b | 2 (40%)c |
DISCUSSION
Severe sepsis was common among patients admitted to the general medical ward in this tertiary care center. Our patient cohort differed in important ways from previously described typical cases of severe sepsis among ICU populations. Severe sepsis on the general medical wards was more commonly associated with Gram‐negative pathogens in the setting of genitourinary tract infections. This is in contrast to Gram‐positive organisms and respiratory tract infections, which are more common in the ICU.[3, 10] Renal and cardiac dysfunction were commonly observed organ failures, whereas in the ICU, severe sepsis has been reported to more likely involve respiratory failure. These results suggest that hospitalists seeking to provide evidence‐based care to prevent postsepsis morbidity and mortality for their non‐ICU patients need to heighten their index of suspicion when caring for an infected patient and appreciate that many severe sepsis patients may not fit neatly into traditional sepsis treatment algorithms.
Studies characterizing severe sepsis in the ICU setting indicate a predominance of pulmonary infections and respiratory failure with occurrence rates of 74% to 95% and 54% to 61%, respectively.[3, 12, 13] Given that either shock or pulmonary dysfunction is often required for admission to many ICUs, it is perhaps not surprising that these rates are dramatically different on the general medicine ward, with a relative scarcity of pulmonary infections (14%) and respiratory dysfunction (30%). Instead, genitourinary infections were noted in 41% (95% CI, 29%‐54%) of the cases, in contrast to the rates of genitourinary infections in ICU patients with severe sepsis, which have rates of 5.4% to 9.1%.[3, 10] Likely as a result of this, a Gram‐negative predominance is noted in the associated microbiology. Furthermore, our study indicates that C difficile and vancomycin‐resistant Enterococcus (VRE) species appear to represent an emerging cause of severe sepsis on the general medicine wards, as they have not been noted to be causative micro‐organisms in previous studies of sepsis. This is concordant with other studies showing increases in incidence and severity of disease for C difficile as well as VRE.[14, 15]
Previous epidemiologic studies of severe sepsis originating outside the ICU are lacking, but some work has been done. One study on the epidemiology of sepsis both with and without organ dysfunction aggregated all hospitalized patients and included those both admitted to the general medicine wards and directly to the ICU.[7] Similar to our study, this study also found a predominance of Gram‐negative causative organisms, as well as comparable in‐hospital mortality rates (12.8% vs 13%). Additionally, genitourinary infections were noted in 20% of the patients, notably higher than rates reported to have been found in patients with severe sepsis in the ICU, but not the magnitude found in our study, perhaps as a result of the combined ICU‐ward population studied. A similar high prevalence of genitourinary infections was also noted in a recent administrative data‐based study of emergency medical services‐transported patients with severe sepsis, half of whom required intensive care during their hospitalization.[16]
Our study is unique in that it focuses on severe sepsis in patients, commonly cared for by hospitalists, who were admitted to the general medical ward, and uses patient level data to elucidate more characteristics of the defining organ dysfunction. Furthermore, our results suggest that severe sepsis was poorly documented in this setting, indicating a potential impact on billing, coding, case mix index, and hospital mortality statistics that rely on very specific wording, as well as a possible need for increased awareness among hospitalists. Without this awareness, an opportunity may be missed for improved patient care via specific sepsis‐targeted measures,[13, 17, 18] including more aggressive resuscitative measures[19] or intensive physical and occupational therapy interventions aimed at impacting the cognitive and functional debilities[20] that result from severe sepsis. Highlighting this growing need to better assist clinicians assess the severity of septic patients and recognize these complex cases on the general medicine wards, 1 recent study evaluated the fitness of several clinical disease‐severity scoring systems for patients with sepsis in general internal medicine departments.[21] Perhaps with the help of tools such as these, which are being piloted in some hospitals, the care of this growing population can be enhanced.
Our study has a number of limitations that should be kept in mind. First, this is a single center study performed at an academic tertiary care center with a relatively high incidence of immunosuppression, which may influence the spectrum of infecting organisms. Our center also has a relatively large, closed‐model ICU, which often operates at near capacity, potentially affecting the severity of our non‐ICU population. Second, although we screened a large number of patients, as necessitated by our intensive and detailed review of clinical information, our sample size with hospitalist‐validated severe sepsis is relatively small. With this small sample size, less prevalent infections, patient characteristics, and organ dysfunctions may by chance have been under or over‐represented, and one could expect some variance in the occurrence rates of organ system dysfunction and infection rates by sampling error alone. Further larger scale studies are warranted to confirm these data and their generalizability. Third, the data necessary to calculate sequential organ failure assessment or multiple organ dysfunction score were not collected. This may limit the ability to directly compare the organ dysfunction noted in this study with others. Additionally, given the ICC definitions of organ dysfunction, some of the organ dysfunction noted, particularly for neurological dysfunction, was reliant on subjective clinical findings documented in the record. Finally, we relied on the lack of specific terminology to indicate a lack of documentation of sepsis, which does not necessarily indicate a lack of recognition or undertreatment of this condition. However, these limitations are offset by the strengths of this study, including the patient‐level medical record validation of severe sepsis by trained hospitalist physicians, high kappa statistic, and strict application of guideline‐based definitions.
This work has important implications for both clinicians and for future research on severe sepsis. The results suggest that severe sepsis may be quite common outside the ICU, and that patients presenting with this condition who are admitted to general medical wards are not routinely characterized by the profound hypoxemia and refractory shock of iconic cases. Certainly, further study looking at larger numbers of cases is needed to better understand the specifics and nuances of this important topic as well as to further evaluate clinicians' ability to recognize and treat such patients in this setting. Furthermore, future research on the treatment of severe sepsis, including both antimicrobials and disease‐modifying agents (eg, anti‐inflammatories) must continue to include and even focus on this large population of non‐ICU patients with severe sepsis, as the risk/benefit ratios of such potential treatments may vary with severity of illness.
In conclusion, severe sepsis was commonly found in patients admitted on the general medicine wards. The epidemiology of the infections and resultant organ dysfunction appears to differ from that found in the ICU. More studies are needed to provide a deeper understanding of this disease process, as this will enable clinicians to better recognize and treat patients thus afflicted, no matter the setting.
Acknowledgments
The authors thank Laetitia Shapiro, AM, for her programming assistance.
Disclosures: This work was supported in part by the US National Institutes of HealthK08, HL091249 (TJI) and the University of Michigan SpecialistHospitalist Allied Research Program (SHARP). This work was also supported in part by VA Ann Arbor Healthcare System, Geriatric Research Education and Clinical Center (GRECC).
The International Consensus Conference (ICC) for sepsis defines severe sepsis as an infection leading to acute organ dysfunction.[1, 2] Severe sepsis afflicts over 1 million patients each year in Medicare alone, and is substantially more common among older Americans than acute myocardial infarction.[3, 4, 5] Recently, the Agency for Healthcare Research and Quality identified severe sepsis as the single most expensive cause of hospitalization in the United States.[6] The incidence of severe sepsis continues to rise.[4, 5]
Severe sepsis is often mischaracterized as a diagnosis cared for primarily in the intensive care unit (ICU). Yet, studies indicate that only 32% to 50% of patients with severe sepsis require ICU care, leaving the majority on the general care wards.[7, 8] These studies also reveal mortality rates of 26% to 30% among patients with severe sepsis who are not admitted to an ICU compared to 11% to 33% in the ICU.[7, 8]
Although a number of epidemiologic and interventional studies have focused on severe sepsis in the ICU,[3, 9, 10] much less is known about patients cared for on the general medicine wards. Without this information, clinicians cannot make informed choices about important management decisions such as targeted diagnostic testing, empirical antimicrobials, and other therapies. To this end, we sought to further characterize the infectious etiologies and resultant organ system dysfunctions in the subset of patients with severe sepsis admitted to non‐ICU medical services at a tertiary academic medical center.
METHODS
Population/Setting
All hospitalizations of adult patients (18 years old) who were initially admitted to non‐ICU medical services at the University of Michigan Hospital during 2009 through 2010 were included. The University of Michigan Hospital has 610 general medical‐surgical beds, including telemetry beds, with closed ICUs comprised of 179 beds staffed by intensivists. Patients transferred from other hospitals and those admitted to non‐medical services were excluded.
Data Abstraction and Definitions
All International Classification of Diseases, 9th Revision, Clinical Modification (ICD‐9‐CM) diagnosis codes for hospitalizations were screened using a previously published and validated algorithm for severe sepsis.[11] Following this screening, 3 randomly selected round‐numbered batches of hospitalizations were sampled with subsequent application of the exclusion criteria. Medical records including physicians' notes, consultants' notes, nurses' notes, physical therapy notes, discharge coordinators' notes, emergency room flow sheets, as well as ward flow sheets were reviewed in detail by 3 practicing hospitalists using a structured instrument closely aligned with the ICC definition of severe sepsis.[2] We also sampled a smaller number of patients whose ICD‐9‐CM diagnoses screened negative for severe sepsis. Sample size was selected as part of a project with multiple objectives, and reflected a pragmatic balance between the anticipated precision of the results and the resources available to conduct chart review.[11] All discrepancies were reconciled among the 3 reviewers.
Reviewers first assessed whether infection was present, then evaluated for evidence of each organ system dysfunction, and finally determined the extent to which those organ dysfunctions were a response to the infection. Infection was defined either as a patient with a microbiologic culture growing a pathologic organism in a normally sterile site or documentation of a suspected infection with other confirmatory evidence (radiological, physical exam finding) with resultant systemic inflammatory response and administration of antimicrobials. Community‐acquired and healthcare‐associated infections were not differentiated. Microbiologic data, confirmatory tests, and site of infection were abstracted in detail.
Organ dysfunction was defined as per the 2001 ICC criteria,[2] and was assessed for neurological, pulmonary, cardiovascular, renal, gastrointestinal, hematological, and hepatic system involvement in all patients. A summary of these clinical definitions is included in Table 1. Data on important comorbidities were also abstracted. Immunosuppression was defined as having any of the following: solid organ transplant, bone marrow/stem cell transplant, human immunodeficiency virus/acquired immunodeficiency syndrome, neutropenia (absolute neutrophil count <1000), hematologic malignancy, solid organ malignancy with chemotherapy within the past 12 months, or pharmacologic immunosuppression (prednisone >20 mg daily for >4 weeks, calcineurin inhibitor, methotrexate, tumor necrosis factor inhibitors, azathioprine, sulfasalazine, hydroxychloroquine). Last, each chart was evaluated for the presence of explicit documentation with the presence of the words or phrases: sepsis, septic shock, or severe sepsis, indicating that the clinical service recognized and fully documented that a patient had severe sepsis.
Organ System | Parameters to Indicate Dysfunction |
---|---|
| |
Cardiovascular | Systolic BP <90, elevated lactate, MAP <70, requiring pressors >2 hours, decrease in systolic BP of >40 |
Renal | Creatinine increase >0.5 mg/dL, oliguria |
Neurological | Acute mental status changes |
Pulmonary | Intubation, BiPAP, supplemental oxygen >6 LPM or 40% face mask, PaO2/FiO2 <300 |
Hematologic | INR >1.5 or PTT >60 not on anticoagulation, platelets <100 or 50% of baseline |
Ileus | Decreased bowel motility requiring a change in diet |
Hepatic | Bilirubin >4 mg/dL and >1.5 baseline |
Data Analysis
Methods for assessment of reviewer concordance have been previously described and were summarized using the kappa statistic.[11] Initial data extraction was performed in SAS 9.1 (SAS Institute, Cary, NC) and all analyses were conducted in Stata 12 (StataCorp LP, College Station, TX). Binomial 95% confidence intervals (CIs) are presented. This project was approved by the University of Michigan Institutional Review Board.
RESULTS
Of 23,288 hospitalizations examined from 2009 through 2010, the ICD‐9based automated screen for severe sepsis was positive for 3,146 (14 %). A random sample of 111 medical records, of which 92 had screened positive for severe sepsis and 19 had screened negative, was reviewed in detail. After review by the hospitalists, 64 of these 111 hospitalizations were judged to have severe sepsis, 61 of the 92 screened positive cases (66%), and 3 of the 19 screened negative cases (16%). The 3 reviewers had a kappa of 0.70, indicating good agreement.
Characteristics of the 64 patients with severe sepsis are shown in Table 2. The mean age was 63 years old (standard deviation [SD]=17.7), and 41% were male. The mean length of stay was 13.7 days (SD=20.8). Thirty‐nine percent (95% CI, 27%‐52%) of patients (25/64) were immunosuppressed. Of patients initially admitted to the general medical ward, 25% (16/64; 95% CI, 15%‐37%) ultimately required ICU care during their admission. The overall in‐hospital mortality rate was 13% (8/64; 95% CI, 6%‐23%). Immunosuppressed patients had a mortality rate of 20% and nonimmunosuppressed patients had a mortality rate of 8%. Only 47% (30/64; 95% CI, 34%‐60%) of the medical records had explicit clinician documentation of severe sepsis.
Age, mean (SD), y | 63 (18) |
---|---|
| |
Male sex, no. (%) | 26 (41) |
Preexisting conditions, no. (%) | |
History of diabetes | 20 (31) |
End stage renal disease on chronic dialysis | 2 (3) |
Chronic obstructive pulmonary disease on oxygen | 3 (5) |
History of cancer | 15 (23) |
Liver cirrhosis | 5 (8) |
Immunosuppression | 25 (39) |
Median length of stay (days) | 7.5 |
Mean length of stay (SD) | 13.7 (20.8) |
The most common site of infection was found to be the genitourinary system, occurring in 41% (26/64; 95% CI, 29%‐54%) of patients (Table 3). Pulmonary and intra‐abdominal sites were also common, accounting for 14% (95% CI, 6.6%‐25%) and 13% (95% CI, 5.6%‐23%) of sites, respectively. An infecting organism was identified by culture in 66% (42/64; 95% CI, 53%‐77%) of case patients with specific pathogens listed in Table 4. Among patients with positive culture results, the majority grew Gram‐negative organisms (57%; 95% CI, 41%‐72%). Non‐Clostridium difficile Gram‐positive organisms were also prominent and identified in 48% (95% CI, 32%‐64%) of positive cultures. Candida was less common (12%, 95% CI, 4.0%‐26%). Fourteen cases (22%, 95% CI, 10%‐30%) had 2 or more concomitant infectious pathogens.
Site | No. (%) |
---|---|
| |
Genitourinary | 26 (41) |
Pulmonary | 9 (14) |
Intra‐abdominal (not intraluminal) | 8 (13) |
Bloodstream/cardiac | 5 (8) |
Skin and soft tissue | 4 (6) |
GI lumen | 4 (6) |
Joint | 2 (3) |
Multiple sites | 4 (6) |
Unknown | 2 (2) |
Absolute Frequency, Total Positive Culture Results, N=64, No. (%)*?>a | Patients With Cultures Growing at Least One of the Pathogens, N=42, No. (%)*?>a | |
---|---|---|
| ||
Gram‐negative pathogens | 30 (47) | 24 (57) |
Escherichia coli | 12 (19) | 12 (29) |
Escherichia coli (multidrug resistant) | 2 (3) | 2 (5) |
Klebsiella | 6 (9) | 5 (12) |
Pseudomonas aeruginosa | 6 (9) | 4 (10) |
Pseudomonas aeruginosa (multidrug resistant) | 2 (3) | 2 (5) |
Otherb | 6 (9) | 6 (14) |
Gram‐positive pathogens | 29 (45) | 25 (59) |
Enterococcus | 14 (22) | 13 (31) |
Vancomycin‐resistant Enterococcus species | 5 (8) | 4 (10) |
Staphylococcus aureus | 7 (11) | 7 (17) |
Methicillin‐resistant Staphylococcus aureus | 3 (5) | 3 (7) |
Streptococcus pneumoniae | 2 (3) | 2 (5) |
Coagulase‐negative staphylococci | 1 (2) | 1 (2) |
Clostridium difficile | 5 (8) | 5 (12) |
Fungi | ||
Candida species | 5 (8) | 5 (12) |
Mycobacterium avium | 1 (2) | 1 (2) |
Two organisms | 9 (21) | |
Three or more organisms | 5 (12) |
All 64 patients had at least 1 organ dysfunction, as required by the ICC definition of severe sepsis. Organ dysfunction in 2 or more organ systems occurred in 77% (95% CI, 64%‐86%) of the cases (49/64). The incidence for each organ system dysfunction is presented in Table 5, as well as its relationship to both mortality and ICU admission. The most common organ system dysfunctions were found to be cardiovascular (hypotension) and renal dysfunction occurring in 66% and 64% of the cases, respectively. In this non‐ICU population, pulmonary dysfunction occurred in 30% of cases, but was frequently associated with transfer to the ICU, as 63% of the patients with pulmonary failure required ICU care. Patients with more organ systems affected were more likely to be transferred to the ICU and to die.
No. (%) | ICU Transfer, No. (%) | Mortality, No. (%) | |
---|---|---|---|
| |||
Number of failed organs, N = 64 | |||
1 | 15 (23%) | 0 (0%) | 0 (0%) |
2 | 25 (39%) | 2 (8%) | 0 (0%) |
3 | 7 (11%) | 2 (29%) | 1 (14%) |
4 | 10 (16%) | 6 (60%) | 3 (30%) |
>4 | 7 (11%) | 6 (86%) | 4 (57%) |
Types of organ system dysfunction, all patients, N = 64*?>a | |||
Cardiovascular | 42 (66%) | 16 (38%)b | 8 (19%)c |
Renal | 41 (64%) | 10 (24%)b | 5 (12%)c |
Central nervous system | 35 (54%) | 14 (40%)b | 7 (18%)c |
Pulmonary | 19 (30%) | 12 (63%)b | 8 (42%)c |
Hematologic | 15 (23%) | 6 (40%)b | 6 (40%)c |
GI (ileus) | 8 (13%) | 5 (63%)b | 1 (13%)c |
Hepatic | 5 (8%) | 4 (80%)b | 2 (40%)c |
DISCUSSION
Severe sepsis was common among patients admitted to the general medical ward in this tertiary care center. Our patient cohort differed in important ways from previously described typical cases of severe sepsis among ICU populations. Severe sepsis on the general medical wards was more commonly associated with Gram‐negative pathogens in the setting of genitourinary tract infections. This is in contrast to Gram‐positive organisms and respiratory tract infections, which are more common in the ICU.[3, 10] Renal and cardiac dysfunction were commonly observed organ failures, whereas in the ICU, severe sepsis has been reported to more likely involve respiratory failure. These results suggest that hospitalists seeking to provide evidence‐based care to prevent postsepsis morbidity and mortality for their non‐ICU patients need to heighten their index of suspicion when caring for an infected patient and appreciate that many severe sepsis patients may not fit neatly into traditional sepsis treatment algorithms.
Studies characterizing severe sepsis in the ICU setting indicate a predominance of pulmonary infections and respiratory failure with occurrence rates of 74% to 95% and 54% to 61%, respectively.[3, 12, 13] Given that either shock or pulmonary dysfunction is often required for admission to many ICUs, it is perhaps not surprising that these rates are dramatically different on the general medicine ward, with a relative scarcity of pulmonary infections (14%) and respiratory dysfunction (30%). Instead, genitourinary infections were noted in 41% (95% CI, 29%‐54%) of the cases, in contrast to the rates of genitourinary infections in ICU patients with severe sepsis, which have rates of 5.4% to 9.1%.[3, 10] Likely as a result of this, a Gram‐negative predominance is noted in the associated microbiology. Furthermore, our study indicates that C difficile and vancomycin‐resistant Enterococcus (VRE) species appear to represent an emerging cause of severe sepsis on the general medicine wards, as they have not been noted to be causative micro‐organisms in previous studies of sepsis. This is concordant with other studies showing increases in incidence and severity of disease for C difficile as well as VRE.[14, 15]
Previous epidemiologic studies of severe sepsis originating outside the ICU are lacking, but some work has been done. One study on the epidemiology of sepsis both with and without organ dysfunction aggregated all hospitalized patients and included those both admitted to the general medicine wards and directly to the ICU.[7] Similar to our study, this study also found a predominance of Gram‐negative causative organisms, as well as comparable in‐hospital mortality rates (12.8% vs 13%). Additionally, genitourinary infections were noted in 20% of the patients, notably higher than rates reported to have been found in patients with severe sepsis in the ICU, but not the magnitude found in our study, perhaps as a result of the combined ICU‐ward population studied. A similar high prevalence of genitourinary infections was also noted in a recent administrative data‐based study of emergency medical services‐transported patients with severe sepsis, half of whom required intensive care during their hospitalization.[16]
Our study is unique in that it focuses on severe sepsis in patients, commonly cared for by hospitalists, who were admitted to the general medical ward, and uses patient level data to elucidate more characteristics of the defining organ dysfunction. Furthermore, our results suggest that severe sepsis was poorly documented in this setting, indicating a potential impact on billing, coding, case mix index, and hospital mortality statistics that rely on very specific wording, as well as a possible need for increased awareness among hospitalists. Without this awareness, an opportunity may be missed for improved patient care via specific sepsis‐targeted measures,[13, 17, 18] including more aggressive resuscitative measures[19] or intensive physical and occupational therapy interventions aimed at impacting the cognitive and functional debilities[20] that result from severe sepsis. Highlighting this growing need to better assist clinicians assess the severity of septic patients and recognize these complex cases on the general medicine wards, 1 recent study evaluated the fitness of several clinical disease‐severity scoring systems for patients with sepsis in general internal medicine departments.[21] Perhaps with the help of tools such as these, which are being piloted in some hospitals, the care of this growing population can be enhanced.
Our study has a number of limitations that should be kept in mind. First, this is a single center study performed at an academic tertiary care center with a relatively high incidence of immunosuppression, which may influence the spectrum of infecting organisms. Our center also has a relatively large, closed‐model ICU, which often operates at near capacity, potentially affecting the severity of our non‐ICU population. Second, although we screened a large number of patients, as necessitated by our intensive and detailed review of clinical information, our sample size with hospitalist‐validated severe sepsis is relatively small. With this small sample size, less prevalent infections, patient characteristics, and organ dysfunctions may by chance have been under or over‐represented, and one could expect some variance in the occurrence rates of organ system dysfunction and infection rates by sampling error alone. Further larger scale studies are warranted to confirm these data and their generalizability. Third, the data necessary to calculate sequential organ failure assessment or multiple organ dysfunction score were not collected. This may limit the ability to directly compare the organ dysfunction noted in this study with others. Additionally, given the ICC definitions of organ dysfunction, some of the organ dysfunction noted, particularly for neurological dysfunction, was reliant on subjective clinical findings documented in the record. Finally, we relied on the lack of specific terminology to indicate a lack of documentation of sepsis, which does not necessarily indicate a lack of recognition or undertreatment of this condition. However, these limitations are offset by the strengths of this study, including the patient‐level medical record validation of severe sepsis by trained hospitalist physicians, high kappa statistic, and strict application of guideline‐based definitions.
This work has important implications for both clinicians and for future research on severe sepsis. The results suggest that severe sepsis may be quite common outside the ICU, and that patients presenting with this condition who are admitted to general medical wards are not routinely characterized by the profound hypoxemia and refractory shock of iconic cases. Certainly, further study looking at larger numbers of cases is needed to better understand the specifics and nuances of this important topic as well as to further evaluate clinicians' ability to recognize and treat such patients in this setting. Furthermore, future research on the treatment of severe sepsis, including both antimicrobials and disease‐modifying agents (eg, anti‐inflammatories) must continue to include and even focus on this large population of non‐ICU patients with severe sepsis, as the risk/benefit ratios of such potential treatments may vary with severity of illness.
In conclusion, severe sepsis was commonly found in patients admitted on the general medicine wards. The epidemiology of the infections and resultant organ dysfunction appears to differ from that found in the ICU. More studies are needed to provide a deeper understanding of this disease process, as this will enable clinicians to better recognize and treat patients thus afflicted, no matter the setting.
Acknowledgments
The authors thank Laetitia Shapiro, AM, for her programming assistance.
Disclosures: This work was supported in part by the US National Institutes of HealthK08, HL091249 (TJI) and the University of Michigan SpecialistHospitalist Allied Research Program (SHARP). This work was also supported in part by VA Ann Arbor Healthcare System, Geriatric Research Education and Clinical Center (GRECC).
- Definitions for sepsis and organ failure and guidelines for the use of innovative therapies in sepsis. The ACCP/SCCM Consensus Conference Committee. American College of Chest Physicians/Society of Critical Care Medicine. Chest. 1992;101(6):1644–1655. , , , et al.
- 2001 SCCM/ESICM/ACCP/ATS/SIS International Sepsis Definitions Conference. Crit Care Med. 2003;31(4):1250–1256. , , , et al.
- Epidemiology of severe sepsis in the United States: analysis of incidence, outcome, and associated costs of care. Crit Care Med. 2001;29(7):1303–1310. , , , , , .
- Population burden of long‐term survivorship after severe sepsis in older americans. J Am Geriatr Soc. 2012;60(6):1070–1077. , , , .
- The epidemiology of sepsis in the United States from 1979 through 2000. N Engl J Med. 2003;348(16):1546–1554. , , , .
- Septicemia in U.S. hospitals, 2009: statistical brief #122. October 2011. In: Healthcare Cost and Utilization Project Statistical Briefs. Rockville, MD: Agency for Health Care Policy and Research; 2006. Available from: http://www.ncbi.nlm.nih.gov/books/NBK65391. Accessed June 2, 2012. , , .
- Sepsis incidence and outcome: contrasting the intensive care unit with the hospital ward. Crit Care Med. 2007;35(5):1284–1289. , , , et al.
- Epidemiology of sepsis in Victoria, Australia. Crit Care Med. 2005;33(1):71–80. , , , , .
- Effect of empirical treatment with moxifloxacin and meropenem vs meropenem on sepsis‐related organ dysfunction in patients with severe sepsis: a randomized trial. JAMA. 2012;307(22):2390–2399. , , , et al.
- Incidence and impact of organ dysfunctions associated with sepsis. Chest. 2005;127(3):942–951. , , , , .
- Identifying patients with severe sepsis using administrative claims: patient‐level validation of the Angus Implementation of the International Consensus Conference definition of severe sepsis [published online ahead of print September 18, 2012]. Medical Care. doi: 10.1097/MLR.0b013e318268ac86. , , , et al.
- Current epidemiology of septic shock: the CUB‐Rea Network. Am J Respir Crit Care Med. 2003;168(2):165–172. , , , .
- Management of sepsis. N Engl J Med. 2006;355(16):1699–1713. .
- Current status of Clostridium difficile infection ipidemiology. Clin Infect Dis. 2012;55(suppl 2):S65–S70. , , .
- Vancomycin‐resistant enterococci. Semin Respir Infect. 2000;15(4):314–326. , .
- Severe sepsis in prehospital emergency care: analysis of incidence, care, and outcome. Am J Respir Crit Care Med. 2012;186(12):1264–1271. , , , , , .
- Novel Therapies for Septic Shock Over the Past 4 Decades. JAMA. 2011;306(2):194–199. , .
- Impact of the Surviving Sepsis Campaign protocols on hospital length of stay and mortality in septic shock patients: results of a three‐year follow‐up quasi‐experimental study. Crit Care Med. 2010;38(4):1036–1043. , , , et al.
- Diagnosis and treatment of severe sepsis. Crit Care. 2007;11(suppl 5):S2. , .
- Long‐term cognitive impairment and functional disability among survivors of severe sepsis. JAMA. 2010;304(16):1787–1794. , , , .
- Assessment of disease‐severity scoring systems for patients with sepsis in general internal medicine departments. Crit Care. 2011;15:R95. , , , , .
- Definitions for sepsis and organ failure and guidelines for the use of innovative therapies in sepsis. The ACCP/SCCM Consensus Conference Committee. American College of Chest Physicians/Society of Critical Care Medicine. Chest. 1992;101(6):1644–1655. , , , et al.
- 2001 SCCM/ESICM/ACCP/ATS/SIS International Sepsis Definitions Conference. Crit Care Med. 2003;31(4):1250–1256. , , , et al.
- Epidemiology of severe sepsis in the United States: analysis of incidence, outcome, and associated costs of care. Crit Care Med. 2001;29(7):1303–1310. , , , , , .
- Population burden of long‐term survivorship after severe sepsis in older americans. J Am Geriatr Soc. 2012;60(6):1070–1077. , , , .
- The epidemiology of sepsis in the United States from 1979 through 2000. N Engl J Med. 2003;348(16):1546–1554. , , , .
- Septicemia in U.S. hospitals, 2009: statistical brief #122. October 2011. In: Healthcare Cost and Utilization Project Statistical Briefs. Rockville, MD: Agency for Health Care Policy and Research; 2006. Available from: http://www.ncbi.nlm.nih.gov/books/NBK65391. Accessed June 2, 2012. , , .
- Sepsis incidence and outcome: contrasting the intensive care unit with the hospital ward. Crit Care Med. 2007;35(5):1284–1289. , , , et al.
- Epidemiology of sepsis in Victoria, Australia. Crit Care Med. 2005;33(1):71–80. , , , , .
- Effect of empirical treatment with moxifloxacin and meropenem vs meropenem on sepsis‐related organ dysfunction in patients with severe sepsis: a randomized trial. JAMA. 2012;307(22):2390–2399. , , , et al.
- Incidence and impact of organ dysfunctions associated with sepsis. Chest. 2005;127(3):942–951. , , , , .
- Identifying patients with severe sepsis using administrative claims: patient‐level validation of the Angus Implementation of the International Consensus Conference definition of severe sepsis [published online ahead of print September 18, 2012]. Medical Care. doi: 10.1097/MLR.0b013e318268ac86. , , , et al.
- Current epidemiology of septic shock: the CUB‐Rea Network. Am J Respir Crit Care Med. 2003;168(2):165–172. , , , .
- Management of sepsis. N Engl J Med. 2006;355(16):1699–1713. .
- Current status of Clostridium difficile infection ipidemiology. Clin Infect Dis. 2012;55(suppl 2):S65–S70. , , .
- Vancomycin‐resistant enterococci. Semin Respir Infect. 2000;15(4):314–326. , .
- Severe sepsis in prehospital emergency care: analysis of incidence, care, and outcome. Am J Respir Crit Care Med. 2012;186(12):1264–1271. , , , , , .
- Novel Therapies for Septic Shock Over the Past 4 Decades. JAMA. 2011;306(2):194–199. , .
- Impact of the Surviving Sepsis Campaign protocols on hospital length of stay and mortality in septic shock patients: results of a three‐year follow‐up quasi‐experimental study. Crit Care Med. 2010;38(4):1036–1043. , , , et al.
- Diagnosis and treatment of severe sepsis. Crit Care. 2007;11(suppl 5):S2. , .
- Long‐term cognitive impairment and functional disability among survivors of severe sepsis. JAMA. 2010;304(16):1787–1794. , , , .
- Assessment of disease‐severity scoring systems for patients with sepsis in general internal medicine departments. Crit Care. 2011;15:R95. , , , , .
Copyright © 2013 Society of Hospital Medicine
Hospitalist Care and Patient Satisfaction
Payers and policymakers are increasingly holding hospitals accountable for patients' experiences with their care. Since 2006, the Centers for Medicare and Medicaid Services (CMS) have collected data on patients' experiences with inpatient care using the Hospital Consumer Assessment of Healthcare Providers and Systems (HCAHPS) survey, a well‐validated and widely used tool. In 2008, these data on patient experience began to be publicly reported, and CMS now plans to base part of its payments to hospitals on HCAHPS performance scores. In this context, hospitals are looking for ways to improve patient satisfaction.
The effort to hold hospitals accountable for patient experience may conflict with another major trend in US hospitals: the increasing use of hospitalists.[1] Although hospitalists may have greater expertise in the day‐to‐day care of the hospitalized patient, they generally do not know the patient and cannot cater to patients' preferences in ways that the primary‐care provider might. Therefore, given that patients may prefer to be seen by their primary‐care provider,[2] greater use of hospitalists may actually lead to a decrease in patient satisfaction. Unfortunately, we are unaware of any national examination of the relationship between hospitalist use in an institution and that entity's performance on patient‐experience scores.
To better understand the relationship between greater hospitalist staffing and patient‐centered care, we examined the association between hospitalist staffing and patient satisfaction with both overall care and specific domains of patient‐centered care. We hypothesized that hospitals that used a high proportion of hospitalists would generally have lower patient‐experience scores. Further, we expected that the relationship would be monotonic (greater use of hospitalists associated with lower scores) and particularly pronounced in 2 domains: patient experience with discharge planning and patient experience with physician communication.
METHODS
Data
We sought to identify acute‐care hospitals with elderly medical patients cared for by hospitalists, non‐hospitalists, or some combination of the 2. To construct this cohort, we used 3 2009 Medicare files. The Beneficiary Summary File contains demographic information on Medicare beneficiaries and data on enrollment in managed‐care plans. To identify medical hospitalizations, we used the Medicare Provider Analysis and Review (MedPAR) 100% Files, which contain the clinical diagnoses and payments for all fee‐for‐service Medicare beneficiaries discharged from acute‐care hospitals. To identify hospitalists and non‐hospitalists, we used the 5% Carrier File, which contains physician billing data for a 5% random sample of fee‐for‐service Medicare beneficiaries. We also obtained information on hospital characteristics from the American Hospital Association (AHA) Annual Survey. We supplemented this with hospital‐level data on patient satisfaction from the HCAHPS survey conducted in 2009. The HCAHPS is a standard survey developed by the Agency for Healthcare Research and Quality (AHRQ) and administered by hospitals to a random sample of adult patients 48 hours to 6 weeks after discharge. The HCAHPS results are adjusted for patient mix and have been tested for nonresponse bias.[3] Details about the development and design of HCAHPS have been described previously.[4]
Patient and Hospital Sample
We started with 48,861,000 Medicare beneficiaries in the Beneficiary Summary File and excluded 38% either because their age was <65 years or they were members of an HMO. At the same time, from the 1,850,000 patients in the 5% Carrier File, we excluded 55% who had not been cared for by a general internist. Finally, we used the MedPAR File to identify 17,387,000 hospital admissions by fee‐for‐service Medicare beneficiaries. From MedPAR, we excluded admissions to a facility other than an acute‐care hospital (24%), surgical admissions identified by diagnosis‐related group (DRG) (29%), and admissions to hospitals with <5 medicine admissions in 2009 (<0.1%). After merging these 3 files (Beneficiary Summary, MedPAR, and 5% Carrier), we were left with 229,496 admissions among 180,399 patients at 3365 hospitals. We subsequently excluded readmissions and were left with 156,333 admissions at 3244 hospitals. Finally, we excluded those patients cared for by both hospitalists and non‐hospitalists during the same hospitalization, and those hospitals missing AHA or HCAHPS data, leaving us with 132,814 patients at 2843 hospitals.
Definition of Hospitalist
We used the claims‐based definition developed and validated by Kuo and Goodwin in earlier work.[1] Hospitalists are defined as those general internists (providers in general practice or internal medicine) who had 5 evaluation and management (E&M) billings (in a 5% sample of Medicare beneficiaries) in 2009 and generated >90% of their claims from the care of hospitalized patients in 2009.
Measures of Patient Satisfaction
There are 2 HCAHPS questions about overall satisfaction, one that asks patients to rate their experience on a scale of 0 to 10 and another that asks whether they would recommend the hospital. Not surprisingly, hospitals' performance on these 2 questions is highly correlated.[5] We measured overall patient experience using commonly used approaches: the proportion of patients who gave the hospital a 9 or 10 (on the 10‐point scale) or the proportion of patients who reported that they would definitely recommend the hospital. The HCAHPS also contains 24 questions, which are reported by CMS in 8 domains: communication with nurse, communication with physician, responsiveness of the staff, pain control, communication about medications, adequacy of discharge planning, cleanliness of the room, and quietness of the room. The patient‐satisfaction score for each of these domains represents the proportion of patients who answered always to each of the questions, or who answered yes to the question about discharge.
Potentially Confounding Variables
Because we were worried that hospitals with hospitalists would be different from hospitals without hospitalists, we identified a series of covariates for adjustment in a multivariable model. We extracted data from the AHA on hospitals' structural characteristics that we assumed might be associated both with having a hospitalist and with patient experience. These variables were size (number of beds), teaching status (membership in the Council of Teaching Hospitals vs no membership), location (urban vs rural), region (the 4 census regions), ownership (for profit, private nonprofit, or public), and presence of advanced clinical capabilities (as measured by having a medical, surgical, and/or cardiac intensive care unit [ICU]). We also used information about the patient population (proportion of patients with Medicare or with Medicaid) as well as nurse‐staffing level (ratio of full‐time equivalent registered nurses to total hospital beds).
Statistical Analyses
We first quantified hospital variation in the proportion of general‐medicine patients cared for by hospitalists, using basic descriptive statistics. Based on these analyses, we categorized hospitals into 3 groups: non‐hospitalist, mixed, and hospitalist (corresponding to lowest, middle, and highest tertile of hospitalist use respectively). We used bivariate techniques to describe the patient and hospital characteristics of hospitals in each group. Patient characteristics included number of comorbidities, which were calculated using software from the Healthcare Cost and Utilization Project (HCUP),[6] based on methods developed by Elixhauser et al.[7] We used the ‐square test to assess whether hospital or patient characteristics differed between hospitalist, mixed, and non‐hospitalist hospitals.
To examine the association between hospitalist care and patient satisfaction, we first constructed bivariate models for each measure of patient satisfaction. In these models, hospital type (hospitalist, mixed, and non‐hospitalist) was our predictor. We next constructed multivariable models, which adjusted for each of the hospital characteristics described above in order to assess the independent relationship between hospitalist care and HCAHPS performance.
In sensitivity analyses, we first examined hospitalist use as a continuous variable and had qualitatively very similar results. Those data are not presented. Additionally, we conducted a propensity score analysis, with results presented in the Appendix (see Supporting Information, Appendix 1, in the online version of this article). In our first‐stage logistic regression model, being a hospitalist hospital (defined as being in the top tertile of hospitalist use vs bottom 2 tertiles) was the outcome. Hospital structural factors were covariates. Based on this first‐stage model, each hospital was assigned a propensity of being a hospitalist hospital. We divided the hospitals into 3 groups (highest propensity tertile, middle propensity tertile, and lowest propensity tertile). In a second‐stage linear regression model, patient satisfaction score was the outcome. The predictors were hospital type (dichotomized, and defined as being in the top tertile of hospitalist use vs bottom 2 tertiles), and propensity of being a hospitalist hospital (3 categories, with low propensity as the reference).
All analyses were performed using SAS version 9.2. The project was reviewed by the Institutional Review Board at the University of Michigan and determined to be not regulated given our use of publicly available datasets.
RESULTS
Among all hospitals, the median proportion of general‐medicine admissions cared for by hospitalists was 41.2% (interquartile range [IQR], 11.5%67.4%). However, US hospitals varied widely in the proportion of general‐medicine patients cared for by hospitalists (Figure 1). Whereas 3.5% of hospitals had all of their general‐medicine patients cared for by hospitalists, 16.6% had none of their general‐medicine patients seen by hospitalists. For hospitals with at least some hospitalist care, the proportion of patients cared for by hospitalists was distributed fairly evenly across the range of possibilities (Figure 1).
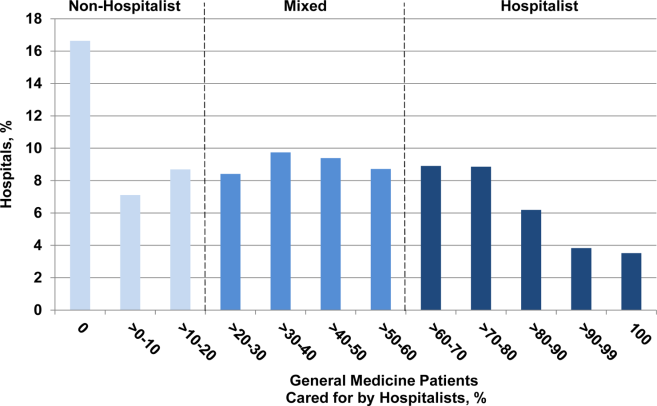
Because hospitalist care varied widely among hospitals, we categorized hospitals into 3 groups (non‐hospitalist, mixed, and hospitalist). The median proportion of patients cared for by hospitalists in the 3 groups was 0%, 39.5%, and 76.5%, respectively (Table 1). The non‐hospitalist hospitals, when compared with mixed and hospitalist hospitals, were more likely to be small, nonteaching, for‐profit institutions located in the Midwestern United States. They also were less likely to have an ICU and had lower nurse‐to‐bed ratios.
Hospital Characteristics | Hospital Type | P Value | ||
---|---|---|---|---|
Non‐Hospitalist (N = 943) | Mixed (N = 948) | Hospitalist (N = 952) | ||
| ||||
GM admissions cared for by hospitalists, median (range), % | 0 (021) | 40 (2158) | 77 (58100) | <0.001 |
Nurse‐to‐bed ratio | 1 | 1 | 2 | <0.001 |
Presence of MICU, % | 79 | 84 | 85 | 0.001 |
Medicaid patients, % | 19 | 18 | 18 | 0.06 |
Hospital beds, % | <0.001 | |||
Small (99) | 36 | 16 | 24 | |
Medium (100399) | 59 | 64 | 58 | |
Large (400) | 6 | 21 | 18 | |
COTH membership, % | <0.001 | |||
Yes | 3 | 13 | 11 | |
No | 97 | 87 | 89 | |
Urban, % | 0.10 | |||
Yes | 88 | 89 | 91 | |
No | 12 | 11 | 9 | |
Profit status, % | <0.001 | |||
For profit | 21 | 17 | 18 | |
Not for profit, private | 62 | 71 | 67 | |
Other | 18 | 12 | 15 | |
Region, % | <0.001 | |||
South | 41 | 42 | 42 | |
Northeast | 14 | 21 | 16 | |
Midwest | 30 | 22 | 18 | |
West | 15 | 15 | 24 |
The types of patients cared for at all 3 hospital types (non‐hospitalist, mixed, and hospitalist) were similar in age and day of admission (Table 2). Patients cared for at non‐hospitalist hospitals were slightly more likely to be female and non‐White, and less likely to be admitted from the emergency department or another hospital or healthcare facility.
Patient Characteristics | Hospital Type | P Value | ||
---|---|---|---|---|
Non‐Hospitalist (N = 33,265) | Mixed (N = 52,844) | Hospitalist (N = 46,705) | ||
| ||||
Age, y | 0.51 | |||
6574 | 27 | 27 | 27 | |
7584 | 39 | 39 | 39 | |
85 | 34 | 34 | 34 | |
Sex | <0.001 | |||
M | 35 | 35 | 36 | |
F | 65 | 65 | 64 | |
Race/ethnicity | <0.001 | |||
White | 85 | 85 | 87 | |
Black | 10 | 11 | 9 | |
Other | 5 | 4 | 4 | |
Unknown | 0 | 0 | 0 | |
Comorbidities, % | <0.001 | |||
0 | 8 | 8 | 7 | |
1 | 23 | 23 | 22 | |
2+ | 69 | 69 | 71 | |
Day of admission | 0.08 | |||
Weekday | 73 | 73 | 73 | |
Weekend | 27 | 27 | 27 | |
Admission source | <0.001 | |||
ED | 75 | 78 | 80 | |
Another ACH | 1 | 2 | 3 | |
Other healthcare facility | 4 | 4 | 4 | |
Other | 20 | 17 | 13 | |
ICU stay | <0.001 | |||
Yes | 13 | 12 | 12 | |
No | 87 | 88 | 88 | |
Length of stay, d | <0.001 | |||
Median (Q1, Q3) | 4 (3, 6) | 4 (2, 6) | 3 (2, 5) | |
DRG | <0.001 | |||
Septicemia or severe sepsis | 3 | 4 | 4 | |
Esophagitis, gastroenteritis | 3 | 3 | 3 | |
Kidney and urinary tract infections | 3 | 3 | 3 | |
Syncope | 3 | 3 | 3 | |
Pneumonia | 3 | 3 | 3 |
When we examined unadjusted relationships between type of hospital and patient experience, we found that patients at hospitalist vs non‐hospitalist hospitals were more likely to recommend the hospital (69.4% vs 65.1%: P < 0.001), and report higher overall satisfaction (65.9% vs 63.6%: P < 0.001) ((see Supporting Information, Appendix, Table A1, in the online version of this article)). Care at hospitalist hospitals was associated with higher satisfaction with discharge, but lower satisfaction with room cleanliness and communication with doctors. These differences were statistically significant at the P < 0.05 level.
When we examined the relationship between having more hospitalists and patient experience using multivariable models that accounted for differences in hospital characteristics, we found largely similar results: The proportion of patients who were satisfied with their overall care was still higher at hospitalist compared with non‐hospitalist hospitals (65.6% vs 63.9%: P < 0.001) (Figure 2). Similarly, patients were more likely to definitely recommend their hospital if they had been cared for at a hospitalist vs non‐hospitalist hospital (66.0% vs 63.4%: P < 0.001).
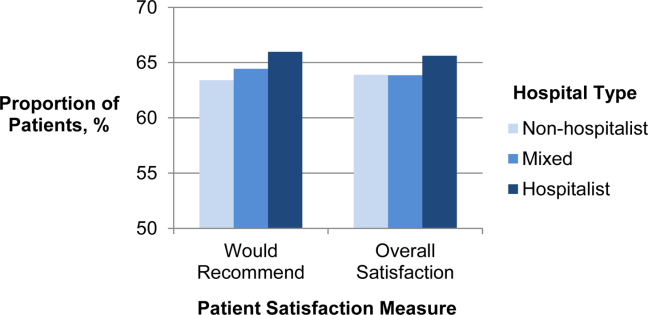
To better understand which domains of care might be contributing to greater overall satisfaction, we also examined patient satisfaction with specific domains of care at hospitalist vs non‐hospitalist hospitals (Table 3) in our adjusted analyses. Among 8 domains, the largest difference in satisfaction between patients cared for at hospitalist vs non‐hospitalist hospitals occurred with discharge. At hospitalist hospitals, 80.3% of patients said they were satisfied with the quality of the discharge planning compared with 78.1% at non‐hospitalist hospitals (P < 0.001). Patients at hospitalist hospitals were more satisfied with most other domains of care as well. Patients cared for at hospitalist hospitals were slightly less likely to be satisfied with communication with doctors, but this difference was not statistically significant (P = 0.45). Results were qualitatively similar in propensity‐score analyses (see Supporting Information, Appendix, Table A2, in the online version of this article).
Specific Domains of Care | Hospital Type, % Satisfied | Hospitalist vs Non‐Hospitalist | |||
---|---|---|---|---|---|
Non‐Hospitalist | Mixed | Hospitalist | Difference in % Satisfied | P Value | |
| |||||
Discharge | 78.1 | 79.1 | 80.3 | 2.1 | <0.001 |
Nursing services | 66.0 | 65.8 | 67.1 | 1.1 | <0.001 |
Quiet | 63.3 | 63.1 | 64.4 | 1.1 | 0.001 |
Communication, nurse | 76.7 | 76.7 | 77.7 | 1.0 | <0.001 |
Pain control | 69.7 | 69.7 | 70.4 | 0.7 | 0.001 |
Medications | 60.5 | 60.5 | 61.2 | 0.7 | 0.002 |
Cleanliness | 72.7 | 72.1 | 72.9 | 0.2 | 0.56 |
Communication, physician | 83.6 | 83.1 | 83.5 | 0.2 | 0.45 |
DISCUSSION
We found that in 2009, US hospitals varied widely in the proportion of general medicine patients cared for by hospitalists. Hospitals with higher levels of hospitalist care did better on most measures of patient satisfaction. Differences were largest in overall satisfaction and for discharge planning. In 5 other domains of care, differences were smaller, but hospitals with more hospitalist care consistently performed better than non‐hospitalist hospitals. Hospitalist care was not associated with patient satisfaction in 2 domains: communication with doctors and cleanliness of room.
Our findings of modestly higher patient satisfaction at hospitalist hospitals along most dimensions of care are surprising and reassuring. Indeed, when hospitalists first began caring for inpatients, some expressed concerns that hospitalist care would decrease patient satisfaction.[8, 9] Though this has been an ongoing concern, we found no evidence to support this contention. It may be that as a response to the concern, hospitals with hospitalists have paid particular attention to issues such as effective handoffs to primary‐care providers.[10, 11, 12, 13] Whether due to these efforts or other factors such as the 24/7 inpatient presence of hospitalists, we found that patients at hospitalist hospitals were more likely to be satisfied with their inpatient care, including their experience at discharge. In contrast, one area that may offer room for improvement for hospitalist hospitals is communication with physicians. It may be that patients cared for by hospitalists do not know their physicians as well as patients whose care is being orchestrated by their primary‐care provider, and thus the benefits of having an ever‐present hospitalist are diminished.
The magnitude of the associations that we found should also be placed in the context of existing research on patient satisfaction. Prior work has described baseline hospital performance, changes over time, and factors associated with greater inpatient satisfaction.[5, 14, 15] The associations that we found between hospitalist care and satisfaction with care at discharge were larger than those found for teaching (vs non‐teaching) hospitals.[5] However, compared with other hospital characteristics such as nurse staffing or profit status, hospitalist care was associated with smaller differences in patient satisfaction. In one study, hospitals in the highest quartile of nurse staffing had HCAHPS scores (ie, willingness to recommend measure) that were 6.7 points higher than those in the lowest quartile of nurse staffing, and similar differences existed between not‐for‐profit, public hospitals vs for‐profit hospitals.[5]
Taken together, our findings address an important gap in knowledge about hospitalist care. Prior research has documented growth in the use of hospitalist care[1] and described the association of hospitalist care with outcomes such as mortality and resource use, and receipt of recommended care.[16, 17, 18, 19] However, we are unaware of any national study that has examined the association of hospitalist care with patient satisfaction. One study surveyed patients in a single health system and found that patients were similarly satisfied with care provided by hospitalists and primary‐care physicians.[20] Our findings should be reassuring to clinical leaders and policymakers who have advocated greater use of hospitalists: the results suggest that there need be no tradeoff between greater use of hospitalist services and patient satisfaction. Indeed, patients appear to be even more satisfied in hospitals that have greater use of hospitalist physicians.
Our study has several limitations. First, it was a cross‐sectional study, and thus we cannot make any conclusions about causality. Although we adjusted for several potential confounders (eg, teaching status, advanced care capabilities, nurse staffing), it is possible that hospitalist care is a surrogate marker for features of hospitals that we could not measure but that directly influence patient experience. In addition, it is possible that patients cared for at hospitalist hospitals differ in unmeasured ways from patients cared for at other types of hospitals. Second, we constructed our primary predictor and outcome from different cohorts. Our primary predictor was derived from the proportion of general‐medicine patients cared for by hospitalists in Medicare claims data. In contrast, our primary outcome was based on HCAHPS responses from a random sampling of all hospital admissions. This misclassification likely would have biased us towards finding small or no associations. Therefore, we are likely underestimating the true association between hospitalist use and patient experience. Third, our findings may not be generalizable to hospitals that serve younger patients or have a large number of specialist hospitalists (who were not included in our definition of hospitalists). For example, compared with older patients with multiple comorbidities, relatively healthy younger patients may derive less benefit from an ever‐present hospitalist who can explain discharge plans or an attentive nurse.
In summary, we found that US hospitals varied widely in their use of hospitalist physicians, and those which a greater proportion of care was delivered by hospitalists generally had better scores on patient experience, especially on the global assessment of satisfaction and in discharge care. Our findings suggest that adoption of the hospitalist modelone of the strategies employed by US hospitals in the past 2 decades to provide efficient careshould not detract from achieving the goal of more patient‐centered care.
Disclosures
Dr. Chen's work is supported in part by the National Institutes of Health/National Institute on Aging (AG024824, University of Michigan Claude D. Pepper Older Americans Independence Center), and the National Institutes of Health/National Center for Research Resources (UL1‐RR024986, Michigan Institute for Clinical and Health Research). Dr. Chen is also supported by a Career Development Grant Award (K08HS020671) from the Agency for Healthcare Research and Quality.
- Growth in the care of older patients by hospitalists in the United States. N Engl J Med. 2009;360(11):1102–1112. , , , .
- How do patients view the role of the primary care physician in inpatient care? Dis Mon. 2002;48(4):230–238. , , .
- Effects of survey mode, patient mix, and nonresponse on CAHPS hospital survey scores. Health Serv Res. 2009;44(2 pt 1):501–518. , , , et al.
- Development, implementation, and public reporting of the HCAHPS survey. Med Care Res Rev. 2010;67(1):27–37. , , , , .
- Patients' perception of hospital care in the United States. N Engl J Med. 2008;359(18):1921–1931. , , , .
- Agency for Healthcare Research and Quality. Healthcare Cost and Utilization Project (HCUP). HCUP Comorbidity Software. http://www.hcup‐us.ahrq.gov/toolssoftware/comorbidity/comorbidity.jsp Accessed November 12, 2012.
- Comorbidity measures for use with administrative data. Med Care. 1998;36(1):8–27. , , , .
- Physician views on caring for hospitalized patients and the hospitalist model of inpatient care. J Gen Intern Med. 2001;16(2):116–119. , , .
- How physicians perceive hospitalist services after implementation: anticipation vs reality. Arch Intern Med. 2003;163(19):2330–2336. , , , .
- Association of communication between hospital‐based physicians and primary care providers with patient outcomes. J Gen Intern Med. 2009;24(3):381–386. , , , et al.
- Passing the clinical baton: 6 principles to guide the hospitalist. Dis Mon. 2002;48(4):260–266. , , .
- Primary care physician attitudes regarding communication with hospitalists. Am J Med. 2001;111(9B):15S–20S. , , , .
- Transitions of Care Consensus policy statement: American College of Physicians, Society of General Internal Medicine, Society of Hospital Medicine, American Geriatrics Society, American College Of Emergency Physicians, and Society for Academic Emergency Medicine. J Hosp Med. 2009;4(6):364–370. , , , et al.
- Hospital survey shows improvements in patient experience. Health Aff (Millwood). 2010;29(11):2061–2067. , , , et al.
- Characteristics of hospitals demonstrating superior performance in patient experience and clinical process measures of care. Med Care Res Rev. 2010;67(1):38–55. , , , , , .
- The impact of hospitalists on the cost and quality of inpatient care in the United States: a research synthesis. Med Care Res Rev. 2005;62(4):379–406. , .
- Quality of care for patients hospitalized with heart failure: assessing the impact of hospitalists. Arch Intern Med. 2002;162(11):1251–1256. , , , , .
- Hospitalists and the quality of care in hospitals. Arch Intern Med. 2009;169(15):1389–1394. , , , , .
- Quality of care for decompensated heart failure: comparable performance between academic hospitalists and non‐hospitalists. J Gen Intern Med. 2008;23(9):1399–1406. , , , et al.
- Patient satisfaction with hospital care provided by hospitalists and primary care physicians. J Hosp Med. 2012;7(2):131–136. , , , et al.
Payers and policymakers are increasingly holding hospitals accountable for patients' experiences with their care. Since 2006, the Centers for Medicare and Medicaid Services (CMS) have collected data on patients' experiences with inpatient care using the Hospital Consumer Assessment of Healthcare Providers and Systems (HCAHPS) survey, a well‐validated and widely used tool. In 2008, these data on patient experience began to be publicly reported, and CMS now plans to base part of its payments to hospitals on HCAHPS performance scores. In this context, hospitals are looking for ways to improve patient satisfaction.
The effort to hold hospitals accountable for patient experience may conflict with another major trend in US hospitals: the increasing use of hospitalists.[1] Although hospitalists may have greater expertise in the day‐to‐day care of the hospitalized patient, they generally do not know the patient and cannot cater to patients' preferences in ways that the primary‐care provider might. Therefore, given that patients may prefer to be seen by their primary‐care provider,[2] greater use of hospitalists may actually lead to a decrease in patient satisfaction. Unfortunately, we are unaware of any national examination of the relationship between hospitalist use in an institution and that entity's performance on patient‐experience scores.
To better understand the relationship between greater hospitalist staffing and patient‐centered care, we examined the association between hospitalist staffing and patient satisfaction with both overall care and specific domains of patient‐centered care. We hypothesized that hospitals that used a high proportion of hospitalists would generally have lower patient‐experience scores. Further, we expected that the relationship would be monotonic (greater use of hospitalists associated with lower scores) and particularly pronounced in 2 domains: patient experience with discharge planning and patient experience with physician communication.
METHODS
Data
We sought to identify acute‐care hospitals with elderly medical patients cared for by hospitalists, non‐hospitalists, or some combination of the 2. To construct this cohort, we used 3 2009 Medicare files. The Beneficiary Summary File contains demographic information on Medicare beneficiaries and data on enrollment in managed‐care plans. To identify medical hospitalizations, we used the Medicare Provider Analysis and Review (MedPAR) 100% Files, which contain the clinical diagnoses and payments for all fee‐for‐service Medicare beneficiaries discharged from acute‐care hospitals. To identify hospitalists and non‐hospitalists, we used the 5% Carrier File, which contains physician billing data for a 5% random sample of fee‐for‐service Medicare beneficiaries. We also obtained information on hospital characteristics from the American Hospital Association (AHA) Annual Survey. We supplemented this with hospital‐level data on patient satisfaction from the HCAHPS survey conducted in 2009. The HCAHPS is a standard survey developed by the Agency for Healthcare Research and Quality (AHRQ) and administered by hospitals to a random sample of adult patients 48 hours to 6 weeks after discharge. The HCAHPS results are adjusted for patient mix and have been tested for nonresponse bias.[3] Details about the development and design of HCAHPS have been described previously.[4]
Patient and Hospital Sample
We started with 48,861,000 Medicare beneficiaries in the Beneficiary Summary File and excluded 38% either because their age was <65 years or they were members of an HMO. At the same time, from the 1,850,000 patients in the 5% Carrier File, we excluded 55% who had not been cared for by a general internist. Finally, we used the MedPAR File to identify 17,387,000 hospital admissions by fee‐for‐service Medicare beneficiaries. From MedPAR, we excluded admissions to a facility other than an acute‐care hospital (24%), surgical admissions identified by diagnosis‐related group (DRG) (29%), and admissions to hospitals with <5 medicine admissions in 2009 (<0.1%). After merging these 3 files (Beneficiary Summary, MedPAR, and 5% Carrier), we were left with 229,496 admissions among 180,399 patients at 3365 hospitals. We subsequently excluded readmissions and were left with 156,333 admissions at 3244 hospitals. Finally, we excluded those patients cared for by both hospitalists and non‐hospitalists during the same hospitalization, and those hospitals missing AHA or HCAHPS data, leaving us with 132,814 patients at 2843 hospitals.
Definition of Hospitalist
We used the claims‐based definition developed and validated by Kuo and Goodwin in earlier work.[1] Hospitalists are defined as those general internists (providers in general practice or internal medicine) who had 5 evaluation and management (E&M) billings (in a 5% sample of Medicare beneficiaries) in 2009 and generated >90% of their claims from the care of hospitalized patients in 2009.
Measures of Patient Satisfaction
There are 2 HCAHPS questions about overall satisfaction, one that asks patients to rate their experience on a scale of 0 to 10 and another that asks whether they would recommend the hospital. Not surprisingly, hospitals' performance on these 2 questions is highly correlated.[5] We measured overall patient experience using commonly used approaches: the proportion of patients who gave the hospital a 9 or 10 (on the 10‐point scale) or the proportion of patients who reported that they would definitely recommend the hospital. The HCAHPS also contains 24 questions, which are reported by CMS in 8 domains: communication with nurse, communication with physician, responsiveness of the staff, pain control, communication about medications, adequacy of discharge planning, cleanliness of the room, and quietness of the room. The patient‐satisfaction score for each of these domains represents the proportion of patients who answered always to each of the questions, or who answered yes to the question about discharge.
Potentially Confounding Variables
Because we were worried that hospitals with hospitalists would be different from hospitals without hospitalists, we identified a series of covariates for adjustment in a multivariable model. We extracted data from the AHA on hospitals' structural characteristics that we assumed might be associated both with having a hospitalist and with patient experience. These variables were size (number of beds), teaching status (membership in the Council of Teaching Hospitals vs no membership), location (urban vs rural), region (the 4 census regions), ownership (for profit, private nonprofit, or public), and presence of advanced clinical capabilities (as measured by having a medical, surgical, and/or cardiac intensive care unit [ICU]). We also used information about the patient population (proportion of patients with Medicare or with Medicaid) as well as nurse‐staffing level (ratio of full‐time equivalent registered nurses to total hospital beds).
Statistical Analyses
We first quantified hospital variation in the proportion of general‐medicine patients cared for by hospitalists, using basic descriptive statistics. Based on these analyses, we categorized hospitals into 3 groups: non‐hospitalist, mixed, and hospitalist (corresponding to lowest, middle, and highest tertile of hospitalist use respectively). We used bivariate techniques to describe the patient and hospital characteristics of hospitals in each group. Patient characteristics included number of comorbidities, which were calculated using software from the Healthcare Cost and Utilization Project (HCUP),[6] based on methods developed by Elixhauser et al.[7] We used the ‐square test to assess whether hospital or patient characteristics differed between hospitalist, mixed, and non‐hospitalist hospitals.
To examine the association between hospitalist care and patient satisfaction, we first constructed bivariate models for each measure of patient satisfaction. In these models, hospital type (hospitalist, mixed, and non‐hospitalist) was our predictor. We next constructed multivariable models, which adjusted for each of the hospital characteristics described above in order to assess the independent relationship between hospitalist care and HCAHPS performance.
In sensitivity analyses, we first examined hospitalist use as a continuous variable and had qualitatively very similar results. Those data are not presented. Additionally, we conducted a propensity score analysis, with results presented in the Appendix (see Supporting Information, Appendix 1, in the online version of this article). In our first‐stage logistic regression model, being a hospitalist hospital (defined as being in the top tertile of hospitalist use vs bottom 2 tertiles) was the outcome. Hospital structural factors were covariates. Based on this first‐stage model, each hospital was assigned a propensity of being a hospitalist hospital. We divided the hospitals into 3 groups (highest propensity tertile, middle propensity tertile, and lowest propensity tertile). In a second‐stage linear regression model, patient satisfaction score was the outcome. The predictors were hospital type (dichotomized, and defined as being in the top tertile of hospitalist use vs bottom 2 tertiles), and propensity of being a hospitalist hospital (3 categories, with low propensity as the reference).
All analyses were performed using SAS version 9.2. The project was reviewed by the Institutional Review Board at the University of Michigan and determined to be not regulated given our use of publicly available datasets.
RESULTS
Among all hospitals, the median proportion of general‐medicine admissions cared for by hospitalists was 41.2% (interquartile range [IQR], 11.5%67.4%). However, US hospitals varied widely in the proportion of general‐medicine patients cared for by hospitalists (Figure 1). Whereas 3.5% of hospitals had all of their general‐medicine patients cared for by hospitalists, 16.6% had none of their general‐medicine patients seen by hospitalists. For hospitals with at least some hospitalist care, the proportion of patients cared for by hospitalists was distributed fairly evenly across the range of possibilities (Figure 1).
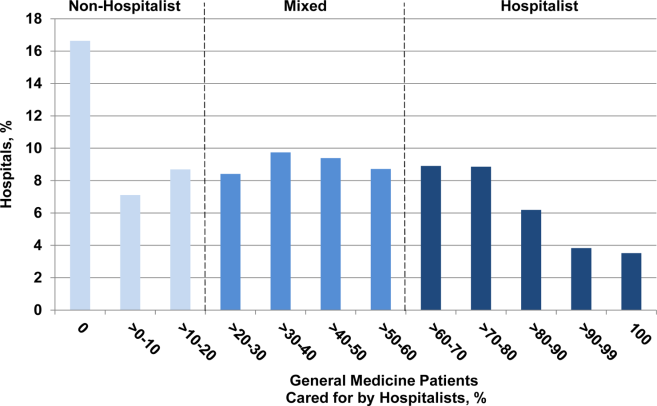
Because hospitalist care varied widely among hospitals, we categorized hospitals into 3 groups (non‐hospitalist, mixed, and hospitalist). The median proportion of patients cared for by hospitalists in the 3 groups was 0%, 39.5%, and 76.5%, respectively (Table 1). The non‐hospitalist hospitals, when compared with mixed and hospitalist hospitals, were more likely to be small, nonteaching, for‐profit institutions located in the Midwestern United States. They also were less likely to have an ICU and had lower nurse‐to‐bed ratios.
Hospital Characteristics | Hospital Type | P Value | ||
---|---|---|---|---|
Non‐Hospitalist (N = 943) | Mixed (N = 948) | Hospitalist (N = 952) | ||
| ||||
GM admissions cared for by hospitalists, median (range), % | 0 (021) | 40 (2158) | 77 (58100) | <0.001 |
Nurse‐to‐bed ratio | 1 | 1 | 2 | <0.001 |
Presence of MICU, % | 79 | 84 | 85 | 0.001 |
Medicaid patients, % | 19 | 18 | 18 | 0.06 |
Hospital beds, % | <0.001 | |||
Small (99) | 36 | 16 | 24 | |
Medium (100399) | 59 | 64 | 58 | |
Large (400) | 6 | 21 | 18 | |
COTH membership, % | <0.001 | |||
Yes | 3 | 13 | 11 | |
No | 97 | 87 | 89 | |
Urban, % | 0.10 | |||
Yes | 88 | 89 | 91 | |
No | 12 | 11 | 9 | |
Profit status, % | <0.001 | |||
For profit | 21 | 17 | 18 | |
Not for profit, private | 62 | 71 | 67 | |
Other | 18 | 12 | 15 | |
Region, % | <0.001 | |||
South | 41 | 42 | 42 | |
Northeast | 14 | 21 | 16 | |
Midwest | 30 | 22 | 18 | |
West | 15 | 15 | 24 |
The types of patients cared for at all 3 hospital types (non‐hospitalist, mixed, and hospitalist) were similar in age and day of admission (Table 2). Patients cared for at non‐hospitalist hospitals were slightly more likely to be female and non‐White, and less likely to be admitted from the emergency department or another hospital or healthcare facility.
Patient Characteristics | Hospital Type | P Value | ||
---|---|---|---|---|
Non‐Hospitalist (N = 33,265) | Mixed (N = 52,844) | Hospitalist (N = 46,705) | ||
| ||||
Age, y | 0.51 | |||
6574 | 27 | 27 | 27 | |
7584 | 39 | 39 | 39 | |
85 | 34 | 34 | 34 | |
Sex | <0.001 | |||
M | 35 | 35 | 36 | |
F | 65 | 65 | 64 | |
Race/ethnicity | <0.001 | |||
White | 85 | 85 | 87 | |
Black | 10 | 11 | 9 | |
Other | 5 | 4 | 4 | |
Unknown | 0 | 0 | 0 | |
Comorbidities, % | <0.001 | |||
0 | 8 | 8 | 7 | |
1 | 23 | 23 | 22 | |
2+ | 69 | 69 | 71 | |
Day of admission | 0.08 | |||
Weekday | 73 | 73 | 73 | |
Weekend | 27 | 27 | 27 | |
Admission source | <0.001 | |||
ED | 75 | 78 | 80 | |
Another ACH | 1 | 2 | 3 | |
Other healthcare facility | 4 | 4 | 4 | |
Other | 20 | 17 | 13 | |
ICU stay | <0.001 | |||
Yes | 13 | 12 | 12 | |
No | 87 | 88 | 88 | |
Length of stay, d | <0.001 | |||
Median (Q1, Q3) | 4 (3, 6) | 4 (2, 6) | 3 (2, 5) | |
DRG | <0.001 | |||
Septicemia or severe sepsis | 3 | 4 | 4 | |
Esophagitis, gastroenteritis | 3 | 3 | 3 | |
Kidney and urinary tract infections | 3 | 3 | 3 | |
Syncope | 3 | 3 | 3 | |
Pneumonia | 3 | 3 | 3 |
When we examined unadjusted relationships between type of hospital and patient experience, we found that patients at hospitalist vs non‐hospitalist hospitals were more likely to recommend the hospital (69.4% vs 65.1%: P < 0.001), and report higher overall satisfaction (65.9% vs 63.6%: P < 0.001) ((see Supporting Information, Appendix, Table A1, in the online version of this article)). Care at hospitalist hospitals was associated with higher satisfaction with discharge, but lower satisfaction with room cleanliness and communication with doctors. These differences were statistically significant at the P < 0.05 level.
When we examined the relationship between having more hospitalists and patient experience using multivariable models that accounted for differences in hospital characteristics, we found largely similar results: The proportion of patients who were satisfied with their overall care was still higher at hospitalist compared with non‐hospitalist hospitals (65.6% vs 63.9%: P < 0.001) (Figure 2). Similarly, patients were more likely to definitely recommend their hospital if they had been cared for at a hospitalist vs non‐hospitalist hospital (66.0% vs 63.4%: P < 0.001).
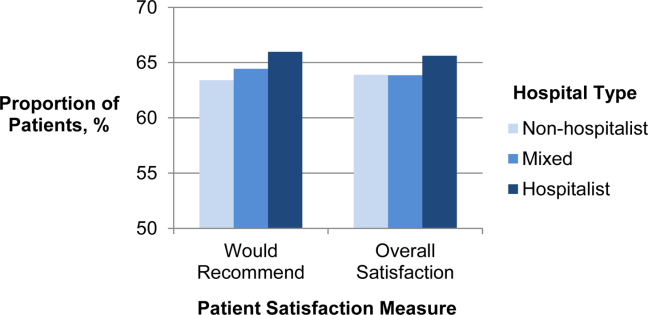
To better understand which domains of care might be contributing to greater overall satisfaction, we also examined patient satisfaction with specific domains of care at hospitalist vs non‐hospitalist hospitals (Table 3) in our adjusted analyses. Among 8 domains, the largest difference in satisfaction between patients cared for at hospitalist vs non‐hospitalist hospitals occurred with discharge. At hospitalist hospitals, 80.3% of patients said they were satisfied with the quality of the discharge planning compared with 78.1% at non‐hospitalist hospitals (P < 0.001). Patients at hospitalist hospitals were more satisfied with most other domains of care as well. Patients cared for at hospitalist hospitals were slightly less likely to be satisfied with communication with doctors, but this difference was not statistically significant (P = 0.45). Results were qualitatively similar in propensity‐score analyses (see Supporting Information, Appendix, Table A2, in the online version of this article).
Specific Domains of Care | Hospital Type, % Satisfied | Hospitalist vs Non‐Hospitalist | |||
---|---|---|---|---|---|
Non‐Hospitalist | Mixed | Hospitalist | Difference in % Satisfied | P Value | |
| |||||
Discharge | 78.1 | 79.1 | 80.3 | 2.1 | <0.001 |
Nursing services | 66.0 | 65.8 | 67.1 | 1.1 | <0.001 |
Quiet | 63.3 | 63.1 | 64.4 | 1.1 | 0.001 |
Communication, nurse | 76.7 | 76.7 | 77.7 | 1.0 | <0.001 |
Pain control | 69.7 | 69.7 | 70.4 | 0.7 | 0.001 |
Medications | 60.5 | 60.5 | 61.2 | 0.7 | 0.002 |
Cleanliness | 72.7 | 72.1 | 72.9 | 0.2 | 0.56 |
Communication, physician | 83.6 | 83.1 | 83.5 | 0.2 | 0.45 |
DISCUSSION
We found that in 2009, US hospitals varied widely in the proportion of general medicine patients cared for by hospitalists. Hospitals with higher levels of hospitalist care did better on most measures of patient satisfaction. Differences were largest in overall satisfaction and for discharge planning. In 5 other domains of care, differences were smaller, but hospitals with more hospitalist care consistently performed better than non‐hospitalist hospitals. Hospitalist care was not associated with patient satisfaction in 2 domains: communication with doctors and cleanliness of room.
Our findings of modestly higher patient satisfaction at hospitalist hospitals along most dimensions of care are surprising and reassuring. Indeed, when hospitalists first began caring for inpatients, some expressed concerns that hospitalist care would decrease patient satisfaction.[8, 9] Though this has been an ongoing concern, we found no evidence to support this contention. It may be that as a response to the concern, hospitals with hospitalists have paid particular attention to issues such as effective handoffs to primary‐care providers.[10, 11, 12, 13] Whether due to these efforts or other factors such as the 24/7 inpatient presence of hospitalists, we found that patients at hospitalist hospitals were more likely to be satisfied with their inpatient care, including their experience at discharge. In contrast, one area that may offer room for improvement for hospitalist hospitals is communication with physicians. It may be that patients cared for by hospitalists do not know their physicians as well as patients whose care is being orchestrated by their primary‐care provider, and thus the benefits of having an ever‐present hospitalist are diminished.
The magnitude of the associations that we found should also be placed in the context of existing research on patient satisfaction. Prior work has described baseline hospital performance, changes over time, and factors associated with greater inpatient satisfaction.[5, 14, 15] The associations that we found between hospitalist care and satisfaction with care at discharge were larger than those found for teaching (vs non‐teaching) hospitals.[5] However, compared with other hospital characteristics such as nurse staffing or profit status, hospitalist care was associated with smaller differences in patient satisfaction. In one study, hospitals in the highest quartile of nurse staffing had HCAHPS scores (ie, willingness to recommend measure) that were 6.7 points higher than those in the lowest quartile of nurse staffing, and similar differences existed between not‐for‐profit, public hospitals vs for‐profit hospitals.[5]
Taken together, our findings address an important gap in knowledge about hospitalist care. Prior research has documented growth in the use of hospitalist care[1] and described the association of hospitalist care with outcomes such as mortality and resource use, and receipt of recommended care.[16, 17, 18, 19] However, we are unaware of any national study that has examined the association of hospitalist care with patient satisfaction. One study surveyed patients in a single health system and found that patients were similarly satisfied with care provided by hospitalists and primary‐care physicians.[20] Our findings should be reassuring to clinical leaders and policymakers who have advocated greater use of hospitalists: the results suggest that there need be no tradeoff between greater use of hospitalist services and patient satisfaction. Indeed, patients appear to be even more satisfied in hospitals that have greater use of hospitalist physicians.
Our study has several limitations. First, it was a cross‐sectional study, and thus we cannot make any conclusions about causality. Although we adjusted for several potential confounders (eg, teaching status, advanced care capabilities, nurse staffing), it is possible that hospitalist care is a surrogate marker for features of hospitals that we could not measure but that directly influence patient experience. In addition, it is possible that patients cared for at hospitalist hospitals differ in unmeasured ways from patients cared for at other types of hospitals. Second, we constructed our primary predictor and outcome from different cohorts. Our primary predictor was derived from the proportion of general‐medicine patients cared for by hospitalists in Medicare claims data. In contrast, our primary outcome was based on HCAHPS responses from a random sampling of all hospital admissions. This misclassification likely would have biased us towards finding small or no associations. Therefore, we are likely underestimating the true association between hospitalist use and patient experience. Third, our findings may not be generalizable to hospitals that serve younger patients or have a large number of specialist hospitalists (who were not included in our definition of hospitalists). For example, compared with older patients with multiple comorbidities, relatively healthy younger patients may derive less benefit from an ever‐present hospitalist who can explain discharge plans or an attentive nurse.
In summary, we found that US hospitals varied widely in their use of hospitalist physicians, and those which a greater proportion of care was delivered by hospitalists generally had better scores on patient experience, especially on the global assessment of satisfaction and in discharge care. Our findings suggest that adoption of the hospitalist modelone of the strategies employed by US hospitals in the past 2 decades to provide efficient careshould not detract from achieving the goal of more patient‐centered care.
Disclosures
Dr. Chen's work is supported in part by the National Institutes of Health/National Institute on Aging (AG024824, University of Michigan Claude D. Pepper Older Americans Independence Center), and the National Institutes of Health/National Center for Research Resources (UL1‐RR024986, Michigan Institute for Clinical and Health Research). Dr. Chen is also supported by a Career Development Grant Award (K08HS020671) from the Agency for Healthcare Research and Quality.
Payers and policymakers are increasingly holding hospitals accountable for patients' experiences with their care. Since 2006, the Centers for Medicare and Medicaid Services (CMS) have collected data on patients' experiences with inpatient care using the Hospital Consumer Assessment of Healthcare Providers and Systems (HCAHPS) survey, a well‐validated and widely used tool. In 2008, these data on patient experience began to be publicly reported, and CMS now plans to base part of its payments to hospitals on HCAHPS performance scores. In this context, hospitals are looking for ways to improve patient satisfaction.
The effort to hold hospitals accountable for patient experience may conflict with another major trend in US hospitals: the increasing use of hospitalists.[1] Although hospitalists may have greater expertise in the day‐to‐day care of the hospitalized patient, they generally do not know the patient and cannot cater to patients' preferences in ways that the primary‐care provider might. Therefore, given that patients may prefer to be seen by their primary‐care provider,[2] greater use of hospitalists may actually lead to a decrease in patient satisfaction. Unfortunately, we are unaware of any national examination of the relationship between hospitalist use in an institution and that entity's performance on patient‐experience scores.
To better understand the relationship between greater hospitalist staffing and patient‐centered care, we examined the association between hospitalist staffing and patient satisfaction with both overall care and specific domains of patient‐centered care. We hypothesized that hospitals that used a high proportion of hospitalists would generally have lower patient‐experience scores. Further, we expected that the relationship would be monotonic (greater use of hospitalists associated with lower scores) and particularly pronounced in 2 domains: patient experience with discharge planning and patient experience with physician communication.
METHODS
Data
We sought to identify acute‐care hospitals with elderly medical patients cared for by hospitalists, non‐hospitalists, or some combination of the 2. To construct this cohort, we used 3 2009 Medicare files. The Beneficiary Summary File contains demographic information on Medicare beneficiaries and data on enrollment in managed‐care plans. To identify medical hospitalizations, we used the Medicare Provider Analysis and Review (MedPAR) 100% Files, which contain the clinical diagnoses and payments for all fee‐for‐service Medicare beneficiaries discharged from acute‐care hospitals. To identify hospitalists and non‐hospitalists, we used the 5% Carrier File, which contains physician billing data for a 5% random sample of fee‐for‐service Medicare beneficiaries. We also obtained information on hospital characteristics from the American Hospital Association (AHA) Annual Survey. We supplemented this with hospital‐level data on patient satisfaction from the HCAHPS survey conducted in 2009. The HCAHPS is a standard survey developed by the Agency for Healthcare Research and Quality (AHRQ) and administered by hospitals to a random sample of adult patients 48 hours to 6 weeks after discharge. The HCAHPS results are adjusted for patient mix and have been tested for nonresponse bias.[3] Details about the development and design of HCAHPS have been described previously.[4]
Patient and Hospital Sample
We started with 48,861,000 Medicare beneficiaries in the Beneficiary Summary File and excluded 38% either because their age was <65 years or they were members of an HMO. At the same time, from the 1,850,000 patients in the 5% Carrier File, we excluded 55% who had not been cared for by a general internist. Finally, we used the MedPAR File to identify 17,387,000 hospital admissions by fee‐for‐service Medicare beneficiaries. From MedPAR, we excluded admissions to a facility other than an acute‐care hospital (24%), surgical admissions identified by diagnosis‐related group (DRG) (29%), and admissions to hospitals with <5 medicine admissions in 2009 (<0.1%). After merging these 3 files (Beneficiary Summary, MedPAR, and 5% Carrier), we were left with 229,496 admissions among 180,399 patients at 3365 hospitals. We subsequently excluded readmissions and were left with 156,333 admissions at 3244 hospitals. Finally, we excluded those patients cared for by both hospitalists and non‐hospitalists during the same hospitalization, and those hospitals missing AHA or HCAHPS data, leaving us with 132,814 patients at 2843 hospitals.
Definition of Hospitalist
We used the claims‐based definition developed and validated by Kuo and Goodwin in earlier work.[1] Hospitalists are defined as those general internists (providers in general practice or internal medicine) who had 5 evaluation and management (E&M) billings (in a 5% sample of Medicare beneficiaries) in 2009 and generated >90% of their claims from the care of hospitalized patients in 2009.
Measures of Patient Satisfaction
There are 2 HCAHPS questions about overall satisfaction, one that asks patients to rate their experience on a scale of 0 to 10 and another that asks whether they would recommend the hospital. Not surprisingly, hospitals' performance on these 2 questions is highly correlated.[5] We measured overall patient experience using commonly used approaches: the proportion of patients who gave the hospital a 9 or 10 (on the 10‐point scale) or the proportion of patients who reported that they would definitely recommend the hospital. The HCAHPS also contains 24 questions, which are reported by CMS in 8 domains: communication with nurse, communication with physician, responsiveness of the staff, pain control, communication about medications, adequacy of discharge planning, cleanliness of the room, and quietness of the room. The patient‐satisfaction score for each of these domains represents the proportion of patients who answered always to each of the questions, or who answered yes to the question about discharge.
Potentially Confounding Variables
Because we were worried that hospitals with hospitalists would be different from hospitals without hospitalists, we identified a series of covariates for adjustment in a multivariable model. We extracted data from the AHA on hospitals' structural characteristics that we assumed might be associated both with having a hospitalist and with patient experience. These variables were size (number of beds), teaching status (membership in the Council of Teaching Hospitals vs no membership), location (urban vs rural), region (the 4 census regions), ownership (for profit, private nonprofit, or public), and presence of advanced clinical capabilities (as measured by having a medical, surgical, and/or cardiac intensive care unit [ICU]). We also used information about the patient population (proportion of patients with Medicare or with Medicaid) as well as nurse‐staffing level (ratio of full‐time equivalent registered nurses to total hospital beds).
Statistical Analyses
We first quantified hospital variation in the proportion of general‐medicine patients cared for by hospitalists, using basic descriptive statistics. Based on these analyses, we categorized hospitals into 3 groups: non‐hospitalist, mixed, and hospitalist (corresponding to lowest, middle, and highest tertile of hospitalist use respectively). We used bivariate techniques to describe the patient and hospital characteristics of hospitals in each group. Patient characteristics included number of comorbidities, which were calculated using software from the Healthcare Cost and Utilization Project (HCUP),[6] based on methods developed by Elixhauser et al.[7] We used the ‐square test to assess whether hospital or patient characteristics differed between hospitalist, mixed, and non‐hospitalist hospitals.
To examine the association between hospitalist care and patient satisfaction, we first constructed bivariate models for each measure of patient satisfaction. In these models, hospital type (hospitalist, mixed, and non‐hospitalist) was our predictor. We next constructed multivariable models, which adjusted for each of the hospital characteristics described above in order to assess the independent relationship between hospitalist care and HCAHPS performance.
In sensitivity analyses, we first examined hospitalist use as a continuous variable and had qualitatively very similar results. Those data are not presented. Additionally, we conducted a propensity score analysis, with results presented in the Appendix (see Supporting Information, Appendix 1, in the online version of this article). In our first‐stage logistic regression model, being a hospitalist hospital (defined as being in the top tertile of hospitalist use vs bottom 2 tertiles) was the outcome. Hospital structural factors were covariates. Based on this first‐stage model, each hospital was assigned a propensity of being a hospitalist hospital. We divided the hospitals into 3 groups (highest propensity tertile, middle propensity tertile, and lowest propensity tertile). In a second‐stage linear regression model, patient satisfaction score was the outcome. The predictors were hospital type (dichotomized, and defined as being in the top tertile of hospitalist use vs bottom 2 tertiles), and propensity of being a hospitalist hospital (3 categories, with low propensity as the reference).
All analyses were performed using SAS version 9.2. The project was reviewed by the Institutional Review Board at the University of Michigan and determined to be not regulated given our use of publicly available datasets.
RESULTS
Among all hospitals, the median proportion of general‐medicine admissions cared for by hospitalists was 41.2% (interquartile range [IQR], 11.5%67.4%). However, US hospitals varied widely in the proportion of general‐medicine patients cared for by hospitalists (Figure 1). Whereas 3.5% of hospitals had all of their general‐medicine patients cared for by hospitalists, 16.6% had none of their general‐medicine patients seen by hospitalists. For hospitals with at least some hospitalist care, the proportion of patients cared for by hospitalists was distributed fairly evenly across the range of possibilities (Figure 1).
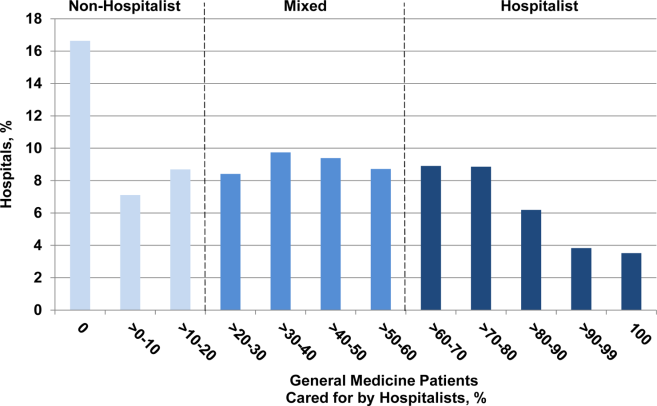
Because hospitalist care varied widely among hospitals, we categorized hospitals into 3 groups (non‐hospitalist, mixed, and hospitalist). The median proportion of patients cared for by hospitalists in the 3 groups was 0%, 39.5%, and 76.5%, respectively (Table 1). The non‐hospitalist hospitals, when compared with mixed and hospitalist hospitals, were more likely to be small, nonteaching, for‐profit institutions located in the Midwestern United States. They also were less likely to have an ICU and had lower nurse‐to‐bed ratios.
Hospital Characteristics | Hospital Type | P Value | ||
---|---|---|---|---|
Non‐Hospitalist (N = 943) | Mixed (N = 948) | Hospitalist (N = 952) | ||
| ||||
GM admissions cared for by hospitalists, median (range), % | 0 (021) | 40 (2158) | 77 (58100) | <0.001 |
Nurse‐to‐bed ratio | 1 | 1 | 2 | <0.001 |
Presence of MICU, % | 79 | 84 | 85 | 0.001 |
Medicaid patients, % | 19 | 18 | 18 | 0.06 |
Hospital beds, % | <0.001 | |||
Small (99) | 36 | 16 | 24 | |
Medium (100399) | 59 | 64 | 58 | |
Large (400) | 6 | 21 | 18 | |
COTH membership, % | <0.001 | |||
Yes | 3 | 13 | 11 | |
No | 97 | 87 | 89 | |
Urban, % | 0.10 | |||
Yes | 88 | 89 | 91 | |
No | 12 | 11 | 9 | |
Profit status, % | <0.001 | |||
For profit | 21 | 17 | 18 | |
Not for profit, private | 62 | 71 | 67 | |
Other | 18 | 12 | 15 | |
Region, % | <0.001 | |||
South | 41 | 42 | 42 | |
Northeast | 14 | 21 | 16 | |
Midwest | 30 | 22 | 18 | |
West | 15 | 15 | 24 |
The types of patients cared for at all 3 hospital types (non‐hospitalist, mixed, and hospitalist) were similar in age and day of admission (Table 2). Patients cared for at non‐hospitalist hospitals were slightly more likely to be female and non‐White, and less likely to be admitted from the emergency department or another hospital or healthcare facility.
Patient Characteristics | Hospital Type | P Value | ||
---|---|---|---|---|
Non‐Hospitalist (N = 33,265) | Mixed (N = 52,844) | Hospitalist (N = 46,705) | ||
| ||||
Age, y | 0.51 | |||
6574 | 27 | 27 | 27 | |
7584 | 39 | 39 | 39 | |
85 | 34 | 34 | 34 | |
Sex | <0.001 | |||
M | 35 | 35 | 36 | |
F | 65 | 65 | 64 | |
Race/ethnicity | <0.001 | |||
White | 85 | 85 | 87 | |
Black | 10 | 11 | 9 | |
Other | 5 | 4 | 4 | |
Unknown | 0 | 0 | 0 | |
Comorbidities, % | <0.001 | |||
0 | 8 | 8 | 7 | |
1 | 23 | 23 | 22 | |
2+ | 69 | 69 | 71 | |
Day of admission | 0.08 | |||
Weekday | 73 | 73 | 73 | |
Weekend | 27 | 27 | 27 | |
Admission source | <0.001 | |||
ED | 75 | 78 | 80 | |
Another ACH | 1 | 2 | 3 | |
Other healthcare facility | 4 | 4 | 4 | |
Other | 20 | 17 | 13 | |
ICU stay | <0.001 | |||
Yes | 13 | 12 | 12 | |
No | 87 | 88 | 88 | |
Length of stay, d | <0.001 | |||
Median (Q1, Q3) | 4 (3, 6) | 4 (2, 6) | 3 (2, 5) | |
DRG | <0.001 | |||
Septicemia or severe sepsis | 3 | 4 | 4 | |
Esophagitis, gastroenteritis | 3 | 3 | 3 | |
Kidney and urinary tract infections | 3 | 3 | 3 | |
Syncope | 3 | 3 | 3 | |
Pneumonia | 3 | 3 | 3 |
When we examined unadjusted relationships between type of hospital and patient experience, we found that patients at hospitalist vs non‐hospitalist hospitals were more likely to recommend the hospital (69.4% vs 65.1%: P < 0.001), and report higher overall satisfaction (65.9% vs 63.6%: P < 0.001) ((see Supporting Information, Appendix, Table A1, in the online version of this article)). Care at hospitalist hospitals was associated with higher satisfaction with discharge, but lower satisfaction with room cleanliness and communication with doctors. These differences were statistically significant at the P < 0.05 level.
When we examined the relationship between having more hospitalists and patient experience using multivariable models that accounted for differences in hospital characteristics, we found largely similar results: The proportion of patients who were satisfied with their overall care was still higher at hospitalist compared with non‐hospitalist hospitals (65.6% vs 63.9%: P < 0.001) (Figure 2). Similarly, patients were more likely to definitely recommend their hospital if they had been cared for at a hospitalist vs non‐hospitalist hospital (66.0% vs 63.4%: P < 0.001).
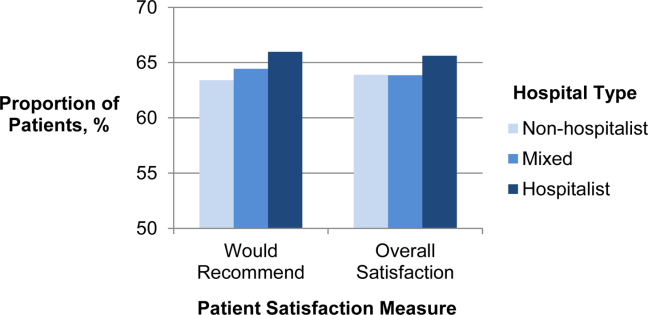
To better understand which domains of care might be contributing to greater overall satisfaction, we also examined patient satisfaction with specific domains of care at hospitalist vs non‐hospitalist hospitals (Table 3) in our adjusted analyses. Among 8 domains, the largest difference in satisfaction between patients cared for at hospitalist vs non‐hospitalist hospitals occurred with discharge. At hospitalist hospitals, 80.3% of patients said they were satisfied with the quality of the discharge planning compared with 78.1% at non‐hospitalist hospitals (P < 0.001). Patients at hospitalist hospitals were more satisfied with most other domains of care as well. Patients cared for at hospitalist hospitals were slightly less likely to be satisfied with communication with doctors, but this difference was not statistically significant (P = 0.45). Results were qualitatively similar in propensity‐score analyses (see Supporting Information, Appendix, Table A2, in the online version of this article).
Specific Domains of Care | Hospital Type, % Satisfied | Hospitalist vs Non‐Hospitalist | |||
---|---|---|---|---|---|
Non‐Hospitalist | Mixed | Hospitalist | Difference in % Satisfied | P Value | |
| |||||
Discharge | 78.1 | 79.1 | 80.3 | 2.1 | <0.001 |
Nursing services | 66.0 | 65.8 | 67.1 | 1.1 | <0.001 |
Quiet | 63.3 | 63.1 | 64.4 | 1.1 | 0.001 |
Communication, nurse | 76.7 | 76.7 | 77.7 | 1.0 | <0.001 |
Pain control | 69.7 | 69.7 | 70.4 | 0.7 | 0.001 |
Medications | 60.5 | 60.5 | 61.2 | 0.7 | 0.002 |
Cleanliness | 72.7 | 72.1 | 72.9 | 0.2 | 0.56 |
Communication, physician | 83.6 | 83.1 | 83.5 | 0.2 | 0.45 |
DISCUSSION
We found that in 2009, US hospitals varied widely in the proportion of general medicine patients cared for by hospitalists. Hospitals with higher levels of hospitalist care did better on most measures of patient satisfaction. Differences were largest in overall satisfaction and for discharge planning. In 5 other domains of care, differences were smaller, but hospitals with more hospitalist care consistently performed better than non‐hospitalist hospitals. Hospitalist care was not associated with patient satisfaction in 2 domains: communication with doctors and cleanliness of room.
Our findings of modestly higher patient satisfaction at hospitalist hospitals along most dimensions of care are surprising and reassuring. Indeed, when hospitalists first began caring for inpatients, some expressed concerns that hospitalist care would decrease patient satisfaction.[8, 9] Though this has been an ongoing concern, we found no evidence to support this contention. It may be that as a response to the concern, hospitals with hospitalists have paid particular attention to issues such as effective handoffs to primary‐care providers.[10, 11, 12, 13] Whether due to these efforts or other factors such as the 24/7 inpatient presence of hospitalists, we found that patients at hospitalist hospitals were more likely to be satisfied with their inpatient care, including their experience at discharge. In contrast, one area that may offer room for improvement for hospitalist hospitals is communication with physicians. It may be that patients cared for by hospitalists do not know their physicians as well as patients whose care is being orchestrated by their primary‐care provider, and thus the benefits of having an ever‐present hospitalist are diminished.
The magnitude of the associations that we found should also be placed in the context of existing research on patient satisfaction. Prior work has described baseline hospital performance, changes over time, and factors associated with greater inpatient satisfaction.[5, 14, 15] The associations that we found between hospitalist care and satisfaction with care at discharge were larger than those found for teaching (vs non‐teaching) hospitals.[5] However, compared with other hospital characteristics such as nurse staffing or profit status, hospitalist care was associated with smaller differences in patient satisfaction. In one study, hospitals in the highest quartile of nurse staffing had HCAHPS scores (ie, willingness to recommend measure) that were 6.7 points higher than those in the lowest quartile of nurse staffing, and similar differences existed between not‐for‐profit, public hospitals vs for‐profit hospitals.[5]
Taken together, our findings address an important gap in knowledge about hospitalist care. Prior research has documented growth in the use of hospitalist care[1] and described the association of hospitalist care with outcomes such as mortality and resource use, and receipt of recommended care.[16, 17, 18, 19] However, we are unaware of any national study that has examined the association of hospitalist care with patient satisfaction. One study surveyed patients in a single health system and found that patients were similarly satisfied with care provided by hospitalists and primary‐care physicians.[20] Our findings should be reassuring to clinical leaders and policymakers who have advocated greater use of hospitalists: the results suggest that there need be no tradeoff between greater use of hospitalist services and patient satisfaction. Indeed, patients appear to be even more satisfied in hospitals that have greater use of hospitalist physicians.
Our study has several limitations. First, it was a cross‐sectional study, and thus we cannot make any conclusions about causality. Although we adjusted for several potential confounders (eg, teaching status, advanced care capabilities, nurse staffing), it is possible that hospitalist care is a surrogate marker for features of hospitals that we could not measure but that directly influence patient experience. In addition, it is possible that patients cared for at hospitalist hospitals differ in unmeasured ways from patients cared for at other types of hospitals. Second, we constructed our primary predictor and outcome from different cohorts. Our primary predictor was derived from the proportion of general‐medicine patients cared for by hospitalists in Medicare claims data. In contrast, our primary outcome was based on HCAHPS responses from a random sampling of all hospital admissions. This misclassification likely would have biased us towards finding small or no associations. Therefore, we are likely underestimating the true association between hospitalist use and patient experience. Third, our findings may not be generalizable to hospitals that serve younger patients or have a large number of specialist hospitalists (who were not included in our definition of hospitalists). For example, compared with older patients with multiple comorbidities, relatively healthy younger patients may derive less benefit from an ever‐present hospitalist who can explain discharge plans or an attentive nurse.
In summary, we found that US hospitals varied widely in their use of hospitalist physicians, and those which a greater proportion of care was delivered by hospitalists generally had better scores on patient experience, especially on the global assessment of satisfaction and in discharge care. Our findings suggest that adoption of the hospitalist modelone of the strategies employed by US hospitals in the past 2 decades to provide efficient careshould not detract from achieving the goal of more patient‐centered care.
Disclosures
Dr. Chen's work is supported in part by the National Institutes of Health/National Institute on Aging (AG024824, University of Michigan Claude D. Pepper Older Americans Independence Center), and the National Institutes of Health/National Center for Research Resources (UL1‐RR024986, Michigan Institute for Clinical and Health Research). Dr. Chen is also supported by a Career Development Grant Award (K08HS020671) from the Agency for Healthcare Research and Quality.
- Growth in the care of older patients by hospitalists in the United States. N Engl J Med. 2009;360(11):1102–1112. , , , .
- How do patients view the role of the primary care physician in inpatient care? Dis Mon. 2002;48(4):230–238. , , .
- Effects of survey mode, patient mix, and nonresponse on CAHPS hospital survey scores. Health Serv Res. 2009;44(2 pt 1):501–518. , , , et al.
- Development, implementation, and public reporting of the HCAHPS survey. Med Care Res Rev. 2010;67(1):27–37. , , , , .
- Patients' perception of hospital care in the United States. N Engl J Med. 2008;359(18):1921–1931. , , , .
- Agency for Healthcare Research and Quality. Healthcare Cost and Utilization Project (HCUP). HCUP Comorbidity Software. http://www.hcup‐us.ahrq.gov/toolssoftware/comorbidity/comorbidity.jsp Accessed November 12, 2012.
- Comorbidity measures for use with administrative data. Med Care. 1998;36(1):8–27. , , , .
- Physician views on caring for hospitalized patients and the hospitalist model of inpatient care. J Gen Intern Med. 2001;16(2):116–119. , , .
- How physicians perceive hospitalist services after implementation: anticipation vs reality. Arch Intern Med. 2003;163(19):2330–2336. , , , .
- Association of communication between hospital‐based physicians and primary care providers with patient outcomes. J Gen Intern Med. 2009;24(3):381–386. , , , et al.
- Passing the clinical baton: 6 principles to guide the hospitalist. Dis Mon. 2002;48(4):260–266. , , .
- Primary care physician attitudes regarding communication with hospitalists. Am J Med. 2001;111(9B):15S–20S. , , , .
- Transitions of Care Consensus policy statement: American College of Physicians, Society of General Internal Medicine, Society of Hospital Medicine, American Geriatrics Society, American College Of Emergency Physicians, and Society for Academic Emergency Medicine. J Hosp Med. 2009;4(6):364–370. , , , et al.
- Hospital survey shows improvements in patient experience. Health Aff (Millwood). 2010;29(11):2061–2067. , , , et al.
- Characteristics of hospitals demonstrating superior performance in patient experience and clinical process measures of care. Med Care Res Rev. 2010;67(1):38–55. , , , , , .
- The impact of hospitalists on the cost and quality of inpatient care in the United States: a research synthesis. Med Care Res Rev. 2005;62(4):379–406. , .
- Quality of care for patients hospitalized with heart failure: assessing the impact of hospitalists. Arch Intern Med. 2002;162(11):1251–1256. , , , , .
- Hospitalists and the quality of care in hospitals. Arch Intern Med. 2009;169(15):1389–1394. , , , , .
- Quality of care for decompensated heart failure: comparable performance between academic hospitalists and non‐hospitalists. J Gen Intern Med. 2008;23(9):1399–1406. , , , et al.
- Patient satisfaction with hospital care provided by hospitalists and primary care physicians. J Hosp Med. 2012;7(2):131–136. , , , et al.
- Growth in the care of older patients by hospitalists in the United States. N Engl J Med. 2009;360(11):1102–1112. , , , .
- How do patients view the role of the primary care physician in inpatient care? Dis Mon. 2002;48(4):230–238. , , .
- Effects of survey mode, patient mix, and nonresponse on CAHPS hospital survey scores. Health Serv Res. 2009;44(2 pt 1):501–518. , , , et al.
- Development, implementation, and public reporting of the HCAHPS survey. Med Care Res Rev. 2010;67(1):27–37. , , , , .
- Patients' perception of hospital care in the United States. N Engl J Med. 2008;359(18):1921–1931. , , , .
- Agency for Healthcare Research and Quality. Healthcare Cost and Utilization Project (HCUP). HCUP Comorbidity Software. http://www.hcup‐us.ahrq.gov/toolssoftware/comorbidity/comorbidity.jsp Accessed November 12, 2012.
- Comorbidity measures for use with administrative data. Med Care. 1998;36(1):8–27. , , , .
- Physician views on caring for hospitalized patients and the hospitalist model of inpatient care. J Gen Intern Med. 2001;16(2):116–119. , , .
- How physicians perceive hospitalist services after implementation: anticipation vs reality. Arch Intern Med. 2003;163(19):2330–2336. , , , .
- Association of communication between hospital‐based physicians and primary care providers with patient outcomes. J Gen Intern Med. 2009;24(3):381–386. , , , et al.
- Passing the clinical baton: 6 principles to guide the hospitalist. Dis Mon. 2002;48(4):260–266. , , .
- Primary care physician attitudes regarding communication with hospitalists. Am J Med. 2001;111(9B):15S–20S. , , , .
- Transitions of Care Consensus policy statement: American College of Physicians, Society of General Internal Medicine, Society of Hospital Medicine, American Geriatrics Society, American College Of Emergency Physicians, and Society for Academic Emergency Medicine. J Hosp Med. 2009;4(6):364–370. , , , et al.
- Hospital survey shows improvements in patient experience. Health Aff (Millwood). 2010;29(11):2061–2067. , , , et al.
- Characteristics of hospitals demonstrating superior performance in patient experience and clinical process measures of care. Med Care Res Rev. 2010;67(1):38–55. , , , , , .
- The impact of hospitalists on the cost and quality of inpatient care in the United States: a research synthesis. Med Care Res Rev. 2005;62(4):379–406. , .
- Quality of care for patients hospitalized with heart failure: assessing the impact of hospitalists. Arch Intern Med. 2002;162(11):1251–1256. , , , , .
- Hospitalists and the quality of care in hospitals. Arch Intern Med. 2009;169(15):1389–1394. , , , , .
- Quality of care for decompensated heart failure: comparable performance between academic hospitalists and non‐hospitalists. J Gen Intern Med. 2008;23(9):1399–1406. , , , et al.
- Patient satisfaction with hospital care provided by hospitalists and primary care physicians. J Hosp Med. 2012;7(2):131–136. , , , et al.
Copyright © 2012 Society of Hospital Medicine
Late Discharges from a Medical Ward
In the past 2 decades, emergency department (ED) overcrowding has become an issue large enough to receive coverage in the popular press, and to spawn research around its causes and effects.116 At the same time, nurses and physicians on the inpatient wards have been urged to shorten the length of stay for patients as health system leaders face an aging population but limited capital to build new beds or hire additional clinical staff. Capacity managementencompassing the flow of patients from ED triage to inpatient dischargehas become a shared concern of clinicians and hospital administrators alike.
How to achieve the goals of diagnosing and healing while ushering patients ever more quickly through the modern hospital is not yet entirely clear. Past research and work by business groups suggests that demand for inpatient beds starts early in the day, but discharges typically occur in the late afternoon.17 This creates a potential bottleneck in patient flow. Many hospitals have implemented measures to improve patient throughput.1821 However, formal research has focused on factors leading to an additional inpatient day.2226 We have found no peer‐reviewed publications that address the problem of same‐day delays by describing hour of day for each step in the discharge process and variables associated with late‐day discharges. To fill this gap, we conducted a prospective cohort study of 209 consecutive discharges from a general medical ward to: (1) describe the natural history of hospital discharge, (2) measure time of day and duration for each step, and (3) identify factors associated with discharges that occur later in the day. We hypothesized that time and duration of discharge would be associated with 5 factors: patient demographics and clinical characteristics, departmental occupancy, type of inpatient testing done immediately prior to discharge, and discharge characteristics such as discharge to a location other than home.
Patients and Methods
Setting
The setting was the Hospitalist Unit of a single teaching hospital in Baltimore (The Johns Hopkins Hospital) from January 1, 2005 to April 30, 2005. Patients entered the cohort upon initiation of the discharge process by the hospitalist team on the Hospitalist Unit, and were followed until they were discharged alive from the hospital.
There were no published data on which to base firm a priori sample size calculations. Based on pilot data, we estimated that a sample size of about 170 would yield precise estimates for means and standard deviations, giving us 80% to 90% power to determine differences in time intervals across categories, with alpha set to 0.05. We estimated that we would need 4 months of data collection to achieve this sample size.
During the period of study, the 16‐bed unit was staffed with in‐house hospitalist attending physicians without house‐staff, from 7 AM (weekdays) or 8 AM (weekends) to 10 PM (Monday to Thursday) or 8 PM (Friday to Sunday). The hospitalist unit had 24‐hour physician coverage, but attending physicians provided overnight coverage from home (backed up by in‐house residents for patient care emergencies). Handoffs of patient care from one attending physician to another typically occurred on Friday afternoon or Monday morning. The unit had 1 dedicated social worker and a nurse clinician who provided part‐time assistance with discharge planning.
Outcome Measurements
We defined the start of the discharge process as the time the patient's last medically necessary test was needed by his or her attending physician. Specifically, physicians were asked when the results of this test first would have been useful in clearing the patient for discharge. In the remainder of this work, we will refer to the start of the discharge process as time decisive test needed.
The end of the discharge process was called the discharge time, and defined as the time the unit clerk saw the patient leave the unit. We defined early discharges as those occurring before the median hour of discharge (3:00 PM), and late discharges as those occurring at or after this hour.
A focus group composed of nurses, physicians, social worker, unit clerks, and support associates (group responsible for cleaning patient rooms) volunteered to map out the discharge process. Based on these discussions, durations in the discharge process were defined as follows: (1) duration 1: time decisive test needed, until time the attending physician was aware of test results; (2) duration 2: time the physician was aware of test results until discharge paperwork was complete; (3) duration 3: time discharge paperwork complete until patient leaves unit; and (4) total discharge duration: time decisive test needed until patient leaves unit.
Exposure Measurements
We categorized exposures into 5 groups: (1) demographics (age, gender, race, source of patient such as outside hospital versus emergency department versus other, and payer on discharge); (2) clinical characteristics (length of stay, any psychiatric diagnosis, any substance abuse diagnosis, and severity of illness); (3) system characteristics (departmental occupancy defined as proportion of hospital beds designated for Department of Medicine patients that were occupied on the day of discharge); (4) last test characteristics (physical exam, laboratory test, procedure, and consult); and (5) discharge characteristics (discharged to home versus not discharged to home, prescriptions filled in hospital pharmacy prior to discharge, and ambulance required for transport).
Psychiatric diagnosis was defined as the presence of any of the following International Classification of Diseases, Ninth Revision, Clinical Modification (ICD‐9‐CM) codes: 290319 (any fourth or fifth digits).27 Substance abuse diagnosis was defined as the presence of any of the following ICD‐9‐CM codes: 303305 (any fourth or fifth digits). Substance abuse codes encompassed drug dependence and abuse, including alcohol dependence and abuse.
The all patient refined diagnosis related group relative weight (APRDRGwt) is a unitless number that estimates the total cost of care for inpatients, based on clinical and demographic characteristics.28 A patient with a relative APRDRGwt of 1 is predicted to have the same cost of care as the national average for inpatients. A patient with a score of 2 is predicted to be twice as costly as the average. In this study, we used APRDRGwt as a gross proxy for severity of illness.
Adjusted length of stay was measured as length of stay minus discharge duration. This adjustment was made to avoid including the exposure (length of stay) in the outcome (discharge duration). Unadjusted length of stay was used when the outcome was discharge time.
Data Sources
We created a separate 4‐item to 9‐item paper questionnaire (included in the Appendix) for each of 4 functional groups participating directly in the discharge process: nurses, physicians, social worker, and unit clerks. Questions were based on staff feedback about the sequence of steps in the discharge process, and potential reasons for delay. The surveys were piloted for several weeks to further refine the wording of questions, and to ensure that the length and location of the surveys minimized workflow interruptions. The questionnaires captured information about the timing of routine events not recorded in existing databases.
Physicians were asked to identify the last test/procedure/consult needed prior to the patient being medically ready for discharge. They were asked when the test results first could have cleared the patient for discharge (time decisive test needed), and when they actually received the test results (time test results back). Nursing and social work surveys provided information on whether or not prescriptions were filled prior to discharge, and the type of transportation used on discharge. Unit clerks documented when the patient left the unit.
Response rates were: nurses (97%), physicians (97%), social worker (99%), and unit clerks (94%). All 4 surveys were completed for 88% of the 209 included patients (prior to 8 exclusions for missing data or extreme outlier observations). Group response rates were tallied at the end of each month and posted on the unit. We did not track how soon after discharge the surveys were completed. However, we reviewed survey responses frequently (often daily, at most every 4 days) and if surveys were incomplete we personally approached staff members to complete the survey.
We supplemented and cross‐checked data from the questionnaire with information from existing hospital databases. These databases were: (1) the patient's medical record for time patient arrived on the floor, and completion time for consults/procedures; (2) the Electronic Bedboard (EBB) for time patient left the unit (as recorded by unit clerk); (3) the Patient Order Entry System for time discharge papers were completed by the physician, and ordering time for select tests; (4) the Electronic Patient Record for demographic information and completion time for select tests; and (5) Datamart, the hospital's administrative/billing database, for information such as length of stay, diagnosis, patient demographics, and insurance status.
Cross‐checking of data and calculation of durations 1, 2, and 3 identified areas of disagreement that were addressed in the following way. Discharge time was provided by 3 sources: social worker and nurses as an ad hoc addition to each of their surveys, unit clerks as a mandatory question on their survey, and unit clerks as entered in the EBB. We used EBB data for discharge time, as this was the most complete and accurate single source of data. However, survey results and knowledge about the sequential process for discharge, suggested that in 20 cases EBB data did not provide the most accurate time. In these cases, discharge time was provided by the unit clerk survey (16 cases), the social work survey (3 cases), and the nursing survey (1 case).
In 28 cases (14%), discharge paperwork was completed before decisive test results were back. And in 8 cases (4%) test results were received earlier than needed. As these were a minority of cases, these negative durations were converted to zero for analysis.
Statistical Analysis
The unit of analysis was the unique hospital discharge. For patients who were discharged from the Hospitalist Unit more than once during the 4‐month study period, each discharge was treated as a separate unit of analysis.
We defined patients discharged before the median discharge time as early discharges, and all others as late discharges. We then categorized patients with discharge durations less than 24 hours as short discharges, and all others as long discharges.
We described the characteristics of 2 groups of patients: early and short discharges versus all others. We used the chi square statistic and Fisher's exact test (when frequency 5 in 1 or both groups) to test the null hypothesis that there was no association between the 2 groups and select patient characteristics. When comparing medians, we used the nonparametric equality of medians test.
For each step in the discharge process, we identified a median time of occurrence. For the first point in the processtime decisive test neededwe also used 1‐way analysis of variance and the F‐test to assess whether or not timing varied significantly by physician.
Because our primary goal was to quantify in hours the association between various factors and discharge time or duration, we used bivariate linear regression models to identify factors associated with time of discharge (primary analysis) and total duration of the discharge process (secondary analysis). We then used multivariate linear regression to identify factors associated with both outcomes. We used forward and backward selection methods to choose the final models for the multivariate analyses, after forcing in the variables for race, sex, and age. Both methods of selection produced identical results. We assessed for colinearity using variance inflation factors.29
Sensitivity Analyses
For both discharge time and discharge duration, we performed regression diagnostics including leverage, Studentized residuals, and influence. Excluding outliers for influence slightly altered the results of our multivariate analyses. However, all variables that were significant at the P < 0.05 level remained significant in the models without outliers.
We chose to include outliers for influence in our final data set after verifying the data as accurate. For discharge time, the number of outliers (3; 1.5%) for influence was in the range expected for a normally distributed data set.
We also tested for normality of the 2 outcome variables. Discharge time was normally distributed, but discharge duration was not. Because of this, we used 2 additional methods to assess the robustness of our results for discharge duration.
First, we log‐transformed the outcome and repeated the analysis. Variables significant in the non‐log‐transformed model remained significant after log‐transformation. Second, we applied bootstrapping30 with 1,000 repetitions for the bivariate and multivariate analyses. The 95% confidence intervals (CIs) (using the bias‐corrected confidence intervals) were modestly altered (some narrowed, some widened), but our conclusions remained the same except for a single variable with borderline significance (payer on discharge) in bivariate analysis. The final reported confidence intervals for discharge duration are based on our analysis without bootstrapping.
Results
Data were collected on 216 patients. Seven patients were excluded from the study, because they were discharged against medical advice. Since these patients left before their decisive test was completed, there was no way to assess duration of the discharge process. Of the remaining 209 patients, 6 patients lacked necessary data to complete analysis (5 without survey data; 1 without administrative data). Two additional patients were eliminated from the final analysis because they bypassed the normal discharge process and were extreme outliers in either discharge time (1 discharged at 1 AM), or discharge duration (1 with discharge duration of 400+ hours). A total of 201 patients were included in the final analyses.
The hospitalist program primarily serves an indigent, local adult population with general medical problems, and this is reflected in the patient characteristics (Table 1). We compared the characteristics of patients discharged early and quickly (discharged prior to median hour of 3:00 PM, and discharge process lasting less than 24 hours) to all other discharges, to identify factors associated with later and longer discharges.
Early and Short Discharges (n = 75)* | All Other Discharges (n = 126) | P Value | |
---|---|---|---|
| |||
Demographics | |||
Age (years) | |||
Median | 55 | 55 | 0.73 |
Range | (19, 94) | (20, 90) | |
Gender (%) | |||
Female | 61.3 | 61.1 | 0.98 |
Race (%) | 0.08 | ||
African American | 76.0 | 60.3 | |
Caucasian | 21.3 | 34.9 | |
Other | 2.7 | 4.8 | |
Payor on discharge (%) | 0.29 | ||
Medicaid | 32.0 | 20.6 | |
Medicare | 32.0 | 38.9 | |
Self‐pay | 12.0 | 16.7 | |
Other | 24.0 | 23.8 | |
Clinical characteristics | |||
Adjusted length of stay (days) | |||
Median | 3 | 3 | 0.19 |
Range | (<1, 20) | (<1, 138) | |
Substance abuse (%) | 41.3 | 37.3 | 0.57 |
Psychiatric diagnosis (%) | 20.0 | 25.4 | 0.38 |
Last test characteristics | |||
Test type (%) | <0.001 | ||
Exam | 42.7 | 26.2 | |
Laboratory test | 38.7 | 13.5 | |
Procedure | 10.7 | 35.7 | |
Consult | 8.0 | 24.6 | |
Discharge characteristics | |||
Discharged to home (%) | 93.3 | 71.4 | <0.001 |
Prescriptions filled prior to discharge (%) | 10.7 | 19.8 | 0.09 |
Ambulance required for transport (%) | 8.0 | 21.4 | 0.01 |
Overall, 81% of patients were admitted from the ED, and 40% of all patients were insured by Medicaid or were self‐pay at time of discharge. Median expected charges were similar to the national average, as demonstrated by the median APRDRGwt of 1.0. Patients stayed an average of 6 days (median = 3 days). Patients with the longest adjusted lengths of stay (>20 days) were never early and short discharges. The most common discharge diagnoses were: congestive heart failure, chest pain or myocardial infarction, pneumonia, asthma/chronic obstructive pulmonary disease, and sickle‐cell disease. Thirty‐nine percent of all patients carried the diagnosis of alcohol or drug dependence or abuse, although for most this was not their discharge diagnosis. None of these demographic or clinical factors were associated with a late or long discharge.
The types of tests patients required on discharge were categorized into 4 groups: consults (18.4%), laboratory tests (22.9%), procedures (26.4%), and physical exam (32.3%). Distribution differed significantly between early and short discharges, and all other discharges (P < 0.001). Procedures and consults were less frequent among early and short discharges (procedures: 10.7% versus 35.7%; consults: 8.0% versus 24.6%).
For all patients, there was fragmentation within the consult and procedure categories. Within the consult group, there were 12 different types of consults ordered, with the dominant category (35.1%) being other. The next highest volume consult was physical/occupational therapy (27.0%). Within the procedure group, there were 11 different types of procedures, with the most common being stress echocardiograms (28.3%). Non‐MRI radiology procedures made up the next largest category (20.8%) and the third was other (18.9%).
Many patients had immediate postdischarge needs, as demonstrated by the 20% of patients not discharged home. The majority (66%) of those who were discharged to a facility required an ambulance. Early and short discharges were less likely to use an ambulance to leave the hospital (8.0% versus 21.4%; P = 0.01), and more likely to be discharged directly to home (99.3% versus 71.4%; P < 0.001).
Based on process mapping, we defined a 4‐step sequential discharge process for all patients (Figure 1). The first step was: decisive test needed by physician to discharge patient. Subgroup analysis demonstrated no significant difference in the timing of this step by individual physician (P = 0.44). The remaining 3 steps were as follows: physician aware of test results, discharge paperwork complete by physician, and patient leaves the unit. Each of the 4 steps showed wide variability in hour of occurrence.

Total discharge duration showed even more variability than the time of day when steps were likely to occur (Figure 2). Median duration of the discharge process was 7.6 hours (25th% to 75th%: 4 to 22 hours). Median duration from decisive test needed until resulted (duration 1) was 2 hours (25th% to 75th%: 0 to 8 hours); between test resulted until discharge paperwork complete (duration 2) was 1.4 hours (25th% to 75th%: 0.3 to 4.2 hours); and between discharge paperwork complete and patient leaving the unit (duration 3) was 2.0 hours (25th% to 75th%: 1.1 to 3.1 hours). All durations were skewed to the right, with durations 1 and 2 each taking at least 24 hours to occur in 10% of patients.
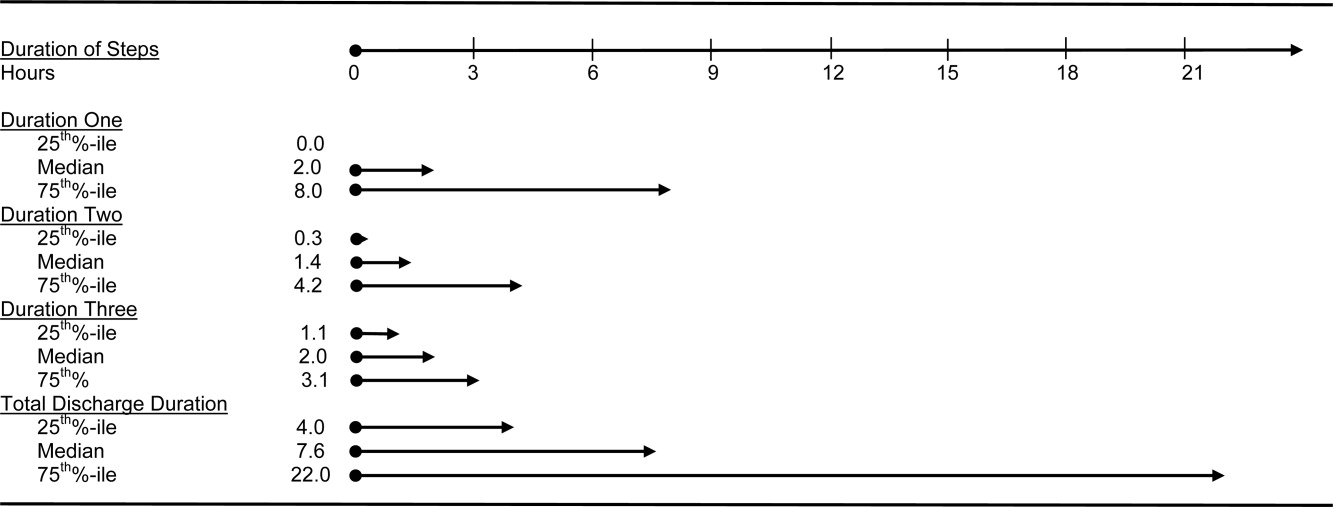
The final multivariate model for time of discharge contained 6 covariates: age, sex, race, test type, prescriptions filled prior to discharge, and need for an ambulance on discharge (Table 2). Special discharge needs continued to be associated with later discharges. Those patients who required an ambulance for transport had mean discharge times that were later by 1.5 hours (95% CI, 0.42.5). If staff obtained discharge medications for patients, these patients left 1.4 hours later than those patients who filled their prescriptions on their own (95% CI, 0.32.4). Patients requiring a consult or procedure also had significantly later discharges (1.2 hours for consults, 95% CI, 0.12.4; 1.1 hours for procedures, 95% CI, 0.12.1) than those needing a bedside exam. Age, sex, and race remained insignificant at the P 0.05 level in the final multivariate model. Length of stay was significantly associated with discharge time in crude analysis, but this variable dropped out of the final multivariate model.
Adjusted Coefficient, Discharge Time as Outcome in Hours (95% CI)* | P Value | Adjusted Coefficient, Discharge Duration as Outcome in Hours (95% CI) | P Value | |
---|---|---|---|---|
| ||||
Demographics | ||||
Age in quartiles (years) | ||||
7294 | 0.5 (0.5, 1.6) | 0.33 | 0.6 (8.3, 7.2) | 0.88 |
5671 | 0.5 (0.6, 1.6) | 0.41 | 1.3 (9.2, 6.6) | 0.75 |
4455 | 0.2 (0.9, 1.3) | 0.74 | 1.2 (9.0, 6.5) | 0.76 |
Male gender | 0.0 (0.8, 0.8) | 0.97 | 1.2 (6.8, 4.5) | 0.69 |
African American race | 0.1 (0.7, 0.9) | 0.80 | 0.3 (5.6, 6.1) | 0.93 |
Last test characteristics | ||||
Test type | ||||
Consult | 1.2 (0.1, 2.4) | 0.04 | 14.8 (6.5, 23.1) | 0.001 |
Procedure | 1.1 (0.1, 2.1) | 0.03 | 13.4 (6.0, 20.7) | <0.001 |
Laboratory test | 0.8 (1.8, 0.3) | 0.14 | 0.9 (8.4, 6.6) | 0.82 |
Exam (reference) | ||||
Discharge needs | ||||
Prescriptions filled prior to discharge | 1.4 (0.3, 2.4) | 0.02 | ||
Not discharged to home | 28.9 (21.9, 35.9) | <0.001 | ||
Ambulance required for transport | 1.5 (0.4, 2.5) | 0.007 |
We used duration of discharge as a secondary outcome measure. The final multivariate model for discharge duration included: age, sex, race, test type, and discharge to a facility (Table 2). Those who went to a facility on average left 28.9 hours (95% CI, 21.935.9) later than those who went home. Test type continued to show a significant association with discharge duration, although the estimates were slightly lower in the adjusted model. Need for a consult was associated with a discharge that was on average 14.8 hours (95% CI, 6.523.1) longer than discharges contingent on a physical exam. Similarly, those patients who had procedures had discharges that were on average 13.4 hours (95% CI, 6.020.7) longer than those whose last test was an exam. Several factors that were significantly associated with discharge duration in unadjusted analyses dropped out of the final multivariate model. These included: need for an ambulance, length of stay, insurance status, and medical complexity as estimated by APRDRGwt.
Conclusions
We found that discharge time and duration had wide variability and that certain factors were associated with only one outcome variabledischarge time or duration. Two factorsneed for an ambulance and filling of prescriptions prior to dischargewere associated with later hour of discharge. Discharge to a location other than home was associated with prolonged discharge duration. Test characteristicsin particular need for a procedure and consultwere significantly associated with both longer and later discharges.
In bivariate analysis, several factors were not associated with discharge time or duration. These were: African‐American race, sex, age, Department of Medicine occupancy on day of discharge, source of admission, psychiatric comorbidity, and substance abuse comorbidity. We had expected higher occupancy to delay discharge as demand exceeded capacity for tests, consults, etc. Our findings suggest that even though our study was conducted during the winter months when hospital occupancy is typically at its peak, supply of staff was still adequate enough to meet high demand. We had also expected that psychiatric and substance abuse comorbidities would prolong discharge as prior studies have found some of these diagnoses to be associated with longer lengths of stay.3134 However, our results do not support such an association, and may reflect our decision to group all psychiatric diagnoses together due to limited sample size.
The main strength of our study is the use of 2 outcome variablestime and durationto define delayed discharges. Our findings demonstrate that few factors are associated with both later and longer discharges. In an era when avoiding emergency room walkouts through early morning hospital discharges can be as important as managing overall length of stay, identifying factors associated with both duration and timing of discharges addresses actual challenges faced by hospitals with limited resources. Prior studies have rarely addressed both outcomes. An additional strength of our study is our use of an interdisciplinary survey. The discharge process is a key component of the inpatient stay, but it is also one for which no group is entirely responsible. Through the development and administration of an interdisciplinary survey, our study adds detail to existing descriptions of this fragmented process, and identifies potential areas for improvement.
Several limitations of our study deserve comment. First, we examined patients discharged from a hospitalist unit without house‐staff at an urban tertiary care hospital. Our findings may need additional interpretation prior to their application in dissimilar settings such as: (1) resident‐covered units in which workflow is shaped by teaching rounds, and (2) nonacademic hospitals in which incentives to provide rapid consults and procedures may be different. Second, we relied on self‐reporting for certain variables such as time decisive test needed. This may be subject to recall bias, as we did not have staff to independently verify recalled times. However, since the discharge process is generally a linear one, we were able to verify the general scope of recalled times with times date‐stamped by the computer during the discharge process (eg, checking that time decisive test needed did not occur after the discharge worksheet had been finalized in the electronic order‐entry system). Third, our sample size was not large enough to control for disease‐specific quality measures. Of note, prior studies have not identified a consistently positive or negative relationship between quality of care and efficiency.3538
Past work has used administrative and survey data to analyze the effect of discharge planning interventions on financial or quality outcomes. Outcomes have included readmissions, mortality, patient satisfaction, length of stay, and inappropriate bed days.22, 3845 However, as capacity management has become a more pressing issue for hospitals, greater focus is being placed on the mismatch between supply and demand of patients at each hour of the day. The relevant unit of measure for efficient discharges has become hour of day, in addition to total length of stay. Some hospital improvement projects have already addressed this shift in thinking.20, 21 Our study adds to this work by formally describing the precise timing and duration of steps in the discharge process, and identifying factors associated with both time and duration.
We believe the results of our study have several implications for hospital administrators and patients interested in more timely care. First, the methods used provide a tangible framework for addressing problems that cross disciplines (eg, nursing, physician, social work) and departments (eg, medicine and radiology), and have a multitude of potential causes and confounders. The survey results offer guidance on where to focus resources, provide a shared baseline metric for improvement, and suggest the cross‐functional team that should be involved in change efforts. Such an approach may be useful for addressing common system‐based challenges in inpatient quality and safety.
Second, with specific regard to discharge planning, our study supports the notion that modifiable factors are associated with discharge time and duration. However, we also describe a fragmented discharge process, with no single bottleneck or department responsible for the majority of late and long discharges. Although procedures and consults were both associated with longer and later discharges, only 26% of patients required a procedure prior to discharge, and 18% required a consult. Moreover, among procedures, different people and events are needed to carry out the 2 most popular procedures: stress echocardiograms, and non‐MRI radiology procedures. Hospital leadership at the highest levels will be required to improve efficiency based on local usage patterns, and to increase coordination among the multiple interdepartmental processes that make up the more general categories of procedure and consult.
Acknowledgements
The authors thank the patients and staff of the Hospitalist Unit for their participation in this study. In addition, they sincerely thank Paul X. Allen, Richard O. Davis, Ronald R. Peterson, and Shuai Shao. They also thank the anonymous reviewers for their thoughtful comments.
- Emergency departments and crowding in United States teaching hospitals.Ann Emerg Med.1991;20:980–986. , , , , .
- A conceptual model of emergency department crowding.Ann Emerg Med.2003;42:173–180. , , , , , .
- Frequent overcrowding in U.S. emergency departments.Acad Emerg Med.2001;8:151–155. , , .
- The effect of hospital occupancy on emergency department length of stay and patient disposition.Acad Emerg Med.2003;10:127–133. , , , , .
- Calculus, chaos, and other models of emergency department crowding.Ann Emerg Med.2003;42:181–184. .
- Increased health care costs associated with ED overcrowding.Am J Emerg Med.1994;12:265–266. , .
- Decreased health care quality associated with emergency department overcrowding.Eur J Emerg Med.1999;6:105–107. , , , et al.
- Can good bed management solve the overcrowding in accident and emergency departments?Emerg Med J.2003;20:149–155. , , .
- Emergency department overcrowding in the United States: an emerging threat to patient safety and public health.Emerg Med J.2003;20:402–405. , .
- General Accounting Office. Hospital emergency departments: crowded conditions vary among hospitals and communities. 2003:GAO‐03–460. Available at: http://www.gao.gov/new.items/d03460.pdf. Accessed October.
- Do you want to die?Time.1990;135:58–60+ , .
- The ER crisis.Ladies Home J.2001;118:70–79. .
- Placing emergency department crowding on the decision agenda.Nurs Econ.2005;23:14–24. .
- Crisis seen in nation's ER care. Washington Post. June 15,2006;A01. .
- Committee on the Future of Emergency Care in the United States Health System.Hospital‐based Emergency Care: at the Breaking Point.Washington DC:National Academies Press;2006.
- Waits to see an emergency department physician: U.S. trends and predictors, 1997–2004.Health Aff.2008;27:w84–w95. , , , et al.
- Advisory Board.Maximizing Hospital Capacity: Expediting Throughput in an Era of Shortage. Health Care Advisory Board research study.Washington DC:Advisory Board; September 12,2002.
- Does U.S. hospital capacity need to be expanded?Health Aff.2003;22:40–54. , , , .
- Institute for Healthcare Improvement.Optimizing Patient Flow: Moving Patients Smoothly Through Acute Care Settings.Cambridge, MA:Institute for Healthcare Improvement;2003.
- http://healthcare.isixsigma.com/library/content/c030708a.asp. Accessed October2008. , . Leveraging six sigma to improve hospital bed availability. Available at:
- University Health System Consortium. Managing patient flow implementation: 2005 benchmarking project field brief. Available at: http://www.musc.edu/medcenter/UHC‐CCP/PFMgmtFldBk.pdf.
- Discharge planning from hospital to home.Cochrane Database Syst Rev.2004;1:CD000313. , , , .
- Predictors of inappropriate hospital days in a department of internal medicine.Int J Epidemiol.1998;27:513–519. , , , et al.
- Utilisation of hospital beds by the elderly—a cohort study of admissions to a teaching hospital.Ir Med J.1995;88:124–126. , , .
- Demographic and clinical characteristics as predictors of length of hospitalization and readmission.J Clin Psychol.1977;33:1093–1099. , , , , , .
- The epidemiology of delays in a teaching hospital: the development and use of a tool that detects unnecessary hospital days.Med Care.1989;27:112–129. , , , .
- American Medical Association.AMA Physician ICD‐9‐CM 2004, Vols 1 and 2.Eden Prairie, MN:Ingenix;2003.
- 3M Health Information Systems and the Maryland Health Care Payment System: frequently asked questions.2004. Available at: http://multimedia.mmm.com/mws/mediawebserver.dyn?6666660Zjcf6lVs6EVs66S7wwCOrrrrQ‐.
- Statistics with STATA.Belmont, CA:Duxbury Press;2004. .
- Statistical data analysis in the computer age.Science.1991;253:390–395. , .
- Psychological comorbidity and length of stay in the general hospital.Am J Psychiatry.1991;148(3):324–329. , , , , .
- Psychiatric comorbidity and greater hospitalization risk, longer length of stay, and higher hospitalization costs in older adults with heart failure.J Am Geriatr Soc.2007;55:1585–1591. , , , , , .
- Does psychiatric comorbidity increase the length of stay in general hospitals?Gen Hosp Psychiatry.2001;23:8–14. , , , .
- The impact of psychiatric comorbidity on length of stay of medical inpatients.Gen Hosp Psychiatry.2003;25:14–19. , , .
- The implications of regional variations in Medicare spending. Part 1: The content, quality, and accessibility of care.Ann Intern Med.2003;138:273–287. , , , , , .
- The implications of regional variations in Medicare spending. Part 2: Health outcomes and satisfaction with care.Ann Intern Med.2003;138:288–298. , , , , , .
- Is early too early? Effect of shorter stays after bypass surgery.Ann Thorac Surg.2007;83:100–107. , , , et al.
- Excess length of stay, charges, and mortality attributable to medical injuries during hospitalization.JAMA.2003;290:1868–1874. , .
- Comprehensive discharge planning and home follow‐up of hospitalized elders, a randomized clinical trial.JAMA.1999;281:613–620. , , , et al.
- Comprehensive discharge planning for the hospitalized elderly, a randomized clinical trial.Ann Intern Med.1994;120:999–1006. , , , , , .
- Evaluating hospital discharge planning: a randomized clinical trial.Med Care.1993;31:358–370. , .
- Does a dedicated discharge coordinator improve the quality of hospital discharge?Qual Health Care.1996;5:89–96. , , , , .
- The effect of a hospitalist service with nurse discharge planner on patient care in an academic teaching hospital.Am J Med.2001;111:627–632. , , , et al.
- Comprehensive discharge planning with postdischarge support for older patients with congestive heart failure: a meta‐analysis.JAMA.2004;291:1358–1367. , , , , , .
- Does increased access to primary care reduce hospital readmissions?N Engl J Med.1996;334:1441–1447. , , .
In the past 2 decades, emergency department (ED) overcrowding has become an issue large enough to receive coverage in the popular press, and to spawn research around its causes and effects.116 At the same time, nurses and physicians on the inpatient wards have been urged to shorten the length of stay for patients as health system leaders face an aging population but limited capital to build new beds or hire additional clinical staff. Capacity managementencompassing the flow of patients from ED triage to inpatient dischargehas become a shared concern of clinicians and hospital administrators alike.
How to achieve the goals of diagnosing and healing while ushering patients ever more quickly through the modern hospital is not yet entirely clear. Past research and work by business groups suggests that demand for inpatient beds starts early in the day, but discharges typically occur in the late afternoon.17 This creates a potential bottleneck in patient flow. Many hospitals have implemented measures to improve patient throughput.1821 However, formal research has focused on factors leading to an additional inpatient day.2226 We have found no peer‐reviewed publications that address the problem of same‐day delays by describing hour of day for each step in the discharge process and variables associated with late‐day discharges. To fill this gap, we conducted a prospective cohort study of 209 consecutive discharges from a general medical ward to: (1) describe the natural history of hospital discharge, (2) measure time of day and duration for each step, and (3) identify factors associated with discharges that occur later in the day. We hypothesized that time and duration of discharge would be associated with 5 factors: patient demographics and clinical characteristics, departmental occupancy, type of inpatient testing done immediately prior to discharge, and discharge characteristics such as discharge to a location other than home.
Patients and Methods
Setting
The setting was the Hospitalist Unit of a single teaching hospital in Baltimore (The Johns Hopkins Hospital) from January 1, 2005 to April 30, 2005. Patients entered the cohort upon initiation of the discharge process by the hospitalist team on the Hospitalist Unit, and were followed until they were discharged alive from the hospital.
There were no published data on which to base firm a priori sample size calculations. Based on pilot data, we estimated that a sample size of about 170 would yield precise estimates for means and standard deviations, giving us 80% to 90% power to determine differences in time intervals across categories, with alpha set to 0.05. We estimated that we would need 4 months of data collection to achieve this sample size.
During the period of study, the 16‐bed unit was staffed with in‐house hospitalist attending physicians without house‐staff, from 7 AM (weekdays) or 8 AM (weekends) to 10 PM (Monday to Thursday) or 8 PM (Friday to Sunday). The hospitalist unit had 24‐hour physician coverage, but attending physicians provided overnight coverage from home (backed up by in‐house residents for patient care emergencies). Handoffs of patient care from one attending physician to another typically occurred on Friday afternoon or Monday morning. The unit had 1 dedicated social worker and a nurse clinician who provided part‐time assistance with discharge planning.
Outcome Measurements
We defined the start of the discharge process as the time the patient's last medically necessary test was needed by his or her attending physician. Specifically, physicians were asked when the results of this test first would have been useful in clearing the patient for discharge. In the remainder of this work, we will refer to the start of the discharge process as time decisive test needed.
The end of the discharge process was called the discharge time, and defined as the time the unit clerk saw the patient leave the unit. We defined early discharges as those occurring before the median hour of discharge (3:00 PM), and late discharges as those occurring at or after this hour.
A focus group composed of nurses, physicians, social worker, unit clerks, and support associates (group responsible for cleaning patient rooms) volunteered to map out the discharge process. Based on these discussions, durations in the discharge process were defined as follows: (1) duration 1: time decisive test needed, until time the attending physician was aware of test results; (2) duration 2: time the physician was aware of test results until discharge paperwork was complete; (3) duration 3: time discharge paperwork complete until patient leaves unit; and (4) total discharge duration: time decisive test needed until patient leaves unit.
Exposure Measurements
We categorized exposures into 5 groups: (1) demographics (age, gender, race, source of patient such as outside hospital versus emergency department versus other, and payer on discharge); (2) clinical characteristics (length of stay, any psychiatric diagnosis, any substance abuse diagnosis, and severity of illness); (3) system characteristics (departmental occupancy defined as proportion of hospital beds designated for Department of Medicine patients that were occupied on the day of discharge); (4) last test characteristics (physical exam, laboratory test, procedure, and consult); and (5) discharge characteristics (discharged to home versus not discharged to home, prescriptions filled in hospital pharmacy prior to discharge, and ambulance required for transport).
Psychiatric diagnosis was defined as the presence of any of the following International Classification of Diseases, Ninth Revision, Clinical Modification (ICD‐9‐CM) codes: 290319 (any fourth or fifth digits).27 Substance abuse diagnosis was defined as the presence of any of the following ICD‐9‐CM codes: 303305 (any fourth or fifth digits). Substance abuse codes encompassed drug dependence and abuse, including alcohol dependence and abuse.
The all patient refined diagnosis related group relative weight (APRDRGwt) is a unitless number that estimates the total cost of care for inpatients, based on clinical and demographic characteristics.28 A patient with a relative APRDRGwt of 1 is predicted to have the same cost of care as the national average for inpatients. A patient with a score of 2 is predicted to be twice as costly as the average. In this study, we used APRDRGwt as a gross proxy for severity of illness.
Adjusted length of stay was measured as length of stay minus discharge duration. This adjustment was made to avoid including the exposure (length of stay) in the outcome (discharge duration). Unadjusted length of stay was used when the outcome was discharge time.
Data Sources
We created a separate 4‐item to 9‐item paper questionnaire (included in the Appendix) for each of 4 functional groups participating directly in the discharge process: nurses, physicians, social worker, and unit clerks. Questions were based on staff feedback about the sequence of steps in the discharge process, and potential reasons for delay. The surveys were piloted for several weeks to further refine the wording of questions, and to ensure that the length and location of the surveys minimized workflow interruptions. The questionnaires captured information about the timing of routine events not recorded in existing databases.
Physicians were asked to identify the last test/procedure/consult needed prior to the patient being medically ready for discharge. They were asked when the test results first could have cleared the patient for discharge (time decisive test needed), and when they actually received the test results (time test results back). Nursing and social work surveys provided information on whether or not prescriptions were filled prior to discharge, and the type of transportation used on discharge. Unit clerks documented when the patient left the unit.
Response rates were: nurses (97%), physicians (97%), social worker (99%), and unit clerks (94%). All 4 surveys were completed for 88% of the 209 included patients (prior to 8 exclusions for missing data or extreme outlier observations). Group response rates were tallied at the end of each month and posted on the unit. We did not track how soon after discharge the surveys were completed. However, we reviewed survey responses frequently (often daily, at most every 4 days) and if surveys were incomplete we personally approached staff members to complete the survey.
We supplemented and cross‐checked data from the questionnaire with information from existing hospital databases. These databases were: (1) the patient's medical record for time patient arrived on the floor, and completion time for consults/procedures; (2) the Electronic Bedboard (EBB) for time patient left the unit (as recorded by unit clerk); (3) the Patient Order Entry System for time discharge papers were completed by the physician, and ordering time for select tests; (4) the Electronic Patient Record for demographic information and completion time for select tests; and (5) Datamart, the hospital's administrative/billing database, for information such as length of stay, diagnosis, patient demographics, and insurance status.
Cross‐checking of data and calculation of durations 1, 2, and 3 identified areas of disagreement that were addressed in the following way. Discharge time was provided by 3 sources: social worker and nurses as an ad hoc addition to each of their surveys, unit clerks as a mandatory question on their survey, and unit clerks as entered in the EBB. We used EBB data for discharge time, as this was the most complete and accurate single source of data. However, survey results and knowledge about the sequential process for discharge, suggested that in 20 cases EBB data did not provide the most accurate time. In these cases, discharge time was provided by the unit clerk survey (16 cases), the social work survey (3 cases), and the nursing survey (1 case).
In 28 cases (14%), discharge paperwork was completed before decisive test results were back. And in 8 cases (4%) test results were received earlier than needed. As these were a minority of cases, these negative durations were converted to zero for analysis.
Statistical Analysis
The unit of analysis was the unique hospital discharge. For patients who were discharged from the Hospitalist Unit more than once during the 4‐month study period, each discharge was treated as a separate unit of analysis.
We defined patients discharged before the median discharge time as early discharges, and all others as late discharges. We then categorized patients with discharge durations less than 24 hours as short discharges, and all others as long discharges.
We described the characteristics of 2 groups of patients: early and short discharges versus all others. We used the chi square statistic and Fisher's exact test (when frequency 5 in 1 or both groups) to test the null hypothesis that there was no association between the 2 groups and select patient characteristics. When comparing medians, we used the nonparametric equality of medians test.
For each step in the discharge process, we identified a median time of occurrence. For the first point in the processtime decisive test neededwe also used 1‐way analysis of variance and the F‐test to assess whether or not timing varied significantly by physician.
Because our primary goal was to quantify in hours the association between various factors and discharge time or duration, we used bivariate linear regression models to identify factors associated with time of discharge (primary analysis) and total duration of the discharge process (secondary analysis). We then used multivariate linear regression to identify factors associated with both outcomes. We used forward and backward selection methods to choose the final models for the multivariate analyses, after forcing in the variables for race, sex, and age. Both methods of selection produced identical results. We assessed for colinearity using variance inflation factors.29
Sensitivity Analyses
For both discharge time and discharge duration, we performed regression diagnostics including leverage, Studentized residuals, and influence. Excluding outliers for influence slightly altered the results of our multivariate analyses. However, all variables that were significant at the P < 0.05 level remained significant in the models without outliers.
We chose to include outliers for influence in our final data set after verifying the data as accurate. For discharge time, the number of outliers (3; 1.5%) for influence was in the range expected for a normally distributed data set.
We also tested for normality of the 2 outcome variables. Discharge time was normally distributed, but discharge duration was not. Because of this, we used 2 additional methods to assess the robustness of our results for discharge duration.
First, we log‐transformed the outcome and repeated the analysis. Variables significant in the non‐log‐transformed model remained significant after log‐transformation. Second, we applied bootstrapping30 with 1,000 repetitions for the bivariate and multivariate analyses. The 95% confidence intervals (CIs) (using the bias‐corrected confidence intervals) were modestly altered (some narrowed, some widened), but our conclusions remained the same except for a single variable with borderline significance (payer on discharge) in bivariate analysis. The final reported confidence intervals for discharge duration are based on our analysis without bootstrapping.
Results
Data were collected on 216 patients. Seven patients were excluded from the study, because they were discharged against medical advice. Since these patients left before their decisive test was completed, there was no way to assess duration of the discharge process. Of the remaining 209 patients, 6 patients lacked necessary data to complete analysis (5 without survey data; 1 without administrative data). Two additional patients were eliminated from the final analysis because they bypassed the normal discharge process and were extreme outliers in either discharge time (1 discharged at 1 AM), or discharge duration (1 with discharge duration of 400+ hours). A total of 201 patients were included in the final analyses.
The hospitalist program primarily serves an indigent, local adult population with general medical problems, and this is reflected in the patient characteristics (Table 1). We compared the characteristics of patients discharged early and quickly (discharged prior to median hour of 3:00 PM, and discharge process lasting less than 24 hours) to all other discharges, to identify factors associated with later and longer discharges.
Early and Short Discharges (n = 75)* | All Other Discharges (n = 126) | P Value | |
---|---|---|---|
| |||
Demographics | |||
Age (years) | |||
Median | 55 | 55 | 0.73 |
Range | (19, 94) | (20, 90) | |
Gender (%) | |||
Female | 61.3 | 61.1 | 0.98 |
Race (%) | 0.08 | ||
African American | 76.0 | 60.3 | |
Caucasian | 21.3 | 34.9 | |
Other | 2.7 | 4.8 | |
Payor on discharge (%) | 0.29 | ||
Medicaid | 32.0 | 20.6 | |
Medicare | 32.0 | 38.9 | |
Self‐pay | 12.0 | 16.7 | |
Other | 24.0 | 23.8 | |
Clinical characteristics | |||
Adjusted length of stay (days) | |||
Median | 3 | 3 | 0.19 |
Range | (<1, 20) | (<1, 138) | |
Substance abuse (%) | 41.3 | 37.3 | 0.57 |
Psychiatric diagnosis (%) | 20.0 | 25.4 | 0.38 |
Last test characteristics | |||
Test type (%) | <0.001 | ||
Exam | 42.7 | 26.2 | |
Laboratory test | 38.7 | 13.5 | |
Procedure | 10.7 | 35.7 | |
Consult | 8.0 | 24.6 | |
Discharge characteristics | |||
Discharged to home (%) | 93.3 | 71.4 | <0.001 |
Prescriptions filled prior to discharge (%) | 10.7 | 19.8 | 0.09 |
Ambulance required for transport (%) | 8.0 | 21.4 | 0.01 |
Overall, 81% of patients were admitted from the ED, and 40% of all patients were insured by Medicaid or were self‐pay at time of discharge. Median expected charges were similar to the national average, as demonstrated by the median APRDRGwt of 1.0. Patients stayed an average of 6 days (median = 3 days). Patients with the longest adjusted lengths of stay (>20 days) were never early and short discharges. The most common discharge diagnoses were: congestive heart failure, chest pain or myocardial infarction, pneumonia, asthma/chronic obstructive pulmonary disease, and sickle‐cell disease. Thirty‐nine percent of all patients carried the diagnosis of alcohol or drug dependence or abuse, although for most this was not their discharge diagnosis. None of these demographic or clinical factors were associated with a late or long discharge.
The types of tests patients required on discharge were categorized into 4 groups: consults (18.4%), laboratory tests (22.9%), procedures (26.4%), and physical exam (32.3%). Distribution differed significantly between early and short discharges, and all other discharges (P < 0.001). Procedures and consults were less frequent among early and short discharges (procedures: 10.7% versus 35.7%; consults: 8.0% versus 24.6%).
For all patients, there was fragmentation within the consult and procedure categories. Within the consult group, there were 12 different types of consults ordered, with the dominant category (35.1%) being other. The next highest volume consult was physical/occupational therapy (27.0%). Within the procedure group, there were 11 different types of procedures, with the most common being stress echocardiograms (28.3%). Non‐MRI radiology procedures made up the next largest category (20.8%) and the third was other (18.9%).
Many patients had immediate postdischarge needs, as demonstrated by the 20% of patients not discharged home. The majority (66%) of those who were discharged to a facility required an ambulance. Early and short discharges were less likely to use an ambulance to leave the hospital (8.0% versus 21.4%; P = 0.01), and more likely to be discharged directly to home (99.3% versus 71.4%; P < 0.001).
Based on process mapping, we defined a 4‐step sequential discharge process for all patients (Figure 1). The first step was: decisive test needed by physician to discharge patient. Subgroup analysis demonstrated no significant difference in the timing of this step by individual physician (P = 0.44). The remaining 3 steps were as follows: physician aware of test results, discharge paperwork complete by physician, and patient leaves the unit. Each of the 4 steps showed wide variability in hour of occurrence.

Total discharge duration showed even more variability than the time of day when steps were likely to occur (Figure 2). Median duration of the discharge process was 7.6 hours (25th% to 75th%: 4 to 22 hours). Median duration from decisive test needed until resulted (duration 1) was 2 hours (25th% to 75th%: 0 to 8 hours); between test resulted until discharge paperwork complete (duration 2) was 1.4 hours (25th% to 75th%: 0.3 to 4.2 hours); and between discharge paperwork complete and patient leaving the unit (duration 3) was 2.0 hours (25th% to 75th%: 1.1 to 3.1 hours). All durations were skewed to the right, with durations 1 and 2 each taking at least 24 hours to occur in 10% of patients.
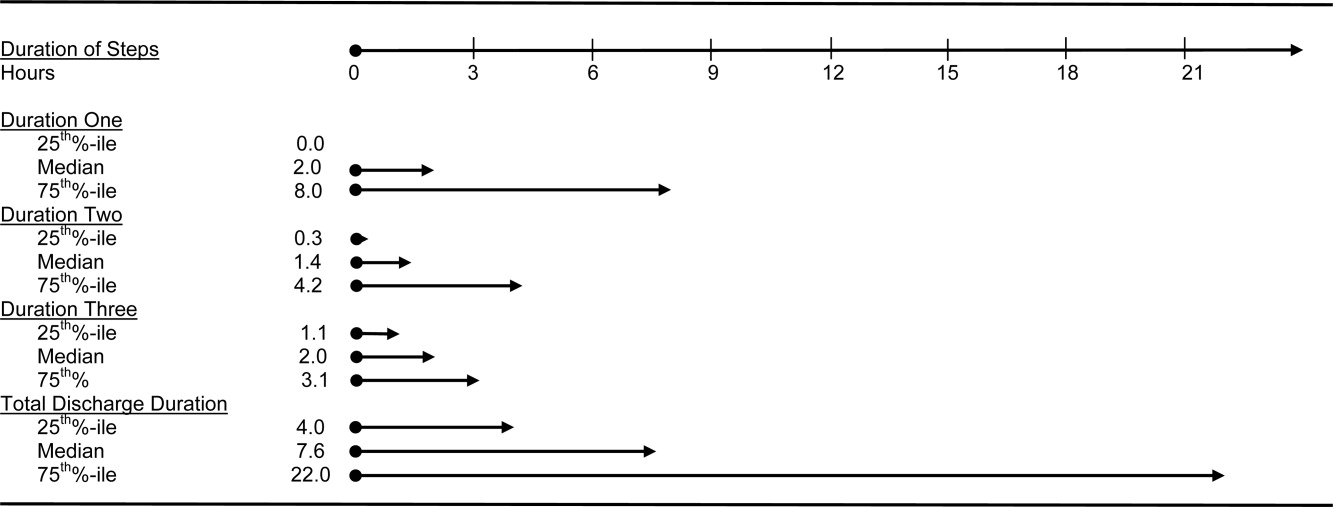
The final multivariate model for time of discharge contained 6 covariates: age, sex, race, test type, prescriptions filled prior to discharge, and need for an ambulance on discharge (Table 2). Special discharge needs continued to be associated with later discharges. Those patients who required an ambulance for transport had mean discharge times that were later by 1.5 hours (95% CI, 0.42.5). If staff obtained discharge medications for patients, these patients left 1.4 hours later than those patients who filled their prescriptions on their own (95% CI, 0.32.4). Patients requiring a consult or procedure also had significantly later discharges (1.2 hours for consults, 95% CI, 0.12.4; 1.1 hours for procedures, 95% CI, 0.12.1) than those needing a bedside exam. Age, sex, and race remained insignificant at the P 0.05 level in the final multivariate model. Length of stay was significantly associated with discharge time in crude analysis, but this variable dropped out of the final multivariate model.
Adjusted Coefficient, Discharge Time as Outcome in Hours (95% CI)* | P Value | Adjusted Coefficient, Discharge Duration as Outcome in Hours (95% CI) | P Value | |
---|---|---|---|---|
| ||||
Demographics | ||||
Age in quartiles (years) | ||||
7294 | 0.5 (0.5, 1.6) | 0.33 | 0.6 (8.3, 7.2) | 0.88 |
5671 | 0.5 (0.6, 1.6) | 0.41 | 1.3 (9.2, 6.6) | 0.75 |
4455 | 0.2 (0.9, 1.3) | 0.74 | 1.2 (9.0, 6.5) | 0.76 |
Male gender | 0.0 (0.8, 0.8) | 0.97 | 1.2 (6.8, 4.5) | 0.69 |
African American race | 0.1 (0.7, 0.9) | 0.80 | 0.3 (5.6, 6.1) | 0.93 |
Last test characteristics | ||||
Test type | ||||
Consult | 1.2 (0.1, 2.4) | 0.04 | 14.8 (6.5, 23.1) | 0.001 |
Procedure | 1.1 (0.1, 2.1) | 0.03 | 13.4 (6.0, 20.7) | <0.001 |
Laboratory test | 0.8 (1.8, 0.3) | 0.14 | 0.9 (8.4, 6.6) | 0.82 |
Exam (reference) | ||||
Discharge needs | ||||
Prescriptions filled prior to discharge | 1.4 (0.3, 2.4) | 0.02 | ||
Not discharged to home | 28.9 (21.9, 35.9) | <0.001 | ||
Ambulance required for transport | 1.5 (0.4, 2.5) | 0.007 |
We used duration of discharge as a secondary outcome measure. The final multivariate model for discharge duration included: age, sex, race, test type, and discharge to a facility (Table 2). Those who went to a facility on average left 28.9 hours (95% CI, 21.935.9) later than those who went home. Test type continued to show a significant association with discharge duration, although the estimates were slightly lower in the adjusted model. Need for a consult was associated with a discharge that was on average 14.8 hours (95% CI, 6.523.1) longer than discharges contingent on a physical exam. Similarly, those patients who had procedures had discharges that were on average 13.4 hours (95% CI, 6.020.7) longer than those whose last test was an exam. Several factors that were significantly associated with discharge duration in unadjusted analyses dropped out of the final multivariate model. These included: need for an ambulance, length of stay, insurance status, and medical complexity as estimated by APRDRGwt.
Conclusions
We found that discharge time and duration had wide variability and that certain factors were associated with only one outcome variabledischarge time or duration. Two factorsneed for an ambulance and filling of prescriptions prior to dischargewere associated with later hour of discharge. Discharge to a location other than home was associated with prolonged discharge duration. Test characteristicsin particular need for a procedure and consultwere significantly associated with both longer and later discharges.
In bivariate analysis, several factors were not associated with discharge time or duration. These were: African‐American race, sex, age, Department of Medicine occupancy on day of discharge, source of admission, psychiatric comorbidity, and substance abuse comorbidity. We had expected higher occupancy to delay discharge as demand exceeded capacity for tests, consults, etc. Our findings suggest that even though our study was conducted during the winter months when hospital occupancy is typically at its peak, supply of staff was still adequate enough to meet high demand. We had also expected that psychiatric and substance abuse comorbidities would prolong discharge as prior studies have found some of these diagnoses to be associated with longer lengths of stay.3134 However, our results do not support such an association, and may reflect our decision to group all psychiatric diagnoses together due to limited sample size.
The main strength of our study is the use of 2 outcome variablestime and durationto define delayed discharges. Our findings demonstrate that few factors are associated with both later and longer discharges. In an era when avoiding emergency room walkouts through early morning hospital discharges can be as important as managing overall length of stay, identifying factors associated with both duration and timing of discharges addresses actual challenges faced by hospitals with limited resources. Prior studies have rarely addressed both outcomes. An additional strength of our study is our use of an interdisciplinary survey. The discharge process is a key component of the inpatient stay, but it is also one for which no group is entirely responsible. Through the development and administration of an interdisciplinary survey, our study adds detail to existing descriptions of this fragmented process, and identifies potential areas for improvement.
Several limitations of our study deserve comment. First, we examined patients discharged from a hospitalist unit without house‐staff at an urban tertiary care hospital. Our findings may need additional interpretation prior to their application in dissimilar settings such as: (1) resident‐covered units in which workflow is shaped by teaching rounds, and (2) nonacademic hospitals in which incentives to provide rapid consults and procedures may be different. Second, we relied on self‐reporting for certain variables such as time decisive test needed. This may be subject to recall bias, as we did not have staff to independently verify recalled times. However, since the discharge process is generally a linear one, we were able to verify the general scope of recalled times with times date‐stamped by the computer during the discharge process (eg, checking that time decisive test needed did not occur after the discharge worksheet had been finalized in the electronic order‐entry system). Third, our sample size was not large enough to control for disease‐specific quality measures. Of note, prior studies have not identified a consistently positive or negative relationship between quality of care and efficiency.3538
Past work has used administrative and survey data to analyze the effect of discharge planning interventions on financial or quality outcomes. Outcomes have included readmissions, mortality, patient satisfaction, length of stay, and inappropriate bed days.22, 3845 However, as capacity management has become a more pressing issue for hospitals, greater focus is being placed on the mismatch between supply and demand of patients at each hour of the day. The relevant unit of measure for efficient discharges has become hour of day, in addition to total length of stay. Some hospital improvement projects have already addressed this shift in thinking.20, 21 Our study adds to this work by formally describing the precise timing and duration of steps in the discharge process, and identifying factors associated with both time and duration.
We believe the results of our study have several implications for hospital administrators and patients interested in more timely care. First, the methods used provide a tangible framework for addressing problems that cross disciplines (eg, nursing, physician, social work) and departments (eg, medicine and radiology), and have a multitude of potential causes and confounders. The survey results offer guidance on where to focus resources, provide a shared baseline metric for improvement, and suggest the cross‐functional team that should be involved in change efforts. Such an approach may be useful for addressing common system‐based challenges in inpatient quality and safety.
Second, with specific regard to discharge planning, our study supports the notion that modifiable factors are associated with discharge time and duration. However, we also describe a fragmented discharge process, with no single bottleneck or department responsible for the majority of late and long discharges. Although procedures and consults were both associated with longer and later discharges, only 26% of patients required a procedure prior to discharge, and 18% required a consult. Moreover, among procedures, different people and events are needed to carry out the 2 most popular procedures: stress echocardiograms, and non‐MRI radiology procedures. Hospital leadership at the highest levels will be required to improve efficiency based on local usage patterns, and to increase coordination among the multiple interdepartmental processes that make up the more general categories of procedure and consult.
Acknowledgements
The authors thank the patients and staff of the Hospitalist Unit for their participation in this study. In addition, they sincerely thank Paul X. Allen, Richard O. Davis, Ronald R. Peterson, and Shuai Shao. They also thank the anonymous reviewers for their thoughtful comments.
In the past 2 decades, emergency department (ED) overcrowding has become an issue large enough to receive coverage in the popular press, and to spawn research around its causes and effects.116 At the same time, nurses and physicians on the inpatient wards have been urged to shorten the length of stay for patients as health system leaders face an aging population but limited capital to build new beds or hire additional clinical staff. Capacity managementencompassing the flow of patients from ED triage to inpatient dischargehas become a shared concern of clinicians and hospital administrators alike.
How to achieve the goals of diagnosing and healing while ushering patients ever more quickly through the modern hospital is not yet entirely clear. Past research and work by business groups suggests that demand for inpatient beds starts early in the day, but discharges typically occur in the late afternoon.17 This creates a potential bottleneck in patient flow. Many hospitals have implemented measures to improve patient throughput.1821 However, formal research has focused on factors leading to an additional inpatient day.2226 We have found no peer‐reviewed publications that address the problem of same‐day delays by describing hour of day for each step in the discharge process and variables associated with late‐day discharges. To fill this gap, we conducted a prospective cohort study of 209 consecutive discharges from a general medical ward to: (1) describe the natural history of hospital discharge, (2) measure time of day and duration for each step, and (3) identify factors associated with discharges that occur later in the day. We hypothesized that time and duration of discharge would be associated with 5 factors: patient demographics and clinical characteristics, departmental occupancy, type of inpatient testing done immediately prior to discharge, and discharge characteristics such as discharge to a location other than home.
Patients and Methods
Setting
The setting was the Hospitalist Unit of a single teaching hospital in Baltimore (The Johns Hopkins Hospital) from January 1, 2005 to April 30, 2005. Patients entered the cohort upon initiation of the discharge process by the hospitalist team on the Hospitalist Unit, and were followed until they were discharged alive from the hospital.
There were no published data on which to base firm a priori sample size calculations. Based on pilot data, we estimated that a sample size of about 170 would yield precise estimates for means and standard deviations, giving us 80% to 90% power to determine differences in time intervals across categories, with alpha set to 0.05. We estimated that we would need 4 months of data collection to achieve this sample size.
During the period of study, the 16‐bed unit was staffed with in‐house hospitalist attending physicians without house‐staff, from 7 AM (weekdays) or 8 AM (weekends) to 10 PM (Monday to Thursday) or 8 PM (Friday to Sunday). The hospitalist unit had 24‐hour physician coverage, but attending physicians provided overnight coverage from home (backed up by in‐house residents for patient care emergencies). Handoffs of patient care from one attending physician to another typically occurred on Friday afternoon or Monday morning. The unit had 1 dedicated social worker and a nurse clinician who provided part‐time assistance with discharge planning.
Outcome Measurements
We defined the start of the discharge process as the time the patient's last medically necessary test was needed by his or her attending physician. Specifically, physicians were asked when the results of this test first would have been useful in clearing the patient for discharge. In the remainder of this work, we will refer to the start of the discharge process as time decisive test needed.
The end of the discharge process was called the discharge time, and defined as the time the unit clerk saw the patient leave the unit. We defined early discharges as those occurring before the median hour of discharge (3:00 PM), and late discharges as those occurring at or after this hour.
A focus group composed of nurses, physicians, social worker, unit clerks, and support associates (group responsible for cleaning patient rooms) volunteered to map out the discharge process. Based on these discussions, durations in the discharge process were defined as follows: (1) duration 1: time decisive test needed, until time the attending physician was aware of test results; (2) duration 2: time the physician was aware of test results until discharge paperwork was complete; (3) duration 3: time discharge paperwork complete until patient leaves unit; and (4) total discharge duration: time decisive test needed until patient leaves unit.
Exposure Measurements
We categorized exposures into 5 groups: (1) demographics (age, gender, race, source of patient such as outside hospital versus emergency department versus other, and payer on discharge); (2) clinical characteristics (length of stay, any psychiatric diagnosis, any substance abuse diagnosis, and severity of illness); (3) system characteristics (departmental occupancy defined as proportion of hospital beds designated for Department of Medicine patients that were occupied on the day of discharge); (4) last test characteristics (physical exam, laboratory test, procedure, and consult); and (5) discharge characteristics (discharged to home versus not discharged to home, prescriptions filled in hospital pharmacy prior to discharge, and ambulance required for transport).
Psychiatric diagnosis was defined as the presence of any of the following International Classification of Diseases, Ninth Revision, Clinical Modification (ICD‐9‐CM) codes: 290319 (any fourth or fifth digits).27 Substance abuse diagnosis was defined as the presence of any of the following ICD‐9‐CM codes: 303305 (any fourth or fifth digits). Substance abuse codes encompassed drug dependence and abuse, including alcohol dependence and abuse.
The all patient refined diagnosis related group relative weight (APRDRGwt) is a unitless number that estimates the total cost of care for inpatients, based on clinical and demographic characteristics.28 A patient with a relative APRDRGwt of 1 is predicted to have the same cost of care as the national average for inpatients. A patient with a score of 2 is predicted to be twice as costly as the average. In this study, we used APRDRGwt as a gross proxy for severity of illness.
Adjusted length of stay was measured as length of stay minus discharge duration. This adjustment was made to avoid including the exposure (length of stay) in the outcome (discharge duration). Unadjusted length of stay was used when the outcome was discharge time.
Data Sources
We created a separate 4‐item to 9‐item paper questionnaire (included in the Appendix) for each of 4 functional groups participating directly in the discharge process: nurses, physicians, social worker, and unit clerks. Questions were based on staff feedback about the sequence of steps in the discharge process, and potential reasons for delay. The surveys were piloted for several weeks to further refine the wording of questions, and to ensure that the length and location of the surveys minimized workflow interruptions. The questionnaires captured information about the timing of routine events not recorded in existing databases.
Physicians were asked to identify the last test/procedure/consult needed prior to the patient being medically ready for discharge. They were asked when the test results first could have cleared the patient for discharge (time decisive test needed), and when they actually received the test results (time test results back). Nursing and social work surveys provided information on whether or not prescriptions were filled prior to discharge, and the type of transportation used on discharge. Unit clerks documented when the patient left the unit.
Response rates were: nurses (97%), physicians (97%), social worker (99%), and unit clerks (94%). All 4 surveys were completed for 88% of the 209 included patients (prior to 8 exclusions for missing data or extreme outlier observations). Group response rates were tallied at the end of each month and posted on the unit. We did not track how soon after discharge the surveys were completed. However, we reviewed survey responses frequently (often daily, at most every 4 days) and if surveys were incomplete we personally approached staff members to complete the survey.
We supplemented and cross‐checked data from the questionnaire with information from existing hospital databases. These databases were: (1) the patient's medical record for time patient arrived on the floor, and completion time for consults/procedures; (2) the Electronic Bedboard (EBB) for time patient left the unit (as recorded by unit clerk); (3) the Patient Order Entry System for time discharge papers were completed by the physician, and ordering time for select tests; (4) the Electronic Patient Record for demographic information and completion time for select tests; and (5) Datamart, the hospital's administrative/billing database, for information such as length of stay, diagnosis, patient demographics, and insurance status.
Cross‐checking of data and calculation of durations 1, 2, and 3 identified areas of disagreement that were addressed in the following way. Discharge time was provided by 3 sources: social worker and nurses as an ad hoc addition to each of their surveys, unit clerks as a mandatory question on their survey, and unit clerks as entered in the EBB. We used EBB data for discharge time, as this was the most complete and accurate single source of data. However, survey results and knowledge about the sequential process for discharge, suggested that in 20 cases EBB data did not provide the most accurate time. In these cases, discharge time was provided by the unit clerk survey (16 cases), the social work survey (3 cases), and the nursing survey (1 case).
In 28 cases (14%), discharge paperwork was completed before decisive test results were back. And in 8 cases (4%) test results were received earlier than needed. As these were a minority of cases, these negative durations were converted to zero for analysis.
Statistical Analysis
The unit of analysis was the unique hospital discharge. For patients who were discharged from the Hospitalist Unit more than once during the 4‐month study period, each discharge was treated as a separate unit of analysis.
We defined patients discharged before the median discharge time as early discharges, and all others as late discharges. We then categorized patients with discharge durations less than 24 hours as short discharges, and all others as long discharges.
We described the characteristics of 2 groups of patients: early and short discharges versus all others. We used the chi square statistic and Fisher's exact test (when frequency 5 in 1 or both groups) to test the null hypothesis that there was no association between the 2 groups and select patient characteristics. When comparing medians, we used the nonparametric equality of medians test.
For each step in the discharge process, we identified a median time of occurrence. For the first point in the processtime decisive test neededwe also used 1‐way analysis of variance and the F‐test to assess whether or not timing varied significantly by physician.
Because our primary goal was to quantify in hours the association between various factors and discharge time or duration, we used bivariate linear regression models to identify factors associated with time of discharge (primary analysis) and total duration of the discharge process (secondary analysis). We then used multivariate linear regression to identify factors associated with both outcomes. We used forward and backward selection methods to choose the final models for the multivariate analyses, after forcing in the variables for race, sex, and age. Both methods of selection produced identical results. We assessed for colinearity using variance inflation factors.29
Sensitivity Analyses
For both discharge time and discharge duration, we performed regression diagnostics including leverage, Studentized residuals, and influence. Excluding outliers for influence slightly altered the results of our multivariate analyses. However, all variables that were significant at the P < 0.05 level remained significant in the models without outliers.
We chose to include outliers for influence in our final data set after verifying the data as accurate. For discharge time, the number of outliers (3; 1.5%) for influence was in the range expected for a normally distributed data set.
We also tested for normality of the 2 outcome variables. Discharge time was normally distributed, but discharge duration was not. Because of this, we used 2 additional methods to assess the robustness of our results for discharge duration.
First, we log‐transformed the outcome and repeated the analysis. Variables significant in the non‐log‐transformed model remained significant after log‐transformation. Second, we applied bootstrapping30 with 1,000 repetitions for the bivariate and multivariate analyses. The 95% confidence intervals (CIs) (using the bias‐corrected confidence intervals) were modestly altered (some narrowed, some widened), but our conclusions remained the same except for a single variable with borderline significance (payer on discharge) in bivariate analysis. The final reported confidence intervals for discharge duration are based on our analysis without bootstrapping.
Results
Data were collected on 216 patients. Seven patients were excluded from the study, because they were discharged against medical advice. Since these patients left before their decisive test was completed, there was no way to assess duration of the discharge process. Of the remaining 209 patients, 6 patients lacked necessary data to complete analysis (5 without survey data; 1 without administrative data). Two additional patients were eliminated from the final analysis because they bypassed the normal discharge process and were extreme outliers in either discharge time (1 discharged at 1 AM), or discharge duration (1 with discharge duration of 400+ hours). A total of 201 patients were included in the final analyses.
The hospitalist program primarily serves an indigent, local adult population with general medical problems, and this is reflected in the patient characteristics (Table 1). We compared the characteristics of patients discharged early and quickly (discharged prior to median hour of 3:00 PM, and discharge process lasting less than 24 hours) to all other discharges, to identify factors associated with later and longer discharges.
Early and Short Discharges (n = 75)* | All Other Discharges (n = 126) | P Value | |
---|---|---|---|
| |||
Demographics | |||
Age (years) | |||
Median | 55 | 55 | 0.73 |
Range | (19, 94) | (20, 90) | |
Gender (%) | |||
Female | 61.3 | 61.1 | 0.98 |
Race (%) | 0.08 | ||
African American | 76.0 | 60.3 | |
Caucasian | 21.3 | 34.9 | |
Other | 2.7 | 4.8 | |
Payor on discharge (%) | 0.29 | ||
Medicaid | 32.0 | 20.6 | |
Medicare | 32.0 | 38.9 | |
Self‐pay | 12.0 | 16.7 | |
Other | 24.0 | 23.8 | |
Clinical characteristics | |||
Adjusted length of stay (days) | |||
Median | 3 | 3 | 0.19 |
Range | (<1, 20) | (<1, 138) | |
Substance abuse (%) | 41.3 | 37.3 | 0.57 |
Psychiatric diagnosis (%) | 20.0 | 25.4 | 0.38 |
Last test characteristics | |||
Test type (%) | <0.001 | ||
Exam | 42.7 | 26.2 | |
Laboratory test | 38.7 | 13.5 | |
Procedure | 10.7 | 35.7 | |
Consult | 8.0 | 24.6 | |
Discharge characteristics | |||
Discharged to home (%) | 93.3 | 71.4 | <0.001 |
Prescriptions filled prior to discharge (%) | 10.7 | 19.8 | 0.09 |
Ambulance required for transport (%) | 8.0 | 21.4 | 0.01 |
Overall, 81% of patients were admitted from the ED, and 40% of all patients were insured by Medicaid or were self‐pay at time of discharge. Median expected charges were similar to the national average, as demonstrated by the median APRDRGwt of 1.0. Patients stayed an average of 6 days (median = 3 days). Patients with the longest adjusted lengths of stay (>20 days) were never early and short discharges. The most common discharge diagnoses were: congestive heart failure, chest pain or myocardial infarction, pneumonia, asthma/chronic obstructive pulmonary disease, and sickle‐cell disease. Thirty‐nine percent of all patients carried the diagnosis of alcohol or drug dependence or abuse, although for most this was not their discharge diagnosis. None of these demographic or clinical factors were associated with a late or long discharge.
The types of tests patients required on discharge were categorized into 4 groups: consults (18.4%), laboratory tests (22.9%), procedures (26.4%), and physical exam (32.3%). Distribution differed significantly between early and short discharges, and all other discharges (P < 0.001). Procedures and consults were less frequent among early and short discharges (procedures: 10.7% versus 35.7%; consults: 8.0% versus 24.6%).
For all patients, there was fragmentation within the consult and procedure categories. Within the consult group, there were 12 different types of consults ordered, with the dominant category (35.1%) being other. The next highest volume consult was physical/occupational therapy (27.0%). Within the procedure group, there were 11 different types of procedures, with the most common being stress echocardiograms (28.3%). Non‐MRI radiology procedures made up the next largest category (20.8%) and the third was other (18.9%).
Many patients had immediate postdischarge needs, as demonstrated by the 20% of patients not discharged home. The majority (66%) of those who were discharged to a facility required an ambulance. Early and short discharges were less likely to use an ambulance to leave the hospital (8.0% versus 21.4%; P = 0.01), and more likely to be discharged directly to home (99.3% versus 71.4%; P < 0.001).
Based on process mapping, we defined a 4‐step sequential discharge process for all patients (Figure 1). The first step was: decisive test needed by physician to discharge patient. Subgroup analysis demonstrated no significant difference in the timing of this step by individual physician (P = 0.44). The remaining 3 steps were as follows: physician aware of test results, discharge paperwork complete by physician, and patient leaves the unit. Each of the 4 steps showed wide variability in hour of occurrence.

Total discharge duration showed even more variability than the time of day when steps were likely to occur (Figure 2). Median duration of the discharge process was 7.6 hours (25th% to 75th%: 4 to 22 hours). Median duration from decisive test needed until resulted (duration 1) was 2 hours (25th% to 75th%: 0 to 8 hours); between test resulted until discharge paperwork complete (duration 2) was 1.4 hours (25th% to 75th%: 0.3 to 4.2 hours); and between discharge paperwork complete and patient leaving the unit (duration 3) was 2.0 hours (25th% to 75th%: 1.1 to 3.1 hours). All durations were skewed to the right, with durations 1 and 2 each taking at least 24 hours to occur in 10% of patients.
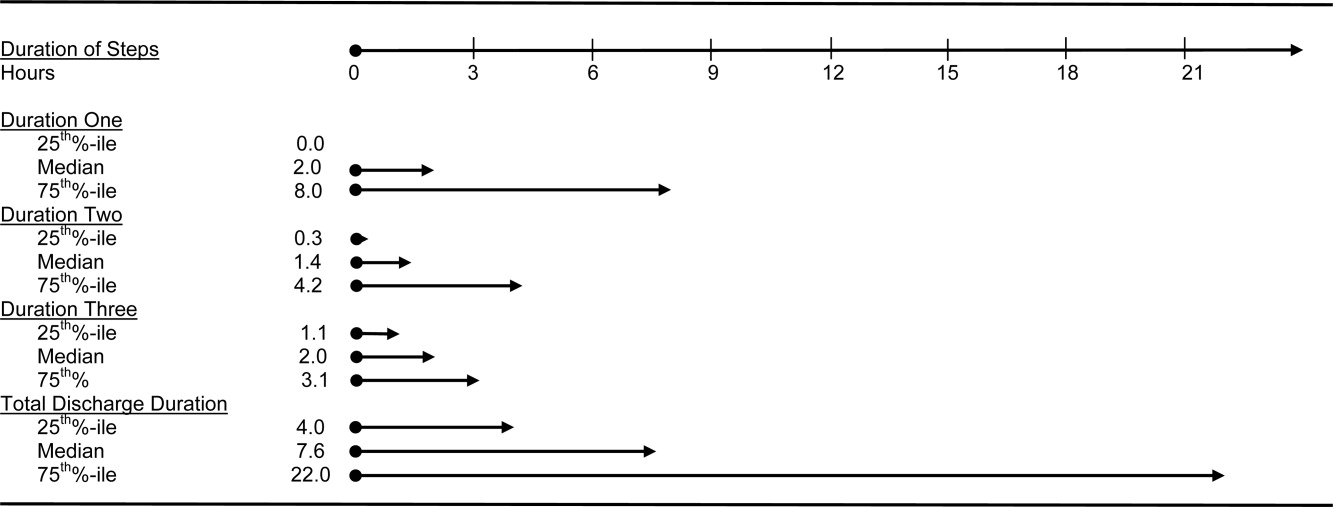
The final multivariate model for time of discharge contained 6 covariates: age, sex, race, test type, prescriptions filled prior to discharge, and need for an ambulance on discharge (Table 2). Special discharge needs continued to be associated with later discharges. Those patients who required an ambulance for transport had mean discharge times that were later by 1.5 hours (95% CI, 0.42.5). If staff obtained discharge medications for patients, these patients left 1.4 hours later than those patients who filled their prescriptions on their own (95% CI, 0.32.4). Patients requiring a consult or procedure also had significantly later discharges (1.2 hours for consults, 95% CI, 0.12.4; 1.1 hours for procedures, 95% CI, 0.12.1) than those needing a bedside exam. Age, sex, and race remained insignificant at the P 0.05 level in the final multivariate model. Length of stay was significantly associated with discharge time in crude analysis, but this variable dropped out of the final multivariate model.
Adjusted Coefficient, Discharge Time as Outcome in Hours (95% CI)* | P Value | Adjusted Coefficient, Discharge Duration as Outcome in Hours (95% CI) | P Value | |
---|---|---|---|---|
| ||||
Demographics | ||||
Age in quartiles (years) | ||||
7294 | 0.5 (0.5, 1.6) | 0.33 | 0.6 (8.3, 7.2) | 0.88 |
5671 | 0.5 (0.6, 1.6) | 0.41 | 1.3 (9.2, 6.6) | 0.75 |
4455 | 0.2 (0.9, 1.3) | 0.74 | 1.2 (9.0, 6.5) | 0.76 |
Male gender | 0.0 (0.8, 0.8) | 0.97 | 1.2 (6.8, 4.5) | 0.69 |
African American race | 0.1 (0.7, 0.9) | 0.80 | 0.3 (5.6, 6.1) | 0.93 |
Last test characteristics | ||||
Test type | ||||
Consult | 1.2 (0.1, 2.4) | 0.04 | 14.8 (6.5, 23.1) | 0.001 |
Procedure | 1.1 (0.1, 2.1) | 0.03 | 13.4 (6.0, 20.7) | <0.001 |
Laboratory test | 0.8 (1.8, 0.3) | 0.14 | 0.9 (8.4, 6.6) | 0.82 |
Exam (reference) | ||||
Discharge needs | ||||
Prescriptions filled prior to discharge | 1.4 (0.3, 2.4) | 0.02 | ||
Not discharged to home | 28.9 (21.9, 35.9) | <0.001 | ||
Ambulance required for transport | 1.5 (0.4, 2.5) | 0.007 |
We used duration of discharge as a secondary outcome measure. The final multivariate model for discharge duration included: age, sex, race, test type, and discharge to a facility (Table 2). Those who went to a facility on average left 28.9 hours (95% CI, 21.935.9) later than those who went home. Test type continued to show a significant association with discharge duration, although the estimates were slightly lower in the adjusted model. Need for a consult was associated with a discharge that was on average 14.8 hours (95% CI, 6.523.1) longer than discharges contingent on a physical exam. Similarly, those patients who had procedures had discharges that were on average 13.4 hours (95% CI, 6.020.7) longer than those whose last test was an exam. Several factors that were significantly associated with discharge duration in unadjusted analyses dropped out of the final multivariate model. These included: need for an ambulance, length of stay, insurance status, and medical complexity as estimated by APRDRGwt.
Conclusions
We found that discharge time and duration had wide variability and that certain factors were associated with only one outcome variabledischarge time or duration. Two factorsneed for an ambulance and filling of prescriptions prior to dischargewere associated with later hour of discharge. Discharge to a location other than home was associated with prolonged discharge duration. Test characteristicsin particular need for a procedure and consultwere significantly associated with both longer and later discharges.
In bivariate analysis, several factors were not associated with discharge time or duration. These were: African‐American race, sex, age, Department of Medicine occupancy on day of discharge, source of admission, psychiatric comorbidity, and substance abuse comorbidity. We had expected higher occupancy to delay discharge as demand exceeded capacity for tests, consults, etc. Our findings suggest that even though our study was conducted during the winter months when hospital occupancy is typically at its peak, supply of staff was still adequate enough to meet high demand. We had also expected that psychiatric and substance abuse comorbidities would prolong discharge as prior studies have found some of these diagnoses to be associated with longer lengths of stay.3134 However, our results do not support such an association, and may reflect our decision to group all psychiatric diagnoses together due to limited sample size.
The main strength of our study is the use of 2 outcome variablestime and durationto define delayed discharges. Our findings demonstrate that few factors are associated with both later and longer discharges. In an era when avoiding emergency room walkouts through early morning hospital discharges can be as important as managing overall length of stay, identifying factors associated with both duration and timing of discharges addresses actual challenges faced by hospitals with limited resources. Prior studies have rarely addressed both outcomes. An additional strength of our study is our use of an interdisciplinary survey. The discharge process is a key component of the inpatient stay, but it is also one for which no group is entirely responsible. Through the development and administration of an interdisciplinary survey, our study adds detail to existing descriptions of this fragmented process, and identifies potential areas for improvement.
Several limitations of our study deserve comment. First, we examined patients discharged from a hospitalist unit without house‐staff at an urban tertiary care hospital. Our findings may need additional interpretation prior to their application in dissimilar settings such as: (1) resident‐covered units in which workflow is shaped by teaching rounds, and (2) nonacademic hospitals in which incentives to provide rapid consults and procedures may be different. Second, we relied on self‐reporting for certain variables such as time decisive test needed. This may be subject to recall bias, as we did not have staff to independently verify recalled times. However, since the discharge process is generally a linear one, we were able to verify the general scope of recalled times with times date‐stamped by the computer during the discharge process (eg, checking that time decisive test needed did not occur after the discharge worksheet had been finalized in the electronic order‐entry system). Third, our sample size was not large enough to control for disease‐specific quality measures. Of note, prior studies have not identified a consistently positive or negative relationship between quality of care and efficiency.3538
Past work has used administrative and survey data to analyze the effect of discharge planning interventions on financial or quality outcomes. Outcomes have included readmissions, mortality, patient satisfaction, length of stay, and inappropriate bed days.22, 3845 However, as capacity management has become a more pressing issue for hospitals, greater focus is being placed on the mismatch between supply and demand of patients at each hour of the day. The relevant unit of measure for efficient discharges has become hour of day, in addition to total length of stay. Some hospital improvement projects have already addressed this shift in thinking.20, 21 Our study adds to this work by formally describing the precise timing and duration of steps in the discharge process, and identifying factors associated with both time and duration.
We believe the results of our study have several implications for hospital administrators and patients interested in more timely care. First, the methods used provide a tangible framework for addressing problems that cross disciplines (eg, nursing, physician, social work) and departments (eg, medicine and radiology), and have a multitude of potential causes and confounders. The survey results offer guidance on where to focus resources, provide a shared baseline metric for improvement, and suggest the cross‐functional team that should be involved in change efforts. Such an approach may be useful for addressing common system‐based challenges in inpatient quality and safety.
Second, with specific regard to discharge planning, our study supports the notion that modifiable factors are associated with discharge time and duration. However, we also describe a fragmented discharge process, with no single bottleneck or department responsible for the majority of late and long discharges. Although procedures and consults were both associated with longer and later discharges, only 26% of patients required a procedure prior to discharge, and 18% required a consult. Moreover, among procedures, different people and events are needed to carry out the 2 most popular procedures: stress echocardiograms, and non‐MRI radiology procedures. Hospital leadership at the highest levels will be required to improve efficiency based on local usage patterns, and to increase coordination among the multiple interdepartmental processes that make up the more general categories of procedure and consult.
Acknowledgements
The authors thank the patients and staff of the Hospitalist Unit for their participation in this study. In addition, they sincerely thank Paul X. Allen, Richard O. Davis, Ronald R. Peterson, and Shuai Shao. They also thank the anonymous reviewers for their thoughtful comments.
- Emergency departments and crowding in United States teaching hospitals.Ann Emerg Med.1991;20:980–986. , , , , .
- A conceptual model of emergency department crowding.Ann Emerg Med.2003;42:173–180. , , , , , .
- Frequent overcrowding in U.S. emergency departments.Acad Emerg Med.2001;8:151–155. , , .
- The effect of hospital occupancy on emergency department length of stay and patient disposition.Acad Emerg Med.2003;10:127–133. , , , , .
- Calculus, chaos, and other models of emergency department crowding.Ann Emerg Med.2003;42:181–184. .
- Increased health care costs associated with ED overcrowding.Am J Emerg Med.1994;12:265–266. , .
- Decreased health care quality associated with emergency department overcrowding.Eur J Emerg Med.1999;6:105–107. , , , et al.
- Can good bed management solve the overcrowding in accident and emergency departments?Emerg Med J.2003;20:149–155. , , .
- Emergency department overcrowding in the United States: an emerging threat to patient safety and public health.Emerg Med J.2003;20:402–405. , .
- General Accounting Office. Hospital emergency departments: crowded conditions vary among hospitals and communities. 2003:GAO‐03–460. Available at: http://www.gao.gov/new.items/d03460.pdf. Accessed October.
- Do you want to die?Time.1990;135:58–60+ , .
- The ER crisis.Ladies Home J.2001;118:70–79. .
- Placing emergency department crowding on the decision agenda.Nurs Econ.2005;23:14–24. .
- Crisis seen in nation's ER care. Washington Post. June 15,2006;A01. .
- Committee on the Future of Emergency Care in the United States Health System.Hospital‐based Emergency Care: at the Breaking Point.Washington DC:National Academies Press;2006.
- Waits to see an emergency department physician: U.S. trends and predictors, 1997–2004.Health Aff.2008;27:w84–w95. , , , et al.
- Advisory Board.Maximizing Hospital Capacity: Expediting Throughput in an Era of Shortage. Health Care Advisory Board research study.Washington DC:Advisory Board; September 12,2002.
- Does U.S. hospital capacity need to be expanded?Health Aff.2003;22:40–54. , , , .
- Institute for Healthcare Improvement.Optimizing Patient Flow: Moving Patients Smoothly Through Acute Care Settings.Cambridge, MA:Institute for Healthcare Improvement;2003.
- http://healthcare.isixsigma.com/library/content/c030708a.asp. Accessed October2008. , . Leveraging six sigma to improve hospital bed availability. Available at:
- University Health System Consortium. Managing patient flow implementation: 2005 benchmarking project field brief. Available at: http://www.musc.edu/medcenter/UHC‐CCP/PFMgmtFldBk.pdf.
- Discharge planning from hospital to home.Cochrane Database Syst Rev.2004;1:CD000313. , , , .
- Predictors of inappropriate hospital days in a department of internal medicine.Int J Epidemiol.1998;27:513–519. , , , et al.
- Utilisation of hospital beds by the elderly—a cohort study of admissions to a teaching hospital.Ir Med J.1995;88:124–126. , , .
- Demographic and clinical characteristics as predictors of length of hospitalization and readmission.J Clin Psychol.1977;33:1093–1099. , , , , , .
- The epidemiology of delays in a teaching hospital: the development and use of a tool that detects unnecessary hospital days.Med Care.1989;27:112–129. , , , .
- American Medical Association.AMA Physician ICD‐9‐CM 2004, Vols 1 and 2.Eden Prairie, MN:Ingenix;2003.
- 3M Health Information Systems and the Maryland Health Care Payment System: frequently asked questions.2004. Available at: http://multimedia.mmm.com/mws/mediawebserver.dyn?6666660Zjcf6lVs6EVs66S7wwCOrrrrQ‐.
- Statistics with STATA.Belmont, CA:Duxbury Press;2004. .
- Statistical data analysis in the computer age.Science.1991;253:390–395. , .
- Psychological comorbidity and length of stay in the general hospital.Am J Psychiatry.1991;148(3):324–329. , , , , .
- Psychiatric comorbidity and greater hospitalization risk, longer length of stay, and higher hospitalization costs in older adults with heart failure.J Am Geriatr Soc.2007;55:1585–1591. , , , , , .
- Does psychiatric comorbidity increase the length of stay in general hospitals?Gen Hosp Psychiatry.2001;23:8–14. , , , .
- The impact of psychiatric comorbidity on length of stay of medical inpatients.Gen Hosp Psychiatry.2003;25:14–19. , , .
- The implications of regional variations in Medicare spending. Part 1: The content, quality, and accessibility of care.Ann Intern Med.2003;138:273–287. , , , , , .
- The implications of regional variations in Medicare spending. Part 2: Health outcomes and satisfaction with care.Ann Intern Med.2003;138:288–298. , , , , , .
- Is early too early? Effect of shorter stays after bypass surgery.Ann Thorac Surg.2007;83:100–107. , , , et al.
- Excess length of stay, charges, and mortality attributable to medical injuries during hospitalization.JAMA.2003;290:1868–1874. , .
- Comprehensive discharge planning and home follow‐up of hospitalized elders, a randomized clinical trial.JAMA.1999;281:613–620. , , , et al.
- Comprehensive discharge planning for the hospitalized elderly, a randomized clinical trial.Ann Intern Med.1994;120:999–1006. , , , , , .
- Evaluating hospital discharge planning: a randomized clinical trial.Med Care.1993;31:358–370. , .
- Does a dedicated discharge coordinator improve the quality of hospital discharge?Qual Health Care.1996;5:89–96. , , , , .
- The effect of a hospitalist service with nurse discharge planner on patient care in an academic teaching hospital.Am J Med.2001;111:627–632. , , , et al.
- Comprehensive discharge planning with postdischarge support for older patients with congestive heart failure: a meta‐analysis.JAMA.2004;291:1358–1367. , , , , , .
- Does increased access to primary care reduce hospital readmissions?N Engl J Med.1996;334:1441–1447. , , .
- Emergency departments and crowding in United States teaching hospitals.Ann Emerg Med.1991;20:980–986. , , , , .
- A conceptual model of emergency department crowding.Ann Emerg Med.2003;42:173–180. , , , , , .
- Frequent overcrowding in U.S. emergency departments.Acad Emerg Med.2001;8:151–155. , , .
- The effect of hospital occupancy on emergency department length of stay and patient disposition.Acad Emerg Med.2003;10:127–133. , , , , .
- Calculus, chaos, and other models of emergency department crowding.Ann Emerg Med.2003;42:181–184. .
- Increased health care costs associated with ED overcrowding.Am J Emerg Med.1994;12:265–266. , .
- Decreased health care quality associated with emergency department overcrowding.Eur J Emerg Med.1999;6:105–107. , , , et al.
- Can good bed management solve the overcrowding in accident and emergency departments?Emerg Med J.2003;20:149–155. , , .
- Emergency department overcrowding in the United States: an emerging threat to patient safety and public health.Emerg Med J.2003;20:402–405. , .
- General Accounting Office. Hospital emergency departments: crowded conditions vary among hospitals and communities. 2003:GAO‐03–460. Available at: http://www.gao.gov/new.items/d03460.pdf. Accessed October.
- Do you want to die?Time.1990;135:58–60+ , .
- The ER crisis.Ladies Home J.2001;118:70–79. .
- Placing emergency department crowding on the decision agenda.Nurs Econ.2005;23:14–24. .
- Crisis seen in nation's ER care. Washington Post. June 15,2006;A01. .
- Committee on the Future of Emergency Care in the United States Health System.Hospital‐based Emergency Care: at the Breaking Point.Washington DC:National Academies Press;2006.
- Waits to see an emergency department physician: U.S. trends and predictors, 1997–2004.Health Aff.2008;27:w84–w95. , , , et al.
- Advisory Board.Maximizing Hospital Capacity: Expediting Throughput in an Era of Shortage. Health Care Advisory Board research study.Washington DC:Advisory Board; September 12,2002.
- Does U.S. hospital capacity need to be expanded?Health Aff.2003;22:40–54. , , , .
- Institute for Healthcare Improvement.Optimizing Patient Flow: Moving Patients Smoothly Through Acute Care Settings.Cambridge, MA:Institute for Healthcare Improvement;2003.
- http://healthcare.isixsigma.com/library/content/c030708a.asp. Accessed October2008. , . Leveraging six sigma to improve hospital bed availability. Available at:
- University Health System Consortium. Managing patient flow implementation: 2005 benchmarking project field brief. Available at: http://www.musc.edu/medcenter/UHC‐CCP/PFMgmtFldBk.pdf.
- Discharge planning from hospital to home.Cochrane Database Syst Rev.2004;1:CD000313. , , , .
- Predictors of inappropriate hospital days in a department of internal medicine.Int J Epidemiol.1998;27:513–519. , , , et al.
- Utilisation of hospital beds by the elderly—a cohort study of admissions to a teaching hospital.Ir Med J.1995;88:124–126. , , .
- Demographic and clinical characteristics as predictors of length of hospitalization and readmission.J Clin Psychol.1977;33:1093–1099. , , , , , .
- The epidemiology of delays in a teaching hospital: the development and use of a tool that detects unnecessary hospital days.Med Care.1989;27:112–129. , , , .
- American Medical Association.AMA Physician ICD‐9‐CM 2004, Vols 1 and 2.Eden Prairie, MN:Ingenix;2003.
- 3M Health Information Systems and the Maryland Health Care Payment System: frequently asked questions.2004. Available at: http://multimedia.mmm.com/mws/mediawebserver.dyn?6666660Zjcf6lVs6EVs66S7wwCOrrrrQ‐.
- Statistics with STATA.Belmont, CA:Duxbury Press;2004. .
- Statistical data analysis in the computer age.Science.1991;253:390–395. , .
- Psychological comorbidity and length of stay in the general hospital.Am J Psychiatry.1991;148(3):324–329. , , , , .
- Psychiatric comorbidity and greater hospitalization risk, longer length of stay, and higher hospitalization costs in older adults with heart failure.J Am Geriatr Soc.2007;55:1585–1591. , , , , , .
- Does psychiatric comorbidity increase the length of stay in general hospitals?Gen Hosp Psychiatry.2001;23:8–14. , , , .
- The impact of psychiatric comorbidity on length of stay of medical inpatients.Gen Hosp Psychiatry.2003;25:14–19. , , .
- The implications of regional variations in Medicare spending. Part 1: The content, quality, and accessibility of care.Ann Intern Med.2003;138:273–287. , , , , , .
- The implications of regional variations in Medicare spending. Part 2: Health outcomes and satisfaction with care.Ann Intern Med.2003;138:288–298. , , , , , .
- Is early too early? Effect of shorter stays after bypass surgery.Ann Thorac Surg.2007;83:100–107. , , , et al.
- Excess length of stay, charges, and mortality attributable to medical injuries during hospitalization.JAMA.2003;290:1868–1874. , .
- Comprehensive discharge planning and home follow‐up of hospitalized elders, a randomized clinical trial.JAMA.1999;281:613–620. , , , et al.
- Comprehensive discharge planning for the hospitalized elderly, a randomized clinical trial.Ann Intern Med.1994;120:999–1006. , , , , , .
- Evaluating hospital discharge planning: a randomized clinical trial.Med Care.1993;31:358–370. , .
- Does a dedicated discharge coordinator improve the quality of hospital discharge?Qual Health Care.1996;5:89–96. , , , , .
- The effect of a hospitalist service with nurse discharge planner on patient care in an academic teaching hospital.Am J Med.2001;111:627–632. , , , et al.
- Comprehensive discharge planning with postdischarge support for older patients with congestive heart failure: a meta‐analysis.JAMA.2004;291:1358–1367. , , , , , .
- Does increased access to primary care reduce hospital readmissions?N Engl J Med.1996;334:1441–1447. , , .
Copyright © 2009 Society of Hospital Medicine