User login
LMW vs UF Heparin
Venous thromboembolism (VTE) is a major source of morbidity and mortality for hospitalized patients, with as many as 16% of high‐risk medical patients developing VTE during their hospital stay.1, 2 Pharmacologic prophylaxis with subcutaneous heparin reduces the risk of VTE by approximately 50%,3, 4 and guidelines produced by the American College of Chest Physicians (ACCP) recommend thromboprophylaxis for patients at moderate‐to‐high risk of VTE with either low‐molecular‐weight heparin (LMWH) or unfractionated heparin (UFH).2 UFH is less expensive per dose, but meta‐analyses have suggested that UFH may be either less effective than LMWH3 or more likely to cause complications, such as bleeding5 or heparin‐induced thrombocytopenia (HIT).6 Others have argued that the efficacy and risk of bleeding with UFH and LMWH are similar.7, 8 In either case, there are few head‐to‐head studies of LMWH and UFH in medical patients and they tend to be small. In the most recent meta‐analysis, which included fewer than 4500 patients, several different low‐molecular‐weight heparins were assessed together, and the observed rate of deep vein thrombosis (DVT) with UFH was high (5.4%), with evidence suggesting publication bias.3
Given the current Joint Commission requirement9 that all medical patients either receive VTE prophylaxis or have documented a reason not to, the implications related to choosing one form of VTE prophylaxis over another are substantial on a national scale. In order to compare the effectiveness of UFH and LMWH in routine practice among hospitalized medical patients, we conducted a retrospective cohort study in a national sample of hospitals and compared the risk of VTE, bleeding, HIT, and death associated with each treatment.
METHODS
Setting and Patients
We conducted a retrospective cohort study of patients discharged between January 1, 2004 and June 30, 2005 from 333 acute care facilities in the United States that participated in Premier's Perspective, a database we have described previously.10 Compared to US hospitals as a whole, Perspective hospitals are more likely to be located in the South and in urban areas. Perspective contains the following data elements: sociodemographic information, International Classification of Diseases, Ninth Revision, Clinical Modification (ICD‐9‐CM) diagnosis and procedure codes, as well as a list of all billed items with a date of service, including diagnostic tests, medications, and other treatments. Hospitals' characteristics include size, region, setting, and teaching status. The Institutional Review Board at Baystate Medical Center granted permission to conduct the study (#132280‐1).
We included general medical patients aged 18 years whose ICD‐9‐CM primary diagnosis code (congestive heart failure, stroke, pneumonia, and urinary tract infection) placed them at moderate‐to‐high risk of VTE according to the ACCP recommendations,2 and who received daily prophylactic dosages of either LMWH (40 mg daily) or UFH (10,00015,000 units daily) initiated by hospital day 2 and continued to discharge or until the patient developed a VTE or a complication attributable to heparin. Patients were included so long as they missed no more than 1 day of prophylaxis or had no more than 1 unusual dose recorded. Patients who switched between heparin types were included and analyzed according to their initial therapy. Patients who received any other regimen were excluded. We also excluded patients who received warfarin on hospital day 1 or 2, because they would not be considered candidates for heparin prophylaxis, and patients whose length of stay was 2 days, because the value of VTE prophylaxis in such cases is unknown.
Data Elements
For each patient, we extracted age, gender, race, and insurance status, principal diagnosis, comorbidities, and specialty of the attending physician. Comorbidities were identified from ICD‐9‐CM secondary diagnosis codes and Diagnosis Related Groups using Healthcare Cost and Utilization Project Comorbidity Software, version 3.1, based on the work of Elixhauser and colleagues.11 We also identified additional risk factors for VTE using a combination of ICD‐9‐CM codes and specific charges. These included cancer, chemotherapy/radiation, prior VTE, use of estrogens and estrogen modulators, inflammatory bowel disease, nephrotic syndrome, myeloproliferative disorders, smoking, central venous catheter, inherited or acquired thrombophilia, mechanical ventilation, urinary catheter, decubitus ulcer, 3‐hydroxy‐3‐methylglutaryl coenzyme A (HMG‐CoA) reductase inhibitors, restraints, and varicose veins. Hospitals were categorized by region (Northeast, South, Midwest, or West), bed size, setting (urban vs rural), and teaching status.
Outcome Variables
We defined hospital‐acquired VTE as a secondary diagnosis of VTE (ICD‐9‐CM diagnoses 453.4, 453.40, 453.41, 453.42, 453.8, 453.9, 415.1, 415.11, 415.19), combined with a diagnostic test for VTE (lower extremity ultrasound, venography, computed tomography (CT) angiogram, ventilation‐perfusion scan, or pulmonary angiogram) after hospital day 2, followed by treatment for VTE (intravenous unfractionated heparin, >60 mg of enoxaparin, 7500 mg of dalteparin, or placement of an inferior vena cava filter) for at least 50% of the remaining hospital days or until initiation of warfarin or appearance of a complication (eg, transfusion or treatment for heparin‐induced thrombocytopenia). We chose this definition to differentiate hospital‐acquired VTE from VTE present on admission.12 In addition, patients who were readmitted within 30 days of discharge with a primary diagnosis of VTE were also considered to have hospital‐acquired VTE.
We also assessed complications of VTE prophylaxis. Major bleeding was defined as the receipt of 2 or more units of packed red blood cells on a single day or a secondary diagnosis of intracranial bleeding. Because there was no ICD‐9‐CM code for HIT, we assessed codes for all thrombocytopenia, as well as secondary thrombocytopenia. Definite HIT was defined as an ICD‐9‐CM code for thrombocytopenia, together with discontinuation of heparin and initiation of treatment with argatroban. A definite complication was defined as HIT or evidence of major bleeding coupled with discontinuation of heparin. Finally, we evaluated all‐cause in‐hospital mortality and total hospital costs.
Statistical Analysis
We computed summary statistics using frequencies and percents for categorical variables, and means, medians, and standard deviations and interquartile range for continuous variables. Associations of prophylaxis type with patient and hospital characteristics and outcomes were assessed using chi‐square tests or Fisher's exact test for categorical variables, and z‐tests or Wilcoxon tests for continuous variables.
We developed a propensity model for treatment with UFH as the outcome; the model included patient characteristics, early treatments, comorbidities, risk factors for VTE, physician specialty, and selected interaction terms. We then developed a series of multivariable models to evaluate the impact of heparin choice on the risk of VTE, complications of treatment, mortality, and total cost. Generalized estimating equation models with a logit link were used to assess the association between the choice of heparin and the risk of VTE, and of complications and mortality, while adjusting for the effects of within‐hospital correlation; identity link models were used for analyses of cost. Costs were trimmed at 3 standard deviations above the mean, and natural log‐transformed values were modeled due to extreme positive skew.
Unadjusted and covariate‐adjusted models were evaluated with and without adjustments for propensity score. In addition, since the hospital was the single strongest predictor of treatment, we developed grouped treatment models, in which a patient's actual treatment was replaced by a probability equal to the proportion of prophylaxed patients receiving UFH at that hospital. This adaptation of instrumental variable analysis uses the hospital as the instrument, and attempts to assess whether patients treated at a hospital which uses UFH more frequently have outcomes that differ from those of patients treated at hospitals which use LMWH more frequently, while adjusting for other patient, physician, and hospital variables. By relying on treatment at the hospital level, this method reduces the opportunity for selection bias at the patient level.
Finally, in order to exclude the possibility that our surrogate bleeding outcome was due to transfusion practices at hospitals that use a particular form of heparin, we compared the hospital rates of transfusion of 2 or more units of packed red cells to the hospital rates of prophylaxis with UFH in a larger dataset of the same hospitals. This set included patients with congestive heart failure, stroke, pneumonia, and urinary tract infection who did not receive daily prophylaxis, as well as patients admitted for chronic obstructive pulmonary disease (COPD) or acute myocardial infarction, and patients who received either warfarin or a treatment dose of heparin in the first 2 hospital days. We also compared the transfusion rates at hospitals that used unfractionated heparin in 80% of patients to hospitals that used LMWH in 80%. All analyses were performed using SAS version 9.1 (SAS Institute Inc, Cary, NC).
RESULTS
Our final sample included 32,104 patients who received prophylaxis at 333 hospitals (see Supporting Information, e‐Figure, in the online version of this article). Patient characteristics appear in Table 1. Most patients (66%) were over age 65; 59% were female and 61% were white. The most common primary diagnoses were pneumonia (40%) and congestive heart failure (25%). Additional risk factors for thromboembolism included cancer (13%), paralysis (8%), or diabetes (35%). Most patients' attending physicians were either internists (61%) or family practitioners (14%). Almost half of the patients were cared for at hospitals in the South (46%).
Total | UFH | LMWH | ||
---|---|---|---|---|
32,104 (100) | 14,591 (45.4) | 17,513 (54.6) | ||
N (%) | N (%) | N (%) | P | |
| ||||
Demographics | ||||
Age | 0.0002 | |||
1849 | 4,061 (12.7) | 1,950 (13.4) | 2,111 (12.1) | |
5064 | 6,962 (21.7) | 3,225 (22.1) | 3,737 (21.3) | |
6579 | 10,909 (34.0) | 4,921 (33.7) | 5,988 (34.2) | |
80+ | 10,172 (31.7) | 4,495 (30.8) | 5,677 (32.4) | |
Sex | 0.0071 | |||
Male | 13,234 (41.2) | 6,133 (42.0) | 7,101 (40.5) | |
Female | 18,870 (58.8) | 8,458 (58.0) | 10,412 (59.5) | |
Race/ethnicity | <0.0001 | |||
White | 19,489 (60.7) | 8,063 (55.3) | 11,426 (65.2) | |
Black | 7,429 (23.1) | 4,101 (28.1) | 3,328 (19.0) | |
Hispanic | 1,304 (4.1) | 591 (4.1) | 713 (4.1) | |
Other | 3,882 (12.1) | 1,836 (12.6) | 2,046 (11.7) | |
Primary diagnosis | <0.0001 | |||
Pneumonia | 12,768 (39.8) | 5,354 (36.7) | 7,414 (42.3) | |
Sepsis* | 1,217 (3.8) | 562 (3.9) | 655 (3.7) | |
Respiratory failure* | 2,017 (6.3) | 814 (5.6) | 1,203 (6.9) | |
Heart failure | 8,157 (25.4) | 3,825 (26.2) | 4,332 (24.7) | |
Stroke | 4,416 (13.8) | 2,295 (15.7) | 2,121 (12.1) | |
Urinary tract infection | 3,529 (11.0) | 1,741 (11.9) | 1,788 (10.2) | |
Attending specialty | <0.0001 | |||
Internist | 19,511 (60.8) | 8,945 (61.3) | 10,566 (60.3) | |
General practice/Family medicine | 4,326 (13.5) | 1,964 (13.5) | 2,362 (13.5) | |
Cardiologist | 1,606 (5.0) | 730 (5.0) | 876 (5.0) | |
Pulmonologist | 2,179 (6.8) | 854 (5.9) | 1,325 (7.6) | |
Nephrology | 583 (1.8) | 380 (2.6) | 203 (1.2) | |
Critical care/Intensivist | 150 (0.5) | 93 (0.6) | 57 (0.3) | |
Other | 3,749 (11.7) | 1,625 (11.1) | 2,124 (12.1) | |
Insurance | <0.0001 | |||
Medicare traditional | 20,281 (63.2) | 8,929 (61.2) | 11,352 (64.8) | |
Medicare managed care | 1,737 (5.4) | 826 (5.7) | 911 (5.2) | |
Medicaid | 2,629 (8.2) | 1,401 (9.6) | 1,228 (7.0) | |
Private | 5,967 (18.6) | 2,830 (19.4) | 3,137 (17.9) | |
Self‐pay/uninsured/other | 1,490 (4.6) | 605 (4.1) | 885 (5.1) | |
Risk factors for VTE | ||||
Admit from skilled nursing facility | 476 (1.5) | 277 (1.9) | 199 (1.1) | <0.0001 |
Paralysis | 2,608 (8.1) | 1,317 (9.0) | 1,291 (7.4) | <0.0001 |
Restraints | 417 (1.3) | 147 (1.0) | 270 (1.5) | <0.0001 |
Decubitus ulcer | 1,190 (3.7) | 631 (4.3) | 559 (3.2) | <0.0001 |
Cancer | 4,154 (12.9) | 1,858 (12.7) | 2,296 (13.1) | 0.3171 |
Chemotherapy | 86 (0.3) | 41 (0.3) | 45 (0.3) | 0.6781 |
Prior venous thromboembolism | 494 (1.5) | 202 (1.4) | 292 (1.7) | 0.0403 |
Pregnancy | 1 (0) | 1 (0) | 0 (0) | 0.2733 |
Estrogens | 438 (1.4) | 143 (1.0) | 295 (1.7) | <0.0001 |
Estrogen modulators | 246 (0.8) | 80 (0.5) | 166 (0.9) | <0.0001 |
Congestive heart failure | 3,107 (9.7) | 1,438 (9.9) | 1,669 (9.5) | 0.3263 |
Respiratory failure | 2,210 (6.9) | 1,037 (7.1) | 1,173 (6.7) | 0.1493 |
Inflammatory bowel disease | 108 (0.3) | 41 (0.3) | 67 (0.4) | 0.1176 |
Nephrotic syndrome | 92 (0.3) | 50 (0.3) | 42 (0.2) | 0.0860 |
Myeloproliferative disorder | 198 (0.6) | 68 (0.5) | 130 (0.7) | 0.0016 |
Obesity | 2,973 (9.3) | 1,211 (8.3) | 1,762 (10.1) | <0.0001 |
Smoking | 4,476 (13.9) | 1,887 (12.9) | 2,589 (14.8) | <0.0001 |
Varicose veins | 19 (0.1) | 6 (0) | 13 (0.1) | 0.2245 |
Central line | 1,070 (3.3) | 502 (3.4) | 568 (3.2) | 0.3271 |
Inherited or acquired thrombophilia | 16 (0) | 9 (0.1) | 7 (0) | 0.3855 |
Diabetes | 11,136 (34.7) | 5,157 (35.3) | 5,979 (34.1) | 0.0241 |
Procedures associated with VTE or bleed | ||||
Mechanical ventilation | 2,282 (7.1) | 1,111 (7.6) | 1,171 (6.7) | 0.0013 |
Urinary catheter | 4,496 (14.0) | 1,545 (10.6) | 2,951 (16.9) | <0.0001 |
Aspirin | 12,865 (40.1) | 6,101 (41.8) | 6,764 (38.6) | <0.0001 |
Clopidogrel | 4,575 (14.3) | 2,087 (14.3) | 2,488 (14.2) | 0.8050 |
Non‐steroidal anti‐inflammatory drugs | 2,147 (6.7) | 867 (5.9) | 1,280 (7.3) | <0.0001 |
Steroids | 7,938 (24.7) | 3,136 (21.5) | 4,802 (27.4) | <0.0001 |
Statins | 7,376 (23.0) | 3,462 (23.7) | 3,914 (22.3) | 0.0035 |
Comorbidities | ||||
AIDS | 124 (0.4) | 73 (0.5) | 51 (0.3) | 0.0026 |
Alcohol abuse | 1,048 (3.3) | 523 (3.6) | 525 (3.0) | 0.0032 |
Deficiency anemia | 7,010 (21.8) | 3,228 (22.1) | 3,782 (21.6) | 0.2543 |
Rheumatoid arthritis/collagen vas | 967 (3.0) | 426 (2.9) | 541 (3.1) | 0.3762 |
Chronic blood loss anemia | 177 (0.6) | 79 (0.5) | 98 (0.6) | 0.8269 |
Chronic pulmonary disease | 12,418 (38.7) | 5,314 (36.4) | 7,104 (40.6) | <0.0001 |
Depression | 3,334 (10.4) | 1433 (9.8) | 1901 (10.9) | 0.0025 |
Drug abuse | 694 (2.2) | 412 (2.8) | 282 (1.6) | <0.0001 |
Hypertension | 16,979 (52.9) | 7,658 (52.5) | 9,321 (53.2) | 0.1866 |
Hypothyroidism | 4,016 (12.5) | 1,716 (11.8) | 2,300 (13.1) | 0.0002 |
Liver disease | 453 (1.4) | 227 (1.6) | 226 (1.3) | 0.0448 |
Other neurological disorders | 4,682 (14.6) | 2,202 (15.1) | 2,480 (14.2) | 0.0187 |
Peripheral vascular disease | 2,134 (6.6) | 980 (6.7) | 1,154 (6.6) | 0.6490 |
Psychoses | 1,295 (4.0) | 574 (3.9) | 721 (4.1) | 0.4066 |
Pulmonary circulation disease | 1,034 (3.2) | 442 (3.0) | 592 (3.4) | 0.0760 |
Renal failure | 2,794 (8.7) | 1,636 (11.2) | 1,158 (6.6) | 0.0000 |
Peptic ulcer disease with bleeding | 563 (1.8) | 232 (1.6) | 331 (1.9) | 0.0414 |
Valvular disease | 2,079 (6.5) | 899 (6.2) | 1,180 (6.7) | 0.0366 |
Weight loss | 1,231 (3.8) | 556 (3.8) | 675 (3.9) | 0.8391 |
Other prophylaxis | ||||
Intermittent pneumatic compression | 1,003 (3.1) | 456 (3.1) | 547 (3.1) | 0.9926 |
Mechanical prophylaxis | 1,281 (4.0) | 524 (3.6) | 757 (4.3) | 0.0009 |
Fifty‐five percent of patients received LMWH and the remainder received UFH; 1274 (4%) patients switched type of heparin during their stay. The proportion of patients receiving LMWH at an individual hospital varied from 0% to 100% with a u‐shaped distribution, with almost one‐third of hospitals prescribing one treatment or the other exclusively (Figure 1). Similarly, the proportion of an individual physician's patients who received prophylaxis with UFH (vs LMWH) varied from 0% to 100% (Figure 1), with 51% prescribing LMWH exclusively and 31% prescribing UFH exclusively. Compared to patients who received UFH, patients receiving LMWH were older and were more likely to be white, female, and to have pneumonia. By far the biggest difference between the groups was the hospitals at which they received their care (see Supporting Information, e‐Table, in the online version of this article). Patients receiving LMWH were much more likely to be from smaller, rural, non‐teaching hospitals in the South or the West. There were also numerous small differences in comorbidities and individual VTE risk factors between the 2 groups. The only large difference was that patients with a secondary diagnosis of renal failure (for which LMWH is not US Food and Drug Administration [FDA] approved) were almost twice as likely to receive UFH.
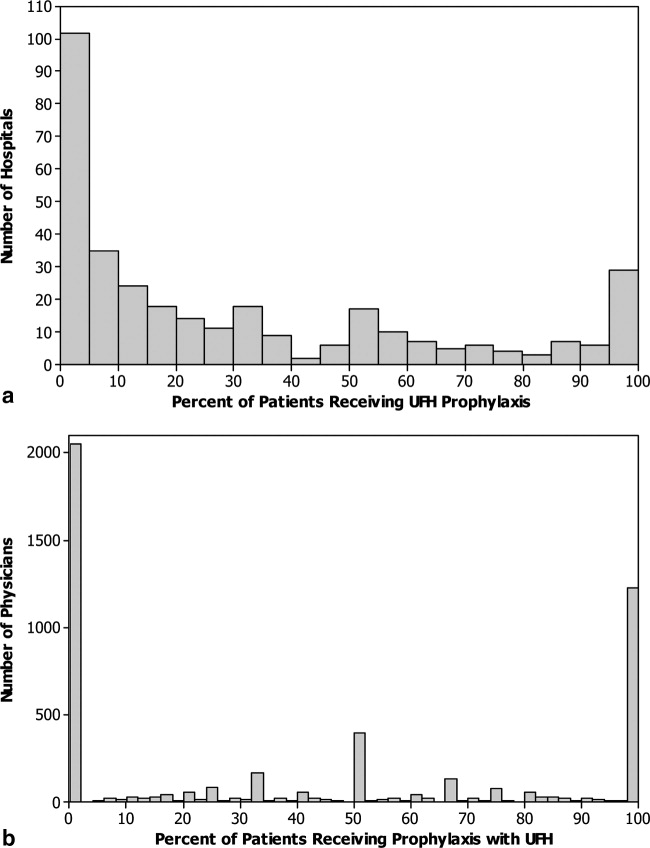
We identified 163 (0.51%) episodes of VTE (Table 2). Compared to patients receiving UFH, those receiving standard LMWH had similar unadjusted rates of VTE (0.53% vs 0.48%; P = 0.54), major bleeding (0.77% vs 0.76%; P = 0.88), thrombocytopenia (1.9% vs 2.0%; P = 0.48), definite HIT (n = 1 vs n = 3; P = 0.34), and mortality (2.8% vs 3.1%; P = 0.07). Definite complications of prophylaxis (HIT or major bleed combined with the discontinuation of heparin) were more common among patients receiving UFH (0.2% vs 0.1%; P = 0.022). Patients treated with UFH had longer unadjusted lengths of stay (P < 0.0001) and higher unadjusted costs (P < 0.0001).
Total | UFH | LMWH | P | |
---|---|---|---|---|
32,104 (100) | 14,591 (45.4) | 17,513 (54.6) | ||
n (%) | n (%) | n (%) | ||
| ||||
Venous thromboembolism | 163 (0.5) | 78 (0.5) | 85 (0.5) | 0.54 |
Heparin‐induced thrombocytopenia | 4 (0) | 3 (0) | 1 (0) | 0.34* |
Any major bleeding | 246 (0.8) | 113 (0.8) | 133 (0.8) | 0.88 |
Transfusion with 2 units of packed red blood cells | 218 (0.7) | 97 (0.7) | 121 (0.7) | 0.78 |
Intracranial hemorrhage | 30 (0.1) | 17 (0.1) | 13 (0.1) | 0.22 |
Complication resulting in stopping heparin | 44 (0.1) | 28 (0.2) | 16 (0.1) | 0.02 |
In‐hospital mortality | 944 (2.9) | 456 (3.1) | 488 (2.8) | 0.07 |
LOS in days; mean (SD) | 6.2 (5.9) | 6.4 (6.2) | 6.0 (5.6) | <0.001 |
Median (IQR) | 5 (37) | 5 (37) | 5 (37) | |
Cost in USD; median (IQR) | 5873 (41718982) | 6007 (41779456) | 5774 (41658660) | <0.001 |
A propensity model for UFH treatment based upon patient characteristics and treatments was not strongly predictive of treatment (c = 0.58) and propensity matching failed to balance many of the patient characteristics. However, hospital alone, ignoring patient characteristics was strongly predictive (c = 0.91) of treatment.
In a model adjusting only for clustering within hospitals, patients treated with UFH had an odds ratio (OR) for VTE of 1.08 (95% confidence interval [CI] 0.79 to 1.49) compared to patients receiving LMWH (Figure 2). Adjustment for propensity for UFH and other covariates attenuated the effect of LMWH (OR 1.04, 95% CI 0.76 to 1.43). When individual patients were assigned a probability of treatment with UFH equal to the hospital rate where they received care, UFH use was associated with a nonsignificant change in the odds of VTE (OR 1.14, 95% CI 0.72 to 1.81).
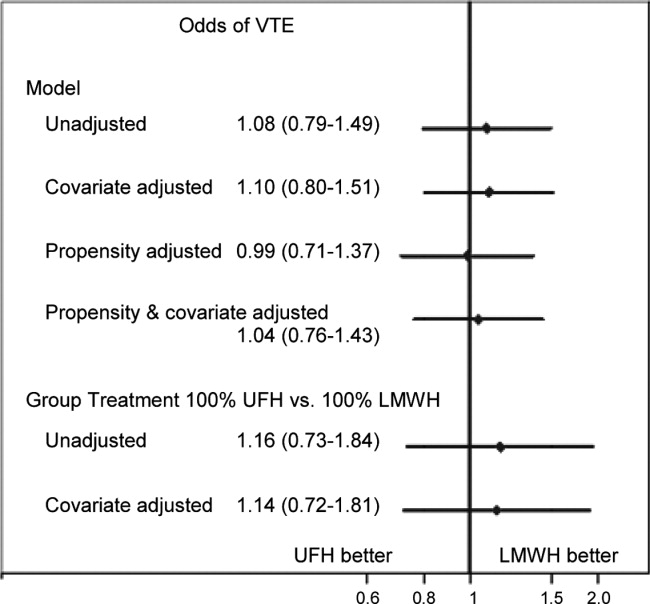
Adjusted for clustering within hospital only, patients treated with UFH had an odds ratio for major bleed of 1.38 (95% CI 1.00 to 1.91) compared to patients receiving LMWH (Figure 3). Adjustment for propensity for UFH and other covariates gave similar results (OR 1.34, 95% CI 0.97 to 1.84). When individual patients were assigned a probability of treatment with UFH equal to the hospital rate where they received care, UFH treatment was associated with a nonsignificant increase in the odds of major bleed (OR 1.64, 95% CI 0.50 to 5.33). When we compared the rate of transfusion across hospitals, including 576,231 additional patients who were excluded from the original analyses because they did not receive daily prophylaxis or had a diagnosis of myocardial infarction or COPD, there was a slight negative correlation between transfusion rates and use of UFH (Spearman Correlation Coefficient 0.03; P = 0.61). Hospitals that used primarily UFH had a transfusion rate of 0.60% versus 0.76% at hospitals using primarily LMWH (P = 0.54), indicating that the increased risk of major bleeding associated with UFH was not confounded by local transfusion practices.
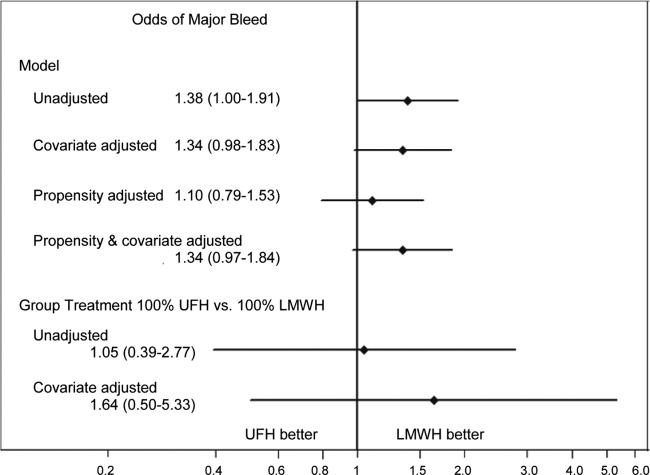
Adjusted for clustering only, patients treated with UFH had an odds ratio for definite complication of 2.35 (95% CI 1.17 to 4.72) compared to those treated with LMWH. Adjustment for propensity and covariates accentuated the association (OR 2.84, 95% CI 1.43 to 5.66). When assigned a probability of treatment with UFH equal to the hospital rate where they received care, UFH treatment was associated with an increase in the risk of definite complication (OR 2.79, 95% CI 1.00 to 7.74).
Adjusted for clustering only, patients treated with UFH had higher costs than those treated with LMWH (cost ratio 1.07, 95% CI 1.05 to 1.09). Adjustment for propensity for UFH and other covariates attenuated the association (cost ratio 1.02, 95% CI 1.00 to 1.03). Finally, when individual patients were assigned a probability of initial treatment with UFH equal to the hospital rate where they received care, treatment with UFH was associated with a nonsignificant change in the relative cost (cost ratio 0.97, 95% CI 0.90 to 1.05).
DISCUSSION
In this retrospective cohort study, we found that low‐molecular‐weight heparin and unfractionated heparin were associated with similar rates of VTE in moderate‐to‐high risk medical patients. However, unfractionated heparin was associated with a small, but higher risk of complications, even after adjustment. There were no statistical differences in rates of heparin‐induced thrombocytopenia, but this complication was exceedingly rare. Finally, overall costs associated with both treatments were similar.
A number of industry‐funded studies have compared LMWH to UFH in randomized clinical trials. These trials have generally been small and used endpoints of uncertain significance, such as asymptomatic deep vein thrombosis assessed by ultrasound. At least 3 meta‐analyses of these trials have been published. Each used different inclusion criteria. The only one to find an efficacy benefit to LMWH over UFH was heavily influenced by the inclusion of a number of studies of stroke patients.3 In that study, LMWH reduced VTE by approximately one‐third relative to UFH. The other 2 analyses found smaller reductions in DVT and pulmonary embolism (PE), and these results were not statistically significant.5, 8 Similarly, 1 analysis5 found a reduction in major bleeding events with LMWH versus UFH, whereas the other 2 studies found smaller reductions which were not statistically significant. The assessment of major bleeding is further complicated by differences in the definition of major bleeding across studies. Using a standard definition of 2 units of packed red blood cells transfused in 1 day to denote major bleeding, we found an associated reduction in bleeding with LMWH that was similar to that observed in the meta‐analyses. Moreover, patients receiving UFH were twice as likely to have a complication that resulted in stopping the prophylaxis, although these complications were overall quite rare. Lastly, there are no cost comparisons based on randomized trials. Several comparisons based on modeling have favored LMWH. One assumed that 3% of patients receiving UFH would develop HIT;13 something we did not observe. At least 3 additional analyses,1416 all funded by the manufacturer of enoxaparin, assumed that LMWH was both more effective and safer than UFH. We found that adjusted costs were similar or slightly lower with UFH than LMWH.
Our study has a number of limitations. First, its observational design makes it vulnerable to selection bias. We attempted to overcome this with rigorous multivariable adjustment, including the propensity for treatment and by using an adaptation of the instrumental variable approach. This method is of particular interest because individual hospitals were strongly predictive of choice of heparin. Still, we cannot exclude the possibility of residual confounding, especially if other outcomes, such as transfusion decisions, were also tied to specific hospital practices. Second, our study used administrative data, and therefore we could not directly adjust for certain differences which may exist between patients who received LMWH and those who received UFH. However, we did adjust for many classic risk factors for VTE. More importantly, it seems that the chance of being treated with a particular form of heparin depends more on the hospital where one receives care than on any combination of patient characteristics. Thus, apart from renal failure, for which we adjusted, it seems unlikely that there were major differences in unmeasured physiological confounders. Third, we limited our analysis to patients who received standard dosing of either type of heparin. We did this to bolster the validity of our findings, but they may not apply to unconventional dosing often observed in clinical practice. Fourth, we measured only outcomes that occurred in the hospital or that prompted a return to the hospital. VTEs which were diagnosed and treated in ambulatory care were not included. While this may have led us to underestimate the true risk of VTE, we have little reason to believe that the choice of whether to admit a patient with VTE is influenced by the original choice of VTE prophylaxis. Finally, our study was conducted before the introduction of generic LMWH, which would be expected to reduce costs associated with LMWH prophylaxis.
VTE prophylaxis for medical patients has emerged as a major focus for quality improvement initiatives. As a result, a significant proportion of general medical patients receive some form of chemoprophylaxis during their hospital stay. Small differences in efficacy or safety of different forms of prophylaxis multiplied by millions of admissions each year can have profound effects on the health of hospitalized patients. Similarly, differences in cost could also have a substantial impact on the healthcare system. We found no difference in efficacy or cost, but treatment with LMWH was less likely to be associated with subsequent transfusion of 2 or more units of packed red blood cells, a surrogate marker for bleeding. In addition, LMWH is more convenient since it can be dosed once daily, and for that reason may be more acceptable to patients. For these reasons, LMWH may be the drug of choice for inpatient prophylaxis of general medical patients. In situations where the cost of the medication itself is important, UFH represents an equally effective alternative.
Acknowledgements
All authors have contributed sufficiently to this study and have provided written permission to be named in the manuscript. No other persons have made substantial contributions to this manuscript. Michael B. Rothberg is the guarantor of the entire manuscript.
Disclosures: This study was supported by a Clinical Scientist Development Award from the Doris Duke Charitable Foundation. The funding source had no role in the study design, analysis, or interpretation of the data. Dr Rothberg served for 1 day as a consultant to Novartis Pharma about an influenza vaccine model. Sandoz, a division of Novartis, was recently granted approval to manufacture a generic form of low‐molecular‐weight heparin. None of the other authors have any conflicts of interest.
- A comparison of enoxaparin with placebo for the prevention of venous thromboembolism in acutely ill medical patients. Prophylaxis in Medical Patients with Enoxaparin Study Group.N Engl J Med.1999;341:793–800. , , , et al.
- Prevention of venous thromboembolism: American College of Chest Physicians Evidence‐Based Clinical Practice Guidelines (8th Edition).Chest.2008;133:381S–453S. , , , et al.
- Pharmacological venous thromboembolism prophylaxis in hospitalized medical patients: a meta‐analysis of randomized controlled trials.Arch Intern Med.2007;167:1476–1486. , , , , .
- Meta‐analysis: anticoagulant prophylaxis to prevent symptomatic venous thromboembolism in hospitalized medical patients.Ann Intern Med.2007;146:278–288. , , , , .
- Prevention of venous thromboembolism in internal medicine with unfractionated or low‐molecular‐weight heparins: a meta‐analysis of randomised clinical trials.Thromb Haemost.2000;83:14–19. , , , et al.
- Risk for heparin‐induced thrombocytopenia with unfractionated and low‐molecular‐weight heparin thromboprophylaxis: a meta‐analysis.Blood.2005;106:2710–2715. , , .
- A safety analysis of thromboprophylaxis in acute medical illness.Thromb Haemost.2003;89:590–591. , .
- How complete is the evidence for thromboembolism prophylaxis in general medicine patients? A meta‐analysis of randomized controlled trials.J Hosp Med.2009;4:289–297. , , , , .
- The Joint Commission on the Accreditation of Healthcare Organizations. Venous Thromboembolism (VTE) Core Measure Set. Available at: http://www.jointcommission.org/PerformanceMeasurement/PerformanceMeasurement/VTE.htm. Accessed June 1,2009.
- Potentially inappropriate medication use in hospitalized elders.J Hosp Med.2008;3:91–102. , , , et al.
- Comorbidity measures for use with administrative data.Med Care.1998;36:8–27. , , , .
- Identification of in‐hospital complications from claims data. Is it valid?Med Care.2000;38:785–795. , , , , , .
- Cost utility of substituting enoxaparin for unfractionated heparin for prophylaxis of venous thrombosis in the hospitalized medical patient.J Hosp Med.2006;1:168–176. , , , .
- Cost effectiveness of thromboprophylaxis with a low‐molecular‐weight heparin versus unfractionated heparin in acutely ill medical inpatients.Am J Manag Care.2004;10:632–642. , , , .
- Comparison of the two‐year outcomes and costs of prophylaxis in medical patients at risk of venous thromboembolism.Thromb Haemost.2008;100:810–820. , , , .
- Cost effectiveness of enoxaparin as prophylaxis against venous thromboembolic complications in acutely ill medical inpatients: modelling study from the hospital perspective in Germany.Pharmacoeconomics.2006;24:571–591. , , , et al.
Venous thromboembolism (VTE) is a major source of morbidity and mortality for hospitalized patients, with as many as 16% of high‐risk medical patients developing VTE during their hospital stay.1, 2 Pharmacologic prophylaxis with subcutaneous heparin reduces the risk of VTE by approximately 50%,3, 4 and guidelines produced by the American College of Chest Physicians (ACCP) recommend thromboprophylaxis for patients at moderate‐to‐high risk of VTE with either low‐molecular‐weight heparin (LMWH) or unfractionated heparin (UFH).2 UFH is less expensive per dose, but meta‐analyses have suggested that UFH may be either less effective than LMWH3 or more likely to cause complications, such as bleeding5 or heparin‐induced thrombocytopenia (HIT).6 Others have argued that the efficacy and risk of bleeding with UFH and LMWH are similar.7, 8 In either case, there are few head‐to‐head studies of LMWH and UFH in medical patients and they tend to be small. In the most recent meta‐analysis, which included fewer than 4500 patients, several different low‐molecular‐weight heparins were assessed together, and the observed rate of deep vein thrombosis (DVT) with UFH was high (5.4%), with evidence suggesting publication bias.3
Given the current Joint Commission requirement9 that all medical patients either receive VTE prophylaxis or have documented a reason not to, the implications related to choosing one form of VTE prophylaxis over another are substantial on a national scale. In order to compare the effectiveness of UFH and LMWH in routine practice among hospitalized medical patients, we conducted a retrospective cohort study in a national sample of hospitals and compared the risk of VTE, bleeding, HIT, and death associated with each treatment.
METHODS
Setting and Patients
We conducted a retrospective cohort study of patients discharged between January 1, 2004 and June 30, 2005 from 333 acute care facilities in the United States that participated in Premier's Perspective, a database we have described previously.10 Compared to US hospitals as a whole, Perspective hospitals are more likely to be located in the South and in urban areas. Perspective contains the following data elements: sociodemographic information, International Classification of Diseases, Ninth Revision, Clinical Modification (ICD‐9‐CM) diagnosis and procedure codes, as well as a list of all billed items with a date of service, including diagnostic tests, medications, and other treatments. Hospitals' characteristics include size, region, setting, and teaching status. The Institutional Review Board at Baystate Medical Center granted permission to conduct the study (#132280‐1).
We included general medical patients aged 18 years whose ICD‐9‐CM primary diagnosis code (congestive heart failure, stroke, pneumonia, and urinary tract infection) placed them at moderate‐to‐high risk of VTE according to the ACCP recommendations,2 and who received daily prophylactic dosages of either LMWH (40 mg daily) or UFH (10,00015,000 units daily) initiated by hospital day 2 and continued to discharge or until the patient developed a VTE or a complication attributable to heparin. Patients were included so long as they missed no more than 1 day of prophylaxis or had no more than 1 unusual dose recorded. Patients who switched between heparin types were included and analyzed according to their initial therapy. Patients who received any other regimen were excluded. We also excluded patients who received warfarin on hospital day 1 or 2, because they would not be considered candidates for heparin prophylaxis, and patients whose length of stay was 2 days, because the value of VTE prophylaxis in such cases is unknown.
Data Elements
For each patient, we extracted age, gender, race, and insurance status, principal diagnosis, comorbidities, and specialty of the attending physician. Comorbidities were identified from ICD‐9‐CM secondary diagnosis codes and Diagnosis Related Groups using Healthcare Cost and Utilization Project Comorbidity Software, version 3.1, based on the work of Elixhauser and colleagues.11 We also identified additional risk factors for VTE using a combination of ICD‐9‐CM codes and specific charges. These included cancer, chemotherapy/radiation, prior VTE, use of estrogens and estrogen modulators, inflammatory bowel disease, nephrotic syndrome, myeloproliferative disorders, smoking, central venous catheter, inherited or acquired thrombophilia, mechanical ventilation, urinary catheter, decubitus ulcer, 3‐hydroxy‐3‐methylglutaryl coenzyme A (HMG‐CoA) reductase inhibitors, restraints, and varicose veins. Hospitals were categorized by region (Northeast, South, Midwest, or West), bed size, setting (urban vs rural), and teaching status.
Outcome Variables
We defined hospital‐acquired VTE as a secondary diagnosis of VTE (ICD‐9‐CM diagnoses 453.4, 453.40, 453.41, 453.42, 453.8, 453.9, 415.1, 415.11, 415.19), combined with a diagnostic test for VTE (lower extremity ultrasound, venography, computed tomography (CT) angiogram, ventilation‐perfusion scan, or pulmonary angiogram) after hospital day 2, followed by treatment for VTE (intravenous unfractionated heparin, >60 mg of enoxaparin, 7500 mg of dalteparin, or placement of an inferior vena cava filter) for at least 50% of the remaining hospital days or until initiation of warfarin or appearance of a complication (eg, transfusion or treatment for heparin‐induced thrombocytopenia). We chose this definition to differentiate hospital‐acquired VTE from VTE present on admission.12 In addition, patients who were readmitted within 30 days of discharge with a primary diagnosis of VTE were also considered to have hospital‐acquired VTE.
We also assessed complications of VTE prophylaxis. Major bleeding was defined as the receipt of 2 or more units of packed red blood cells on a single day or a secondary diagnosis of intracranial bleeding. Because there was no ICD‐9‐CM code for HIT, we assessed codes for all thrombocytopenia, as well as secondary thrombocytopenia. Definite HIT was defined as an ICD‐9‐CM code for thrombocytopenia, together with discontinuation of heparin and initiation of treatment with argatroban. A definite complication was defined as HIT or evidence of major bleeding coupled with discontinuation of heparin. Finally, we evaluated all‐cause in‐hospital mortality and total hospital costs.
Statistical Analysis
We computed summary statistics using frequencies and percents for categorical variables, and means, medians, and standard deviations and interquartile range for continuous variables. Associations of prophylaxis type with patient and hospital characteristics and outcomes were assessed using chi‐square tests or Fisher's exact test for categorical variables, and z‐tests or Wilcoxon tests for continuous variables.
We developed a propensity model for treatment with UFH as the outcome; the model included patient characteristics, early treatments, comorbidities, risk factors for VTE, physician specialty, and selected interaction terms. We then developed a series of multivariable models to evaluate the impact of heparin choice on the risk of VTE, complications of treatment, mortality, and total cost. Generalized estimating equation models with a logit link were used to assess the association between the choice of heparin and the risk of VTE, and of complications and mortality, while adjusting for the effects of within‐hospital correlation; identity link models were used for analyses of cost. Costs were trimmed at 3 standard deviations above the mean, and natural log‐transformed values were modeled due to extreme positive skew.
Unadjusted and covariate‐adjusted models were evaluated with and without adjustments for propensity score. In addition, since the hospital was the single strongest predictor of treatment, we developed grouped treatment models, in which a patient's actual treatment was replaced by a probability equal to the proportion of prophylaxed patients receiving UFH at that hospital. This adaptation of instrumental variable analysis uses the hospital as the instrument, and attempts to assess whether patients treated at a hospital which uses UFH more frequently have outcomes that differ from those of patients treated at hospitals which use LMWH more frequently, while adjusting for other patient, physician, and hospital variables. By relying on treatment at the hospital level, this method reduces the opportunity for selection bias at the patient level.
Finally, in order to exclude the possibility that our surrogate bleeding outcome was due to transfusion practices at hospitals that use a particular form of heparin, we compared the hospital rates of transfusion of 2 or more units of packed red cells to the hospital rates of prophylaxis with UFH in a larger dataset of the same hospitals. This set included patients with congestive heart failure, stroke, pneumonia, and urinary tract infection who did not receive daily prophylaxis, as well as patients admitted for chronic obstructive pulmonary disease (COPD) or acute myocardial infarction, and patients who received either warfarin or a treatment dose of heparin in the first 2 hospital days. We also compared the transfusion rates at hospitals that used unfractionated heparin in 80% of patients to hospitals that used LMWH in 80%. All analyses were performed using SAS version 9.1 (SAS Institute Inc, Cary, NC).
RESULTS
Our final sample included 32,104 patients who received prophylaxis at 333 hospitals (see Supporting Information, e‐Figure, in the online version of this article). Patient characteristics appear in Table 1. Most patients (66%) were over age 65; 59% were female and 61% were white. The most common primary diagnoses were pneumonia (40%) and congestive heart failure (25%). Additional risk factors for thromboembolism included cancer (13%), paralysis (8%), or diabetes (35%). Most patients' attending physicians were either internists (61%) or family practitioners (14%). Almost half of the patients were cared for at hospitals in the South (46%).
Total | UFH | LMWH | ||
---|---|---|---|---|
32,104 (100) | 14,591 (45.4) | 17,513 (54.6) | ||
N (%) | N (%) | N (%) | P | |
| ||||
Demographics | ||||
Age | 0.0002 | |||
1849 | 4,061 (12.7) | 1,950 (13.4) | 2,111 (12.1) | |
5064 | 6,962 (21.7) | 3,225 (22.1) | 3,737 (21.3) | |
6579 | 10,909 (34.0) | 4,921 (33.7) | 5,988 (34.2) | |
80+ | 10,172 (31.7) | 4,495 (30.8) | 5,677 (32.4) | |
Sex | 0.0071 | |||
Male | 13,234 (41.2) | 6,133 (42.0) | 7,101 (40.5) | |
Female | 18,870 (58.8) | 8,458 (58.0) | 10,412 (59.5) | |
Race/ethnicity | <0.0001 | |||
White | 19,489 (60.7) | 8,063 (55.3) | 11,426 (65.2) | |
Black | 7,429 (23.1) | 4,101 (28.1) | 3,328 (19.0) | |
Hispanic | 1,304 (4.1) | 591 (4.1) | 713 (4.1) | |
Other | 3,882 (12.1) | 1,836 (12.6) | 2,046 (11.7) | |
Primary diagnosis | <0.0001 | |||
Pneumonia | 12,768 (39.8) | 5,354 (36.7) | 7,414 (42.3) | |
Sepsis* | 1,217 (3.8) | 562 (3.9) | 655 (3.7) | |
Respiratory failure* | 2,017 (6.3) | 814 (5.6) | 1,203 (6.9) | |
Heart failure | 8,157 (25.4) | 3,825 (26.2) | 4,332 (24.7) | |
Stroke | 4,416 (13.8) | 2,295 (15.7) | 2,121 (12.1) | |
Urinary tract infection | 3,529 (11.0) | 1,741 (11.9) | 1,788 (10.2) | |
Attending specialty | <0.0001 | |||
Internist | 19,511 (60.8) | 8,945 (61.3) | 10,566 (60.3) | |
General practice/Family medicine | 4,326 (13.5) | 1,964 (13.5) | 2,362 (13.5) | |
Cardiologist | 1,606 (5.0) | 730 (5.0) | 876 (5.0) | |
Pulmonologist | 2,179 (6.8) | 854 (5.9) | 1,325 (7.6) | |
Nephrology | 583 (1.8) | 380 (2.6) | 203 (1.2) | |
Critical care/Intensivist | 150 (0.5) | 93 (0.6) | 57 (0.3) | |
Other | 3,749 (11.7) | 1,625 (11.1) | 2,124 (12.1) | |
Insurance | <0.0001 | |||
Medicare traditional | 20,281 (63.2) | 8,929 (61.2) | 11,352 (64.8) | |
Medicare managed care | 1,737 (5.4) | 826 (5.7) | 911 (5.2) | |
Medicaid | 2,629 (8.2) | 1,401 (9.6) | 1,228 (7.0) | |
Private | 5,967 (18.6) | 2,830 (19.4) | 3,137 (17.9) | |
Self‐pay/uninsured/other | 1,490 (4.6) | 605 (4.1) | 885 (5.1) | |
Risk factors for VTE | ||||
Admit from skilled nursing facility | 476 (1.5) | 277 (1.9) | 199 (1.1) | <0.0001 |
Paralysis | 2,608 (8.1) | 1,317 (9.0) | 1,291 (7.4) | <0.0001 |
Restraints | 417 (1.3) | 147 (1.0) | 270 (1.5) | <0.0001 |
Decubitus ulcer | 1,190 (3.7) | 631 (4.3) | 559 (3.2) | <0.0001 |
Cancer | 4,154 (12.9) | 1,858 (12.7) | 2,296 (13.1) | 0.3171 |
Chemotherapy | 86 (0.3) | 41 (0.3) | 45 (0.3) | 0.6781 |
Prior venous thromboembolism | 494 (1.5) | 202 (1.4) | 292 (1.7) | 0.0403 |
Pregnancy | 1 (0) | 1 (0) | 0 (0) | 0.2733 |
Estrogens | 438 (1.4) | 143 (1.0) | 295 (1.7) | <0.0001 |
Estrogen modulators | 246 (0.8) | 80 (0.5) | 166 (0.9) | <0.0001 |
Congestive heart failure | 3,107 (9.7) | 1,438 (9.9) | 1,669 (9.5) | 0.3263 |
Respiratory failure | 2,210 (6.9) | 1,037 (7.1) | 1,173 (6.7) | 0.1493 |
Inflammatory bowel disease | 108 (0.3) | 41 (0.3) | 67 (0.4) | 0.1176 |
Nephrotic syndrome | 92 (0.3) | 50 (0.3) | 42 (0.2) | 0.0860 |
Myeloproliferative disorder | 198 (0.6) | 68 (0.5) | 130 (0.7) | 0.0016 |
Obesity | 2,973 (9.3) | 1,211 (8.3) | 1,762 (10.1) | <0.0001 |
Smoking | 4,476 (13.9) | 1,887 (12.9) | 2,589 (14.8) | <0.0001 |
Varicose veins | 19 (0.1) | 6 (0) | 13 (0.1) | 0.2245 |
Central line | 1,070 (3.3) | 502 (3.4) | 568 (3.2) | 0.3271 |
Inherited or acquired thrombophilia | 16 (0) | 9 (0.1) | 7 (0) | 0.3855 |
Diabetes | 11,136 (34.7) | 5,157 (35.3) | 5,979 (34.1) | 0.0241 |
Procedures associated with VTE or bleed | ||||
Mechanical ventilation | 2,282 (7.1) | 1,111 (7.6) | 1,171 (6.7) | 0.0013 |
Urinary catheter | 4,496 (14.0) | 1,545 (10.6) | 2,951 (16.9) | <0.0001 |
Aspirin | 12,865 (40.1) | 6,101 (41.8) | 6,764 (38.6) | <0.0001 |
Clopidogrel | 4,575 (14.3) | 2,087 (14.3) | 2,488 (14.2) | 0.8050 |
Non‐steroidal anti‐inflammatory drugs | 2,147 (6.7) | 867 (5.9) | 1,280 (7.3) | <0.0001 |
Steroids | 7,938 (24.7) | 3,136 (21.5) | 4,802 (27.4) | <0.0001 |
Statins | 7,376 (23.0) | 3,462 (23.7) | 3,914 (22.3) | 0.0035 |
Comorbidities | ||||
AIDS | 124 (0.4) | 73 (0.5) | 51 (0.3) | 0.0026 |
Alcohol abuse | 1,048 (3.3) | 523 (3.6) | 525 (3.0) | 0.0032 |
Deficiency anemia | 7,010 (21.8) | 3,228 (22.1) | 3,782 (21.6) | 0.2543 |
Rheumatoid arthritis/collagen vas | 967 (3.0) | 426 (2.9) | 541 (3.1) | 0.3762 |
Chronic blood loss anemia | 177 (0.6) | 79 (0.5) | 98 (0.6) | 0.8269 |
Chronic pulmonary disease | 12,418 (38.7) | 5,314 (36.4) | 7,104 (40.6) | <0.0001 |
Depression | 3,334 (10.4) | 1433 (9.8) | 1901 (10.9) | 0.0025 |
Drug abuse | 694 (2.2) | 412 (2.8) | 282 (1.6) | <0.0001 |
Hypertension | 16,979 (52.9) | 7,658 (52.5) | 9,321 (53.2) | 0.1866 |
Hypothyroidism | 4,016 (12.5) | 1,716 (11.8) | 2,300 (13.1) | 0.0002 |
Liver disease | 453 (1.4) | 227 (1.6) | 226 (1.3) | 0.0448 |
Other neurological disorders | 4,682 (14.6) | 2,202 (15.1) | 2,480 (14.2) | 0.0187 |
Peripheral vascular disease | 2,134 (6.6) | 980 (6.7) | 1,154 (6.6) | 0.6490 |
Psychoses | 1,295 (4.0) | 574 (3.9) | 721 (4.1) | 0.4066 |
Pulmonary circulation disease | 1,034 (3.2) | 442 (3.0) | 592 (3.4) | 0.0760 |
Renal failure | 2,794 (8.7) | 1,636 (11.2) | 1,158 (6.6) | 0.0000 |
Peptic ulcer disease with bleeding | 563 (1.8) | 232 (1.6) | 331 (1.9) | 0.0414 |
Valvular disease | 2,079 (6.5) | 899 (6.2) | 1,180 (6.7) | 0.0366 |
Weight loss | 1,231 (3.8) | 556 (3.8) | 675 (3.9) | 0.8391 |
Other prophylaxis | ||||
Intermittent pneumatic compression | 1,003 (3.1) | 456 (3.1) | 547 (3.1) | 0.9926 |
Mechanical prophylaxis | 1,281 (4.0) | 524 (3.6) | 757 (4.3) | 0.0009 |
Fifty‐five percent of patients received LMWH and the remainder received UFH; 1274 (4%) patients switched type of heparin during their stay. The proportion of patients receiving LMWH at an individual hospital varied from 0% to 100% with a u‐shaped distribution, with almost one‐third of hospitals prescribing one treatment or the other exclusively (Figure 1). Similarly, the proportion of an individual physician's patients who received prophylaxis with UFH (vs LMWH) varied from 0% to 100% (Figure 1), with 51% prescribing LMWH exclusively and 31% prescribing UFH exclusively. Compared to patients who received UFH, patients receiving LMWH were older and were more likely to be white, female, and to have pneumonia. By far the biggest difference between the groups was the hospitals at which they received their care (see Supporting Information, e‐Table, in the online version of this article). Patients receiving LMWH were much more likely to be from smaller, rural, non‐teaching hospitals in the South or the West. There were also numerous small differences in comorbidities and individual VTE risk factors between the 2 groups. The only large difference was that patients with a secondary diagnosis of renal failure (for which LMWH is not US Food and Drug Administration [FDA] approved) were almost twice as likely to receive UFH.
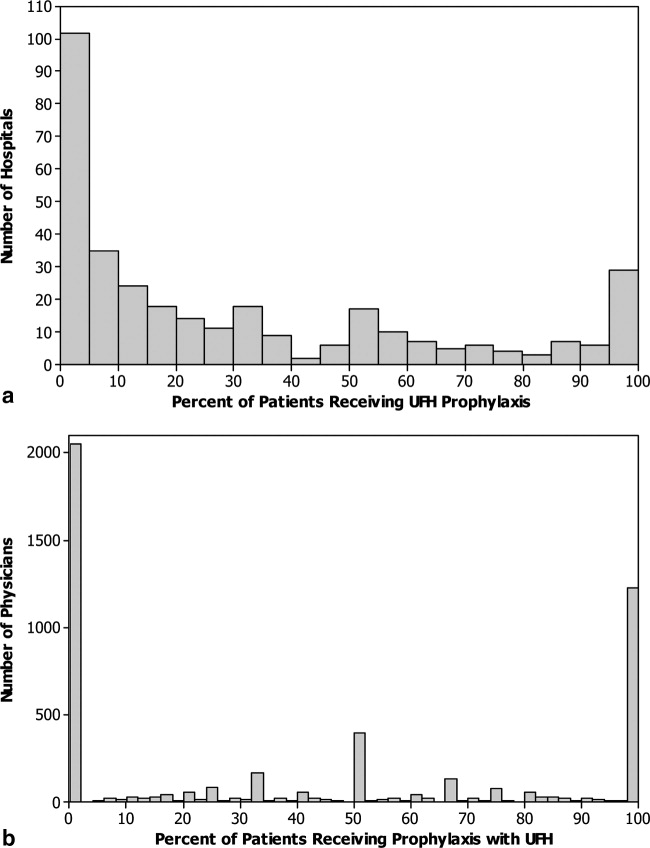
We identified 163 (0.51%) episodes of VTE (Table 2). Compared to patients receiving UFH, those receiving standard LMWH had similar unadjusted rates of VTE (0.53% vs 0.48%; P = 0.54), major bleeding (0.77% vs 0.76%; P = 0.88), thrombocytopenia (1.9% vs 2.0%; P = 0.48), definite HIT (n = 1 vs n = 3; P = 0.34), and mortality (2.8% vs 3.1%; P = 0.07). Definite complications of prophylaxis (HIT or major bleed combined with the discontinuation of heparin) were more common among patients receiving UFH (0.2% vs 0.1%; P = 0.022). Patients treated with UFH had longer unadjusted lengths of stay (P < 0.0001) and higher unadjusted costs (P < 0.0001).
Total | UFH | LMWH | P | |
---|---|---|---|---|
32,104 (100) | 14,591 (45.4) | 17,513 (54.6) | ||
n (%) | n (%) | n (%) | ||
| ||||
Venous thromboembolism | 163 (0.5) | 78 (0.5) | 85 (0.5) | 0.54 |
Heparin‐induced thrombocytopenia | 4 (0) | 3 (0) | 1 (0) | 0.34* |
Any major bleeding | 246 (0.8) | 113 (0.8) | 133 (0.8) | 0.88 |
Transfusion with 2 units of packed red blood cells | 218 (0.7) | 97 (0.7) | 121 (0.7) | 0.78 |
Intracranial hemorrhage | 30 (0.1) | 17 (0.1) | 13 (0.1) | 0.22 |
Complication resulting in stopping heparin | 44 (0.1) | 28 (0.2) | 16 (0.1) | 0.02 |
In‐hospital mortality | 944 (2.9) | 456 (3.1) | 488 (2.8) | 0.07 |
LOS in days; mean (SD) | 6.2 (5.9) | 6.4 (6.2) | 6.0 (5.6) | <0.001 |
Median (IQR) | 5 (37) | 5 (37) | 5 (37) | |
Cost in USD; median (IQR) | 5873 (41718982) | 6007 (41779456) | 5774 (41658660) | <0.001 |
A propensity model for UFH treatment based upon patient characteristics and treatments was not strongly predictive of treatment (c = 0.58) and propensity matching failed to balance many of the patient characteristics. However, hospital alone, ignoring patient characteristics was strongly predictive (c = 0.91) of treatment.
In a model adjusting only for clustering within hospitals, patients treated with UFH had an odds ratio (OR) for VTE of 1.08 (95% confidence interval [CI] 0.79 to 1.49) compared to patients receiving LMWH (Figure 2). Adjustment for propensity for UFH and other covariates attenuated the effect of LMWH (OR 1.04, 95% CI 0.76 to 1.43). When individual patients were assigned a probability of treatment with UFH equal to the hospital rate where they received care, UFH use was associated with a nonsignificant change in the odds of VTE (OR 1.14, 95% CI 0.72 to 1.81).
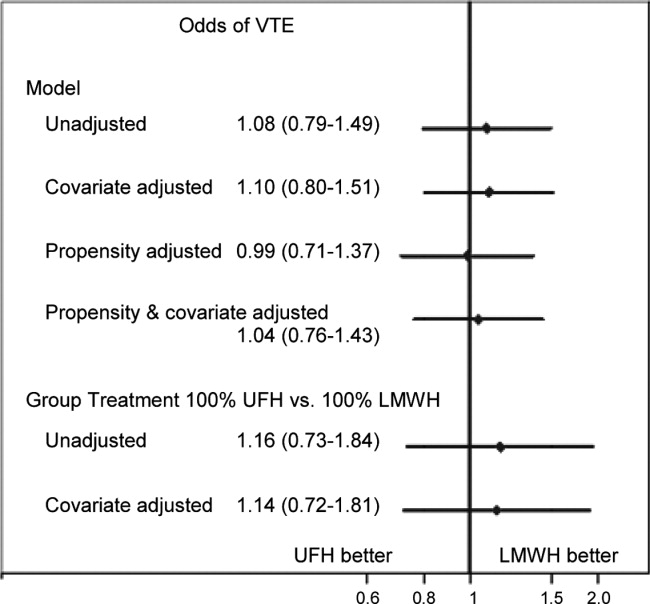
Adjusted for clustering within hospital only, patients treated with UFH had an odds ratio for major bleed of 1.38 (95% CI 1.00 to 1.91) compared to patients receiving LMWH (Figure 3). Adjustment for propensity for UFH and other covariates gave similar results (OR 1.34, 95% CI 0.97 to 1.84). When individual patients were assigned a probability of treatment with UFH equal to the hospital rate where they received care, UFH treatment was associated with a nonsignificant increase in the odds of major bleed (OR 1.64, 95% CI 0.50 to 5.33). When we compared the rate of transfusion across hospitals, including 576,231 additional patients who were excluded from the original analyses because they did not receive daily prophylaxis or had a diagnosis of myocardial infarction or COPD, there was a slight negative correlation between transfusion rates and use of UFH (Spearman Correlation Coefficient 0.03; P = 0.61). Hospitals that used primarily UFH had a transfusion rate of 0.60% versus 0.76% at hospitals using primarily LMWH (P = 0.54), indicating that the increased risk of major bleeding associated with UFH was not confounded by local transfusion practices.
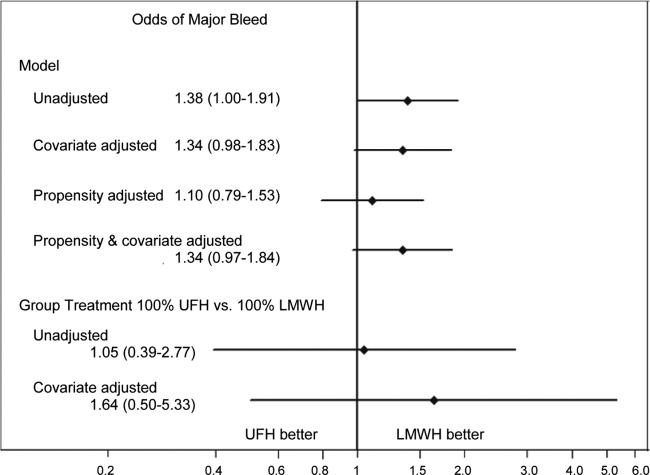
Adjusted for clustering only, patients treated with UFH had an odds ratio for definite complication of 2.35 (95% CI 1.17 to 4.72) compared to those treated with LMWH. Adjustment for propensity and covariates accentuated the association (OR 2.84, 95% CI 1.43 to 5.66). When assigned a probability of treatment with UFH equal to the hospital rate where they received care, UFH treatment was associated with an increase in the risk of definite complication (OR 2.79, 95% CI 1.00 to 7.74).
Adjusted for clustering only, patients treated with UFH had higher costs than those treated with LMWH (cost ratio 1.07, 95% CI 1.05 to 1.09). Adjustment for propensity for UFH and other covariates attenuated the association (cost ratio 1.02, 95% CI 1.00 to 1.03). Finally, when individual patients were assigned a probability of initial treatment with UFH equal to the hospital rate where they received care, treatment with UFH was associated with a nonsignificant change in the relative cost (cost ratio 0.97, 95% CI 0.90 to 1.05).
DISCUSSION
In this retrospective cohort study, we found that low‐molecular‐weight heparin and unfractionated heparin were associated with similar rates of VTE in moderate‐to‐high risk medical patients. However, unfractionated heparin was associated with a small, but higher risk of complications, even after adjustment. There were no statistical differences in rates of heparin‐induced thrombocytopenia, but this complication was exceedingly rare. Finally, overall costs associated with both treatments were similar.
A number of industry‐funded studies have compared LMWH to UFH in randomized clinical trials. These trials have generally been small and used endpoints of uncertain significance, such as asymptomatic deep vein thrombosis assessed by ultrasound. At least 3 meta‐analyses of these trials have been published. Each used different inclusion criteria. The only one to find an efficacy benefit to LMWH over UFH was heavily influenced by the inclusion of a number of studies of stroke patients.3 In that study, LMWH reduced VTE by approximately one‐third relative to UFH. The other 2 analyses found smaller reductions in DVT and pulmonary embolism (PE), and these results were not statistically significant.5, 8 Similarly, 1 analysis5 found a reduction in major bleeding events with LMWH versus UFH, whereas the other 2 studies found smaller reductions which were not statistically significant. The assessment of major bleeding is further complicated by differences in the definition of major bleeding across studies. Using a standard definition of 2 units of packed red blood cells transfused in 1 day to denote major bleeding, we found an associated reduction in bleeding with LMWH that was similar to that observed in the meta‐analyses. Moreover, patients receiving UFH were twice as likely to have a complication that resulted in stopping the prophylaxis, although these complications were overall quite rare. Lastly, there are no cost comparisons based on randomized trials. Several comparisons based on modeling have favored LMWH. One assumed that 3% of patients receiving UFH would develop HIT;13 something we did not observe. At least 3 additional analyses,1416 all funded by the manufacturer of enoxaparin, assumed that LMWH was both more effective and safer than UFH. We found that adjusted costs were similar or slightly lower with UFH than LMWH.
Our study has a number of limitations. First, its observational design makes it vulnerable to selection bias. We attempted to overcome this with rigorous multivariable adjustment, including the propensity for treatment and by using an adaptation of the instrumental variable approach. This method is of particular interest because individual hospitals were strongly predictive of choice of heparin. Still, we cannot exclude the possibility of residual confounding, especially if other outcomes, such as transfusion decisions, were also tied to specific hospital practices. Second, our study used administrative data, and therefore we could not directly adjust for certain differences which may exist between patients who received LMWH and those who received UFH. However, we did adjust for many classic risk factors for VTE. More importantly, it seems that the chance of being treated with a particular form of heparin depends more on the hospital where one receives care than on any combination of patient characteristics. Thus, apart from renal failure, for which we adjusted, it seems unlikely that there were major differences in unmeasured physiological confounders. Third, we limited our analysis to patients who received standard dosing of either type of heparin. We did this to bolster the validity of our findings, but they may not apply to unconventional dosing often observed in clinical practice. Fourth, we measured only outcomes that occurred in the hospital or that prompted a return to the hospital. VTEs which were diagnosed and treated in ambulatory care were not included. While this may have led us to underestimate the true risk of VTE, we have little reason to believe that the choice of whether to admit a patient with VTE is influenced by the original choice of VTE prophylaxis. Finally, our study was conducted before the introduction of generic LMWH, which would be expected to reduce costs associated with LMWH prophylaxis.
VTE prophylaxis for medical patients has emerged as a major focus for quality improvement initiatives. As a result, a significant proportion of general medical patients receive some form of chemoprophylaxis during their hospital stay. Small differences in efficacy or safety of different forms of prophylaxis multiplied by millions of admissions each year can have profound effects on the health of hospitalized patients. Similarly, differences in cost could also have a substantial impact on the healthcare system. We found no difference in efficacy or cost, but treatment with LMWH was less likely to be associated with subsequent transfusion of 2 or more units of packed red blood cells, a surrogate marker for bleeding. In addition, LMWH is more convenient since it can be dosed once daily, and for that reason may be more acceptable to patients. For these reasons, LMWH may be the drug of choice for inpatient prophylaxis of general medical patients. In situations where the cost of the medication itself is important, UFH represents an equally effective alternative.
Acknowledgements
All authors have contributed sufficiently to this study and have provided written permission to be named in the manuscript. No other persons have made substantial contributions to this manuscript. Michael B. Rothberg is the guarantor of the entire manuscript.
Disclosures: This study was supported by a Clinical Scientist Development Award from the Doris Duke Charitable Foundation. The funding source had no role in the study design, analysis, or interpretation of the data. Dr Rothberg served for 1 day as a consultant to Novartis Pharma about an influenza vaccine model. Sandoz, a division of Novartis, was recently granted approval to manufacture a generic form of low‐molecular‐weight heparin. None of the other authors have any conflicts of interest.
Venous thromboembolism (VTE) is a major source of morbidity and mortality for hospitalized patients, with as many as 16% of high‐risk medical patients developing VTE during their hospital stay.1, 2 Pharmacologic prophylaxis with subcutaneous heparin reduces the risk of VTE by approximately 50%,3, 4 and guidelines produced by the American College of Chest Physicians (ACCP) recommend thromboprophylaxis for patients at moderate‐to‐high risk of VTE with either low‐molecular‐weight heparin (LMWH) or unfractionated heparin (UFH).2 UFH is less expensive per dose, but meta‐analyses have suggested that UFH may be either less effective than LMWH3 or more likely to cause complications, such as bleeding5 or heparin‐induced thrombocytopenia (HIT).6 Others have argued that the efficacy and risk of bleeding with UFH and LMWH are similar.7, 8 In either case, there are few head‐to‐head studies of LMWH and UFH in medical patients and they tend to be small. In the most recent meta‐analysis, which included fewer than 4500 patients, several different low‐molecular‐weight heparins were assessed together, and the observed rate of deep vein thrombosis (DVT) with UFH was high (5.4%), with evidence suggesting publication bias.3
Given the current Joint Commission requirement9 that all medical patients either receive VTE prophylaxis or have documented a reason not to, the implications related to choosing one form of VTE prophylaxis over another are substantial on a national scale. In order to compare the effectiveness of UFH and LMWH in routine practice among hospitalized medical patients, we conducted a retrospective cohort study in a national sample of hospitals and compared the risk of VTE, bleeding, HIT, and death associated with each treatment.
METHODS
Setting and Patients
We conducted a retrospective cohort study of patients discharged between January 1, 2004 and June 30, 2005 from 333 acute care facilities in the United States that participated in Premier's Perspective, a database we have described previously.10 Compared to US hospitals as a whole, Perspective hospitals are more likely to be located in the South and in urban areas. Perspective contains the following data elements: sociodemographic information, International Classification of Diseases, Ninth Revision, Clinical Modification (ICD‐9‐CM) diagnosis and procedure codes, as well as a list of all billed items with a date of service, including diagnostic tests, medications, and other treatments. Hospitals' characteristics include size, region, setting, and teaching status. The Institutional Review Board at Baystate Medical Center granted permission to conduct the study (#132280‐1).
We included general medical patients aged 18 years whose ICD‐9‐CM primary diagnosis code (congestive heart failure, stroke, pneumonia, and urinary tract infection) placed them at moderate‐to‐high risk of VTE according to the ACCP recommendations,2 and who received daily prophylactic dosages of either LMWH (40 mg daily) or UFH (10,00015,000 units daily) initiated by hospital day 2 and continued to discharge or until the patient developed a VTE or a complication attributable to heparin. Patients were included so long as they missed no more than 1 day of prophylaxis or had no more than 1 unusual dose recorded. Patients who switched between heparin types were included and analyzed according to their initial therapy. Patients who received any other regimen were excluded. We also excluded patients who received warfarin on hospital day 1 or 2, because they would not be considered candidates for heparin prophylaxis, and patients whose length of stay was 2 days, because the value of VTE prophylaxis in such cases is unknown.
Data Elements
For each patient, we extracted age, gender, race, and insurance status, principal diagnosis, comorbidities, and specialty of the attending physician. Comorbidities were identified from ICD‐9‐CM secondary diagnosis codes and Diagnosis Related Groups using Healthcare Cost and Utilization Project Comorbidity Software, version 3.1, based on the work of Elixhauser and colleagues.11 We also identified additional risk factors for VTE using a combination of ICD‐9‐CM codes and specific charges. These included cancer, chemotherapy/radiation, prior VTE, use of estrogens and estrogen modulators, inflammatory bowel disease, nephrotic syndrome, myeloproliferative disorders, smoking, central venous catheter, inherited or acquired thrombophilia, mechanical ventilation, urinary catheter, decubitus ulcer, 3‐hydroxy‐3‐methylglutaryl coenzyme A (HMG‐CoA) reductase inhibitors, restraints, and varicose veins. Hospitals were categorized by region (Northeast, South, Midwest, or West), bed size, setting (urban vs rural), and teaching status.
Outcome Variables
We defined hospital‐acquired VTE as a secondary diagnosis of VTE (ICD‐9‐CM diagnoses 453.4, 453.40, 453.41, 453.42, 453.8, 453.9, 415.1, 415.11, 415.19), combined with a diagnostic test for VTE (lower extremity ultrasound, venography, computed tomography (CT) angiogram, ventilation‐perfusion scan, or pulmonary angiogram) after hospital day 2, followed by treatment for VTE (intravenous unfractionated heparin, >60 mg of enoxaparin, 7500 mg of dalteparin, or placement of an inferior vena cava filter) for at least 50% of the remaining hospital days or until initiation of warfarin or appearance of a complication (eg, transfusion or treatment for heparin‐induced thrombocytopenia). We chose this definition to differentiate hospital‐acquired VTE from VTE present on admission.12 In addition, patients who were readmitted within 30 days of discharge with a primary diagnosis of VTE were also considered to have hospital‐acquired VTE.
We also assessed complications of VTE prophylaxis. Major bleeding was defined as the receipt of 2 or more units of packed red blood cells on a single day or a secondary diagnosis of intracranial bleeding. Because there was no ICD‐9‐CM code for HIT, we assessed codes for all thrombocytopenia, as well as secondary thrombocytopenia. Definite HIT was defined as an ICD‐9‐CM code for thrombocytopenia, together with discontinuation of heparin and initiation of treatment with argatroban. A definite complication was defined as HIT or evidence of major bleeding coupled with discontinuation of heparin. Finally, we evaluated all‐cause in‐hospital mortality and total hospital costs.
Statistical Analysis
We computed summary statistics using frequencies and percents for categorical variables, and means, medians, and standard deviations and interquartile range for continuous variables. Associations of prophylaxis type with patient and hospital characteristics and outcomes were assessed using chi‐square tests or Fisher's exact test for categorical variables, and z‐tests or Wilcoxon tests for continuous variables.
We developed a propensity model for treatment with UFH as the outcome; the model included patient characteristics, early treatments, comorbidities, risk factors for VTE, physician specialty, and selected interaction terms. We then developed a series of multivariable models to evaluate the impact of heparin choice on the risk of VTE, complications of treatment, mortality, and total cost. Generalized estimating equation models with a logit link were used to assess the association between the choice of heparin and the risk of VTE, and of complications and mortality, while adjusting for the effects of within‐hospital correlation; identity link models were used for analyses of cost. Costs were trimmed at 3 standard deviations above the mean, and natural log‐transformed values were modeled due to extreme positive skew.
Unadjusted and covariate‐adjusted models were evaluated with and without adjustments for propensity score. In addition, since the hospital was the single strongest predictor of treatment, we developed grouped treatment models, in which a patient's actual treatment was replaced by a probability equal to the proportion of prophylaxed patients receiving UFH at that hospital. This adaptation of instrumental variable analysis uses the hospital as the instrument, and attempts to assess whether patients treated at a hospital which uses UFH more frequently have outcomes that differ from those of patients treated at hospitals which use LMWH more frequently, while adjusting for other patient, physician, and hospital variables. By relying on treatment at the hospital level, this method reduces the opportunity for selection bias at the patient level.
Finally, in order to exclude the possibility that our surrogate bleeding outcome was due to transfusion practices at hospitals that use a particular form of heparin, we compared the hospital rates of transfusion of 2 or more units of packed red cells to the hospital rates of prophylaxis with UFH in a larger dataset of the same hospitals. This set included patients with congestive heart failure, stroke, pneumonia, and urinary tract infection who did not receive daily prophylaxis, as well as patients admitted for chronic obstructive pulmonary disease (COPD) or acute myocardial infarction, and patients who received either warfarin or a treatment dose of heparin in the first 2 hospital days. We also compared the transfusion rates at hospitals that used unfractionated heparin in 80% of patients to hospitals that used LMWH in 80%. All analyses were performed using SAS version 9.1 (SAS Institute Inc, Cary, NC).
RESULTS
Our final sample included 32,104 patients who received prophylaxis at 333 hospitals (see Supporting Information, e‐Figure, in the online version of this article). Patient characteristics appear in Table 1. Most patients (66%) were over age 65; 59% were female and 61% were white. The most common primary diagnoses were pneumonia (40%) and congestive heart failure (25%). Additional risk factors for thromboembolism included cancer (13%), paralysis (8%), or diabetes (35%). Most patients' attending physicians were either internists (61%) or family practitioners (14%). Almost half of the patients were cared for at hospitals in the South (46%).
Total | UFH | LMWH | ||
---|---|---|---|---|
32,104 (100) | 14,591 (45.4) | 17,513 (54.6) | ||
N (%) | N (%) | N (%) | P | |
| ||||
Demographics | ||||
Age | 0.0002 | |||
1849 | 4,061 (12.7) | 1,950 (13.4) | 2,111 (12.1) | |
5064 | 6,962 (21.7) | 3,225 (22.1) | 3,737 (21.3) | |
6579 | 10,909 (34.0) | 4,921 (33.7) | 5,988 (34.2) | |
80+ | 10,172 (31.7) | 4,495 (30.8) | 5,677 (32.4) | |
Sex | 0.0071 | |||
Male | 13,234 (41.2) | 6,133 (42.0) | 7,101 (40.5) | |
Female | 18,870 (58.8) | 8,458 (58.0) | 10,412 (59.5) | |
Race/ethnicity | <0.0001 | |||
White | 19,489 (60.7) | 8,063 (55.3) | 11,426 (65.2) | |
Black | 7,429 (23.1) | 4,101 (28.1) | 3,328 (19.0) | |
Hispanic | 1,304 (4.1) | 591 (4.1) | 713 (4.1) | |
Other | 3,882 (12.1) | 1,836 (12.6) | 2,046 (11.7) | |
Primary diagnosis | <0.0001 | |||
Pneumonia | 12,768 (39.8) | 5,354 (36.7) | 7,414 (42.3) | |
Sepsis* | 1,217 (3.8) | 562 (3.9) | 655 (3.7) | |
Respiratory failure* | 2,017 (6.3) | 814 (5.6) | 1,203 (6.9) | |
Heart failure | 8,157 (25.4) | 3,825 (26.2) | 4,332 (24.7) | |
Stroke | 4,416 (13.8) | 2,295 (15.7) | 2,121 (12.1) | |
Urinary tract infection | 3,529 (11.0) | 1,741 (11.9) | 1,788 (10.2) | |
Attending specialty | <0.0001 | |||
Internist | 19,511 (60.8) | 8,945 (61.3) | 10,566 (60.3) | |
General practice/Family medicine | 4,326 (13.5) | 1,964 (13.5) | 2,362 (13.5) | |
Cardiologist | 1,606 (5.0) | 730 (5.0) | 876 (5.0) | |
Pulmonologist | 2,179 (6.8) | 854 (5.9) | 1,325 (7.6) | |
Nephrology | 583 (1.8) | 380 (2.6) | 203 (1.2) | |
Critical care/Intensivist | 150 (0.5) | 93 (0.6) | 57 (0.3) | |
Other | 3,749 (11.7) | 1,625 (11.1) | 2,124 (12.1) | |
Insurance | <0.0001 | |||
Medicare traditional | 20,281 (63.2) | 8,929 (61.2) | 11,352 (64.8) | |
Medicare managed care | 1,737 (5.4) | 826 (5.7) | 911 (5.2) | |
Medicaid | 2,629 (8.2) | 1,401 (9.6) | 1,228 (7.0) | |
Private | 5,967 (18.6) | 2,830 (19.4) | 3,137 (17.9) | |
Self‐pay/uninsured/other | 1,490 (4.6) | 605 (4.1) | 885 (5.1) | |
Risk factors for VTE | ||||
Admit from skilled nursing facility | 476 (1.5) | 277 (1.9) | 199 (1.1) | <0.0001 |
Paralysis | 2,608 (8.1) | 1,317 (9.0) | 1,291 (7.4) | <0.0001 |
Restraints | 417 (1.3) | 147 (1.0) | 270 (1.5) | <0.0001 |
Decubitus ulcer | 1,190 (3.7) | 631 (4.3) | 559 (3.2) | <0.0001 |
Cancer | 4,154 (12.9) | 1,858 (12.7) | 2,296 (13.1) | 0.3171 |
Chemotherapy | 86 (0.3) | 41 (0.3) | 45 (0.3) | 0.6781 |
Prior venous thromboembolism | 494 (1.5) | 202 (1.4) | 292 (1.7) | 0.0403 |
Pregnancy | 1 (0) | 1 (0) | 0 (0) | 0.2733 |
Estrogens | 438 (1.4) | 143 (1.0) | 295 (1.7) | <0.0001 |
Estrogen modulators | 246 (0.8) | 80 (0.5) | 166 (0.9) | <0.0001 |
Congestive heart failure | 3,107 (9.7) | 1,438 (9.9) | 1,669 (9.5) | 0.3263 |
Respiratory failure | 2,210 (6.9) | 1,037 (7.1) | 1,173 (6.7) | 0.1493 |
Inflammatory bowel disease | 108 (0.3) | 41 (0.3) | 67 (0.4) | 0.1176 |
Nephrotic syndrome | 92 (0.3) | 50 (0.3) | 42 (0.2) | 0.0860 |
Myeloproliferative disorder | 198 (0.6) | 68 (0.5) | 130 (0.7) | 0.0016 |
Obesity | 2,973 (9.3) | 1,211 (8.3) | 1,762 (10.1) | <0.0001 |
Smoking | 4,476 (13.9) | 1,887 (12.9) | 2,589 (14.8) | <0.0001 |
Varicose veins | 19 (0.1) | 6 (0) | 13 (0.1) | 0.2245 |
Central line | 1,070 (3.3) | 502 (3.4) | 568 (3.2) | 0.3271 |
Inherited or acquired thrombophilia | 16 (0) | 9 (0.1) | 7 (0) | 0.3855 |
Diabetes | 11,136 (34.7) | 5,157 (35.3) | 5,979 (34.1) | 0.0241 |
Procedures associated with VTE or bleed | ||||
Mechanical ventilation | 2,282 (7.1) | 1,111 (7.6) | 1,171 (6.7) | 0.0013 |
Urinary catheter | 4,496 (14.0) | 1,545 (10.6) | 2,951 (16.9) | <0.0001 |
Aspirin | 12,865 (40.1) | 6,101 (41.8) | 6,764 (38.6) | <0.0001 |
Clopidogrel | 4,575 (14.3) | 2,087 (14.3) | 2,488 (14.2) | 0.8050 |
Non‐steroidal anti‐inflammatory drugs | 2,147 (6.7) | 867 (5.9) | 1,280 (7.3) | <0.0001 |
Steroids | 7,938 (24.7) | 3,136 (21.5) | 4,802 (27.4) | <0.0001 |
Statins | 7,376 (23.0) | 3,462 (23.7) | 3,914 (22.3) | 0.0035 |
Comorbidities | ||||
AIDS | 124 (0.4) | 73 (0.5) | 51 (0.3) | 0.0026 |
Alcohol abuse | 1,048 (3.3) | 523 (3.6) | 525 (3.0) | 0.0032 |
Deficiency anemia | 7,010 (21.8) | 3,228 (22.1) | 3,782 (21.6) | 0.2543 |
Rheumatoid arthritis/collagen vas | 967 (3.0) | 426 (2.9) | 541 (3.1) | 0.3762 |
Chronic blood loss anemia | 177 (0.6) | 79 (0.5) | 98 (0.6) | 0.8269 |
Chronic pulmonary disease | 12,418 (38.7) | 5,314 (36.4) | 7,104 (40.6) | <0.0001 |
Depression | 3,334 (10.4) | 1433 (9.8) | 1901 (10.9) | 0.0025 |
Drug abuse | 694 (2.2) | 412 (2.8) | 282 (1.6) | <0.0001 |
Hypertension | 16,979 (52.9) | 7,658 (52.5) | 9,321 (53.2) | 0.1866 |
Hypothyroidism | 4,016 (12.5) | 1,716 (11.8) | 2,300 (13.1) | 0.0002 |
Liver disease | 453 (1.4) | 227 (1.6) | 226 (1.3) | 0.0448 |
Other neurological disorders | 4,682 (14.6) | 2,202 (15.1) | 2,480 (14.2) | 0.0187 |
Peripheral vascular disease | 2,134 (6.6) | 980 (6.7) | 1,154 (6.6) | 0.6490 |
Psychoses | 1,295 (4.0) | 574 (3.9) | 721 (4.1) | 0.4066 |
Pulmonary circulation disease | 1,034 (3.2) | 442 (3.0) | 592 (3.4) | 0.0760 |
Renal failure | 2,794 (8.7) | 1,636 (11.2) | 1,158 (6.6) | 0.0000 |
Peptic ulcer disease with bleeding | 563 (1.8) | 232 (1.6) | 331 (1.9) | 0.0414 |
Valvular disease | 2,079 (6.5) | 899 (6.2) | 1,180 (6.7) | 0.0366 |
Weight loss | 1,231 (3.8) | 556 (3.8) | 675 (3.9) | 0.8391 |
Other prophylaxis | ||||
Intermittent pneumatic compression | 1,003 (3.1) | 456 (3.1) | 547 (3.1) | 0.9926 |
Mechanical prophylaxis | 1,281 (4.0) | 524 (3.6) | 757 (4.3) | 0.0009 |
Fifty‐five percent of patients received LMWH and the remainder received UFH; 1274 (4%) patients switched type of heparin during their stay. The proportion of patients receiving LMWH at an individual hospital varied from 0% to 100% with a u‐shaped distribution, with almost one‐third of hospitals prescribing one treatment or the other exclusively (Figure 1). Similarly, the proportion of an individual physician's patients who received prophylaxis with UFH (vs LMWH) varied from 0% to 100% (Figure 1), with 51% prescribing LMWH exclusively and 31% prescribing UFH exclusively. Compared to patients who received UFH, patients receiving LMWH were older and were more likely to be white, female, and to have pneumonia. By far the biggest difference between the groups was the hospitals at which they received their care (see Supporting Information, e‐Table, in the online version of this article). Patients receiving LMWH were much more likely to be from smaller, rural, non‐teaching hospitals in the South or the West. There were also numerous small differences in comorbidities and individual VTE risk factors between the 2 groups. The only large difference was that patients with a secondary diagnosis of renal failure (for which LMWH is not US Food and Drug Administration [FDA] approved) were almost twice as likely to receive UFH.
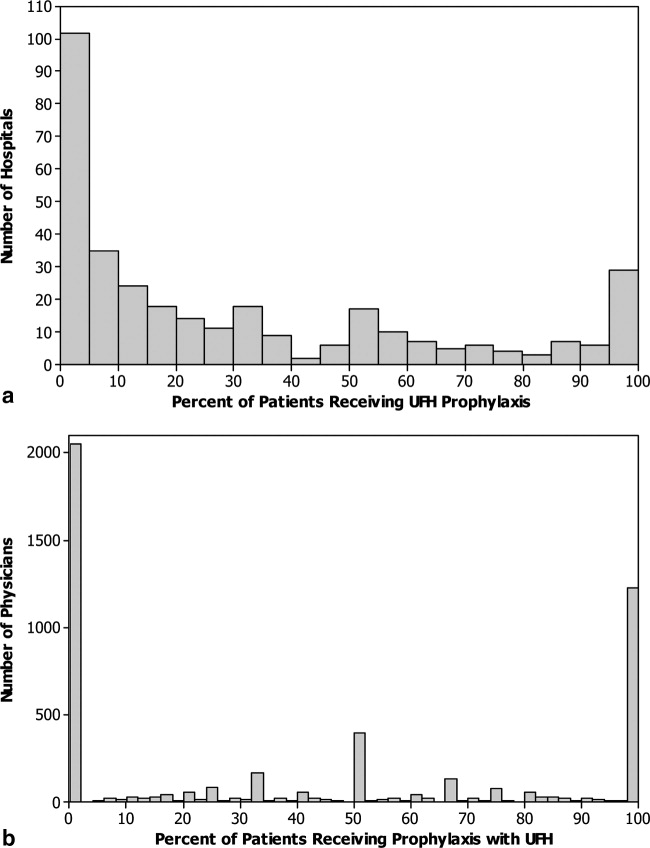
We identified 163 (0.51%) episodes of VTE (Table 2). Compared to patients receiving UFH, those receiving standard LMWH had similar unadjusted rates of VTE (0.53% vs 0.48%; P = 0.54), major bleeding (0.77% vs 0.76%; P = 0.88), thrombocytopenia (1.9% vs 2.0%; P = 0.48), definite HIT (n = 1 vs n = 3; P = 0.34), and mortality (2.8% vs 3.1%; P = 0.07). Definite complications of prophylaxis (HIT or major bleed combined with the discontinuation of heparin) were more common among patients receiving UFH (0.2% vs 0.1%; P = 0.022). Patients treated with UFH had longer unadjusted lengths of stay (P < 0.0001) and higher unadjusted costs (P < 0.0001).
Total | UFH | LMWH | P | |
---|---|---|---|---|
32,104 (100) | 14,591 (45.4) | 17,513 (54.6) | ||
n (%) | n (%) | n (%) | ||
| ||||
Venous thromboembolism | 163 (0.5) | 78 (0.5) | 85 (0.5) | 0.54 |
Heparin‐induced thrombocytopenia | 4 (0) | 3 (0) | 1 (0) | 0.34* |
Any major bleeding | 246 (0.8) | 113 (0.8) | 133 (0.8) | 0.88 |
Transfusion with 2 units of packed red blood cells | 218 (0.7) | 97 (0.7) | 121 (0.7) | 0.78 |
Intracranial hemorrhage | 30 (0.1) | 17 (0.1) | 13 (0.1) | 0.22 |
Complication resulting in stopping heparin | 44 (0.1) | 28 (0.2) | 16 (0.1) | 0.02 |
In‐hospital mortality | 944 (2.9) | 456 (3.1) | 488 (2.8) | 0.07 |
LOS in days; mean (SD) | 6.2 (5.9) | 6.4 (6.2) | 6.0 (5.6) | <0.001 |
Median (IQR) | 5 (37) | 5 (37) | 5 (37) | |
Cost in USD; median (IQR) | 5873 (41718982) | 6007 (41779456) | 5774 (41658660) | <0.001 |
A propensity model for UFH treatment based upon patient characteristics and treatments was not strongly predictive of treatment (c = 0.58) and propensity matching failed to balance many of the patient characteristics. However, hospital alone, ignoring patient characteristics was strongly predictive (c = 0.91) of treatment.
In a model adjusting only for clustering within hospitals, patients treated with UFH had an odds ratio (OR) for VTE of 1.08 (95% confidence interval [CI] 0.79 to 1.49) compared to patients receiving LMWH (Figure 2). Adjustment for propensity for UFH and other covariates attenuated the effect of LMWH (OR 1.04, 95% CI 0.76 to 1.43). When individual patients were assigned a probability of treatment with UFH equal to the hospital rate where they received care, UFH use was associated with a nonsignificant change in the odds of VTE (OR 1.14, 95% CI 0.72 to 1.81).
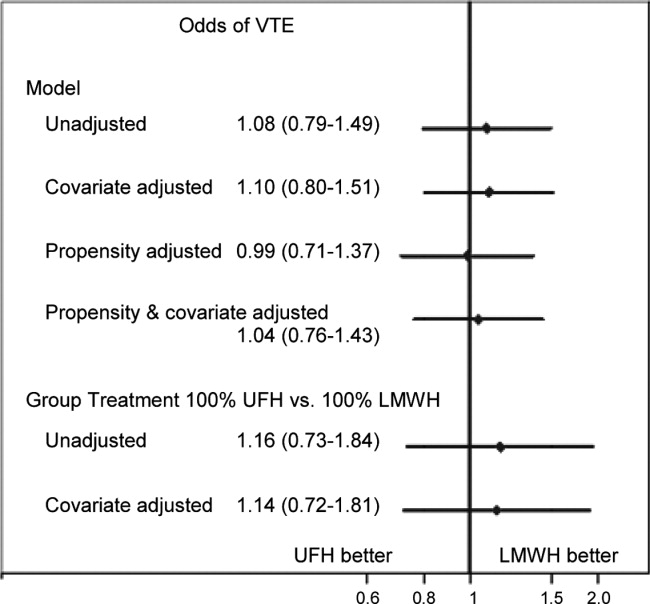
Adjusted for clustering within hospital only, patients treated with UFH had an odds ratio for major bleed of 1.38 (95% CI 1.00 to 1.91) compared to patients receiving LMWH (Figure 3). Adjustment for propensity for UFH and other covariates gave similar results (OR 1.34, 95% CI 0.97 to 1.84). When individual patients were assigned a probability of treatment with UFH equal to the hospital rate where they received care, UFH treatment was associated with a nonsignificant increase in the odds of major bleed (OR 1.64, 95% CI 0.50 to 5.33). When we compared the rate of transfusion across hospitals, including 576,231 additional patients who were excluded from the original analyses because they did not receive daily prophylaxis or had a diagnosis of myocardial infarction or COPD, there was a slight negative correlation between transfusion rates and use of UFH (Spearman Correlation Coefficient 0.03; P = 0.61). Hospitals that used primarily UFH had a transfusion rate of 0.60% versus 0.76% at hospitals using primarily LMWH (P = 0.54), indicating that the increased risk of major bleeding associated with UFH was not confounded by local transfusion practices.
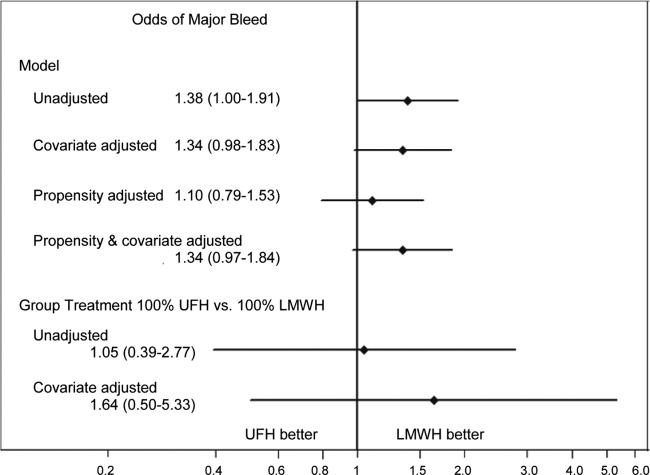
Adjusted for clustering only, patients treated with UFH had an odds ratio for definite complication of 2.35 (95% CI 1.17 to 4.72) compared to those treated with LMWH. Adjustment for propensity and covariates accentuated the association (OR 2.84, 95% CI 1.43 to 5.66). When assigned a probability of treatment with UFH equal to the hospital rate where they received care, UFH treatment was associated with an increase in the risk of definite complication (OR 2.79, 95% CI 1.00 to 7.74).
Adjusted for clustering only, patients treated with UFH had higher costs than those treated with LMWH (cost ratio 1.07, 95% CI 1.05 to 1.09). Adjustment for propensity for UFH and other covariates attenuated the association (cost ratio 1.02, 95% CI 1.00 to 1.03). Finally, when individual patients were assigned a probability of initial treatment with UFH equal to the hospital rate where they received care, treatment with UFH was associated with a nonsignificant change in the relative cost (cost ratio 0.97, 95% CI 0.90 to 1.05).
DISCUSSION
In this retrospective cohort study, we found that low‐molecular‐weight heparin and unfractionated heparin were associated with similar rates of VTE in moderate‐to‐high risk medical patients. However, unfractionated heparin was associated with a small, but higher risk of complications, even after adjustment. There were no statistical differences in rates of heparin‐induced thrombocytopenia, but this complication was exceedingly rare. Finally, overall costs associated with both treatments were similar.
A number of industry‐funded studies have compared LMWH to UFH in randomized clinical trials. These trials have generally been small and used endpoints of uncertain significance, such as asymptomatic deep vein thrombosis assessed by ultrasound. At least 3 meta‐analyses of these trials have been published. Each used different inclusion criteria. The only one to find an efficacy benefit to LMWH over UFH was heavily influenced by the inclusion of a number of studies of stroke patients.3 In that study, LMWH reduced VTE by approximately one‐third relative to UFH. The other 2 analyses found smaller reductions in DVT and pulmonary embolism (PE), and these results were not statistically significant.5, 8 Similarly, 1 analysis5 found a reduction in major bleeding events with LMWH versus UFH, whereas the other 2 studies found smaller reductions which were not statistically significant. The assessment of major bleeding is further complicated by differences in the definition of major bleeding across studies. Using a standard definition of 2 units of packed red blood cells transfused in 1 day to denote major bleeding, we found an associated reduction in bleeding with LMWH that was similar to that observed in the meta‐analyses. Moreover, patients receiving UFH were twice as likely to have a complication that resulted in stopping the prophylaxis, although these complications were overall quite rare. Lastly, there are no cost comparisons based on randomized trials. Several comparisons based on modeling have favored LMWH. One assumed that 3% of patients receiving UFH would develop HIT;13 something we did not observe. At least 3 additional analyses,1416 all funded by the manufacturer of enoxaparin, assumed that LMWH was both more effective and safer than UFH. We found that adjusted costs were similar or slightly lower with UFH than LMWH.
Our study has a number of limitations. First, its observational design makes it vulnerable to selection bias. We attempted to overcome this with rigorous multivariable adjustment, including the propensity for treatment and by using an adaptation of the instrumental variable approach. This method is of particular interest because individual hospitals were strongly predictive of choice of heparin. Still, we cannot exclude the possibility of residual confounding, especially if other outcomes, such as transfusion decisions, were also tied to specific hospital practices. Second, our study used administrative data, and therefore we could not directly adjust for certain differences which may exist between patients who received LMWH and those who received UFH. However, we did adjust for many classic risk factors for VTE. More importantly, it seems that the chance of being treated with a particular form of heparin depends more on the hospital where one receives care than on any combination of patient characteristics. Thus, apart from renal failure, for which we adjusted, it seems unlikely that there were major differences in unmeasured physiological confounders. Third, we limited our analysis to patients who received standard dosing of either type of heparin. We did this to bolster the validity of our findings, but they may not apply to unconventional dosing often observed in clinical practice. Fourth, we measured only outcomes that occurred in the hospital or that prompted a return to the hospital. VTEs which were diagnosed and treated in ambulatory care were not included. While this may have led us to underestimate the true risk of VTE, we have little reason to believe that the choice of whether to admit a patient with VTE is influenced by the original choice of VTE prophylaxis. Finally, our study was conducted before the introduction of generic LMWH, which would be expected to reduce costs associated with LMWH prophylaxis.
VTE prophylaxis for medical patients has emerged as a major focus for quality improvement initiatives. As a result, a significant proportion of general medical patients receive some form of chemoprophylaxis during their hospital stay. Small differences in efficacy or safety of different forms of prophylaxis multiplied by millions of admissions each year can have profound effects on the health of hospitalized patients. Similarly, differences in cost could also have a substantial impact on the healthcare system. We found no difference in efficacy or cost, but treatment with LMWH was less likely to be associated with subsequent transfusion of 2 or more units of packed red blood cells, a surrogate marker for bleeding. In addition, LMWH is more convenient since it can be dosed once daily, and for that reason may be more acceptable to patients. For these reasons, LMWH may be the drug of choice for inpatient prophylaxis of general medical patients. In situations where the cost of the medication itself is important, UFH represents an equally effective alternative.
Acknowledgements
All authors have contributed sufficiently to this study and have provided written permission to be named in the manuscript. No other persons have made substantial contributions to this manuscript. Michael B. Rothberg is the guarantor of the entire manuscript.
Disclosures: This study was supported by a Clinical Scientist Development Award from the Doris Duke Charitable Foundation. The funding source had no role in the study design, analysis, or interpretation of the data. Dr Rothberg served for 1 day as a consultant to Novartis Pharma about an influenza vaccine model. Sandoz, a division of Novartis, was recently granted approval to manufacture a generic form of low‐molecular‐weight heparin. None of the other authors have any conflicts of interest.
- A comparison of enoxaparin with placebo for the prevention of venous thromboembolism in acutely ill medical patients. Prophylaxis in Medical Patients with Enoxaparin Study Group.N Engl J Med.1999;341:793–800. , , , et al.
- Prevention of venous thromboembolism: American College of Chest Physicians Evidence‐Based Clinical Practice Guidelines (8th Edition).Chest.2008;133:381S–453S. , , , et al.
- Pharmacological venous thromboembolism prophylaxis in hospitalized medical patients: a meta‐analysis of randomized controlled trials.Arch Intern Med.2007;167:1476–1486. , , , , .
- Meta‐analysis: anticoagulant prophylaxis to prevent symptomatic venous thromboembolism in hospitalized medical patients.Ann Intern Med.2007;146:278–288. , , , , .
- Prevention of venous thromboembolism in internal medicine with unfractionated or low‐molecular‐weight heparins: a meta‐analysis of randomised clinical trials.Thromb Haemost.2000;83:14–19. , , , et al.
- Risk for heparin‐induced thrombocytopenia with unfractionated and low‐molecular‐weight heparin thromboprophylaxis: a meta‐analysis.Blood.2005;106:2710–2715. , , .
- A safety analysis of thromboprophylaxis in acute medical illness.Thromb Haemost.2003;89:590–591. , .
- How complete is the evidence for thromboembolism prophylaxis in general medicine patients? A meta‐analysis of randomized controlled trials.J Hosp Med.2009;4:289–297. , , , , .
- The Joint Commission on the Accreditation of Healthcare Organizations. Venous Thromboembolism (VTE) Core Measure Set. Available at: http://www.jointcommission.org/PerformanceMeasurement/PerformanceMeasurement/VTE.htm. Accessed June 1,2009.
- Potentially inappropriate medication use in hospitalized elders.J Hosp Med.2008;3:91–102. , , , et al.
- Comorbidity measures for use with administrative data.Med Care.1998;36:8–27. , , , .
- Identification of in‐hospital complications from claims data. Is it valid?Med Care.2000;38:785–795. , , , , , .
- Cost utility of substituting enoxaparin for unfractionated heparin for prophylaxis of venous thrombosis in the hospitalized medical patient.J Hosp Med.2006;1:168–176. , , , .
- Cost effectiveness of thromboprophylaxis with a low‐molecular‐weight heparin versus unfractionated heparin in acutely ill medical inpatients.Am J Manag Care.2004;10:632–642. , , , .
- Comparison of the two‐year outcomes and costs of prophylaxis in medical patients at risk of venous thromboembolism.Thromb Haemost.2008;100:810–820. , , , .
- Cost effectiveness of enoxaparin as prophylaxis against venous thromboembolic complications in acutely ill medical inpatients: modelling study from the hospital perspective in Germany.Pharmacoeconomics.2006;24:571–591. , , , et al.
- A comparison of enoxaparin with placebo for the prevention of venous thromboembolism in acutely ill medical patients. Prophylaxis in Medical Patients with Enoxaparin Study Group.N Engl J Med.1999;341:793–800. , , , et al.
- Prevention of venous thromboembolism: American College of Chest Physicians Evidence‐Based Clinical Practice Guidelines (8th Edition).Chest.2008;133:381S–453S. , , , et al.
- Pharmacological venous thromboembolism prophylaxis in hospitalized medical patients: a meta‐analysis of randomized controlled trials.Arch Intern Med.2007;167:1476–1486. , , , , .
- Meta‐analysis: anticoagulant prophylaxis to prevent symptomatic venous thromboembolism in hospitalized medical patients.Ann Intern Med.2007;146:278–288. , , , , .
- Prevention of venous thromboembolism in internal medicine with unfractionated or low‐molecular‐weight heparins: a meta‐analysis of randomised clinical trials.Thromb Haemost.2000;83:14–19. , , , et al.
- Risk for heparin‐induced thrombocytopenia with unfractionated and low‐molecular‐weight heparin thromboprophylaxis: a meta‐analysis.Blood.2005;106:2710–2715. , , .
- A safety analysis of thromboprophylaxis in acute medical illness.Thromb Haemost.2003;89:590–591. , .
- How complete is the evidence for thromboembolism prophylaxis in general medicine patients? A meta‐analysis of randomized controlled trials.J Hosp Med.2009;4:289–297. , , , , .
- The Joint Commission on the Accreditation of Healthcare Organizations. Venous Thromboembolism (VTE) Core Measure Set. Available at: http://www.jointcommission.org/PerformanceMeasurement/PerformanceMeasurement/VTE.htm. Accessed June 1,2009.
- Potentially inappropriate medication use in hospitalized elders.J Hosp Med.2008;3:91–102. , , , et al.
- Comorbidity measures for use with administrative data.Med Care.1998;36:8–27. , , , .
- Identification of in‐hospital complications from claims data. Is it valid?Med Care.2000;38:785–795. , , , , , .
- Cost utility of substituting enoxaparin for unfractionated heparin for prophylaxis of venous thrombosis in the hospitalized medical patient.J Hosp Med.2006;1:168–176. , , , .
- Cost effectiveness of thromboprophylaxis with a low‐molecular‐weight heparin versus unfractionated heparin in acutely ill medical inpatients.Am J Manag Care.2004;10:632–642. , , , .
- Comparison of the two‐year outcomes and costs of prophylaxis in medical patients at risk of venous thromboembolism.Thromb Haemost.2008;100:810–820. , , , .
- Cost effectiveness of enoxaparin as prophylaxis against venous thromboembolic complications in acutely ill medical inpatients: modelling study from the hospital perspective in Germany.Pharmacoeconomics.2006;24:571–591. , , , et al.
Copyright © 2012 Society of Hospital Medicine
Risk Model for VTE
Venous thromboembolism (VTE) is a major source of morbidity and mortality for hospitalized patients. Among medical patients at the highest risk, as many as 15% can be expected to develop a VTE during their hospital stay1, 2; however, among general medical patients, the incidence of symptomatic VTE is less than 1%,1 and potentially as low as 0.3%.3 Thromboprophylaxis with subcutaneous heparin reduces the risk of VTE by approximately 50%,4 and is therefore recommended for medical patients at high risk. However, heparin also increases the risk of bleeding and thrombocytopenia and thus should be avoided for patients at low risk of VTE. Consequently, the Joint Commission on Accreditation of Healthcare Organizations (JCAHO) recommends that all hospitalized medical patients receive a risk assessment for VTE.5
Certain disease states, including stroke, acute myocardial infarction, heart failure, respiratory disease, sepsis, and cancer, have been associated with increased risk for VTE, and, based on the inclusion criteria of several randomized trials, current American College of Chest Physicians (ACCP) guidelines recommend thromboprophylaxis for patients hospitalized with these diagnoses.2 However, evidence that these factors actually increase a patient's risk for VTE comes from studies of ambulatory patients and is often weak or conflicting. Existing risk‐stratification tools,6, 7 as well as the ACCP guidelines, have not been validated, and accordingly JCAHO does not specify how risk assessment should be conducted. In order to help clinicians better estimate the risk of VTE in medical patients and therefore to provide more targeted thromboprophylaxis, we examined a large cohort of patients with high‐risk diagnoses and created a risk stratification model.
Methods
Setting and Patients
We identified a retrospective cohort of patients discharged between January 1, 2004 and June 30, 2005 from 374 acute care facilities in the US that participated in Premier's Perspective, a database developed for measuring quality and healthcare utilization. Participating hospitals represent all regions of the US, and are generally similar in composition to US hospitals; however, in comparison to information contained in the American Hospital Association annual survey, Perspective hospitals are more likely to be located in the South and in urban areas. Available data elements include those derived from the uniform billing 04 form, such as sociodemographic information about each patient, their International Classification of Diseases, Ninth Revision, Clinical Modification (ICD‐9‐CM) diagnosis and procedure codes, as well as hospital and physician information. This information is supplemented with a date‐stamped log of all items and services billed to the patient or insurer, including diagnostic tests, medications, and other treatments. Permission to conduct the study was obtained from the Institutional Review Board at Baystate Medical Center.
We included all patients age 18 years at moderate‐to‐high risk of VTE according to the ACCP recommendations,8 based on a principal diagnosis of pneumonia, septicemia or respiratory failure with pneumonia, heart failure, chronic obstructive pulmonary disease (COPD), stroke, and urinary tract infection. Diagnoses were assessed using ICD‐9‐CM codes. Patients who were prescribed warfarin or therapeutic doses of heparin on hospital day 1 or 2, and those who received >1 therapeutic dose of heparin but otherwise did not fulfill criteria for VTE, were excluded because we could not evaluate whether they experienced a VTE event during hospitalization. We also excluded patients whose length of stay was <3 days, because our definition of hospital‐acquired VTE required treatment begun on day 3 or later, and those with an indication for anticoagulation other than VTE (eg, prosthetic cardiac valve or atrial fibrillation), because we could not reliably distinguish treatment for VTE from treatment of the underlying condition.
Risk Factors
For each patient, we extracted age, gender, race/ethnicity, and insurance status, principal diagnosis, comorbidities, and specialty of the attending physician. Comorbidities were identified from ICD‐9‐CM secondary diagnosis codes and Diagnosis Related Groups using Healthcare Cost and Utilization Project Comorbidity Software, version 3.1, based on the work of Elixhauser et al.9 We also assessed risk factors which have been previously linked to VTE: paralysis, cancer (metastatic, solid tumor, and lymphoma), chemotherapy/radiation, prior VTE, use of estrogens and estrogen modulators, inflammatory bowel disease, nephrotic syndrome, myeloproliferative disorders, obesity, smoking, central venous catheter, inherited or acquired thrombophilia, steroid use, mechanical ventilation, urinary catheter, decubitus ulcer, HMGco‐A reductase inhibitors, restraints, diabetes, varicose veins, and length‐of‐stay 6 days. These additional comorbidities were defined based on the presence of specific ICD‐9 codes, while use of HMG‐co‐A reductase inhibitors were identified from medication charge files. We also noted whether patients received anticoagulants, the dosages and days of administration, as well as intermittent pneumatic compression devices.
Identification of VTE
Because the presence of a secondary diagnosis of VTE in medical patients is not a reliable way of differentiating hospital‐acquired VTE from those present at the time of admission,10 subjects were considered to have experienced a hospital‐acquired VTE only if they underwent a diagnostic test for VTE (lower extremity ultrasound, venography, CT angiogram, ventilation‐perfusion scan, or pulmonary angiogram) on hospital day 3 or later, received treatment for VTE for at least 50% of the remaining hospital stay, or until initiation of warfarin or appearance of a complication (eg, transfusion or treatment for heparin‐induced thrombocytopenia) and were given a secondary diagnosis of VTE (ICD‐9 diagnoses 453.4, 453.40, 453.41, 453.42, 453.8, 453.9, 415.1, 415.11, 415.19). We considered the following to be treatments for VTE: intravenous unfractionated heparin, >60 mg of enoxaparin, 7500 mg of dalteparin, or placement of an inferior vena cava filter. In addition, patients who were readmitted within 30 days of discharge with a primary diagnosis of VTE were also considered to have developed a VTE as a complication of their previous hospital stay.
Statistical Analysis
Univariate predictors of VTE were assessed using chi‐square tests. We developed a multivariable logistic regression model for VTE on an 80% randomly selected subset of the eligible admissions (the derivation cohort) using all measured risk factors for VTE and selected interaction terms. Generalized estimating equations (GEE) models with a logit link (SAS PROC GENMOD) were used to account for the clustering of patients within hospitals. Initial models were stratified on VTE prophylaxis. Factors significant at P < 0.05 were retained. Parameter estimates derived from the model were used to compute individual VTE risk in the remaining 20% of the admissions (the validation cohort). Discrimination in the validation model was assessed by the c‐statistic, as well as the expected/observed ratio. Both cohorts were categorized by decile of risk, based on the probability distribution in the derivation cohort, and observed VTE events compared to those predicted by the model. All analyses were performed using the Statistical Analysis System (version 9.1, SAS Institute, Inc., Cary, NC).
Role of the Funding Source
This study was supported by a Clinical Scientist Development Award from the Doris Duke Charitable Foundation. The funding source had no role in the study design, analysis, or interpretation of the data.
Results
Our sample contained 242,738 patients, 194,198 (80%) assigned to the derivation set and 48,540 (20%) to the validation set. Patient characteristics were similar in both sets (Supporting Information Appendix Table 1). Most patients were over age 65, 59% were female, and 64% were white (Table 1). The most common primary diagnoses were pneumonia (33%) and congestive heart failure (19%). The most common comorbidities were hypertension (50%), diabetes (31%), chronic pulmonary disease (30%), and anemia (20%). Most patients were cared for by internists (54%) or family practitioners (21%), and 30% received some form of anticoagulant VTE prophylaxis (Table 2). Of patients with an ICD‐9 code for VTE during hospitalization, just over half lacked either diagnostic testing, treatment, or both, leaving 612 (0.25%) patients who fulfilled our criteria for VTE; an additional 440 (0.18%) were readmitted for VTE, for an overall incidence of 0.43%. Patients with a length of stay 6 days had an incidence of 0.79% vs 0.19% for patients with shorter stays.
Total | No VTE | VTE | |||||
---|---|---|---|---|---|---|---|
Variable | N | % | N | % | N | % | P‐Value |
Total | 242,738 | 100 | 241,686 | 100.0 | 1,052 | 100.0 | |
Demographics | |||||||
Age | 0.20 | ||||||
18‐49 | 31,065 | 12.8 | 30,952 | 12.8 | 113 | 10.7 | |
50‐64 | 51,309 | 21.1 | 51,083 | 21.1 | 226 | 21.5 | |
65‐74 | 51,230 | 21.1 | 50,993 | 21.1 | 237 | 22.5 | |
75+ | 109,134 | 45.0 | 108,658 | 45.0 | 476 | 45.2 | |
Female | 142,910 | 58.9 | 142,330 | 58.9 | 580 | 55.1 | 0.01 |
Race/ethnicity | 0.49 | ||||||
White | 155,866 | 64.2 | 155,189 | 64.2 | 677 | 64.4 | |
Black | 41,556 | 17.1 | 41,374 | 17.1 | 182 | 17.3 | |
Hispanic | 9,809 | 4.0 | 9,776 | 4.0 | 33 | 3.1 | |
Other | 35,507 | 14.6 | 35,347 | 14.6 | 160 | 15.2 | |
Marital status | 0.28 | ||||||
Married/life partner | 88,035 | 36.3 | 87,627 | 36.3 | 408 | 38.8 | |
Single | 39,254 | 16.2 | 39,103 | 16.2 | 151 | 14.4 | |
Separated/divorced | 23,492 | 9.7 | 23,394 | 9.7 | 98 | 9.3 | |
Widowed | 58,669 | 24.2 | 58,426 | 24.2 | 243 | 23.1 | |
Other | 33,288 | 13.7 | 33,136 | 13.7 | 152 | 14.4 | |
Admission characteristics | |||||||
Primary diagnosis | <0.001 | ||||||
Community‐acquired pneumonia | 81,171 | 33.4 | 80,792 | 33.4 | 379 | 36.0 | |
Septicemia | 7,643 | 3.2 | 7,568 | 3.1 | 75 | 7.1 | |
Chronic obstructive pulmonary disease | 35,116 | 14.5 | 35,027 | 14.5 | 89 | 8.5 | |
Respiratory failure | 7,098 | 2.9 | 7,012 | 2.9 | 86 | 8.2 | |
Congestive heart failure | 46,503 | 19.2 | 46,336 | 19.2 | 167 | 15.9 | |
Cardiovascular disease | 33,044 | 13.6 | 32,931 | 13.6 | 113 | 10.7 | |
Urinary tract infection | 32,163 | 13.3 | 32,020 | 13.2 | 143 | 13.6 | |
Insurance payer | 0.93 | ||||||
Medicare traditional | 157,609 | 64.9 | 156,927 | 64.9 | 682 | 64.8 | |
Medicare managed care | 10,649 | 4.4 | 10,597 | 4.4 | 52 | 4.9 | |
Medicaid | 17,796 | 7.3 | 17,720 | 7.3 | 76 | 7.2 | |
Private | 44,858 | 18.5 | 44,665 | 18.5 | 193 | 18.3 | |
Self‐pay/uninsured/other | 11,826 | 4.9 | 11,777 | 4.9 | 49 | 4.7 | |
Admitted from skilled nursing facility | 3,003 | 1.2 | 2,980 | 1.2 | 23 | 2.2 | 0.005 |
Risk factors | |||||||
Any VTE prophylaxis | 72,558 | 29.9 | 72,164 | 29.9 | 394 | 37.5 | <0.001 |
Length of stay 6 days | 99,463 | 41.0 | 98,680 | 40.8 | 783 | 74.4 | <0.001 |
Paralysis | 16,764 | 6.9 | 16,689 | 6.9 | 75 | 7.1 | 0.77 |
Metastatic cancer | 5,013 | 2.1 | 4,928 | 2.0 | 85 | 8.1 | <0.001 |
Solid tumor without metastasis | 25,127 | 10.4 | 24,995 | 10.3 | 132 | 12.5 | 0.02 |
Lymphoma | 3,026 | 1.2 | 2,995 | 1.2 | 31 | 2.9 | <0.001 |
Cancer chemotherapy/radiation | 1,254 | 0.5 | 1,231 | 0.5 | 23 | 2.2 | <0.001 |
Prior venous thromboembolism | 2,945 | 1.2 | 2,926 | 1.2 | 19 | 1.8 | 0.08 |
Estrogens | 4,819 | 2.0 | 4,807 | 2.0 | 12 | 1.1 | 0.05 |
Estrogen modulators | 2,102 | 0.9 | 2,091 | 0.9 | 11 | 1.0 | 0.53 |
Inflammatory bowel disease | 814 | 0.3 | 803 | 0.3 | 11 | 1.0 | <0.001 |
Nephrotic syndrome | 520 | 0.2 | 517 | 0.2 | 3 | 0.3 | 0.62 |
Myeloproliferative disorder | 1,983 | 0.8 | 1,973 | 0.8 | 10 | 1.0 | 0.63 |
Obesity | 16,938 | 7.0 | 16,856 | 7.0 | 82 | 7.8 | 0.30 |
Smoking | 35,386 | 14.6 | 35,284 | 14.6 | 102 | 9.7 | <0.001 |
Central venous catheter | 14,754 | 6.1 | 14,525 | 6.0 | 229 | 21.8 | <0.001 |
Inherited or acquired thrombophilia | 114 | 0.1 | 108 | 0.0 | 6 | 0.6 | <0.001 |
Steroids | 82,606 | 34.0 | 82,185 | 34.0 | 421 | 40.0 | <0.001 |
Mechanical ventilation | 13,347 | 5.5 | 13,167 | 5.4 | 180 | 17.1 | <0.001 |
Urinary catheter | 39,080 | 16.1 | 38,816 | 16.1 | 264 | 25.1 | <0.001 |
Decubitus ulcer | 6,829 | 2.8 | 6,776 | 2.8 | 53 | 5.0 | <0.001 |
Statins use | 57,282 | 23.6 | 57,068 | 23.6 | 214 | 20.3 | 0.01 |
Use of restraints | 5,970 | 2.5 | 5,914 | 2.4 | 56 | 5.3 | <0.001 |
Diabetes mellitus | 75,103 | 30.9 | 74,799 | 30.9 | 304 | 28.9 | 0.15 |
Varicose veins | 166 | 0.1 | 165 | 0.1 | 1 | 0.1 | 0.74 |
Comorbidities | |||||||
Hypertension | 120,606 | 49.7 | 120,126 | 49.7 | 480 | 45.6 | 0.008 |
Congestive heart failure | 18,900 | 7.8 | 18,793 | 7.8 | 107 | 10.2 | 0.004 |
Peripheral vascular disease | 16,705 | 6.9 | 16,639 | 6.9 | 66 | 6.3 | 0.43 |
Valvular disease | 13,683 | 5.6 | 13,628 | 5.6 | 55 | 5.2 | 0.56 |
Pulmonary circulation disease | 5,530 | 2.3 | 5,492 | 2.3 | 38 | 3.6 | 0.004 |
Chronic pulmonary disease | 72,028 | 29.7 | 71,698 | 29.7 | 330 | 31.4 | 0.23 |
Respiratory failure second diagnosis | 13,027 | 5.4 | 12,893 | 5.3 | 134 | 12.7 | <0.001 |
Rheumatoid arthritis/collagen vascular disease | 7,090 | 2.9 | 7,050 | 2.9 | 40 | 3.8 | 0.09 |
Deficiency anemias | 49,605 | 20.4 | 49,352 | 20.4 | 253 | 24.0 | 0.004 |
Weight loss | 8,810 | 3.6 | 8,714 | 3.6 | 96 | 9.1 | <0.001 |
Peptic ulcer disease bleeding | 4,736 | 2.0 | 4,723 | 2.0 | 13 | 1.2 | 0.09 |
Chronic blood loss anemia | 2,354 | 1.0 | 2,338 | 1.0 | 16 | 1.5 | 0.07 |
Hypothyroidism | 28,773 | 11.9 | 28,668 | 11.9 | 105 | 10.0 | 0.06 |
Renal failure | 19,768 | 8.1 | 19,669 | 8.1 | 99 | 9.4 | 0.13 |
Liver disease | 4,682 | 1.9 | 4,657 | 1.9 | 25 | 2.4 | 0.29 |
Other neurological disorders | 33,094 | 13.6 | 32,905 | 13.6 | 189 | 18.0 | <0.001 |
Psychoses | 9,330 | 3.8 | 9,283 | 3.8 | 47 | 4.5 | 0.29 |
Depression | 25,561 | 10.5 | 25,442 | 10.5 | 119 | 11.3 | 0.41 |
Alcohol abuse | 7,756 | 3.2 | 7,727 | 3.2 | 29 | 2.8 | 0.42 |
Drug abuse | 4,336 | 1.8 | 4,318 | 1.8 | 18 | 1.7 | 0.85 |
Acquired immune deficiency syndrome | 1,048 | 0.4 | 1,045 | 0.4 | 3 | 0.3 | 0.47 |
Total | Derivation | Validation | |||||
---|---|---|---|---|---|---|---|
Variable | N | % | N | % | N | % | P‐Value |
| |||||||
Total | 242,738 | 100 | 194,198 | 100 | 48,540 | 100 | |
VTE prophylaxis | 0.97 | ||||||
No prophylaxis | 170,180 | 70.1 | 136,153 | 70.1 | 34,027 | 70.1 | |
Any prophylaxis | 72,558 | 29.9 | 58,045 | 29.9 | 14,513 | 29.9 | |
Outcomes | |||||||
ICD‐9 code for VTE | 1,304 | 0.5 | 1,025 | 0.5 | 279 | 0.6 | 0.21 |
ICD‐9 code + diagnostic test | 989 | 0.4 | 777 | 0.4 | 212 | 0.4 | 0.26 |
ICD‐9 code + diagnostic test + treatment for VTE | 612 | 0.3 | 471 | 0.2 | 141 | 0.3 | 0.06 |
Readmission for VTE within 30 days | 446 | 0.2 | 363 | 0.2 | 83 | 0.2 | 0.46 |
Total hospital‐acquired VTE | 1,052 | 0.4 | 829 | 0.4 | 223 | 0.5 | 0.33 |
In‐hospital mortality | 8,019 | 3.3 | 6,403 | 3.3 | 1,616 | 3.3 | 0.72 |
Any readmission within 30 days | 28,664 | 11.8 | 22,885 | 11.8 | 5,779 | 11.9 | 0.46 |
Risk factors for VTE
A large number of patient and hospital factors were associated with the development of VTE (Table 1). Due to the large sample size, even weak associations appear highly statistically significant. Compared to patients without VTE, those with VTE were more likely to have received VTE prophylaxis (37% vs 30%, P < 0.001). However, models of patients receiving prophylaxis and of patients not receiving prophylaxis produced similar odds ratios for the various risk factors (Supporting Information Appendix Table 2); therefore, the final model includes both patients who did, and did not, receive VTE prophylaxis. In the multivariable model (Supporting Information Appendix Table 3), age, length of stay, gender, primary diagnosis, cancer, inflammatory bowel disease, obesity, central venous catheter, inherited thrombophilia, steroid use, mechanical ventilation, active chemotherapy, and urinary catheters were all associated with VTE (Table 3). The strongest risk factors were length of stay 6 days (OR 3.22, 95% CI 2.73, 3.79), central venous catheter (OR 1.87, 95% CI 1.52, 2.29), inflammatory bowel disease (OR 3.11, 95% CI 1.59, 6.08), and inherited thrombophilia (OR 4.00, 95% CI 0.98, 16.40). In addition, there were important interactions between age and cancer; cancer was a strong risk factor among younger patients, but is not as strong a risk factor among older patients (OR compared to young patients without cancer was 4.62 (95% CI 2.72, 7.87) for those age 1849 years, and 3.64 (95% CI 2.52, 5.25) for those aged 5064 years).
Risk Factor | OR | 95% CI |
---|---|---|
| ||
Any prophylaxis | 0.98 | (0.84, 1.14) |
Female | 0.85 | (0.74, 0.98) |
Length of stay 6 days | 3.22 | (2.73, 3.79) |
Age* | ||
18‐49 years | 1 | Referent |
50‐64 years | 1.15 | (0.86, 1.56) |
>65 years | 1.51 | (1.17, 1.96) |
Primary diagnosis | ||
Pneumonia | 1 | Referent |
Chronic obstructive pulmonary disease | 0.57 | (0.44, 0.75) |
Stroke | 0.84 | (0.66, 1.08) |
Congestive heart failure | 0.86 | (0.70, 1.06) |
Urinary tract infection | 1.19 | (0.95, 1.50) |
Respiratory failure | 1.15 | (0.85, 1.55) |
Septicemia | 1.11 | (0.82, 1.50) |
Comorbidities | ||
Inflammatory bowel disease | 3.11 | (1.59, 6.08) |
Obesity | 1.28 | (0.99, 1.66) |
Inherited thrombophilia | 4.00 | (0.98, 16.40) |
Cancer | ||
18‐49 years | 4.62 | (2.72, 7.87) |
50‐64 years | 3.64 | (2.52, 5.25) |
>65 years | 2.17 | (1.61, 2.92) |
Treatments | ||
Central venous catheter | 1.87 | (1.52, 2.29) |
Mechanical ventilation | 1.61 | (1.27, 2.05) |
Urinary catheter | 1.17 | (0.99, 1.38) |
Chemotherapy | 1.71 | (1.03, 2.83) |
Steroids | 1.22 | (1.04, 1.43) |
In the derivation set, the multivariable model produced deciles of mean predicted risk from 0.11% to 1.45%, while mean observed risk over the same deciles ranged from 0.12% to 1.42% (Figure 1). Within the validation cohort, the observed rate of VTE was 0.46% (223 cases among 48,543 subjects). The expected rate according to the model was 0.43% (expected/observed ratio: 0.93 [95% CI 0.82, 1.06]). Model discrimination measured by the c‐statistic in the validation set was 0.75 (95% CI 0.71, 0.78). The model produced deciles of mean predicted risk from 0.11% to 1.46%, with mean observed risk over the same deciles from 0.17% to 1.81%. Risk gradient was relatively flat across the first 6 deciles, began to rise at the seventh decile, and rose sharply in the highest one. Using a risk threshold of 1%, the model had a sensitivity of 28% and a specificity of 93%. In the validation set, this translated into a positive predictive value of 2.2% and a negative predictive value of 99.7%. Assuming that VTE prophylaxis has an efficacy of 50%, the number‐needed‐to‐treat to prevent one VTE among high‐risk patients (predicted risk >1%) would be 91. In contrast, providing prophylaxis to the entire validation sample would result in a number‐needed‐to‐treat of 435. Using a lower treatment threshold of 0.4% produced a positive predictive value of 1% and a negative predictive value of 99.8%. At this threshold, the model would detect 73% of patients with VTE and the number‐needed‐to‐treat to prevent one VTE would be 200.
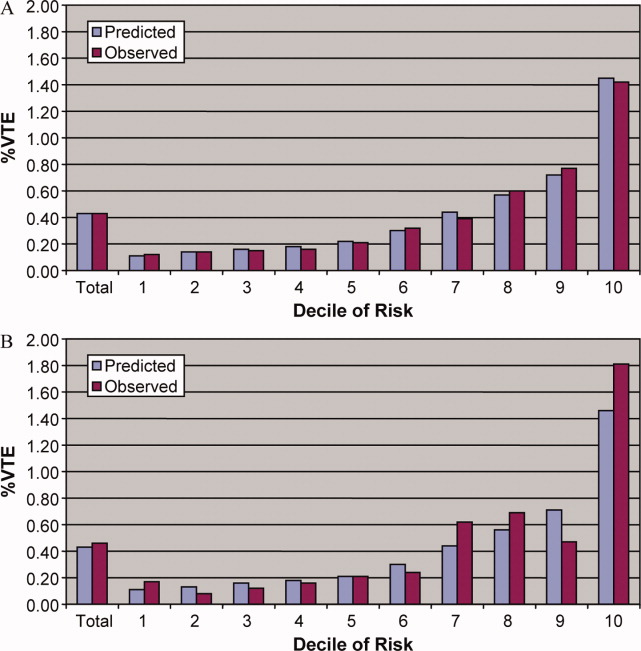
Discussion
In a representative sample of 243,000 hospitalized medical patients with at least one major risk factor for VTE, we found that symptomatic VTE was an uncommon event, occurring in approximately 1 in 231 patients. We identified a number of factors that were associated with an increased risk of VTE, but many previously cited risk factors did not show an association in multivariable models. In particular, patients with a primary diagnosis of COPD appeared not to share the same high risk of VTE as patients with the other diagnoses we examined, a finding reported by others.11 The risk model we developed accurately stratifies patients across a wide range of VTE probabilities, but even among those with the highest predicted rates, symptomatic VTE occurred in less than 2%.
VTE is often described as a frequent complication of hospitalization for medical illness and one of the most common potentially preventable causes of death. Indeed, rates of asymptomatic VTE have been demonstrated to be 3.7% to 26%.12 Although some of these might have fatal consequences, most are distal vein thromboses and their significance is unknown. In contrast, symptomatic events are uncommon, with previous estimates among general medical patients in observational studies in the range of 0.3%3 to 0.8%,12 similar to the rate observed in our study. Symptomatic event rates among control patients in landmark randomized trials have ranged from 0.86%13 to 2.3%,14 but these studies enrolled only very high‐risk patients with more extended hospitalizations, and may involve follow‐up periods of a month or more.
Because it is unlikely that our diagnostic algorithm was 100% sensitive, and because 30% of our patients received chemoprophylaxis, it is probable that we have underestimated the true rate of VTE in our sample. Among the patients who received prophylaxis, the observed rate of VTE was 0.54%. If we assume that prophylaxis is 50% effective, then had these patients not received prophylaxis, their rate of VTE would have been 1.08% (vs 0.39% among those patients who received no prophylaxis) and the overall rate of VTE for the sample would have been 0.60% (1.08 0.30 + 0.39 0.70). If we further assume that our algorithm was only 80% sensitive and 100% specific, the true underlying rate of symptomatic VTE could have been as high as 0.75%, still less than half that seen in randomized trials.
Prophylaxis with heparin has been shown to decrease the rate of both asymptomatic and symptomatic events, but because of the low prevalence, the number‐needed‐to‐treat to prevent one symptomatic pulmonary embolism has been estimated at 345, and prophylaxis has not been shown to affect all‐cause mortality.4, 15 At the same time, prophylaxis costs money, is uncomfortable, and carries a small risk of bleeding and heparin‐induced thrombocytopenia. Given the generally low incidence of symptomatic VTE, it therefore makes sense to reserve prophylaxis for patients at higher risk of thromboembolism.
To decide whether prophylaxis is appropriate for a given patient, it is necessary to quantify the patient's risk and then apply an appropriate threshold for treatment. The National Quality Forum (NQF) recommends,16 and JCAHO has adopted, that a clinician must evaluate each patient upon admission, and regularly thereafter, for the risk of developing DVT [deep vein thrombosis]/VTE. Until now, however, there has been no widely accepted, validated method to risk stratify medical patients. The ACCP recommendations cite just three studies of VTE risk factors in hospitalized medical patients.11, 17, 18 Together they examined 477 cases and 1197 controls, identifying congestive heart failure, pneumonia, cancer, and previous VTE as risk factors. Predictive models based on these factors17, 1921 have not been subjected to validation or have performed poorly.18 Acknowledging this lack of standardized risk assessment, JCAHO leaves the means of assessment to individual hospitals. A quality improvement guide published by the Agency for Healthcare Research and Quality goes one step further, stating that In a typical hospital, it is estimated that fewer than 5% of medical patients could be considered at low risk by most VTE risk stratification methods.22 The guide recommends near universal VTE prophylaxis.
In light of the JCAHO requirements, our model should be welcomed by hospitalists. Rather than assuming that all patients over 40 years of age are at high risk, our model will enable clinicians to risk stratify patients from a low of 0.1% to >1.4% (>10‐fold increase in risk). Moreover, the model was derived from more than 800 episodes of symptomatic VTE among almost 190,000 general medical patients and validated on almost 50,000 more. The observed patients were cared for in clinical practice at a nationally representative group of US hospitals, not in a highly selected clinical trial, increasing the generalizability of our findings. Finally, the model includes ten common risk factors that can easily be entered into decision support software or extracted automatically from the electronic medical record. Electronic reminder systems have already been shown to increase use of VTE prophylaxis, and prevent VTE, especially among cancer patients.23
A more challenging task is defining the appropriate risk threshold to initiate VTE prophylaxis. The Thromboembolic Risk Factors (THRIFT) Consensus Group classified patients according to risk of proximal DVT as low (<1%), moderate (1%‐10%), and high (>10%).21 They recommended heparin prophylaxis for all patients at moderate risk or higher. Although the patients included in our study all had a diagnosis that warranted prophylaxis according to the ACCP guidelines, using the THRIFT threshold for moderate‐to‐high risk, only 7% of our patients should have received prophylaxis. The recommendation not to offer heparin prophylaxis to patients with less than 1% chance of developing symptomatic VTE seems reasonable, given the large number‐needed‐to‐treat, but formal decision analyses should be conducted to better define this threshold. Many hospitalists, however, may feel uncomfortable using the 1% threshold, because our model failed to identify almost three out of four patients who ultimately experienced symptomatic VTE. At that threshold, it would seem that hospital‐acquired VTE is not a preventable complication in most medical patients, as others have pointed out.3, 24 Alternatively, if the threshold were lowered to 0.4%, our model could reduce the use of prophylaxis by 60%, while still identifying three‐fourths of all VTE cases. Further research is needed to know whether such a threshold is reasonable.
Our study has a number of important limitations. First, we relied on claims data, not chart review. We do not know for certain which patients experienced VTE, although our definition of VTE required diagnosis codes plus charges for both diagnosis and treatment. Moreover, our rates are similar to those observed in other trials where symptomatic events were confirmed. Second, about 30% of our patients received at least some VTE prophylaxis, and this may have prevented as many as half of the VTEs in that group. Without prophylaxis, rates might have been 20%30% higher. Similarly, we could not detect patients who were diagnosed after discharge but not admitted to hospital. While we believe this number to be small, it would again increase the rate slightly. Third, we could not assess certain clinical circumstances that are not associated with hospital charges or diagnosis codes, especially prolonged bed rest. Other risk factors, such as the urinary catheter, were probably surrogate markers for immobilization rather than true risk factors. Fourth, we included length of stay in our prediction model. We did this because most randomized trials of VTE prophylaxis included only patients with an expected length of stay 6 days. Physicians' estimates about probable length of stay may be less accurate than actual length of stay as a predictor of VTE. Moreover, the relationship may have been confounded if hospital‐acquired VTE led to longer lengths of stay. We think this unlikely since many of the events were discovered on readmission. Fifth, we studied only patients carrying high‐risk diagnoses, and therefore do not know the baseline risk for patients with less risky conditions, although it should be lower than what we observed. It seems probable that COPD, rather than being protective, as it appears in our model, actually represents the baseline risk for low‐risk diagnoses. It should be noted that we did include a number of other high‐risk diagnoses, such as cancer and inflammatory bowel disease, as secondary diagnoses. A larger, more inclusive study should be conducted to validate our model in other populations. Finally, we cannot know who died of undiagnosed VTE, either in the hospital or after discharge. Such an outcome would be important, but those events are likely to be rare, and VTE prophylaxis has not been shown to affect mortality.
VTE remains a daunting problem in hospitalized medical patients. Although VTE is responsible for a large number of hospital deaths each year, identifying patients at high risk for clinically important VTE is challenging, and may contribute to the persistently low rates of VTE prophylaxis seen in hospitals.25 Current efforts to treat nearly all patients are likely to lead to unnecessary cost, discomfort, and side effects. We present a simple logistic regression model that can easily identify patients at moderate‐to‐high risk (>1%) of developing symptomatic VTE. Future studies should focus on prospectively validating the model in a wider spectrum of medical illness, and better defining the appropriate risk cutoff for general prophylaxis.
Acknowledgements
The authors thank Aruna Priya, MS, for her help with some of the statistical analyses.
- A comparison of enoxaparin with placebo for the prevention of venous thromboembolism in acutely ill medical patients. Prophylaxis in Medical Patients with Enoxaparin Study Group.N Engl J Med.1999;341(11):793–800. , , , et al.
- Prevention of venous thromboembolism: American College of Chest Physicians Evidence‐Based Clinical Practice Guidelines (8th ed).Chest.2008;133(6 suppl):381S–453S. , , , et al.
- Thrombosis prophylaxis in hospitalised medical patients: does prophylaxis in all patients make sense?Neth J Med.2000;56(5):171–176. , , .
- Pharmacological venous thromboembolism prophylaxis in hospitalized medical patients: a meta‐analysis of randomized controlled trials.Arch Intern Med.2007;167(14):1476–1486. , , , , .
- The Joint Commission on the Accreditation of Healthcare Organizations. Venous thromboembolism (VTE) core measure set. Available at: http://www. jointcommission.org/PerformanceMeasurement/PerformanceMeasurement/VTE.htm. Accessed June 1,2009.
- Effective risk stratification of surgical and nonsurgical patients for venous thromboembolic disease.Semin Hematol.2001;38(2 suppl 5):12–19. , , .
- Assessment of venous thromboembolism risk and the benefits of thromboprophylaxis in medical patients.Thromb Haemost.2005;94(4):750–759. , , , et al.
- Prevention of venous thromboembolism: the Seventh ACCP Conference on Antithrombotic and Thrombolytic Therapy.Chest.2004;126(3 suppl):338S–400S. , , , et al.
- Comorbidity measures for use with administrative data.Med Care.1998;36(1):8–27. , , , .
- Identification of in‐hospital complications from claims data. Is it valid?Med Care.2000;38(8):785–795. , , , , , .
- Risk factors for venous thromboembolism in hospitalized patients with acute medical illness: analysis of the MEDENOX Study.Arch Intern Med.2004;164(9):963–968. , , , et al.
- The magnitude of an iatrogenic disorder: a systematic review of the incidence of venous thromboembolism for general medical inpatients.Thromb Haemost.2006;95(5):758–762. , , .
- Randomized, placebo‐controlled trial of dalteparin for the prevention of venous thromboembolism in acutely ill medical patients.Circulation.2004;110(7):874–879. , , , , , .
- Randomised, controlled trial of low‐dose heparin for prevention of fatal pulmonary embolism in patients with infectious diseases. The Heparin Prophylaxis Study Group.Lancet.1996;347(9012):1357–1361. .
- Meta‐analysis: anticoagulant prophylaxis to prevent symptomatic venous thromboembolism in hospitalized medical patients.Ann Intern Med.2007;146(4):278–288. , , , , .
- National Quality Forum.National Voluntary Consensus Standards for Prevention and Care of Venous Thromboembolism: Policy, Preferred Practices, and Initial Performance Measures.Washington, DC;2006.
- Risk factors for deep vein thrombosis in inpatients aged 65 and older: a case‐control multicenter study.J Am Geriatr Soc.2004;52(8):1299–1304. , , , et al.
- Risk factors for venous thrombosis in medical inpatients: validation of a thrombosis risk score.J Thromb Haemost.2004;2(12):2156–2161. , , .
- Venous thromboembolism prophylaxis and risk assessment in medical patients.Semin Thromb Hemost.1991;17(suppl 3):313–318. , , , , , .
- A population‐based perspective of the hospital incidence and case‐fatality rates of deep vein thrombosis and pulmonary embolism. The Worcester DVT Study.Arch Intern Med.1991;151(5):933–938. , , , et al.
- Thromboembolic Risk Factors (THRIFT) Consensus Group.Risk of and prophylaxis for venous thromboembolism in hospital patients.BMJ.1992;305(6853):567–574.
- Preventing Hospital‐Acquired Venous Thromboembolism: A Guide for Effective Quality Improvement. AHRQ Publication No. 08–0075.Rockville, MD:Agency for Healthcare Research and Quality;2008. , .
- Electronic alerts to prevent venous thromboembolism among hospitalized patients.N Engl J Med.2005;352(10):969–977. , , , et al.
- Prophylaxis against venous thromboembolism.BMJ.1992;305(6862):1156. , , .
- Prevention of in‐hospital VTE: why can't we do better?Lancet.2008;371(9610):361–362. , .
Venous thromboembolism (VTE) is a major source of morbidity and mortality for hospitalized patients. Among medical patients at the highest risk, as many as 15% can be expected to develop a VTE during their hospital stay1, 2; however, among general medical patients, the incidence of symptomatic VTE is less than 1%,1 and potentially as low as 0.3%.3 Thromboprophylaxis with subcutaneous heparin reduces the risk of VTE by approximately 50%,4 and is therefore recommended for medical patients at high risk. However, heparin also increases the risk of bleeding and thrombocytopenia and thus should be avoided for patients at low risk of VTE. Consequently, the Joint Commission on Accreditation of Healthcare Organizations (JCAHO) recommends that all hospitalized medical patients receive a risk assessment for VTE.5
Certain disease states, including stroke, acute myocardial infarction, heart failure, respiratory disease, sepsis, and cancer, have been associated with increased risk for VTE, and, based on the inclusion criteria of several randomized trials, current American College of Chest Physicians (ACCP) guidelines recommend thromboprophylaxis for patients hospitalized with these diagnoses.2 However, evidence that these factors actually increase a patient's risk for VTE comes from studies of ambulatory patients and is often weak or conflicting. Existing risk‐stratification tools,6, 7 as well as the ACCP guidelines, have not been validated, and accordingly JCAHO does not specify how risk assessment should be conducted. In order to help clinicians better estimate the risk of VTE in medical patients and therefore to provide more targeted thromboprophylaxis, we examined a large cohort of patients with high‐risk diagnoses and created a risk stratification model.
Methods
Setting and Patients
We identified a retrospective cohort of patients discharged between January 1, 2004 and June 30, 2005 from 374 acute care facilities in the US that participated in Premier's Perspective, a database developed for measuring quality and healthcare utilization. Participating hospitals represent all regions of the US, and are generally similar in composition to US hospitals; however, in comparison to information contained in the American Hospital Association annual survey, Perspective hospitals are more likely to be located in the South and in urban areas. Available data elements include those derived from the uniform billing 04 form, such as sociodemographic information about each patient, their International Classification of Diseases, Ninth Revision, Clinical Modification (ICD‐9‐CM) diagnosis and procedure codes, as well as hospital and physician information. This information is supplemented with a date‐stamped log of all items and services billed to the patient or insurer, including diagnostic tests, medications, and other treatments. Permission to conduct the study was obtained from the Institutional Review Board at Baystate Medical Center.
We included all patients age 18 years at moderate‐to‐high risk of VTE according to the ACCP recommendations,8 based on a principal diagnosis of pneumonia, septicemia or respiratory failure with pneumonia, heart failure, chronic obstructive pulmonary disease (COPD), stroke, and urinary tract infection. Diagnoses were assessed using ICD‐9‐CM codes. Patients who were prescribed warfarin or therapeutic doses of heparin on hospital day 1 or 2, and those who received >1 therapeutic dose of heparin but otherwise did not fulfill criteria for VTE, were excluded because we could not evaluate whether they experienced a VTE event during hospitalization. We also excluded patients whose length of stay was <3 days, because our definition of hospital‐acquired VTE required treatment begun on day 3 or later, and those with an indication for anticoagulation other than VTE (eg, prosthetic cardiac valve or atrial fibrillation), because we could not reliably distinguish treatment for VTE from treatment of the underlying condition.
Risk Factors
For each patient, we extracted age, gender, race/ethnicity, and insurance status, principal diagnosis, comorbidities, and specialty of the attending physician. Comorbidities were identified from ICD‐9‐CM secondary diagnosis codes and Diagnosis Related Groups using Healthcare Cost and Utilization Project Comorbidity Software, version 3.1, based on the work of Elixhauser et al.9 We also assessed risk factors which have been previously linked to VTE: paralysis, cancer (metastatic, solid tumor, and lymphoma), chemotherapy/radiation, prior VTE, use of estrogens and estrogen modulators, inflammatory bowel disease, nephrotic syndrome, myeloproliferative disorders, obesity, smoking, central venous catheter, inherited or acquired thrombophilia, steroid use, mechanical ventilation, urinary catheter, decubitus ulcer, HMGco‐A reductase inhibitors, restraints, diabetes, varicose veins, and length‐of‐stay 6 days. These additional comorbidities were defined based on the presence of specific ICD‐9 codes, while use of HMG‐co‐A reductase inhibitors were identified from medication charge files. We also noted whether patients received anticoagulants, the dosages and days of administration, as well as intermittent pneumatic compression devices.
Identification of VTE
Because the presence of a secondary diagnosis of VTE in medical patients is not a reliable way of differentiating hospital‐acquired VTE from those present at the time of admission,10 subjects were considered to have experienced a hospital‐acquired VTE only if they underwent a diagnostic test for VTE (lower extremity ultrasound, venography, CT angiogram, ventilation‐perfusion scan, or pulmonary angiogram) on hospital day 3 or later, received treatment for VTE for at least 50% of the remaining hospital stay, or until initiation of warfarin or appearance of a complication (eg, transfusion or treatment for heparin‐induced thrombocytopenia) and were given a secondary diagnosis of VTE (ICD‐9 diagnoses 453.4, 453.40, 453.41, 453.42, 453.8, 453.9, 415.1, 415.11, 415.19). We considered the following to be treatments for VTE: intravenous unfractionated heparin, >60 mg of enoxaparin, 7500 mg of dalteparin, or placement of an inferior vena cava filter. In addition, patients who were readmitted within 30 days of discharge with a primary diagnosis of VTE were also considered to have developed a VTE as a complication of their previous hospital stay.
Statistical Analysis
Univariate predictors of VTE were assessed using chi‐square tests. We developed a multivariable logistic regression model for VTE on an 80% randomly selected subset of the eligible admissions (the derivation cohort) using all measured risk factors for VTE and selected interaction terms. Generalized estimating equations (GEE) models with a logit link (SAS PROC GENMOD) were used to account for the clustering of patients within hospitals. Initial models were stratified on VTE prophylaxis. Factors significant at P < 0.05 were retained. Parameter estimates derived from the model were used to compute individual VTE risk in the remaining 20% of the admissions (the validation cohort). Discrimination in the validation model was assessed by the c‐statistic, as well as the expected/observed ratio. Both cohorts were categorized by decile of risk, based on the probability distribution in the derivation cohort, and observed VTE events compared to those predicted by the model. All analyses were performed using the Statistical Analysis System (version 9.1, SAS Institute, Inc., Cary, NC).
Role of the Funding Source
This study was supported by a Clinical Scientist Development Award from the Doris Duke Charitable Foundation. The funding source had no role in the study design, analysis, or interpretation of the data.
Results
Our sample contained 242,738 patients, 194,198 (80%) assigned to the derivation set and 48,540 (20%) to the validation set. Patient characteristics were similar in both sets (Supporting Information Appendix Table 1). Most patients were over age 65, 59% were female, and 64% were white (Table 1). The most common primary diagnoses were pneumonia (33%) and congestive heart failure (19%). The most common comorbidities were hypertension (50%), diabetes (31%), chronic pulmonary disease (30%), and anemia (20%). Most patients were cared for by internists (54%) or family practitioners (21%), and 30% received some form of anticoagulant VTE prophylaxis (Table 2). Of patients with an ICD‐9 code for VTE during hospitalization, just over half lacked either diagnostic testing, treatment, or both, leaving 612 (0.25%) patients who fulfilled our criteria for VTE; an additional 440 (0.18%) were readmitted for VTE, for an overall incidence of 0.43%. Patients with a length of stay 6 days had an incidence of 0.79% vs 0.19% for patients with shorter stays.
Total | No VTE | VTE | |||||
---|---|---|---|---|---|---|---|
Variable | N | % | N | % | N | % | P‐Value |
Total | 242,738 | 100 | 241,686 | 100.0 | 1,052 | 100.0 | |
Demographics | |||||||
Age | 0.20 | ||||||
18‐49 | 31,065 | 12.8 | 30,952 | 12.8 | 113 | 10.7 | |
50‐64 | 51,309 | 21.1 | 51,083 | 21.1 | 226 | 21.5 | |
65‐74 | 51,230 | 21.1 | 50,993 | 21.1 | 237 | 22.5 | |
75+ | 109,134 | 45.0 | 108,658 | 45.0 | 476 | 45.2 | |
Female | 142,910 | 58.9 | 142,330 | 58.9 | 580 | 55.1 | 0.01 |
Race/ethnicity | 0.49 | ||||||
White | 155,866 | 64.2 | 155,189 | 64.2 | 677 | 64.4 | |
Black | 41,556 | 17.1 | 41,374 | 17.1 | 182 | 17.3 | |
Hispanic | 9,809 | 4.0 | 9,776 | 4.0 | 33 | 3.1 | |
Other | 35,507 | 14.6 | 35,347 | 14.6 | 160 | 15.2 | |
Marital status | 0.28 | ||||||
Married/life partner | 88,035 | 36.3 | 87,627 | 36.3 | 408 | 38.8 | |
Single | 39,254 | 16.2 | 39,103 | 16.2 | 151 | 14.4 | |
Separated/divorced | 23,492 | 9.7 | 23,394 | 9.7 | 98 | 9.3 | |
Widowed | 58,669 | 24.2 | 58,426 | 24.2 | 243 | 23.1 | |
Other | 33,288 | 13.7 | 33,136 | 13.7 | 152 | 14.4 | |
Admission characteristics | |||||||
Primary diagnosis | <0.001 | ||||||
Community‐acquired pneumonia | 81,171 | 33.4 | 80,792 | 33.4 | 379 | 36.0 | |
Septicemia | 7,643 | 3.2 | 7,568 | 3.1 | 75 | 7.1 | |
Chronic obstructive pulmonary disease | 35,116 | 14.5 | 35,027 | 14.5 | 89 | 8.5 | |
Respiratory failure | 7,098 | 2.9 | 7,012 | 2.9 | 86 | 8.2 | |
Congestive heart failure | 46,503 | 19.2 | 46,336 | 19.2 | 167 | 15.9 | |
Cardiovascular disease | 33,044 | 13.6 | 32,931 | 13.6 | 113 | 10.7 | |
Urinary tract infection | 32,163 | 13.3 | 32,020 | 13.2 | 143 | 13.6 | |
Insurance payer | 0.93 | ||||||
Medicare traditional | 157,609 | 64.9 | 156,927 | 64.9 | 682 | 64.8 | |
Medicare managed care | 10,649 | 4.4 | 10,597 | 4.4 | 52 | 4.9 | |
Medicaid | 17,796 | 7.3 | 17,720 | 7.3 | 76 | 7.2 | |
Private | 44,858 | 18.5 | 44,665 | 18.5 | 193 | 18.3 | |
Self‐pay/uninsured/other | 11,826 | 4.9 | 11,777 | 4.9 | 49 | 4.7 | |
Admitted from skilled nursing facility | 3,003 | 1.2 | 2,980 | 1.2 | 23 | 2.2 | 0.005 |
Risk factors | |||||||
Any VTE prophylaxis | 72,558 | 29.9 | 72,164 | 29.9 | 394 | 37.5 | <0.001 |
Length of stay 6 days | 99,463 | 41.0 | 98,680 | 40.8 | 783 | 74.4 | <0.001 |
Paralysis | 16,764 | 6.9 | 16,689 | 6.9 | 75 | 7.1 | 0.77 |
Metastatic cancer | 5,013 | 2.1 | 4,928 | 2.0 | 85 | 8.1 | <0.001 |
Solid tumor without metastasis | 25,127 | 10.4 | 24,995 | 10.3 | 132 | 12.5 | 0.02 |
Lymphoma | 3,026 | 1.2 | 2,995 | 1.2 | 31 | 2.9 | <0.001 |
Cancer chemotherapy/radiation | 1,254 | 0.5 | 1,231 | 0.5 | 23 | 2.2 | <0.001 |
Prior venous thromboembolism | 2,945 | 1.2 | 2,926 | 1.2 | 19 | 1.8 | 0.08 |
Estrogens | 4,819 | 2.0 | 4,807 | 2.0 | 12 | 1.1 | 0.05 |
Estrogen modulators | 2,102 | 0.9 | 2,091 | 0.9 | 11 | 1.0 | 0.53 |
Inflammatory bowel disease | 814 | 0.3 | 803 | 0.3 | 11 | 1.0 | <0.001 |
Nephrotic syndrome | 520 | 0.2 | 517 | 0.2 | 3 | 0.3 | 0.62 |
Myeloproliferative disorder | 1,983 | 0.8 | 1,973 | 0.8 | 10 | 1.0 | 0.63 |
Obesity | 16,938 | 7.0 | 16,856 | 7.0 | 82 | 7.8 | 0.30 |
Smoking | 35,386 | 14.6 | 35,284 | 14.6 | 102 | 9.7 | <0.001 |
Central venous catheter | 14,754 | 6.1 | 14,525 | 6.0 | 229 | 21.8 | <0.001 |
Inherited or acquired thrombophilia | 114 | 0.1 | 108 | 0.0 | 6 | 0.6 | <0.001 |
Steroids | 82,606 | 34.0 | 82,185 | 34.0 | 421 | 40.0 | <0.001 |
Mechanical ventilation | 13,347 | 5.5 | 13,167 | 5.4 | 180 | 17.1 | <0.001 |
Urinary catheter | 39,080 | 16.1 | 38,816 | 16.1 | 264 | 25.1 | <0.001 |
Decubitus ulcer | 6,829 | 2.8 | 6,776 | 2.8 | 53 | 5.0 | <0.001 |
Statins use | 57,282 | 23.6 | 57,068 | 23.6 | 214 | 20.3 | 0.01 |
Use of restraints | 5,970 | 2.5 | 5,914 | 2.4 | 56 | 5.3 | <0.001 |
Diabetes mellitus | 75,103 | 30.9 | 74,799 | 30.9 | 304 | 28.9 | 0.15 |
Varicose veins | 166 | 0.1 | 165 | 0.1 | 1 | 0.1 | 0.74 |
Comorbidities | |||||||
Hypertension | 120,606 | 49.7 | 120,126 | 49.7 | 480 | 45.6 | 0.008 |
Congestive heart failure | 18,900 | 7.8 | 18,793 | 7.8 | 107 | 10.2 | 0.004 |
Peripheral vascular disease | 16,705 | 6.9 | 16,639 | 6.9 | 66 | 6.3 | 0.43 |
Valvular disease | 13,683 | 5.6 | 13,628 | 5.6 | 55 | 5.2 | 0.56 |
Pulmonary circulation disease | 5,530 | 2.3 | 5,492 | 2.3 | 38 | 3.6 | 0.004 |
Chronic pulmonary disease | 72,028 | 29.7 | 71,698 | 29.7 | 330 | 31.4 | 0.23 |
Respiratory failure second diagnosis | 13,027 | 5.4 | 12,893 | 5.3 | 134 | 12.7 | <0.001 |
Rheumatoid arthritis/collagen vascular disease | 7,090 | 2.9 | 7,050 | 2.9 | 40 | 3.8 | 0.09 |
Deficiency anemias | 49,605 | 20.4 | 49,352 | 20.4 | 253 | 24.0 | 0.004 |
Weight loss | 8,810 | 3.6 | 8,714 | 3.6 | 96 | 9.1 | <0.001 |
Peptic ulcer disease bleeding | 4,736 | 2.0 | 4,723 | 2.0 | 13 | 1.2 | 0.09 |
Chronic blood loss anemia | 2,354 | 1.0 | 2,338 | 1.0 | 16 | 1.5 | 0.07 |
Hypothyroidism | 28,773 | 11.9 | 28,668 | 11.9 | 105 | 10.0 | 0.06 |
Renal failure | 19,768 | 8.1 | 19,669 | 8.1 | 99 | 9.4 | 0.13 |
Liver disease | 4,682 | 1.9 | 4,657 | 1.9 | 25 | 2.4 | 0.29 |
Other neurological disorders | 33,094 | 13.6 | 32,905 | 13.6 | 189 | 18.0 | <0.001 |
Psychoses | 9,330 | 3.8 | 9,283 | 3.8 | 47 | 4.5 | 0.29 |
Depression | 25,561 | 10.5 | 25,442 | 10.5 | 119 | 11.3 | 0.41 |
Alcohol abuse | 7,756 | 3.2 | 7,727 | 3.2 | 29 | 2.8 | 0.42 |
Drug abuse | 4,336 | 1.8 | 4,318 | 1.8 | 18 | 1.7 | 0.85 |
Acquired immune deficiency syndrome | 1,048 | 0.4 | 1,045 | 0.4 | 3 | 0.3 | 0.47 |
Total | Derivation | Validation | |||||
---|---|---|---|---|---|---|---|
Variable | N | % | N | % | N | % | P‐Value |
| |||||||
Total | 242,738 | 100 | 194,198 | 100 | 48,540 | 100 | |
VTE prophylaxis | 0.97 | ||||||
No prophylaxis | 170,180 | 70.1 | 136,153 | 70.1 | 34,027 | 70.1 | |
Any prophylaxis | 72,558 | 29.9 | 58,045 | 29.9 | 14,513 | 29.9 | |
Outcomes | |||||||
ICD‐9 code for VTE | 1,304 | 0.5 | 1,025 | 0.5 | 279 | 0.6 | 0.21 |
ICD‐9 code + diagnostic test | 989 | 0.4 | 777 | 0.4 | 212 | 0.4 | 0.26 |
ICD‐9 code + diagnostic test + treatment for VTE | 612 | 0.3 | 471 | 0.2 | 141 | 0.3 | 0.06 |
Readmission for VTE within 30 days | 446 | 0.2 | 363 | 0.2 | 83 | 0.2 | 0.46 |
Total hospital‐acquired VTE | 1,052 | 0.4 | 829 | 0.4 | 223 | 0.5 | 0.33 |
In‐hospital mortality | 8,019 | 3.3 | 6,403 | 3.3 | 1,616 | 3.3 | 0.72 |
Any readmission within 30 days | 28,664 | 11.8 | 22,885 | 11.8 | 5,779 | 11.9 | 0.46 |
Risk factors for VTE
A large number of patient and hospital factors were associated with the development of VTE (Table 1). Due to the large sample size, even weak associations appear highly statistically significant. Compared to patients without VTE, those with VTE were more likely to have received VTE prophylaxis (37% vs 30%, P < 0.001). However, models of patients receiving prophylaxis and of patients not receiving prophylaxis produced similar odds ratios for the various risk factors (Supporting Information Appendix Table 2); therefore, the final model includes both patients who did, and did not, receive VTE prophylaxis. In the multivariable model (Supporting Information Appendix Table 3), age, length of stay, gender, primary diagnosis, cancer, inflammatory bowel disease, obesity, central venous catheter, inherited thrombophilia, steroid use, mechanical ventilation, active chemotherapy, and urinary catheters were all associated with VTE (Table 3). The strongest risk factors were length of stay 6 days (OR 3.22, 95% CI 2.73, 3.79), central venous catheter (OR 1.87, 95% CI 1.52, 2.29), inflammatory bowel disease (OR 3.11, 95% CI 1.59, 6.08), and inherited thrombophilia (OR 4.00, 95% CI 0.98, 16.40). In addition, there were important interactions between age and cancer; cancer was a strong risk factor among younger patients, but is not as strong a risk factor among older patients (OR compared to young patients without cancer was 4.62 (95% CI 2.72, 7.87) for those age 1849 years, and 3.64 (95% CI 2.52, 5.25) for those aged 5064 years).
Risk Factor | OR | 95% CI |
---|---|---|
| ||
Any prophylaxis | 0.98 | (0.84, 1.14) |
Female | 0.85 | (0.74, 0.98) |
Length of stay 6 days | 3.22 | (2.73, 3.79) |
Age* | ||
18‐49 years | 1 | Referent |
50‐64 years | 1.15 | (0.86, 1.56) |
>65 years | 1.51 | (1.17, 1.96) |
Primary diagnosis | ||
Pneumonia | 1 | Referent |
Chronic obstructive pulmonary disease | 0.57 | (0.44, 0.75) |
Stroke | 0.84 | (0.66, 1.08) |
Congestive heart failure | 0.86 | (0.70, 1.06) |
Urinary tract infection | 1.19 | (0.95, 1.50) |
Respiratory failure | 1.15 | (0.85, 1.55) |
Septicemia | 1.11 | (0.82, 1.50) |
Comorbidities | ||
Inflammatory bowel disease | 3.11 | (1.59, 6.08) |
Obesity | 1.28 | (0.99, 1.66) |
Inherited thrombophilia | 4.00 | (0.98, 16.40) |
Cancer | ||
18‐49 years | 4.62 | (2.72, 7.87) |
50‐64 years | 3.64 | (2.52, 5.25) |
>65 years | 2.17 | (1.61, 2.92) |
Treatments | ||
Central venous catheter | 1.87 | (1.52, 2.29) |
Mechanical ventilation | 1.61 | (1.27, 2.05) |
Urinary catheter | 1.17 | (0.99, 1.38) |
Chemotherapy | 1.71 | (1.03, 2.83) |
Steroids | 1.22 | (1.04, 1.43) |
In the derivation set, the multivariable model produced deciles of mean predicted risk from 0.11% to 1.45%, while mean observed risk over the same deciles ranged from 0.12% to 1.42% (Figure 1). Within the validation cohort, the observed rate of VTE was 0.46% (223 cases among 48,543 subjects). The expected rate according to the model was 0.43% (expected/observed ratio: 0.93 [95% CI 0.82, 1.06]). Model discrimination measured by the c‐statistic in the validation set was 0.75 (95% CI 0.71, 0.78). The model produced deciles of mean predicted risk from 0.11% to 1.46%, with mean observed risk over the same deciles from 0.17% to 1.81%. Risk gradient was relatively flat across the first 6 deciles, began to rise at the seventh decile, and rose sharply in the highest one. Using a risk threshold of 1%, the model had a sensitivity of 28% and a specificity of 93%. In the validation set, this translated into a positive predictive value of 2.2% and a negative predictive value of 99.7%. Assuming that VTE prophylaxis has an efficacy of 50%, the number‐needed‐to‐treat to prevent one VTE among high‐risk patients (predicted risk >1%) would be 91. In contrast, providing prophylaxis to the entire validation sample would result in a number‐needed‐to‐treat of 435. Using a lower treatment threshold of 0.4% produced a positive predictive value of 1% and a negative predictive value of 99.8%. At this threshold, the model would detect 73% of patients with VTE and the number‐needed‐to‐treat to prevent one VTE would be 200.
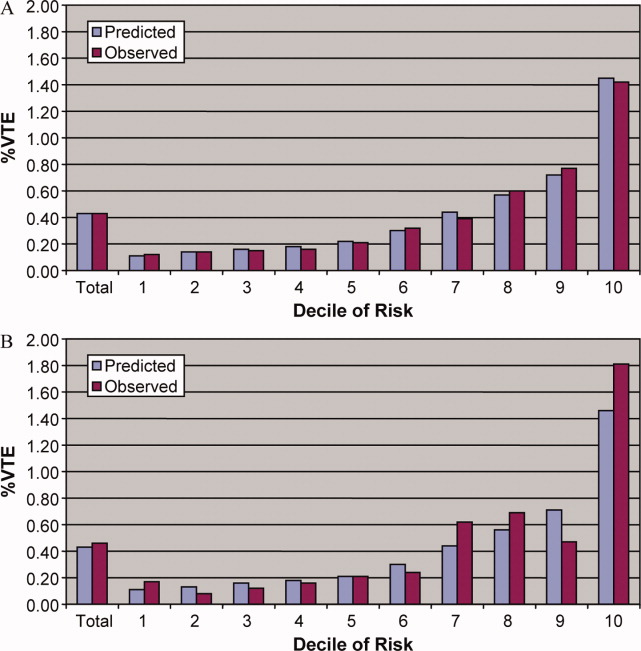
Discussion
In a representative sample of 243,000 hospitalized medical patients with at least one major risk factor for VTE, we found that symptomatic VTE was an uncommon event, occurring in approximately 1 in 231 patients. We identified a number of factors that were associated with an increased risk of VTE, but many previously cited risk factors did not show an association in multivariable models. In particular, patients with a primary diagnosis of COPD appeared not to share the same high risk of VTE as patients with the other diagnoses we examined, a finding reported by others.11 The risk model we developed accurately stratifies patients across a wide range of VTE probabilities, but even among those with the highest predicted rates, symptomatic VTE occurred in less than 2%.
VTE is often described as a frequent complication of hospitalization for medical illness and one of the most common potentially preventable causes of death. Indeed, rates of asymptomatic VTE have been demonstrated to be 3.7% to 26%.12 Although some of these might have fatal consequences, most are distal vein thromboses and their significance is unknown. In contrast, symptomatic events are uncommon, with previous estimates among general medical patients in observational studies in the range of 0.3%3 to 0.8%,12 similar to the rate observed in our study. Symptomatic event rates among control patients in landmark randomized trials have ranged from 0.86%13 to 2.3%,14 but these studies enrolled only very high‐risk patients with more extended hospitalizations, and may involve follow‐up periods of a month or more.
Because it is unlikely that our diagnostic algorithm was 100% sensitive, and because 30% of our patients received chemoprophylaxis, it is probable that we have underestimated the true rate of VTE in our sample. Among the patients who received prophylaxis, the observed rate of VTE was 0.54%. If we assume that prophylaxis is 50% effective, then had these patients not received prophylaxis, their rate of VTE would have been 1.08% (vs 0.39% among those patients who received no prophylaxis) and the overall rate of VTE for the sample would have been 0.60% (1.08 0.30 + 0.39 0.70). If we further assume that our algorithm was only 80% sensitive and 100% specific, the true underlying rate of symptomatic VTE could have been as high as 0.75%, still less than half that seen in randomized trials.
Prophylaxis with heparin has been shown to decrease the rate of both asymptomatic and symptomatic events, but because of the low prevalence, the number‐needed‐to‐treat to prevent one symptomatic pulmonary embolism has been estimated at 345, and prophylaxis has not been shown to affect all‐cause mortality.4, 15 At the same time, prophylaxis costs money, is uncomfortable, and carries a small risk of bleeding and heparin‐induced thrombocytopenia. Given the generally low incidence of symptomatic VTE, it therefore makes sense to reserve prophylaxis for patients at higher risk of thromboembolism.
To decide whether prophylaxis is appropriate for a given patient, it is necessary to quantify the patient's risk and then apply an appropriate threshold for treatment. The National Quality Forum (NQF) recommends,16 and JCAHO has adopted, that a clinician must evaluate each patient upon admission, and regularly thereafter, for the risk of developing DVT [deep vein thrombosis]/VTE. Until now, however, there has been no widely accepted, validated method to risk stratify medical patients. The ACCP recommendations cite just three studies of VTE risk factors in hospitalized medical patients.11, 17, 18 Together they examined 477 cases and 1197 controls, identifying congestive heart failure, pneumonia, cancer, and previous VTE as risk factors. Predictive models based on these factors17, 1921 have not been subjected to validation or have performed poorly.18 Acknowledging this lack of standardized risk assessment, JCAHO leaves the means of assessment to individual hospitals. A quality improvement guide published by the Agency for Healthcare Research and Quality goes one step further, stating that In a typical hospital, it is estimated that fewer than 5% of medical patients could be considered at low risk by most VTE risk stratification methods.22 The guide recommends near universal VTE prophylaxis.
In light of the JCAHO requirements, our model should be welcomed by hospitalists. Rather than assuming that all patients over 40 years of age are at high risk, our model will enable clinicians to risk stratify patients from a low of 0.1% to >1.4% (>10‐fold increase in risk). Moreover, the model was derived from more than 800 episodes of symptomatic VTE among almost 190,000 general medical patients and validated on almost 50,000 more. The observed patients were cared for in clinical practice at a nationally representative group of US hospitals, not in a highly selected clinical trial, increasing the generalizability of our findings. Finally, the model includes ten common risk factors that can easily be entered into decision support software or extracted automatically from the electronic medical record. Electronic reminder systems have already been shown to increase use of VTE prophylaxis, and prevent VTE, especially among cancer patients.23
A more challenging task is defining the appropriate risk threshold to initiate VTE prophylaxis. The Thromboembolic Risk Factors (THRIFT) Consensus Group classified patients according to risk of proximal DVT as low (<1%), moderate (1%‐10%), and high (>10%).21 They recommended heparin prophylaxis for all patients at moderate risk or higher. Although the patients included in our study all had a diagnosis that warranted prophylaxis according to the ACCP guidelines, using the THRIFT threshold for moderate‐to‐high risk, only 7% of our patients should have received prophylaxis. The recommendation not to offer heparin prophylaxis to patients with less than 1% chance of developing symptomatic VTE seems reasonable, given the large number‐needed‐to‐treat, but formal decision analyses should be conducted to better define this threshold. Many hospitalists, however, may feel uncomfortable using the 1% threshold, because our model failed to identify almost three out of four patients who ultimately experienced symptomatic VTE. At that threshold, it would seem that hospital‐acquired VTE is not a preventable complication in most medical patients, as others have pointed out.3, 24 Alternatively, if the threshold were lowered to 0.4%, our model could reduce the use of prophylaxis by 60%, while still identifying three‐fourths of all VTE cases. Further research is needed to know whether such a threshold is reasonable.
Our study has a number of important limitations. First, we relied on claims data, not chart review. We do not know for certain which patients experienced VTE, although our definition of VTE required diagnosis codes plus charges for both diagnosis and treatment. Moreover, our rates are similar to those observed in other trials where symptomatic events were confirmed. Second, about 30% of our patients received at least some VTE prophylaxis, and this may have prevented as many as half of the VTEs in that group. Without prophylaxis, rates might have been 20%30% higher. Similarly, we could not detect patients who were diagnosed after discharge but not admitted to hospital. While we believe this number to be small, it would again increase the rate slightly. Third, we could not assess certain clinical circumstances that are not associated with hospital charges or diagnosis codes, especially prolonged bed rest. Other risk factors, such as the urinary catheter, were probably surrogate markers for immobilization rather than true risk factors. Fourth, we included length of stay in our prediction model. We did this because most randomized trials of VTE prophylaxis included only patients with an expected length of stay 6 days. Physicians' estimates about probable length of stay may be less accurate than actual length of stay as a predictor of VTE. Moreover, the relationship may have been confounded if hospital‐acquired VTE led to longer lengths of stay. We think this unlikely since many of the events were discovered on readmission. Fifth, we studied only patients carrying high‐risk diagnoses, and therefore do not know the baseline risk for patients with less risky conditions, although it should be lower than what we observed. It seems probable that COPD, rather than being protective, as it appears in our model, actually represents the baseline risk for low‐risk diagnoses. It should be noted that we did include a number of other high‐risk diagnoses, such as cancer and inflammatory bowel disease, as secondary diagnoses. A larger, more inclusive study should be conducted to validate our model in other populations. Finally, we cannot know who died of undiagnosed VTE, either in the hospital or after discharge. Such an outcome would be important, but those events are likely to be rare, and VTE prophylaxis has not been shown to affect mortality.
VTE remains a daunting problem in hospitalized medical patients. Although VTE is responsible for a large number of hospital deaths each year, identifying patients at high risk for clinically important VTE is challenging, and may contribute to the persistently low rates of VTE prophylaxis seen in hospitals.25 Current efforts to treat nearly all patients are likely to lead to unnecessary cost, discomfort, and side effects. We present a simple logistic regression model that can easily identify patients at moderate‐to‐high risk (>1%) of developing symptomatic VTE. Future studies should focus on prospectively validating the model in a wider spectrum of medical illness, and better defining the appropriate risk cutoff for general prophylaxis.
Acknowledgements
The authors thank Aruna Priya, MS, for her help with some of the statistical analyses.
Venous thromboembolism (VTE) is a major source of morbidity and mortality for hospitalized patients. Among medical patients at the highest risk, as many as 15% can be expected to develop a VTE during their hospital stay1, 2; however, among general medical patients, the incidence of symptomatic VTE is less than 1%,1 and potentially as low as 0.3%.3 Thromboprophylaxis with subcutaneous heparin reduces the risk of VTE by approximately 50%,4 and is therefore recommended for medical patients at high risk. However, heparin also increases the risk of bleeding and thrombocytopenia and thus should be avoided for patients at low risk of VTE. Consequently, the Joint Commission on Accreditation of Healthcare Organizations (JCAHO) recommends that all hospitalized medical patients receive a risk assessment for VTE.5
Certain disease states, including stroke, acute myocardial infarction, heart failure, respiratory disease, sepsis, and cancer, have been associated with increased risk for VTE, and, based on the inclusion criteria of several randomized trials, current American College of Chest Physicians (ACCP) guidelines recommend thromboprophylaxis for patients hospitalized with these diagnoses.2 However, evidence that these factors actually increase a patient's risk for VTE comes from studies of ambulatory patients and is often weak or conflicting. Existing risk‐stratification tools,6, 7 as well as the ACCP guidelines, have not been validated, and accordingly JCAHO does not specify how risk assessment should be conducted. In order to help clinicians better estimate the risk of VTE in medical patients and therefore to provide more targeted thromboprophylaxis, we examined a large cohort of patients with high‐risk diagnoses and created a risk stratification model.
Methods
Setting and Patients
We identified a retrospective cohort of patients discharged between January 1, 2004 and June 30, 2005 from 374 acute care facilities in the US that participated in Premier's Perspective, a database developed for measuring quality and healthcare utilization. Participating hospitals represent all regions of the US, and are generally similar in composition to US hospitals; however, in comparison to information contained in the American Hospital Association annual survey, Perspective hospitals are more likely to be located in the South and in urban areas. Available data elements include those derived from the uniform billing 04 form, such as sociodemographic information about each patient, their International Classification of Diseases, Ninth Revision, Clinical Modification (ICD‐9‐CM) diagnosis and procedure codes, as well as hospital and physician information. This information is supplemented with a date‐stamped log of all items and services billed to the patient or insurer, including diagnostic tests, medications, and other treatments. Permission to conduct the study was obtained from the Institutional Review Board at Baystate Medical Center.
We included all patients age 18 years at moderate‐to‐high risk of VTE according to the ACCP recommendations,8 based on a principal diagnosis of pneumonia, septicemia or respiratory failure with pneumonia, heart failure, chronic obstructive pulmonary disease (COPD), stroke, and urinary tract infection. Diagnoses were assessed using ICD‐9‐CM codes. Patients who were prescribed warfarin or therapeutic doses of heparin on hospital day 1 or 2, and those who received >1 therapeutic dose of heparin but otherwise did not fulfill criteria for VTE, were excluded because we could not evaluate whether they experienced a VTE event during hospitalization. We also excluded patients whose length of stay was <3 days, because our definition of hospital‐acquired VTE required treatment begun on day 3 or later, and those with an indication for anticoagulation other than VTE (eg, prosthetic cardiac valve or atrial fibrillation), because we could not reliably distinguish treatment for VTE from treatment of the underlying condition.
Risk Factors
For each patient, we extracted age, gender, race/ethnicity, and insurance status, principal diagnosis, comorbidities, and specialty of the attending physician. Comorbidities were identified from ICD‐9‐CM secondary diagnosis codes and Diagnosis Related Groups using Healthcare Cost and Utilization Project Comorbidity Software, version 3.1, based on the work of Elixhauser et al.9 We also assessed risk factors which have been previously linked to VTE: paralysis, cancer (metastatic, solid tumor, and lymphoma), chemotherapy/radiation, prior VTE, use of estrogens and estrogen modulators, inflammatory bowel disease, nephrotic syndrome, myeloproliferative disorders, obesity, smoking, central venous catheter, inherited or acquired thrombophilia, steroid use, mechanical ventilation, urinary catheter, decubitus ulcer, HMGco‐A reductase inhibitors, restraints, diabetes, varicose veins, and length‐of‐stay 6 days. These additional comorbidities were defined based on the presence of specific ICD‐9 codes, while use of HMG‐co‐A reductase inhibitors were identified from medication charge files. We also noted whether patients received anticoagulants, the dosages and days of administration, as well as intermittent pneumatic compression devices.
Identification of VTE
Because the presence of a secondary diagnosis of VTE in medical patients is not a reliable way of differentiating hospital‐acquired VTE from those present at the time of admission,10 subjects were considered to have experienced a hospital‐acquired VTE only if they underwent a diagnostic test for VTE (lower extremity ultrasound, venography, CT angiogram, ventilation‐perfusion scan, or pulmonary angiogram) on hospital day 3 or later, received treatment for VTE for at least 50% of the remaining hospital stay, or until initiation of warfarin or appearance of a complication (eg, transfusion or treatment for heparin‐induced thrombocytopenia) and were given a secondary diagnosis of VTE (ICD‐9 diagnoses 453.4, 453.40, 453.41, 453.42, 453.8, 453.9, 415.1, 415.11, 415.19). We considered the following to be treatments for VTE: intravenous unfractionated heparin, >60 mg of enoxaparin, 7500 mg of dalteparin, or placement of an inferior vena cava filter. In addition, patients who were readmitted within 30 days of discharge with a primary diagnosis of VTE were also considered to have developed a VTE as a complication of their previous hospital stay.
Statistical Analysis
Univariate predictors of VTE were assessed using chi‐square tests. We developed a multivariable logistic regression model for VTE on an 80% randomly selected subset of the eligible admissions (the derivation cohort) using all measured risk factors for VTE and selected interaction terms. Generalized estimating equations (GEE) models with a logit link (SAS PROC GENMOD) were used to account for the clustering of patients within hospitals. Initial models were stratified on VTE prophylaxis. Factors significant at P < 0.05 were retained. Parameter estimates derived from the model were used to compute individual VTE risk in the remaining 20% of the admissions (the validation cohort). Discrimination in the validation model was assessed by the c‐statistic, as well as the expected/observed ratio. Both cohorts were categorized by decile of risk, based on the probability distribution in the derivation cohort, and observed VTE events compared to those predicted by the model. All analyses were performed using the Statistical Analysis System (version 9.1, SAS Institute, Inc., Cary, NC).
Role of the Funding Source
This study was supported by a Clinical Scientist Development Award from the Doris Duke Charitable Foundation. The funding source had no role in the study design, analysis, or interpretation of the data.
Results
Our sample contained 242,738 patients, 194,198 (80%) assigned to the derivation set and 48,540 (20%) to the validation set. Patient characteristics were similar in both sets (Supporting Information Appendix Table 1). Most patients were over age 65, 59% were female, and 64% were white (Table 1). The most common primary diagnoses were pneumonia (33%) and congestive heart failure (19%). The most common comorbidities were hypertension (50%), diabetes (31%), chronic pulmonary disease (30%), and anemia (20%). Most patients were cared for by internists (54%) or family practitioners (21%), and 30% received some form of anticoagulant VTE prophylaxis (Table 2). Of patients with an ICD‐9 code for VTE during hospitalization, just over half lacked either diagnostic testing, treatment, or both, leaving 612 (0.25%) patients who fulfilled our criteria for VTE; an additional 440 (0.18%) were readmitted for VTE, for an overall incidence of 0.43%. Patients with a length of stay 6 days had an incidence of 0.79% vs 0.19% for patients with shorter stays.
Total | No VTE | VTE | |||||
---|---|---|---|---|---|---|---|
Variable | N | % | N | % | N | % | P‐Value |
Total | 242,738 | 100 | 241,686 | 100.0 | 1,052 | 100.0 | |
Demographics | |||||||
Age | 0.20 | ||||||
18‐49 | 31,065 | 12.8 | 30,952 | 12.8 | 113 | 10.7 | |
50‐64 | 51,309 | 21.1 | 51,083 | 21.1 | 226 | 21.5 | |
65‐74 | 51,230 | 21.1 | 50,993 | 21.1 | 237 | 22.5 | |
75+ | 109,134 | 45.0 | 108,658 | 45.0 | 476 | 45.2 | |
Female | 142,910 | 58.9 | 142,330 | 58.9 | 580 | 55.1 | 0.01 |
Race/ethnicity | 0.49 | ||||||
White | 155,866 | 64.2 | 155,189 | 64.2 | 677 | 64.4 | |
Black | 41,556 | 17.1 | 41,374 | 17.1 | 182 | 17.3 | |
Hispanic | 9,809 | 4.0 | 9,776 | 4.0 | 33 | 3.1 | |
Other | 35,507 | 14.6 | 35,347 | 14.6 | 160 | 15.2 | |
Marital status | 0.28 | ||||||
Married/life partner | 88,035 | 36.3 | 87,627 | 36.3 | 408 | 38.8 | |
Single | 39,254 | 16.2 | 39,103 | 16.2 | 151 | 14.4 | |
Separated/divorced | 23,492 | 9.7 | 23,394 | 9.7 | 98 | 9.3 | |
Widowed | 58,669 | 24.2 | 58,426 | 24.2 | 243 | 23.1 | |
Other | 33,288 | 13.7 | 33,136 | 13.7 | 152 | 14.4 | |
Admission characteristics | |||||||
Primary diagnosis | <0.001 | ||||||
Community‐acquired pneumonia | 81,171 | 33.4 | 80,792 | 33.4 | 379 | 36.0 | |
Septicemia | 7,643 | 3.2 | 7,568 | 3.1 | 75 | 7.1 | |
Chronic obstructive pulmonary disease | 35,116 | 14.5 | 35,027 | 14.5 | 89 | 8.5 | |
Respiratory failure | 7,098 | 2.9 | 7,012 | 2.9 | 86 | 8.2 | |
Congestive heart failure | 46,503 | 19.2 | 46,336 | 19.2 | 167 | 15.9 | |
Cardiovascular disease | 33,044 | 13.6 | 32,931 | 13.6 | 113 | 10.7 | |
Urinary tract infection | 32,163 | 13.3 | 32,020 | 13.2 | 143 | 13.6 | |
Insurance payer | 0.93 | ||||||
Medicare traditional | 157,609 | 64.9 | 156,927 | 64.9 | 682 | 64.8 | |
Medicare managed care | 10,649 | 4.4 | 10,597 | 4.4 | 52 | 4.9 | |
Medicaid | 17,796 | 7.3 | 17,720 | 7.3 | 76 | 7.2 | |
Private | 44,858 | 18.5 | 44,665 | 18.5 | 193 | 18.3 | |
Self‐pay/uninsured/other | 11,826 | 4.9 | 11,777 | 4.9 | 49 | 4.7 | |
Admitted from skilled nursing facility | 3,003 | 1.2 | 2,980 | 1.2 | 23 | 2.2 | 0.005 |
Risk factors | |||||||
Any VTE prophylaxis | 72,558 | 29.9 | 72,164 | 29.9 | 394 | 37.5 | <0.001 |
Length of stay 6 days | 99,463 | 41.0 | 98,680 | 40.8 | 783 | 74.4 | <0.001 |
Paralysis | 16,764 | 6.9 | 16,689 | 6.9 | 75 | 7.1 | 0.77 |
Metastatic cancer | 5,013 | 2.1 | 4,928 | 2.0 | 85 | 8.1 | <0.001 |
Solid tumor without metastasis | 25,127 | 10.4 | 24,995 | 10.3 | 132 | 12.5 | 0.02 |
Lymphoma | 3,026 | 1.2 | 2,995 | 1.2 | 31 | 2.9 | <0.001 |
Cancer chemotherapy/radiation | 1,254 | 0.5 | 1,231 | 0.5 | 23 | 2.2 | <0.001 |
Prior venous thromboembolism | 2,945 | 1.2 | 2,926 | 1.2 | 19 | 1.8 | 0.08 |
Estrogens | 4,819 | 2.0 | 4,807 | 2.0 | 12 | 1.1 | 0.05 |
Estrogen modulators | 2,102 | 0.9 | 2,091 | 0.9 | 11 | 1.0 | 0.53 |
Inflammatory bowel disease | 814 | 0.3 | 803 | 0.3 | 11 | 1.0 | <0.001 |
Nephrotic syndrome | 520 | 0.2 | 517 | 0.2 | 3 | 0.3 | 0.62 |
Myeloproliferative disorder | 1,983 | 0.8 | 1,973 | 0.8 | 10 | 1.0 | 0.63 |
Obesity | 16,938 | 7.0 | 16,856 | 7.0 | 82 | 7.8 | 0.30 |
Smoking | 35,386 | 14.6 | 35,284 | 14.6 | 102 | 9.7 | <0.001 |
Central venous catheter | 14,754 | 6.1 | 14,525 | 6.0 | 229 | 21.8 | <0.001 |
Inherited or acquired thrombophilia | 114 | 0.1 | 108 | 0.0 | 6 | 0.6 | <0.001 |
Steroids | 82,606 | 34.0 | 82,185 | 34.0 | 421 | 40.0 | <0.001 |
Mechanical ventilation | 13,347 | 5.5 | 13,167 | 5.4 | 180 | 17.1 | <0.001 |
Urinary catheter | 39,080 | 16.1 | 38,816 | 16.1 | 264 | 25.1 | <0.001 |
Decubitus ulcer | 6,829 | 2.8 | 6,776 | 2.8 | 53 | 5.0 | <0.001 |
Statins use | 57,282 | 23.6 | 57,068 | 23.6 | 214 | 20.3 | 0.01 |
Use of restraints | 5,970 | 2.5 | 5,914 | 2.4 | 56 | 5.3 | <0.001 |
Diabetes mellitus | 75,103 | 30.9 | 74,799 | 30.9 | 304 | 28.9 | 0.15 |
Varicose veins | 166 | 0.1 | 165 | 0.1 | 1 | 0.1 | 0.74 |
Comorbidities | |||||||
Hypertension | 120,606 | 49.7 | 120,126 | 49.7 | 480 | 45.6 | 0.008 |
Congestive heart failure | 18,900 | 7.8 | 18,793 | 7.8 | 107 | 10.2 | 0.004 |
Peripheral vascular disease | 16,705 | 6.9 | 16,639 | 6.9 | 66 | 6.3 | 0.43 |
Valvular disease | 13,683 | 5.6 | 13,628 | 5.6 | 55 | 5.2 | 0.56 |
Pulmonary circulation disease | 5,530 | 2.3 | 5,492 | 2.3 | 38 | 3.6 | 0.004 |
Chronic pulmonary disease | 72,028 | 29.7 | 71,698 | 29.7 | 330 | 31.4 | 0.23 |
Respiratory failure second diagnosis | 13,027 | 5.4 | 12,893 | 5.3 | 134 | 12.7 | <0.001 |
Rheumatoid arthritis/collagen vascular disease | 7,090 | 2.9 | 7,050 | 2.9 | 40 | 3.8 | 0.09 |
Deficiency anemias | 49,605 | 20.4 | 49,352 | 20.4 | 253 | 24.0 | 0.004 |
Weight loss | 8,810 | 3.6 | 8,714 | 3.6 | 96 | 9.1 | <0.001 |
Peptic ulcer disease bleeding | 4,736 | 2.0 | 4,723 | 2.0 | 13 | 1.2 | 0.09 |
Chronic blood loss anemia | 2,354 | 1.0 | 2,338 | 1.0 | 16 | 1.5 | 0.07 |
Hypothyroidism | 28,773 | 11.9 | 28,668 | 11.9 | 105 | 10.0 | 0.06 |
Renal failure | 19,768 | 8.1 | 19,669 | 8.1 | 99 | 9.4 | 0.13 |
Liver disease | 4,682 | 1.9 | 4,657 | 1.9 | 25 | 2.4 | 0.29 |
Other neurological disorders | 33,094 | 13.6 | 32,905 | 13.6 | 189 | 18.0 | <0.001 |
Psychoses | 9,330 | 3.8 | 9,283 | 3.8 | 47 | 4.5 | 0.29 |
Depression | 25,561 | 10.5 | 25,442 | 10.5 | 119 | 11.3 | 0.41 |
Alcohol abuse | 7,756 | 3.2 | 7,727 | 3.2 | 29 | 2.8 | 0.42 |
Drug abuse | 4,336 | 1.8 | 4,318 | 1.8 | 18 | 1.7 | 0.85 |
Acquired immune deficiency syndrome | 1,048 | 0.4 | 1,045 | 0.4 | 3 | 0.3 | 0.47 |
Total | Derivation | Validation | |||||
---|---|---|---|---|---|---|---|
Variable | N | % | N | % | N | % | P‐Value |
| |||||||
Total | 242,738 | 100 | 194,198 | 100 | 48,540 | 100 | |
VTE prophylaxis | 0.97 | ||||||
No prophylaxis | 170,180 | 70.1 | 136,153 | 70.1 | 34,027 | 70.1 | |
Any prophylaxis | 72,558 | 29.9 | 58,045 | 29.9 | 14,513 | 29.9 | |
Outcomes | |||||||
ICD‐9 code for VTE | 1,304 | 0.5 | 1,025 | 0.5 | 279 | 0.6 | 0.21 |
ICD‐9 code + diagnostic test | 989 | 0.4 | 777 | 0.4 | 212 | 0.4 | 0.26 |
ICD‐9 code + diagnostic test + treatment for VTE | 612 | 0.3 | 471 | 0.2 | 141 | 0.3 | 0.06 |
Readmission for VTE within 30 days | 446 | 0.2 | 363 | 0.2 | 83 | 0.2 | 0.46 |
Total hospital‐acquired VTE | 1,052 | 0.4 | 829 | 0.4 | 223 | 0.5 | 0.33 |
In‐hospital mortality | 8,019 | 3.3 | 6,403 | 3.3 | 1,616 | 3.3 | 0.72 |
Any readmission within 30 days | 28,664 | 11.8 | 22,885 | 11.8 | 5,779 | 11.9 | 0.46 |
Risk factors for VTE
A large number of patient and hospital factors were associated with the development of VTE (Table 1). Due to the large sample size, even weak associations appear highly statistically significant. Compared to patients without VTE, those with VTE were more likely to have received VTE prophylaxis (37% vs 30%, P < 0.001). However, models of patients receiving prophylaxis and of patients not receiving prophylaxis produced similar odds ratios for the various risk factors (Supporting Information Appendix Table 2); therefore, the final model includes both patients who did, and did not, receive VTE prophylaxis. In the multivariable model (Supporting Information Appendix Table 3), age, length of stay, gender, primary diagnosis, cancer, inflammatory bowel disease, obesity, central venous catheter, inherited thrombophilia, steroid use, mechanical ventilation, active chemotherapy, and urinary catheters were all associated with VTE (Table 3). The strongest risk factors were length of stay 6 days (OR 3.22, 95% CI 2.73, 3.79), central venous catheter (OR 1.87, 95% CI 1.52, 2.29), inflammatory bowel disease (OR 3.11, 95% CI 1.59, 6.08), and inherited thrombophilia (OR 4.00, 95% CI 0.98, 16.40). In addition, there were important interactions between age and cancer; cancer was a strong risk factor among younger patients, but is not as strong a risk factor among older patients (OR compared to young patients without cancer was 4.62 (95% CI 2.72, 7.87) for those age 1849 years, and 3.64 (95% CI 2.52, 5.25) for those aged 5064 years).
Risk Factor | OR | 95% CI |
---|---|---|
| ||
Any prophylaxis | 0.98 | (0.84, 1.14) |
Female | 0.85 | (0.74, 0.98) |
Length of stay 6 days | 3.22 | (2.73, 3.79) |
Age* | ||
18‐49 years | 1 | Referent |
50‐64 years | 1.15 | (0.86, 1.56) |
>65 years | 1.51 | (1.17, 1.96) |
Primary diagnosis | ||
Pneumonia | 1 | Referent |
Chronic obstructive pulmonary disease | 0.57 | (0.44, 0.75) |
Stroke | 0.84 | (0.66, 1.08) |
Congestive heart failure | 0.86 | (0.70, 1.06) |
Urinary tract infection | 1.19 | (0.95, 1.50) |
Respiratory failure | 1.15 | (0.85, 1.55) |
Septicemia | 1.11 | (0.82, 1.50) |
Comorbidities | ||
Inflammatory bowel disease | 3.11 | (1.59, 6.08) |
Obesity | 1.28 | (0.99, 1.66) |
Inherited thrombophilia | 4.00 | (0.98, 16.40) |
Cancer | ||
18‐49 years | 4.62 | (2.72, 7.87) |
50‐64 years | 3.64 | (2.52, 5.25) |
>65 years | 2.17 | (1.61, 2.92) |
Treatments | ||
Central venous catheter | 1.87 | (1.52, 2.29) |
Mechanical ventilation | 1.61 | (1.27, 2.05) |
Urinary catheter | 1.17 | (0.99, 1.38) |
Chemotherapy | 1.71 | (1.03, 2.83) |
Steroids | 1.22 | (1.04, 1.43) |
In the derivation set, the multivariable model produced deciles of mean predicted risk from 0.11% to 1.45%, while mean observed risk over the same deciles ranged from 0.12% to 1.42% (Figure 1). Within the validation cohort, the observed rate of VTE was 0.46% (223 cases among 48,543 subjects). The expected rate according to the model was 0.43% (expected/observed ratio: 0.93 [95% CI 0.82, 1.06]). Model discrimination measured by the c‐statistic in the validation set was 0.75 (95% CI 0.71, 0.78). The model produced deciles of mean predicted risk from 0.11% to 1.46%, with mean observed risk over the same deciles from 0.17% to 1.81%. Risk gradient was relatively flat across the first 6 deciles, began to rise at the seventh decile, and rose sharply in the highest one. Using a risk threshold of 1%, the model had a sensitivity of 28% and a specificity of 93%. In the validation set, this translated into a positive predictive value of 2.2% and a negative predictive value of 99.7%. Assuming that VTE prophylaxis has an efficacy of 50%, the number‐needed‐to‐treat to prevent one VTE among high‐risk patients (predicted risk >1%) would be 91. In contrast, providing prophylaxis to the entire validation sample would result in a number‐needed‐to‐treat of 435. Using a lower treatment threshold of 0.4% produced a positive predictive value of 1% and a negative predictive value of 99.8%. At this threshold, the model would detect 73% of patients with VTE and the number‐needed‐to‐treat to prevent one VTE would be 200.
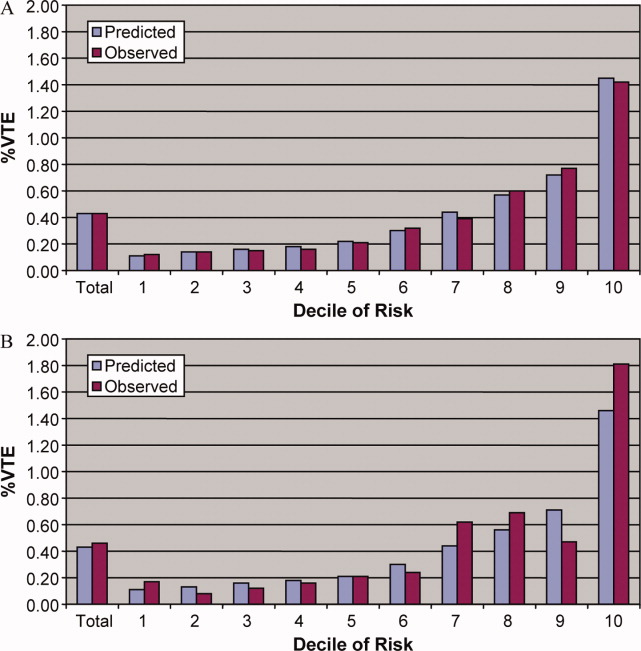
Discussion
In a representative sample of 243,000 hospitalized medical patients with at least one major risk factor for VTE, we found that symptomatic VTE was an uncommon event, occurring in approximately 1 in 231 patients. We identified a number of factors that were associated with an increased risk of VTE, but many previously cited risk factors did not show an association in multivariable models. In particular, patients with a primary diagnosis of COPD appeared not to share the same high risk of VTE as patients with the other diagnoses we examined, a finding reported by others.11 The risk model we developed accurately stratifies patients across a wide range of VTE probabilities, but even among those with the highest predicted rates, symptomatic VTE occurred in less than 2%.
VTE is often described as a frequent complication of hospitalization for medical illness and one of the most common potentially preventable causes of death. Indeed, rates of asymptomatic VTE have been demonstrated to be 3.7% to 26%.12 Although some of these might have fatal consequences, most are distal vein thromboses and their significance is unknown. In contrast, symptomatic events are uncommon, with previous estimates among general medical patients in observational studies in the range of 0.3%3 to 0.8%,12 similar to the rate observed in our study. Symptomatic event rates among control patients in landmark randomized trials have ranged from 0.86%13 to 2.3%,14 but these studies enrolled only very high‐risk patients with more extended hospitalizations, and may involve follow‐up periods of a month or more.
Because it is unlikely that our diagnostic algorithm was 100% sensitive, and because 30% of our patients received chemoprophylaxis, it is probable that we have underestimated the true rate of VTE in our sample. Among the patients who received prophylaxis, the observed rate of VTE was 0.54%. If we assume that prophylaxis is 50% effective, then had these patients not received prophylaxis, their rate of VTE would have been 1.08% (vs 0.39% among those patients who received no prophylaxis) and the overall rate of VTE for the sample would have been 0.60% (1.08 0.30 + 0.39 0.70). If we further assume that our algorithm was only 80% sensitive and 100% specific, the true underlying rate of symptomatic VTE could have been as high as 0.75%, still less than half that seen in randomized trials.
Prophylaxis with heparin has been shown to decrease the rate of both asymptomatic and symptomatic events, but because of the low prevalence, the number‐needed‐to‐treat to prevent one symptomatic pulmonary embolism has been estimated at 345, and prophylaxis has not been shown to affect all‐cause mortality.4, 15 At the same time, prophylaxis costs money, is uncomfortable, and carries a small risk of bleeding and heparin‐induced thrombocytopenia. Given the generally low incidence of symptomatic VTE, it therefore makes sense to reserve prophylaxis for patients at higher risk of thromboembolism.
To decide whether prophylaxis is appropriate for a given patient, it is necessary to quantify the patient's risk and then apply an appropriate threshold for treatment. The National Quality Forum (NQF) recommends,16 and JCAHO has adopted, that a clinician must evaluate each patient upon admission, and regularly thereafter, for the risk of developing DVT [deep vein thrombosis]/VTE. Until now, however, there has been no widely accepted, validated method to risk stratify medical patients. The ACCP recommendations cite just three studies of VTE risk factors in hospitalized medical patients.11, 17, 18 Together they examined 477 cases and 1197 controls, identifying congestive heart failure, pneumonia, cancer, and previous VTE as risk factors. Predictive models based on these factors17, 1921 have not been subjected to validation or have performed poorly.18 Acknowledging this lack of standardized risk assessment, JCAHO leaves the means of assessment to individual hospitals. A quality improvement guide published by the Agency for Healthcare Research and Quality goes one step further, stating that In a typical hospital, it is estimated that fewer than 5% of medical patients could be considered at low risk by most VTE risk stratification methods.22 The guide recommends near universal VTE prophylaxis.
In light of the JCAHO requirements, our model should be welcomed by hospitalists. Rather than assuming that all patients over 40 years of age are at high risk, our model will enable clinicians to risk stratify patients from a low of 0.1% to >1.4% (>10‐fold increase in risk). Moreover, the model was derived from more than 800 episodes of symptomatic VTE among almost 190,000 general medical patients and validated on almost 50,000 more. The observed patients were cared for in clinical practice at a nationally representative group of US hospitals, not in a highly selected clinical trial, increasing the generalizability of our findings. Finally, the model includes ten common risk factors that can easily be entered into decision support software or extracted automatically from the electronic medical record. Electronic reminder systems have already been shown to increase use of VTE prophylaxis, and prevent VTE, especially among cancer patients.23
A more challenging task is defining the appropriate risk threshold to initiate VTE prophylaxis. The Thromboembolic Risk Factors (THRIFT) Consensus Group classified patients according to risk of proximal DVT as low (<1%), moderate (1%‐10%), and high (>10%).21 They recommended heparin prophylaxis for all patients at moderate risk or higher. Although the patients included in our study all had a diagnosis that warranted prophylaxis according to the ACCP guidelines, using the THRIFT threshold for moderate‐to‐high risk, only 7% of our patients should have received prophylaxis. The recommendation not to offer heparin prophylaxis to patients with less than 1% chance of developing symptomatic VTE seems reasonable, given the large number‐needed‐to‐treat, but formal decision analyses should be conducted to better define this threshold. Many hospitalists, however, may feel uncomfortable using the 1% threshold, because our model failed to identify almost three out of four patients who ultimately experienced symptomatic VTE. At that threshold, it would seem that hospital‐acquired VTE is not a preventable complication in most medical patients, as others have pointed out.3, 24 Alternatively, if the threshold were lowered to 0.4%, our model could reduce the use of prophylaxis by 60%, while still identifying three‐fourths of all VTE cases. Further research is needed to know whether such a threshold is reasonable.
Our study has a number of important limitations. First, we relied on claims data, not chart review. We do not know for certain which patients experienced VTE, although our definition of VTE required diagnosis codes plus charges for both diagnosis and treatment. Moreover, our rates are similar to those observed in other trials where symptomatic events were confirmed. Second, about 30% of our patients received at least some VTE prophylaxis, and this may have prevented as many as half of the VTEs in that group. Without prophylaxis, rates might have been 20%30% higher. Similarly, we could not detect patients who were diagnosed after discharge but not admitted to hospital. While we believe this number to be small, it would again increase the rate slightly. Third, we could not assess certain clinical circumstances that are not associated with hospital charges or diagnosis codes, especially prolonged bed rest. Other risk factors, such as the urinary catheter, were probably surrogate markers for immobilization rather than true risk factors. Fourth, we included length of stay in our prediction model. We did this because most randomized trials of VTE prophylaxis included only patients with an expected length of stay 6 days. Physicians' estimates about probable length of stay may be less accurate than actual length of stay as a predictor of VTE. Moreover, the relationship may have been confounded if hospital‐acquired VTE led to longer lengths of stay. We think this unlikely since many of the events were discovered on readmission. Fifth, we studied only patients carrying high‐risk diagnoses, and therefore do not know the baseline risk for patients with less risky conditions, although it should be lower than what we observed. It seems probable that COPD, rather than being protective, as it appears in our model, actually represents the baseline risk for low‐risk diagnoses. It should be noted that we did include a number of other high‐risk diagnoses, such as cancer and inflammatory bowel disease, as secondary diagnoses. A larger, more inclusive study should be conducted to validate our model in other populations. Finally, we cannot know who died of undiagnosed VTE, either in the hospital or after discharge. Such an outcome would be important, but those events are likely to be rare, and VTE prophylaxis has not been shown to affect mortality.
VTE remains a daunting problem in hospitalized medical patients. Although VTE is responsible for a large number of hospital deaths each year, identifying patients at high risk for clinically important VTE is challenging, and may contribute to the persistently low rates of VTE prophylaxis seen in hospitals.25 Current efforts to treat nearly all patients are likely to lead to unnecessary cost, discomfort, and side effects. We present a simple logistic regression model that can easily identify patients at moderate‐to‐high risk (>1%) of developing symptomatic VTE. Future studies should focus on prospectively validating the model in a wider spectrum of medical illness, and better defining the appropriate risk cutoff for general prophylaxis.
Acknowledgements
The authors thank Aruna Priya, MS, for her help with some of the statistical analyses.
- A comparison of enoxaparin with placebo for the prevention of venous thromboembolism in acutely ill medical patients. Prophylaxis in Medical Patients with Enoxaparin Study Group.N Engl J Med.1999;341(11):793–800. , , , et al.
- Prevention of venous thromboembolism: American College of Chest Physicians Evidence‐Based Clinical Practice Guidelines (8th ed).Chest.2008;133(6 suppl):381S–453S. , , , et al.
- Thrombosis prophylaxis in hospitalised medical patients: does prophylaxis in all patients make sense?Neth J Med.2000;56(5):171–176. , , .
- Pharmacological venous thromboembolism prophylaxis in hospitalized medical patients: a meta‐analysis of randomized controlled trials.Arch Intern Med.2007;167(14):1476–1486. , , , , .
- The Joint Commission on the Accreditation of Healthcare Organizations. Venous thromboembolism (VTE) core measure set. Available at: http://www. jointcommission.org/PerformanceMeasurement/PerformanceMeasurement/VTE.htm. Accessed June 1,2009.
- Effective risk stratification of surgical and nonsurgical patients for venous thromboembolic disease.Semin Hematol.2001;38(2 suppl 5):12–19. , , .
- Assessment of venous thromboembolism risk and the benefits of thromboprophylaxis in medical patients.Thromb Haemost.2005;94(4):750–759. , , , et al.
- Prevention of venous thromboembolism: the Seventh ACCP Conference on Antithrombotic and Thrombolytic Therapy.Chest.2004;126(3 suppl):338S–400S. , , , et al.
- Comorbidity measures for use with administrative data.Med Care.1998;36(1):8–27. , , , .
- Identification of in‐hospital complications from claims data. Is it valid?Med Care.2000;38(8):785–795. , , , , , .
- Risk factors for venous thromboembolism in hospitalized patients with acute medical illness: analysis of the MEDENOX Study.Arch Intern Med.2004;164(9):963–968. , , , et al.
- The magnitude of an iatrogenic disorder: a systematic review of the incidence of venous thromboembolism for general medical inpatients.Thromb Haemost.2006;95(5):758–762. , , .
- Randomized, placebo‐controlled trial of dalteparin for the prevention of venous thromboembolism in acutely ill medical patients.Circulation.2004;110(7):874–879. , , , , , .
- Randomised, controlled trial of low‐dose heparin for prevention of fatal pulmonary embolism in patients with infectious diseases. The Heparin Prophylaxis Study Group.Lancet.1996;347(9012):1357–1361. .
- Meta‐analysis: anticoagulant prophylaxis to prevent symptomatic venous thromboembolism in hospitalized medical patients.Ann Intern Med.2007;146(4):278–288. , , , , .
- National Quality Forum.National Voluntary Consensus Standards for Prevention and Care of Venous Thromboembolism: Policy, Preferred Practices, and Initial Performance Measures.Washington, DC;2006.
- Risk factors for deep vein thrombosis in inpatients aged 65 and older: a case‐control multicenter study.J Am Geriatr Soc.2004;52(8):1299–1304. , , , et al.
- Risk factors for venous thrombosis in medical inpatients: validation of a thrombosis risk score.J Thromb Haemost.2004;2(12):2156–2161. , , .
- Venous thromboembolism prophylaxis and risk assessment in medical patients.Semin Thromb Hemost.1991;17(suppl 3):313–318. , , , , , .
- A population‐based perspective of the hospital incidence and case‐fatality rates of deep vein thrombosis and pulmonary embolism. The Worcester DVT Study.Arch Intern Med.1991;151(5):933–938. , , , et al.
- Thromboembolic Risk Factors (THRIFT) Consensus Group.Risk of and prophylaxis for venous thromboembolism in hospital patients.BMJ.1992;305(6853):567–574.
- Preventing Hospital‐Acquired Venous Thromboembolism: A Guide for Effective Quality Improvement. AHRQ Publication No. 08–0075.Rockville, MD:Agency for Healthcare Research and Quality;2008. , .
- Electronic alerts to prevent venous thromboembolism among hospitalized patients.N Engl J Med.2005;352(10):969–977. , , , et al.
- Prophylaxis against venous thromboembolism.BMJ.1992;305(6862):1156. , , .
- Prevention of in‐hospital VTE: why can't we do better?Lancet.2008;371(9610):361–362. , .
- A comparison of enoxaparin with placebo for the prevention of venous thromboembolism in acutely ill medical patients. Prophylaxis in Medical Patients with Enoxaparin Study Group.N Engl J Med.1999;341(11):793–800. , , , et al.
- Prevention of venous thromboembolism: American College of Chest Physicians Evidence‐Based Clinical Practice Guidelines (8th ed).Chest.2008;133(6 suppl):381S–453S. , , , et al.
- Thrombosis prophylaxis in hospitalised medical patients: does prophylaxis in all patients make sense?Neth J Med.2000;56(5):171–176. , , .
- Pharmacological venous thromboembolism prophylaxis in hospitalized medical patients: a meta‐analysis of randomized controlled trials.Arch Intern Med.2007;167(14):1476–1486. , , , , .
- The Joint Commission on the Accreditation of Healthcare Organizations. Venous thromboembolism (VTE) core measure set. Available at: http://www. jointcommission.org/PerformanceMeasurement/PerformanceMeasurement/VTE.htm. Accessed June 1,2009.
- Effective risk stratification of surgical and nonsurgical patients for venous thromboembolic disease.Semin Hematol.2001;38(2 suppl 5):12–19. , , .
- Assessment of venous thromboembolism risk and the benefits of thromboprophylaxis in medical patients.Thromb Haemost.2005;94(4):750–759. , , , et al.
- Prevention of venous thromboembolism: the Seventh ACCP Conference on Antithrombotic and Thrombolytic Therapy.Chest.2004;126(3 suppl):338S–400S. , , , et al.
- Comorbidity measures for use with administrative data.Med Care.1998;36(1):8–27. , , , .
- Identification of in‐hospital complications from claims data. Is it valid?Med Care.2000;38(8):785–795. , , , , , .
- Risk factors for venous thromboembolism in hospitalized patients with acute medical illness: analysis of the MEDENOX Study.Arch Intern Med.2004;164(9):963–968. , , , et al.
- The magnitude of an iatrogenic disorder: a systematic review of the incidence of venous thromboembolism for general medical inpatients.Thromb Haemost.2006;95(5):758–762. , , .
- Randomized, placebo‐controlled trial of dalteparin for the prevention of venous thromboembolism in acutely ill medical patients.Circulation.2004;110(7):874–879. , , , , , .
- Randomised, controlled trial of low‐dose heparin for prevention of fatal pulmonary embolism in patients with infectious diseases. The Heparin Prophylaxis Study Group.Lancet.1996;347(9012):1357–1361. .
- Meta‐analysis: anticoagulant prophylaxis to prevent symptomatic venous thromboembolism in hospitalized medical patients.Ann Intern Med.2007;146(4):278–288. , , , , .
- National Quality Forum.National Voluntary Consensus Standards for Prevention and Care of Venous Thromboembolism: Policy, Preferred Practices, and Initial Performance Measures.Washington, DC;2006.
- Risk factors for deep vein thrombosis in inpatients aged 65 and older: a case‐control multicenter study.J Am Geriatr Soc.2004;52(8):1299–1304. , , , et al.
- Risk factors for venous thrombosis in medical inpatients: validation of a thrombosis risk score.J Thromb Haemost.2004;2(12):2156–2161. , , .
- Venous thromboembolism prophylaxis and risk assessment in medical patients.Semin Thromb Hemost.1991;17(suppl 3):313–318. , , , , , .
- A population‐based perspective of the hospital incidence and case‐fatality rates of deep vein thrombosis and pulmonary embolism. The Worcester DVT Study.Arch Intern Med.1991;151(5):933–938. , , , et al.
- Thromboembolic Risk Factors (THRIFT) Consensus Group.Risk of and prophylaxis for venous thromboembolism in hospital patients.BMJ.1992;305(6853):567–574.
- Preventing Hospital‐Acquired Venous Thromboembolism: A Guide for Effective Quality Improvement. AHRQ Publication No. 08–0075.Rockville, MD:Agency for Healthcare Research and Quality;2008. , .
- Electronic alerts to prevent venous thromboembolism among hospitalized patients.N Engl J Med.2005;352(10):969–977. , , , et al.
- Prophylaxis against venous thromboembolism.BMJ.1992;305(6862):1156. , , .
- Prevention of in‐hospital VTE: why can't we do better?Lancet.2008;371(9610):361–362. , .
Copyright © 2011 Society of Hospital Medicine
Macrolides and Quinolones for AECOPD
Acute exacerbations of chronic obstructive pulmonary disease (AECOPD) are responsible for more than 600,000 hospitalizations annually, resulting in direct costs of over $20 billion.1 Bacterial infections appear responsible for 50% of such exacerbations,25 and current COPD guidelines recommend treatment with antibiotics for patients with severe exacerbations or a change in sputum.1,69 These recommendations are based on a number of small randomized trials, most of which were conducted more than 20 years ago using narrow spectrum antibiotics that are no longer commonly used.10 Only 4 studies, totaling 321 subjects, included hospitalized patients, and most studies excluded patients who required steroids. Because no clinical trials have compared different antibiotic regimens for AECOPD, existing guidelines offer a range of treatment options, including amoxicillin‐clavulonate, macrolides, quinolones, cephalosporins, aminopenicillins, and tetracyclines.
Among hospitalized patients, macrolides and quinolones appear to be the most frequently prescribed antibiotics.11 Both are available in oral formulations, have excellent bioavailability, and are administered once daily. In addition to their antimicrobial activity, macrolides are believed to have antiinflammatory effects, which could be especially advantageous in AECOPD.1214 In trials of chronic bronchitis, however, fluoroquinolones have been shown to reduce the risk of recurrent exacerbation when compared to macrolides.15 The wide variation that has been observed in antibiotic selection for patients hospitalized for AECOPD suggests a high degree of uncertainty among clinicians about the benefits of different treatment options.11 Given the limited evidence from randomized trials, we sought to evaluate the comparative effectiveness of macrolides and quinolones among a large, representative sample of patients hospitalized with AECOPD.
Subjects and Methods
Setting and Subjects
We conducted a retrospective cohort study of all patients hospitalized between January 1 and December 31, 2001 for AECOPD at any 1 of 375 acute care facilities in the United States that participated in Premier's Perspective, a voluntary, fee‐supported database developed for measuring quality and healthcare utilization. Participating hospitals represent all geographical regions, and are primarily small‐sized to medium‐sized nonteaching hospitals located mostly in urban areas. In addition to the information contained in the standard hospital discharge file (Uniform Billing 92) such as patient age, International Classification of Disease, 9th Edition, Clinical Modification (ICD‐9‐CM) codes, the Perspective database includes a date‐stamped log of all billed items, including diagnostic tests, medications, and other treatments, as well as costs, for individual patients. The study was approved by the Institutional Review Board of Baystate Medical Center.
Patients were included if they had a primary diagnosis consistent with AECOPD (ICD‐9 codes 491.21 and 493.22) or a primary diagnosis of respiratory failure (ICD‐9 codes 518.81 and 518.84) paired with secondary diagnosis of AECOPD; they also had to receive at least 2 consecutive days of either a macrolide or a quinolone, started within 48 hours of hospitalization. Patients receiving both antibiotics were excluded, but patients who received additional antibiotics were included. To enhance the specificity of our diagnosis codes, we limited our study to patients age 40 years.16 Because mechanical ventilation initiated after hospital day 2 was an outcome measure, we excluded patients admitted directly to the intensive care unit. We also excluded: those with other bacterial infections, such as pneumonia or cellulitis, who might have another indication for antibiotics; those with a length of stay <2 days, because we could not ascertain whether they received a full course of antibiotics; patients with a secondary diagnosis of pulmonary embolism or pneumothorax; and those whose attending physicians were not internists, family practitioners, hospitalists, pulmonologists, or intensivists. For patients with more than 1 admission during the study period, we included only the first admission.
Data Elements
For each patient, we assessed age, gender, race, marital and insurance status, principal diagnosis, comorbidities, and specialty of the attending physician. Comorbidities were identified from ICD‐9 secondary diagnosis codes and Diagnosis Related Groups using Healthcare Cost and Utilization Project Comorbidity Software (version 3.1), based on the work of Elixhauser et al.17 In addition, to assess disease severity we recorded the presence of chronic pulmonary heart disease, the number of admissions for COPD during the 12 months prior to the index admission, and arterial blood gas testing.18,19 We also identified pharmacy or diagnostic charges for interventions that were recommended in current guidelines (beta‐adrenergic and anticholinergic bronchodilators, steroids, and noninvasive positive‐pressure ventilation); those that were not recommended or were of uncertain benefit (methylxanthine bronchodilators, spirometry/pulmonary function testing, mucolytic medications, chest physiotherapy, and sputum testing); and drugs that might be associated with severe exacerbations or end‐stage COPD (loop diuretics, morphine, and nutritional supplements).1,69 Hospitals were categorized by region (Northeast, South, Midwest, or West), bed size, setting (urban vs. rural), ownership, and teaching status.
Antibiotic Class and Outcome Variables
Our primary predictor variable was the antibiotic initiated during the first 2 hospital days and continued for at least 2 days, regardless of other antibiotics the patient may have received during the course of hospitalization. Because we anticipated low in‐hospital mortality, our primary outcome was a composite measure of treatment failure, defined as initiation of mechanical ventilation after hospital day 2, in‐hospital mortality, or readmission for COPD within 30 days of discharge.20 Secondary outcomes included hospital costs and length of stay, as well as allergic reactions identified by ICD‐9 code, and antibiotic‐associated diarrhea, defined as treatment with either metronidazole or oral vancomycin begun after hospital day 3.
Statistical Analysis
Summary statistics were computed using frequencies and percents for categorical variables; and means, medians, standard deviations, and interquartile ranges for continuous variables. Associations between antibiotic selection and patient and hospital characteristics were assessed using chi‐square tests for categorical variables and z‐tests for continuous variables.
We developed a series of multivariable models to evaluate the impact of initial antibiotic selection on the risk of treatment failure, length of stay, and total cost. In order to account for the effects of within‐hospital correlation, generalized estimating equation (GEE) models with a logit link were used to assess the effect of antibiotic selection on the risk of treatment failure, and identity link models were used for analyses of length of stay and cost. Unadjusted and covariate‐adjusted models for treatment failure were evaluated with and without adjustments for propensity score. A propensity score is the probability that a given patient would receive treatment with a macrolide, derived from a nonparsimonious model in which treatment with a macrolide was considered the outcome. The propensity model included all patient characteristics, other early treatments and tests, comorbidities, hospital and physician characteristics, and selected interaction terms.21 Length of stay and cost were trimmed at 3 standard deviations above the mean, and natural log‐transformed values were modeled due to extreme positive skew. In addition, we carried out matched analyses in which we compared the outcomes of patients who were treated with a macrolide to those with similar propensity scores (ie, with similar likelihood of receiving a macrolide) who received a quinolone.22
Finally, to reduce the threat of residual confounding by indication, which can occur if sicker patients are more likely to receive a particular antibiotic, we developed a grouped treatment model, in which all patients treated at the same hospital were assigned a probability of treatment with a macrolide equal to the overall treatment rate at that hospital.23 This is an adaptation of instrumental variable analysis, a well‐accepted technique in econometrics with growing use in health care.24,25 It attempts to assess whether patients treated at a hospital at which quinolones are used more frequently have better outcomes than patients treated at hospitals at which macrolides are used more frequently, while adjusting for other patient, physician, and hospital variables. It ignores the actual treatment the patient received, and instead substitutes the hospital's rate of macrolide use. By grouping treatment at the hospital level, this method greatly reduces the possibility of residual selection bias, unless hospitals that use a lot of macrolides have patients who differ in a consistent way from hospitals which use mostly quinolones.
All analyses were performed using SAS version 9.1 (SAS Institute, Inc., Cary, NC).
Results
Of 26,248 AECOPD patients treated with antibiotics, 19,608 patients met the inclusion criteria; of these, 6139 (31%) were treated initially with a macrolide; the median age was 70 years; 60% were female; and 78% were white. A total of 86% of patients had a primary diagnosis of obstructive chronic bronchitis with acute exacerbation, and 6% had respiratory failure. The most common comorbidities were hypertension, diabetes, and congestive heart failure. Twenty‐two percent had been admitted at least once in the preceding 12 months. Treatment failure occurred in 7.7% of patients, and 1.3% died in the hospital. Mean length of stay was 4.8 days. Hospital prescribing rates for macrolides varied from 0% to 100%, with a mean of 33% and an interquartile range of 14% to 46% (Supporting Appendix Figure 1).
Compared to patients receiving macrolides, those receiving quinolones were older, more likely to have respiratory failure, to be cared for by a pulmonologist, and to have an admission in the previous year (Table 1). They were also more likely to be treated with bronchodilators, methylxanthines, steroids, diuretics, and noninvasive positive pressure ventilation, and to have an arterial blood gas, but less likely to receive concomitant treatment with a cephalosporin (11% vs. 57%). With the exception of cephalosporin treatment, these differences were small, but due to the large sample were statistically significant. Comorbidities were similar in both groups. Patients in the quinolone group were also more likely to experience treatment failure (8.1% vs. 6.8%), death (1.5% vs. 1.0%), and antibiotic‐associated diarrhea (1.1% vs. 0.5%).
Complete Cohort | Propensity‐matched Subsample | |||||
---|---|---|---|---|---|---|
Characteristic | Quinolone (n = 13469) | Macrolide (n = 6139) | P Value | Quinolone (n = 5610) | Macrolide (n = 5610) | P Value |
| ||||||
Antibiotics received during hospitalization* [n (%)] | ||||||
Macrolide | 264 (2) | 6139 (100) | 119 (2) | 5610 (100) | ||
Quinolone | 13469 (100) | 459 (8) | 5610 (100) | 424 (8) | ||
Cephalosporin | 1696 (13) | 3579 (59) | <0.001 | 726 (13) | 3305 (59) | <0.001 |
Tetracycline | 231 (2) | 75 (2) | 0.01 | 101 (2) | 73 (2) | 0.06 |
Other antibiotics | 397 (3) | 220 (4) | 0.02 | 166 (3) | 193 (3) | 0.03 |
Age (years) (mean [SD]) | 69.1 (11.4) | 68.2 (11.8) | <0.001 | 68.6 (11.7) | 68.5 (11.7) | 0.58 |
Male sex (n [%]) | 5447 (40) | 2440 (40) | 0.36 | 2207 (39) | 2196 (39) | 0.85 |
Race/ethnic group (n [%]) | <0.001 | 0.44 | ||||
White | 10454 (78) | 4758 (78) | 4359 (78) | 4368 (78) | ||
Black | 1060 (8) | 540 (9) | 470 (8) | 455 (8) | ||
Hispanic | 463 (3) | 144 (2) | 157 (3) | 134 (2) | ||
Other | 1492 (11) | 697 (11) | 624 (11) | 653 (12) | ||
Primary diagnosis (n [%]) | <0.001 | 0.78 | ||||
Obstructive chronic bronchitis with acute exacerbation | 11650 (87) | 5298 (86) | 4884 (87) | 4860 (87) | ||
Chronic obstructive asthma/asthma with COPD | 908 (7) | 569 (9) | 466 (8) | 486 (9) | ||
Respiratory failure | 911 (7) | 272 (4) | 260 (5) | 264 (5) | ||
Admissions in the prior year (n [%]) | <0.001 | 0.84 | ||||
0 | 9846 (73) | 4654 (76) | 4249 (76) | 4231 (75) | ||
1 | 1918 (14) | 816 (13) | 747 (13) | 750 (13) | ||
2+ | 1085 (8) | 445 (7) | 397 (7) | 420 (8) | ||
Missing | 620 (5) | 224 (4) | 217 (4) | 209 (4) | ||
Physician specialty (n [%]) | <0.001 | 0.84 | ||||
Internal medicine/hospitalist | 7069 (53) | 3321 (54) | 3032 (54) | 3072 (55) | ||
Family/general medicine | 3569 (27) | 2074 (34) | 1824 (33) | 1812 (32) | ||
Pulmonologist | 2776 (21) | 727 (12) | 738 (13) | 711 (13) | ||
Critical care/emntensivist | 55 (0) | 17 (0) | 16 (0) | 15 (0) | ||
Tests on hospital day 1 or 2 (n [%]) | ||||||
Arterial blood gas | 8084 (60) | 3377 (55) | <0.001 | 3195 (57) | 3129 (56) | 0.22 |
Sputum test | 1741 (13) | 766 (13) | 0.39 | 20 (0) | 16 (0) | 0.62 |
Medications/therapies on hospital day 1 or 2 (n [%]) | ||||||
Short‐acting bronchodilators | 7555 (56) | 3242 (53) | <0.001 | 2969 (53) | 2820 (50) | 0.005 |
Long‐acting beta‐2 agonists | 2068 (15) | 748 (12) | <0.001 | 704 (13) | 719 (13) | 0.69 |
Methylxanthine bronchodilators | 3051 (23) | 1149 (19) | <0.001 | 1102 (20) | 1093 (20) | 0.85 |
Steroids | 0.04 | 0.68 | ||||
Intravenous | 11148 (83) | 4989 (81) | 4547 (81) | 4581 (82) | ||
Oral | 772 (6) | 376 (6) | 334 (6) | 330 (6) | ||
Severity indicators (n [%]) | ||||||
Chronic pulmonary heart disease | 890 (7) | 401 (7) | 0.85 | 337 (6) | 368 (7) | 0.24 |
Sleep apnea | 586 (4) | 234 (4) | 0.08 | 211 (4) | 218 (4) | 0.77 |
Noninvasive positive pressure ventilation | 391 (3) | 128 (2) | <0.001 | 128 (2) | 114 (2) | 0.40 |
Loop diuretics | 4838 (36) | 1971 (32) | <0.001 | 1884 (34) | 1862 (33) | 0.67 |
Hospital characteristics (n [%]) | ||||||
Staffed beds | <0.001 | 0.71 | ||||
6200 | 3483 (26) | 1688 (28) | 1610 (29) | 1586 (28) | ||
201300 | 3132 (23) | 1198 (20) | 1174 (21) | 1154 (21) | ||
301500 | 4265 (32) | 2047 (33) | 1809 (32) | 1867 (33) | ||
500+ | 2589 (19) | 1206 (20) | 1017 (18) | 1003 (18) | ||
Hospital region (n [%]) | <0.001 | 0.65 | ||||
South | 8562 (64) | 3270 (53) | 3212 (57) | 3160 (56) | ||
Midwest | 2602 (19) | 1444 (24) | 1170 (21) | 1216 (22) | ||
Northeast | 1163 (9) | 871 (14) | 687 (12) | 704 (13) | ||
West | 1142 (9) | 554 (9) | 541 (10) | 530 (9) | ||
Teaching hospital | <0.001 | 0.63 | ||||
No | 12090 (90) | 5037 (82) | 4896 (87) | 4878 (87) | ||
Yes | 1379 (10) | 1102 (18) | 714 (13) | 732 (13) | ||
Comorbidities (n [%]) | ||||||
Congestive heart failure | 2673 (20) | 1147 (19) | 0.06 | 1081 (19) | 1060 (19) | 0.63 |
Metastatic cancer | 134 (1) | 27 (0) | <0.001 | 34 (1) | 38 (1) | 0.72 |
Depression | 1419 (11) | 669 (11) | 0.45 | 598 (11) | 603 (11) | 0.90 |
Deficiency anemias | 1155 (9) | 476 (8) | 0.05 | 426 (8) | 432 (8) | 0.86 |
Solid tumor without metastasis | 1487 (11) | 586 (10) | 0.002 | 550 (10) | 552 (10) | 0.97 |
Hypothyroidism | 1267 (9) | 527 (9) | 0.07 | 481 (9) | 482 (9) | 1.00 |
Peripheral vascular disease | 821 (6) | 312 (5) | 0.005 | 287 (5) | 288 (5) | 1.00 |
Paralysis | 165 (1) | 46 (1) | 0.003 | 49 (1) | 51 (1) | 0.92 |
Obesity | 957 (7) | 435 (7) | 0.98 | 386 (7) | 398 (7) | 0.68 |
Hypertension | 5793 (43) | 2688 (44) | 0.31 | 2474 (44) | 2468 (44) | 0.92 |
Diabetes | 0.04 | 0.45 | ||||
Without chronic complications | 2630 (20) | 1127 (18) | 1057 (19) | 1066 (19) | ||
With chronic complications | 298 (2) | 116 (2) | 115 (2) | 97 (2) |
In the unadjusted analysis, compared to patients receiving quinolones, those treated with macrolides were less likely to experience treatment failure (OR, 0.83; 95% CI, 0.740.94) (Table 2). Adjusting for all patient, hospital, and physician covariates, including the propensity for treatment with macrolides, increased the OR to 0.89 and the results were no longer significant (95% CI, 0.781.01). Propensity matching successfully balanced all measured covariates except for the use of short‐acting bronchodilators and additional antibiotics (Table 1). In the propensity‐matched sample (Figure 1), quinolone‐treated patients were more likely to experience antibiotic‐associated diarrhea (1.2% vs. 0.6%; P = 0.0003) and late mechanical ventilation (1.3% vs. 0.8%; P = 0.02). There were no differences in adjusted cost or length of stay between the 2 groups. The results of the grouped treatment analysis, substituting the hospital's specific rate of macrolide use in place of the actual treatment that each patient received suggested that the 2 antibiotics were associated with similar rates of treatment failure. The OR for a 100% hospital rate of macrolide treatment vs. a 0% rate was 1.01 (95% CI, 0.751.35).
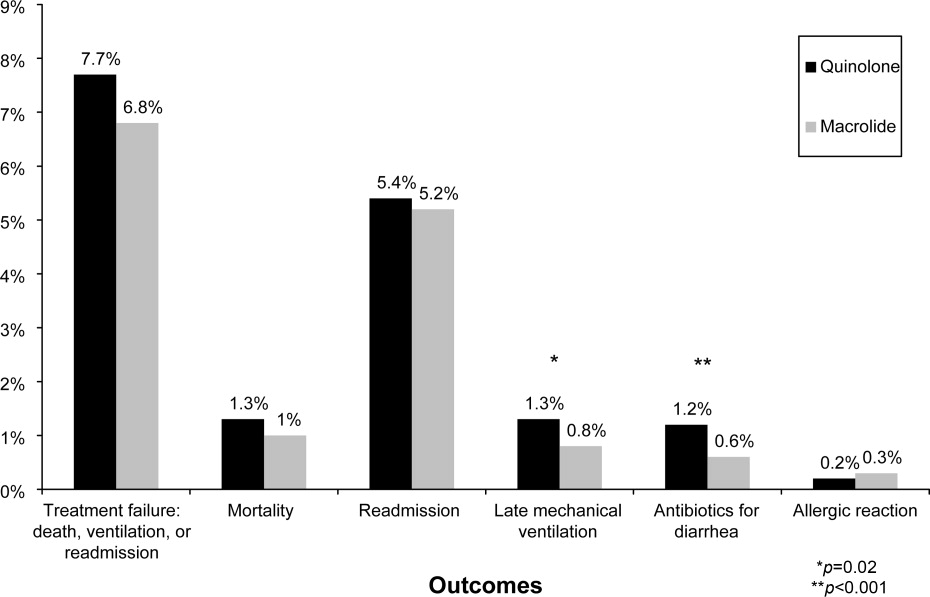
Treatment Failure | Cost | LOS | ||||
---|---|---|---|---|---|---|
Models | OR | 95% CI | Ratio | 95% CI | Ratio | 95% CI |
| ||||||
Unadjusted | 0.83 | 0.730.93 | 0.98 | 0.971.00 | 0.96 | 0.950.98 |
Adjusted for propensity score only* | 0.89 | 0.791.01 | 1.00 | 0.981.01 | 0.98 | 0.971.00 |
Adjusted for covariates | 0.87 | 0.770.99 | 1.00 | 0.991.02 | 0.99 | 0.971.00 |
Adjusted for covariates and propensity score | 0.89 | 0.781.01 | 1.00 | 0.991.02 | 0.98 | 0.971.00 |
Matched sample, unadjusted | 0.87 | 0.751.00 | 0.99 | 0.981.01 | 0.99 | 0.971.01 |
Matched sample, adjusted for unbalanced variables | 0.87 | 0.751.01 | 1.00 | 0.981.02 | 0.99 | 0.971.01 |
Grouped treatment model, unadjusted | 0.90 | 0.681.19 | 0.97 | 0.891.06 | 0.92 | 0.870.96 |
Group treatment model, adjusted for covariates∥ | 1.01 | 0.751.35 | 0.96 | 0.881.05 | 0.96 | 0.911.00 |
Discussion
In this large observational study conducted at 375 hospitals, we took advantage of a natural experiment in which antibiotic prescribing patterns varied widely across hospitals to compare the effectiveness of 2 common antibiotic regimens for AECOPD. Treatment with macrolides and quinolones were associated with a similar risk of treatment failure, costs, and length of stay; however, patients treated with macrolides were less likely to experience late mechanical ventilation or treatment for antibiotic‐associated diarrhea.
Despite broad consensus in COPD guidelines that patients with severe acute exacerbations should receive antibiotics, there is little agreement about the preferred empiric agent. Controversy exists regarding antibiotics' comparative effectiveness, and even over which pathogens cause COPD exacerbations. Given the frequency of hospitalization for AECOPD, understanding the comparative effectiveness of treatments in this setting could have important implications for health outcomes and costs. Unfortunately, most antibiotic studies in AECOPD were conducted >20 years ago, using antibiotics that rarely appeared in our sample.26 Consequently, clinical practice guidelines offer conflicting recommendations. For example, the National Institute for Clinical Excellence recommends empirical treatment with an aminopenicillin, a macrolide, or a tetracycline,8 while the American Thoracic Society recommends amoxicillin‐clavulonate or a fluoroquinolone.9
As might be expected in light of so much uncertainty, we found wide variation in prescribing patterns across hospitals. Overall, approximately one‐third of patients received a macrolide and two‐thirds a quinolone. Both regimens provide adequate coverage of H. influenza, S. pneumoniae, and M. catarrhalis, and conform to at least 1 COPD guideline. Nevertheless, patients receiving macrolides often received a cephalosporin as well; this pattern of treatment suggests that antibiotic selection is likely to have been influenced more by guidelines for the treatment of community‐acquired pneumonia than COPD.
Previous studies comparing antibiotic effectiveness suffer from shortcomings that limit their application to patients hospitalized with AECOPD. First, they enrolled patients with chronic bronchitis, and included patients without obstructive lung disease, and most studies included patients as young as 18 years old. Second, many either did not include treatment with steroids or excluded patients receiving more than 10 mg of prednisone daily. Third, almost all enrolled only ambulatory patients.
While there are no studies comparing quinolones and macrolides in patients hospitalized for AECOPD, a meta‐analysis comparing quinolones, macrolides, and amoxicillin‐clavulonate identified 19 trials of ambulatory patients with chronic bronchitis. That study found that all 3 drugs had similar efficacy initially, but that quinolones resulted in the fewest relapses over a 26‐week period.27 Macrolides and quinolones had similar rates of adverse effects. In contrast, we did not find a difference in treatment failure, cost, or length of stay, but did find a higher rate of diarrhea associated with quinolones. Others have also documented an association between fluoroquinolones and C. difficile diarrhea.2830 This trend, first noted in 2001, is of particular concern because the fluoroquinolone‐resistant strains appear to be hypervirulent and have been associated with nosocomial epidemics.3134
Our study has several limitations. First, its observational design leaves open the possibility of selection bias. For this reason we analyzed our data in several ways, including using a grouped treatment approach, an adaptation of the instrumental variable technique, and accepted only those differences which were consistent across all models. Second, our study used claims data, and therefore we could not directly adjust for physiological measures of severity. However, the highly detailed nature of the data allowed us to adjust for numerous tests and treatments that reflected the clinician's assessment of the patient's severity, as well as the number of prior COPD admissions. Third, we cannot exclude the possibility that some patients may have had concurrent pneumonia without an ICD‐9 code. We think that the number would be small because reimbursement for pneumonia is generally higher than for COPD, so hospitals have an incentive to code pneumonia as the principal diagnosis when present. Finally, we compared initial antibiotics only. More than one‐quarter of our patients received an additional antibiotic before discharge. In particular, patients receiving macrolides were often prescribed a concomitant cephalosporin. We do not know to what extent these additional antibiotics may have affected the outcomes.
Despite the large number of patients hospitalized annually for AECOPD, there are no randomized trials comparing different antibiotics in this population. Studies comparing antibiotics in chronic bronchitis can offer little guidance, since they have primarily focused on proving equivalence between existing antibiotics and newer, more expensive formulations.35 Because many of the patients enrolled in such trials do not benefit from antibiotics at all, either because they do not have COPD or because their exacerbation is not caused by bacteria, it is relatively easy to prove equivalence. Given that AECOPD is one of the leading causes of hospitalization in the United States, large, randomized trials comparing the effectiveness of different antibiotics should be a high priority. In the meantime, macrolides (often given together with cephalosporins) and quinolones appear to be equally effective initial antibiotic choices; considering antibiotic‐associated diarrhea, macrolides appear to be the safer of the 2.
- Evidence base for management of acute exacerbations of chronic obstructive pulmonary disease.Ann Intern Med.2001;134:595–599. , , .
- Infectious etiologies in acute exacerbation of COPD.Diagn Microbiol Infect Dis.2001;40:95–102. , , , et al.
- Bacterial infections in patients requiring admission for an acute exacerbation of COPD; a 1‐year prospective study.Respir Med.2003;97:770–777. , .
- Microbiologic determinants of exacerbation in chronic obstructive pulmonary disease.Arch Intern Med.2005;165:891–897. , , , et al.
- Infection in the pathogenesis and course of chronic obstructive pulmonary disease.N Engl J Med.2008;359:2355–2365. , .
- Global strategy for the diagnosis, management, and prevention of chronic obstructive pulmonary disease: GOLD executive summary.Am J Respir Crit Care Med.2007;176:532–555. , , , et al.
- Canadian Thoracic Society recommendations for management of chronic obstructive pulmonary disease—2008 update—highlights for primary care.Can Respir J.2008;15(suppl A):1A–8A. , , , et al.
- Chronic obstructive pulmonary disease.National clinical guideline on management of chronic obstructive pulmonary disease in adults in primary and secondary care.Thorax.2004;59(suppl 1):1–232.
- Standards for the diagnosis and treatment of patients with COPD: a summary of the ATS/ERS position paper.Eur Respir J.2004;23:932–946. , , , et al.
- Antibiotics for exacerbations of chronic obstructive pulmonary disease.Cochrane Database Syst Rev.2006:CD004403. , , , , .
- Quality of care for patients hospitalized for acute exacerbations of chronic obstructive pulmonary disease.Ann Intern Med.2006;144:894–903. , , , , , .
- Modulation of neutrophil and inflammation markers in chronic obstructive pulmonary disease by short‐term azithromycin treatment.Eur J Pharmacol.2005;517:132–143. , , , et al.
- Azithromycin modulates neutrophil function and circulating inflammatory mediators in healthy human subjects.Eur J Pharmacol.2002;450:277–289. , , , et al.
- The effect of clarithromycin on inflammatory markers in chronic obstructive pulmonary disease: preliminary data.Ann Pharmacother.2004;38:1400–1405. , , , , , .
- A comparison of gemifloxacin and clarithromycin in acute exacerbations of chronic bronchitis and long‐term clinical outcomes.Clin Ther.2002;24:639–652. , , , .
- In‐hospital mortality following acute exacerbations of chronic obstructive pulmonary disease.Arch Intern Med.2003;163:1180–1186. , , , .
- Comorbidity measures for use with administrative data.Med Care.1998;36:8–27. , , , .
- Outcomes following acute exacerbation of severe chronic obstructive lung disease. The SUPPORT investigators (Study to Understand Prognoses and Preferences for Outcomes and Risks of Treatments).Am J Respir Crit Care Med.1996;154:959–967. , , , et al.
- Mortality and mortality‐related factors after hospitalization for acute exacerbation of COPD.Chest.2003;124:459–467. , , .
- Effect of systemic glucocorticoids on exacerbations of chronic obstructive pulmonary disease.N Engl J Med.1999;340:1941–1947. , , , et al.
- Propensity score methods for bias reduction in the comparison of a treatment to a non‐randomized control group.Stat Med.1998;17:2265–2281. .
- Reducing bias in a propensity score matched‐pair sample using greedy matching techniques. Proceedings of the Twenty‐Sixth Annual SAS Users Group International Conference. Cary, NC: SAS Institute;2001,214–216. .
- Modeling treatment effects on binary outcomes with grouped‐treatment variables and individual covariates.Am J Epidemiol.2002;156:753–760. , , , .
- Does more intensive treatment of acute myocardial infarction in the elderly reduce mortality? Analysis using instrumental variables. [see Comment].JAMA.1994;272:859–866. , , .
- Analysis of observational studies in the presence of treatment selection bias: effects of invasive cardiac management on AMI survival using propensity score and instrumental variable methods.JAMA.2007;297:278–285. , , , , , .
- Antibiotics in chronic obstructive pulmonary disease exacerbations. A meta‐analysis.JAMA.1995;273:957–960. , , , .
- Macrolides, quinolones and amoxicillin/clavulanate for chronic bronchitis: a meta‐analysis.Eur Respir J.2007;29:1127–1137. , , , , .
- Antimicrobial‐associated risk factors for Clostridium difficile infection.Clin Infect Dis.2008;46(suppl 1):S19–S31. , , , , .
- Short‐term and long‐term outcomes of moxifloxacin compared to standard antibiotic treatment in acute exacerbations of chronic bronchitis.Chest.2004;125:953–964. , , , et al.
- Oral gemifloxacin once daily for 5 days compared with sequential therapy with i.v. ceftriaxone/oral cefuroxime (maximum of 10 days) in the treatment of hospitalized patients with acute exacerbations of chronic bronchitis.Respir Med.2003;97:242–249. , , , , .
- A large outbreak of Clostridium difficile‐associated disease with an unexpected proportion of deaths and colectomies at a teaching hospital following increased fluoroquinolone use.Infect Control Hosp Epidemiol.2005;26:273–280. , , , et al.
- An epidemic, toxin gene‐variant strain of Clostridium difficile.N Engl J Med.2005;353:2433–2441. , , , et al.
- Outbreak of Clostridium difficile infection in a long‐term care facility: association with gatifloxacin use.Clin Infect Dis.2004;38:640–645. , , , et al.
- A predominantly clonal multi‐institutional outbreak of Clostridium difficile‐associated diarrhea with high morbidity and mortality.N Engl J Med.2005;353:2442–2449. , , , et al.
- No more equivalence trials for antibiotics in exacerbations of COPD, please.Chest.2004;125:811–813. , .
Acute exacerbations of chronic obstructive pulmonary disease (AECOPD) are responsible for more than 600,000 hospitalizations annually, resulting in direct costs of over $20 billion.1 Bacterial infections appear responsible for 50% of such exacerbations,25 and current COPD guidelines recommend treatment with antibiotics for patients with severe exacerbations or a change in sputum.1,69 These recommendations are based on a number of small randomized trials, most of which were conducted more than 20 years ago using narrow spectrum antibiotics that are no longer commonly used.10 Only 4 studies, totaling 321 subjects, included hospitalized patients, and most studies excluded patients who required steroids. Because no clinical trials have compared different antibiotic regimens for AECOPD, existing guidelines offer a range of treatment options, including amoxicillin‐clavulonate, macrolides, quinolones, cephalosporins, aminopenicillins, and tetracyclines.
Among hospitalized patients, macrolides and quinolones appear to be the most frequently prescribed antibiotics.11 Both are available in oral formulations, have excellent bioavailability, and are administered once daily. In addition to their antimicrobial activity, macrolides are believed to have antiinflammatory effects, which could be especially advantageous in AECOPD.1214 In trials of chronic bronchitis, however, fluoroquinolones have been shown to reduce the risk of recurrent exacerbation when compared to macrolides.15 The wide variation that has been observed in antibiotic selection for patients hospitalized for AECOPD suggests a high degree of uncertainty among clinicians about the benefits of different treatment options.11 Given the limited evidence from randomized trials, we sought to evaluate the comparative effectiveness of macrolides and quinolones among a large, representative sample of patients hospitalized with AECOPD.
Subjects and Methods
Setting and Subjects
We conducted a retrospective cohort study of all patients hospitalized between January 1 and December 31, 2001 for AECOPD at any 1 of 375 acute care facilities in the United States that participated in Premier's Perspective, a voluntary, fee‐supported database developed for measuring quality and healthcare utilization. Participating hospitals represent all geographical regions, and are primarily small‐sized to medium‐sized nonteaching hospitals located mostly in urban areas. In addition to the information contained in the standard hospital discharge file (Uniform Billing 92) such as patient age, International Classification of Disease, 9th Edition, Clinical Modification (ICD‐9‐CM) codes, the Perspective database includes a date‐stamped log of all billed items, including diagnostic tests, medications, and other treatments, as well as costs, for individual patients. The study was approved by the Institutional Review Board of Baystate Medical Center.
Patients were included if they had a primary diagnosis consistent with AECOPD (ICD‐9 codes 491.21 and 493.22) or a primary diagnosis of respiratory failure (ICD‐9 codes 518.81 and 518.84) paired with secondary diagnosis of AECOPD; they also had to receive at least 2 consecutive days of either a macrolide or a quinolone, started within 48 hours of hospitalization. Patients receiving both antibiotics were excluded, but patients who received additional antibiotics were included. To enhance the specificity of our diagnosis codes, we limited our study to patients age 40 years.16 Because mechanical ventilation initiated after hospital day 2 was an outcome measure, we excluded patients admitted directly to the intensive care unit. We also excluded: those with other bacterial infections, such as pneumonia or cellulitis, who might have another indication for antibiotics; those with a length of stay <2 days, because we could not ascertain whether they received a full course of antibiotics; patients with a secondary diagnosis of pulmonary embolism or pneumothorax; and those whose attending physicians were not internists, family practitioners, hospitalists, pulmonologists, or intensivists. For patients with more than 1 admission during the study period, we included only the first admission.
Data Elements
For each patient, we assessed age, gender, race, marital and insurance status, principal diagnosis, comorbidities, and specialty of the attending physician. Comorbidities were identified from ICD‐9 secondary diagnosis codes and Diagnosis Related Groups using Healthcare Cost and Utilization Project Comorbidity Software (version 3.1), based on the work of Elixhauser et al.17 In addition, to assess disease severity we recorded the presence of chronic pulmonary heart disease, the number of admissions for COPD during the 12 months prior to the index admission, and arterial blood gas testing.18,19 We also identified pharmacy or diagnostic charges for interventions that were recommended in current guidelines (beta‐adrenergic and anticholinergic bronchodilators, steroids, and noninvasive positive‐pressure ventilation); those that were not recommended or were of uncertain benefit (methylxanthine bronchodilators, spirometry/pulmonary function testing, mucolytic medications, chest physiotherapy, and sputum testing); and drugs that might be associated with severe exacerbations or end‐stage COPD (loop diuretics, morphine, and nutritional supplements).1,69 Hospitals were categorized by region (Northeast, South, Midwest, or West), bed size, setting (urban vs. rural), ownership, and teaching status.
Antibiotic Class and Outcome Variables
Our primary predictor variable was the antibiotic initiated during the first 2 hospital days and continued for at least 2 days, regardless of other antibiotics the patient may have received during the course of hospitalization. Because we anticipated low in‐hospital mortality, our primary outcome was a composite measure of treatment failure, defined as initiation of mechanical ventilation after hospital day 2, in‐hospital mortality, or readmission for COPD within 30 days of discharge.20 Secondary outcomes included hospital costs and length of stay, as well as allergic reactions identified by ICD‐9 code, and antibiotic‐associated diarrhea, defined as treatment with either metronidazole or oral vancomycin begun after hospital day 3.
Statistical Analysis
Summary statistics were computed using frequencies and percents for categorical variables; and means, medians, standard deviations, and interquartile ranges for continuous variables. Associations between antibiotic selection and patient and hospital characteristics were assessed using chi‐square tests for categorical variables and z‐tests for continuous variables.
We developed a series of multivariable models to evaluate the impact of initial antibiotic selection on the risk of treatment failure, length of stay, and total cost. In order to account for the effects of within‐hospital correlation, generalized estimating equation (GEE) models with a logit link were used to assess the effect of antibiotic selection on the risk of treatment failure, and identity link models were used for analyses of length of stay and cost. Unadjusted and covariate‐adjusted models for treatment failure were evaluated with and without adjustments for propensity score. A propensity score is the probability that a given patient would receive treatment with a macrolide, derived from a nonparsimonious model in which treatment with a macrolide was considered the outcome. The propensity model included all patient characteristics, other early treatments and tests, comorbidities, hospital and physician characteristics, and selected interaction terms.21 Length of stay and cost were trimmed at 3 standard deviations above the mean, and natural log‐transformed values were modeled due to extreme positive skew. In addition, we carried out matched analyses in which we compared the outcomes of patients who were treated with a macrolide to those with similar propensity scores (ie, with similar likelihood of receiving a macrolide) who received a quinolone.22
Finally, to reduce the threat of residual confounding by indication, which can occur if sicker patients are more likely to receive a particular antibiotic, we developed a grouped treatment model, in which all patients treated at the same hospital were assigned a probability of treatment with a macrolide equal to the overall treatment rate at that hospital.23 This is an adaptation of instrumental variable analysis, a well‐accepted technique in econometrics with growing use in health care.24,25 It attempts to assess whether patients treated at a hospital at which quinolones are used more frequently have better outcomes than patients treated at hospitals at which macrolides are used more frequently, while adjusting for other patient, physician, and hospital variables. It ignores the actual treatment the patient received, and instead substitutes the hospital's rate of macrolide use. By grouping treatment at the hospital level, this method greatly reduces the possibility of residual selection bias, unless hospitals that use a lot of macrolides have patients who differ in a consistent way from hospitals which use mostly quinolones.
All analyses were performed using SAS version 9.1 (SAS Institute, Inc., Cary, NC).
Results
Of 26,248 AECOPD patients treated with antibiotics, 19,608 patients met the inclusion criteria; of these, 6139 (31%) were treated initially with a macrolide; the median age was 70 years; 60% were female; and 78% were white. A total of 86% of patients had a primary diagnosis of obstructive chronic bronchitis with acute exacerbation, and 6% had respiratory failure. The most common comorbidities were hypertension, diabetes, and congestive heart failure. Twenty‐two percent had been admitted at least once in the preceding 12 months. Treatment failure occurred in 7.7% of patients, and 1.3% died in the hospital. Mean length of stay was 4.8 days. Hospital prescribing rates for macrolides varied from 0% to 100%, with a mean of 33% and an interquartile range of 14% to 46% (Supporting Appendix Figure 1).
Compared to patients receiving macrolides, those receiving quinolones were older, more likely to have respiratory failure, to be cared for by a pulmonologist, and to have an admission in the previous year (Table 1). They were also more likely to be treated with bronchodilators, methylxanthines, steroids, diuretics, and noninvasive positive pressure ventilation, and to have an arterial blood gas, but less likely to receive concomitant treatment with a cephalosporin (11% vs. 57%). With the exception of cephalosporin treatment, these differences were small, but due to the large sample were statistically significant. Comorbidities were similar in both groups. Patients in the quinolone group were also more likely to experience treatment failure (8.1% vs. 6.8%), death (1.5% vs. 1.0%), and antibiotic‐associated diarrhea (1.1% vs. 0.5%).
Complete Cohort | Propensity‐matched Subsample | |||||
---|---|---|---|---|---|---|
Characteristic | Quinolone (n = 13469) | Macrolide (n = 6139) | P Value | Quinolone (n = 5610) | Macrolide (n = 5610) | P Value |
| ||||||
Antibiotics received during hospitalization* [n (%)] | ||||||
Macrolide | 264 (2) | 6139 (100) | 119 (2) | 5610 (100) | ||
Quinolone | 13469 (100) | 459 (8) | 5610 (100) | 424 (8) | ||
Cephalosporin | 1696 (13) | 3579 (59) | <0.001 | 726 (13) | 3305 (59) | <0.001 |
Tetracycline | 231 (2) | 75 (2) | 0.01 | 101 (2) | 73 (2) | 0.06 |
Other antibiotics | 397 (3) | 220 (4) | 0.02 | 166 (3) | 193 (3) | 0.03 |
Age (years) (mean [SD]) | 69.1 (11.4) | 68.2 (11.8) | <0.001 | 68.6 (11.7) | 68.5 (11.7) | 0.58 |
Male sex (n [%]) | 5447 (40) | 2440 (40) | 0.36 | 2207 (39) | 2196 (39) | 0.85 |
Race/ethnic group (n [%]) | <0.001 | 0.44 | ||||
White | 10454 (78) | 4758 (78) | 4359 (78) | 4368 (78) | ||
Black | 1060 (8) | 540 (9) | 470 (8) | 455 (8) | ||
Hispanic | 463 (3) | 144 (2) | 157 (3) | 134 (2) | ||
Other | 1492 (11) | 697 (11) | 624 (11) | 653 (12) | ||
Primary diagnosis (n [%]) | <0.001 | 0.78 | ||||
Obstructive chronic bronchitis with acute exacerbation | 11650 (87) | 5298 (86) | 4884 (87) | 4860 (87) | ||
Chronic obstructive asthma/asthma with COPD | 908 (7) | 569 (9) | 466 (8) | 486 (9) | ||
Respiratory failure | 911 (7) | 272 (4) | 260 (5) | 264 (5) | ||
Admissions in the prior year (n [%]) | <0.001 | 0.84 | ||||
0 | 9846 (73) | 4654 (76) | 4249 (76) | 4231 (75) | ||
1 | 1918 (14) | 816 (13) | 747 (13) | 750 (13) | ||
2+ | 1085 (8) | 445 (7) | 397 (7) | 420 (8) | ||
Missing | 620 (5) | 224 (4) | 217 (4) | 209 (4) | ||
Physician specialty (n [%]) | <0.001 | 0.84 | ||||
Internal medicine/hospitalist | 7069 (53) | 3321 (54) | 3032 (54) | 3072 (55) | ||
Family/general medicine | 3569 (27) | 2074 (34) | 1824 (33) | 1812 (32) | ||
Pulmonologist | 2776 (21) | 727 (12) | 738 (13) | 711 (13) | ||
Critical care/emntensivist | 55 (0) | 17 (0) | 16 (0) | 15 (0) | ||
Tests on hospital day 1 or 2 (n [%]) | ||||||
Arterial blood gas | 8084 (60) | 3377 (55) | <0.001 | 3195 (57) | 3129 (56) | 0.22 |
Sputum test | 1741 (13) | 766 (13) | 0.39 | 20 (0) | 16 (0) | 0.62 |
Medications/therapies on hospital day 1 or 2 (n [%]) | ||||||
Short‐acting bronchodilators | 7555 (56) | 3242 (53) | <0.001 | 2969 (53) | 2820 (50) | 0.005 |
Long‐acting beta‐2 agonists | 2068 (15) | 748 (12) | <0.001 | 704 (13) | 719 (13) | 0.69 |
Methylxanthine bronchodilators | 3051 (23) | 1149 (19) | <0.001 | 1102 (20) | 1093 (20) | 0.85 |
Steroids | 0.04 | 0.68 | ||||
Intravenous | 11148 (83) | 4989 (81) | 4547 (81) | 4581 (82) | ||
Oral | 772 (6) | 376 (6) | 334 (6) | 330 (6) | ||
Severity indicators (n [%]) | ||||||
Chronic pulmonary heart disease | 890 (7) | 401 (7) | 0.85 | 337 (6) | 368 (7) | 0.24 |
Sleep apnea | 586 (4) | 234 (4) | 0.08 | 211 (4) | 218 (4) | 0.77 |
Noninvasive positive pressure ventilation | 391 (3) | 128 (2) | <0.001 | 128 (2) | 114 (2) | 0.40 |
Loop diuretics | 4838 (36) | 1971 (32) | <0.001 | 1884 (34) | 1862 (33) | 0.67 |
Hospital characteristics (n [%]) | ||||||
Staffed beds | <0.001 | 0.71 | ||||
6200 | 3483 (26) | 1688 (28) | 1610 (29) | 1586 (28) | ||
201300 | 3132 (23) | 1198 (20) | 1174 (21) | 1154 (21) | ||
301500 | 4265 (32) | 2047 (33) | 1809 (32) | 1867 (33) | ||
500+ | 2589 (19) | 1206 (20) | 1017 (18) | 1003 (18) | ||
Hospital region (n [%]) | <0.001 | 0.65 | ||||
South | 8562 (64) | 3270 (53) | 3212 (57) | 3160 (56) | ||
Midwest | 2602 (19) | 1444 (24) | 1170 (21) | 1216 (22) | ||
Northeast | 1163 (9) | 871 (14) | 687 (12) | 704 (13) | ||
West | 1142 (9) | 554 (9) | 541 (10) | 530 (9) | ||
Teaching hospital | <0.001 | 0.63 | ||||
No | 12090 (90) | 5037 (82) | 4896 (87) | 4878 (87) | ||
Yes | 1379 (10) | 1102 (18) | 714 (13) | 732 (13) | ||
Comorbidities (n [%]) | ||||||
Congestive heart failure | 2673 (20) | 1147 (19) | 0.06 | 1081 (19) | 1060 (19) | 0.63 |
Metastatic cancer | 134 (1) | 27 (0) | <0.001 | 34 (1) | 38 (1) | 0.72 |
Depression | 1419 (11) | 669 (11) | 0.45 | 598 (11) | 603 (11) | 0.90 |
Deficiency anemias | 1155 (9) | 476 (8) | 0.05 | 426 (8) | 432 (8) | 0.86 |
Solid tumor without metastasis | 1487 (11) | 586 (10) | 0.002 | 550 (10) | 552 (10) | 0.97 |
Hypothyroidism | 1267 (9) | 527 (9) | 0.07 | 481 (9) | 482 (9) | 1.00 |
Peripheral vascular disease | 821 (6) | 312 (5) | 0.005 | 287 (5) | 288 (5) | 1.00 |
Paralysis | 165 (1) | 46 (1) | 0.003 | 49 (1) | 51 (1) | 0.92 |
Obesity | 957 (7) | 435 (7) | 0.98 | 386 (7) | 398 (7) | 0.68 |
Hypertension | 5793 (43) | 2688 (44) | 0.31 | 2474 (44) | 2468 (44) | 0.92 |
Diabetes | 0.04 | 0.45 | ||||
Without chronic complications | 2630 (20) | 1127 (18) | 1057 (19) | 1066 (19) | ||
With chronic complications | 298 (2) | 116 (2) | 115 (2) | 97 (2) |
In the unadjusted analysis, compared to patients receiving quinolones, those treated with macrolides were less likely to experience treatment failure (OR, 0.83; 95% CI, 0.740.94) (Table 2). Adjusting for all patient, hospital, and physician covariates, including the propensity for treatment with macrolides, increased the OR to 0.89 and the results were no longer significant (95% CI, 0.781.01). Propensity matching successfully balanced all measured covariates except for the use of short‐acting bronchodilators and additional antibiotics (Table 1). In the propensity‐matched sample (Figure 1), quinolone‐treated patients were more likely to experience antibiotic‐associated diarrhea (1.2% vs. 0.6%; P = 0.0003) and late mechanical ventilation (1.3% vs. 0.8%; P = 0.02). There were no differences in adjusted cost or length of stay between the 2 groups. The results of the grouped treatment analysis, substituting the hospital's specific rate of macrolide use in place of the actual treatment that each patient received suggested that the 2 antibiotics were associated with similar rates of treatment failure. The OR for a 100% hospital rate of macrolide treatment vs. a 0% rate was 1.01 (95% CI, 0.751.35).
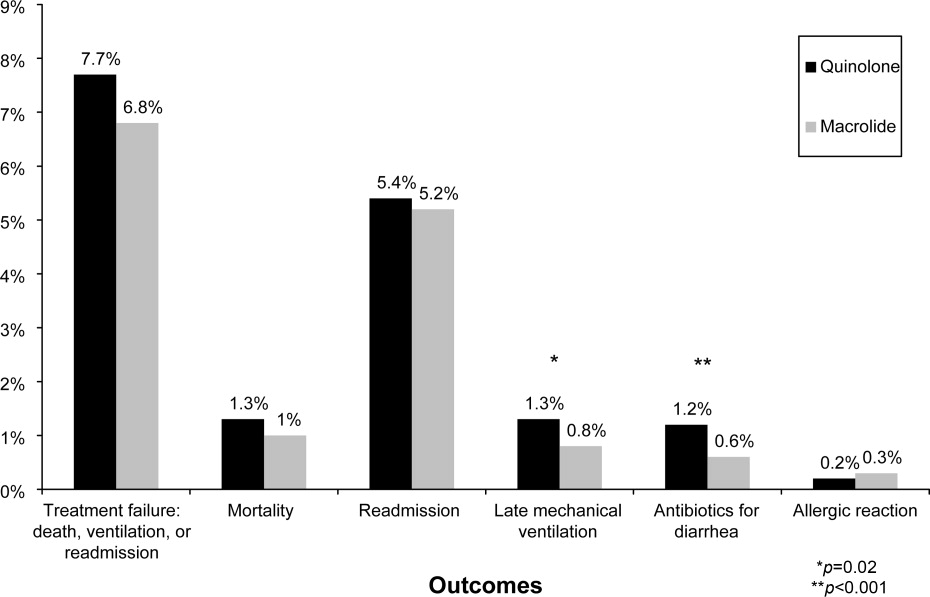
Treatment Failure | Cost | LOS | ||||
---|---|---|---|---|---|---|
Models | OR | 95% CI | Ratio | 95% CI | Ratio | 95% CI |
| ||||||
Unadjusted | 0.83 | 0.730.93 | 0.98 | 0.971.00 | 0.96 | 0.950.98 |
Adjusted for propensity score only* | 0.89 | 0.791.01 | 1.00 | 0.981.01 | 0.98 | 0.971.00 |
Adjusted for covariates | 0.87 | 0.770.99 | 1.00 | 0.991.02 | 0.99 | 0.971.00 |
Adjusted for covariates and propensity score | 0.89 | 0.781.01 | 1.00 | 0.991.02 | 0.98 | 0.971.00 |
Matched sample, unadjusted | 0.87 | 0.751.00 | 0.99 | 0.981.01 | 0.99 | 0.971.01 |
Matched sample, adjusted for unbalanced variables | 0.87 | 0.751.01 | 1.00 | 0.981.02 | 0.99 | 0.971.01 |
Grouped treatment model, unadjusted | 0.90 | 0.681.19 | 0.97 | 0.891.06 | 0.92 | 0.870.96 |
Group treatment model, adjusted for covariates∥ | 1.01 | 0.751.35 | 0.96 | 0.881.05 | 0.96 | 0.911.00 |
Discussion
In this large observational study conducted at 375 hospitals, we took advantage of a natural experiment in which antibiotic prescribing patterns varied widely across hospitals to compare the effectiveness of 2 common antibiotic regimens for AECOPD. Treatment with macrolides and quinolones were associated with a similar risk of treatment failure, costs, and length of stay; however, patients treated with macrolides were less likely to experience late mechanical ventilation or treatment for antibiotic‐associated diarrhea.
Despite broad consensus in COPD guidelines that patients with severe acute exacerbations should receive antibiotics, there is little agreement about the preferred empiric agent. Controversy exists regarding antibiotics' comparative effectiveness, and even over which pathogens cause COPD exacerbations. Given the frequency of hospitalization for AECOPD, understanding the comparative effectiveness of treatments in this setting could have important implications for health outcomes and costs. Unfortunately, most antibiotic studies in AECOPD were conducted >20 years ago, using antibiotics that rarely appeared in our sample.26 Consequently, clinical practice guidelines offer conflicting recommendations. For example, the National Institute for Clinical Excellence recommends empirical treatment with an aminopenicillin, a macrolide, or a tetracycline,8 while the American Thoracic Society recommends amoxicillin‐clavulonate or a fluoroquinolone.9
As might be expected in light of so much uncertainty, we found wide variation in prescribing patterns across hospitals. Overall, approximately one‐third of patients received a macrolide and two‐thirds a quinolone. Both regimens provide adequate coverage of H. influenza, S. pneumoniae, and M. catarrhalis, and conform to at least 1 COPD guideline. Nevertheless, patients receiving macrolides often received a cephalosporin as well; this pattern of treatment suggests that antibiotic selection is likely to have been influenced more by guidelines for the treatment of community‐acquired pneumonia than COPD.
Previous studies comparing antibiotic effectiveness suffer from shortcomings that limit their application to patients hospitalized with AECOPD. First, they enrolled patients with chronic bronchitis, and included patients without obstructive lung disease, and most studies included patients as young as 18 years old. Second, many either did not include treatment with steroids or excluded patients receiving more than 10 mg of prednisone daily. Third, almost all enrolled only ambulatory patients.
While there are no studies comparing quinolones and macrolides in patients hospitalized for AECOPD, a meta‐analysis comparing quinolones, macrolides, and amoxicillin‐clavulonate identified 19 trials of ambulatory patients with chronic bronchitis. That study found that all 3 drugs had similar efficacy initially, but that quinolones resulted in the fewest relapses over a 26‐week period.27 Macrolides and quinolones had similar rates of adverse effects. In contrast, we did not find a difference in treatment failure, cost, or length of stay, but did find a higher rate of diarrhea associated with quinolones. Others have also documented an association between fluoroquinolones and C. difficile diarrhea.2830 This trend, first noted in 2001, is of particular concern because the fluoroquinolone‐resistant strains appear to be hypervirulent and have been associated with nosocomial epidemics.3134
Our study has several limitations. First, its observational design leaves open the possibility of selection bias. For this reason we analyzed our data in several ways, including using a grouped treatment approach, an adaptation of the instrumental variable technique, and accepted only those differences which were consistent across all models. Second, our study used claims data, and therefore we could not directly adjust for physiological measures of severity. However, the highly detailed nature of the data allowed us to adjust for numerous tests and treatments that reflected the clinician's assessment of the patient's severity, as well as the number of prior COPD admissions. Third, we cannot exclude the possibility that some patients may have had concurrent pneumonia without an ICD‐9 code. We think that the number would be small because reimbursement for pneumonia is generally higher than for COPD, so hospitals have an incentive to code pneumonia as the principal diagnosis when present. Finally, we compared initial antibiotics only. More than one‐quarter of our patients received an additional antibiotic before discharge. In particular, patients receiving macrolides were often prescribed a concomitant cephalosporin. We do not know to what extent these additional antibiotics may have affected the outcomes.
Despite the large number of patients hospitalized annually for AECOPD, there are no randomized trials comparing different antibiotics in this population. Studies comparing antibiotics in chronic bronchitis can offer little guidance, since they have primarily focused on proving equivalence between existing antibiotics and newer, more expensive formulations.35 Because many of the patients enrolled in such trials do not benefit from antibiotics at all, either because they do not have COPD or because their exacerbation is not caused by bacteria, it is relatively easy to prove equivalence. Given that AECOPD is one of the leading causes of hospitalization in the United States, large, randomized trials comparing the effectiveness of different antibiotics should be a high priority. In the meantime, macrolides (often given together with cephalosporins) and quinolones appear to be equally effective initial antibiotic choices; considering antibiotic‐associated diarrhea, macrolides appear to be the safer of the 2.
Acute exacerbations of chronic obstructive pulmonary disease (AECOPD) are responsible for more than 600,000 hospitalizations annually, resulting in direct costs of over $20 billion.1 Bacterial infections appear responsible for 50% of such exacerbations,25 and current COPD guidelines recommend treatment with antibiotics for patients with severe exacerbations or a change in sputum.1,69 These recommendations are based on a number of small randomized trials, most of which were conducted more than 20 years ago using narrow spectrum antibiotics that are no longer commonly used.10 Only 4 studies, totaling 321 subjects, included hospitalized patients, and most studies excluded patients who required steroids. Because no clinical trials have compared different antibiotic regimens for AECOPD, existing guidelines offer a range of treatment options, including amoxicillin‐clavulonate, macrolides, quinolones, cephalosporins, aminopenicillins, and tetracyclines.
Among hospitalized patients, macrolides and quinolones appear to be the most frequently prescribed antibiotics.11 Both are available in oral formulations, have excellent bioavailability, and are administered once daily. In addition to their antimicrobial activity, macrolides are believed to have antiinflammatory effects, which could be especially advantageous in AECOPD.1214 In trials of chronic bronchitis, however, fluoroquinolones have been shown to reduce the risk of recurrent exacerbation when compared to macrolides.15 The wide variation that has been observed in antibiotic selection for patients hospitalized for AECOPD suggests a high degree of uncertainty among clinicians about the benefits of different treatment options.11 Given the limited evidence from randomized trials, we sought to evaluate the comparative effectiveness of macrolides and quinolones among a large, representative sample of patients hospitalized with AECOPD.
Subjects and Methods
Setting and Subjects
We conducted a retrospective cohort study of all patients hospitalized between January 1 and December 31, 2001 for AECOPD at any 1 of 375 acute care facilities in the United States that participated in Premier's Perspective, a voluntary, fee‐supported database developed for measuring quality and healthcare utilization. Participating hospitals represent all geographical regions, and are primarily small‐sized to medium‐sized nonteaching hospitals located mostly in urban areas. In addition to the information contained in the standard hospital discharge file (Uniform Billing 92) such as patient age, International Classification of Disease, 9th Edition, Clinical Modification (ICD‐9‐CM) codes, the Perspective database includes a date‐stamped log of all billed items, including diagnostic tests, medications, and other treatments, as well as costs, for individual patients. The study was approved by the Institutional Review Board of Baystate Medical Center.
Patients were included if they had a primary diagnosis consistent with AECOPD (ICD‐9 codes 491.21 and 493.22) or a primary diagnosis of respiratory failure (ICD‐9 codes 518.81 and 518.84) paired with secondary diagnosis of AECOPD; they also had to receive at least 2 consecutive days of either a macrolide or a quinolone, started within 48 hours of hospitalization. Patients receiving both antibiotics were excluded, but patients who received additional antibiotics were included. To enhance the specificity of our diagnosis codes, we limited our study to patients age 40 years.16 Because mechanical ventilation initiated after hospital day 2 was an outcome measure, we excluded patients admitted directly to the intensive care unit. We also excluded: those with other bacterial infections, such as pneumonia or cellulitis, who might have another indication for antibiotics; those with a length of stay <2 days, because we could not ascertain whether they received a full course of antibiotics; patients with a secondary diagnosis of pulmonary embolism or pneumothorax; and those whose attending physicians were not internists, family practitioners, hospitalists, pulmonologists, or intensivists. For patients with more than 1 admission during the study period, we included only the first admission.
Data Elements
For each patient, we assessed age, gender, race, marital and insurance status, principal diagnosis, comorbidities, and specialty of the attending physician. Comorbidities were identified from ICD‐9 secondary diagnosis codes and Diagnosis Related Groups using Healthcare Cost and Utilization Project Comorbidity Software (version 3.1), based on the work of Elixhauser et al.17 In addition, to assess disease severity we recorded the presence of chronic pulmonary heart disease, the number of admissions for COPD during the 12 months prior to the index admission, and arterial blood gas testing.18,19 We also identified pharmacy or diagnostic charges for interventions that were recommended in current guidelines (beta‐adrenergic and anticholinergic bronchodilators, steroids, and noninvasive positive‐pressure ventilation); those that were not recommended or were of uncertain benefit (methylxanthine bronchodilators, spirometry/pulmonary function testing, mucolytic medications, chest physiotherapy, and sputum testing); and drugs that might be associated with severe exacerbations or end‐stage COPD (loop diuretics, morphine, and nutritional supplements).1,69 Hospitals were categorized by region (Northeast, South, Midwest, or West), bed size, setting (urban vs. rural), ownership, and teaching status.
Antibiotic Class and Outcome Variables
Our primary predictor variable was the antibiotic initiated during the first 2 hospital days and continued for at least 2 days, regardless of other antibiotics the patient may have received during the course of hospitalization. Because we anticipated low in‐hospital mortality, our primary outcome was a composite measure of treatment failure, defined as initiation of mechanical ventilation after hospital day 2, in‐hospital mortality, or readmission for COPD within 30 days of discharge.20 Secondary outcomes included hospital costs and length of stay, as well as allergic reactions identified by ICD‐9 code, and antibiotic‐associated diarrhea, defined as treatment with either metronidazole or oral vancomycin begun after hospital day 3.
Statistical Analysis
Summary statistics were computed using frequencies and percents for categorical variables; and means, medians, standard deviations, and interquartile ranges for continuous variables. Associations between antibiotic selection and patient and hospital characteristics were assessed using chi‐square tests for categorical variables and z‐tests for continuous variables.
We developed a series of multivariable models to evaluate the impact of initial antibiotic selection on the risk of treatment failure, length of stay, and total cost. In order to account for the effects of within‐hospital correlation, generalized estimating equation (GEE) models with a logit link were used to assess the effect of antibiotic selection on the risk of treatment failure, and identity link models were used for analyses of length of stay and cost. Unadjusted and covariate‐adjusted models for treatment failure were evaluated with and without adjustments for propensity score. A propensity score is the probability that a given patient would receive treatment with a macrolide, derived from a nonparsimonious model in which treatment with a macrolide was considered the outcome. The propensity model included all patient characteristics, other early treatments and tests, comorbidities, hospital and physician characteristics, and selected interaction terms.21 Length of stay and cost were trimmed at 3 standard deviations above the mean, and natural log‐transformed values were modeled due to extreme positive skew. In addition, we carried out matched analyses in which we compared the outcomes of patients who were treated with a macrolide to those with similar propensity scores (ie, with similar likelihood of receiving a macrolide) who received a quinolone.22
Finally, to reduce the threat of residual confounding by indication, which can occur if sicker patients are more likely to receive a particular antibiotic, we developed a grouped treatment model, in which all patients treated at the same hospital were assigned a probability of treatment with a macrolide equal to the overall treatment rate at that hospital.23 This is an adaptation of instrumental variable analysis, a well‐accepted technique in econometrics with growing use in health care.24,25 It attempts to assess whether patients treated at a hospital at which quinolones are used more frequently have better outcomes than patients treated at hospitals at which macrolides are used more frequently, while adjusting for other patient, physician, and hospital variables. It ignores the actual treatment the patient received, and instead substitutes the hospital's rate of macrolide use. By grouping treatment at the hospital level, this method greatly reduces the possibility of residual selection bias, unless hospitals that use a lot of macrolides have patients who differ in a consistent way from hospitals which use mostly quinolones.
All analyses were performed using SAS version 9.1 (SAS Institute, Inc., Cary, NC).
Results
Of 26,248 AECOPD patients treated with antibiotics, 19,608 patients met the inclusion criteria; of these, 6139 (31%) were treated initially with a macrolide; the median age was 70 years; 60% were female; and 78% were white. A total of 86% of patients had a primary diagnosis of obstructive chronic bronchitis with acute exacerbation, and 6% had respiratory failure. The most common comorbidities were hypertension, diabetes, and congestive heart failure. Twenty‐two percent had been admitted at least once in the preceding 12 months. Treatment failure occurred in 7.7% of patients, and 1.3% died in the hospital. Mean length of stay was 4.8 days. Hospital prescribing rates for macrolides varied from 0% to 100%, with a mean of 33% and an interquartile range of 14% to 46% (Supporting Appendix Figure 1).
Compared to patients receiving macrolides, those receiving quinolones were older, more likely to have respiratory failure, to be cared for by a pulmonologist, and to have an admission in the previous year (Table 1). They were also more likely to be treated with bronchodilators, methylxanthines, steroids, diuretics, and noninvasive positive pressure ventilation, and to have an arterial blood gas, but less likely to receive concomitant treatment with a cephalosporin (11% vs. 57%). With the exception of cephalosporin treatment, these differences were small, but due to the large sample were statistically significant. Comorbidities were similar in both groups. Patients in the quinolone group were also more likely to experience treatment failure (8.1% vs. 6.8%), death (1.5% vs. 1.0%), and antibiotic‐associated diarrhea (1.1% vs. 0.5%).
Complete Cohort | Propensity‐matched Subsample | |||||
---|---|---|---|---|---|---|
Characteristic | Quinolone (n = 13469) | Macrolide (n = 6139) | P Value | Quinolone (n = 5610) | Macrolide (n = 5610) | P Value |
| ||||||
Antibiotics received during hospitalization* [n (%)] | ||||||
Macrolide | 264 (2) | 6139 (100) | 119 (2) | 5610 (100) | ||
Quinolone | 13469 (100) | 459 (8) | 5610 (100) | 424 (8) | ||
Cephalosporin | 1696 (13) | 3579 (59) | <0.001 | 726 (13) | 3305 (59) | <0.001 |
Tetracycline | 231 (2) | 75 (2) | 0.01 | 101 (2) | 73 (2) | 0.06 |
Other antibiotics | 397 (3) | 220 (4) | 0.02 | 166 (3) | 193 (3) | 0.03 |
Age (years) (mean [SD]) | 69.1 (11.4) | 68.2 (11.8) | <0.001 | 68.6 (11.7) | 68.5 (11.7) | 0.58 |
Male sex (n [%]) | 5447 (40) | 2440 (40) | 0.36 | 2207 (39) | 2196 (39) | 0.85 |
Race/ethnic group (n [%]) | <0.001 | 0.44 | ||||
White | 10454 (78) | 4758 (78) | 4359 (78) | 4368 (78) | ||
Black | 1060 (8) | 540 (9) | 470 (8) | 455 (8) | ||
Hispanic | 463 (3) | 144 (2) | 157 (3) | 134 (2) | ||
Other | 1492 (11) | 697 (11) | 624 (11) | 653 (12) | ||
Primary diagnosis (n [%]) | <0.001 | 0.78 | ||||
Obstructive chronic bronchitis with acute exacerbation | 11650 (87) | 5298 (86) | 4884 (87) | 4860 (87) | ||
Chronic obstructive asthma/asthma with COPD | 908 (7) | 569 (9) | 466 (8) | 486 (9) | ||
Respiratory failure | 911 (7) | 272 (4) | 260 (5) | 264 (5) | ||
Admissions in the prior year (n [%]) | <0.001 | 0.84 | ||||
0 | 9846 (73) | 4654 (76) | 4249 (76) | 4231 (75) | ||
1 | 1918 (14) | 816 (13) | 747 (13) | 750 (13) | ||
2+ | 1085 (8) | 445 (7) | 397 (7) | 420 (8) | ||
Missing | 620 (5) | 224 (4) | 217 (4) | 209 (4) | ||
Physician specialty (n [%]) | <0.001 | 0.84 | ||||
Internal medicine/hospitalist | 7069 (53) | 3321 (54) | 3032 (54) | 3072 (55) | ||
Family/general medicine | 3569 (27) | 2074 (34) | 1824 (33) | 1812 (32) | ||
Pulmonologist | 2776 (21) | 727 (12) | 738 (13) | 711 (13) | ||
Critical care/emntensivist | 55 (0) | 17 (0) | 16 (0) | 15 (0) | ||
Tests on hospital day 1 or 2 (n [%]) | ||||||
Arterial blood gas | 8084 (60) | 3377 (55) | <0.001 | 3195 (57) | 3129 (56) | 0.22 |
Sputum test | 1741 (13) | 766 (13) | 0.39 | 20 (0) | 16 (0) | 0.62 |
Medications/therapies on hospital day 1 or 2 (n [%]) | ||||||
Short‐acting bronchodilators | 7555 (56) | 3242 (53) | <0.001 | 2969 (53) | 2820 (50) | 0.005 |
Long‐acting beta‐2 agonists | 2068 (15) | 748 (12) | <0.001 | 704 (13) | 719 (13) | 0.69 |
Methylxanthine bronchodilators | 3051 (23) | 1149 (19) | <0.001 | 1102 (20) | 1093 (20) | 0.85 |
Steroids | 0.04 | 0.68 | ||||
Intravenous | 11148 (83) | 4989 (81) | 4547 (81) | 4581 (82) | ||
Oral | 772 (6) | 376 (6) | 334 (6) | 330 (6) | ||
Severity indicators (n [%]) | ||||||
Chronic pulmonary heart disease | 890 (7) | 401 (7) | 0.85 | 337 (6) | 368 (7) | 0.24 |
Sleep apnea | 586 (4) | 234 (4) | 0.08 | 211 (4) | 218 (4) | 0.77 |
Noninvasive positive pressure ventilation | 391 (3) | 128 (2) | <0.001 | 128 (2) | 114 (2) | 0.40 |
Loop diuretics | 4838 (36) | 1971 (32) | <0.001 | 1884 (34) | 1862 (33) | 0.67 |
Hospital characteristics (n [%]) | ||||||
Staffed beds | <0.001 | 0.71 | ||||
6200 | 3483 (26) | 1688 (28) | 1610 (29) | 1586 (28) | ||
201300 | 3132 (23) | 1198 (20) | 1174 (21) | 1154 (21) | ||
301500 | 4265 (32) | 2047 (33) | 1809 (32) | 1867 (33) | ||
500+ | 2589 (19) | 1206 (20) | 1017 (18) | 1003 (18) | ||
Hospital region (n [%]) | <0.001 | 0.65 | ||||
South | 8562 (64) | 3270 (53) | 3212 (57) | 3160 (56) | ||
Midwest | 2602 (19) | 1444 (24) | 1170 (21) | 1216 (22) | ||
Northeast | 1163 (9) | 871 (14) | 687 (12) | 704 (13) | ||
West | 1142 (9) | 554 (9) | 541 (10) | 530 (9) | ||
Teaching hospital | <0.001 | 0.63 | ||||
No | 12090 (90) | 5037 (82) | 4896 (87) | 4878 (87) | ||
Yes | 1379 (10) | 1102 (18) | 714 (13) | 732 (13) | ||
Comorbidities (n [%]) | ||||||
Congestive heart failure | 2673 (20) | 1147 (19) | 0.06 | 1081 (19) | 1060 (19) | 0.63 |
Metastatic cancer | 134 (1) | 27 (0) | <0.001 | 34 (1) | 38 (1) | 0.72 |
Depression | 1419 (11) | 669 (11) | 0.45 | 598 (11) | 603 (11) | 0.90 |
Deficiency anemias | 1155 (9) | 476 (8) | 0.05 | 426 (8) | 432 (8) | 0.86 |
Solid tumor without metastasis | 1487 (11) | 586 (10) | 0.002 | 550 (10) | 552 (10) | 0.97 |
Hypothyroidism | 1267 (9) | 527 (9) | 0.07 | 481 (9) | 482 (9) | 1.00 |
Peripheral vascular disease | 821 (6) | 312 (5) | 0.005 | 287 (5) | 288 (5) | 1.00 |
Paralysis | 165 (1) | 46 (1) | 0.003 | 49 (1) | 51 (1) | 0.92 |
Obesity | 957 (7) | 435 (7) | 0.98 | 386 (7) | 398 (7) | 0.68 |
Hypertension | 5793 (43) | 2688 (44) | 0.31 | 2474 (44) | 2468 (44) | 0.92 |
Diabetes | 0.04 | 0.45 | ||||
Without chronic complications | 2630 (20) | 1127 (18) | 1057 (19) | 1066 (19) | ||
With chronic complications | 298 (2) | 116 (2) | 115 (2) | 97 (2) |
In the unadjusted analysis, compared to patients receiving quinolones, those treated with macrolides were less likely to experience treatment failure (OR, 0.83; 95% CI, 0.740.94) (Table 2). Adjusting for all patient, hospital, and physician covariates, including the propensity for treatment with macrolides, increased the OR to 0.89 and the results were no longer significant (95% CI, 0.781.01). Propensity matching successfully balanced all measured covariates except for the use of short‐acting bronchodilators and additional antibiotics (Table 1). In the propensity‐matched sample (Figure 1), quinolone‐treated patients were more likely to experience antibiotic‐associated diarrhea (1.2% vs. 0.6%; P = 0.0003) and late mechanical ventilation (1.3% vs. 0.8%; P = 0.02). There were no differences in adjusted cost or length of stay between the 2 groups. The results of the grouped treatment analysis, substituting the hospital's specific rate of macrolide use in place of the actual treatment that each patient received suggested that the 2 antibiotics were associated with similar rates of treatment failure. The OR for a 100% hospital rate of macrolide treatment vs. a 0% rate was 1.01 (95% CI, 0.751.35).
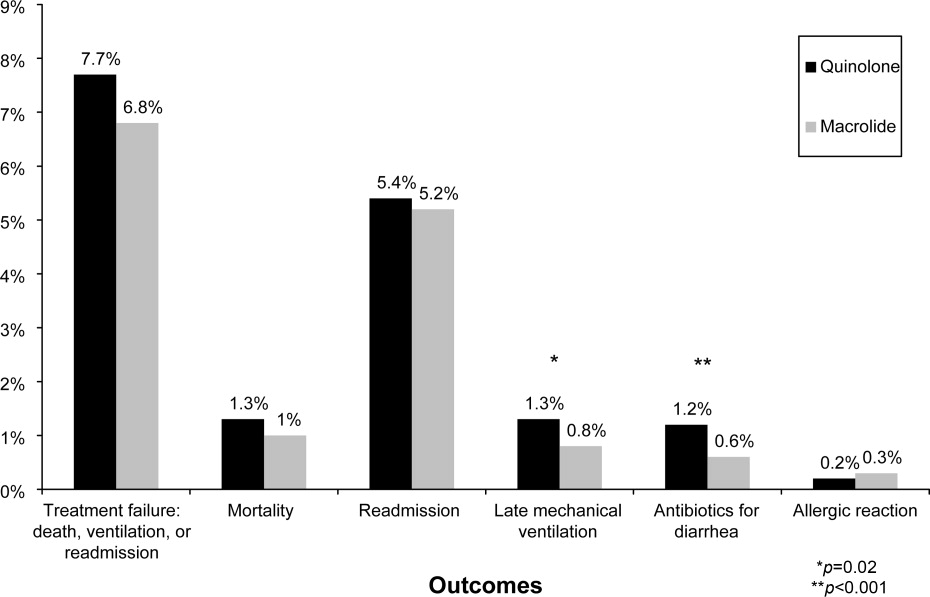
Treatment Failure | Cost | LOS | ||||
---|---|---|---|---|---|---|
Models | OR | 95% CI | Ratio | 95% CI | Ratio | 95% CI |
| ||||||
Unadjusted | 0.83 | 0.730.93 | 0.98 | 0.971.00 | 0.96 | 0.950.98 |
Adjusted for propensity score only* | 0.89 | 0.791.01 | 1.00 | 0.981.01 | 0.98 | 0.971.00 |
Adjusted for covariates | 0.87 | 0.770.99 | 1.00 | 0.991.02 | 0.99 | 0.971.00 |
Adjusted for covariates and propensity score | 0.89 | 0.781.01 | 1.00 | 0.991.02 | 0.98 | 0.971.00 |
Matched sample, unadjusted | 0.87 | 0.751.00 | 0.99 | 0.981.01 | 0.99 | 0.971.01 |
Matched sample, adjusted for unbalanced variables | 0.87 | 0.751.01 | 1.00 | 0.981.02 | 0.99 | 0.971.01 |
Grouped treatment model, unadjusted | 0.90 | 0.681.19 | 0.97 | 0.891.06 | 0.92 | 0.870.96 |
Group treatment model, adjusted for covariates∥ | 1.01 | 0.751.35 | 0.96 | 0.881.05 | 0.96 | 0.911.00 |
Discussion
In this large observational study conducted at 375 hospitals, we took advantage of a natural experiment in which antibiotic prescribing patterns varied widely across hospitals to compare the effectiveness of 2 common antibiotic regimens for AECOPD. Treatment with macrolides and quinolones were associated with a similar risk of treatment failure, costs, and length of stay; however, patients treated with macrolides were less likely to experience late mechanical ventilation or treatment for antibiotic‐associated diarrhea.
Despite broad consensus in COPD guidelines that patients with severe acute exacerbations should receive antibiotics, there is little agreement about the preferred empiric agent. Controversy exists regarding antibiotics' comparative effectiveness, and even over which pathogens cause COPD exacerbations. Given the frequency of hospitalization for AECOPD, understanding the comparative effectiveness of treatments in this setting could have important implications for health outcomes and costs. Unfortunately, most antibiotic studies in AECOPD were conducted >20 years ago, using antibiotics that rarely appeared in our sample.26 Consequently, clinical practice guidelines offer conflicting recommendations. For example, the National Institute for Clinical Excellence recommends empirical treatment with an aminopenicillin, a macrolide, or a tetracycline,8 while the American Thoracic Society recommends amoxicillin‐clavulonate or a fluoroquinolone.9
As might be expected in light of so much uncertainty, we found wide variation in prescribing patterns across hospitals. Overall, approximately one‐third of patients received a macrolide and two‐thirds a quinolone. Both regimens provide adequate coverage of H. influenza, S. pneumoniae, and M. catarrhalis, and conform to at least 1 COPD guideline. Nevertheless, patients receiving macrolides often received a cephalosporin as well; this pattern of treatment suggests that antibiotic selection is likely to have been influenced more by guidelines for the treatment of community‐acquired pneumonia than COPD.
Previous studies comparing antibiotic effectiveness suffer from shortcomings that limit their application to patients hospitalized with AECOPD. First, they enrolled patients with chronic bronchitis, and included patients without obstructive lung disease, and most studies included patients as young as 18 years old. Second, many either did not include treatment with steroids or excluded patients receiving more than 10 mg of prednisone daily. Third, almost all enrolled only ambulatory patients.
While there are no studies comparing quinolones and macrolides in patients hospitalized for AECOPD, a meta‐analysis comparing quinolones, macrolides, and amoxicillin‐clavulonate identified 19 trials of ambulatory patients with chronic bronchitis. That study found that all 3 drugs had similar efficacy initially, but that quinolones resulted in the fewest relapses over a 26‐week period.27 Macrolides and quinolones had similar rates of adverse effects. In contrast, we did not find a difference in treatment failure, cost, or length of stay, but did find a higher rate of diarrhea associated with quinolones. Others have also documented an association between fluoroquinolones and C. difficile diarrhea.2830 This trend, first noted in 2001, is of particular concern because the fluoroquinolone‐resistant strains appear to be hypervirulent and have been associated with nosocomial epidemics.3134
Our study has several limitations. First, its observational design leaves open the possibility of selection bias. For this reason we analyzed our data in several ways, including using a grouped treatment approach, an adaptation of the instrumental variable technique, and accepted only those differences which were consistent across all models. Second, our study used claims data, and therefore we could not directly adjust for physiological measures of severity. However, the highly detailed nature of the data allowed us to adjust for numerous tests and treatments that reflected the clinician's assessment of the patient's severity, as well as the number of prior COPD admissions. Third, we cannot exclude the possibility that some patients may have had concurrent pneumonia without an ICD‐9 code. We think that the number would be small because reimbursement for pneumonia is generally higher than for COPD, so hospitals have an incentive to code pneumonia as the principal diagnosis when present. Finally, we compared initial antibiotics only. More than one‐quarter of our patients received an additional antibiotic before discharge. In particular, patients receiving macrolides were often prescribed a concomitant cephalosporin. We do not know to what extent these additional antibiotics may have affected the outcomes.
Despite the large number of patients hospitalized annually for AECOPD, there are no randomized trials comparing different antibiotics in this population. Studies comparing antibiotics in chronic bronchitis can offer little guidance, since they have primarily focused on proving equivalence between existing antibiotics and newer, more expensive formulations.35 Because many of the patients enrolled in such trials do not benefit from antibiotics at all, either because they do not have COPD or because their exacerbation is not caused by bacteria, it is relatively easy to prove equivalence. Given that AECOPD is one of the leading causes of hospitalization in the United States, large, randomized trials comparing the effectiveness of different antibiotics should be a high priority. In the meantime, macrolides (often given together with cephalosporins) and quinolones appear to be equally effective initial antibiotic choices; considering antibiotic‐associated diarrhea, macrolides appear to be the safer of the 2.
- Evidence base for management of acute exacerbations of chronic obstructive pulmonary disease.Ann Intern Med.2001;134:595–599. , , .
- Infectious etiologies in acute exacerbation of COPD.Diagn Microbiol Infect Dis.2001;40:95–102. , , , et al.
- Bacterial infections in patients requiring admission for an acute exacerbation of COPD; a 1‐year prospective study.Respir Med.2003;97:770–777. , .
- Microbiologic determinants of exacerbation in chronic obstructive pulmonary disease.Arch Intern Med.2005;165:891–897. , , , et al.
- Infection in the pathogenesis and course of chronic obstructive pulmonary disease.N Engl J Med.2008;359:2355–2365. , .
- Global strategy for the diagnosis, management, and prevention of chronic obstructive pulmonary disease: GOLD executive summary.Am J Respir Crit Care Med.2007;176:532–555. , , , et al.
- Canadian Thoracic Society recommendations for management of chronic obstructive pulmonary disease—2008 update—highlights for primary care.Can Respir J.2008;15(suppl A):1A–8A. , , , et al.
- Chronic obstructive pulmonary disease.National clinical guideline on management of chronic obstructive pulmonary disease in adults in primary and secondary care.Thorax.2004;59(suppl 1):1–232.
- Standards for the diagnosis and treatment of patients with COPD: a summary of the ATS/ERS position paper.Eur Respir J.2004;23:932–946. , , , et al.
- Antibiotics for exacerbations of chronic obstructive pulmonary disease.Cochrane Database Syst Rev.2006:CD004403. , , , , .
- Quality of care for patients hospitalized for acute exacerbations of chronic obstructive pulmonary disease.Ann Intern Med.2006;144:894–903. , , , , , .
- Modulation of neutrophil and inflammation markers in chronic obstructive pulmonary disease by short‐term azithromycin treatment.Eur J Pharmacol.2005;517:132–143. , , , et al.
- Azithromycin modulates neutrophil function and circulating inflammatory mediators in healthy human subjects.Eur J Pharmacol.2002;450:277–289. , , , et al.
- The effect of clarithromycin on inflammatory markers in chronic obstructive pulmonary disease: preliminary data.Ann Pharmacother.2004;38:1400–1405. , , , , , .
- A comparison of gemifloxacin and clarithromycin in acute exacerbations of chronic bronchitis and long‐term clinical outcomes.Clin Ther.2002;24:639–652. , , , .
- In‐hospital mortality following acute exacerbations of chronic obstructive pulmonary disease.Arch Intern Med.2003;163:1180–1186. , , , .
- Comorbidity measures for use with administrative data.Med Care.1998;36:8–27. , , , .
- Outcomes following acute exacerbation of severe chronic obstructive lung disease. The SUPPORT investigators (Study to Understand Prognoses and Preferences for Outcomes and Risks of Treatments).Am J Respir Crit Care Med.1996;154:959–967. , , , et al.
- Mortality and mortality‐related factors after hospitalization for acute exacerbation of COPD.Chest.2003;124:459–467. , , .
- Effect of systemic glucocorticoids on exacerbations of chronic obstructive pulmonary disease.N Engl J Med.1999;340:1941–1947. , , , et al.
- Propensity score methods for bias reduction in the comparison of a treatment to a non‐randomized control group.Stat Med.1998;17:2265–2281. .
- Reducing bias in a propensity score matched‐pair sample using greedy matching techniques. Proceedings of the Twenty‐Sixth Annual SAS Users Group International Conference. Cary, NC: SAS Institute;2001,214–216. .
- Modeling treatment effects on binary outcomes with grouped‐treatment variables and individual covariates.Am J Epidemiol.2002;156:753–760. , , , .
- Does more intensive treatment of acute myocardial infarction in the elderly reduce mortality? Analysis using instrumental variables. [see Comment].JAMA.1994;272:859–866. , , .
- Analysis of observational studies in the presence of treatment selection bias: effects of invasive cardiac management on AMI survival using propensity score and instrumental variable methods.JAMA.2007;297:278–285. , , , , , .
- Antibiotics in chronic obstructive pulmonary disease exacerbations. A meta‐analysis.JAMA.1995;273:957–960. , , , .
- Macrolides, quinolones and amoxicillin/clavulanate for chronic bronchitis: a meta‐analysis.Eur Respir J.2007;29:1127–1137. , , , , .
- Antimicrobial‐associated risk factors for Clostridium difficile infection.Clin Infect Dis.2008;46(suppl 1):S19–S31. , , , , .
- Short‐term and long‐term outcomes of moxifloxacin compared to standard antibiotic treatment in acute exacerbations of chronic bronchitis.Chest.2004;125:953–964. , , , et al.
- Oral gemifloxacin once daily for 5 days compared with sequential therapy with i.v. ceftriaxone/oral cefuroxime (maximum of 10 days) in the treatment of hospitalized patients with acute exacerbations of chronic bronchitis.Respir Med.2003;97:242–249. , , , , .
- A large outbreak of Clostridium difficile‐associated disease with an unexpected proportion of deaths and colectomies at a teaching hospital following increased fluoroquinolone use.Infect Control Hosp Epidemiol.2005;26:273–280. , , , et al.
- An epidemic, toxin gene‐variant strain of Clostridium difficile.N Engl J Med.2005;353:2433–2441. , , , et al.
- Outbreak of Clostridium difficile infection in a long‐term care facility: association with gatifloxacin use.Clin Infect Dis.2004;38:640–645. , , , et al.
- A predominantly clonal multi‐institutional outbreak of Clostridium difficile‐associated diarrhea with high morbidity and mortality.N Engl J Med.2005;353:2442–2449. , , , et al.
- No more equivalence trials for antibiotics in exacerbations of COPD, please.Chest.2004;125:811–813. , .
- Evidence base for management of acute exacerbations of chronic obstructive pulmonary disease.Ann Intern Med.2001;134:595–599. , , .
- Infectious etiologies in acute exacerbation of COPD.Diagn Microbiol Infect Dis.2001;40:95–102. , , , et al.
- Bacterial infections in patients requiring admission for an acute exacerbation of COPD; a 1‐year prospective study.Respir Med.2003;97:770–777. , .
- Microbiologic determinants of exacerbation in chronic obstructive pulmonary disease.Arch Intern Med.2005;165:891–897. , , , et al.
- Infection in the pathogenesis and course of chronic obstructive pulmonary disease.N Engl J Med.2008;359:2355–2365. , .
- Global strategy for the diagnosis, management, and prevention of chronic obstructive pulmonary disease: GOLD executive summary.Am J Respir Crit Care Med.2007;176:532–555. , , , et al.
- Canadian Thoracic Society recommendations for management of chronic obstructive pulmonary disease—2008 update—highlights for primary care.Can Respir J.2008;15(suppl A):1A–8A. , , , et al.
- Chronic obstructive pulmonary disease.National clinical guideline on management of chronic obstructive pulmonary disease in adults in primary and secondary care.Thorax.2004;59(suppl 1):1–232.
- Standards for the diagnosis and treatment of patients with COPD: a summary of the ATS/ERS position paper.Eur Respir J.2004;23:932–946. , , , et al.
- Antibiotics for exacerbations of chronic obstructive pulmonary disease.Cochrane Database Syst Rev.2006:CD004403. , , , , .
- Quality of care for patients hospitalized for acute exacerbations of chronic obstructive pulmonary disease.Ann Intern Med.2006;144:894–903. , , , , , .
- Modulation of neutrophil and inflammation markers in chronic obstructive pulmonary disease by short‐term azithromycin treatment.Eur J Pharmacol.2005;517:132–143. , , , et al.
- Azithromycin modulates neutrophil function and circulating inflammatory mediators in healthy human subjects.Eur J Pharmacol.2002;450:277–289. , , , et al.
- The effect of clarithromycin on inflammatory markers in chronic obstructive pulmonary disease: preliminary data.Ann Pharmacother.2004;38:1400–1405. , , , , , .
- A comparison of gemifloxacin and clarithromycin in acute exacerbations of chronic bronchitis and long‐term clinical outcomes.Clin Ther.2002;24:639–652. , , , .
- In‐hospital mortality following acute exacerbations of chronic obstructive pulmonary disease.Arch Intern Med.2003;163:1180–1186. , , , .
- Comorbidity measures for use with administrative data.Med Care.1998;36:8–27. , , , .
- Outcomes following acute exacerbation of severe chronic obstructive lung disease. The SUPPORT investigators (Study to Understand Prognoses and Preferences for Outcomes and Risks of Treatments).Am J Respir Crit Care Med.1996;154:959–967. , , , et al.
- Mortality and mortality‐related factors after hospitalization for acute exacerbation of COPD.Chest.2003;124:459–467. , , .
- Effect of systemic glucocorticoids on exacerbations of chronic obstructive pulmonary disease.N Engl J Med.1999;340:1941–1947. , , , et al.
- Propensity score methods for bias reduction in the comparison of a treatment to a non‐randomized control group.Stat Med.1998;17:2265–2281. .
- Reducing bias in a propensity score matched‐pair sample using greedy matching techniques. Proceedings of the Twenty‐Sixth Annual SAS Users Group International Conference. Cary, NC: SAS Institute;2001,214–216. .
- Modeling treatment effects on binary outcomes with grouped‐treatment variables and individual covariates.Am J Epidemiol.2002;156:753–760. , , , .
- Does more intensive treatment of acute myocardial infarction in the elderly reduce mortality? Analysis using instrumental variables. [see Comment].JAMA.1994;272:859–866. , , .
- Analysis of observational studies in the presence of treatment selection bias: effects of invasive cardiac management on AMI survival using propensity score and instrumental variable methods.JAMA.2007;297:278–285. , , , , , .
- Antibiotics in chronic obstructive pulmonary disease exacerbations. A meta‐analysis.JAMA.1995;273:957–960. , , , .
- Macrolides, quinolones and amoxicillin/clavulanate for chronic bronchitis: a meta‐analysis.Eur Respir J.2007;29:1127–1137. , , , , .
- Antimicrobial‐associated risk factors for Clostridium difficile infection.Clin Infect Dis.2008;46(suppl 1):S19–S31. , , , , .
- Short‐term and long‐term outcomes of moxifloxacin compared to standard antibiotic treatment in acute exacerbations of chronic bronchitis.Chest.2004;125:953–964. , , , et al.
- Oral gemifloxacin once daily for 5 days compared with sequential therapy with i.v. ceftriaxone/oral cefuroxime (maximum of 10 days) in the treatment of hospitalized patients with acute exacerbations of chronic bronchitis.Respir Med.2003;97:242–249. , , , , .
- A large outbreak of Clostridium difficile‐associated disease with an unexpected proportion of deaths and colectomies at a teaching hospital following increased fluoroquinolone use.Infect Control Hosp Epidemiol.2005;26:273–280. , , , et al.
- An epidemic, toxin gene‐variant strain of Clostridium difficile.N Engl J Med.2005;353:2433–2441. , , , et al.
- Outbreak of Clostridium difficile infection in a long‐term care facility: association with gatifloxacin use.Clin Infect Dis.2004;38:640–645. , , , et al.
- A predominantly clonal multi‐institutional outbreak of Clostridium difficile‐associated diarrhea with high morbidity and mortality.N Engl J Med.2005;353:2442–2449. , , , et al.
- No more equivalence trials for antibiotics in exacerbations of COPD, please.Chest.2004;125:811–813. , .
Copyright © 2010 Society of Hospital Medicine