User login
Patients who deteriorate in the hospital and are transferred to the intensive care unit (ICU) have higher mortality and greater morbidity than those directly admitted from the emergency department.[1, 2, 3] Rapid response teams (RRTs) were created to address this problem.[4, 5] Quantitative tools, such as the Modified Early Warning Score (MEWS),[6] have been used to support RRTs almost since their inception. Nonetheless, work on developing scores that can serve as triggers for RRT evaluation or intervention continues. The notion that comprehensive inpatient electronic medical records (EMRs) could support RRTs (both as a source of patient data and a platform for providing alerts) has intuitive appeal. Not surprisingly, in addition to newer versions of manual scores,[7] electronic scores are now entering clinical practice. These newer systems are being tested in research institutions,[8] hospitals with advanced capabilities,[9] and as part of proprietary systems.[10] Although a fair amount of statistical information (eg, area under the receiver operator characteristic curve of a given predictive model) on the performance of various trigger systems has been published, existing reports have not described details of how the electronic architecture is integrated with clinical practice.
Electronic alert systems generated from physiology‐based predictive models do not yet constitute mature technologies. No consensus or legal mandate regarding their role yet exists. Given this situation, studying different implementation approaches and their outcomes has value. It is instructive to consider how a given institutional solution addresses common contingenciesoperational constraints that are likely to be present, albeit in different forms, in most placesto help others understand the limitations and issues they may present. In this article we describe the structure of an EMR‐based early warning system in 2 pilot hospitals at Kaiser Permanente Northern California (KPNC). In this pilot, we embedded an updated version of a previously described early warning score[11] into the EMR. We will emphasize how its components address institutional, operational, and technological constraints. Finally, we will also describe unfinished businesschanges we would like to see in a future dissemination phase. Two important aspects of the pilot (development of a clinical response arm and addressing patient preferences with respect to supportive care) are being described elsewhere in this issue of the Journal of Hospital Medicine. Analyses of the actual impact on patient outcomes will be reported elsewhere; initial results appear favorable.[12]
INITIAL CONSTRAINTS
The ability to actually prevent inpatient deteriorations may be limited,[13] and doubts regarding the value of RRTs persist.[14, 15, 16] Consequently, work that led to the pilot occurred in stages. In the first stage (prior to 2010), our team presented data to internal audiences documenting the rates and outcomes of unplanned transfers from the ward to the ICU. Concurrently, our team developed a first generation risk adjustment methodology that was published in 2008.[17] We used this methodology to show that unplanned transfers did, in fact, have elevated mortality, and that this persisted after risk adjustment.[1, 2, 3] This phase of our work coincided with KPNC's deployment of the Epic inpatient EMR (
Once we demonstrated that we could, in fact, predict inpatient deteriorations, we still had to address medicallegal considerations, the need for a clinical response arm, and how to address patient preferences with respect to supportive or palliative care. To address these concerns and ensure that the implementation would be seamlessly integrated with routine clinical practice, our team worked for 1 year with hospitalists and other clinicians at the pilot sites prior to the go‐live date.
The primary concern from a medicallegal perspective is that once results from a predictive model (which could be an alert, severity score, comorbidity score, or other probability estimate) are displayed in the chart, relevant clinical information has been changed. Thus, failure to address such an EMR item could lead to malpractice risk for individuals and/or enterprise liability for an organization. After discussing this with senior leadership, they specified that it would be permissible to go forward so long as we could document that an educational intervention was in place to make sure that clinicians understood the system and that it was linked to specific protocols approved by hospitalists.
Current predictive models, including ours, generate a probability estimate. They do not necessarily identify the etiology of a problem or what solutions ought to be considered. Consequently, our senior leadership insisted that we be able to answer clinicians' basic question: What do we do when we get an alert? The article by Dummett et al.[20] in this issue of the Journal of Hospital Medicine describes how we addressed this constraint. Lastly, not all patients can be rescued. The article by Granich et al.[21] describes how we handled the need to respect patient choices.
PROCEDURAL COMPONENTS
The Gordon and Betty Moore Foundation, which funded the pilot, only had 1 restriction (inclusion of a hospital in the Sacramento, California area). The other site was selected based on 2 initial criteria: (1) the chosen site had to be 1 of the smaller KPNC hospitals, and (2) the chosen site had to be easily accessible for the lead author (G.J.E.). The KPNC South San Francisco hospital was selected as the alpha site and the KPNC Sacramento hospital as the beta site. One of the major drivers for these decisions was that both had robust palliative care services. The Sacramento hospital is a larger hospital with a more complex caseload.
Prior to the go‐live dates (November 19, 2013 for South San Francisco and April 16, 2014 for Sacramento), the executive committees at both hospitals reviewed preliminary data and the implementation plans for the early warning system. Following these reviews, the executive committees approved the deployment. Also during this phase, in consultation with our communications departments, we adopted the name Advance Alert Monitoring (AAM) as the outward facing name for the system. We also developed recommended scripts for clinical staff to employ when approaching a patient in whom an alert had been issued (this is because the alert is calibrated so as to predict increased risk of deterioration within the next 12 hours, which means that a patient might be surprised as to why clinicians were suddenly evaluating them). Facility approvals occurred approximately 1 month prior to the go‐live date at each hospital, permitting a shadowing phase. In this phase, selected physicians were provided with probability estimates and severity scores, but these were not displayed in the EMR front end. This shadowing phase permitted clinicians to finalize the response arms' protocols that are described in the articles by Dummett et al.[20] and Granich et al.[21] We obtained approval from the KPNC Institutional Review Board for the Protection of Human Subjects for the evaluation component that is described below.
EARLY DETECTION ALGORITHMS
The early detection algorithms we employed, which are being updated periodically, were based on our previously published work.[11, 18] Even though admitting diagnoses were found to be predictive in our original model, during actual development of the real‐time data extraction algorithms, we found that diagnoses could not be obtained reliably, so we made the decision to use a single predictive equation for all patients. The core components of the AAM score equation are the above‐mentioned LAPS2 and COPS2; these are combined with other data elements (Table 1). None of the scores are proprietary, and our equations could be replicated by any entity with a comprehensive inpatient EMR. Our early detection system is calibrated using outcomes that occurred 12 hours from when the alert is issued. For prediction, it uses data from the preceding 12 months for the COPS2 and the preceding 24 to 72 hours for physiologic data.
Category | Elements Included | Comment |
---|---|---|
Demographics | Age, sex | |
Patient location | Unit indicators (eg, 3 West); also known as bed history indicators | Only patients in general medicalsurgical ward, transitional care unit, and telemetry unit are eligible. Patients in the operating room, postanesthesia recovery room, labor and delivery service, and pediatrics are ineligible. |
Health services | Admission venue | Emergency department admission or not. |
Elapsed length of stay in hospital up to the point when data are scanned | Interhospital transport is common in our integrated delivery system; this data element requires linking both unit stays as well as stays involving different hospitals. | |
Status | Care directive orders | Patients with a comfort careonly order are not eligible; all other patients (full code, partial code, and do not resuscitate) are. |
Admission status | Inpatients and patients admitted for observation status are eligible. | |
Physiologic | Vital signs, laboratory tests, neurological status checks | See online Appendices and references [6] and [15] for details on how we extract, format, and transform these variables. |
Composite indices | Generic severity of illness score | See text and description in reference [15] for details on the Laboratory‐based Acute Physiology score, version 2 and the Comorbidity Point Score, version 2. |
Longitudinal comorbidity score |
During the course of developing the real‐time extraction algorithms, we encountered a number of delays in real‐time data acquisition. These fall into 2 categories: charting delay and server delay. Charting delay is due to nonautomated charting of vital signs by nurses (eg, a nurse obtains vital signs on a patient, writes them down on paper, and then enters them later). In general, this delay was in the 15‐ to 30‐minute range, but occasionally was as high as 2 hours. Server delay, which was variable and ranged from a few minutes to (occasionally) 1 to 2 hours, is due to 2 factors. The first is that certain point of care tests were not always uploaded into the EMR immediately. This is because the testing units, which can display results to clinicians within minutes, must be physically connected to a computer for uploading results. The second is the processing time required for the system to cycle through hundreds of patient records in the context of a very large EMR system (the KPNC Epic build runs in 6 separate geographic instances, and our system runs in 2 of these). Figure 1 shows that each probability estimate thus has what we called an uncertainty period of 2 hours (the +2 hours addresses the fact that we needed to give clinicians a minimum time to respond to an alert). Given limited resources and the need to balance accuracy of the alerts, adequate lead time, the presence of an uncertainty period, and alert fatigue, we elected to issue alerts every 6 hours (with the exact timing based on facility preferences).
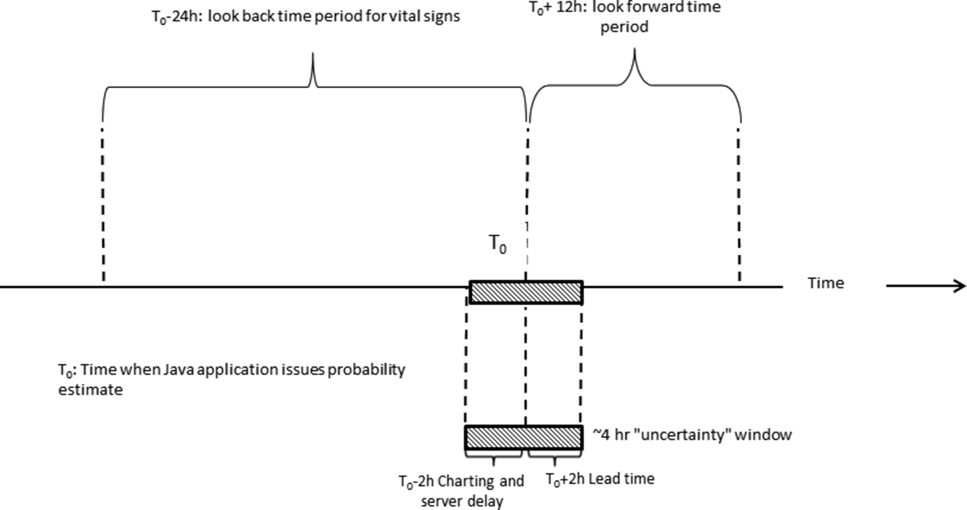
A summary of the components of our equation is provided in the Supporting Information, Appendices, in the online version of this article. The statistical performance characteristics of our final equation, which are based on approximately 262 million individual data points from 650,684 hospitalizations in which patients experienced 20,471 deteriorations, is being reported elsewhere. Between November 19, 2013 and November 30, 2015 (the most recent data currently available to us for analysis), a total of 26,386 patients admitted to the ward or transitional care unit at the 2 pilot sites were scored by the AAM system, and these patients generated 3,881 alerts involving a total of 1,413 patients, which meant an average of 2 alerts per day at South San Francisco and 4 alerts per day in Sacramento. Resource limitations have precluded us from conducting formal surveys to assess clinician acceptance. However, repeated meetings with both hospitalists as well as RRT nurses indicated that favorable departmental consensus exists.
INSTANTIATION OF ALGORITHMS IN THE EMR
Given the complexity of the calculations involving many variables (Table 1), we elected to employ Web services to extract data for processing using a Java application outside the EMR, which then pushed results into the EMR front end (Figure 2). Additional details on this decision are provided in the Supporting Information, Appendices, in the online version of this article. Our team had to expend considerable resources and time to map all necessary data elements in the real time environment, whose identifying characteristics are not the same as those employed by the KPHC data warehouse. Considerable debugging was required during the first 7 months of the pilot. Troubleshooting for the application was often required on very short notice (eg, when the system unexpectedly stopped issuing alerts during a weekend, or when 1 class of patients suddenly stopped receiving scores). It is likely that future efforts to embed algorithms in EMRs will experience similar difficulties, and it is wise to budget so as maximize available analytic and application programmer resources.
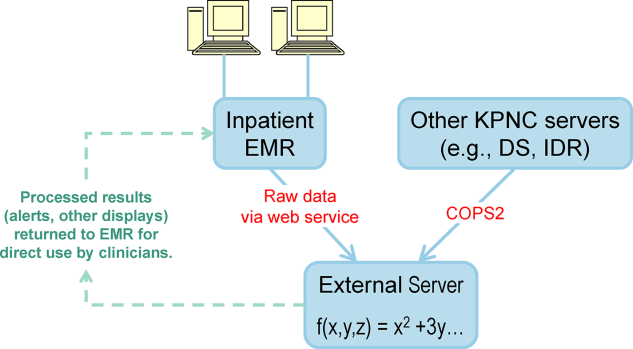
Figure 3 shows the final appearance of the graphical user interface at KPHC, which provides clinicians with 3 numbers: ADV ALERT SCORE (AAM score) is the probability of experiencing unplanned transfer within the next 12 hours, COPS is the COPS2, and LAPS is the LAPS2 assigned at the time a patient is placed in a hospital room. The current protocol in place is that the clinical response arm is triggered when the AAM score is 8.
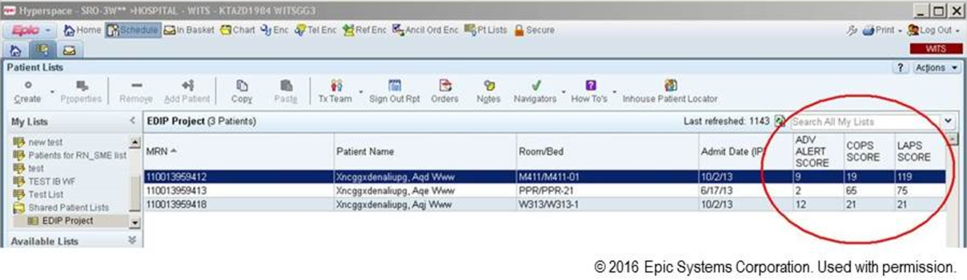
LIMITATIONS
One of the limitations of working with a commercial EMR in a large system, such as KPNC, is that of scalability. Understandably, the organization is reluctant to make changes in the EMR that will not ultimately be deployed across all hospitals in the system. Thus, any significant modification of the EMR or its associated workflows must, from the outset, be structured for subsequent spread to the remaining hospitals (19 in our case). Because we had not deployed a system like this before, we did not know what to expect and, had we known then what experience has taught us, our initial requests would have been different. Table 2 summarizes the major changes we would have made to our implementation strategy had we known then what we know now.
Component | Status in Pilot Application | Desirable Changes |
---|---|---|
| ||
Degree of disaster recovery support | System outages are handled on an ad hoc basis. | Same level of support as is seen in regular clinical systems (24/7 technical support). |
Laboratory data feed | Web service. | It would be extremely valuable to have a definite answer about whether alternative data feeds would be faster and more reliable. |
LAPS2 score | Score appears only on ward or TCU patients. | Display for all hospitalized adults (include anyone 18 years and include ICU patients). |
Score appears only on inpatient physician dashboard. | Display scores in multiple dashboards (eg, emergency department dashboard). | |
COPS2 score | Score appears only on ward or TCU patients. | Display for all hospitalized adults (include anyone 18 years and include ICU patients). |
Score appears only on inpatient physician dashboard. | Display scores in multiple dashboards (eg, emergency department dashboard). | |
Alert response tracking | None is available. | Functionality that permits tracking what the status is of patients in whom an alert was issued (who responded, where it is charted, etc.)could be structured as a workbench report in KP HealthConnectvery important because of medical legal reasons. |
Trending capability for scores | None is available. | Trending display available in same location where vital signs and laboratory test results are displayed. |
Messaging capability | Not currently available. | Transmission of scores to rapid response team (or other designated first responder) via a smartphone, thus obviating the need for staff to check the inpatient dashboard manually every 6 hours. |
EVALUATION STRATEGY
Due to institutional constraints, it is not possible for us to conduct a gold standard pilot using patient‐level randomization, as described by Kollef et al.[8] Consequently, in addition to using the pilot to surface specific implementation issues, we had to develop a parallel scoring system for capturing key data points (scores, outcomes) not just at the 2 pilot sites, but also at the remaining 19 KPNC hospitals. This required that we develop electronic tools that would permit us to capture these data elements continuously, both prospectively as well as retrospectively. Thus, to give an example, we developed a macro that we call LAPS2 any time that permits us to assign a retrospective severity score given any T0. Our ultimate goal is to evaluate the system's deployment using a stepped wedge design[22] in which geographically contiguous clusters of 2 to 4 hospitals go live periodically. The silver standard (a cluster trial involving randomization at the individual hospital level[23]) is not feasible because KPNC hospitals span a very broad geographic area, and it is more resource intensive in a shorter time span. In this context, the most important output from a pilot such as this is to generate an estimate of likely impact; this estimate then becomes a critical component for power calculations for the stepped wedge.
Our ongoing evaluation has all the limitations inherent in the analysis of nonrandomized interventions. Because it only involves 2 hospitals, it is difficult to assess variation due to facility‐specific factors. Finally, because our priority was to avoid alert fatigue, the total number of patients who experience an alert is small, limiting available sample size. Given these constraints, we will employ a counterfactual method, multivariate matching,[24, 25, 26] so as to come as close as possible to simulating a randomized trial. To control for hospital‐specific factors, matching will be combined with difference‐in‐differences[27, 28] methodology. Our basic approach takes advantage of the fact that, although our alert system is currently running in 2 hospitals, it is possible for us to assign a retrospective alert to patients at all KPNC hospitals. Using multivariate matching techniques, we will then create a cohort in which each patient who received an alert is matched to 2 patients who are given a retrospective virtual alert during the same time period in control facilities. The pre‐ and postimplementation outcomes of pilot and matched controls are compared. The matching algorithms specify exact matches on membership status, whether or not the patient had been admitted to the ICU prior to the first alert, and whether or not the patient was full code at the time of an alert. Once potential matches are found using the above procedures, our algorithms seek the closest match for the following variables: age, alert probability, COPS2, and admission LAPS2. Membership status is important, because many individuals who are not covered by the Kaiser Foundation Health Plan, Inc., are hospitalized at KPNC hospitals. Because these nonmembers' postdischarge outcomes cannot be tracked, it is important to control for this variable in our analyses.
Our electronic evaluation strategy also can be used to quantify pilot effects on length of stay (total, after an alert, and ICU), rehospitalization, use of hospice, mortality, and cost. However, it is not adequate for the evaluation of whether or not patient preferences are respected. Consequently, we have also developed manual review instruments for structured electronic chart review (the coding form and manual are provided in the online Appendix of the article in this issue of Journal of Hospital Medicine by Granich et al.[21]). This review will focus on issues such as whether or not patients' surrogates were identified, whether goals of care were discussed, and so forth. In those cases where patients died in the hospital, we will also review whether death occurred after resuscitation, whether family members were present, and so forth.
As noted above and in Figure 1, charting delays can result in uncertainty periods. We have found that these delays can also result in discrepancies in which data extracted from the real time system do not match those extracted from the data warehouse. These discrepancies can complicate creation of analysis datasets, which in turn can lead to delays in completing analyses. Such delays can cause significant problems with stakeholders. In retrospect, we should have devoted more resources to ongoing electronic audits and to the development of algorithms that formally address charting delays.
LESSONS LEARNED AND THOUGHTS ON FUTURE DISSEMINATION
We believe that embedding predictive models in the EMR will become an essential component of clinical care. Despite resource limitations and having to work in a frontier area, we did 3 things well. We were able to embed a complex set of equations and display their outputs in a commercial EMR outside the research setting. In a setting where hospitalists could have requested discontinuation of the system, we achieved consensus that it should remain the standard of care. Lastly, as a result of this work, KPNC will be deploying this early warning system in all its hospitals, so our overall implementation and communication strategy has been sound.
Nonetheless, our road to implementation has been a bumpy one, and we have learned a number of valuable lessons that are being incorporated into our future work. They merit sharing with the broader medical community. Using the title of a song by Ricky SkaggsIf I Had It All Again to Dowe can summarize what we learned with 3 phrases: engage leadership early, provide simpler explanations, and embed the evaluation in the solution.
Although our research on risk adjustment and the epidemiology was known to many KPNC leaders and clinicians, our initial engagement focus was on connecting with hospital physicians and operational leaders who worked in quality improvement. In retrospect, the research team should have engaged with 2 different communities much soonerthe information technology community and that component of leadership that focused on the EMR and information technology issues. Although these 2 broad communities interact with operations all the time, they do not necessarily have regular contact with research developments that might affect both EMR as well as quality improvement operations simultaneously. Not seeking this early engagement probably slowed our work by 9 to 15 months, because of repeated delays resulting from our assumption that the information technology teams understood things that were clear to us but not to them. One major result of this at KPNC is that we now have a regular quarterly meeting between researchers and the EMR leadership. The goal of this regular meeting is to make sure that operational leaders and researchers contemplating projects with an informatics component communicate early, long before any consideration of implementation occurs.
Whereas the notion of providing early warning seems intuitive and simple, translating this into a set of equations is challenging. However, we have found that developing equations is much easier than developing communication strategies suitable for people who are not interested in statistics, a group that probably constitutes the majority of clinicians. One major result of this learning now guiding our work is that our team devotes more time to considering existing and possible workflows. This process includes spending more time engaging with clinicians around how they use information. We are also experimenting with different ways of illustrating statistical concepts (eg, probabilities, likelihood ratios).
As is discussed in the article by Dummett et al.,[20] 1 workflow component that remains unresolved is that of documentation. It is not clear what the documentation standard should be for a deterioration probability. Solving this particular conundrum is not something that can be done by electronic or statistical means. However, also with the benefit of hindsight, we now know that we should have put more energy into automated electronic tools that provide support for documentation after an alert. In addition to being requested by clinicians, having tools that automatically generate tracers as part of both the alerting and documentation process would also make evaluation easier. For example, it would permit a better delineation of the causal path between the intervention (providing a deterioration probability) and patient outcomes. In future projects, incorporation of such tools will get much more prominence.
Acknowledgements
The authors thank Dr. Michelle Caughey, Dr. Philip Madvig, Dr. Patricia Conolly, and Ms. Barbara Crawford for their administrative support, Dr. Tracy Lieu for reviewing the manuscript, and Ms. Rachel Lesser for formatting the manuscript.
Disclosures: This work was supported by a grant from the Gordon and Betty Moore Foundation (Early Detection, Prevention, and Mitigation of Impending Physiologic Deterioration in Hospitalized Patients Outside Intensive Care: Phase 3, pilot), The Permanente Medical Group, Inc., and Kaiser Foundation Hospitals, Inc. As part of our agreement with the Gordon and Betty Moore Foundation, we made a commitment to disseminate our findings in articles such as this one. However, the Foundation and its staff played no role in how we actually structured our articles, nor did they review or preapprove any of the manuscripts submitted as part of the dissemination component. Dr. Liu was supported by the National Institute for General Medical Sciences award K23GM112018. None of the sponsors had any involvement in our decision to submit this manuscript or in the determination of its contents. None of the authors has any conflicts of interest to declare of relevance to this work
- Intra‐hospital transfers to a higher level of care: contribution to total hospital and intensive care unit (ICU) mortality and length of stay (LOS). J Hosp Med. 2011;6(2):74–80. , , , , , .
- Adverse outcomes associated with delayed intensive care unit transfers in an integrated healthcare system. J Hosp Med. 2012;7(3):224–230. , , , .
- Risk factors for unplanned transfer to intensive care within 24 hours of admission from the emergency department in an integrated healthcare system. J Hosp Med. 2012;8(1):13–19. , , , , , .
- The medical emergency team: a new strategy to identify and intervene in high‐risk surgical patients. Clin Intensive Care. 1995;6:269–272. , , , , .
- The medical emergency team. Anaesth Intensive Care. 1995;23(2):183–186. , , , .
- The critically ill: following your MEWS. QJM. 2001;94(10):507–510. .
- National Health Service. National Early Warning Score (NEWS). Standardising the Assessment Of Acute‐Illness Severity in the NHS. Report of a Working Party. London, United Kingdom: Royal College of Physicians; 2012.
- A randomized trial of real‐time automated clinical deterioration alerts sent to a rapid response team. J Hosp Med. 2014;9(7):424–429. , , , et al.
- Automated detection of physiologic deterioration in hospitalized patients. J Am Med Inform Assoc. 2015;22(2):350–360. , , , et al.
- Identifying patients at increased risk for unplanned readmission. Med Care. 2013;51(9):761–766. , , , , .
- Early detection of impending physiologic deterioration among patients who are not in intensive care: development of predictive models using data from an automated electronic medical record. J Hosp Med. 2012;7(5):388–395. , , , , , .
- Early detection of impending deterioration outside the ICU: a difference‐in‐differences (DiD) study. Presented at: American Thoracic Society International Conference, San Francisco, California; May 13–18, 2016; A7614. , , , et al.
- Unplanned transfers to a medical intensive care unit: causes and relationship to preventable errors in care. J Hosp Med. 2011;6(2):68–72. , , , .
- Rapid response teams—walk, don't run. JAMA. 2006;296(13):1645–1647. , , .
- Rapid response systems: a systematic review. Crit Care Med. 2007;35(5):1238–1243. , , , , , .
- Rethinking rapid response teams. JAMA. 2010;304(12):1375–1376. , .
- Risk adjusting hospital inpatient mortality using automated inpatient, outpatient, and laboratory databases. Med Care. 2008;46(3):232–239. , , , , , .
- Risk‐adjusting hospital mortality using a comprehensive electronic record in an integrated healthcare delivery system. Med Care. 2013;51(5):446–453. , , , , .
- Nonelective rehospitalizations and post‐discharge mortality: predictive models suitable for use in real time. Med Care. 2015;53(11):916–923. , , , , , .
- J Hosp Med. 2016;11:000–000. et al.
- J Hosp Med. 2016;11:000–000. et al.
- Design and analysis of stepped wedge cluster randomized trials. Contemp Clin Trials. 2007;28(2):182–191. , .
- Cluster randomized trials: evaluating treatments applied to groups. JAMA. 2015;313(20):2068–2069. , .
- Comparison of multivariate matching methods: structures, distances, and algorithms. J Comput Graph Stat. 1993;2(4):405–420. , .
- A method/macro based on propensity score and Mahalanobis distance to reduce bias in treatment comparison in observational study. Eli Lilly working paper available at: http://www.lexjansen.com/pharmasug/2006/publichealthresearch/pr05.pdf. , , .
- Matching methods for causal inference: a review and a look forward. Stat Sci. 2010;25(1):1–21. .
- Methods for evaluating changes in health care policy: the difference‐in‐differences approach. JAMA. 2014;312(22):2401–2402. , .
- Why we should not be indifferent to specification choices for difference‐in‐differences. Health Serv Res. 2015;50(4):1211–1235. , , .
Patients who deteriorate in the hospital and are transferred to the intensive care unit (ICU) have higher mortality and greater morbidity than those directly admitted from the emergency department.[1, 2, 3] Rapid response teams (RRTs) were created to address this problem.[4, 5] Quantitative tools, such as the Modified Early Warning Score (MEWS),[6] have been used to support RRTs almost since their inception. Nonetheless, work on developing scores that can serve as triggers for RRT evaluation or intervention continues. The notion that comprehensive inpatient electronic medical records (EMRs) could support RRTs (both as a source of patient data and a platform for providing alerts) has intuitive appeal. Not surprisingly, in addition to newer versions of manual scores,[7] electronic scores are now entering clinical practice. These newer systems are being tested in research institutions,[8] hospitals with advanced capabilities,[9] and as part of proprietary systems.[10] Although a fair amount of statistical information (eg, area under the receiver operator characteristic curve of a given predictive model) on the performance of various trigger systems has been published, existing reports have not described details of how the electronic architecture is integrated with clinical practice.
Electronic alert systems generated from physiology‐based predictive models do not yet constitute mature technologies. No consensus or legal mandate regarding their role yet exists. Given this situation, studying different implementation approaches and their outcomes has value. It is instructive to consider how a given institutional solution addresses common contingenciesoperational constraints that are likely to be present, albeit in different forms, in most placesto help others understand the limitations and issues they may present. In this article we describe the structure of an EMR‐based early warning system in 2 pilot hospitals at Kaiser Permanente Northern California (KPNC). In this pilot, we embedded an updated version of a previously described early warning score[11] into the EMR. We will emphasize how its components address institutional, operational, and technological constraints. Finally, we will also describe unfinished businesschanges we would like to see in a future dissemination phase. Two important aspects of the pilot (development of a clinical response arm and addressing patient preferences with respect to supportive care) are being described elsewhere in this issue of the Journal of Hospital Medicine. Analyses of the actual impact on patient outcomes will be reported elsewhere; initial results appear favorable.[12]
INITIAL CONSTRAINTS
The ability to actually prevent inpatient deteriorations may be limited,[13] and doubts regarding the value of RRTs persist.[14, 15, 16] Consequently, work that led to the pilot occurred in stages. In the first stage (prior to 2010), our team presented data to internal audiences documenting the rates and outcomes of unplanned transfers from the ward to the ICU. Concurrently, our team developed a first generation risk adjustment methodology that was published in 2008.[17] We used this methodology to show that unplanned transfers did, in fact, have elevated mortality, and that this persisted after risk adjustment.[1, 2, 3] This phase of our work coincided with KPNC's deployment of the Epic inpatient EMR (
Once we demonstrated that we could, in fact, predict inpatient deteriorations, we still had to address medicallegal considerations, the need for a clinical response arm, and how to address patient preferences with respect to supportive or palliative care. To address these concerns and ensure that the implementation would be seamlessly integrated with routine clinical practice, our team worked for 1 year with hospitalists and other clinicians at the pilot sites prior to the go‐live date.
The primary concern from a medicallegal perspective is that once results from a predictive model (which could be an alert, severity score, comorbidity score, or other probability estimate) are displayed in the chart, relevant clinical information has been changed. Thus, failure to address such an EMR item could lead to malpractice risk for individuals and/or enterprise liability for an organization. After discussing this with senior leadership, they specified that it would be permissible to go forward so long as we could document that an educational intervention was in place to make sure that clinicians understood the system and that it was linked to specific protocols approved by hospitalists.
Current predictive models, including ours, generate a probability estimate. They do not necessarily identify the etiology of a problem or what solutions ought to be considered. Consequently, our senior leadership insisted that we be able to answer clinicians' basic question: What do we do when we get an alert? The article by Dummett et al.[20] in this issue of the Journal of Hospital Medicine describes how we addressed this constraint. Lastly, not all patients can be rescued. The article by Granich et al.[21] describes how we handled the need to respect patient choices.
PROCEDURAL COMPONENTS
The Gordon and Betty Moore Foundation, which funded the pilot, only had 1 restriction (inclusion of a hospital in the Sacramento, California area). The other site was selected based on 2 initial criteria: (1) the chosen site had to be 1 of the smaller KPNC hospitals, and (2) the chosen site had to be easily accessible for the lead author (G.J.E.). The KPNC South San Francisco hospital was selected as the alpha site and the KPNC Sacramento hospital as the beta site. One of the major drivers for these decisions was that both had robust palliative care services. The Sacramento hospital is a larger hospital with a more complex caseload.
Prior to the go‐live dates (November 19, 2013 for South San Francisco and April 16, 2014 for Sacramento), the executive committees at both hospitals reviewed preliminary data and the implementation plans for the early warning system. Following these reviews, the executive committees approved the deployment. Also during this phase, in consultation with our communications departments, we adopted the name Advance Alert Monitoring (AAM) as the outward facing name for the system. We also developed recommended scripts for clinical staff to employ when approaching a patient in whom an alert had been issued (this is because the alert is calibrated so as to predict increased risk of deterioration within the next 12 hours, which means that a patient might be surprised as to why clinicians were suddenly evaluating them). Facility approvals occurred approximately 1 month prior to the go‐live date at each hospital, permitting a shadowing phase. In this phase, selected physicians were provided with probability estimates and severity scores, but these were not displayed in the EMR front end. This shadowing phase permitted clinicians to finalize the response arms' protocols that are described in the articles by Dummett et al.[20] and Granich et al.[21] We obtained approval from the KPNC Institutional Review Board for the Protection of Human Subjects for the evaluation component that is described below.
EARLY DETECTION ALGORITHMS
The early detection algorithms we employed, which are being updated periodically, were based on our previously published work.[11, 18] Even though admitting diagnoses were found to be predictive in our original model, during actual development of the real‐time data extraction algorithms, we found that diagnoses could not be obtained reliably, so we made the decision to use a single predictive equation for all patients. The core components of the AAM score equation are the above‐mentioned LAPS2 and COPS2; these are combined with other data elements (Table 1). None of the scores are proprietary, and our equations could be replicated by any entity with a comprehensive inpatient EMR. Our early detection system is calibrated using outcomes that occurred 12 hours from when the alert is issued. For prediction, it uses data from the preceding 12 months for the COPS2 and the preceding 24 to 72 hours for physiologic data.
Category | Elements Included | Comment |
---|---|---|
Demographics | Age, sex | |
Patient location | Unit indicators (eg, 3 West); also known as bed history indicators | Only patients in general medicalsurgical ward, transitional care unit, and telemetry unit are eligible. Patients in the operating room, postanesthesia recovery room, labor and delivery service, and pediatrics are ineligible. |
Health services | Admission venue | Emergency department admission or not. |
Elapsed length of stay in hospital up to the point when data are scanned | Interhospital transport is common in our integrated delivery system; this data element requires linking both unit stays as well as stays involving different hospitals. | |
Status | Care directive orders | Patients with a comfort careonly order are not eligible; all other patients (full code, partial code, and do not resuscitate) are. |
Admission status | Inpatients and patients admitted for observation status are eligible. | |
Physiologic | Vital signs, laboratory tests, neurological status checks | See online Appendices and references [6] and [15] for details on how we extract, format, and transform these variables. |
Composite indices | Generic severity of illness score | See text and description in reference [15] for details on the Laboratory‐based Acute Physiology score, version 2 and the Comorbidity Point Score, version 2. |
Longitudinal comorbidity score |
During the course of developing the real‐time extraction algorithms, we encountered a number of delays in real‐time data acquisition. These fall into 2 categories: charting delay and server delay. Charting delay is due to nonautomated charting of vital signs by nurses (eg, a nurse obtains vital signs on a patient, writes them down on paper, and then enters them later). In general, this delay was in the 15‐ to 30‐minute range, but occasionally was as high as 2 hours. Server delay, which was variable and ranged from a few minutes to (occasionally) 1 to 2 hours, is due to 2 factors. The first is that certain point of care tests were not always uploaded into the EMR immediately. This is because the testing units, which can display results to clinicians within minutes, must be physically connected to a computer for uploading results. The second is the processing time required for the system to cycle through hundreds of patient records in the context of a very large EMR system (the KPNC Epic build runs in 6 separate geographic instances, and our system runs in 2 of these). Figure 1 shows that each probability estimate thus has what we called an uncertainty period of 2 hours (the +2 hours addresses the fact that we needed to give clinicians a minimum time to respond to an alert). Given limited resources and the need to balance accuracy of the alerts, adequate lead time, the presence of an uncertainty period, and alert fatigue, we elected to issue alerts every 6 hours (with the exact timing based on facility preferences).
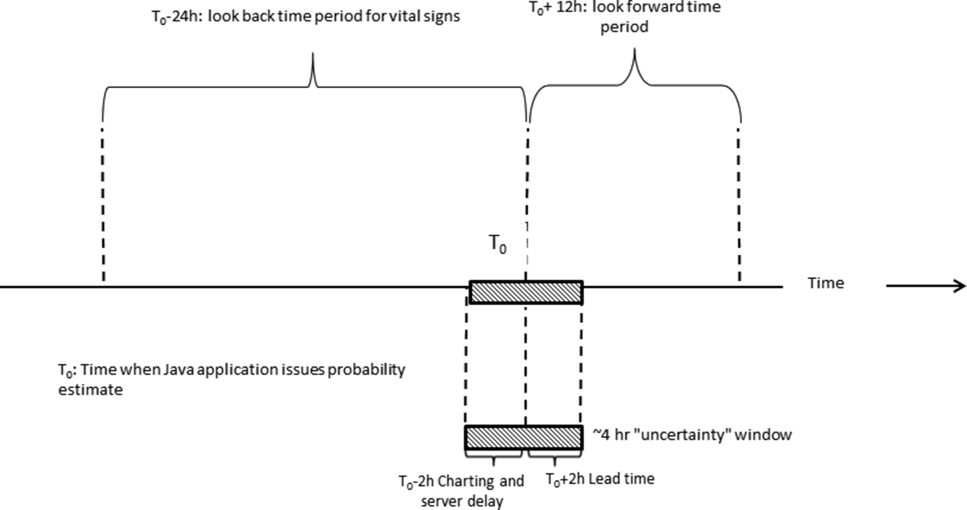
A summary of the components of our equation is provided in the Supporting Information, Appendices, in the online version of this article. The statistical performance characteristics of our final equation, which are based on approximately 262 million individual data points from 650,684 hospitalizations in which patients experienced 20,471 deteriorations, is being reported elsewhere. Between November 19, 2013 and November 30, 2015 (the most recent data currently available to us for analysis), a total of 26,386 patients admitted to the ward or transitional care unit at the 2 pilot sites were scored by the AAM system, and these patients generated 3,881 alerts involving a total of 1,413 patients, which meant an average of 2 alerts per day at South San Francisco and 4 alerts per day in Sacramento. Resource limitations have precluded us from conducting formal surveys to assess clinician acceptance. However, repeated meetings with both hospitalists as well as RRT nurses indicated that favorable departmental consensus exists.
INSTANTIATION OF ALGORITHMS IN THE EMR
Given the complexity of the calculations involving many variables (Table 1), we elected to employ Web services to extract data for processing using a Java application outside the EMR, which then pushed results into the EMR front end (Figure 2). Additional details on this decision are provided in the Supporting Information, Appendices, in the online version of this article. Our team had to expend considerable resources and time to map all necessary data elements in the real time environment, whose identifying characteristics are not the same as those employed by the KPHC data warehouse. Considerable debugging was required during the first 7 months of the pilot. Troubleshooting for the application was often required on very short notice (eg, when the system unexpectedly stopped issuing alerts during a weekend, or when 1 class of patients suddenly stopped receiving scores). It is likely that future efforts to embed algorithms in EMRs will experience similar difficulties, and it is wise to budget so as maximize available analytic and application programmer resources.
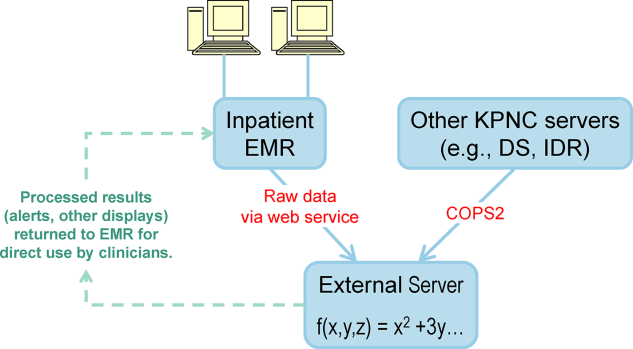
Figure 3 shows the final appearance of the graphical user interface at KPHC, which provides clinicians with 3 numbers: ADV ALERT SCORE (AAM score) is the probability of experiencing unplanned transfer within the next 12 hours, COPS is the COPS2, and LAPS is the LAPS2 assigned at the time a patient is placed in a hospital room. The current protocol in place is that the clinical response arm is triggered when the AAM score is 8.
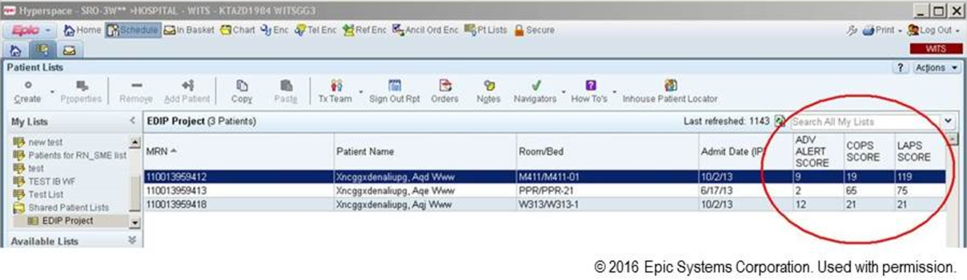
LIMITATIONS
One of the limitations of working with a commercial EMR in a large system, such as KPNC, is that of scalability. Understandably, the organization is reluctant to make changes in the EMR that will not ultimately be deployed across all hospitals in the system. Thus, any significant modification of the EMR or its associated workflows must, from the outset, be structured for subsequent spread to the remaining hospitals (19 in our case). Because we had not deployed a system like this before, we did not know what to expect and, had we known then what experience has taught us, our initial requests would have been different. Table 2 summarizes the major changes we would have made to our implementation strategy had we known then what we know now.
Component | Status in Pilot Application | Desirable Changes |
---|---|---|
| ||
Degree of disaster recovery support | System outages are handled on an ad hoc basis. | Same level of support as is seen in regular clinical systems (24/7 technical support). |
Laboratory data feed | Web service. | It would be extremely valuable to have a definite answer about whether alternative data feeds would be faster and more reliable. |
LAPS2 score | Score appears only on ward or TCU patients. | Display for all hospitalized adults (include anyone 18 years and include ICU patients). |
Score appears only on inpatient physician dashboard. | Display scores in multiple dashboards (eg, emergency department dashboard). | |
COPS2 score | Score appears only on ward or TCU patients. | Display for all hospitalized adults (include anyone 18 years and include ICU patients). |
Score appears only on inpatient physician dashboard. | Display scores in multiple dashboards (eg, emergency department dashboard). | |
Alert response tracking | None is available. | Functionality that permits tracking what the status is of patients in whom an alert was issued (who responded, where it is charted, etc.)could be structured as a workbench report in KP HealthConnectvery important because of medical legal reasons. |
Trending capability for scores | None is available. | Trending display available in same location where vital signs and laboratory test results are displayed. |
Messaging capability | Not currently available. | Transmission of scores to rapid response team (or other designated first responder) via a smartphone, thus obviating the need for staff to check the inpatient dashboard manually every 6 hours. |
EVALUATION STRATEGY
Due to institutional constraints, it is not possible for us to conduct a gold standard pilot using patient‐level randomization, as described by Kollef et al.[8] Consequently, in addition to using the pilot to surface specific implementation issues, we had to develop a parallel scoring system for capturing key data points (scores, outcomes) not just at the 2 pilot sites, but also at the remaining 19 KPNC hospitals. This required that we develop electronic tools that would permit us to capture these data elements continuously, both prospectively as well as retrospectively. Thus, to give an example, we developed a macro that we call LAPS2 any time that permits us to assign a retrospective severity score given any T0. Our ultimate goal is to evaluate the system's deployment using a stepped wedge design[22] in which geographically contiguous clusters of 2 to 4 hospitals go live periodically. The silver standard (a cluster trial involving randomization at the individual hospital level[23]) is not feasible because KPNC hospitals span a very broad geographic area, and it is more resource intensive in a shorter time span. In this context, the most important output from a pilot such as this is to generate an estimate of likely impact; this estimate then becomes a critical component for power calculations for the stepped wedge.
Our ongoing evaluation has all the limitations inherent in the analysis of nonrandomized interventions. Because it only involves 2 hospitals, it is difficult to assess variation due to facility‐specific factors. Finally, because our priority was to avoid alert fatigue, the total number of patients who experience an alert is small, limiting available sample size. Given these constraints, we will employ a counterfactual method, multivariate matching,[24, 25, 26] so as to come as close as possible to simulating a randomized trial. To control for hospital‐specific factors, matching will be combined with difference‐in‐differences[27, 28] methodology. Our basic approach takes advantage of the fact that, although our alert system is currently running in 2 hospitals, it is possible for us to assign a retrospective alert to patients at all KPNC hospitals. Using multivariate matching techniques, we will then create a cohort in which each patient who received an alert is matched to 2 patients who are given a retrospective virtual alert during the same time period in control facilities. The pre‐ and postimplementation outcomes of pilot and matched controls are compared. The matching algorithms specify exact matches on membership status, whether or not the patient had been admitted to the ICU prior to the first alert, and whether or not the patient was full code at the time of an alert. Once potential matches are found using the above procedures, our algorithms seek the closest match for the following variables: age, alert probability, COPS2, and admission LAPS2. Membership status is important, because many individuals who are not covered by the Kaiser Foundation Health Plan, Inc., are hospitalized at KPNC hospitals. Because these nonmembers' postdischarge outcomes cannot be tracked, it is important to control for this variable in our analyses.
Our electronic evaluation strategy also can be used to quantify pilot effects on length of stay (total, after an alert, and ICU), rehospitalization, use of hospice, mortality, and cost. However, it is not adequate for the evaluation of whether or not patient preferences are respected. Consequently, we have also developed manual review instruments for structured electronic chart review (the coding form and manual are provided in the online Appendix of the article in this issue of Journal of Hospital Medicine by Granich et al.[21]). This review will focus on issues such as whether or not patients' surrogates were identified, whether goals of care were discussed, and so forth. In those cases where patients died in the hospital, we will also review whether death occurred after resuscitation, whether family members were present, and so forth.
As noted above and in Figure 1, charting delays can result in uncertainty periods. We have found that these delays can also result in discrepancies in which data extracted from the real time system do not match those extracted from the data warehouse. These discrepancies can complicate creation of analysis datasets, which in turn can lead to delays in completing analyses. Such delays can cause significant problems with stakeholders. In retrospect, we should have devoted more resources to ongoing electronic audits and to the development of algorithms that formally address charting delays.
LESSONS LEARNED AND THOUGHTS ON FUTURE DISSEMINATION
We believe that embedding predictive models in the EMR will become an essential component of clinical care. Despite resource limitations and having to work in a frontier area, we did 3 things well. We were able to embed a complex set of equations and display their outputs in a commercial EMR outside the research setting. In a setting where hospitalists could have requested discontinuation of the system, we achieved consensus that it should remain the standard of care. Lastly, as a result of this work, KPNC will be deploying this early warning system in all its hospitals, so our overall implementation and communication strategy has been sound.
Nonetheless, our road to implementation has been a bumpy one, and we have learned a number of valuable lessons that are being incorporated into our future work. They merit sharing with the broader medical community. Using the title of a song by Ricky SkaggsIf I Had It All Again to Dowe can summarize what we learned with 3 phrases: engage leadership early, provide simpler explanations, and embed the evaluation in the solution.
Although our research on risk adjustment and the epidemiology was known to many KPNC leaders and clinicians, our initial engagement focus was on connecting with hospital physicians and operational leaders who worked in quality improvement. In retrospect, the research team should have engaged with 2 different communities much soonerthe information technology community and that component of leadership that focused on the EMR and information technology issues. Although these 2 broad communities interact with operations all the time, they do not necessarily have regular contact with research developments that might affect both EMR as well as quality improvement operations simultaneously. Not seeking this early engagement probably slowed our work by 9 to 15 months, because of repeated delays resulting from our assumption that the information technology teams understood things that were clear to us but not to them. One major result of this at KPNC is that we now have a regular quarterly meeting between researchers and the EMR leadership. The goal of this regular meeting is to make sure that operational leaders and researchers contemplating projects with an informatics component communicate early, long before any consideration of implementation occurs.
Whereas the notion of providing early warning seems intuitive and simple, translating this into a set of equations is challenging. However, we have found that developing equations is much easier than developing communication strategies suitable for people who are not interested in statistics, a group that probably constitutes the majority of clinicians. One major result of this learning now guiding our work is that our team devotes more time to considering existing and possible workflows. This process includes spending more time engaging with clinicians around how they use information. We are also experimenting with different ways of illustrating statistical concepts (eg, probabilities, likelihood ratios).
As is discussed in the article by Dummett et al.,[20] 1 workflow component that remains unresolved is that of documentation. It is not clear what the documentation standard should be for a deterioration probability. Solving this particular conundrum is not something that can be done by electronic or statistical means. However, also with the benefit of hindsight, we now know that we should have put more energy into automated electronic tools that provide support for documentation after an alert. In addition to being requested by clinicians, having tools that automatically generate tracers as part of both the alerting and documentation process would also make evaluation easier. For example, it would permit a better delineation of the causal path between the intervention (providing a deterioration probability) and patient outcomes. In future projects, incorporation of such tools will get much more prominence.
Acknowledgements
The authors thank Dr. Michelle Caughey, Dr. Philip Madvig, Dr. Patricia Conolly, and Ms. Barbara Crawford for their administrative support, Dr. Tracy Lieu for reviewing the manuscript, and Ms. Rachel Lesser for formatting the manuscript.
Disclosures: This work was supported by a grant from the Gordon and Betty Moore Foundation (Early Detection, Prevention, and Mitigation of Impending Physiologic Deterioration in Hospitalized Patients Outside Intensive Care: Phase 3, pilot), The Permanente Medical Group, Inc., and Kaiser Foundation Hospitals, Inc. As part of our agreement with the Gordon and Betty Moore Foundation, we made a commitment to disseminate our findings in articles such as this one. However, the Foundation and its staff played no role in how we actually structured our articles, nor did they review or preapprove any of the manuscripts submitted as part of the dissemination component. Dr. Liu was supported by the National Institute for General Medical Sciences award K23GM112018. None of the sponsors had any involvement in our decision to submit this manuscript or in the determination of its contents. None of the authors has any conflicts of interest to declare of relevance to this work
Patients who deteriorate in the hospital and are transferred to the intensive care unit (ICU) have higher mortality and greater morbidity than those directly admitted from the emergency department.[1, 2, 3] Rapid response teams (RRTs) were created to address this problem.[4, 5] Quantitative tools, such as the Modified Early Warning Score (MEWS),[6] have been used to support RRTs almost since their inception. Nonetheless, work on developing scores that can serve as triggers for RRT evaluation or intervention continues. The notion that comprehensive inpatient electronic medical records (EMRs) could support RRTs (both as a source of patient data and a platform for providing alerts) has intuitive appeal. Not surprisingly, in addition to newer versions of manual scores,[7] electronic scores are now entering clinical practice. These newer systems are being tested in research institutions,[8] hospitals with advanced capabilities,[9] and as part of proprietary systems.[10] Although a fair amount of statistical information (eg, area under the receiver operator characteristic curve of a given predictive model) on the performance of various trigger systems has been published, existing reports have not described details of how the electronic architecture is integrated with clinical practice.
Electronic alert systems generated from physiology‐based predictive models do not yet constitute mature technologies. No consensus or legal mandate regarding their role yet exists. Given this situation, studying different implementation approaches and their outcomes has value. It is instructive to consider how a given institutional solution addresses common contingenciesoperational constraints that are likely to be present, albeit in different forms, in most placesto help others understand the limitations and issues they may present. In this article we describe the structure of an EMR‐based early warning system in 2 pilot hospitals at Kaiser Permanente Northern California (KPNC). In this pilot, we embedded an updated version of a previously described early warning score[11] into the EMR. We will emphasize how its components address institutional, operational, and technological constraints. Finally, we will also describe unfinished businesschanges we would like to see in a future dissemination phase. Two important aspects of the pilot (development of a clinical response arm and addressing patient preferences with respect to supportive care) are being described elsewhere in this issue of the Journal of Hospital Medicine. Analyses of the actual impact on patient outcomes will be reported elsewhere; initial results appear favorable.[12]
INITIAL CONSTRAINTS
The ability to actually prevent inpatient deteriorations may be limited,[13] and doubts regarding the value of RRTs persist.[14, 15, 16] Consequently, work that led to the pilot occurred in stages. In the first stage (prior to 2010), our team presented data to internal audiences documenting the rates and outcomes of unplanned transfers from the ward to the ICU. Concurrently, our team developed a first generation risk adjustment methodology that was published in 2008.[17] We used this methodology to show that unplanned transfers did, in fact, have elevated mortality, and that this persisted after risk adjustment.[1, 2, 3] This phase of our work coincided with KPNC's deployment of the Epic inpatient EMR (
Once we demonstrated that we could, in fact, predict inpatient deteriorations, we still had to address medicallegal considerations, the need for a clinical response arm, and how to address patient preferences with respect to supportive or palliative care. To address these concerns and ensure that the implementation would be seamlessly integrated with routine clinical practice, our team worked for 1 year with hospitalists and other clinicians at the pilot sites prior to the go‐live date.
The primary concern from a medicallegal perspective is that once results from a predictive model (which could be an alert, severity score, comorbidity score, or other probability estimate) are displayed in the chart, relevant clinical information has been changed. Thus, failure to address such an EMR item could lead to malpractice risk for individuals and/or enterprise liability for an organization. After discussing this with senior leadership, they specified that it would be permissible to go forward so long as we could document that an educational intervention was in place to make sure that clinicians understood the system and that it was linked to specific protocols approved by hospitalists.
Current predictive models, including ours, generate a probability estimate. They do not necessarily identify the etiology of a problem or what solutions ought to be considered. Consequently, our senior leadership insisted that we be able to answer clinicians' basic question: What do we do when we get an alert? The article by Dummett et al.[20] in this issue of the Journal of Hospital Medicine describes how we addressed this constraint. Lastly, not all patients can be rescued. The article by Granich et al.[21] describes how we handled the need to respect patient choices.
PROCEDURAL COMPONENTS
The Gordon and Betty Moore Foundation, which funded the pilot, only had 1 restriction (inclusion of a hospital in the Sacramento, California area). The other site was selected based on 2 initial criteria: (1) the chosen site had to be 1 of the smaller KPNC hospitals, and (2) the chosen site had to be easily accessible for the lead author (G.J.E.). The KPNC South San Francisco hospital was selected as the alpha site and the KPNC Sacramento hospital as the beta site. One of the major drivers for these decisions was that both had robust palliative care services. The Sacramento hospital is a larger hospital with a more complex caseload.
Prior to the go‐live dates (November 19, 2013 for South San Francisco and April 16, 2014 for Sacramento), the executive committees at both hospitals reviewed preliminary data and the implementation plans for the early warning system. Following these reviews, the executive committees approved the deployment. Also during this phase, in consultation with our communications departments, we adopted the name Advance Alert Monitoring (AAM) as the outward facing name for the system. We also developed recommended scripts for clinical staff to employ when approaching a patient in whom an alert had been issued (this is because the alert is calibrated so as to predict increased risk of deterioration within the next 12 hours, which means that a patient might be surprised as to why clinicians were suddenly evaluating them). Facility approvals occurred approximately 1 month prior to the go‐live date at each hospital, permitting a shadowing phase. In this phase, selected physicians were provided with probability estimates and severity scores, but these were not displayed in the EMR front end. This shadowing phase permitted clinicians to finalize the response arms' protocols that are described in the articles by Dummett et al.[20] and Granich et al.[21] We obtained approval from the KPNC Institutional Review Board for the Protection of Human Subjects for the evaluation component that is described below.
EARLY DETECTION ALGORITHMS
The early detection algorithms we employed, which are being updated periodically, were based on our previously published work.[11, 18] Even though admitting diagnoses were found to be predictive in our original model, during actual development of the real‐time data extraction algorithms, we found that diagnoses could not be obtained reliably, so we made the decision to use a single predictive equation for all patients. The core components of the AAM score equation are the above‐mentioned LAPS2 and COPS2; these are combined with other data elements (Table 1). None of the scores are proprietary, and our equations could be replicated by any entity with a comprehensive inpatient EMR. Our early detection system is calibrated using outcomes that occurred 12 hours from when the alert is issued. For prediction, it uses data from the preceding 12 months for the COPS2 and the preceding 24 to 72 hours for physiologic data.
Category | Elements Included | Comment |
---|---|---|
Demographics | Age, sex | |
Patient location | Unit indicators (eg, 3 West); also known as bed history indicators | Only patients in general medicalsurgical ward, transitional care unit, and telemetry unit are eligible. Patients in the operating room, postanesthesia recovery room, labor and delivery service, and pediatrics are ineligible. |
Health services | Admission venue | Emergency department admission or not. |
Elapsed length of stay in hospital up to the point when data are scanned | Interhospital transport is common in our integrated delivery system; this data element requires linking both unit stays as well as stays involving different hospitals. | |
Status | Care directive orders | Patients with a comfort careonly order are not eligible; all other patients (full code, partial code, and do not resuscitate) are. |
Admission status | Inpatients and patients admitted for observation status are eligible. | |
Physiologic | Vital signs, laboratory tests, neurological status checks | See online Appendices and references [6] and [15] for details on how we extract, format, and transform these variables. |
Composite indices | Generic severity of illness score | See text and description in reference [15] for details on the Laboratory‐based Acute Physiology score, version 2 and the Comorbidity Point Score, version 2. |
Longitudinal comorbidity score |
During the course of developing the real‐time extraction algorithms, we encountered a number of delays in real‐time data acquisition. These fall into 2 categories: charting delay and server delay. Charting delay is due to nonautomated charting of vital signs by nurses (eg, a nurse obtains vital signs on a patient, writes them down on paper, and then enters them later). In general, this delay was in the 15‐ to 30‐minute range, but occasionally was as high as 2 hours. Server delay, which was variable and ranged from a few minutes to (occasionally) 1 to 2 hours, is due to 2 factors. The first is that certain point of care tests were not always uploaded into the EMR immediately. This is because the testing units, which can display results to clinicians within minutes, must be physically connected to a computer for uploading results. The second is the processing time required for the system to cycle through hundreds of patient records in the context of a very large EMR system (the KPNC Epic build runs in 6 separate geographic instances, and our system runs in 2 of these). Figure 1 shows that each probability estimate thus has what we called an uncertainty period of 2 hours (the +2 hours addresses the fact that we needed to give clinicians a minimum time to respond to an alert). Given limited resources and the need to balance accuracy of the alerts, adequate lead time, the presence of an uncertainty period, and alert fatigue, we elected to issue alerts every 6 hours (with the exact timing based on facility preferences).
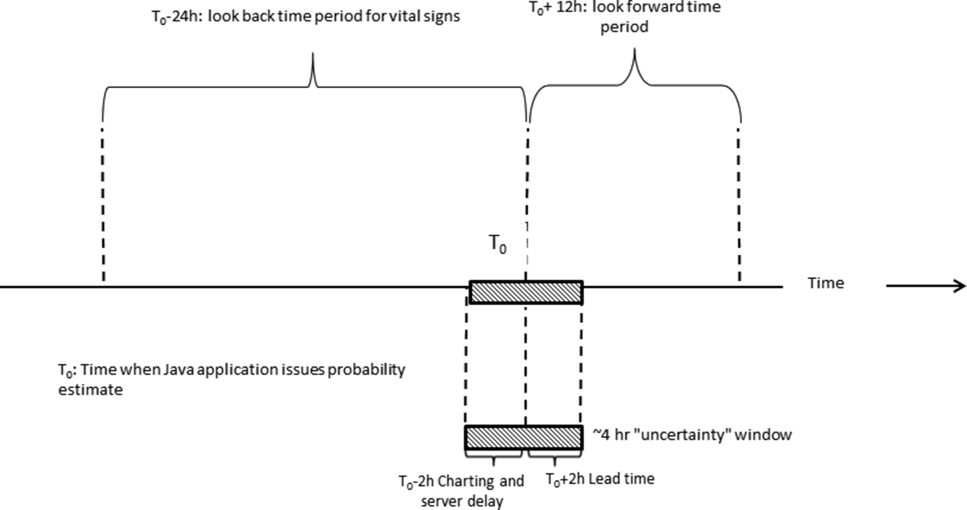
A summary of the components of our equation is provided in the Supporting Information, Appendices, in the online version of this article. The statistical performance characteristics of our final equation, which are based on approximately 262 million individual data points from 650,684 hospitalizations in which patients experienced 20,471 deteriorations, is being reported elsewhere. Between November 19, 2013 and November 30, 2015 (the most recent data currently available to us for analysis), a total of 26,386 patients admitted to the ward or transitional care unit at the 2 pilot sites were scored by the AAM system, and these patients generated 3,881 alerts involving a total of 1,413 patients, which meant an average of 2 alerts per day at South San Francisco and 4 alerts per day in Sacramento. Resource limitations have precluded us from conducting formal surveys to assess clinician acceptance. However, repeated meetings with both hospitalists as well as RRT nurses indicated that favorable departmental consensus exists.
INSTANTIATION OF ALGORITHMS IN THE EMR
Given the complexity of the calculations involving many variables (Table 1), we elected to employ Web services to extract data for processing using a Java application outside the EMR, which then pushed results into the EMR front end (Figure 2). Additional details on this decision are provided in the Supporting Information, Appendices, in the online version of this article. Our team had to expend considerable resources and time to map all necessary data elements in the real time environment, whose identifying characteristics are not the same as those employed by the KPHC data warehouse. Considerable debugging was required during the first 7 months of the pilot. Troubleshooting for the application was often required on very short notice (eg, when the system unexpectedly stopped issuing alerts during a weekend, or when 1 class of patients suddenly stopped receiving scores). It is likely that future efforts to embed algorithms in EMRs will experience similar difficulties, and it is wise to budget so as maximize available analytic and application programmer resources.
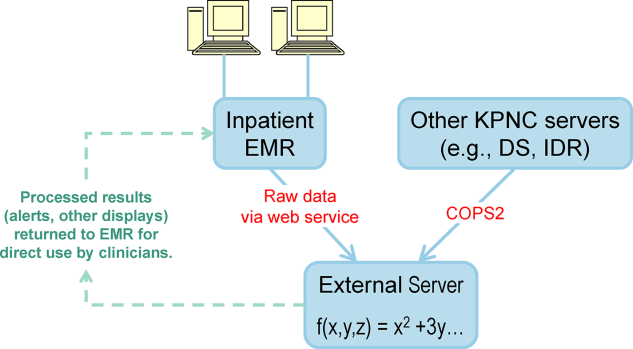
Figure 3 shows the final appearance of the graphical user interface at KPHC, which provides clinicians with 3 numbers: ADV ALERT SCORE (AAM score) is the probability of experiencing unplanned transfer within the next 12 hours, COPS is the COPS2, and LAPS is the LAPS2 assigned at the time a patient is placed in a hospital room. The current protocol in place is that the clinical response arm is triggered when the AAM score is 8.
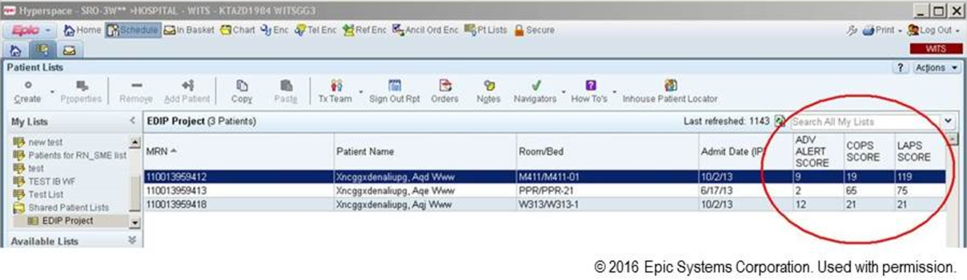
LIMITATIONS
One of the limitations of working with a commercial EMR in a large system, such as KPNC, is that of scalability. Understandably, the organization is reluctant to make changes in the EMR that will not ultimately be deployed across all hospitals in the system. Thus, any significant modification of the EMR or its associated workflows must, from the outset, be structured for subsequent spread to the remaining hospitals (19 in our case). Because we had not deployed a system like this before, we did not know what to expect and, had we known then what experience has taught us, our initial requests would have been different. Table 2 summarizes the major changes we would have made to our implementation strategy had we known then what we know now.
Component | Status in Pilot Application | Desirable Changes |
---|---|---|
| ||
Degree of disaster recovery support | System outages are handled on an ad hoc basis. | Same level of support as is seen in regular clinical systems (24/7 technical support). |
Laboratory data feed | Web service. | It would be extremely valuable to have a definite answer about whether alternative data feeds would be faster and more reliable. |
LAPS2 score | Score appears only on ward or TCU patients. | Display for all hospitalized adults (include anyone 18 years and include ICU patients). |
Score appears only on inpatient physician dashboard. | Display scores in multiple dashboards (eg, emergency department dashboard). | |
COPS2 score | Score appears only on ward or TCU patients. | Display for all hospitalized adults (include anyone 18 years and include ICU patients). |
Score appears only on inpatient physician dashboard. | Display scores in multiple dashboards (eg, emergency department dashboard). | |
Alert response tracking | None is available. | Functionality that permits tracking what the status is of patients in whom an alert was issued (who responded, where it is charted, etc.)could be structured as a workbench report in KP HealthConnectvery important because of medical legal reasons. |
Trending capability for scores | None is available. | Trending display available in same location where vital signs and laboratory test results are displayed. |
Messaging capability | Not currently available. | Transmission of scores to rapid response team (or other designated first responder) via a smartphone, thus obviating the need for staff to check the inpatient dashboard manually every 6 hours. |
EVALUATION STRATEGY
Due to institutional constraints, it is not possible for us to conduct a gold standard pilot using patient‐level randomization, as described by Kollef et al.[8] Consequently, in addition to using the pilot to surface specific implementation issues, we had to develop a parallel scoring system for capturing key data points (scores, outcomes) not just at the 2 pilot sites, but also at the remaining 19 KPNC hospitals. This required that we develop electronic tools that would permit us to capture these data elements continuously, both prospectively as well as retrospectively. Thus, to give an example, we developed a macro that we call LAPS2 any time that permits us to assign a retrospective severity score given any T0. Our ultimate goal is to evaluate the system's deployment using a stepped wedge design[22] in which geographically contiguous clusters of 2 to 4 hospitals go live periodically. The silver standard (a cluster trial involving randomization at the individual hospital level[23]) is not feasible because KPNC hospitals span a very broad geographic area, and it is more resource intensive in a shorter time span. In this context, the most important output from a pilot such as this is to generate an estimate of likely impact; this estimate then becomes a critical component for power calculations for the stepped wedge.
Our ongoing evaluation has all the limitations inherent in the analysis of nonrandomized interventions. Because it only involves 2 hospitals, it is difficult to assess variation due to facility‐specific factors. Finally, because our priority was to avoid alert fatigue, the total number of patients who experience an alert is small, limiting available sample size. Given these constraints, we will employ a counterfactual method, multivariate matching,[24, 25, 26] so as to come as close as possible to simulating a randomized trial. To control for hospital‐specific factors, matching will be combined with difference‐in‐differences[27, 28] methodology. Our basic approach takes advantage of the fact that, although our alert system is currently running in 2 hospitals, it is possible for us to assign a retrospective alert to patients at all KPNC hospitals. Using multivariate matching techniques, we will then create a cohort in which each patient who received an alert is matched to 2 patients who are given a retrospective virtual alert during the same time period in control facilities. The pre‐ and postimplementation outcomes of pilot and matched controls are compared. The matching algorithms specify exact matches on membership status, whether or not the patient had been admitted to the ICU prior to the first alert, and whether or not the patient was full code at the time of an alert. Once potential matches are found using the above procedures, our algorithms seek the closest match for the following variables: age, alert probability, COPS2, and admission LAPS2. Membership status is important, because many individuals who are not covered by the Kaiser Foundation Health Plan, Inc., are hospitalized at KPNC hospitals. Because these nonmembers' postdischarge outcomes cannot be tracked, it is important to control for this variable in our analyses.
Our electronic evaluation strategy also can be used to quantify pilot effects on length of stay (total, after an alert, and ICU), rehospitalization, use of hospice, mortality, and cost. However, it is not adequate for the evaluation of whether or not patient preferences are respected. Consequently, we have also developed manual review instruments for structured electronic chart review (the coding form and manual are provided in the online Appendix of the article in this issue of Journal of Hospital Medicine by Granich et al.[21]). This review will focus on issues such as whether or not patients' surrogates were identified, whether goals of care were discussed, and so forth. In those cases where patients died in the hospital, we will also review whether death occurred after resuscitation, whether family members were present, and so forth.
As noted above and in Figure 1, charting delays can result in uncertainty periods. We have found that these delays can also result in discrepancies in which data extracted from the real time system do not match those extracted from the data warehouse. These discrepancies can complicate creation of analysis datasets, which in turn can lead to delays in completing analyses. Such delays can cause significant problems with stakeholders. In retrospect, we should have devoted more resources to ongoing electronic audits and to the development of algorithms that formally address charting delays.
LESSONS LEARNED AND THOUGHTS ON FUTURE DISSEMINATION
We believe that embedding predictive models in the EMR will become an essential component of clinical care. Despite resource limitations and having to work in a frontier area, we did 3 things well. We were able to embed a complex set of equations and display their outputs in a commercial EMR outside the research setting. In a setting where hospitalists could have requested discontinuation of the system, we achieved consensus that it should remain the standard of care. Lastly, as a result of this work, KPNC will be deploying this early warning system in all its hospitals, so our overall implementation and communication strategy has been sound.
Nonetheless, our road to implementation has been a bumpy one, and we have learned a number of valuable lessons that are being incorporated into our future work. They merit sharing with the broader medical community. Using the title of a song by Ricky SkaggsIf I Had It All Again to Dowe can summarize what we learned with 3 phrases: engage leadership early, provide simpler explanations, and embed the evaluation in the solution.
Although our research on risk adjustment and the epidemiology was known to many KPNC leaders and clinicians, our initial engagement focus was on connecting with hospital physicians and operational leaders who worked in quality improvement. In retrospect, the research team should have engaged with 2 different communities much soonerthe information technology community and that component of leadership that focused on the EMR and information technology issues. Although these 2 broad communities interact with operations all the time, they do not necessarily have regular contact with research developments that might affect both EMR as well as quality improvement operations simultaneously. Not seeking this early engagement probably slowed our work by 9 to 15 months, because of repeated delays resulting from our assumption that the information technology teams understood things that were clear to us but not to them. One major result of this at KPNC is that we now have a regular quarterly meeting between researchers and the EMR leadership. The goal of this regular meeting is to make sure that operational leaders and researchers contemplating projects with an informatics component communicate early, long before any consideration of implementation occurs.
Whereas the notion of providing early warning seems intuitive and simple, translating this into a set of equations is challenging. However, we have found that developing equations is much easier than developing communication strategies suitable for people who are not interested in statistics, a group that probably constitutes the majority of clinicians. One major result of this learning now guiding our work is that our team devotes more time to considering existing and possible workflows. This process includes spending more time engaging with clinicians around how they use information. We are also experimenting with different ways of illustrating statistical concepts (eg, probabilities, likelihood ratios).
As is discussed in the article by Dummett et al.,[20] 1 workflow component that remains unresolved is that of documentation. It is not clear what the documentation standard should be for a deterioration probability. Solving this particular conundrum is not something that can be done by electronic or statistical means. However, also with the benefit of hindsight, we now know that we should have put more energy into automated electronic tools that provide support for documentation after an alert. In addition to being requested by clinicians, having tools that automatically generate tracers as part of both the alerting and documentation process would also make evaluation easier. For example, it would permit a better delineation of the causal path between the intervention (providing a deterioration probability) and patient outcomes. In future projects, incorporation of such tools will get much more prominence.
Acknowledgements
The authors thank Dr. Michelle Caughey, Dr. Philip Madvig, Dr. Patricia Conolly, and Ms. Barbara Crawford for their administrative support, Dr. Tracy Lieu for reviewing the manuscript, and Ms. Rachel Lesser for formatting the manuscript.
Disclosures: This work was supported by a grant from the Gordon and Betty Moore Foundation (Early Detection, Prevention, and Mitigation of Impending Physiologic Deterioration in Hospitalized Patients Outside Intensive Care: Phase 3, pilot), The Permanente Medical Group, Inc., and Kaiser Foundation Hospitals, Inc. As part of our agreement with the Gordon and Betty Moore Foundation, we made a commitment to disseminate our findings in articles such as this one. However, the Foundation and its staff played no role in how we actually structured our articles, nor did they review or preapprove any of the manuscripts submitted as part of the dissemination component. Dr. Liu was supported by the National Institute for General Medical Sciences award K23GM112018. None of the sponsors had any involvement in our decision to submit this manuscript or in the determination of its contents. None of the authors has any conflicts of interest to declare of relevance to this work
- Intra‐hospital transfers to a higher level of care: contribution to total hospital and intensive care unit (ICU) mortality and length of stay (LOS). J Hosp Med. 2011;6(2):74–80. , , , , , .
- Adverse outcomes associated with delayed intensive care unit transfers in an integrated healthcare system. J Hosp Med. 2012;7(3):224–230. , , , .
- Risk factors for unplanned transfer to intensive care within 24 hours of admission from the emergency department in an integrated healthcare system. J Hosp Med. 2012;8(1):13–19. , , , , , .
- The medical emergency team: a new strategy to identify and intervene in high‐risk surgical patients. Clin Intensive Care. 1995;6:269–272. , , , , .
- The medical emergency team. Anaesth Intensive Care. 1995;23(2):183–186. , , , .
- The critically ill: following your MEWS. QJM. 2001;94(10):507–510. .
- National Health Service. National Early Warning Score (NEWS). Standardising the Assessment Of Acute‐Illness Severity in the NHS. Report of a Working Party. London, United Kingdom: Royal College of Physicians; 2012.
- A randomized trial of real‐time automated clinical deterioration alerts sent to a rapid response team. J Hosp Med. 2014;9(7):424–429. , , , et al.
- Automated detection of physiologic deterioration in hospitalized patients. J Am Med Inform Assoc. 2015;22(2):350–360. , , , et al.
- Identifying patients at increased risk for unplanned readmission. Med Care. 2013;51(9):761–766. , , , , .
- Early detection of impending physiologic deterioration among patients who are not in intensive care: development of predictive models using data from an automated electronic medical record. J Hosp Med. 2012;7(5):388–395. , , , , , .
- Early detection of impending deterioration outside the ICU: a difference‐in‐differences (DiD) study. Presented at: American Thoracic Society International Conference, San Francisco, California; May 13–18, 2016; A7614. , , , et al.
- Unplanned transfers to a medical intensive care unit: causes and relationship to preventable errors in care. J Hosp Med. 2011;6(2):68–72. , , , .
- Rapid response teams—walk, don't run. JAMA. 2006;296(13):1645–1647. , , .
- Rapid response systems: a systematic review. Crit Care Med. 2007;35(5):1238–1243. , , , , , .
- Rethinking rapid response teams. JAMA. 2010;304(12):1375–1376. , .
- Risk adjusting hospital inpatient mortality using automated inpatient, outpatient, and laboratory databases. Med Care. 2008;46(3):232–239. , , , , , .
- Risk‐adjusting hospital mortality using a comprehensive electronic record in an integrated healthcare delivery system. Med Care. 2013;51(5):446–453. , , , , .
- Nonelective rehospitalizations and post‐discharge mortality: predictive models suitable for use in real time. Med Care. 2015;53(11):916–923. , , , , , .
- J Hosp Med. 2016;11:000–000. et al.
- J Hosp Med. 2016;11:000–000. et al.
- Design and analysis of stepped wedge cluster randomized trials. Contemp Clin Trials. 2007;28(2):182–191. , .
- Cluster randomized trials: evaluating treatments applied to groups. JAMA. 2015;313(20):2068–2069. , .
- Comparison of multivariate matching methods: structures, distances, and algorithms. J Comput Graph Stat. 1993;2(4):405–420. , .
- A method/macro based on propensity score and Mahalanobis distance to reduce bias in treatment comparison in observational study. Eli Lilly working paper available at: http://www.lexjansen.com/pharmasug/2006/publichealthresearch/pr05.pdf. , , .
- Matching methods for causal inference: a review and a look forward. Stat Sci. 2010;25(1):1–21. .
- Methods for evaluating changes in health care policy: the difference‐in‐differences approach. JAMA. 2014;312(22):2401–2402. , .
- Why we should not be indifferent to specification choices for difference‐in‐differences. Health Serv Res. 2015;50(4):1211–1235. , , .
- Intra‐hospital transfers to a higher level of care: contribution to total hospital and intensive care unit (ICU) mortality and length of stay (LOS). J Hosp Med. 2011;6(2):74–80. , , , , , .
- Adverse outcomes associated with delayed intensive care unit transfers in an integrated healthcare system. J Hosp Med. 2012;7(3):224–230. , , , .
- Risk factors for unplanned transfer to intensive care within 24 hours of admission from the emergency department in an integrated healthcare system. J Hosp Med. 2012;8(1):13–19. , , , , , .
- The medical emergency team: a new strategy to identify and intervene in high‐risk surgical patients. Clin Intensive Care. 1995;6:269–272. , , , , .
- The medical emergency team. Anaesth Intensive Care. 1995;23(2):183–186. , , , .
- The critically ill: following your MEWS. QJM. 2001;94(10):507–510. .
- National Health Service. National Early Warning Score (NEWS). Standardising the Assessment Of Acute‐Illness Severity in the NHS. Report of a Working Party. London, United Kingdom: Royal College of Physicians; 2012.
- A randomized trial of real‐time automated clinical deterioration alerts sent to a rapid response team. J Hosp Med. 2014;9(7):424–429. , , , et al.
- Automated detection of physiologic deterioration in hospitalized patients. J Am Med Inform Assoc. 2015;22(2):350–360. , , , et al.
- Identifying patients at increased risk for unplanned readmission. Med Care. 2013;51(9):761–766. , , , , .
- Early detection of impending physiologic deterioration among patients who are not in intensive care: development of predictive models using data from an automated electronic medical record. J Hosp Med. 2012;7(5):388–395. , , , , , .
- Early detection of impending deterioration outside the ICU: a difference‐in‐differences (DiD) study. Presented at: American Thoracic Society International Conference, San Francisco, California; May 13–18, 2016; A7614. , , , et al.
- Unplanned transfers to a medical intensive care unit: causes and relationship to preventable errors in care. J Hosp Med. 2011;6(2):68–72. , , , .
- Rapid response teams—walk, don't run. JAMA. 2006;296(13):1645–1647. , , .
- Rapid response systems: a systematic review. Crit Care Med. 2007;35(5):1238–1243. , , , , , .
- Rethinking rapid response teams. JAMA. 2010;304(12):1375–1376. , .
- Risk adjusting hospital inpatient mortality using automated inpatient, outpatient, and laboratory databases. Med Care. 2008;46(3):232–239. , , , , , .
- Risk‐adjusting hospital mortality using a comprehensive electronic record in an integrated healthcare delivery system. Med Care. 2013;51(5):446–453. , , , , .
- Nonelective rehospitalizations and post‐discharge mortality: predictive models suitable for use in real time. Med Care. 2015;53(11):916–923. , , , , , .
- J Hosp Med. 2016;11:000–000. et al.
- J Hosp Med. 2016;11:000–000. et al.
- Design and analysis of stepped wedge cluster randomized trials. Contemp Clin Trials. 2007;28(2):182–191. , .
- Cluster randomized trials: evaluating treatments applied to groups. JAMA. 2015;313(20):2068–2069. , .
- Comparison of multivariate matching methods: structures, distances, and algorithms. J Comput Graph Stat. 1993;2(4):405–420. , .
- A method/macro based on propensity score and Mahalanobis distance to reduce bias in treatment comparison in observational study. Eli Lilly working paper available at: http://www.lexjansen.com/pharmasug/2006/publichealthresearch/pr05.pdf. , , .
- Matching methods for causal inference: a review and a look forward. Stat Sci. 2010;25(1):1–21. .
- Methods for evaluating changes in health care policy: the difference‐in‐differences approach. JAMA. 2014;312(22):2401–2402. , .
- Why we should not be indifferent to specification choices for difference‐in‐differences. Health Serv Res. 2015;50(4):1211–1235. , , .
© 2016 Society of Hospital Medicine