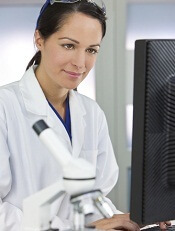
Photo by Darren Baker
Researchers say they have developed the first computer machine-learning model to accurately predict which patients diagnosed with acute
myeloid leukemia (AML) will go into remission after treatment and which will relapse.
“It’s pretty straightforward to teach a computer to recognize AML, once you develop a robust algorithm, and, in previous work, we did it with almost 100% accuracy,” said study author Murat Dundar, PhD of Indiana University-Purdue University Indianapolis.
“What was challenging was to go beyond that work and teach the computer to accurately predict the direction of change in disease progression in AML patients, interpreting new data to predict the unknown: which new AML patients will go into remission and which will relapse.”
Dr Dundar and his colleagues described this work in IEEE Transactions on Biomedical Engineering.
The researchers said they modeled data from multiple flow cytometry samples to identify functionally distinct cell populations and their local realizations. Each sample was characterized by the proportions of recovered cell populations, which were used to predict the direction of change in disease progression for each AML patient.
“As the input, our computational system employs data from flow cytometry, a widely utilized technology that can rapidly provide detailed characteristics of single cells in samples such as blood or bone marrow,” explained study author Bartek Rajwa, PhD, of Purdue University in Lafayette, Indiana.
“Traditionally, the results of flow cytometry analyses are evaluated by highly trained human experts rather than by machine-learning algorithms. But computers are often better at extracting knowledge from complex data than humans are.”
The researchers used 200 diseased and non-diseased immunophenotypic panels for training and tested
the computational system with samples collected at multiple time points from 36 additional AML patients.
The system was able to predict remission with 100% accuracy (26 of 26 cases) and relapse with 90% accuracy (9 of 10 cases).
“Machine learning is not about modeling data,” Dr Dundar noted. “It’s about extracting knowledge from the data you have so you can build a powerful, intuitive tool that can make predictions about future data that the computer has not previously seen. The machine is learning, not memorizing, and that’s what we did.”
