User login
HN‐Associated Healthcare Burden
Hyponatremia is an electrolyte disorder most commonly defined as a serum sodium concentration <135 mEq/L.1 Its exact definition can vary across studies, but typically ranges between <130 and <138 mEq/L.2, 3 Signs and symptoms of hyponatremia can include malaise, headache, disorientation, confusion, muscle weakness, and cramps. If severe, seizures, respiratory arrest, brainstem herniation, coma, and death may result.
The incidence of hyponatremia in the general hospitalized population has been reported to range between 1% and 6% when defined as <130135 mEq/L,4, 5 and its occurrence increases with a more prolonged hospital stay to 13%.6 A recent study reported that when hyponatremia was defined with a less stringent threshold of <138 mEq/L, the incidence at admission rose to 38%.3 Hyponatremia is a comorbid condition of multiple diseases, occurring in approximately 20% of patients with heart failure,7, 8 and 40% to 57% of patients with advanced cirrhosis.9, 10 The syndrome of the inappropriate release of antidiuretic hormone (SIADH) is additionally a predominant cause of hyponatremia, with a prevalence reported as high as 35% in hospitalized patients.11
Hyponatremia is not only widespread, but also an independent predictor of mortality. In a retrospective cohort analysis, Waikar et al reported that in comparison to patients who were normonatremic, patients with serum sodium concentrations <135 mEq/L had a risk of in‐hospital mortality as high as 47%, and that this risk doubled for patients with serum sodium concentrations between 125 and 129 mEq/L.6 In the study by Wald et al, which defined hyponatremia as <138 mEq/L, the risk of in‐hospital mortality was similar.3 In both of these studies, even mild hyponatremia (130137 mEq/L) was associated with increased risk of in‐hospital mortality.3, 6
The overall cost of hyponatremia is estimated to range between $1.6 and $3.6 billion for 2011.12, 13 Hospital readmissions are a significant contributor to total healthcare costs, with some being entirely avoidable with increased standards of care. The Centers for Medicare and Medicaid Services has begun to not only publicly report hospital readmission rates, but also penalize hospitals for early readmissions.14 Strategies to reduce hospital readmissions are currently being integrated into healthcare reform policy.14 In the present study, the incremental burden of hospitalized hyponatremic (HN) versus non‐HN patients in terms of hospital resource utilization, costs, and early hospital readmission in the real‐world was evaluated.
METHODS
Study Design
This study was a retrospective analysis that examined healthcare utilization and costs among HN patients using the Premier Hospital Database. The database contains over 310 million hospital encounters from more than 700 US hospitals, or 1 out of every 4 discharges in the US. The administrative data available included patient and provider demographics, diagnoses and procedures, as well as date‐stamped billing records for all pharmacy, laboratory, imaging, procedures, and supplies.
Patient Selection and Matching
HN patients were eligible for study inclusion if they were a hospital inpatient discharged between January 1, 2007 and March 31, 2010, were >18 years of age at admission, and had either a primary or secondary diagnosis of hyponatremia or hyposmolality (defined as International Classification of Diseases, Ninth Revision (ICD‐9‐CM) code: 276.1x). Patients were excluded if they had been transferred from another acute care facility, transferred to another acute care or critical access facility, or left against medical advice. Labor and delivery patients (ICD‐9‐CM codes: 72.xx‐74.xx, V22.x, V23.x, V27.x, and V28.x), and patients classified as observational were also excluded. A second cohort of non‐hyponatremic patients was created using the same inclusion and exclusion criteria, with the exception that patients not have a primary or secondary diagnosis of hyponatremia or hyposmolality (defined as ICD‐9‐CM code: 276.1x).
The matching of hyponatremia (HN) and control (non‐HN) cohorts was accomplished using a combination of exact and propensity score matching techniques. Patients were first exact matched on age, gender, Medicare Severity‐Diagnosis Related Group (MS‐DRG) assignment, and hospital geographic region. Propensity score matching was further utilized to create the final study cohorts for outcomes comparisons. Propensity score matching is commonly used in retrospective cohort studies to correct for sample selection bias due to observable differences between groups.15, 16 The propensity score was generated using logistic regression with the dependent variable as hyponatremia (yes vs no) and the following covariates: age, race, admission source, attending physician specialty, 3M All Patient Refined‐Diagnosis Related Group (APR‐DRG) Severity of Illness and Risk of Mortality index scores, Deyo‐Charlson Comorbidity Index score, selected hyponatremia‐related comorbidity conditions, and hospital size, region, and urban/rural designation. These covariates were initially selected by an expert panel of physicians, and backward selection was utilized in the logistic regression using the most parsimonious model.17
Following generation of the propensity scores, HN patients were matched to non‐HN patients 1:1 using a nearest neighbor matching algorithm, including hospital identification and propensity score.18 Inclusion of hospital identification in the matching sequence, as well as provider characteristics, especially hospital size, attending physician specialty, and geographic region in the propensity score, was used to control for potential clustering effects at the physician and hospital level.19 During the propensity score matching process, likelihood‐ratio test, Hosmer‐Lemshow goodness of fit, and concordance c statistics were utilized to assess the fitness of the models.20 The final propensity score model produced a concordance c statistic of 0.8.
Outcome Measures and Statistical Analyses
The following outcome measures were compared between the matched HN and non‐HN patient cohorts: total and intensive care unit (ICU) hospitalization costs, total and ICU length of stay (LOS), ICU admission, and 30‐day hospital readmission. Bivariate descriptive statistics were employed to test for significant differences in demographics, patient clinical characteristics, and unadjusted costs and healthcare resource utilization and readmission rates between patient cohorts. To detect statistically significant differences in continuous and categorical variables, respectively, t tests and chi‐square tests were performed.
Multivariate analysis of outcome measures utilized generalized linear models. Due to the skewed nature of LOS and cost data, LOS was analyzed using multivariate negative binomial regression and cost was analyzed using multivariate gamma regression.21 Binary outcomes (ICU admission and 30‐day readmission) were analyzed using multivariate logistic regression. The analysis accounted for potential confounding factors by inclusion of the following covariates: age group, gender, race, admission source, and Deyo‐Charlson Comorbidity Index score. These covariates were previously identified in the Wald et al hyponatremia study,3 and were verified using likelihood‐ratio, Hosmer‐Lemshow goodness of fit, and concordance c statistics.
Subgroup Sensitivity Analysis
For the subgroup sensitivity analyses, patients were identified as having community‐acquired pneumonia (CAP), congestive heart failure (CHF), urinary tract infection (UTI), or chronic obstructive pulmonary disease (COPD) based upon principal diagnosis codes. Patients were categorized according to these subgroup definitions, and then previously matched patients with the same subgroup classification constituted the final analysis set for each subgroup. The methods that were used for the overall matched analysis were then applied to each subgroup to evaluate the incremental burden of overall cost and LOS associated with hyponatremia.
RESULTS
Patient Population
Of the 606,057 HN patients eligible for matching, a total of 558,815 HN patients were matched to 558,815 non‐HN patients, a 92% match ratio. Table 1 describes the overall characteristics of the patient populations. For both cohorts, median age was 70 years, 57% of patients were female, and approximately 67% were white. The majority of patients in either cohort had Medicare coverage (55%), and approximately 75% of patients entered the hospital via the emergency room with nearly 70% having a 3M APR‐DRG disease severity level of major or extreme. Patients of both cohorts were most often attended by an internist or a hospitalist, with a combined percentage of approximately 60%. A small, but greater proportion of HN patients had comorbidities of cancer, pulmonary disease, and SIADH. Comorbid conditions of liver cirrhosis/hepatic disease and human immunodeficiency virus (HIV) were similarly distributed among both patient cohorts.
Hyponatremic | Non‐Hyponatremic | |
---|---|---|
Discharges N (%) | Discharges N (%) | |
| ||
Sample Discharges | 558,815 (100.0%) | 558,815 (100.0%) |
Age median (IQR) | 70.00 (57.081.0) | 70.00 (57.081.0) |
Gender | ||
Female | 319,069 (57.1%) | 319,069 (57.1%) |
Male | 239,746 (42.9%) | 239,746 (42.9%) |
Race | ||
American Indian | 3,465 (0.6%) | 3,448 (0.6%) |
Asian/Pacific | 10,065 (1.8%) | 9,690 (1.7%) |
Black | 63,776 (11.4%) | 66,233 (11.9%) |
Hispanic | 24,341 (4.4%) | 24,426 (4.4%) |
White | 377,434 (67.5%) | 376,639 (67.4%) |
Other/unknown | 79,734 (14.3%) | 78,379 (14.0%) |
Primary payer | ||
Medicaretraditional | 310,312 (55.5%) | 310,643 (55.6%) |
Managed care | 75,476 (13.5%) | 78,184 (14.0%) |
Medicaremanaged care | 45,439 (8.1%) | 47,947 (8.6%) |
Non‐cap | ||
Medicaid | 44,690 (8.0%) | 43,767 (7.8%) |
Other | 82,898 (14.8%) | 78,274 (14.0%) |
Admission source | ||
Physician referral | 5,022 (0.9%) | 4,636 (0.8%) |
Transfer from another nonacute health facility | 18,031 (3.2%) | 18,163 (3.3%) |
Emergency room | 417,556 (74.7%) | 420,401 (75.2%) |
Other/unknown | 118,206 (21.2%) | 115,615 (20.7%) |
APR‐DRG severity of illness | ||
1Minor | 14,257 (2.6%) | 12,993 (2.3%) |
2Moderate | 174,859 (31.3%) | 179,356 (32.1%) |
3Major | 263,814 (47.2%) | 265,422 (47.5%) |
4Extreme | 105,885 (19.0%) | 101,044 (18.1%) |
Attending physician specialty | ||
Internal Medicine | 235,628 (42.1%) | 240,875 (43.1%) |
Hospitalist | 88,250 (15.8%) | 87,720 (15.7%) |
Family Practice (FP) | 73,346 (13.1%) | 72,828 (13.0%) |
Orthopedic Surgery (ORS) | 23,595 (4.2%) | 22,949 (4.1%) |
Cardiovascular Diseases (CD) | 19,521 (3.5%) | 18,057 (3.2%) |
Comorbidities | ||
Human immunodeficiency virus | 3,971 (0.7%) | 4,018 (0.7%) |
Cancer/neoplasm/malignancy | 107,851 (19.3%) | 105,199 (18.8%) |
Pulmonary disease | 77,849 (13.9%) | 77,184 (13.8%) |
Cirrhosis/hepatic disease | 23,038 (4.1%) | 23,418 (4.2%) |
SIADH | 1,972 (0.4%) | 1,278 (0.2%) |
Subgroup populations | ||
Community‐acquired pneumonia | 26,291 (4.7%) | 26,291 (4.7%) |
Congestive heart failure | 23,020 (4.1%) | 23,020 (4.1%) |
Urinary tract infection | 14,238 (2.6%) | 14,238 (2.6%) |
COPD | 8,696 (1.6%) | 8,696 (1.6%) |
CCI median (IQR) | 3.0 (1.05.0) | 3.0 (1.05.0) |
No. of premier hospitals | 459 | |
Provider region | ||
East North Central | 78,332 (14.0%) | 78,332 (14.0%) |
East South Central | 32,122 (5.8%) | 32,122 (5.8%) |
Middle Atlantic | 73,846 (13.2%) | 73,846 (13.2%) |
Mountain | 23,761 (4.3%) | 23,761 (4.3%) |
New England | 9,493 (1.7%) | 9,493 (1.7%) |
Pacific | 73,059 (13.1%) | 73,059 (13.1%) |
South Atlantic | 175,194 (31.4%) | 175,194 (31.4%) |
West North Central | 37,913 (6.8%) | 37,913 (6.8%) |
West South Central | 55,095 (9.9%) | 55,095 (9.9%) |
Population served | ||
Rural | 69,749 (12.5%) | 68,414 (12.2%) |
Urban | 489,066 (87.5%) | 490,401 (87.8%) |
Teaching status | ||
Non‐teaching | 337,620 (60.4%) | 337,513 (60.4%) |
Teaching | 221,195 (39.6%) | 221,302 (39.6%) |
No. of hospital beds | ||
699 | 22,067 (4.0%) | 21,777 (3.9%) |
100199 | 57,367 (10.3%) | 56,097 (10.0%) |
200299 | 87,563 (15.7%) | 86,639 (15.5%) |
300499 | 218,834 (39.2%) | 220,248 (39.4%) |
500+ | 172,984 (31.0%) | 174,054 (31.2%) |
Hospital Characteristics
Patient cohorts had similar distributions with respect to hospital characteristics (Table 1). Approximately 30% of patients were provided care from hospitals located in the South Atlantic region, and between 10% and 15% were serviced from hospitals in the East North Central, Middle Atlantic, Pacific, and West South Central regions. Most hospitals providing care for patient cohorts served urban populations (88%) and were large, with hospital bed numbers 300. Approximately 60% of hospitals were non‐teaching hospitals.
Healthcare Utilization, Readmission, and Cost Differences Among Patient Cohorts
The mean LOS (8.8 10.3 vs 7.7 8.5, P < 0.001), a difference of 1.1 days and mean ICU LOS (5.5 7.9 vs 4.9 7.1 days, P < 0.001), a difference of 0.6 days were significantly greater for the HN cohort in comparison to the non‐HN cohort (Table 2). The increase in healthcare resource utilization of patients with HN was reflected in their significantly higher mean total hospital costs per admission ($15,281 $24,054 vs $13,439 $22,198, P < 0.001), a difference of $1842; and mean costs incurred in the ICU ($8525 $13,342 vs $7597 $12,695, P < 0.001), a difference of $928 (Table 2). Furthermore, patients in the HN cohort were significantly more likely to be readmitted to the hospital for any cause (17.5% vs 16.4%, P < 0.001) (Table 2).
Hyponatremic | Non‐Hyponatremic | P Value | |
---|---|---|---|
| |||
Total LOS (mean SD) | 8.8 10.3 | 7.7 8.5 | <0.001 |
Total hospitalization cost (mean SD) | $15,281 $24,054 | $13,439 $22,198 | <0.001 |
ICU admission (N, %) | 129,235 (23.1%) | 123,502 (22.1%) | <0.001 |
ICU LOS (mean SD) | 5.5 7.9 | 4.9 7.1 | <0.001 |
ICU cost (mean SD) | $8,525 $13,342 | $7,597 $12,695 | <0.001 |
30‐Day all cause readmission (N, %) | 96,063 (17.5%) | 87,058 (16.4%) | <0.001 |
Multivariate Analysis
Multivariate analysis demonstrated hyponatremia was associated with an increase in mean hospital LOS of 10.9%, [95% confidence interval: 10.4%11.5%], (P < 0.0001) and an increase in mean total hospital costs of 8.2%, [7.4%9.0%], (P < 0.0001) (Table 3). Additionally, hyponatremia was associated with an increase in ICU LOS of 10.2%, [8.7%11.8%], (P < 0.0001), and a higher ICU cost of 8.9%, [7.2%10.7%], (P < 0.0001) (Table 3). Hyponatremia was not associated with a greater likelihood of ICU admission (odds ratio = 1.0; [1.01.0], P =.5760). However, the condition was associated with a significantly greater chance of hospital readmission (odds ratio = 1.2, [1.11.2], P < 0.0001) within 30 days postdischarge (Table 3).
Overall Cohort N = 1,117,630 | CAP N = 52,582 | CHF N = 46,040 | UTI N = 28,746 | COPD N = 17,392 | |
---|---|---|---|---|---|
| |||||
Total LOS difference | 10.9% [10.4%, 11.5%] P < 0.0001 | 5.4% [4.4%, 6.5%] P < 0.0001 | 20.6% [19.0%, 22.2%] P < 0.0001 | 2.7% [1.2%, 4.2%] P = 0.0003 | 6.7% [4.8%, 8.5%] P < 0.0001 |
Total cost difference | 8.2% [7.4%, 9.0%] P < 0.0001 | 5.6% [4.0%, 7.0%] P < 0.0001 | 19.2% [17.1%, 21.4%] P < 0.0001 | 2.2% [1.4%, 4.4%] P = 0.0582 | 5.6% [3.0%, 8.2%] P < 0.0001 |
ICU admission* odds ratio | 1.0 [1.0, 1.0] P = 0.5760 | 1.0 [1.0, 1.1] P = 0.8363 | 1.2 [1.1, 1.3] P < 0.0001 | 1.2 [1.1, 1.3] P = 0.0038 | 1.0 [0.9, 1.1] P = 0.5995 |
ICU LOS difference | 10.2% [8.7%, 11.8%] P < 0.0001 | 8.4% [3.4%, 13.7%] P = 0.0008 | 24.8% [19.9%, 29.9%] P < 0.0001 | 4.9% [4.0%, 14.7%] P = 0.2893 | 10.1% [1.5%, 19.3%] P = 0.0204 |
ICU cost difference | 8.9% [7.2%, 10.7%] P < 0.0001 | 8.2% [2.7%, 14.0%] P = 0.0029 | 24.1% [18.9%, 29.7%] P < 0.0001 | 2.8% [6.7%, 13.4%] P = 0.5739 | 8.4% [0.6%, 18.1%] P = 0.6680 |
30‐Day readmission odds ratio | 1.2 [1.1, 1.2] P < 0.0001 | 1.0 [0.9, 1.0] P = 0.5141 | 1.1 [1.1, 1.2] P < 0.0001 | 1.0 [1.0, 1.1] P = 0.5211 | 1.0 [1.0, 1.1] P = 0.6119 |
Subgroup Sensitivity Analyses
For patients with CAP (n = 52,582), CHF (n = 46,040), a UTI (n = 28,476), or COPD (n = 17,392), the percent increases in LOS and all cause hospitalization cost of the HN cohort in comparison to the non‐HN cohort were 5.4% (P < 0.0001) and 5.6% (P < 0.0001), 20.6% (P < 0.0001) and 19.2% (P < 0.0001), 2.7% (P = 0.0003) and 2.2% (P = 0.0582), and 6.7% (P < 0.0001) and 5.6% (P < 0.0001), respectively (Table 3).
DISCUSSION
This large‐scale, real‐world hospital database study provides healthcare utilization and cost data on the largest population of HN patients that has currently been studied. The results of this study are consistent with others in showing that HN patients use healthcare services more extensively, and represent a patient population which is more expensive to treat in the inpatient setting.5, 22 Additionally, this study yields new findings in that patients in the real‐world with hyponatremia resulting from various etiologies are more likely to be readmitted to the hospital than patients with similar demographics and characteristics who do not have hyponatremia. The results of the subgroup analysis were generally consistent with the results for the overall matched population, as the incremental burden estimates were directionally consistent. However, among the specific subgroups of patients, although it appears that hyponatremia is predictive of hospital readmission in patients with CHF, it did not necessarily correspond with hospital readmission rates among patients with CAP, UTI, or COPD. Therefore, hyponatremia, at least for patients with these latter conditions, may not be predictive of readmission, but remains associated with increased healthcare utilization during the initial hospitalization. Further evaluation of the incremental burden of hyponatremia among patients with specific disease conditions is needed to validate the findings.
The difference in hospital LOS at first admission between HN and non‐HN patients in this study was 1.1 days, and comparable to that reported by Shorr et al.23 In the study by Shorr et al, patients hospitalized for congestive heart failure (CHF), who were HN, had a 0.5 day increased LOS, and those who were severely HN had a 1.3 day increased LOS.23 Two other retrospective cohort analyses reported a 1.4 day5 and 2.0 day22 increased LOS for HN patients in comparison to non‐HN patients. Zilberberg et al and Callahan et al additionally reported that HN patients had a significantly greater need for ICU (4%10%).5, 22 In the present study, LOS in the ICU and associated costs were also compared among HN and non‐HN cohorts and, after adjustment for key patient characteristics, hyponatremia was associated with an incremental increase of 10.2% for ICU LOS and an 8.9% increase in ICU cost.
In this study, patients with hyponatremia of any severity were found to have a mean increase in hospital cost per admission of $1842, with multivariate analysis demonstrating an associated incremental increase of 8.2%. These results are also comparable to that reported in other retrospective cohort analyses. Shorr et al reported that in‐hospital costs attributed to hyponatremia were $509, and for severe hyponatremia were $1132.23 Callahan et al reported a $1200 increase in costs per admission for patients with mild‐to‐moderate hyponatremia, and a $3540 increase for patients with moderate‐to‐severe hyponatremia, in comparison to non‐HN patients.22
The Institute for Healthcare Improvement reports that hospitalizations account for nearly one‐third of the $882 billion spent on healthcare in the US in 2011, and that a substantial fraction of all hospitalizations are readmissions.13, 24 A recent meta‐analysis of 30‐day hospital readmission rates found 23.1% were classified as avoidable.25 Readmission rates of HN patients in comparison to non‐HN patients have been reported in 3 other studies, all of which were conducted on clinical trial patients with heart failure.7, 8, 26 Two of these studies, which evaluated only patients with acute class IV heart failure,8, 26 reported that hyponatremia was associated with a significant increase in readmission rates (up to 20% higher), and the other, which evaluated any patients hospitalized for heart failure, did not.7 The differences there may be partially attributed to the differences in heart failure severity among patient populations, as hyponatremia is an independent predictor of worsening heart failure. The only published study to date on the influence of hyponatremia on readmission rates in the real‐world was conducted by Scherz et al, who reported that the co‐occurrence of hyponatremia in patients with acute pulmonary embolism, discharged from 185 hospitals in Pennsylvania, was independently associated with an increased readmission rate of 19.3%.27 In the present study, hyponatremia was associated with an incremental increase ranging between 14% and 17% for hospital readmission for any cause. It was conducted on a patient population in which hyponatremia was resultant from many causes, and not all patients had a serious comorbid condition. Also, the HN and non‐HN cohorts were matched for comorbidity prevalence and disease severity, and in other studies they were not. Therefore, the results of this study importantly imply that hyponatremia, whether it is resultant from a serious disease or any other cause substantially increases the healthcare burden. The implementation of strategies to prevent hospital readmissions may play an important role in reducing the healthcare burden of hyponatremia, and future studies are warranted to evaluate this hypothesis alongside evaluation of the outpatient hyponatremia burden.
The limitations of this study include, firstly, that it is only representative of inpatient hospital costs, and excludes outpatient healthcare utilization and costs. Secondly, this study utilized the Premier Hospital Database for patient selection, and laboratory testing data for serum sodium level are not available in this database; therefore, the severity of hyponatremia could not be accurately established in the HN patient population. Thirdly, the occurrence of hyponatremia in patients with some diseases is a marker of disease severity, as is the case with congestive heart failure and cirrhosis.23, 28 Our study did not adjust for the specific disease (eg, CHF) severity, which may influence the results. Future research is needed to evaluate the impact of hyponatremia on underlying disease severity of other diseases, and how its co‐occurrence may influence healthcare resource utilization and cost in each case. Although the Premier Hospital Database contains information from a large number of hospitals across the US, it is possible that it may not be representative of the entire US population of HN patients. Additionally, billing and coding errors and missing data could potentially have occurred, although the large patient population size likely precludes a large impact on the results of this study. Finally, the frequency of use of fluid restriction in these hospital settings could not be chronicled, thus limiting the ability to assess therapies and treatment modalities in use.
Acknowledgements
The authors acknowledge Melissa Lingohr‐Smith from Novosys Health in the editorial support and review of this manuscript.
Disclosures: This research was supported by Otsuka America Pharmaceutical, Inc, Princeton, NJ, which manufactures tolvaptan for the treatment of hyponatremia. Drs Amin and Deitelzweig are consultants for, and have received honoraria from, Otsuka America Pharmaceutical in connection with conducting this study. Drs Christian and Friend are employees of Otsuka America Pharmaceutical. Dr Lin is an employee of Novosys Health, which has received research funds from Otsuka America Pharmaceutical in connection with conducting this study and development of this manuscript. D. Baumer and Dr. Lowe are employees of Premier Inc, which has received research funds from Otsuka America Pharmaceutical. K. Belk was previously employed with Premier Inc.
- Management of hyponatremia: providing treatment and avoiding harm.Cleve Clin J Med.2010;77(10):715–726. , , .
- Causes and management of hyponatremia.Ann Pharmacother.2003;37(11):1694–1702. , , .
- Impact of hospital‐associated hyponatremia on selected outcomes.Arch Intern Med.2010;170(3):294–302. , , , , .
- Hyponatremia: a prospective analysis of its epidemiology and the pathogenetic role of vasopressin.Ann Intern Med.1985;102(2):164–168. , , , .
- Epidemiology, clinical and economic outcomes of admission hyponatremia among hospitalized patients.Curr Med Res Opin.2008;24(6):1601–1608. , , , et al.
- Mortality after hospitalization with mild, moderate, and severe hyponatremia.Am J Med.2009;122(9):857–865. , , .
- Relationship between admission serum sodium concentration and clinical outcomes in patients hospitalized for heart failure: an analysis from the OPTIMIZE‐HF registry.Eur Heart J.2007;28(8):980–988. , , , et al.
- Characterization and prognostic value of persistent hyponatremia in patients with severe heart failure in the ESCAPE Trial.Arch Intern Med.2007;167(18):1998–2005. , , , et al.
- Hyponatremia in cirrhosis: results of a patient population survey.Hepatology.2006;44(6):1535–1542. , , , .
- Hyponatremia in cirrhosis: from pathogenesis to treatment.Hepatology.1998;28(3):851–864. , , , et al.
- The syndrome of inappropriate antidiuresis: pathophysiology, clinical management and new therapeutic options.Nephron Clin Pract.2011;119(1):c62–c73. , , , , .
- Cost of illness of hyponatremia in the United States.Cost Eff Resour Alloc.2006;4:10. , , .
- US Health Care Budget: US Budget Breakdown for FY12—Charts. Available at: http://www.usgovernmentspending.com/health_care_budget_2012_1.html. Accessed December 19, 2011.
- 111th Congress. Patient Protection and Affordable Care Act. Public Law 111–148.1–906.
- An evaluation of statistical matching.JBES.1984;2(1):91–102. .
- Propensity score‐matching methods for nonexperimental causal studies.Rev Econ Stat.2002;84(1):151–161. , .
- Applied Logistic Regression Analysis. Quantitative Applications in the Social Sciences, Vol106.2nd ed.Thousand Oaks, CA:Sage;2002. .
- Reducing bias in a propensity score matched‐pair sample using greedy matching techniques. Paper presented at: Proceedings of the Twenty‐Sixth Annual SAS Users Group International Conference; April 22–25,2001; Long Beach, CA. .
- The effect of clustering of outcomes on the association of procedure volume and surgical outcomes.Ann Intern Med.2003;139(8):658–665. , , , , .
- Regression Modeling Strategies: With Applications to Linear Models, Logistic Regression, and Survival Analysis.New York, NY:Springer‐Verlag,2001. .
- Generalized Modeling Approaches to Risk Adjustment of Skewed Outcomes Data.Cambridge, MA:National Bureau of Economic Research, Inc;2003. , , .
- Economic impact of hyponatremia in hospitalized patients: a retrospective cohort study.Postgrad Med.2009;121(2):186–191. , , , .
- Burden of sodium abnormalities in patients hospitalized for heart failure.Congest Heart Fail.2011;17(1):1–7. , , , et al.
- Institute for Healthcare Improvement. Reduce Avoidable Hospital Readmissions. Available at: http://www.ihi.org/explore/readmissions/Pages/default.aspx. Accessed December 18, 2011.
- A meta‐analysis of hospital 30‐day avoidable readmission rates.J Eval Clin Pract.2011 Nov 9. doi: 10.1111/j.1365–2753.2011.01773.x. Published online August 17, 2012. , , .
- Critical elements of clinical follow‐up after hospital discharge for heart failure: insights from the EVEREST trial.Eur J Heart Fail.2010;12(4):367–374. , , , et al.
- Prognostic importance of hyponatremia in patients with acute pulmonary embolism.Am J Respir Crit Care Med.2010;182(9):1178–1183. , , , et al.
- Serum sodium predicts prognosis in critically ill cirrhotic patients.J Clin Gastroenterol.2010;44(3):220–226. , , , et al.
Hyponatremia is an electrolyte disorder most commonly defined as a serum sodium concentration <135 mEq/L.1 Its exact definition can vary across studies, but typically ranges between <130 and <138 mEq/L.2, 3 Signs and symptoms of hyponatremia can include malaise, headache, disorientation, confusion, muscle weakness, and cramps. If severe, seizures, respiratory arrest, brainstem herniation, coma, and death may result.
The incidence of hyponatremia in the general hospitalized population has been reported to range between 1% and 6% when defined as <130135 mEq/L,4, 5 and its occurrence increases with a more prolonged hospital stay to 13%.6 A recent study reported that when hyponatremia was defined with a less stringent threshold of <138 mEq/L, the incidence at admission rose to 38%.3 Hyponatremia is a comorbid condition of multiple diseases, occurring in approximately 20% of patients with heart failure,7, 8 and 40% to 57% of patients with advanced cirrhosis.9, 10 The syndrome of the inappropriate release of antidiuretic hormone (SIADH) is additionally a predominant cause of hyponatremia, with a prevalence reported as high as 35% in hospitalized patients.11
Hyponatremia is not only widespread, but also an independent predictor of mortality. In a retrospective cohort analysis, Waikar et al reported that in comparison to patients who were normonatremic, patients with serum sodium concentrations <135 mEq/L had a risk of in‐hospital mortality as high as 47%, and that this risk doubled for patients with serum sodium concentrations between 125 and 129 mEq/L.6 In the study by Wald et al, which defined hyponatremia as <138 mEq/L, the risk of in‐hospital mortality was similar.3 In both of these studies, even mild hyponatremia (130137 mEq/L) was associated with increased risk of in‐hospital mortality.3, 6
The overall cost of hyponatremia is estimated to range between $1.6 and $3.6 billion for 2011.12, 13 Hospital readmissions are a significant contributor to total healthcare costs, with some being entirely avoidable with increased standards of care. The Centers for Medicare and Medicaid Services has begun to not only publicly report hospital readmission rates, but also penalize hospitals for early readmissions.14 Strategies to reduce hospital readmissions are currently being integrated into healthcare reform policy.14 In the present study, the incremental burden of hospitalized hyponatremic (HN) versus non‐HN patients in terms of hospital resource utilization, costs, and early hospital readmission in the real‐world was evaluated.
METHODS
Study Design
This study was a retrospective analysis that examined healthcare utilization and costs among HN patients using the Premier Hospital Database. The database contains over 310 million hospital encounters from more than 700 US hospitals, or 1 out of every 4 discharges in the US. The administrative data available included patient and provider demographics, diagnoses and procedures, as well as date‐stamped billing records for all pharmacy, laboratory, imaging, procedures, and supplies.
Patient Selection and Matching
HN patients were eligible for study inclusion if they were a hospital inpatient discharged between January 1, 2007 and March 31, 2010, were >18 years of age at admission, and had either a primary or secondary diagnosis of hyponatremia or hyposmolality (defined as International Classification of Diseases, Ninth Revision (ICD‐9‐CM) code: 276.1x). Patients were excluded if they had been transferred from another acute care facility, transferred to another acute care or critical access facility, or left against medical advice. Labor and delivery patients (ICD‐9‐CM codes: 72.xx‐74.xx, V22.x, V23.x, V27.x, and V28.x), and patients classified as observational were also excluded. A second cohort of non‐hyponatremic patients was created using the same inclusion and exclusion criteria, with the exception that patients not have a primary or secondary diagnosis of hyponatremia or hyposmolality (defined as ICD‐9‐CM code: 276.1x).
The matching of hyponatremia (HN) and control (non‐HN) cohorts was accomplished using a combination of exact and propensity score matching techniques. Patients were first exact matched on age, gender, Medicare Severity‐Diagnosis Related Group (MS‐DRG) assignment, and hospital geographic region. Propensity score matching was further utilized to create the final study cohorts for outcomes comparisons. Propensity score matching is commonly used in retrospective cohort studies to correct for sample selection bias due to observable differences between groups.15, 16 The propensity score was generated using logistic regression with the dependent variable as hyponatremia (yes vs no) and the following covariates: age, race, admission source, attending physician specialty, 3M All Patient Refined‐Diagnosis Related Group (APR‐DRG) Severity of Illness and Risk of Mortality index scores, Deyo‐Charlson Comorbidity Index score, selected hyponatremia‐related comorbidity conditions, and hospital size, region, and urban/rural designation. These covariates were initially selected by an expert panel of physicians, and backward selection was utilized in the logistic regression using the most parsimonious model.17
Following generation of the propensity scores, HN patients were matched to non‐HN patients 1:1 using a nearest neighbor matching algorithm, including hospital identification and propensity score.18 Inclusion of hospital identification in the matching sequence, as well as provider characteristics, especially hospital size, attending physician specialty, and geographic region in the propensity score, was used to control for potential clustering effects at the physician and hospital level.19 During the propensity score matching process, likelihood‐ratio test, Hosmer‐Lemshow goodness of fit, and concordance c statistics were utilized to assess the fitness of the models.20 The final propensity score model produced a concordance c statistic of 0.8.
Outcome Measures and Statistical Analyses
The following outcome measures were compared between the matched HN and non‐HN patient cohorts: total and intensive care unit (ICU) hospitalization costs, total and ICU length of stay (LOS), ICU admission, and 30‐day hospital readmission. Bivariate descriptive statistics were employed to test for significant differences in demographics, patient clinical characteristics, and unadjusted costs and healthcare resource utilization and readmission rates between patient cohorts. To detect statistically significant differences in continuous and categorical variables, respectively, t tests and chi‐square tests were performed.
Multivariate analysis of outcome measures utilized generalized linear models. Due to the skewed nature of LOS and cost data, LOS was analyzed using multivariate negative binomial regression and cost was analyzed using multivariate gamma regression.21 Binary outcomes (ICU admission and 30‐day readmission) were analyzed using multivariate logistic regression. The analysis accounted for potential confounding factors by inclusion of the following covariates: age group, gender, race, admission source, and Deyo‐Charlson Comorbidity Index score. These covariates were previously identified in the Wald et al hyponatremia study,3 and were verified using likelihood‐ratio, Hosmer‐Lemshow goodness of fit, and concordance c statistics.
Subgroup Sensitivity Analysis
For the subgroup sensitivity analyses, patients were identified as having community‐acquired pneumonia (CAP), congestive heart failure (CHF), urinary tract infection (UTI), or chronic obstructive pulmonary disease (COPD) based upon principal diagnosis codes. Patients were categorized according to these subgroup definitions, and then previously matched patients with the same subgroup classification constituted the final analysis set for each subgroup. The methods that were used for the overall matched analysis were then applied to each subgroup to evaluate the incremental burden of overall cost and LOS associated with hyponatremia.
RESULTS
Patient Population
Of the 606,057 HN patients eligible for matching, a total of 558,815 HN patients were matched to 558,815 non‐HN patients, a 92% match ratio. Table 1 describes the overall characteristics of the patient populations. For both cohorts, median age was 70 years, 57% of patients were female, and approximately 67% were white. The majority of patients in either cohort had Medicare coverage (55%), and approximately 75% of patients entered the hospital via the emergency room with nearly 70% having a 3M APR‐DRG disease severity level of major or extreme. Patients of both cohorts were most often attended by an internist or a hospitalist, with a combined percentage of approximately 60%. A small, but greater proportion of HN patients had comorbidities of cancer, pulmonary disease, and SIADH. Comorbid conditions of liver cirrhosis/hepatic disease and human immunodeficiency virus (HIV) were similarly distributed among both patient cohorts.
Hyponatremic | Non‐Hyponatremic | |
---|---|---|
Discharges N (%) | Discharges N (%) | |
| ||
Sample Discharges | 558,815 (100.0%) | 558,815 (100.0%) |
Age median (IQR) | 70.00 (57.081.0) | 70.00 (57.081.0) |
Gender | ||
Female | 319,069 (57.1%) | 319,069 (57.1%) |
Male | 239,746 (42.9%) | 239,746 (42.9%) |
Race | ||
American Indian | 3,465 (0.6%) | 3,448 (0.6%) |
Asian/Pacific | 10,065 (1.8%) | 9,690 (1.7%) |
Black | 63,776 (11.4%) | 66,233 (11.9%) |
Hispanic | 24,341 (4.4%) | 24,426 (4.4%) |
White | 377,434 (67.5%) | 376,639 (67.4%) |
Other/unknown | 79,734 (14.3%) | 78,379 (14.0%) |
Primary payer | ||
Medicaretraditional | 310,312 (55.5%) | 310,643 (55.6%) |
Managed care | 75,476 (13.5%) | 78,184 (14.0%) |
Medicaremanaged care | 45,439 (8.1%) | 47,947 (8.6%) |
Non‐cap | ||
Medicaid | 44,690 (8.0%) | 43,767 (7.8%) |
Other | 82,898 (14.8%) | 78,274 (14.0%) |
Admission source | ||
Physician referral | 5,022 (0.9%) | 4,636 (0.8%) |
Transfer from another nonacute health facility | 18,031 (3.2%) | 18,163 (3.3%) |
Emergency room | 417,556 (74.7%) | 420,401 (75.2%) |
Other/unknown | 118,206 (21.2%) | 115,615 (20.7%) |
APR‐DRG severity of illness | ||
1Minor | 14,257 (2.6%) | 12,993 (2.3%) |
2Moderate | 174,859 (31.3%) | 179,356 (32.1%) |
3Major | 263,814 (47.2%) | 265,422 (47.5%) |
4Extreme | 105,885 (19.0%) | 101,044 (18.1%) |
Attending physician specialty | ||
Internal Medicine | 235,628 (42.1%) | 240,875 (43.1%) |
Hospitalist | 88,250 (15.8%) | 87,720 (15.7%) |
Family Practice (FP) | 73,346 (13.1%) | 72,828 (13.0%) |
Orthopedic Surgery (ORS) | 23,595 (4.2%) | 22,949 (4.1%) |
Cardiovascular Diseases (CD) | 19,521 (3.5%) | 18,057 (3.2%) |
Comorbidities | ||
Human immunodeficiency virus | 3,971 (0.7%) | 4,018 (0.7%) |
Cancer/neoplasm/malignancy | 107,851 (19.3%) | 105,199 (18.8%) |
Pulmonary disease | 77,849 (13.9%) | 77,184 (13.8%) |
Cirrhosis/hepatic disease | 23,038 (4.1%) | 23,418 (4.2%) |
SIADH | 1,972 (0.4%) | 1,278 (0.2%) |
Subgroup populations | ||
Community‐acquired pneumonia | 26,291 (4.7%) | 26,291 (4.7%) |
Congestive heart failure | 23,020 (4.1%) | 23,020 (4.1%) |
Urinary tract infection | 14,238 (2.6%) | 14,238 (2.6%) |
COPD | 8,696 (1.6%) | 8,696 (1.6%) |
CCI median (IQR) | 3.0 (1.05.0) | 3.0 (1.05.0) |
No. of premier hospitals | 459 | |
Provider region | ||
East North Central | 78,332 (14.0%) | 78,332 (14.0%) |
East South Central | 32,122 (5.8%) | 32,122 (5.8%) |
Middle Atlantic | 73,846 (13.2%) | 73,846 (13.2%) |
Mountain | 23,761 (4.3%) | 23,761 (4.3%) |
New England | 9,493 (1.7%) | 9,493 (1.7%) |
Pacific | 73,059 (13.1%) | 73,059 (13.1%) |
South Atlantic | 175,194 (31.4%) | 175,194 (31.4%) |
West North Central | 37,913 (6.8%) | 37,913 (6.8%) |
West South Central | 55,095 (9.9%) | 55,095 (9.9%) |
Population served | ||
Rural | 69,749 (12.5%) | 68,414 (12.2%) |
Urban | 489,066 (87.5%) | 490,401 (87.8%) |
Teaching status | ||
Non‐teaching | 337,620 (60.4%) | 337,513 (60.4%) |
Teaching | 221,195 (39.6%) | 221,302 (39.6%) |
No. of hospital beds | ||
699 | 22,067 (4.0%) | 21,777 (3.9%) |
100199 | 57,367 (10.3%) | 56,097 (10.0%) |
200299 | 87,563 (15.7%) | 86,639 (15.5%) |
300499 | 218,834 (39.2%) | 220,248 (39.4%) |
500+ | 172,984 (31.0%) | 174,054 (31.2%) |
Hospital Characteristics
Patient cohorts had similar distributions with respect to hospital characteristics (Table 1). Approximately 30% of patients were provided care from hospitals located in the South Atlantic region, and between 10% and 15% were serviced from hospitals in the East North Central, Middle Atlantic, Pacific, and West South Central regions. Most hospitals providing care for patient cohorts served urban populations (88%) and were large, with hospital bed numbers 300. Approximately 60% of hospitals were non‐teaching hospitals.
Healthcare Utilization, Readmission, and Cost Differences Among Patient Cohorts
The mean LOS (8.8 10.3 vs 7.7 8.5, P < 0.001), a difference of 1.1 days and mean ICU LOS (5.5 7.9 vs 4.9 7.1 days, P < 0.001), a difference of 0.6 days were significantly greater for the HN cohort in comparison to the non‐HN cohort (Table 2). The increase in healthcare resource utilization of patients with HN was reflected in their significantly higher mean total hospital costs per admission ($15,281 $24,054 vs $13,439 $22,198, P < 0.001), a difference of $1842; and mean costs incurred in the ICU ($8525 $13,342 vs $7597 $12,695, P < 0.001), a difference of $928 (Table 2). Furthermore, patients in the HN cohort were significantly more likely to be readmitted to the hospital for any cause (17.5% vs 16.4%, P < 0.001) (Table 2).
Hyponatremic | Non‐Hyponatremic | P Value | |
---|---|---|---|
| |||
Total LOS (mean SD) | 8.8 10.3 | 7.7 8.5 | <0.001 |
Total hospitalization cost (mean SD) | $15,281 $24,054 | $13,439 $22,198 | <0.001 |
ICU admission (N, %) | 129,235 (23.1%) | 123,502 (22.1%) | <0.001 |
ICU LOS (mean SD) | 5.5 7.9 | 4.9 7.1 | <0.001 |
ICU cost (mean SD) | $8,525 $13,342 | $7,597 $12,695 | <0.001 |
30‐Day all cause readmission (N, %) | 96,063 (17.5%) | 87,058 (16.4%) | <0.001 |
Multivariate Analysis
Multivariate analysis demonstrated hyponatremia was associated with an increase in mean hospital LOS of 10.9%, [95% confidence interval: 10.4%11.5%], (P < 0.0001) and an increase in mean total hospital costs of 8.2%, [7.4%9.0%], (P < 0.0001) (Table 3). Additionally, hyponatremia was associated with an increase in ICU LOS of 10.2%, [8.7%11.8%], (P < 0.0001), and a higher ICU cost of 8.9%, [7.2%10.7%], (P < 0.0001) (Table 3). Hyponatremia was not associated with a greater likelihood of ICU admission (odds ratio = 1.0; [1.01.0], P =.5760). However, the condition was associated with a significantly greater chance of hospital readmission (odds ratio = 1.2, [1.11.2], P < 0.0001) within 30 days postdischarge (Table 3).
Overall Cohort N = 1,117,630 | CAP N = 52,582 | CHF N = 46,040 | UTI N = 28,746 | COPD N = 17,392 | |
---|---|---|---|---|---|
| |||||
Total LOS difference | 10.9% [10.4%, 11.5%] P < 0.0001 | 5.4% [4.4%, 6.5%] P < 0.0001 | 20.6% [19.0%, 22.2%] P < 0.0001 | 2.7% [1.2%, 4.2%] P = 0.0003 | 6.7% [4.8%, 8.5%] P < 0.0001 |
Total cost difference | 8.2% [7.4%, 9.0%] P < 0.0001 | 5.6% [4.0%, 7.0%] P < 0.0001 | 19.2% [17.1%, 21.4%] P < 0.0001 | 2.2% [1.4%, 4.4%] P = 0.0582 | 5.6% [3.0%, 8.2%] P < 0.0001 |
ICU admission* odds ratio | 1.0 [1.0, 1.0] P = 0.5760 | 1.0 [1.0, 1.1] P = 0.8363 | 1.2 [1.1, 1.3] P < 0.0001 | 1.2 [1.1, 1.3] P = 0.0038 | 1.0 [0.9, 1.1] P = 0.5995 |
ICU LOS difference | 10.2% [8.7%, 11.8%] P < 0.0001 | 8.4% [3.4%, 13.7%] P = 0.0008 | 24.8% [19.9%, 29.9%] P < 0.0001 | 4.9% [4.0%, 14.7%] P = 0.2893 | 10.1% [1.5%, 19.3%] P = 0.0204 |
ICU cost difference | 8.9% [7.2%, 10.7%] P < 0.0001 | 8.2% [2.7%, 14.0%] P = 0.0029 | 24.1% [18.9%, 29.7%] P < 0.0001 | 2.8% [6.7%, 13.4%] P = 0.5739 | 8.4% [0.6%, 18.1%] P = 0.6680 |
30‐Day readmission odds ratio | 1.2 [1.1, 1.2] P < 0.0001 | 1.0 [0.9, 1.0] P = 0.5141 | 1.1 [1.1, 1.2] P < 0.0001 | 1.0 [1.0, 1.1] P = 0.5211 | 1.0 [1.0, 1.1] P = 0.6119 |
Subgroup Sensitivity Analyses
For patients with CAP (n = 52,582), CHF (n = 46,040), a UTI (n = 28,476), or COPD (n = 17,392), the percent increases in LOS and all cause hospitalization cost of the HN cohort in comparison to the non‐HN cohort were 5.4% (P < 0.0001) and 5.6% (P < 0.0001), 20.6% (P < 0.0001) and 19.2% (P < 0.0001), 2.7% (P = 0.0003) and 2.2% (P = 0.0582), and 6.7% (P < 0.0001) and 5.6% (P < 0.0001), respectively (Table 3).
DISCUSSION
This large‐scale, real‐world hospital database study provides healthcare utilization and cost data on the largest population of HN patients that has currently been studied. The results of this study are consistent with others in showing that HN patients use healthcare services more extensively, and represent a patient population which is more expensive to treat in the inpatient setting.5, 22 Additionally, this study yields new findings in that patients in the real‐world with hyponatremia resulting from various etiologies are more likely to be readmitted to the hospital than patients with similar demographics and characteristics who do not have hyponatremia. The results of the subgroup analysis were generally consistent with the results for the overall matched population, as the incremental burden estimates were directionally consistent. However, among the specific subgroups of patients, although it appears that hyponatremia is predictive of hospital readmission in patients with CHF, it did not necessarily correspond with hospital readmission rates among patients with CAP, UTI, or COPD. Therefore, hyponatremia, at least for patients with these latter conditions, may not be predictive of readmission, but remains associated with increased healthcare utilization during the initial hospitalization. Further evaluation of the incremental burden of hyponatremia among patients with specific disease conditions is needed to validate the findings.
The difference in hospital LOS at first admission between HN and non‐HN patients in this study was 1.1 days, and comparable to that reported by Shorr et al.23 In the study by Shorr et al, patients hospitalized for congestive heart failure (CHF), who were HN, had a 0.5 day increased LOS, and those who were severely HN had a 1.3 day increased LOS.23 Two other retrospective cohort analyses reported a 1.4 day5 and 2.0 day22 increased LOS for HN patients in comparison to non‐HN patients. Zilberberg et al and Callahan et al additionally reported that HN patients had a significantly greater need for ICU (4%10%).5, 22 In the present study, LOS in the ICU and associated costs were also compared among HN and non‐HN cohorts and, after adjustment for key patient characteristics, hyponatremia was associated with an incremental increase of 10.2% for ICU LOS and an 8.9% increase in ICU cost.
In this study, patients with hyponatremia of any severity were found to have a mean increase in hospital cost per admission of $1842, with multivariate analysis demonstrating an associated incremental increase of 8.2%. These results are also comparable to that reported in other retrospective cohort analyses. Shorr et al reported that in‐hospital costs attributed to hyponatremia were $509, and for severe hyponatremia were $1132.23 Callahan et al reported a $1200 increase in costs per admission for patients with mild‐to‐moderate hyponatremia, and a $3540 increase for patients with moderate‐to‐severe hyponatremia, in comparison to non‐HN patients.22
The Institute for Healthcare Improvement reports that hospitalizations account for nearly one‐third of the $882 billion spent on healthcare in the US in 2011, and that a substantial fraction of all hospitalizations are readmissions.13, 24 A recent meta‐analysis of 30‐day hospital readmission rates found 23.1% were classified as avoidable.25 Readmission rates of HN patients in comparison to non‐HN patients have been reported in 3 other studies, all of which were conducted on clinical trial patients with heart failure.7, 8, 26 Two of these studies, which evaluated only patients with acute class IV heart failure,8, 26 reported that hyponatremia was associated with a significant increase in readmission rates (up to 20% higher), and the other, which evaluated any patients hospitalized for heart failure, did not.7 The differences there may be partially attributed to the differences in heart failure severity among patient populations, as hyponatremia is an independent predictor of worsening heart failure. The only published study to date on the influence of hyponatremia on readmission rates in the real‐world was conducted by Scherz et al, who reported that the co‐occurrence of hyponatremia in patients with acute pulmonary embolism, discharged from 185 hospitals in Pennsylvania, was independently associated with an increased readmission rate of 19.3%.27 In the present study, hyponatremia was associated with an incremental increase ranging between 14% and 17% for hospital readmission for any cause. It was conducted on a patient population in which hyponatremia was resultant from many causes, and not all patients had a serious comorbid condition. Also, the HN and non‐HN cohorts were matched for comorbidity prevalence and disease severity, and in other studies they were not. Therefore, the results of this study importantly imply that hyponatremia, whether it is resultant from a serious disease or any other cause substantially increases the healthcare burden. The implementation of strategies to prevent hospital readmissions may play an important role in reducing the healthcare burden of hyponatremia, and future studies are warranted to evaluate this hypothesis alongside evaluation of the outpatient hyponatremia burden.
The limitations of this study include, firstly, that it is only representative of inpatient hospital costs, and excludes outpatient healthcare utilization and costs. Secondly, this study utilized the Premier Hospital Database for patient selection, and laboratory testing data for serum sodium level are not available in this database; therefore, the severity of hyponatremia could not be accurately established in the HN patient population. Thirdly, the occurrence of hyponatremia in patients with some diseases is a marker of disease severity, as is the case with congestive heart failure and cirrhosis.23, 28 Our study did not adjust for the specific disease (eg, CHF) severity, which may influence the results. Future research is needed to evaluate the impact of hyponatremia on underlying disease severity of other diseases, and how its co‐occurrence may influence healthcare resource utilization and cost in each case. Although the Premier Hospital Database contains information from a large number of hospitals across the US, it is possible that it may not be representative of the entire US population of HN patients. Additionally, billing and coding errors and missing data could potentially have occurred, although the large patient population size likely precludes a large impact on the results of this study. Finally, the frequency of use of fluid restriction in these hospital settings could not be chronicled, thus limiting the ability to assess therapies and treatment modalities in use.
Acknowledgements
The authors acknowledge Melissa Lingohr‐Smith from Novosys Health in the editorial support and review of this manuscript.
Disclosures: This research was supported by Otsuka America Pharmaceutical, Inc, Princeton, NJ, which manufactures tolvaptan for the treatment of hyponatremia. Drs Amin and Deitelzweig are consultants for, and have received honoraria from, Otsuka America Pharmaceutical in connection with conducting this study. Drs Christian and Friend are employees of Otsuka America Pharmaceutical. Dr Lin is an employee of Novosys Health, which has received research funds from Otsuka America Pharmaceutical in connection with conducting this study and development of this manuscript. D. Baumer and Dr. Lowe are employees of Premier Inc, which has received research funds from Otsuka America Pharmaceutical. K. Belk was previously employed with Premier Inc.
Hyponatremia is an electrolyte disorder most commonly defined as a serum sodium concentration <135 mEq/L.1 Its exact definition can vary across studies, but typically ranges between <130 and <138 mEq/L.2, 3 Signs and symptoms of hyponatremia can include malaise, headache, disorientation, confusion, muscle weakness, and cramps. If severe, seizures, respiratory arrest, brainstem herniation, coma, and death may result.
The incidence of hyponatremia in the general hospitalized population has been reported to range between 1% and 6% when defined as <130135 mEq/L,4, 5 and its occurrence increases with a more prolonged hospital stay to 13%.6 A recent study reported that when hyponatremia was defined with a less stringent threshold of <138 mEq/L, the incidence at admission rose to 38%.3 Hyponatremia is a comorbid condition of multiple diseases, occurring in approximately 20% of patients with heart failure,7, 8 and 40% to 57% of patients with advanced cirrhosis.9, 10 The syndrome of the inappropriate release of antidiuretic hormone (SIADH) is additionally a predominant cause of hyponatremia, with a prevalence reported as high as 35% in hospitalized patients.11
Hyponatremia is not only widespread, but also an independent predictor of mortality. In a retrospective cohort analysis, Waikar et al reported that in comparison to patients who were normonatremic, patients with serum sodium concentrations <135 mEq/L had a risk of in‐hospital mortality as high as 47%, and that this risk doubled for patients with serum sodium concentrations between 125 and 129 mEq/L.6 In the study by Wald et al, which defined hyponatremia as <138 mEq/L, the risk of in‐hospital mortality was similar.3 In both of these studies, even mild hyponatremia (130137 mEq/L) was associated with increased risk of in‐hospital mortality.3, 6
The overall cost of hyponatremia is estimated to range between $1.6 and $3.6 billion for 2011.12, 13 Hospital readmissions are a significant contributor to total healthcare costs, with some being entirely avoidable with increased standards of care. The Centers for Medicare and Medicaid Services has begun to not only publicly report hospital readmission rates, but also penalize hospitals for early readmissions.14 Strategies to reduce hospital readmissions are currently being integrated into healthcare reform policy.14 In the present study, the incremental burden of hospitalized hyponatremic (HN) versus non‐HN patients in terms of hospital resource utilization, costs, and early hospital readmission in the real‐world was evaluated.
METHODS
Study Design
This study was a retrospective analysis that examined healthcare utilization and costs among HN patients using the Premier Hospital Database. The database contains over 310 million hospital encounters from more than 700 US hospitals, or 1 out of every 4 discharges in the US. The administrative data available included patient and provider demographics, diagnoses and procedures, as well as date‐stamped billing records for all pharmacy, laboratory, imaging, procedures, and supplies.
Patient Selection and Matching
HN patients were eligible for study inclusion if they were a hospital inpatient discharged between January 1, 2007 and March 31, 2010, were >18 years of age at admission, and had either a primary or secondary diagnosis of hyponatremia or hyposmolality (defined as International Classification of Diseases, Ninth Revision (ICD‐9‐CM) code: 276.1x). Patients were excluded if they had been transferred from another acute care facility, transferred to another acute care or critical access facility, or left against medical advice. Labor and delivery patients (ICD‐9‐CM codes: 72.xx‐74.xx, V22.x, V23.x, V27.x, and V28.x), and patients classified as observational were also excluded. A second cohort of non‐hyponatremic patients was created using the same inclusion and exclusion criteria, with the exception that patients not have a primary or secondary diagnosis of hyponatremia or hyposmolality (defined as ICD‐9‐CM code: 276.1x).
The matching of hyponatremia (HN) and control (non‐HN) cohorts was accomplished using a combination of exact and propensity score matching techniques. Patients were first exact matched on age, gender, Medicare Severity‐Diagnosis Related Group (MS‐DRG) assignment, and hospital geographic region. Propensity score matching was further utilized to create the final study cohorts for outcomes comparisons. Propensity score matching is commonly used in retrospective cohort studies to correct for sample selection bias due to observable differences between groups.15, 16 The propensity score was generated using logistic regression with the dependent variable as hyponatremia (yes vs no) and the following covariates: age, race, admission source, attending physician specialty, 3M All Patient Refined‐Diagnosis Related Group (APR‐DRG) Severity of Illness and Risk of Mortality index scores, Deyo‐Charlson Comorbidity Index score, selected hyponatremia‐related comorbidity conditions, and hospital size, region, and urban/rural designation. These covariates were initially selected by an expert panel of physicians, and backward selection was utilized in the logistic regression using the most parsimonious model.17
Following generation of the propensity scores, HN patients were matched to non‐HN patients 1:1 using a nearest neighbor matching algorithm, including hospital identification and propensity score.18 Inclusion of hospital identification in the matching sequence, as well as provider characteristics, especially hospital size, attending physician specialty, and geographic region in the propensity score, was used to control for potential clustering effects at the physician and hospital level.19 During the propensity score matching process, likelihood‐ratio test, Hosmer‐Lemshow goodness of fit, and concordance c statistics were utilized to assess the fitness of the models.20 The final propensity score model produced a concordance c statistic of 0.8.
Outcome Measures and Statistical Analyses
The following outcome measures were compared between the matched HN and non‐HN patient cohorts: total and intensive care unit (ICU) hospitalization costs, total and ICU length of stay (LOS), ICU admission, and 30‐day hospital readmission. Bivariate descriptive statistics were employed to test for significant differences in demographics, patient clinical characteristics, and unadjusted costs and healthcare resource utilization and readmission rates between patient cohorts. To detect statistically significant differences in continuous and categorical variables, respectively, t tests and chi‐square tests were performed.
Multivariate analysis of outcome measures utilized generalized linear models. Due to the skewed nature of LOS and cost data, LOS was analyzed using multivariate negative binomial regression and cost was analyzed using multivariate gamma regression.21 Binary outcomes (ICU admission and 30‐day readmission) were analyzed using multivariate logistic regression. The analysis accounted for potential confounding factors by inclusion of the following covariates: age group, gender, race, admission source, and Deyo‐Charlson Comorbidity Index score. These covariates were previously identified in the Wald et al hyponatremia study,3 and were verified using likelihood‐ratio, Hosmer‐Lemshow goodness of fit, and concordance c statistics.
Subgroup Sensitivity Analysis
For the subgroup sensitivity analyses, patients were identified as having community‐acquired pneumonia (CAP), congestive heart failure (CHF), urinary tract infection (UTI), or chronic obstructive pulmonary disease (COPD) based upon principal diagnosis codes. Patients were categorized according to these subgroup definitions, and then previously matched patients with the same subgroup classification constituted the final analysis set for each subgroup. The methods that were used for the overall matched analysis were then applied to each subgroup to evaluate the incremental burden of overall cost and LOS associated with hyponatremia.
RESULTS
Patient Population
Of the 606,057 HN patients eligible for matching, a total of 558,815 HN patients were matched to 558,815 non‐HN patients, a 92% match ratio. Table 1 describes the overall characteristics of the patient populations. For both cohorts, median age was 70 years, 57% of patients were female, and approximately 67% were white. The majority of patients in either cohort had Medicare coverage (55%), and approximately 75% of patients entered the hospital via the emergency room with nearly 70% having a 3M APR‐DRG disease severity level of major or extreme. Patients of both cohorts were most often attended by an internist or a hospitalist, with a combined percentage of approximately 60%. A small, but greater proportion of HN patients had comorbidities of cancer, pulmonary disease, and SIADH. Comorbid conditions of liver cirrhosis/hepatic disease and human immunodeficiency virus (HIV) were similarly distributed among both patient cohorts.
Hyponatremic | Non‐Hyponatremic | |
---|---|---|
Discharges N (%) | Discharges N (%) | |
| ||
Sample Discharges | 558,815 (100.0%) | 558,815 (100.0%) |
Age median (IQR) | 70.00 (57.081.0) | 70.00 (57.081.0) |
Gender | ||
Female | 319,069 (57.1%) | 319,069 (57.1%) |
Male | 239,746 (42.9%) | 239,746 (42.9%) |
Race | ||
American Indian | 3,465 (0.6%) | 3,448 (0.6%) |
Asian/Pacific | 10,065 (1.8%) | 9,690 (1.7%) |
Black | 63,776 (11.4%) | 66,233 (11.9%) |
Hispanic | 24,341 (4.4%) | 24,426 (4.4%) |
White | 377,434 (67.5%) | 376,639 (67.4%) |
Other/unknown | 79,734 (14.3%) | 78,379 (14.0%) |
Primary payer | ||
Medicaretraditional | 310,312 (55.5%) | 310,643 (55.6%) |
Managed care | 75,476 (13.5%) | 78,184 (14.0%) |
Medicaremanaged care | 45,439 (8.1%) | 47,947 (8.6%) |
Non‐cap | ||
Medicaid | 44,690 (8.0%) | 43,767 (7.8%) |
Other | 82,898 (14.8%) | 78,274 (14.0%) |
Admission source | ||
Physician referral | 5,022 (0.9%) | 4,636 (0.8%) |
Transfer from another nonacute health facility | 18,031 (3.2%) | 18,163 (3.3%) |
Emergency room | 417,556 (74.7%) | 420,401 (75.2%) |
Other/unknown | 118,206 (21.2%) | 115,615 (20.7%) |
APR‐DRG severity of illness | ||
1Minor | 14,257 (2.6%) | 12,993 (2.3%) |
2Moderate | 174,859 (31.3%) | 179,356 (32.1%) |
3Major | 263,814 (47.2%) | 265,422 (47.5%) |
4Extreme | 105,885 (19.0%) | 101,044 (18.1%) |
Attending physician specialty | ||
Internal Medicine | 235,628 (42.1%) | 240,875 (43.1%) |
Hospitalist | 88,250 (15.8%) | 87,720 (15.7%) |
Family Practice (FP) | 73,346 (13.1%) | 72,828 (13.0%) |
Orthopedic Surgery (ORS) | 23,595 (4.2%) | 22,949 (4.1%) |
Cardiovascular Diseases (CD) | 19,521 (3.5%) | 18,057 (3.2%) |
Comorbidities | ||
Human immunodeficiency virus | 3,971 (0.7%) | 4,018 (0.7%) |
Cancer/neoplasm/malignancy | 107,851 (19.3%) | 105,199 (18.8%) |
Pulmonary disease | 77,849 (13.9%) | 77,184 (13.8%) |
Cirrhosis/hepatic disease | 23,038 (4.1%) | 23,418 (4.2%) |
SIADH | 1,972 (0.4%) | 1,278 (0.2%) |
Subgroup populations | ||
Community‐acquired pneumonia | 26,291 (4.7%) | 26,291 (4.7%) |
Congestive heart failure | 23,020 (4.1%) | 23,020 (4.1%) |
Urinary tract infection | 14,238 (2.6%) | 14,238 (2.6%) |
COPD | 8,696 (1.6%) | 8,696 (1.6%) |
CCI median (IQR) | 3.0 (1.05.0) | 3.0 (1.05.0) |
No. of premier hospitals | 459 | |
Provider region | ||
East North Central | 78,332 (14.0%) | 78,332 (14.0%) |
East South Central | 32,122 (5.8%) | 32,122 (5.8%) |
Middle Atlantic | 73,846 (13.2%) | 73,846 (13.2%) |
Mountain | 23,761 (4.3%) | 23,761 (4.3%) |
New England | 9,493 (1.7%) | 9,493 (1.7%) |
Pacific | 73,059 (13.1%) | 73,059 (13.1%) |
South Atlantic | 175,194 (31.4%) | 175,194 (31.4%) |
West North Central | 37,913 (6.8%) | 37,913 (6.8%) |
West South Central | 55,095 (9.9%) | 55,095 (9.9%) |
Population served | ||
Rural | 69,749 (12.5%) | 68,414 (12.2%) |
Urban | 489,066 (87.5%) | 490,401 (87.8%) |
Teaching status | ||
Non‐teaching | 337,620 (60.4%) | 337,513 (60.4%) |
Teaching | 221,195 (39.6%) | 221,302 (39.6%) |
No. of hospital beds | ||
699 | 22,067 (4.0%) | 21,777 (3.9%) |
100199 | 57,367 (10.3%) | 56,097 (10.0%) |
200299 | 87,563 (15.7%) | 86,639 (15.5%) |
300499 | 218,834 (39.2%) | 220,248 (39.4%) |
500+ | 172,984 (31.0%) | 174,054 (31.2%) |
Hospital Characteristics
Patient cohorts had similar distributions with respect to hospital characteristics (Table 1). Approximately 30% of patients were provided care from hospitals located in the South Atlantic region, and between 10% and 15% were serviced from hospitals in the East North Central, Middle Atlantic, Pacific, and West South Central regions. Most hospitals providing care for patient cohorts served urban populations (88%) and were large, with hospital bed numbers 300. Approximately 60% of hospitals were non‐teaching hospitals.
Healthcare Utilization, Readmission, and Cost Differences Among Patient Cohorts
The mean LOS (8.8 10.3 vs 7.7 8.5, P < 0.001), a difference of 1.1 days and mean ICU LOS (5.5 7.9 vs 4.9 7.1 days, P < 0.001), a difference of 0.6 days were significantly greater for the HN cohort in comparison to the non‐HN cohort (Table 2). The increase in healthcare resource utilization of patients with HN was reflected in their significantly higher mean total hospital costs per admission ($15,281 $24,054 vs $13,439 $22,198, P < 0.001), a difference of $1842; and mean costs incurred in the ICU ($8525 $13,342 vs $7597 $12,695, P < 0.001), a difference of $928 (Table 2). Furthermore, patients in the HN cohort were significantly more likely to be readmitted to the hospital for any cause (17.5% vs 16.4%, P < 0.001) (Table 2).
Hyponatremic | Non‐Hyponatremic | P Value | |
---|---|---|---|
| |||
Total LOS (mean SD) | 8.8 10.3 | 7.7 8.5 | <0.001 |
Total hospitalization cost (mean SD) | $15,281 $24,054 | $13,439 $22,198 | <0.001 |
ICU admission (N, %) | 129,235 (23.1%) | 123,502 (22.1%) | <0.001 |
ICU LOS (mean SD) | 5.5 7.9 | 4.9 7.1 | <0.001 |
ICU cost (mean SD) | $8,525 $13,342 | $7,597 $12,695 | <0.001 |
30‐Day all cause readmission (N, %) | 96,063 (17.5%) | 87,058 (16.4%) | <0.001 |
Multivariate Analysis
Multivariate analysis demonstrated hyponatremia was associated with an increase in mean hospital LOS of 10.9%, [95% confidence interval: 10.4%11.5%], (P < 0.0001) and an increase in mean total hospital costs of 8.2%, [7.4%9.0%], (P < 0.0001) (Table 3). Additionally, hyponatremia was associated with an increase in ICU LOS of 10.2%, [8.7%11.8%], (P < 0.0001), and a higher ICU cost of 8.9%, [7.2%10.7%], (P < 0.0001) (Table 3). Hyponatremia was not associated with a greater likelihood of ICU admission (odds ratio = 1.0; [1.01.0], P =.5760). However, the condition was associated with a significantly greater chance of hospital readmission (odds ratio = 1.2, [1.11.2], P < 0.0001) within 30 days postdischarge (Table 3).
Overall Cohort N = 1,117,630 | CAP N = 52,582 | CHF N = 46,040 | UTI N = 28,746 | COPD N = 17,392 | |
---|---|---|---|---|---|
| |||||
Total LOS difference | 10.9% [10.4%, 11.5%] P < 0.0001 | 5.4% [4.4%, 6.5%] P < 0.0001 | 20.6% [19.0%, 22.2%] P < 0.0001 | 2.7% [1.2%, 4.2%] P = 0.0003 | 6.7% [4.8%, 8.5%] P < 0.0001 |
Total cost difference | 8.2% [7.4%, 9.0%] P < 0.0001 | 5.6% [4.0%, 7.0%] P < 0.0001 | 19.2% [17.1%, 21.4%] P < 0.0001 | 2.2% [1.4%, 4.4%] P = 0.0582 | 5.6% [3.0%, 8.2%] P < 0.0001 |
ICU admission* odds ratio | 1.0 [1.0, 1.0] P = 0.5760 | 1.0 [1.0, 1.1] P = 0.8363 | 1.2 [1.1, 1.3] P < 0.0001 | 1.2 [1.1, 1.3] P = 0.0038 | 1.0 [0.9, 1.1] P = 0.5995 |
ICU LOS difference | 10.2% [8.7%, 11.8%] P < 0.0001 | 8.4% [3.4%, 13.7%] P = 0.0008 | 24.8% [19.9%, 29.9%] P < 0.0001 | 4.9% [4.0%, 14.7%] P = 0.2893 | 10.1% [1.5%, 19.3%] P = 0.0204 |
ICU cost difference | 8.9% [7.2%, 10.7%] P < 0.0001 | 8.2% [2.7%, 14.0%] P = 0.0029 | 24.1% [18.9%, 29.7%] P < 0.0001 | 2.8% [6.7%, 13.4%] P = 0.5739 | 8.4% [0.6%, 18.1%] P = 0.6680 |
30‐Day readmission odds ratio | 1.2 [1.1, 1.2] P < 0.0001 | 1.0 [0.9, 1.0] P = 0.5141 | 1.1 [1.1, 1.2] P < 0.0001 | 1.0 [1.0, 1.1] P = 0.5211 | 1.0 [1.0, 1.1] P = 0.6119 |
Subgroup Sensitivity Analyses
For patients with CAP (n = 52,582), CHF (n = 46,040), a UTI (n = 28,476), or COPD (n = 17,392), the percent increases in LOS and all cause hospitalization cost of the HN cohort in comparison to the non‐HN cohort were 5.4% (P < 0.0001) and 5.6% (P < 0.0001), 20.6% (P < 0.0001) and 19.2% (P < 0.0001), 2.7% (P = 0.0003) and 2.2% (P = 0.0582), and 6.7% (P < 0.0001) and 5.6% (P < 0.0001), respectively (Table 3).
DISCUSSION
This large‐scale, real‐world hospital database study provides healthcare utilization and cost data on the largest population of HN patients that has currently been studied. The results of this study are consistent with others in showing that HN patients use healthcare services more extensively, and represent a patient population which is more expensive to treat in the inpatient setting.5, 22 Additionally, this study yields new findings in that patients in the real‐world with hyponatremia resulting from various etiologies are more likely to be readmitted to the hospital than patients with similar demographics and characteristics who do not have hyponatremia. The results of the subgroup analysis were generally consistent with the results for the overall matched population, as the incremental burden estimates were directionally consistent. However, among the specific subgroups of patients, although it appears that hyponatremia is predictive of hospital readmission in patients with CHF, it did not necessarily correspond with hospital readmission rates among patients with CAP, UTI, or COPD. Therefore, hyponatremia, at least for patients with these latter conditions, may not be predictive of readmission, but remains associated with increased healthcare utilization during the initial hospitalization. Further evaluation of the incremental burden of hyponatremia among patients with specific disease conditions is needed to validate the findings.
The difference in hospital LOS at first admission between HN and non‐HN patients in this study was 1.1 days, and comparable to that reported by Shorr et al.23 In the study by Shorr et al, patients hospitalized for congestive heart failure (CHF), who were HN, had a 0.5 day increased LOS, and those who were severely HN had a 1.3 day increased LOS.23 Two other retrospective cohort analyses reported a 1.4 day5 and 2.0 day22 increased LOS for HN patients in comparison to non‐HN patients. Zilberberg et al and Callahan et al additionally reported that HN patients had a significantly greater need for ICU (4%10%).5, 22 In the present study, LOS in the ICU and associated costs were also compared among HN and non‐HN cohorts and, after adjustment for key patient characteristics, hyponatremia was associated with an incremental increase of 10.2% for ICU LOS and an 8.9% increase in ICU cost.
In this study, patients with hyponatremia of any severity were found to have a mean increase in hospital cost per admission of $1842, with multivariate analysis demonstrating an associated incremental increase of 8.2%. These results are also comparable to that reported in other retrospective cohort analyses. Shorr et al reported that in‐hospital costs attributed to hyponatremia were $509, and for severe hyponatremia were $1132.23 Callahan et al reported a $1200 increase in costs per admission for patients with mild‐to‐moderate hyponatremia, and a $3540 increase for patients with moderate‐to‐severe hyponatremia, in comparison to non‐HN patients.22
The Institute for Healthcare Improvement reports that hospitalizations account for nearly one‐third of the $882 billion spent on healthcare in the US in 2011, and that a substantial fraction of all hospitalizations are readmissions.13, 24 A recent meta‐analysis of 30‐day hospital readmission rates found 23.1% were classified as avoidable.25 Readmission rates of HN patients in comparison to non‐HN patients have been reported in 3 other studies, all of which were conducted on clinical trial patients with heart failure.7, 8, 26 Two of these studies, which evaluated only patients with acute class IV heart failure,8, 26 reported that hyponatremia was associated with a significant increase in readmission rates (up to 20% higher), and the other, which evaluated any patients hospitalized for heart failure, did not.7 The differences there may be partially attributed to the differences in heart failure severity among patient populations, as hyponatremia is an independent predictor of worsening heart failure. The only published study to date on the influence of hyponatremia on readmission rates in the real‐world was conducted by Scherz et al, who reported that the co‐occurrence of hyponatremia in patients with acute pulmonary embolism, discharged from 185 hospitals in Pennsylvania, was independently associated with an increased readmission rate of 19.3%.27 In the present study, hyponatremia was associated with an incremental increase ranging between 14% and 17% for hospital readmission for any cause. It was conducted on a patient population in which hyponatremia was resultant from many causes, and not all patients had a serious comorbid condition. Also, the HN and non‐HN cohorts were matched for comorbidity prevalence and disease severity, and in other studies they were not. Therefore, the results of this study importantly imply that hyponatremia, whether it is resultant from a serious disease or any other cause substantially increases the healthcare burden. The implementation of strategies to prevent hospital readmissions may play an important role in reducing the healthcare burden of hyponatremia, and future studies are warranted to evaluate this hypothesis alongside evaluation of the outpatient hyponatremia burden.
The limitations of this study include, firstly, that it is only representative of inpatient hospital costs, and excludes outpatient healthcare utilization and costs. Secondly, this study utilized the Premier Hospital Database for patient selection, and laboratory testing data for serum sodium level are not available in this database; therefore, the severity of hyponatremia could not be accurately established in the HN patient population. Thirdly, the occurrence of hyponatremia in patients with some diseases is a marker of disease severity, as is the case with congestive heart failure and cirrhosis.23, 28 Our study did not adjust for the specific disease (eg, CHF) severity, which may influence the results. Future research is needed to evaluate the impact of hyponatremia on underlying disease severity of other diseases, and how its co‐occurrence may influence healthcare resource utilization and cost in each case. Although the Premier Hospital Database contains information from a large number of hospitals across the US, it is possible that it may not be representative of the entire US population of HN patients. Additionally, billing and coding errors and missing data could potentially have occurred, although the large patient population size likely precludes a large impact on the results of this study. Finally, the frequency of use of fluid restriction in these hospital settings could not be chronicled, thus limiting the ability to assess therapies and treatment modalities in use.
Acknowledgements
The authors acknowledge Melissa Lingohr‐Smith from Novosys Health in the editorial support and review of this manuscript.
Disclosures: This research was supported by Otsuka America Pharmaceutical, Inc, Princeton, NJ, which manufactures tolvaptan for the treatment of hyponatremia. Drs Amin and Deitelzweig are consultants for, and have received honoraria from, Otsuka America Pharmaceutical in connection with conducting this study. Drs Christian and Friend are employees of Otsuka America Pharmaceutical. Dr Lin is an employee of Novosys Health, which has received research funds from Otsuka America Pharmaceutical in connection with conducting this study and development of this manuscript. D. Baumer and Dr. Lowe are employees of Premier Inc, which has received research funds from Otsuka America Pharmaceutical. K. Belk was previously employed with Premier Inc.
- Management of hyponatremia: providing treatment and avoiding harm.Cleve Clin J Med.2010;77(10):715–726. , , .
- Causes and management of hyponatremia.Ann Pharmacother.2003;37(11):1694–1702. , , .
- Impact of hospital‐associated hyponatremia on selected outcomes.Arch Intern Med.2010;170(3):294–302. , , , , .
- Hyponatremia: a prospective analysis of its epidemiology and the pathogenetic role of vasopressin.Ann Intern Med.1985;102(2):164–168. , , , .
- Epidemiology, clinical and economic outcomes of admission hyponatremia among hospitalized patients.Curr Med Res Opin.2008;24(6):1601–1608. , , , et al.
- Mortality after hospitalization with mild, moderate, and severe hyponatremia.Am J Med.2009;122(9):857–865. , , .
- Relationship between admission serum sodium concentration and clinical outcomes in patients hospitalized for heart failure: an analysis from the OPTIMIZE‐HF registry.Eur Heart J.2007;28(8):980–988. , , , et al.
- Characterization and prognostic value of persistent hyponatremia in patients with severe heart failure in the ESCAPE Trial.Arch Intern Med.2007;167(18):1998–2005. , , , et al.
- Hyponatremia in cirrhosis: results of a patient population survey.Hepatology.2006;44(6):1535–1542. , , , .
- Hyponatremia in cirrhosis: from pathogenesis to treatment.Hepatology.1998;28(3):851–864. , , , et al.
- The syndrome of inappropriate antidiuresis: pathophysiology, clinical management and new therapeutic options.Nephron Clin Pract.2011;119(1):c62–c73. , , , , .
- Cost of illness of hyponatremia in the United States.Cost Eff Resour Alloc.2006;4:10. , , .
- US Health Care Budget: US Budget Breakdown for FY12—Charts. Available at: http://www.usgovernmentspending.com/health_care_budget_2012_1.html. Accessed December 19, 2011.
- 111th Congress. Patient Protection and Affordable Care Act. Public Law 111–148.1–906.
- An evaluation of statistical matching.JBES.1984;2(1):91–102. .
- Propensity score‐matching methods for nonexperimental causal studies.Rev Econ Stat.2002;84(1):151–161. , .
- Applied Logistic Regression Analysis. Quantitative Applications in the Social Sciences, Vol106.2nd ed.Thousand Oaks, CA:Sage;2002. .
- Reducing bias in a propensity score matched‐pair sample using greedy matching techniques. Paper presented at: Proceedings of the Twenty‐Sixth Annual SAS Users Group International Conference; April 22–25,2001; Long Beach, CA. .
- The effect of clustering of outcomes on the association of procedure volume and surgical outcomes.Ann Intern Med.2003;139(8):658–665. , , , , .
- Regression Modeling Strategies: With Applications to Linear Models, Logistic Regression, and Survival Analysis.New York, NY:Springer‐Verlag,2001. .
- Generalized Modeling Approaches to Risk Adjustment of Skewed Outcomes Data.Cambridge, MA:National Bureau of Economic Research, Inc;2003. , , .
- Economic impact of hyponatremia in hospitalized patients: a retrospective cohort study.Postgrad Med.2009;121(2):186–191. , , , .
- Burden of sodium abnormalities in patients hospitalized for heart failure.Congest Heart Fail.2011;17(1):1–7. , , , et al.
- Institute for Healthcare Improvement. Reduce Avoidable Hospital Readmissions. Available at: http://www.ihi.org/explore/readmissions/Pages/default.aspx. Accessed December 18, 2011.
- A meta‐analysis of hospital 30‐day avoidable readmission rates.J Eval Clin Pract.2011 Nov 9. doi: 10.1111/j.1365–2753.2011.01773.x. Published online August 17, 2012. , , .
- Critical elements of clinical follow‐up after hospital discharge for heart failure: insights from the EVEREST trial.Eur J Heart Fail.2010;12(4):367–374. , , , et al.
- Prognostic importance of hyponatremia in patients with acute pulmonary embolism.Am J Respir Crit Care Med.2010;182(9):1178–1183. , , , et al.
- Serum sodium predicts prognosis in critically ill cirrhotic patients.J Clin Gastroenterol.2010;44(3):220–226. , , , et al.
- Management of hyponatremia: providing treatment and avoiding harm.Cleve Clin J Med.2010;77(10):715–726. , , .
- Causes and management of hyponatremia.Ann Pharmacother.2003;37(11):1694–1702. , , .
- Impact of hospital‐associated hyponatremia on selected outcomes.Arch Intern Med.2010;170(3):294–302. , , , , .
- Hyponatremia: a prospective analysis of its epidemiology and the pathogenetic role of vasopressin.Ann Intern Med.1985;102(2):164–168. , , , .
- Epidemiology, clinical and economic outcomes of admission hyponatremia among hospitalized patients.Curr Med Res Opin.2008;24(6):1601–1608. , , , et al.
- Mortality after hospitalization with mild, moderate, and severe hyponatremia.Am J Med.2009;122(9):857–865. , , .
- Relationship between admission serum sodium concentration and clinical outcomes in patients hospitalized for heart failure: an analysis from the OPTIMIZE‐HF registry.Eur Heart J.2007;28(8):980–988. , , , et al.
- Characterization and prognostic value of persistent hyponatremia in patients with severe heart failure in the ESCAPE Trial.Arch Intern Med.2007;167(18):1998–2005. , , , et al.
- Hyponatremia in cirrhosis: results of a patient population survey.Hepatology.2006;44(6):1535–1542. , , , .
- Hyponatremia in cirrhosis: from pathogenesis to treatment.Hepatology.1998;28(3):851–864. , , , et al.
- The syndrome of inappropriate antidiuresis: pathophysiology, clinical management and new therapeutic options.Nephron Clin Pract.2011;119(1):c62–c73. , , , , .
- Cost of illness of hyponatremia in the United States.Cost Eff Resour Alloc.2006;4:10. , , .
- US Health Care Budget: US Budget Breakdown for FY12—Charts. Available at: http://www.usgovernmentspending.com/health_care_budget_2012_1.html. Accessed December 19, 2011.
- 111th Congress. Patient Protection and Affordable Care Act. Public Law 111–148.1–906.
- An evaluation of statistical matching.JBES.1984;2(1):91–102. .
- Propensity score‐matching methods for nonexperimental causal studies.Rev Econ Stat.2002;84(1):151–161. , .
- Applied Logistic Regression Analysis. Quantitative Applications in the Social Sciences, Vol106.2nd ed.Thousand Oaks, CA:Sage;2002. .
- Reducing bias in a propensity score matched‐pair sample using greedy matching techniques. Paper presented at: Proceedings of the Twenty‐Sixth Annual SAS Users Group International Conference; April 22–25,2001; Long Beach, CA. .
- The effect of clustering of outcomes on the association of procedure volume and surgical outcomes.Ann Intern Med.2003;139(8):658–665. , , , , .
- Regression Modeling Strategies: With Applications to Linear Models, Logistic Regression, and Survival Analysis.New York, NY:Springer‐Verlag,2001. .
- Generalized Modeling Approaches to Risk Adjustment of Skewed Outcomes Data.Cambridge, MA:National Bureau of Economic Research, Inc;2003. , , .
- Economic impact of hyponatremia in hospitalized patients: a retrospective cohort study.Postgrad Med.2009;121(2):186–191. , , , .
- Burden of sodium abnormalities in patients hospitalized for heart failure.Congest Heart Fail.2011;17(1):1–7. , , , et al.
- Institute for Healthcare Improvement. Reduce Avoidable Hospital Readmissions. Available at: http://www.ihi.org/explore/readmissions/Pages/default.aspx. Accessed December 18, 2011.
- A meta‐analysis of hospital 30‐day avoidable readmission rates.J Eval Clin Pract.2011 Nov 9. doi: 10.1111/j.1365–2753.2011.01773.x. Published online August 17, 2012. , , .
- Critical elements of clinical follow‐up after hospital discharge for heart failure: insights from the EVEREST trial.Eur J Heart Fail.2010;12(4):367–374. , , , et al.
- Prognostic importance of hyponatremia in patients with acute pulmonary embolism.Am J Respir Crit Care Med.2010;182(9):1178–1183. , , , et al.
- Serum sodium predicts prognosis in critically ill cirrhotic patients.J Clin Gastroenterol.2010;44(3):220–226. , , , et al.
Copyright © 2012 Society of Hospital Medicine
Duration of VTE Risk in Medically Ill Patients
Patients who are hospitalized for acute medical illness are at an increased risk of developing venous thromboembolism (VTE), which comprises deep‐vein thrombosis (DVT) and pulmonary embolism (PE).13 In a recent real‐world study of 158,325 US medical patients by Spyropoulos et al,4 4.0% of patients developed DVT, 1.5% developed PE, and 0.2% developed both DVT and PE. Furthermore, results from a population‐based case‐control study indicate that hospitalization for medical illness accounted for a proportion of VTE events similar to that of hospitalization for surgery (22% and 24%, respectively).5
Thromboprophylaxis reduces VTE incidence in at‐risk medical patients and is recommended according to evidence‐based guidelines from the American College of Chest Physicians (ACCP).1 The ACCP guidelines advocate that acutely ill medical patients admitted to the hospital with congestive heart failure (CHF) or severe lung disease/chronic obstructive pulmonary disease (COPD) or those who are confined to bed and have one or more additional risk factors (including active cancer, previous VTE, sepsis, acute neurologic disease, or inflammatory bowel disease) receive pharmacological prophylaxis with lowmolecular weight heparin (LMWH), low‐dose unfractionated heparin (UFH), or fondaparinux.1 Although guidelines provide recommendations for the duration of prophylaxis after major orthopedic surgery, such recommendations are unavailable for medical patients. In clinical trials of acutely ill medical patients, prophylaxis regimens found to be effective were provided for a duration of hospitalization of 6‐14 days.68 The mean length of hospital stay for medical illnesses is decreasing and is currently shorter than 6‐14 days.9, 10
In clinical practice, the duration of VTE risk during and after hospitalization is not well understood in medical patients, particularly in the context of shortening hospital stays. Such information could, however, provide insight into whether current thromboprophylaxis practices reflect real‐world need. To gain a greater understanding of the period during which patients are at risk of VTE, this retrospective, observational study assessed the incidence and time course of symptomatic VTE events during and after hospitalization in a large population of US medical patients.
METHODS
Data and Patient Selection
This study employed linked administrative claims data and hospital billing data contained in the Thomson Reuters MarketScan Inpatient Drug Link File. This combines longitudinal patient‐level inpatient and outpatient medical and pharmaceutical claims data from the MarketScan Commercial claims data from the MarketScan Commercial Claims and Encounters (Commercial) and Medicare Supplemental and Coordination of Benefits (Medicare Supplemental) databases, with hospital discharge records detailing services used and drugs administered during a hospitalization, which are included in the Hospital Drug Database. The linked data sources enable analysis of a patient's experience before, during, and after a hospitalization. The present study was not designed to obtain bleeding rates.
The study cohort comprised patients considered to be at‐risk for VTE as a result of a medical hospitalization occurring between January 1, 2005, and December 31, 2008. At‐risk medical hospitalizations were those for which the primary diagnosis was for cancer, CHF, severe lung disease/COPD, or infectious disease (see Supporting Information, Appendix I, for International Classification of Diseases, 9th Revision, Clinical Modification [ICD‐9‐CM] codes used to identify patients with medical illnesses). Included patients were required to be at least 18 years of age at the time of admission and were required to be continuously enrolled in their insurance benefits for at least 12 months before admission (the baseline period) and for at least 180 days after the admission date (the evaluation period) to ensure that all administrative claims data during that period were captured. Patients who died in‐hospital from any cause were exempted from the continuous enrollment criterion, as long as they had been continuously enrolled prior to inpatient death. Patients transferred from or discharged to another acute‐care facility were excluded because of the possibility for incomplete inpatient data capture. For patients who had multiple medical hospitalizations between January 1, 2005, and December 31, 2008, the hospitalization around which the analysis focused was randomly selected from the set of potential medical hospitalizations.
Prophylaxis
Pharmacological prophylaxis was identified via charge codes during hospitalization or via pharmacy claims after discharge for UFH, enoxaparin, dalteparin, warfarin, and fondaparinux. All dosages of a pharmacological agent were considered prophylactic only if there was no evidence of VTE during the admission, with the exception of warfarin (Supporting Information, Appendix II). Post‐discharge use of anticoagulation therapy was measured for up to 35 days after discharge from the hospital. Use of mechanical prophylaxis during hospitalization was identified via charge codes for graduated compression stockings and charge codes indicating use of intermittent pneumatic compression devices and/or venous foot pumps. The appropriateness of prophylaxis was not assessed.
Analysis
The risk of VTE was estimated across an evaluation period of 180 days by measuring VTE occurrence and person‐time exposure. Inpatient VTE occurrence was defined as any nonprimary diagnosis of DVT and/or PE during the at‐risk hospitalization. VTE after discharge was defined as an ICD‐9‐CM diagnosis code, whether primary or secondary, for DVT or PE in the evaluation period during an emergency room or inpatient admission, or on an outpatient claim with 1 or more of the following confirmatory events: an emergency room or inpatient admission for VTE within 2 days of the outpatient diagnosis; a prescription claim for enoxaparin, fondaparinux, or UFH within 15 days after diagnosis; or a prescription claim for warfarin within 15 days after diagnosis and no evidence of atrial fibrillation or atrial flutter in the 6 months before the outpatient diagnosis for DVT or PE. Person‐time exposure was measured as the number of days from the hospital admission date to the first occurrence of VTE, or censoring at a subsequent at‐risk hospitalization, death, or 180 days after admission.
Cumulative risk of VTE over the 180‐day evaluation period was calculated by the Kaplan‐Meier product limit method of survival analysis and displayed for deciles of cumulative risk at 180 days after the hospital admission date. The risk of VTE at each point of time during the evaluation period (the hazard function) was first calculated on a daily basis and then smoothed via LOESS regression, a locally weighted regression procedure.
RESULTS
Patient Demographics
A total of 11,139 medical patients were included in the analysis (Figure 1), with a mean standard deviation (SD) age of 67.6 13.9 years, and 51.6% were women (Table 1). Of the reasons for admission to the hospital, 51.5% of patients were admitted for severe lung disease/COPD, 20.1% were admitted for cancer, 15.3% were admitted for CHF, and 13.1% were admitted for severe infectious disease. Most patients were treated in an urban hospital (87.5%), in a hospital without teaching status (87.9%), and in the South Census region (74.1%). The majority of patients were treated in medium‐sized to large care facilities. Risk factors for VTE during the baseline period included hospitalization for a medical condition with a high risk for VTE (75.6%), a prior at‐risk hospitalization (18.6%), cancer therapy (10.0% of all medical patients combined and 18.5% of cancer patients), trauma (9.2%), and previous VTE (4.3%).
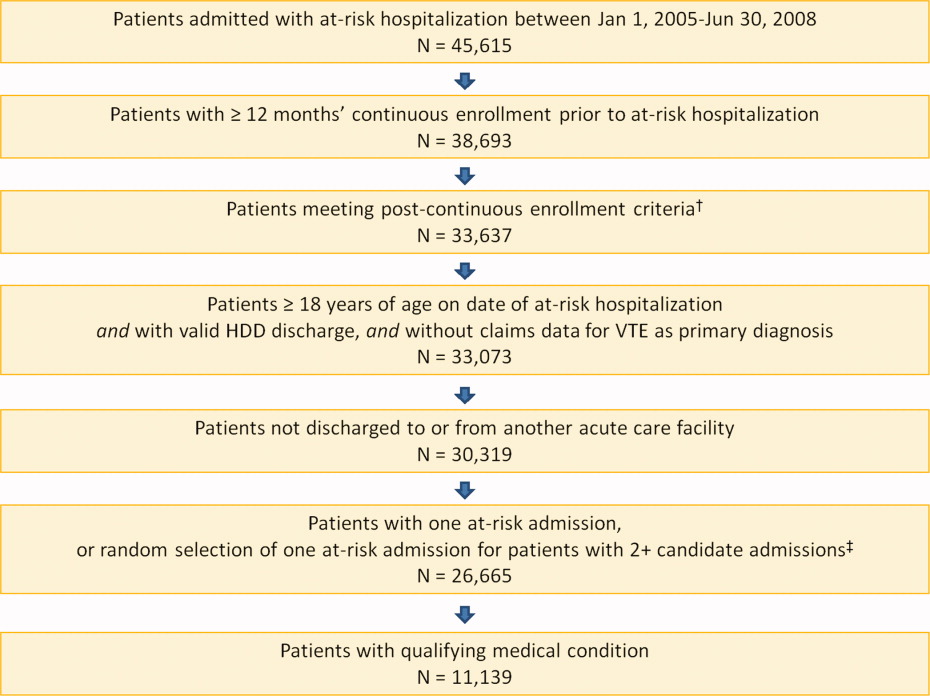
Characteristic | Medical Patients (N = 11,139) |
---|---|
| |
Gender | |
Men | 5389 (48.4) |
Women | 5750 (51.6) |
Reason for hospitalization | |
Cancer | 2243 (20.1) |
CHF | 1705 (15.3) |
Severe lung disease/COPD | 5736 (51.5) |
Severe infectious disease | 1455 (13.1) |
Age group, years | |
1834 | 230 (2.1) |
3544 | 442 (4.0) |
4554 | 1188 (10.7) |
5564 | 2644 (23.7) |
6574 | 2657 (23.9) |
7584 | 2969 (26.7) |
85 years | 1009 (9.1) |
Median age SD, years | 67.6 13.9 |
Primary payer* | |
Medicare | 6819 (61.2) |
Commercial | 4320 (38.8) |
Geographical area | |
Northeast | 122 (1.1) |
North Central | 2649 (23.8) |
South | 8258 (74.1) |
West | 110 (1.0) |
Urban location | 9743 (87.5) |
Teaching hospital | 1345 (12.1) |
Licensed bed size | |
1199 | 1621 (14.6) |
200299 | 2869 (25.8) |
300499 | 4005 (36.0) |
500 | 2644 (23.7) |
VTE Prophylaxis
Patients stayed in hospital for a mean SD duration of 5.3 5.3 days, varying from 4.6 3.9 days in patients with CHF to 6.7 6.5 days in patients with infectious disease, during which 46.7% of patients received pharmacological VTE prophylaxis. Inpatient pharmacological prophylaxis rates ranged from 64.1% in patients with CHF to 30.7% in patients with cancer (Table 2). Overall, the most commonly used form of inpatient pharmacological prophylaxis was enoxaparin (26.8% of all patients), followed by UFH (13.5% of all patients). Mechanical prophylaxis was received by 12.2% of all patients. Mean SD VTE prophylaxis duration during hospitalization was 5.0 4.7 days, varying from 4.2 4.0 days in patients with cancer to 6.2 5.5 days in patients with infectious disease.
n (%) | Infectious Disease (n = 1455) | CHF (n = 1705) | Severe Lung Disease/COPD (n = 5736) | Cancer (n = 2243) | Any Medical (N = 11,139) |
---|---|---|---|---|---|
| |||||
Pharmacological prophylaxis during hospitalization* | 599 (41.2) | 1093 (64.1) | 2820 (49.2) | 688 (30.7) | 5200 (46.7) |
Enoxaparin | 362 (24.9) | 466 (27.3) | 1877 (32.7) | 282 (12.6) | 2987 (26.8) |
UFH | 191 (13.1) | 400 (23.5) | 527 (9.2) | 383 (17.1) | 1501 (13.5) |
Warfarin | 135 (9.3) | 498 (29.2) | 622 (10.8) | 95 (4.2) | 1350 (12.1) |
Dalteparin | 16 (1.1) | 21 (1.2) | 109 (1.9) | 16 (0.7) | 162 (1.5) |
Fondaparinux | 5 (0.3) | 4 (0.2) | 22 (0.4) | 2 (0.1) | 33 (0.3) |
Mechanical prophylaxis in hospital | 148 (10.2) | 65 (3.8) | 343 (6.0) | 803 (35.8) | 1359 (12.2) |
Anticoagulation within 35 days after discharge | 104 (7.1) | 315 (18.5) | 397 (6.9) | 166 (7.4) | 982 (8.8) |
Enoxaparin | 15 (1.0) | 14 (0.8) | 32 (0.6) | 25 (1.1) | 86 (0.8) |
UFH | 17 (1.2) | 10 (0.6) | 23 (0.4) | 35 (1.6) | 85 (0.8) |
Warfarin | 79 (5.4) | 302 (17.7) | 357 (6.2) | 116 (5.2) | 854 (7.7) |
Dalteparin | 0 | 0 | 2 (<0.1) | 1 (<0.1) | 3 (<0.1) |
Fondaparinux | 1 (0.1) | 0 | 0 | 2 (0.1) | 3 (<0.1) |
Antiplatelet therapy within 35 days after discharge | 72 (4.9) | 217 (12.7) | 351 (6.1) | 53 (2.4) | 693 (6.2) |
In the 35 days after discharge, 8.8% of patients received anticoagulation therapy, most commonly warfarin (7.7%). The rate of outpatient prophylaxis was highest in patients hospitalized for CHF (18.5%) compared with other medical conditions (7%).
Time Course of VTE Risk and Hazard Function
Overall, there were 366 symptomatic VTE events, representing a VTE rate of 3.3%. These events comprised 241 DVT‐only events, 98 PE‐only events, and 27 events with evidence of both DVT and PE. In total, 43.4% of events occurred during hospitalization (Figure 2). The VTE rate was 5.7% in patients with cancer (30.5% of events occurring in hospital), 4.3% with infectious disease (61.9% in hospital), 3.1% with CHF (54.7% in hospital), and 2.1% with severe lung disease/COPD (42.6% in hospital). The highest number of VTE events, 97 events (62 DVT only, 26 PE only, and 9 events both DVT and PE), occurred in the first 9 days after the hospital admission date, of which 87.6% were during hospitalization. During days 10‐19, there were 82 VTE events (50 DVT only, 24 PE only, and 8 both DVT and PE), 70.7% of which occurred in the hospital. Over the following 10‐day periods, VTE incidence gradually declined (Figure 2) and fluctuated at a background level of 4‐8 events during each 10‐day interval from 120 to 180 days.
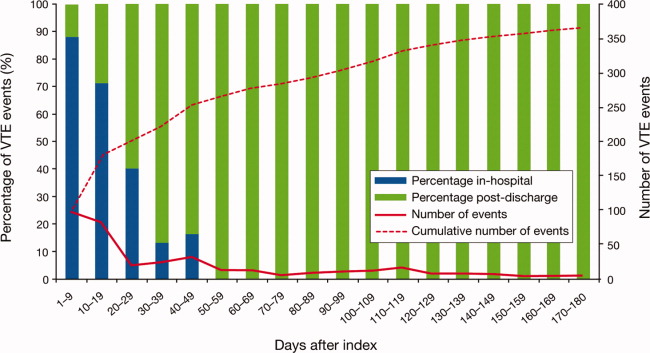
The cumulative probability of VTE among all patients was 0.035 (Figure 3A). Half of the VTE risk had accumulated by day 23, and 75% had accumulated by day 71. By day 30, the proportion of cumulative risk was 52.6% overall, and ranged from 41.9% with cancer to 72.9% with infectious disease (Figure 3).
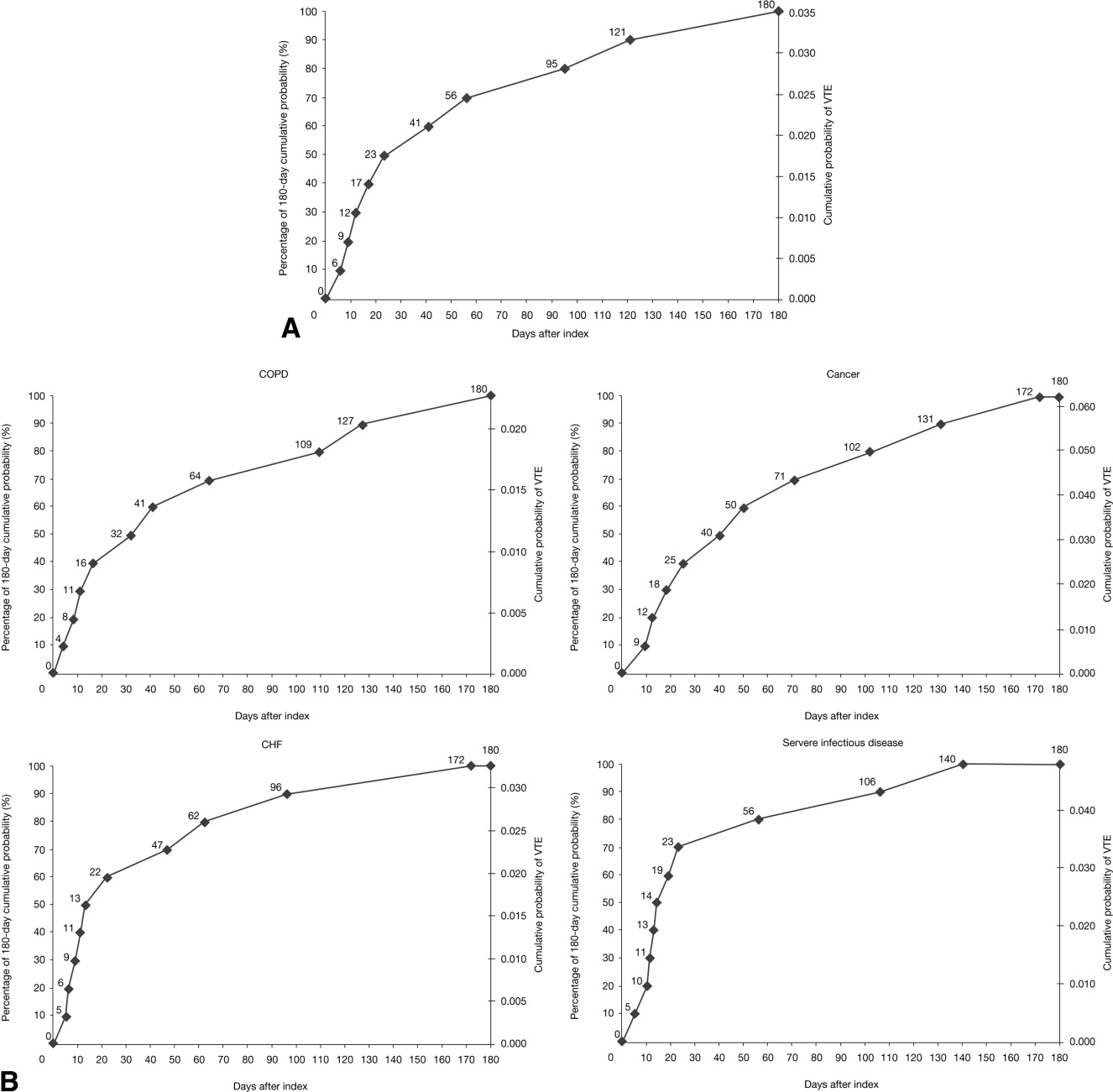
The VTE hazard peaked at approximately 1.05 VTE events per 1000 person‐days on day 8 after the hospital admission date overall (Figure 4A). The cumulative hazard at the peak day was 18.2% of the total VTE hazard over the 180‐day evaluation period. The hazard peak ranged from day 7 in patients with severe lung disease/COPD to day 12 in patients with infectious disease (Figure 4B). The cumulative hazard at the peak day was 39.7% for patients with infectious disease, 29.2% for patients with CHF, and approximately 19% for cancer or severe lung disease/COPD. After the peak risk day, the VTE hazard function decreased until the curve reached an inflection point, at day 28, when the cumulative risk was 51.8% (Figure 4A). After the inflection point, the VTE hazard increased to 0.3 VTE events per 1000 person‐days at approximately day 40‐45 and then decreased to <0.2 events per 1000 person‐days. The timing of the inflection varied by approximately 1 week across the medical illnesses (ranging from day 25 for severe lung disease/COPD to day 33 for CHF), with the cumulative risk at the inflection point ranging from 41.9% with cancer to 72.9% with infectious disease.
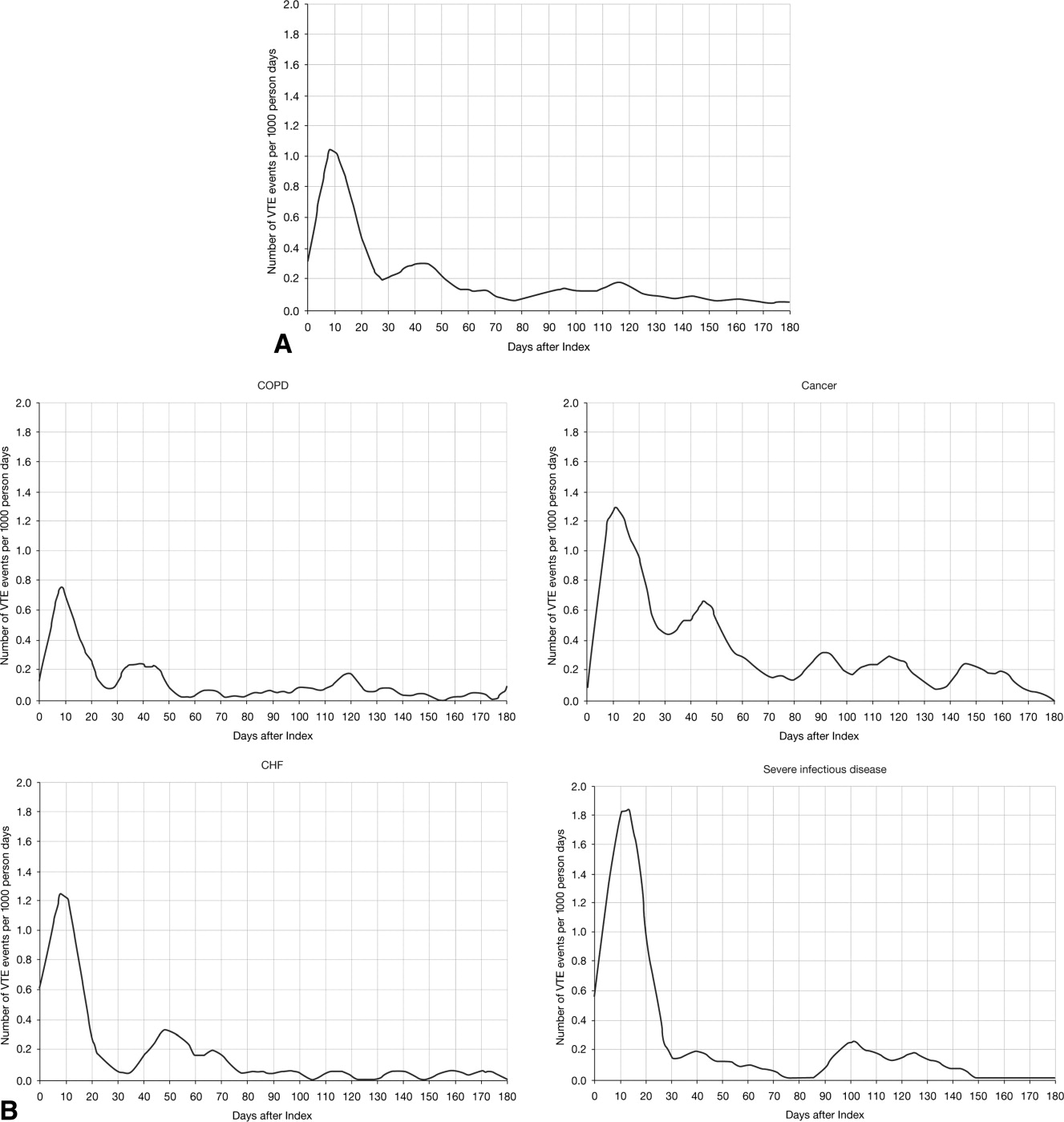
DISCUSSION
The results from this large, real‐world study provide new insights into the duration of risk of symptomatic VTE in medical patients and demonstrate that the number of VTE events was highest during days 0‐19, with the peak of VTE hazard at day 8. Half of the total 180‐day cumulative risk had been incurred by day 23 after hospital admission, and the period of greatest increased risk extended up to at least 30 days. Importantly, more than half of VTE events occurred after discharge (56.6%). A particularly high proportion of VTE events (69.5%) had occurred after discharge in patients with cancer. Although it was assumed that most VTE events that could be reasonably attributed to an at‐risk hospitalization would occur within 90 days as shown previously,4, 11 the 180‐day evaluation period was used to examine whether there was a prolonged period of continually diminished VTE risk from 90 to 180 days. Thus, events occurring within the later portions of the evaluation period may or may not have been attributable to the index hospitalization, potentially reflecting a background rate of VTE as noted above. Although these events are included in our estimate of the 180‐day cumulative risk of VTE, interpretation of the study results excluding such events is possible by examining the cumulative risk that had been incurred at each time point during the evaluation period.
Few other studies have assessed the duration of VTE risk in hospitalized medical patients. In a study by Spyropoulos et al,4 the median time to a DVT and/or PE event was 74 days, ranging from 62 days in severe infectious disease to 126 days in CHF. In another observational study that included patients who had recently been hospitalized but had not undergone surgery, 66.9% of patients who experienced DVT and/or PE events were diagnosed with DVT and/or PE within the first month after hospital discharge; 19.9% between months 1 and 2, and 13.2% between months 2 and 3.12
Fewer than half of the patients in the present study received thromboprophylaxis, which is consistent with other studies demonstrating the low prophylaxis rates in medical inpatients.9, 1315 In a recently published US study of discharge records that included 22,455 medical inpatients, prophylaxis rates were 59.4% in patients with CHF, 52.3% with cancer, 45.8% with severe lung disease/COPD, and 40.4% with infectious disease.14 Fewer than 10% of patients in the present study received prophylaxis after discharge, a result that is consistent with other studies.4, 9
The effect of extended prophylaxis in acutely ill medical patients with the LMWH enoxaparin beyond 6‐14 days has been investigated in the EXCLAIM study.16 This trial included approximately 5800 acutely ill medical patients at significant risk of developing VTE due to a recent reduction in mobility. Patients in the extended prophylaxis group had a lower risk of VTE (2.5% vs 4% for placebo; absolute risk reduction 1.5% [95.8% confidence interval 2.54% to 0.52%]), but had increased major bleeding events (0.8% vs 0.3% for placebo; absolute risk difference favoring placebo, 0.51% [95% confidence interval, 0.12% to 0.89%]). The patient populations with most benefit from an additional 28 days prophylaxis with enoxaparin, in addition to the usual short‐term prophylaxis of 10 days, were patients with restricted mobility (level 1; total bed rest/sedentary), elderly patients (age >75 years), and women. A limitation of the EXCLAIM trial is that estimates of efficacy and safety are difficult to interpret: after an interim analysis of adjudicated efficacy and safety outcomes, amendments were made to the original study protocol by changing eligibility criteria for patients with level 2 immobility (level 1 with bathroom privileges).16
The optimal duration of prophylaxis for medical patients has not been determined; prophylaxis is generally administered to at‐risk medical patients for the duration of hospitalization. In the current study, mean length of stay was 5.3 5.3 days overall. As hospital stays shorten, many medical patients who are prescribed inpatient prophylaxis alone are unlikely to receive the standard 6‐14 days of prophylaxis shown to be effective in clinical trials.68 Furthermore, the extended period of VTE risk in the present study and the finding that 56.6% of events occurred after discharge also suggest that current practices for inpatient prophylaxis alone may need to be evaluated.
This study reports real‐world data from a large, well‐defined population and obtains the incidence of symptomatic VTE events. Even though certain demographic data deviate from the national averagefor example, 74.1% of patients were treated in the South Census region, whereas this region is served by 37.6% of US hospitals17; 87.5% of hospitals had an urban location (compared with 60.1% of US hospitals18), and 85.4% of hospitals had a licensed bed size of at least 200 beds (compared with 28.2% of US hospitals, with the average US hospital having fewer than 100 beds19)these data may be beneficial in guiding policy and health care strategies for gaining understanding of the duration of risk for VTE.
Limitations of the study include characterization of the VTE risk period through examination of the cumulative risk and hazard of VTE across time, as the actual VTE risk period cannot be determined with exact precision. We used ICD‐9‐CM diagnosis coding to identify VTE. Since many cases of PE are asymptomatic and detected at autopsy,20 our approach may have missed such cases, as they would not have been recorded within the database. Furthermore, validation studies suggest that suboptimal specificity exists for ICD‐9‐CM diagnosis codes used to identify VTE.21 In an attempt to improve the specificity of our VTE identification algorithm, we required that post‐discharge VTE was recorded either during an emergency room or subsequent inpatient admission (which would be indicative of acute care for VTE) or on an outpatient claim with subsequent evidence of treatment for VTE. The true sensitivity and specificity of the VTE identification algorithms used for this study remain unknown, however, so the study findings should be interpreted in light of this limitation. The databases used for the analysis may not be representative of the US population as a whole; for example, this study used claims data from commercial and Medicare supplemental databases, which do not include Medicaid patients. Another limitation was that outpatient mechanical prophylaxis, such as graded compression stockings, was not captured due to over‐the‐counter availability. In addition, appropriateness of prophylaxis was not determined in this study, because these data could not be obtained from the claims database used. Further studies are warranted to obtain information on the incidence of VTE after hospitalization for medical illness in patients who received appropriate prophylaxis during hospitalization.
Finally, all dosages of a pharmacological agent were considered prophylactic only if a VTE event did not occur, with the exception of warfarin; any dose of warfarin was considered for prophylaxis, regardless of a VTE diagnosis. Warfarin may be used for purposes other than VTE prophylaxis (eg, prophylaxis for a thromboembolic cerebrovascular accident). The data source does not allow for identifying the exact reason for anticoagulation therapy with warfarin. Nonetheless, warfarin therapy will confer a decreased risk of VTE regardless of its purpose.
Results from this large cohort of medical patients indicate that symptomatic VTE risk is highest within the first 19 days after hospital admission (a period that may encompass both the duration of hospitalization as well as the period after discharge) with a considerable risk of VTE extending into the period after discharge. Receiving appropriate prophylaxis in‐hospital remains of great importance to prevent inpatient and likely post‐discharge VTE in patients with acute medical illness. In addition, given the time course of VTE events, with VTE incidence peaking at 8 days but with increased risk extending to 30 days, and the number of out‐of‐hospital VTE events incurred, the results of this study suggest that future research is warranted to investigate the risks and benefits of improving thromboprophylaxis practices in the period after hospitalization.
Acknowledgements
Funding Source: sanofi‐aventis U.S. provided funding to Thomson Reuters to perform this study. The authors received editorial/writing support from Tessa Hartog of Excerpta Medica in the preparation of the manuscript funded by sanofi‐aventis U.S.
Disclosure: Alpesh Amin has received research honorarium and is on the speakers bureau for sanofi‐aventis U.S. Otsuka Pharmaceutical, and Boehringer‐Ingelheim. Helen Varker, Nicole Princic, and Stephen Johnston are employees at Thomson Reuters, which received funding from sanofi‐aventis U.S. Jay Lin is an employee of Novosys Health, which received funding from sanofi‐aventis U.S. Stephen Thompson is an employee of sanofi‐aventis U.S.
- Prevention of venous thromboembolism: American College of Chest Physicians Evidence‐Based Clinical Practice Guidelines (8th Edition). Chest. 2008;133(6 Suppl):381S–453S. , , , et al.
- Risk factors for deep vein thrombosis and pulmonary embolism: a population‐based case‐control study. Arch Intern Med. 2000;160:809–815. , , , , , .
- The epidemiology of venous thromboembolism in the community. Arterioscler Thromb Vasc Biol. 2008;28:370–372. .
- Rates of venous thromboembolism occurrence in medical patients among the insured population. Thromb Haemost. 2009;102:951–957. , , , .
- Relative impact of risk factors for deep vein thrombosis and pulmonary embolism: a population‐based study. Arch Intern Med. 2002;162:1245–1248. , , , et al.
- A comparison of enoxaparin with placebo for the prevention of venous thromboembolism in acutely ill medical patients. Prophylaxis in Medical Patients with Enoxaparin Study Group. N Engl J Med. 1999;341:793–800. , , , et al.
- Randomized, placebo‐controlled trial of dalteparin for the prevention of venous thromboembolism in acutely ill medical patients. Circulation. 2004;110:874–879. , , , et al.
- Efficacy and safety of fondaparinux for the prevention of venous thromboembolism in older acute medical patients: randomised placebo controlled trial. BMJ. 2006;332:325–329. , , , et al.
- Lack of thromboprophylaxis across the care continuum in US medical patients. Hosp Pract (Minneap). 2010;38:17–25. , , .
- HCUP NIS Related Reports. Healthcare Cost and Utilization Project (HCUP), September 2008. Available at: www.hcup‐us.ahrq.gov/db/nation/nis/nisrelatedreports.jsp. Accessed June 2011.
- Direct medical costs of venous thromboembolism and subsequent hospital readmission rates: an administrative claims analysis from 30 managed care organizations. J Manag Care Pharm. 2007;13:475–486. , .
- Venous thromboembolism in the outpatient setting. Arch Intern Med. 2007;167:1471–1475. , , , , .
- Inpatient thromboprophylaxis use in U.S. hospitals: adherence to the seventh American College of Chest Physician's recommendations for at‐risk medical and surgical patients. J Hosp Med. 2009;4:E15–E21. , , , .
- Are hospitals delivering appropriate VTE prevention? The venous thromboembolism study to assess the rate of thromboprophylaxis (VTE start). J Thromb Thrombolysis. 2010;29:326–339. , , , et al.
- Venous thromboembolism risk and prophylaxis in hospitalised medically ill patients. The ENDORSE Global Survey. Thromb Haemost. 2010;103:736–748. , , , et al.
- Extended‐duration venous thromboembolism prophylaxis in acutely ill medical patients with recently reduced mobility: a randomized trial. Ann Intern Med. 2010;153:8–18. , , , et al.
- American Society for Healthcare Engineering of the American Hospital Association. Overview of the Hospital Market, 2009. Available from: www.ashe.org/e2c/pdfs/energy/heg_ch2_background.pdf. Accessed June 2011.
- American Hospital Association. Fast Facts on US Hospitals, 2009. Available at: http://www.aha.org/aha/resource‐center/Statistics‐and‐Studies/fast‐facts.html. Accessed June 2011.
- American Hospital Association. AHA Annual Survey of Hospitals Database, 2009. Available from: http://www.ahadata.com/ahadata_app/index.jsp. Accessed June 2011.
- The epidemiology of venous thromboembolism. Circulation. 2003;107(23 suppl 1):I4–I8. .
- How valid is the ICD‐9‐CM based AHRQ patient safety indicator for postoperative venous thromboembolism? Med Care. 2009;47:1237–1243. , , , et al.
Patients who are hospitalized for acute medical illness are at an increased risk of developing venous thromboembolism (VTE), which comprises deep‐vein thrombosis (DVT) and pulmonary embolism (PE).13 In a recent real‐world study of 158,325 US medical patients by Spyropoulos et al,4 4.0% of patients developed DVT, 1.5% developed PE, and 0.2% developed both DVT and PE. Furthermore, results from a population‐based case‐control study indicate that hospitalization for medical illness accounted for a proportion of VTE events similar to that of hospitalization for surgery (22% and 24%, respectively).5
Thromboprophylaxis reduces VTE incidence in at‐risk medical patients and is recommended according to evidence‐based guidelines from the American College of Chest Physicians (ACCP).1 The ACCP guidelines advocate that acutely ill medical patients admitted to the hospital with congestive heart failure (CHF) or severe lung disease/chronic obstructive pulmonary disease (COPD) or those who are confined to bed and have one or more additional risk factors (including active cancer, previous VTE, sepsis, acute neurologic disease, or inflammatory bowel disease) receive pharmacological prophylaxis with lowmolecular weight heparin (LMWH), low‐dose unfractionated heparin (UFH), or fondaparinux.1 Although guidelines provide recommendations for the duration of prophylaxis after major orthopedic surgery, such recommendations are unavailable for medical patients. In clinical trials of acutely ill medical patients, prophylaxis regimens found to be effective were provided for a duration of hospitalization of 6‐14 days.68 The mean length of hospital stay for medical illnesses is decreasing and is currently shorter than 6‐14 days.9, 10
In clinical practice, the duration of VTE risk during and after hospitalization is not well understood in medical patients, particularly in the context of shortening hospital stays. Such information could, however, provide insight into whether current thromboprophylaxis practices reflect real‐world need. To gain a greater understanding of the period during which patients are at risk of VTE, this retrospective, observational study assessed the incidence and time course of symptomatic VTE events during and after hospitalization in a large population of US medical patients.
METHODS
Data and Patient Selection
This study employed linked administrative claims data and hospital billing data contained in the Thomson Reuters MarketScan Inpatient Drug Link File. This combines longitudinal patient‐level inpatient and outpatient medical and pharmaceutical claims data from the MarketScan Commercial claims data from the MarketScan Commercial Claims and Encounters (Commercial) and Medicare Supplemental and Coordination of Benefits (Medicare Supplemental) databases, with hospital discharge records detailing services used and drugs administered during a hospitalization, which are included in the Hospital Drug Database. The linked data sources enable analysis of a patient's experience before, during, and after a hospitalization. The present study was not designed to obtain bleeding rates.
The study cohort comprised patients considered to be at‐risk for VTE as a result of a medical hospitalization occurring between January 1, 2005, and December 31, 2008. At‐risk medical hospitalizations were those for which the primary diagnosis was for cancer, CHF, severe lung disease/COPD, or infectious disease (see Supporting Information, Appendix I, for International Classification of Diseases, 9th Revision, Clinical Modification [ICD‐9‐CM] codes used to identify patients with medical illnesses). Included patients were required to be at least 18 years of age at the time of admission and were required to be continuously enrolled in their insurance benefits for at least 12 months before admission (the baseline period) and for at least 180 days after the admission date (the evaluation period) to ensure that all administrative claims data during that period were captured. Patients who died in‐hospital from any cause were exempted from the continuous enrollment criterion, as long as they had been continuously enrolled prior to inpatient death. Patients transferred from or discharged to another acute‐care facility were excluded because of the possibility for incomplete inpatient data capture. For patients who had multiple medical hospitalizations between January 1, 2005, and December 31, 2008, the hospitalization around which the analysis focused was randomly selected from the set of potential medical hospitalizations.
Prophylaxis
Pharmacological prophylaxis was identified via charge codes during hospitalization or via pharmacy claims after discharge for UFH, enoxaparin, dalteparin, warfarin, and fondaparinux. All dosages of a pharmacological agent were considered prophylactic only if there was no evidence of VTE during the admission, with the exception of warfarin (Supporting Information, Appendix II). Post‐discharge use of anticoagulation therapy was measured for up to 35 days after discharge from the hospital. Use of mechanical prophylaxis during hospitalization was identified via charge codes for graduated compression stockings and charge codes indicating use of intermittent pneumatic compression devices and/or venous foot pumps. The appropriateness of prophylaxis was not assessed.
Analysis
The risk of VTE was estimated across an evaluation period of 180 days by measuring VTE occurrence and person‐time exposure. Inpatient VTE occurrence was defined as any nonprimary diagnosis of DVT and/or PE during the at‐risk hospitalization. VTE after discharge was defined as an ICD‐9‐CM diagnosis code, whether primary or secondary, for DVT or PE in the evaluation period during an emergency room or inpatient admission, or on an outpatient claim with 1 or more of the following confirmatory events: an emergency room or inpatient admission for VTE within 2 days of the outpatient diagnosis; a prescription claim for enoxaparin, fondaparinux, or UFH within 15 days after diagnosis; or a prescription claim for warfarin within 15 days after diagnosis and no evidence of atrial fibrillation or atrial flutter in the 6 months before the outpatient diagnosis for DVT or PE. Person‐time exposure was measured as the number of days from the hospital admission date to the first occurrence of VTE, or censoring at a subsequent at‐risk hospitalization, death, or 180 days after admission.
Cumulative risk of VTE over the 180‐day evaluation period was calculated by the Kaplan‐Meier product limit method of survival analysis and displayed for deciles of cumulative risk at 180 days after the hospital admission date. The risk of VTE at each point of time during the evaluation period (the hazard function) was first calculated on a daily basis and then smoothed via LOESS regression, a locally weighted regression procedure.
RESULTS
Patient Demographics
A total of 11,139 medical patients were included in the analysis (Figure 1), with a mean standard deviation (SD) age of 67.6 13.9 years, and 51.6% were women (Table 1). Of the reasons for admission to the hospital, 51.5% of patients were admitted for severe lung disease/COPD, 20.1% were admitted for cancer, 15.3% were admitted for CHF, and 13.1% were admitted for severe infectious disease. Most patients were treated in an urban hospital (87.5%), in a hospital without teaching status (87.9%), and in the South Census region (74.1%). The majority of patients were treated in medium‐sized to large care facilities. Risk factors for VTE during the baseline period included hospitalization for a medical condition with a high risk for VTE (75.6%), a prior at‐risk hospitalization (18.6%), cancer therapy (10.0% of all medical patients combined and 18.5% of cancer patients), trauma (9.2%), and previous VTE (4.3%).
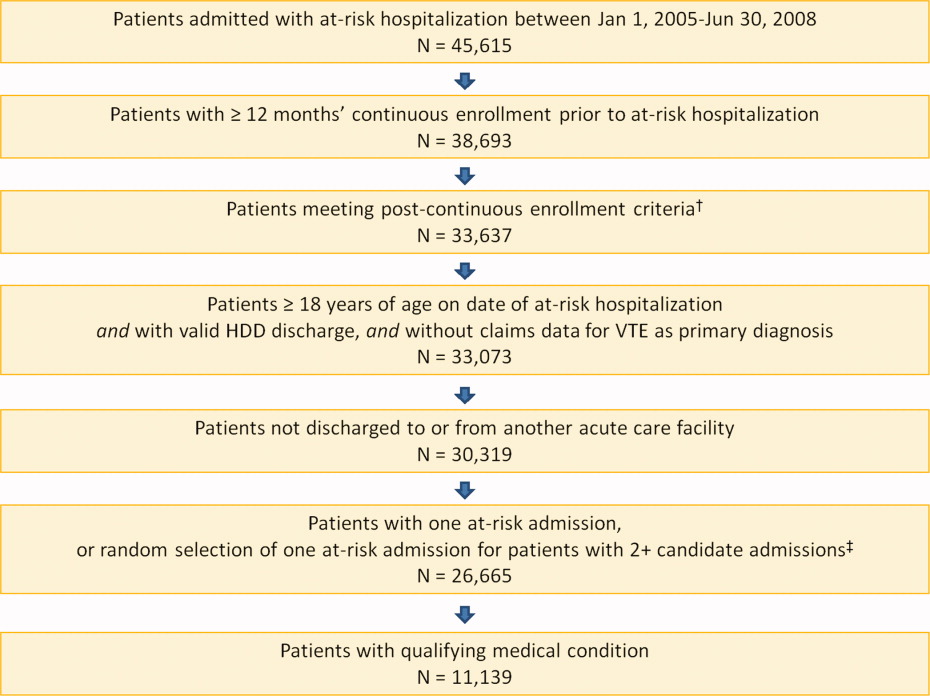
Characteristic | Medical Patients (N = 11,139) |
---|---|
| |
Gender | |
Men | 5389 (48.4) |
Women | 5750 (51.6) |
Reason for hospitalization | |
Cancer | 2243 (20.1) |
CHF | 1705 (15.3) |
Severe lung disease/COPD | 5736 (51.5) |
Severe infectious disease | 1455 (13.1) |
Age group, years | |
1834 | 230 (2.1) |
3544 | 442 (4.0) |
4554 | 1188 (10.7) |
5564 | 2644 (23.7) |
6574 | 2657 (23.9) |
7584 | 2969 (26.7) |
85 years | 1009 (9.1) |
Median age SD, years | 67.6 13.9 |
Primary payer* | |
Medicare | 6819 (61.2) |
Commercial | 4320 (38.8) |
Geographical area | |
Northeast | 122 (1.1) |
North Central | 2649 (23.8) |
South | 8258 (74.1) |
West | 110 (1.0) |
Urban location | 9743 (87.5) |
Teaching hospital | 1345 (12.1) |
Licensed bed size | |
1199 | 1621 (14.6) |
200299 | 2869 (25.8) |
300499 | 4005 (36.0) |
500 | 2644 (23.7) |
VTE Prophylaxis
Patients stayed in hospital for a mean SD duration of 5.3 5.3 days, varying from 4.6 3.9 days in patients with CHF to 6.7 6.5 days in patients with infectious disease, during which 46.7% of patients received pharmacological VTE prophylaxis. Inpatient pharmacological prophylaxis rates ranged from 64.1% in patients with CHF to 30.7% in patients with cancer (Table 2). Overall, the most commonly used form of inpatient pharmacological prophylaxis was enoxaparin (26.8% of all patients), followed by UFH (13.5% of all patients). Mechanical prophylaxis was received by 12.2% of all patients. Mean SD VTE prophylaxis duration during hospitalization was 5.0 4.7 days, varying from 4.2 4.0 days in patients with cancer to 6.2 5.5 days in patients with infectious disease.
n (%) | Infectious Disease (n = 1455) | CHF (n = 1705) | Severe Lung Disease/COPD (n = 5736) | Cancer (n = 2243) | Any Medical (N = 11,139) |
---|---|---|---|---|---|
| |||||
Pharmacological prophylaxis during hospitalization* | 599 (41.2) | 1093 (64.1) | 2820 (49.2) | 688 (30.7) | 5200 (46.7) |
Enoxaparin | 362 (24.9) | 466 (27.3) | 1877 (32.7) | 282 (12.6) | 2987 (26.8) |
UFH | 191 (13.1) | 400 (23.5) | 527 (9.2) | 383 (17.1) | 1501 (13.5) |
Warfarin | 135 (9.3) | 498 (29.2) | 622 (10.8) | 95 (4.2) | 1350 (12.1) |
Dalteparin | 16 (1.1) | 21 (1.2) | 109 (1.9) | 16 (0.7) | 162 (1.5) |
Fondaparinux | 5 (0.3) | 4 (0.2) | 22 (0.4) | 2 (0.1) | 33 (0.3) |
Mechanical prophylaxis in hospital | 148 (10.2) | 65 (3.8) | 343 (6.0) | 803 (35.8) | 1359 (12.2) |
Anticoagulation within 35 days after discharge | 104 (7.1) | 315 (18.5) | 397 (6.9) | 166 (7.4) | 982 (8.8) |
Enoxaparin | 15 (1.0) | 14 (0.8) | 32 (0.6) | 25 (1.1) | 86 (0.8) |
UFH | 17 (1.2) | 10 (0.6) | 23 (0.4) | 35 (1.6) | 85 (0.8) |
Warfarin | 79 (5.4) | 302 (17.7) | 357 (6.2) | 116 (5.2) | 854 (7.7) |
Dalteparin | 0 | 0 | 2 (<0.1) | 1 (<0.1) | 3 (<0.1) |
Fondaparinux | 1 (0.1) | 0 | 0 | 2 (0.1) | 3 (<0.1) |
Antiplatelet therapy within 35 days after discharge | 72 (4.9) | 217 (12.7) | 351 (6.1) | 53 (2.4) | 693 (6.2) |
In the 35 days after discharge, 8.8% of patients received anticoagulation therapy, most commonly warfarin (7.7%). The rate of outpatient prophylaxis was highest in patients hospitalized for CHF (18.5%) compared with other medical conditions (7%).
Time Course of VTE Risk and Hazard Function
Overall, there were 366 symptomatic VTE events, representing a VTE rate of 3.3%. These events comprised 241 DVT‐only events, 98 PE‐only events, and 27 events with evidence of both DVT and PE. In total, 43.4% of events occurred during hospitalization (Figure 2). The VTE rate was 5.7% in patients with cancer (30.5% of events occurring in hospital), 4.3% with infectious disease (61.9% in hospital), 3.1% with CHF (54.7% in hospital), and 2.1% with severe lung disease/COPD (42.6% in hospital). The highest number of VTE events, 97 events (62 DVT only, 26 PE only, and 9 events both DVT and PE), occurred in the first 9 days after the hospital admission date, of which 87.6% were during hospitalization. During days 10‐19, there were 82 VTE events (50 DVT only, 24 PE only, and 8 both DVT and PE), 70.7% of which occurred in the hospital. Over the following 10‐day periods, VTE incidence gradually declined (Figure 2) and fluctuated at a background level of 4‐8 events during each 10‐day interval from 120 to 180 days.
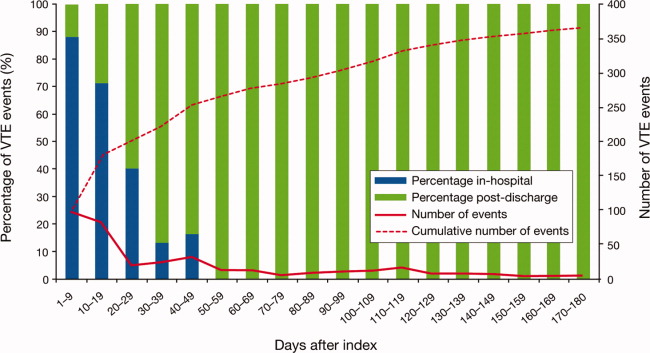
The cumulative probability of VTE among all patients was 0.035 (Figure 3A). Half of the VTE risk had accumulated by day 23, and 75% had accumulated by day 71. By day 30, the proportion of cumulative risk was 52.6% overall, and ranged from 41.9% with cancer to 72.9% with infectious disease (Figure 3).
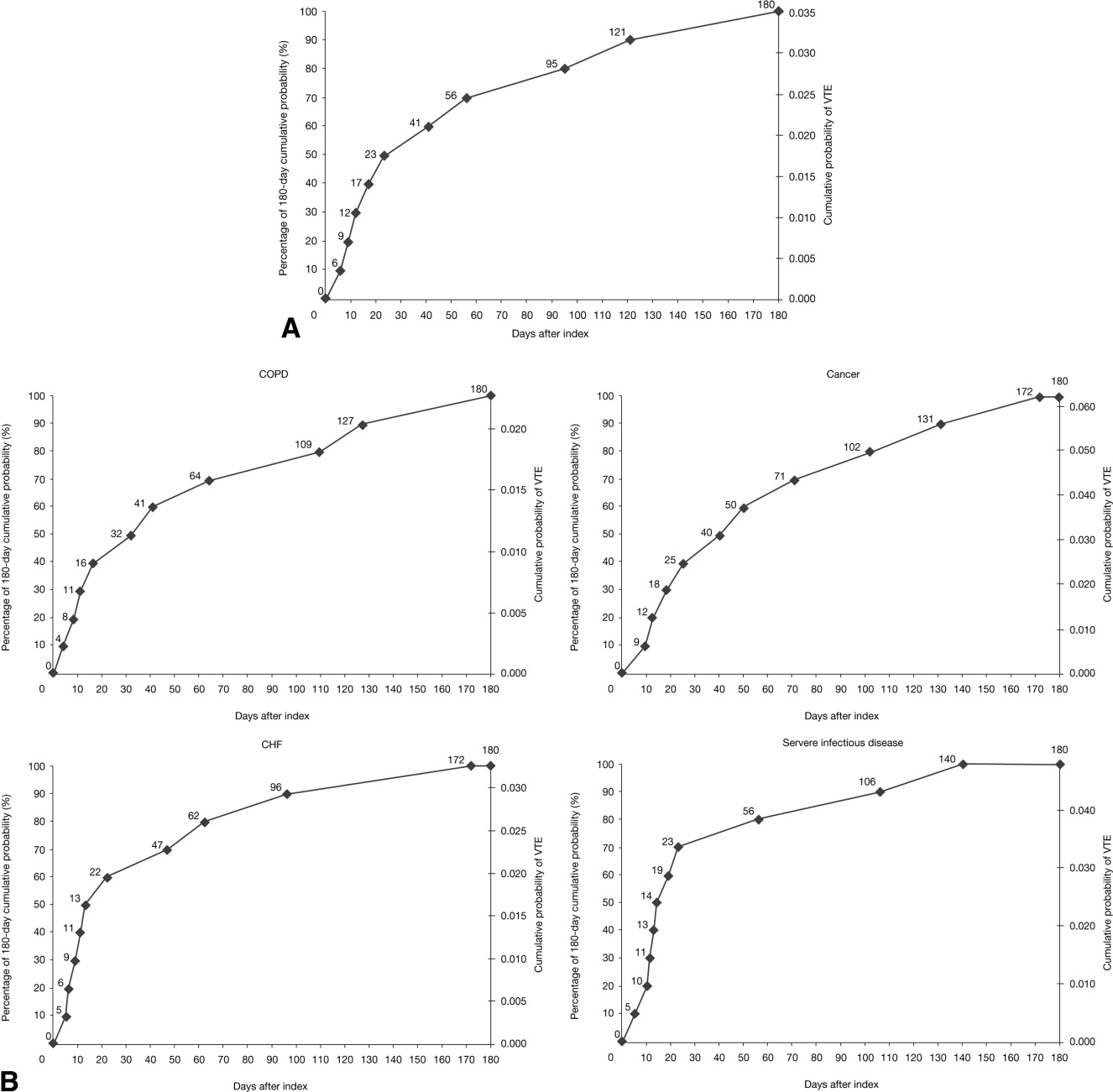
The VTE hazard peaked at approximately 1.05 VTE events per 1000 person‐days on day 8 after the hospital admission date overall (Figure 4A). The cumulative hazard at the peak day was 18.2% of the total VTE hazard over the 180‐day evaluation period. The hazard peak ranged from day 7 in patients with severe lung disease/COPD to day 12 in patients with infectious disease (Figure 4B). The cumulative hazard at the peak day was 39.7% for patients with infectious disease, 29.2% for patients with CHF, and approximately 19% for cancer or severe lung disease/COPD. After the peak risk day, the VTE hazard function decreased until the curve reached an inflection point, at day 28, when the cumulative risk was 51.8% (Figure 4A). After the inflection point, the VTE hazard increased to 0.3 VTE events per 1000 person‐days at approximately day 40‐45 and then decreased to <0.2 events per 1000 person‐days. The timing of the inflection varied by approximately 1 week across the medical illnesses (ranging from day 25 for severe lung disease/COPD to day 33 for CHF), with the cumulative risk at the inflection point ranging from 41.9% with cancer to 72.9% with infectious disease.
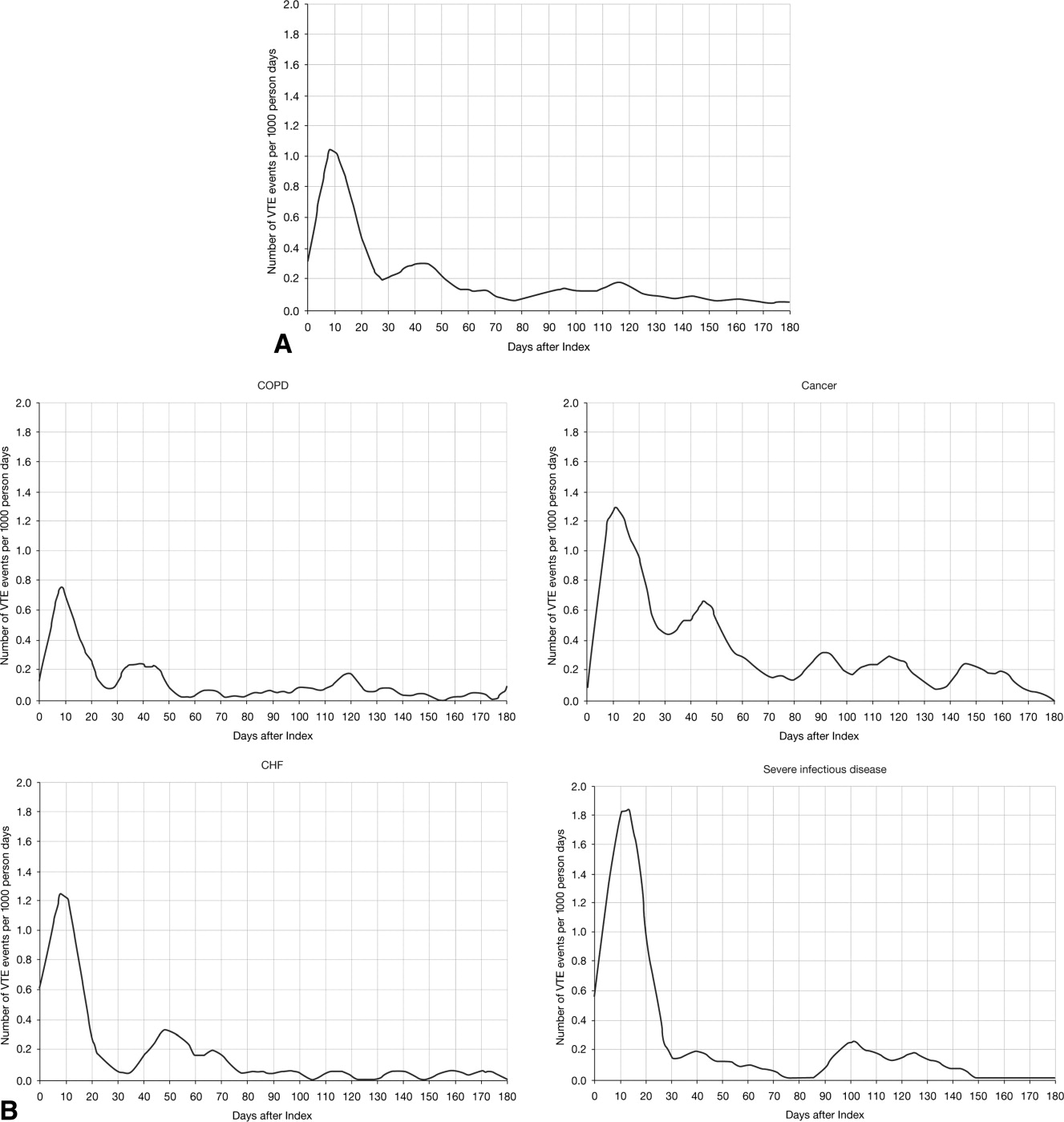
DISCUSSION
The results from this large, real‐world study provide new insights into the duration of risk of symptomatic VTE in medical patients and demonstrate that the number of VTE events was highest during days 0‐19, with the peak of VTE hazard at day 8. Half of the total 180‐day cumulative risk had been incurred by day 23 after hospital admission, and the period of greatest increased risk extended up to at least 30 days. Importantly, more than half of VTE events occurred after discharge (56.6%). A particularly high proportion of VTE events (69.5%) had occurred after discharge in patients with cancer. Although it was assumed that most VTE events that could be reasonably attributed to an at‐risk hospitalization would occur within 90 days as shown previously,4, 11 the 180‐day evaluation period was used to examine whether there was a prolonged period of continually diminished VTE risk from 90 to 180 days. Thus, events occurring within the later portions of the evaluation period may or may not have been attributable to the index hospitalization, potentially reflecting a background rate of VTE as noted above. Although these events are included in our estimate of the 180‐day cumulative risk of VTE, interpretation of the study results excluding such events is possible by examining the cumulative risk that had been incurred at each time point during the evaluation period.
Few other studies have assessed the duration of VTE risk in hospitalized medical patients. In a study by Spyropoulos et al,4 the median time to a DVT and/or PE event was 74 days, ranging from 62 days in severe infectious disease to 126 days in CHF. In another observational study that included patients who had recently been hospitalized but had not undergone surgery, 66.9% of patients who experienced DVT and/or PE events were diagnosed with DVT and/or PE within the first month after hospital discharge; 19.9% between months 1 and 2, and 13.2% between months 2 and 3.12
Fewer than half of the patients in the present study received thromboprophylaxis, which is consistent with other studies demonstrating the low prophylaxis rates in medical inpatients.9, 1315 In a recently published US study of discharge records that included 22,455 medical inpatients, prophylaxis rates were 59.4% in patients with CHF, 52.3% with cancer, 45.8% with severe lung disease/COPD, and 40.4% with infectious disease.14 Fewer than 10% of patients in the present study received prophylaxis after discharge, a result that is consistent with other studies.4, 9
The effect of extended prophylaxis in acutely ill medical patients with the LMWH enoxaparin beyond 6‐14 days has been investigated in the EXCLAIM study.16 This trial included approximately 5800 acutely ill medical patients at significant risk of developing VTE due to a recent reduction in mobility. Patients in the extended prophylaxis group had a lower risk of VTE (2.5% vs 4% for placebo; absolute risk reduction 1.5% [95.8% confidence interval 2.54% to 0.52%]), but had increased major bleeding events (0.8% vs 0.3% for placebo; absolute risk difference favoring placebo, 0.51% [95% confidence interval, 0.12% to 0.89%]). The patient populations with most benefit from an additional 28 days prophylaxis with enoxaparin, in addition to the usual short‐term prophylaxis of 10 days, were patients with restricted mobility (level 1; total bed rest/sedentary), elderly patients (age >75 years), and women. A limitation of the EXCLAIM trial is that estimates of efficacy and safety are difficult to interpret: after an interim analysis of adjudicated efficacy and safety outcomes, amendments were made to the original study protocol by changing eligibility criteria for patients with level 2 immobility (level 1 with bathroom privileges).16
The optimal duration of prophylaxis for medical patients has not been determined; prophylaxis is generally administered to at‐risk medical patients for the duration of hospitalization. In the current study, mean length of stay was 5.3 5.3 days overall. As hospital stays shorten, many medical patients who are prescribed inpatient prophylaxis alone are unlikely to receive the standard 6‐14 days of prophylaxis shown to be effective in clinical trials.68 Furthermore, the extended period of VTE risk in the present study and the finding that 56.6% of events occurred after discharge also suggest that current practices for inpatient prophylaxis alone may need to be evaluated.
This study reports real‐world data from a large, well‐defined population and obtains the incidence of symptomatic VTE events. Even though certain demographic data deviate from the national averagefor example, 74.1% of patients were treated in the South Census region, whereas this region is served by 37.6% of US hospitals17; 87.5% of hospitals had an urban location (compared with 60.1% of US hospitals18), and 85.4% of hospitals had a licensed bed size of at least 200 beds (compared with 28.2% of US hospitals, with the average US hospital having fewer than 100 beds19)these data may be beneficial in guiding policy and health care strategies for gaining understanding of the duration of risk for VTE.
Limitations of the study include characterization of the VTE risk period through examination of the cumulative risk and hazard of VTE across time, as the actual VTE risk period cannot be determined with exact precision. We used ICD‐9‐CM diagnosis coding to identify VTE. Since many cases of PE are asymptomatic and detected at autopsy,20 our approach may have missed such cases, as they would not have been recorded within the database. Furthermore, validation studies suggest that suboptimal specificity exists for ICD‐9‐CM diagnosis codes used to identify VTE.21 In an attempt to improve the specificity of our VTE identification algorithm, we required that post‐discharge VTE was recorded either during an emergency room or subsequent inpatient admission (which would be indicative of acute care for VTE) or on an outpatient claim with subsequent evidence of treatment for VTE. The true sensitivity and specificity of the VTE identification algorithms used for this study remain unknown, however, so the study findings should be interpreted in light of this limitation. The databases used for the analysis may not be representative of the US population as a whole; for example, this study used claims data from commercial and Medicare supplemental databases, which do not include Medicaid patients. Another limitation was that outpatient mechanical prophylaxis, such as graded compression stockings, was not captured due to over‐the‐counter availability. In addition, appropriateness of prophylaxis was not determined in this study, because these data could not be obtained from the claims database used. Further studies are warranted to obtain information on the incidence of VTE after hospitalization for medical illness in patients who received appropriate prophylaxis during hospitalization.
Finally, all dosages of a pharmacological agent were considered prophylactic only if a VTE event did not occur, with the exception of warfarin; any dose of warfarin was considered for prophylaxis, regardless of a VTE diagnosis. Warfarin may be used for purposes other than VTE prophylaxis (eg, prophylaxis for a thromboembolic cerebrovascular accident). The data source does not allow for identifying the exact reason for anticoagulation therapy with warfarin. Nonetheless, warfarin therapy will confer a decreased risk of VTE regardless of its purpose.
Results from this large cohort of medical patients indicate that symptomatic VTE risk is highest within the first 19 days after hospital admission (a period that may encompass both the duration of hospitalization as well as the period after discharge) with a considerable risk of VTE extending into the period after discharge. Receiving appropriate prophylaxis in‐hospital remains of great importance to prevent inpatient and likely post‐discharge VTE in patients with acute medical illness. In addition, given the time course of VTE events, with VTE incidence peaking at 8 days but with increased risk extending to 30 days, and the number of out‐of‐hospital VTE events incurred, the results of this study suggest that future research is warranted to investigate the risks and benefits of improving thromboprophylaxis practices in the period after hospitalization.
Acknowledgements
Funding Source: sanofi‐aventis U.S. provided funding to Thomson Reuters to perform this study. The authors received editorial/writing support from Tessa Hartog of Excerpta Medica in the preparation of the manuscript funded by sanofi‐aventis U.S.
Disclosure: Alpesh Amin has received research honorarium and is on the speakers bureau for sanofi‐aventis U.S. Otsuka Pharmaceutical, and Boehringer‐Ingelheim. Helen Varker, Nicole Princic, and Stephen Johnston are employees at Thomson Reuters, which received funding from sanofi‐aventis U.S. Jay Lin is an employee of Novosys Health, which received funding from sanofi‐aventis U.S. Stephen Thompson is an employee of sanofi‐aventis U.S.
Patients who are hospitalized for acute medical illness are at an increased risk of developing venous thromboembolism (VTE), which comprises deep‐vein thrombosis (DVT) and pulmonary embolism (PE).13 In a recent real‐world study of 158,325 US medical patients by Spyropoulos et al,4 4.0% of patients developed DVT, 1.5% developed PE, and 0.2% developed both DVT and PE. Furthermore, results from a population‐based case‐control study indicate that hospitalization for medical illness accounted for a proportion of VTE events similar to that of hospitalization for surgery (22% and 24%, respectively).5
Thromboprophylaxis reduces VTE incidence in at‐risk medical patients and is recommended according to evidence‐based guidelines from the American College of Chest Physicians (ACCP).1 The ACCP guidelines advocate that acutely ill medical patients admitted to the hospital with congestive heart failure (CHF) or severe lung disease/chronic obstructive pulmonary disease (COPD) or those who are confined to bed and have one or more additional risk factors (including active cancer, previous VTE, sepsis, acute neurologic disease, or inflammatory bowel disease) receive pharmacological prophylaxis with lowmolecular weight heparin (LMWH), low‐dose unfractionated heparin (UFH), or fondaparinux.1 Although guidelines provide recommendations for the duration of prophylaxis after major orthopedic surgery, such recommendations are unavailable for medical patients. In clinical trials of acutely ill medical patients, prophylaxis regimens found to be effective were provided for a duration of hospitalization of 6‐14 days.68 The mean length of hospital stay for medical illnesses is decreasing and is currently shorter than 6‐14 days.9, 10
In clinical practice, the duration of VTE risk during and after hospitalization is not well understood in medical patients, particularly in the context of shortening hospital stays. Such information could, however, provide insight into whether current thromboprophylaxis practices reflect real‐world need. To gain a greater understanding of the period during which patients are at risk of VTE, this retrospective, observational study assessed the incidence and time course of symptomatic VTE events during and after hospitalization in a large population of US medical patients.
METHODS
Data and Patient Selection
This study employed linked administrative claims data and hospital billing data contained in the Thomson Reuters MarketScan Inpatient Drug Link File. This combines longitudinal patient‐level inpatient and outpatient medical and pharmaceutical claims data from the MarketScan Commercial claims data from the MarketScan Commercial Claims and Encounters (Commercial) and Medicare Supplemental and Coordination of Benefits (Medicare Supplemental) databases, with hospital discharge records detailing services used and drugs administered during a hospitalization, which are included in the Hospital Drug Database. The linked data sources enable analysis of a patient's experience before, during, and after a hospitalization. The present study was not designed to obtain bleeding rates.
The study cohort comprised patients considered to be at‐risk for VTE as a result of a medical hospitalization occurring between January 1, 2005, and December 31, 2008. At‐risk medical hospitalizations were those for which the primary diagnosis was for cancer, CHF, severe lung disease/COPD, or infectious disease (see Supporting Information, Appendix I, for International Classification of Diseases, 9th Revision, Clinical Modification [ICD‐9‐CM] codes used to identify patients with medical illnesses). Included patients were required to be at least 18 years of age at the time of admission and were required to be continuously enrolled in their insurance benefits for at least 12 months before admission (the baseline period) and for at least 180 days after the admission date (the evaluation period) to ensure that all administrative claims data during that period were captured. Patients who died in‐hospital from any cause were exempted from the continuous enrollment criterion, as long as they had been continuously enrolled prior to inpatient death. Patients transferred from or discharged to another acute‐care facility were excluded because of the possibility for incomplete inpatient data capture. For patients who had multiple medical hospitalizations between January 1, 2005, and December 31, 2008, the hospitalization around which the analysis focused was randomly selected from the set of potential medical hospitalizations.
Prophylaxis
Pharmacological prophylaxis was identified via charge codes during hospitalization or via pharmacy claims after discharge for UFH, enoxaparin, dalteparin, warfarin, and fondaparinux. All dosages of a pharmacological agent were considered prophylactic only if there was no evidence of VTE during the admission, with the exception of warfarin (Supporting Information, Appendix II). Post‐discharge use of anticoagulation therapy was measured for up to 35 days after discharge from the hospital. Use of mechanical prophylaxis during hospitalization was identified via charge codes for graduated compression stockings and charge codes indicating use of intermittent pneumatic compression devices and/or venous foot pumps. The appropriateness of prophylaxis was not assessed.
Analysis
The risk of VTE was estimated across an evaluation period of 180 days by measuring VTE occurrence and person‐time exposure. Inpatient VTE occurrence was defined as any nonprimary diagnosis of DVT and/or PE during the at‐risk hospitalization. VTE after discharge was defined as an ICD‐9‐CM diagnosis code, whether primary or secondary, for DVT or PE in the evaluation period during an emergency room or inpatient admission, or on an outpatient claim with 1 or more of the following confirmatory events: an emergency room or inpatient admission for VTE within 2 days of the outpatient diagnosis; a prescription claim for enoxaparin, fondaparinux, or UFH within 15 days after diagnosis; or a prescription claim for warfarin within 15 days after diagnosis and no evidence of atrial fibrillation or atrial flutter in the 6 months before the outpatient diagnosis for DVT or PE. Person‐time exposure was measured as the number of days from the hospital admission date to the first occurrence of VTE, or censoring at a subsequent at‐risk hospitalization, death, or 180 days after admission.
Cumulative risk of VTE over the 180‐day evaluation period was calculated by the Kaplan‐Meier product limit method of survival analysis and displayed for deciles of cumulative risk at 180 days after the hospital admission date. The risk of VTE at each point of time during the evaluation period (the hazard function) was first calculated on a daily basis and then smoothed via LOESS regression, a locally weighted regression procedure.
RESULTS
Patient Demographics
A total of 11,139 medical patients were included in the analysis (Figure 1), with a mean standard deviation (SD) age of 67.6 13.9 years, and 51.6% were women (Table 1). Of the reasons for admission to the hospital, 51.5% of patients were admitted for severe lung disease/COPD, 20.1% were admitted for cancer, 15.3% were admitted for CHF, and 13.1% were admitted for severe infectious disease. Most patients were treated in an urban hospital (87.5%), in a hospital without teaching status (87.9%), and in the South Census region (74.1%). The majority of patients were treated in medium‐sized to large care facilities. Risk factors for VTE during the baseline period included hospitalization for a medical condition with a high risk for VTE (75.6%), a prior at‐risk hospitalization (18.6%), cancer therapy (10.0% of all medical patients combined and 18.5% of cancer patients), trauma (9.2%), and previous VTE (4.3%).
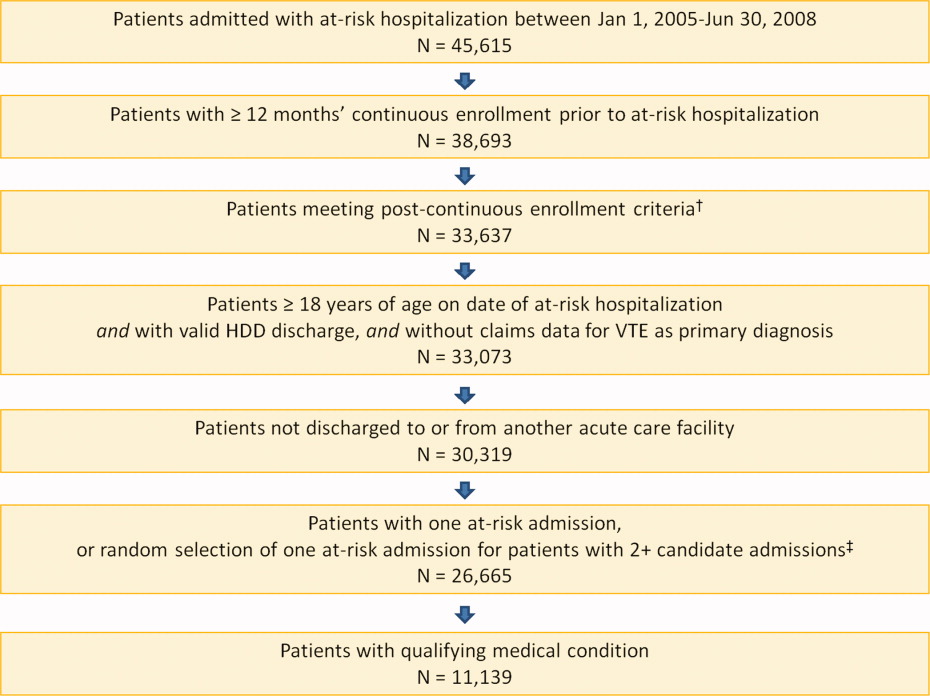
Characteristic | Medical Patients (N = 11,139) |
---|---|
| |
Gender | |
Men | 5389 (48.4) |
Women | 5750 (51.6) |
Reason for hospitalization | |
Cancer | 2243 (20.1) |
CHF | 1705 (15.3) |
Severe lung disease/COPD | 5736 (51.5) |
Severe infectious disease | 1455 (13.1) |
Age group, years | |
1834 | 230 (2.1) |
3544 | 442 (4.0) |
4554 | 1188 (10.7) |
5564 | 2644 (23.7) |
6574 | 2657 (23.9) |
7584 | 2969 (26.7) |
85 years | 1009 (9.1) |
Median age SD, years | 67.6 13.9 |
Primary payer* | |
Medicare | 6819 (61.2) |
Commercial | 4320 (38.8) |
Geographical area | |
Northeast | 122 (1.1) |
North Central | 2649 (23.8) |
South | 8258 (74.1) |
West | 110 (1.0) |
Urban location | 9743 (87.5) |
Teaching hospital | 1345 (12.1) |
Licensed bed size | |
1199 | 1621 (14.6) |
200299 | 2869 (25.8) |
300499 | 4005 (36.0) |
500 | 2644 (23.7) |
VTE Prophylaxis
Patients stayed in hospital for a mean SD duration of 5.3 5.3 days, varying from 4.6 3.9 days in patients with CHF to 6.7 6.5 days in patients with infectious disease, during which 46.7% of patients received pharmacological VTE prophylaxis. Inpatient pharmacological prophylaxis rates ranged from 64.1% in patients with CHF to 30.7% in patients with cancer (Table 2). Overall, the most commonly used form of inpatient pharmacological prophylaxis was enoxaparin (26.8% of all patients), followed by UFH (13.5% of all patients). Mechanical prophylaxis was received by 12.2% of all patients. Mean SD VTE prophylaxis duration during hospitalization was 5.0 4.7 days, varying from 4.2 4.0 days in patients with cancer to 6.2 5.5 days in patients with infectious disease.
n (%) | Infectious Disease (n = 1455) | CHF (n = 1705) | Severe Lung Disease/COPD (n = 5736) | Cancer (n = 2243) | Any Medical (N = 11,139) |
---|---|---|---|---|---|
| |||||
Pharmacological prophylaxis during hospitalization* | 599 (41.2) | 1093 (64.1) | 2820 (49.2) | 688 (30.7) | 5200 (46.7) |
Enoxaparin | 362 (24.9) | 466 (27.3) | 1877 (32.7) | 282 (12.6) | 2987 (26.8) |
UFH | 191 (13.1) | 400 (23.5) | 527 (9.2) | 383 (17.1) | 1501 (13.5) |
Warfarin | 135 (9.3) | 498 (29.2) | 622 (10.8) | 95 (4.2) | 1350 (12.1) |
Dalteparin | 16 (1.1) | 21 (1.2) | 109 (1.9) | 16 (0.7) | 162 (1.5) |
Fondaparinux | 5 (0.3) | 4 (0.2) | 22 (0.4) | 2 (0.1) | 33 (0.3) |
Mechanical prophylaxis in hospital | 148 (10.2) | 65 (3.8) | 343 (6.0) | 803 (35.8) | 1359 (12.2) |
Anticoagulation within 35 days after discharge | 104 (7.1) | 315 (18.5) | 397 (6.9) | 166 (7.4) | 982 (8.8) |
Enoxaparin | 15 (1.0) | 14 (0.8) | 32 (0.6) | 25 (1.1) | 86 (0.8) |
UFH | 17 (1.2) | 10 (0.6) | 23 (0.4) | 35 (1.6) | 85 (0.8) |
Warfarin | 79 (5.4) | 302 (17.7) | 357 (6.2) | 116 (5.2) | 854 (7.7) |
Dalteparin | 0 | 0 | 2 (<0.1) | 1 (<0.1) | 3 (<0.1) |
Fondaparinux | 1 (0.1) | 0 | 0 | 2 (0.1) | 3 (<0.1) |
Antiplatelet therapy within 35 days after discharge | 72 (4.9) | 217 (12.7) | 351 (6.1) | 53 (2.4) | 693 (6.2) |
In the 35 days after discharge, 8.8% of patients received anticoagulation therapy, most commonly warfarin (7.7%). The rate of outpatient prophylaxis was highest in patients hospitalized for CHF (18.5%) compared with other medical conditions (7%).
Time Course of VTE Risk and Hazard Function
Overall, there were 366 symptomatic VTE events, representing a VTE rate of 3.3%. These events comprised 241 DVT‐only events, 98 PE‐only events, and 27 events with evidence of both DVT and PE. In total, 43.4% of events occurred during hospitalization (Figure 2). The VTE rate was 5.7% in patients with cancer (30.5% of events occurring in hospital), 4.3% with infectious disease (61.9% in hospital), 3.1% with CHF (54.7% in hospital), and 2.1% with severe lung disease/COPD (42.6% in hospital). The highest number of VTE events, 97 events (62 DVT only, 26 PE only, and 9 events both DVT and PE), occurred in the first 9 days after the hospital admission date, of which 87.6% were during hospitalization. During days 10‐19, there were 82 VTE events (50 DVT only, 24 PE only, and 8 both DVT and PE), 70.7% of which occurred in the hospital. Over the following 10‐day periods, VTE incidence gradually declined (Figure 2) and fluctuated at a background level of 4‐8 events during each 10‐day interval from 120 to 180 days.
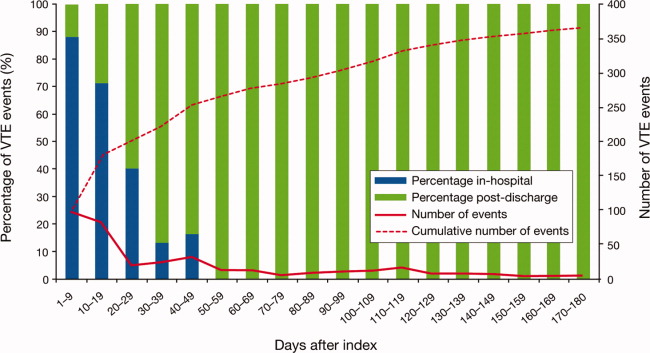
The cumulative probability of VTE among all patients was 0.035 (Figure 3A). Half of the VTE risk had accumulated by day 23, and 75% had accumulated by day 71. By day 30, the proportion of cumulative risk was 52.6% overall, and ranged from 41.9% with cancer to 72.9% with infectious disease (Figure 3).
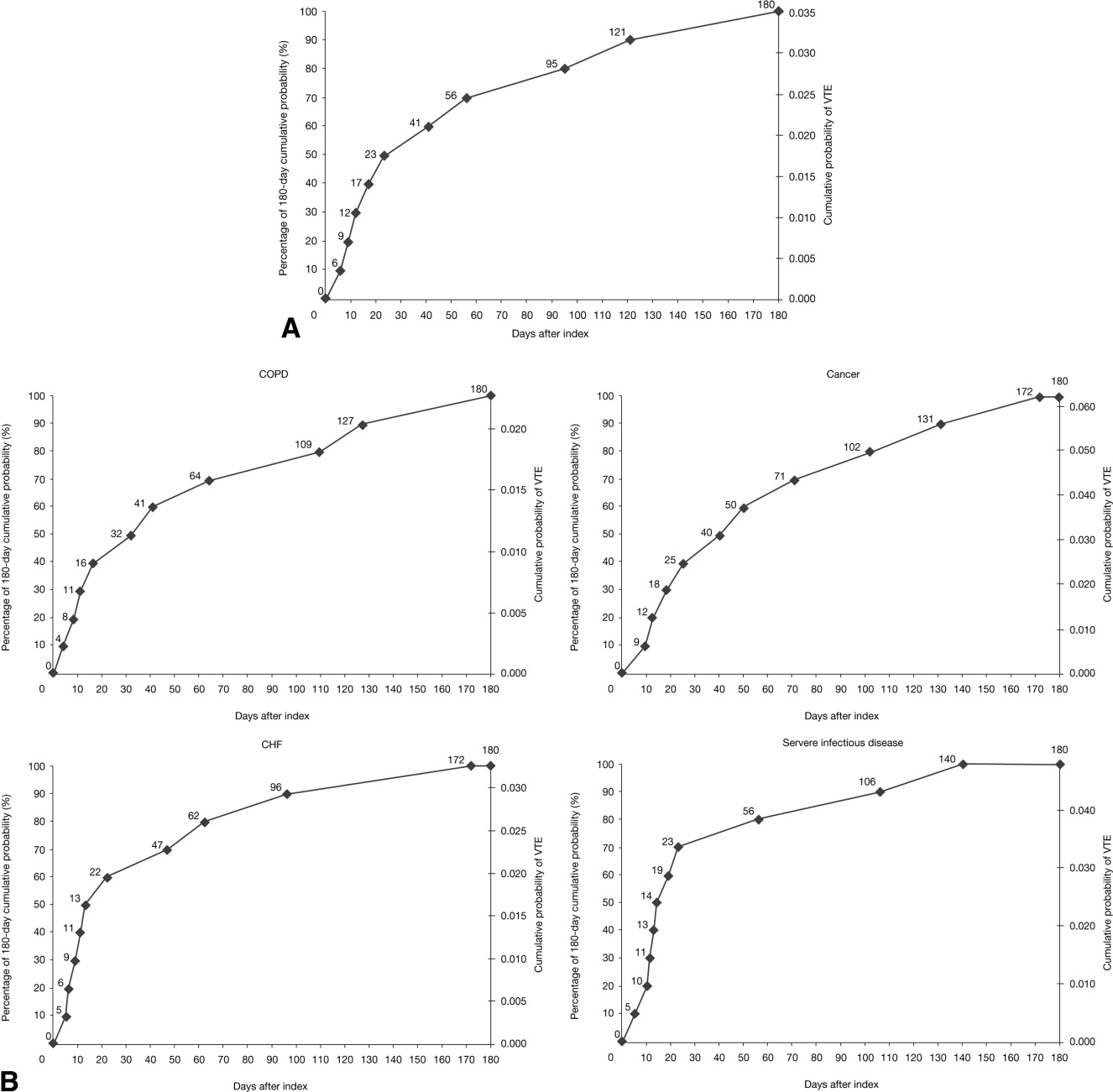
The VTE hazard peaked at approximately 1.05 VTE events per 1000 person‐days on day 8 after the hospital admission date overall (Figure 4A). The cumulative hazard at the peak day was 18.2% of the total VTE hazard over the 180‐day evaluation period. The hazard peak ranged from day 7 in patients with severe lung disease/COPD to day 12 in patients with infectious disease (Figure 4B). The cumulative hazard at the peak day was 39.7% for patients with infectious disease, 29.2% for patients with CHF, and approximately 19% for cancer or severe lung disease/COPD. After the peak risk day, the VTE hazard function decreased until the curve reached an inflection point, at day 28, when the cumulative risk was 51.8% (Figure 4A). After the inflection point, the VTE hazard increased to 0.3 VTE events per 1000 person‐days at approximately day 40‐45 and then decreased to <0.2 events per 1000 person‐days. The timing of the inflection varied by approximately 1 week across the medical illnesses (ranging from day 25 for severe lung disease/COPD to day 33 for CHF), with the cumulative risk at the inflection point ranging from 41.9% with cancer to 72.9% with infectious disease.
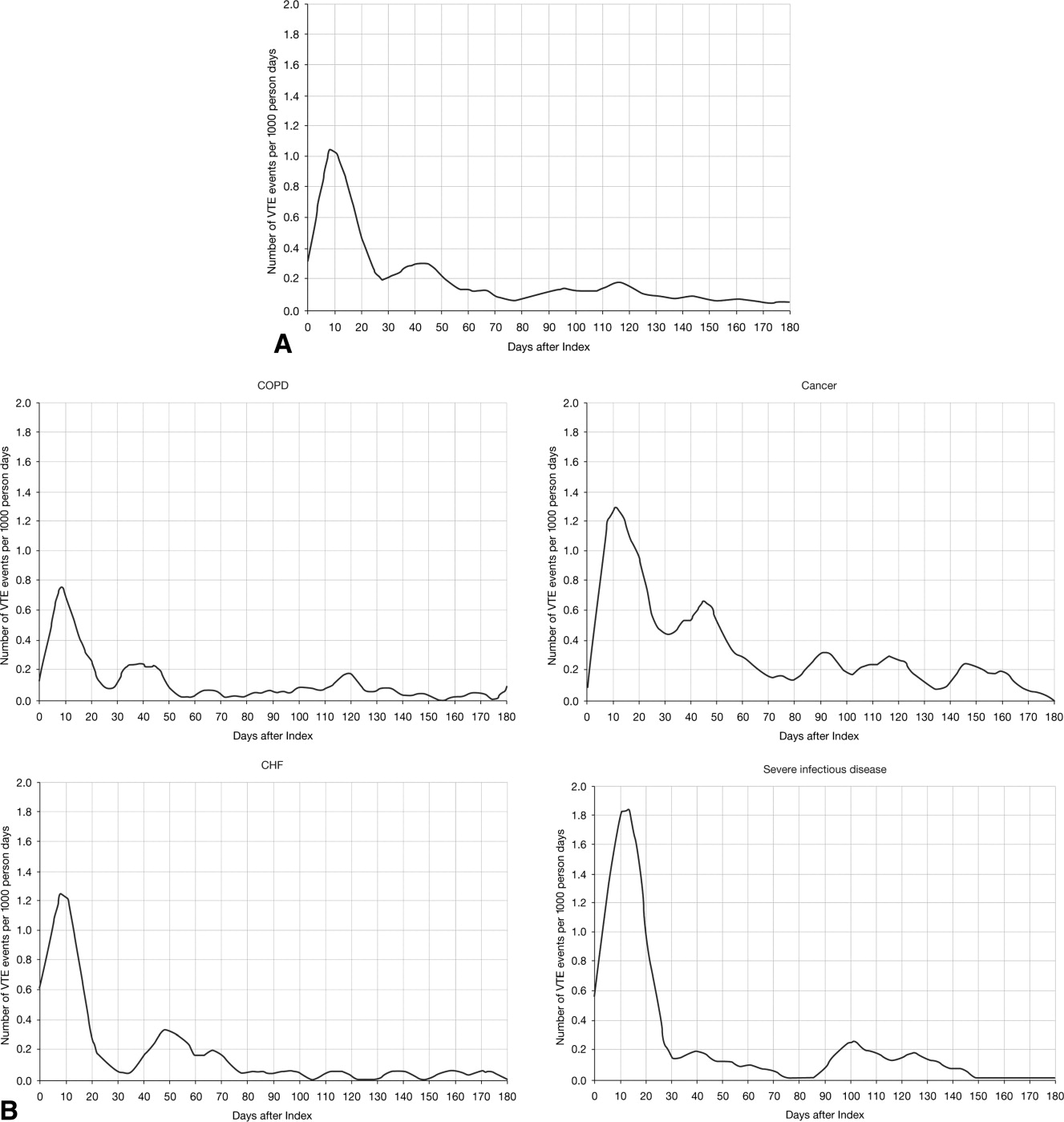
DISCUSSION
The results from this large, real‐world study provide new insights into the duration of risk of symptomatic VTE in medical patients and demonstrate that the number of VTE events was highest during days 0‐19, with the peak of VTE hazard at day 8. Half of the total 180‐day cumulative risk had been incurred by day 23 after hospital admission, and the period of greatest increased risk extended up to at least 30 days. Importantly, more than half of VTE events occurred after discharge (56.6%). A particularly high proportion of VTE events (69.5%) had occurred after discharge in patients with cancer. Although it was assumed that most VTE events that could be reasonably attributed to an at‐risk hospitalization would occur within 90 days as shown previously,4, 11 the 180‐day evaluation period was used to examine whether there was a prolonged period of continually diminished VTE risk from 90 to 180 days. Thus, events occurring within the later portions of the evaluation period may or may not have been attributable to the index hospitalization, potentially reflecting a background rate of VTE as noted above. Although these events are included in our estimate of the 180‐day cumulative risk of VTE, interpretation of the study results excluding such events is possible by examining the cumulative risk that had been incurred at each time point during the evaluation period.
Few other studies have assessed the duration of VTE risk in hospitalized medical patients. In a study by Spyropoulos et al,4 the median time to a DVT and/or PE event was 74 days, ranging from 62 days in severe infectious disease to 126 days in CHF. In another observational study that included patients who had recently been hospitalized but had not undergone surgery, 66.9% of patients who experienced DVT and/or PE events were diagnosed with DVT and/or PE within the first month after hospital discharge; 19.9% between months 1 and 2, and 13.2% between months 2 and 3.12
Fewer than half of the patients in the present study received thromboprophylaxis, which is consistent with other studies demonstrating the low prophylaxis rates in medical inpatients.9, 1315 In a recently published US study of discharge records that included 22,455 medical inpatients, prophylaxis rates were 59.4% in patients with CHF, 52.3% with cancer, 45.8% with severe lung disease/COPD, and 40.4% with infectious disease.14 Fewer than 10% of patients in the present study received prophylaxis after discharge, a result that is consistent with other studies.4, 9
The effect of extended prophylaxis in acutely ill medical patients with the LMWH enoxaparin beyond 6‐14 days has been investigated in the EXCLAIM study.16 This trial included approximately 5800 acutely ill medical patients at significant risk of developing VTE due to a recent reduction in mobility. Patients in the extended prophylaxis group had a lower risk of VTE (2.5% vs 4% for placebo; absolute risk reduction 1.5% [95.8% confidence interval 2.54% to 0.52%]), but had increased major bleeding events (0.8% vs 0.3% for placebo; absolute risk difference favoring placebo, 0.51% [95% confidence interval, 0.12% to 0.89%]). The patient populations with most benefit from an additional 28 days prophylaxis with enoxaparin, in addition to the usual short‐term prophylaxis of 10 days, were patients with restricted mobility (level 1; total bed rest/sedentary), elderly patients (age >75 years), and women. A limitation of the EXCLAIM trial is that estimates of efficacy and safety are difficult to interpret: after an interim analysis of adjudicated efficacy and safety outcomes, amendments were made to the original study protocol by changing eligibility criteria for patients with level 2 immobility (level 1 with bathroom privileges).16
The optimal duration of prophylaxis for medical patients has not been determined; prophylaxis is generally administered to at‐risk medical patients for the duration of hospitalization. In the current study, mean length of stay was 5.3 5.3 days overall. As hospital stays shorten, many medical patients who are prescribed inpatient prophylaxis alone are unlikely to receive the standard 6‐14 days of prophylaxis shown to be effective in clinical trials.68 Furthermore, the extended period of VTE risk in the present study and the finding that 56.6% of events occurred after discharge also suggest that current practices for inpatient prophylaxis alone may need to be evaluated.
This study reports real‐world data from a large, well‐defined population and obtains the incidence of symptomatic VTE events. Even though certain demographic data deviate from the national averagefor example, 74.1% of patients were treated in the South Census region, whereas this region is served by 37.6% of US hospitals17; 87.5% of hospitals had an urban location (compared with 60.1% of US hospitals18), and 85.4% of hospitals had a licensed bed size of at least 200 beds (compared with 28.2% of US hospitals, with the average US hospital having fewer than 100 beds19)these data may be beneficial in guiding policy and health care strategies for gaining understanding of the duration of risk for VTE.
Limitations of the study include characterization of the VTE risk period through examination of the cumulative risk and hazard of VTE across time, as the actual VTE risk period cannot be determined with exact precision. We used ICD‐9‐CM diagnosis coding to identify VTE. Since many cases of PE are asymptomatic and detected at autopsy,20 our approach may have missed such cases, as they would not have been recorded within the database. Furthermore, validation studies suggest that suboptimal specificity exists for ICD‐9‐CM diagnosis codes used to identify VTE.21 In an attempt to improve the specificity of our VTE identification algorithm, we required that post‐discharge VTE was recorded either during an emergency room or subsequent inpatient admission (which would be indicative of acute care for VTE) or on an outpatient claim with subsequent evidence of treatment for VTE. The true sensitivity and specificity of the VTE identification algorithms used for this study remain unknown, however, so the study findings should be interpreted in light of this limitation. The databases used for the analysis may not be representative of the US population as a whole; for example, this study used claims data from commercial and Medicare supplemental databases, which do not include Medicaid patients. Another limitation was that outpatient mechanical prophylaxis, such as graded compression stockings, was not captured due to over‐the‐counter availability. In addition, appropriateness of prophylaxis was not determined in this study, because these data could not be obtained from the claims database used. Further studies are warranted to obtain information on the incidence of VTE after hospitalization for medical illness in patients who received appropriate prophylaxis during hospitalization.
Finally, all dosages of a pharmacological agent were considered prophylactic only if a VTE event did not occur, with the exception of warfarin; any dose of warfarin was considered for prophylaxis, regardless of a VTE diagnosis. Warfarin may be used for purposes other than VTE prophylaxis (eg, prophylaxis for a thromboembolic cerebrovascular accident). The data source does not allow for identifying the exact reason for anticoagulation therapy with warfarin. Nonetheless, warfarin therapy will confer a decreased risk of VTE regardless of its purpose.
Results from this large cohort of medical patients indicate that symptomatic VTE risk is highest within the first 19 days after hospital admission (a period that may encompass both the duration of hospitalization as well as the period after discharge) with a considerable risk of VTE extending into the period after discharge. Receiving appropriate prophylaxis in‐hospital remains of great importance to prevent inpatient and likely post‐discharge VTE in patients with acute medical illness. In addition, given the time course of VTE events, with VTE incidence peaking at 8 days but with increased risk extending to 30 days, and the number of out‐of‐hospital VTE events incurred, the results of this study suggest that future research is warranted to investigate the risks and benefits of improving thromboprophylaxis practices in the period after hospitalization.
Acknowledgements
Funding Source: sanofi‐aventis U.S. provided funding to Thomson Reuters to perform this study. The authors received editorial/writing support from Tessa Hartog of Excerpta Medica in the preparation of the manuscript funded by sanofi‐aventis U.S.
Disclosure: Alpesh Amin has received research honorarium and is on the speakers bureau for sanofi‐aventis U.S. Otsuka Pharmaceutical, and Boehringer‐Ingelheim. Helen Varker, Nicole Princic, and Stephen Johnston are employees at Thomson Reuters, which received funding from sanofi‐aventis U.S. Jay Lin is an employee of Novosys Health, which received funding from sanofi‐aventis U.S. Stephen Thompson is an employee of sanofi‐aventis U.S.
- Prevention of venous thromboembolism: American College of Chest Physicians Evidence‐Based Clinical Practice Guidelines (8th Edition). Chest. 2008;133(6 Suppl):381S–453S. , , , et al.
- Risk factors for deep vein thrombosis and pulmonary embolism: a population‐based case‐control study. Arch Intern Med. 2000;160:809–815. , , , , , .
- The epidemiology of venous thromboembolism in the community. Arterioscler Thromb Vasc Biol. 2008;28:370–372. .
- Rates of venous thromboembolism occurrence in medical patients among the insured population. Thromb Haemost. 2009;102:951–957. , , , .
- Relative impact of risk factors for deep vein thrombosis and pulmonary embolism: a population‐based study. Arch Intern Med. 2002;162:1245–1248. , , , et al.
- A comparison of enoxaparin with placebo for the prevention of venous thromboembolism in acutely ill medical patients. Prophylaxis in Medical Patients with Enoxaparin Study Group. N Engl J Med. 1999;341:793–800. , , , et al.
- Randomized, placebo‐controlled trial of dalteparin for the prevention of venous thromboembolism in acutely ill medical patients. Circulation. 2004;110:874–879. , , , et al.
- Efficacy and safety of fondaparinux for the prevention of venous thromboembolism in older acute medical patients: randomised placebo controlled trial. BMJ. 2006;332:325–329. , , , et al.
- Lack of thromboprophylaxis across the care continuum in US medical patients. Hosp Pract (Minneap). 2010;38:17–25. , , .
- HCUP NIS Related Reports. Healthcare Cost and Utilization Project (HCUP), September 2008. Available at: www.hcup‐us.ahrq.gov/db/nation/nis/nisrelatedreports.jsp. Accessed June 2011.
- Direct medical costs of venous thromboembolism and subsequent hospital readmission rates: an administrative claims analysis from 30 managed care organizations. J Manag Care Pharm. 2007;13:475–486. , .
- Venous thromboembolism in the outpatient setting. Arch Intern Med. 2007;167:1471–1475. , , , , .
- Inpatient thromboprophylaxis use in U.S. hospitals: adherence to the seventh American College of Chest Physician's recommendations for at‐risk medical and surgical patients. J Hosp Med. 2009;4:E15–E21. , , , .
- Are hospitals delivering appropriate VTE prevention? The venous thromboembolism study to assess the rate of thromboprophylaxis (VTE start). J Thromb Thrombolysis. 2010;29:326–339. , , , et al.
- Venous thromboembolism risk and prophylaxis in hospitalised medically ill patients. The ENDORSE Global Survey. Thromb Haemost. 2010;103:736–748. , , , et al.
- Extended‐duration venous thromboembolism prophylaxis in acutely ill medical patients with recently reduced mobility: a randomized trial. Ann Intern Med. 2010;153:8–18. , , , et al.
- American Society for Healthcare Engineering of the American Hospital Association. Overview of the Hospital Market, 2009. Available from: www.ashe.org/e2c/pdfs/energy/heg_ch2_background.pdf. Accessed June 2011.
- American Hospital Association. Fast Facts on US Hospitals, 2009. Available at: http://www.aha.org/aha/resource‐center/Statistics‐and‐Studies/fast‐facts.html. Accessed June 2011.
- American Hospital Association. AHA Annual Survey of Hospitals Database, 2009. Available from: http://www.ahadata.com/ahadata_app/index.jsp. Accessed June 2011.
- The epidemiology of venous thromboembolism. Circulation. 2003;107(23 suppl 1):I4–I8. .
- How valid is the ICD‐9‐CM based AHRQ patient safety indicator for postoperative venous thromboembolism? Med Care. 2009;47:1237–1243. , , , et al.
- Prevention of venous thromboembolism: American College of Chest Physicians Evidence‐Based Clinical Practice Guidelines (8th Edition). Chest. 2008;133(6 Suppl):381S–453S. , , , et al.
- Risk factors for deep vein thrombosis and pulmonary embolism: a population‐based case‐control study. Arch Intern Med. 2000;160:809–815. , , , , , .
- The epidemiology of venous thromboembolism in the community. Arterioscler Thromb Vasc Biol. 2008;28:370–372. .
- Rates of venous thromboembolism occurrence in medical patients among the insured population. Thromb Haemost. 2009;102:951–957. , , , .
- Relative impact of risk factors for deep vein thrombosis and pulmonary embolism: a population‐based study. Arch Intern Med. 2002;162:1245–1248. , , , et al.
- A comparison of enoxaparin with placebo for the prevention of venous thromboembolism in acutely ill medical patients. Prophylaxis in Medical Patients with Enoxaparin Study Group. N Engl J Med. 1999;341:793–800. , , , et al.
- Randomized, placebo‐controlled trial of dalteparin for the prevention of venous thromboembolism in acutely ill medical patients. Circulation. 2004;110:874–879. , , , et al.
- Efficacy and safety of fondaparinux for the prevention of venous thromboembolism in older acute medical patients: randomised placebo controlled trial. BMJ. 2006;332:325–329. , , , et al.
- Lack of thromboprophylaxis across the care continuum in US medical patients. Hosp Pract (Minneap). 2010;38:17–25. , , .
- HCUP NIS Related Reports. Healthcare Cost and Utilization Project (HCUP), September 2008. Available at: www.hcup‐us.ahrq.gov/db/nation/nis/nisrelatedreports.jsp. Accessed June 2011.
- Direct medical costs of venous thromboembolism and subsequent hospital readmission rates: an administrative claims analysis from 30 managed care organizations. J Manag Care Pharm. 2007;13:475–486. , .
- Venous thromboembolism in the outpatient setting. Arch Intern Med. 2007;167:1471–1475. , , , , .
- Inpatient thromboprophylaxis use in U.S. hospitals: adherence to the seventh American College of Chest Physician's recommendations for at‐risk medical and surgical patients. J Hosp Med. 2009;4:E15–E21. , , , .
- Are hospitals delivering appropriate VTE prevention? The venous thromboembolism study to assess the rate of thromboprophylaxis (VTE start). J Thromb Thrombolysis. 2010;29:326–339. , , , et al.
- Venous thromboembolism risk and prophylaxis in hospitalised medically ill patients. The ENDORSE Global Survey. Thromb Haemost. 2010;103:736–748. , , , et al.
- Extended‐duration venous thromboembolism prophylaxis in acutely ill medical patients with recently reduced mobility: a randomized trial. Ann Intern Med. 2010;153:8–18. , , , et al.
- American Society for Healthcare Engineering of the American Hospital Association. Overview of the Hospital Market, 2009. Available from: www.ashe.org/e2c/pdfs/energy/heg_ch2_background.pdf. Accessed June 2011.
- American Hospital Association. Fast Facts on US Hospitals, 2009. Available at: http://www.aha.org/aha/resource‐center/Statistics‐and‐Studies/fast‐facts.html. Accessed June 2011.
- American Hospital Association. AHA Annual Survey of Hospitals Database, 2009. Available from: http://www.ahadata.com/ahadata_app/index.jsp. Accessed June 2011.
- The epidemiology of venous thromboembolism. Circulation. 2003;107(23 suppl 1):I4–I8. .
- How valid is the ICD‐9‐CM based AHRQ patient safety indicator for postoperative venous thromboembolism? Med Care. 2009;47:1237–1243. , , , et al.
Copyright © 2011 Society of Hospital Medicine
Economic Impact of Enoxaparin in Stroke
Venous thromboembolism (VTE), which encompasses both deep‐vein thrombosis (DVT) and pulmonary embolism (PE), is a major health problem in the United States and worldwide. It represents one of the most significant causes of morbidity and mortality with an estimated 300,000 VTE‐related deaths,1 and 300,000‐600,000 hospitalizations in the United States annually.2 Hospitalization for medical illness is associated with a similar proportion of VTE cases as hospitalization for surgery.3 Several groups of medical patients have been shown to be at an increased risk of VTE, including those with cancer, severe respiratory disease, acute infectious illness, heart failure, myocardial infarction, and acute ischemic stroke.47 Ischemic stroke patients represent approximately 4.6% of medical patients at high risk of VTE in US hospitals.8 The incidence of DVT in such patients has been reported to be as high as 75%9 and PE has been reported to be responsible for up to 25% of early deaths after stroke.10
Several studies have demonstrated the efficacy of unfractionated heparin (UFH) or a low‐molecular‐weight heparin (LMWH) in the prevention of VTE in stroke patients, and have demonstrated that LMWHs are at least as effective as UFH.1114 The open‐label, randomized Prevention of VTE after acute ischemic stroke with LMWH and UFH (PREVAIL) trial demonstrated that in patients with acute ischemic stroke, prophylaxis for 10 days with the LMWH enoxaparin reduces the risk of VTE by 43% compared with UFH (10.2% vs 18.1%, respectively; relative risk = 0.57; 95% confidence interval [CI] = 0.44‐0.76; P = 0.0001) without increasing the incidence of overall bleeding events (7.9% vs 8.1%, respectively; P = 0.83), or the composite of symptomatic intracranial and major extracranial hemorrhage (1% in each group; P = 0.23). There was, however, a slight but significant increase in major extracranial hemorrhage alone with enoxaparin (1% vs 0%; P = 0.015).14 Evidence‐based guidelines from the American College of Chest Physicians (ACCP) provide recommendations for appropriate thromboprophylaxis regimens for patients at risk of VTE.15 Thromboprophylaxis with UFH, LMWH, and, more recently, fondaparinux is recommended for medical patients admitted to hospital with congestive heart failure or severe respiratory disease, or those who are confined to bed and have one or more additional risk factors, including active cancer, previous VTE, or acute neurologic disease.15 Similarly, in the Eighth ACCP Clinical Practice Guidelines, low‐dose UFH or LMWH are recommended for VTE prevention in patients with ischemic stroke who have restricted mobility.16
VTE is also associated with a substantial economic burden on the healthcare system, costing an estimated $1.5 billion annually in the United States.17 Thromboprophylaxis has been shown to be a cost‐effective strategy in hospitalized medical patients. Prophylaxis with a LMWH has been shown to be more cost‐effective than UFH in these patients.1821
However, despite the clinical and economic benefits, prophylaxis is still commonly underused in medical patients.22, 23 In surgical patients, the Surgical Care Improvement Project (SCIP) focuses on reducing surgical complications, and has endorsed 2 measures: VTE‐1, relating to the proportion of patients for whom VTE prophylaxis is ordered; and VTE‐2, relating to those who receive the recommended regimen (
The objective of the current study was to determine the economic impact, in terms of hospital costs, of enoxaparin compared with UFH for VTE prophylaxis after acute ischemic stroke. A decision‐analytic model was constructed using data from the PREVAIL study and historical inpatient data from a multi‐hospital database.
METHODS
In this study, the cost implications, from the hospital perspective, of VTE prophylaxis with enoxaparin or UFH in patients with acute ischemic stroke, were determined using a decision‐analytic model in TreeAge Pro Suite (TreeAge Software, Inc., Williamstown, MA, USA). The decision‐tree was based on 3 stages: (a) whether patients received enoxaparin or UFH; (b) how patients were classified according to their National Institutes of Health Stroke Scale (NIHSS) classification scores (<14 or 14); and (c) which clinical event each patient experienced, as defined per the PREVAIL trial (DVT, PE, intracranial hemorrhage, major extracranial hemorrhage, and minor extracranial hemorrhage) (Figure 1). The time horizon for the model was established at 90 days to mirror the length of follow‐up in the PREVAIL trial.
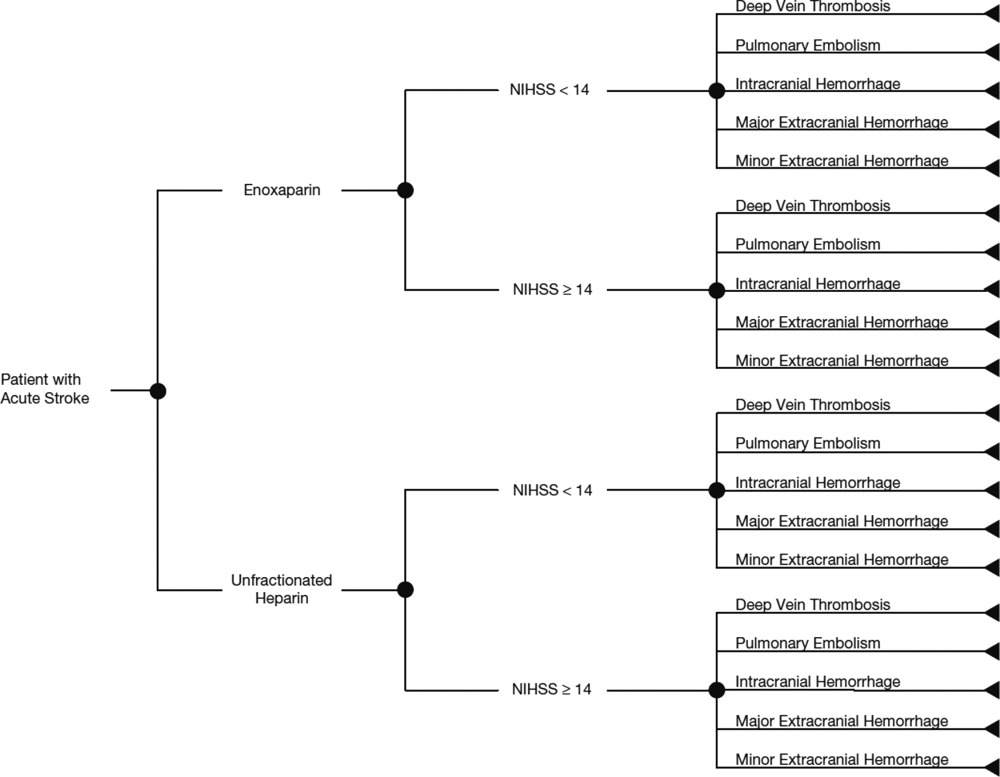
Total hospital costs were calculated based on clinical event rates (from the PREVAIL trial) and the costs of each clinical event, which were calculated separately according to the descriptions below, and then inserted into the decision‐analytic model. The clinical event rates were calculated from the efficacy and safety endpoints collected in the PREVAIL trial, and included VTE events (DVT and PE) and bleeding events (intracranial hemorrhage, major extracranial hemorrhage, and minor extracranial hemorrhage). Details of the patient population, eligibility criteria, and treatment regimen have previously been published in full elsewhere.14, 27
The costs of clinical events during hospitalization were estimated using a multivariate cost‐evaluation model, based on mean hospital costs for the events in the (Premier Inc., Charlotte, NC, USA) multi‐hospital database, one of the largest US hospital clinical and economic databases. The data are received from over 600 hospitals, representing all geographical areas of the United States, a broad range of bed sizes, teaching and non‐teaching, and urban and rural facilities. This database contains detailed US inpatient care records of principal and secondary diagnoses, inpatient procedures, administered laboratory tests, dispensed drugs, and demographic information. The evaluation of hospital cost for each type of clinical event was conducted by i3 Innovus (Ingenix, Inc., Eden Prairie, MN, USA). Total hospital costs were cumulative from all events, so if patients experienced multiple clinical events, the costs of the events were additive. The cost for stroke treatment and management was not included because it is an inclusion criterion of the PREVAIL trial and, thus, all patients in the trial have such costs.
Default drug costs were taken from the 2008 US wholesalers' acquisition cost data. The default dosing schedule is based on information extracted from the PREVAIL trial: enoxaparin 40 mg (once‐daily) and UFH 5000 U (twice‐daily) for 10 days each ($25.97 and $2.97, respectively). A drug‐administration fee was added for each dose of either enoxaparin or UFH ($10 for each).19
The estimated hospital cost of clinical events, along with drug costs, were inserted into the decision‐analytic model in TreeAge Pro Suite to estimate the cost per discharge from the hospital perspective in patients with ischemic stroke receiving VTE prophylaxis with enoxaparin or UFH. An additional analysis was performed to investigate the costs and cost differences in patients with less severe stroke (NIHSS scores <14) and more severe stroke (NIHSS scores 14).
Sensitivity analyses were performed to examine the impact of varying the cost inputs on the total hospital cost of each treatment arm by 5%, 10%, 15%, 20%, 30%, and 40%, and the robustness in the difference in costs between the enoxaparin and UFH groups. Univariate (via tornado diagram in TreeAge Pro Suite) and multivariate (via Monte Carlo simulation in TreeAge Pro Suite) analyses were performed. For the univariate analysis, each clinical event cost was adjusted individually, increasing or decreasing by 5%, 10%, 15%, 20%, 30% and 40% while other parameters remained unchanged. For the Monte Carlo simulation (TreeAge Pro Suite), all the parameters were simultaneously varied in a random fashion, within a range of 5%, 10%, 15%, 20%, 30%, and 40% over 10,000 trials. The simulation adopted a gamma distribution assumption for input sampling for cost parameters and a beta distribution for the event probability parameters. The confidence intervals for the probability parameters were obtained from the PREVAIL trial. The differences between the enoxaparin and UFH treatment groups were plotted in a graph against the variation in costs of each clinical event.
RESULTS
The clinical VTE and bleeding event rates as collected from the PREVAIL trial are shown in Table 1. The hospital costs per clinical event are shown in Table 2. The most costly clinical event from the hospital perspective was intracranial hemorrhage at $4001, followed by major extracranial hemorrhage at $3534. The costs of DVT and PE were $3003 and $2143, respectively.
Event Rate | 95% CI | |
---|---|---|
| ||
Enoxaparin (NIHSS <14) | ||
Deep‐vein thrombosis | 0.081 | 0.05730.1048 |
Pulmonary embolism | 0.002 | 0.000050.011 |
Intracranial hemorrhage | 0.0031 | 00.0074 |
Major extracranial hemorrhage | 0.0047 | 00.0099 |
Minor hemorrhage | 0.0372 | 0.0240.0549 |
Enoxaparin (NIHSS 14) | ||
Deep‐vein thrombosis | 0.1625 | 0.10530.2197 |
Pulmonary embolism | 0 | 00 |
Intracranial hemorrhage | 0.0086 | 00.0205 |
Major extracranial hemorrhage | 0.0172 | 0.04660.1198 |
Minor hemorrhage | 0.0776 | 0.04660.1198 |
UFH (NIHSS <14) | ||
Deep‐vein thrombosis | 0.1356 | 0.10540.1658 |
Pulmonary embolism | 0.004 | 0.00050.0145 |
Intracranial hemorrhage | 0.0032 | 00.0077 |
Major extracranial hemorrhage | 0 | 00 |
Minor hemorrhage | 0.0514 | 0.03550.0719 |
UFH (NIHSS 14) | ||
Deep‐vein thrombosis | 0.2914 | 0.22410.3588 |
Pulmonary embolism | 0.0229 | 0.00630.0575 |
Intracranial hemorrhage | 0.016 | 0.00040.0316 |
Major extracranial hemorrhage | 0 | 00 |
Minor hemorrhage | 0.064 | 0.0370.1019 |
Event | Cost per Event ($)* | ||
---|---|---|---|
Likeliest | Minimum | Maximum | |
| |||
Deep‐vein thrombosis | 3,003 | 2,402 | 3,604 |
Pulmonary embolism | 2,143 | 1,714 | 2,572 |
Intracranial hemorrhage | 4,001 | 3,201 | 4,801 |
Major extracranial hemorrhage | 3,534 | 2,827 | 4,241 |
Minor hemorrhage | 1,322 | 1,058 | 1,586 |
Enoxaparin cost per dose | 26 | 21 | 31 |
Unfractionated heparin cost per dose | 3 | 2 | 4 |
The average hospital cost with enoxaparin, when taking into account the costs of VTE and bleeding, was lower than with UFH ($422 vs $662, respectively), with a net savings of $240 per patient if enoxaparin was used. The average drug costs, including drug‐administration costs, were higher in the enoxaparin group ($360) compared with the UFH group ($259; difference $101). Nevertheless, the total hospital cost when clinical events and drug costs were considered together, was lower with enoxaparin than UFH. The total hospital costs per patient were $782 in patients receiving prophylaxis with enoxaparin and $922 in patients receiving UFH. Thus, enoxaparin was associated with a total cost‐savings of $140 per patient (Figure 2).
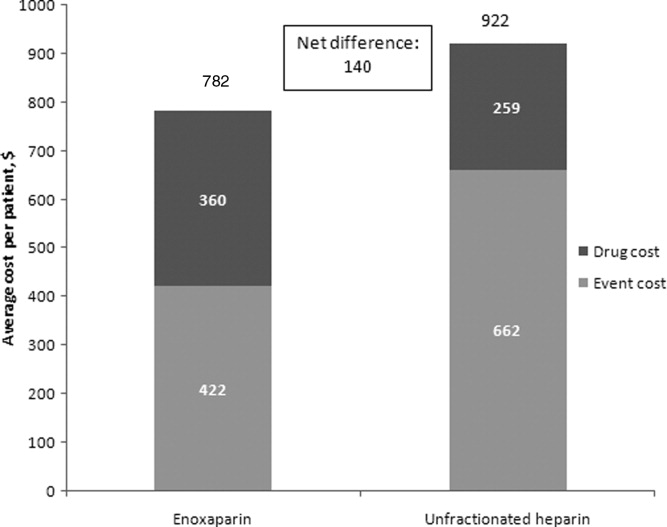
The cost estimates according to the stroke severity score (NIHSS scores <14 vs 14) are described in Table 3. The drug costs were consistent, regardless of stroke severity, for enoxaparin ($360) and for UFH ($259). However, in both treatment groups, the event costs were higher in patients with more severe stroke, compared with less severe stroke. For example, in the enoxaparin group, the event costs were $686 in patients with NIHSS scores 14 and $326 in patients with NIHSS scores <14. Nevertheless, the overall costs (event costs plus drug costs) were lower with enoxaparin compared with UFH, both in patients with less severe and more severe stroke. In fact, the total hospital cost‐savings were greater when enoxaparin was used instead of UFH in patients with more severe stroke (cost‐saving $287 if NIHSS score 14 vs $71 if NIHSS score <14) (Table 3).
Enoxaparin ($) | UFH ($) | Difference ($ [UFHEnoxaparin]) | |
---|---|---|---|
| |||
NIHSS score <14 | |||
Mean event costs per patient | 326 | 497 | 171 |
Mean drug costs per patient* | 360 | 259 | 101 |
Total costs | 685 | 756 | 71 |
NIHSS score 14 | |||
Mean event costs per patient | 686 | 1,073 | 387 |
Mean drug costs per patient* | 360 | 259 | 101 |
Total costs | 1,046 | 1,332 | 287 |
Multiple sensitivity analyses were performed. In the base case univariate sensitivity analysis, individual costs were adjusted by 20% (Table 4). If the cost of DVT increased by 20% (from $3003 to $3604) the difference between the enoxaparin and UFH groups was $187. When the cost of DVT was decreased by 20% to $2402, enoxaparin was still cost‐saving, with a difference of $94. For each of the individual cost parameters that were varied (DVT, PE, intracranial hemorrhage, major extracranial hemorrhage, and minor hemorrhage), enoxaparin was always less costly than UFH. Subsequent sensitivity analyses were performed (not shown) where cost parameters were varied by 5%, 10%, 15%, 30%, and 40%. Enoxaparin remained less costly than UFH in all cases.
Event | Baseline Cost Input ($) | +20% Cost Input ($) | +20% Difference ($ [UFH Enoxaparin]) (% Change) | 20% Cost Input ($) | 20% Difference ($ [UFH Enoxaparin]) (% Change) |
---|---|---|---|---|---|
| |||||
Deep‐vein thrombosis | 3,003 | 3,604 | 187 (33) | 2,402 | 94 (33) |
Pulmonary embolism | 2,143 | 2,572 | 144 (2.5) | 1,714 | 137 (2.5) |
Intracranial hemorrhage | 4,001 | 4,801 | 142 (1.3) | 3,201 | 138 (1.3) |
Major extracranial hemorrhage | 3,534 | 4,241 | 134 (4.0) | 2,827 | 146 (4.0) |
Minor hemorrhage | 1,322 | 1,586 | 142 (1.3) | 1,058 | 138 (1.3) |
A multivariate analysis was performed using a Monte Carlo simulation in TreeAge Pro (Figure 3). When all parameters were varied simultaneously (by 5%, 10%, 15%, 20%, 30%, and 40%) and the differences in costs between the enoxaparin and UFH groups were measured and plotted, the mean (standard deviation) difference between enoxaparin and UFH prophylaxis was $140 ($79) (Figure 3). Figure 4 shows a graphical presentation of the sensitivity analysis results for event probabilities and costs. Differences in enoxaparin drug costs, hospital costs for DVT, and probability of DVT for patients on enoxaparin are the factors that have the greatest effect on the overall cost.
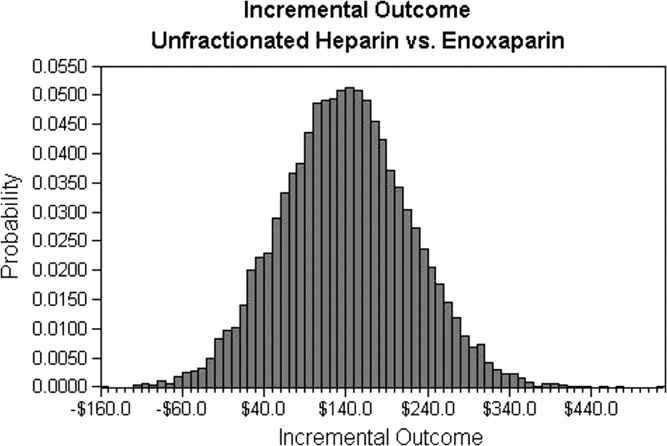
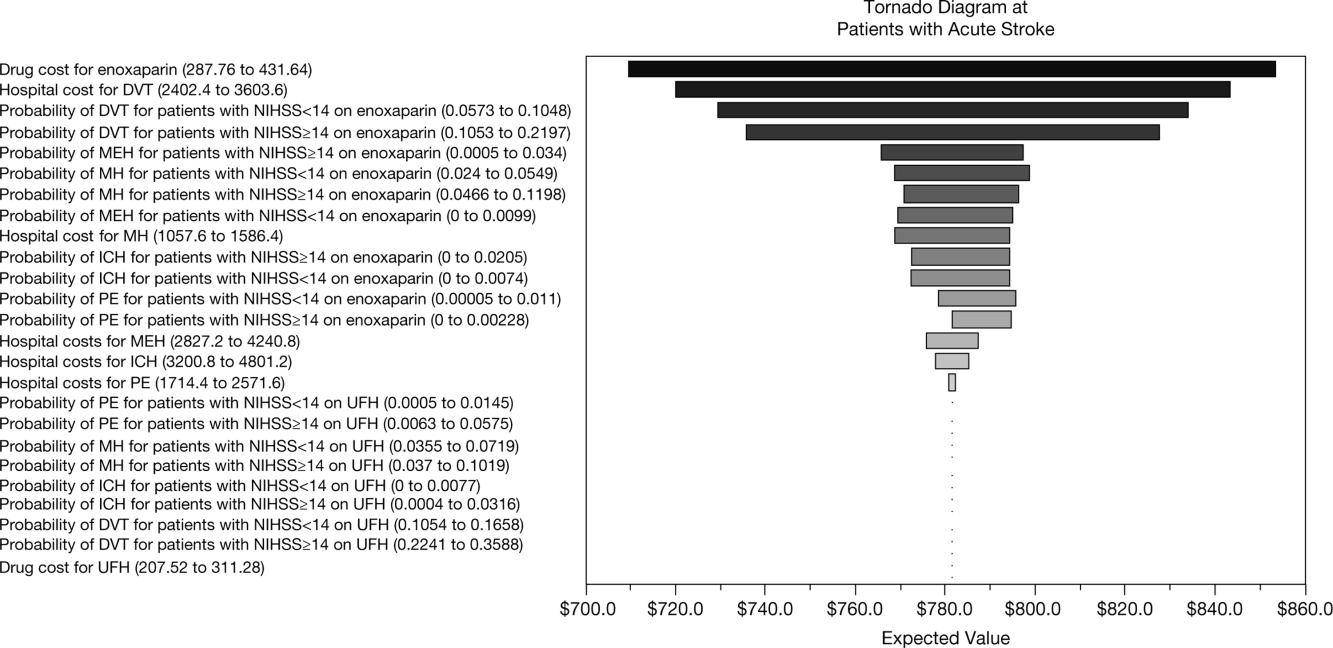
Finally, an additional scenario was performed using a published ratio of asymptomatic DVT to symptomatic VTE, due to the fact that not all VTE events in the real‐world present with symptoms prompting treatment. Quinlan et al. determined a ratio of asymptomatic DVT to symptomatic VTE of 5 for total hip replacement patients and of 21 for total knee replacement patients.28 Although derived from different patient populations who received different anticoagulants, we utilized the symptomatic event rates from the pooled studies to recalculate cost differences between enoxaparin and UFH in acute ischemic stroke. Using only symptomatic event rates, based on the 21:1 ratio in patients undergoing total knee replacement, the total cost for enoxaparin was $485 compared to $386 for UFH. Similar results were found based on the 5:1 ratio in patients with total hip replacement (enoxaparin $532 vs $472 for UFH). This was the only scenario where the higher drug cost of prophylaxis with enoxaparin was not completely offset by the reduction in events compared to UFH, likely due to the smaller difference in event rates once examining only symptomatic VTE.
DISCUSSION
This analysis demonstrates that, from the hospital perspective, enoxaparin 40 mg subcutaneously once‐daily is associated with lower total hospital costs and is more cost‐effective than twice‐daily UFH 5000 U subcutaneously for the prevention of VTE in patients with acute ischemic stroke. Despite higher drug‐acquisition costs, enoxaparin was associated with total cost‐savings of $140 per patient. This is due to the lower event rates with enoxaparin compared with UFH.
Previous studies, using hospital or payer information, have shown that VTE prophylaxis is more cost‐effective compared with no prophylaxis. In terms of the different VTE prophylaxis regimens, enoxaparin represents a more cost‐effective option in comparison with UFH19, 21, 2932 and also when compared with fondaparinux.21, 33 When comparing the results between different trials, it should be noted that previous analyses were mainly modeled on the Prophylaxis in Medical Patients with Enoxaparin (MEDENOX) study, which was performed in general medical patients and reported a VTE rate of 5.5%.6 However, patients with acute ischemic stroke are at a higher risk of VTE, with a 10% incidence of VTE reported in the PREVAIL study.14 Furthermore, twice‐daily rather than three‐timesdaily administration of UFH was used in the PREVAIL study, based on the current practice patterns seen during the PREVAIL trial design.
A recent retrospective analysis of transactional billing records demonstrated that, despite higher mean costs of anticoagulation therapy, the mean, total, adjusted direct hospital costs were lower with LMWH thromboprophylaxis compared with UFH ($7358 vs $8680, respectively; difference $1322; P < 0.001).21 A previous study by Burleigh and colleagues based on hospital discharge information extracted from both medical and surgical patients, has a sub‐analysis in patients with stroke. In these patients also, the total costs were lower for enoxaparin compared with UFH ($8608 vs $8911, respectively; difference $303).29 In the Burleigh study, drug costs and total discharge costs (eg, room and board, laboratory, and diagnostic imaging) were derived from drug charges and total charges, and were converted to estimated costs using cost‐to‐charge methods, so the absolute figures are not directly comparable with the current analysis.
This study adds to current literature by using data from a prospective study to analyze the hospital costs of VTE prophylaxis in stroke patients. The current study also provides a valuable cost‐analysis regarding a specific subgroup of medical patients at particularly high risk of VTE, and provides an economic comparison among stroke patients with NIHSS scores of <14 versus 14. In the PREVAIL study, despite a 2‐fold higher incidence of VTE in patients with more severe stroke (16.3% vs 8.3%), a similar reduction in VTE risk was observed with enoxaparin versus UFH in patients with NIHSS scores of 14 (odds ratio = 0.56; 95% CI = 0.37‐0.84; P = 0.0036) and <14 (odds ratio = 0.46; 95% CI = 0.27‐0.78; P = 0.0043).14 Enoxaparin was shown to be cost‐saving relative to UFH in both patient groups and, in particular, in patients with more severe stroke.
Potential limitations of the current analysis include the applicability of the figures obtained from the highly selected clinical trial population to real‐world clinical practice, and the fact that it is difficult to match cost estimates to trial data definitions. For example, this analysis was conducted with a comparator of twice‐daily UFH (as opposed to three‐timesdaily) which may be used in the real‐world setting and may have resulted in the increased number of events in the UFH group seen in the PREVAIL study. Due to a variety of differences between real‐world practice patterns and the PREVAIL clinical trial, we can only speculate as to the true cost‐consequences of utilizing enoxaparin versus UFH.
Furthermore, the original model did not include a sub‐analysis regarding the rates and, therefore, costs of proximal/symptomatic VTE. In the primary study of PREVAIL, the rates of symptomatic DVT were 1 in 666 patients (<1%) for enoxaparin and 4 in 669 patients (1%) for UFH, whereas the rates of proximal DVT were 30 in 666 patients (5%) and 64 in 669 patients (10%), respectively. Sensitivity analyses were performed to investigate the impact of lower rates of both DVT and PE (up to 40%), and the differences between groups were found to be robust. However, it is important to note that overall costs for both groups may have been increased through the inclusion of asymptomatic costs, with a more distinct separation of these costs making for a good follow‐up study. In a similar cost‐analysis we performed based on the PREVAIL study, which assessed the cost to the payer, we included an analysis of costs according to 3 different VTE definitions: the PREVAIL VTE definition (as in the current study); a definition of major VTE (PE, symptomatic DVT, and asymptomatic proximal DVT); and primary endpoints recommended by the European Medicines Agency Committee for Medicinal Products for Human Use for studies on VTE (proximal DVT, nonfatal PE, and all‐cause mortality). We found similar results irrespective of clinical event definitions.34 In an additional model scenario using a published ratio of asymptomatic DVT to symptomatic VTE,28 the higher drug cost of prophylaxis with enoxaparin was not completely offset by the reduction in events compared to UFH. This was likely due to the smaller difference in event rates once examining only symptomatic VTE. This scenario was limited by the fact that the ratio was derived from different patient populations receiving different anticoagulants than stroke patients.
In conclusion, data from this analysis adds to the evidence that, from the hospital perspective, the higher drug cost of enoxaparin is offset by the economic consequences of the events avoided as compared with UFH for the prevention of VTE following acute ischemic stroke, particularly in patients with severe stroke.
Acknowledgements
The authors thank Aylin Lee from I3 Innovus for her contribution to this study. The authors also acknowledge Min Chen for her assistance in statistical analysis, and Essy Mozaffari for his contribution to this study.
- Venous thromboembolism: disease burden, outcomes and risk factors.J Thromb Haemost.2005;3:1611–1617. .
- The epidemiology of venous thromboembolism in the community: implications for prevention and management.J Thromb Thrombolysis.2006;21:23–29. .
- Relative impact of risk factors for deep vein thrombosis and pulmonary embolism: a population‐based study.Arch Intern Med.2002;162:1245–1248. , , , et al.
- Risk factors for deep vein thrombosis and pulmonary embolism: a population‐based case‐control study.Arch Intern Med.2000;160:809–815. , , , , , .
- A safety analysis of thromboprophylaxis in acute medical illness.Thromb Haemost.2003;89:590–591. , .
- Quantification of risk factors for venous thromboembolism: a preliminary study for the development of a risk assessment tool.Haematologica.2003;88:1410–1421. , , , , .
- Prevalence of venous thromboembolism in acute hemorrhagic and thromboembolic stroke.Am J Phys Med Rehabil.2003;82:364–369. , .
- Thromboprophylaxis rates in US medical centers: success or failure?J Thromb Haemost.2007;5:1610–1616. , , , .
- Complications after acute stroke.Stroke.1996;27:415–420. , , , .
- Venous thromboembolism after acute stroke.Stroke.2001;32:262–267. , , , .
- Enoxaparin vs heparin for prevention of deep‐vein thrombosis in acute ischaemic stroke: a randomized, double‐blind study.Acta Neurol Scand.2002;106:84–92. , , , , , .
- Low‐molecular‐weight heparins or heparinoids versus standard unfractionated heparin for acute ischaemic stroke.Cochrane Database Syst Rev.2008;(3):CD000119. , , .
- for the PROTECT Trial Group.Prophylaxis of thrombotic and embolic events in acute ischemic stroke with the low‐molecular‐weight heparin certoparin: results of the PROTECT Trial.Stroke.2006;37:139–144. , , , et al;
- for the PREVAIL Investigators.The efficacy and safety of enoxaparin versus unfractionated heparin for the prevention of venous thromboembolism after acute ischaemic stroke (PREVAIL study): an open‐label randomised comparison.Lancet.2007;369:1347–1355. , , , et al;
- for the American College of Chest Physicians.Prevention of venous thromboembolism: American College of Chest Physicians Evidence‐Based Clinical Practice Guidelines (8th ed).Chest.2008;133(6 suppl):381S–453S. , , , et al;
- for the American College of Chest Physicians.Antithrombotic and thrombolytic therapy for ischemic stroke: American College of Chest Physicians Evidence‐Based Clinical Practice Guidelines (8th ed).Chest.2008;133(6 suppl):630S–669S. , , , , ;
- Management of acute proximal deep vein thrombosis: pharmacoeconomic evaluation of outpatient treatment with enoxaparin vs inpatient treatment with unfractionated heparin.Chest.2002;122:108–114. , , , .
- Economic evaluation of enoxaparin as prophylaxis against venous thromboembolism in seriously ill medical patients: a US perspective.Am J Manag Care.2002;8:1082–1088. , .
- Cost effectiveness of thromboprophylaxis with a low‐molecular‐weight heparin versus unfractionated heparin in acutely ill medical inpatients.Am J Manag Care.2004;10:632–642. , , , .
- Cost effectiveness of enoxaparin as prophylaxis against venous thromboembolic complications in acutely ill medical inpatients: modelling study from the hospital perspective in Germany.Pharmacoeconomics.2006;24:571–591. , , , et al.
- Hospital‐based costs associated with venous thromboembolism prophylaxis regimens.J Thromb Thrombolysis.2010;29:449–458. , , , , .
- for the ENDORSE Investigators.Venous thromboembolism risk and prophylaxis in the acute hospital care setting (ENDORSE study): a multinational cross‐sectional study.Lancet.2008;371:387–394. , , , et al;
- for the IMPROVE Investigators.Venous thromboembolism prophylaxis in acutely ill hospitalized medical patients: findings from the International Medical Prevention Registry on Venous Thromboembolism.Chest.2007;132:936–945. , , , et al;
- United States Department of Health August 2008. Available at: http://www.ahrq.gov/qual/vtguide/. Accessed August 18,2010.
- Enoxaparin versus unfractionated heparin in the prevention of venous thromboembolism after acute ischemic stroke: rationale, design, and methods of an open‐label, randomized, parallel‐group multicenter trial.J Stroke Cerebrovasc Dis.2005;14:95–100. , , , .
- Association between asymptomatic deep vein thrombosis detected by venography and symptomatic venous thromboembolism in patients undergoing elective hip or knee surgery.J Thromb Haemost.2007;5:1438–1443. , , , , , .
- Thromboprophylaxis in medically ill patients at risk for venous thromboembolism.Am J Health Syst Pharm.2006;63(20 suppl 6):S23–S29. , , , et al.
- Comparison of the two‐year outcomes and costs of prophylaxis in medical patients at risk of venous thromboembolism.Thromb Haemost.2008;100:810–820. , , , .
- Cost for inpatient care of venous thrombosis: a trial of enoxaparin vs standard heparin.Arch Intern Med.2000;160:3160–3165. , , , , , .
- Economic evaluation of enoxaparin for the prevention of venous thromboembolism in acutely ill medical patients.Pharm World Sci.2004;26:214–220. , , , .
- Total hospital‐based costs of enoxaparin or fondaparinux prophylaxis in patients at risk of venous thromboembolism [abstract]. Presented at the Chest 2008 Annual Meeting; October 25–30,2008; Philadelphia, PA. , , , , .
- Economic impact of enoxaparin after acute ischemic stroke based on PREVAIL.Clin Appl Thromb Hemost.2011;17:150–157. , , , , .
Venous thromboembolism (VTE), which encompasses both deep‐vein thrombosis (DVT) and pulmonary embolism (PE), is a major health problem in the United States and worldwide. It represents one of the most significant causes of morbidity and mortality with an estimated 300,000 VTE‐related deaths,1 and 300,000‐600,000 hospitalizations in the United States annually.2 Hospitalization for medical illness is associated with a similar proportion of VTE cases as hospitalization for surgery.3 Several groups of medical patients have been shown to be at an increased risk of VTE, including those with cancer, severe respiratory disease, acute infectious illness, heart failure, myocardial infarction, and acute ischemic stroke.47 Ischemic stroke patients represent approximately 4.6% of medical patients at high risk of VTE in US hospitals.8 The incidence of DVT in such patients has been reported to be as high as 75%9 and PE has been reported to be responsible for up to 25% of early deaths after stroke.10
Several studies have demonstrated the efficacy of unfractionated heparin (UFH) or a low‐molecular‐weight heparin (LMWH) in the prevention of VTE in stroke patients, and have demonstrated that LMWHs are at least as effective as UFH.1114 The open‐label, randomized Prevention of VTE after acute ischemic stroke with LMWH and UFH (PREVAIL) trial demonstrated that in patients with acute ischemic stroke, prophylaxis for 10 days with the LMWH enoxaparin reduces the risk of VTE by 43% compared with UFH (10.2% vs 18.1%, respectively; relative risk = 0.57; 95% confidence interval [CI] = 0.44‐0.76; P = 0.0001) without increasing the incidence of overall bleeding events (7.9% vs 8.1%, respectively; P = 0.83), or the composite of symptomatic intracranial and major extracranial hemorrhage (1% in each group; P = 0.23). There was, however, a slight but significant increase in major extracranial hemorrhage alone with enoxaparin (1% vs 0%; P = 0.015).14 Evidence‐based guidelines from the American College of Chest Physicians (ACCP) provide recommendations for appropriate thromboprophylaxis regimens for patients at risk of VTE.15 Thromboprophylaxis with UFH, LMWH, and, more recently, fondaparinux is recommended for medical patients admitted to hospital with congestive heart failure or severe respiratory disease, or those who are confined to bed and have one or more additional risk factors, including active cancer, previous VTE, or acute neurologic disease.15 Similarly, in the Eighth ACCP Clinical Practice Guidelines, low‐dose UFH or LMWH are recommended for VTE prevention in patients with ischemic stroke who have restricted mobility.16
VTE is also associated with a substantial economic burden on the healthcare system, costing an estimated $1.5 billion annually in the United States.17 Thromboprophylaxis has been shown to be a cost‐effective strategy in hospitalized medical patients. Prophylaxis with a LMWH has been shown to be more cost‐effective than UFH in these patients.1821
However, despite the clinical and economic benefits, prophylaxis is still commonly underused in medical patients.22, 23 In surgical patients, the Surgical Care Improvement Project (SCIP) focuses on reducing surgical complications, and has endorsed 2 measures: VTE‐1, relating to the proportion of patients for whom VTE prophylaxis is ordered; and VTE‐2, relating to those who receive the recommended regimen (
The objective of the current study was to determine the economic impact, in terms of hospital costs, of enoxaparin compared with UFH for VTE prophylaxis after acute ischemic stroke. A decision‐analytic model was constructed using data from the PREVAIL study and historical inpatient data from a multi‐hospital database.
METHODS
In this study, the cost implications, from the hospital perspective, of VTE prophylaxis with enoxaparin or UFH in patients with acute ischemic stroke, were determined using a decision‐analytic model in TreeAge Pro Suite (TreeAge Software, Inc., Williamstown, MA, USA). The decision‐tree was based on 3 stages: (a) whether patients received enoxaparin or UFH; (b) how patients were classified according to their National Institutes of Health Stroke Scale (NIHSS) classification scores (<14 or 14); and (c) which clinical event each patient experienced, as defined per the PREVAIL trial (DVT, PE, intracranial hemorrhage, major extracranial hemorrhage, and minor extracranial hemorrhage) (Figure 1). The time horizon for the model was established at 90 days to mirror the length of follow‐up in the PREVAIL trial.
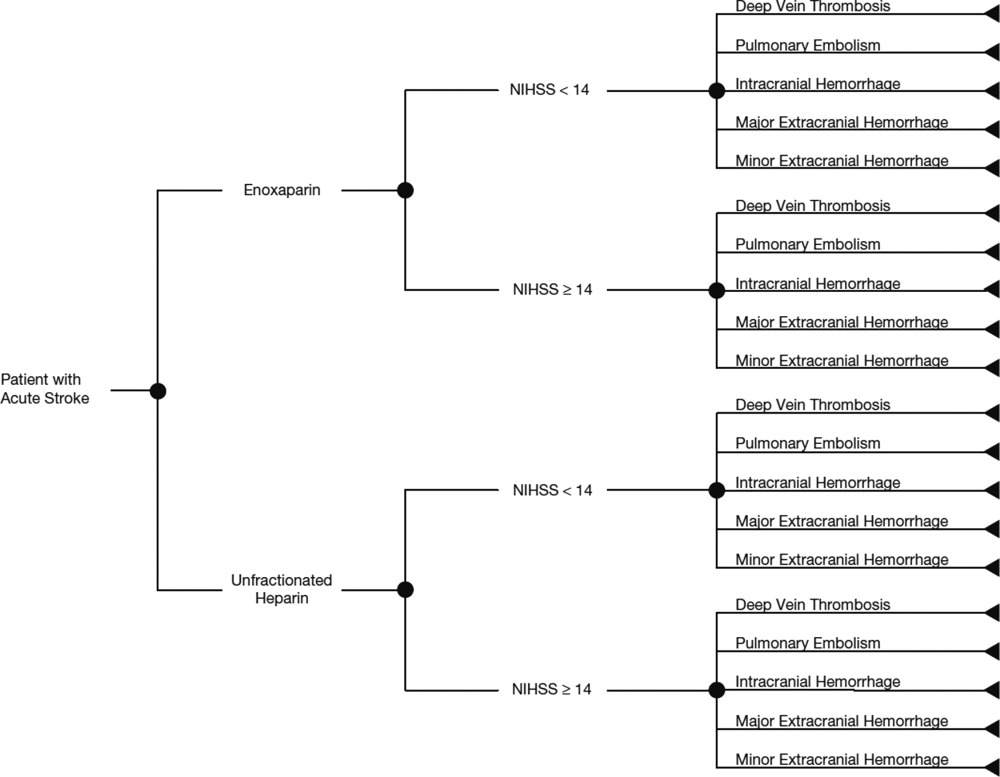
Total hospital costs were calculated based on clinical event rates (from the PREVAIL trial) and the costs of each clinical event, which were calculated separately according to the descriptions below, and then inserted into the decision‐analytic model. The clinical event rates were calculated from the efficacy and safety endpoints collected in the PREVAIL trial, and included VTE events (DVT and PE) and bleeding events (intracranial hemorrhage, major extracranial hemorrhage, and minor extracranial hemorrhage). Details of the patient population, eligibility criteria, and treatment regimen have previously been published in full elsewhere.14, 27
The costs of clinical events during hospitalization were estimated using a multivariate cost‐evaluation model, based on mean hospital costs for the events in the (Premier Inc., Charlotte, NC, USA) multi‐hospital database, one of the largest US hospital clinical and economic databases. The data are received from over 600 hospitals, representing all geographical areas of the United States, a broad range of bed sizes, teaching and non‐teaching, and urban and rural facilities. This database contains detailed US inpatient care records of principal and secondary diagnoses, inpatient procedures, administered laboratory tests, dispensed drugs, and demographic information. The evaluation of hospital cost for each type of clinical event was conducted by i3 Innovus (Ingenix, Inc., Eden Prairie, MN, USA). Total hospital costs were cumulative from all events, so if patients experienced multiple clinical events, the costs of the events were additive. The cost for stroke treatment and management was not included because it is an inclusion criterion of the PREVAIL trial and, thus, all patients in the trial have such costs.
Default drug costs were taken from the 2008 US wholesalers' acquisition cost data. The default dosing schedule is based on information extracted from the PREVAIL trial: enoxaparin 40 mg (once‐daily) and UFH 5000 U (twice‐daily) for 10 days each ($25.97 and $2.97, respectively). A drug‐administration fee was added for each dose of either enoxaparin or UFH ($10 for each).19
The estimated hospital cost of clinical events, along with drug costs, were inserted into the decision‐analytic model in TreeAge Pro Suite to estimate the cost per discharge from the hospital perspective in patients with ischemic stroke receiving VTE prophylaxis with enoxaparin or UFH. An additional analysis was performed to investigate the costs and cost differences in patients with less severe stroke (NIHSS scores <14) and more severe stroke (NIHSS scores 14).
Sensitivity analyses were performed to examine the impact of varying the cost inputs on the total hospital cost of each treatment arm by 5%, 10%, 15%, 20%, 30%, and 40%, and the robustness in the difference in costs between the enoxaparin and UFH groups. Univariate (via tornado diagram in TreeAge Pro Suite) and multivariate (via Monte Carlo simulation in TreeAge Pro Suite) analyses were performed. For the univariate analysis, each clinical event cost was adjusted individually, increasing or decreasing by 5%, 10%, 15%, 20%, 30% and 40% while other parameters remained unchanged. For the Monte Carlo simulation (TreeAge Pro Suite), all the parameters were simultaneously varied in a random fashion, within a range of 5%, 10%, 15%, 20%, 30%, and 40% over 10,000 trials. The simulation adopted a gamma distribution assumption for input sampling for cost parameters and a beta distribution for the event probability parameters. The confidence intervals for the probability parameters were obtained from the PREVAIL trial. The differences between the enoxaparin and UFH treatment groups were plotted in a graph against the variation in costs of each clinical event.
RESULTS
The clinical VTE and bleeding event rates as collected from the PREVAIL trial are shown in Table 1. The hospital costs per clinical event are shown in Table 2. The most costly clinical event from the hospital perspective was intracranial hemorrhage at $4001, followed by major extracranial hemorrhage at $3534. The costs of DVT and PE were $3003 and $2143, respectively.
Event Rate | 95% CI | |
---|---|---|
| ||
Enoxaparin (NIHSS <14) | ||
Deep‐vein thrombosis | 0.081 | 0.05730.1048 |
Pulmonary embolism | 0.002 | 0.000050.011 |
Intracranial hemorrhage | 0.0031 | 00.0074 |
Major extracranial hemorrhage | 0.0047 | 00.0099 |
Minor hemorrhage | 0.0372 | 0.0240.0549 |
Enoxaparin (NIHSS 14) | ||
Deep‐vein thrombosis | 0.1625 | 0.10530.2197 |
Pulmonary embolism | 0 | 00 |
Intracranial hemorrhage | 0.0086 | 00.0205 |
Major extracranial hemorrhage | 0.0172 | 0.04660.1198 |
Minor hemorrhage | 0.0776 | 0.04660.1198 |
UFH (NIHSS <14) | ||
Deep‐vein thrombosis | 0.1356 | 0.10540.1658 |
Pulmonary embolism | 0.004 | 0.00050.0145 |
Intracranial hemorrhage | 0.0032 | 00.0077 |
Major extracranial hemorrhage | 0 | 00 |
Minor hemorrhage | 0.0514 | 0.03550.0719 |
UFH (NIHSS 14) | ||
Deep‐vein thrombosis | 0.2914 | 0.22410.3588 |
Pulmonary embolism | 0.0229 | 0.00630.0575 |
Intracranial hemorrhage | 0.016 | 0.00040.0316 |
Major extracranial hemorrhage | 0 | 00 |
Minor hemorrhage | 0.064 | 0.0370.1019 |
Event | Cost per Event ($)* | ||
---|---|---|---|
Likeliest | Minimum | Maximum | |
| |||
Deep‐vein thrombosis | 3,003 | 2,402 | 3,604 |
Pulmonary embolism | 2,143 | 1,714 | 2,572 |
Intracranial hemorrhage | 4,001 | 3,201 | 4,801 |
Major extracranial hemorrhage | 3,534 | 2,827 | 4,241 |
Minor hemorrhage | 1,322 | 1,058 | 1,586 |
Enoxaparin cost per dose | 26 | 21 | 31 |
Unfractionated heparin cost per dose | 3 | 2 | 4 |
The average hospital cost with enoxaparin, when taking into account the costs of VTE and bleeding, was lower than with UFH ($422 vs $662, respectively), with a net savings of $240 per patient if enoxaparin was used. The average drug costs, including drug‐administration costs, were higher in the enoxaparin group ($360) compared with the UFH group ($259; difference $101). Nevertheless, the total hospital cost when clinical events and drug costs were considered together, was lower with enoxaparin than UFH. The total hospital costs per patient were $782 in patients receiving prophylaxis with enoxaparin and $922 in patients receiving UFH. Thus, enoxaparin was associated with a total cost‐savings of $140 per patient (Figure 2).
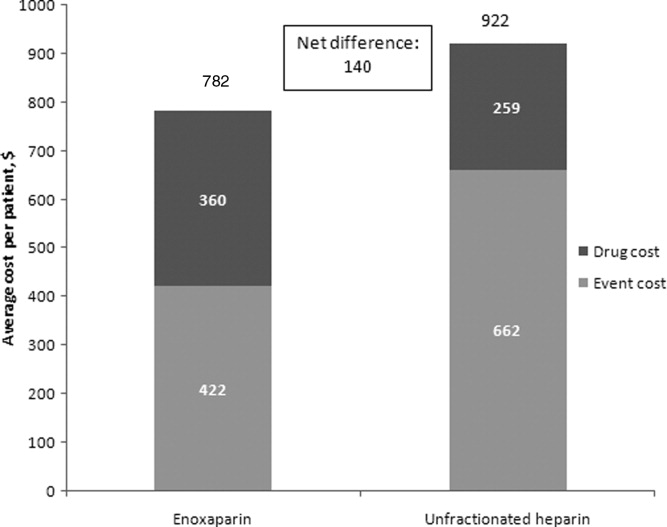
The cost estimates according to the stroke severity score (NIHSS scores <14 vs 14) are described in Table 3. The drug costs were consistent, regardless of stroke severity, for enoxaparin ($360) and for UFH ($259). However, in both treatment groups, the event costs were higher in patients with more severe stroke, compared with less severe stroke. For example, in the enoxaparin group, the event costs were $686 in patients with NIHSS scores 14 and $326 in patients with NIHSS scores <14. Nevertheless, the overall costs (event costs plus drug costs) were lower with enoxaparin compared with UFH, both in patients with less severe and more severe stroke. In fact, the total hospital cost‐savings were greater when enoxaparin was used instead of UFH in patients with more severe stroke (cost‐saving $287 if NIHSS score 14 vs $71 if NIHSS score <14) (Table 3).
Enoxaparin ($) | UFH ($) | Difference ($ [UFHEnoxaparin]) | |
---|---|---|---|
| |||
NIHSS score <14 | |||
Mean event costs per patient | 326 | 497 | 171 |
Mean drug costs per patient* | 360 | 259 | 101 |
Total costs | 685 | 756 | 71 |
NIHSS score 14 | |||
Mean event costs per patient | 686 | 1,073 | 387 |
Mean drug costs per patient* | 360 | 259 | 101 |
Total costs | 1,046 | 1,332 | 287 |
Multiple sensitivity analyses were performed. In the base case univariate sensitivity analysis, individual costs were adjusted by 20% (Table 4). If the cost of DVT increased by 20% (from $3003 to $3604) the difference between the enoxaparin and UFH groups was $187. When the cost of DVT was decreased by 20% to $2402, enoxaparin was still cost‐saving, with a difference of $94. For each of the individual cost parameters that were varied (DVT, PE, intracranial hemorrhage, major extracranial hemorrhage, and minor hemorrhage), enoxaparin was always less costly than UFH. Subsequent sensitivity analyses were performed (not shown) where cost parameters were varied by 5%, 10%, 15%, 30%, and 40%. Enoxaparin remained less costly than UFH in all cases.
Event | Baseline Cost Input ($) | +20% Cost Input ($) | +20% Difference ($ [UFH Enoxaparin]) (% Change) | 20% Cost Input ($) | 20% Difference ($ [UFH Enoxaparin]) (% Change) |
---|---|---|---|---|---|
| |||||
Deep‐vein thrombosis | 3,003 | 3,604 | 187 (33) | 2,402 | 94 (33) |
Pulmonary embolism | 2,143 | 2,572 | 144 (2.5) | 1,714 | 137 (2.5) |
Intracranial hemorrhage | 4,001 | 4,801 | 142 (1.3) | 3,201 | 138 (1.3) |
Major extracranial hemorrhage | 3,534 | 4,241 | 134 (4.0) | 2,827 | 146 (4.0) |
Minor hemorrhage | 1,322 | 1,586 | 142 (1.3) | 1,058 | 138 (1.3) |
A multivariate analysis was performed using a Monte Carlo simulation in TreeAge Pro (Figure 3). When all parameters were varied simultaneously (by 5%, 10%, 15%, 20%, 30%, and 40%) and the differences in costs between the enoxaparin and UFH groups were measured and plotted, the mean (standard deviation) difference between enoxaparin and UFH prophylaxis was $140 ($79) (Figure 3). Figure 4 shows a graphical presentation of the sensitivity analysis results for event probabilities and costs. Differences in enoxaparin drug costs, hospital costs for DVT, and probability of DVT for patients on enoxaparin are the factors that have the greatest effect on the overall cost.
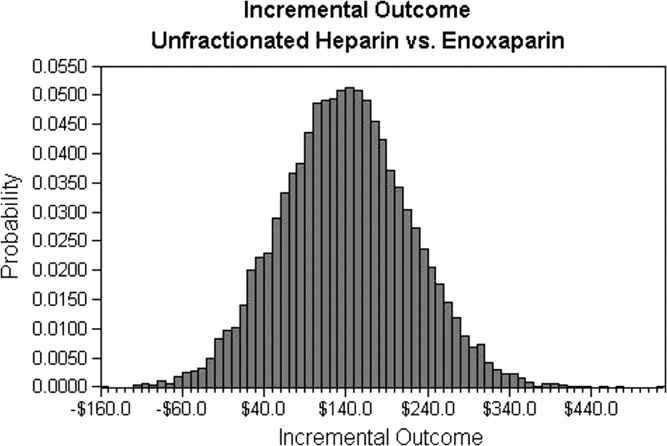
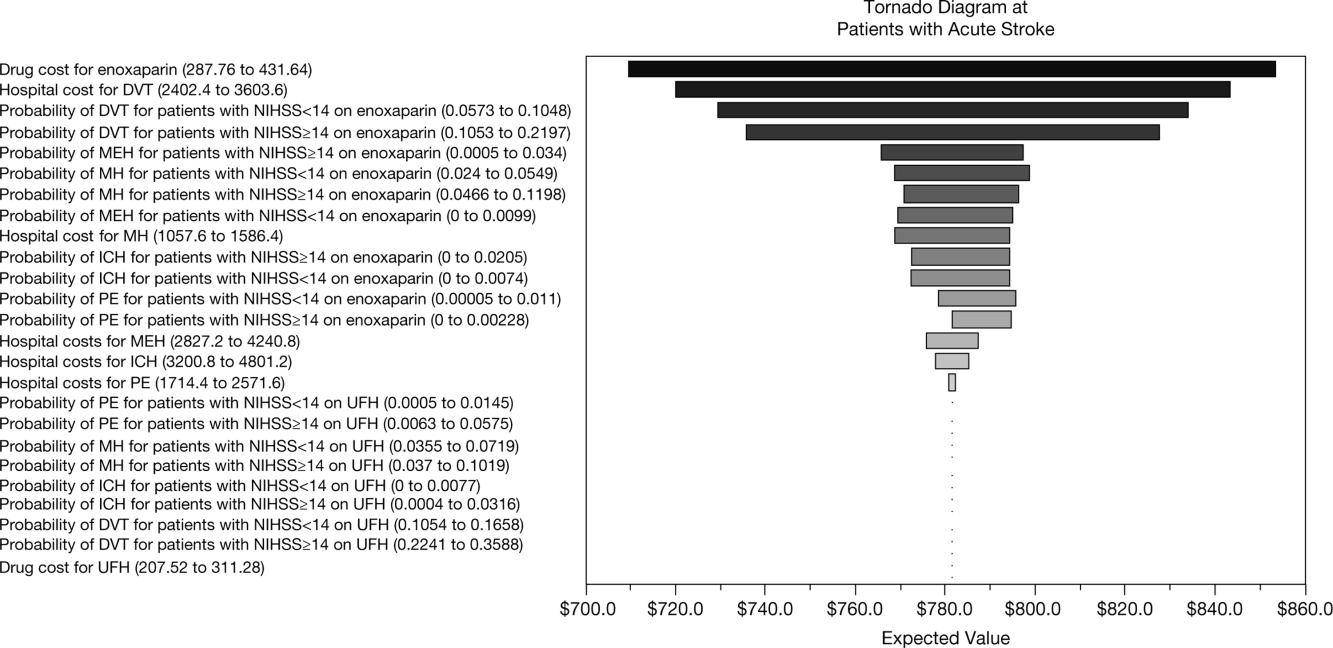
Finally, an additional scenario was performed using a published ratio of asymptomatic DVT to symptomatic VTE, due to the fact that not all VTE events in the real‐world present with symptoms prompting treatment. Quinlan et al. determined a ratio of asymptomatic DVT to symptomatic VTE of 5 for total hip replacement patients and of 21 for total knee replacement patients.28 Although derived from different patient populations who received different anticoagulants, we utilized the symptomatic event rates from the pooled studies to recalculate cost differences between enoxaparin and UFH in acute ischemic stroke. Using only symptomatic event rates, based on the 21:1 ratio in patients undergoing total knee replacement, the total cost for enoxaparin was $485 compared to $386 for UFH. Similar results were found based on the 5:1 ratio in patients with total hip replacement (enoxaparin $532 vs $472 for UFH). This was the only scenario where the higher drug cost of prophylaxis with enoxaparin was not completely offset by the reduction in events compared to UFH, likely due to the smaller difference in event rates once examining only symptomatic VTE.
DISCUSSION
This analysis demonstrates that, from the hospital perspective, enoxaparin 40 mg subcutaneously once‐daily is associated with lower total hospital costs and is more cost‐effective than twice‐daily UFH 5000 U subcutaneously for the prevention of VTE in patients with acute ischemic stroke. Despite higher drug‐acquisition costs, enoxaparin was associated with total cost‐savings of $140 per patient. This is due to the lower event rates with enoxaparin compared with UFH.
Previous studies, using hospital or payer information, have shown that VTE prophylaxis is more cost‐effective compared with no prophylaxis. In terms of the different VTE prophylaxis regimens, enoxaparin represents a more cost‐effective option in comparison with UFH19, 21, 2932 and also when compared with fondaparinux.21, 33 When comparing the results between different trials, it should be noted that previous analyses were mainly modeled on the Prophylaxis in Medical Patients with Enoxaparin (MEDENOX) study, which was performed in general medical patients and reported a VTE rate of 5.5%.6 However, patients with acute ischemic stroke are at a higher risk of VTE, with a 10% incidence of VTE reported in the PREVAIL study.14 Furthermore, twice‐daily rather than three‐timesdaily administration of UFH was used in the PREVAIL study, based on the current practice patterns seen during the PREVAIL trial design.
A recent retrospective analysis of transactional billing records demonstrated that, despite higher mean costs of anticoagulation therapy, the mean, total, adjusted direct hospital costs were lower with LMWH thromboprophylaxis compared with UFH ($7358 vs $8680, respectively; difference $1322; P < 0.001).21 A previous study by Burleigh and colleagues based on hospital discharge information extracted from both medical and surgical patients, has a sub‐analysis in patients with stroke. In these patients also, the total costs were lower for enoxaparin compared with UFH ($8608 vs $8911, respectively; difference $303).29 In the Burleigh study, drug costs and total discharge costs (eg, room and board, laboratory, and diagnostic imaging) were derived from drug charges and total charges, and were converted to estimated costs using cost‐to‐charge methods, so the absolute figures are not directly comparable with the current analysis.
This study adds to current literature by using data from a prospective study to analyze the hospital costs of VTE prophylaxis in stroke patients. The current study also provides a valuable cost‐analysis regarding a specific subgroup of medical patients at particularly high risk of VTE, and provides an economic comparison among stroke patients with NIHSS scores of <14 versus 14. In the PREVAIL study, despite a 2‐fold higher incidence of VTE in patients with more severe stroke (16.3% vs 8.3%), a similar reduction in VTE risk was observed with enoxaparin versus UFH in patients with NIHSS scores of 14 (odds ratio = 0.56; 95% CI = 0.37‐0.84; P = 0.0036) and <14 (odds ratio = 0.46; 95% CI = 0.27‐0.78; P = 0.0043).14 Enoxaparin was shown to be cost‐saving relative to UFH in both patient groups and, in particular, in patients with more severe stroke.
Potential limitations of the current analysis include the applicability of the figures obtained from the highly selected clinical trial population to real‐world clinical practice, and the fact that it is difficult to match cost estimates to trial data definitions. For example, this analysis was conducted with a comparator of twice‐daily UFH (as opposed to three‐timesdaily) which may be used in the real‐world setting and may have resulted in the increased number of events in the UFH group seen in the PREVAIL study. Due to a variety of differences between real‐world practice patterns and the PREVAIL clinical trial, we can only speculate as to the true cost‐consequences of utilizing enoxaparin versus UFH.
Furthermore, the original model did not include a sub‐analysis regarding the rates and, therefore, costs of proximal/symptomatic VTE. In the primary study of PREVAIL, the rates of symptomatic DVT were 1 in 666 patients (<1%) for enoxaparin and 4 in 669 patients (1%) for UFH, whereas the rates of proximal DVT were 30 in 666 patients (5%) and 64 in 669 patients (10%), respectively. Sensitivity analyses were performed to investigate the impact of lower rates of both DVT and PE (up to 40%), and the differences between groups were found to be robust. However, it is important to note that overall costs for both groups may have been increased through the inclusion of asymptomatic costs, with a more distinct separation of these costs making for a good follow‐up study. In a similar cost‐analysis we performed based on the PREVAIL study, which assessed the cost to the payer, we included an analysis of costs according to 3 different VTE definitions: the PREVAIL VTE definition (as in the current study); a definition of major VTE (PE, symptomatic DVT, and asymptomatic proximal DVT); and primary endpoints recommended by the European Medicines Agency Committee for Medicinal Products for Human Use for studies on VTE (proximal DVT, nonfatal PE, and all‐cause mortality). We found similar results irrespective of clinical event definitions.34 In an additional model scenario using a published ratio of asymptomatic DVT to symptomatic VTE,28 the higher drug cost of prophylaxis with enoxaparin was not completely offset by the reduction in events compared to UFH. This was likely due to the smaller difference in event rates once examining only symptomatic VTE. This scenario was limited by the fact that the ratio was derived from different patient populations receiving different anticoagulants than stroke patients.
In conclusion, data from this analysis adds to the evidence that, from the hospital perspective, the higher drug cost of enoxaparin is offset by the economic consequences of the events avoided as compared with UFH for the prevention of VTE following acute ischemic stroke, particularly in patients with severe stroke.
Acknowledgements
The authors thank Aylin Lee from I3 Innovus for her contribution to this study. The authors also acknowledge Min Chen for her assistance in statistical analysis, and Essy Mozaffari for his contribution to this study.
Venous thromboembolism (VTE), which encompasses both deep‐vein thrombosis (DVT) and pulmonary embolism (PE), is a major health problem in the United States and worldwide. It represents one of the most significant causes of morbidity and mortality with an estimated 300,000 VTE‐related deaths,1 and 300,000‐600,000 hospitalizations in the United States annually.2 Hospitalization for medical illness is associated with a similar proportion of VTE cases as hospitalization for surgery.3 Several groups of medical patients have been shown to be at an increased risk of VTE, including those with cancer, severe respiratory disease, acute infectious illness, heart failure, myocardial infarction, and acute ischemic stroke.47 Ischemic stroke patients represent approximately 4.6% of medical patients at high risk of VTE in US hospitals.8 The incidence of DVT in such patients has been reported to be as high as 75%9 and PE has been reported to be responsible for up to 25% of early deaths after stroke.10
Several studies have demonstrated the efficacy of unfractionated heparin (UFH) or a low‐molecular‐weight heparin (LMWH) in the prevention of VTE in stroke patients, and have demonstrated that LMWHs are at least as effective as UFH.1114 The open‐label, randomized Prevention of VTE after acute ischemic stroke with LMWH and UFH (PREVAIL) trial demonstrated that in patients with acute ischemic stroke, prophylaxis for 10 days with the LMWH enoxaparin reduces the risk of VTE by 43% compared with UFH (10.2% vs 18.1%, respectively; relative risk = 0.57; 95% confidence interval [CI] = 0.44‐0.76; P = 0.0001) without increasing the incidence of overall bleeding events (7.9% vs 8.1%, respectively; P = 0.83), or the composite of symptomatic intracranial and major extracranial hemorrhage (1% in each group; P = 0.23). There was, however, a slight but significant increase in major extracranial hemorrhage alone with enoxaparin (1% vs 0%; P = 0.015).14 Evidence‐based guidelines from the American College of Chest Physicians (ACCP) provide recommendations for appropriate thromboprophylaxis regimens for patients at risk of VTE.15 Thromboprophylaxis with UFH, LMWH, and, more recently, fondaparinux is recommended for medical patients admitted to hospital with congestive heart failure or severe respiratory disease, or those who are confined to bed and have one or more additional risk factors, including active cancer, previous VTE, or acute neurologic disease.15 Similarly, in the Eighth ACCP Clinical Practice Guidelines, low‐dose UFH or LMWH are recommended for VTE prevention in patients with ischemic stroke who have restricted mobility.16
VTE is also associated with a substantial economic burden on the healthcare system, costing an estimated $1.5 billion annually in the United States.17 Thromboprophylaxis has been shown to be a cost‐effective strategy in hospitalized medical patients. Prophylaxis with a LMWH has been shown to be more cost‐effective than UFH in these patients.1821
However, despite the clinical and economic benefits, prophylaxis is still commonly underused in medical patients.22, 23 In surgical patients, the Surgical Care Improvement Project (SCIP) focuses on reducing surgical complications, and has endorsed 2 measures: VTE‐1, relating to the proportion of patients for whom VTE prophylaxis is ordered; and VTE‐2, relating to those who receive the recommended regimen (
The objective of the current study was to determine the economic impact, in terms of hospital costs, of enoxaparin compared with UFH for VTE prophylaxis after acute ischemic stroke. A decision‐analytic model was constructed using data from the PREVAIL study and historical inpatient data from a multi‐hospital database.
METHODS
In this study, the cost implications, from the hospital perspective, of VTE prophylaxis with enoxaparin or UFH in patients with acute ischemic stroke, were determined using a decision‐analytic model in TreeAge Pro Suite (TreeAge Software, Inc., Williamstown, MA, USA). The decision‐tree was based on 3 stages: (a) whether patients received enoxaparin or UFH; (b) how patients were classified according to their National Institutes of Health Stroke Scale (NIHSS) classification scores (<14 or 14); and (c) which clinical event each patient experienced, as defined per the PREVAIL trial (DVT, PE, intracranial hemorrhage, major extracranial hemorrhage, and minor extracranial hemorrhage) (Figure 1). The time horizon for the model was established at 90 days to mirror the length of follow‐up in the PREVAIL trial.
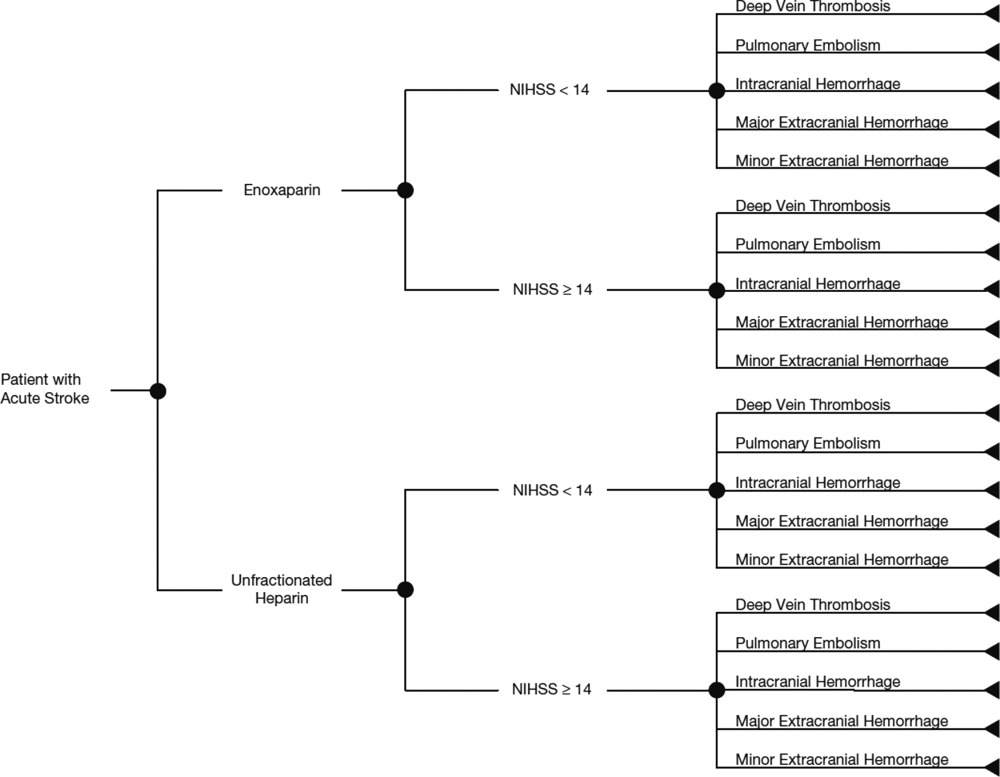
Total hospital costs were calculated based on clinical event rates (from the PREVAIL trial) and the costs of each clinical event, which were calculated separately according to the descriptions below, and then inserted into the decision‐analytic model. The clinical event rates were calculated from the efficacy and safety endpoints collected in the PREVAIL trial, and included VTE events (DVT and PE) and bleeding events (intracranial hemorrhage, major extracranial hemorrhage, and minor extracranial hemorrhage). Details of the patient population, eligibility criteria, and treatment regimen have previously been published in full elsewhere.14, 27
The costs of clinical events during hospitalization were estimated using a multivariate cost‐evaluation model, based on mean hospital costs for the events in the (Premier Inc., Charlotte, NC, USA) multi‐hospital database, one of the largest US hospital clinical and economic databases. The data are received from over 600 hospitals, representing all geographical areas of the United States, a broad range of bed sizes, teaching and non‐teaching, and urban and rural facilities. This database contains detailed US inpatient care records of principal and secondary diagnoses, inpatient procedures, administered laboratory tests, dispensed drugs, and demographic information. The evaluation of hospital cost for each type of clinical event was conducted by i3 Innovus (Ingenix, Inc., Eden Prairie, MN, USA). Total hospital costs were cumulative from all events, so if patients experienced multiple clinical events, the costs of the events were additive. The cost for stroke treatment and management was not included because it is an inclusion criterion of the PREVAIL trial and, thus, all patients in the trial have such costs.
Default drug costs were taken from the 2008 US wholesalers' acquisition cost data. The default dosing schedule is based on information extracted from the PREVAIL trial: enoxaparin 40 mg (once‐daily) and UFH 5000 U (twice‐daily) for 10 days each ($25.97 and $2.97, respectively). A drug‐administration fee was added for each dose of either enoxaparin or UFH ($10 for each).19
The estimated hospital cost of clinical events, along with drug costs, were inserted into the decision‐analytic model in TreeAge Pro Suite to estimate the cost per discharge from the hospital perspective in patients with ischemic stroke receiving VTE prophylaxis with enoxaparin or UFH. An additional analysis was performed to investigate the costs and cost differences in patients with less severe stroke (NIHSS scores <14) and more severe stroke (NIHSS scores 14).
Sensitivity analyses were performed to examine the impact of varying the cost inputs on the total hospital cost of each treatment arm by 5%, 10%, 15%, 20%, 30%, and 40%, and the robustness in the difference in costs between the enoxaparin and UFH groups. Univariate (via tornado diagram in TreeAge Pro Suite) and multivariate (via Monte Carlo simulation in TreeAge Pro Suite) analyses were performed. For the univariate analysis, each clinical event cost was adjusted individually, increasing or decreasing by 5%, 10%, 15%, 20%, 30% and 40% while other parameters remained unchanged. For the Monte Carlo simulation (TreeAge Pro Suite), all the parameters were simultaneously varied in a random fashion, within a range of 5%, 10%, 15%, 20%, 30%, and 40% over 10,000 trials. The simulation adopted a gamma distribution assumption for input sampling for cost parameters and a beta distribution for the event probability parameters. The confidence intervals for the probability parameters were obtained from the PREVAIL trial. The differences between the enoxaparin and UFH treatment groups were plotted in a graph against the variation in costs of each clinical event.
RESULTS
The clinical VTE and bleeding event rates as collected from the PREVAIL trial are shown in Table 1. The hospital costs per clinical event are shown in Table 2. The most costly clinical event from the hospital perspective was intracranial hemorrhage at $4001, followed by major extracranial hemorrhage at $3534. The costs of DVT and PE were $3003 and $2143, respectively.
Event Rate | 95% CI | |
---|---|---|
| ||
Enoxaparin (NIHSS <14) | ||
Deep‐vein thrombosis | 0.081 | 0.05730.1048 |
Pulmonary embolism | 0.002 | 0.000050.011 |
Intracranial hemorrhage | 0.0031 | 00.0074 |
Major extracranial hemorrhage | 0.0047 | 00.0099 |
Minor hemorrhage | 0.0372 | 0.0240.0549 |
Enoxaparin (NIHSS 14) | ||
Deep‐vein thrombosis | 0.1625 | 0.10530.2197 |
Pulmonary embolism | 0 | 00 |
Intracranial hemorrhage | 0.0086 | 00.0205 |
Major extracranial hemorrhage | 0.0172 | 0.04660.1198 |
Minor hemorrhage | 0.0776 | 0.04660.1198 |
UFH (NIHSS <14) | ||
Deep‐vein thrombosis | 0.1356 | 0.10540.1658 |
Pulmonary embolism | 0.004 | 0.00050.0145 |
Intracranial hemorrhage | 0.0032 | 00.0077 |
Major extracranial hemorrhage | 0 | 00 |
Minor hemorrhage | 0.0514 | 0.03550.0719 |
UFH (NIHSS 14) | ||
Deep‐vein thrombosis | 0.2914 | 0.22410.3588 |
Pulmonary embolism | 0.0229 | 0.00630.0575 |
Intracranial hemorrhage | 0.016 | 0.00040.0316 |
Major extracranial hemorrhage | 0 | 00 |
Minor hemorrhage | 0.064 | 0.0370.1019 |
Event | Cost per Event ($)* | ||
---|---|---|---|
Likeliest | Minimum | Maximum | |
| |||
Deep‐vein thrombosis | 3,003 | 2,402 | 3,604 |
Pulmonary embolism | 2,143 | 1,714 | 2,572 |
Intracranial hemorrhage | 4,001 | 3,201 | 4,801 |
Major extracranial hemorrhage | 3,534 | 2,827 | 4,241 |
Minor hemorrhage | 1,322 | 1,058 | 1,586 |
Enoxaparin cost per dose | 26 | 21 | 31 |
Unfractionated heparin cost per dose | 3 | 2 | 4 |
The average hospital cost with enoxaparin, when taking into account the costs of VTE and bleeding, was lower than with UFH ($422 vs $662, respectively), with a net savings of $240 per patient if enoxaparin was used. The average drug costs, including drug‐administration costs, were higher in the enoxaparin group ($360) compared with the UFH group ($259; difference $101). Nevertheless, the total hospital cost when clinical events and drug costs were considered together, was lower with enoxaparin than UFH. The total hospital costs per patient were $782 in patients receiving prophylaxis with enoxaparin and $922 in patients receiving UFH. Thus, enoxaparin was associated with a total cost‐savings of $140 per patient (Figure 2).
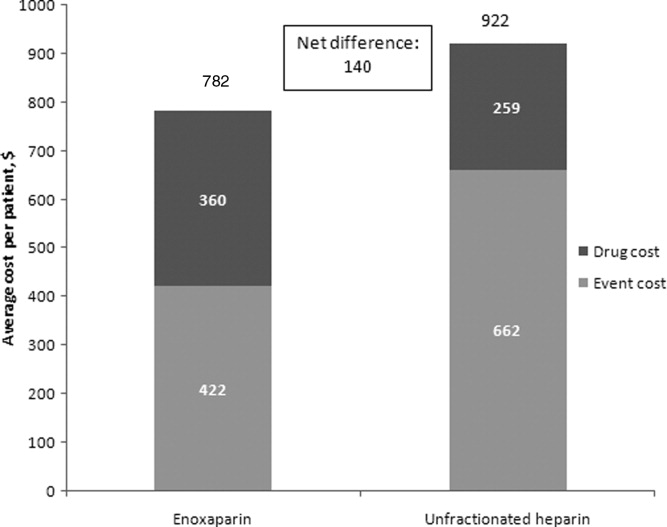
The cost estimates according to the stroke severity score (NIHSS scores <14 vs 14) are described in Table 3. The drug costs were consistent, regardless of stroke severity, for enoxaparin ($360) and for UFH ($259). However, in both treatment groups, the event costs were higher in patients with more severe stroke, compared with less severe stroke. For example, in the enoxaparin group, the event costs were $686 in patients with NIHSS scores 14 and $326 in patients with NIHSS scores <14. Nevertheless, the overall costs (event costs plus drug costs) were lower with enoxaparin compared with UFH, both in patients with less severe and more severe stroke. In fact, the total hospital cost‐savings were greater when enoxaparin was used instead of UFH in patients with more severe stroke (cost‐saving $287 if NIHSS score 14 vs $71 if NIHSS score <14) (Table 3).
Enoxaparin ($) | UFH ($) | Difference ($ [UFHEnoxaparin]) | |
---|---|---|---|
| |||
NIHSS score <14 | |||
Mean event costs per patient | 326 | 497 | 171 |
Mean drug costs per patient* | 360 | 259 | 101 |
Total costs | 685 | 756 | 71 |
NIHSS score 14 | |||
Mean event costs per patient | 686 | 1,073 | 387 |
Mean drug costs per patient* | 360 | 259 | 101 |
Total costs | 1,046 | 1,332 | 287 |
Multiple sensitivity analyses were performed. In the base case univariate sensitivity analysis, individual costs were adjusted by 20% (Table 4). If the cost of DVT increased by 20% (from $3003 to $3604) the difference between the enoxaparin and UFH groups was $187. When the cost of DVT was decreased by 20% to $2402, enoxaparin was still cost‐saving, with a difference of $94. For each of the individual cost parameters that were varied (DVT, PE, intracranial hemorrhage, major extracranial hemorrhage, and minor hemorrhage), enoxaparin was always less costly than UFH. Subsequent sensitivity analyses were performed (not shown) where cost parameters were varied by 5%, 10%, 15%, 30%, and 40%. Enoxaparin remained less costly than UFH in all cases.
Event | Baseline Cost Input ($) | +20% Cost Input ($) | +20% Difference ($ [UFH Enoxaparin]) (% Change) | 20% Cost Input ($) | 20% Difference ($ [UFH Enoxaparin]) (% Change) |
---|---|---|---|---|---|
| |||||
Deep‐vein thrombosis | 3,003 | 3,604 | 187 (33) | 2,402 | 94 (33) |
Pulmonary embolism | 2,143 | 2,572 | 144 (2.5) | 1,714 | 137 (2.5) |
Intracranial hemorrhage | 4,001 | 4,801 | 142 (1.3) | 3,201 | 138 (1.3) |
Major extracranial hemorrhage | 3,534 | 4,241 | 134 (4.0) | 2,827 | 146 (4.0) |
Minor hemorrhage | 1,322 | 1,586 | 142 (1.3) | 1,058 | 138 (1.3) |
A multivariate analysis was performed using a Monte Carlo simulation in TreeAge Pro (Figure 3). When all parameters were varied simultaneously (by 5%, 10%, 15%, 20%, 30%, and 40%) and the differences in costs between the enoxaparin and UFH groups were measured and plotted, the mean (standard deviation) difference between enoxaparin and UFH prophylaxis was $140 ($79) (Figure 3). Figure 4 shows a graphical presentation of the sensitivity analysis results for event probabilities and costs. Differences in enoxaparin drug costs, hospital costs for DVT, and probability of DVT for patients on enoxaparin are the factors that have the greatest effect on the overall cost.
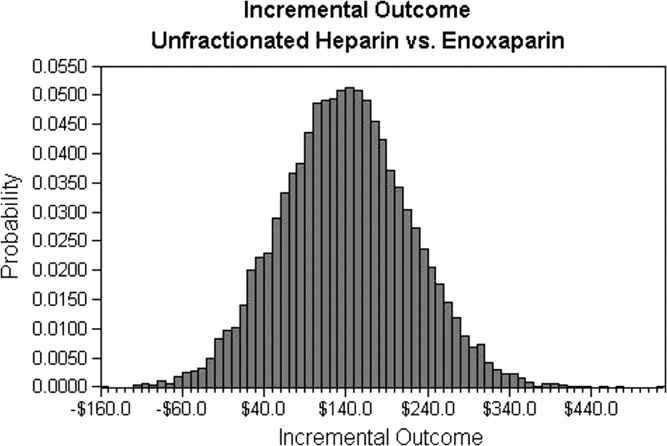
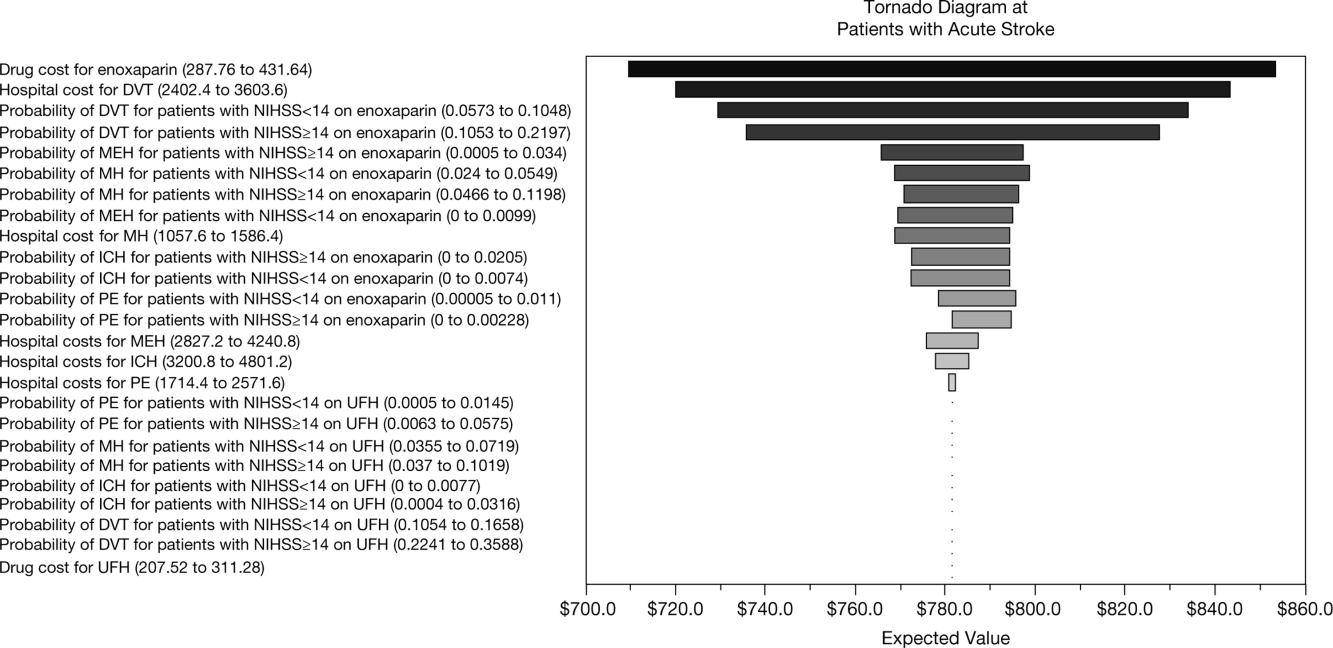
Finally, an additional scenario was performed using a published ratio of asymptomatic DVT to symptomatic VTE, due to the fact that not all VTE events in the real‐world present with symptoms prompting treatment. Quinlan et al. determined a ratio of asymptomatic DVT to symptomatic VTE of 5 for total hip replacement patients and of 21 for total knee replacement patients.28 Although derived from different patient populations who received different anticoagulants, we utilized the symptomatic event rates from the pooled studies to recalculate cost differences between enoxaparin and UFH in acute ischemic stroke. Using only symptomatic event rates, based on the 21:1 ratio in patients undergoing total knee replacement, the total cost for enoxaparin was $485 compared to $386 for UFH. Similar results were found based on the 5:1 ratio in patients with total hip replacement (enoxaparin $532 vs $472 for UFH). This was the only scenario where the higher drug cost of prophylaxis with enoxaparin was not completely offset by the reduction in events compared to UFH, likely due to the smaller difference in event rates once examining only symptomatic VTE.
DISCUSSION
This analysis demonstrates that, from the hospital perspective, enoxaparin 40 mg subcutaneously once‐daily is associated with lower total hospital costs and is more cost‐effective than twice‐daily UFH 5000 U subcutaneously for the prevention of VTE in patients with acute ischemic stroke. Despite higher drug‐acquisition costs, enoxaparin was associated with total cost‐savings of $140 per patient. This is due to the lower event rates with enoxaparin compared with UFH.
Previous studies, using hospital or payer information, have shown that VTE prophylaxis is more cost‐effective compared with no prophylaxis. In terms of the different VTE prophylaxis regimens, enoxaparin represents a more cost‐effective option in comparison with UFH19, 21, 2932 and also when compared with fondaparinux.21, 33 When comparing the results between different trials, it should be noted that previous analyses were mainly modeled on the Prophylaxis in Medical Patients with Enoxaparin (MEDENOX) study, which was performed in general medical patients and reported a VTE rate of 5.5%.6 However, patients with acute ischemic stroke are at a higher risk of VTE, with a 10% incidence of VTE reported in the PREVAIL study.14 Furthermore, twice‐daily rather than three‐timesdaily administration of UFH was used in the PREVAIL study, based on the current practice patterns seen during the PREVAIL trial design.
A recent retrospective analysis of transactional billing records demonstrated that, despite higher mean costs of anticoagulation therapy, the mean, total, adjusted direct hospital costs were lower with LMWH thromboprophylaxis compared with UFH ($7358 vs $8680, respectively; difference $1322; P < 0.001).21 A previous study by Burleigh and colleagues based on hospital discharge information extracted from both medical and surgical patients, has a sub‐analysis in patients with stroke. In these patients also, the total costs were lower for enoxaparin compared with UFH ($8608 vs $8911, respectively; difference $303).29 In the Burleigh study, drug costs and total discharge costs (eg, room and board, laboratory, and diagnostic imaging) were derived from drug charges and total charges, and were converted to estimated costs using cost‐to‐charge methods, so the absolute figures are not directly comparable with the current analysis.
This study adds to current literature by using data from a prospective study to analyze the hospital costs of VTE prophylaxis in stroke patients. The current study also provides a valuable cost‐analysis regarding a specific subgroup of medical patients at particularly high risk of VTE, and provides an economic comparison among stroke patients with NIHSS scores of <14 versus 14. In the PREVAIL study, despite a 2‐fold higher incidence of VTE in patients with more severe stroke (16.3% vs 8.3%), a similar reduction in VTE risk was observed with enoxaparin versus UFH in patients with NIHSS scores of 14 (odds ratio = 0.56; 95% CI = 0.37‐0.84; P = 0.0036) and <14 (odds ratio = 0.46; 95% CI = 0.27‐0.78; P = 0.0043).14 Enoxaparin was shown to be cost‐saving relative to UFH in both patient groups and, in particular, in patients with more severe stroke.
Potential limitations of the current analysis include the applicability of the figures obtained from the highly selected clinical trial population to real‐world clinical practice, and the fact that it is difficult to match cost estimates to trial data definitions. For example, this analysis was conducted with a comparator of twice‐daily UFH (as opposed to three‐timesdaily) which may be used in the real‐world setting and may have resulted in the increased number of events in the UFH group seen in the PREVAIL study. Due to a variety of differences between real‐world practice patterns and the PREVAIL clinical trial, we can only speculate as to the true cost‐consequences of utilizing enoxaparin versus UFH.
Furthermore, the original model did not include a sub‐analysis regarding the rates and, therefore, costs of proximal/symptomatic VTE. In the primary study of PREVAIL, the rates of symptomatic DVT were 1 in 666 patients (<1%) for enoxaparin and 4 in 669 patients (1%) for UFH, whereas the rates of proximal DVT were 30 in 666 patients (5%) and 64 in 669 patients (10%), respectively. Sensitivity analyses were performed to investigate the impact of lower rates of both DVT and PE (up to 40%), and the differences between groups were found to be robust. However, it is important to note that overall costs for both groups may have been increased through the inclusion of asymptomatic costs, with a more distinct separation of these costs making for a good follow‐up study. In a similar cost‐analysis we performed based on the PREVAIL study, which assessed the cost to the payer, we included an analysis of costs according to 3 different VTE definitions: the PREVAIL VTE definition (as in the current study); a definition of major VTE (PE, symptomatic DVT, and asymptomatic proximal DVT); and primary endpoints recommended by the European Medicines Agency Committee for Medicinal Products for Human Use for studies on VTE (proximal DVT, nonfatal PE, and all‐cause mortality). We found similar results irrespective of clinical event definitions.34 In an additional model scenario using a published ratio of asymptomatic DVT to symptomatic VTE,28 the higher drug cost of prophylaxis with enoxaparin was not completely offset by the reduction in events compared to UFH. This was likely due to the smaller difference in event rates once examining only symptomatic VTE. This scenario was limited by the fact that the ratio was derived from different patient populations receiving different anticoagulants than stroke patients.
In conclusion, data from this analysis adds to the evidence that, from the hospital perspective, the higher drug cost of enoxaparin is offset by the economic consequences of the events avoided as compared with UFH for the prevention of VTE following acute ischemic stroke, particularly in patients with severe stroke.
Acknowledgements
The authors thank Aylin Lee from I3 Innovus for her contribution to this study. The authors also acknowledge Min Chen for her assistance in statistical analysis, and Essy Mozaffari for his contribution to this study.
- Venous thromboembolism: disease burden, outcomes and risk factors.J Thromb Haemost.2005;3:1611–1617. .
- The epidemiology of venous thromboembolism in the community: implications for prevention and management.J Thromb Thrombolysis.2006;21:23–29. .
- Relative impact of risk factors for deep vein thrombosis and pulmonary embolism: a population‐based study.Arch Intern Med.2002;162:1245–1248. , , , et al.
- Risk factors for deep vein thrombosis and pulmonary embolism: a population‐based case‐control study.Arch Intern Med.2000;160:809–815. , , , , , .
- A safety analysis of thromboprophylaxis in acute medical illness.Thromb Haemost.2003;89:590–591. , .
- Quantification of risk factors for venous thromboembolism: a preliminary study for the development of a risk assessment tool.Haematologica.2003;88:1410–1421. , , , , .
- Prevalence of venous thromboembolism in acute hemorrhagic and thromboembolic stroke.Am J Phys Med Rehabil.2003;82:364–369. , .
- Thromboprophylaxis rates in US medical centers: success or failure?J Thromb Haemost.2007;5:1610–1616. , , , .
- Complications after acute stroke.Stroke.1996;27:415–420. , , , .
- Venous thromboembolism after acute stroke.Stroke.2001;32:262–267. , , , .
- Enoxaparin vs heparin for prevention of deep‐vein thrombosis in acute ischaemic stroke: a randomized, double‐blind study.Acta Neurol Scand.2002;106:84–92. , , , , , .
- Low‐molecular‐weight heparins or heparinoids versus standard unfractionated heparin for acute ischaemic stroke.Cochrane Database Syst Rev.2008;(3):CD000119. , , .
- for the PROTECT Trial Group.Prophylaxis of thrombotic and embolic events in acute ischemic stroke with the low‐molecular‐weight heparin certoparin: results of the PROTECT Trial.Stroke.2006;37:139–144. , , , et al;
- for the PREVAIL Investigators.The efficacy and safety of enoxaparin versus unfractionated heparin for the prevention of venous thromboembolism after acute ischaemic stroke (PREVAIL study): an open‐label randomised comparison.Lancet.2007;369:1347–1355. , , , et al;
- for the American College of Chest Physicians.Prevention of venous thromboembolism: American College of Chest Physicians Evidence‐Based Clinical Practice Guidelines (8th ed).Chest.2008;133(6 suppl):381S–453S. , , , et al;
- for the American College of Chest Physicians.Antithrombotic and thrombolytic therapy for ischemic stroke: American College of Chest Physicians Evidence‐Based Clinical Practice Guidelines (8th ed).Chest.2008;133(6 suppl):630S–669S. , , , , ;
- Management of acute proximal deep vein thrombosis: pharmacoeconomic evaluation of outpatient treatment with enoxaparin vs inpatient treatment with unfractionated heparin.Chest.2002;122:108–114. , , , .
- Economic evaluation of enoxaparin as prophylaxis against venous thromboembolism in seriously ill medical patients: a US perspective.Am J Manag Care.2002;8:1082–1088. , .
- Cost effectiveness of thromboprophylaxis with a low‐molecular‐weight heparin versus unfractionated heparin in acutely ill medical inpatients.Am J Manag Care.2004;10:632–642. , , , .
- Cost effectiveness of enoxaparin as prophylaxis against venous thromboembolic complications in acutely ill medical inpatients: modelling study from the hospital perspective in Germany.Pharmacoeconomics.2006;24:571–591. , , , et al.
- Hospital‐based costs associated with venous thromboembolism prophylaxis regimens.J Thromb Thrombolysis.2010;29:449–458. , , , , .
- for the ENDORSE Investigators.Venous thromboembolism risk and prophylaxis in the acute hospital care setting (ENDORSE study): a multinational cross‐sectional study.Lancet.2008;371:387–394. , , , et al;
- for the IMPROVE Investigators.Venous thromboembolism prophylaxis in acutely ill hospitalized medical patients: findings from the International Medical Prevention Registry on Venous Thromboembolism.Chest.2007;132:936–945. , , , et al;
- United States Department of Health August 2008. Available at: http://www.ahrq.gov/qual/vtguide/. Accessed August 18,2010.
- Enoxaparin versus unfractionated heparin in the prevention of venous thromboembolism after acute ischemic stroke: rationale, design, and methods of an open‐label, randomized, parallel‐group multicenter trial.J Stroke Cerebrovasc Dis.2005;14:95–100. , , , .
- Association between asymptomatic deep vein thrombosis detected by venography and symptomatic venous thromboembolism in patients undergoing elective hip or knee surgery.J Thromb Haemost.2007;5:1438–1443. , , , , , .
- Thromboprophylaxis in medically ill patients at risk for venous thromboembolism.Am J Health Syst Pharm.2006;63(20 suppl 6):S23–S29. , , , et al.
- Comparison of the two‐year outcomes and costs of prophylaxis in medical patients at risk of venous thromboembolism.Thromb Haemost.2008;100:810–820. , , , .
- Cost for inpatient care of venous thrombosis: a trial of enoxaparin vs standard heparin.Arch Intern Med.2000;160:3160–3165. , , , , , .
- Economic evaluation of enoxaparin for the prevention of venous thromboembolism in acutely ill medical patients.Pharm World Sci.2004;26:214–220. , , , .
- Total hospital‐based costs of enoxaparin or fondaparinux prophylaxis in patients at risk of venous thromboembolism [abstract]. Presented at the Chest 2008 Annual Meeting; October 25–30,2008; Philadelphia, PA. , , , , .
- Economic impact of enoxaparin after acute ischemic stroke based on PREVAIL.Clin Appl Thromb Hemost.2011;17:150–157. , , , , .
- Venous thromboembolism: disease burden, outcomes and risk factors.J Thromb Haemost.2005;3:1611–1617. .
- The epidemiology of venous thromboembolism in the community: implications for prevention and management.J Thromb Thrombolysis.2006;21:23–29. .
- Relative impact of risk factors for deep vein thrombosis and pulmonary embolism: a population‐based study.Arch Intern Med.2002;162:1245–1248. , , , et al.
- Risk factors for deep vein thrombosis and pulmonary embolism: a population‐based case‐control study.Arch Intern Med.2000;160:809–815. , , , , , .
- A safety analysis of thromboprophylaxis in acute medical illness.Thromb Haemost.2003;89:590–591. , .
- Quantification of risk factors for venous thromboembolism: a preliminary study for the development of a risk assessment tool.Haematologica.2003;88:1410–1421. , , , , .
- Prevalence of venous thromboembolism in acute hemorrhagic and thromboembolic stroke.Am J Phys Med Rehabil.2003;82:364–369. , .
- Thromboprophylaxis rates in US medical centers: success or failure?J Thromb Haemost.2007;5:1610–1616. , , , .
- Complications after acute stroke.Stroke.1996;27:415–420. , , , .
- Venous thromboembolism after acute stroke.Stroke.2001;32:262–267. , , , .
- Enoxaparin vs heparin for prevention of deep‐vein thrombosis in acute ischaemic stroke: a randomized, double‐blind study.Acta Neurol Scand.2002;106:84–92. , , , , , .
- Low‐molecular‐weight heparins or heparinoids versus standard unfractionated heparin for acute ischaemic stroke.Cochrane Database Syst Rev.2008;(3):CD000119. , , .
- for the PROTECT Trial Group.Prophylaxis of thrombotic and embolic events in acute ischemic stroke with the low‐molecular‐weight heparin certoparin: results of the PROTECT Trial.Stroke.2006;37:139–144. , , , et al;
- for the PREVAIL Investigators.The efficacy and safety of enoxaparin versus unfractionated heparin for the prevention of venous thromboembolism after acute ischaemic stroke (PREVAIL study): an open‐label randomised comparison.Lancet.2007;369:1347–1355. , , , et al;
- for the American College of Chest Physicians.Prevention of venous thromboembolism: American College of Chest Physicians Evidence‐Based Clinical Practice Guidelines (8th ed).Chest.2008;133(6 suppl):381S–453S. , , , et al;
- for the American College of Chest Physicians.Antithrombotic and thrombolytic therapy for ischemic stroke: American College of Chest Physicians Evidence‐Based Clinical Practice Guidelines (8th ed).Chest.2008;133(6 suppl):630S–669S. , , , , ;
- Management of acute proximal deep vein thrombosis: pharmacoeconomic evaluation of outpatient treatment with enoxaparin vs inpatient treatment with unfractionated heparin.Chest.2002;122:108–114. , , , .
- Economic evaluation of enoxaparin as prophylaxis against venous thromboembolism in seriously ill medical patients: a US perspective.Am J Manag Care.2002;8:1082–1088. , .
- Cost effectiveness of thromboprophylaxis with a low‐molecular‐weight heparin versus unfractionated heparin in acutely ill medical inpatients.Am J Manag Care.2004;10:632–642. , , , .
- Cost effectiveness of enoxaparin as prophylaxis against venous thromboembolic complications in acutely ill medical inpatients: modelling study from the hospital perspective in Germany.Pharmacoeconomics.2006;24:571–591. , , , et al.
- Hospital‐based costs associated with venous thromboembolism prophylaxis regimens.J Thromb Thrombolysis.2010;29:449–458. , , , , .
- for the ENDORSE Investigators.Venous thromboembolism risk and prophylaxis in the acute hospital care setting (ENDORSE study): a multinational cross‐sectional study.Lancet.2008;371:387–394. , , , et al;
- for the IMPROVE Investigators.Venous thromboembolism prophylaxis in acutely ill hospitalized medical patients: findings from the International Medical Prevention Registry on Venous Thromboembolism.Chest.2007;132:936–945. , , , et al;
- United States Department of Health August 2008. Available at: http://www.ahrq.gov/qual/vtguide/. Accessed August 18,2010.
- Enoxaparin versus unfractionated heparin in the prevention of venous thromboembolism after acute ischemic stroke: rationale, design, and methods of an open‐label, randomized, parallel‐group multicenter trial.J Stroke Cerebrovasc Dis.2005;14:95–100. , , , .
- Association between asymptomatic deep vein thrombosis detected by venography and symptomatic venous thromboembolism in patients undergoing elective hip or knee surgery.J Thromb Haemost.2007;5:1438–1443. , , , , , .
- Thromboprophylaxis in medically ill patients at risk for venous thromboembolism.Am J Health Syst Pharm.2006;63(20 suppl 6):S23–S29. , , , et al.
- Comparison of the two‐year outcomes and costs of prophylaxis in medical patients at risk of venous thromboembolism.Thromb Haemost.2008;100:810–820. , , , .
- Cost for inpatient care of venous thrombosis: a trial of enoxaparin vs standard heparin.Arch Intern Med.2000;160:3160–3165. , , , , , .
- Economic evaluation of enoxaparin for the prevention of venous thromboembolism in acutely ill medical patients.Pharm World Sci.2004;26:214–220. , , , .
- Total hospital‐based costs of enoxaparin or fondaparinux prophylaxis in patients at risk of venous thromboembolism [abstract]. Presented at the Chest 2008 Annual Meeting; October 25–30,2008; Philadelphia, PA. , , , , .
- Economic impact of enoxaparin after acute ischemic stroke based on PREVAIL.Clin Appl Thromb Hemost.2011;17:150–157. , , , , .
Copyright © 2011 Society of Hospital Medicine