User login
Lean-Based Redesign of Multidisciplinary Rounds on General Medicine Service
Given that multiple disciplines are often involved in caring for patients admitted to the hospital, timely communication, collaboration, and coordination amongst various disciplines is necessary for safe and effective patient care.1 With the focus on improving patient satisfaction and throughput in hospitals, it is also important to make more accurate predictions of the discharge date and allow time for patients and their families to prepare for discharge.2-4
Multidisciplinary rounds (MDR) are defined as structured daily communication amongst key members of the patient’s care team (eg, nurses, physicians, case managers, social workers, pharmacists, and rehabilitation services). MDR have shown to be a useful strategy for ensuring that all members of the care team are updated on the plan of care for the patient.5 During MDR, a brief “check-in” discussing the patient’s plan of care, pending needs, and barriers to discharge allows all team members, patients, and families to effectively coordinate care and plan and prepare for discharge.
Multiple studies have reported increased collaboration and improved communication between disciplines with the use of such multidisciplinary rounding.2,5-7 Additionally, MDR have been shown to improve patient outcomes8 and reduce adverse events,9 length of stay (LOS),6,8 cost of care,8 and readmissions.1
We redesigned MDR on the general medicine wards at our institution in October 2014 by using Lean management techniques. Lean is defined as a set of philosophies and methods that aim to create transformation in thinking, behavior, and culture in each process, with the goal of maximizing the value for the patients and providers, adding efficiency, and reducing waste and waits.10
In this study, we evaluate whether this new model of MDR was associated with a decrease in the LOS. We also evaluate whether this new model of MDR was associated with an increase in discharges before noon, documentation of estimated discharge date (EDD) in our electronic health record (EHR), and patient satisfaction.
METHODS
Setting, Design, and Patients
The study was conducted on the teaching general medicine service at our institution, an urban, 484-bed academic hospital. The general medicine service has patients on 4 inpatient units (total of 95 beds) and is managed by 5 teaching service teams.
We performed a pre-post study. The preperiod (in which the old model of MDR was followed) included 4000 patients discharged between September 1, 2013, and October 22, 2014. The postperiod (in which the new model of MDR was followed) included 2085 patients discharged between October 23, 2014, and April 30, 2015. We excluded 139 patients that died in the hospital prior to discharge and patients on the nonteaching and/or private practice service.
All data were provided by our institution’s Digital Solutions Department. Our institutional review board issued a letter of determination exempting this study from further review because it was deemed to be a quality improvement initiative.
Use of Lean Management to Redesign our MDR
Our institution has incorporated the Lean management system to continually add value to services through the elimination of waste, thus simultaneously optimizing the quality of patient care, cost, and patient satisfaction.11 Lean, derived from the Toyota Production System, has long been used in manufacturing and in recent decades has spread to healthcare.12 We leveraged the following 3 key Lean techniques to redesign our MDR: (1) value stream management (VSM), (2) rapid process improvement workshops (RPIW), and (3) active daily management (ADM), as detailed in supplementary Appendix 1.
Interventions
Outcomes
The primary outcome was mean LOS. The secondary outcomes were (1) discharges before noon, (2) recording of the EDD in our EHR within 24 hours of admission (as time stamped on our EHR), and (3) patient satisfaction.
Data for patient satisfaction were obtained using the Press Ganey survey. We used data on patient satisfaction scores for the following 2 relevant questions on this survey: (1) extent to which the patient felt ready to be discharged and (2) how well staff worked together to care for the patient. Proportions of the “top-box” (“very good”) were used for the analysis. These survey data were available on 467 patients (11.7%) in the preperiod and 188 patients (9.0%) in the postperiod.
Data Analysis
A sensitivity analysis was conducted on a second cohort that included a subset of patients from the preperiod between November 1, 2013, and April 30, 2014, and a subset of patients from the postperiod between November 1, 2014, and April 1, 2015, to control for the calendar period (supplementary Appendix 2).
All analyses were conducted in R version 3.3.0, with the linear mixed-effects model lme4 statistical package.13,14
RESULTS
Table 3 shows the differences in the outcomes between the pre- and postperiods. There was no change in the LOS or LOS adjusted for CMI. There was a 3.9% increase in discharges before noon in the postperiod compared with the preperiod (95% CI, 2.4% to 5.3%; P < .001). There was a 9.9% increase in the percentage of patients for whom the EDD was recorded in our EHR within 24 hours of admission (95% CI, 7.4% to 12.4%; P < .001). There was no change in the “top-box” patient satisfaction scores.
There were only marginal differences in the results between the entire cohort and a second subset cohort used for sensitivity analysis (supplementary Appendix 2).
DISCUSSION
In our study, there was no change in the mean LOS with the new model of MDR. There was an increase in discharges before noon and in recording of the EDD in our EHR within 24 hours of admission in the postperiod when the Lean-based new model of MDR was utilized. There was no change in patient satisfaction. With no change in staffing, we were able to accommodate the increase in the discharge volume in the postperiod.
We believe our results are attributable to several factors, including clearly defined roles and responsibilities for all participants of MDR, the inclusion of more experienced general medicine attending physician (compared with housestaff), Lean management techniques to identify gaps in the patient’s journey from emergency department to discharge using VSM, the development of appropriate workflows and standard work on how the multidisciplinary teams would work together at RPIWs, and ADM to ensure sustainability and engagement among frontline members and institutional leaders. In order to sustain this, we planned to continue monitoring data in daily, weekly, and monthly forums with senior physician and administrative leaders. Planning for additional interventions is underway, including moving MDR to the bedside, instituting an afternoon “check-in” that would enable more detailed action planning, and addressing barriers in a timely manner for patients ready to discharge the following day.
Our study has a few limitations. First, this is an observational study that cannot determine causation. Second, this is a single-center study conducted on patients only on the general medicine teaching service. Third, there were several concurrent interventions implemented at our institution to improve LOS, throughput, and patient satisfaction in addition to MDR, thus making it difficult to isolate the impact of our intervention. Fourth, in the new model of MDR, rounds took place only 5 days per week, thereby possibly limiting the potential impact on our outcomes. Fifth, while we showed improvements in the discharges before noon and recording of EDD in the post period, we were not able to achieve our target of 25% discharges before noon or 100% recording of EDD in this time period. We believe the limited amount of time between the pre- and postperiods to allow for adoption and learning of the processes might have contributed to the underestimation of the impact of the new model of MDR, thereby limiting our ability to achieve our targets. Sixth, the response rate on the Press Ganey survey was low, and we did not directly survey patients or families for their satisfaction with MDR.
Our study has several strengths. To our knowledge, this is the first study to embed Lean management techniques in the design of MDR in the inpatient setting. While several studies have demonstrated improvements in discharges before noon through the implementation of MDR, they have not incorporated Lean management techniques, which we believe are critical to ensure the sustainability of results.1,3,5,6,8,15 Second, while it was not measured, there was a high level of provider engagement in the process in the new model of MDR. Third, because the MDR were conducted at the nurse’s station on each inpatient unit in the new model instead of in a conference room, it was well attended by all members of the multidisciplinary team. Fourth, the presence of a visibility board allowed for all team members to have easy access to visual feedback throughout the day, even if they were not present at the MDR. Fifth, we believe that there was also more accurate estimation of the date and time of discharge in the new model of MDR because the discussion was facilitated by the case manager, who is experienced in identifying barriers to discharge (compared with the housestaff in the old model of MDR), and included the more experienced attending physician. Finally, the consistent presence of a multidisciplinary team at MDR allowed for the incorporation of everyone’s concerns at one time, thereby limiting the need for paging multiple disciplines throughout the day, which led to quicker resolution of issues and assignment of pending tasks.
In conclusion, our study shows no change in the mean LOS when the Lean-based model of MDR was utilized. Our study demonstrates an increase in discharges before noon and in recording of EDD on our EHR within 24 hours of admission in the post period when the Lean-based model of MDR was utilized. There was no change in patient satisfaction. While this study was conducted at an academic medical center on the general medicine wards, we believe our new model of MDR, which leveraged Lean management techniques, may successfully impact patient flow in all inpatient clinical services and nonteaching hospitals.
Disclosure
The authors report no financial conflicts of interest and have nothing to disclose.
1. Townsend-Gervis M, Cornell P, Vardaman JM. Interdisciplinary Rounds and Structured Communication Reduce Re-Admissions and Improve Some Patient Outcomes. West J Nurs Res. 2014;36(7):917-928. PubMed
2. Vazirani S, Hays RD, Shapiro MF, Cowan M. Effect of a multidisciplinary intervention on communication and collaboration among physicians and nurses. Am J Crit Care. 2005;14(1):71-77. PubMed
3. Wertheimer B, Jacobs RE, Bailey M, et al. Discharge before noon: an achievable hospital goal. J Hosp Med. 2014;9(4):210-214. PubMed
4. Wertheimer B, Jacobs RE, Iturrate E, Bailey M, Hochman K. Discharge before noon: Effect on throughput and sustainability. J Hosp Med. 2015;10(10):664-669. PubMed
5. Halm MA, Gagner S, Goering M, Sabo J, Smith M, Zaccagnini M. Interdisciplinary rounds: impact on patients, families, and staff. Clin Nurse Spec. 2003;17(3):133-142. PubMed
6. O’Mahony S, Mazur E, Charney P, Wang Y, Fine J. Use of multidisciplinary rounds to simultaneously improve quality outcomes, enhance resident education, and shorten length of stay. J Gen Intern Med. 2007;22(8):1073-1079. PubMed
7. Reimer N, Herbener L. Round and round we go: rounding strategies to impact exemplary professional practice. Clin J Oncol Nurs. 2014;18(6):654-660. PubMed
8. Curley C, McEachern JE, Speroff T. A firm trial of interdisciplinary rounds on the inpatient medical wards: an intervention designed using continuous quality improvement. Med Care. 1998;36(8 Suppl):AS4-AS12. PubMed
9. Baggs JG, Ryan SA, Phelps CE, Richeson JF, Johnson JE. The association between interdisciplinary collaboration and patient outcomes in a medical intensive care unit. Heart Lung. 1992;21(1):18-24. PubMed
10. Lawal AK, Rotter T, Kinsman L, et al. Lean management in health care: definition, concepts, methodology and effects reported (systematic review protocol). Syst Rev. 2014;3:103. PubMed
11. Liker JK. Toyota Way: 14 Management Principles from the World’s Greatest Manufacturer. New York, Chicago, San Francisco, Athens, London, Madrid, Mexico City, Milan, New Delhi, Singapore, Sydney, Toronto: McGraw-Hill Education; 2004.
12. Kane M, Chui K, Rimicci J, et al. Lean Manufacturing Improves Emergency Department Throughput and Patient Satisfaction. J Nurs Adm. 2015;45(9):429-434. PubMed
13. R Core Team. R: A language and environment for statistical computing. R Foundation for Statistical Computing, Vienna, Austria. 2016. http://www.R-project.org/. Accessed November 7, 2017.
14. Bates D, Mächler M, Bolker B, Walker S. Fitting Linear Mixed-Effects Models Using lme4. J Stat Softw. 2015;67(1):1-48.
15. O’Leary KJ, Buck R, Fligiel HM, et al. Structured interdisciplinary rounds in a medical teaching unit: improving patient safety. Arch Intern Med. 2011;171(7):678-684. PubMed
Given that multiple disciplines are often involved in caring for patients admitted to the hospital, timely communication, collaboration, and coordination amongst various disciplines is necessary for safe and effective patient care.1 With the focus on improving patient satisfaction and throughput in hospitals, it is also important to make more accurate predictions of the discharge date and allow time for patients and their families to prepare for discharge.2-4
Multidisciplinary rounds (MDR) are defined as structured daily communication amongst key members of the patient’s care team (eg, nurses, physicians, case managers, social workers, pharmacists, and rehabilitation services). MDR have shown to be a useful strategy for ensuring that all members of the care team are updated on the plan of care for the patient.5 During MDR, a brief “check-in” discussing the patient’s plan of care, pending needs, and barriers to discharge allows all team members, patients, and families to effectively coordinate care and plan and prepare for discharge.
Multiple studies have reported increased collaboration and improved communication between disciplines with the use of such multidisciplinary rounding.2,5-7 Additionally, MDR have been shown to improve patient outcomes8 and reduce adverse events,9 length of stay (LOS),6,8 cost of care,8 and readmissions.1
We redesigned MDR on the general medicine wards at our institution in October 2014 by using Lean management techniques. Lean is defined as a set of philosophies and methods that aim to create transformation in thinking, behavior, and culture in each process, with the goal of maximizing the value for the patients and providers, adding efficiency, and reducing waste and waits.10
In this study, we evaluate whether this new model of MDR was associated with a decrease in the LOS. We also evaluate whether this new model of MDR was associated with an increase in discharges before noon, documentation of estimated discharge date (EDD) in our electronic health record (EHR), and patient satisfaction.
METHODS
Setting, Design, and Patients
The study was conducted on the teaching general medicine service at our institution, an urban, 484-bed academic hospital. The general medicine service has patients on 4 inpatient units (total of 95 beds) and is managed by 5 teaching service teams.
We performed a pre-post study. The preperiod (in which the old model of MDR was followed) included 4000 patients discharged between September 1, 2013, and October 22, 2014. The postperiod (in which the new model of MDR was followed) included 2085 patients discharged between October 23, 2014, and April 30, 2015. We excluded 139 patients that died in the hospital prior to discharge and patients on the nonteaching and/or private practice service.
All data were provided by our institution’s Digital Solutions Department. Our institutional review board issued a letter of determination exempting this study from further review because it was deemed to be a quality improvement initiative.
Use of Lean Management to Redesign our MDR
Our institution has incorporated the Lean management system to continually add value to services through the elimination of waste, thus simultaneously optimizing the quality of patient care, cost, and patient satisfaction.11 Lean, derived from the Toyota Production System, has long been used in manufacturing and in recent decades has spread to healthcare.12 We leveraged the following 3 key Lean techniques to redesign our MDR: (1) value stream management (VSM), (2) rapid process improvement workshops (RPIW), and (3) active daily management (ADM), as detailed in supplementary Appendix 1.
Interventions
Outcomes
The primary outcome was mean LOS. The secondary outcomes were (1) discharges before noon, (2) recording of the EDD in our EHR within 24 hours of admission (as time stamped on our EHR), and (3) patient satisfaction.
Data for patient satisfaction were obtained using the Press Ganey survey. We used data on patient satisfaction scores for the following 2 relevant questions on this survey: (1) extent to which the patient felt ready to be discharged and (2) how well staff worked together to care for the patient. Proportions of the “top-box” (“very good”) were used for the analysis. These survey data were available on 467 patients (11.7%) in the preperiod and 188 patients (9.0%) in the postperiod.
Data Analysis
A sensitivity analysis was conducted on a second cohort that included a subset of patients from the preperiod between November 1, 2013, and April 30, 2014, and a subset of patients from the postperiod between November 1, 2014, and April 1, 2015, to control for the calendar period (supplementary Appendix 2).
All analyses were conducted in R version 3.3.0, with the linear mixed-effects model lme4 statistical package.13,14
RESULTS
Table 3 shows the differences in the outcomes between the pre- and postperiods. There was no change in the LOS or LOS adjusted for CMI. There was a 3.9% increase in discharges before noon in the postperiod compared with the preperiod (95% CI, 2.4% to 5.3%; P < .001). There was a 9.9% increase in the percentage of patients for whom the EDD was recorded in our EHR within 24 hours of admission (95% CI, 7.4% to 12.4%; P < .001). There was no change in the “top-box” patient satisfaction scores.
There were only marginal differences in the results between the entire cohort and a second subset cohort used for sensitivity analysis (supplementary Appendix 2).
DISCUSSION
In our study, there was no change in the mean LOS with the new model of MDR. There was an increase in discharges before noon and in recording of the EDD in our EHR within 24 hours of admission in the postperiod when the Lean-based new model of MDR was utilized. There was no change in patient satisfaction. With no change in staffing, we were able to accommodate the increase in the discharge volume in the postperiod.
We believe our results are attributable to several factors, including clearly defined roles and responsibilities for all participants of MDR, the inclusion of more experienced general medicine attending physician (compared with housestaff), Lean management techniques to identify gaps in the patient’s journey from emergency department to discharge using VSM, the development of appropriate workflows and standard work on how the multidisciplinary teams would work together at RPIWs, and ADM to ensure sustainability and engagement among frontline members and institutional leaders. In order to sustain this, we planned to continue monitoring data in daily, weekly, and monthly forums with senior physician and administrative leaders. Planning for additional interventions is underway, including moving MDR to the bedside, instituting an afternoon “check-in” that would enable more detailed action planning, and addressing barriers in a timely manner for patients ready to discharge the following day.
Our study has a few limitations. First, this is an observational study that cannot determine causation. Second, this is a single-center study conducted on patients only on the general medicine teaching service. Third, there were several concurrent interventions implemented at our institution to improve LOS, throughput, and patient satisfaction in addition to MDR, thus making it difficult to isolate the impact of our intervention. Fourth, in the new model of MDR, rounds took place only 5 days per week, thereby possibly limiting the potential impact on our outcomes. Fifth, while we showed improvements in the discharges before noon and recording of EDD in the post period, we were not able to achieve our target of 25% discharges before noon or 100% recording of EDD in this time period. We believe the limited amount of time between the pre- and postperiods to allow for adoption and learning of the processes might have contributed to the underestimation of the impact of the new model of MDR, thereby limiting our ability to achieve our targets. Sixth, the response rate on the Press Ganey survey was low, and we did not directly survey patients or families for their satisfaction with MDR.
Our study has several strengths. To our knowledge, this is the first study to embed Lean management techniques in the design of MDR in the inpatient setting. While several studies have demonstrated improvements in discharges before noon through the implementation of MDR, they have not incorporated Lean management techniques, which we believe are critical to ensure the sustainability of results.1,3,5,6,8,15 Second, while it was not measured, there was a high level of provider engagement in the process in the new model of MDR. Third, because the MDR were conducted at the nurse’s station on each inpatient unit in the new model instead of in a conference room, it was well attended by all members of the multidisciplinary team. Fourth, the presence of a visibility board allowed for all team members to have easy access to visual feedback throughout the day, even if they were not present at the MDR. Fifth, we believe that there was also more accurate estimation of the date and time of discharge in the new model of MDR because the discussion was facilitated by the case manager, who is experienced in identifying barriers to discharge (compared with the housestaff in the old model of MDR), and included the more experienced attending physician. Finally, the consistent presence of a multidisciplinary team at MDR allowed for the incorporation of everyone’s concerns at one time, thereby limiting the need for paging multiple disciplines throughout the day, which led to quicker resolution of issues and assignment of pending tasks.
In conclusion, our study shows no change in the mean LOS when the Lean-based model of MDR was utilized. Our study demonstrates an increase in discharges before noon and in recording of EDD on our EHR within 24 hours of admission in the post period when the Lean-based model of MDR was utilized. There was no change in patient satisfaction. While this study was conducted at an academic medical center on the general medicine wards, we believe our new model of MDR, which leveraged Lean management techniques, may successfully impact patient flow in all inpatient clinical services and nonteaching hospitals.
Disclosure
The authors report no financial conflicts of interest and have nothing to disclose.
Given that multiple disciplines are often involved in caring for patients admitted to the hospital, timely communication, collaboration, and coordination amongst various disciplines is necessary for safe and effective patient care.1 With the focus on improving patient satisfaction and throughput in hospitals, it is also important to make more accurate predictions of the discharge date and allow time for patients and their families to prepare for discharge.2-4
Multidisciplinary rounds (MDR) are defined as structured daily communication amongst key members of the patient’s care team (eg, nurses, physicians, case managers, social workers, pharmacists, and rehabilitation services). MDR have shown to be a useful strategy for ensuring that all members of the care team are updated on the plan of care for the patient.5 During MDR, a brief “check-in” discussing the patient’s plan of care, pending needs, and barriers to discharge allows all team members, patients, and families to effectively coordinate care and plan and prepare for discharge.
Multiple studies have reported increased collaboration and improved communication between disciplines with the use of such multidisciplinary rounding.2,5-7 Additionally, MDR have been shown to improve patient outcomes8 and reduce adverse events,9 length of stay (LOS),6,8 cost of care,8 and readmissions.1
We redesigned MDR on the general medicine wards at our institution in October 2014 by using Lean management techniques. Lean is defined as a set of philosophies and methods that aim to create transformation in thinking, behavior, and culture in each process, with the goal of maximizing the value for the patients and providers, adding efficiency, and reducing waste and waits.10
In this study, we evaluate whether this new model of MDR was associated with a decrease in the LOS. We also evaluate whether this new model of MDR was associated with an increase in discharges before noon, documentation of estimated discharge date (EDD) in our electronic health record (EHR), and patient satisfaction.
METHODS
Setting, Design, and Patients
The study was conducted on the teaching general medicine service at our institution, an urban, 484-bed academic hospital. The general medicine service has patients on 4 inpatient units (total of 95 beds) and is managed by 5 teaching service teams.
We performed a pre-post study. The preperiod (in which the old model of MDR was followed) included 4000 patients discharged between September 1, 2013, and October 22, 2014. The postperiod (in which the new model of MDR was followed) included 2085 patients discharged between October 23, 2014, and April 30, 2015. We excluded 139 patients that died in the hospital prior to discharge and patients on the nonteaching and/or private practice service.
All data were provided by our institution’s Digital Solutions Department. Our institutional review board issued a letter of determination exempting this study from further review because it was deemed to be a quality improvement initiative.
Use of Lean Management to Redesign our MDR
Our institution has incorporated the Lean management system to continually add value to services through the elimination of waste, thus simultaneously optimizing the quality of patient care, cost, and patient satisfaction.11 Lean, derived from the Toyota Production System, has long been used in manufacturing and in recent decades has spread to healthcare.12 We leveraged the following 3 key Lean techniques to redesign our MDR: (1) value stream management (VSM), (2) rapid process improvement workshops (RPIW), and (3) active daily management (ADM), as detailed in supplementary Appendix 1.
Interventions
Outcomes
The primary outcome was mean LOS. The secondary outcomes were (1) discharges before noon, (2) recording of the EDD in our EHR within 24 hours of admission (as time stamped on our EHR), and (3) patient satisfaction.
Data for patient satisfaction were obtained using the Press Ganey survey. We used data on patient satisfaction scores for the following 2 relevant questions on this survey: (1) extent to which the patient felt ready to be discharged and (2) how well staff worked together to care for the patient. Proportions of the “top-box” (“very good”) were used for the analysis. These survey data were available on 467 patients (11.7%) in the preperiod and 188 patients (9.0%) in the postperiod.
Data Analysis
A sensitivity analysis was conducted on a second cohort that included a subset of patients from the preperiod between November 1, 2013, and April 30, 2014, and a subset of patients from the postperiod between November 1, 2014, and April 1, 2015, to control for the calendar period (supplementary Appendix 2).
All analyses were conducted in R version 3.3.0, with the linear mixed-effects model lme4 statistical package.13,14
RESULTS
Table 3 shows the differences in the outcomes between the pre- and postperiods. There was no change in the LOS or LOS adjusted for CMI. There was a 3.9% increase in discharges before noon in the postperiod compared with the preperiod (95% CI, 2.4% to 5.3%; P < .001). There was a 9.9% increase in the percentage of patients for whom the EDD was recorded in our EHR within 24 hours of admission (95% CI, 7.4% to 12.4%; P < .001). There was no change in the “top-box” patient satisfaction scores.
There were only marginal differences in the results between the entire cohort and a second subset cohort used for sensitivity analysis (supplementary Appendix 2).
DISCUSSION
In our study, there was no change in the mean LOS with the new model of MDR. There was an increase in discharges before noon and in recording of the EDD in our EHR within 24 hours of admission in the postperiod when the Lean-based new model of MDR was utilized. There was no change in patient satisfaction. With no change in staffing, we were able to accommodate the increase in the discharge volume in the postperiod.
We believe our results are attributable to several factors, including clearly defined roles and responsibilities for all participants of MDR, the inclusion of more experienced general medicine attending physician (compared with housestaff), Lean management techniques to identify gaps in the patient’s journey from emergency department to discharge using VSM, the development of appropriate workflows and standard work on how the multidisciplinary teams would work together at RPIWs, and ADM to ensure sustainability and engagement among frontline members and institutional leaders. In order to sustain this, we planned to continue monitoring data in daily, weekly, and monthly forums with senior physician and administrative leaders. Planning for additional interventions is underway, including moving MDR to the bedside, instituting an afternoon “check-in” that would enable more detailed action planning, and addressing barriers in a timely manner for patients ready to discharge the following day.
Our study has a few limitations. First, this is an observational study that cannot determine causation. Second, this is a single-center study conducted on patients only on the general medicine teaching service. Third, there were several concurrent interventions implemented at our institution to improve LOS, throughput, and patient satisfaction in addition to MDR, thus making it difficult to isolate the impact of our intervention. Fourth, in the new model of MDR, rounds took place only 5 days per week, thereby possibly limiting the potential impact on our outcomes. Fifth, while we showed improvements in the discharges before noon and recording of EDD in the post period, we were not able to achieve our target of 25% discharges before noon or 100% recording of EDD in this time period. We believe the limited amount of time between the pre- and postperiods to allow for adoption and learning of the processes might have contributed to the underestimation of the impact of the new model of MDR, thereby limiting our ability to achieve our targets. Sixth, the response rate on the Press Ganey survey was low, and we did not directly survey patients or families for their satisfaction with MDR.
Our study has several strengths. To our knowledge, this is the first study to embed Lean management techniques in the design of MDR in the inpatient setting. While several studies have demonstrated improvements in discharges before noon through the implementation of MDR, they have not incorporated Lean management techniques, which we believe are critical to ensure the sustainability of results.1,3,5,6,8,15 Second, while it was not measured, there was a high level of provider engagement in the process in the new model of MDR. Third, because the MDR were conducted at the nurse’s station on each inpatient unit in the new model instead of in a conference room, it was well attended by all members of the multidisciplinary team. Fourth, the presence of a visibility board allowed for all team members to have easy access to visual feedback throughout the day, even if they were not present at the MDR. Fifth, we believe that there was also more accurate estimation of the date and time of discharge in the new model of MDR because the discussion was facilitated by the case manager, who is experienced in identifying barriers to discharge (compared with the housestaff in the old model of MDR), and included the more experienced attending physician. Finally, the consistent presence of a multidisciplinary team at MDR allowed for the incorporation of everyone’s concerns at one time, thereby limiting the need for paging multiple disciplines throughout the day, which led to quicker resolution of issues and assignment of pending tasks.
In conclusion, our study shows no change in the mean LOS when the Lean-based model of MDR was utilized. Our study demonstrates an increase in discharges before noon and in recording of EDD on our EHR within 24 hours of admission in the post period when the Lean-based model of MDR was utilized. There was no change in patient satisfaction. While this study was conducted at an academic medical center on the general medicine wards, we believe our new model of MDR, which leveraged Lean management techniques, may successfully impact patient flow in all inpatient clinical services and nonteaching hospitals.
Disclosure
The authors report no financial conflicts of interest and have nothing to disclose.
1. Townsend-Gervis M, Cornell P, Vardaman JM. Interdisciplinary Rounds and Structured Communication Reduce Re-Admissions and Improve Some Patient Outcomes. West J Nurs Res. 2014;36(7):917-928. PubMed
2. Vazirani S, Hays RD, Shapiro MF, Cowan M. Effect of a multidisciplinary intervention on communication and collaboration among physicians and nurses. Am J Crit Care. 2005;14(1):71-77. PubMed
3. Wertheimer B, Jacobs RE, Bailey M, et al. Discharge before noon: an achievable hospital goal. J Hosp Med. 2014;9(4):210-214. PubMed
4. Wertheimer B, Jacobs RE, Iturrate E, Bailey M, Hochman K. Discharge before noon: Effect on throughput and sustainability. J Hosp Med. 2015;10(10):664-669. PubMed
5. Halm MA, Gagner S, Goering M, Sabo J, Smith M, Zaccagnini M. Interdisciplinary rounds: impact on patients, families, and staff. Clin Nurse Spec. 2003;17(3):133-142. PubMed
6. O’Mahony S, Mazur E, Charney P, Wang Y, Fine J. Use of multidisciplinary rounds to simultaneously improve quality outcomes, enhance resident education, and shorten length of stay. J Gen Intern Med. 2007;22(8):1073-1079. PubMed
7. Reimer N, Herbener L. Round and round we go: rounding strategies to impact exemplary professional practice. Clin J Oncol Nurs. 2014;18(6):654-660. PubMed
8. Curley C, McEachern JE, Speroff T. A firm trial of interdisciplinary rounds on the inpatient medical wards: an intervention designed using continuous quality improvement. Med Care. 1998;36(8 Suppl):AS4-AS12. PubMed
9. Baggs JG, Ryan SA, Phelps CE, Richeson JF, Johnson JE. The association between interdisciplinary collaboration and patient outcomes in a medical intensive care unit. Heart Lung. 1992;21(1):18-24. PubMed
10. Lawal AK, Rotter T, Kinsman L, et al. Lean management in health care: definition, concepts, methodology and effects reported (systematic review protocol). Syst Rev. 2014;3:103. PubMed
11. Liker JK. Toyota Way: 14 Management Principles from the World’s Greatest Manufacturer. New York, Chicago, San Francisco, Athens, London, Madrid, Mexico City, Milan, New Delhi, Singapore, Sydney, Toronto: McGraw-Hill Education; 2004.
12. Kane M, Chui K, Rimicci J, et al. Lean Manufacturing Improves Emergency Department Throughput and Patient Satisfaction. J Nurs Adm. 2015;45(9):429-434. PubMed
13. R Core Team. R: A language and environment for statistical computing. R Foundation for Statistical Computing, Vienna, Austria. 2016. http://www.R-project.org/. Accessed November 7, 2017.
14. Bates D, Mächler M, Bolker B, Walker S. Fitting Linear Mixed-Effects Models Using lme4. J Stat Softw. 2015;67(1):1-48.
15. O’Leary KJ, Buck R, Fligiel HM, et al. Structured interdisciplinary rounds in a medical teaching unit: improving patient safety. Arch Intern Med. 2011;171(7):678-684. PubMed
1. Townsend-Gervis M, Cornell P, Vardaman JM. Interdisciplinary Rounds and Structured Communication Reduce Re-Admissions and Improve Some Patient Outcomes. West J Nurs Res. 2014;36(7):917-928. PubMed
2. Vazirani S, Hays RD, Shapiro MF, Cowan M. Effect of a multidisciplinary intervention on communication and collaboration among physicians and nurses. Am J Crit Care. 2005;14(1):71-77. PubMed
3. Wertheimer B, Jacobs RE, Bailey M, et al. Discharge before noon: an achievable hospital goal. J Hosp Med. 2014;9(4):210-214. PubMed
4. Wertheimer B, Jacobs RE, Iturrate E, Bailey M, Hochman K. Discharge before noon: Effect on throughput and sustainability. J Hosp Med. 2015;10(10):664-669. PubMed
5. Halm MA, Gagner S, Goering M, Sabo J, Smith M, Zaccagnini M. Interdisciplinary rounds: impact on patients, families, and staff. Clin Nurse Spec. 2003;17(3):133-142. PubMed
6. O’Mahony S, Mazur E, Charney P, Wang Y, Fine J. Use of multidisciplinary rounds to simultaneously improve quality outcomes, enhance resident education, and shorten length of stay. J Gen Intern Med. 2007;22(8):1073-1079. PubMed
7. Reimer N, Herbener L. Round and round we go: rounding strategies to impact exemplary professional practice. Clin J Oncol Nurs. 2014;18(6):654-660. PubMed
8. Curley C, McEachern JE, Speroff T. A firm trial of interdisciplinary rounds on the inpatient medical wards: an intervention designed using continuous quality improvement. Med Care. 1998;36(8 Suppl):AS4-AS12. PubMed
9. Baggs JG, Ryan SA, Phelps CE, Richeson JF, Johnson JE. The association between interdisciplinary collaboration and patient outcomes in a medical intensive care unit. Heart Lung. 1992;21(1):18-24. PubMed
10. Lawal AK, Rotter T, Kinsman L, et al. Lean management in health care: definition, concepts, methodology and effects reported (systematic review protocol). Syst Rev. 2014;3:103. PubMed
11. Liker JK. Toyota Way: 14 Management Principles from the World’s Greatest Manufacturer. New York, Chicago, San Francisco, Athens, London, Madrid, Mexico City, Milan, New Delhi, Singapore, Sydney, Toronto: McGraw-Hill Education; 2004.
12. Kane M, Chui K, Rimicci J, et al. Lean Manufacturing Improves Emergency Department Throughput and Patient Satisfaction. J Nurs Adm. 2015;45(9):429-434. PubMed
13. R Core Team. R: A language and environment for statistical computing. R Foundation for Statistical Computing, Vienna, Austria. 2016. http://www.R-project.org/. Accessed November 7, 2017.
14. Bates D, Mächler M, Bolker B, Walker S. Fitting Linear Mixed-Effects Models Using lme4. J Stat Softw. 2015;67(1):1-48.
15. O’Leary KJ, Buck R, Fligiel HM, et al. Structured interdisciplinary rounds in a medical teaching unit: improving patient safety. Arch Intern Med. 2011;171(7):678-684. PubMed
© 2018 Society of Hospital Medicine
Telemetry Use for LOS and Cost Reduction
Inpatient hospital services are a major component of total US civilian noninstitutionalized healthcare expenses, accounting for 29.3% of spending in 2009[1] when the average cost per stay was $9700.[2] Telemetry monitoring, a widely used resource for the identification of life‐threatening arrhythmias, contributes to these costs. In 1998, Sivaram et al. estimated the cost per patient at $683; in 2010, Ivonye et al. published the cost difference between a telemetry bed and a nonmonitored bed in their inner‐city public teaching facility reached $800.[3, 4]
In 1991, the American College of Cardiology published guidelines for telemetry use, which were later revised by the American Heart Association in 2004.[5, 6] Notably, the guidelines are based on expert opinion and on research data in electrocardiography.[7] The guidelines divide patients into 3 classes based on clinical condition: recommending telemetry monitoring for almost all class I patients, stating possible benefit in class II patients, and discouraging cardiac monitoring for the low‐risk class III patients.[5, 6] The Choosing Wisely campaign, an initiative of the American Board of Internal Medicine and the Society of Hospital Medicine, highlights telemetry monitoring as 1 of the top 5 interventions that physicians and patients should question when determining tests and procedures.[8] Choosing Wisely suggests using a protocol to govern continuation of telemetry outside of the intensive care unit (ICU), as inappropriate monitoring increases care costs and may result in patient harm.[8] The Joint Commission 2014 National Patient Safety Goals notes that numerous alarm signals and the resulting noise and displayed information tends to desensitize staff and cause them to miss or ignore alarm signals or even disable them.[9]
Few studies have examined implementation methods for improved telemetry bed utilization. One study evaluated the impact of a multispecialty telemetry policy with enforcement by an outside cardiologist and nurse team, noting improved cardiac monitoring bed utilization and decreased academic hospital closure, which previously resulted in inability to accept new patients or procedure cancellation.[10] Another study provided an orientation handout discussed by the chief resident and telemetry indication reviews during multidisciplinary rounds 3 times a week.[11]
Our study is one the first to demonstrate a model for a hospitalist‐led approach to guide appropriate telemetry use. We investigated the impact of a multipronged approach to guide telemetry usage: (1) a hospitalist‐led, daily review of bed utilization during attending rounds, (2) a hospitalist attending‐driven, trainee‐focused education module on telemetry utilization, (3) quarterly feedback on telemetry bed utilization rates, and (4) financial incentives. We analyzed pre‐ and post‐evaluation results from the education module to measure impact on knowledge, skills, and attitudes. Additionally, we evaluated the effect of the intervention on length of stay (LOS) and bed utilization costs, while monitoring case mix index (CMI) and overall mortality.
METHODS
Setting
This study took place at Stanford Hospital and Clinics, a teaching academic center in Stanford, California. Stanford Hospital is a 444‐bed, urban medical center with 114 telemetry intermediate ICU beds, and 66 ICU beds. The 264 medicalsurgical beds lack telemetry monitoring, which can only be completed in the intermediate and full ICU. All patients on telemetry units receive both cardiac monitoring and increased nursing ratios. Transfer orders are placed in the electronic medical record to shift patients between care levels. Bed control attempts to transfer patients as soon as an open bed in the appropriate care level exists.
The study included all 5 housestaff inpatient general internal medicine wards teams (which excludes cardiology, pulmonary hypertension, hematology, oncology, and post‐transplant patients). Hospitalists and nonhospitalists attend on the wards for 1‐ to 2‐week blocks. Teaching teams are staffed by 1 to 2 medical students, 2 interns, 1 resident, and 1 attending. The university institutional review board notice of determination waived review for this study because it was classified as quality improvement.
Participants
Ten full‐ and part‐time hospitalist physicians participated in the standardized telemetry teaching. Fifty‐six of the approximately 80 medical students and housestaff on hospitalists' teams completed the educational evaluation. Both hospitalist and nonhospitalist teams participated in daily multidisciplinary rounds, focusing on barriers to discharge including telemetry use. Twelve nonhospitalists served on the wards during the intervention period. Hospitalists covered 72% of the internal medicine wards during the intervention period.
Study Design
We investigated the impact of a multipronged approach to guide telemetry usage from January 2013 to August 2013 (intervention period).
Hospitalist‐Led Daily Review of Bed Utilization
Hospitalists were encouraged to discuss the need of telemetry on daily attending rounds and review indications for telemetry while on service. Prior to starting a ward block, attendings were emailed the teaching module with a reminder to discuss the need for telemetry on attending rounds. Reminders to discuss telemetry utilization were also provided during every‐other‐week hospitalist meetings. Compliance of daily discussion was not tracked.
Hospitalist‐Driven, Trainee‐Focused, Education Module on Telemetry Utilization
The educational module was taught during teaching sessions only by the hospitalists. Trainees on nonhospitalist teams did not receive dedicated teaching about telemetry usage. The module was given to learners only once. The module was a 10‐slide, Microsoft PowerPoint (Microsoft Corp., Redmond, WA) presentation that reviewed the history of telemetry, the American College of Cardiology and the American Heart Association guidelines, the cost difference between telemetry and nonmonitored beds, and the perceived barriers to discontinuation. The presentation was accompanied by a pre‐ and post‐evaluation to elicit knowledge, skills, and attitudes of telemetry use (see Supporting Information, Appendix A, in the online version of this article). The pre‐ and post‐evaluations were created through consensus with a multidisciplinary, expert panel after reviewing the evidence‐based literature.
Quarterly Feedback on Telemetry Bed Utilization Rates
Hospital beduse and CMI data were obtained from the Stanford finance department for the intervention period and for the baseline period, which was the year prior to the study, January 1, 2012 to December 31, 2012. Hospital beduse data included the number of days patients were on telemetry units versus medicalsurgical units (nontelemetry units), differentiated by hospitalists and nonhospitalists. Cost savings were calculated by the Stanford finance department that used Stanford‐specific, internal cost accounting data to determine the impact of the intervention. These data were reviewed at hospitalist meetings on a quarterly basis. We also obtained the University Healthsystem Consortium mortality index (observed to expected) for the general internal medicine service during the baseline and intervention periods.
To measure sustainment of telemetry reduction in the postintervention period, we measured telemetry LOS from September 2014 to March 2015 (extension period).
Financial Incentives
Hospitalists were provided a $2000 bonus at the end of fiscal year 2013 if the group showed a decrease in telemetry bed use in comparison to the baseline period.
Statistical Analysis of Clinical Outcome Measures
Continuous outcomes were tested using 2‐tailed t tests. Comparison of continuous outcome included differences in telemetry and nontelemetry LOS and CMI. Pairwise comparisons were made for various time periods. A P value of <0.05 was considered statistically significant. Statistical analyses were performed using Stata 12.0 software (StataCorp, College Station, TX).
RESULTS
Clinical and Value Outcomes
Baseline (January 2012December 2012) Versus Intervention Period (January 2013August 2013)
LOS for telemetry beds was significantly reduced over the intervention period (2.75 days vs 2.13 days, P=0.005) for hospitalists. Notably, there was no significant difference in mean LOS between baseline and intervention periods for nontelemetry beds (2.84 days vs 2.72 days, P=0.32) for hospitalists. In comparison, for nonhospitalists, there was no difference in mean LOS for telemetry beds between baseline and intervention periods (2.75 days vs 2.46 days, P=0.33) and nontelemetry beds (2.64 days vs 2.89 days, P=0.26) (Table 1).
Baseline Period | Intervention Period | P Value | Extension Period | P Value | |
---|---|---|---|---|---|
| |||||
Length of stay | |||||
Hospitalists | |||||
Telemetry beds | 2.75 | 2.13 | 0.005 | 1.93 | 0.09 |
Nontelemetry beds | 2.84 | 2.72 | 0.324 | 2.44 | 0.21 |
Nonhospitalists | |||||
Telemetry beds | 2.75 | 2.46 | 0.331 | 2.22 | 0.43 |
Nontelemetry beds | 2.64 | 2.89 | 0.261 | 2.26 | 0.05 |
Case mix index | |||||
Hospitalists | 1.44 | 1.45 | 0.68 | 1.40 | 0.21 |
Nonhospitalists | 1.46 | 1.40 | 0.53 | 1.53 | 0.18 |
Costs of hospital stay were also reduced in the multipronged, hospitalist‐driven intervention group. Expenditures for telemetry beds were reduced by 22.5% over the intervention period for hospitalists (Table 2).
Baseline to Intervention Period | Intervention to Extension Period | |
---|---|---|
| ||
Hospitalists | ||
Telemetry beds | 22.55% | 9.55% |
Nontelemetry beds | 4.23% | 10.14% |
Nonhospitalists | ||
Telemetry beds | 10.55% | 9.89% |
Nontelemetry beds | 9.47% | 21.84% |
The mean CMI of the patient cohort managed by the hospitalists in the baseline and intervention periods was not significantly different (1.44 vs 1.45, P=0.68). The mean CMI of the patients managed by the nonhospitalists in the baseline and intervention periods was also not significantly different (1.46 vs 1.40, P=0.53) (Table 1). Mortality index during the baseline and intervention periods was not significantly different (0.770.22 vs 0.660.23, P=0.54), as during the intervention and extension periods (0.660.23 vs 0.650.15, P=0.95).
Intervention Period (January 2013August 2013) Versus Extension Period (September 2014‐March 2015)
The decreased telemetry LOS for hospitalists was sustained from the intervention period to the extension period, from 2.13 to 1.93 (P=0.09). There was no significant change in the nontelemetry LOS in the intervention period compared to the extension period (2.72 vs 2.44, P=0.21). There was no change in the telemetry LOS for nonhospitalists from the intervention period to the extension period (2.46 vs 2.22, P=0.43).
The mean CMI in the hospitalist group was not significantly different in the intervention period compared to the extension period (1.45 to 1.40, P=0.21). The mean CMI in the nonhospitalist group did not change from the intervention period to the extension period (1.40 vs 1.53, P=0.18) (Table 1).
Education Outcomes
Out of the 56 participants completing the education module and survey, 28.6% were medical students, 53.6% were interns, 12.5% were second‐year residents, and 5.4% were third‐year residents. Several findings were seen at baseline via pretest. In evaluating patterns of current telemetry use, 32.2% of participants reported evaluating the necessity of telemetry for patients on admission only, 26.3% during transitions of care, 5.1% after discharge plans were cemented, 33.1% on a daily basis, and 3.4% rarely. When asked which member of the care team was most likely to encourage use of appropriate telemetry, 20.8% identified another resident, 13.9% nursing, 37.5% attending physician, 20.8% self, 4.2% the team as a whole, and 2.8% as not any.
Figure 1 shows premodule results regarding the trainees perceived percentage of patient encounters during which a participant's team discussed their patient's need for telemetry.
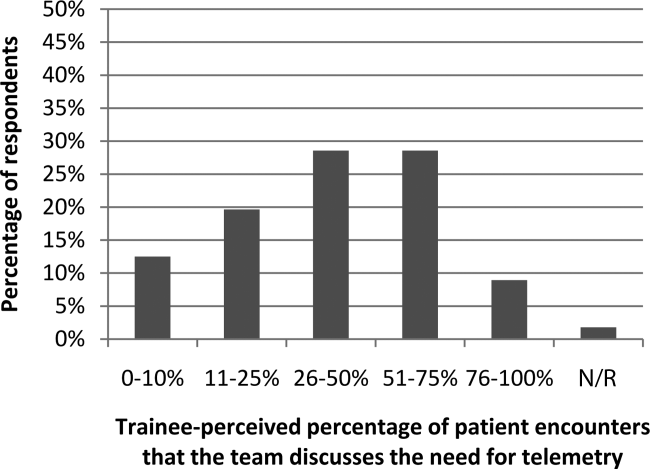
In assessing perception of current telemetry utilization, 1.8% of participants thought 0% to 10% of patients were currently on telemetry, 19.6% thought 11% to 20%, 42.9% thought 21% to 31%, 30.4% thought 31% to 40%, and 3.6% thought 41% to 50%.
Two areas were assessed at both baseline and after the intervention: knowledge of indications of telemetry use and cost related to telemetry use. We saw increased awareness of cost‐saving actions. To assess current knowledge of the indications of proper telemetry use according to American Heart Association guidelines, participants were presented with a list of 5 patients with different clinical indications for telemetry use and asked which patient required telemetry the most. Of the participants, 54.5% identified the correct answer in the pretest and 61.8% identified the correct answer in the post‐test. To assess knowledge of the costs of telemetry relative to other patient care, participants were presented with a patient case and asked to identify the most and least cost‐saving actions to safely care for the patient. When asked to identify the most cost‐saving action, 20.3% identified the correct answer in the pretest and 61.0% identified the correct answer in the post‐test. Of those who answered incorrectly in the pretest, 51.1% answered correctly in the post‐test (P=0.002). When asked to identify the least cost‐saving action, 23.7% identified the correct answer in the pretest and 50.9% identified the correct answer in the posttest. Of those who answered incorrectly in the pretest, 60.0% answered correctly in the post‐test (P=0.003).
In the post‐test, when asked about the importance of appropriate telemetry usage in providing cost‐conscious care and assuring appropriate hospital resource management, 76.8% of participants found the need very important, 21.4% somewhat important, and 1.8% as not applicable. The most commonly perceived barriers impeding discontinuation of telemetry, as reported by participants via post‐test, were nursing desires and time. Figure 2 shows all perceived barriers.
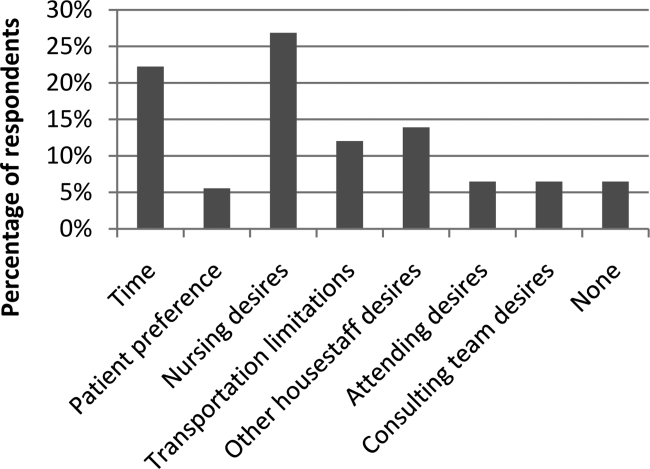
DISCUSSION
Our study is one of the first to our knowledge to demonstrate reductions in telemetry LOS by a hospitalist intervention for telemetry utilization. Others[10, 11] have studied the impact of an orientation handout by chief residents or a multispecialty telemetry policy with enforcement by an outside cardiologist and nurse team. Dressler et al. later sustained a 70% reduction in telemetry use without adversely affecting patient safety, as assessed through numbers of rapid response activations, codes, and deaths, through integrating the AHA guidelines into their electronic ordering system.[12] However, our study has the advantage of the primary team, who knows the patient and clinical scenario best, driving the change during attending rounds. In an era where cost consciousness intersects the practice of medicine, any intervention in patient care that demonstrates cost savings without an adverse impact on patient care and resource utilization must be emphasized. This is particularly important in academic institutions, where residents and medical students are learning to integrate the principles of patient safety and quality improvement into their clinical practice.[13] We actually showed sustained telemetry LOS reductions into the extension period after our intervention. We believe this may be due to telemetry triage being integrated into our attending and resident rounding practices. Future work should include integration of telemetry triage into clinical decision support in the electronic medical record and multidisciplinary rounds to disseminate telemetry triage hospital‐wide in both the academic and community settings.
Our study also revealed that nearly half of participants were not aware of the criteria for appropriate utilization of telemetry before our intervention; in the preintervention period, there were many anecdotal and objective findings of inappropriate utilization of telemetry as well as prolonged continuation beyond the clinical needs in both the hospitalist and nonhospitalist group. For the hospitalist group (ie, the group receiving guideline‐based education on appropriate indications for telemetry utilization), there was an assessment of both appropriate usage and timely discontinuation of telemetry in the postintervention period, which we attribute in large part to adherence to the education provided to this group.
We were able to show increased knowledge of cost‐saving actions among trainees with our educational module. We believe it is imperative to educate our providers (physicians, nurses, case managers, and students within these disciplines) on the appropriate indications for telemetry use, not only to help with cost savings and resource availability (ie, allowing telemetry beds to be available for patients who need them most), but also to instill consistent expectations among our patients. For the hospitalist group (ie, the group receiving guideline‐based education on appropriate indications for telemetry utilization), there was an assessment of both appropriate usage and timely discontinuation of telemetry in the postintervention period, which we attribute in large part to adherence to the education provided to this group.
Additionally, we feel it is important to consider the impacts of inappropriate use of telemetry from a patient's perspective: it is physically restrictive/emnconvenient, alarms are disruptive, it can be a barrier for other treatments such as physical therapy, it may increase the time it takes for imaging studies, a nurse may be required to accompany patients on telemetry, and poses additional costs to their medical bill.
We believe our success is due to several strategies. First, at the start of the fiscal year when quality improvement metrics are established, this particular metric (improving the appropriate utilization and timely discontinuation of telemetry) was deemed important by all hospitalists, engendering group buy‐in prior to the intervention. Our hospitalists received a detailed and interactive tutorial session in person at the beginning of the study. This tutorial provided the hospitalists with a comprehensive understanding of the appropriate (and inappropriate) indications for telemetry monitoring, hence facilitating guideline‐directed utilization. Email reminders and the tutorial tool were provided each time a hospitalist attended on the wards, and hospitalists received a small financial incentive to comply with appropriate telemetry utilization.
Our study has several strengths. First, the time frame of our study was long enough (8 months) to allow consistent trends to emerge and to optimize exposure of housestaff and medical students to this quality‐improvement initiative. Second, our cost savings came from 2 factors, direct reduction of inappropriate telemetry use and reduction in length of stay, highlighting the dual impact of appropriate telemetry utilization on cost. The overall reductions in telemetry utilization for the intervention group were a result of both reductions in initial placement on telemetry for patients who did not meet criteria for such monitoring as well as timely discontinuation of telemetry during the patient's hospitalization. Third, our study demonstrates that physicians can be effective in driving appropriate telemetry usage by participating in the clinical decision making regarding necessity and educating providers, trainees/students, and patients on appropriate indications. Finally, we show sustainment of our intervention in the extension period, suggesting telemetry triage integration into rounding practice.
Our study has limitations as well. First, our sample size is relatively small at a single academic center. Second, due to complexities in our faculty scheduling, we were unable to completely randomize patients to a hospitalist versus nonhospitalist team. However, we believe that despite the inability to randomize, our study does show the benefit of a hospitalist attending to reduce telemetry LOS given there was no change in nonhospitalist telemetry LOS despite all of the other hospital‐wide interventions (multidisciplinary rounds, similar housestaff). Third, our study was limited in that the CMI was used as a proxy for patient complexity, and the mortality index was used as the overall marker of safety. Further studies should monitor frequency and outcomes of arrhythmic events of patients transferred from telemetry monitoring to medicalsurgical beds. Finally, as the intervention was multipronged, we are unable to determine which component led to the reductions in telemetry utilization. Each component, however, remains easily transferrable to outside institutions. We demonstrated both a reduction in initiation of telemetry as well as timely discontinuation; however, due to the complexity in capturing this accurately, we were unable to numerically quantify these individual outcomes.
Additionally, there were approximately 10 nonhospitalist attendings who also staffed the wards during the intervention time period of our study; these attendings did not undergo the telemetry tutorial/orientation. This difference, along with the Hawthorne effect for the hospitalist attendings, also likely contributed to the difference in outcomes between the 2 attending cohorts in the intervention period.
CONCLUSIONS
Our results demonstrate that a multipronged hospitalist‐driven intervention to improve appropriate use of telemetry reduces telemetry LOS and cost. Hence, we believe that targeted, education‐driven interventions with monitoring of progress can have demonstrable impacts on changing practice. Physicians will need to make trade‐offs in clinical practice to balance efficient resource utilization with the patient's evolving condition in the inpatient setting, the complexities of clinical workflow, and the patient's expectations.[14] Appropriate telemetry utilization is a prime example of what needs to be done well in the future for high‐value care.
Acknowledgements
The authors acknowledge the hospitalists who participated in the intervention: Jeffrey Chi, Willliam Daines, Sumbul Desai, Poonam Hosamani, John Kugler, Charles Liao, Errol Ozdalga, and Sang Hoon Woo. The authors also acknowledge Joan Hendershott in the Finance Department and Joseph Hopkins in the Quality Department.
Disclosures: All coauthors have seen and agree with the contents of the article; submission (aside from abstracts) was not under review by any other publication. The authors report no disclosures of financial support from, or equity positions in, manufacturers of drugs or products mentioned in the article.
- 2009. Statistical brief 355. 2012. Agency for Healthcare Research and Quality, Rockville, MD. , National health care expenses in the U.S. civilian noninstitutionalized population,
- Costs for hospital stays in the United States, 2010. Statistical brief 146. 2013. Agency for Healthcare Research and Quality, Rockville, MD. , ,
- Telemetry outside critical care units: patterns of utilization and influence on management decisions. Clin Cardiol. 1998;21(7):503–505. , ,
- Evaluation of telemetry utilization, policy, and outcomes in an inner‐city academic medical center. J Natl Med Assoc. 2010;102(7):598–604. , , , et al.
- Recommended guidelines for in‐hospital cardiac monitoring of adults for detection of arrhythmia. Emergency Cardiac Care Committee members. J Am Coll Cardiol. 1991;18(6):1431–1433. , ,
- Practice standards for electrocardiographic monitoring in hospital settings: an American Heart Association scientific statement from the Councils on Cardiovascular Nursing, Clinical Cardiology, and Cardiovascular Disease in the Young: endorsed by the International Society of Computerized Electrocardiology and the American Association of Critical‐Care Nurses. Circulation. 2004;110(17):2721–2746. , , , et al.
- Is telemetry overused? Is it as helpful as thought? Cleve Clin J Med. 2009;76(6):368–372. , , , , ,
- Society of Hospital Medicine. Adult Hospital Medicine. Five things physicians and patients should question. Available at: http://www.choosingwisely.org/societies/society‐of‐hospital‐medicine‐adult. Published February 21, 2013. Accessed October 5, 2014.
- Joint Commission on Accreditation of Healthcare Organizations. The Joint Commission announces 2014 national patient safety goal. Jt Comm Perspect. 2013;33(7):1–4.
- Optimizing telemetry utilization in an academic medical center. J Clin Outcomes Manage. 2008;15(9):435–440. , , , ,
- Improving utilization of telemetry in a university hospital. J Clin Outcomes Manage. 2005;12(10):519–522. ,
- Altering overuse of cardiac telemetry in non‐intensive care unit settings by hardwiring the use of American Heart Association guidelines. JAMA Intern Med. 2014;174:1852–1854. , , , ,
- "Innovation" institutes in academic health centers: enhancing value through leadership, education, engagement, and scholarship. Acad Med. 2014;89(9):1204–1206. , ,
- Controlling health costs: physician responses to patient expectations for medical care. J Gen Intern Med. 2014;29(9):1234–1241. , , , , ,
Inpatient hospital services are a major component of total US civilian noninstitutionalized healthcare expenses, accounting for 29.3% of spending in 2009[1] when the average cost per stay was $9700.[2] Telemetry monitoring, a widely used resource for the identification of life‐threatening arrhythmias, contributes to these costs. In 1998, Sivaram et al. estimated the cost per patient at $683; in 2010, Ivonye et al. published the cost difference between a telemetry bed and a nonmonitored bed in their inner‐city public teaching facility reached $800.[3, 4]
In 1991, the American College of Cardiology published guidelines for telemetry use, which were later revised by the American Heart Association in 2004.[5, 6] Notably, the guidelines are based on expert opinion and on research data in electrocardiography.[7] The guidelines divide patients into 3 classes based on clinical condition: recommending telemetry monitoring for almost all class I patients, stating possible benefit in class II patients, and discouraging cardiac monitoring for the low‐risk class III patients.[5, 6] The Choosing Wisely campaign, an initiative of the American Board of Internal Medicine and the Society of Hospital Medicine, highlights telemetry monitoring as 1 of the top 5 interventions that physicians and patients should question when determining tests and procedures.[8] Choosing Wisely suggests using a protocol to govern continuation of telemetry outside of the intensive care unit (ICU), as inappropriate monitoring increases care costs and may result in patient harm.[8] The Joint Commission 2014 National Patient Safety Goals notes that numerous alarm signals and the resulting noise and displayed information tends to desensitize staff and cause them to miss or ignore alarm signals or even disable them.[9]
Few studies have examined implementation methods for improved telemetry bed utilization. One study evaluated the impact of a multispecialty telemetry policy with enforcement by an outside cardiologist and nurse team, noting improved cardiac monitoring bed utilization and decreased academic hospital closure, which previously resulted in inability to accept new patients or procedure cancellation.[10] Another study provided an orientation handout discussed by the chief resident and telemetry indication reviews during multidisciplinary rounds 3 times a week.[11]
Our study is one the first to demonstrate a model for a hospitalist‐led approach to guide appropriate telemetry use. We investigated the impact of a multipronged approach to guide telemetry usage: (1) a hospitalist‐led, daily review of bed utilization during attending rounds, (2) a hospitalist attending‐driven, trainee‐focused education module on telemetry utilization, (3) quarterly feedback on telemetry bed utilization rates, and (4) financial incentives. We analyzed pre‐ and post‐evaluation results from the education module to measure impact on knowledge, skills, and attitudes. Additionally, we evaluated the effect of the intervention on length of stay (LOS) and bed utilization costs, while monitoring case mix index (CMI) and overall mortality.
METHODS
Setting
This study took place at Stanford Hospital and Clinics, a teaching academic center in Stanford, California. Stanford Hospital is a 444‐bed, urban medical center with 114 telemetry intermediate ICU beds, and 66 ICU beds. The 264 medicalsurgical beds lack telemetry monitoring, which can only be completed in the intermediate and full ICU. All patients on telemetry units receive both cardiac monitoring and increased nursing ratios. Transfer orders are placed in the electronic medical record to shift patients between care levels. Bed control attempts to transfer patients as soon as an open bed in the appropriate care level exists.
The study included all 5 housestaff inpatient general internal medicine wards teams (which excludes cardiology, pulmonary hypertension, hematology, oncology, and post‐transplant patients). Hospitalists and nonhospitalists attend on the wards for 1‐ to 2‐week blocks. Teaching teams are staffed by 1 to 2 medical students, 2 interns, 1 resident, and 1 attending. The university institutional review board notice of determination waived review for this study because it was classified as quality improvement.
Participants
Ten full‐ and part‐time hospitalist physicians participated in the standardized telemetry teaching. Fifty‐six of the approximately 80 medical students and housestaff on hospitalists' teams completed the educational evaluation. Both hospitalist and nonhospitalist teams participated in daily multidisciplinary rounds, focusing on barriers to discharge including telemetry use. Twelve nonhospitalists served on the wards during the intervention period. Hospitalists covered 72% of the internal medicine wards during the intervention period.
Study Design
We investigated the impact of a multipronged approach to guide telemetry usage from January 2013 to August 2013 (intervention period).
Hospitalist‐Led Daily Review of Bed Utilization
Hospitalists were encouraged to discuss the need of telemetry on daily attending rounds and review indications for telemetry while on service. Prior to starting a ward block, attendings were emailed the teaching module with a reminder to discuss the need for telemetry on attending rounds. Reminders to discuss telemetry utilization were also provided during every‐other‐week hospitalist meetings. Compliance of daily discussion was not tracked.
Hospitalist‐Driven, Trainee‐Focused, Education Module on Telemetry Utilization
The educational module was taught during teaching sessions only by the hospitalists. Trainees on nonhospitalist teams did not receive dedicated teaching about telemetry usage. The module was given to learners only once. The module was a 10‐slide, Microsoft PowerPoint (Microsoft Corp., Redmond, WA) presentation that reviewed the history of telemetry, the American College of Cardiology and the American Heart Association guidelines, the cost difference between telemetry and nonmonitored beds, and the perceived barriers to discontinuation. The presentation was accompanied by a pre‐ and post‐evaluation to elicit knowledge, skills, and attitudes of telemetry use (see Supporting Information, Appendix A, in the online version of this article). The pre‐ and post‐evaluations were created through consensus with a multidisciplinary, expert panel after reviewing the evidence‐based literature.
Quarterly Feedback on Telemetry Bed Utilization Rates
Hospital beduse and CMI data were obtained from the Stanford finance department for the intervention period and for the baseline period, which was the year prior to the study, January 1, 2012 to December 31, 2012. Hospital beduse data included the number of days patients were on telemetry units versus medicalsurgical units (nontelemetry units), differentiated by hospitalists and nonhospitalists. Cost savings were calculated by the Stanford finance department that used Stanford‐specific, internal cost accounting data to determine the impact of the intervention. These data were reviewed at hospitalist meetings on a quarterly basis. We also obtained the University Healthsystem Consortium mortality index (observed to expected) for the general internal medicine service during the baseline and intervention periods.
To measure sustainment of telemetry reduction in the postintervention period, we measured telemetry LOS from September 2014 to March 2015 (extension period).
Financial Incentives
Hospitalists were provided a $2000 bonus at the end of fiscal year 2013 if the group showed a decrease in telemetry bed use in comparison to the baseline period.
Statistical Analysis of Clinical Outcome Measures
Continuous outcomes were tested using 2‐tailed t tests. Comparison of continuous outcome included differences in telemetry and nontelemetry LOS and CMI. Pairwise comparisons were made for various time periods. A P value of <0.05 was considered statistically significant. Statistical analyses were performed using Stata 12.0 software (StataCorp, College Station, TX).
RESULTS
Clinical and Value Outcomes
Baseline (January 2012December 2012) Versus Intervention Period (January 2013August 2013)
LOS for telemetry beds was significantly reduced over the intervention period (2.75 days vs 2.13 days, P=0.005) for hospitalists. Notably, there was no significant difference in mean LOS between baseline and intervention periods for nontelemetry beds (2.84 days vs 2.72 days, P=0.32) for hospitalists. In comparison, for nonhospitalists, there was no difference in mean LOS for telemetry beds between baseline and intervention periods (2.75 days vs 2.46 days, P=0.33) and nontelemetry beds (2.64 days vs 2.89 days, P=0.26) (Table 1).
Baseline Period | Intervention Period | P Value | Extension Period | P Value | |
---|---|---|---|---|---|
| |||||
Length of stay | |||||
Hospitalists | |||||
Telemetry beds | 2.75 | 2.13 | 0.005 | 1.93 | 0.09 |
Nontelemetry beds | 2.84 | 2.72 | 0.324 | 2.44 | 0.21 |
Nonhospitalists | |||||
Telemetry beds | 2.75 | 2.46 | 0.331 | 2.22 | 0.43 |
Nontelemetry beds | 2.64 | 2.89 | 0.261 | 2.26 | 0.05 |
Case mix index | |||||
Hospitalists | 1.44 | 1.45 | 0.68 | 1.40 | 0.21 |
Nonhospitalists | 1.46 | 1.40 | 0.53 | 1.53 | 0.18 |
Costs of hospital stay were also reduced in the multipronged, hospitalist‐driven intervention group. Expenditures for telemetry beds were reduced by 22.5% over the intervention period for hospitalists (Table 2).
Baseline to Intervention Period | Intervention to Extension Period | |
---|---|---|
| ||
Hospitalists | ||
Telemetry beds | 22.55% | 9.55% |
Nontelemetry beds | 4.23% | 10.14% |
Nonhospitalists | ||
Telemetry beds | 10.55% | 9.89% |
Nontelemetry beds | 9.47% | 21.84% |
The mean CMI of the patient cohort managed by the hospitalists in the baseline and intervention periods was not significantly different (1.44 vs 1.45, P=0.68). The mean CMI of the patients managed by the nonhospitalists in the baseline and intervention periods was also not significantly different (1.46 vs 1.40, P=0.53) (Table 1). Mortality index during the baseline and intervention periods was not significantly different (0.770.22 vs 0.660.23, P=0.54), as during the intervention and extension periods (0.660.23 vs 0.650.15, P=0.95).
Intervention Period (January 2013August 2013) Versus Extension Period (September 2014‐March 2015)
The decreased telemetry LOS for hospitalists was sustained from the intervention period to the extension period, from 2.13 to 1.93 (P=0.09). There was no significant change in the nontelemetry LOS in the intervention period compared to the extension period (2.72 vs 2.44, P=0.21). There was no change in the telemetry LOS for nonhospitalists from the intervention period to the extension period (2.46 vs 2.22, P=0.43).
The mean CMI in the hospitalist group was not significantly different in the intervention period compared to the extension period (1.45 to 1.40, P=0.21). The mean CMI in the nonhospitalist group did not change from the intervention period to the extension period (1.40 vs 1.53, P=0.18) (Table 1).
Education Outcomes
Out of the 56 participants completing the education module and survey, 28.6% were medical students, 53.6% were interns, 12.5% were second‐year residents, and 5.4% were third‐year residents. Several findings were seen at baseline via pretest. In evaluating patterns of current telemetry use, 32.2% of participants reported evaluating the necessity of telemetry for patients on admission only, 26.3% during transitions of care, 5.1% after discharge plans were cemented, 33.1% on a daily basis, and 3.4% rarely. When asked which member of the care team was most likely to encourage use of appropriate telemetry, 20.8% identified another resident, 13.9% nursing, 37.5% attending physician, 20.8% self, 4.2% the team as a whole, and 2.8% as not any.
Figure 1 shows premodule results regarding the trainees perceived percentage of patient encounters during which a participant's team discussed their patient's need for telemetry.
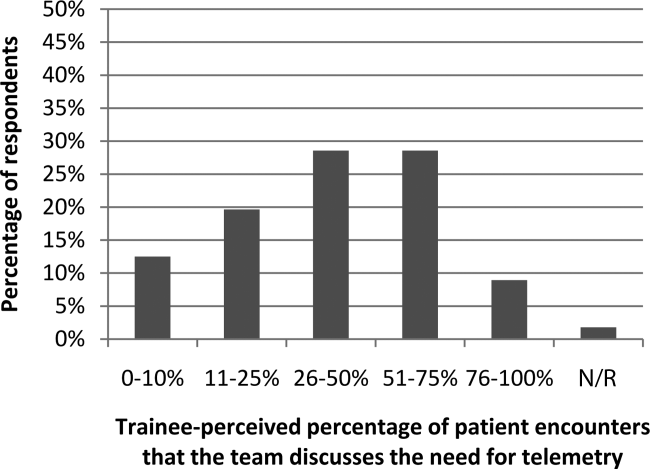
In assessing perception of current telemetry utilization, 1.8% of participants thought 0% to 10% of patients were currently on telemetry, 19.6% thought 11% to 20%, 42.9% thought 21% to 31%, 30.4% thought 31% to 40%, and 3.6% thought 41% to 50%.
Two areas were assessed at both baseline and after the intervention: knowledge of indications of telemetry use and cost related to telemetry use. We saw increased awareness of cost‐saving actions. To assess current knowledge of the indications of proper telemetry use according to American Heart Association guidelines, participants were presented with a list of 5 patients with different clinical indications for telemetry use and asked which patient required telemetry the most. Of the participants, 54.5% identified the correct answer in the pretest and 61.8% identified the correct answer in the post‐test. To assess knowledge of the costs of telemetry relative to other patient care, participants were presented with a patient case and asked to identify the most and least cost‐saving actions to safely care for the patient. When asked to identify the most cost‐saving action, 20.3% identified the correct answer in the pretest and 61.0% identified the correct answer in the post‐test. Of those who answered incorrectly in the pretest, 51.1% answered correctly in the post‐test (P=0.002). When asked to identify the least cost‐saving action, 23.7% identified the correct answer in the pretest and 50.9% identified the correct answer in the posttest. Of those who answered incorrectly in the pretest, 60.0% answered correctly in the post‐test (P=0.003).
In the post‐test, when asked about the importance of appropriate telemetry usage in providing cost‐conscious care and assuring appropriate hospital resource management, 76.8% of participants found the need very important, 21.4% somewhat important, and 1.8% as not applicable. The most commonly perceived barriers impeding discontinuation of telemetry, as reported by participants via post‐test, were nursing desires and time. Figure 2 shows all perceived barriers.
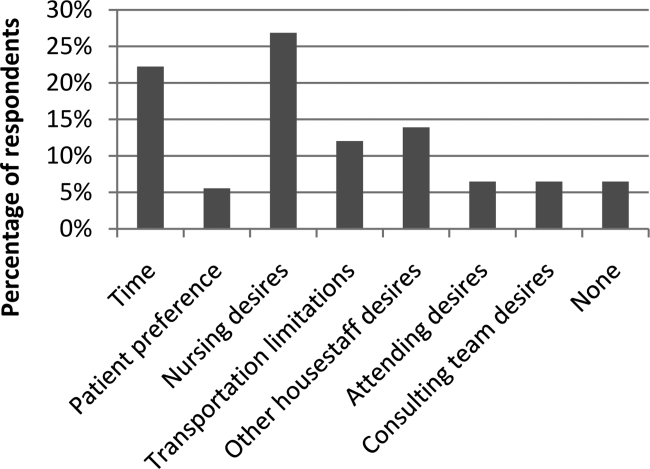
DISCUSSION
Our study is one of the first to our knowledge to demonstrate reductions in telemetry LOS by a hospitalist intervention for telemetry utilization. Others[10, 11] have studied the impact of an orientation handout by chief residents or a multispecialty telemetry policy with enforcement by an outside cardiologist and nurse team. Dressler et al. later sustained a 70% reduction in telemetry use without adversely affecting patient safety, as assessed through numbers of rapid response activations, codes, and deaths, through integrating the AHA guidelines into their electronic ordering system.[12] However, our study has the advantage of the primary team, who knows the patient and clinical scenario best, driving the change during attending rounds. In an era where cost consciousness intersects the practice of medicine, any intervention in patient care that demonstrates cost savings without an adverse impact on patient care and resource utilization must be emphasized. This is particularly important in academic institutions, where residents and medical students are learning to integrate the principles of patient safety and quality improvement into their clinical practice.[13] We actually showed sustained telemetry LOS reductions into the extension period after our intervention. We believe this may be due to telemetry triage being integrated into our attending and resident rounding practices. Future work should include integration of telemetry triage into clinical decision support in the electronic medical record and multidisciplinary rounds to disseminate telemetry triage hospital‐wide in both the academic and community settings.
Our study also revealed that nearly half of participants were not aware of the criteria for appropriate utilization of telemetry before our intervention; in the preintervention period, there were many anecdotal and objective findings of inappropriate utilization of telemetry as well as prolonged continuation beyond the clinical needs in both the hospitalist and nonhospitalist group. For the hospitalist group (ie, the group receiving guideline‐based education on appropriate indications for telemetry utilization), there was an assessment of both appropriate usage and timely discontinuation of telemetry in the postintervention period, which we attribute in large part to adherence to the education provided to this group.
We were able to show increased knowledge of cost‐saving actions among trainees with our educational module. We believe it is imperative to educate our providers (physicians, nurses, case managers, and students within these disciplines) on the appropriate indications for telemetry use, not only to help with cost savings and resource availability (ie, allowing telemetry beds to be available for patients who need them most), but also to instill consistent expectations among our patients. For the hospitalist group (ie, the group receiving guideline‐based education on appropriate indications for telemetry utilization), there was an assessment of both appropriate usage and timely discontinuation of telemetry in the postintervention period, which we attribute in large part to adherence to the education provided to this group.
Additionally, we feel it is important to consider the impacts of inappropriate use of telemetry from a patient's perspective: it is physically restrictive/emnconvenient, alarms are disruptive, it can be a barrier for other treatments such as physical therapy, it may increase the time it takes for imaging studies, a nurse may be required to accompany patients on telemetry, and poses additional costs to their medical bill.
We believe our success is due to several strategies. First, at the start of the fiscal year when quality improvement metrics are established, this particular metric (improving the appropriate utilization and timely discontinuation of telemetry) was deemed important by all hospitalists, engendering group buy‐in prior to the intervention. Our hospitalists received a detailed and interactive tutorial session in person at the beginning of the study. This tutorial provided the hospitalists with a comprehensive understanding of the appropriate (and inappropriate) indications for telemetry monitoring, hence facilitating guideline‐directed utilization. Email reminders and the tutorial tool were provided each time a hospitalist attended on the wards, and hospitalists received a small financial incentive to comply with appropriate telemetry utilization.
Our study has several strengths. First, the time frame of our study was long enough (8 months) to allow consistent trends to emerge and to optimize exposure of housestaff and medical students to this quality‐improvement initiative. Second, our cost savings came from 2 factors, direct reduction of inappropriate telemetry use and reduction in length of stay, highlighting the dual impact of appropriate telemetry utilization on cost. The overall reductions in telemetry utilization for the intervention group were a result of both reductions in initial placement on telemetry for patients who did not meet criteria for such monitoring as well as timely discontinuation of telemetry during the patient's hospitalization. Third, our study demonstrates that physicians can be effective in driving appropriate telemetry usage by participating in the clinical decision making regarding necessity and educating providers, trainees/students, and patients on appropriate indications. Finally, we show sustainment of our intervention in the extension period, suggesting telemetry triage integration into rounding practice.
Our study has limitations as well. First, our sample size is relatively small at a single academic center. Second, due to complexities in our faculty scheduling, we were unable to completely randomize patients to a hospitalist versus nonhospitalist team. However, we believe that despite the inability to randomize, our study does show the benefit of a hospitalist attending to reduce telemetry LOS given there was no change in nonhospitalist telemetry LOS despite all of the other hospital‐wide interventions (multidisciplinary rounds, similar housestaff). Third, our study was limited in that the CMI was used as a proxy for patient complexity, and the mortality index was used as the overall marker of safety. Further studies should monitor frequency and outcomes of arrhythmic events of patients transferred from telemetry monitoring to medicalsurgical beds. Finally, as the intervention was multipronged, we are unable to determine which component led to the reductions in telemetry utilization. Each component, however, remains easily transferrable to outside institutions. We demonstrated both a reduction in initiation of telemetry as well as timely discontinuation; however, due to the complexity in capturing this accurately, we were unable to numerically quantify these individual outcomes.
Additionally, there were approximately 10 nonhospitalist attendings who also staffed the wards during the intervention time period of our study; these attendings did not undergo the telemetry tutorial/orientation. This difference, along with the Hawthorne effect for the hospitalist attendings, also likely contributed to the difference in outcomes between the 2 attending cohorts in the intervention period.
CONCLUSIONS
Our results demonstrate that a multipronged hospitalist‐driven intervention to improve appropriate use of telemetry reduces telemetry LOS and cost. Hence, we believe that targeted, education‐driven interventions with monitoring of progress can have demonstrable impacts on changing practice. Physicians will need to make trade‐offs in clinical practice to balance efficient resource utilization with the patient's evolving condition in the inpatient setting, the complexities of clinical workflow, and the patient's expectations.[14] Appropriate telemetry utilization is a prime example of what needs to be done well in the future for high‐value care.
Acknowledgements
The authors acknowledge the hospitalists who participated in the intervention: Jeffrey Chi, Willliam Daines, Sumbul Desai, Poonam Hosamani, John Kugler, Charles Liao, Errol Ozdalga, and Sang Hoon Woo. The authors also acknowledge Joan Hendershott in the Finance Department and Joseph Hopkins in the Quality Department.
Disclosures: All coauthors have seen and agree with the contents of the article; submission (aside from abstracts) was not under review by any other publication. The authors report no disclosures of financial support from, or equity positions in, manufacturers of drugs or products mentioned in the article.
Inpatient hospital services are a major component of total US civilian noninstitutionalized healthcare expenses, accounting for 29.3% of spending in 2009[1] when the average cost per stay was $9700.[2] Telemetry monitoring, a widely used resource for the identification of life‐threatening arrhythmias, contributes to these costs. In 1998, Sivaram et al. estimated the cost per patient at $683; in 2010, Ivonye et al. published the cost difference between a telemetry bed and a nonmonitored bed in their inner‐city public teaching facility reached $800.[3, 4]
In 1991, the American College of Cardiology published guidelines for telemetry use, which were later revised by the American Heart Association in 2004.[5, 6] Notably, the guidelines are based on expert opinion and on research data in electrocardiography.[7] The guidelines divide patients into 3 classes based on clinical condition: recommending telemetry monitoring for almost all class I patients, stating possible benefit in class II patients, and discouraging cardiac monitoring for the low‐risk class III patients.[5, 6] The Choosing Wisely campaign, an initiative of the American Board of Internal Medicine and the Society of Hospital Medicine, highlights telemetry monitoring as 1 of the top 5 interventions that physicians and patients should question when determining tests and procedures.[8] Choosing Wisely suggests using a protocol to govern continuation of telemetry outside of the intensive care unit (ICU), as inappropriate monitoring increases care costs and may result in patient harm.[8] The Joint Commission 2014 National Patient Safety Goals notes that numerous alarm signals and the resulting noise and displayed information tends to desensitize staff and cause them to miss or ignore alarm signals or even disable them.[9]
Few studies have examined implementation methods for improved telemetry bed utilization. One study evaluated the impact of a multispecialty telemetry policy with enforcement by an outside cardiologist and nurse team, noting improved cardiac monitoring bed utilization and decreased academic hospital closure, which previously resulted in inability to accept new patients or procedure cancellation.[10] Another study provided an orientation handout discussed by the chief resident and telemetry indication reviews during multidisciplinary rounds 3 times a week.[11]
Our study is one the first to demonstrate a model for a hospitalist‐led approach to guide appropriate telemetry use. We investigated the impact of a multipronged approach to guide telemetry usage: (1) a hospitalist‐led, daily review of bed utilization during attending rounds, (2) a hospitalist attending‐driven, trainee‐focused education module on telemetry utilization, (3) quarterly feedback on telemetry bed utilization rates, and (4) financial incentives. We analyzed pre‐ and post‐evaluation results from the education module to measure impact on knowledge, skills, and attitudes. Additionally, we evaluated the effect of the intervention on length of stay (LOS) and bed utilization costs, while monitoring case mix index (CMI) and overall mortality.
METHODS
Setting
This study took place at Stanford Hospital and Clinics, a teaching academic center in Stanford, California. Stanford Hospital is a 444‐bed, urban medical center with 114 telemetry intermediate ICU beds, and 66 ICU beds. The 264 medicalsurgical beds lack telemetry monitoring, which can only be completed in the intermediate and full ICU. All patients on telemetry units receive both cardiac monitoring and increased nursing ratios. Transfer orders are placed in the electronic medical record to shift patients between care levels. Bed control attempts to transfer patients as soon as an open bed in the appropriate care level exists.
The study included all 5 housestaff inpatient general internal medicine wards teams (which excludes cardiology, pulmonary hypertension, hematology, oncology, and post‐transplant patients). Hospitalists and nonhospitalists attend on the wards for 1‐ to 2‐week blocks. Teaching teams are staffed by 1 to 2 medical students, 2 interns, 1 resident, and 1 attending. The university institutional review board notice of determination waived review for this study because it was classified as quality improvement.
Participants
Ten full‐ and part‐time hospitalist physicians participated in the standardized telemetry teaching. Fifty‐six of the approximately 80 medical students and housestaff on hospitalists' teams completed the educational evaluation. Both hospitalist and nonhospitalist teams participated in daily multidisciplinary rounds, focusing on barriers to discharge including telemetry use. Twelve nonhospitalists served on the wards during the intervention period. Hospitalists covered 72% of the internal medicine wards during the intervention period.
Study Design
We investigated the impact of a multipronged approach to guide telemetry usage from January 2013 to August 2013 (intervention period).
Hospitalist‐Led Daily Review of Bed Utilization
Hospitalists were encouraged to discuss the need of telemetry on daily attending rounds and review indications for telemetry while on service. Prior to starting a ward block, attendings were emailed the teaching module with a reminder to discuss the need for telemetry on attending rounds. Reminders to discuss telemetry utilization were also provided during every‐other‐week hospitalist meetings. Compliance of daily discussion was not tracked.
Hospitalist‐Driven, Trainee‐Focused, Education Module on Telemetry Utilization
The educational module was taught during teaching sessions only by the hospitalists. Trainees on nonhospitalist teams did not receive dedicated teaching about telemetry usage. The module was given to learners only once. The module was a 10‐slide, Microsoft PowerPoint (Microsoft Corp., Redmond, WA) presentation that reviewed the history of telemetry, the American College of Cardiology and the American Heart Association guidelines, the cost difference between telemetry and nonmonitored beds, and the perceived barriers to discontinuation. The presentation was accompanied by a pre‐ and post‐evaluation to elicit knowledge, skills, and attitudes of telemetry use (see Supporting Information, Appendix A, in the online version of this article). The pre‐ and post‐evaluations were created through consensus with a multidisciplinary, expert panel after reviewing the evidence‐based literature.
Quarterly Feedback on Telemetry Bed Utilization Rates
Hospital beduse and CMI data were obtained from the Stanford finance department for the intervention period and for the baseline period, which was the year prior to the study, January 1, 2012 to December 31, 2012. Hospital beduse data included the number of days patients were on telemetry units versus medicalsurgical units (nontelemetry units), differentiated by hospitalists and nonhospitalists. Cost savings were calculated by the Stanford finance department that used Stanford‐specific, internal cost accounting data to determine the impact of the intervention. These data were reviewed at hospitalist meetings on a quarterly basis. We also obtained the University Healthsystem Consortium mortality index (observed to expected) for the general internal medicine service during the baseline and intervention periods.
To measure sustainment of telemetry reduction in the postintervention period, we measured telemetry LOS from September 2014 to March 2015 (extension period).
Financial Incentives
Hospitalists were provided a $2000 bonus at the end of fiscal year 2013 if the group showed a decrease in telemetry bed use in comparison to the baseline period.
Statistical Analysis of Clinical Outcome Measures
Continuous outcomes were tested using 2‐tailed t tests. Comparison of continuous outcome included differences in telemetry and nontelemetry LOS and CMI. Pairwise comparisons were made for various time periods. A P value of <0.05 was considered statistically significant. Statistical analyses were performed using Stata 12.0 software (StataCorp, College Station, TX).
RESULTS
Clinical and Value Outcomes
Baseline (January 2012December 2012) Versus Intervention Period (January 2013August 2013)
LOS for telemetry beds was significantly reduced over the intervention period (2.75 days vs 2.13 days, P=0.005) for hospitalists. Notably, there was no significant difference in mean LOS between baseline and intervention periods for nontelemetry beds (2.84 days vs 2.72 days, P=0.32) for hospitalists. In comparison, for nonhospitalists, there was no difference in mean LOS for telemetry beds between baseline and intervention periods (2.75 days vs 2.46 days, P=0.33) and nontelemetry beds (2.64 days vs 2.89 days, P=0.26) (Table 1).
Baseline Period | Intervention Period | P Value | Extension Period | P Value | |
---|---|---|---|---|---|
| |||||
Length of stay | |||||
Hospitalists | |||||
Telemetry beds | 2.75 | 2.13 | 0.005 | 1.93 | 0.09 |
Nontelemetry beds | 2.84 | 2.72 | 0.324 | 2.44 | 0.21 |
Nonhospitalists | |||||
Telemetry beds | 2.75 | 2.46 | 0.331 | 2.22 | 0.43 |
Nontelemetry beds | 2.64 | 2.89 | 0.261 | 2.26 | 0.05 |
Case mix index | |||||
Hospitalists | 1.44 | 1.45 | 0.68 | 1.40 | 0.21 |
Nonhospitalists | 1.46 | 1.40 | 0.53 | 1.53 | 0.18 |
Costs of hospital stay were also reduced in the multipronged, hospitalist‐driven intervention group. Expenditures for telemetry beds were reduced by 22.5% over the intervention period for hospitalists (Table 2).
Baseline to Intervention Period | Intervention to Extension Period | |
---|---|---|
| ||
Hospitalists | ||
Telemetry beds | 22.55% | 9.55% |
Nontelemetry beds | 4.23% | 10.14% |
Nonhospitalists | ||
Telemetry beds | 10.55% | 9.89% |
Nontelemetry beds | 9.47% | 21.84% |
The mean CMI of the patient cohort managed by the hospitalists in the baseline and intervention periods was not significantly different (1.44 vs 1.45, P=0.68). The mean CMI of the patients managed by the nonhospitalists in the baseline and intervention periods was also not significantly different (1.46 vs 1.40, P=0.53) (Table 1). Mortality index during the baseline and intervention periods was not significantly different (0.770.22 vs 0.660.23, P=0.54), as during the intervention and extension periods (0.660.23 vs 0.650.15, P=0.95).
Intervention Period (January 2013August 2013) Versus Extension Period (September 2014‐March 2015)
The decreased telemetry LOS for hospitalists was sustained from the intervention period to the extension period, from 2.13 to 1.93 (P=0.09). There was no significant change in the nontelemetry LOS in the intervention period compared to the extension period (2.72 vs 2.44, P=0.21). There was no change in the telemetry LOS for nonhospitalists from the intervention period to the extension period (2.46 vs 2.22, P=0.43).
The mean CMI in the hospitalist group was not significantly different in the intervention period compared to the extension period (1.45 to 1.40, P=0.21). The mean CMI in the nonhospitalist group did not change from the intervention period to the extension period (1.40 vs 1.53, P=0.18) (Table 1).
Education Outcomes
Out of the 56 participants completing the education module and survey, 28.6% were medical students, 53.6% were interns, 12.5% were second‐year residents, and 5.4% were third‐year residents. Several findings were seen at baseline via pretest. In evaluating patterns of current telemetry use, 32.2% of participants reported evaluating the necessity of telemetry for patients on admission only, 26.3% during transitions of care, 5.1% after discharge plans were cemented, 33.1% on a daily basis, and 3.4% rarely. When asked which member of the care team was most likely to encourage use of appropriate telemetry, 20.8% identified another resident, 13.9% nursing, 37.5% attending physician, 20.8% self, 4.2% the team as a whole, and 2.8% as not any.
Figure 1 shows premodule results regarding the trainees perceived percentage of patient encounters during which a participant's team discussed their patient's need for telemetry.
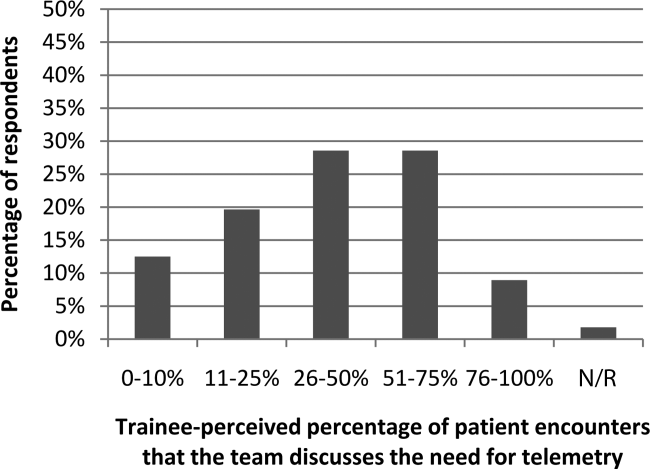
In assessing perception of current telemetry utilization, 1.8% of participants thought 0% to 10% of patients were currently on telemetry, 19.6% thought 11% to 20%, 42.9% thought 21% to 31%, 30.4% thought 31% to 40%, and 3.6% thought 41% to 50%.
Two areas were assessed at both baseline and after the intervention: knowledge of indications of telemetry use and cost related to telemetry use. We saw increased awareness of cost‐saving actions. To assess current knowledge of the indications of proper telemetry use according to American Heart Association guidelines, participants were presented with a list of 5 patients with different clinical indications for telemetry use and asked which patient required telemetry the most. Of the participants, 54.5% identified the correct answer in the pretest and 61.8% identified the correct answer in the post‐test. To assess knowledge of the costs of telemetry relative to other patient care, participants were presented with a patient case and asked to identify the most and least cost‐saving actions to safely care for the patient. When asked to identify the most cost‐saving action, 20.3% identified the correct answer in the pretest and 61.0% identified the correct answer in the post‐test. Of those who answered incorrectly in the pretest, 51.1% answered correctly in the post‐test (P=0.002). When asked to identify the least cost‐saving action, 23.7% identified the correct answer in the pretest and 50.9% identified the correct answer in the posttest. Of those who answered incorrectly in the pretest, 60.0% answered correctly in the post‐test (P=0.003).
In the post‐test, when asked about the importance of appropriate telemetry usage in providing cost‐conscious care and assuring appropriate hospital resource management, 76.8% of participants found the need very important, 21.4% somewhat important, and 1.8% as not applicable. The most commonly perceived barriers impeding discontinuation of telemetry, as reported by participants via post‐test, were nursing desires and time. Figure 2 shows all perceived barriers.
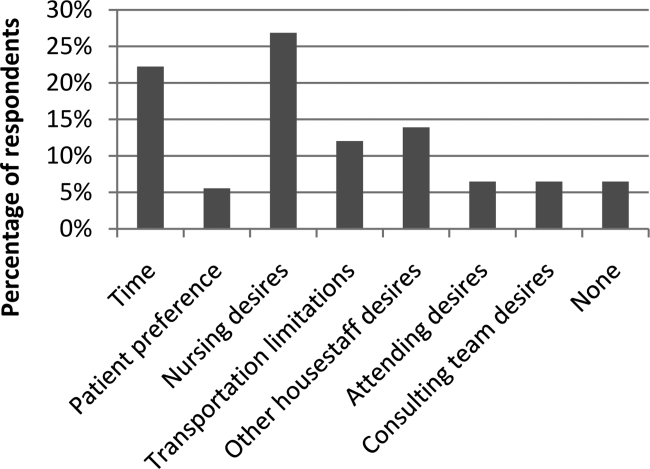
DISCUSSION
Our study is one of the first to our knowledge to demonstrate reductions in telemetry LOS by a hospitalist intervention for telemetry utilization. Others[10, 11] have studied the impact of an orientation handout by chief residents or a multispecialty telemetry policy with enforcement by an outside cardiologist and nurse team. Dressler et al. later sustained a 70% reduction in telemetry use without adversely affecting patient safety, as assessed through numbers of rapid response activations, codes, and deaths, through integrating the AHA guidelines into their electronic ordering system.[12] However, our study has the advantage of the primary team, who knows the patient and clinical scenario best, driving the change during attending rounds. In an era where cost consciousness intersects the practice of medicine, any intervention in patient care that demonstrates cost savings without an adverse impact on patient care and resource utilization must be emphasized. This is particularly important in academic institutions, where residents and medical students are learning to integrate the principles of patient safety and quality improvement into their clinical practice.[13] We actually showed sustained telemetry LOS reductions into the extension period after our intervention. We believe this may be due to telemetry triage being integrated into our attending and resident rounding practices. Future work should include integration of telemetry triage into clinical decision support in the electronic medical record and multidisciplinary rounds to disseminate telemetry triage hospital‐wide in both the academic and community settings.
Our study also revealed that nearly half of participants were not aware of the criteria for appropriate utilization of telemetry before our intervention; in the preintervention period, there were many anecdotal and objective findings of inappropriate utilization of telemetry as well as prolonged continuation beyond the clinical needs in both the hospitalist and nonhospitalist group. For the hospitalist group (ie, the group receiving guideline‐based education on appropriate indications for telemetry utilization), there was an assessment of both appropriate usage and timely discontinuation of telemetry in the postintervention period, which we attribute in large part to adherence to the education provided to this group.
We were able to show increased knowledge of cost‐saving actions among trainees with our educational module. We believe it is imperative to educate our providers (physicians, nurses, case managers, and students within these disciplines) on the appropriate indications for telemetry use, not only to help with cost savings and resource availability (ie, allowing telemetry beds to be available for patients who need them most), but also to instill consistent expectations among our patients. For the hospitalist group (ie, the group receiving guideline‐based education on appropriate indications for telemetry utilization), there was an assessment of both appropriate usage and timely discontinuation of telemetry in the postintervention period, which we attribute in large part to adherence to the education provided to this group.
Additionally, we feel it is important to consider the impacts of inappropriate use of telemetry from a patient's perspective: it is physically restrictive/emnconvenient, alarms are disruptive, it can be a barrier for other treatments such as physical therapy, it may increase the time it takes for imaging studies, a nurse may be required to accompany patients on telemetry, and poses additional costs to their medical bill.
We believe our success is due to several strategies. First, at the start of the fiscal year when quality improvement metrics are established, this particular metric (improving the appropriate utilization and timely discontinuation of telemetry) was deemed important by all hospitalists, engendering group buy‐in prior to the intervention. Our hospitalists received a detailed and interactive tutorial session in person at the beginning of the study. This tutorial provided the hospitalists with a comprehensive understanding of the appropriate (and inappropriate) indications for telemetry monitoring, hence facilitating guideline‐directed utilization. Email reminders and the tutorial tool were provided each time a hospitalist attended on the wards, and hospitalists received a small financial incentive to comply with appropriate telemetry utilization.
Our study has several strengths. First, the time frame of our study was long enough (8 months) to allow consistent trends to emerge and to optimize exposure of housestaff and medical students to this quality‐improvement initiative. Second, our cost savings came from 2 factors, direct reduction of inappropriate telemetry use and reduction in length of stay, highlighting the dual impact of appropriate telemetry utilization on cost. The overall reductions in telemetry utilization for the intervention group were a result of both reductions in initial placement on telemetry for patients who did not meet criteria for such monitoring as well as timely discontinuation of telemetry during the patient's hospitalization. Third, our study demonstrates that physicians can be effective in driving appropriate telemetry usage by participating in the clinical decision making regarding necessity and educating providers, trainees/students, and patients on appropriate indications. Finally, we show sustainment of our intervention in the extension period, suggesting telemetry triage integration into rounding practice.
Our study has limitations as well. First, our sample size is relatively small at a single academic center. Second, due to complexities in our faculty scheduling, we were unable to completely randomize patients to a hospitalist versus nonhospitalist team. However, we believe that despite the inability to randomize, our study does show the benefit of a hospitalist attending to reduce telemetry LOS given there was no change in nonhospitalist telemetry LOS despite all of the other hospital‐wide interventions (multidisciplinary rounds, similar housestaff). Third, our study was limited in that the CMI was used as a proxy for patient complexity, and the mortality index was used as the overall marker of safety. Further studies should monitor frequency and outcomes of arrhythmic events of patients transferred from telemetry monitoring to medicalsurgical beds. Finally, as the intervention was multipronged, we are unable to determine which component led to the reductions in telemetry utilization. Each component, however, remains easily transferrable to outside institutions. We demonstrated both a reduction in initiation of telemetry as well as timely discontinuation; however, due to the complexity in capturing this accurately, we were unable to numerically quantify these individual outcomes.
Additionally, there were approximately 10 nonhospitalist attendings who also staffed the wards during the intervention time period of our study; these attendings did not undergo the telemetry tutorial/orientation. This difference, along with the Hawthorne effect for the hospitalist attendings, also likely contributed to the difference in outcomes between the 2 attending cohorts in the intervention period.
CONCLUSIONS
Our results demonstrate that a multipronged hospitalist‐driven intervention to improve appropriate use of telemetry reduces telemetry LOS and cost. Hence, we believe that targeted, education‐driven interventions with monitoring of progress can have demonstrable impacts on changing practice. Physicians will need to make trade‐offs in clinical practice to balance efficient resource utilization with the patient's evolving condition in the inpatient setting, the complexities of clinical workflow, and the patient's expectations.[14] Appropriate telemetry utilization is a prime example of what needs to be done well in the future for high‐value care.
Acknowledgements
The authors acknowledge the hospitalists who participated in the intervention: Jeffrey Chi, Willliam Daines, Sumbul Desai, Poonam Hosamani, John Kugler, Charles Liao, Errol Ozdalga, and Sang Hoon Woo. The authors also acknowledge Joan Hendershott in the Finance Department and Joseph Hopkins in the Quality Department.
Disclosures: All coauthors have seen and agree with the contents of the article; submission (aside from abstracts) was not under review by any other publication. The authors report no disclosures of financial support from, or equity positions in, manufacturers of drugs or products mentioned in the article.
- 2009. Statistical brief 355. 2012. Agency for Healthcare Research and Quality, Rockville, MD. , National health care expenses in the U.S. civilian noninstitutionalized population,
- Costs for hospital stays in the United States, 2010. Statistical brief 146. 2013. Agency for Healthcare Research and Quality, Rockville, MD. , ,
- Telemetry outside critical care units: patterns of utilization and influence on management decisions. Clin Cardiol. 1998;21(7):503–505. , ,
- Evaluation of telemetry utilization, policy, and outcomes in an inner‐city academic medical center. J Natl Med Assoc. 2010;102(7):598–604. , , , et al.
- Recommended guidelines for in‐hospital cardiac monitoring of adults for detection of arrhythmia. Emergency Cardiac Care Committee members. J Am Coll Cardiol. 1991;18(6):1431–1433. , ,
- Practice standards for electrocardiographic monitoring in hospital settings: an American Heart Association scientific statement from the Councils on Cardiovascular Nursing, Clinical Cardiology, and Cardiovascular Disease in the Young: endorsed by the International Society of Computerized Electrocardiology and the American Association of Critical‐Care Nurses. Circulation. 2004;110(17):2721–2746. , , , et al.
- Is telemetry overused? Is it as helpful as thought? Cleve Clin J Med. 2009;76(6):368–372. , , , , ,
- Society of Hospital Medicine. Adult Hospital Medicine. Five things physicians and patients should question. Available at: http://www.choosingwisely.org/societies/society‐of‐hospital‐medicine‐adult. Published February 21, 2013. Accessed October 5, 2014.
- Joint Commission on Accreditation of Healthcare Organizations. The Joint Commission announces 2014 national patient safety goal. Jt Comm Perspect. 2013;33(7):1–4.
- Optimizing telemetry utilization in an academic medical center. J Clin Outcomes Manage. 2008;15(9):435–440. , , , ,
- Improving utilization of telemetry in a university hospital. J Clin Outcomes Manage. 2005;12(10):519–522. ,
- Altering overuse of cardiac telemetry in non‐intensive care unit settings by hardwiring the use of American Heart Association guidelines. JAMA Intern Med. 2014;174:1852–1854. , , , ,
- "Innovation" institutes in academic health centers: enhancing value through leadership, education, engagement, and scholarship. Acad Med. 2014;89(9):1204–1206. , ,
- Controlling health costs: physician responses to patient expectations for medical care. J Gen Intern Med. 2014;29(9):1234–1241. , , , , ,
- 2009. Statistical brief 355. 2012. Agency for Healthcare Research and Quality, Rockville, MD. , National health care expenses in the U.S. civilian noninstitutionalized population,
- Costs for hospital stays in the United States, 2010. Statistical brief 146. 2013. Agency for Healthcare Research and Quality, Rockville, MD. , ,
- Telemetry outside critical care units: patterns of utilization and influence on management decisions. Clin Cardiol. 1998;21(7):503–505. , ,
- Evaluation of telemetry utilization, policy, and outcomes in an inner‐city academic medical center. J Natl Med Assoc. 2010;102(7):598–604. , , , et al.
- Recommended guidelines for in‐hospital cardiac monitoring of adults for detection of arrhythmia. Emergency Cardiac Care Committee members. J Am Coll Cardiol. 1991;18(6):1431–1433. , ,
- Practice standards for electrocardiographic monitoring in hospital settings: an American Heart Association scientific statement from the Councils on Cardiovascular Nursing, Clinical Cardiology, and Cardiovascular Disease in the Young: endorsed by the International Society of Computerized Electrocardiology and the American Association of Critical‐Care Nurses. Circulation. 2004;110(17):2721–2746. , , , et al.
- Is telemetry overused? Is it as helpful as thought? Cleve Clin J Med. 2009;76(6):368–372. , , , , ,
- Society of Hospital Medicine. Adult Hospital Medicine. Five things physicians and patients should question. Available at: http://www.choosingwisely.org/societies/society‐of‐hospital‐medicine‐adult. Published February 21, 2013. Accessed October 5, 2014.
- Joint Commission on Accreditation of Healthcare Organizations. The Joint Commission announces 2014 national patient safety goal. Jt Comm Perspect. 2013;33(7):1–4.
- Optimizing telemetry utilization in an academic medical center. J Clin Outcomes Manage. 2008;15(9):435–440. , , , ,
- Improving utilization of telemetry in a university hospital. J Clin Outcomes Manage. 2005;12(10):519–522. ,
- Altering overuse of cardiac telemetry in non‐intensive care unit settings by hardwiring the use of American Heart Association guidelines. JAMA Intern Med. 2014;174:1852–1854. , , , ,
- "Innovation" institutes in academic health centers: enhancing value through leadership, education, engagement, and scholarship. Acad Med. 2014;89(9):1204–1206. , ,
- Controlling health costs: physician responses to patient expectations for medical care. J Gen Intern Med. 2014;29(9):1234–1241. , , , , ,
Reduction in Iatrogenic Pneumothorax
Iatrogenic pneumothorax (IAP) is a complication of invasive procedures that is associated with substantial morbidity and some mortality.[1] IAP is often avoidable, and in many cases can be prevented through adherence to evidence‐based guidelines and procedural techniques known to reduce the incidence of IAP.[2] IAP may occur with a subclavian (SC) or internal jugular (IJ) central venous catheter (CVC) insertion, but is more frequently associated with the SC approach.[3] Ultrasound guidance during IJ CVC insertion is associated with a lower risk as compared to guidance by anatomical landmarks.[4, 5] Other bedside procedures that are known to cause IAP include thoracentesis. This risk can also be reduced with the use of ultrasound guidance.[6]
Including simulation in training for CVC insertion has been demonstrated in meta‐analyses to improve both learner outcomes, including simulator performance and perceived confidence, and patient outcomes, including fewer failed CVC attempts and reduced incidence of IAP.[7] Even brief simulation workshops lasting less than two hours can improve patient safety during CVC insertion.[8]
The implementation of ultrasound‐based simulation and improved adherence to the actual use of ultrasound at the bedside can be motivated by tying competency‐based educational objectives (eg, CVC insertion) to clinical outcomes (ie, rates of IAP) and tracking both as part of a continuous quality‐improvement cycle.[9] Adherence to best practices for CVC insertion can also be improved through standardizing hospital‐wide policies and hands‐on training.[10] Involving many stakeholders, including nurses, physicians, nurse practioners and physician assistants, in a multidisciplinary team has been shown to help alter entrenched behaviors and reduce the incidence of central‐line associated bloodstream infections through long‐term adherence to evidence‐based interventions.[11]
LOCAL PROBLEM
The Agency for Healthcare Research and Quality (AHRQ) has designed Patient Safety Indicators (PSIs) (
Our hospital is a member of the University HealthSystem Consortium (UHC) (
Despite this, the PSI can highlight areas where quality‐improvement efforts might be best directed. In 2005 and 2006, our hospital was ranked within the lowest UHC performance quartile for all‐cause IAP PSI.
During FY 2006 (September 2005August 2006), root‐cause analysis on cases of IAP at our hospital found that CVC insertion (40%) was the most common procedure associated with IAP, with SC insertion causing 69% of CVC‐associated IAP. Other common procedures associated with IAP were operative/pacemaker (30%), thoracentesis (25%), and ventilator associated (5%). Ultrasound was not used in 2/5 cases of IJ CVC placement and 3/5 thoracentesis cases. Only 44% of CVC insertions had a procedure note.
Intended Improvement/Study Question
Our team set out to plan and implement a set of multifaceted interventions within 90 days. The short‐term goal was a 50% reduction in the CVC IAP and all‐cause IAP rate within 18 months, and the long‐term goal was sustained reduction of CVC IAP and all‐cause IAP rate.
METHODS
The format of this article is based on the standards for quality‐improvement reporting excellence guidelines for the reporting of studies on the effectiveness of quality‐improvement interventions.[14]
Setting
Stanford University Medical Center is an academic medical center with 465 beds and over 25,000 inpatient admissions per year, providing both general acute care services and tertiary medical care. Residents perform CVC bedside procedures when central venous access is needed, in the intensive care unit (ICU), operating room (OR), and inpatient units. Prior to this project, ultrasound equipment was only available in the emergency department (ED) and ICUs. There was no formal CVC procedure supervision policy, CVC training curriculum, and procedure note templates for documentation of CVC insertion.
Planning the Interventions
A multidisciplinary quality‐improvement team met weekly during the 90‐day design period from January 2007 to March 2007. Our team included representatives from the departments of medicine, anesthesia and critical care, surgery, nursing, and emergency medicine. We also partnered with our institution's clinical and administrative leaders, experts in simulation, and the hospital quality department.
We hypothesized that a standardized set of education and training interventions promoting ultrasound‐guided IJ CVC insertion as the method of choice at our hospital would significantly reduce our rate of CVC‐associated IAP. Our multifaceted intervention included: (1) clinical and documentation standards based on evidence, (2) cognitive aids, (3) simulation training, (4) purchase and deployment of ultrasound equipment, and (5) feedback to clinical services.
Our team followed the define, measure, analyze, improve, control (DMAIC) framework.[15] We set interval goals with target completion dates throughout the 90‐day period, identified owners of each goal, and tracked progress with a shared spreadsheet.
In the 90‐day intervention, we accomplished the following: (1) conducted root‐cause analysis of IAP cases for fiscal year 2006, (2) created clinical and documentation standards around CVC placement, (3) created cognitive aids and procedure note templates, (4) developed simulation training courses, and (5) requested purchase of additional ultrasound equipment.
Data Collection
To evaluate our progress in reducing the rates of IAP, we tracked the incidence of IAP using UHC and AHRQ PSI methodology. In collaboration with our hospital's quality department, we manually reviewed every PSI‐identified case of IAP. This review has focused on identifying whether or not pneumothorax actually occurred, and whether it was associated with CVC insertion. For those associated with CVC, data were collected for patient location and service, the procedure site, whether ultrasound was used, whether a chest tube was required, and the final disposition of the patient.
Demographic data (age, gender, case mix index [CMI]) shown in Table 1 were obtained through MIDAS+ Solutions (Tucson, Arizona), a proprietary database that contains healthcare management coded data. Total hospital CVC insertion rates were calculated using International Classification of Diseases, Ninth Revision (ICD‐9) coding for 38.93 and 38.97. ICU central lineassociated blood stream infections (CLABSI) data were obtained from internal collection by our infection control team. Number and location of CVCs placed in the ICU data were obtained from nursing flow sheets in our electronic medical record (EMR). Cost information was provided by our finance department using internal accounting.
Patients With CVC Insertion | Year | ||||||||
---|---|---|---|---|---|---|---|---|---|
2006 | 2007 | 2008 | 2009 | 2010 | 2011 | 2012 | 2013 | 2014 | |
| |||||||||
Age, y (mean) | 55.0 | 55.5 | 55.0 | 57.0 | 56.5 | 58.5 | 57.5 | 59.0 | 58.5 |
% female | 47.0 | 49.5 | 47.0 | 48.8 | 46.2 | 46.1 | 45.7 | 46.2 | 45.7 |
Case‐mix index | 3.08 | 3.35 | 3.21 | 3.40 | 3.71 | 3.91 | 3.92 | 3.92 | 4.08 |
Total no. of CVCs/year* | 1,593 | 1,141 | 1,589 | 2,250 | 2,441 | 2,774 | 2,754 | 2,722 | 2,845 |
No. of CVCs/year in ICU | NA | NA | NA | 1,502 | 1,357 | 1,345 | 1,316 | 1,421 | 1,590 |
No. of subclavians/year in ICU | NA | NA | NA | 167 | 75 | 70 | 83 | 75 | 97 |
No. of IJs/year in ICU | NA | NA | NA | 898 | 773 | 681 | 677 | 713 | 876 |
No. of femorals/year in ICU | NA | NA | NA | 212 | 152 | 203 | 171 | 198 | 206 |
No. of PICCs/year in ICU | NA | NA | NA | 225 | 357 | 391 | 385 | 435 | 411 |
Preintervention (2006) | Postintervention (20082014) | P Value | |||||||
Age, y (mean) | 55.2 | 58.7 | <0.0001 | ||||||
% female | 47.0% | 46.4% | 0.642 | ||||||
Case‐mix index | 3.08 | 3.73 | <0.0001 | ||||||
CVC insertion rate | 8.1% | 11.4% | <0.0001 | ||||||
All Inpatients | Year | ||||||||
2006 | 2007 | 2008 | 2009 | 2010 | 2011 | 2012 | 2013 | 2014 | |
Age, y (mean) | 57.1 | 57.2 | 56.8 | 57.2 | 57.5 | 58.0 | 58.0 | 57.9 | 58.3 |
% female | 51.6 | 51.2 | 52.4 | 51.7 | 51.1 | 51.5 | 50.3 | 49.9 | 50.1 |
Case‐mix index | 1.86 | 1.98 | 1.96 | 1.99 | 1.96 | 2.02 | 2.03 | 2.07 | 2.23 |
Preintervention (2006) | Postintervention (20082014) | P Value | |||||||
Age, y (mean) | 57.1 | 57.6 | <0.01 | ||||||
% female | 51.6% | 50.9% | 0.07 | ||||||
Case‐mix index | 1.86 | 2.03 | 0.13 | ||||||
Central Line‐Associated Bloodstream Infections per 1,000 Central Line Days | |||||||||
Preintervention | Postintervention | P Value | |||||||
Short term (2006 vs 2008) | 1.8 | 0.60 | 0.004 | ||||||
Long term (2006 vs 20082014) | 1.8 | 0.68 | <0.0001 |
The project granted a Notice of Determination of Approval from the Stanford Administrative Panels for the Protection of Human Subjects (institutional review board).
Methods of Evaluation/Analysis
For the purpose of this analysis, the preintervention period was defined as January 1, 2006 through December 31, 2006, our first year of IAP case review. We defined the intervention period as January 1, 2007 through December 31, 2007, during which we planned and implemented hospital‐wide standardization of CVC insertion practices and incorporated CVC insertion training simulation into resident orientation in July 2007. The postintervention period was defined as January 1, 2008 through December 31, 2014.
All statistical analyses were performed using Stata version 12.1 (StataCorp, College Station, TX). [2] tests were used to determine statistical differences in pre‐ versus postintervention patient demographic data (age, gender, CMI), CVC insertion rates, and CLABSI rates. Because IAP is a rare event, a statistical process control g‐chart was created using QI Macros (KnowWare International, Inc., Denver, CO) to show the number of CVC procedures between IAP. [2] and Fisher exact tests were used to determine statistical differences in CVC anatomic location and use of ultrasound pre‐ and postintervention. A 2‐sided Z test to show a difference in proportions was used to determine statistical differences in CVC‐related IAP rate and all‐cause IAP rate pre‐ and postintervention.
Measuring Adherence to Intervention
Location of CVC Placement and Ultrasound Guidance Pre‐ Versus Postintervention
We utilized the Stanford Clinical Informatics Center (SCCI) services for obtaining counts of patients. Custom queries were performed on SCCI's Stanford Translational Research Integrated Database Environment (STRIDE) platform[16] to search Stanford Hospital electronic heath records for patients. This search primarily involved getting counts for the number of patients with clinical notes that contained the keywords of interest. To identify documentation for placement of CVC from 2006 to 2014, procedure or operative notes containing the words central line or CVC were counted. Further subcounts were obtained by searching for additional keywords such as PICC [peripherally inserted central catheters], femoral, jugular, subclavian, and ultrasound.
Adherence to Intervention in the ICU in 2014
A total of 100 charts were reviewed from patients in our medical and surgical ICU with a CVC in 2014 to evaluate the current trend of central line placement and sustainability of our intervention. Fifty charts were initially randomly selected from the ICU cohort. For those who had multiple lines placed, only the first line was reviewed. Because the initial audit did not provide enough SC lines and we wanted to review more IJ lines, we randomly selected an additional 25 patients who had SC and 25 patients who had IJ to review. The following was collected during chart review: primary team, location of line placement, usage of ultrasound, usage of standard procedure template, supervision, level of training for supervisor, and level of training for staff who performed procedure.
Outcomes
The rate of CVC‐associated IAP was calculated as the total number of IAPs attributed to CVCs divided by the total number of CVCs inserted determined by ICD‐9 coding for 38.93 and 38.97. The total IAP rate was calculated as the total number of IAP/1000 discharges.
RESULTS
Interventions
Our team began the intervention in early 2007 with promotion of ultrasound‐guided IJ catheterization. Clinical exceptions included: (1) trauma or code situations where access to the neck is limited, (2) suspected or confirmed neck injuries, (3) presence of a tracheostomy, and (4) bilateral internal jugular sites unsuitable for catheterization.
Our hospital adopted new formal CVC insertion policies consistent with the above training and education efforts. All physicians were required to document CVC insertions using the template available in the EMR. To be certified to perform CVC insertion independently, trainee physicians were required to complete the simulation training and successfully place a minimum of 5 CVCs directly supervised by an already‐certified physician. This was consistent with the Accreditation Council for Graduate Medical Education suggested minimum requirement in 2007. In our critical care units, all CVC insertions must be supervised by an ICU fellow or attending.
To reinforce the on‐the‐ground work by our physician leaders, we created 2 education tools to embed best practices into our CVC insertion workflow. A checklist with best practices for CVC insertion that was distributed throughout the hospital via central line kits and educational flyers, and a CVC insertion procedure note template consistent with California Department of Public Health documentation requirements was made available in our EMR.
In June 2007, we integrated CVC insertion simulation training into procedure workshops required for all medicine, surgery, anesthesia, and emergency medicine trainees during their intern year. These workshops promoted ultrasound‐guided IJ catheterization and supporting evidence for the new IJ site preference. Training sessions were 2 to 3 hours, and included a demonstration of best‐practice CVC insertion, as well as training with simulation models supervised by an instructor using a standardized CVC checklist. These trainings used both the Blue Phantom human torso model (
Hospital administration provided funds to purchase ultrasound machines for patient units such as medicine, cardiology, ED, and ICU). A total of 4 Site‐Rite (Bard Access Systems, Inc., Salt Lake City, UT) ultrasounds were purchased in 2007. The hospital has continued to purchase ultrasound units yearly, and had 53 ultrasound units in 2014
Cases of IAP were continuously reviewed throughout the intervention period. Based on their higher CVC‐associated IAP rates, the ORs and catheterization lab were identified as having opportunities for improvement. In 2008, Hospital quality‐improvement leadership met with physician leaders in these areas to review their CVC‐related IAP data and to discuss strategies to reduce their IAP rates. These strategies included lessons learned from other services that had successfully decreased their IAP rates.
To sustain our gains, we continue to review all IAP through our coding quality, clinical documentation, quality reporting departments, and peer review. We have implemented other strategies to decrease IAP, such as the use of ultrasound guidance for bedside thoracentesis procedures, which became possible after the availability of more ultrasound devices. Training for ultrasound‐guided thoracentesis was done by our procedure‐team attending during supervision of residents.
Outcomes
Preintervention (January 1, 2006 to December 31, 2006)
There were a total of 26 cases of IAP in 2006. Of these, 15 (58%) were associated with CVC insertion (Figure 1). The single procedure associated with the largest proportion of IAP was SC CVC insertion (11 cases, 42% of all IAP cases). Eleven CVC‐associated IAPs were significant enough to require chest tube placement. Our hospital recorded a total of 1593 CVC insertions (ICD‐9 codes 38.93 and 38.97) in 2006.
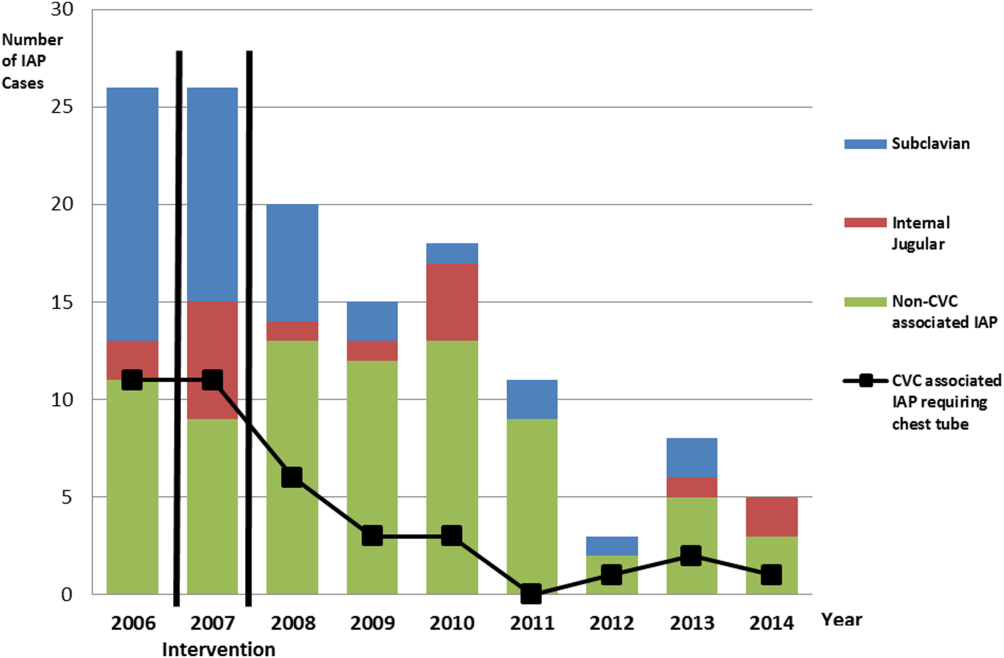
Postintervention (January 1, 2008 to December 31, 2014)
There were a total of 80 cases of IAP over 7 years, of which 24 (30%) were associated with CVC insertion. Of these, 16 required chest tube placement. In the last 4 years of the postintervention period (20112014), there were only 5 cases of CVC‐associated IAP requiring chest tube placement (Figure 1). There were a total of 12,000 CVC insertions recorded over the same period.
We successfully met both our short‐ and long‐term goals. Our preintervention CVC‐associated IAP rate was 0.94%, and our post‐intervention rate during 2008 was 0.44%, a short‐term reduction of 53% (P=0.088). Our average postintervention CVC‐associated IAP rate for the years 2008 through 2014 was 0.13%, a significant long‐term reduction of 86% (P<0.0001) (Table 2). The decrease in CVC‐associated IAP rates occurred despite an older patient population (P<0.001) and a higher CMI (P<0.001) in postintervention patients who received a CVC (Table 1). Special cause variation corresponding to a change in our process is demonstrated in Figure 2. The preintervention average number of procedures between IAP was 114.8 and increased to 460.7 in the postintervention period.
Total CVC (n=95) | Subclavian (n=29) | Internal Jugular (n=58) | Femoral (n=8) | ||||||||||
---|---|---|---|---|---|---|---|---|---|---|---|---|---|
| |||||||||||||
Compliance to intervention | |||||||||||||
US guided | 68.1% | 20.7% | 86.2% | 100.0% | |||||||||
Procedure note completion | 90.4% | 93.1% | 86.2% | 100.0% | |||||||||
Supervision | 70.2% | 77.8% | 73.1% | 87.5% | |||||||||
Level of training | |||||||||||||
Resident | 61.1% | 58.6% | 60.3% | 75.0% | |||||||||
Fellow | 25.3% | 27.6% | 24.1% | 25.0% | |||||||||
Attending | 4.2% | 6.9% | 3.4% | 0.0% | |||||||||
Advance practitioner | 3.2% | 3.4% | 3.4% | 0.0% | |||||||||
Unknown | 6.3% | 3.4% | 8.6% | 0.0% | |||||||||
Supervisor type | |||||||||||||
Resident | 3.0% | 4.8% | 2.6% | 0.0% | |||||||||
Fellow | 54.5% | 33.3% | 57.9% | 100.0% | |||||||||
Attending | 42.4% | 61.9% | 39.5% | 0.0% | |||||||||
Location of CVC Placement | Internal Jugular (n=25) | Subclavian (n=25) | |||||||||||
MICU | 32.0% | 32.0% | |||||||||||
SICU* | 40.0% | 52.0% | |||||||||||
Operating room | 28.0% | 16.0% | |||||||||||
Average no. of attempts/procedure | 1.4 | 1.5 | |||||||||||
Indications for subclavian insertion (n=25) | |||||||||||||
Trauma/surgical site | 60.0% | ||||||||||||
Need for additional access | 16.0% | ||||||||||||
Unsuccessful IJ placement | 4.0% | ||||||||||||
Unclear | 20.0% | ||||||||||||
Iatrogenic Pneumothorax Rate (20062014) | Year | ||||||||||||
2006 | 2007 | 2008 | 2009 | 2010 | 2011 | 2012 | 2013 | 2014 | |||||
% of CVC insertions associated with IAP | 0.94 | 1.49 | 0.44 | 0.13 | 0.20 | 0.07 | 0.04 | 0.11 | 0.07 | ||||
All‐cause IAP per 1,000 discharges | 1.32 | 1.29 | 0.98 | 0.71 | 0.83 | 0.49 | 0.13 | 0.35 | 0.23 | ||||
Preintervention | Postintervention | P Value | |||||||||||
CVC‐ associated IAP short term (2006 vs 2008) | 0.94% | 0.44% | 0.088 | ||||||||||
CVC‐associated IAP long term (2006 vs 20082014) | 0.94% | 0.13% | <0.0001 | ||||||||||
All‐cause IAP per 1,000 discharges short term (2006 vs 2008) | 1.32 | 0.98 | <0.0001 | ||||||||||
All‐cause IAP per 1,000 discharges long term (2006 vs 2008‐14) | 1.32 | 0.52 | <0.0001 |
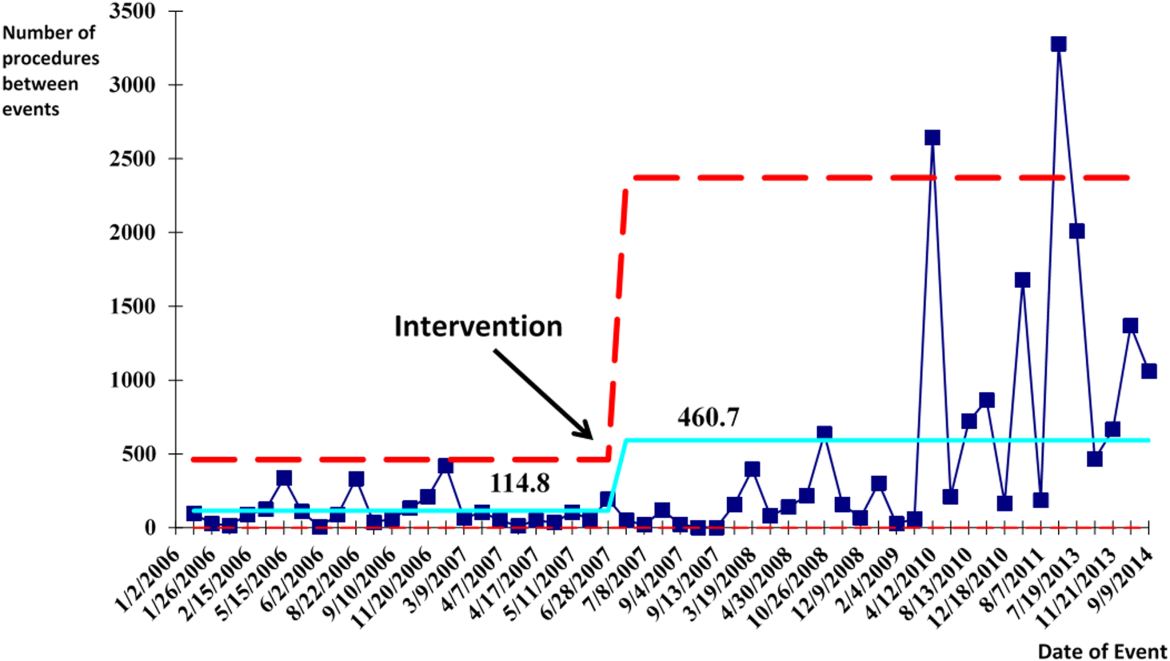
With the decrease in CVC‐associated IAP, we also saw a decrease in our all‐cause IAP rate per 1000 discharges from 1.32 in 2006 to 0.98 in 2008. This represents a 26% short‐term reduction (P<0.0001). We also saw a decrease in our all‐cause IAP rate per 1000 discharges to 0.52 from 2008 to 2014, representing a 61% long‐term reduction (P<0.0001). This decrease in all‐cause IAP postintervention occurred despite an older patient population (P<0.01) for all discharges. Our hospital is now in the highest performance UHC quartile for all‐cause IAP in 2012 to 2014.
After our multifaceted intervention in 2007, there was substantially more and consistent documentation of CVC procedure notes from less than 500 in 2006 to greater than 2000 in 2009. The distribution of CVC procedure notes in the pre‐ (2006) versus postintervention (20082014) period showed a decrease in the proportion of femoral lines from 15% to 11%, increase in IJ lines from 31% to 49%, and a decrease in SC from 54% to 40% (P=0.001). The distribution of IJ CVC procedure notes in the pre‐ (2006) versus postintervention (20082014) period showed an increase in the proportion of procedures with ultrasound documentation from 13% to 93% (P<0.001) (Figure 3).
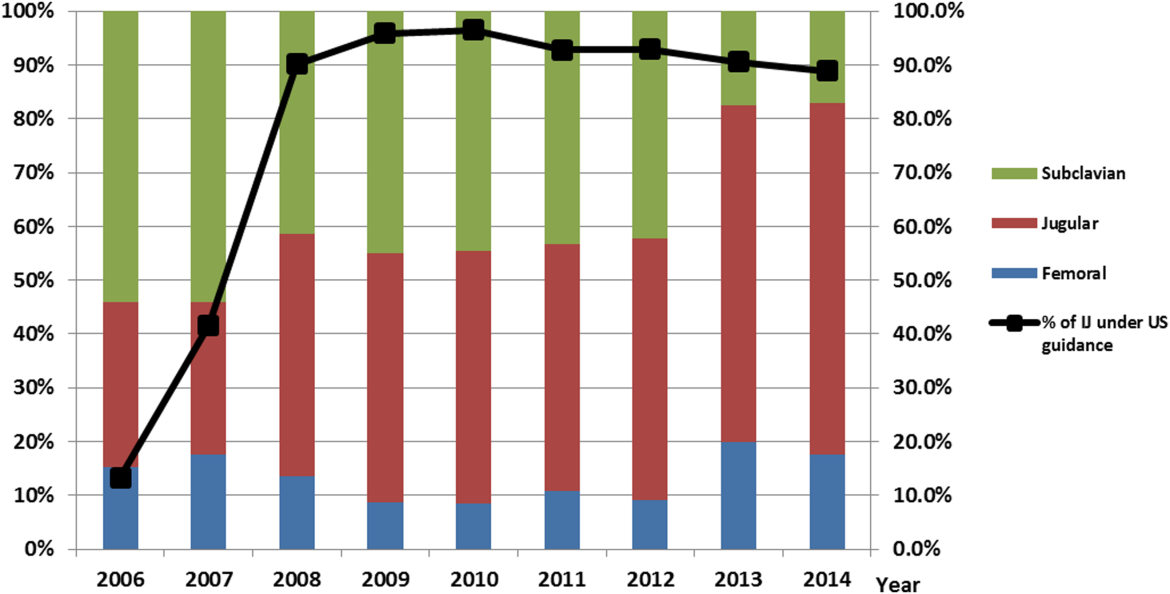
In our ICU 2014 audit, the majority of CVC lines were placed by residents under supervision (>70%), and most used the standard CVC note template to document the procedure (90%). Of the total CVC approach, 66% were IJ and 4% were SC. Eighty‐six percent used ultrasound during IJ placement. The majority of SC insertions were placed in the surgical ICU and had clear indications (80%) for placement. Of those, 75% were due to trauma (limited access to neck) or surgery (interfering with surgical site) (Table 2).
DISCUSSION
Summary
This quality‐improvement intervention demonstrates that a multidisciplinary team can successfully implement a multifaceted intervention that sustainably reduces the rate of IAP complications from CVC placement and improves patient safety over 7 years. We found high compliance with our intervention, which included an increase in CVC notes and documentation of ultrasound guidance. There was also an increase in the IJ approach in our postintervention period. We showed statistically significant long‐term reductions in both CVC‐associated and all‐cause IAP rates. From 2011 to 2014, there were only 5 cases of CVC‐associated IAP requiring chest tube placement. Post hoc analysis showed a statistically significant decrease in CLABSI rates (P<0.0001) from a preintervention rate of 1.6 infections per 1000 central line days to postintervention average rate of 0.68 infections per 1000 central line days. This decrease may be related to the incorporation of wide sterile barrier techniques in our CVC training workshops, checklists, and template procedure notes.
A strength of this study is the sustained significant long‐term reduction in IAP. There are few data that exist to describe sustained interventions in this area. Sustainability was achieved by integrating our interventions into ongoing programs that already existed in the hospital; we incorporated our simulation training into the existing new resident orientation, increased the availability of existing ultrasound equipment, and continued our IAP chart review through coding quality with feedback to involved services. The procedure note template continues to be easily available in our EMR, and the SC approach to CVC placement is limited to select cases.
Based on a post hoc cost‐benefit analysis, the financial benefits of decreasing the rate of IAP outweigh the costs associated with implementation of this initiative. The purchase cost for a Site‐Rite (Bard Access Systems) ultrasound machine was $18,000. The cost of materials for 1 workshop is $5000 annually. Cases from the Nationwide Inpatient Sample that were flagged by this PSI had 7.0% excess mortality, 4.4 days of excess hospitalization, and approximately $18,000 in excess hospital charges.[17, 18] Based on these data, if we had continued at our preintervention rate of CVC‐associated IAP requiring chest tube placement, we would estimate 9 additional CVC‐associated IAPs requiring chest tube insertion per year. This would result in over $180,000 of additional costs annually. Based on an initial cost of $100,000 for 4 workshops and the necessary equipment, we would have realized our cost savings in less than 1 year postintervention. These are all approximate costs, and further detailed analysis is needed.
One challenge with this intervention is the culture change away from using the SC approach, and the concern from trainees of how they would learn to perform SC CVC if needed. We would suggest dedicated SC CVC ultrasound training for those services who may need to use this approach (eg, neuroanesthesia and trauma).
Interpretation/Relation to Other Evidence
The field of implementation science can help explain why some projects are successful and others fail. We can further dissect the success of this project using an implementation science model similar to that described by French et al.[19] French et al. describe 4 behavior‐change techniques. These steps include (1) who needs to do what differently, (2) which barriers and enablers need to be addressed, (3) which intervention component could overcome the barriers and enhance enablers, and (4) how can behavior change be measured and understood. Barriers included suboptimal skills of residents, low awareness of evidence‐based guidelines, and entrenched practices inconsistent with best evidence. There was also a belief that IJ lines were more likely to become infected. Targeted behaviors needing to be done differently were the choice of CVC placement site and insertion technique. Barriers to change were assessed by asking members of the project team to explore with members of their service what led them to do CVC lines without ultrasound guidance. Enhancements focused on information provision, simulation practice, and persuasive communication. Behavior change was measured by tracking the number of IAPs, site of CVC, and documentation of technique. Continuation of these interventions based on this theoretical framework drove maintenance of gains.
We completed our main intervention planning in 90 days, and met our short‐term goal on schedule. The Institute for Healthcare Improvement (IHI) advocates that such short timelines are efficient mechanisms for developing and acting on projects. Other institutions have reported on similar rapid‐cycle planning and short‐term goal setting[20]
Limitations
Our study captures the experience of a quality‐improvement team at a single academic center, and our results may not be generalizable to other institutions. Our chart review process only occurred once a case had been identified through AHRQ PSI methodology. It is possible that the PSI does not capture all cases of IAP, although we believe our coding department has a very rigorous process to look for all IAP evidence in the patient's record. We used administrative data to determine the number of hospital‐wide CVC procedures.
Our compliance data with interventions from STRIDE are based on looking for key words in procedure note documentation (so undocumented notes are not captured). To validate this, we performed a manual audit of our adherence to our intervention in 2014, and those data are consistent with the results from our STRIDE data.
Our study's observational design also cannot control for exogenous effects on physician practice relating to CVC insertion or the overall risk of IAP. Some of our decrease in complications may be from the increase in PICC line use. Nevertheless, our CVC‐associated IAP rate has decreased despite >6000 non‐PICC CVCs in our ICU over the past 5 years, and a rising CMI (18% increase in postintervention period) and older population of patients with CVC insertion (P<0.0001)
CONCLUSIONS
We are the first, to our knowledge, to report a measurable improvement in reducing IAP patient outcomes that has been sustained for over 7 years. Our hospital is in the highest performance UHC quartile for all‐cause IAP in 2012 to 2014. A multidisciplinary quality‐improvement team, focused on evidence, patient safety, and standardization, can use a multifaceted intervention to sustainably improve patient outcomes. Promoting ultrasound‐guided IJ catheterization as the CVC insertion method of choice significantly reduced our hospital's rate of CVC‐associated IAP.
Acknowledgements
The authors acknowledge many who have contributed to this quality‐improvement project:
Irina Tokareva, Jay Lee, Kourt Bowes, and Gomathi Krishnan for data analysis; Laura Meinke for significant website curriculum; Fred Mihm, Sarah Williams, and John Kugler for leadership in ultrasound training; Kevin Tabb and Norm Rizk for hospital financial support of simulation workshops and ultrasound machines; Pooja Loftus and Helene Grossman for statistical analysis; Eric Hadhazy for data support; Joan Hendershott for cost information; Nancy Szaflarski for project management and manuscript review; and Isabella Chu for manuscript review.
Disclosures: STRIDE (Stanford Translational Research Integrated Database Environment) is a research and development project at Stanford University to create a standards‐based informatics platform supporting clinical and translational research. This STRIDE project was supported by the National Center for Research Resources and the National Center for Advancing Translational Sciences, National Institutes of Health, through grant UL1 RR025744. The content is solely the responsibility of the authors and does not necessarily represent the official views of the NIH. The authors report no conflicts of interest.
- Significance of iatrogenic pneumothoraces. Chest. 1994;105(4):1147–1150. , , .
- How to avoid and manage a pneumothorax. J Vasc Access. 2006;7(1):7–14. , , , , , .
- Iatrogenic pneumothorax: etiology, incidence and risk factors. Thorac Cardiovasc Surg. 2009;57(5):286–290. , , , .
- Real‐time ultrasound‐guided catheterisation of the internal jugular vein: a prospective comparison with the landmark technique in critical care patients. Crit Care. 2006;10(6):R162. , , , et al.
- Safe placement of central venous catheters: a measured approach. J Intens Care Med. 2011;26(6):392–396. , , .
- Reducing iatrogenic risk in thoracentesis: establishing best practice via experiential training in a zero‐risk environment. Chest. 2009;135(5):1315–1320. , , , .
- Use of simulation‐based education to improve outcomes of central venous catheterization: a systematic review and meta‐analysis. Acad Med. 2011;86(9):1137–1147. , , , , , .
- A prerotational, simulation‐based workshop improves the safety of central venous catheter insertion: results of a successful internal medicine house staff training program. Chest. 2011;140(3):652–658. , , , , , .
- Linking residency training effectiveness to clinical outcomes: a quality improvement approach. Jt Comm J Qual Patient Saf. 2010;36(5):203–208. , , , , .
- Education of physicians‐in‐training can decrease the risk for vascular catheter infection. Ann Intern Med. 2000;132(8):641–648. , , , et al.
- A multidisciplinary approach to reduce central line‐associated bloodstream infections. Jt Comm J Qual Patient Saf. 2013;39(2):61–69. , , , et al.
- Validity of selected Patient Safety Indicators: opportunities and concerns. J Am Coll Surg. 2011;212(6):924–934. , , , et al.
- Cases of iatrogenic pneumothorax can be identified from ICD‐9‐CM coded data. Am J Med Qual. 2010;25(3):218–224. , , , et al.
- SQUIRE development group. Publication guidelines for quality improvement studies in health care: evolution of the SQUIRE project. BMJ. 2009;338:a3152. , , , , ;
- The Quality Toolbox. 2nd ed. Milwaukee, WI: ASQ Quality Press; 2005. .
- STRIDE—an integrated standards‐based translational research informatics platform. AMIA Annu Symp Proc. 2009;2009:391–395. , , , .
- Accidental iatrogenic pneumothorax in hospitalized patients. Med Care. 2006;44(2):182–186. , , .
- Excess length of stay, charges, and mortality attributable to medical injuries during hospitalization. JAMA. 2003;290(14):1868–1874. , .
- Developing theory‐informed behaviour change interventions to implement evidence into practice: a systematic approach using the Theoretical Domains Framework. Implement Sci. 2012;7:38. , , , et al.
- Using rapid‐cycle quality improvement methodology to reduce feeding tubes in patients with advanced dementia: before and after study. BMJ. 2004;329(7464):491–494. , .
Iatrogenic pneumothorax (IAP) is a complication of invasive procedures that is associated with substantial morbidity and some mortality.[1] IAP is often avoidable, and in many cases can be prevented through adherence to evidence‐based guidelines and procedural techniques known to reduce the incidence of IAP.[2] IAP may occur with a subclavian (SC) or internal jugular (IJ) central venous catheter (CVC) insertion, but is more frequently associated with the SC approach.[3] Ultrasound guidance during IJ CVC insertion is associated with a lower risk as compared to guidance by anatomical landmarks.[4, 5] Other bedside procedures that are known to cause IAP include thoracentesis. This risk can also be reduced with the use of ultrasound guidance.[6]
Including simulation in training for CVC insertion has been demonstrated in meta‐analyses to improve both learner outcomes, including simulator performance and perceived confidence, and patient outcomes, including fewer failed CVC attempts and reduced incidence of IAP.[7] Even brief simulation workshops lasting less than two hours can improve patient safety during CVC insertion.[8]
The implementation of ultrasound‐based simulation and improved adherence to the actual use of ultrasound at the bedside can be motivated by tying competency‐based educational objectives (eg, CVC insertion) to clinical outcomes (ie, rates of IAP) and tracking both as part of a continuous quality‐improvement cycle.[9] Adherence to best practices for CVC insertion can also be improved through standardizing hospital‐wide policies and hands‐on training.[10] Involving many stakeholders, including nurses, physicians, nurse practioners and physician assistants, in a multidisciplinary team has been shown to help alter entrenched behaviors and reduce the incidence of central‐line associated bloodstream infections through long‐term adherence to evidence‐based interventions.[11]
LOCAL PROBLEM
The Agency for Healthcare Research and Quality (AHRQ) has designed Patient Safety Indicators (PSIs) (
Our hospital is a member of the University HealthSystem Consortium (UHC) (
Despite this, the PSI can highlight areas where quality‐improvement efforts might be best directed. In 2005 and 2006, our hospital was ranked within the lowest UHC performance quartile for all‐cause IAP PSI.
During FY 2006 (September 2005August 2006), root‐cause analysis on cases of IAP at our hospital found that CVC insertion (40%) was the most common procedure associated with IAP, with SC insertion causing 69% of CVC‐associated IAP. Other common procedures associated with IAP were operative/pacemaker (30%), thoracentesis (25%), and ventilator associated (5%). Ultrasound was not used in 2/5 cases of IJ CVC placement and 3/5 thoracentesis cases. Only 44% of CVC insertions had a procedure note.
Intended Improvement/Study Question
Our team set out to plan and implement a set of multifaceted interventions within 90 days. The short‐term goal was a 50% reduction in the CVC IAP and all‐cause IAP rate within 18 months, and the long‐term goal was sustained reduction of CVC IAP and all‐cause IAP rate.
METHODS
The format of this article is based on the standards for quality‐improvement reporting excellence guidelines for the reporting of studies on the effectiveness of quality‐improvement interventions.[14]
Setting
Stanford University Medical Center is an academic medical center with 465 beds and over 25,000 inpatient admissions per year, providing both general acute care services and tertiary medical care. Residents perform CVC bedside procedures when central venous access is needed, in the intensive care unit (ICU), operating room (OR), and inpatient units. Prior to this project, ultrasound equipment was only available in the emergency department (ED) and ICUs. There was no formal CVC procedure supervision policy, CVC training curriculum, and procedure note templates for documentation of CVC insertion.
Planning the Interventions
A multidisciplinary quality‐improvement team met weekly during the 90‐day design period from January 2007 to March 2007. Our team included representatives from the departments of medicine, anesthesia and critical care, surgery, nursing, and emergency medicine. We also partnered with our institution's clinical and administrative leaders, experts in simulation, and the hospital quality department.
We hypothesized that a standardized set of education and training interventions promoting ultrasound‐guided IJ CVC insertion as the method of choice at our hospital would significantly reduce our rate of CVC‐associated IAP. Our multifaceted intervention included: (1) clinical and documentation standards based on evidence, (2) cognitive aids, (3) simulation training, (4) purchase and deployment of ultrasound equipment, and (5) feedback to clinical services.
Our team followed the define, measure, analyze, improve, control (DMAIC) framework.[15] We set interval goals with target completion dates throughout the 90‐day period, identified owners of each goal, and tracked progress with a shared spreadsheet.
In the 90‐day intervention, we accomplished the following: (1) conducted root‐cause analysis of IAP cases for fiscal year 2006, (2) created clinical and documentation standards around CVC placement, (3) created cognitive aids and procedure note templates, (4) developed simulation training courses, and (5) requested purchase of additional ultrasound equipment.
Data Collection
To evaluate our progress in reducing the rates of IAP, we tracked the incidence of IAP using UHC and AHRQ PSI methodology. In collaboration with our hospital's quality department, we manually reviewed every PSI‐identified case of IAP. This review has focused on identifying whether or not pneumothorax actually occurred, and whether it was associated with CVC insertion. For those associated with CVC, data were collected for patient location and service, the procedure site, whether ultrasound was used, whether a chest tube was required, and the final disposition of the patient.
Demographic data (age, gender, case mix index [CMI]) shown in Table 1 were obtained through MIDAS+ Solutions (Tucson, Arizona), a proprietary database that contains healthcare management coded data. Total hospital CVC insertion rates were calculated using International Classification of Diseases, Ninth Revision (ICD‐9) coding for 38.93 and 38.97. ICU central lineassociated blood stream infections (CLABSI) data were obtained from internal collection by our infection control team. Number and location of CVCs placed in the ICU data were obtained from nursing flow sheets in our electronic medical record (EMR). Cost information was provided by our finance department using internal accounting.
Patients With CVC Insertion | Year | ||||||||
---|---|---|---|---|---|---|---|---|---|
2006 | 2007 | 2008 | 2009 | 2010 | 2011 | 2012 | 2013 | 2014 | |
| |||||||||
Age, y (mean) | 55.0 | 55.5 | 55.0 | 57.0 | 56.5 | 58.5 | 57.5 | 59.0 | 58.5 |
% female | 47.0 | 49.5 | 47.0 | 48.8 | 46.2 | 46.1 | 45.7 | 46.2 | 45.7 |
Case‐mix index | 3.08 | 3.35 | 3.21 | 3.40 | 3.71 | 3.91 | 3.92 | 3.92 | 4.08 |
Total no. of CVCs/year* | 1,593 | 1,141 | 1,589 | 2,250 | 2,441 | 2,774 | 2,754 | 2,722 | 2,845 |
No. of CVCs/year in ICU | NA | NA | NA | 1,502 | 1,357 | 1,345 | 1,316 | 1,421 | 1,590 |
No. of subclavians/year in ICU | NA | NA | NA | 167 | 75 | 70 | 83 | 75 | 97 |
No. of IJs/year in ICU | NA | NA | NA | 898 | 773 | 681 | 677 | 713 | 876 |
No. of femorals/year in ICU | NA | NA | NA | 212 | 152 | 203 | 171 | 198 | 206 |
No. of PICCs/year in ICU | NA | NA | NA | 225 | 357 | 391 | 385 | 435 | 411 |
Preintervention (2006) | Postintervention (20082014) | P Value | |||||||
Age, y (mean) | 55.2 | 58.7 | <0.0001 | ||||||
% female | 47.0% | 46.4% | 0.642 | ||||||
Case‐mix index | 3.08 | 3.73 | <0.0001 | ||||||
CVC insertion rate | 8.1% | 11.4% | <0.0001 | ||||||
All Inpatients | Year | ||||||||
2006 | 2007 | 2008 | 2009 | 2010 | 2011 | 2012 | 2013 | 2014 | |
Age, y (mean) | 57.1 | 57.2 | 56.8 | 57.2 | 57.5 | 58.0 | 58.0 | 57.9 | 58.3 |
% female | 51.6 | 51.2 | 52.4 | 51.7 | 51.1 | 51.5 | 50.3 | 49.9 | 50.1 |
Case‐mix index | 1.86 | 1.98 | 1.96 | 1.99 | 1.96 | 2.02 | 2.03 | 2.07 | 2.23 |
Preintervention (2006) | Postintervention (20082014) | P Value | |||||||
Age, y (mean) | 57.1 | 57.6 | <0.01 | ||||||
% female | 51.6% | 50.9% | 0.07 | ||||||
Case‐mix index | 1.86 | 2.03 | 0.13 | ||||||
Central Line‐Associated Bloodstream Infections per 1,000 Central Line Days | |||||||||
Preintervention | Postintervention | P Value | |||||||
Short term (2006 vs 2008) | 1.8 | 0.60 | 0.004 | ||||||
Long term (2006 vs 20082014) | 1.8 | 0.68 | <0.0001 |
The project granted a Notice of Determination of Approval from the Stanford Administrative Panels for the Protection of Human Subjects (institutional review board).
Methods of Evaluation/Analysis
For the purpose of this analysis, the preintervention period was defined as January 1, 2006 through December 31, 2006, our first year of IAP case review. We defined the intervention period as January 1, 2007 through December 31, 2007, during which we planned and implemented hospital‐wide standardization of CVC insertion practices and incorporated CVC insertion training simulation into resident orientation in July 2007. The postintervention period was defined as January 1, 2008 through December 31, 2014.
All statistical analyses were performed using Stata version 12.1 (StataCorp, College Station, TX). [2] tests were used to determine statistical differences in pre‐ versus postintervention patient demographic data (age, gender, CMI), CVC insertion rates, and CLABSI rates. Because IAP is a rare event, a statistical process control g‐chart was created using QI Macros (KnowWare International, Inc., Denver, CO) to show the number of CVC procedures between IAP. [2] and Fisher exact tests were used to determine statistical differences in CVC anatomic location and use of ultrasound pre‐ and postintervention. A 2‐sided Z test to show a difference in proportions was used to determine statistical differences in CVC‐related IAP rate and all‐cause IAP rate pre‐ and postintervention.
Measuring Adherence to Intervention
Location of CVC Placement and Ultrasound Guidance Pre‐ Versus Postintervention
We utilized the Stanford Clinical Informatics Center (SCCI) services for obtaining counts of patients. Custom queries were performed on SCCI's Stanford Translational Research Integrated Database Environment (STRIDE) platform[16] to search Stanford Hospital electronic heath records for patients. This search primarily involved getting counts for the number of patients with clinical notes that contained the keywords of interest. To identify documentation for placement of CVC from 2006 to 2014, procedure or operative notes containing the words central line or CVC were counted. Further subcounts were obtained by searching for additional keywords such as PICC [peripherally inserted central catheters], femoral, jugular, subclavian, and ultrasound.
Adherence to Intervention in the ICU in 2014
A total of 100 charts were reviewed from patients in our medical and surgical ICU with a CVC in 2014 to evaluate the current trend of central line placement and sustainability of our intervention. Fifty charts were initially randomly selected from the ICU cohort. For those who had multiple lines placed, only the first line was reviewed. Because the initial audit did not provide enough SC lines and we wanted to review more IJ lines, we randomly selected an additional 25 patients who had SC and 25 patients who had IJ to review. The following was collected during chart review: primary team, location of line placement, usage of ultrasound, usage of standard procedure template, supervision, level of training for supervisor, and level of training for staff who performed procedure.
Outcomes
The rate of CVC‐associated IAP was calculated as the total number of IAPs attributed to CVCs divided by the total number of CVCs inserted determined by ICD‐9 coding for 38.93 and 38.97. The total IAP rate was calculated as the total number of IAP/1000 discharges.
RESULTS
Interventions
Our team began the intervention in early 2007 with promotion of ultrasound‐guided IJ catheterization. Clinical exceptions included: (1) trauma or code situations where access to the neck is limited, (2) suspected or confirmed neck injuries, (3) presence of a tracheostomy, and (4) bilateral internal jugular sites unsuitable for catheterization.
Our hospital adopted new formal CVC insertion policies consistent with the above training and education efforts. All physicians were required to document CVC insertions using the template available in the EMR. To be certified to perform CVC insertion independently, trainee physicians were required to complete the simulation training and successfully place a minimum of 5 CVCs directly supervised by an already‐certified physician. This was consistent with the Accreditation Council for Graduate Medical Education suggested minimum requirement in 2007. In our critical care units, all CVC insertions must be supervised by an ICU fellow or attending.
To reinforce the on‐the‐ground work by our physician leaders, we created 2 education tools to embed best practices into our CVC insertion workflow. A checklist with best practices for CVC insertion that was distributed throughout the hospital via central line kits and educational flyers, and a CVC insertion procedure note template consistent with California Department of Public Health documentation requirements was made available in our EMR.
In June 2007, we integrated CVC insertion simulation training into procedure workshops required for all medicine, surgery, anesthesia, and emergency medicine trainees during their intern year. These workshops promoted ultrasound‐guided IJ catheterization and supporting evidence for the new IJ site preference. Training sessions were 2 to 3 hours, and included a demonstration of best‐practice CVC insertion, as well as training with simulation models supervised by an instructor using a standardized CVC checklist. These trainings used both the Blue Phantom human torso model (
Hospital administration provided funds to purchase ultrasound machines for patient units such as medicine, cardiology, ED, and ICU). A total of 4 Site‐Rite (Bard Access Systems, Inc., Salt Lake City, UT) ultrasounds were purchased in 2007. The hospital has continued to purchase ultrasound units yearly, and had 53 ultrasound units in 2014
Cases of IAP were continuously reviewed throughout the intervention period. Based on their higher CVC‐associated IAP rates, the ORs and catheterization lab were identified as having opportunities for improvement. In 2008, Hospital quality‐improvement leadership met with physician leaders in these areas to review their CVC‐related IAP data and to discuss strategies to reduce their IAP rates. These strategies included lessons learned from other services that had successfully decreased their IAP rates.
To sustain our gains, we continue to review all IAP through our coding quality, clinical documentation, quality reporting departments, and peer review. We have implemented other strategies to decrease IAP, such as the use of ultrasound guidance for bedside thoracentesis procedures, which became possible after the availability of more ultrasound devices. Training for ultrasound‐guided thoracentesis was done by our procedure‐team attending during supervision of residents.
Outcomes
Preintervention (January 1, 2006 to December 31, 2006)
There were a total of 26 cases of IAP in 2006. Of these, 15 (58%) were associated with CVC insertion (Figure 1). The single procedure associated with the largest proportion of IAP was SC CVC insertion (11 cases, 42% of all IAP cases). Eleven CVC‐associated IAPs were significant enough to require chest tube placement. Our hospital recorded a total of 1593 CVC insertions (ICD‐9 codes 38.93 and 38.97) in 2006.
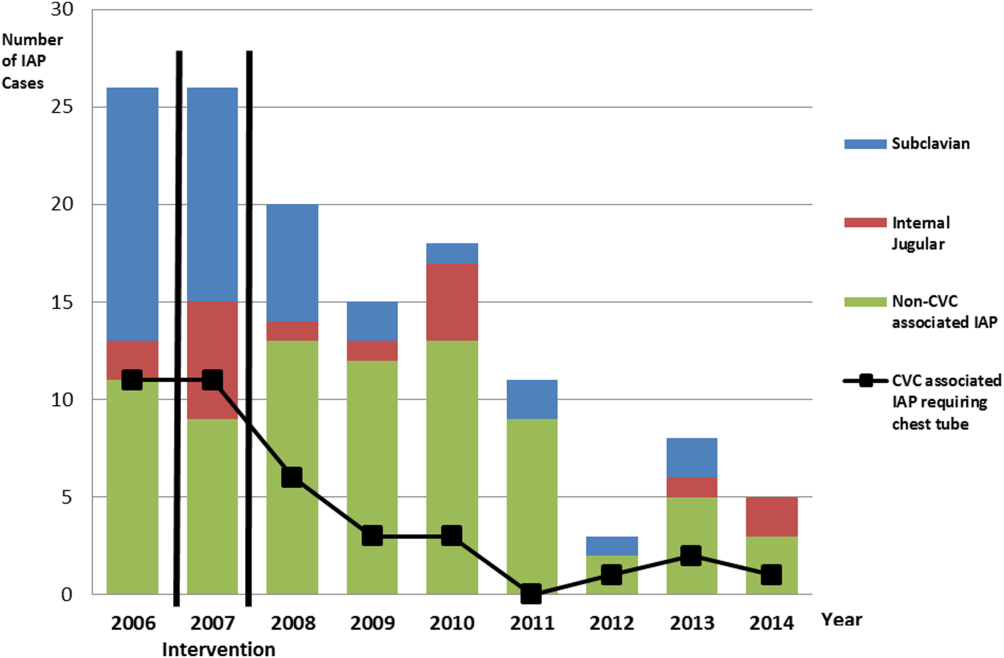
Postintervention (January 1, 2008 to December 31, 2014)
There were a total of 80 cases of IAP over 7 years, of which 24 (30%) were associated with CVC insertion. Of these, 16 required chest tube placement. In the last 4 years of the postintervention period (20112014), there were only 5 cases of CVC‐associated IAP requiring chest tube placement (Figure 1). There were a total of 12,000 CVC insertions recorded over the same period.
We successfully met both our short‐ and long‐term goals. Our preintervention CVC‐associated IAP rate was 0.94%, and our post‐intervention rate during 2008 was 0.44%, a short‐term reduction of 53% (P=0.088). Our average postintervention CVC‐associated IAP rate for the years 2008 through 2014 was 0.13%, a significant long‐term reduction of 86% (P<0.0001) (Table 2). The decrease in CVC‐associated IAP rates occurred despite an older patient population (P<0.001) and a higher CMI (P<0.001) in postintervention patients who received a CVC (Table 1). Special cause variation corresponding to a change in our process is demonstrated in Figure 2. The preintervention average number of procedures between IAP was 114.8 and increased to 460.7 in the postintervention period.
Total CVC (n=95) | Subclavian (n=29) | Internal Jugular (n=58) | Femoral (n=8) | ||||||||||
---|---|---|---|---|---|---|---|---|---|---|---|---|---|
| |||||||||||||
Compliance to intervention | |||||||||||||
US guided | 68.1% | 20.7% | 86.2% | 100.0% | |||||||||
Procedure note completion | 90.4% | 93.1% | 86.2% | 100.0% | |||||||||
Supervision | 70.2% | 77.8% | 73.1% | 87.5% | |||||||||
Level of training | |||||||||||||
Resident | 61.1% | 58.6% | 60.3% | 75.0% | |||||||||
Fellow | 25.3% | 27.6% | 24.1% | 25.0% | |||||||||
Attending | 4.2% | 6.9% | 3.4% | 0.0% | |||||||||
Advance practitioner | 3.2% | 3.4% | 3.4% | 0.0% | |||||||||
Unknown | 6.3% | 3.4% | 8.6% | 0.0% | |||||||||
Supervisor type | |||||||||||||
Resident | 3.0% | 4.8% | 2.6% | 0.0% | |||||||||
Fellow | 54.5% | 33.3% | 57.9% | 100.0% | |||||||||
Attending | 42.4% | 61.9% | 39.5% | 0.0% | |||||||||
Location of CVC Placement | Internal Jugular (n=25) | Subclavian (n=25) | |||||||||||
MICU | 32.0% | 32.0% | |||||||||||
SICU* | 40.0% | 52.0% | |||||||||||
Operating room | 28.0% | 16.0% | |||||||||||
Average no. of attempts/procedure | 1.4 | 1.5 | |||||||||||
Indications for subclavian insertion (n=25) | |||||||||||||
Trauma/surgical site | 60.0% | ||||||||||||
Need for additional access | 16.0% | ||||||||||||
Unsuccessful IJ placement | 4.0% | ||||||||||||
Unclear | 20.0% | ||||||||||||
Iatrogenic Pneumothorax Rate (20062014) | Year | ||||||||||||
2006 | 2007 | 2008 | 2009 | 2010 | 2011 | 2012 | 2013 | 2014 | |||||
% of CVC insertions associated with IAP | 0.94 | 1.49 | 0.44 | 0.13 | 0.20 | 0.07 | 0.04 | 0.11 | 0.07 | ||||
All‐cause IAP per 1,000 discharges | 1.32 | 1.29 | 0.98 | 0.71 | 0.83 | 0.49 | 0.13 | 0.35 | 0.23 | ||||
Preintervention | Postintervention | P Value | |||||||||||
CVC‐ associated IAP short term (2006 vs 2008) | 0.94% | 0.44% | 0.088 | ||||||||||
CVC‐associated IAP long term (2006 vs 20082014) | 0.94% | 0.13% | <0.0001 | ||||||||||
All‐cause IAP per 1,000 discharges short term (2006 vs 2008) | 1.32 | 0.98 | <0.0001 | ||||||||||
All‐cause IAP per 1,000 discharges long term (2006 vs 2008‐14) | 1.32 | 0.52 | <0.0001 |
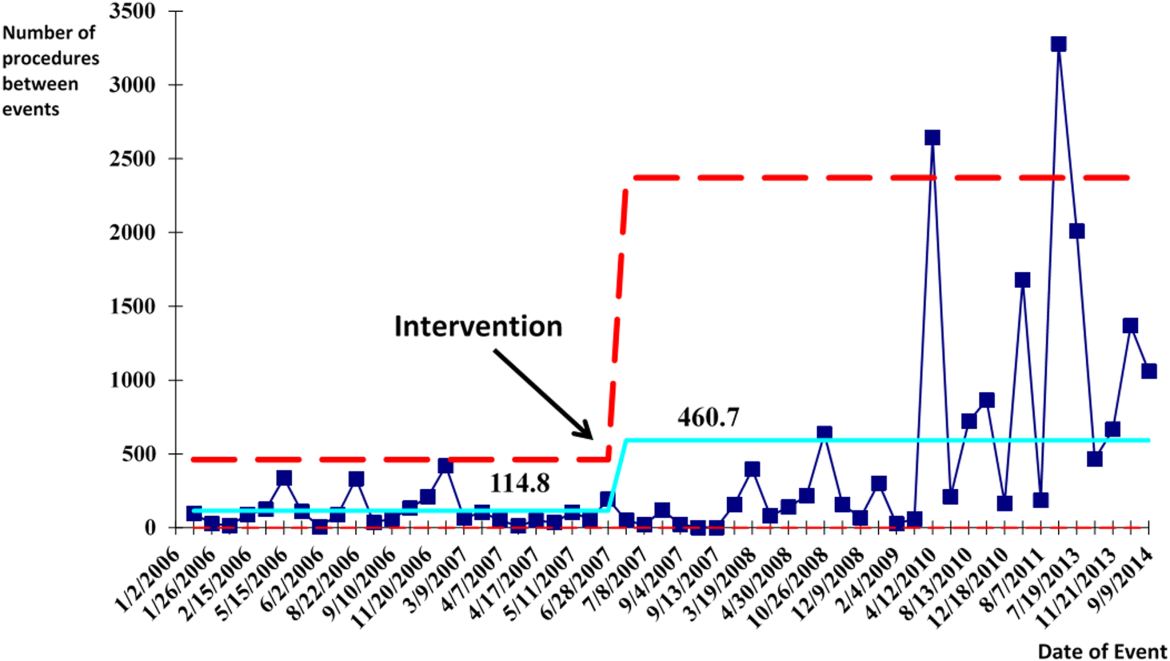
With the decrease in CVC‐associated IAP, we also saw a decrease in our all‐cause IAP rate per 1000 discharges from 1.32 in 2006 to 0.98 in 2008. This represents a 26% short‐term reduction (P<0.0001). We also saw a decrease in our all‐cause IAP rate per 1000 discharges to 0.52 from 2008 to 2014, representing a 61% long‐term reduction (P<0.0001). This decrease in all‐cause IAP postintervention occurred despite an older patient population (P<0.01) for all discharges. Our hospital is now in the highest performance UHC quartile for all‐cause IAP in 2012 to 2014.
After our multifaceted intervention in 2007, there was substantially more and consistent documentation of CVC procedure notes from less than 500 in 2006 to greater than 2000 in 2009. The distribution of CVC procedure notes in the pre‐ (2006) versus postintervention (20082014) period showed a decrease in the proportion of femoral lines from 15% to 11%, increase in IJ lines from 31% to 49%, and a decrease in SC from 54% to 40% (P=0.001). The distribution of IJ CVC procedure notes in the pre‐ (2006) versus postintervention (20082014) period showed an increase in the proportion of procedures with ultrasound documentation from 13% to 93% (P<0.001) (Figure 3).
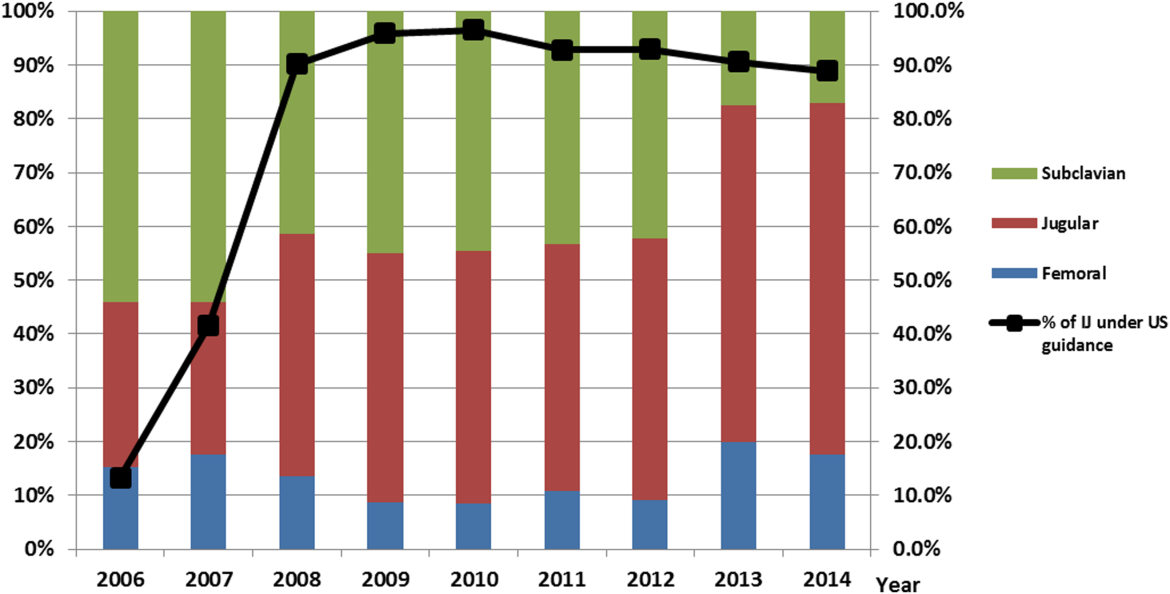
In our ICU 2014 audit, the majority of CVC lines were placed by residents under supervision (>70%), and most used the standard CVC note template to document the procedure (90%). Of the total CVC approach, 66% were IJ and 4% were SC. Eighty‐six percent used ultrasound during IJ placement. The majority of SC insertions were placed in the surgical ICU and had clear indications (80%) for placement. Of those, 75% were due to trauma (limited access to neck) or surgery (interfering with surgical site) (Table 2).
DISCUSSION
Summary
This quality‐improvement intervention demonstrates that a multidisciplinary team can successfully implement a multifaceted intervention that sustainably reduces the rate of IAP complications from CVC placement and improves patient safety over 7 years. We found high compliance with our intervention, which included an increase in CVC notes and documentation of ultrasound guidance. There was also an increase in the IJ approach in our postintervention period. We showed statistically significant long‐term reductions in both CVC‐associated and all‐cause IAP rates. From 2011 to 2014, there were only 5 cases of CVC‐associated IAP requiring chest tube placement. Post hoc analysis showed a statistically significant decrease in CLABSI rates (P<0.0001) from a preintervention rate of 1.6 infections per 1000 central line days to postintervention average rate of 0.68 infections per 1000 central line days. This decrease may be related to the incorporation of wide sterile barrier techniques in our CVC training workshops, checklists, and template procedure notes.
A strength of this study is the sustained significant long‐term reduction in IAP. There are few data that exist to describe sustained interventions in this area. Sustainability was achieved by integrating our interventions into ongoing programs that already existed in the hospital; we incorporated our simulation training into the existing new resident orientation, increased the availability of existing ultrasound equipment, and continued our IAP chart review through coding quality with feedback to involved services. The procedure note template continues to be easily available in our EMR, and the SC approach to CVC placement is limited to select cases.
Based on a post hoc cost‐benefit analysis, the financial benefits of decreasing the rate of IAP outweigh the costs associated with implementation of this initiative. The purchase cost for a Site‐Rite (Bard Access Systems) ultrasound machine was $18,000. The cost of materials for 1 workshop is $5000 annually. Cases from the Nationwide Inpatient Sample that were flagged by this PSI had 7.0% excess mortality, 4.4 days of excess hospitalization, and approximately $18,000 in excess hospital charges.[17, 18] Based on these data, if we had continued at our preintervention rate of CVC‐associated IAP requiring chest tube placement, we would estimate 9 additional CVC‐associated IAPs requiring chest tube insertion per year. This would result in over $180,000 of additional costs annually. Based on an initial cost of $100,000 for 4 workshops and the necessary equipment, we would have realized our cost savings in less than 1 year postintervention. These are all approximate costs, and further detailed analysis is needed.
One challenge with this intervention is the culture change away from using the SC approach, and the concern from trainees of how they would learn to perform SC CVC if needed. We would suggest dedicated SC CVC ultrasound training for those services who may need to use this approach (eg, neuroanesthesia and trauma).
Interpretation/Relation to Other Evidence
The field of implementation science can help explain why some projects are successful and others fail. We can further dissect the success of this project using an implementation science model similar to that described by French et al.[19] French et al. describe 4 behavior‐change techniques. These steps include (1) who needs to do what differently, (2) which barriers and enablers need to be addressed, (3) which intervention component could overcome the barriers and enhance enablers, and (4) how can behavior change be measured and understood. Barriers included suboptimal skills of residents, low awareness of evidence‐based guidelines, and entrenched practices inconsistent with best evidence. There was also a belief that IJ lines were more likely to become infected. Targeted behaviors needing to be done differently were the choice of CVC placement site and insertion technique. Barriers to change were assessed by asking members of the project team to explore with members of their service what led them to do CVC lines without ultrasound guidance. Enhancements focused on information provision, simulation practice, and persuasive communication. Behavior change was measured by tracking the number of IAPs, site of CVC, and documentation of technique. Continuation of these interventions based on this theoretical framework drove maintenance of gains.
We completed our main intervention planning in 90 days, and met our short‐term goal on schedule. The Institute for Healthcare Improvement (IHI) advocates that such short timelines are efficient mechanisms for developing and acting on projects. Other institutions have reported on similar rapid‐cycle planning and short‐term goal setting[20]
Limitations
Our study captures the experience of a quality‐improvement team at a single academic center, and our results may not be generalizable to other institutions. Our chart review process only occurred once a case had been identified through AHRQ PSI methodology. It is possible that the PSI does not capture all cases of IAP, although we believe our coding department has a very rigorous process to look for all IAP evidence in the patient's record. We used administrative data to determine the number of hospital‐wide CVC procedures.
Our compliance data with interventions from STRIDE are based on looking for key words in procedure note documentation (so undocumented notes are not captured). To validate this, we performed a manual audit of our adherence to our intervention in 2014, and those data are consistent with the results from our STRIDE data.
Our study's observational design also cannot control for exogenous effects on physician practice relating to CVC insertion or the overall risk of IAP. Some of our decrease in complications may be from the increase in PICC line use. Nevertheless, our CVC‐associated IAP rate has decreased despite >6000 non‐PICC CVCs in our ICU over the past 5 years, and a rising CMI (18% increase in postintervention period) and older population of patients with CVC insertion (P<0.0001)
CONCLUSIONS
We are the first, to our knowledge, to report a measurable improvement in reducing IAP patient outcomes that has been sustained for over 7 years. Our hospital is in the highest performance UHC quartile for all‐cause IAP in 2012 to 2014. A multidisciplinary quality‐improvement team, focused on evidence, patient safety, and standardization, can use a multifaceted intervention to sustainably improve patient outcomes. Promoting ultrasound‐guided IJ catheterization as the CVC insertion method of choice significantly reduced our hospital's rate of CVC‐associated IAP.
Acknowledgements
The authors acknowledge many who have contributed to this quality‐improvement project:
Irina Tokareva, Jay Lee, Kourt Bowes, and Gomathi Krishnan for data analysis; Laura Meinke for significant website curriculum; Fred Mihm, Sarah Williams, and John Kugler for leadership in ultrasound training; Kevin Tabb and Norm Rizk for hospital financial support of simulation workshops and ultrasound machines; Pooja Loftus and Helene Grossman for statistical analysis; Eric Hadhazy for data support; Joan Hendershott for cost information; Nancy Szaflarski for project management and manuscript review; and Isabella Chu for manuscript review.
Disclosures: STRIDE (Stanford Translational Research Integrated Database Environment) is a research and development project at Stanford University to create a standards‐based informatics platform supporting clinical and translational research. This STRIDE project was supported by the National Center for Research Resources and the National Center for Advancing Translational Sciences, National Institutes of Health, through grant UL1 RR025744. The content is solely the responsibility of the authors and does not necessarily represent the official views of the NIH. The authors report no conflicts of interest.
Iatrogenic pneumothorax (IAP) is a complication of invasive procedures that is associated with substantial morbidity and some mortality.[1] IAP is often avoidable, and in many cases can be prevented through adherence to evidence‐based guidelines and procedural techniques known to reduce the incidence of IAP.[2] IAP may occur with a subclavian (SC) or internal jugular (IJ) central venous catheter (CVC) insertion, but is more frequently associated with the SC approach.[3] Ultrasound guidance during IJ CVC insertion is associated with a lower risk as compared to guidance by anatomical landmarks.[4, 5] Other bedside procedures that are known to cause IAP include thoracentesis. This risk can also be reduced with the use of ultrasound guidance.[6]
Including simulation in training for CVC insertion has been demonstrated in meta‐analyses to improve both learner outcomes, including simulator performance and perceived confidence, and patient outcomes, including fewer failed CVC attempts and reduced incidence of IAP.[7] Even brief simulation workshops lasting less than two hours can improve patient safety during CVC insertion.[8]
The implementation of ultrasound‐based simulation and improved adherence to the actual use of ultrasound at the bedside can be motivated by tying competency‐based educational objectives (eg, CVC insertion) to clinical outcomes (ie, rates of IAP) and tracking both as part of a continuous quality‐improvement cycle.[9] Adherence to best practices for CVC insertion can also be improved through standardizing hospital‐wide policies and hands‐on training.[10] Involving many stakeholders, including nurses, physicians, nurse practioners and physician assistants, in a multidisciplinary team has been shown to help alter entrenched behaviors and reduce the incidence of central‐line associated bloodstream infections through long‐term adherence to evidence‐based interventions.[11]
LOCAL PROBLEM
The Agency for Healthcare Research and Quality (AHRQ) has designed Patient Safety Indicators (PSIs) (
Our hospital is a member of the University HealthSystem Consortium (UHC) (
Despite this, the PSI can highlight areas where quality‐improvement efforts might be best directed. In 2005 and 2006, our hospital was ranked within the lowest UHC performance quartile for all‐cause IAP PSI.
During FY 2006 (September 2005August 2006), root‐cause analysis on cases of IAP at our hospital found that CVC insertion (40%) was the most common procedure associated with IAP, with SC insertion causing 69% of CVC‐associated IAP. Other common procedures associated with IAP were operative/pacemaker (30%), thoracentesis (25%), and ventilator associated (5%). Ultrasound was not used in 2/5 cases of IJ CVC placement and 3/5 thoracentesis cases. Only 44% of CVC insertions had a procedure note.
Intended Improvement/Study Question
Our team set out to plan and implement a set of multifaceted interventions within 90 days. The short‐term goal was a 50% reduction in the CVC IAP and all‐cause IAP rate within 18 months, and the long‐term goal was sustained reduction of CVC IAP and all‐cause IAP rate.
METHODS
The format of this article is based on the standards for quality‐improvement reporting excellence guidelines for the reporting of studies on the effectiveness of quality‐improvement interventions.[14]
Setting
Stanford University Medical Center is an academic medical center with 465 beds and over 25,000 inpatient admissions per year, providing both general acute care services and tertiary medical care. Residents perform CVC bedside procedures when central venous access is needed, in the intensive care unit (ICU), operating room (OR), and inpatient units. Prior to this project, ultrasound equipment was only available in the emergency department (ED) and ICUs. There was no formal CVC procedure supervision policy, CVC training curriculum, and procedure note templates for documentation of CVC insertion.
Planning the Interventions
A multidisciplinary quality‐improvement team met weekly during the 90‐day design period from January 2007 to March 2007. Our team included representatives from the departments of medicine, anesthesia and critical care, surgery, nursing, and emergency medicine. We also partnered with our institution's clinical and administrative leaders, experts in simulation, and the hospital quality department.
We hypothesized that a standardized set of education and training interventions promoting ultrasound‐guided IJ CVC insertion as the method of choice at our hospital would significantly reduce our rate of CVC‐associated IAP. Our multifaceted intervention included: (1) clinical and documentation standards based on evidence, (2) cognitive aids, (3) simulation training, (4) purchase and deployment of ultrasound equipment, and (5) feedback to clinical services.
Our team followed the define, measure, analyze, improve, control (DMAIC) framework.[15] We set interval goals with target completion dates throughout the 90‐day period, identified owners of each goal, and tracked progress with a shared spreadsheet.
In the 90‐day intervention, we accomplished the following: (1) conducted root‐cause analysis of IAP cases for fiscal year 2006, (2) created clinical and documentation standards around CVC placement, (3) created cognitive aids and procedure note templates, (4) developed simulation training courses, and (5) requested purchase of additional ultrasound equipment.
Data Collection
To evaluate our progress in reducing the rates of IAP, we tracked the incidence of IAP using UHC and AHRQ PSI methodology. In collaboration with our hospital's quality department, we manually reviewed every PSI‐identified case of IAP. This review has focused on identifying whether or not pneumothorax actually occurred, and whether it was associated with CVC insertion. For those associated with CVC, data were collected for patient location and service, the procedure site, whether ultrasound was used, whether a chest tube was required, and the final disposition of the patient.
Demographic data (age, gender, case mix index [CMI]) shown in Table 1 were obtained through MIDAS+ Solutions (Tucson, Arizona), a proprietary database that contains healthcare management coded data. Total hospital CVC insertion rates were calculated using International Classification of Diseases, Ninth Revision (ICD‐9) coding for 38.93 and 38.97. ICU central lineassociated blood stream infections (CLABSI) data were obtained from internal collection by our infection control team. Number and location of CVCs placed in the ICU data were obtained from nursing flow sheets in our electronic medical record (EMR). Cost information was provided by our finance department using internal accounting.
Patients With CVC Insertion | Year | ||||||||
---|---|---|---|---|---|---|---|---|---|
2006 | 2007 | 2008 | 2009 | 2010 | 2011 | 2012 | 2013 | 2014 | |
| |||||||||
Age, y (mean) | 55.0 | 55.5 | 55.0 | 57.0 | 56.5 | 58.5 | 57.5 | 59.0 | 58.5 |
% female | 47.0 | 49.5 | 47.0 | 48.8 | 46.2 | 46.1 | 45.7 | 46.2 | 45.7 |
Case‐mix index | 3.08 | 3.35 | 3.21 | 3.40 | 3.71 | 3.91 | 3.92 | 3.92 | 4.08 |
Total no. of CVCs/year* | 1,593 | 1,141 | 1,589 | 2,250 | 2,441 | 2,774 | 2,754 | 2,722 | 2,845 |
No. of CVCs/year in ICU | NA | NA | NA | 1,502 | 1,357 | 1,345 | 1,316 | 1,421 | 1,590 |
No. of subclavians/year in ICU | NA | NA | NA | 167 | 75 | 70 | 83 | 75 | 97 |
No. of IJs/year in ICU | NA | NA | NA | 898 | 773 | 681 | 677 | 713 | 876 |
No. of femorals/year in ICU | NA | NA | NA | 212 | 152 | 203 | 171 | 198 | 206 |
No. of PICCs/year in ICU | NA | NA | NA | 225 | 357 | 391 | 385 | 435 | 411 |
Preintervention (2006) | Postintervention (20082014) | P Value | |||||||
Age, y (mean) | 55.2 | 58.7 | <0.0001 | ||||||
% female | 47.0% | 46.4% | 0.642 | ||||||
Case‐mix index | 3.08 | 3.73 | <0.0001 | ||||||
CVC insertion rate | 8.1% | 11.4% | <0.0001 | ||||||
All Inpatients | Year | ||||||||
2006 | 2007 | 2008 | 2009 | 2010 | 2011 | 2012 | 2013 | 2014 | |
Age, y (mean) | 57.1 | 57.2 | 56.8 | 57.2 | 57.5 | 58.0 | 58.0 | 57.9 | 58.3 |
% female | 51.6 | 51.2 | 52.4 | 51.7 | 51.1 | 51.5 | 50.3 | 49.9 | 50.1 |
Case‐mix index | 1.86 | 1.98 | 1.96 | 1.99 | 1.96 | 2.02 | 2.03 | 2.07 | 2.23 |
Preintervention (2006) | Postintervention (20082014) | P Value | |||||||
Age, y (mean) | 57.1 | 57.6 | <0.01 | ||||||
% female | 51.6% | 50.9% | 0.07 | ||||||
Case‐mix index | 1.86 | 2.03 | 0.13 | ||||||
Central Line‐Associated Bloodstream Infections per 1,000 Central Line Days | |||||||||
Preintervention | Postintervention | P Value | |||||||
Short term (2006 vs 2008) | 1.8 | 0.60 | 0.004 | ||||||
Long term (2006 vs 20082014) | 1.8 | 0.68 | <0.0001 |
The project granted a Notice of Determination of Approval from the Stanford Administrative Panels for the Protection of Human Subjects (institutional review board).
Methods of Evaluation/Analysis
For the purpose of this analysis, the preintervention period was defined as January 1, 2006 through December 31, 2006, our first year of IAP case review. We defined the intervention period as January 1, 2007 through December 31, 2007, during which we planned and implemented hospital‐wide standardization of CVC insertion practices and incorporated CVC insertion training simulation into resident orientation in July 2007. The postintervention period was defined as January 1, 2008 through December 31, 2014.
All statistical analyses were performed using Stata version 12.1 (StataCorp, College Station, TX). [2] tests were used to determine statistical differences in pre‐ versus postintervention patient demographic data (age, gender, CMI), CVC insertion rates, and CLABSI rates. Because IAP is a rare event, a statistical process control g‐chart was created using QI Macros (KnowWare International, Inc., Denver, CO) to show the number of CVC procedures between IAP. [2] and Fisher exact tests were used to determine statistical differences in CVC anatomic location and use of ultrasound pre‐ and postintervention. A 2‐sided Z test to show a difference in proportions was used to determine statistical differences in CVC‐related IAP rate and all‐cause IAP rate pre‐ and postintervention.
Measuring Adherence to Intervention
Location of CVC Placement and Ultrasound Guidance Pre‐ Versus Postintervention
We utilized the Stanford Clinical Informatics Center (SCCI) services for obtaining counts of patients. Custom queries were performed on SCCI's Stanford Translational Research Integrated Database Environment (STRIDE) platform[16] to search Stanford Hospital electronic heath records for patients. This search primarily involved getting counts for the number of patients with clinical notes that contained the keywords of interest. To identify documentation for placement of CVC from 2006 to 2014, procedure or operative notes containing the words central line or CVC were counted. Further subcounts were obtained by searching for additional keywords such as PICC [peripherally inserted central catheters], femoral, jugular, subclavian, and ultrasound.
Adherence to Intervention in the ICU in 2014
A total of 100 charts were reviewed from patients in our medical and surgical ICU with a CVC in 2014 to evaluate the current trend of central line placement and sustainability of our intervention. Fifty charts were initially randomly selected from the ICU cohort. For those who had multiple lines placed, only the first line was reviewed. Because the initial audit did not provide enough SC lines and we wanted to review more IJ lines, we randomly selected an additional 25 patients who had SC and 25 patients who had IJ to review. The following was collected during chart review: primary team, location of line placement, usage of ultrasound, usage of standard procedure template, supervision, level of training for supervisor, and level of training for staff who performed procedure.
Outcomes
The rate of CVC‐associated IAP was calculated as the total number of IAPs attributed to CVCs divided by the total number of CVCs inserted determined by ICD‐9 coding for 38.93 and 38.97. The total IAP rate was calculated as the total number of IAP/1000 discharges.
RESULTS
Interventions
Our team began the intervention in early 2007 with promotion of ultrasound‐guided IJ catheterization. Clinical exceptions included: (1) trauma or code situations where access to the neck is limited, (2) suspected or confirmed neck injuries, (3) presence of a tracheostomy, and (4) bilateral internal jugular sites unsuitable for catheterization.
Our hospital adopted new formal CVC insertion policies consistent with the above training and education efforts. All physicians were required to document CVC insertions using the template available in the EMR. To be certified to perform CVC insertion independently, trainee physicians were required to complete the simulation training and successfully place a minimum of 5 CVCs directly supervised by an already‐certified physician. This was consistent with the Accreditation Council for Graduate Medical Education suggested minimum requirement in 2007. In our critical care units, all CVC insertions must be supervised by an ICU fellow or attending.
To reinforce the on‐the‐ground work by our physician leaders, we created 2 education tools to embed best practices into our CVC insertion workflow. A checklist with best practices for CVC insertion that was distributed throughout the hospital via central line kits and educational flyers, and a CVC insertion procedure note template consistent with California Department of Public Health documentation requirements was made available in our EMR.
In June 2007, we integrated CVC insertion simulation training into procedure workshops required for all medicine, surgery, anesthesia, and emergency medicine trainees during their intern year. These workshops promoted ultrasound‐guided IJ catheterization and supporting evidence for the new IJ site preference. Training sessions were 2 to 3 hours, and included a demonstration of best‐practice CVC insertion, as well as training with simulation models supervised by an instructor using a standardized CVC checklist. These trainings used both the Blue Phantom human torso model (
Hospital administration provided funds to purchase ultrasound machines for patient units such as medicine, cardiology, ED, and ICU). A total of 4 Site‐Rite (Bard Access Systems, Inc., Salt Lake City, UT) ultrasounds were purchased in 2007. The hospital has continued to purchase ultrasound units yearly, and had 53 ultrasound units in 2014
Cases of IAP were continuously reviewed throughout the intervention period. Based on their higher CVC‐associated IAP rates, the ORs and catheterization lab were identified as having opportunities for improvement. In 2008, Hospital quality‐improvement leadership met with physician leaders in these areas to review their CVC‐related IAP data and to discuss strategies to reduce their IAP rates. These strategies included lessons learned from other services that had successfully decreased their IAP rates.
To sustain our gains, we continue to review all IAP through our coding quality, clinical documentation, quality reporting departments, and peer review. We have implemented other strategies to decrease IAP, such as the use of ultrasound guidance for bedside thoracentesis procedures, which became possible after the availability of more ultrasound devices. Training for ultrasound‐guided thoracentesis was done by our procedure‐team attending during supervision of residents.
Outcomes
Preintervention (January 1, 2006 to December 31, 2006)
There were a total of 26 cases of IAP in 2006. Of these, 15 (58%) were associated with CVC insertion (Figure 1). The single procedure associated with the largest proportion of IAP was SC CVC insertion (11 cases, 42% of all IAP cases). Eleven CVC‐associated IAPs were significant enough to require chest tube placement. Our hospital recorded a total of 1593 CVC insertions (ICD‐9 codes 38.93 and 38.97) in 2006.
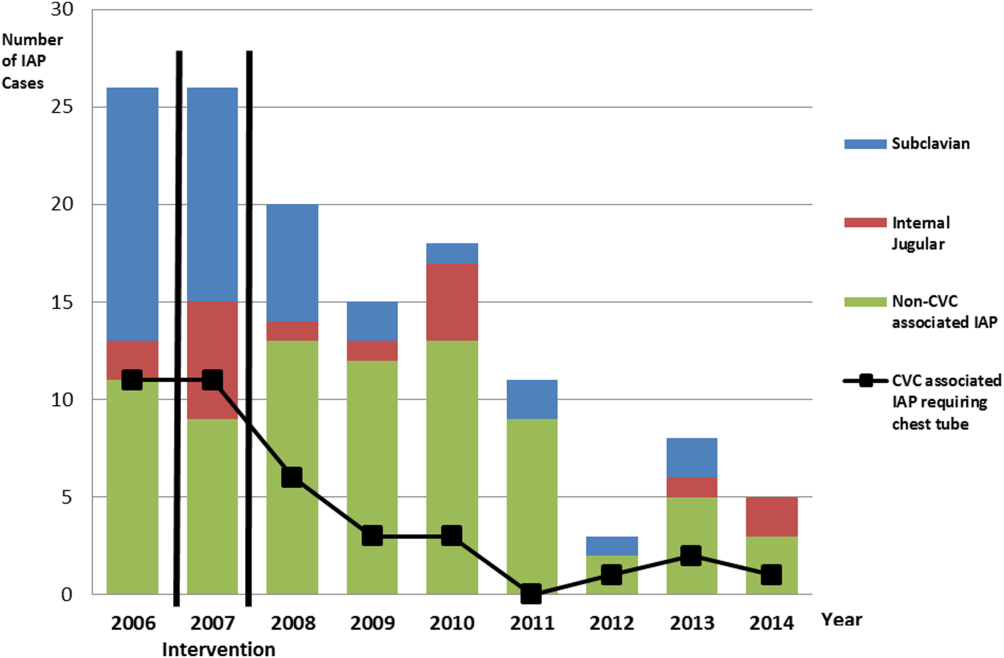
Postintervention (January 1, 2008 to December 31, 2014)
There were a total of 80 cases of IAP over 7 years, of which 24 (30%) were associated with CVC insertion. Of these, 16 required chest tube placement. In the last 4 years of the postintervention period (20112014), there were only 5 cases of CVC‐associated IAP requiring chest tube placement (Figure 1). There were a total of 12,000 CVC insertions recorded over the same period.
We successfully met both our short‐ and long‐term goals. Our preintervention CVC‐associated IAP rate was 0.94%, and our post‐intervention rate during 2008 was 0.44%, a short‐term reduction of 53% (P=0.088). Our average postintervention CVC‐associated IAP rate for the years 2008 through 2014 was 0.13%, a significant long‐term reduction of 86% (P<0.0001) (Table 2). The decrease in CVC‐associated IAP rates occurred despite an older patient population (P<0.001) and a higher CMI (P<0.001) in postintervention patients who received a CVC (Table 1). Special cause variation corresponding to a change in our process is demonstrated in Figure 2. The preintervention average number of procedures between IAP was 114.8 and increased to 460.7 in the postintervention period.
Total CVC (n=95) | Subclavian (n=29) | Internal Jugular (n=58) | Femoral (n=8) | ||||||||||
---|---|---|---|---|---|---|---|---|---|---|---|---|---|
| |||||||||||||
Compliance to intervention | |||||||||||||
US guided | 68.1% | 20.7% | 86.2% | 100.0% | |||||||||
Procedure note completion | 90.4% | 93.1% | 86.2% | 100.0% | |||||||||
Supervision | 70.2% | 77.8% | 73.1% | 87.5% | |||||||||
Level of training | |||||||||||||
Resident | 61.1% | 58.6% | 60.3% | 75.0% | |||||||||
Fellow | 25.3% | 27.6% | 24.1% | 25.0% | |||||||||
Attending | 4.2% | 6.9% | 3.4% | 0.0% | |||||||||
Advance practitioner | 3.2% | 3.4% | 3.4% | 0.0% | |||||||||
Unknown | 6.3% | 3.4% | 8.6% | 0.0% | |||||||||
Supervisor type | |||||||||||||
Resident | 3.0% | 4.8% | 2.6% | 0.0% | |||||||||
Fellow | 54.5% | 33.3% | 57.9% | 100.0% | |||||||||
Attending | 42.4% | 61.9% | 39.5% | 0.0% | |||||||||
Location of CVC Placement | Internal Jugular (n=25) | Subclavian (n=25) | |||||||||||
MICU | 32.0% | 32.0% | |||||||||||
SICU* | 40.0% | 52.0% | |||||||||||
Operating room | 28.0% | 16.0% | |||||||||||
Average no. of attempts/procedure | 1.4 | 1.5 | |||||||||||
Indications for subclavian insertion (n=25) | |||||||||||||
Trauma/surgical site | 60.0% | ||||||||||||
Need for additional access | 16.0% | ||||||||||||
Unsuccessful IJ placement | 4.0% | ||||||||||||
Unclear | 20.0% | ||||||||||||
Iatrogenic Pneumothorax Rate (20062014) | Year | ||||||||||||
2006 | 2007 | 2008 | 2009 | 2010 | 2011 | 2012 | 2013 | 2014 | |||||
% of CVC insertions associated with IAP | 0.94 | 1.49 | 0.44 | 0.13 | 0.20 | 0.07 | 0.04 | 0.11 | 0.07 | ||||
All‐cause IAP per 1,000 discharges | 1.32 | 1.29 | 0.98 | 0.71 | 0.83 | 0.49 | 0.13 | 0.35 | 0.23 | ||||
Preintervention | Postintervention | P Value | |||||||||||
CVC‐ associated IAP short term (2006 vs 2008) | 0.94% | 0.44% | 0.088 | ||||||||||
CVC‐associated IAP long term (2006 vs 20082014) | 0.94% | 0.13% | <0.0001 | ||||||||||
All‐cause IAP per 1,000 discharges short term (2006 vs 2008) | 1.32 | 0.98 | <0.0001 | ||||||||||
All‐cause IAP per 1,000 discharges long term (2006 vs 2008‐14) | 1.32 | 0.52 | <0.0001 |
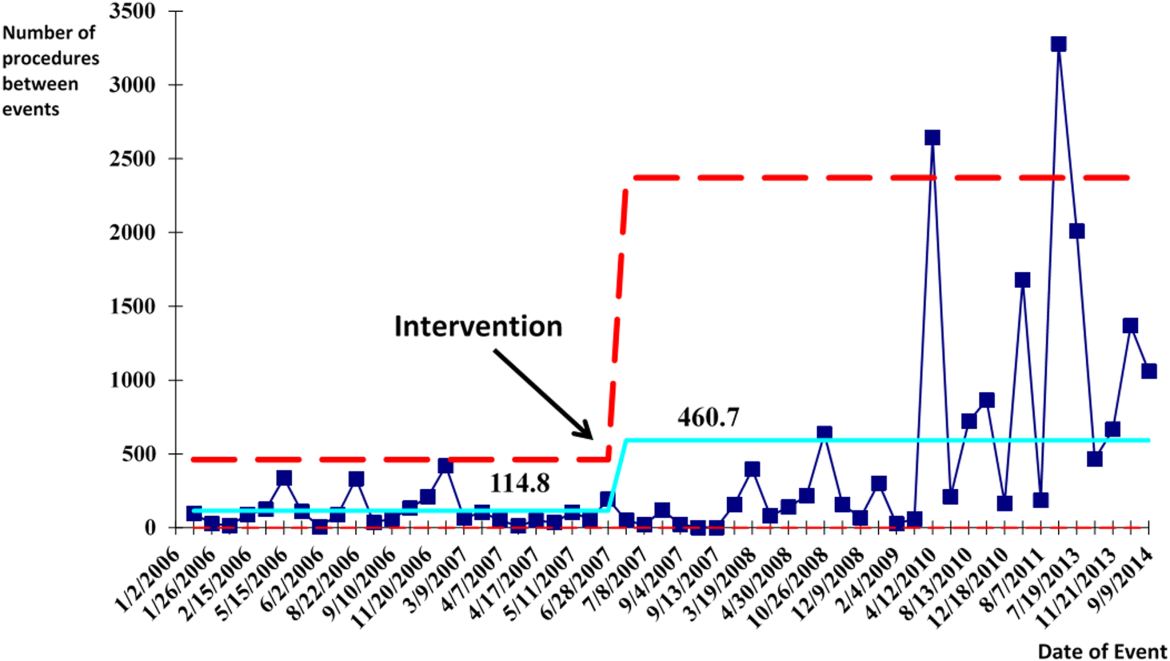
With the decrease in CVC‐associated IAP, we also saw a decrease in our all‐cause IAP rate per 1000 discharges from 1.32 in 2006 to 0.98 in 2008. This represents a 26% short‐term reduction (P<0.0001). We also saw a decrease in our all‐cause IAP rate per 1000 discharges to 0.52 from 2008 to 2014, representing a 61% long‐term reduction (P<0.0001). This decrease in all‐cause IAP postintervention occurred despite an older patient population (P<0.01) for all discharges. Our hospital is now in the highest performance UHC quartile for all‐cause IAP in 2012 to 2014.
After our multifaceted intervention in 2007, there was substantially more and consistent documentation of CVC procedure notes from less than 500 in 2006 to greater than 2000 in 2009. The distribution of CVC procedure notes in the pre‐ (2006) versus postintervention (20082014) period showed a decrease in the proportion of femoral lines from 15% to 11%, increase in IJ lines from 31% to 49%, and a decrease in SC from 54% to 40% (P=0.001). The distribution of IJ CVC procedure notes in the pre‐ (2006) versus postintervention (20082014) period showed an increase in the proportion of procedures with ultrasound documentation from 13% to 93% (P<0.001) (Figure 3).
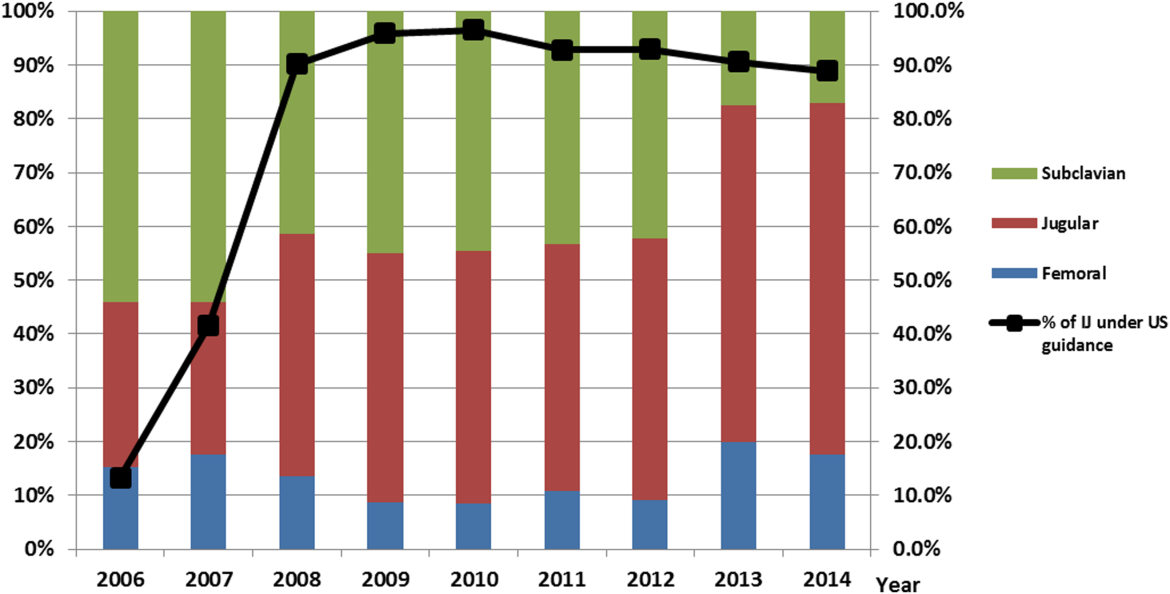
In our ICU 2014 audit, the majority of CVC lines were placed by residents under supervision (>70%), and most used the standard CVC note template to document the procedure (90%). Of the total CVC approach, 66% were IJ and 4% were SC. Eighty‐six percent used ultrasound during IJ placement. The majority of SC insertions were placed in the surgical ICU and had clear indications (80%) for placement. Of those, 75% were due to trauma (limited access to neck) or surgery (interfering with surgical site) (Table 2).
DISCUSSION
Summary
This quality‐improvement intervention demonstrates that a multidisciplinary team can successfully implement a multifaceted intervention that sustainably reduces the rate of IAP complications from CVC placement and improves patient safety over 7 years. We found high compliance with our intervention, which included an increase in CVC notes and documentation of ultrasound guidance. There was also an increase in the IJ approach in our postintervention period. We showed statistically significant long‐term reductions in both CVC‐associated and all‐cause IAP rates. From 2011 to 2014, there were only 5 cases of CVC‐associated IAP requiring chest tube placement. Post hoc analysis showed a statistically significant decrease in CLABSI rates (P<0.0001) from a preintervention rate of 1.6 infections per 1000 central line days to postintervention average rate of 0.68 infections per 1000 central line days. This decrease may be related to the incorporation of wide sterile barrier techniques in our CVC training workshops, checklists, and template procedure notes.
A strength of this study is the sustained significant long‐term reduction in IAP. There are few data that exist to describe sustained interventions in this area. Sustainability was achieved by integrating our interventions into ongoing programs that already existed in the hospital; we incorporated our simulation training into the existing new resident orientation, increased the availability of existing ultrasound equipment, and continued our IAP chart review through coding quality with feedback to involved services. The procedure note template continues to be easily available in our EMR, and the SC approach to CVC placement is limited to select cases.
Based on a post hoc cost‐benefit analysis, the financial benefits of decreasing the rate of IAP outweigh the costs associated with implementation of this initiative. The purchase cost for a Site‐Rite (Bard Access Systems) ultrasound machine was $18,000. The cost of materials for 1 workshop is $5000 annually. Cases from the Nationwide Inpatient Sample that were flagged by this PSI had 7.0% excess mortality, 4.4 days of excess hospitalization, and approximately $18,000 in excess hospital charges.[17, 18] Based on these data, if we had continued at our preintervention rate of CVC‐associated IAP requiring chest tube placement, we would estimate 9 additional CVC‐associated IAPs requiring chest tube insertion per year. This would result in over $180,000 of additional costs annually. Based on an initial cost of $100,000 for 4 workshops and the necessary equipment, we would have realized our cost savings in less than 1 year postintervention. These are all approximate costs, and further detailed analysis is needed.
One challenge with this intervention is the culture change away from using the SC approach, and the concern from trainees of how they would learn to perform SC CVC if needed. We would suggest dedicated SC CVC ultrasound training for those services who may need to use this approach (eg, neuroanesthesia and trauma).
Interpretation/Relation to Other Evidence
The field of implementation science can help explain why some projects are successful and others fail. We can further dissect the success of this project using an implementation science model similar to that described by French et al.[19] French et al. describe 4 behavior‐change techniques. These steps include (1) who needs to do what differently, (2) which barriers and enablers need to be addressed, (3) which intervention component could overcome the barriers and enhance enablers, and (4) how can behavior change be measured and understood. Barriers included suboptimal skills of residents, low awareness of evidence‐based guidelines, and entrenched practices inconsistent with best evidence. There was also a belief that IJ lines were more likely to become infected. Targeted behaviors needing to be done differently were the choice of CVC placement site and insertion technique. Barriers to change were assessed by asking members of the project team to explore with members of their service what led them to do CVC lines without ultrasound guidance. Enhancements focused on information provision, simulation practice, and persuasive communication. Behavior change was measured by tracking the number of IAPs, site of CVC, and documentation of technique. Continuation of these interventions based on this theoretical framework drove maintenance of gains.
We completed our main intervention planning in 90 days, and met our short‐term goal on schedule. The Institute for Healthcare Improvement (IHI) advocates that such short timelines are efficient mechanisms for developing and acting on projects. Other institutions have reported on similar rapid‐cycle planning and short‐term goal setting[20]
Limitations
Our study captures the experience of a quality‐improvement team at a single academic center, and our results may not be generalizable to other institutions. Our chart review process only occurred once a case had been identified through AHRQ PSI methodology. It is possible that the PSI does not capture all cases of IAP, although we believe our coding department has a very rigorous process to look for all IAP evidence in the patient's record. We used administrative data to determine the number of hospital‐wide CVC procedures.
Our compliance data with interventions from STRIDE are based on looking for key words in procedure note documentation (so undocumented notes are not captured). To validate this, we performed a manual audit of our adherence to our intervention in 2014, and those data are consistent with the results from our STRIDE data.
Our study's observational design also cannot control for exogenous effects on physician practice relating to CVC insertion or the overall risk of IAP. Some of our decrease in complications may be from the increase in PICC line use. Nevertheless, our CVC‐associated IAP rate has decreased despite >6000 non‐PICC CVCs in our ICU over the past 5 years, and a rising CMI (18% increase in postintervention period) and older population of patients with CVC insertion (P<0.0001)
CONCLUSIONS
We are the first, to our knowledge, to report a measurable improvement in reducing IAP patient outcomes that has been sustained for over 7 years. Our hospital is in the highest performance UHC quartile for all‐cause IAP in 2012 to 2014. A multidisciplinary quality‐improvement team, focused on evidence, patient safety, and standardization, can use a multifaceted intervention to sustainably improve patient outcomes. Promoting ultrasound‐guided IJ catheterization as the CVC insertion method of choice significantly reduced our hospital's rate of CVC‐associated IAP.
Acknowledgements
The authors acknowledge many who have contributed to this quality‐improvement project:
Irina Tokareva, Jay Lee, Kourt Bowes, and Gomathi Krishnan for data analysis; Laura Meinke for significant website curriculum; Fred Mihm, Sarah Williams, and John Kugler for leadership in ultrasound training; Kevin Tabb and Norm Rizk for hospital financial support of simulation workshops and ultrasound machines; Pooja Loftus and Helene Grossman for statistical analysis; Eric Hadhazy for data support; Joan Hendershott for cost information; Nancy Szaflarski for project management and manuscript review; and Isabella Chu for manuscript review.
Disclosures: STRIDE (Stanford Translational Research Integrated Database Environment) is a research and development project at Stanford University to create a standards‐based informatics platform supporting clinical and translational research. This STRIDE project was supported by the National Center for Research Resources and the National Center for Advancing Translational Sciences, National Institutes of Health, through grant UL1 RR025744. The content is solely the responsibility of the authors and does not necessarily represent the official views of the NIH. The authors report no conflicts of interest.
- Significance of iatrogenic pneumothoraces. Chest. 1994;105(4):1147–1150. , , .
- How to avoid and manage a pneumothorax. J Vasc Access. 2006;7(1):7–14. , , , , , .
- Iatrogenic pneumothorax: etiology, incidence and risk factors. Thorac Cardiovasc Surg. 2009;57(5):286–290. , , , .
- Real‐time ultrasound‐guided catheterisation of the internal jugular vein: a prospective comparison with the landmark technique in critical care patients. Crit Care. 2006;10(6):R162. , , , et al.
- Safe placement of central venous catheters: a measured approach. J Intens Care Med. 2011;26(6):392–396. , , .
- Reducing iatrogenic risk in thoracentesis: establishing best practice via experiential training in a zero‐risk environment. Chest. 2009;135(5):1315–1320. , , , .
- Use of simulation‐based education to improve outcomes of central venous catheterization: a systematic review and meta‐analysis. Acad Med. 2011;86(9):1137–1147. , , , , , .
- A prerotational, simulation‐based workshop improves the safety of central venous catheter insertion: results of a successful internal medicine house staff training program. Chest. 2011;140(3):652–658. , , , , , .
- Linking residency training effectiveness to clinical outcomes: a quality improvement approach. Jt Comm J Qual Patient Saf. 2010;36(5):203–208. , , , , .
- Education of physicians‐in‐training can decrease the risk for vascular catheter infection. Ann Intern Med. 2000;132(8):641–648. , , , et al.
- A multidisciplinary approach to reduce central line‐associated bloodstream infections. Jt Comm J Qual Patient Saf. 2013;39(2):61–69. , , , et al.
- Validity of selected Patient Safety Indicators: opportunities and concerns. J Am Coll Surg. 2011;212(6):924–934. , , , et al.
- Cases of iatrogenic pneumothorax can be identified from ICD‐9‐CM coded data. Am J Med Qual. 2010;25(3):218–224. , , , et al.
- SQUIRE development group. Publication guidelines for quality improvement studies in health care: evolution of the SQUIRE project. BMJ. 2009;338:a3152. , , , , ;
- The Quality Toolbox. 2nd ed. Milwaukee, WI: ASQ Quality Press; 2005. .
- STRIDE—an integrated standards‐based translational research informatics platform. AMIA Annu Symp Proc. 2009;2009:391–395. , , , .
- Accidental iatrogenic pneumothorax in hospitalized patients. Med Care. 2006;44(2):182–186. , , .
- Excess length of stay, charges, and mortality attributable to medical injuries during hospitalization. JAMA. 2003;290(14):1868–1874. , .
- Developing theory‐informed behaviour change interventions to implement evidence into practice: a systematic approach using the Theoretical Domains Framework. Implement Sci. 2012;7:38. , , , et al.
- Using rapid‐cycle quality improvement methodology to reduce feeding tubes in patients with advanced dementia: before and after study. BMJ. 2004;329(7464):491–494. , .
- Significance of iatrogenic pneumothoraces. Chest. 1994;105(4):1147–1150. , , .
- How to avoid and manage a pneumothorax. J Vasc Access. 2006;7(1):7–14. , , , , , .
- Iatrogenic pneumothorax: etiology, incidence and risk factors. Thorac Cardiovasc Surg. 2009;57(5):286–290. , , , .
- Real‐time ultrasound‐guided catheterisation of the internal jugular vein: a prospective comparison with the landmark technique in critical care patients. Crit Care. 2006;10(6):R162. , , , et al.
- Safe placement of central venous catheters: a measured approach. J Intens Care Med. 2011;26(6):392–396. , , .
- Reducing iatrogenic risk in thoracentesis: establishing best practice via experiential training in a zero‐risk environment. Chest. 2009;135(5):1315–1320. , , , .
- Use of simulation‐based education to improve outcomes of central venous catheterization: a systematic review and meta‐analysis. Acad Med. 2011;86(9):1137–1147. , , , , , .
- A prerotational, simulation‐based workshop improves the safety of central venous catheter insertion: results of a successful internal medicine house staff training program. Chest. 2011;140(3):652–658. , , , , , .
- Linking residency training effectiveness to clinical outcomes: a quality improvement approach. Jt Comm J Qual Patient Saf. 2010;36(5):203–208. , , , , .
- Education of physicians‐in‐training can decrease the risk for vascular catheter infection. Ann Intern Med. 2000;132(8):641–648. , , , et al.
- A multidisciplinary approach to reduce central line‐associated bloodstream infections. Jt Comm J Qual Patient Saf. 2013;39(2):61–69. , , , et al.
- Validity of selected Patient Safety Indicators: opportunities and concerns. J Am Coll Surg. 2011;212(6):924–934. , , , et al.
- Cases of iatrogenic pneumothorax can be identified from ICD‐9‐CM coded data. Am J Med Qual. 2010;25(3):218–224. , , , et al.
- SQUIRE development group. Publication guidelines for quality improvement studies in health care: evolution of the SQUIRE project. BMJ. 2009;338:a3152. , , , , ;
- The Quality Toolbox. 2nd ed. Milwaukee, WI: ASQ Quality Press; 2005. .
- STRIDE—an integrated standards‐based translational research informatics platform. AMIA Annu Symp Proc. 2009;2009:391–395. , , , .
- Accidental iatrogenic pneumothorax in hospitalized patients. Med Care. 2006;44(2):182–186. , , .
- Excess length of stay, charges, and mortality attributable to medical injuries during hospitalization. JAMA. 2003;290(14):1868–1874. , .
- Developing theory‐informed behaviour change interventions to implement evidence into practice: a systematic approach using the Theoretical Domains Framework. Implement Sci. 2012;7:38. , , , et al.
- Using rapid‐cycle quality improvement methodology to reduce feeding tubes in patients with advanced dementia: before and after study. BMJ. 2004;329(7464):491–494. , .
© 2015 Society of Hospital Medicine
Severe‐Sepsis Screening Tool
Sepsis remains a significant healthcare burden and is the sixth most common reason for hospitalization in the United States. For patients presenting with severe sepsis, mortality rates are approximately 30%,[1, 2] and sepsis remains the most expensive reason for hospitalization. In 2009, septicemia accounted for nearly $15.4 billion in aggregate hospital costs.[2]
Early identification of sepsis and the timely implementation of goal‐directed therapy significantly decrease sepsis‐related mortality and are cost‐effective,[3, 4, 5] highlighting the need for new clinical strategies to aid in early diagnosis. To date, most studies have focused on the screening and management of sepsis in the emergency department and intensive care unit (ICU),[6, 7] and less is known about the benefits of screening in non‐ICU settings. In the non‐ICU setting, conditions may go unrecognized and treatments delayed. Evidence suggests that patients diagnosed with severe sepsis in the non‐ICU setting are almost twice as likely to die as those diagnosed in an emergency department.[8, 9]
Application of a sepsis screening tool to both medical and surgical patients poses an additional challenge that may impact the screen's performance. The specificity may be compromised by noninfectious causes of systemic inflammatory response syndrome (SIRS) commonly seen in the postsurgical patient. For example, the tachycardia and fever often seen in the postoperative patient are sufficient to qualify for SIRS, making the diagnosis of sepsis more challenging. The purpose of this study was to examine the performance of a nurse‐driven, simple sepsis screening tool in a mixed medical and surgical non‐ICU setting.
METHODS
Setting
This was an observational pilot study of prospectively screened patients admitted to a 26‐bed medical/surgical intermediate care unit with telemetry monitoring in a 613‐bed university tertiary referral hospital over a 1‐month time period. The surgical patient population of this floor consisted of cardiothoracic (50%), general (24%), and vascular surgery (17%) patients as well as a small number of trauma (7%) patients. The medical patient population admitted to this unit included pretransplant and complex medical patients requiring telemetry monitoring. Though the incidence of sepsis specific to this unit was unknown prior to the study, after an analysis of discharges the study team surmised there would be sufficient volume for testing of a nurse‐based screening tool.
Nurse Education
Registered nurses (RNs) working on the study unit had an average of 5 to 7 years of experience. The all‐RN unit was staffed predominantly at a 1:3 RN to patient ratio. RNs were supported by a clinical nurse specialist (CNS) and clinical educator (CE) RN who provided regular ongoing education about infection prevention and identification of common conditions that are seen on the unit.
In the 6 months prior to our sepsis screening initiative, nursing staff had been given more than 8 hours of education on infection‐ and sepsis‐related topics in 15‐ to 20‐minute blocks of time. This dedicated education took place during the nurses' shift in groups of 2 to 3, and was run by the CNS, assistant nurse manager, and CE RN. Nurses were also encouraged to attend an optional 8‐hour sepsis continuing medical education (CME) program. Approximately 20% of the nurses on the study unit attended.
Just prior to the pilot study, nursing staff completed a 1‐hour refresher self‐study module on severe sepsis stressing the importance of early identification. There was also a training month prior to the actual data collection time frame, where unit core trainers (RNs) or champions who had attended the optional 8‐hour sepsis CME conducted 1:1 follow‐up with each RN, reviewing at least 1 of their screens to validate understanding of screening concepts. Each RN was checked off after correctly completing a screen. During the study, unit educators and the CNS provided additional on‐unit in‐service training with screening tool completion instructions and advice on how to incorporate the tool into the RN's current assessment workflow. In addition, the charge nurses were asked to review the screens collected each shift and validate any that may have seemed inconsistent with the RN's verbal report of the patient's status.
The university's institutional review board notice of determination waived review for this study because it was classified as quality improvement.
Screening Tool
A sepsis screening tool was developed as part of a broader initiative to improve sepsis‐related morbidity and mortality at our hospital. The screening tool was adapted from the severe sepsis screening tool created by the Surviving Sepsis Campaign and Institute for Healthcare Improvement,[10] and consisted of a simple 3‐tiered paper‐based screening assessment that was to be completed by the bedside RN (Figure 1). RNs on the pilot medical/surgical intermediate care unit performed the screening assessment with their regular patient assessment at the beginning of each shift.
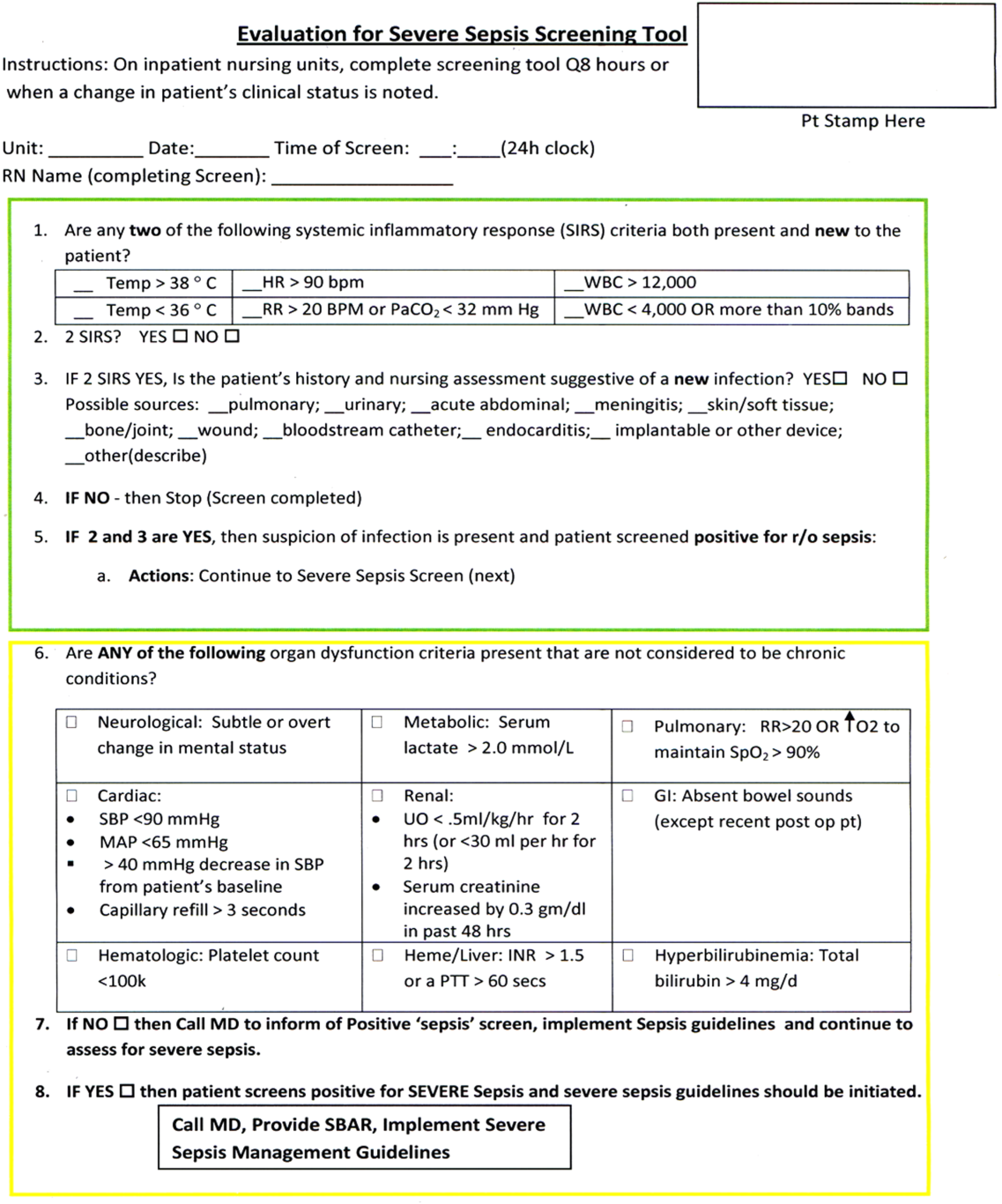
The first tier of the tool screened for the presence of SIRS. Positive parameters included heart rate >90, temperature >38C or <36C, white blood cell count >12,000 or<4000 or >10% bands, and/or respiratory rate >20 or partial pressure of carbon dioxide (PaCO2) <32 mm Hg. To decrease the number of false‐positive screens in patients whose abnormal vitals could already be attributed to a condition other than sepsis, these symptoms were only scored if they had emerged within the previous 8 hours.
If patients met 2 SIRS criteria, the nurse would move to the second tier of the tool, which involved consideration of possible infection as a contributor to a patient's clinical condition as well as a source of infection. If infection was not suspected, further screening was terminated. If infection was suspected, the patient then met criteria for a positive sepsis screen, and a third tier of screening involving assessment of organ dysfunction was initiated.
If the patient screened positive for sepsis (2 SIRS and suspicion for new infection) or severe sepsis (sepsis with end‐organ dysfunction), nurses were instructed to document this in the patient's electronic medical record (EMR) and call the primary team to initiate actions following the hospital‐wide sepsis guidelines. Any subsequent actions were recorded in the patient's EMR.
Data Collection
Completed sepsis screening forms during the month of October 2010 were reviewed by the authors (E.G., L.S., and P.M.). Data including age, gender, International Classification of Diseases, Ninth Revision (ICD‐9) admission and discharge diagnoses, vital signs, lab results, clinical interventions, and documented clinical decision processes by healthcare staff were collected on patients with a positive screen or those who did not screen positive but had an ICD‐9 code for sepsis, severe sepsis, or septic shock during their hospitalization or at discharge. We also collected demographic and clinical data for a random sample of patients who consistently screened negative for sepsis.
Performance Measurement
The sensitivity and specificity of the screening tool was determined by identifying true‐positive, false‐positive, true‐negative, and false‐negative results and calculating accordingly using a 2 2 contingency table. True positives were defined as cases where patients screened positive for sepsis and had a documented diagnosis of sepsis in their EMR within 24 hours of the positive screening or had an ICD‐9 billing code for sepsis. False‐positive cases were those in which patients screened positive for sepsis but did not have a diagnosis of sepsis by manual chart review nor was there an ICD‐9 code for sepsis for their hospital stay. True‐negative cases were those where patients screened negative and did not have an ICD‐9 code for sepsis. False negatives were cases where patients consistently screened negative for sepsis but had an ICD‐9 code for sepsis.
Clinical Activities
To examine the impact of a positive sepsis screen on subsequent clinical action, we assessed the frequency with which a treatment or diagnostic workup was initiated after a positive screen and compared this to clinical activity initiated after a negative screen. Specifically, the patient's EMR was reviewed for actions including measurement of lactate, blood cultures, administration of broad spectrum antibiotics, administration of fluid boluses, or consultation with or transfer to the ICU. These actions were chosen because they are part of the Surviving Sepsis Bundle, which has been demonstrated to improve mortality rates after diagnosis of severe sepsis or septic shock,[11, 12] and can be done outside of an ICU setting. Because screening was done every 8 hours, clinical activity was only attributed to a positive or negative sepsis screen if it occurred within 8 hours of the screening result. Patients were excluded if there were missing data points that precluded full analysis of their clinical course.
Statistical Analysis
To compare the performance of the screening tool between surgical and medical patients, we calculated 95% confidence intervals of screening test sensitivity and specificity. To test if performance was significantly different between these groups, we performed a nonparametric, 2‐sided, 2‐sample test of proportions. Though similar to a [2] test, the 2‐sided test of proportions allowed us to determine if there was a directional difference in test performance (ie, Does the screening tool perform better or worse in a certain patient group?). We also used the test of proportions to compare differences in the proportion of patients receiving sepsis‐related interventions before and after a positive or negative screening result. For comparisons of demographic variables we used nonparametric tests including the [2] test for categorical variables and the Kruskal‐Wallis test for continuous variables. We used SAS 9.3 (SAS Institute Inc., Cary, NC) to perform our analyses.
RESULTS
Over a 1‐month time period, 2143 screens were completed on 245 patients (169 surgical, 76 medical). The overall incidence of sepsis on the treatment unit during this time period was 9%. Surgical patients had an 8.9% incidence of sepsis, and medical patients had an incidence of 9.2%.
Screening tool performance is presented in Table 1. The screening tool had 95.5% sensitivity and 91.9% specificity, with no significant differences in performance between surgical and medical patients. The overall negative predictive value was 99.5%, also with comparable performance in both surgical and medical patients (P = 0.89). The overall positive predictive value (PPV) was 70% in medical patients and 48% in surgical patients (P = 0.12). Screening tool accuracy for medical and surgical patients was 92%.
Overall, N = 245 (95% CI) | Surgery, N = 169 (95% CI) | Medicine, N = 76 (95% CI) | P Value* | |
---|---|---|---|---|
| ||||
Sensitivity | 95.5% (75%‐99.7%) | 93% (66%‐99.6%) | 100% (56%‐100%) | 0.17 |
Specificity | 91.9% (87%‐95%) | 90% (84%‐94%) | 95% (87%‐99%) | 0.48 |
NPV | 99.5% (81%‐100%) | 99.3% (71%‐100%) | 100% (67%‐100%) | 0.89 |
PPV | 53.8% (39%‐70%) | 48% (23%‐73%) | 70% (30%‐100%) | 0.12 |
LR+ | 11.8 | 9.3 | 20 | |
LR | 0.05 | 0.08 | 0 | |
Confirmed patient diagnosis, overall | ||||
Sepsis | No sepsis | |||
Screen positive | 21 (TP) | 18 (FP) | ||
Screen negative | 1 (FN) | 205 (TN) | ||
Confirmed patient diagnosis, medicine | ||||
Sepsis | No sepsis | |||
Screen positive | 7 (TP) | 3 (FP) | ||
Screen negative | 0 (FN) | 66 (TN) | ||
Confirmed patient diagnosis, surgery | ||||
Sepsis | No sepsis | |||
Screen positive | 14 (TP) | 15 (FP) | ||
Screen negative | 1 (FN) | 139 (TN) |
Clinical Activities
Of the 39 patients who screened positive for sepsis, nurses classified 20 with sepsis and 19 with severe sepsis. Of these 39 patients, 33 were included in our descriptive analysis of the effect of positive screening results on clinical activity (3 were excluded for admission for sepsis and 3 for missing data). As a comparison, we randomly selected 30 patients of the 206 patients who screened negative for sepsis to evaluate clinical activity before and after a negative screen.
Characteristics of patients screening positive and negative for sepsis are reported in Table 2. We found no statistically significant differences in age, sex, length of hospital stay, or mortality amongst all groups.
Patient Characteristics | Surgery (Positive) | Medicine (Positive) | Surgery (Negative) | Medicine (Negative) | P Value |
---|---|---|---|---|---|
| |||||
No. | 26 | 7 | 20 | 10 | |
Age, y, mean | 57.8 ( 16.5) | 72.4 ( 16.8) | 64.6 ( 19.4) | 63.6 ( 16.8) | 0.25 |
% Male (no.) | 50% (13) | 57% (4) | 60% (12) | 60% (6) | 0.27 |
Length of stay, d, median (IQR) | 9 (716.7) | 7 (5.511.5) | 11 (7.722) | 8 (421) | 0.38 |
No. of PODs until first positive screen, d, median (IQR) | 2 (13) | N/A | N/A | N/A | |
% Mortality (no.) | 0% | 14% (1) | 5% (1) | 10% (1) | 0.19 |
Figure 2 illustrates differences in the proportion of patients receiving a clinical action before and after a negative or positive screening test result. In the cohort of 33 patients screening positive for sepsis, clinical action after a positive screen was taken in 4 of the 7 (50%) medical patients and 11 of 26 (42%) surgical patients. In patients screening negative for sepsis we found only 1 incident in which a sepsis‐related action was taken after a negative screen. In this case the patient was admitted to the ICU within 8 hours of a negative screen, though there was no explicit documentation that sepsis was the reason for this admission.
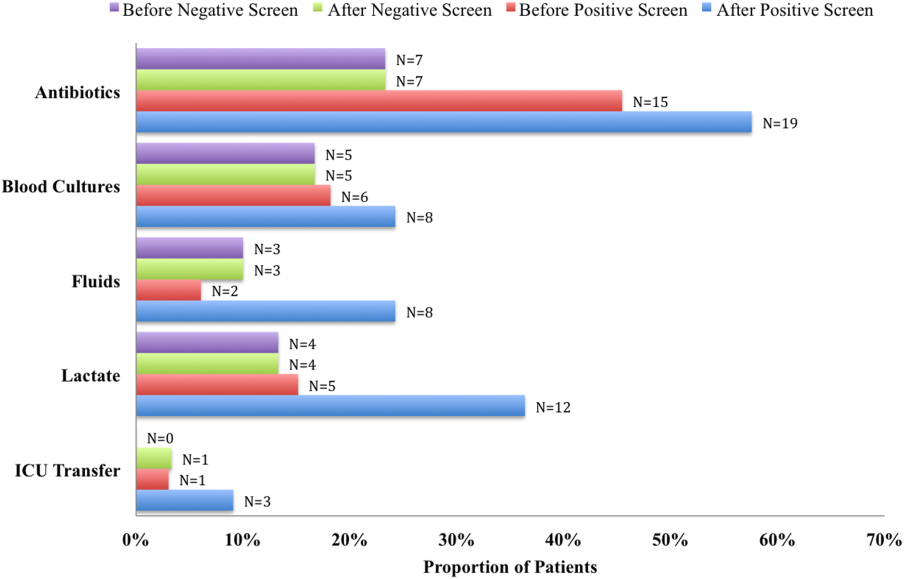
We compared the proportion of patients receiving sepsis‐related treatment before either a negative or positive screen and found no significant difference (Table 3). We then compared the proportion of patients receiving sepsis‐related actions after a positive or negative screening test result and found that the proportion of patients receiving antibiotics, blood cultures, and lactate measurement was significantly higher for patients with a positive sepsis screening result compared to those with a negative screening result (Table 3).
Intervention and Group | Proportion | P Value |
---|---|---|
| ||
Before screening test | ||
Antibiotics | 0.066 | |
Positive screen | 45% | |
Negative screen | 23% | |
Lactate | 0.837 | |
Positive screen | 15% | |
Negative screen | 13% | |
Blood culture | 0.181 | |
Positive screen | 18% | |
Negative screen | 17% | |
Fluid administration | 0.564 | |
Positive screen | 6% | |
Negative screen | 10% | |
ICU transfer/consult | 0.337 | |
Positive screen | 3% | |
Negative screen | 0% | |
After screening test | ||
Antibiotics | 0.006 | |
Positive screen | 58% | |
Negative screen | 23% | |
Lactate | 0.018 | |
Positive screen | 36% | |
Negative screen | 13% | |
Blood Culture | 0.002 | |
Positive screen | 24% | |
Negative screen | 17% | |
Fluid administration | 0.112 | |
Positive screen | 24% | |
Negative screen | 10% | |
ICU transfer/consult | 0.175 | |
Positive screen | 9% | |
Negative screen | 3% |
DISCUSSION
Improving recognition and time to treatment of sepsis in a non‐ICU setting is an important step toward decreasing sepsis‐related mortality. Lundberg and colleagues found that mortality rates for patients diagnosed with septic shock on a general ward were higher than for patients diagnosed in the ICU, even though ward patients were younger and healthier at baseline.[8] For ward patients, treatment delays were most profound in initiating vasoactive therapies, and minor delays were encountered in initiating fluid resuscitation. In their international study on the impact of early goal‐directed therapy guidelines, Levy and colleagues found that patients diagnosed with severe sepsis on the wards were almost twice as likely to die as patients diagnosed with sepsis in the emergency department.[9]
We are the first to report about an accurate nurse‐driven SIRS‐based sepsis screening protocol that is effective in the early identification of sepsis in both medical and surgical patients in an intermediate care setting. We found no significant difference in the screening tool performance between the medical and surgical cohorts. This is an important comparison given that SIRS criteria alone can be nonspecific in the postoperative population, where it is common to have hemodynamic changes, elevation of inflammatory markers, and fevers from noninfectious sources.
Our sepsis screening tool was designed in 3 tiers to improve its specificity. The first tier was based strictly on SIRS criteria (eg, tachycardia or fever), whereas the second and third tiers served to increase the specificity of the screening tool by instructing the evaluator to assess possible sources of infection and assess for objective signs of organ dysfunction. We relied heavily on the nursing staff to assess for the presence or absence of infection and believe that the educational component prior to initiating the screening protocol was vital.
EMR‐based screening tools that rely purely on physiologic data have been considered for the early detection and management of sepsis, although they lack the specificity gained through the incorporation of clinical judgment.[13] Sawyer and colleagues report using a real‐time EMR‐based method for early sepsis detection in non‐ICU patients that is based solely on objective measures; however, their PPV was only 19.5%. The model we describe in this study is one that incorporates real‐time physiologic data available from an EMR coupled with the clinical judgment of a bedside registered nurse. As our data suggest, this provides a screen that is both sensitive and specific.
It is interesting to note that in our assessment of clinical action taken 8 hours after a positive screening test (the interval after which a new screening test was performed), the rate of diagnostic workup and/or treatment for sepsis was relatively low. One reason for this could have been that the treating team had suspicion for sepsis prior to a positive screen and had already initiated clinical action. Of the 51 recorded clinical actions taken around the time of a positive screen, the majority (67%) occurred before the screening result. It is also possible that clinical action was not pursued because the treatment team disagreed with a diagnosis of sepsis. Of all the false positive screening cases, manual chart review confirmed that these patients did not have sepsis, nor did they develop sepsis during their index hospital stay. Last, we only recorded clinical actions taken within 8 hours of the first positive screen for sepsis and measured 5 very specific actions. Thus, our analysis may have missed actions taken after 8 hours or actions that differed from the 5 we chose to assess.
Even with the apparently low levels of new clinical activity after a positive screen, when compared to patients who screened negative for sepsis, a significantly higher number of patients who had a positive screen received antibiotics, had lactate measured, and had blood cultures drawn. We did not find a significant difference in the proportion of patients receiving a sepsis‐related clinical action before a screening result (positive or negative), which suggests that a positive screening test may have led to increased clinical action.
A limitation of our study is its small size and that it was conducted in 1 pilot unit. Additionally, our retrospective analysis of clinical care inhibited our ability to fully understand a patient's clinical course or retrieve missing data points. A related limitation is that we could not ascertain how often the screening tool did not identify a case of sepsis before it was clinically diagnosed. Assessing the temporal performance of our screening tool is of great interest and may be more easily performed using an electronic version of the screening tool, which is currently in development.
Using ICD‐9 codes to determine the true‐negative cohort is another limitation of our study. It is well documented that use of administrative data can lead to inaccurate classification of patients.[14] To address this, we performed random audits of 30 test‐negative patients. In doing so we did not find any errors in classification.
Although we did not find a significant difference in screening tool performance between surgical and medical patients, the PPV of the tool was lower in the surgical population (48%) compared to the medical population (70%). The lower PPV observed in surgical patients could be attributable to an overall lower incidence of sepsis in this cohort as well as possible errors in initial assessment of infection, which can be difficult in postsurgical patients. Our retrospective analysis included data from the early months of the screening protocol, a time in which nursing staff was still developing clinical acumen in identifying sepsis. However, this could have led nurses to either overestimate or underestimate the presence of infection in either patient group.
Suspicion for infection is the cornerstone definition of sepsis, and in our screening protocol nurses were charged with making this decision based on their knowledge of the patient's clinical course and current status. Issues concerning nurses' recognition of infection symptoms are an area of opportunity for further research and education and could aid in improving PPV. Clinical judgment could be further bolstered by adding promising laboratory tests such as C‐reactive protein or procalcitonin as objective adjuncts to an initial assessment for sepsis,[15] which could potentially increase screening test PPV.
CONCLUSIONS
A simple screening tool for sepsis performed by the bedside nurse can provide a means to successfully identify sepsis early and lead to more timely diagnostics and treatment in both medical and surgical patients in an intermediate care setting.
ACKNOWLEDGEMENTS
The authors thank Eileen Pummer, quality manager for the sepsis team; Pauline Regner, patient care manager of the pilot study unit; and the nurses who contributed to the screening tool design team and data collection. The authors acknowledge Pooja Loftus for her statistical expertise, and Isabella Chu for her review of the manuscript. Disclosures: Presented as a poster at the 31st Annual Meeting of the Surgical Infection Society, Palm Beach, Florida, May 2011. The authors report no conflicts of interest.
- Epidemiology of severe sepsis in the United States: analysis of incidence, outcome, and associated costs of care. Crit Care Med. 2001;29(7):1303–1310. , , , , ,
- http://www.hcup-us.ahrq.gov/reports/statbriefs/sb122.pdf. Published October 2011. Accessed on September 4, 2012. , , Septicemia in U.S. hospitals, 2009. HCUP statistical brief #122. Agency for Healthcare Research and Quality. Available at:
- Economic implications of an evidence‐based sepsis protocol: can we improve outcomes and lower costs? Crit Care Med. 2007;35(5):1257–1262. , , ,
- Late compliance with the sepsis resuscitation bundle: impact on mortality. Shock. 2011;36(6):542–547. , , , , ,
- The costs and cost‐effectiveness of an integrated sepsis treatment protocol. Crit Care Med. 2008;36(4):1168–1174. , , , , ,
- A simple prediction algorithm for bacteraemia in patients with acute febrile illness. QJM. 2005;98(11):813–820. , ,
- Validation of a screening tool for the early identification of sepsis. J Trauma. 2009;66(6):1539–1546; discussion 1546–1547. , , , et al.
- Septic shock: an analysis of outcomes for patients with onset on hospital wards versus intensive care units. Crit Care Med. 1998;26(6):1020–1024. , , , et al.
- The Surviving Sepsis Campaign: results of an international guideline‐based performance improvement program targeting severe sepsis. Crit Care Med. 2010;38(2):367–374. , , , et al.
- Institute of Healthcare Improvement. Evaluation for severe sepsis screening tool. Surviving Sepsis Campaign. Available at: http://www.survivingsepsis.org/About_the_Campaign/Documents/evaluationforseveresepsisscreeningtool.pdf. Accessed on September 30, 2012.
- Impact of the Surviving Sepsis Campaign protocols on hospital length of stay and mortality in septic shock patients: results of a three‐year follow‐up quasi‐experimental study. Crit Care Med. 2010;38(4):1036–1043. , , , et al.
- Reduction of the severe sepsis or septic shock associated mortality by reinforcement of the recommendations bundle: a multicenter study. Ann Fr Anesth Reanim. 2010;29(9):621–628. , , , et al.
- Implementation of a real‐time computerized sepsis alert in nonintensive care unit patients. Crit Care Med. 2011;39(3):469–473. , , , et al.
- Accuracy of administrative data for identifying patients with pneumonia. Am J Med Qual. 2005;20(6):319–328. , , ,
- Comparison of procalcitonin and C‐reactive protein as markers of sepsis. Crit Care Med. 2003;31(6):1737–1741. , , , , ,
Sepsis remains a significant healthcare burden and is the sixth most common reason for hospitalization in the United States. For patients presenting with severe sepsis, mortality rates are approximately 30%,[1, 2] and sepsis remains the most expensive reason for hospitalization. In 2009, septicemia accounted for nearly $15.4 billion in aggregate hospital costs.[2]
Early identification of sepsis and the timely implementation of goal‐directed therapy significantly decrease sepsis‐related mortality and are cost‐effective,[3, 4, 5] highlighting the need for new clinical strategies to aid in early diagnosis. To date, most studies have focused on the screening and management of sepsis in the emergency department and intensive care unit (ICU),[6, 7] and less is known about the benefits of screening in non‐ICU settings. In the non‐ICU setting, conditions may go unrecognized and treatments delayed. Evidence suggests that patients diagnosed with severe sepsis in the non‐ICU setting are almost twice as likely to die as those diagnosed in an emergency department.[8, 9]
Application of a sepsis screening tool to both medical and surgical patients poses an additional challenge that may impact the screen's performance. The specificity may be compromised by noninfectious causes of systemic inflammatory response syndrome (SIRS) commonly seen in the postsurgical patient. For example, the tachycardia and fever often seen in the postoperative patient are sufficient to qualify for SIRS, making the diagnosis of sepsis more challenging. The purpose of this study was to examine the performance of a nurse‐driven, simple sepsis screening tool in a mixed medical and surgical non‐ICU setting.
METHODS
Setting
This was an observational pilot study of prospectively screened patients admitted to a 26‐bed medical/surgical intermediate care unit with telemetry monitoring in a 613‐bed university tertiary referral hospital over a 1‐month time period. The surgical patient population of this floor consisted of cardiothoracic (50%), general (24%), and vascular surgery (17%) patients as well as a small number of trauma (7%) patients. The medical patient population admitted to this unit included pretransplant and complex medical patients requiring telemetry monitoring. Though the incidence of sepsis specific to this unit was unknown prior to the study, after an analysis of discharges the study team surmised there would be sufficient volume for testing of a nurse‐based screening tool.
Nurse Education
Registered nurses (RNs) working on the study unit had an average of 5 to 7 years of experience. The all‐RN unit was staffed predominantly at a 1:3 RN to patient ratio. RNs were supported by a clinical nurse specialist (CNS) and clinical educator (CE) RN who provided regular ongoing education about infection prevention and identification of common conditions that are seen on the unit.
In the 6 months prior to our sepsis screening initiative, nursing staff had been given more than 8 hours of education on infection‐ and sepsis‐related topics in 15‐ to 20‐minute blocks of time. This dedicated education took place during the nurses' shift in groups of 2 to 3, and was run by the CNS, assistant nurse manager, and CE RN. Nurses were also encouraged to attend an optional 8‐hour sepsis continuing medical education (CME) program. Approximately 20% of the nurses on the study unit attended.
Just prior to the pilot study, nursing staff completed a 1‐hour refresher self‐study module on severe sepsis stressing the importance of early identification. There was also a training month prior to the actual data collection time frame, where unit core trainers (RNs) or champions who had attended the optional 8‐hour sepsis CME conducted 1:1 follow‐up with each RN, reviewing at least 1 of their screens to validate understanding of screening concepts. Each RN was checked off after correctly completing a screen. During the study, unit educators and the CNS provided additional on‐unit in‐service training with screening tool completion instructions and advice on how to incorporate the tool into the RN's current assessment workflow. In addition, the charge nurses were asked to review the screens collected each shift and validate any that may have seemed inconsistent with the RN's verbal report of the patient's status.
The university's institutional review board notice of determination waived review for this study because it was classified as quality improvement.
Screening Tool
A sepsis screening tool was developed as part of a broader initiative to improve sepsis‐related morbidity and mortality at our hospital. The screening tool was adapted from the severe sepsis screening tool created by the Surviving Sepsis Campaign and Institute for Healthcare Improvement,[10] and consisted of a simple 3‐tiered paper‐based screening assessment that was to be completed by the bedside RN (Figure 1). RNs on the pilot medical/surgical intermediate care unit performed the screening assessment with their regular patient assessment at the beginning of each shift.
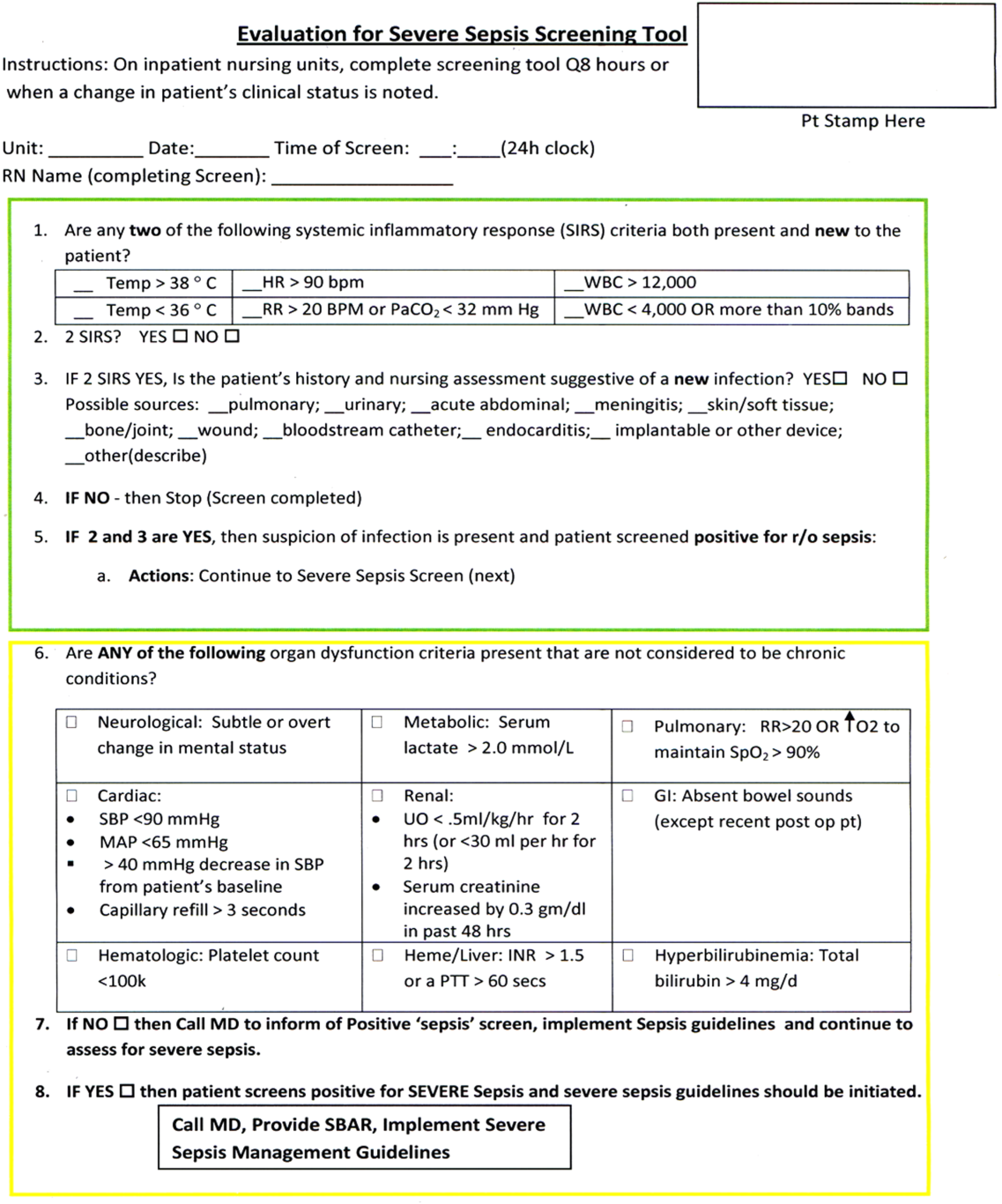
The first tier of the tool screened for the presence of SIRS. Positive parameters included heart rate >90, temperature >38C or <36C, white blood cell count >12,000 or<4000 or >10% bands, and/or respiratory rate >20 or partial pressure of carbon dioxide (PaCO2) <32 mm Hg. To decrease the number of false‐positive screens in patients whose abnormal vitals could already be attributed to a condition other than sepsis, these symptoms were only scored if they had emerged within the previous 8 hours.
If patients met 2 SIRS criteria, the nurse would move to the second tier of the tool, which involved consideration of possible infection as a contributor to a patient's clinical condition as well as a source of infection. If infection was not suspected, further screening was terminated. If infection was suspected, the patient then met criteria for a positive sepsis screen, and a third tier of screening involving assessment of organ dysfunction was initiated.
If the patient screened positive for sepsis (2 SIRS and suspicion for new infection) or severe sepsis (sepsis with end‐organ dysfunction), nurses were instructed to document this in the patient's electronic medical record (EMR) and call the primary team to initiate actions following the hospital‐wide sepsis guidelines. Any subsequent actions were recorded in the patient's EMR.
Data Collection
Completed sepsis screening forms during the month of October 2010 were reviewed by the authors (E.G., L.S., and P.M.). Data including age, gender, International Classification of Diseases, Ninth Revision (ICD‐9) admission and discharge diagnoses, vital signs, lab results, clinical interventions, and documented clinical decision processes by healthcare staff were collected on patients with a positive screen or those who did not screen positive but had an ICD‐9 code for sepsis, severe sepsis, or septic shock during their hospitalization or at discharge. We also collected demographic and clinical data for a random sample of patients who consistently screened negative for sepsis.
Performance Measurement
The sensitivity and specificity of the screening tool was determined by identifying true‐positive, false‐positive, true‐negative, and false‐negative results and calculating accordingly using a 2 2 contingency table. True positives were defined as cases where patients screened positive for sepsis and had a documented diagnosis of sepsis in their EMR within 24 hours of the positive screening or had an ICD‐9 billing code for sepsis. False‐positive cases were those in which patients screened positive for sepsis but did not have a diagnosis of sepsis by manual chart review nor was there an ICD‐9 code for sepsis for their hospital stay. True‐negative cases were those where patients screened negative and did not have an ICD‐9 code for sepsis. False negatives were cases where patients consistently screened negative for sepsis but had an ICD‐9 code for sepsis.
Clinical Activities
To examine the impact of a positive sepsis screen on subsequent clinical action, we assessed the frequency with which a treatment or diagnostic workup was initiated after a positive screen and compared this to clinical activity initiated after a negative screen. Specifically, the patient's EMR was reviewed for actions including measurement of lactate, blood cultures, administration of broad spectrum antibiotics, administration of fluid boluses, or consultation with or transfer to the ICU. These actions were chosen because they are part of the Surviving Sepsis Bundle, which has been demonstrated to improve mortality rates after diagnosis of severe sepsis or septic shock,[11, 12] and can be done outside of an ICU setting. Because screening was done every 8 hours, clinical activity was only attributed to a positive or negative sepsis screen if it occurred within 8 hours of the screening result. Patients were excluded if there were missing data points that precluded full analysis of their clinical course.
Statistical Analysis
To compare the performance of the screening tool between surgical and medical patients, we calculated 95% confidence intervals of screening test sensitivity and specificity. To test if performance was significantly different between these groups, we performed a nonparametric, 2‐sided, 2‐sample test of proportions. Though similar to a [2] test, the 2‐sided test of proportions allowed us to determine if there was a directional difference in test performance (ie, Does the screening tool perform better or worse in a certain patient group?). We also used the test of proportions to compare differences in the proportion of patients receiving sepsis‐related interventions before and after a positive or negative screening result. For comparisons of demographic variables we used nonparametric tests including the [2] test for categorical variables and the Kruskal‐Wallis test for continuous variables. We used SAS 9.3 (SAS Institute Inc., Cary, NC) to perform our analyses.
RESULTS
Over a 1‐month time period, 2143 screens were completed on 245 patients (169 surgical, 76 medical). The overall incidence of sepsis on the treatment unit during this time period was 9%. Surgical patients had an 8.9% incidence of sepsis, and medical patients had an incidence of 9.2%.
Screening tool performance is presented in Table 1. The screening tool had 95.5% sensitivity and 91.9% specificity, with no significant differences in performance between surgical and medical patients. The overall negative predictive value was 99.5%, also with comparable performance in both surgical and medical patients (P = 0.89). The overall positive predictive value (PPV) was 70% in medical patients and 48% in surgical patients (P = 0.12). Screening tool accuracy for medical and surgical patients was 92%.
Overall, N = 245 (95% CI) | Surgery, N = 169 (95% CI) | Medicine, N = 76 (95% CI) | P Value* | |
---|---|---|---|---|
| ||||
Sensitivity | 95.5% (75%‐99.7%) | 93% (66%‐99.6%) | 100% (56%‐100%) | 0.17 |
Specificity | 91.9% (87%‐95%) | 90% (84%‐94%) | 95% (87%‐99%) | 0.48 |
NPV | 99.5% (81%‐100%) | 99.3% (71%‐100%) | 100% (67%‐100%) | 0.89 |
PPV | 53.8% (39%‐70%) | 48% (23%‐73%) | 70% (30%‐100%) | 0.12 |
LR+ | 11.8 | 9.3 | 20 | |
LR | 0.05 | 0.08 | 0 | |
Confirmed patient diagnosis, overall | ||||
Sepsis | No sepsis | |||
Screen positive | 21 (TP) | 18 (FP) | ||
Screen negative | 1 (FN) | 205 (TN) | ||
Confirmed patient diagnosis, medicine | ||||
Sepsis | No sepsis | |||
Screen positive | 7 (TP) | 3 (FP) | ||
Screen negative | 0 (FN) | 66 (TN) | ||
Confirmed patient diagnosis, surgery | ||||
Sepsis | No sepsis | |||
Screen positive | 14 (TP) | 15 (FP) | ||
Screen negative | 1 (FN) | 139 (TN) |
Clinical Activities
Of the 39 patients who screened positive for sepsis, nurses classified 20 with sepsis and 19 with severe sepsis. Of these 39 patients, 33 were included in our descriptive analysis of the effect of positive screening results on clinical activity (3 were excluded for admission for sepsis and 3 for missing data). As a comparison, we randomly selected 30 patients of the 206 patients who screened negative for sepsis to evaluate clinical activity before and after a negative screen.
Characteristics of patients screening positive and negative for sepsis are reported in Table 2. We found no statistically significant differences in age, sex, length of hospital stay, or mortality amongst all groups.
Patient Characteristics | Surgery (Positive) | Medicine (Positive) | Surgery (Negative) | Medicine (Negative) | P Value |
---|---|---|---|---|---|
| |||||
No. | 26 | 7 | 20 | 10 | |
Age, y, mean | 57.8 ( 16.5) | 72.4 ( 16.8) | 64.6 ( 19.4) | 63.6 ( 16.8) | 0.25 |
% Male (no.) | 50% (13) | 57% (4) | 60% (12) | 60% (6) | 0.27 |
Length of stay, d, median (IQR) | 9 (716.7) | 7 (5.511.5) | 11 (7.722) | 8 (421) | 0.38 |
No. of PODs until first positive screen, d, median (IQR) | 2 (13) | N/A | N/A | N/A | |
% Mortality (no.) | 0% | 14% (1) | 5% (1) | 10% (1) | 0.19 |
Figure 2 illustrates differences in the proportion of patients receiving a clinical action before and after a negative or positive screening test result. In the cohort of 33 patients screening positive for sepsis, clinical action after a positive screen was taken in 4 of the 7 (50%) medical patients and 11 of 26 (42%) surgical patients. In patients screening negative for sepsis we found only 1 incident in which a sepsis‐related action was taken after a negative screen. In this case the patient was admitted to the ICU within 8 hours of a negative screen, though there was no explicit documentation that sepsis was the reason for this admission.
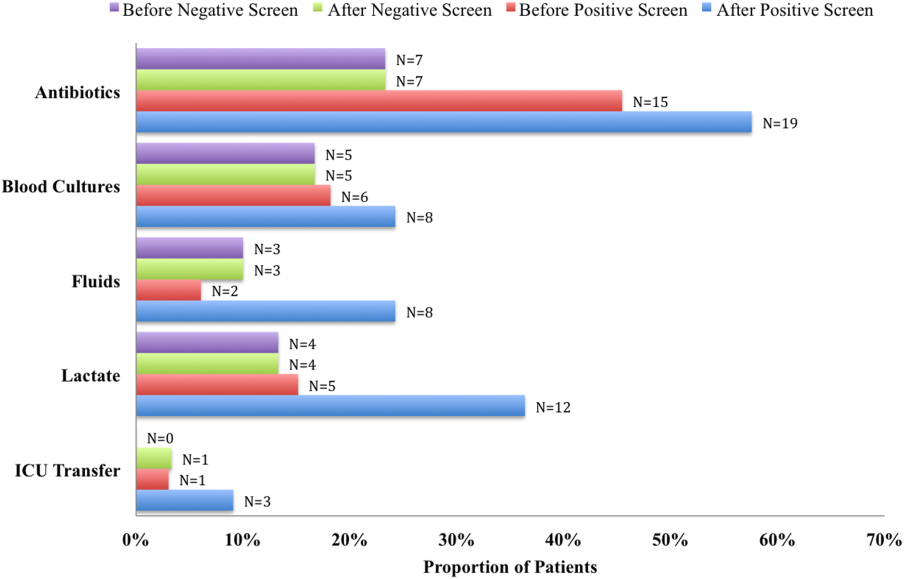
We compared the proportion of patients receiving sepsis‐related treatment before either a negative or positive screen and found no significant difference (Table 3). We then compared the proportion of patients receiving sepsis‐related actions after a positive or negative screening test result and found that the proportion of patients receiving antibiotics, blood cultures, and lactate measurement was significantly higher for patients with a positive sepsis screening result compared to those with a negative screening result (Table 3).
Intervention and Group | Proportion | P Value |
---|---|---|
| ||
Before screening test | ||
Antibiotics | 0.066 | |
Positive screen | 45% | |
Negative screen | 23% | |
Lactate | 0.837 | |
Positive screen | 15% | |
Negative screen | 13% | |
Blood culture | 0.181 | |
Positive screen | 18% | |
Negative screen | 17% | |
Fluid administration | 0.564 | |
Positive screen | 6% | |
Negative screen | 10% | |
ICU transfer/consult | 0.337 | |
Positive screen | 3% | |
Negative screen | 0% | |
After screening test | ||
Antibiotics | 0.006 | |
Positive screen | 58% | |
Negative screen | 23% | |
Lactate | 0.018 | |
Positive screen | 36% | |
Negative screen | 13% | |
Blood Culture | 0.002 | |
Positive screen | 24% | |
Negative screen | 17% | |
Fluid administration | 0.112 | |
Positive screen | 24% | |
Negative screen | 10% | |
ICU transfer/consult | 0.175 | |
Positive screen | 9% | |
Negative screen | 3% |
DISCUSSION
Improving recognition and time to treatment of sepsis in a non‐ICU setting is an important step toward decreasing sepsis‐related mortality. Lundberg and colleagues found that mortality rates for patients diagnosed with septic shock on a general ward were higher than for patients diagnosed in the ICU, even though ward patients were younger and healthier at baseline.[8] For ward patients, treatment delays were most profound in initiating vasoactive therapies, and minor delays were encountered in initiating fluid resuscitation. In their international study on the impact of early goal‐directed therapy guidelines, Levy and colleagues found that patients diagnosed with severe sepsis on the wards were almost twice as likely to die as patients diagnosed with sepsis in the emergency department.[9]
We are the first to report about an accurate nurse‐driven SIRS‐based sepsis screening protocol that is effective in the early identification of sepsis in both medical and surgical patients in an intermediate care setting. We found no significant difference in the screening tool performance between the medical and surgical cohorts. This is an important comparison given that SIRS criteria alone can be nonspecific in the postoperative population, where it is common to have hemodynamic changes, elevation of inflammatory markers, and fevers from noninfectious sources.
Our sepsis screening tool was designed in 3 tiers to improve its specificity. The first tier was based strictly on SIRS criteria (eg, tachycardia or fever), whereas the second and third tiers served to increase the specificity of the screening tool by instructing the evaluator to assess possible sources of infection and assess for objective signs of organ dysfunction. We relied heavily on the nursing staff to assess for the presence or absence of infection and believe that the educational component prior to initiating the screening protocol was vital.
EMR‐based screening tools that rely purely on physiologic data have been considered for the early detection and management of sepsis, although they lack the specificity gained through the incorporation of clinical judgment.[13] Sawyer and colleagues report using a real‐time EMR‐based method for early sepsis detection in non‐ICU patients that is based solely on objective measures; however, their PPV was only 19.5%. The model we describe in this study is one that incorporates real‐time physiologic data available from an EMR coupled with the clinical judgment of a bedside registered nurse. As our data suggest, this provides a screen that is both sensitive and specific.
It is interesting to note that in our assessment of clinical action taken 8 hours after a positive screening test (the interval after which a new screening test was performed), the rate of diagnostic workup and/or treatment for sepsis was relatively low. One reason for this could have been that the treating team had suspicion for sepsis prior to a positive screen and had already initiated clinical action. Of the 51 recorded clinical actions taken around the time of a positive screen, the majority (67%) occurred before the screening result. It is also possible that clinical action was not pursued because the treatment team disagreed with a diagnosis of sepsis. Of all the false positive screening cases, manual chart review confirmed that these patients did not have sepsis, nor did they develop sepsis during their index hospital stay. Last, we only recorded clinical actions taken within 8 hours of the first positive screen for sepsis and measured 5 very specific actions. Thus, our analysis may have missed actions taken after 8 hours or actions that differed from the 5 we chose to assess.
Even with the apparently low levels of new clinical activity after a positive screen, when compared to patients who screened negative for sepsis, a significantly higher number of patients who had a positive screen received antibiotics, had lactate measured, and had blood cultures drawn. We did not find a significant difference in the proportion of patients receiving a sepsis‐related clinical action before a screening result (positive or negative), which suggests that a positive screening test may have led to increased clinical action.
A limitation of our study is its small size and that it was conducted in 1 pilot unit. Additionally, our retrospective analysis of clinical care inhibited our ability to fully understand a patient's clinical course or retrieve missing data points. A related limitation is that we could not ascertain how often the screening tool did not identify a case of sepsis before it was clinically diagnosed. Assessing the temporal performance of our screening tool is of great interest and may be more easily performed using an electronic version of the screening tool, which is currently in development.
Using ICD‐9 codes to determine the true‐negative cohort is another limitation of our study. It is well documented that use of administrative data can lead to inaccurate classification of patients.[14] To address this, we performed random audits of 30 test‐negative patients. In doing so we did not find any errors in classification.
Although we did not find a significant difference in screening tool performance between surgical and medical patients, the PPV of the tool was lower in the surgical population (48%) compared to the medical population (70%). The lower PPV observed in surgical patients could be attributable to an overall lower incidence of sepsis in this cohort as well as possible errors in initial assessment of infection, which can be difficult in postsurgical patients. Our retrospective analysis included data from the early months of the screening protocol, a time in which nursing staff was still developing clinical acumen in identifying sepsis. However, this could have led nurses to either overestimate or underestimate the presence of infection in either patient group.
Suspicion for infection is the cornerstone definition of sepsis, and in our screening protocol nurses were charged with making this decision based on their knowledge of the patient's clinical course and current status. Issues concerning nurses' recognition of infection symptoms are an area of opportunity for further research and education and could aid in improving PPV. Clinical judgment could be further bolstered by adding promising laboratory tests such as C‐reactive protein or procalcitonin as objective adjuncts to an initial assessment for sepsis,[15] which could potentially increase screening test PPV.
CONCLUSIONS
A simple screening tool for sepsis performed by the bedside nurse can provide a means to successfully identify sepsis early and lead to more timely diagnostics and treatment in both medical and surgical patients in an intermediate care setting.
ACKNOWLEDGEMENTS
The authors thank Eileen Pummer, quality manager for the sepsis team; Pauline Regner, patient care manager of the pilot study unit; and the nurses who contributed to the screening tool design team and data collection. The authors acknowledge Pooja Loftus for her statistical expertise, and Isabella Chu for her review of the manuscript. Disclosures: Presented as a poster at the 31st Annual Meeting of the Surgical Infection Society, Palm Beach, Florida, May 2011. The authors report no conflicts of interest.
Sepsis remains a significant healthcare burden and is the sixth most common reason for hospitalization in the United States. For patients presenting with severe sepsis, mortality rates are approximately 30%,[1, 2] and sepsis remains the most expensive reason for hospitalization. In 2009, septicemia accounted for nearly $15.4 billion in aggregate hospital costs.[2]
Early identification of sepsis and the timely implementation of goal‐directed therapy significantly decrease sepsis‐related mortality and are cost‐effective,[3, 4, 5] highlighting the need for new clinical strategies to aid in early diagnosis. To date, most studies have focused on the screening and management of sepsis in the emergency department and intensive care unit (ICU),[6, 7] and less is known about the benefits of screening in non‐ICU settings. In the non‐ICU setting, conditions may go unrecognized and treatments delayed. Evidence suggests that patients diagnosed with severe sepsis in the non‐ICU setting are almost twice as likely to die as those diagnosed in an emergency department.[8, 9]
Application of a sepsis screening tool to both medical and surgical patients poses an additional challenge that may impact the screen's performance. The specificity may be compromised by noninfectious causes of systemic inflammatory response syndrome (SIRS) commonly seen in the postsurgical patient. For example, the tachycardia and fever often seen in the postoperative patient are sufficient to qualify for SIRS, making the diagnosis of sepsis more challenging. The purpose of this study was to examine the performance of a nurse‐driven, simple sepsis screening tool in a mixed medical and surgical non‐ICU setting.
METHODS
Setting
This was an observational pilot study of prospectively screened patients admitted to a 26‐bed medical/surgical intermediate care unit with telemetry monitoring in a 613‐bed university tertiary referral hospital over a 1‐month time period. The surgical patient population of this floor consisted of cardiothoracic (50%), general (24%), and vascular surgery (17%) patients as well as a small number of trauma (7%) patients. The medical patient population admitted to this unit included pretransplant and complex medical patients requiring telemetry monitoring. Though the incidence of sepsis specific to this unit was unknown prior to the study, after an analysis of discharges the study team surmised there would be sufficient volume for testing of a nurse‐based screening tool.
Nurse Education
Registered nurses (RNs) working on the study unit had an average of 5 to 7 years of experience. The all‐RN unit was staffed predominantly at a 1:3 RN to patient ratio. RNs were supported by a clinical nurse specialist (CNS) and clinical educator (CE) RN who provided regular ongoing education about infection prevention and identification of common conditions that are seen on the unit.
In the 6 months prior to our sepsis screening initiative, nursing staff had been given more than 8 hours of education on infection‐ and sepsis‐related topics in 15‐ to 20‐minute blocks of time. This dedicated education took place during the nurses' shift in groups of 2 to 3, and was run by the CNS, assistant nurse manager, and CE RN. Nurses were also encouraged to attend an optional 8‐hour sepsis continuing medical education (CME) program. Approximately 20% of the nurses on the study unit attended.
Just prior to the pilot study, nursing staff completed a 1‐hour refresher self‐study module on severe sepsis stressing the importance of early identification. There was also a training month prior to the actual data collection time frame, where unit core trainers (RNs) or champions who had attended the optional 8‐hour sepsis CME conducted 1:1 follow‐up with each RN, reviewing at least 1 of their screens to validate understanding of screening concepts. Each RN was checked off after correctly completing a screen. During the study, unit educators and the CNS provided additional on‐unit in‐service training with screening tool completion instructions and advice on how to incorporate the tool into the RN's current assessment workflow. In addition, the charge nurses were asked to review the screens collected each shift and validate any that may have seemed inconsistent with the RN's verbal report of the patient's status.
The university's institutional review board notice of determination waived review for this study because it was classified as quality improvement.
Screening Tool
A sepsis screening tool was developed as part of a broader initiative to improve sepsis‐related morbidity and mortality at our hospital. The screening tool was adapted from the severe sepsis screening tool created by the Surviving Sepsis Campaign and Institute for Healthcare Improvement,[10] and consisted of a simple 3‐tiered paper‐based screening assessment that was to be completed by the bedside RN (Figure 1). RNs on the pilot medical/surgical intermediate care unit performed the screening assessment with their regular patient assessment at the beginning of each shift.
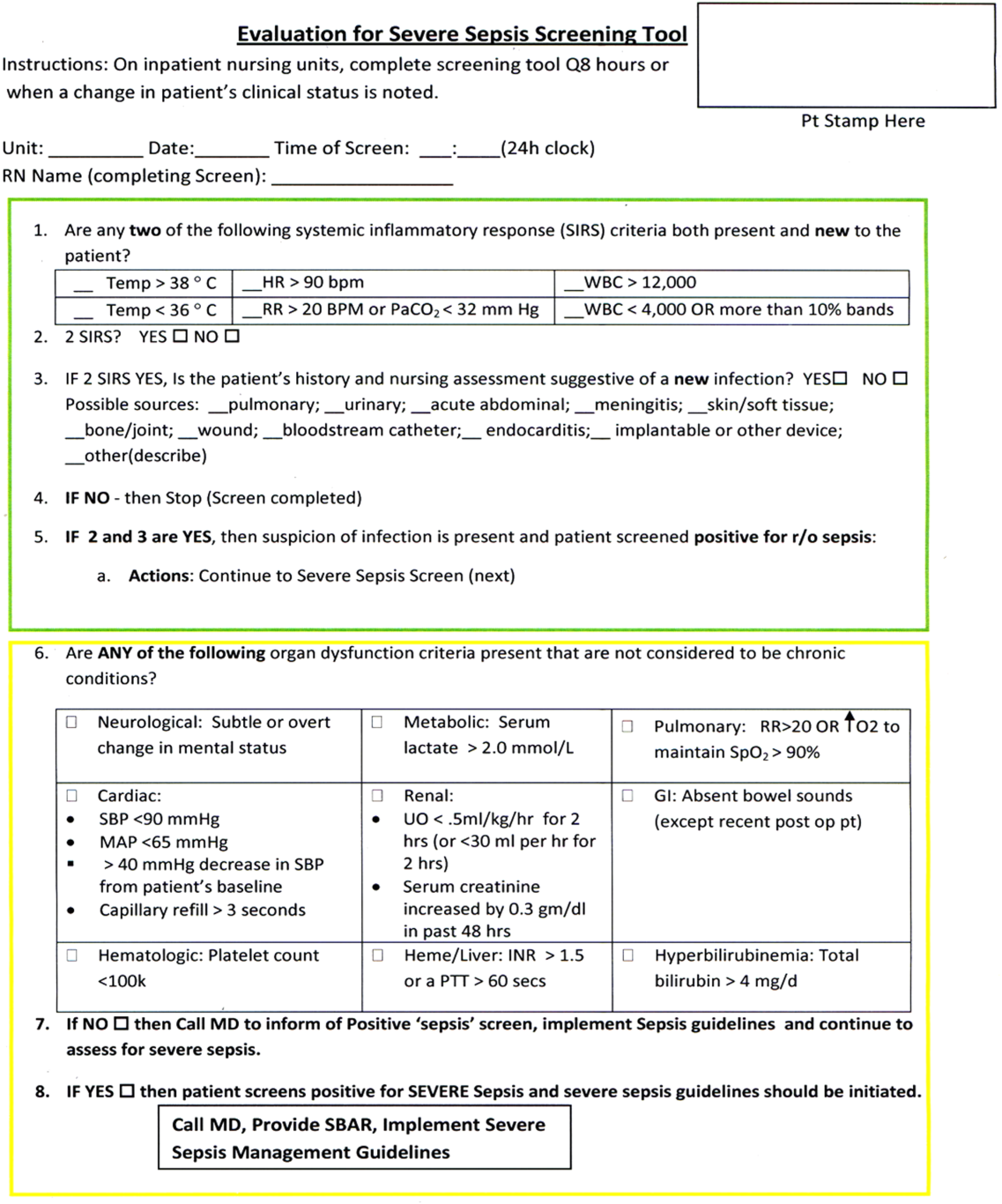
The first tier of the tool screened for the presence of SIRS. Positive parameters included heart rate >90, temperature >38C or <36C, white blood cell count >12,000 or<4000 or >10% bands, and/or respiratory rate >20 or partial pressure of carbon dioxide (PaCO2) <32 mm Hg. To decrease the number of false‐positive screens in patients whose abnormal vitals could already be attributed to a condition other than sepsis, these symptoms were only scored if they had emerged within the previous 8 hours.
If patients met 2 SIRS criteria, the nurse would move to the second tier of the tool, which involved consideration of possible infection as a contributor to a patient's clinical condition as well as a source of infection. If infection was not suspected, further screening was terminated. If infection was suspected, the patient then met criteria for a positive sepsis screen, and a third tier of screening involving assessment of organ dysfunction was initiated.
If the patient screened positive for sepsis (2 SIRS and suspicion for new infection) or severe sepsis (sepsis with end‐organ dysfunction), nurses were instructed to document this in the patient's electronic medical record (EMR) and call the primary team to initiate actions following the hospital‐wide sepsis guidelines. Any subsequent actions were recorded in the patient's EMR.
Data Collection
Completed sepsis screening forms during the month of October 2010 were reviewed by the authors (E.G., L.S., and P.M.). Data including age, gender, International Classification of Diseases, Ninth Revision (ICD‐9) admission and discharge diagnoses, vital signs, lab results, clinical interventions, and documented clinical decision processes by healthcare staff were collected on patients with a positive screen or those who did not screen positive but had an ICD‐9 code for sepsis, severe sepsis, or septic shock during their hospitalization or at discharge. We also collected demographic and clinical data for a random sample of patients who consistently screened negative for sepsis.
Performance Measurement
The sensitivity and specificity of the screening tool was determined by identifying true‐positive, false‐positive, true‐negative, and false‐negative results and calculating accordingly using a 2 2 contingency table. True positives were defined as cases where patients screened positive for sepsis and had a documented diagnosis of sepsis in their EMR within 24 hours of the positive screening or had an ICD‐9 billing code for sepsis. False‐positive cases were those in which patients screened positive for sepsis but did not have a diagnosis of sepsis by manual chart review nor was there an ICD‐9 code for sepsis for their hospital stay. True‐negative cases were those where patients screened negative and did not have an ICD‐9 code for sepsis. False negatives were cases where patients consistently screened negative for sepsis but had an ICD‐9 code for sepsis.
Clinical Activities
To examine the impact of a positive sepsis screen on subsequent clinical action, we assessed the frequency with which a treatment or diagnostic workup was initiated after a positive screen and compared this to clinical activity initiated after a negative screen. Specifically, the patient's EMR was reviewed for actions including measurement of lactate, blood cultures, administration of broad spectrum antibiotics, administration of fluid boluses, or consultation with or transfer to the ICU. These actions were chosen because they are part of the Surviving Sepsis Bundle, which has been demonstrated to improve mortality rates after diagnosis of severe sepsis or septic shock,[11, 12] and can be done outside of an ICU setting. Because screening was done every 8 hours, clinical activity was only attributed to a positive or negative sepsis screen if it occurred within 8 hours of the screening result. Patients were excluded if there were missing data points that precluded full analysis of their clinical course.
Statistical Analysis
To compare the performance of the screening tool between surgical and medical patients, we calculated 95% confidence intervals of screening test sensitivity and specificity. To test if performance was significantly different between these groups, we performed a nonparametric, 2‐sided, 2‐sample test of proportions. Though similar to a [2] test, the 2‐sided test of proportions allowed us to determine if there was a directional difference in test performance (ie, Does the screening tool perform better or worse in a certain patient group?). We also used the test of proportions to compare differences in the proportion of patients receiving sepsis‐related interventions before and after a positive or negative screening result. For comparisons of demographic variables we used nonparametric tests including the [2] test for categorical variables and the Kruskal‐Wallis test for continuous variables. We used SAS 9.3 (SAS Institute Inc., Cary, NC) to perform our analyses.
RESULTS
Over a 1‐month time period, 2143 screens were completed on 245 patients (169 surgical, 76 medical). The overall incidence of sepsis on the treatment unit during this time period was 9%. Surgical patients had an 8.9% incidence of sepsis, and medical patients had an incidence of 9.2%.
Screening tool performance is presented in Table 1. The screening tool had 95.5% sensitivity and 91.9% specificity, with no significant differences in performance between surgical and medical patients. The overall negative predictive value was 99.5%, also with comparable performance in both surgical and medical patients (P = 0.89). The overall positive predictive value (PPV) was 70% in medical patients and 48% in surgical patients (P = 0.12). Screening tool accuracy for medical and surgical patients was 92%.
Overall, N = 245 (95% CI) | Surgery, N = 169 (95% CI) | Medicine, N = 76 (95% CI) | P Value* | |
---|---|---|---|---|
| ||||
Sensitivity | 95.5% (75%‐99.7%) | 93% (66%‐99.6%) | 100% (56%‐100%) | 0.17 |
Specificity | 91.9% (87%‐95%) | 90% (84%‐94%) | 95% (87%‐99%) | 0.48 |
NPV | 99.5% (81%‐100%) | 99.3% (71%‐100%) | 100% (67%‐100%) | 0.89 |
PPV | 53.8% (39%‐70%) | 48% (23%‐73%) | 70% (30%‐100%) | 0.12 |
LR+ | 11.8 | 9.3 | 20 | |
LR | 0.05 | 0.08 | 0 | |
Confirmed patient diagnosis, overall | ||||
Sepsis | No sepsis | |||
Screen positive | 21 (TP) | 18 (FP) | ||
Screen negative | 1 (FN) | 205 (TN) | ||
Confirmed patient diagnosis, medicine | ||||
Sepsis | No sepsis | |||
Screen positive | 7 (TP) | 3 (FP) | ||
Screen negative | 0 (FN) | 66 (TN) | ||
Confirmed patient diagnosis, surgery | ||||
Sepsis | No sepsis | |||
Screen positive | 14 (TP) | 15 (FP) | ||
Screen negative | 1 (FN) | 139 (TN) |
Clinical Activities
Of the 39 patients who screened positive for sepsis, nurses classified 20 with sepsis and 19 with severe sepsis. Of these 39 patients, 33 were included in our descriptive analysis of the effect of positive screening results on clinical activity (3 were excluded for admission for sepsis and 3 for missing data). As a comparison, we randomly selected 30 patients of the 206 patients who screened negative for sepsis to evaluate clinical activity before and after a negative screen.
Characteristics of patients screening positive and negative for sepsis are reported in Table 2. We found no statistically significant differences in age, sex, length of hospital stay, or mortality amongst all groups.
Patient Characteristics | Surgery (Positive) | Medicine (Positive) | Surgery (Negative) | Medicine (Negative) | P Value |
---|---|---|---|---|---|
| |||||
No. | 26 | 7 | 20 | 10 | |
Age, y, mean | 57.8 ( 16.5) | 72.4 ( 16.8) | 64.6 ( 19.4) | 63.6 ( 16.8) | 0.25 |
% Male (no.) | 50% (13) | 57% (4) | 60% (12) | 60% (6) | 0.27 |
Length of stay, d, median (IQR) | 9 (716.7) | 7 (5.511.5) | 11 (7.722) | 8 (421) | 0.38 |
No. of PODs until first positive screen, d, median (IQR) | 2 (13) | N/A | N/A | N/A | |
% Mortality (no.) | 0% | 14% (1) | 5% (1) | 10% (1) | 0.19 |
Figure 2 illustrates differences in the proportion of patients receiving a clinical action before and after a negative or positive screening test result. In the cohort of 33 patients screening positive for sepsis, clinical action after a positive screen was taken in 4 of the 7 (50%) medical patients and 11 of 26 (42%) surgical patients. In patients screening negative for sepsis we found only 1 incident in which a sepsis‐related action was taken after a negative screen. In this case the patient was admitted to the ICU within 8 hours of a negative screen, though there was no explicit documentation that sepsis was the reason for this admission.
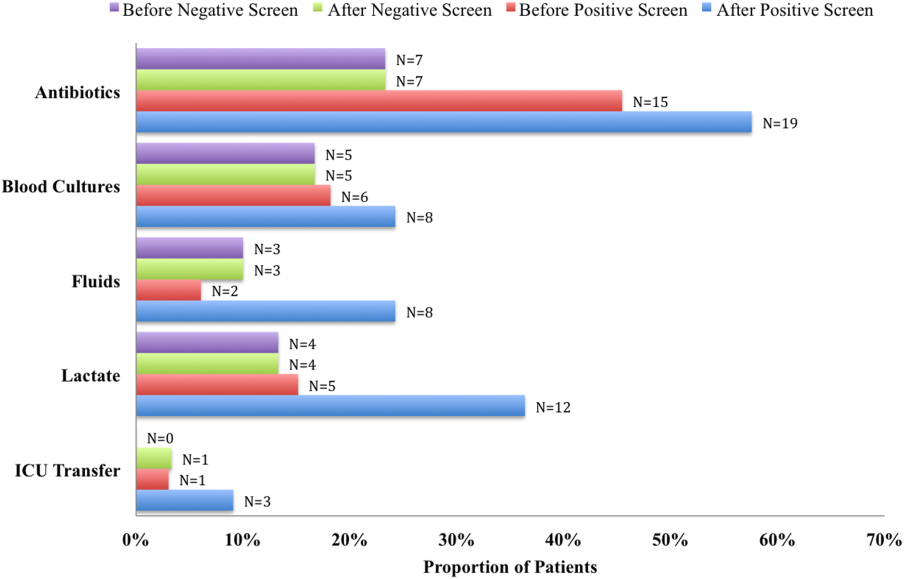
We compared the proportion of patients receiving sepsis‐related treatment before either a negative or positive screen and found no significant difference (Table 3). We then compared the proportion of patients receiving sepsis‐related actions after a positive or negative screening test result and found that the proportion of patients receiving antibiotics, blood cultures, and lactate measurement was significantly higher for patients with a positive sepsis screening result compared to those with a negative screening result (Table 3).
Intervention and Group | Proportion | P Value |
---|---|---|
| ||
Before screening test | ||
Antibiotics | 0.066 | |
Positive screen | 45% | |
Negative screen | 23% | |
Lactate | 0.837 | |
Positive screen | 15% | |
Negative screen | 13% | |
Blood culture | 0.181 | |
Positive screen | 18% | |
Negative screen | 17% | |
Fluid administration | 0.564 | |
Positive screen | 6% | |
Negative screen | 10% | |
ICU transfer/consult | 0.337 | |
Positive screen | 3% | |
Negative screen | 0% | |
After screening test | ||
Antibiotics | 0.006 | |
Positive screen | 58% | |
Negative screen | 23% | |
Lactate | 0.018 | |
Positive screen | 36% | |
Negative screen | 13% | |
Blood Culture | 0.002 | |
Positive screen | 24% | |
Negative screen | 17% | |
Fluid administration | 0.112 | |
Positive screen | 24% | |
Negative screen | 10% | |
ICU transfer/consult | 0.175 | |
Positive screen | 9% | |
Negative screen | 3% |
DISCUSSION
Improving recognition and time to treatment of sepsis in a non‐ICU setting is an important step toward decreasing sepsis‐related mortality. Lundberg and colleagues found that mortality rates for patients diagnosed with septic shock on a general ward were higher than for patients diagnosed in the ICU, even though ward patients were younger and healthier at baseline.[8] For ward patients, treatment delays were most profound in initiating vasoactive therapies, and minor delays were encountered in initiating fluid resuscitation. In their international study on the impact of early goal‐directed therapy guidelines, Levy and colleagues found that patients diagnosed with severe sepsis on the wards were almost twice as likely to die as patients diagnosed with sepsis in the emergency department.[9]
We are the first to report about an accurate nurse‐driven SIRS‐based sepsis screening protocol that is effective in the early identification of sepsis in both medical and surgical patients in an intermediate care setting. We found no significant difference in the screening tool performance between the medical and surgical cohorts. This is an important comparison given that SIRS criteria alone can be nonspecific in the postoperative population, where it is common to have hemodynamic changes, elevation of inflammatory markers, and fevers from noninfectious sources.
Our sepsis screening tool was designed in 3 tiers to improve its specificity. The first tier was based strictly on SIRS criteria (eg, tachycardia or fever), whereas the second and third tiers served to increase the specificity of the screening tool by instructing the evaluator to assess possible sources of infection and assess for objective signs of organ dysfunction. We relied heavily on the nursing staff to assess for the presence or absence of infection and believe that the educational component prior to initiating the screening protocol was vital.
EMR‐based screening tools that rely purely on physiologic data have been considered for the early detection and management of sepsis, although they lack the specificity gained through the incorporation of clinical judgment.[13] Sawyer and colleagues report using a real‐time EMR‐based method for early sepsis detection in non‐ICU patients that is based solely on objective measures; however, their PPV was only 19.5%. The model we describe in this study is one that incorporates real‐time physiologic data available from an EMR coupled with the clinical judgment of a bedside registered nurse. As our data suggest, this provides a screen that is both sensitive and specific.
It is interesting to note that in our assessment of clinical action taken 8 hours after a positive screening test (the interval after which a new screening test was performed), the rate of diagnostic workup and/or treatment for sepsis was relatively low. One reason for this could have been that the treating team had suspicion for sepsis prior to a positive screen and had already initiated clinical action. Of the 51 recorded clinical actions taken around the time of a positive screen, the majority (67%) occurred before the screening result. It is also possible that clinical action was not pursued because the treatment team disagreed with a diagnosis of sepsis. Of all the false positive screening cases, manual chart review confirmed that these patients did not have sepsis, nor did they develop sepsis during their index hospital stay. Last, we only recorded clinical actions taken within 8 hours of the first positive screen for sepsis and measured 5 very specific actions. Thus, our analysis may have missed actions taken after 8 hours or actions that differed from the 5 we chose to assess.
Even with the apparently low levels of new clinical activity after a positive screen, when compared to patients who screened negative for sepsis, a significantly higher number of patients who had a positive screen received antibiotics, had lactate measured, and had blood cultures drawn. We did not find a significant difference in the proportion of patients receiving a sepsis‐related clinical action before a screening result (positive or negative), which suggests that a positive screening test may have led to increased clinical action.
A limitation of our study is its small size and that it was conducted in 1 pilot unit. Additionally, our retrospective analysis of clinical care inhibited our ability to fully understand a patient's clinical course or retrieve missing data points. A related limitation is that we could not ascertain how often the screening tool did not identify a case of sepsis before it was clinically diagnosed. Assessing the temporal performance of our screening tool is of great interest and may be more easily performed using an electronic version of the screening tool, which is currently in development.
Using ICD‐9 codes to determine the true‐negative cohort is another limitation of our study. It is well documented that use of administrative data can lead to inaccurate classification of patients.[14] To address this, we performed random audits of 30 test‐negative patients. In doing so we did not find any errors in classification.
Although we did not find a significant difference in screening tool performance between surgical and medical patients, the PPV of the tool was lower in the surgical population (48%) compared to the medical population (70%). The lower PPV observed in surgical patients could be attributable to an overall lower incidence of sepsis in this cohort as well as possible errors in initial assessment of infection, which can be difficult in postsurgical patients. Our retrospective analysis included data from the early months of the screening protocol, a time in which nursing staff was still developing clinical acumen in identifying sepsis. However, this could have led nurses to either overestimate or underestimate the presence of infection in either patient group.
Suspicion for infection is the cornerstone definition of sepsis, and in our screening protocol nurses were charged with making this decision based on their knowledge of the patient's clinical course and current status. Issues concerning nurses' recognition of infection symptoms are an area of opportunity for further research and education and could aid in improving PPV. Clinical judgment could be further bolstered by adding promising laboratory tests such as C‐reactive protein or procalcitonin as objective adjuncts to an initial assessment for sepsis,[15] which could potentially increase screening test PPV.
CONCLUSIONS
A simple screening tool for sepsis performed by the bedside nurse can provide a means to successfully identify sepsis early and lead to more timely diagnostics and treatment in both medical and surgical patients in an intermediate care setting.
ACKNOWLEDGEMENTS
The authors thank Eileen Pummer, quality manager for the sepsis team; Pauline Regner, patient care manager of the pilot study unit; and the nurses who contributed to the screening tool design team and data collection. The authors acknowledge Pooja Loftus for her statistical expertise, and Isabella Chu for her review of the manuscript. Disclosures: Presented as a poster at the 31st Annual Meeting of the Surgical Infection Society, Palm Beach, Florida, May 2011. The authors report no conflicts of interest.
- Epidemiology of severe sepsis in the United States: analysis of incidence, outcome, and associated costs of care. Crit Care Med. 2001;29(7):1303–1310. , , , , ,
- http://www.hcup-us.ahrq.gov/reports/statbriefs/sb122.pdf. Published October 2011. Accessed on September 4, 2012. , , Septicemia in U.S. hospitals, 2009. HCUP statistical brief #122. Agency for Healthcare Research and Quality. Available at:
- Economic implications of an evidence‐based sepsis protocol: can we improve outcomes and lower costs? Crit Care Med. 2007;35(5):1257–1262. , , ,
- Late compliance with the sepsis resuscitation bundle: impact on mortality. Shock. 2011;36(6):542–547. , , , , ,
- The costs and cost‐effectiveness of an integrated sepsis treatment protocol. Crit Care Med. 2008;36(4):1168–1174. , , , , ,
- A simple prediction algorithm for bacteraemia in patients with acute febrile illness. QJM. 2005;98(11):813–820. , ,
- Validation of a screening tool for the early identification of sepsis. J Trauma. 2009;66(6):1539–1546; discussion 1546–1547. , , , et al.
- Septic shock: an analysis of outcomes for patients with onset on hospital wards versus intensive care units. Crit Care Med. 1998;26(6):1020–1024. , , , et al.
- The Surviving Sepsis Campaign: results of an international guideline‐based performance improvement program targeting severe sepsis. Crit Care Med. 2010;38(2):367–374. , , , et al.
- Institute of Healthcare Improvement. Evaluation for severe sepsis screening tool. Surviving Sepsis Campaign. Available at: http://www.survivingsepsis.org/About_the_Campaign/Documents/evaluationforseveresepsisscreeningtool.pdf. Accessed on September 30, 2012.
- Impact of the Surviving Sepsis Campaign protocols on hospital length of stay and mortality in septic shock patients: results of a three‐year follow‐up quasi‐experimental study. Crit Care Med. 2010;38(4):1036–1043. , , , et al.
- Reduction of the severe sepsis or septic shock associated mortality by reinforcement of the recommendations bundle: a multicenter study. Ann Fr Anesth Reanim. 2010;29(9):621–628. , , , et al.
- Implementation of a real‐time computerized sepsis alert in nonintensive care unit patients. Crit Care Med. 2011;39(3):469–473. , , , et al.
- Accuracy of administrative data for identifying patients with pneumonia. Am J Med Qual. 2005;20(6):319–328. , , ,
- Comparison of procalcitonin and C‐reactive protein as markers of sepsis. Crit Care Med. 2003;31(6):1737–1741. , , , , ,
- Epidemiology of severe sepsis in the United States: analysis of incidence, outcome, and associated costs of care. Crit Care Med. 2001;29(7):1303–1310. , , , , ,
- http://www.hcup-us.ahrq.gov/reports/statbriefs/sb122.pdf. Published October 2011. Accessed on September 4, 2012. , , Septicemia in U.S. hospitals, 2009. HCUP statistical brief #122. Agency for Healthcare Research and Quality. Available at:
- Economic implications of an evidence‐based sepsis protocol: can we improve outcomes and lower costs? Crit Care Med. 2007;35(5):1257–1262. , , ,
- Late compliance with the sepsis resuscitation bundle: impact on mortality. Shock. 2011;36(6):542–547. , , , , ,
- The costs and cost‐effectiveness of an integrated sepsis treatment protocol. Crit Care Med. 2008;36(4):1168–1174. , , , , ,
- A simple prediction algorithm for bacteraemia in patients with acute febrile illness. QJM. 2005;98(11):813–820. , ,
- Validation of a screening tool for the early identification of sepsis. J Trauma. 2009;66(6):1539–1546; discussion 1546–1547. , , , et al.
- Septic shock: an analysis of outcomes for patients with onset on hospital wards versus intensive care units. Crit Care Med. 1998;26(6):1020–1024. , , , et al.
- The Surviving Sepsis Campaign: results of an international guideline‐based performance improvement program targeting severe sepsis. Crit Care Med. 2010;38(2):367–374. , , , et al.
- Institute of Healthcare Improvement. Evaluation for severe sepsis screening tool. Surviving Sepsis Campaign. Available at: http://www.survivingsepsis.org/About_the_Campaign/Documents/evaluationforseveresepsisscreeningtool.pdf. Accessed on September 30, 2012.
- Impact of the Surviving Sepsis Campaign protocols on hospital length of stay and mortality in septic shock patients: results of a three‐year follow‐up quasi‐experimental study. Crit Care Med. 2010;38(4):1036–1043. , , , et al.
- Reduction of the severe sepsis or septic shock associated mortality by reinforcement of the recommendations bundle: a multicenter study. Ann Fr Anesth Reanim. 2010;29(9):621–628. , , , et al.
- Implementation of a real‐time computerized sepsis alert in nonintensive care unit patients. Crit Care Med. 2011;39(3):469–473. , , , et al.
- Accuracy of administrative data for identifying patients with pneumonia. Am J Med Qual. 2005;20(6):319–328. , , ,
- Comparison of procalcitonin and C‐reactive protein as markers of sepsis. Crit Care Med. 2003;31(6):1737–1741. , , , , ,
© 2014 Society of Hospital Medicine
Secure Texting Improves Hospital Communication
Pagers, though reliable and familiar technology, can be suboptimal for facilitating healthcare team communication.[1, 2] Most paging systems utilize single‐function pagers and only allow one‐way communication, requiring recipients to disrupt workflow to respond to pages. Paging transmissions can also be intercepted, and the information presented on pager displays can be viewed by anyone in possession of the pager.
Smartphones allow for instantaneous two‐way and group communication through advanced technological features. Their use is widespread; over 81% of American physicians owned a smartphone in 2011.[3] Previous studies demonstrate that healthcare providers rate smartphone‐based email positively, and that team smartphones can facilitate communication between nurses and physicians.[4, 5] However, these studies specifically examined the utility of smartphone‐based email and voice calls, and did not include text messaging. Limitations of traditional smartphone‐based text messaging include Health Insurance Portability and Accountability Act (HIPAA) noncompliance and dependence on in‐hospital cellular reception, which can be unreliable. HIPAA is a 1996 US federal law that established a set of privacy and security rules governing not only what is considered protected health information (PHI), but also minimum standards for the protection of such information. HIPAA compliance is defined as meeting these minimum standards for physical, network, and process security.[6, 7] Though PHI is often transmitted via paging systems and commercial carrier‐based text messaging, these modalities are not secure and are thus not HIPAA‐compliant.
Text messaging applications that address these security and reliability issues have the potential to greatly enhance in‐hospital communication. We hypothesized that a smartphone‐based HIPAA‐compliant group messaging application could improve in‐hospital communication on the inpatient medicine service. To our knowledge, our study is the first to examine a HIPAA‐compliant text messaging system, and also the first to compare a combination paging/HIPAA‐compliant group messaging (HCGM) system with a paging‐only system in assessing healthcare provider perception of communication efficiency.
METHODS
Intervention
This study utilized Medigram (Medigram, Inc.,
Study Design
In an eight‐week prospective, cluster‐randomized, controlled trial conducted at Stanford Hospital (June 25, 2012August 17, 2012), three of five inpatient medicine teams were randomized to use Medigram in addition to the existing hospital paging system (HCGM teams); the remaining two teams were assigned to use hospital paging only (control teams). Each team included one attending physician, one resident, two interns, two medical students, and a case manager. According to prescheduled rotations, attendings rotated every two weeks, and residents, interns, and medical students rotated every four weeks. All rotations were either off‐service or off‐site, with the exception of two attendings who rotated between study teams but within their experimental designations. Case managers remained with the same team. Additionally, the satellite pharmacy was provided with an HCGM‐equipped smartphone to communicate with experimental teams.
Participation was voluntary, with a 96% participation rate (n=75). HCGM teams downloaded the free application onto their smartphones. Participants without smartphones were provided with one for the duration of the study. Proper application use was demonstrated by one researcher in a 10‐minute standardized presentation. HCGM teams were encouraged to use the application in lieu of paging, except when patient care could be compromised.
All participants completed linked baseline and post‐study surveys. Gift cards valued at $10 were provided on completion of each survey. Though participants were assigned to either HCGM or control groups based on the randomized assignment of their preset cluster (hospital team) to an HCGM or control group, analysis was performed on the individual level due to the hospital's set rotation schedule, which resulted in dynamic, frequently changing clusters. We also compared average length of stay and time of discharge for patients treated by control versus HCGM teams. Clinical outcome data were obtained from the hospital's database using Midas+ Statit Solutions (Midas+ Statit Solutions Group, Tucson, AZ). Survey and clinical outcome data were analyzed in Stata (StataCorp, College Station, TX) and R (R Foundation for Statistical Computing, Vienna, Austria).
Survey Design and Analysis
Identical, anonymous baseline surveys were administered to control and HCGM teams. These surveys assessed attitudes toward the hospital paging system using a 5‐point Likert scale (1=low, 5=high) to evaluate perceived measures of effectiveness, workflow integration, and overall satisfaction. Wilcoxon rank sum tests were used to compare control and HCGM group responses to these questions. Free response questions asked participants to list the most effective and ineffective aspects of the paging system.
Post‐study surveys included all baseline survey questions, as well as questions about personal texting behavior. Post‐study HCGM surveys also included a parallel set of questions rating the HCGM application on the same measures of perceived effectiveness, workflow integration, and overall satisfaction. Wilcoxon signed rank tests were used to compare HCGM participants' baseline evaluations of paging to their post‐study evaluations of the HCGM application. Baseline and post‐study surveys were linked by the last four digits of respondent cell phone numbers. To compare control and HCGM group perceptions of the hospital paging system at study completion, post‐study survey responses were evaluated using Wilcoxon rank sum tests. The family‐wise error rate was left unadjusted due to concerns around inflated type II errors, given the high degree of correlation between survey questions.
All free response questions were analyzed using thematic analysis and grounded theory. After reviewing responses to each question, a list of overarching themes was constructed. Two researchers then independently reviewed each free‐response entry to assign it to one or more of these themes (some responses included several ideas with distinct themes). Entries with concordant theme assignments (90%) were coded as such; nonconcordant entries required an additional round of review to reach concordance. Finally, objective outcome measures including length of stay and time of discharge were analyzed by two‐sample t test.
Control Group | HCGM Group | |
---|---|---|
| ||
Paired surveys collected (completion rate) | 22 (85%) | 41 (84%) |
Average age 95% CI | 30.10 1.71 | 30.95 2.94 |
Gender | ||
Male | 13 (59%) | 24 (59%) |
Female | 9 (41%) | 17 (41%) |
Role | ||
Medical students | 6 (27%) | 11 (27%) |
Interns (PGY 1) | 7 (32%) | 12 (29%) |
Residents (PGY2 and 3) | 3 (14%) | 6 (15%) |
Attending physicians | 5 (23%) | 5 (12%) |
Case managers | 1 (5%) | 3 (7%) |
Pharmacists | 0 (0%) | 4 (10%) |
Information Security
The HCGM application in this study features 256‐bit encryption technology and requires a six‐digit password to access texts. For added security, a study‐dedicated server (HP ProLiant DL 180 G6; Hewlett‐Packard Co., Palo Alto, CA) with 4‐TB hard drive capacity (4 Seagate Barracuda ST1000DM003 1 TB 7200 RPM internal hard drives; Seagate Technology PLC, Cupertino, CA) was installed in the Stanford School of Medicine Data Center to store encrypted text messages. Data stored on the phones/server were accessible only to study participants, not researchers. These security measures were approved by Stanford Hospital and Stanford School of Medicine's security and privacy review process.
Hospital Paging System
Stanford Hospital and Clinics is a quaternary care academic medical center with 613 beds, 49 operating rooms, and over 25,000 inpatient admissions per year.[8] The institution uses one‐way alphanumeric pagers (primary model: Daviscomm BR802 Flex Pager from USA Mobility, secondary model: Sun Telecom Titan 3 Plus from USA Mobility; USA Mobility Inc., Springfield, VA). USA Mobility operates the largest one‐ and two‐way paging networks in the United States.[9]
RESULTS
Of 26 control and 49 HCGM group members participating in the study, linked baseline and post‐study surveys were collected for 22 control and 41 HCGM participants (completion rates of 84.6% and 83.7%, respectively). To minimize recall bias, surveys not completed within a prespecified timeframe upon entering or leaving a team (two days attendings, four days others) were excluded.
Control and HCGM Group Characteristics
Control and HCGM groups were well matched demographically (Table 1). The average ages of control and HCGM group members were 30.10 and 30.95, respectively. Both groups were 59% male and 41% female.
What do you find effective about the current hospital paging system? | What do you find ineffective about the current hospital paging system? | ||||
---|---|---|---|---|---|
Theme | No. of Respondents, (% of Total) | Response Example | Theme | No. of Respondents, (% of Total) | Response Example |
| |||||
Reliability of message transmission | 17 (30.4%) | Everyone is able to receive the pages I send, regardless of service | Time wasted waiting for a response | 17 (29.3%) | Inefficient use of time waiting for reply |
Ability to text page | 14 (25.0%) | Text paging allows targeted questions | One‐way nature of communication | 14 (24.1%) | Cannot text back instantly |
Ease of use | 8 (14.3%) | Easy to use | Needing to find a computer to send a text page | 12 (20.7%) | Have to find an available computer to send a page |
Search function | 5 (8.9%) | Search function is pretty effective in finding the people you're looking for | Character limitation | 10 (17.2%) | Length of text allowed too short |
Ubiquity | 5 (8.9%) | Everyone is on paging system | Search function | 6 (10.3%) | Delay in looking people up in the system |
Speed | 4 (7.1%) | Fast | Finding a phone to return a page | 5 (8.6%) | When you receive a page you need to find a phone |
Loud alerts | 4 (7.1%) | Pager loud enough to hear all the time | Receipt of page uncertain | 3 (5.2%) | Unknown if page received |
Staff responsiveness to pages | 4 (7.1%) | I know MD has to be onsite or covering the pager so someone eventually will call back | Sender's pager number not always included in page | 3 (5.2%) | Not everyone puts their pager number when they page. Then it's impossible to get back to them. |
Brevity of messages | 3 (5.4%) | Requires very brief messages (easier for recipient) | Needing to remain near a phone while waiting for a page response | 3 (5.2%) | Wait by a phone for someone to call back; sometimes they do not call back |
Helpful page operators | 2 (3.6%) | Page operators very helpful | Reliability of message transmission | 3 (5.2%) | Sometimes messages don't go through |
Other | 10 (17.9%) | It's online and allows paging from anywhere there's internet access | Other | 11 (19.0%) | You cannot text with patient info on it |
A similar distribution of team member roles was observed in both groups, with two exceptions. First, the proportion of attending respondents in the HCGM group was lower than in the control group. This was due to the fact that several HCGM attendings entered discrepant ID codes on their surveys, thus making it impossible to link baseline and post‐study responses; these data were excluded. Additionally, two HCGM attendings were on service for four, rather than the standard two weeks, meaning two additional data points from unique attendings could not be obtained. Second, the experimental group included four pharmacists, whereas the control group did not. As a sensitivity test, we analyzed the data excluding the pharmacists, and this did not change our results.
Baseline Evaluations of the Hospital Paging System
At baseline, there were no significant differences between control and HCGM participants' perceptions of paging effectiveness (see Supporting Table 1, in the online version of this article). On a 5‐point rating scale (1=low, 5=high), 63 subjects rated their overall satisfaction with the paging system an average of 2.79 (95% confidence interval: 2.55‐3.03).
In free response questions, components of the paging system most frequently cited as effective included: reliability of message transmission, alphanumeric text paging, and ease of use (30.4%, 25.0%, and 14.3% of 56 respondents, respectively) (Table 2). Ineffective aspects included: time wasted waiting for responses to pages, the unidirectional nature of pagers, and needing to find a computer to send a text page (29.3%, 24.1%, and 20.7% of 58 respondents, respectively) (Table 2).
Question | Baseline Average Rating of Paging System* | Post‐Study Average Rating of HCGM Application | P Value |
---|---|---|---|
| |||
Rate the effectiveness of each in allowing you to | |||
Communicate your thoughts clearly | 3.194 | 3.806 | 0.010 |
Communicate your thoughts efficiently | 3.200 | 3.829 | 0.009 |
Send messages to other hospital staff | 3.543 | 3.571 | 0.480 |
Receive messages/stay informed in real time | 3.222 | 3.306 | 0.405 |
Rate the effectiveness of each in integrating into your workflow during | |||
Work rounds | 2.313 | 3.000 | 0.018 |
Patient discharge | 2.448 | 3.276 | 0.012 |
Patient admissions | 2.862 | 2.621 | 0.238 |
Teaching sessions | 2.292 | 2.458 | 0.448 |
Overall satisfaction | 2.811 | 3.459 | 0.003 |
Baseline Utilization of Text Messaging
The majority of participants were familiar with text messaging and regularly used it personally and professionally prior to the start of the study. 90.5% of participants (n = 63) reported sending an average of 1 personal text messages per day, with the largest proportion (39.7%) sending 1‐5 texts per day (see Supporting Figure 1A in the online version of this article). 58.1% of respondents (n=62) reported sending an average of 1 text messages per day related to patient care (see Supporting Figure 1B in the online version of this article), with the largest fraction (58.3%) sending 1‐5 texts per day.
HCGM Adoption and Usage Patterns
Active use of HCGM was defined as using the application to send or receive an average of 1 text messages per day. Of HCGM participants, 67% self‐reported 1 week of active use of the application, indicating a strong compliance rate. Among non‐attendings, 70% reported sending 1 or more texts to other team members per day; this percentage increased to 86% among those whose attendings texted them at least once per day (47% of non‐attendings). Respondents who text frequently in their personal lives (>5 texts/day) were more likely to use the application; 90% of these respondents sent 1 or more HCGM texts per day.
Among 12 subjects who did not report sending or receiving 1 HCGM text/day, the top three reasons were: other team members were not using it (67%), no need to use it given the close proximity of other team members (67%), and other (33%). A Wilcoxon rank sum test was used to compare the ages of active versus nonactive users; no significant age difference was found (P=0.200).
To provide an objective measure of application adoption, usage data for each HCGM participant were obtained from the application developers. Because much of the study's first week was spent onboarding and instructing participant, the first week was not included in the analysis. Of 43 individuals enrolled in the study for at least one of the seven remaining weeks, 56% sent a total of 5 texts, 44% sent 10 texts, and 28% sent 20 texts. HCGM users on three teams sent an aggregate mean of 123 texts/week. Data on number of messages received by each user were not available.
Perceived Effectiveness: Paging Versus HCGM
In post‐study surveys, HCGM participants rated HCGM significantly higher (P<0.05) than paging (Table 3) in terms of ability to communicate thoughts clearly (P=0.010) and efficiently (P=0.009). HCGM was also deemed more effective at integrating into workflow during rounds (P=0.018) and patient discharge (P=0.012). Overall satisfaction with HCGM was also significantly higher (P=0.003).
Control (n=22) | HCGM (n=41) | |||||
---|---|---|---|---|---|---|
Baseline Mean | Post‐Study Mean | P Value* | Baseline Mean | Post‐Study Mean | P Value* | |
| ||||||
Rate the effectiveness of each in allowing you to | ||||||
Communicate your thoughts clearly | 2.905 | 2.619 | 0.103 | 3.250 | 2.850 | 0.004 |
Communicate your thoughts efficiently | 2.952 | 2.762 | 0.106 | 3.250 | 2.825 | 0.018 |
Send messages to other hospital staff | 3.762 | 3.190 | 0.019 | 3.550 | 3.450 | 0.253 |
Receive messages/stay informed in real time | 3.667 | 2.857 | 0.002 | 3.300 | 2.900 | 0.031 |
Rate the effectiveness of each in integrating into your workflow during | ||||||
Work rounds | 2.429 | 2.476 | 0.303 | 2.410 | 2.718 | 0.078 |
Patient discharge | 2.500 | 2.350 | 0.251 | 2.472 | 2.861 | 0.071 |
Patient admissions | 2.905 | 2.524 | 0.020 | 2.889 | 3.000 | 0.384 |
Teaching sessions | 2.143 | 2.200 | 0.386 | 2.367 | 2.400 | 0.418 |
Comparison of Pre‐ and Post‐study Perceived Effectiveness of the Hospital Paging System
In post‐study evaluations, both control and HCGM participants rated the paging system's effectiveness less favorably (P<0.05) compared to baseline in terms of ability to receive messages/stay informed in real time (control P=0.002, HCGM P=0.031) (Table 4). Controls also reported a decrease from baseline in perceived effectiveness of paging in terms of ability to send messages (P=0.019) and integrate into workflow during patient admissions (P=0.020). HCGM participants found paging less effective at communicating thoughts clearly (P=0.004) and efficiently (P=0.018). No significant differences existed between control and HCGM groups' average assessments of paging at the conclusion of the study (see Supporting Table 2, in the online version of this article).
What do you find effective about the Medigram system? | What do you find ineffective about the Medigram system? | ||||
---|---|---|---|---|---|
Theme | No. of Respondents, (% of Total) | Response Example | Theme | No. of Respondents, (% of Total) | Response Example |
| |||||
Ease of use | 11 (32.4%) | Easy to use | Lack of ubiquity | 10 (30.3%) | Not enough people using it |
Group texting feature | 11 (32.4%) | Ability to communicate with entire teameveryone seeing same message | Inconsistent usage | 8 (24.2%) | No one used it reliably |
Speed | 8 (23.5%) | Faster than a page to send a message | Reliability of message transmission | 5 (15.2%) | Big negative is it requires Wi‐Fi |
Accessibility | 5 (14.7%) | Able to get messages across quickly and anywhere without a computer | Missed message alerts | 4 (12.1%) | Unable to reliably know message was received if phone on silent |
Efficiency | 4 (11.8%) | Very efficient way to communicate | Password login | 3 (9.1%) | Having to type a 6‐digit password in |
Real‐time communication | 2 (5.9%) | Real‐time results | User interface | 2 (6.1%) | Interface is a little convoluted |
No character limitation | 2 (5.9%) | No limit on words | Other | 10 (30.3%) | Not sure if all of the texts were relevant |
Other | 4 (11.8%) | Great UI |
HCGM User Experience
When asked if they would recommend using an HCGM system to facilitate communication on the internal medicine wards, 85% of HCGM participants replied yes, 15% reported not sure, and 0% reported no. Based on free response entries, HCGM's most effective features (Table 5) included ease of use, group texting capacity, and speed (32.4%, 32.4%, and 23.5% of 34 respondents, respectively); its most ineffective aspects (Table 5) included lack of ubiquity, inconsistent usage by those with access to the application, and reliability of message transmission (30.3%, 24.2%, and 15.2% of 33 respondents, respectively).
DISCUSSION
We are the first to report that smartphone‐based, HIPAA‐compliant, group messaging applications improve provider perception of in‐hospital communication, while providing the information security that paging and commercial cellular networks do not. HCGM participants rated the application more favorably than paging in terms of clarity and efficiency of communication. These findings may be attributed to the expanded functionality offered by the application, including no character limit per HCGM text, the ability to use special characters such as slashes and ampersands, group texting, and the ability to reply immediately. HCGM may result in more efficient communication by facilitating direct two‐way communication via smartphones, whereas sending or returning pages requires a landline or computer.
HCGM participants rated the application higher than paging in terms of workflow integration during rounds and patient discharge, but not during patient admissions and teaching sessions. We had hypothesized that HCGM would integrate better into participants' workflows because HCGM texts could be replied to immediately. The reasons for the equivalence of HCGM and paging for workflow integration during patient admissions and teaching sessions may have been due to weak Wi‐Fi in certain areas of the hospital, and may warrant further investigation.
Analysis of HCGM utilization indicated that there were factors that made participants more or less likely to use the application. Individuals who reported that their attendings used HCGM regularly were more likely to use it themselves. Attending usage may legitimize use of HCGM for housestaff and medical students, who may otherwise feel that texting appears unprofessional. Participants who texted frequently in their personal lives were also more likely to utilize HCGM regularly, perhaps due to increased familiarity with/affinity for the platform.
HCGM participants who did not utilize the application regularly most often cited the fact that other team members did not use it. Among all users, the most frequently noted ineffective aspects of the application were its lack of ubiquity (HCGM was made available only to the small subset of individuals involved in the study) and inconsistent usage by those who did have access to the application. These findings suggest that HCGM effectiveness may be maximized with unrestricted access and mandated use; patchwork implementation, as in this study, detracts from perceived effectiveness.
Though objective outcome measures (average length of stay and average time of discharge) for patients of control attendings and HCGM attendings were examined, no significant differences were observed (P=0.089 and 0.494, respectively). These results may be due to the small size and short duration of the study.
Limitations
Our study had several limitations. HCGM was available only to individuals in the experimental arm of the study; most members of the internal medicine department and all other departments were not reachable through the application. This lack of ubiquity was a frequently cited frustration. Among individuals to whom HCGM was made available, barriers to adoption included: close proximity to would‐be message recipients, concern that smartphone usage in front of patients might appear unprofessional, and inconsistent or dropped service (weak or no Wi‐Fi signal in some areas). A technical problem with the Android platform midway through the study served as a potential frustration to several participants.
Due to the aforementioned issues, some participants used the HCGM application in a very limited way. We also did not replace hospital pagers (infeasible in this hospital setting); the HCGM application was added as a supplemental system. Future studies might explore the replacement of paging systems with HCGM‐type applications, as well as delve further into quantitative patient care outcomes.
It should be noted that the start of the study unintentionally coincided with the start of new interns and medical students in the hospital. Although it is possible that their relative unfamiliarity with the hospital may have made them more amenable to adopting a new technology, it is also possible that they may have been less likely to do so in the midst of such a major transitional period. Finally, this was a single‐site study, and as such, its findings may not be broadly generalizable. More research on such interventions is warranted, particularly in the context of current insecure communication methods such as paging that may make hospital‐wide adoption of new methods of secure communication, such as HCGM, mandatory.
CONCLUSION
Our study is the first to demonstrate that HCGM applications improve healthcare provider perception of multiple measures of in‐hospital communication, including efficiency of communication, workflow integration, and overall satisfaction. Notably, 85% of HCGM team respondents said they would recommend using an HCGM system on the wards. As smartphone use is expected to continue to increase among physicians and the general population, it is increasingly important to understand how to utilize these powerful communication tools to improve healthcare in an effective and secure manner.
Acknowledgements
The authors would like to thank the Stanford Medicine Residency Program and Stanford Hospital and Clinics for allowing them to perform the study, as well as Medigram, Inc., for supplying gift cards to incentivize survey completion and loaner iPhones to allow individuals without smartphones to participate in the study.
Disclosures: Stanford University School of Medicine's Medical Scholars Program provided financial support to the first author over the course of the study. Medigram, Inc. funded gift cards given to both control and HCGM participants upon survey completion and provided loaner iPhones to allow individuals without smartphones to participate in the study. Medigram, Inc. has also paid the fee for OnlineOpen to provide open access to this article. This study received an institutional review board exemption as quality improvement research. Participation was voluntary, and all participants signed a consent form. The authors report no conflicts of interest.
- Communication systems in healthcare. Clin Biochem Rev. 2006;27:89–98. .
- Alphanumeric paging: a potential source of problems in patient care and communication. J Surg Educ. 2011;68:447–451. , , .
- Manhattan Research. Taking the pulse: US market research report. Available at: http://manhattanresearch.com/Products‐and‐Services/Physician/Physician‐Research‐Modules. Accessed February 6, 2013.
- The use of wireless e‐mail to improve healthcare team communication. J Am Med Inform Assoc. 2009;16:705–713. , , , et al.
- The use of smartphones for clinical communication on internal medicine wards. J Hosp Med. 2010;5:553–559. , , , et al.
- US Department of Health and Human Services. Summary of the HIPAA privacy rule. Available at: http://www.hhs.gov/ocr/privacy/hipaa/understanding/summary/. Accessed May 11, 2013.
- United States Department of Health and Human Services. Summary of the HIPAA security rule. Available at: http://www.hhs.gov/ocr/privacy/hipaa/understanding/srsummary.html. Accessed May 11, 2013.
- Stanford University. Stanford facts: about Stanford. Available at: http://facts.stanford.edu/about/hospital. Accessed October 12, 2013.
- Wikinvest. USA mobility. Available at: http://www.wikinvest.com/stock/USA_Mobility_(USMO). Accessed February 6, 2013.
Pagers, though reliable and familiar technology, can be suboptimal for facilitating healthcare team communication.[1, 2] Most paging systems utilize single‐function pagers and only allow one‐way communication, requiring recipients to disrupt workflow to respond to pages. Paging transmissions can also be intercepted, and the information presented on pager displays can be viewed by anyone in possession of the pager.
Smartphones allow for instantaneous two‐way and group communication through advanced technological features. Their use is widespread; over 81% of American physicians owned a smartphone in 2011.[3] Previous studies demonstrate that healthcare providers rate smartphone‐based email positively, and that team smartphones can facilitate communication between nurses and physicians.[4, 5] However, these studies specifically examined the utility of smartphone‐based email and voice calls, and did not include text messaging. Limitations of traditional smartphone‐based text messaging include Health Insurance Portability and Accountability Act (HIPAA) noncompliance and dependence on in‐hospital cellular reception, which can be unreliable. HIPAA is a 1996 US federal law that established a set of privacy and security rules governing not only what is considered protected health information (PHI), but also minimum standards for the protection of such information. HIPAA compliance is defined as meeting these minimum standards for physical, network, and process security.[6, 7] Though PHI is often transmitted via paging systems and commercial carrier‐based text messaging, these modalities are not secure and are thus not HIPAA‐compliant.
Text messaging applications that address these security and reliability issues have the potential to greatly enhance in‐hospital communication. We hypothesized that a smartphone‐based HIPAA‐compliant group messaging application could improve in‐hospital communication on the inpatient medicine service. To our knowledge, our study is the first to examine a HIPAA‐compliant text messaging system, and also the first to compare a combination paging/HIPAA‐compliant group messaging (HCGM) system with a paging‐only system in assessing healthcare provider perception of communication efficiency.
METHODS
Intervention
This study utilized Medigram (Medigram, Inc.,
Study Design
In an eight‐week prospective, cluster‐randomized, controlled trial conducted at Stanford Hospital (June 25, 2012August 17, 2012), three of five inpatient medicine teams were randomized to use Medigram in addition to the existing hospital paging system (HCGM teams); the remaining two teams were assigned to use hospital paging only (control teams). Each team included one attending physician, one resident, two interns, two medical students, and a case manager. According to prescheduled rotations, attendings rotated every two weeks, and residents, interns, and medical students rotated every four weeks. All rotations were either off‐service or off‐site, with the exception of two attendings who rotated between study teams but within their experimental designations. Case managers remained with the same team. Additionally, the satellite pharmacy was provided with an HCGM‐equipped smartphone to communicate with experimental teams.
Participation was voluntary, with a 96% participation rate (n=75). HCGM teams downloaded the free application onto their smartphones. Participants without smartphones were provided with one for the duration of the study. Proper application use was demonstrated by one researcher in a 10‐minute standardized presentation. HCGM teams were encouraged to use the application in lieu of paging, except when patient care could be compromised.
All participants completed linked baseline and post‐study surveys. Gift cards valued at $10 were provided on completion of each survey. Though participants were assigned to either HCGM or control groups based on the randomized assignment of their preset cluster (hospital team) to an HCGM or control group, analysis was performed on the individual level due to the hospital's set rotation schedule, which resulted in dynamic, frequently changing clusters. We also compared average length of stay and time of discharge for patients treated by control versus HCGM teams. Clinical outcome data were obtained from the hospital's database using Midas+ Statit Solutions (Midas+ Statit Solutions Group, Tucson, AZ). Survey and clinical outcome data were analyzed in Stata (StataCorp, College Station, TX) and R (R Foundation for Statistical Computing, Vienna, Austria).
Survey Design and Analysis
Identical, anonymous baseline surveys were administered to control and HCGM teams. These surveys assessed attitudes toward the hospital paging system using a 5‐point Likert scale (1=low, 5=high) to evaluate perceived measures of effectiveness, workflow integration, and overall satisfaction. Wilcoxon rank sum tests were used to compare control and HCGM group responses to these questions. Free response questions asked participants to list the most effective and ineffective aspects of the paging system.
Post‐study surveys included all baseline survey questions, as well as questions about personal texting behavior. Post‐study HCGM surveys also included a parallel set of questions rating the HCGM application on the same measures of perceived effectiveness, workflow integration, and overall satisfaction. Wilcoxon signed rank tests were used to compare HCGM participants' baseline evaluations of paging to their post‐study evaluations of the HCGM application. Baseline and post‐study surveys were linked by the last four digits of respondent cell phone numbers. To compare control and HCGM group perceptions of the hospital paging system at study completion, post‐study survey responses were evaluated using Wilcoxon rank sum tests. The family‐wise error rate was left unadjusted due to concerns around inflated type II errors, given the high degree of correlation between survey questions.
All free response questions were analyzed using thematic analysis and grounded theory. After reviewing responses to each question, a list of overarching themes was constructed. Two researchers then independently reviewed each free‐response entry to assign it to one or more of these themes (some responses included several ideas with distinct themes). Entries with concordant theme assignments (90%) were coded as such; nonconcordant entries required an additional round of review to reach concordance. Finally, objective outcome measures including length of stay and time of discharge were analyzed by two‐sample t test.
Control Group | HCGM Group | |
---|---|---|
| ||
Paired surveys collected (completion rate) | 22 (85%) | 41 (84%) |
Average age 95% CI | 30.10 1.71 | 30.95 2.94 |
Gender | ||
Male | 13 (59%) | 24 (59%) |
Female | 9 (41%) | 17 (41%) |
Role | ||
Medical students | 6 (27%) | 11 (27%) |
Interns (PGY 1) | 7 (32%) | 12 (29%) |
Residents (PGY2 and 3) | 3 (14%) | 6 (15%) |
Attending physicians | 5 (23%) | 5 (12%) |
Case managers | 1 (5%) | 3 (7%) |
Pharmacists | 0 (0%) | 4 (10%) |
Information Security
The HCGM application in this study features 256‐bit encryption technology and requires a six‐digit password to access texts. For added security, a study‐dedicated server (HP ProLiant DL 180 G6; Hewlett‐Packard Co., Palo Alto, CA) with 4‐TB hard drive capacity (4 Seagate Barracuda ST1000DM003 1 TB 7200 RPM internal hard drives; Seagate Technology PLC, Cupertino, CA) was installed in the Stanford School of Medicine Data Center to store encrypted text messages. Data stored on the phones/server were accessible only to study participants, not researchers. These security measures were approved by Stanford Hospital and Stanford School of Medicine's security and privacy review process.
Hospital Paging System
Stanford Hospital and Clinics is a quaternary care academic medical center with 613 beds, 49 operating rooms, and over 25,000 inpatient admissions per year.[8] The institution uses one‐way alphanumeric pagers (primary model: Daviscomm BR802 Flex Pager from USA Mobility, secondary model: Sun Telecom Titan 3 Plus from USA Mobility; USA Mobility Inc., Springfield, VA). USA Mobility operates the largest one‐ and two‐way paging networks in the United States.[9]
RESULTS
Of 26 control and 49 HCGM group members participating in the study, linked baseline and post‐study surveys were collected for 22 control and 41 HCGM participants (completion rates of 84.6% and 83.7%, respectively). To minimize recall bias, surveys not completed within a prespecified timeframe upon entering or leaving a team (two days attendings, four days others) were excluded.
Control and HCGM Group Characteristics
Control and HCGM groups were well matched demographically (Table 1). The average ages of control and HCGM group members were 30.10 and 30.95, respectively. Both groups were 59% male and 41% female.
What do you find effective about the current hospital paging system? | What do you find ineffective about the current hospital paging system? | ||||
---|---|---|---|---|---|
Theme | No. of Respondents, (% of Total) | Response Example | Theme | No. of Respondents, (% of Total) | Response Example |
| |||||
Reliability of message transmission | 17 (30.4%) | Everyone is able to receive the pages I send, regardless of service | Time wasted waiting for a response | 17 (29.3%) | Inefficient use of time waiting for reply |
Ability to text page | 14 (25.0%) | Text paging allows targeted questions | One‐way nature of communication | 14 (24.1%) | Cannot text back instantly |
Ease of use | 8 (14.3%) | Easy to use | Needing to find a computer to send a text page | 12 (20.7%) | Have to find an available computer to send a page |
Search function | 5 (8.9%) | Search function is pretty effective in finding the people you're looking for | Character limitation | 10 (17.2%) | Length of text allowed too short |
Ubiquity | 5 (8.9%) | Everyone is on paging system | Search function | 6 (10.3%) | Delay in looking people up in the system |
Speed | 4 (7.1%) | Fast | Finding a phone to return a page | 5 (8.6%) | When you receive a page you need to find a phone |
Loud alerts | 4 (7.1%) | Pager loud enough to hear all the time | Receipt of page uncertain | 3 (5.2%) | Unknown if page received |
Staff responsiveness to pages | 4 (7.1%) | I know MD has to be onsite or covering the pager so someone eventually will call back | Sender's pager number not always included in page | 3 (5.2%) | Not everyone puts their pager number when they page. Then it's impossible to get back to them. |
Brevity of messages | 3 (5.4%) | Requires very brief messages (easier for recipient) | Needing to remain near a phone while waiting for a page response | 3 (5.2%) | Wait by a phone for someone to call back; sometimes they do not call back |
Helpful page operators | 2 (3.6%) | Page operators very helpful | Reliability of message transmission | 3 (5.2%) | Sometimes messages don't go through |
Other | 10 (17.9%) | It's online and allows paging from anywhere there's internet access | Other | 11 (19.0%) | You cannot text with patient info on it |
A similar distribution of team member roles was observed in both groups, with two exceptions. First, the proportion of attending respondents in the HCGM group was lower than in the control group. This was due to the fact that several HCGM attendings entered discrepant ID codes on their surveys, thus making it impossible to link baseline and post‐study responses; these data were excluded. Additionally, two HCGM attendings were on service for four, rather than the standard two weeks, meaning two additional data points from unique attendings could not be obtained. Second, the experimental group included four pharmacists, whereas the control group did not. As a sensitivity test, we analyzed the data excluding the pharmacists, and this did not change our results.
Baseline Evaluations of the Hospital Paging System
At baseline, there were no significant differences between control and HCGM participants' perceptions of paging effectiveness (see Supporting Table 1, in the online version of this article). On a 5‐point rating scale (1=low, 5=high), 63 subjects rated their overall satisfaction with the paging system an average of 2.79 (95% confidence interval: 2.55‐3.03).
In free response questions, components of the paging system most frequently cited as effective included: reliability of message transmission, alphanumeric text paging, and ease of use (30.4%, 25.0%, and 14.3% of 56 respondents, respectively) (Table 2). Ineffective aspects included: time wasted waiting for responses to pages, the unidirectional nature of pagers, and needing to find a computer to send a text page (29.3%, 24.1%, and 20.7% of 58 respondents, respectively) (Table 2).
Question | Baseline Average Rating of Paging System* | Post‐Study Average Rating of HCGM Application | P Value |
---|---|---|---|
| |||
Rate the effectiveness of each in allowing you to | |||
Communicate your thoughts clearly | 3.194 | 3.806 | 0.010 |
Communicate your thoughts efficiently | 3.200 | 3.829 | 0.009 |
Send messages to other hospital staff | 3.543 | 3.571 | 0.480 |
Receive messages/stay informed in real time | 3.222 | 3.306 | 0.405 |
Rate the effectiveness of each in integrating into your workflow during | |||
Work rounds | 2.313 | 3.000 | 0.018 |
Patient discharge | 2.448 | 3.276 | 0.012 |
Patient admissions | 2.862 | 2.621 | 0.238 |
Teaching sessions | 2.292 | 2.458 | 0.448 |
Overall satisfaction | 2.811 | 3.459 | 0.003 |
Baseline Utilization of Text Messaging
The majority of participants were familiar with text messaging and regularly used it personally and professionally prior to the start of the study. 90.5% of participants (n = 63) reported sending an average of 1 personal text messages per day, with the largest proportion (39.7%) sending 1‐5 texts per day (see Supporting Figure 1A in the online version of this article). 58.1% of respondents (n=62) reported sending an average of 1 text messages per day related to patient care (see Supporting Figure 1B in the online version of this article), with the largest fraction (58.3%) sending 1‐5 texts per day.
HCGM Adoption and Usage Patterns
Active use of HCGM was defined as using the application to send or receive an average of 1 text messages per day. Of HCGM participants, 67% self‐reported 1 week of active use of the application, indicating a strong compliance rate. Among non‐attendings, 70% reported sending 1 or more texts to other team members per day; this percentage increased to 86% among those whose attendings texted them at least once per day (47% of non‐attendings). Respondents who text frequently in their personal lives (>5 texts/day) were more likely to use the application; 90% of these respondents sent 1 or more HCGM texts per day.
Among 12 subjects who did not report sending or receiving 1 HCGM text/day, the top three reasons were: other team members were not using it (67%), no need to use it given the close proximity of other team members (67%), and other (33%). A Wilcoxon rank sum test was used to compare the ages of active versus nonactive users; no significant age difference was found (P=0.200).
To provide an objective measure of application adoption, usage data for each HCGM participant were obtained from the application developers. Because much of the study's first week was spent onboarding and instructing participant, the first week was not included in the analysis. Of 43 individuals enrolled in the study for at least one of the seven remaining weeks, 56% sent a total of 5 texts, 44% sent 10 texts, and 28% sent 20 texts. HCGM users on three teams sent an aggregate mean of 123 texts/week. Data on number of messages received by each user were not available.
Perceived Effectiveness: Paging Versus HCGM
In post‐study surveys, HCGM participants rated HCGM significantly higher (P<0.05) than paging (Table 3) in terms of ability to communicate thoughts clearly (P=0.010) and efficiently (P=0.009). HCGM was also deemed more effective at integrating into workflow during rounds (P=0.018) and patient discharge (P=0.012). Overall satisfaction with HCGM was also significantly higher (P=0.003).
Control (n=22) | HCGM (n=41) | |||||
---|---|---|---|---|---|---|
Baseline Mean | Post‐Study Mean | P Value* | Baseline Mean | Post‐Study Mean | P Value* | |
| ||||||
Rate the effectiveness of each in allowing you to | ||||||
Communicate your thoughts clearly | 2.905 | 2.619 | 0.103 | 3.250 | 2.850 | 0.004 |
Communicate your thoughts efficiently | 2.952 | 2.762 | 0.106 | 3.250 | 2.825 | 0.018 |
Send messages to other hospital staff | 3.762 | 3.190 | 0.019 | 3.550 | 3.450 | 0.253 |
Receive messages/stay informed in real time | 3.667 | 2.857 | 0.002 | 3.300 | 2.900 | 0.031 |
Rate the effectiveness of each in integrating into your workflow during | ||||||
Work rounds | 2.429 | 2.476 | 0.303 | 2.410 | 2.718 | 0.078 |
Patient discharge | 2.500 | 2.350 | 0.251 | 2.472 | 2.861 | 0.071 |
Patient admissions | 2.905 | 2.524 | 0.020 | 2.889 | 3.000 | 0.384 |
Teaching sessions | 2.143 | 2.200 | 0.386 | 2.367 | 2.400 | 0.418 |
Comparison of Pre‐ and Post‐study Perceived Effectiveness of the Hospital Paging System
In post‐study evaluations, both control and HCGM participants rated the paging system's effectiveness less favorably (P<0.05) compared to baseline in terms of ability to receive messages/stay informed in real time (control P=0.002, HCGM P=0.031) (Table 4). Controls also reported a decrease from baseline in perceived effectiveness of paging in terms of ability to send messages (P=0.019) and integrate into workflow during patient admissions (P=0.020). HCGM participants found paging less effective at communicating thoughts clearly (P=0.004) and efficiently (P=0.018). No significant differences existed between control and HCGM groups' average assessments of paging at the conclusion of the study (see Supporting Table 2, in the online version of this article).
What do you find effective about the Medigram system? | What do you find ineffective about the Medigram system? | ||||
---|---|---|---|---|---|
Theme | No. of Respondents, (% of Total) | Response Example | Theme | No. of Respondents, (% of Total) | Response Example |
| |||||
Ease of use | 11 (32.4%) | Easy to use | Lack of ubiquity | 10 (30.3%) | Not enough people using it |
Group texting feature | 11 (32.4%) | Ability to communicate with entire teameveryone seeing same message | Inconsistent usage | 8 (24.2%) | No one used it reliably |
Speed | 8 (23.5%) | Faster than a page to send a message | Reliability of message transmission | 5 (15.2%) | Big negative is it requires Wi‐Fi |
Accessibility | 5 (14.7%) | Able to get messages across quickly and anywhere without a computer | Missed message alerts | 4 (12.1%) | Unable to reliably know message was received if phone on silent |
Efficiency | 4 (11.8%) | Very efficient way to communicate | Password login | 3 (9.1%) | Having to type a 6‐digit password in |
Real‐time communication | 2 (5.9%) | Real‐time results | User interface | 2 (6.1%) | Interface is a little convoluted |
No character limitation | 2 (5.9%) | No limit on words | Other | 10 (30.3%) | Not sure if all of the texts were relevant |
Other | 4 (11.8%) | Great UI |
HCGM User Experience
When asked if they would recommend using an HCGM system to facilitate communication on the internal medicine wards, 85% of HCGM participants replied yes, 15% reported not sure, and 0% reported no. Based on free response entries, HCGM's most effective features (Table 5) included ease of use, group texting capacity, and speed (32.4%, 32.4%, and 23.5% of 34 respondents, respectively); its most ineffective aspects (Table 5) included lack of ubiquity, inconsistent usage by those with access to the application, and reliability of message transmission (30.3%, 24.2%, and 15.2% of 33 respondents, respectively).
DISCUSSION
We are the first to report that smartphone‐based, HIPAA‐compliant, group messaging applications improve provider perception of in‐hospital communication, while providing the information security that paging and commercial cellular networks do not. HCGM participants rated the application more favorably than paging in terms of clarity and efficiency of communication. These findings may be attributed to the expanded functionality offered by the application, including no character limit per HCGM text, the ability to use special characters such as slashes and ampersands, group texting, and the ability to reply immediately. HCGM may result in more efficient communication by facilitating direct two‐way communication via smartphones, whereas sending or returning pages requires a landline or computer.
HCGM participants rated the application higher than paging in terms of workflow integration during rounds and patient discharge, but not during patient admissions and teaching sessions. We had hypothesized that HCGM would integrate better into participants' workflows because HCGM texts could be replied to immediately. The reasons for the equivalence of HCGM and paging for workflow integration during patient admissions and teaching sessions may have been due to weak Wi‐Fi in certain areas of the hospital, and may warrant further investigation.
Analysis of HCGM utilization indicated that there were factors that made participants more or less likely to use the application. Individuals who reported that their attendings used HCGM regularly were more likely to use it themselves. Attending usage may legitimize use of HCGM for housestaff and medical students, who may otherwise feel that texting appears unprofessional. Participants who texted frequently in their personal lives were also more likely to utilize HCGM regularly, perhaps due to increased familiarity with/affinity for the platform.
HCGM participants who did not utilize the application regularly most often cited the fact that other team members did not use it. Among all users, the most frequently noted ineffective aspects of the application were its lack of ubiquity (HCGM was made available only to the small subset of individuals involved in the study) and inconsistent usage by those who did have access to the application. These findings suggest that HCGM effectiveness may be maximized with unrestricted access and mandated use; patchwork implementation, as in this study, detracts from perceived effectiveness.
Though objective outcome measures (average length of stay and average time of discharge) for patients of control attendings and HCGM attendings were examined, no significant differences were observed (P=0.089 and 0.494, respectively). These results may be due to the small size and short duration of the study.
Limitations
Our study had several limitations. HCGM was available only to individuals in the experimental arm of the study; most members of the internal medicine department and all other departments were not reachable through the application. This lack of ubiquity was a frequently cited frustration. Among individuals to whom HCGM was made available, barriers to adoption included: close proximity to would‐be message recipients, concern that smartphone usage in front of patients might appear unprofessional, and inconsistent or dropped service (weak or no Wi‐Fi signal in some areas). A technical problem with the Android platform midway through the study served as a potential frustration to several participants.
Due to the aforementioned issues, some participants used the HCGM application in a very limited way. We also did not replace hospital pagers (infeasible in this hospital setting); the HCGM application was added as a supplemental system. Future studies might explore the replacement of paging systems with HCGM‐type applications, as well as delve further into quantitative patient care outcomes.
It should be noted that the start of the study unintentionally coincided with the start of new interns and medical students in the hospital. Although it is possible that their relative unfamiliarity with the hospital may have made them more amenable to adopting a new technology, it is also possible that they may have been less likely to do so in the midst of such a major transitional period. Finally, this was a single‐site study, and as such, its findings may not be broadly generalizable. More research on such interventions is warranted, particularly in the context of current insecure communication methods such as paging that may make hospital‐wide adoption of new methods of secure communication, such as HCGM, mandatory.
CONCLUSION
Our study is the first to demonstrate that HCGM applications improve healthcare provider perception of multiple measures of in‐hospital communication, including efficiency of communication, workflow integration, and overall satisfaction. Notably, 85% of HCGM team respondents said they would recommend using an HCGM system on the wards. As smartphone use is expected to continue to increase among physicians and the general population, it is increasingly important to understand how to utilize these powerful communication tools to improve healthcare in an effective and secure manner.
Acknowledgements
The authors would like to thank the Stanford Medicine Residency Program and Stanford Hospital and Clinics for allowing them to perform the study, as well as Medigram, Inc., for supplying gift cards to incentivize survey completion and loaner iPhones to allow individuals without smartphones to participate in the study.
Disclosures: Stanford University School of Medicine's Medical Scholars Program provided financial support to the first author over the course of the study. Medigram, Inc. funded gift cards given to both control and HCGM participants upon survey completion and provided loaner iPhones to allow individuals without smartphones to participate in the study. Medigram, Inc. has also paid the fee for OnlineOpen to provide open access to this article. This study received an institutional review board exemption as quality improvement research. Participation was voluntary, and all participants signed a consent form. The authors report no conflicts of interest.
Pagers, though reliable and familiar technology, can be suboptimal for facilitating healthcare team communication.[1, 2] Most paging systems utilize single‐function pagers and only allow one‐way communication, requiring recipients to disrupt workflow to respond to pages. Paging transmissions can also be intercepted, and the information presented on pager displays can be viewed by anyone in possession of the pager.
Smartphones allow for instantaneous two‐way and group communication through advanced technological features. Their use is widespread; over 81% of American physicians owned a smartphone in 2011.[3] Previous studies demonstrate that healthcare providers rate smartphone‐based email positively, and that team smartphones can facilitate communication between nurses and physicians.[4, 5] However, these studies specifically examined the utility of smartphone‐based email and voice calls, and did not include text messaging. Limitations of traditional smartphone‐based text messaging include Health Insurance Portability and Accountability Act (HIPAA) noncompliance and dependence on in‐hospital cellular reception, which can be unreliable. HIPAA is a 1996 US federal law that established a set of privacy and security rules governing not only what is considered protected health information (PHI), but also minimum standards for the protection of such information. HIPAA compliance is defined as meeting these minimum standards for physical, network, and process security.[6, 7] Though PHI is often transmitted via paging systems and commercial carrier‐based text messaging, these modalities are not secure and are thus not HIPAA‐compliant.
Text messaging applications that address these security and reliability issues have the potential to greatly enhance in‐hospital communication. We hypothesized that a smartphone‐based HIPAA‐compliant group messaging application could improve in‐hospital communication on the inpatient medicine service. To our knowledge, our study is the first to examine a HIPAA‐compliant text messaging system, and also the first to compare a combination paging/HIPAA‐compliant group messaging (HCGM) system with a paging‐only system in assessing healthcare provider perception of communication efficiency.
METHODS
Intervention
This study utilized Medigram (Medigram, Inc.,
Study Design
In an eight‐week prospective, cluster‐randomized, controlled trial conducted at Stanford Hospital (June 25, 2012August 17, 2012), three of five inpatient medicine teams were randomized to use Medigram in addition to the existing hospital paging system (HCGM teams); the remaining two teams were assigned to use hospital paging only (control teams). Each team included one attending physician, one resident, two interns, two medical students, and a case manager. According to prescheduled rotations, attendings rotated every two weeks, and residents, interns, and medical students rotated every four weeks. All rotations were either off‐service or off‐site, with the exception of two attendings who rotated between study teams but within their experimental designations. Case managers remained with the same team. Additionally, the satellite pharmacy was provided with an HCGM‐equipped smartphone to communicate with experimental teams.
Participation was voluntary, with a 96% participation rate (n=75). HCGM teams downloaded the free application onto their smartphones. Participants without smartphones were provided with one for the duration of the study. Proper application use was demonstrated by one researcher in a 10‐minute standardized presentation. HCGM teams were encouraged to use the application in lieu of paging, except when patient care could be compromised.
All participants completed linked baseline and post‐study surveys. Gift cards valued at $10 were provided on completion of each survey. Though participants were assigned to either HCGM or control groups based on the randomized assignment of their preset cluster (hospital team) to an HCGM or control group, analysis was performed on the individual level due to the hospital's set rotation schedule, which resulted in dynamic, frequently changing clusters. We also compared average length of stay and time of discharge for patients treated by control versus HCGM teams. Clinical outcome data were obtained from the hospital's database using Midas+ Statit Solutions (Midas+ Statit Solutions Group, Tucson, AZ). Survey and clinical outcome data were analyzed in Stata (StataCorp, College Station, TX) and R (R Foundation for Statistical Computing, Vienna, Austria).
Survey Design and Analysis
Identical, anonymous baseline surveys were administered to control and HCGM teams. These surveys assessed attitudes toward the hospital paging system using a 5‐point Likert scale (1=low, 5=high) to evaluate perceived measures of effectiveness, workflow integration, and overall satisfaction. Wilcoxon rank sum tests were used to compare control and HCGM group responses to these questions. Free response questions asked participants to list the most effective and ineffective aspects of the paging system.
Post‐study surveys included all baseline survey questions, as well as questions about personal texting behavior. Post‐study HCGM surveys also included a parallel set of questions rating the HCGM application on the same measures of perceived effectiveness, workflow integration, and overall satisfaction. Wilcoxon signed rank tests were used to compare HCGM participants' baseline evaluations of paging to their post‐study evaluations of the HCGM application. Baseline and post‐study surveys were linked by the last four digits of respondent cell phone numbers. To compare control and HCGM group perceptions of the hospital paging system at study completion, post‐study survey responses were evaluated using Wilcoxon rank sum tests. The family‐wise error rate was left unadjusted due to concerns around inflated type II errors, given the high degree of correlation between survey questions.
All free response questions were analyzed using thematic analysis and grounded theory. After reviewing responses to each question, a list of overarching themes was constructed. Two researchers then independently reviewed each free‐response entry to assign it to one or more of these themes (some responses included several ideas with distinct themes). Entries with concordant theme assignments (90%) were coded as such; nonconcordant entries required an additional round of review to reach concordance. Finally, objective outcome measures including length of stay and time of discharge were analyzed by two‐sample t test.
Control Group | HCGM Group | |
---|---|---|
| ||
Paired surveys collected (completion rate) | 22 (85%) | 41 (84%) |
Average age 95% CI | 30.10 1.71 | 30.95 2.94 |
Gender | ||
Male | 13 (59%) | 24 (59%) |
Female | 9 (41%) | 17 (41%) |
Role | ||
Medical students | 6 (27%) | 11 (27%) |
Interns (PGY 1) | 7 (32%) | 12 (29%) |
Residents (PGY2 and 3) | 3 (14%) | 6 (15%) |
Attending physicians | 5 (23%) | 5 (12%) |
Case managers | 1 (5%) | 3 (7%) |
Pharmacists | 0 (0%) | 4 (10%) |
Information Security
The HCGM application in this study features 256‐bit encryption technology and requires a six‐digit password to access texts. For added security, a study‐dedicated server (HP ProLiant DL 180 G6; Hewlett‐Packard Co., Palo Alto, CA) with 4‐TB hard drive capacity (4 Seagate Barracuda ST1000DM003 1 TB 7200 RPM internal hard drives; Seagate Technology PLC, Cupertino, CA) was installed in the Stanford School of Medicine Data Center to store encrypted text messages. Data stored on the phones/server were accessible only to study participants, not researchers. These security measures were approved by Stanford Hospital and Stanford School of Medicine's security and privacy review process.
Hospital Paging System
Stanford Hospital and Clinics is a quaternary care academic medical center with 613 beds, 49 operating rooms, and over 25,000 inpatient admissions per year.[8] The institution uses one‐way alphanumeric pagers (primary model: Daviscomm BR802 Flex Pager from USA Mobility, secondary model: Sun Telecom Titan 3 Plus from USA Mobility; USA Mobility Inc., Springfield, VA). USA Mobility operates the largest one‐ and two‐way paging networks in the United States.[9]
RESULTS
Of 26 control and 49 HCGM group members participating in the study, linked baseline and post‐study surveys were collected for 22 control and 41 HCGM participants (completion rates of 84.6% and 83.7%, respectively). To minimize recall bias, surveys not completed within a prespecified timeframe upon entering or leaving a team (two days attendings, four days others) were excluded.
Control and HCGM Group Characteristics
Control and HCGM groups were well matched demographically (Table 1). The average ages of control and HCGM group members were 30.10 and 30.95, respectively. Both groups were 59% male and 41% female.
What do you find effective about the current hospital paging system? | What do you find ineffective about the current hospital paging system? | ||||
---|---|---|---|---|---|
Theme | No. of Respondents, (% of Total) | Response Example | Theme | No. of Respondents, (% of Total) | Response Example |
| |||||
Reliability of message transmission | 17 (30.4%) | Everyone is able to receive the pages I send, regardless of service | Time wasted waiting for a response | 17 (29.3%) | Inefficient use of time waiting for reply |
Ability to text page | 14 (25.0%) | Text paging allows targeted questions | One‐way nature of communication | 14 (24.1%) | Cannot text back instantly |
Ease of use | 8 (14.3%) | Easy to use | Needing to find a computer to send a text page | 12 (20.7%) | Have to find an available computer to send a page |
Search function | 5 (8.9%) | Search function is pretty effective in finding the people you're looking for | Character limitation | 10 (17.2%) | Length of text allowed too short |
Ubiquity | 5 (8.9%) | Everyone is on paging system | Search function | 6 (10.3%) | Delay in looking people up in the system |
Speed | 4 (7.1%) | Fast | Finding a phone to return a page | 5 (8.6%) | When you receive a page you need to find a phone |
Loud alerts | 4 (7.1%) | Pager loud enough to hear all the time | Receipt of page uncertain | 3 (5.2%) | Unknown if page received |
Staff responsiveness to pages | 4 (7.1%) | I know MD has to be onsite or covering the pager so someone eventually will call back | Sender's pager number not always included in page | 3 (5.2%) | Not everyone puts their pager number when they page. Then it's impossible to get back to them. |
Brevity of messages | 3 (5.4%) | Requires very brief messages (easier for recipient) | Needing to remain near a phone while waiting for a page response | 3 (5.2%) | Wait by a phone for someone to call back; sometimes they do not call back |
Helpful page operators | 2 (3.6%) | Page operators very helpful | Reliability of message transmission | 3 (5.2%) | Sometimes messages don't go through |
Other | 10 (17.9%) | It's online and allows paging from anywhere there's internet access | Other | 11 (19.0%) | You cannot text with patient info on it |
A similar distribution of team member roles was observed in both groups, with two exceptions. First, the proportion of attending respondents in the HCGM group was lower than in the control group. This was due to the fact that several HCGM attendings entered discrepant ID codes on their surveys, thus making it impossible to link baseline and post‐study responses; these data were excluded. Additionally, two HCGM attendings were on service for four, rather than the standard two weeks, meaning two additional data points from unique attendings could not be obtained. Second, the experimental group included four pharmacists, whereas the control group did not. As a sensitivity test, we analyzed the data excluding the pharmacists, and this did not change our results.
Baseline Evaluations of the Hospital Paging System
At baseline, there were no significant differences between control and HCGM participants' perceptions of paging effectiveness (see Supporting Table 1, in the online version of this article). On a 5‐point rating scale (1=low, 5=high), 63 subjects rated their overall satisfaction with the paging system an average of 2.79 (95% confidence interval: 2.55‐3.03).
In free response questions, components of the paging system most frequently cited as effective included: reliability of message transmission, alphanumeric text paging, and ease of use (30.4%, 25.0%, and 14.3% of 56 respondents, respectively) (Table 2). Ineffective aspects included: time wasted waiting for responses to pages, the unidirectional nature of pagers, and needing to find a computer to send a text page (29.3%, 24.1%, and 20.7% of 58 respondents, respectively) (Table 2).
Question | Baseline Average Rating of Paging System* | Post‐Study Average Rating of HCGM Application | P Value |
---|---|---|---|
| |||
Rate the effectiveness of each in allowing you to | |||
Communicate your thoughts clearly | 3.194 | 3.806 | 0.010 |
Communicate your thoughts efficiently | 3.200 | 3.829 | 0.009 |
Send messages to other hospital staff | 3.543 | 3.571 | 0.480 |
Receive messages/stay informed in real time | 3.222 | 3.306 | 0.405 |
Rate the effectiveness of each in integrating into your workflow during | |||
Work rounds | 2.313 | 3.000 | 0.018 |
Patient discharge | 2.448 | 3.276 | 0.012 |
Patient admissions | 2.862 | 2.621 | 0.238 |
Teaching sessions | 2.292 | 2.458 | 0.448 |
Overall satisfaction | 2.811 | 3.459 | 0.003 |
Baseline Utilization of Text Messaging
The majority of participants were familiar with text messaging and regularly used it personally and professionally prior to the start of the study. 90.5% of participants (n = 63) reported sending an average of 1 personal text messages per day, with the largest proportion (39.7%) sending 1‐5 texts per day (see Supporting Figure 1A in the online version of this article). 58.1% of respondents (n=62) reported sending an average of 1 text messages per day related to patient care (see Supporting Figure 1B in the online version of this article), with the largest fraction (58.3%) sending 1‐5 texts per day.
HCGM Adoption and Usage Patterns
Active use of HCGM was defined as using the application to send or receive an average of 1 text messages per day. Of HCGM participants, 67% self‐reported 1 week of active use of the application, indicating a strong compliance rate. Among non‐attendings, 70% reported sending 1 or more texts to other team members per day; this percentage increased to 86% among those whose attendings texted them at least once per day (47% of non‐attendings). Respondents who text frequently in their personal lives (>5 texts/day) were more likely to use the application; 90% of these respondents sent 1 or more HCGM texts per day.
Among 12 subjects who did not report sending or receiving 1 HCGM text/day, the top three reasons were: other team members were not using it (67%), no need to use it given the close proximity of other team members (67%), and other (33%). A Wilcoxon rank sum test was used to compare the ages of active versus nonactive users; no significant age difference was found (P=0.200).
To provide an objective measure of application adoption, usage data for each HCGM participant were obtained from the application developers. Because much of the study's first week was spent onboarding and instructing participant, the first week was not included in the analysis. Of 43 individuals enrolled in the study for at least one of the seven remaining weeks, 56% sent a total of 5 texts, 44% sent 10 texts, and 28% sent 20 texts. HCGM users on three teams sent an aggregate mean of 123 texts/week. Data on number of messages received by each user were not available.
Perceived Effectiveness: Paging Versus HCGM
In post‐study surveys, HCGM participants rated HCGM significantly higher (P<0.05) than paging (Table 3) in terms of ability to communicate thoughts clearly (P=0.010) and efficiently (P=0.009). HCGM was also deemed more effective at integrating into workflow during rounds (P=0.018) and patient discharge (P=0.012). Overall satisfaction with HCGM was also significantly higher (P=0.003).
Control (n=22) | HCGM (n=41) | |||||
---|---|---|---|---|---|---|
Baseline Mean | Post‐Study Mean | P Value* | Baseline Mean | Post‐Study Mean | P Value* | |
| ||||||
Rate the effectiveness of each in allowing you to | ||||||
Communicate your thoughts clearly | 2.905 | 2.619 | 0.103 | 3.250 | 2.850 | 0.004 |
Communicate your thoughts efficiently | 2.952 | 2.762 | 0.106 | 3.250 | 2.825 | 0.018 |
Send messages to other hospital staff | 3.762 | 3.190 | 0.019 | 3.550 | 3.450 | 0.253 |
Receive messages/stay informed in real time | 3.667 | 2.857 | 0.002 | 3.300 | 2.900 | 0.031 |
Rate the effectiveness of each in integrating into your workflow during | ||||||
Work rounds | 2.429 | 2.476 | 0.303 | 2.410 | 2.718 | 0.078 |
Patient discharge | 2.500 | 2.350 | 0.251 | 2.472 | 2.861 | 0.071 |
Patient admissions | 2.905 | 2.524 | 0.020 | 2.889 | 3.000 | 0.384 |
Teaching sessions | 2.143 | 2.200 | 0.386 | 2.367 | 2.400 | 0.418 |
Comparison of Pre‐ and Post‐study Perceived Effectiveness of the Hospital Paging System
In post‐study evaluations, both control and HCGM participants rated the paging system's effectiveness less favorably (P<0.05) compared to baseline in terms of ability to receive messages/stay informed in real time (control P=0.002, HCGM P=0.031) (Table 4). Controls also reported a decrease from baseline in perceived effectiveness of paging in terms of ability to send messages (P=0.019) and integrate into workflow during patient admissions (P=0.020). HCGM participants found paging less effective at communicating thoughts clearly (P=0.004) and efficiently (P=0.018). No significant differences existed between control and HCGM groups' average assessments of paging at the conclusion of the study (see Supporting Table 2, in the online version of this article).
What do you find effective about the Medigram system? | What do you find ineffective about the Medigram system? | ||||
---|---|---|---|---|---|
Theme | No. of Respondents, (% of Total) | Response Example | Theme | No. of Respondents, (% of Total) | Response Example |
| |||||
Ease of use | 11 (32.4%) | Easy to use | Lack of ubiquity | 10 (30.3%) | Not enough people using it |
Group texting feature | 11 (32.4%) | Ability to communicate with entire teameveryone seeing same message | Inconsistent usage | 8 (24.2%) | No one used it reliably |
Speed | 8 (23.5%) | Faster than a page to send a message | Reliability of message transmission | 5 (15.2%) | Big negative is it requires Wi‐Fi |
Accessibility | 5 (14.7%) | Able to get messages across quickly and anywhere without a computer | Missed message alerts | 4 (12.1%) | Unable to reliably know message was received if phone on silent |
Efficiency | 4 (11.8%) | Very efficient way to communicate | Password login | 3 (9.1%) | Having to type a 6‐digit password in |
Real‐time communication | 2 (5.9%) | Real‐time results | User interface | 2 (6.1%) | Interface is a little convoluted |
No character limitation | 2 (5.9%) | No limit on words | Other | 10 (30.3%) | Not sure if all of the texts were relevant |
Other | 4 (11.8%) | Great UI |
HCGM User Experience
When asked if they would recommend using an HCGM system to facilitate communication on the internal medicine wards, 85% of HCGM participants replied yes, 15% reported not sure, and 0% reported no. Based on free response entries, HCGM's most effective features (Table 5) included ease of use, group texting capacity, and speed (32.4%, 32.4%, and 23.5% of 34 respondents, respectively); its most ineffective aspects (Table 5) included lack of ubiquity, inconsistent usage by those with access to the application, and reliability of message transmission (30.3%, 24.2%, and 15.2% of 33 respondents, respectively).
DISCUSSION
We are the first to report that smartphone‐based, HIPAA‐compliant, group messaging applications improve provider perception of in‐hospital communication, while providing the information security that paging and commercial cellular networks do not. HCGM participants rated the application more favorably than paging in terms of clarity and efficiency of communication. These findings may be attributed to the expanded functionality offered by the application, including no character limit per HCGM text, the ability to use special characters such as slashes and ampersands, group texting, and the ability to reply immediately. HCGM may result in more efficient communication by facilitating direct two‐way communication via smartphones, whereas sending or returning pages requires a landline or computer.
HCGM participants rated the application higher than paging in terms of workflow integration during rounds and patient discharge, but not during patient admissions and teaching sessions. We had hypothesized that HCGM would integrate better into participants' workflows because HCGM texts could be replied to immediately. The reasons for the equivalence of HCGM and paging for workflow integration during patient admissions and teaching sessions may have been due to weak Wi‐Fi in certain areas of the hospital, and may warrant further investigation.
Analysis of HCGM utilization indicated that there were factors that made participants more or less likely to use the application. Individuals who reported that their attendings used HCGM regularly were more likely to use it themselves. Attending usage may legitimize use of HCGM for housestaff and medical students, who may otherwise feel that texting appears unprofessional. Participants who texted frequently in their personal lives were also more likely to utilize HCGM regularly, perhaps due to increased familiarity with/affinity for the platform.
HCGM participants who did not utilize the application regularly most often cited the fact that other team members did not use it. Among all users, the most frequently noted ineffective aspects of the application were its lack of ubiquity (HCGM was made available only to the small subset of individuals involved in the study) and inconsistent usage by those who did have access to the application. These findings suggest that HCGM effectiveness may be maximized with unrestricted access and mandated use; patchwork implementation, as in this study, detracts from perceived effectiveness.
Though objective outcome measures (average length of stay and average time of discharge) for patients of control attendings and HCGM attendings were examined, no significant differences were observed (P=0.089 and 0.494, respectively). These results may be due to the small size and short duration of the study.
Limitations
Our study had several limitations. HCGM was available only to individuals in the experimental arm of the study; most members of the internal medicine department and all other departments were not reachable through the application. This lack of ubiquity was a frequently cited frustration. Among individuals to whom HCGM was made available, barriers to adoption included: close proximity to would‐be message recipients, concern that smartphone usage in front of patients might appear unprofessional, and inconsistent or dropped service (weak or no Wi‐Fi signal in some areas). A technical problem with the Android platform midway through the study served as a potential frustration to several participants.
Due to the aforementioned issues, some participants used the HCGM application in a very limited way. We also did not replace hospital pagers (infeasible in this hospital setting); the HCGM application was added as a supplemental system. Future studies might explore the replacement of paging systems with HCGM‐type applications, as well as delve further into quantitative patient care outcomes.
It should be noted that the start of the study unintentionally coincided with the start of new interns and medical students in the hospital. Although it is possible that their relative unfamiliarity with the hospital may have made them more amenable to adopting a new technology, it is also possible that they may have been less likely to do so in the midst of such a major transitional period. Finally, this was a single‐site study, and as such, its findings may not be broadly generalizable. More research on such interventions is warranted, particularly in the context of current insecure communication methods such as paging that may make hospital‐wide adoption of new methods of secure communication, such as HCGM, mandatory.
CONCLUSION
Our study is the first to demonstrate that HCGM applications improve healthcare provider perception of multiple measures of in‐hospital communication, including efficiency of communication, workflow integration, and overall satisfaction. Notably, 85% of HCGM team respondents said they would recommend using an HCGM system on the wards. As smartphone use is expected to continue to increase among physicians and the general population, it is increasingly important to understand how to utilize these powerful communication tools to improve healthcare in an effective and secure manner.
Acknowledgements
The authors would like to thank the Stanford Medicine Residency Program and Stanford Hospital and Clinics for allowing them to perform the study, as well as Medigram, Inc., for supplying gift cards to incentivize survey completion and loaner iPhones to allow individuals without smartphones to participate in the study.
Disclosures: Stanford University School of Medicine's Medical Scholars Program provided financial support to the first author over the course of the study. Medigram, Inc. funded gift cards given to both control and HCGM participants upon survey completion and provided loaner iPhones to allow individuals without smartphones to participate in the study. Medigram, Inc. has also paid the fee for OnlineOpen to provide open access to this article. This study received an institutional review board exemption as quality improvement research. Participation was voluntary, and all participants signed a consent form. The authors report no conflicts of interest.
- Communication systems in healthcare. Clin Biochem Rev. 2006;27:89–98. .
- Alphanumeric paging: a potential source of problems in patient care and communication. J Surg Educ. 2011;68:447–451. , , .
- Manhattan Research. Taking the pulse: US market research report. Available at: http://manhattanresearch.com/Products‐and‐Services/Physician/Physician‐Research‐Modules. Accessed February 6, 2013.
- The use of wireless e‐mail to improve healthcare team communication. J Am Med Inform Assoc. 2009;16:705–713. , , , et al.
- The use of smartphones for clinical communication on internal medicine wards. J Hosp Med. 2010;5:553–559. , , , et al.
- US Department of Health and Human Services. Summary of the HIPAA privacy rule. Available at: http://www.hhs.gov/ocr/privacy/hipaa/understanding/summary/. Accessed May 11, 2013.
- United States Department of Health and Human Services. Summary of the HIPAA security rule. Available at: http://www.hhs.gov/ocr/privacy/hipaa/understanding/srsummary.html. Accessed May 11, 2013.
- Stanford University. Stanford facts: about Stanford. Available at: http://facts.stanford.edu/about/hospital. Accessed October 12, 2013.
- Wikinvest. USA mobility. Available at: http://www.wikinvest.com/stock/USA_Mobility_(USMO). Accessed February 6, 2013.
- Communication systems in healthcare. Clin Biochem Rev. 2006;27:89–98. .
- Alphanumeric paging: a potential source of problems in patient care and communication. J Surg Educ. 2011;68:447–451. , , .
- Manhattan Research. Taking the pulse: US market research report. Available at: http://manhattanresearch.com/Products‐and‐Services/Physician/Physician‐Research‐Modules. Accessed February 6, 2013.
- The use of wireless e‐mail to improve healthcare team communication. J Am Med Inform Assoc. 2009;16:705–713. , , , et al.
- The use of smartphones for clinical communication on internal medicine wards. J Hosp Med. 2010;5:553–559. , , , et al.
- US Department of Health and Human Services. Summary of the HIPAA privacy rule. Available at: http://www.hhs.gov/ocr/privacy/hipaa/understanding/summary/. Accessed May 11, 2013.
- United States Department of Health and Human Services. Summary of the HIPAA security rule. Available at: http://www.hhs.gov/ocr/privacy/hipaa/understanding/srsummary.html. Accessed May 11, 2013.
- Stanford University. Stanford facts: about Stanford. Available at: http://facts.stanford.edu/about/hospital. Accessed October 12, 2013.
- Wikinvest. USA mobility. Available at: http://www.wikinvest.com/stock/USA_Mobility_(USMO). Accessed February 6, 2013.
© 2014 The Authors Journal of Hospital Medicine published by Wiley Periodicals, Inc. on behalf of Society of Hospital Medicine
Why Transfusion BPAs Are Overridden
Transfusion of blood products has multiple clinical applications, yet when used outside of recommended guidelines it is associated with increased cost, waste, morbidity, and mortality.[1] Studies reviewing restrictive versus liberal blood transfusion strategies have demonstrated no benefit to liberal strategies over restrictive strategies[2, 3, 4, 5, 6, 7, 8, 9, 10] and possibly even harm.[11] Given the risks and real costs of blood transfusions occurring outside of recommended guidelines, professional societies in hematology, anesthesiology, and hospital medicine each include excessive blood transfusions among their top 5 questionable clinical practices in the American Board of Internal Medicine Foundation's Choosing Wisely initiative.[12] To modify behaviors around blood transfusion practices, hospitals and blood banks may need to provide clinical decision support (CDS) for physicians.
The conventional approach to CDS is direct education and training campaigns, but there is a unique opportunity for intelligent decision support at the point of care through electronic medical record (EMR) systems directly integrated with computerized physician order entry (CPOE).[13, 14, 15] Prior work at Stanford toward reducing unnecessary blood transfusions started with hospital‐wide education campaigns, which brought down the percentage of transfusions ordered for patients with hemoglobin (Hgb) >8 g/dL from 57% to 52%. Further reduction to <30% was achieved after the introduction of an interruptive best practice alert (BPA) integrated into the CPOE transfusion ordering process.[16, 17] Specifically, providers attempting to order a blood transfusion for patients with an EMR‐determined Hgb >7 are presented with a BPA popup reminding them of best practice guidelines and a prompt to either abort the transfusion or provide a reason to override the BPA and proceed.
It remains uncertain why up to 30% of transfusions continue to occur outside of recommended guidelines in spite of interruptive prompts. This study demonstrates a general approach to secondary use of clinical data from the EMR toward understanding provider behavior, specifically by analyzing free‐text comments linked to transfusion override behavior and identifying the type of providers interacting with the BPAs.
MATERIALS AND METHODS
At Stanford University Hospital, a 447‐bed academic tertiary care center servicing adult patients, clinicians order blood transfusions through an EMR+CPOE system. When an order for red blood cell transfusion is attempted, the EMR evaluates the patient chart for specific criteria based on previously published guidelines.[18] Specifically, the BPA will review the last recorded Hgb value and trigger if the Hgb is >8, or if the Hgb is >7 and there is no concurrent EMR problem‐list entry related to acute coronary syndrome or acute hemorrhage. Once the blood transfusion BPA triggers, the ordering provider is presented with an interruptive prompt reminding them of best practice guidelines and the 3 most recent Hgb values for the patient.[16] From there, the provider may either abort the transfusion or override the BPA and proceed. Overrides require the provider to select a reason from a predefined list of institutionally accepted transfusion indications including acute bleeding, acute coronary syndrome and Hgb <8, and postoperative cardiothoracic surgery and Hgb <8. If none of the predefined override reasons are selected, the provider simply selects other, with the option of a free‐text comment to elaborate their rationale.
Data from provider interactions with the BPA were collected from the EMR across all inpatient wards for 8 months after the implementation period. Data collected from each interaction included the patient identifier, alert description, action taken, ordering provider identifier, ordering provider type (job title), optional comments for overriding the BPA, and date and time.
The free‐text override comments were independently reviewed by 2 licensed physicians, tagging them into a set of general categories by iterative inductive analysis of the comment content. Individual comments were allowed multiple possible category tags (eg, coronary artery disease and symptomatic). The initial tagging process was expedited by isolating common keywords in the override comments and assigning likely category tags to each, as in Table 2. The 2 physician reviewers then manually inspected all comments with the option to revise the initial category tagging to ensure validity. Many category tags occurred sporadically and were aggregated into more general categories, such as hematologic disorder (includes myelodysplastic syndrome, myelofibrosis, multiple myeloma, sickle cell, thalassemia, Waldenstrom's), symptomatic (includes fatigue, lightheaded, short of breath), and per other medical doctor [MD] (includes any other physician direction, primarily specialty consultation services).
To assess the inter‐rater agreement of this category tagging between the 2 reviewers, a confusion matrix similar to the example in Table 1 was setup for each tag used. Several agreement statistics are calculated based on the confusion matrix, including the positive agreement rate (Pa+) and Cohen's kappa statistic (). Kappa statistic values range from 1 to +1, with values <0 indicating no agreement and values >0.8 indicating near perfect agreement.[19] To reject the null hypothesis that the 2 reviewers could have independently arrived at their similar tagging assignments by chance, a 2 test was applied for each confusion matrix, with Yates' correction to avoid overestimating statistical significance given the low rates of inter‐rater disagreement.[20]
Reviewer 1 Tags "Surgery" | ||||
---|---|---|---|---|
Yes | No | Total | ||
| ||||
Reviewer 2 Tags "Surgery" | Yes | 143 | 11 | 154 |
No | 5 | 820 | 825 | |
Total | 148 | 831 | 979 |
Category Tag | Keyword | Keyword Count |
---|---|---|
| ||
Hgb 78 | 7.1, 7.2, 7.8, 7.9, 8, <8 | 360 |
BMT | BMT | 359 |
Symptomatic | Symptomatic | 187 |
Surgery | Postop, post‐op, surgery, surgical | 176 |
Dropping Hgb | Down, drop, dropping | 117 |
Chemotherapy | Chemo, chemotherapy | 88 |
Per other MD | Per | 87 |
Transplant | Transplant | 70 |
Cardiac | Cardiac | 66 |
Bleeding | Bleeding | 65 |
Procedure | Procedure | 65 |
Hgb <7 | 7 | 58 |
Hypotension | Hypotension | 51 |
Protocol | Protocol | 51 |
Cirrhosis | Liver | 50 |
Imminent discharge | Discharge | 49 |
Leukemia, acute | AML | 44 |
Cancer | Cancer | 37 |
Sepsis | Sepsis | 32 |
Tachycardia | Tachycardia | 28 |
RESULTS
During the data collection period from January 1, 2011 to August 31, 2012, the blood transfusion BPA fired in 11,791 instances, of which 10,642 recorded sufficient data for analysis (Figure 1). The ordering provider proceeded to override the BPA and continued with transfusion in 98% of cases (10,442/10,642). Acute bleeding" was the most common structured response (34%). The majority of BPA overrides used the general purpose other structured response option accounting for 56% (5886/10,442) of override responses, of which 37% (2185/5886) entered a free‐text comment elaborating the override reason. With 3701 nonresponders (other overrides with no free‐text comment), the overall response rate was 65% (6941/10,642).
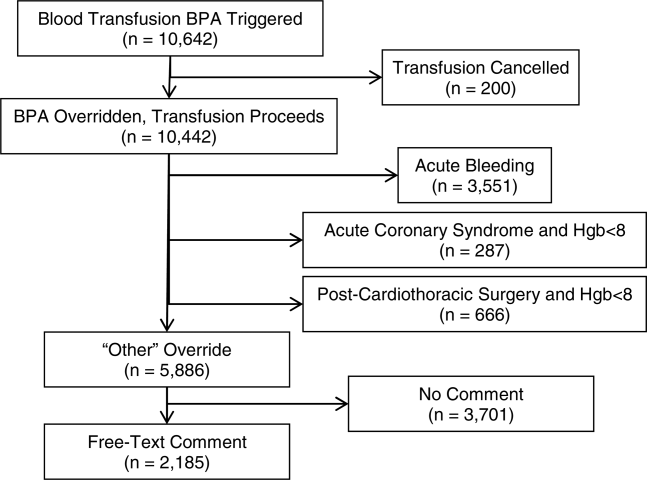
With a handful of free‐text comments included with structured override responses (eg, 28 acute bleeding overrides included additional comment from the provider), a total of 2216 override comments were available for analysis. Using an initial selection of keyword‐tag associations, as in Table 2, 95% (2104/2216) of the override comments had a preliminary category tagging assigned. After manual review and revision by the first physician reviewer, 74% (1633/2216) of the comments retained their automated tags, whereas 26% (583/2216) were updated based on the reviewer's assessment of validity. This included 112 comments lacking automated tags the reviewer manually added, as well as 471 comments with automated tags revised by the reviewer.
The most common override category tags are presented in Figure 2 and Figure 3 for BPAs triggered in response to blood transfusions ordered for patients with Hgb 78 and Hgb >8, respectively. The agreed+ percentages correspond to the number of comments where the 2 physician reviewers both assigned the respective category tag (Pa+), whereas the disagreed percentages correspond to comments where the reviewers differed (Pd=1‐Pa). By Yates' corrected 2 analysis, P was <1010 for independence between reviewer tag assignments for all tags assessed. Kappa statistics are reported in the figures to describe inter‐rater agreement.
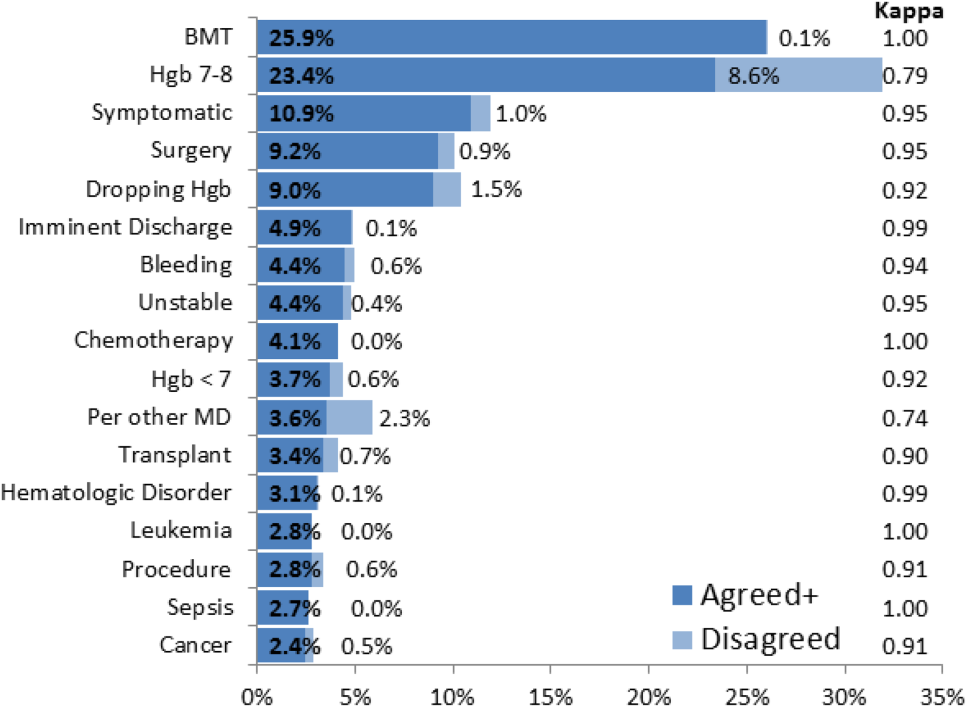
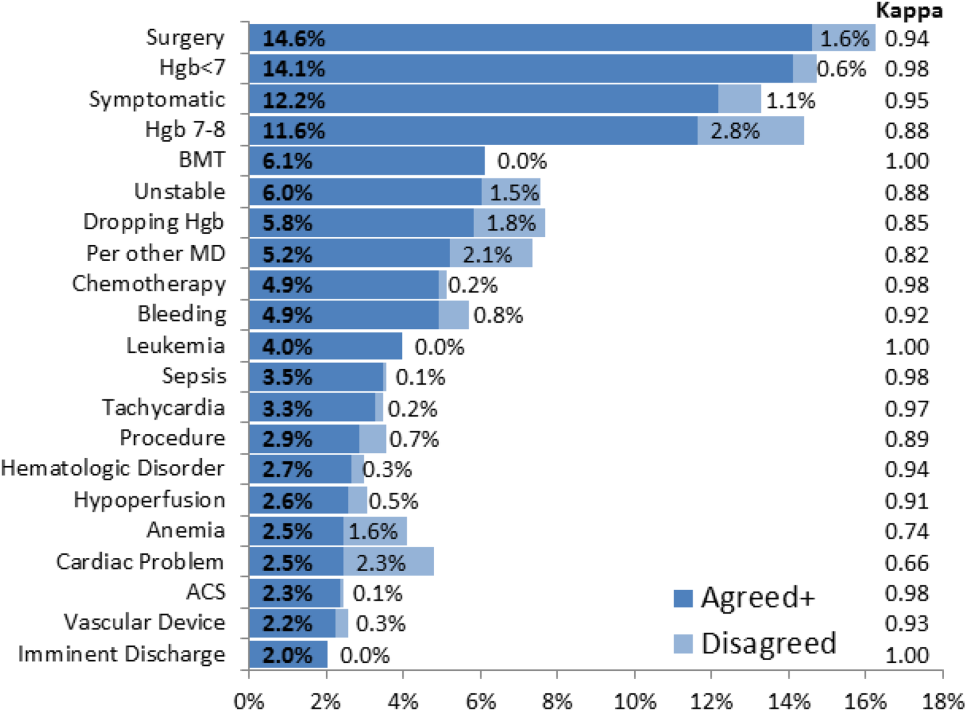
Resident physicians were the primary ordering provider group, accounting for approximately 55% (5863/10,642) of BPA interactions, followed by registered nurses, fellows, and attending physicians.
DISCUSSION
Prior work has established that an interruptive BPA to provide decision support against inappropriate blood transfusions can drive a significant and sustained reduction in unnecessary transfusions,[16] especially when satisfying the primary features of effective decision support.[14] The quantity of transfusions directly aborted by the BPA (only 2% in this case) does not nearly account for the total observed reductions in transfusions, suggesting that the BPA yields an indirect teaching effect over time beyond direct interruption. In other words, once a user has been interrupted by the BPA several times, they will be discouraged from even attempting to order inappropriate blood transfusions in the future.
Despite the improvements above, there remains a substantial fraction (up to 30%) of blood transfusions occurring outside of recommended guidelines where providers specifically override the BPA prompt.[21] This work is the first structured analysis of such BPA override behavior by generalizable methods of EMR data analysis to guide further improvements.
Analysis of the override behavior focused primarily on the free‐text comments explaining provider rationales for overriding the BPA. These comments were categorized by 2 physician reviewers, with P<1010 for all category tags, rejecting the null hypothesis that the 2 reviewers could have independently arrived at their similar category tags by chance. Further assessment of inter‐rater agreement was generally excellent, with >0.8 in the majority of cases. The remaining cases (ie, Hgb 78, per other MD, anemia, cardiac) still had substantial agreement, with >0.6. These disagreements almost universally occurred when a reviewer assigned a subset of the category tags assigned by the other reviewer. For example, 1 reviewer may tag a comment bone marrow transplant (BMT) patient with Hgb 7.2 with BMT and Hgb 78, whereas the other would only cite BMT.
Figure 2 and Figure 3 highlight the varied categories of responses, with most categories comprising <4% of the available responses. Among the most common override reasons are structured protocols for specialty services, as in the stem cell transplant service, whose protocol recommends transfusing blood when Hgb <8 rather than Hgb <7. In these cases, the BPA is unlikely to change protocolized behavior, thus overall workflow would likely be improved by adding a structured BPA override option for these protocols.
Analysis of the override comments did expose some relatively common and questionable transfusion practices, including perioperative and periprocedural transfusions, as well as anticipation of imminent discharge. Prior studies indicate that transfusions in anticipation of surgical or procedural intervention provide no benefit compared to responding to blood requirements intraoperatively as needed,[3, 22, 23] and imminent discharge of a patient is not a well‐recognized reason to transfuse outside of guidelines. The identification of these questionable and relatively common practices identifies opportunities for targeted education and training campaigns.
Symptomatic anemia was 1 of the more commonly cited BPA override reasons with a fraction providing a specific symptom such as fatigue, lightheadedness, or shortness of breath. Although the pervasiveness of this rationale may speak to adding it as a structured BPA override option, the nonspecific, subjective, and nonevidence‐based nature of symptom‐driven blood transfusions suggests that ongoing interruptive BPA prompts can still be useful to remind providers of the risks and guideline‐based approaches to such cases.
Limitations of the analysis are revealed as a fraction of BPA events did not fully record all relevant data, preventing proper analysis. Override comments suggest the BPA was triggering inappropriately for patients appropriately below the recommended transfusion threshold of Hgb <7, assuming provider free‐textentered values were accurate. Review of these cases showed some variability, such as when providers based their transfusion decision on a hematocrit of 20 rather than a Hgb of 7. Many comments also stated nonthreshold Hgb values, such as Hgb 7.2, seeming to imply that the value was close enough to the recommended threshold to justify overriding the transfusion prompt.
The most significant limitation of this study is the substantial fraction of nonresponder BPA transfusion overrides with a nonspecific other reason and no text commentary, comprising 34% (3670/10,642) of all BPA interactions and 62% (3670/5886) of all other overrides. Although the BPA is easily overridden by design to avoid workflow disruption that could compromise the priority of patient care, the nonresponses raise concern for skewed interpretation of the override data. General studies in survey responses provide reassurance that lower response rates do not necessarily indicate response bias,[24] with response rates as low as 25% yielding results statistically indistinguishable from more rigorous methods achieving >50% response rates.[25] In this specific case, response bias is better characterized by comparing ordering provider characteristics for the other overrides with and without free‐text comments. Specifically, Figure 4 shows the distribution of other overrides by provider type (job title) and provider home department (where available from physician department registries), separated by whether a free‐text comment was left. For each sub‐group, a 2 analysis compared the observed versus expected proportion of providers leaving comments based on the null hypothesis that leaving a comment was independent of membership in the subgroup. Similar proportions with nonsignificant P values suggest against significant response bias for most subgroups, but the data do indicate that this work likely under‐represents the opinion of fellows, physician assistants, and neurosurgeons, while slightly over‐representing the opinion of medicine, general surgery, and obstetrics/gynecology practitioners. Given that the under‐represented groups overall constitute a small minority of the total BPA interactions, this work should still be generalizable to the majority of transfusion behavior.
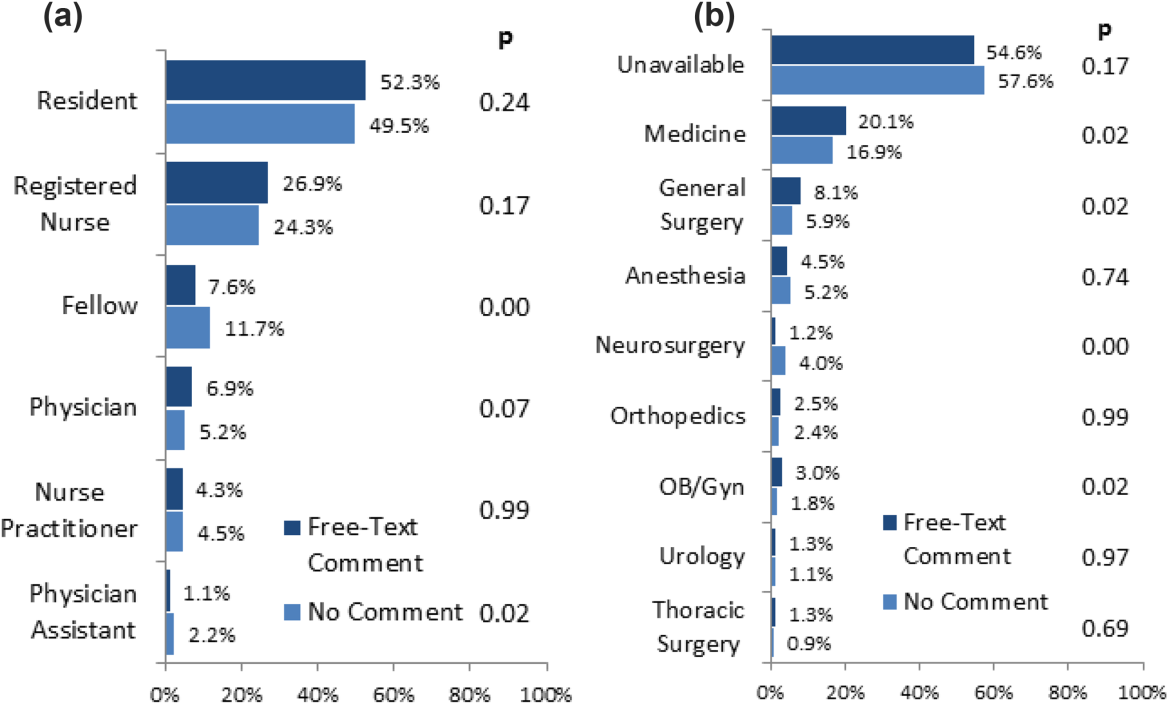
Further review of the ordering provider type (job title) distribution indicates that the vast majority of providers who actually interact with BPAs in this academic hospital are resident physicians. Similarly notable is that 25% of BPAs trigger for registered nurses. Upon review of hospital practices, we confirmed that nurses can enter transfusion orders on behalf of MD cosigners, generally in the context of a verbal order or routine treatment protocol, most notably on the stem cell transplant service. The prevalence of resident, nurse, nurse practitioner, and physician assistant order entry overall indicates that the providers encountering the BPA often do not have the negotiating power to accept or override the prompts, specifically defying the meaningful use goal of decision‐making providers directly interacting with CPOE and decision support prompts.[26]
The theoretical intelligence of the BPA not to trigger in well‐accepted cases of transfusion, where acute bleeding is occurring based on the presence of bleeding related items in the patient's problem list, is demonstrated to be limited. Acute bleeding represented the most common structured override response. This is likely multifactorial, but is largely the result of inconsistent usage of the patient problem lists that the BPA would depend upon. Another commonly cited override explanation was for a dropping Hgb without a specific bleeding source. This could point toward the BPA triggering an algorithm incorporating the last several Hgb values to assess the trajectory. Even then, however, guidelines would advocate holding transfusion and proceeding with serial monitoring of Hgb levels until an accepted transfusion threshold was actually breached.
This analysis demonstrates a general approach to secondary analysis of EMR data, in this case to provide insight into the specific question of why providers continue to order costly, unnecessary, and potentially harmful blood transfusions in spite of an interruptive BPA CDS tool. Limitations of the BPA are now better understood, including technical aspects of accounting for all decision‐making data, the frequency of nonresponse for override rationale, and that the providers interacting with the BPAs often do not have the negotiating power to change ordering behavior. The analysis recognizes protocolized behaviors that should be integrated into the CDS algorithms, and specification of symptomatic anemia as a common albeit subjective indication for blood transfusion. A wide swath of individually uncommon rationales drive transfusion override behavior, motivating further EMR‐based analysis to more efficiently recognize common scenarios (eg, perioperative/procedural, imminent discharge) for directed education and training interventions than can be achieved by conventional chart review.
Acknowledgements
The authors thank Sylvia Bereknyei for consultation on qualitative analysis methods, C.J. Garst for providing the dataset, David Iberri for contributing to early manuscript drafts, Paul Maggio for designing the original BPA, and Debra Green and Brett Toensing for providing staff department registries.
Disclosure: Nothing to report.
Transfusion of blood products has multiple clinical applications, yet when used outside of recommended guidelines it is associated with increased cost, waste, morbidity, and mortality.[1] Studies reviewing restrictive versus liberal blood transfusion strategies have demonstrated no benefit to liberal strategies over restrictive strategies[2, 3, 4, 5, 6, 7, 8, 9, 10] and possibly even harm.[11] Given the risks and real costs of blood transfusions occurring outside of recommended guidelines, professional societies in hematology, anesthesiology, and hospital medicine each include excessive blood transfusions among their top 5 questionable clinical practices in the American Board of Internal Medicine Foundation's Choosing Wisely initiative.[12] To modify behaviors around blood transfusion practices, hospitals and blood banks may need to provide clinical decision support (CDS) for physicians.
The conventional approach to CDS is direct education and training campaigns, but there is a unique opportunity for intelligent decision support at the point of care through electronic medical record (EMR) systems directly integrated with computerized physician order entry (CPOE).[13, 14, 15] Prior work at Stanford toward reducing unnecessary blood transfusions started with hospital‐wide education campaigns, which brought down the percentage of transfusions ordered for patients with hemoglobin (Hgb) >8 g/dL from 57% to 52%. Further reduction to <30% was achieved after the introduction of an interruptive best practice alert (BPA) integrated into the CPOE transfusion ordering process.[16, 17] Specifically, providers attempting to order a blood transfusion for patients with an EMR‐determined Hgb >7 are presented with a BPA popup reminding them of best practice guidelines and a prompt to either abort the transfusion or provide a reason to override the BPA and proceed.
It remains uncertain why up to 30% of transfusions continue to occur outside of recommended guidelines in spite of interruptive prompts. This study demonstrates a general approach to secondary use of clinical data from the EMR toward understanding provider behavior, specifically by analyzing free‐text comments linked to transfusion override behavior and identifying the type of providers interacting with the BPAs.
MATERIALS AND METHODS
At Stanford University Hospital, a 447‐bed academic tertiary care center servicing adult patients, clinicians order blood transfusions through an EMR+CPOE system. When an order for red blood cell transfusion is attempted, the EMR evaluates the patient chart for specific criteria based on previously published guidelines.[18] Specifically, the BPA will review the last recorded Hgb value and trigger if the Hgb is >8, or if the Hgb is >7 and there is no concurrent EMR problem‐list entry related to acute coronary syndrome or acute hemorrhage. Once the blood transfusion BPA triggers, the ordering provider is presented with an interruptive prompt reminding them of best practice guidelines and the 3 most recent Hgb values for the patient.[16] From there, the provider may either abort the transfusion or override the BPA and proceed. Overrides require the provider to select a reason from a predefined list of institutionally accepted transfusion indications including acute bleeding, acute coronary syndrome and Hgb <8, and postoperative cardiothoracic surgery and Hgb <8. If none of the predefined override reasons are selected, the provider simply selects other, with the option of a free‐text comment to elaborate their rationale.
Data from provider interactions with the BPA were collected from the EMR across all inpatient wards for 8 months after the implementation period. Data collected from each interaction included the patient identifier, alert description, action taken, ordering provider identifier, ordering provider type (job title), optional comments for overriding the BPA, and date and time.
The free‐text override comments were independently reviewed by 2 licensed physicians, tagging them into a set of general categories by iterative inductive analysis of the comment content. Individual comments were allowed multiple possible category tags (eg, coronary artery disease and symptomatic). The initial tagging process was expedited by isolating common keywords in the override comments and assigning likely category tags to each, as in Table 2. The 2 physician reviewers then manually inspected all comments with the option to revise the initial category tagging to ensure validity. Many category tags occurred sporadically and were aggregated into more general categories, such as hematologic disorder (includes myelodysplastic syndrome, myelofibrosis, multiple myeloma, sickle cell, thalassemia, Waldenstrom's), symptomatic (includes fatigue, lightheaded, short of breath), and per other medical doctor [MD] (includes any other physician direction, primarily specialty consultation services).
To assess the inter‐rater agreement of this category tagging between the 2 reviewers, a confusion matrix similar to the example in Table 1 was setup for each tag used. Several agreement statistics are calculated based on the confusion matrix, including the positive agreement rate (Pa+) and Cohen's kappa statistic (). Kappa statistic values range from 1 to +1, with values <0 indicating no agreement and values >0.8 indicating near perfect agreement.[19] To reject the null hypothesis that the 2 reviewers could have independently arrived at their similar tagging assignments by chance, a 2 test was applied for each confusion matrix, with Yates' correction to avoid overestimating statistical significance given the low rates of inter‐rater disagreement.[20]
Reviewer 1 Tags "Surgery" | ||||
---|---|---|---|---|
Yes | No | Total | ||
| ||||
Reviewer 2 Tags "Surgery" | Yes | 143 | 11 | 154 |
No | 5 | 820 | 825 | |
Total | 148 | 831 | 979 |
Category Tag | Keyword | Keyword Count |
---|---|---|
| ||
Hgb 78 | 7.1, 7.2, 7.8, 7.9, 8, <8 | 360 |
BMT | BMT | 359 |
Symptomatic | Symptomatic | 187 |
Surgery | Postop, post‐op, surgery, surgical | 176 |
Dropping Hgb | Down, drop, dropping | 117 |
Chemotherapy | Chemo, chemotherapy | 88 |
Per other MD | Per | 87 |
Transplant | Transplant | 70 |
Cardiac | Cardiac | 66 |
Bleeding | Bleeding | 65 |
Procedure | Procedure | 65 |
Hgb <7 | 7 | 58 |
Hypotension | Hypotension | 51 |
Protocol | Protocol | 51 |
Cirrhosis | Liver | 50 |
Imminent discharge | Discharge | 49 |
Leukemia, acute | AML | 44 |
Cancer | Cancer | 37 |
Sepsis | Sepsis | 32 |
Tachycardia | Tachycardia | 28 |
RESULTS
During the data collection period from January 1, 2011 to August 31, 2012, the blood transfusion BPA fired in 11,791 instances, of which 10,642 recorded sufficient data for analysis (Figure 1). The ordering provider proceeded to override the BPA and continued with transfusion in 98% of cases (10,442/10,642). Acute bleeding" was the most common structured response (34%). The majority of BPA overrides used the general purpose other structured response option accounting for 56% (5886/10,442) of override responses, of which 37% (2185/5886) entered a free‐text comment elaborating the override reason. With 3701 nonresponders (other overrides with no free‐text comment), the overall response rate was 65% (6941/10,642).
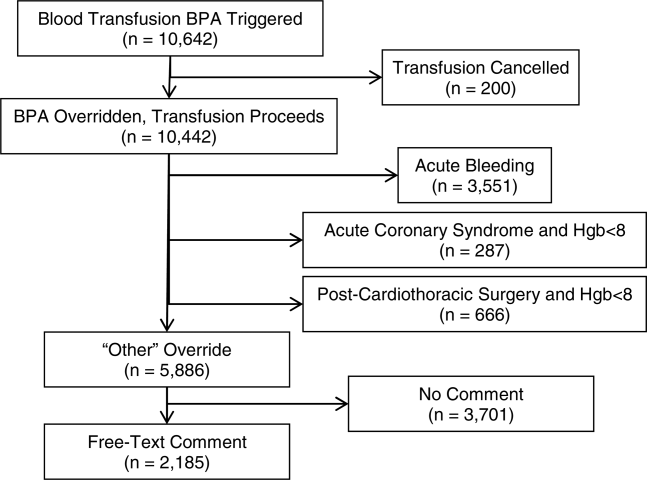
With a handful of free‐text comments included with structured override responses (eg, 28 acute bleeding overrides included additional comment from the provider), a total of 2216 override comments were available for analysis. Using an initial selection of keyword‐tag associations, as in Table 2, 95% (2104/2216) of the override comments had a preliminary category tagging assigned. After manual review and revision by the first physician reviewer, 74% (1633/2216) of the comments retained their automated tags, whereas 26% (583/2216) were updated based on the reviewer's assessment of validity. This included 112 comments lacking automated tags the reviewer manually added, as well as 471 comments with automated tags revised by the reviewer.
The most common override category tags are presented in Figure 2 and Figure 3 for BPAs triggered in response to blood transfusions ordered for patients with Hgb 78 and Hgb >8, respectively. The agreed+ percentages correspond to the number of comments where the 2 physician reviewers both assigned the respective category tag (Pa+), whereas the disagreed percentages correspond to comments where the reviewers differed (Pd=1‐Pa). By Yates' corrected 2 analysis, P was <1010 for independence between reviewer tag assignments for all tags assessed. Kappa statistics are reported in the figures to describe inter‐rater agreement.
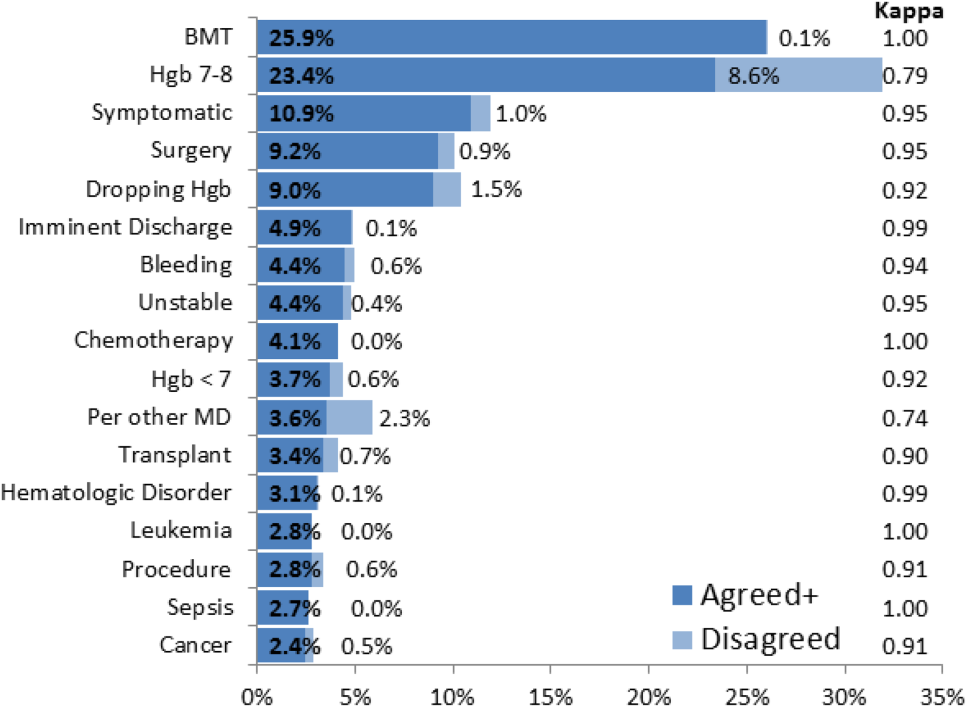
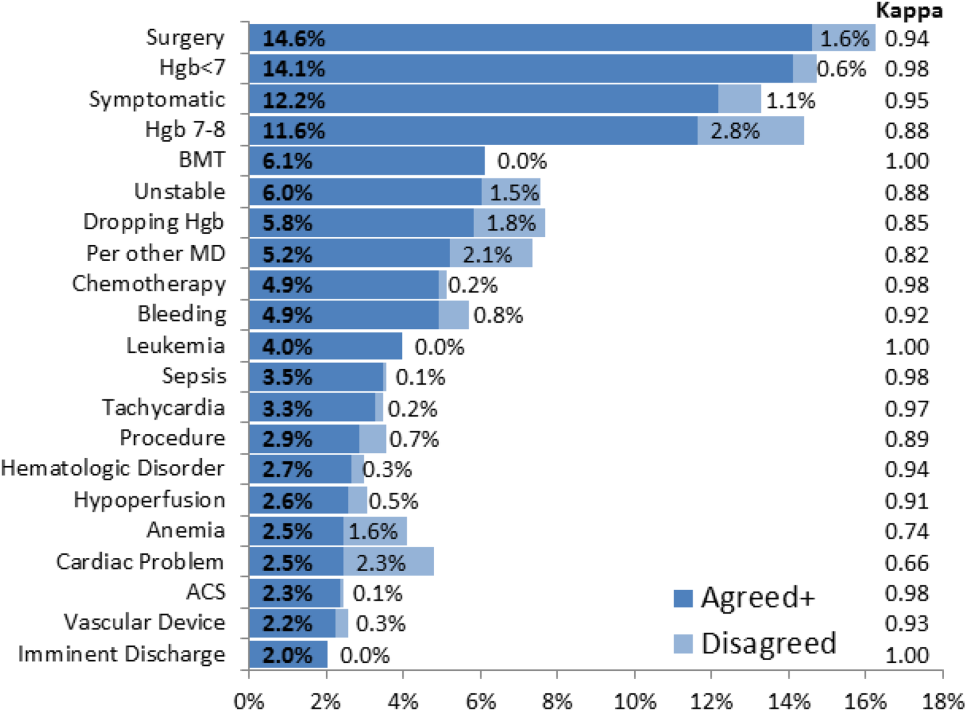
Resident physicians were the primary ordering provider group, accounting for approximately 55% (5863/10,642) of BPA interactions, followed by registered nurses, fellows, and attending physicians.
DISCUSSION
Prior work has established that an interruptive BPA to provide decision support against inappropriate blood transfusions can drive a significant and sustained reduction in unnecessary transfusions,[16] especially when satisfying the primary features of effective decision support.[14] The quantity of transfusions directly aborted by the BPA (only 2% in this case) does not nearly account for the total observed reductions in transfusions, suggesting that the BPA yields an indirect teaching effect over time beyond direct interruption. In other words, once a user has been interrupted by the BPA several times, they will be discouraged from even attempting to order inappropriate blood transfusions in the future.
Despite the improvements above, there remains a substantial fraction (up to 30%) of blood transfusions occurring outside of recommended guidelines where providers specifically override the BPA prompt.[21] This work is the first structured analysis of such BPA override behavior by generalizable methods of EMR data analysis to guide further improvements.
Analysis of the override behavior focused primarily on the free‐text comments explaining provider rationales for overriding the BPA. These comments were categorized by 2 physician reviewers, with P<1010 for all category tags, rejecting the null hypothesis that the 2 reviewers could have independently arrived at their similar category tags by chance. Further assessment of inter‐rater agreement was generally excellent, with >0.8 in the majority of cases. The remaining cases (ie, Hgb 78, per other MD, anemia, cardiac) still had substantial agreement, with >0.6. These disagreements almost universally occurred when a reviewer assigned a subset of the category tags assigned by the other reviewer. For example, 1 reviewer may tag a comment bone marrow transplant (BMT) patient with Hgb 7.2 with BMT and Hgb 78, whereas the other would only cite BMT.
Figure 2 and Figure 3 highlight the varied categories of responses, with most categories comprising <4% of the available responses. Among the most common override reasons are structured protocols for specialty services, as in the stem cell transplant service, whose protocol recommends transfusing blood when Hgb <8 rather than Hgb <7. In these cases, the BPA is unlikely to change protocolized behavior, thus overall workflow would likely be improved by adding a structured BPA override option for these protocols.
Analysis of the override comments did expose some relatively common and questionable transfusion practices, including perioperative and periprocedural transfusions, as well as anticipation of imminent discharge. Prior studies indicate that transfusions in anticipation of surgical or procedural intervention provide no benefit compared to responding to blood requirements intraoperatively as needed,[3, 22, 23] and imminent discharge of a patient is not a well‐recognized reason to transfuse outside of guidelines. The identification of these questionable and relatively common practices identifies opportunities for targeted education and training campaigns.
Symptomatic anemia was 1 of the more commonly cited BPA override reasons with a fraction providing a specific symptom such as fatigue, lightheadedness, or shortness of breath. Although the pervasiveness of this rationale may speak to adding it as a structured BPA override option, the nonspecific, subjective, and nonevidence‐based nature of symptom‐driven blood transfusions suggests that ongoing interruptive BPA prompts can still be useful to remind providers of the risks and guideline‐based approaches to such cases.
Limitations of the analysis are revealed as a fraction of BPA events did not fully record all relevant data, preventing proper analysis. Override comments suggest the BPA was triggering inappropriately for patients appropriately below the recommended transfusion threshold of Hgb <7, assuming provider free‐textentered values were accurate. Review of these cases showed some variability, such as when providers based their transfusion decision on a hematocrit of 20 rather than a Hgb of 7. Many comments also stated nonthreshold Hgb values, such as Hgb 7.2, seeming to imply that the value was close enough to the recommended threshold to justify overriding the transfusion prompt.
The most significant limitation of this study is the substantial fraction of nonresponder BPA transfusion overrides with a nonspecific other reason and no text commentary, comprising 34% (3670/10,642) of all BPA interactions and 62% (3670/5886) of all other overrides. Although the BPA is easily overridden by design to avoid workflow disruption that could compromise the priority of patient care, the nonresponses raise concern for skewed interpretation of the override data. General studies in survey responses provide reassurance that lower response rates do not necessarily indicate response bias,[24] with response rates as low as 25% yielding results statistically indistinguishable from more rigorous methods achieving >50% response rates.[25] In this specific case, response bias is better characterized by comparing ordering provider characteristics for the other overrides with and without free‐text comments. Specifically, Figure 4 shows the distribution of other overrides by provider type (job title) and provider home department (where available from physician department registries), separated by whether a free‐text comment was left. For each sub‐group, a 2 analysis compared the observed versus expected proportion of providers leaving comments based on the null hypothesis that leaving a comment was independent of membership in the subgroup. Similar proportions with nonsignificant P values suggest against significant response bias for most subgroups, but the data do indicate that this work likely under‐represents the opinion of fellows, physician assistants, and neurosurgeons, while slightly over‐representing the opinion of medicine, general surgery, and obstetrics/gynecology practitioners. Given that the under‐represented groups overall constitute a small minority of the total BPA interactions, this work should still be generalizable to the majority of transfusion behavior.
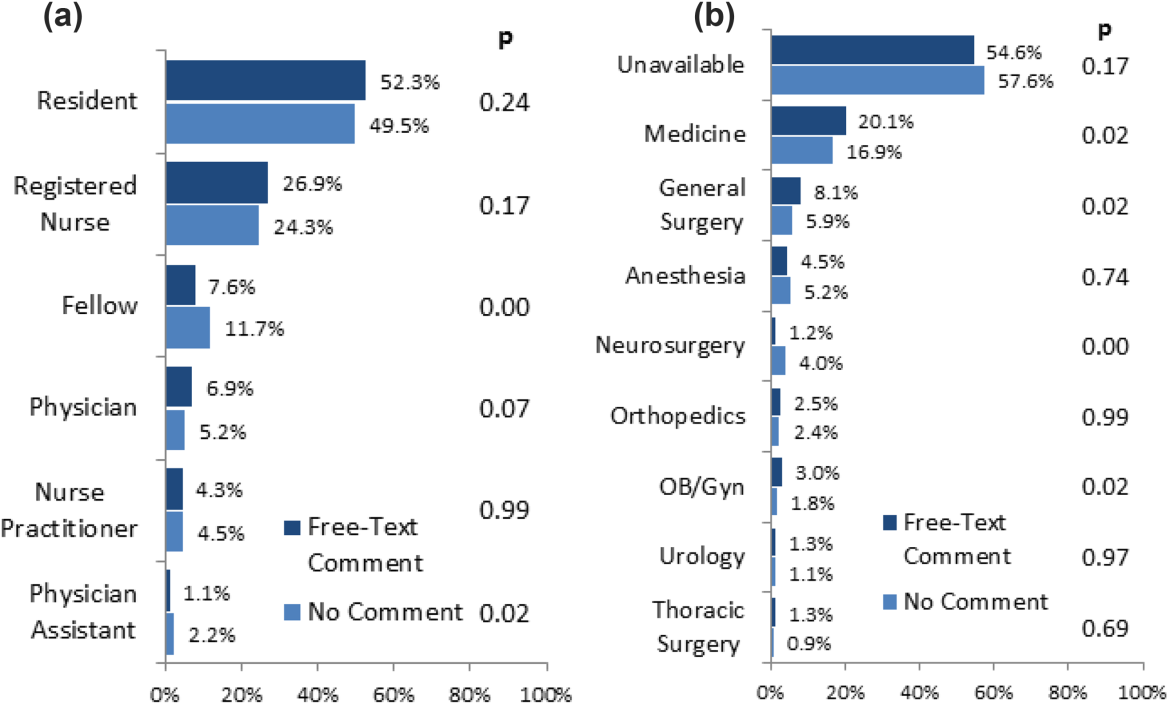
Further review of the ordering provider type (job title) distribution indicates that the vast majority of providers who actually interact with BPAs in this academic hospital are resident physicians. Similarly notable is that 25% of BPAs trigger for registered nurses. Upon review of hospital practices, we confirmed that nurses can enter transfusion orders on behalf of MD cosigners, generally in the context of a verbal order or routine treatment protocol, most notably on the stem cell transplant service. The prevalence of resident, nurse, nurse practitioner, and physician assistant order entry overall indicates that the providers encountering the BPA often do not have the negotiating power to accept or override the prompts, specifically defying the meaningful use goal of decision‐making providers directly interacting with CPOE and decision support prompts.[26]
The theoretical intelligence of the BPA not to trigger in well‐accepted cases of transfusion, where acute bleeding is occurring based on the presence of bleeding related items in the patient's problem list, is demonstrated to be limited. Acute bleeding represented the most common structured override response. This is likely multifactorial, but is largely the result of inconsistent usage of the patient problem lists that the BPA would depend upon. Another commonly cited override explanation was for a dropping Hgb without a specific bleeding source. This could point toward the BPA triggering an algorithm incorporating the last several Hgb values to assess the trajectory. Even then, however, guidelines would advocate holding transfusion and proceeding with serial monitoring of Hgb levels until an accepted transfusion threshold was actually breached.
This analysis demonstrates a general approach to secondary analysis of EMR data, in this case to provide insight into the specific question of why providers continue to order costly, unnecessary, and potentially harmful blood transfusions in spite of an interruptive BPA CDS tool. Limitations of the BPA are now better understood, including technical aspects of accounting for all decision‐making data, the frequency of nonresponse for override rationale, and that the providers interacting with the BPAs often do not have the negotiating power to change ordering behavior. The analysis recognizes protocolized behaviors that should be integrated into the CDS algorithms, and specification of symptomatic anemia as a common albeit subjective indication for blood transfusion. A wide swath of individually uncommon rationales drive transfusion override behavior, motivating further EMR‐based analysis to more efficiently recognize common scenarios (eg, perioperative/procedural, imminent discharge) for directed education and training interventions than can be achieved by conventional chart review.
Acknowledgements
The authors thank Sylvia Bereknyei for consultation on qualitative analysis methods, C.J. Garst for providing the dataset, David Iberri for contributing to early manuscript drafts, Paul Maggio for designing the original BPA, and Debra Green and Brett Toensing for providing staff department registries.
Disclosure: Nothing to report.
Transfusion of blood products has multiple clinical applications, yet when used outside of recommended guidelines it is associated with increased cost, waste, morbidity, and mortality.[1] Studies reviewing restrictive versus liberal blood transfusion strategies have demonstrated no benefit to liberal strategies over restrictive strategies[2, 3, 4, 5, 6, 7, 8, 9, 10] and possibly even harm.[11] Given the risks and real costs of blood transfusions occurring outside of recommended guidelines, professional societies in hematology, anesthesiology, and hospital medicine each include excessive blood transfusions among their top 5 questionable clinical practices in the American Board of Internal Medicine Foundation's Choosing Wisely initiative.[12] To modify behaviors around blood transfusion practices, hospitals and blood banks may need to provide clinical decision support (CDS) for physicians.
The conventional approach to CDS is direct education and training campaigns, but there is a unique opportunity for intelligent decision support at the point of care through electronic medical record (EMR) systems directly integrated with computerized physician order entry (CPOE).[13, 14, 15] Prior work at Stanford toward reducing unnecessary blood transfusions started with hospital‐wide education campaigns, which brought down the percentage of transfusions ordered for patients with hemoglobin (Hgb) >8 g/dL from 57% to 52%. Further reduction to <30% was achieved after the introduction of an interruptive best practice alert (BPA) integrated into the CPOE transfusion ordering process.[16, 17] Specifically, providers attempting to order a blood transfusion for patients with an EMR‐determined Hgb >7 are presented with a BPA popup reminding them of best practice guidelines and a prompt to either abort the transfusion or provide a reason to override the BPA and proceed.
It remains uncertain why up to 30% of transfusions continue to occur outside of recommended guidelines in spite of interruptive prompts. This study demonstrates a general approach to secondary use of clinical data from the EMR toward understanding provider behavior, specifically by analyzing free‐text comments linked to transfusion override behavior and identifying the type of providers interacting with the BPAs.
MATERIALS AND METHODS
At Stanford University Hospital, a 447‐bed academic tertiary care center servicing adult patients, clinicians order blood transfusions through an EMR+CPOE system. When an order for red blood cell transfusion is attempted, the EMR evaluates the patient chart for specific criteria based on previously published guidelines.[18] Specifically, the BPA will review the last recorded Hgb value and trigger if the Hgb is >8, or if the Hgb is >7 and there is no concurrent EMR problem‐list entry related to acute coronary syndrome or acute hemorrhage. Once the blood transfusion BPA triggers, the ordering provider is presented with an interruptive prompt reminding them of best practice guidelines and the 3 most recent Hgb values for the patient.[16] From there, the provider may either abort the transfusion or override the BPA and proceed. Overrides require the provider to select a reason from a predefined list of institutionally accepted transfusion indications including acute bleeding, acute coronary syndrome and Hgb <8, and postoperative cardiothoracic surgery and Hgb <8. If none of the predefined override reasons are selected, the provider simply selects other, with the option of a free‐text comment to elaborate their rationale.
Data from provider interactions with the BPA were collected from the EMR across all inpatient wards for 8 months after the implementation period. Data collected from each interaction included the patient identifier, alert description, action taken, ordering provider identifier, ordering provider type (job title), optional comments for overriding the BPA, and date and time.
The free‐text override comments were independently reviewed by 2 licensed physicians, tagging them into a set of general categories by iterative inductive analysis of the comment content. Individual comments were allowed multiple possible category tags (eg, coronary artery disease and symptomatic). The initial tagging process was expedited by isolating common keywords in the override comments and assigning likely category tags to each, as in Table 2. The 2 physician reviewers then manually inspected all comments with the option to revise the initial category tagging to ensure validity. Many category tags occurred sporadically and were aggregated into more general categories, such as hematologic disorder (includes myelodysplastic syndrome, myelofibrosis, multiple myeloma, sickle cell, thalassemia, Waldenstrom's), symptomatic (includes fatigue, lightheaded, short of breath), and per other medical doctor [MD] (includes any other physician direction, primarily specialty consultation services).
To assess the inter‐rater agreement of this category tagging between the 2 reviewers, a confusion matrix similar to the example in Table 1 was setup for each tag used. Several agreement statistics are calculated based on the confusion matrix, including the positive agreement rate (Pa+) and Cohen's kappa statistic (). Kappa statistic values range from 1 to +1, with values <0 indicating no agreement and values >0.8 indicating near perfect agreement.[19] To reject the null hypothesis that the 2 reviewers could have independently arrived at their similar tagging assignments by chance, a 2 test was applied for each confusion matrix, with Yates' correction to avoid overestimating statistical significance given the low rates of inter‐rater disagreement.[20]
Reviewer 1 Tags "Surgery" | ||||
---|---|---|---|---|
Yes | No | Total | ||
| ||||
Reviewer 2 Tags "Surgery" | Yes | 143 | 11 | 154 |
No | 5 | 820 | 825 | |
Total | 148 | 831 | 979 |
Category Tag | Keyword | Keyword Count |
---|---|---|
| ||
Hgb 78 | 7.1, 7.2, 7.8, 7.9, 8, <8 | 360 |
BMT | BMT | 359 |
Symptomatic | Symptomatic | 187 |
Surgery | Postop, post‐op, surgery, surgical | 176 |
Dropping Hgb | Down, drop, dropping | 117 |
Chemotherapy | Chemo, chemotherapy | 88 |
Per other MD | Per | 87 |
Transplant | Transplant | 70 |
Cardiac | Cardiac | 66 |
Bleeding | Bleeding | 65 |
Procedure | Procedure | 65 |
Hgb <7 | 7 | 58 |
Hypotension | Hypotension | 51 |
Protocol | Protocol | 51 |
Cirrhosis | Liver | 50 |
Imminent discharge | Discharge | 49 |
Leukemia, acute | AML | 44 |
Cancer | Cancer | 37 |
Sepsis | Sepsis | 32 |
Tachycardia | Tachycardia | 28 |
RESULTS
During the data collection period from January 1, 2011 to August 31, 2012, the blood transfusion BPA fired in 11,791 instances, of which 10,642 recorded sufficient data for analysis (Figure 1). The ordering provider proceeded to override the BPA and continued with transfusion in 98% of cases (10,442/10,642). Acute bleeding" was the most common structured response (34%). The majority of BPA overrides used the general purpose other structured response option accounting for 56% (5886/10,442) of override responses, of which 37% (2185/5886) entered a free‐text comment elaborating the override reason. With 3701 nonresponders (other overrides with no free‐text comment), the overall response rate was 65% (6941/10,642).
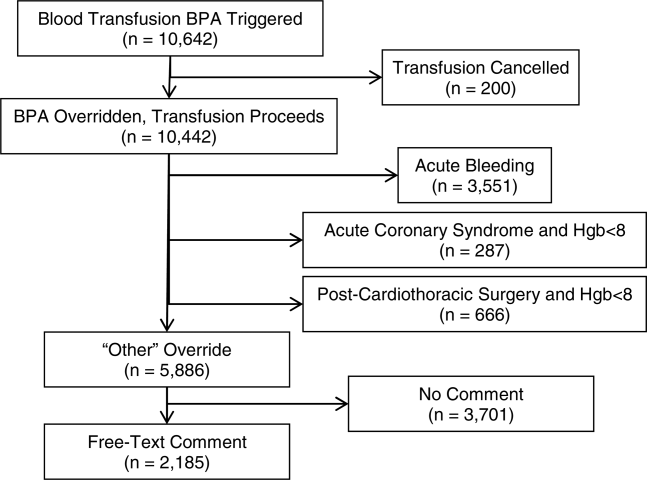
With a handful of free‐text comments included with structured override responses (eg, 28 acute bleeding overrides included additional comment from the provider), a total of 2216 override comments were available for analysis. Using an initial selection of keyword‐tag associations, as in Table 2, 95% (2104/2216) of the override comments had a preliminary category tagging assigned. After manual review and revision by the first physician reviewer, 74% (1633/2216) of the comments retained their automated tags, whereas 26% (583/2216) were updated based on the reviewer's assessment of validity. This included 112 comments lacking automated tags the reviewer manually added, as well as 471 comments with automated tags revised by the reviewer.
The most common override category tags are presented in Figure 2 and Figure 3 for BPAs triggered in response to blood transfusions ordered for patients with Hgb 78 and Hgb >8, respectively. The agreed+ percentages correspond to the number of comments where the 2 physician reviewers both assigned the respective category tag (Pa+), whereas the disagreed percentages correspond to comments where the reviewers differed (Pd=1‐Pa). By Yates' corrected 2 analysis, P was <1010 for independence between reviewer tag assignments for all tags assessed. Kappa statistics are reported in the figures to describe inter‐rater agreement.
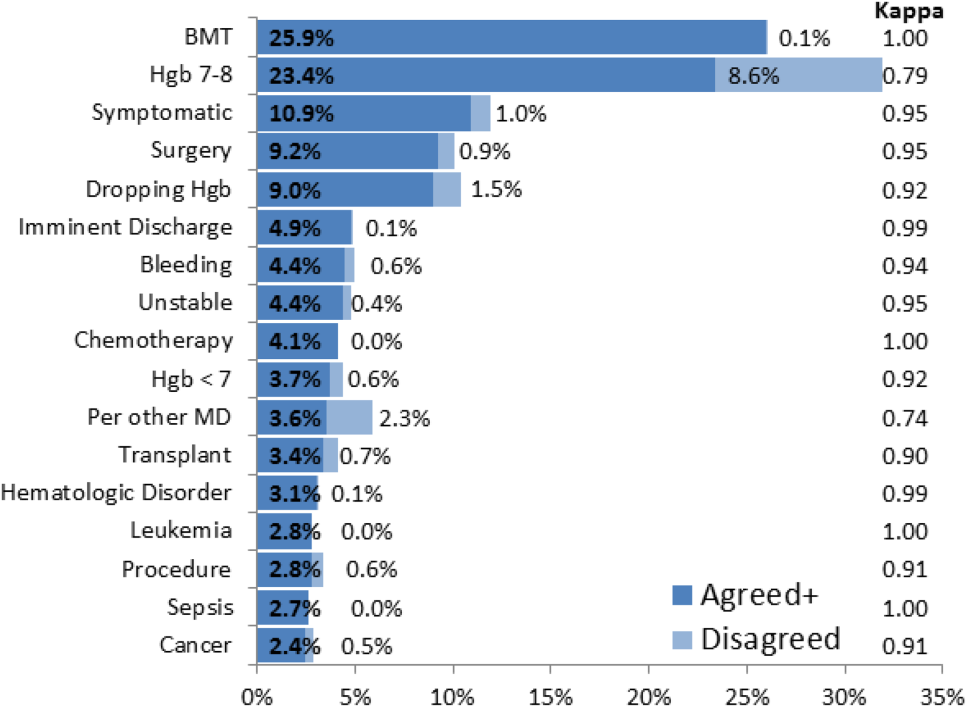
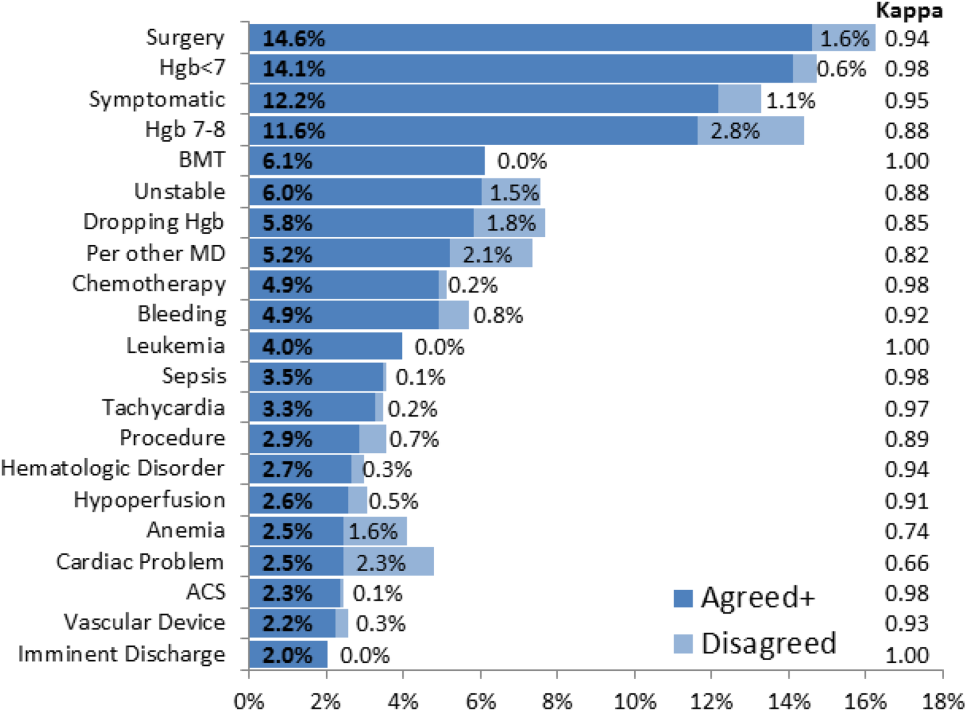
Resident physicians were the primary ordering provider group, accounting for approximately 55% (5863/10,642) of BPA interactions, followed by registered nurses, fellows, and attending physicians.
DISCUSSION
Prior work has established that an interruptive BPA to provide decision support against inappropriate blood transfusions can drive a significant and sustained reduction in unnecessary transfusions,[16] especially when satisfying the primary features of effective decision support.[14] The quantity of transfusions directly aborted by the BPA (only 2% in this case) does not nearly account for the total observed reductions in transfusions, suggesting that the BPA yields an indirect teaching effect over time beyond direct interruption. In other words, once a user has been interrupted by the BPA several times, they will be discouraged from even attempting to order inappropriate blood transfusions in the future.
Despite the improvements above, there remains a substantial fraction (up to 30%) of blood transfusions occurring outside of recommended guidelines where providers specifically override the BPA prompt.[21] This work is the first structured analysis of such BPA override behavior by generalizable methods of EMR data analysis to guide further improvements.
Analysis of the override behavior focused primarily on the free‐text comments explaining provider rationales for overriding the BPA. These comments were categorized by 2 physician reviewers, with P<1010 for all category tags, rejecting the null hypothesis that the 2 reviewers could have independently arrived at their similar category tags by chance. Further assessment of inter‐rater agreement was generally excellent, with >0.8 in the majority of cases. The remaining cases (ie, Hgb 78, per other MD, anemia, cardiac) still had substantial agreement, with >0.6. These disagreements almost universally occurred when a reviewer assigned a subset of the category tags assigned by the other reviewer. For example, 1 reviewer may tag a comment bone marrow transplant (BMT) patient with Hgb 7.2 with BMT and Hgb 78, whereas the other would only cite BMT.
Figure 2 and Figure 3 highlight the varied categories of responses, with most categories comprising <4% of the available responses. Among the most common override reasons are structured protocols for specialty services, as in the stem cell transplant service, whose protocol recommends transfusing blood when Hgb <8 rather than Hgb <7. In these cases, the BPA is unlikely to change protocolized behavior, thus overall workflow would likely be improved by adding a structured BPA override option for these protocols.
Analysis of the override comments did expose some relatively common and questionable transfusion practices, including perioperative and periprocedural transfusions, as well as anticipation of imminent discharge. Prior studies indicate that transfusions in anticipation of surgical or procedural intervention provide no benefit compared to responding to blood requirements intraoperatively as needed,[3, 22, 23] and imminent discharge of a patient is not a well‐recognized reason to transfuse outside of guidelines. The identification of these questionable and relatively common practices identifies opportunities for targeted education and training campaigns.
Symptomatic anemia was 1 of the more commonly cited BPA override reasons with a fraction providing a specific symptom such as fatigue, lightheadedness, or shortness of breath. Although the pervasiveness of this rationale may speak to adding it as a structured BPA override option, the nonspecific, subjective, and nonevidence‐based nature of symptom‐driven blood transfusions suggests that ongoing interruptive BPA prompts can still be useful to remind providers of the risks and guideline‐based approaches to such cases.
Limitations of the analysis are revealed as a fraction of BPA events did not fully record all relevant data, preventing proper analysis. Override comments suggest the BPA was triggering inappropriately for patients appropriately below the recommended transfusion threshold of Hgb <7, assuming provider free‐textentered values were accurate. Review of these cases showed some variability, such as when providers based their transfusion decision on a hematocrit of 20 rather than a Hgb of 7. Many comments also stated nonthreshold Hgb values, such as Hgb 7.2, seeming to imply that the value was close enough to the recommended threshold to justify overriding the transfusion prompt.
The most significant limitation of this study is the substantial fraction of nonresponder BPA transfusion overrides with a nonspecific other reason and no text commentary, comprising 34% (3670/10,642) of all BPA interactions and 62% (3670/5886) of all other overrides. Although the BPA is easily overridden by design to avoid workflow disruption that could compromise the priority of patient care, the nonresponses raise concern for skewed interpretation of the override data. General studies in survey responses provide reassurance that lower response rates do not necessarily indicate response bias,[24] with response rates as low as 25% yielding results statistically indistinguishable from more rigorous methods achieving >50% response rates.[25] In this specific case, response bias is better characterized by comparing ordering provider characteristics for the other overrides with and without free‐text comments. Specifically, Figure 4 shows the distribution of other overrides by provider type (job title) and provider home department (where available from physician department registries), separated by whether a free‐text comment was left. For each sub‐group, a 2 analysis compared the observed versus expected proportion of providers leaving comments based on the null hypothesis that leaving a comment was independent of membership in the subgroup. Similar proportions with nonsignificant P values suggest against significant response bias for most subgroups, but the data do indicate that this work likely under‐represents the opinion of fellows, physician assistants, and neurosurgeons, while slightly over‐representing the opinion of medicine, general surgery, and obstetrics/gynecology practitioners. Given that the under‐represented groups overall constitute a small minority of the total BPA interactions, this work should still be generalizable to the majority of transfusion behavior.
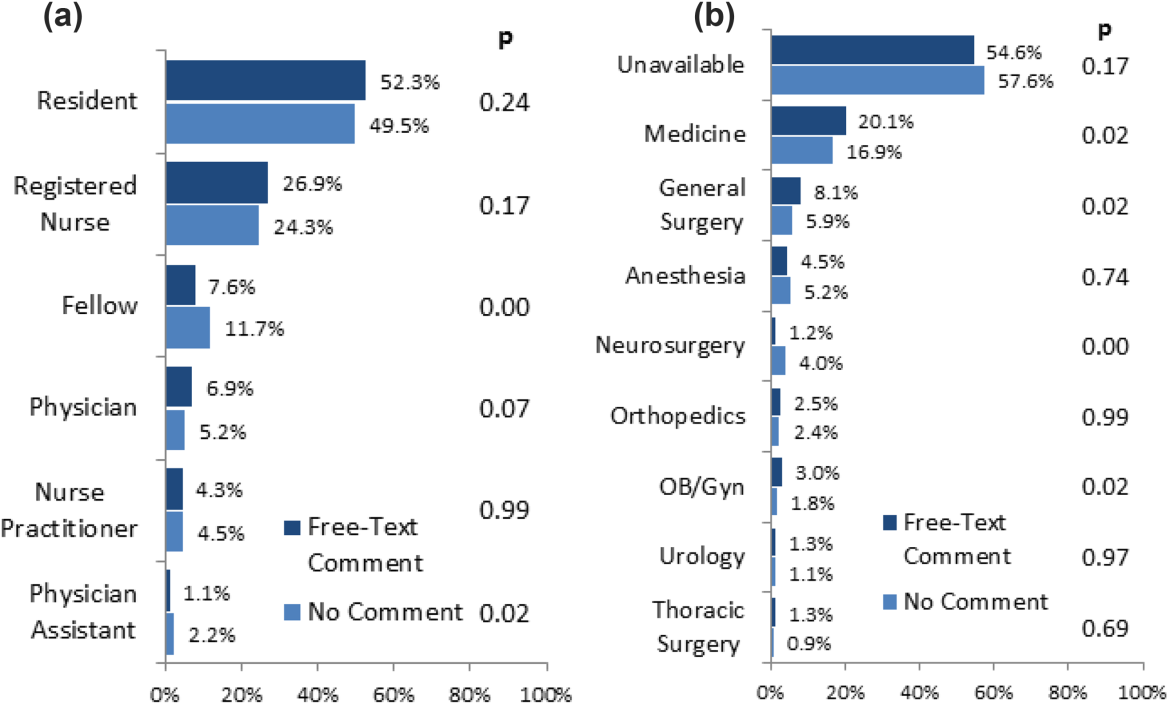
Further review of the ordering provider type (job title) distribution indicates that the vast majority of providers who actually interact with BPAs in this academic hospital are resident physicians. Similarly notable is that 25% of BPAs trigger for registered nurses. Upon review of hospital practices, we confirmed that nurses can enter transfusion orders on behalf of MD cosigners, generally in the context of a verbal order or routine treatment protocol, most notably on the stem cell transplant service. The prevalence of resident, nurse, nurse practitioner, and physician assistant order entry overall indicates that the providers encountering the BPA often do not have the negotiating power to accept or override the prompts, specifically defying the meaningful use goal of decision‐making providers directly interacting with CPOE and decision support prompts.[26]
The theoretical intelligence of the BPA not to trigger in well‐accepted cases of transfusion, where acute bleeding is occurring based on the presence of bleeding related items in the patient's problem list, is demonstrated to be limited. Acute bleeding represented the most common structured override response. This is likely multifactorial, but is largely the result of inconsistent usage of the patient problem lists that the BPA would depend upon. Another commonly cited override explanation was for a dropping Hgb without a specific bleeding source. This could point toward the BPA triggering an algorithm incorporating the last several Hgb values to assess the trajectory. Even then, however, guidelines would advocate holding transfusion and proceeding with serial monitoring of Hgb levels until an accepted transfusion threshold was actually breached.
This analysis demonstrates a general approach to secondary analysis of EMR data, in this case to provide insight into the specific question of why providers continue to order costly, unnecessary, and potentially harmful blood transfusions in spite of an interruptive BPA CDS tool. Limitations of the BPA are now better understood, including technical aspects of accounting for all decision‐making data, the frequency of nonresponse for override rationale, and that the providers interacting with the BPAs often do not have the negotiating power to change ordering behavior. The analysis recognizes protocolized behaviors that should be integrated into the CDS algorithms, and specification of symptomatic anemia as a common albeit subjective indication for blood transfusion. A wide swath of individually uncommon rationales drive transfusion override behavior, motivating further EMR‐based analysis to more efficiently recognize common scenarios (eg, perioperative/procedural, imminent discharge) for directed education and training interventions than can be achieved by conventional chart review.
Acknowledgements
The authors thank Sylvia Bereknyei for consultation on qualitative analysis methods, C.J. Garst for providing the dataset, David Iberri for contributing to early manuscript drafts, Paul Maggio for designing the original BPA, and Debra Green and Brett Toensing for providing staff department registries.
Disclosure: Nothing to report.
© 2014 Society of Hospital Medicine