User login
Post-Intensive Care Unit Psychiatric Comorbidity and Quality of Life
The prevalence of depression, anxiety, and posttraumatic stress disorder (PTSD) symptoms in intensive care unit (ICU) survivors ranges from 17% to 44%.1-4 Psychiatric comorbidity, the presence of 2 or more psychiatric disorders, is highly prevalent in survivors of acute respiratory distress syndrome and is associated with higher mortality in postsurgical ICU survivors.5-7 While long-term cognitive impairment in patients with ICU delirium has been associated with poor quality of life (QoL),1 the effects of psychiatric comorbidity on QoL among similar patients are not as well understood. In this study, we examined whether psychiatric comorbidity was associated with poorer QoL in survivors of ICU delirium.
METHODS
We examined subjects who participated in the Pharmacologic Management of Delirium (PMD) clinical trial. This trial examined the efficacy of a pharmacological intervention for patients who developed ICU delirium at a local tertiary-care academic hospital.8 Out of 62 patients who participated in the follow-up of the PMD study, 58 completed QoL interviews and validated psychiatric screens (Patient Health Questionnaire-9 [PHQ-9] for depression, the Generalized Anxiety Disorder-7 [GAD-7] questionnaire for anxiety, and the Post-Traumatic Stress Syndrome [PTSS-10] questionnaire for PTSD) at 3 months after hospital discharge. High psychiatric comorbidity was defined as having significant symptoms for all 3 conditions (depression: PHQ-9 score ≥ 10; anxiety: GAD-7 ≥ 10; and PTSD: PTSS-10 > 35). No psychiatric morbidity was defined as having no significant symptoms for all 3 conditions. Low to moderate (low-moderate) psychiatric morbidity was defined as having symptoms for 1 to 2 conditions.
Participants also completed 2 complementary QoL measures: the EuroQol 5 dimensions questionnaire 3-level (EQ-5D-3L) Index and the EuroQol 5 dimensions Visual Analog Scale (EQ-5D-VAS).9 The EQ-5D-3L Index asks participants to rate themselves as having (1) no problems, (2) some problems, or (3) extreme problems on the following 5 scales: mobility, self-care, usual activities, pain/discomfort, and anxiety/depression. The scores are then indexed against the US population to create a continuous index scale ranging from −0.11 to 1.00.
Fisher’s exact tests were used to compare dichotomous outcomes. Analysis of variance (ANOVA) was used to compare continuous outcomes across the 3 psychiatric groups. Analysis of covariance (ANCOVA) was used to determine whether psychiatric comorbidity in survivors of ICU delirium was associated with QoL measures. Models were adjusted for the following covariates: age, gender, Charlson Comorbidity Index, discharged to home, prior history of depression, and prior history of anxiety. To assess the relationship of psychiatric comorbidity with QoL, we chose the 2 continuous QoL measures as the outcome. Because we were interested in the effect of psychiatric burden on QoL, we used ANCOVA with QoL as the dependent variable and psychiatric burden as an independent variable. Pairwise comparisons were then performed when overall differences were significant (P < 0.05). We performed 2 separate sensitivity analyses. The first analysis looked solely at the subgroup of patients from the medical intensive care unit. We also recalculated the EQ-5D-3L index excluding the anxiety/depression item.
RESULTS
Nearly one-third of patients (18/58) had high psychiatric burden. The table looks at the demographic and clinical characteristics of patients with high psychiatric comorbidity versus those of low-moderate psychiatric comorbidity and those with no psychiatric morbidity. Patient groups did not differ significantly in terms of demographics. For clinical characteristics, patients with high psychiatric comorbidity were more likely than patients with low-moderate psychiatric comorbidity to have a prior history of depression (P < 0.05).
Patients with high psychiatric comorbidity were more likely to have a poorer QoL when compared with patients with low-moderate psychiatric comorbidity and to those with no morbidity as measured by a lower EQ-5D-3L Index (no, 0.69 ± 0.25; low-moderate, 0.70 ± 0.19; high, 0.48 ± 0.24; P = 0.006) and EQ-5D-VAS (no, 67.0 ± 20.7; low-moderate, 76.6 ± 20.0; high, 50.8 ± 22.4; P = 0.004). After adjusting for covariates, patients with high psychiatric comorbidity had a poorer QoL compared with those with no morbidity or low-moderate comorbidity on the EQ-5D-3L Index (P = 0.017 for overall differences), whereas patients who had high psychiatric comorbidity had a poorer QoL compared to those with low-moderate comorbidity on the EQ-5D-VAS (P = 0.039 for overall differences; Figure). Subgroup analysis of MICU patients yielded similar results. Patients with high psychiatric burden had significantly poorer QoL as measured by the EQ-5D-3L (unadjusted P = 0.044, adjusted P = 0.003) and the EQ-5D-VAS (unadjusted P = 0.007, adjusted P = 0.021). After excluding the anxiety/depression item from the EQ-5D-3L, we observed similar differences (no, 0.71 ± 0.24; low-moderate, 0.75 ± 0.15; high, 0.58 ± 0.22; unadjusted P = 0.062; adjusted P = 0.040).
DISCUSSION/CONCLUSION
Psychiatric comorbidities in ICU survivors are common and pose a significant clinical issue. Patients with multiple psychiatric comorbidities can be more complicated to identify from a diagnostic standpoint and often require more prolonged, intensive mental health treatment when compared with patients with a single psychiatric disorder.10,11 Our study showed that high psychiatric comorbidity in survivors of ICU delirium is associated with a decreased QoL compared with those with no psychiatric comorbidity or with low-moderate psychiatric comorbidity. This finding is consistent with previous studies in the general population that patients with multiple psychiatric comorbidities are associated with a poorer QoL compared with patients with a single psychiatric comorbidity.10,11
There is a pressing need to better characterize psychiatric comorbidities in ICU survivors because our current evidence suggests that the prevalence of psychiatric comorbidities of ICU survivors is substantially higher than that of the general population. We found that nearly one-third of survivors of ICU delirium had comorbid depression, anxiety, and PTSD symptoms at 3 months. This is consistent with the few other studies of ICU survivors, which showed a prevalence of psychiatric comorbidity of 25% to 33%.5,12 These rates are substantially higher than the prevalence in the general population of 6%.13
The high rate of psychiatric comorbidities may render it difficult to effectively treat the mental health symptoms in ICU survivors.14 Treating multiple psychiatric comorbidities may also be especially challenging in survivors of ICU delirium because they have a high prevalence of cognitive impairment. Mental health treatments for patients with psychiatric disorders and comorbid cognitive impairment are limited. Better characterization of psychiatric comorbidity in ICU survivors, particularly those with ICU delirium, is vital to the development of more effective, bundled treatments for this population with multiple comorbidities.
Standardized screenings of ICU survivors at a high risk for psychiatric disorders, such as survivors of ICU delirium, may help to identify patients with comorbid psychiatric disorder symptoms and have them referred to appropriate treatment earlier with the hope of improving their QoL sooner. Although opportunities to deliver integrated outpatient collaborative mental health and medical care for a subspecialty population are limited, one potential model of care would be to utilize a collaborative-care model in an ICU survivor clinic.15
Strengths of our study include the examination of psychiatric comorbidities in survivors of ICU delirium, who often have a poor QoL. A deeper understanding of psychiatric comorbidity and its relationship with QoL is needed to better understand how to deliver more effective treatments for these survivors. Limitations include the small sample size, a one-time measurement of psychiatric comorbidities at the 3-month follow-up based on screenings tools, and a lack of objective measures of physical functioning to determine the effects of psychiatric comorbidities on physical functioning. There may also have been differences in how patients with no psychiatric comorbidity responded to the EQ-5D-VAS as a result of premorbid differences (eg, they were healthier prior to their ICU stay and perceived their survivor status more negatively). This may explain why we did not see a statistically significant difference between no psychiatric comorbidity and high psychiatric comorbidity groups on the EQ-5D-VAS. Nevertheless, we did see a difference between the low-moderate psychiatric comorbidity group on EQ-5D-VAS and differences between the no comorbidity and low-moderate comorbidity groups versus the high comorbidity group on the EQ-5D-3L. Finally, data about psychiatric history and QoL prior to ICU hospitalization were limited. Therefore, truly determining incidence versus prevalence of post-ICU comorbidities and whether psychiatric symptoms and its effects on QoL were due to ICU hospitalization or to premorbid psychiatric symptoms is difficult.
Our study demonstrated that in survivors of ICU delirium, higher comorbidity of psychiatric symptoms was associated with poorer QoL. Future studies will need to confirm these findings. We will also need to identify potentially reversible risk factors for psychiatric comorbidity and poorer QoL and develop treatments to effectively target the mental health symptoms of survivors of ICU delirium.
Disclosure
Grant support: The PMD trial is funded through the National Institutes of Health grant R01AG054205-02. SW is supported by NIA 2P30AG010133. AP is supported by CMS 1 L1 CMS331444-02-00, Indiana CTSI, and NIA R01AG054205-02. SG is supported by NIA 2P30AG010133, NIA 5R01AG045350, and NIA R01AG054205-02. SK is supported by NHBLI 5T32HL091816-07. MB is supported by NIA R01 AG040220-05, AHRQ P30 HS024384-02, CMS 1 L1 CMS331444-02-00, NIA R01 AG030618-05A1 and NIA R01AG054205-02. BK is supported by NIA K23-AG043476 and NHLBI R01HL131730. The funding agency had no role in the development of the study design, collection, analysis, interpretation of data, manuscript development, or the decision to submit the manuscript for publication. Conflicts of interest include MB, SG, and AP being funded by NIA R01AG054205-02 for the PMD study.
1. Jutte JE, Erb CT, Jackson JC. Physical, cognitive, and psychological disability following critical illness: what is the risk? Semin Respir Crit Care Med. 2015;36(6):943-958. PubMed
2. Nikayin S, Rabiee A, Hashem MD, et al. Anxiety symptoms in survivors of critical illness: a systematic review and meta-analysis. Gen Hosp Psychiatry. 2016;43:23-29. PubMed
3. Rabiee A, Nikayin S, Hashem MD, et al. Depressive symptoms after critical illness: a systematic review and meta-analysis. Crit Care Med. 2016;44(9):1744-1753. PubMed
4. Parker AM, Sricharoenchai T, Raparla S, Schneck KW, Bienvenu OJ, Needham DM. Posttraumatic stress disorder in critical illness survivors: a metaanalysis. Crit Care Med. 2015;43(5):1121-1129. PubMed
5. Bienvenu OJ, Colantuoni E, Mendez-Tellez PA, et al. Cooccurrence of and remission from general anxiety, depression, and posttraumatic stress disorder symptoms after acute lung injury: a 2-year longitudinal study. Crit Care Med. 2015;43(3):642-653. PubMed
6. Huang M, Parker AM, Bienvenu OJ, et al. Psychiatric Symptoms in Acute Respiratory Distress Syndrome Survivors: A 1-Year National Multicenter Study. Crit Care Med. 2016;44(5):954-965. PubMed
7. Abrams TE, Vaughan-Sarrazin M, Rosenthal GE. Influence of psychiatric comorbidity on surgical mortality. Arch Surg. 2010;145(10):947-953. PubMed
8. Campbell NL, Khan BA, Farber M, et al. Improving delirium care in the intensive care unit: the design of a pragmatic study. Trials. 2011;12:139. PubMed
9. EuroQol Group. EuroQol--a new facility for the measurement of health-related quality of life. Health Policy. 1990;16(3):199-208. PubMed
10. Hirschfeld RM. The comorbidity of major depression and anxiety disorders: recognition and management in primary care. Prim Care Companion J Clin Psychiatry. 2001;3(6):244–254. PubMed
11. Campbell DG, Felker BL, Liu CF, et al. Prevalence of depression–PTSD comorbidity: implications for clinical practice guidelines and primary care-based interventions. J Gen Intern Med. 2007;22(6):711–718. PubMed
12. Wolters AE, Peelen LM, Welling MC, et al. Long-term mental health problems after delirium in the ICU. Crit Care Med. 2016;44(10):1808-1813. PubMed
13. Kessler RC, Chiu WT, Demler O, Merikangas KR, Walters EE. Prevalence, severity, and comorbidity of 12-month DSM-IV disorders in the National Comorbidity Survey Replication. Arch Gen Psychiatry. 2005;62(6):617-627. PubMed
14. Mehlhorn J, Freytag A, Schmidt K, et al. Rehabilitation interventions for postintensive care syndrome: a systematic review. Crit Care Med. 2014;42(5):1263-1271. PubMed
15. Khan BA, Lasiter S, Boustani MA. CE: critical care recovery center: an innovative collaborative care model for ICU survivors. Am J Nurs. 2015;115(3):24-31. PubMed
The prevalence of depression, anxiety, and posttraumatic stress disorder (PTSD) symptoms in intensive care unit (ICU) survivors ranges from 17% to 44%.1-4 Psychiatric comorbidity, the presence of 2 or more psychiatric disorders, is highly prevalent in survivors of acute respiratory distress syndrome and is associated with higher mortality in postsurgical ICU survivors.5-7 While long-term cognitive impairment in patients with ICU delirium has been associated with poor quality of life (QoL),1 the effects of psychiatric comorbidity on QoL among similar patients are not as well understood. In this study, we examined whether psychiatric comorbidity was associated with poorer QoL in survivors of ICU delirium.
METHODS
We examined subjects who participated in the Pharmacologic Management of Delirium (PMD) clinical trial. This trial examined the efficacy of a pharmacological intervention for patients who developed ICU delirium at a local tertiary-care academic hospital.8 Out of 62 patients who participated in the follow-up of the PMD study, 58 completed QoL interviews and validated psychiatric screens (Patient Health Questionnaire-9 [PHQ-9] for depression, the Generalized Anxiety Disorder-7 [GAD-7] questionnaire for anxiety, and the Post-Traumatic Stress Syndrome [PTSS-10] questionnaire for PTSD) at 3 months after hospital discharge. High psychiatric comorbidity was defined as having significant symptoms for all 3 conditions (depression: PHQ-9 score ≥ 10; anxiety: GAD-7 ≥ 10; and PTSD: PTSS-10 > 35). No psychiatric morbidity was defined as having no significant symptoms for all 3 conditions. Low to moderate (low-moderate) psychiatric morbidity was defined as having symptoms for 1 to 2 conditions.
Participants also completed 2 complementary QoL measures: the EuroQol 5 dimensions questionnaire 3-level (EQ-5D-3L) Index and the EuroQol 5 dimensions Visual Analog Scale (EQ-5D-VAS).9 The EQ-5D-3L Index asks participants to rate themselves as having (1) no problems, (2) some problems, or (3) extreme problems on the following 5 scales: mobility, self-care, usual activities, pain/discomfort, and anxiety/depression. The scores are then indexed against the US population to create a continuous index scale ranging from −0.11 to 1.00.
Fisher’s exact tests were used to compare dichotomous outcomes. Analysis of variance (ANOVA) was used to compare continuous outcomes across the 3 psychiatric groups. Analysis of covariance (ANCOVA) was used to determine whether psychiatric comorbidity in survivors of ICU delirium was associated with QoL measures. Models were adjusted for the following covariates: age, gender, Charlson Comorbidity Index, discharged to home, prior history of depression, and prior history of anxiety. To assess the relationship of psychiatric comorbidity with QoL, we chose the 2 continuous QoL measures as the outcome. Because we were interested in the effect of psychiatric burden on QoL, we used ANCOVA with QoL as the dependent variable and psychiatric burden as an independent variable. Pairwise comparisons were then performed when overall differences were significant (P < 0.05). We performed 2 separate sensitivity analyses. The first analysis looked solely at the subgroup of patients from the medical intensive care unit. We also recalculated the EQ-5D-3L index excluding the anxiety/depression item.
RESULTS
Nearly one-third of patients (18/58) had high psychiatric burden. The table looks at the demographic and clinical characteristics of patients with high psychiatric comorbidity versus those of low-moderate psychiatric comorbidity and those with no psychiatric morbidity. Patient groups did not differ significantly in terms of demographics. For clinical characteristics, patients with high psychiatric comorbidity were more likely than patients with low-moderate psychiatric comorbidity to have a prior history of depression (P < 0.05).
Patients with high psychiatric comorbidity were more likely to have a poorer QoL when compared with patients with low-moderate psychiatric comorbidity and to those with no morbidity as measured by a lower EQ-5D-3L Index (no, 0.69 ± 0.25; low-moderate, 0.70 ± 0.19; high, 0.48 ± 0.24; P = 0.006) and EQ-5D-VAS (no, 67.0 ± 20.7; low-moderate, 76.6 ± 20.0; high, 50.8 ± 22.4; P = 0.004). After adjusting for covariates, patients with high psychiatric comorbidity had a poorer QoL compared with those with no morbidity or low-moderate comorbidity on the EQ-5D-3L Index (P = 0.017 for overall differences), whereas patients who had high psychiatric comorbidity had a poorer QoL compared to those with low-moderate comorbidity on the EQ-5D-VAS (P = 0.039 for overall differences; Figure). Subgroup analysis of MICU patients yielded similar results. Patients with high psychiatric burden had significantly poorer QoL as measured by the EQ-5D-3L (unadjusted P = 0.044, adjusted P = 0.003) and the EQ-5D-VAS (unadjusted P = 0.007, adjusted P = 0.021). After excluding the anxiety/depression item from the EQ-5D-3L, we observed similar differences (no, 0.71 ± 0.24; low-moderate, 0.75 ± 0.15; high, 0.58 ± 0.22; unadjusted P = 0.062; adjusted P = 0.040).
DISCUSSION/CONCLUSION
Psychiatric comorbidities in ICU survivors are common and pose a significant clinical issue. Patients with multiple psychiatric comorbidities can be more complicated to identify from a diagnostic standpoint and often require more prolonged, intensive mental health treatment when compared with patients with a single psychiatric disorder.10,11 Our study showed that high psychiatric comorbidity in survivors of ICU delirium is associated with a decreased QoL compared with those with no psychiatric comorbidity or with low-moderate psychiatric comorbidity. This finding is consistent with previous studies in the general population that patients with multiple psychiatric comorbidities are associated with a poorer QoL compared with patients with a single psychiatric comorbidity.10,11
There is a pressing need to better characterize psychiatric comorbidities in ICU survivors because our current evidence suggests that the prevalence of psychiatric comorbidities of ICU survivors is substantially higher than that of the general population. We found that nearly one-third of survivors of ICU delirium had comorbid depression, anxiety, and PTSD symptoms at 3 months. This is consistent with the few other studies of ICU survivors, which showed a prevalence of psychiatric comorbidity of 25% to 33%.5,12 These rates are substantially higher than the prevalence in the general population of 6%.13
The high rate of psychiatric comorbidities may render it difficult to effectively treat the mental health symptoms in ICU survivors.14 Treating multiple psychiatric comorbidities may also be especially challenging in survivors of ICU delirium because they have a high prevalence of cognitive impairment. Mental health treatments for patients with psychiatric disorders and comorbid cognitive impairment are limited. Better characterization of psychiatric comorbidity in ICU survivors, particularly those with ICU delirium, is vital to the development of more effective, bundled treatments for this population with multiple comorbidities.
Standardized screenings of ICU survivors at a high risk for psychiatric disorders, such as survivors of ICU delirium, may help to identify patients with comorbid psychiatric disorder symptoms and have them referred to appropriate treatment earlier with the hope of improving their QoL sooner. Although opportunities to deliver integrated outpatient collaborative mental health and medical care for a subspecialty population are limited, one potential model of care would be to utilize a collaborative-care model in an ICU survivor clinic.15
Strengths of our study include the examination of psychiatric comorbidities in survivors of ICU delirium, who often have a poor QoL. A deeper understanding of psychiatric comorbidity and its relationship with QoL is needed to better understand how to deliver more effective treatments for these survivors. Limitations include the small sample size, a one-time measurement of psychiatric comorbidities at the 3-month follow-up based on screenings tools, and a lack of objective measures of physical functioning to determine the effects of psychiatric comorbidities on physical functioning. There may also have been differences in how patients with no psychiatric comorbidity responded to the EQ-5D-VAS as a result of premorbid differences (eg, they were healthier prior to their ICU stay and perceived their survivor status more negatively). This may explain why we did not see a statistically significant difference between no psychiatric comorbidity and high psychiatric comorbidity groups on the EQ-5D-VAS. Nevertheless, we did see a difference between the low-moderate psychiatric comorbidity group on EQ-5D-VAS and differences between the no comorbidity and low-moderate comorbidity groups versus the high comorbidity group on the EQ-5D-3L. Finally, data about psychiatric history and QoL prior to ICU hospitalization were limited. Therefore, truly determining incidence versus prevalence of post-ICU comorbidities and whether psychiatric symptoms and its effects on QoL were due to ICU hospitalization or to premorbid psychiatric symptoms is difficult.
Our study demonstrated that in survivors of ICU delirium, higher comorbidity of psychiatric symptoms was associated with poorer QoL. Future studies will need to confirm these findings. We will also need to identify potentially reversible risk factors for psychiatric comorbidity and poorer QoL and develop treatments to effectively target the mental health symptoms of survivors of ICU delirium.
Disclosure
Grant support: The PMD trial is funded through the National Institutes of Health grant R01AG054205-02. SW is supported by NIA 2P30AG010133. AP is supported by CMS 1 L1 CMS331444-02-00, Indiana CTSI, and NIA R01AG054205-02. SG is supported by NIA 2P30AG010133, NIA 5R01AG045350, and NIA R01AG054205-02. SK is supported by NHBLI 5T32HL091816-07. MB is supported by NIA R01 AG040220-05, AHRQ P30 HS024384-02, CMS 1 L1 CMS331444-02-00, NIA R01 AG030618-05A1 and NIA R01AG054205-02. BK is supported by NIA K23-AG043476 and NHLBI R01HL131730. The funding agency had no role in the development of the study design, collection, analysis, interpretation of data, manuscript development, or the decision to submit the manuscript for publication. Conflicts of interest include MB, SG, and AP being funded by NIA R01AG054205-02 for the PMD study.
The prevalence of depression, anxiety, and posttraumatic stress disorder (PTSD) symptoms in intensive care unit (ICU) survivors ranges from 17% to 44%.1-4 Psychiatric comorbidity, the presence of 2 or more psychiatric disorders, is highly prevalent in survivors of acute respiratory distress syndrome and is associated with higher mortality in postsurgical ICU survivors.5-7 While long-term cognitive impairment in patients with ICU delirium has been associated with poor quality of life (QoL),1 the effects of psychiatric comorbidity on QoL among similar patients are not as well understood. In this study, we examined whether psychiatric comorbidity was associated with poorer QoL in survivors of ICU delirium.
METHODS
We examined subjects who participated in the Pharmacologic Management of Delirium (PMD) clinical trial. This trial examined the efficacy of a pharmacological intervention for patients who developed ICU delirium at a local tertiary-care academic hospital.8 Out of 62 patients who participated in the follow-up of the PMD study, 58 completed QoL interviews and validated psychiatric screens (Patient Health Questionnaire-9 [PHQ-9] for depression, the Generalized Anxiety Disorder-7 [GAD-7] questionnaire for anxiety, and the Post-Traumatic Stress Syndrome [PTSS-10] questionnaire for PTSD) at 3 months after hospital discharge. High psychiatric comorbidity was defined as having significant symptoms for all 3 conditions (depression: PHQ-9 score ≥ 10; anxiety: GAD-7 ≥ 10; and PTSD: PTSS-10 > 35). No psychiatric morbidity was defined as having no significant symptoms for all 3 conditions. Low to moderate (low-moderate) psychiatric morbidity was defined as having symptoms for 1 to 2 conditions.
Participants also completed 2 complementary QoL measures: the EuroQol 5 dimensions questionnaire 3-level (EQ-5D-3L) Index and the EuroQol 5 dimensions Visual Analog Scale (EQ-5D-VAS).9 The EQ-5D-3L Index asks participants to rate themselves as having (1) no problems, (2) some problems, or (3) extreme problems on the following 5 scales: mobility, self-care, usual activities, pain/discomfort, and anxiety/depression. The scores are then indexed against the US population to create a continuous index scale ranging from −0.11 to 1.00.
Fisher’s exact tests were used to compare dichotomous outcomes. Analysis of variance (ANOVA) was used to compare continuous outcomes across the 3 psychiatric groups. Analysis of covariance (ANCOVA) was used to determine whether psychiatric comorbidity in survivors of ICU delirium was associated with QoL measures. Models were adjusted for the following covariates: age, gender, Charlson Comorbidity Index, discharged to home, prior history of depression, and prior history of anxiety. To assess the relationship of psychiatric comorbidity with QoL, we chose the 2 continuous QoL measures as the outcome. Because we were interested in the effect of psychiatric burden on QoL, we used ANCOVA with QoL as the dependent variable and psychiatric burden as an independent variable. Pairwise comparisons were then performed when overall differences were significant (P < 0.05). We performed 2 separate sensitivity analyses. The first analysis looked solely at the subgroup of patients from the medical intensive care unit. We also recalculated the EQ-5D-3L index excluding the anxiety/depression item.
RESULTS
Nearly one-third of patients (18/58) had high psychiatric burden. The table looks at the demographic and clinical characteristics of patients with high psychiatric comorbidity versus those of low-moderate psychiatric comorbidity and those with no psychiatric morbidity. Patient groups did not differ significantly in terms of demographics. For clinical characteristics, patients with high psychiatric comorbidity were more likely than patients with low-moderate psychiatric comorbidity to have a prior history of depression (P < 0.05).
Patients with high psychiatric comorbidity were more likely to have a poorer QoL when compared with patients with low-moderate psychiatric comorbidity and to those with no morbidity as measured by a lower EQ-5D-3L Index (no, 0.69 ± 0.25; low-moderate, 0.70 ± 0.19; high, 0.48 ± 0.24; P = 0.006) and EQ-5D-VAS (no, 67.0 ± 20.7; low-moderate, 76.6 ± 20.0; high, 50.8 ± 22.4; P = 0.004). After adjusting for covariates, patients with high psychiatric comorbidity had a poorer QoL compared with those with no morbidity or low-moderate comorbidity on the EQ-5D-3L Index (P = 0.017 for overall differences), whereas patients who had high psychiatric comorbidity had a poorer QoL compared to those with low-moderate comorbidity on the EQ-5D-VAS (P = 0.039 for overall differences; Figure). Subgroup analysis of MICU patients yielded similar results. Patients with high psychiatric burden had significantly poorer QoL as measured by the EQ-5D-3L (unadjusted P = 0.044, adjusted P = 0.003) and the EQ-5D-VAS (unadjusted P = 0.007, adjusted P = 0.021). After excluding the anxiety/depression item from the EQ-5D-3L, we observed similar differences (no, 0.71 ± 0.24; low-moderate, 0.75 ± 0.15; high, 0.58 ± 0.22; unadjusted P = 0.062; adjusted P = 0.040).
DISCUSSION/CONCLUSION
Psychiatric comorbidities in ICU survivors are common and pose a significant clinical issue. Patients with multiple psychiatric comorbidities can be more complicated to identify from a diagnostic standpoint and often require more prolonged, intensive mental health treatment when compared with patients with a single psychiatric disorder.10,11 Our study showed that high psychiatric comorbidity in survivors of ICU delirium is associated with a decreased QoL compared with those with no psychiatric comorbidity or with low-moderate psychiatric comorbidity. This finding is consistent with previous studies in the general population that patients with multiple psychiatric comorbidities are associated with a poorer QoL compared with patients with a single psychiatric comorbidity.10,11
There is a pressing need to better characterize psychiatric comorbidities in ICU survivors because our current evidence suggests that the prevalence of psychiatric comorbidities of ICU survivors is substantially higher than that of the general population. We found that nearly one-third of survivors of ICU delirium had comorbid depression, anxiety, and PTSD symptoms at 3 months. This is consistent with the few other studies of ICU survivors, which showed a prevalence of psychiatric comorbidity of 25% to 33%.5,12 These rates are substantially higher than the prevalence in the general population of 6%.13
The high rate of psychiatric comorbidities may render it difficult to effectively treat the mental health symptoms in ICU survivors.14 Treating multiple psychiatric comorbidities may also be especially challenging in survivors of ICU delirium because they have a high prevalence of cognitive impairment. Mental health treatments for patients with psychiatric disorders and comorbid cognitive impairment are limited. Better characterization of psychiatric comorbidity in ICU survivors, particularly those with ICU delirium, is vital to the development of more effective, bundled treatments for this population with multiple comorbidities.
Standardized screenings of ICU survivors at a high risk for psychiatric disorders, such as survivors of ICU delirium, may help to identify patients with comorbid psychiatric disorder symptoms and have them referred to appropriate treatment earlier with the hope of improving their QoL sooner. Although opportunities to deliver integrated outpatient collaborative mental health and medical care for a subspecialty population are limited, one potential model of care would be to utilize a collaborative-care model in an ICU survivor clinic.15
Strengths of our study include the examination of psychiatric comorbidities in survivors of ICU delirium, who often have a poor QoL. A deeper understanding of psychiatric comorbidity and its relationship with QoL is needed to better understand how to deliver more effective treatments for these survivors. Limitations include the small sample size, a one-time measurement of psychiatric comorbidities at the 3-month follow-up based on screenings tools, and a lack of objective measures of physical functioning to determine the effects of psychiatric comorbidities on physical functioning. There may also have been differences in how patients with no psychiatric comorbidity responded to the EQ-5D-VAS as a result of premorbid differences (eg, they were healthier prior to their ICU stay and perceived their survivor status more negatively). This may explain why we did not see a statistically significant difference between no psychiatric comorbidity and high psychiatric comorbidity groups on the EQ-5D-VAS. Nevertheless, we did see a difference between the low-moderate psychiatric comorbidity group on EQ-5D-VAS and differences between the no comorbidity and low-moderate comorbidity groups versus the high comorbidity group on the EQ-5D-3L. Finally, data about psychiatric history and QoL prior to ICU hospitalization were limited. Therefore, truly determining incidence versus prevalence of post-ICU comorbidities and whether psychiatric symptoms and its effects on QoL were due to ICU hospitalization or to premorbid psychiatric symptoms is difficult.
Our study demonstrated that in survivors of ICU delirium, higher comorbidity of psychiatric symptoms was associated with poorer QoL. Future studies will need to confirm these findings. We will also need to identify potentially reversible risk factors for psychiatric comorbidity and poorer QoL and develop treatments to effectively target the mental health symptoms of survivors of ICU delirium.
Disclosure
Grant support: The PMD trial is funded through the National Institutes of Health grant R01AG054205-02. SW is supported by NIA 2P30AG010133. AP is supported by CMS 1 L1 CMS331444-02-00, Indiana CTSI, and NIA R01AG054205-02. SG is supported by NIA 2P30AG010133, NIA 5R01AG045350, and NIA R01AG054205-02. SK is supported by NHBLI 5T32HL091816-07. MB is supported by NIA R01 AG040220-05, AHRQ P30 HS024384-02, CMS 1 L1 CMS331444-02-00, NIA R01 AG030618-05A1 and NIA R01AG054205-02. BK is supported by NIA K23-AG043476 and NHLBI R01HL131730. The funding agency had no role in the development of the study design, collection, analysis, interpretation of data, manuscript development, or the decision to submit the manuscript for publication. Conflicts of interest include MB, SG, and AP being funded by NIA R01AG054205-02 for the PMD study.
1. Jutte JE, Erb CT, Jackson JC. Physical, cognitive, and psychological disability following critical illness: what is the risk? Semin Respir Crit Care Med. 2015;36(6):943-958. PubMed
2. Nikayin S, Rabiee A, Hashem MD, et al. Anxiety symptoms in survivors of critical illness: a systematic review and meta-analysis. Gen Hosp Psychiatry. 2016;43:23-29. PubMed
3. Rabiee A, Nikayin S, Hashem MD, et al. Depressive symptoms after critical illness: a systematic review and meta-analysis. Crit Care Med. 2016;44(9):1744-1753. PubMed
4. Parker AM, Sricharoenchai T, Raparla S, Schneck KW, Bienvenu OJ, Needham DM. Posttraumatic stress disorder in critical illness survivors: a metaanalysis. Crit Care Med. 2015;43(5):1121-1129. PubMed
5. Bienvenu OJ, Colantuoni E, Mendez-Tellez PA, et al. Cooccurrence of and remission from general anxiety, depression, and posttraumatic stress disorder symptoms after acute lung injury: a 2-year longitudinal study. Crit Care Med. 2015;43(3):642-653. PubMed
6. Huang M, Parker AM, Bienvenu OJ, et al. Psychiatric Symptoms in Acute Respiratory Distress Syndrome Survivors: A 1-Year National Multicenter Study. Crit Care Med. 2016;44(5):954-965. PubMed
7. Abrams TE, Vaughan-Sarrazin M, Rosenthal GE. Influence of psychiatric comorbidity on surgical mortality. Arch Surg. 2010;145(10):947-953. PubMed
8. Campbell NL, Khan BA, Farber M, et al. Improving delirium care in the intensive care unit: the design of a pragmatic study. Trials. 2011;12:139. PubMed
9. EuroQol Group. EuroQol--a new facility for the measurement of health-related quality of life. Health Policy. 1990;16(3):199-208. PubMed
10. Hirschfeld RM. The comorbidity of major depression and anxiety disorders: recognition and management in primary care. Prim Care Companion J Clin Psychiatry. 2001;3(6):244–254. PubMed
11. Campbell DG, Felker BL, Liu CF, et al. Prevalence of depression–PTSD comorbidity: implications for clinical practice guidelines and primary care-based interventions. J Gen Intern Med. 2007;22(6):711–718. PubMed
12. Wolters AE, Peelen LM, Welling MC, et al. Long-term mental health problems after delirium in the ICU. Crit Care Med. 2016;44(10):1808-1813. PubMed
13. Kessler RC, Chiu WT, Demler O, Merikangas KR, Walters EE. Prevalence, severity, and comorbidity of 12-month DSM-IV disorders in the National Comorbidity Survey Replication. Arch Gen Psychiatry. 2005;62(6):617-627. PubMed
14. Mehlhorn J, Freytag A, Schmidt K, et al. Rehabilitation interventions for postintensive care syndrome: a systematic review. Crit Care Med. 2014;42(5):1263-1271. PubMed
15. Khan BA, Lasiter S, Boustani MA. CE: critical care recovery center: an innovative collaborative care model for ICU survivors. Am J Nurs. 2015;115(3):24-31. PubMed
1. Jutte JE, Erb CT, Jackson JC. Physical, cognitive, and psychological disability following critical illness: what is the risk? Semin Respir Crit Care Med. 2015;36(6):943-958. PubMed
2. Nikayin S, Rabiee A, Hashem MD, et al. Anxiety symptoms in survivors of critical illness: a systematic review and meta-analysis. Gen Hosp Psychiatry. 2016;43:23-29. PubMed
3. Rabiee A, Nikayin S, Hashem MD, et al. Depressive symptoms after critical illness: a systematic review and meta-analysis. Crit Care Med. 2016;44(9):1744-1753. PubMed
4. Parker AM, Sricharoenchai T, Raparla S, Schneck KW, Bienvenu OJ, Needham DM. Posttraumatic stress disorder in critical illness survivors: a metaanalysis. Crit Care Med. 2015;43(5):1121-1129. PubMed
5. Bienvenu OJ, Colantuoni E, Mendez-Tellez PA, et al. Cooccurrence of and remission from general anxiety, depression, and posttraumatic stress disorder symptoms after acute lung injury: a 2-year longitudinal study. Crit Care Med. 2015;43(3):642-653. PubMed
6. Huang M, Parker AM, Bienvenu OJ, et al. Psychiatric Symptoms in Acute Respiratory Distress Syndrome Survivors: A 1-Year National Multicenter Study. Crit Care Med. 2016;44(5):954-965. PubMed
7. Abrams TE, Vaughan-Sarrazin M, Rosenthal GE. Influence of psychiatric comorbidity on surgical mortality. Arch Surg. 2010;145(10):947-953. PubMed
8. Campbell NL, Khan BA, Farber M, et al. Improving delirium care in the intensive care unit: the design of a pragmatic study. Trials. 2011;12:139. PubMed
9. EuroQol Group. EuroQol--a new facility for the measurement of health-related quality of life. Health Policy. 1990;16(3):199-208. PubMed
10. Hirschfeld RM. The comorbidity of major depression and anxiety disorders: recognition and management in primary care. Prim Care Companion J Clin Psychiatry. 2001;3(6):244–254. PubMed
11. Campbell DG, Felker BL, Liu CF, et al. Prevalence of depression–PTSD comorbidity: implications for clinical practice guidelines and primary care-based interventions. J Gen Intern Med. 2007;22(6):711–718. PubMed
12. Wolters AE, Peelen LM, Welling MC, et al. Long-term mental health problems after delirium in the ICU. Crit Care Med. 2016;44(10):1808-1813. PubMed
13. Kessler RC, Chiu WT, Demler O, Merikangas KR, Walters EE. Prevalence, severity, and comorbidity of 12-month DSM-IV disorders in the National Comorbidity Survey Replication. Arch Gen Psychiatry. 2005;62(6):617-627. PubMed
14. Mehlhorn J, Freytag A, Schmidt K, et al. Rehabilitation interventions for postintensive care syndrome: a systematic review. Crit Care Med. 2014;42(5):1263-1271. PubMed
15. Khan BA, Lasiter S, Boustani MA. CE: critical care recovery center: an innovative collaborative care model for ICU survivors. Am J Nurs. 2015;115(3):24-31. PubMed
© 2017 Society of Hospital Medicine
Antidepressant Use and Depressive Symptoms in Intensive Care Unit Survivors
As the number of intensive care unit (ICU) survivors has steadily increased over the past few decades, there is growing awareness of the long-term physical, cognitive, and psychological impairments after ICU hospitalization, collectively known as post–intensive care syndrome (PICS).1 Systematic reviews based mostly on research studies suggest that the prevalence of depressive symptoms 2-12 months after ICU discharge is nearly 30%.2-5 Due to the scarcity of established models of care for ICU survivors, there is limited characterization of depressive symptoms and antidepressant regimens in this clinical population. The Critical Care Recovery Center (CCRC) at Eskenazi Hospital is one of the first ICU survivor clinics in the United States and targets a racially diverse, underserved population in the Indianapolis metropolitan area.6 In this study, we examined whether patients had depressive symptoms at their initial CCRC visit, and whether the risk factors for depressive symptoms differed if they were on an antidepressant at their initial CCRC visit.
METHODS
Referral criteria to the CCRC were 18 years or older, admitted to the Eskenazi ICU, were on mechanical ventilation or delirious for ≥48 hours (major risk factors for the development of PICS), and recommended for follow-up by a critical care physician. The exclusion criterion included was enrollment in hospice or palliative care services. Institutional review board approval was obtained to conduct retrospective analyses of de-identified clinical data. Medical history and medication lists were collected from patients, informal caregivers, and electronic medical records.
Two hundred thirty-three patients were seen in the CCRC from July 2011 to August 2016. Two hundred four patients rated symptoms of depression with either the Patient Health Questionnaire (PHQ-9; N = 99) or Geriatric Depression Scale (GDS-30; N = 105) at their initial visit to the CCRC prior to receiving any treatment at the CCRC. Twenty-nine patients who did not complete depression questionnaires were excluded from the analyses. Patients with PHQ-9 score ≥10 or GDS score ≥20 were categorized as having moderate to severe depressive symptoms.7,8
Electronic medical records were reviewed to determine whether patients were on an antidepressant at hospital admission, hospital discharge, and the initial CCRC visit prior to any treatment in the CCRC. Patients who were on a tricyclic antidepressant, selective serotonin reuptake inhibitor, selective serotonin-norepinephrine reuptake inhibitor, noradrenergic and specific serotonergic antidepressant (eg, mirtazapine), or norepinephrine and dopaminergic reuptake inhibitor (eg, bupropion) at any dose were designated as being on an antidepressant. Prescribers of antidepressants included primary care providers, clinical providers during their hospital stay, and various outpatient subspecialists other than those in the CCRC.
We then examined whether the risk factors for depressive symptoms differed if patients were on an antidepressant at their initial CCRC visit. We compared demographic and clinical characteristics between depressed and nondepressed patients not on an antidepressant. We repeated these analyses for those on an antidepressant. Dichotomous outcomes were compared using chi-square testing, and two-way Student t tests for continuous outcomes. Demographic and clinical variables with P < 0.1 were included as covariates in a logistic regression model for depressive symptoms separately for those not an antidepressant and those on an antidepressant. History of depression was not included as a covariate because it is highly collinear with post-ICU depression.
RESULTS
Two hundred four ICU survivors in this study reflected a racially diverse and underserved population (monthly income $745.3 ± $931.5). Although most had respiratory failure and/or delirium during their hospital stay, 94.1% (N = 160) mostly lived independently after discharge. Nearly one-third of patients (N = 69) were on at least 1 antidepressant at their initial CCRC visit. Of these 69 patients, 60.9% (N = 42) had an antidepressant prescription on hospital admission, and 60.9% (N = 42) had an antidepressant prescription on hospital discharge.
We first compared the demographic and clinical characteristics of patients with and without depressive symptoms at their initial CCRC visit. Patients with depressive symptoms were younger, less likely to have cardiac disease, more likely to have a history of depression, more likely to have been prescribed an antidepressant on hospital admission, more likely to be prescribed an antidepressant on hospital discharge, and more likely to be on an antidepressant at their initial CCRC visit (Table 1).
Patients with depressive symptoms on an antidepressant (n = 65) were younger and more likely to be African American (borderline significance; Supplementary Table 2). Multivariate logistic regression showed that both younger age (OR = 0.92 per year, P = 0.003) and African American race (OR = 4.3, P = 0.024) remained significantly associated with depressive symptoms (Table 2).
DISCUSSION
Our study demonstrated that about one-third of our ICU survivor clinical cohort had untreated or inadequately treated depressive symptoms at their CCRC initial visit. Many patients with depressive symptoms had a history of depression and/or antidepressant prescription on hospital admission. This suggests that pre-ICU depression is a major contributor to post-ICU depression. These findings are consistent with the results of a large retrospective analysis of Danish ICU survivors that found that patients were more likely to have premorbid psychiatric diagnoses, compared with the general population.9 Another ICU survivor research study that excluded patients who were on antidepressants prior to ICU hospitalization found that 49% of these patients were on an antidepressant after their ICU stay.10 Our much lower rate of patients on an antidepressant after their ICU stay may reflect the differences between patient populations, differences in healthcare systems, and differences in clinician prescribing practices.
Younger age was associated with a higher likelihood of depressive symptoms independent of antidepressant status. Findings about the relationship between age and post-ICU depression have varied. The Bringing to Light the Risk Factors and Incidence of Neuropsychological Dysfunction in ICU Survivors group found that older age was associated with more depressive symptoms at 12 months postdischarge.11 On the other hand, a systematic review of post-ICU depression did not find any relationship between age and post-ICU depression.2,3 These differences may be due in part to demographic variations in cohorts.
Our logistic regression models suggest that there may also be different risk factors in patients who had untreated vs inadequately treated depressive symptoms. Patients who were not on an antidepressant at their initial CCRC visit were more likely to have a lower level of education. This is consistent with the Medical Expenditure Panel Surveys study, which showed that adults with less than a high school education were less likely to receive depression treatment.12 In patients who were on antidepressants at their initial CCRC visit, African Americans were more likely to have depressive symptoms. Possible reasons may include differences in receiving guideline-concordant antidepressant medication treatment, access to mental health subspecialty services, higher prevalence of treatment refractory depression, and differences in responses to antidepressant treatments.13,14
Strengths of our study include detailed characterization for a fairly large ICU survivor clinic population and a racially diverse cohort. To the best of our knowledge, our study is also the first to examine whether there may be different risk factors for depressive symptoms based on antidepressant status. Limitations include the lack of information about nonpharmacologic antidepressant treatment and the inability to assess whether noncompliance, insufficient dose, or insufficient time on antidepressants contributed to inadequate antidepressant treatment. Antidepressants may have also been prescribed for other purposes such as smoking cessation, neuropathic pain, and migraine headaches. However, because 72.4% of patients on antidepressants had a history of depression, it is likely that most of them were on antidepressants to treat depression.
Other limitations include potential biases in our clinical cohort. Over the last 5 years, the CCRC has provided care to more than 200 ICU survivors. With 1100 mechanically ventilated admissions per year, only 1.8% of survivors are seen. The referral criteria for the CCRC is a major source of selection bias, which likely overrepresents PICS. Because patients are seen in the CCRC about 3 months after hospital discharge, there is also informant censoring due to death. Physically sicker survivors in nursing home facilities were less likely to be included. Finally, the small cohort size may have resulted in an underpowered study.
Future studies will need to confirm our findings about the high prevalence of post-ICU depression and different responses to antidepressant medications by certain groups. Pre-ICU depression, lack of antidepressant treatment, and inadequate antidepressant treatment are major causes of post-ICU depression. Currently, the CCRC offers pharmacotherapy, problem-solving therapy, or referral to mental health specialists to treat patients with depressive symptoms. ICU survivor clinics, such as the CCRC, may become important settings that allow for increased access to depression treatment for those at higher risk for post-ICU depression as well as the testing of new antidepressant regimens for those with inadequately treated depression.
Acknowledgments
The authors thank Dr. Adil Sheikh for assistance with data entry and Cynthia Reynolds for her clinical services. Grant support: The Critical Care Recovery Center (CCRC) is supported by Eskenazi Health Services. SW is supported by NIA 2P30AG010133. SG is supported by NIA 2P30AG010133 and NIA 5R01AG045350. SK is supported by NHBLI 5T32HL091816-07. MB is supported by NIA R01 AG040220-05, AHRQ P30 HS024384-02, CMS 1 L1 CMS331444-02-00 and NIA R01 AG030618-05A1. BK is supported by NIA K23-AG043476 and NHLBI R01HL131730.
Disclosure
There are no conflicts of interest. None of the above NIH grants supported the CCRC or this work.
1. Needham DM, Davidson J, Cohen H, et al. Improving long-term outcomes after discharge from intensive care unit: report from a stakeholders’ conference. Crit Care Med. 2012;40:502-509. PubMed
2. Davydow DS, Gifford JM, Desai SV, Bienvenu OJ, Needham DM. Depression in general intensive care unit survivors: a systematic review. Intensive Care Med. 2009;35:796-809. PubMed
3. Rabiee A, Nikayin S, Hashem MD, et al. Depressive symptoms after critical illness: a systematic review and meta-analysis. Crit Care Med. 2016;44(9):1744-1753. PubMed
4. Huang M, Parker AM, Bienvenu OJ, et al. Psychiatric symptoms in acute respiratory distress syndrome survivors: A 1-year national multicenter study. Crit Care Med 2016;44:954-965. PubMed
5. Bienvenu OJ, Colantuoni E, Mendez-Tellez PA, et al. Cooccurrence of and remission from general anxiety, depression, and posttraumatic stress disorder symptoms after acute lung injury: a 2-year longitudinal study. Crit Care Med. 2015;43:642-653. PubMed
6. Khan BA, Lasiter S, Boustani MA. CE: critical care recovery center: an innovative collaborative care model for ICU survivors. Am J Nurs. 2015;115:24-31. PubMed
7. Kroenke K, Spitzer RL, Williams JB. The PHQ-9: validity of a brief depression severity measure. J Gen Intern Med. 2001;16:606-613. PubMed
8. Yesavage JA, Brink TL, Rose TL, et al. Development and validation of a geriatric depression screening scale: a preliminary report. J Psychiatr Res. 1982-1983;17:37-49. PubMed
9. Wuns ch H, Christiansen CF, Johansen MB, et al. Psychiatric diagnoses and psychoactive medication use among nonsurgical critically ill patients receiving mechanical ventilation. JAMA. 2014;311:1133-1142. PubMed
10. Weinert C, Meller W. Epidemiology of depression and antidepressant therapy after acute respiratory failure. Psychosomatics. 2006;47(5):399-407. PubMed
11. Jackson JC, Pandharipande PP, Girard TD, et al. Depression, post-traumatic stress disorder, and functional disability in survivors of critical illness in the BRAIN-ICU study: a longitudinal cohort study. Lancet Respir Med. 2014;2:369-379. PubMed
12. Olfson M, Blanco C, Marcus SC. Treatment of adult depression in the United States. JAMA Intern Med. 2016;176:1482-1491. PubMed
13. González HM, Vega WA, Williams DR, Tarraf W, West BT, Neighbors HW. Depression care in the United States: too little for too few. Arch Gen Psychiatry. 2010;67:37-46. PubMed
14. Bailey RK, Patel M, Barker NC, Ali S, Jabeen S. Major depressive disorder in the African American population. J Natl Med Assoc. 2011;103:548-557. PubMed
As the number of intensive care unit (ICU) survivors has steadily increased over the past few decades, there is growing awareness of the long-term physical, cognitive, and psychological impairments after ICU hospitalization, collectively known as post–intensive care syndrome (PICS).1 Systematic reviews based mostly on research studies suggest that the prevalence of depressive symptoms 2-12 months after ICU discharge is nearly 30%.2-5 Due to the scarcity of established models of care for ICU survivors, there is limited characterization of depressive symptoms and antidepressant regimens in this clinical population. The Critical Care Recovery Center (CCRC) at Eskenazi Hospital is one of the first ICU survivor clinics in the United States and targets a racially diverse, underserved population in the Indianapolis metropolitan area.6 In this study, we examined whether patients had depressive symptoms at their initial CCRC visit, and whether the risk factors for depressive symptoms differed if they were on an antidepressant at their initial CCRC visit.
METHODS
Referral criteria to the CCRC were 18 years or older, admitted to the Eskenazi ICU, were on mechanical ventilation or delirious for ≥48 hours (major risk factors for the development of PICS), and recommended for follow-up by a critical care physician. The exclusion criterion included was enrollment in hospice or palliative care services. Institutional review board approval was obtained to conduct retrospective analyses of de-identified clinical data. Medical history and medication lists were collected from patients, informal caregivers, and electronic medical records.
Two hundred thirty-three patients were seen in the CCRC from July 2011 to August 2016. Two hundred four patients rated symptoms of depression with either the Patient Health Questionnaire (PHQ-9; N = 99) or Geriatric Depression Scale (GDS-30; N = 105) at their initial visit to the CCRC prior to receiving any treatment at the CCRC. Twenty-nine patients who did not complete depression questionnaires were excluded from the analyses. Patients with PHQ-9 score ≥10 or GDS score ≥20 were categorized as having moderate to severe depressive symptoms.7,8
Electronic medical records were reviewed to determine whether patients were on an antidepressant at hospital admission, hospital discharge, and the initial CCRC visit prior to any treatment in the CCRC. Patients who were on a tricyclic antidepressant, selective serotonin reuptake inhibitor, selective serotonin-norepinephrine reuptake inhibitor, noradrenergic and specific serotonergic antidepressant (eg, mirtazapine), or norepinephrine and dopaminergic reuptake inhibitor (eg, bupropion) at any dose were designated as being on an antidepressant. Prescribers of antidepressants included primary care providers, clinical providers during their hospital stay, and various outpatient subspecialists other than those in the CCRC.
We then examined whether the risk factors for depressive symptoms differed if patients were on an antidepressant at their initial CCRC visit. We compared demographic and clinical characteristics between depressed and nondepressed patients not on an antidepressant. We repeated these analyses for those on an antidepressant. Dichotomous outcomes were compared using chi-square testing, and two-way Student t tests for continuous outcomes. Demographic and clinical variables with P < 0.1 were included as covariates in a logistic regression model for depressive symptoms separately for those not an antidepressant and those on an antidepressant. History of depression was not included as a covariate because it is highly collinear with post-ICU depression.
RESULTS
Two hundred four ICU survivors in this study reflected a racially diverse and underserved population (monthly income $745.3 ± $931.5). Although most had respiratory failure and/or delirium during their hospital stay, 94.1% (N = 160) mostly lived independently after discharge. Nearly one-third of patients (N = 69) were on at least 1 antidepressant at their initial CCRC visit. Of these 69 patients, 60.9% (N = 42) had an antidepressant prescription on hospital admission, and 60.9% (N = 42) had an antidepressant prescription on hospital discharge.
We first compared the demographic and clinical characteristics of patients with and without depressive symptoms at their initial CCRC visit. Patients with depressive symptoms were younger, less likely to have cardiac disease, more likely to have a history of depression, more likely to have been prescribed an antidepressant on hospital admission, more likely to be prescribed an antidepressant on hospital discharge, and more likely to be on an antidepressant at their initial CCRC visit (Table 1).
Patients with depressive symptoms on an antidepressant (n = 65) were younger and more likely to be African American (borderline significance; Supplementary Table 2). Multivariate logistic regression showed that both younger age (OR = 0.92 per year, P = 0.003) and African American race (OR = 4.3, P = 0.024) remained significantly associated with depressive symptoms (Table 2).
DISCUSSION
Our study demonstrated that about one-third of our ICU survivor clinical cohort had untreated or inadequately treated depressive symptoms at their CCRC initial visit. Many patients with depressive symptoms had a history of depression and/or antidepressant prescription on hospital admission. This suggests that pre-ICU depression is a major contributor to post-ICU depression. These findings are consistent with the results of a large retrospective analysis of Danish ICU survivors that found that patients were more likely to have premorbid psychiatric diagnoses, compared with the general population.9 Another ICU survivor research study that excluded patients who were on antidepressants prior to ICU hospitalization found that 49% of these patients were on an antidepressant after their ICU stay.10 Our much lower rate of patients on an antidepressant after their ICU stay may reflect the differences between patient populations, differences in healthcare systems, and differences in clinician prescribing practices.
Younger age was associated with a higher likelihood of depressive symptoms independent of antidepressant status. Findings about the relationship between age and post-ICU depression have varied. The Bringing to Light the Risk Factors and Incidence of Neuropsychological Dysfunction in ICU Survivors group found that older age was associated with more depressive symptoms at 12 months postdischarge.11 On the other hand, a systematic review of post-ICU depression did not find any relationship between age and post-ICU depression.2,3 These differences may be due in part to demographic variations in cohorts.
Our logistic regression models suggest that there may also be different risk factors in patients who had untreated vs inadequately treated depressive symptoms. Patients who were not on an antidepressant at their initial CCRC visit were more likely to have a lower level of education. This is consistent with the Medical Expenditure Panel Surveys study, which showed that adults with less than a high school education were less likely to receive depression treatment.12 In patients who were on antidepressants at their initial CCRC visit, African Americans were more likely to have depressive symptoms. Possible reasons may include differences in receiving guideline-concordant antidepressant medication treatment, access to mental health subspecialty services, higher prevalence of treatment refractory depression, and differences in responses to antidepressant treatments.13,14
Strengths of our study include detailed characterization for a fairly large ICU survivor clinic population and a racially diverse cohort. To the best of our knowledge, our study is also the first to examine whether there may be different risk factors for depressive symptoms based on antidepressant status. Limitations include the lack of information about nonpharmacologic antidepressant treatment and the inability to assess whether noncompliance, insufficient dose, or insufficient time on antidepressants contributed to inadequate antidepressant treatment. Antidepressants may have also been prescribed for other purposes such as smoking cessation, neuropathic pain, and migraine headaches. However, because 72.4% of patients on antidepressants had a history of depression, it is likely that most of them were on antidepressants to treat depression.
Other limitations include potential biases in our clinical cohort. Over the last 5 years, the CCRC has provided care to more than 200 ICU survivors. With 1100 mechanically ventilated admissions per year, only 1.8% of survivors are seen. The referral criteria for the CCRC is a major source of selection bias, which likely overrepresents PICS. Because patients are seen in the CCRC about 3 months after hospital discharge, there is also informant censoring due to death. Physically sicker survivors in nursing home facilities were less likely to be included. Finally, the small cohort size may have resulted in an underpowered study.
Future studies will need to confirm our findings about the high prevalence of post-ICU depression and different responses to antidepressant medications by certain groups. Pre-ICU depression, lack of antidepressant treatment, and inadequate antidepressant treatment are major causes of post-ICU depression. Currently, the CCRC offers pharmacotherapy, problem-solving therapy, or referral to mental health specialists to treat patients with depressive symptoms. ICU survivor clinics, such as the CCRC, may become important settings that allow for increased access to depression treatment for those at higher risk for post-ICU depression as well as the testing of new antidepressant regimens for those with inadequately treated depression.
Acknowledgments
The authors thank Dr. Adil Sheikh for assistance with data entry and Cynthia Reynolds for her clinical services. Grant support: The Critical Care Recovery Center (CCRC) is supported by Eskenazi Health Services. SW is supported by NIA 2P30AG010133. SG is supported by NIA 2P30AG010133 and NIA 5R01AG045350. SK is supported by NHBLI 5T32HL091816-07. MB is supported by NIA R01 AG040220-05, AHRQ P30 HS024384-02, CMS 1 L1 CMS331444-02-00 and NIA R01 AG030618-05A1. BK is supported by NIA K23-AG043476 and NHLBI R01HL131730.
Disclosure
There are no conflicts of interest. None of the above NIH grants supported the CCRC or this work.
As the number of intensive care unit (ICU) survivors has steadily increased over the past few decades, there is growing awareness of the long-term physical, cognitive, and psychological impairments after ICU hospitalization, collectively known as post–intensive care syndrome (PICS).1 Systematic reviews based mostly on research studies suggest that the prevalence of depressive symptoms 2-12 months after ICU discharge is nearly 30%.2-5 Due to the scarcity of established models of care for ICU survivors, there is limited characterization of depressive symptoms and antidepressant regimens in this clinical population. The Critical Care Recovery Center (CCRC) at Eskenazi Hospital is one of the first ICU survivor clinics in the United States and targets a racially diverse, underserved population in the Indianapolis metropolitan area.6 In this study, we examined whether patients had depressive symptoms at their initial CCRC visit, and whether the risk factors for depressive symptoms differed if they were on an antidepressant at their initial CCRC visit.
METHODS
Referral criteria to the CCRC were 18 years or older, admitted to the Eskenazi ICU, were on mechanical ventilation or delirious for ≥48 hours (major risk factors for the development of PICS), and recommended for follow-up by a critical care physician. The exclusion criterion included was enrollment in hospice or palliative care services. Institutional review board approval was obtained to conduct retrospective analyses of de-identified clinical data. Medical history and medication lists were collected from patients, informal caregivers, and electronic medical records.
Two hundred thirty-three patients were seen in the CCRC from July 2011 to August 2016. Two hundred four patients rated symptoms of depression with either the Patient Health Questionnaire (PHQ-9; N = 99) or Geriatric Depression Scale (GDS-30; N = 105) at their initial visit to the CCRC prior to receiving any treatment at the CCRC. Twenty-nine patients who did not complete depression questionnaires were excluded from the analyses. Patients with PHQ-9 score ≥10 or GDS score ≥20 were categorized as having moderate to severe depressive symptoms.7,8
Electronic medical records were reviewed to determine whether patients were on an antidepressant at hospital admission, hospital discharge, and the initial CCRC visit prior to any treatment in the CCRC. Patients who were on a tricyclic antidepressant, selective serotonin reuptake inhibitor, selective serotonin-norepinephrine reuptake inhibitor, noradrenergic and specific serotonergic antidepressant (eg, mirtazapine), or norepinephrine and dopaminergic reuptake inhibitor (eg, bupropion) at any dose were designated as being on an antidepressant. Prescribers of antidepressants included primary care providers, clinical providers during their hospital stay, and various outpatient subspecialists other than those in the CCRC.
We then examined whether the risk factors for depressive symptoms differed if patients were on an antidepressant at their initial CCRC visit. We compared demographic and clinical characteristics between depressed and nondepressed patients not on an antidepressant. We repeated these analyses for those on an antidepressant. Dichotomous outcomes were compared using chi-square testing, and two-way Student t tests for continuous outcomes. Demographic and clinical variables with P < 0.1 were included as covariates in a logistic regression model for depressive symptoms separately for those not an antidepressant and those on an antidepressant. History of depression was not included as a covariate because it is highly collinear with post-ICU depression.
RESULTS
Two hundred four ICU survivors in this study reflected a racially diverse and underserved population (monthly income $745.3 ± $931.5). Although most had respiratory failure and/or delirium during their hospital stay, 94.1% (N = 160) mostly lived independently after discharge. Nearly one-third of patients (N = 69) were on at least 1 antidepressant at their initial CCRC visit. Of these 69 patients, 60.9% (N = 42) had an antidepressant prescription on hospital admission, and 60.9% (N = 42) had an antidepressant prescription on hospital discharge.
We first compared the demographic and clinical characteristics of patients with and without depressive symptoms at their initial CCRC visit. Patients with depressive symptoms were younger, less likely to have cardiac disease, more likely to have a history of depression, more likely to have been prescribed an antidepressant on hospital admission, more likely to be prescribed an antidepressant on hospital discharge, and more likely to be on an antidepressant at their initial CCRC visit (Table 1).
Patients with depressive symptoms on an antidepressant (n = 65) were younger and more likely to be African American (borderline significance; Supplementary Table 2). Multivariate logistic regression showed that both younger age (OR = 0.92 per year, P = 0.003) and African American race (OR = 4.3, P = 0.024) remained significantly associated with depressive symptoms (Table 2).
DISCUSSION
Our study demonstrated that about one-third of our ICU survivor clinical cohort had untreated or inadequately treated depressive symptoms at their CCRC initial visit. Many patients with depressive symptoms had a history of depression and/or antidepressant prescription on hospital admission. This suggests that pre-ICU depression is a major contributor to post-ICU depression. These findings are consistent with the results of a large retrospective analysis of Danish ICU survivors that found that patients were more likely to have premorbid psychiatric diagnoses, compared with the general population.9 Another ICU survivor research study that excluded patients who were on antidepressants prior to ICU hospitalization found that 49% of these patients were on an antidepressant after their ICU stay.10 Our much lower rate of patients on an antidepressant after their ICU stay may reflect the differences between patient populations, differences in healthcare systems, and differences in clinician prescribing practices.
Younger age was associated with a higher likelihood of depressive symptoms independent of antidepressant status. Findings about the relationship between age and post-ICU depression have varied. The Bringing to Light the Risk Factors and Incidence of Neuropsychological Dysfunction in ICU Survivors group found that older age was associated with more depressive symptoms at 12 months postdischarge.11 On the other hand, a systematic review of post-ICU depression did not find any relationship between age and post-ICU depression.2,3 These differences may be due in part to demographic variations in cohorts.
Our logistic regression models suggest that there may also be different risk factors in patients who had untreated vs inadequately treated depressive symptoms. Patients who were not on an antidepressant at their initial CCRC visit were more likely to have a lower level of education. This is consistent with the Medical Expenditure Panel Surveys study, which showed that adults with less than a high school education were less likely to receive depression treatment.12 In patients who were on antidepressants at their initial CCRC visit, African Americans were more likely to have depressive symptoms. Possible reasons may include differences in receiving guideline-concordant antidepressant medication treatment, access to mental health subspecialty services, higher prevalence of treatment refractory depression, and differences in responses to antidepressant treatments.13,14
Strengths of our study include detailed characterization for a fairly large ICU survivor clinic population and a racially diverse cohort. To the best of our knowledge, our study is also the first to examine whether there may be different risk factors for depressive symptoms based on antidepressant status. Limitations include the lack of information about nonpharmacologic antidepressant treatment and the inability to assess whether noncompliance, insufficient dose, or insufficient time on antidepressants contributed to inadequate antidepressant treatment. Antidepressants may have also been prescribed for other purposes such as smoking cessation, neuropathic pain, and migraine headaches. However, because 72.4% of patients on antidepressants had a history of depression, it is likely that most of them were on antidepressants to treat depression.
Other limitations include potential biases in our clinical cohort. Over the last 5 years, the CCRC has provided care to more than 200 ICU survivors. With 1100 mechanically ventilated admissions per year, only 1.8% of survivors are seen. The referral criteria for the CCRC is a major source of selection bias, which likely overrepresents PICS. Because patients are seen in the CCRC about 3 months after hospital discharge, there is also informant censoring due to death. Physically sicker survivors in nursing home facilities were less likely to be included. Finally, the small cohort size may have resulted in an underpowered study.
Future studies will need to confirm our findings about the high prevalence of post-ICU depression and different responses to antidepressant medications by certain groups. Pre-ICU depression, lack of antidepressant treatment, and inadequate antidepressant treatment are major causes of post-ICU depression. Currently, the CCRC offers pharmacotherapy, problem-solving therapy, or referral to mental health specialists to treat patients with depressive symptoms. ICU survivor clinics, such as the CCRC, may become important settings that allow for increased access to depression treatment for those at higher risk for post-ICU depression as well as the testing of new antidepressant regimens for those with inadequately treated depression.
Acknowledgments
The authors thank Dr. Adil Sheikh for assistance with data entry and Cynthia Reynolds for her clinical services. Grant support: The Critical Care Recovery Center (CCRC) is supported by Eskenazi Health Services. SW is supported by NIA 2P30AG010133. SG is supported by NIA 2P30AG010133 and NIA 5R01AG045350. SK is supported by NHBLI 5T32HL091816-07. MB is supported by NIA R01 AG040220-05, AHRQ P30 HS024384-02, CMS 1 L1 CMS331444-02-00 and NIA R01 AG030618-05A1. BK is supported by NIA K23-AG043476 and NHLBI R01HL131730.
Disclosure
There are no conflicts of interest. None of the above NIH grants supported the CCRC or this work.
1. Needham DM, Davidson J, Cohen H, et al. Improving long-term outcomes after discharge from intensive care unit: report from a stakeholders’ conference. Crit Care Med. 2012;40:502-509. PubMed
2. Davydow DS, Gifford JM, Desai SV, Bienvenu OJ, Needham DM. Depression in general intensive care unit survivors: a systematic review. Intensive Care Med. 2009;35:796-809. PubMed
3. Rabiee A, Nikayin S, Hashem MD, et al. Depressive symptoms after critical illness: a systematic review and meta-analysis. Crit Care Med. 2016;44(9):1744-1753. PubMed
4. Huang M, Parker AM, Bienvenu OJ, et al. Psychiatric symptoms in acute respiratory distress syndrome survivors: A 1-year national multicenter study. Crit Care Med 2016;44:954-965. PubMed
5. Bienvenu OJ, Colantuoni E, Mendez-Tellez PA, et al. Cooccurrence of and remission from general anxiety, depression, and posttraumatic stress disorder symptoms after acute lung injury: a 2-year longitudinal study. Crit Care Med. 2015;43:642-653. PubMed
6. Khan BA, Lasiter S, Boustani MA. CE: critical care recovery center: an innovative collaborative care model for ICU survivors. Am J Nurs. 2015;115:24-31. PubMed
7. Kroenke K, Spitzer RL, Williams JB. The PHQ-9: validity of a brief depression severity measure. J Gen Intern Med. 2001;16:606-613. PubMed
8. Yesavage JA, Brink TL, Rose TL, et al. Development and validation of a geriatric depression screening scale: a preliminary report. J Psychiatr Res. 1982-1983;17:37-49. PubMed
9. Wuns ch H, Christiansen CF, Johansen MB, et al. Psychiatric diagnoses and psychoactive medication use among nonsurgical critically ill patients receiving mechanical ventilation. JAMA. 2014;311:1133-1142. PubMed
10. Weinert C, Meller W. Epidemiology of depression and antidepressant therapy after acute respiratory failure. Psychosomatics. 2006;47(5):399-407. PubMed
11. Jackson JC, Pandharipande PP, Girard TD, et al. Depression, post-traumatic stress disorder, and functional disability in survivors of critical illness in the BRAIN-ICU study: a longitudinal cohort study. Lancet Respir Med. 2014;2:369-379. PubMed
12. Olfson M, Blanco C, Marcus SC. Treatment of adult depression in the United States. JAMA Intern Med. 2016;176:1482-1491. PubMed
13. González HM, Vega WA, Williams DR, Tarraf W, West BT, Neighbors HW. Depression care in the United States: too little for too few. Arch Gen Psychiatry. 2010;67:37-46. PubMed
14. Bailey RK, Patel M, Barker NC, Ali S, Jabeen S. Major depressive disorder in the African American population. J Natl Med Assoc. 2011;103:548-557. PubMed
1. Needham DM, Davidson J, Cohen H, et al. Improving long-term outcomes after discharge from intensive care unit: report from a stakeholders’ conference. Crit Care Med. 2012;40:502-509. PubMed
2. Davydow DS, Gifford JM, Desai SV, Bienvenu OJ, Needham DM. Depression in general intensive care unit survivors: a systematic review. Intensive Care Med. 2009;35:796-809. PubMed
3. Rabiee A, Nikayin S, Hashem MD, et al. Depressive symptoms after critical illness: a systematic review and meta-analysis. Crit Care Med. 2016;44(9):1744-1753. PubMed
4. Huang M, Parker AM, Bienvenu OJ, et al. Psychiatric symptoms in acute respiratory distress syndrome survivors: A 1-year national multicenter study. Crit Care Med 2016;44:954-965. PubMed
5. Bienvenu OJ, Colantuoni E, Mendez-Tellez PA, et al. Cooccurrence of and remission from general anxiety, depression, and posttraumatic stress disorder symptoms after acute lung injury: a 2-year longitudinal study. Crit Care Med. 2015;43:642-653. PubMed
6. Khan BA, Lasiter S, Boustani MA. CE: critical care recovery center: an innovative collaborative care model for ICU survivors. Am J Nurs. 2015;115:24-31. PubMed
7. Kroenke K, Spitzer RL, Williams JB. The PHQ-9: validity of a brief depression severity measure. J Gen Intern Med. 2001;16:606-613. PubMed
8. Yesavage JA, Brink TL, Rose TL, et al. Development and validation of a geriatric depression screening scale: a preliminary report. J Psychiatr Res. 1982-1983;17:37-49. PubMed
9. Wuns ch H, Christiansen CF, Johansen MB, et al. Psychiatric diagnoses and psychoactive medication use among nonsurgical critically ill patients receiving mechanical ventilation. JAMA. 2014;311:1133-1142. PubMed
10. Weinert C, Meller W. Epidemiology of depression and antidepressant therapy after acute respiratory failure. Psychosomatics. 2006;47(5):399-407. PubMed
11. Jackson JC, Pandharipande PP, Girard TD, et al. Depression, post-traumatic stress disorder, and functional disability in survivors of critical illness in the BRAIN-ICU study: a longitudinal cohort study. Lancet Respir Med. 2014;2:369-379. PubMed
12. Olfson M, Blanco C, Marcus SC. Treatment of adult depression in the United States. JAMA Intern Med. 2016;176:1482-1491. PubMed
13. González HM, Vega WA, Williams DR, Tarraf W, West BT, Neighbors HW. Depression care in the United States: too little for too few. Arch Gen Psychiatry. 2010;67:37-46. PubMed
14. Bailey RK, Patel M, Barker NC, Ali S, Jabeen S. Major depressive disorder in the African American population. J Natl Med Assoc. 2011;103:548-557. PubMed
© 2017 Society of Hospital Medicine
Impact of Inpatient GCS on CI Patients
Under the Patient Protection and Affordable Care Act of 2010, commonly referred to as the Affordable Care Act, hospitals face up to a 3% penalty in Medicare reimbursements for patients readmitted within 30 days of initial discharge, and measures have been proposed for modifying payments to hospitals based on their performance on this metric.[1] Cognitive impairment (CI) is considered a major risk factor for poor postdischarge outcomes including mortality and hospital readmission.[2, 3] Hospitals are seeking strategies to reduce postdischarge mortality and rehospitalization among patients with and without CI.[4] Such strategies include use of transitional care coaches, patient and caregiver education, postdischarge follow‐up, and provision of geriatric consultative services (GCS) for the care of complex patients in the hospital setting.[5, 6, 7]
GCS utilize comprehensive geriatric assessments and multidisciplinary processes to recognize and modify risk factors that may lead to poor outcomes among hospitalized patients.[8, 9, 10, 11] Implementation of GCS models including Acute Care for Elders and, recently, the Mobile Acute Care of the Elderly services have shown many benefits among older patients including a reduction in the hospital length of stay and readmission rates.[12, 13] The benefits of such services among hospitalized elders suffering from CI, however, are not well established. The objective of this article was to evaluate the impact of GCS on the readmission and mortality rates of older adults with CI within 12 months of their hospitalization to an urban, public hospital. We hypothesized that GCS will reduce both 12‐month hospital readmissions and mortality rates among this vulnerable group of older adults.
METHODS
The study was approved by the Indiana University institutional review board, and informed consent for identifiable chart review was obtained from subjects or their legally authorized representatives.
Setting
The study was conducted at Eskenazi hospital, Indianapolis, Indiana, a 340‐bed, university‐affiliated, public hospital with over 2300 admissions of patients aged 65 years or older every year.
Population
Four hundred fifteen hospitalized patients aged 65 years or older suffering from CI were enrolled into an original, randomized, controlled trial that evaluated the effect of a computerized decision support system on their quality and outcome of care between July 1, 2006 and May 30, 2008.[14] The computerized decision support included reminders for physicians to reduce the prescription of 18 anticholinergics, minimize physical restraints and Foley catheterization, and increase referral to the local GCS.[15] That previous trial neither showed an impact on quality of care nor health utilization among older patients, including mortality and hospital readmission rates. The current study uses the data from the clinical trial cohort to evaluate the effect of GCS on the 12‐month mortality and hospital readmission rates for hospitalized elders with CI (Figure 1).
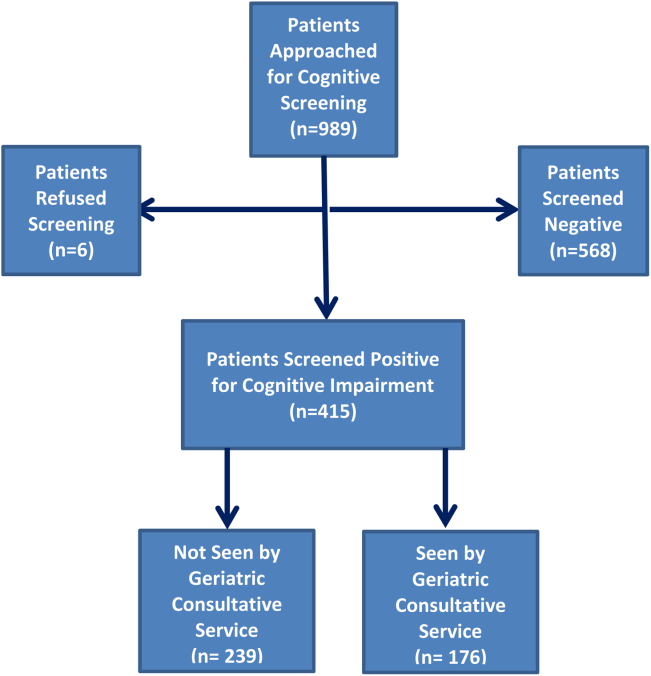
Inclusion and Exclusion Criteria
Individuals were eligible for enrollment if they were aged 65 years or older, hospitalized on a medical ward, able to speak English, and had evidence of CI within 48 hours of hospital admission. Individuals were excluded if they were previously enrolled, were aphasic, or unresponsive. The presence of CI was based on the Short Portable Mental Status Questionnaire (SPMSQ),[16] a brief 10‐item screening test with a sensitivity of 86% and specificity of 99% for dementia using a score of 7 or less (maximum possible score of 10).[16] The SPMSQ scoring process adjusts for participant educational and racial status, which was a benefit to its use given the urban setting of our hospital serving a large proportion of minority and low‐education patients. A physician‐trained research assistant administered the SPMSQ within 48 hours of hospital admission.
Geriatric Consultative Services
GCS is an interdisciplinary team of a geriatrician, a geriatric pharmacist, a case manager nurse, a social worker, a medical assistant, physical therapists, and a representative of the local Area Agency on Aging. There may be a geriatric fellow and/or medicine resident available to the team based on their rotation structure. Team‐based bedside rounds are performed on new consults only, but all patients are seen individually by the team clinicians. The team emphasizes prevention of functional decline and polypharmacy, recognition and treatment of geriatric syndromes including dementia and delirium, and early discharge/transition planning. Consensus recommendations are prepared and documented in the consult notes section of the electronic medical records. Recommendations deemed critical are discussed directly with the primary teams, but no orders are placed by the GCS team. The GCS team is available on all weekdays but not on weekends or major holidays.
Study Outcomes
For this secondary analysis, we used the Regenstrief Medical Record System (RMRS) to measure 2 outcomes: hospital readmission and mortality rates up to 1 year from discharge following index hospitalization, defined as the first admission in the original clinical trial. The RMRS is the primary instrument for processing data and monitoring patient and physician activity for the hospital.[17, 18] The RMRS is linked with a state‐wide health information exchange to capture data on hospitalization outside the hospital. The RMRS also contains death certificate information for all registered patients who die in or outside the Eskenazi hospital.
Other Data Collections
Delirium was assessed at screening and then every weekday using the Confusion Assessment Method (CAM) by a trained research assistant.[19] CAM evaluates 10 symptoms of delirium specified in the Diagnostic and Statistical Manual of Mental Disorders‐III‐Revision: acute onset, fluctuating course, inattention, disorganized thinking, altered level of consciousness, disorientation, memory impairment, perceptual disturbances, psychomotor agitation or retardation, and sleep/wake disturbance. Participant demographic characteristics, including age, sex, ethnicity, and years of education, were collected from the RMRS and from interviews performed at the time of cognitive screening. Information on length of hospital stay and discharge destination (eg, home vs facility, including skilled nursing and acute rehabilitation facilities) was also obtained from the RMRS. Charlson Comorbidity Index score was calculated using International Classification of Diseases, Ninth Revision codes gathered from 1 year before admission until the time of each participant's discharge from the hospital.[20] The Acute Physiology Score (APS) from the Acute Physiology and Chronic Health Evaluation (APACHE) III was derived from data available in the RMRS to measure the severity of illness.[21] Although the APACHE III was developed in the intensive care unit using data from the first 24 hours after admission, for our study we used the worst laboratory test value during the entire hospital stay to calculate the APS.[22]
Statistical Analysis
Baseline variables are presented as means and standard deviations for continuous variables, and percentages for binary categorical variables. Comparisons between patients receiving GCS and those who did not were performed using 2 tests for categorical variables and Kruskal‐Wallis test for continuous variables. Cox proportional hazard models were used to determine the association between receiving GCS and time to hospital readmission or mortality within 30 days or 1‐year postindex admission while adjusting for other covariates. For the models using time to readmission, patients without readmission were censored either at the endpoint (30 days or 1 year) or at time of death for those who died within the time frame in each model. Because GCS was not randomly assigned, we also conducted a propensity score analysis.[23] A logistic model for the probability of receiving GCS was conducted using patient demographic variables and information collected before and at the time of GCS. Stratified Cox proportional models using quintiles of predicted probability of receiving GCS were used in a propensity‐adjusted Cox model. All data analyses were performed using SAS version 9.3 (SAS Institute, Inc., Cary, NC).
RESULTS
Between July 1, 2006 and May 30, 2008, 415 CI patients were enrolled in the original trial, with 176 receiving the GCS. As shown in Table 1, the GCS and non‐GCS groups differed significantly. The GCS group was older (79.2 years old, 8.1 standard deviation [SD] vs 75.8 years old, 7.8 SD; P0.001), scored lower on the SPMSQ (4.7, 2.7 SD vs 5.5, 2.7 SD; P=0.002), had fewer chronic conditions with a lower mean Charlson Comorbidity Index Score (2.1, 1.86 SD vs 2.8, 2.6 SD; P=0.023), but a higher percentage of delirium (48.9% vs 29.3%), a lower percentage of being discharged home (37.5% vs 56.1%), and a higher mean length of stay (6.4 days, 6.4 SD vs 5.6 days, 5.9 SD; P=0.004). They also had a lower malignancy rate (6.2% vs 14.6%; P=0.007) and a lower number of hospitalizations in the previous year (0.5 admissions, 0.9 SD vs 0.7 admissions, 1.1 SD; P=0.035). No differences were observed in regard to gender, ethnicity, history of myocardial infarctions, chronic obstructive pulmonary disease, cerebrovascular disease, peripheral vascular disease, diabetes, and use of anticholinergic medicines.
No GCS, n=239 | GCS, n=176 | P Value* | |
---|---|---|---|
| |||
Baseline characteristics | |||
Mean age (SD) | 75.8 (7.8) | 79.2 (8.1) | <0.001 |
% Female | 66.1 [n=158] | 68.2 [n=120] | 0.657 |
% African American | 54.8 [n=131] | 63.6 [n=112] | 0.071 |
Mean SPMSQ score (SD) | 5.5 (2.7) | 4.7 (2.7) | 0.002 |
Admission diagnoses | |||
MI | 15.5 [n=37] | 13.6 [n=24] | 0.675 |
CHF | 38.1 [n=91] | 34.7 [n=61] | 0.475 |
PVD | 7.1 [n=17] | 9.7 [n=17] | 0.370 |
Cerebrovascular | 13.8 [n=33] | 19.3 [n=34] | 0.140 |
COPD | 41.0 [n=98] | 33.0 [n=58] | 0.094 |
Diabetes | 47.7 [n=114] | 40.9 [n=72] | 0.169 |
Malignancy | 14.6 [n=35] | 6.2 [n=11] | 0.007 |
Metastatic cancer | 8.8 [n=21] | 1.7 [n=3] | 0.002 |
Mean Charlson Comorbidity (SD) | 2.8 (2.6) | 2.1 (1.8) | 0.023 |
Mean APS (SD) | 24.5 (13.8) | 25.9 (13.5) | 0.231 |
Definite ACB Use | 35.2 [n=84] | 27.8 [n=49] | 0.136 |
Length of stay | 5.6 (5.9) | 6.4 (6.4) | 0.004 |
% Any delirium | 29.3 [n=70] | 48.9 [n=156] | <0.001 |
% Discharged home | 56.1 [n=134] | 37.5 [n=66] | <0.001 |
No. of inpatient stays prior year | 0.7 (1.1) | 0.5 (0.9) | 0.035 |
Follow‐up outcomes | |||
% Readmission within 30 days | 15.1 [n=36] | 22.7 [n=40] | 0.054 |
% Readmission within 1 year | 54.4 [n=130] | 56.3 [n=99] | 0.765 |
% Death within 30 days | 4.2 [n=10] | 1.7 [n=3] | 0.253 |
% Death within 1 year | 26.8 [n=64] | 23.9 [n=42] | 0.569 |
% Readmission or death within 30 days | 18.0 [n=43] | 24.4 [n=43] | 0.113 |
% Readmission or death within 1 year | 64.8 [n=155] | 63.1 [n=111] | 0.708 |
Table 2 describes the association of various factors with receiving GCS. Patients who were positive for delirium (odds ratio [OR]=1.65; 95% confidence interval=0.98‐2.77) and were older (OR=1.04; 95% confidence interval=1.01‐1.08) had a higher propensity to receive GCS, whereas, the presence of metastatic cancer resulted in a lower propensity (OR=0.15; 95% confidence interval=0.02‐1.16) of receiving GCS. The logistic model estimated area under the receiver operating characteristic curve was 0.707.
Adjusted OR (95% CI) | P Value | |
---|---|---|
| ||
Age | 1.04 (1.011.08) | 0.006 |
Female | 1.02 (0.641.63) | 0.942 |
African American | 1.11 (0.711.72) | 0.657 |
Short Portable Mental Status Questionnaire score | 1.00 (0.911.10) | 0.990 |
Acute Physiology Score | 1.00 (0.981.02) | 0.769 |
Charlson Comorbidity Score | 1.11 (0.841.46) | 0.471 |
Length of hospital stay | 1.02 (0.981.07) | 0.299 |
Definite anticholinergic use* | 0.74 (0.461.20) | 0.219 |
Any delirium during hospital stay | 1.65 (0.982.77) | 0.061 |
Diabetes mellitus | 0.72 (0.411.26) | 0.253 |
Myocardial infarction | 0.83 (0.411.66) | 0.593 |
Congestive heart failure | 0.83 (0.471.47) | 0.524 |
Peripheral vascular disease | 1.39 (0.613.18) | 0.433 |
Cerebrovascular disease | 1.30 (0.652.59) | 0.464 |
Malignancy | 0.45 (0.171.21) | 0.113 |
Metastatic cancer | 0.15 (0.021.16) | 0.069 |
Chronic obstructive pulmonary disease | 0.91 (0.531.55) | 0.727 |
Table 3 provides results from the Cox models for receiving GCS on readmission and mortality outcomes adjusting for various sets of covariates and with the propensity score adjustment. Model 1 presents unadjusted hazard ratio (HR). Model 2 presents HRs adjusting for a common set of covariates that were significantly associated with at least 1 of the outcomes, whereas model 3 presents the results adjusting for all covariates. All 4 models yielded similar results. As evident from this table, propensity‐adjusted HR for 30‐day readmission was still significantly higher among patients receiving GCS (HR=1.75; 95% confidence interval=1.06‐2.88) but not at 1 year (HR=1.19; 95% confidence interval=0.89‐1.59). There was a trend for decreased mortality for the GCS group at 30 days (HR=0.35; 95% confidence interval=0.09‐1.35), but it disappeared at 1 year (HR=0.91; 95% confidence interval=0.59‐1.40). A composite outcome of readmissions and mortality did not show any difference between the GCS and no‐GCS groups.
Outcome Variables | Model 1 | Model 2 | Model 3 | Propensity Adjusted | ||||
---|---|---|---|---|---|---|---|---|
HR (95% CI) | P Value | HR (95% CI) | P Value | HR (95% CI) | P Value | HR (95% CI) | P Value | |
| ||||||||
Readmission within 30 days | 1.65 (1.05, 2.59) | 0.030 | 1.73 (1.08, 2.78) | 0.024 | 1.84 (1.133.00) | 0.015 | 1.75 (1.062.88) | 0.029 |
Readmission within 1 year | 1.13 (0.87, 1.46) | 0.373 | 1.24 (0.94, 1.63) | 0.125 | 1.26 (0.941.68) | 0.117 | 1.19 (0.891.59) | 0.245 |
Death within 30 days | 0.43 (0.12, 1.56) | 0.199 | 0.34 (0.09, 1.28) | 0.110 | 0.25 (0.061.02) | 0.053 | 0.35 (0.091.35) | 0.126 |
Death within 1 year | 0.95 (0.65, 1.45) | 0.806 | 0.87 (0.58 1.31) | 0.506 | 0.93 (0.601.42) | 0.724 | 0.91 (0.591.40) | 0.669 |
Readmission or Death within 30 days | 1.48 (0.97, 2.26) | 0.070 | 1.49 (0.96, 2.33) | 0.078 | 1.56 (0.982.47) | 0.061 | 1.55 (0.972.48) | 0.069 |
Readmission or death within 1 year | 1.05 (0.82, 1.34) | 0.699 | 1.11 (0.86, 1.43) | 0.412 | 1.15 (0.881.50) | 0.318 | 1.08 (0.831.42) | 0.569 |
DISCUSSION
To our knowledge, this is the first study to analyze the impact of GCS on hospital readmission and mortality rates of CI patients. Our results did not show any short‐term or long‐term benefits of GCS for CI patients. Recent studies exploring cost benefits of the GCS have found trends toward lower readmission, but none focused on patients with CI.[6, 24, 25] It is important to note that our study did not use random allocation to assigning the patient into the GCS or control group, thus raising the possibility that patients who received GCS were sicker and were medically and socially more complex than those who did not receive the consult. Moreover, GCS consultation is preferentially sought for and completed for patients with CI and functional limitations, consistent with our finding that GCS patients more often have delirium and are less‐often discharged home.
The nature of the GCS team is another important consideration. Our GCS model did not include unit cohorting of patients, an important component of other proposed GCS models.[26] A recent meta‐analysis found that the GCS models without unit cohorting of patients did not have an impact on 1‐ or 12‐month readmission rates.[27] Low adherence to consultant recommendations (less than 33%) was thought to be a reason for such results. Importance of cohorting with regard to accomplishing recommendations by primary teams, importance of unit staff expertise in geriatric principles, and impact of a unit model on teamwork has also been highlighted by another review.[28] These findings lend to the hypothesis that unit cohorting and direct order placement by the GCS team may improve outcomes among CI patients, including a reduction in readmission rates.
Although readmissions rates were not statistically different between GCS and control groups at 1‐year postdischarge, 30‐day readmission rates were higher among the GCS group. Previous research among older heart failure patients found that a comprehensive transitional care intervention at the time of hospital discharge significantly shortened the time to readmission in the intervention group (P=0.026).[29] The factors identified by the study authors included enhanced supervision by the transitional healthcare teams along with improved awareness and education among treated patients that may have facilitated early recognition of clinical deterioration.[29] A recent study with intensive outpatient care that resulted in increased admissions among chronically ill adults provided a similar conclusion.[30]
GCS patients showed a trend toward decreased mortality as did patients enrolled in previous studies evaluating GCS models in the inpatient setting, as suggested by a recent review.[27] A caveat to note is that these trends favored ward‐styled GCS services as compared to our open GCS model,[27, 28] although the factors cited in these dedicated units affecting mortality included prompt attention to early rehabilitation, delirium management, and prevention of pressure ulcers and are also frequently implemented for patients in our GCS service model and therefore may have produced similar results.
Our neutral results in regard to the readmissions need to be interpreted with caution. First, this study was conducted in a hospital that supports expert geriatric and palliative care teams, both in the inpatient and the ambulatory settings, that provide consultative services and train medicine teams and hospital nursing staff. On the outpatient side, the presence of a robust geriatrics house‐calls program and the Geriatric Resources for Assessment and Care of Elders team results in above‐average care for the control group, and thus may also impact apparent outcomes.[31, 32] Second, 30‐day readmissions represent a complex outcome. Two recent reviews of hospital‐initiated interventions have shown that evidence regarding best strategies to decrease 30‐day readmissions is unclear.[33] Neither review included studies that targeted patients with CI only. The 2 programs that reduced 30‐day readmissions were multifaceted and included personnel who provide bridging between the hospital and the outpatient setting.[34] The GCS does include a focus on postdischarge resources, but does that on a case‐by‐case basis and no formal posthospital follow‐ups are provided. Moreover, the value of 30‐day readmission rates as a marker of quality, even though used by policymakers as an indicator of hospital quality, remains controversial.[35, 36] Broadening the outcomes of interest to include patient‐centered outcomes including satisfaction with care, that have shown to impact other health outcomes, may help improve understanding the benefits of GCS in hospitals.[37] Other comprehensive transitional care models that failed to show a benefit on 30‐day readmissions in older patients still resulted in higher satisfaction among patients.[38] Unfortunately, our evaluation did not include an assessment of patient satisfaction and quality of transitions.
Since the study period, GCS at our hospital now has incorporated a more robust focus on advance care planning (ACP) and execution of Physician Orders for Scope of Treatment that were legislated in the state in July 2013. The GCS team members are expert in carrying out complex ACP discussions and also partner with the inpatient palliative care team. It is quite possible that a study of more recent outcomes will yield more positive results for the selected outcomes. Thus, for future trials that aim to study the impact of GCS in the inpatient settings, it may be advisable to include important quality markers such as implementation of ACP and patient satisfaction along with the health utilization outcomes.
Limitations
As mentioned previously, it is possible that our risk adjustment was insufficient to account for all the medical and psychosocial differences among groups. For example, the overall anticholinergic impact of various medications such as antipsychotic medications and histamine‐2 blockers was assessed via the Anticholinergic Burden Scale on admission, but we did not have information on medication prescribing during the stay. We were further limited by lack of baseline functional status and socioeconomic details, both of which are related to 30‐day readmissions. For example, living alone, prior use of assist devices, and belonging to lower socioeconomic status are correlated with higher readmission rates.[39, 40] Patients with available social support may receive more intense supervision and may seek medical attention sooner. On the other hand, worsening health among CI patients without any approximate social support may be unnoticed for days. Absence of details of inpatient interventions may also have resulted in unmeasurable confounders that could have impacted our study outcomes. Finally, lack of information on the uptake of GCS recommendations by the primary teams is another limitation of this analysis. Future trials should include strategies to address these information gaps.
CONCLUSION
Our results comparing inpatient geriatrics consultative services with usual care in hospitalized elders having cognitive impairment failed to demonstrate an impact on readmissions and mortality. A clinical lesson learned, however, is that much work is still required to reduce readmission and mortality rates in this especially vulnerable patient population.
Disclosures
Disclosures: This work was supported by grants from a Geriatric Academic Career Award (K01HP20517) through Health Resources and Services Administration, R01AG034205 and K23‐AG043476 from the National Institute on Aging, and the John A. Hartford Foundation Center for Excellence in Geriatric Medicine. The sponsors had no role in the study design, evaluation, or manuscript development. The authors report no conflicts of interest.
- Relationship between hospital readmission and mortality rates for patients hospitalized with acute myocardial infarction, heart failure, or pneumonia. JAMA. 2013;309(6):587–593. , , , et al.
- Cognitive impairment. Can it predict the course of hospitalized patients? J Am Geriatr Soc. 1986;34(8):579–585. , , ,
- Importance of functional measures in predicting mortality among older hospitalized patients. JAMA. 1998;279(15):1187–1193. , , , , ,
- Understanding preventable hospital readmissions: masqueraders, markers, and true causal factors. J Hosp Med. 2011;6(2):51–53. ,
- A multicomponent intervention to prevent delirium in hospitalized older patients. N Engl J Med. 1999;340(9):669–676. , , , et al.
- Effects of a multicomponent intervention on functional outcomes and process of care in hospitalized older patients: a randomized controlled trial of Acute Care for Elders (ACE) in a community hospital. J Am Geriatr Soc. 2000;48(12):1572–1581. , , , et al.
- American Geriatrics Society Health Care Systems Committee. Improving the quality of transitional care for persons with complex care needs. J Am Geriatr Soc. 2003;51(4):556–557. , ;
- Development and implementation of a proactive geriatrics consultation model in collaboration with hospitalists. J Am Geriatr Soc. 2009;57(11):2139–2145. , , ,
- Screening of the risk of functional decline performed by an inpatient geriatric consultation team in a general hospital [in French]. Revue medicale de Bruxelles. 2013;34(6):462–468. , , , et al.
- Systematic detection and multidisciplinary care of delirium in older medical inpatients: a randomized trial. CMAJ. 2002;167(7):753–759. , , , et al.
- Potentially inappropriate prescribing for geriatric inpatients: an acute care of the elderly unit compared to a general medicine service. Consult Pharm. 2003;18(1):37–42, 47–39. , ,
- Evaluation of the Mobile Acute Care of the Elderly (MACE) service. JAMA Intern Med. 2013;173(11):990–996. , , ,
- Effects of an acute care for elders unit on costs and 30‐day readmissions. JAMA Intern Med. 2013;173(11):981–987. , , , , ,
- Impact and recognition of cognitive impairment among hospitalized elders. J Hosp Med. 2010;5(2):69–75. , , , et al.
- Enhancing care for hospitalized older adults with cognitive impairment: a randomized controlled trial. J Gen Intern Med. 2012;27(5):561–567. , , , et al.
- Short Portable Mental Status Questionnaire as a screening test for dementia and delirium among the elderly. J Am Geriatr Soc. 1987;35(5):412–416. , , ,
- The Regenstrief Medical Record System: a quarter century experience. Int J Med Inform. 1999;54(3):225–253. , , , et al.
- Factors determining the decision to institutionalize dementing individuals: a prospective study. Gerontologist. 1993;33(6):714–720. , , , , ,
- Clarifying confusion: the confusion assessment method. A new method for detection of delirium. Ann Intern Med. 1990;113(12):941–948. , , , , ,
- Resuscitation: how do we decide? A prospective study of physicians' preferences and the clinical course of hospitalized patients. JAMA. 1986;255(10):1316–1322. , , , , ,
- The APACHE III prognostic system. Risk prediction of hospital mortality for critically ill hospitalized adults. Chest. 1991;100(6):1619–1636. , , , et al.
- Interaction between cognitive impairment and discharge destination and its effect on rehospitalization. J Am Geriatr Soc. 2013;61(11):1958–1963. , , , et al.
- Propensity scores in intensive care and anaesthesiology literature: a systematic review. Intensive Care Med. 2010;36(12):1993–2003. , , , , ,
- Improving functional outcomes in older patients: lessons from an acute care for elders unit. Jt Comm J Qual Improv. 1998;24(2):63–76. , , , et al.
- Developing a stroke unit using the acute care for elders intervention and model of care. J Am Geriatr Soc. 2003;51(11):1660–1667. , , , et al.
- A medical unit for the acute care of the elderly. J Am Geriatr Soc. 1994;42(5):545–552. , , ,
- Impact of geriatric consultation teams on clinical outcome in acute hospitals: a systematic review and meta‐analysis. BMC Med. 2013;11:48. , , , ,
- Comprehensive geriatric assessment for older adults admitted to hospital: meta‐analysis of randomised controlled trials. BMJ. 2011;343:d6553. , , , ,
- Prevention of readmission in elderly patients with congestive heart failure: results of a prospective, randomized pilot study. J Gen Intern Med. 1993;8(11):585–590. , , , et al.
- Effect of a pharmacist intervention on clinically important medication errors after hospital discharge: a randomized trial. Ann Intern Med. 2012;157(1):1–10. , , , et al.
- House calls for seniors: building and sustaining a model of care for homebound seniors. J Am Geriatr Soc. 2009;57(6):1103–1109. , , , ,
- Geriatric care management for low‐income seniors: a randomized controlled trial. JAMA. 2007;298(22):2623–2633. , , , et al.
- Interventions to reduce 30‐day rehospitalization: a systematic review. Ann Intern Med. 2011;155(8):520–528. , , , ,
- The effects of a discharge planning and home follow‐up intervention on elders hospitalized with common medical and surgical cardiac conditions. J Cardiovasc Nurs. 1999;14(1):44–54. ,
- Readmission to hospital: a measure of quality or outcome? Qual Saf Health Care. 2004;13(1):10–11.
- Unintended consequences of steps to cut readmissions and reform payment may threaten care of vulnerable older adults. Health Aff (Millwood). 2012;31(7):1623–1632. , , , , ,
- Analyzing the effects of shared decision‐making, empathy and team interaction on patient satisfaction and treatment acceptance in medical rehabilitation using a structural equation modeling approach. Patient Educ Couns. 2013;91(2):167–175. , ,
- The Care Transitions Innovation (C‐TraIn) for Socioeconomically Disadvantaged Adults: results of a cluster randomized controlled trial. J Gen Intern Med. 2014;29(11):1460–1467. , , ,
- Risk factors for early hospital readmission in low‐income elderly adults. J Am Geriatr Soc. 2014;62(3):489–494. , , ,
- Understanding why patients of low socioeconomic status prefer hospitals over ambulatory care. Health Aff (Millwood). 2013;32(7):1196–1203. , , , , ,
Under the Patient Protection and Affordable Care Act of 2010, commonly referred to as the Affordable Care Act, hospitals face up to a 3% penalty in Medicare reimbursements for patients readmitted within 30 days of initial discharge, and measures have been proposed for modifying payments to hospitals based on their performance on this metric.[1] Cognitive impairment (CI) is considered a major risk factor for poor postdischarge outcomes including mortality and hospital readmission.[2, 3] Hospitals are seeking strategies to reduce postdischarge mortality and rehospitalization among patients with and without CI.[4] Such strategies include use of transitional care coaches, patient and caregiver education, postdischarge follow‐up, and provision of geriatric consultative services (GCS) for the care of complex patients in the hospital setting.[5, 6, 7]
GCS utilize comprehensive geriatric assessments and multidisciplinary processes to recognize and modify risk factors that may lead to poor outcomes among hospitalized patients.[8, 9, 10, 11] Implementation of GCS models including Acute Care for Elders and, recently, the Mobile Acute Care of the Elderly services have shown many benefits among older patients including a reduction in the hospital length of stay and readmission rates.[12, 13] The benefits of such services among hospitalized elders suffering from CI, however, are not well established. The objective of this article was to evaluate the impact of GCS on the readmission and mortality rates of older adults with CI within 12 months of their hospitalization to an urban, public hospital. We hypothesized that GCS will reduce both 12‐month hospital readmissions and mortality rates among this vulnerable group of older adults.
METHODS
The study was approved by the Indiana University institutional review board, and informed consent for identifiable chart review was obtained from subjects or their legally authorized representatives.
Setting
The study was conducted at Eskenazi hospital, Indianapolis, Indiana, a 340‐bed, university‐affiliated, public hospital with over 2300 admissions of patients aged 65 years or older every year.
Population
Four hundred fifteen hospitalized patients aged 65 years or older suffering from CI were enrolled into an original, randomized, controlled trial that evaluated the effect of a computerized decision support system on their quality and outcome of care between July 1, 2006 and May 30, 2008.[14] The computerized decision support included reminders for physicians to reduce the prescription of 18 anticholinergics, minimize physical restraints and Foley catheterization, and increase referral to the local GCS.[15] That previous trial neither showed an impact on quality of care nor health utilization among older patients, including mortality and hospital readmission rates. The current study uses the data from the clinical trial cohort to evaluate the effect of GCS on the 12‐month mortality and hospital readmission rates for hospitalized elders with CI (Figure 1).
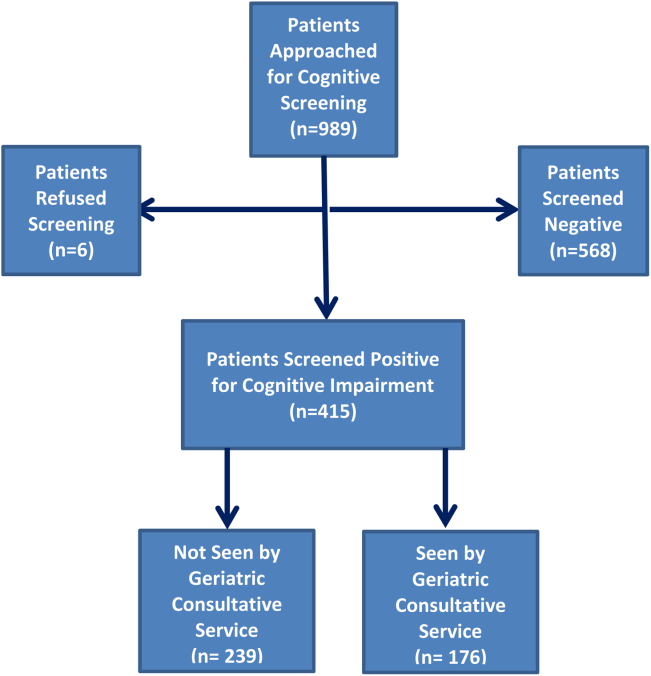
Inclusion and Exclusion Criteria
Individuals were eligible for enrollment if they were aged 65 years or older, hospitalized on a medical ward, able to speak English, and had evidence of CI within 48 hours of hospital admission. Individuals were excluded if they were previously enrolled, were aphasic, or unresponsive. The presence of CI was based on the Short Portable Mental Status Questionnaire (SPMSQ),[16] a brief 10‐item screening test with a sensitivity of 86% and specificity of 99% for dementia using a score of 7 or less (maximum possible score of 10).[16] The SPMSQ scoring process adjusts for participant educational and racial status, which was a benefit to its use given the urban setting of our hospital serving a large proportion of minority and low‐education patients. A physician‐trained research assistant administered the SPMSQ within 48 hours of hospital admission.
Geriatric Consultative Services
GCS is an interdisciplinary team of a geriatrician, a geriatric pharmacist, a case manager nurse, a social worker, a medical assistant, physical therapists, and a representative of the local Area Agency on Aging. There may be a geriatric fellow and/or medicine resident available to the team based on their rotation structure. Team‐based bedside rounds are performed on new consults only, but all patients are seen individually by the team clinicians. The team emphasizes prevention of functional decline and polypharmacy, recognition and treatment of geriatric syndromes including dementia and delirium, and early discharge/transition planning. Consensus recommendations are prepared and documented in the consult notes section of the electronic medical records. Recommendations deemed critical are discussed directly with the primary teams, but no orders are placed by the GCS team. The GCS team is available on all weekdays but not on weekends or major holidays.
Study Outcomes
For this secondary analysis, we used the Regenstrief Medical Record System (RMRS) to measure 2 outcomes: hospital readmission and mortality rates up to 1 year from discharge following index hospitalization, defined as the first admission in the original clinical trial. The RMRS is the primary instrument for processing data and monitoring patient and physician activity for the hospital.[17, 18] The RMRS is linked with a state‐wide health information exchange to capture data on hospitalization outside the hospital. The RMRS also contains death certificate information for all registered patients who die in or outside the Eskenazi hospital.
Other Data Collections
Delirium was assessed at screening and then every weekday using the Confusion Assessment Method (CAM) by a trained research assistant.[19] CAM evaluates 10 symptoms of delirium specified in the Diagnostic and Statistical Manual of Mental Disorders‐III‐Revision: acute onset, fluctuating course, inattention, disorganized thinking, altered level of consciousness, disorientation, memory impairment, perceptual disturbances, psychomotor agitation or retardation, and sleep/wake disturbance. Participant demographic characteristics, including age, sex, ethnicity, and years of education, were collected from the RMRS and from interviews performed at the time of cognitive screening. Information on length of hospital stay and discharge destination (eg, home vs facility, including skilled nursing and acute rehabilitation facilities) was also obtained from the RMRS. Charlson Comorbidity Index score was calculated using International Classification of Diseases, Ninth Revision codes gathered from 1 year before admission until the time of each participant's discharge from the hospital.[20] The Acute Physiology Score (APS) from the Acute Physiology and Chronic Health Evaluation (APACHE) III was derived from data available in the RMRS to measure the severity of illness.[21] Although the APACHE III was developed in the intensive care unit using data from the first 24 hours after admission, for our study we used the worst laboratory test value during the entire hospital stay to calculate the APS.[22]
Statistical Analysis
Baseline variables are presented as means and standard deviations for continuous variables, and percentages for binary categorical variables. Comparisons between patients receiving GCS and those who did not were performed using 2 tests for categorical variables and Kruskal‐Wallis test for continuous variables. Cox proportional hazard models were used to determine the association between receiving GCS and time to hospital readmission or mortality within 30 days or 1‐year postindex admission while adjusting for other covariates. For the models using time to readmission, patients without readmission were censored either at the endpoint (30 days or 1 year) or at time of death for those who died within the time frame in each model. Because GCS was not randomly assigned, we also conducted a propensity score analysis.[23] A logistic model for the probability of receiving GCS was conducted using patient demographic variables and information collected before and at the time of GCS. Stratified Cox proportional models using quintiles of predicted probability of receiving GCS were used in a propensity‐adjusted Cox model. All data analyses were performed using SAS version 9.3 (SAS Institute, Inc., Cary, NC).
RESULTS
Between July 1, 2006 and May 30, 2008, 415 CI patients were enrolled in the original trial, with 176 receiving the GCS. As shown in Table 1, the GCS and non‐GCS groups differed significantly. The GCS group was older (79.2 years old, 8.1 standard deviation [SD] vs 75.8 years old, 7.8 SD; P0.001), scored lower on the SPMSQ (4.7, 2.7 SD vs 5.5, 2.7 SD; P=0.002), had fewer chronic conditions with a lower mean Charlson Comorbidity Index Score (2.1, 1.86 SD vs 2.8, 2.6 SD; P=0.023), but a higher percentage of delirium (48.9% vs 29.3%), a lower percentage of being discharged home (37.5% vs 56.1%), and a higher mean length of stay (6.4 days, 6.4 SD vs 5.6 days, 5.9 SD; P=0.004). They also had a lower malignancy rate (6.2% vs 14.6%; P=0.007) and a lower number of hospitalizations in the previous year (0.5 admissions, 0.9 SD vs 0.7 admissions, 1.1 SD; P=0.035). No differences were observed in regard to gender, ethnicity, history of myocardial infarctions, chronic obstructive pulmonary disease, cerebrovascular disease, peripheral vascular disease, diabetes, and use of anticholinergic medicines.
No GCS, n=239 | GCS, n=176 | P Value* | |
---|---|---|---|
| |||
Baseline characteristics | |||
Mean age (SD) | 75.8 (7.8) | 79.2 (8.1) | <0.001 |
% Female | 66.1 [n=158] | 68.2 [n=120] | 0.657 |
% African American | 54.8 [n=131] | 63.6 [n=112] | 0.071 |
Mean SPMSQ score (SD) | 5.5 (2.7) | 4.7 (2.7) | 0.002 |
Admission diagnoses | |||
MI | 15.5 [n=37] | 13.6 [n=24] | 0.675 |
CHF | 38.1 [n=91] | 34.7 [n=61] | 0.475 |
PVD | 7.1 [n=17] | 9.7 [n=17] | 0.370 |
Cerebrovascular | 13.8 [n=33] | 19.3 [n=34] | 0.140 |
COPD | 41.0 [n=98] | 33.0 [n=58] | 0.094 |
Diabetes | 47.7 [n=114] | 40.9 [n=72] | 0.169 |
Malignancy | 14.6 [n=35] | 6.2 [n=11] | 0.007 |
Metastatic cancer | 8.8 [n=21] | 1.7 [n=3] | 0.002 |
Mean Charlson Comorbidity (SD) | 2.8 (2.6) | 2.1 (1.8) | 0.023 |
Mean APS (SD) | 24.5 (13.8) | 25.9 (13.5) | 0.231 |
Definite ACB Use | 35.2 [n=84] | 27.8 [n=49] | 0.136 |
Length of stay | 5.6 (5.9) | 6.4 (6.4) | 0.004 |
% Any delirium | 29.3 [n=70] | 48.9 [n=156] | <0.001 |
% Discharged home | 56.1 [n=134] | 37.5 [n=66] | <0.001 |
No. of inpatient stays prior year | 0.7 (1.1) | 0.5 (0.9) | 0.035 |
Follow‐up outcomes | |||
% Readmission within 30 days | 15.1 [n=36] | 22.7 [n=40] | 0.054 |
% Readmission within 1 year | 54.4 [n=130] | 56.3 [n=99] | 0.765 |
% Death within 30 days | 4.2 [n=10] | 1.7 [n=3] | 0.253 |
% Death within 1 year | 26.8 [n=64] | 23.9 [n=42] | 0.569 |
% Readmission or death within 30 days | 18.0 [n=43] | 24.4 [n=43] | 0.113 |
% Readmission or death within 1 year | 64.8 [n=155] | 63.1 [n=111] | 0.708 |
Table 2 describes the association of various factors with receiving GCS. Patients who were positive for delirium (odds ratio [OR]=1.65; 95% confidence interval=0.98‐2.77) and were older (OR=1.04; 95% confidence interval=1.01‐1.08) had a higher propensity to receive GCS, whereas, the presence of metastatic cancer resulted in a lower propensity (OR=0.15; 95% confidence interval=0.02‐1.16) of receiving GCS. The logistic model estimated area under the receiver operating characteristic curve was 0.707.
Adjusted OR (95% CI) | P Value | |
---|---|---|
| ||
Age | 1.04 (1.011.08) | 0.006 |
Female | 1.02 (0.641.63) | 0.942 |
African American | 1.11 (0.711.72) | 0.657 |
Short Portable Mental Status Questionnaire score | 1.00 (0.911.10) | 0.990 |
Acute Physiology Score | 1.00 (0.981.02) | 0.769 |
Charlson Comorbidity Score | 1.11 (0.841.46) | 0.471 |
Length of hospital stay | 1.02 (0.981.07) | 0.299 |
Definite anticholinergic use* | 0.74 (0.461.20) | 0.219 |
Any delirium during hospital stay | 1.65 (0.982.77) | 0.061 |
Diabetes mellitus | 0.72 (0.411.26) | 0.253 |
Myocardial infarction | 0.83 (0.411.66) | 0.593 |
Congestive heart failure | 0.83 (0.471.47) | 0.524 |
Peripheral vascular disease | 1.39 (0.613.18) | 0.433 |
Cerebrovascular disease | 1.30 (0.652.59) | 0.464 |
Malignancy | 0.45 (0.171.21) | 0.113 |
Metastatic cancer | 0.15 (0.021.16) | 0.069 |
Chronic obstructive pulmonary disease | 0.91 (0.531.55) | 0.727 |
Table 3 provides results from the Cox models for receiving GCS on readmission and mortality outcomes adjusting for various sets of covariates and with the propensity score adjustment. Model 1 presents unadjusted hazard ratio (HR). Model 2 presents HRs adjusting for a common set of covariates that were significantly associated with at least 1 of the outcomes, whereas model 3 presents the results adjusting for all covariates. All 4 models yielded similar results. As evident from this table, propensity‐adjusted HR for 30‐day readmission was still significantly higher among patients receiving GCS (HR=1.75; 95% confidence interval=1.06‐2.88) but not at 1 year (HR=1.19; 95% confidence interval=0.89‐1.59). There was a trend for decreased mortality for the GCS group at 30 days (HR=0.35; 95% confidence interval=0.09‐1.35), but it disappeared at 1 year (HR=0.91; 95% confidence interval=0.59‐1.40). A composite outcome of readmissions and mortality did not show any difference between the GCS and no‐GCS groups.
Outcome Variables | Model 1 | Model 2 | Model 3 | Propensity Adjusted | ||||
---|---|---|---|---|---|---|---|---|
HR (95% CI) | P Value | HR (95% CI) | P Value | HR (95% CI) | P Value | HR (95% CI) | P Value | |
| ||||||||
Readmission within 30 days | 1.65 (1.05, 2.59) | 0.030 | 1.73 (1.08, 2.78) | 0.024 | 1.84 (1.133.00) | 0.015 | 1.75 (1.062.88) | 0.029 |
Readmission within 1 year | 1.13 (0.87, 1.46) | 0.373 | 1.24 (0.94, 1.63) | 0.125 | 1.26 (0.941.68) | 0.117 | 1.19 (0.891.59) | 0.245 |
Death within 30 days | 0.43 (0.12, 1.56) | 0.199 | 0.34 (0.09, 1.28) | 0.110 | 0.25 (0.061.02) | 0.053 | 0.35 (0.091.35) | 0.126 |
Death within 1 year | 0.95 (0.65, 1.45) | 0.806 | 0.87 (0.58 1.31) | 0.506 | 0.93 (0.601.42) | 0.724 | 0.91 (0.591.40) | 0.669 |
Readmission or Death within 30 days | 1.48 (0.97, 2.26) | 0.070 | 1.49 (0.96, 2.33) | 0.078 | 1.56 (0.982.47) | 0.061 | 1.55 (0.972.48) | 0.069 |
Readmission or death within 1 year | 1.05 (0.82, 1.34) | 0.699 | 1.11 (0.86, 1.43) | 0.412 | 1.15 (0.881.50) | 0.318 | 1.08 (0.831.42) | 0.569 |
DISCUSSION
To our knowledge, this is the first study to analyze the impact of GCS on hospital readmission and mortality rates of CI patients. Our results did not show any short‐term or long‐term benefits of GCS for CI patients. Recent studies exploring cost benefits of the GCS have found trends toward lower readmission, but none focused on patients with CI.[6, 24, 25] It is important to note that our study did not use random allocation to assigning the patient into the GCS or control group, thus raising the possibility that patients who received GCS were sicker and were medically and socially more complex than those who did not receive the consult. Moreover, GCS consultation is preferentially sought for and completed for patients with CI and functional limitations, consistent with our finding that GCS patients more often have delirium and are less‐often discharged home.
The nature of the GCS team is another important consideration. Our GCS model did not include unit cohorting of patients, an important component of other proposed GCS models.[26] A recent meta‐analysis found that the GCS models without unit cohorting of patients did not have an impact on 1‐ or 12‐month readmission rates.[27] Low adherence to consultant recommendations (less than 33%) was thought to be a reason for such results. Importance of cohorting with regard to accomplishing recommendations by primary teams, importance of unit staff expertise in geriatric principles, and impact of a unit model on teamwork has also been highlighted by another review.[28] These findings lend to the hypothesis that unit cohorting and direct order placement by the GCS team may improve outcomes among CI patients, including a reduction in readmission rates.
Although readmissions rates were not statistically different between GCS and control groups at 1‐year postdischarge, 30‐day readmission rates were higher among the GCS group. Previous research among older heart failure patients found that a comprehensive transitional care intervention at the time of hospital discharge significantly shortened the time to readmission in the intervention group (P=0.026).[29] The factors identified by the study authors included enhanced supervision by the transitional healthcare teams along with improved awareness and education among treated patients that may have facilitated early recognition of clinical deterioration.[29] A recent study with intensive outpatient care that resulted in increased admissions among chronically ill adults provided a similar conclusion.[30]
GCS patients showed a trend toward decreased mortality as did patients enrolled in previous studies evaluating GCS models in the inpatient setting, as suggested by a recent review.[27] A caveat to note is that these trends favored ward‐styled GCS services as compared to our open GCS model,[27, 28] although the factors cited in these dedicated units affecting mortality included prompt attention to early rehabilitation, delirium management, and prevention of pressure ulcers and are also frequently implemented for patients in our GCS service model and therefore may have produced similar results.
Our neutral results in regard to the readmissions need to be interpreted with caution. First, this study was conducted in a hospital that supports expert geriatric and palliative care teams, both in the inpatient and the ambulatory settings, that provide consultative services and train medicine teams and hospital nursing staff. On the outpatient side, the presence of a robust geriatrics house‐calls program and the Geriatric Resources for Assessment and Care of Elders team results in above‐average care for the control group, and thus may also impact apparent outcomes.[31, 32] Second, 30‐day readmissions represent a complex outcome. Two recent reviews of hospital‐initiated interventions have shown that evidence regarding best strategies to decrease 30‐day readmissions is unclear.[33] Neither review included studies that targeted patients with CI only. The 2 programs that reduced 30‐day readmissions were multifaceted and included personnel who provide bridging between the hospital and the outpatient setting.[34] The GCS does include a focus on postdischarge resources, but does that on a case‐by‐case basis and no formal posthospital follow‐ups are provided. Moreover, the value of 30‐day readmission rates as a marker of quality, even though used by policymakers as an indicator of hospital quality, remains controversial.[35, 36] Broadening the outcomes of interest to include patient‐centered outcomes including satisfaction with care, that have shown to impact other health outcomes, may help improve understanding the benefits of GCS in hospitals.[37] Other comprehensive transitional care models that failed to show a benefit on 30‐day readmissions in older patients still resulted in higher satisfaction among patients.[38] Unfortunately, our evaluation did not include an assessment of patient satisfaction and quality of transitions.
Since the study period, GCS at our hospital now has incorporated a more robust focus on advance care planning (ACP) and execution of Physician Orders for Scope of Treatment that were legislated in the state in July 2013. The GCS team members are expert in carrying out complex ACP discussions and also partner with the inpatient palliative care team. It is quite possible that a study of more recent outcomes will yield more positive results for the selected outcomes. Thus, for future trials that aim to study the impact of GCS in the inpatient settings, it may be advisable to include important quality markers such as implementation of ACP and patient satisfaction along with the health utilization outcomes.
Limitations
As mentioned previously, it is possible that our risk adjustment was insufficient to account for all the medical and psychosocial differences among groups. For example, the overall anticholinergic impact of various medications such as antipsychotic medications and histamine‐2 blockers was assessed via the Anticholinergic Burden Scale on admission, but we did not have information on medication prescribing during the stay. We were further limited by lack of baseline functional status and socioeconomic details, both of which are related to 30‐day readmissions. For example, living alone, prior use of assist devices, and belonging to lower socioeconomic status are correlated with higher readmission rates.[39, 40] Patients with available social support may receive more intense supervision and may seek medical attention sooner. On the other hand, worsening health among CI patients without any approximate social support may be unnoticed for days. Absence of details of inpatient interventions may also have resulted in unmeasurable confounders that could have impacted our study outcomes. Finally, lack of information on the uptake of GCS recommendations by the primary teams is another limitation of this analysis. Future trials should include strategies to address these information gaps.
CONCLUSION
Our results comparing inpatient geriatrics consultative services with usual care in hospitalized elders having cognitive impairment failed to demonstrate an impact on readmissions and mortality. A clinical lesson learned, however, is that much work is still required to reduce readmission and mortality rates in this especially vulnerable patient population.
Disclosures
Disclosures: This work was supported by grants from a Geriatric Academic Career Award (K01HP20517) through Health Resources and Services Administration, R01AG034205 and K23‐AG043476 from the National Institute on Aging, and the John A. Hartford Foundation Center for Excellence in Geriatric Medicine. The sponsors had no role in the study design, evaluation, or manuscript development. The authors report no conflicts of interest.
Under the Patient Protection and Affordable Care Act of 2010, commonly referred to as the Affordable Care Act, hospitals face up to a 3% penalty in Medicare reimbursements for patients readmitted within 30 days of initial discharge, and measures have been proposed for modifying payments to hospitals based on their performance on this metric.[1] Cognitive impairment (CI) is considered a major risk factor for poor postdischarge outcomes including mortality and hospital readmission.[2, 3] Hospitals are seeking strategies to reduce postdischarge mortality and rehospitalization among patients with and without CI.[4] Such strategies include use of transitional care coaches, patient and caregiver education, postdischarge follow‐up, and provision of geriatric consultative services (GCS) for the care of complex patients in the hospital setting.[5, 6, 7]
GCS utilize comprehensive geriatric assessments and multidisciplinary processes to recognize and modify risk factors that may lead to poor outcomes among hospitalized patients.[8, 9, 10, 11] Implementation of GCS models including Acute Care for Elders and, recently, the Mobile Acute Care of the Elderly services have shown many benefits among older patients including a reduction in the hospital length of stay and readmission rates.[12, 13] The benefits of such services among hospitalized elders suffering from CI, however, are not well established. The objective of this article was to evaluate the impact of GCS on the readmission and mortality rates of older adults with CI within 12 months of their hospitalization to an urban, public hospital. We hypothesized that GCS will reduce both 12‐month hospital readmissions and mortality rates among this vulnerable group of older adults.
METHODS
The study was approved by the Indiana University institutional review board, and informed consent for identifiable chart review was obtained from subjects or their legally authorized representatives.
Setting
The study was conducted at Eskenazi hospital, Indianapolis, Indiana, a 340‐bed, university‐affiliated, public hospital with over 2300 admissions of patients aged 65 years or older every year.
Population
Four hundred fifteen hospitalized patients aged 65 years or older suffering from CI were enrolled into an original, randomized, controlled trial that evaluated the effect of a computerized decision support system on their quality and outcome of care between July 1, 2006 and May 30, 2008.[14] The computerized decision support included reminders for physicians to reduce the prescription of 18 anticholinergics, minimize physical restraints and Foley catheterization, and increase referral to the local GCS.[15] That previous trial neither showed an impact on quality of care nor health utilization among older patients, including mortality and hospital readmission rates. The current study uses the data from the clinical trial cohort to evaluate the effect of GCS on the 12‐month mortality and hospital readmission rates for hospitalized elders with CI (Figure 1).
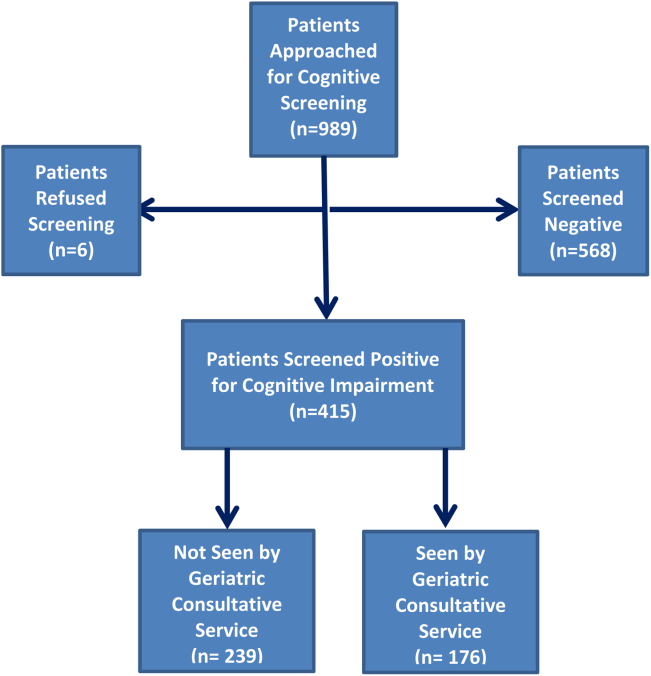
Inclusion and Exclusion Criteria
Individuals were eligible for enrollment if they were aged 65 years or older, hospitalized on a medical ward, able to speak English, and had evidence of CI within 48 hours of hospital admission. Individuals were excluded if they were previously enrolled, were aphasic, or unresponsive. The presence of CI was based on the Short Portable Mental Status Questionnaire (SPMSQ),[16] a brief 10‐item screening test with a sensitivity of 86% and specificity of 99% for dementia using a score of 7 or less (maximum possible score of 10).[16] The SPMSQ scoring process adjusts for participant educational and racial status, which was a benefit to its use given the urban setting of our hospital serving a large proportion of minority and low‐education patients. A physician‐trained research assistant administered the SPMSQ within 48 hours of hospital admission.
Geriatric Consultative Services
GCS is an interdisciplinary team of a geriatrician, a geriatric pharmacist, a case manager nurse, a social worker, a medical assistant, physical therapists, and a representative of the local Area Agency on Aging. There may be a geriatric fellow and/or medicine resident available to the team based on their rotation structure. Team‐based bedside rounds are performed on new consults only, but all patients are seen individually by the team clinicians. The team emphasizes prevention of functional decline and polypharmacy, recognition and treatment of geriatric syndromes including dementia and delirium, and early discharge/transition planning. Consensus recommendations are prepared and documented in the consult notes section of the electronic medical records. Recommendations deemed critical are discussed directly with the primary teams, but no orders are placed by the GCS team. The GCS team is available on all weekdays but not on weekends or major holidays.
Study Outcomes
For this secondary analysis, we used the Regenstrief Medical Record System (RMRS) to measure 2 outcomes: hospital readmission and mortality rates up to 1 year from discharge following index hospitalization, defined as the first admission in the original clinical trial. The RMRS is the primary instrument for processing data and monitoring patient and physician activity for the hospital.[17, 18] The RMRS is linked with a state‐wide health information exchange to capture data on hospitalization outside the hospital. The RMRS also contains death certificate information for all registered patients who die in or outside the Eskenazi hospital.
Other Data Collections
Delirium was assessed at screening and then every weekday using the Confusion Assessment Method (CAM) by a trained research assistant.[19] CAM evaluates 10 symptoms of delirium specified in the Diagnostic and Statistical Manual of Mental Disorders‐III‐Revision: acute onset, fluctuating course, inattention, disorganized thinking, altered level of consciousness, disorientation, memory impairment, perceptual disturbances, psychomotor agitation or retardation, and sleep/wake disturbance. Participant demographic characteristics, including age, sex, ethnicity, and years of education, were collected from the RMRS and from interviews performed at the time of cognitive screening. Information on length of hospital stay and discharge destination (eg, home vs facility, including skilled nursing and acute rehabilitation facilities) was also obtained from the RMRS. Charlson Comorbidity Index score was calculated using International Classification of Diseases, Ninth Revision codes gathered from 1 year before admission until the time of each participant's discharge from the hospital.[20] The Acute Physiology Score (APS) from the Acute Physiology and Chronic Health Evaluation (APACHE) III was derived from data available in the RMRS to measure the severity of illness.[21] Although the APACHE III was developed in the intensive care unit using data from the first 24 hours after admission, for our study we used the worst laboratory test value during the entire hospital stay to calculate the APS.[22]
Statistical Analysis
Baseline variables are presented as means and standard deviations for continuous variables, and percentages for binary categorical variables. Comparisons between patients receiving GCS and those who did not were performed using 2 tests for categorical variables and Kruskal‐Wallis test for continuous variables. Cox proportional hazard models were used to determine the association between receiving GCS and time to hospital readmission or mortality within 30 days or 1‐year postindex admission while adjusting for other covariates. For the models using time to readmission, patients without readmission were censored either at the endpoint (30 days or 1 year) or at time of death for those who died within the time frame in each model. Because GCS was not randomly assigned, we also conducted a propensity score analysis.[23] A logistic model for the probability of receiving GCS was conducted using patient demographic variables and information collected before and at the time of GCS. Stratified Cox proportional models using quintiles of predicted probability of receiving GCS were used in a propensity‐adjusted Cox model. All data analyses were performed using SAS version 9.3 (SAS Institute, Inc., Cary, NC).
RESULTS
Between July 1, 2006 and May 30, 2008, 415 CI patients were enrolled in the original trial, with 176 receiving the GCS. As shown in Table 1, the GCS and non‐GCS groups differed significantly. The GCS group was older (79.2 years old, 8.1 standard deviation [SD] vs 75.8 years old, 7.8 SD; P0.001), scored lower on the SPMSQ (4.7, 2.7 SD vs 5.5, 2.7 SD; P=0.002), had fewer chronic conditions with a lower mean Charlson Comorbidity Index Score (2.1, 1.86 SD vs 2.8, 2.6 SD; P=0.023), but a higher percentage of delirium (48.9% vs 29.3%), a lower percentage of being discharged home (37.5% vs 56.1%), and a higher mean length of stay (6.4 days, 6.4 SD vs 5.6 days, 5.9 SD; P=0.004). They also had a lower malignancy rate (6.2% vs 14.6%; P=0.007) and a lower number of hospitalizations in the previous year (0.5 admissions, 0.9 SD vs 0.7 admissions, 1.1 SD; P=0.035). No differences were observed in regard to gender, ethnicity, history of myocardial infarctions, chronic obstructive pulmonary disease, cerebrovascular disease, peripheral vascular disease, diabetes, and use of anticholinergic medicines.
No GCS, n=239 | GCS, n=176 | P Value* | |
---|---|---|---|
| |||
Baseline characteristics | |||
Mean age (SD) | 75.8 (7.8) | 79.2 (8.1) | <0.001 |
% Female | 66.1 [n=158] | 68.2 [n=120] | 0.657 |
% African American | 54.8 [n=131] | 63.6 [n=112] | 0.071 |
Mean SPMSQ score (SD) | 5.5 (2.7) | 4.7 (2.7) | 0.002 |
Admission diagnoses | |||
MI | 15.5 [n=37] | 13.6 [n=24] | 0.675 |
CHF | 38.1 [n=91] | 34.7 [n=61] | 0.475 |
PVD | 7.1 [n=17] | 9.7 [n=17] | 0.370 |
Cerebrovascular | 13.8 [n=33] | 19.3 [n=34] | 0.140 |
COPD | 41.0 [n=98] | 33.0 [n=58] | 0.094 |
Diabetes | 47.7 [n=114] | 40.9 [n=72] | 0.169 |
Malignancy | 14.6 [n=35] | 6.2 [n=11] | 0.007 |
Metastatic cancer | 8.8 [n=21] | 1.7 [n=3] | 0.002 |
Mean Charlson Comorbidity (SD) | 2.8 (2.6) | 2.1 (1.8) | 0.023 |
Mean APS (SD) | 24.5 (13.8) | 25.9 (13.5) | 0.231 |
Definite ACB Use | 35.2 [n=84] | 27.8 [n=49] | 0.136 |
Length of stay | 5.6 (5.9) | 6.4 (6.4) | 0.004 |
% Any delirium | 29.3 [n=70] | 48.9 [n=156] | <0.001 |
% Discharged home | 56.1 [n=134] | 37.5 [n=66] | <0.001 |
No. of inpatient stays prior year | 0.7 (1.1) | 0.5 (0.9) | 0.035 |
Follow‐up outcomes | |||
% Readmission within 30 days | 15.1 [n=36] | 22.7 [n=40] | 0.054 |
% Readmission within 1 year | 54.4 [n=130] | 56.3 [n=99] | 0.765 |
% Death within 30 days | 4.2 [n=10] | 1.7 [n=3] | 0.253 |
% Death within 1 year | 26.8 [n=64] | 23.9 [n=42] | 0.569 |
% Readmission or death within 30 days | 18.0 [n=43] | 24.4 [n=43] | 0.113 |
% Readmission or death within 1 year | 64.8 [n=155] | 63.1 [n=111] | 0.708 |
Table 2 describes the association of various factors with receiving GCS. Patients who were positive for delirium (odds ratio [OR]=1.65; 95% confidence interval=0.98‐2.77) and were older (OR=1.04; 95% confidence interval=1.01‐1.08) had a higher propensity to receive GCS, whereas, the presence of metastatic cancer resulted in a lower propensity (OR=0.15; 95% confidence interval=0.02‐1.16) of receiving GCS. The logistic model estimated area under the receiver operating characteristic curve was 0.707.
Adjusted OR (95% CI) | P Value | |
---|---|---|
| ||
Age | 1.04 (1.011.08) | 0.006 |
Female | 1.02 (0.641.63) | 0.942 |
African American | 1.11 (0.711.72) | 0.657 |
Short Portable Mental Status Questionnaire score | 1.00 (0.911.10) | 0.990 |
Acute Physiology Score | 1.00 (0.981.02) | 0.769 |
Charlson Comorbidity Score | 1.11 (0.841.46) | 0.471 |
Length of hospital stay | 1.02 (0.981.07) | 0.299 |
Definite anticholinergic use* | 0.74 (0.461.20) | 0.219 |
Any delirium during hospital stay | 1.65 (0.982.77) | 0.061 |
Diabetes mellitus | 0.72 (0.411.26) | 0.253 |
Myocardial infarction | 0.83 (0.411.66) | 0.593 |
Congestive heart failure | 0.83 (0.471.47) | 0.524 |
Peripheral vascular disease | 1.39 (0.613.18) | 0.433 |
Cerebrovascular disease | 1.30 (0.652.59) | 0.464 |
Malignancy | 0.45 (0.171.21) | 0.113 |
Metastatic cancer | 0.15 (0.021.16) | 0.069 |
Chronic obstructive pulmonary disease | 0.91 (0.531.55) | 0.727 |
Table 3 provides results from the Cox models for receiving GCS on readmission and mortality outcomes adjusting for various sets of covariates and with the propensity score adjustment. Model 1 presents unadjusted hazard ratio (HR). Model 2 presents HRs adjusting for a common set of covariates that were significantly associated with at least 1 of the outcomes, whereas model 3 presents the results adjusting for all covariates. All 4 models yielded similar results. As evident from this table, propensity‐adjusted HR for 30‐day readmission was still significantly higher among patients receiving GCS (HR=1.75; 95% confidence interval=1.06‐2.88) but not at 1 year (HR=1.19; 95% confidence interval=0.89‐1.59). There was a trend for decreased mortality for the GCS group at 30 days (HR=0.35; 95% confidence interval=0.09‐1.35), but it disappeared at 1 year (HR=0.91; 95% confidence interval=0.59‐1.40). A composite outcome of readmissions and mortality did not show any difference between the GCS and no‐GCS groups.
Outcome Variables | Model 1 | Model 2 | Model 3 | Propensity Adjusted | ||||
---|---|---|---|---|---|---|---|---|
HR (95% CI) | P Value | HR (95% CI) | P Value | HR (95% CI) | P Value | HR (95% CI) | P Value | |
| ||||||||
Readmission within 30 days | 1.65 (1.05, 2.59) | 0.030 | 1.73 (1.08, 2.78) | 0.024 | 1.84 (1.133.00) | 0.015 | 1.75 (1.062.88) | 0.029 |
Readmission within 1 year | 1.13 (0.87, 1.46) | 0.373 | 1.24 (0.94, 1.63) | 0.125 | 1.26 (0.941.68) | 0.117 | 1.19 (0.891.59) | 0.245 |
Death within 30 days | 0.43 (0.12, 1.56) | 0.199 | 0.34 (0.09, 1.28) | 0.110 | 0.25 (0.061.02) | 0.053 | 0.35 (0.091.35) | 0.126 |
Death within 1 year | 0.95 (0.65, 1.45) | 0.806 | 0.87 (0.58 1.31) | 0.506 | 0.93 (0.601.42) | 0.724 | 0.91 (0.591.40) | 0.669 |
Readmission or Death within 30 days | 1.48 (0.97, 2.26) | 0.070 | 1.49 (0.96, 2.33) | 0.078 | 1.56 (0.982.47) | 0.061 | 1.55 (0.972.48) | 0.069 |
Readmission or death within 1 year | 1.05 (0.82, 1.34) | 0.699 | 1.11 (0.86, 1.43) | 0.412 | 1.15 (0.881.50) | 0.318 | 1.08 (0.831.42) | 0.569 |
DISCUSSION
To our knowledge, this is the first study to analyze the impact of GCS on hospital readmission and mortality rates of CI patients. Our results did not show any short‐term or long‐term benefits of GCS for CI patients. Recent studies exploring cost benefits of the GCS have found trends toward lower readmission, but none focused on patients with CI.[6, 24, 25] It is important to note that our study did not use random allocation to assigning the patient into the GCS or control group, thus raising the possibility that patients who received GCS were sicker and were medically and socially more complex than those who did not receive the consult. Moreover, GCS consultation is preferentially sought for and completed for patients with CI and functional limitations, consistent with our finding that GCS patients more often have delirium and are less‐often discharged home.
The nature of the GCS team is another important consideration. Our GCS model did not include unit cohorting of patients, an important component of other proposed GCS models.[26] A recent meta‐analysis found that the GCS models without unit cohorting of patients did not have an impact on 1‐ or 12‐month readmission rates.[27] Low adherence to consultant recommendations (less than 33%) was thought to be a reason for such results. Importance of cohorting with regard to accomplishing recommendations by primary teams, importance of unit staff expertise in geriatric principles, and impact of a unit model on teamwork has also been highlighted by another review.[28] These findings lend to the hypothesis that unit cohorting and direct order placement by the GCS team may improve outcomes among CI patients, including a reduction in readmission rates.
Although readmissions rates were not statistically different between GCS and control groups at 1‐year postdischarge, 30‐day readmission rates were higher among the GCS group. Previous research among older heart failure patients found that a comprehensive transitional care intervention at the time of hospital discharge significantly shortened the time to readmission in the intervention group (P=0.026).[29] The factors identified by the study authors included enhanced supervision by the transitional healthcare teams along with improved awareness and education among treated patients that may have facilitated early recognition of clinical deterioration.[29] A recent study with intensive outpatient care that resulted in increased admissions among chronically ill adults provided a similar conclusion.[30]
GCS patients showed a trend toward decreased mortality as did patients enrolled in previous studies evaluating GCS models in the inpatient setting, as suggested by a recent review.[27] A caveat to note is that these trends favored ward‐styled GCS services as compared to our open GCS model,[27, 28] although the factors cited in these dedicated units affecting mortality included prompt attention to early rehabilitation, delirium management, and prevention of pressure ulcers and are also frequently implemented for patients in our GCS service model and therefore may have produced similar results.
Our neutral results in regard to the readmissions need to be interpreted with caution. First, this study was conducted in a hospital that supports expert geriatric and palliative care teams, both in the inpatient and the ambulatory settings, that provide consultative services and train medicine teams and hospital nursing staff. On the outpatient side, the presence of a robust geriatrics house‐calls program and the Geriatric Resources for Assessment and Care of Elders team results in above‐average care for the control group, and thus may also impact apparent outcomes.[31, 32] Second, 30‐day readmissions represent a complex outcome. Two recent reviews of hospital‐initiated interventions have shown that evidence regarding best strategies to decrease 30‐day readmissions is unclear.[33] Neither review included studies that targeted patients with CI only. The 2 programs that reduced 30‐day readmissions were multifaceted and included personnel who provide bridging between the hospital and the outpatient setting.[34] The GCS does include a focus on postdischarge resources, but does that on a case‐by‐case basis and no formal posthospital follow‐ups are provided. Moreover, the value of 30‐day readmission rates as a marker of quality, even though used by policymakers as an indicator of hospital quality, remains controversial.[35, 36] Broadening the outcomes of interest to include patient‐centered outcomes including satisfaction with care, that have shown to impact other health outcomes, may help improve understanding the benefits of GCS in hospitals.[37] Other comprehensive transitional care models that failed to show a benefit on 30‐day readmissions in older patients still resulted in higher satisfaction among patients.[38] Unfortunately, our evaluation did not include an assessment of patient satisfaction and quality of transitions.
Since the study period, GCS at our hospital now has incorporated a more robust focus on advance care planning (ACP) and execution of Physician Orders for Scope of Treatment that were legislated in the state in July 2013. The GCS team members are expert in carrying out complex ACP discussions and also partner with the inpatient palliative care team. It is quite possible that a study of more recent outcomes will yield more positive results for the selected outcomes. Thus, for future trials that aim to study the impact of GCS in the inpatient settings, it may be advisable to include important quality markers such as implementation of ACP and patient satisfaction along with the health utilization outcomes.
Limitations
As mentioned previously, it is possible that our risk adjustment was insufficient to account for all the medical and psychosocial differences among groups. For example, the overall anticholinergic impact of various medications such as antipsychotic medications and histamine‐2 blockers was assessed via the Anticholinergic Burden Scale on admission, but we did not have information on medication prescribing during the stay. We were further limited by lack of baseline functional status and socioeconomic details, both of which are related to 30‐day readmissions. For example, living alone, prior use of assist devices, and belonging to lower socioeconomic status are correlated with higher readmission rates.[39, 40] Patients with available social support may receive more intense supervision and may seek medical attention sooner. On the other hand, worsening health among CI patients without any approximate social support may be unnoticed for days. Absence of details of inpatient interventions may also have resulted in unmeasurable confounders that could have impacted our study outcomes. Finally, lack of information on the uptake of GCS recommendations by the primary teams is another limitation of this analysis. Future trials should include strategies to address these information gaps.
CONCLUSION
Our results comparing inpatient geriatrics consultative services with usual care in hospitalized elders having cognitive impairment failed to demonstrate an impact on readmissions and mortality. A clinical lesson learned, however, is that much work is still required to reduce readmission and mortality rates in this especially vulnerable patient population.
Disclosures
Disclosures: This work was supported by grants from a Geriatric Academic Career Award (K01HP20517) through Health Resources and Services Administration, R01AG034205 and K23‐AG043476 from the National Institute on Aging, and the John A. Hartford Foundation Center for Excellence in Geriatric Medicine. The sponsors had no role in the study design, evaluation, or manuscript development. The authors report no conflicts of interest.
- Relationship between hospital readmission and mortality rates for patients hospitalized with acute myocardial infarction, heart failure, or pneumonia. JAMA. 2013;309(6):587–593. , , , et al.
- Cognitive impairment. Can it predict the course of hospitalized patients? J Am Geriatr Soc. 1986;34(8):579–585. , , ,
- Importance of functional measures in predicting mortality among older hospitalized patients. JAMA. 1998;279(15):1187–1193. , , , , ,
- Understanding preventable hospital readmissions: masqueraders, markers, and true causal factors. J Hosp Med. 2011;6(2):51–53. ,
- A multicomponent intervention to prevent delirium in hospitalized older patients. N Engl J Med. 1999;340(9):669–676. , , , et al.
- Effects of a multicomponent intervention on functional outcomes and process of care in hospitalized older patients: a randomized controlled trial of Acute Care for Elders (ACE) in a community hospital. J Am Geriatr Soc. 2000;48(12):1572–1581. , , , et al.
- American Geriatrics Society Health Care Systems Committee. Improving the quality of transitional care for persons with complex care needs. J Am Geriatr Soc. 2003;51(4):556–557. , ;
- Development and implementation of a proactive geriatrics consultation model in collaboration with hospitalists. J Am Geriatr Soc. 2009;57(11):2139–2145. , , ,
- Screening of the risk of functional decline performed by an inpatient geriatric consultation team in a general hospital [in French]. Revue medicale de Bruxelles. 2013;34(6):462–468. , , , et al.
- Systematic detection and multidisciplinary care of delirium in older medical inpatients: a randomized trial. CMAJ. 2002;167(7):753–759. , , , et al.
- Potentially inappropriate prescribing for geriatric inpatients: an acute care of the elderly unit compared to a general medicine service. Consult Pharm. 2003;18(1):37–42, 47–39. , ,
- Evaluation of the Mobile Acute Care of the Elderly (MACE) service. JAMA Intern Med. 2013;173(11):990–996. , , ,
- Effects of an acute care for elders unit on costs and 30‐day readmissions. JAMA Intern Med. 2013;173(11):981–987. , , , , ,
- Impact and recognition of cognitive impairment among hospitalized elders. J Hosp Med. 2010;5(2):69–75. , , , et al.
- Enhancing care for hospitalized older adults with cognitive impairment: a randomized controlled trial. J Gen Intern Med. 2012;27(5):561–567. , , , et al.
- Short Portable Mental Status Questionnaire as a screening test for dementia and delirium among the elderly. J Am Geriatr Soc. 1987;35(5):412–416. , , ,
- The Regenstrief Medical Record System: a quarter century experience. Int J Med Inform. 1999;54(3):225–253. , , , et al.
- Factors determining the decision to institutionalize dementing individuals: a prospective study. Gerontologist. 1993;33(6):714–720. , , , , ,
- Clarifying confusion: the confusion assessment method. A new method for detection of delirium. Ann Intern Med. 1990;113(12):941–948. , , , , ,
- Resuscitation: how do we decide? A prospective study of physicians' preferences and the clinical course of hospitalized patients. JAMA. 1986;255(10):1316–1322. , , , , ,
- The APACHE III prognostic system. Risk prediction of hospital mortality for critically ill hospitalized adults. Chest. 1991;100(6):1619–1636. , , , et al.
- Interaction between cognitive impairment and discharge destination and its effect on rehospitalization. J Am Geriatr Soc. 2013;61(11):1958–1963. , , , et al.
- Propensity scores in intensive care and anaesthesiology literature: a systematic review. Intensive Care Med. 2010;36(12):1993–2003. , , , , ,
- Improving functional outcomes in older patients: lessons from an acute care for elders unit. Jt Comm J Qual Improv. 1998;24(2):63–76. , , , et al.
- Developing a stroke unit using the acute care for elders intervention and model of care. J Am Geriatr Soc. 2003;51(11):1660–1667. , , , et al.
- A medical unit for the acute care of the elderly. J Am Geriatr Soc. 1994;42(5):545–552. , , ,
- Impact of geriatric consultation teams on clinical outcome in acute hospitals: a systematic review and meta‐analysis. BMC Med. 2013;11:48. , , , ,
- Comprehensive geriatric assessment for older adults admitted to hospital: meta‐analysis of randomised controlled trials. BMJ. 2011;343:d6553. , , , ,
- Prevention of readmission in elderly patients with congestive heart failure: results of a prospective, randomized pilot study. J Gen Intern Med. 1993;8(11):585–590. , , , et al.
- Effect of a pharmacist intervention on clinically important medication errors after hospital discharge: a randomized trial. Ann Intern Med. 2012;157(1):1–10. , , , et al.
- House calls for seniors: building and sustaining a model of care for homebound seniors. J Am Geriatr Soc. 2009;57(6):1103–1109. , , , ,
- Geriatric care management for low‐income seniors: a randomized controlled trial. JAMA. 2007;298(22):2623–2633. , , , et al.
- Interventions to reduce 30‐day rehospitalization: a systematic review. Ann Intern Med. 2011;155(8):520–528. , , , ,
- The effects of a discharge planning and home follow‐up intervention on elders hospitalized with common medical and surgical cardiac conditions. J Cardiovasc Nurs. 1999;14(1):44–54. ,
- Readmission to hospital: a measure of quality or outcome? Qual Saf Health Care. 2004;13(1):10–11.
- Unintended consequences of steps to cut readmissions and reform payment may threaten care of vulnerable older adults. Health Aff (Millwood). 2012;31(7):1623–1632. , , , , ,
- Analyzing the effects of shared decision‐making, empathy and team interaction on patient satisfaction and treatment acceptance in medical rehabilitation using a structural equation modeling approach. Patient Educ Couns. 2013;91(2):167–175. , ,
- The Care Transitions Innovation (C‐TraIn) for Socioeconomically Disadvantaged Adults: results of a cluster randomized controlled trial. J Gen Intern Med. 2014;29(11):1460–1467. , , ,
- Risk factors for early hospital readmission in low‐income elderly adults. J Am Geriatr Soc. 2014;62(3):489–494. , , ,
- Understanding why patients of low socioeconomic status prefer hospitals over ambulatory care. Health Aff (Millwood). 2013;32(7):1196–1203. , , , , ,
- Relationship between hospital readmission and mortality rates for patients hospitalized with acute myocardial infarction, heart failure, or pneumonia. JAMA. 2013;309(6):587–593. , , , et al.
- Cognitive impairment. Can it predict the course of hospitalized patients? J Am Geriatr Soc. 1986;34(8):579–585. , , ,
- Importance of functional measures in predicting mortality among older hospitalized patients. JAMA. 1998;279(15):1187–1193. , , , , ,
- Understanding preventable hospital readmissions: masqueraders, markers, and true causal factors. J Hosp Med. 2011;6(2):51–53. ,
- A multicomponent intervention to prevent delirium in hospitalized older patients. N Engl J Med. 1999;340(9):669–676. , , , et al.
- Effects of a multicomponent intervention on functional outcomes and process of care in hospitalized older patients: a randomized controlled trial of Acute Care for Elders (ACE) in a community hospital. J Am Geriatr Soc. 2000;48(12):1572–1581. , , , et al.
- American Geriatrics Society Health Care Systems Committee. Improving the quality of transitional care for persons with complex care needs. J Am Geriatr Soc. 2003;51(4):556–557. , ;
- Development and implementation of a proactive geriatrics consultation model in collaboration with hospitalists. J Am Geriatr Soc. 2009;57(11):2139–2145. , , ,
- Screening of the risk of functional decline performed by an inpatient geriatric consultation team in a general hospital [in French]. Revue medicale de Bruxelles. 2013;34(6):462–468. , , , et al.
- Systematic detection and multidisciplinary care of delirium in older medical inpatients: a randomized trial. CMAJ. 2002;167(7):753–759. , , , et al.
- Potentially inappropriate prescribing for geriatric inpatients: an acute care of the elderly unit compared to a general medicine service. Consult Pharm. 2003;18(1):37–42, 47–39. , ,
- Evaluation of the Mobile Acute Care of the Elderly (MACE) service. JAMA Intern Med. 2013;173(11):990–996. , , ,
- Effects of an acute care for elders unit on costs and 30‐day readmissions. JAMA Intern Med. 2013;173(11):981–987. , , , , ,
- Impact and recognition of cognitive impairment among hospitalized elders. J Hosp Med. 2010;5(2):69–75. , , , et al.
- Enhancing care for hospitalized older adults with cognitive impairment: a randomized controlled trial. J Gen Intern Med. 2012;27(5):561–567. , , , et al.
- Short Portable Mental Status Questionnaire as a screening test for dementia and delirium among the elderly. J Am Geriatr Soc. 1987;35(5):412–416. , , ,
- The Regenstrief Medical Record System: a quarter century experience. Int J Med Inform. 1999;54(3):225–253. , , , et al.
- Factors determining the decision to institutionalize dementing individuals: a prospective study. Gerontologist. 1993;33(6):714–720. , , , , ,
- Clarifying confusion: the confusion assessment method. A new method for detection of delirium. Ann Intern Med. 1990;113(12):941–948. , , , , ,
- Resuscitation: how do we decide? A prospective study of physicians' preferences and the clinical course of hospitalized patients. JAMA. 1986;255(10):1316–1322. , , , , ,
- The APACHE III prognostic system. Risk prediction of hospital mortality for critically ill hospitalized adults. Chest. 1991;100(6):1619–1636. , , , et al.
- Interaction between cognitive impairment and discharge destination and its effect on rehospitalization. J Am Geriatr Soc. 2013;61(11):1958–1963. , , , et al.
- Propensity scores in intensive care and anaesthesiology literature: a systematic review. Intensive Care Med. 2010;36(12):1993–2003. , , , , ,
- Improving functional outcomes in older patients: lessons from an acute care for elders unit. Jt Comm J Qual Improv. 1998;24(2):63–76. , , , et al.
- Developing a stroke unit using the acute care for elders intervention and model of care. J Am Geriatr Soc. 2003;51(11):1660–1667. , , , et al.
- A medical unit for the acute care of the elderly. J Am Geriatr Soc. 1994;42(5):545–552. , , ,
- Impact of geriatric consultation teams on clinical outcome in acute hospitals: a systematic review and meta‐analysis. BMC Med. 2013;11:48. , , , ,
- Comprehensive geriatric assessment for older adults admitted to hospital: meta‐analysis of randomised controlled trials. BMJ. 2011;343:d6553. , , , ,
- Prevention of readmission in elderly patients with congestive heart failure: results of a prospective, randomized pilot study. J Gen Intern Med. 1993;8(11):585–590. , , , et al.
- Effect of a pharmacist intervention on clinically important medication errors after hospital discharge: a randomized trial. Ann Intern Med. 2012;157(1):1–10. , , , et al.
- House calls for seniors: building and sustaining a model of care for homebound seniors. J Am Geriatr Soc. 2009;57(6):1103–1109. , , , ,
- Geriatric care management for low‐income seniors: a randomized controlled trial. JAMA. 2007;298(22):2623–2633. , , , et al.
- Interventions to reduce 30‐day rehospitalization: a systematic review. Ann Intern Med. 2011;155(8):520–528. , , , ,
- The effects of a discharge planning and home follow‐up intervention on elders hospitalized with common medical and surgical cardiac conditions. J Cardiovasc Nurs. 1999;14(1):44–54. ,
- Readmission to hospital: a measure of quality or outcome? Qual Saf Health Care. 2004;13(1):10–11.
- Unintended consequences of steps to cut readmissions and reform payment may threaten care of vulnerable older adults. Health Aff (Millwood). 2012;31(7):1623–1632. , , , , ,
- Analyzing the effects of shared decision‐making, empathy and team interaction on patient satisfaction and treatment acceptance in medical rehabilitation using a structural equation modeling approach. Patient Educ Couns. 2013;91(2):167–175. , ,
- The Care Transitions Innovation (C‐TraIn) for Socioeconomically Disadvantaged Adults: results of a cluster randomized controlled trial. J Gen Intern Med. 2014;29(11):1460–1467. , , ,
- Risk factors for early hospital readmission in low‐income elderly adults. J Am Geriatr Soc. 2014;62(3):489–494. , , ,
- Understanding why patients of low socioeconomic status prefer hospitals over ambulatory care. Health Aff (Millwood). 2013;32(7):1196–1203. , , , , ,
© 2015 Society of Hospital Medicine