User login
Hospital Antipsychotic Use
Antipsychotic medications are frequently used off label for management of behavioral symptoms associated with delirium and/or dementia. Despite regulations designed to curb inappropriate prescribing of these medications in nursing homes, substantial levels of use and variation in use have been observed in this setting.[1] Although antipsychotic medications are also frequently used in the hospital, the scope and variation in use have not been adequately investigated. Given the lack of oversight for medication prescribing in the hospital setting and the frequency of delirium, occurring in 15% to 26% of hospitalized older adults,[2, 3, 4] off‐label use of antipsychotic medications and variation in use could be substantial.
Because variation in practice is known to increase in the setting of controversy or lack of clarity regarding appropriate management,[5] large degrees of variation can draw attention to priority areas for clinical effectiveness studies, and the need for guidelines, clinical decision support, or regulatory oversight. In the absence of clear guidelines for the use of antipsychotic medication in nonpsychiatric hospitalized patients, we hypothesized that significant variation in use would persist after controlling for patient characteristics. Using a large, nationally representative cohort of admissions to 300 hospitals from July 2009 to June 2010, we sought to investigate prescribing patterns and hospital variation in use of antipsychotic medications in nonpsychiatric admissions to US hospitals.
METHODS
Setting and Data Collection
We conducted a retrospective cohort study using data from 300 US, nonfederal, acute care facilities contributing to the database maintained by Premier (Premier Healthcare Solutions, Inc., Charlotte, NC). This nationally representative database, created to measure healthcare utilization and quality of care, is drawn from voluntarily participating hospitals and contains data on approximately 1 in every 4 discharges nationwide.[6] Participating hospitals are similar in geographic distribution and urban/rural status to hospitals nationwide, although large, nonteaching hospitals are slightly over‐represented. The study was approved by the institutional review board at Beth Israel Deaconess Medical Center.
Inclusion and Exclusion Criteria
We studied a cohort of all adult (18 years) nonpsychiatric admissions to participating hospitals from July 1, 2009 through June 30, 2010. We excluded patients admitted to a psychiatry service or with any discharge diagnosis of a psychotic disorder (defined by the Elixhauser comorbidity Psychoses: 295.00‐298.9, 299.10‐299.11), because we were interested in use of antipsychotics for conditions other than primary psychiatric disorders. We also excluded patients with a charge for labor and delivery owing to the nonrepresentativeness of this patient population for the general hospitalized patient. We excluded admissions with unknown gender, and admissions with a length of stay greater than 365 days, as these admissions are not representative of the typical admission to an acute care hospital. We also excluded hospitals contributing less than 100 admissions owing to lack of precision in corresponding hospital prescribing rates.
Antipsychotic Medication Utilization
In‐hospital antipsychotic use was ascertained from pharmacy charges, reflecting each medication dispensed during the hospitalization. We categorized antipsychotic medications as typical (haloperidol, loxapine, thioridazine, molindone, thiothixine, pimozide, fluphenazine, trifluoperazine, chlorpromazine, and perphenazine) and atypical (aripiprazole, asenapine, clozapine, iloperidone, lurasidone, olanzapine, paliperidone, quetiapine, risperidone, and ziprasidone) based on classification by the Food and Drug Administration.[7, 8] We excluded prochlorperazine (Compazine) from our typical antipsychotic definition, as this medication is almost exclusively used as an antiemetic.
In the absence of guidelines for use of antipsychotic agents in hospitalized patients, we used the Centers for Medicare and Medicaid Services (CMS) guidelines for long‐term care facilities to define measures of potentially excessive dosing in the hospital setting.[9] These guidelines define the daily dosage levels of antipsychotics above which the medical necessity of the higher dose should be explained in the medical record. We defined any daily dosage above these specified levels as a potentially excessive daily dose.
Characteristics Associated With Use
We investigated the association between antipsychotic use and patient and hospital characteristics, selected based on clinical grounds. Patient characteristics included: (1) demographic variables such as age (<65, 6574, 75+ years), gender, self‐reported race, marital status, and primary insurance; (2) admission characteristic variables, including admitting department (surgical vs nonsurgical, defined by a surgical attending of record and presence of operating room charges), whether the patient spent any time in the intensive care unit (ICU), and whether they received mechanical ventilation; and (3) potential indications for use, including delirium (included delirium superimposed upon dementia), dementia (without delirium), and insomnia (see Supporting Information, Appendix, in the online version of this article for International Classification of Diseases, Ninth Revision, Clinical Modification [ICD‐9‐CM] codes). Hospital characteristics included number of beds, urban versus rural status, teaching status, and US Census region.
Statistical Analysis
We report the proportion of admissions with in‐hospital use of any antipsychotic, and the number of days of exposure, overall and stratified by typical and atypical.
We determined potentially excessive dosing by taking the sum of the doses for a specific antipsychotic charged on a given day and comparing it to the CMS guidelines for long‐term care settings described above. We report the percentage of exposed admissions with at least 1 day of potentially excessive dosing.
All multivariable models below were operationalized as generalized estimating equations with a Poisson error term, log link, robust variance estimator,[10] and an exchangeable correlation structure to account for repeated admissions of the same patient.
We investigated patient and hospital characteristics associated with use of any antipsychotic medication using a multivariable model that simultaneously included all patient and hospital characteristics in Table 1 as independent variables.
% of Cohort | Any Exposure, % | Typical Exposure, % | Atypical Exposure, % | |
---|---|---|---|---|
| ||||
Patient characteristics | ||||
Age group, y | ||||
<65 | 52.1 | 4.6 | 2.0 | 3.1 |
6574 | 18.5 | 5.2 | 2.7 | 3.1 |
75+ | 29.4 | 8.8 | 4.6 | 5.4 |
Gender | ||||
Male | 43.8 | 6.6 | 3.6 | 3.8 |
Female | 56.2 | 5.5 | 2.3 | 3.8 |
Race | ||||
White | 64.6 | 6.1 | 2.9 | 4.0 |
Black | 13.5 | 5.5 | 2.8 | 3.3 |
Hispanic | 5.0 | 4.9 | 2.2 | 3.2 |
Other | 19.9 | 6.1 | 3.1 | 3.7 |
Marital Status | ||||
Married | 42.5 | 4.6 | 2.4 | 2.7 |
Single | 46.7 | 7.2 | 3.2 | 4.7 |
Unknown/other | 10.8 | 6.4 | 3.1 | 4.1 |
Primary insurance | ||||
Private (commercial) | 28.8 | 3.0 | 1.5 | 1.8 |
Medicaid | 10.3 | 6.4 | 2.4 | 4.6 |
Medicare managed | 10.6 | 7.1 | 4.1 | 4.0 |
Medicare traditional | 40.9 | 8.0 | 3.7 | 5.3 |
Self‐pay or other | 9.4 | 4.3 | 2.5 | 2.2 |
Admitting department | ||||
Surgical | 60.6 | 5.8 | 3.1 | 3.4 |
Nonsurgical | 39.4 | 6.2 | 2.4 | 4.4 |
Any ICU stay | 16.6 | 10.4 | 7.2 | 4.9 |
Mechanical ventilation | 4.7 | 17.4 | 12.9 | 7.9 |
Diagnoses | ||||
Delirium | 3.2 | 28.6 | 19.4 | 15.7 |
Dementia | 3.1 | 27.4 | 12.0 | 20.2 |
Insomnia | 1.3 | 10.2 | 3.9 | 7.5 |
Discharge disposition | ||||
Home | 77.9 | 3.8 | 1.6 | 2.5 |
SNF/Rehab | 15.5 | 13.7 | 6.8 | 9.0 |
Hospice | 1.7 | 16.0 | 10.3 | 8.1 |
Other | 4.9 | 11.6 | 7.6 | 5.7 |
Hospital characteristics, % | ||||
No. of beds | ||||
200 | 14.1 | 6.1 | 2.8 | 3.8 |
201300 | 18.6 | 6.1 | 2.9 | 3.9 |
301500 | 37.7 | 5.9 | 2.9 | 3.7 |
500+ | 29.7 | 5.9 | 2.8 | 3.8 |
Population served | ||||
Urban | 89.4 | 6.0 | 2.9 | 3.8 |
Rural | 10.6 | 5.8 | 2.4 | 3.9 |
Teaching status | ||||
Teaching | 39.2 | 5.8 | 2.9 | 3.7 |
Nonteaching | 60.8 | 6.0 | 2.8 | 3.9 |
US Census region | ||||
West | 16.9 | 5.9 | 3.2 | 3.5 |
Northeast | 20.1 | 6.1 | 2.9 | 3.9 |
Midwest | 21.9 | 5.7 | 2.5 | 3.8 |
South | 41.0 | 6.1 | 2.9 | 3.9 |
To determine hospital variation in antipsychotic use, we first determined the proportion of admissions at each hospital with at least 1 charge for antipsychotic medication. We then divided hospitals into quintiles based on their facility‐level antipsychotic prescribing rates and assigned all admissions to their corresponding hospital quintile. We then used a multivariable model to measure the adjusted association between prescribing quintile and patient‐level receipt of antipsychotic medication, controlling for all patient characteristics listed in Table 1 (except discharge disposition), and comorbidities using the Healthcare Cost and Utilization Project Comorbidity Software version 3.7 (Agency for Healthcare Research and Quality, Rockville, MD).[11] We used the lowest prescribing quintile as the reference group. We also report in the Supporting Information, Appendix, in the online version of this article, the distribution of prescribing rates for the hospitals in our cohort before and after adjustment for patient characteristics. For both approaches, we conducted stratified analyses in admissions with delirium and dementia.
All analyses were carried out using SAS software (SAS Institute Inc., Cary, NC).
RESULTS
Admission Characteristics
There were 3,190,934 admissions aged 18 years and over to 300 hospitals from July 1, 2009 to June 30, 2010. After excluding admissions with unknown gender (n = 17), length of stay greater than 365 days (n = 25), charges for labor and delivery (n = 323,111) or a psychiatric attending of record or psychiatric comorbidity (n = 172,669), and admissions to hospitals with fewer than 100 admissions (n = 31), our cohort included 2,695,081 admissions. The median age was 63 years (25th, 75th percentile 48, 77 years), and 1,514,986 (56%) were women. Table 1 shows the overall admission characteristics of the cohort and the percent exposed to antipsychotics among each patient and hospital characteristic.
Antipsychotic Use
There were 160,773 (6%) admissions with antipsychotic exposure. Among exposed admissions, 102,148 (64%) received atypical and 76,979 (48%) received typical antipsychotics, with 18,354 (11%) exposed to both. The median (25th, 75th percentile) length of stay among exposed was 5 days (3, 9 days), and the median (25th, 75th percentile) number of days of exposure was 3 (1, 5 days) overall, 3 days (2, 6 days) for atypical and 2 days (1, 3 days) for typical exposure.
Among admissions aged 65 to 74 years, 25,855 (5%) were exposed. Among admissions aged 75 years or older, 69,792 (9%) were exposed. Among admissions with delirium, exposure occurred in 24,787 (29%), with 13,640 (55%) receiving atypical, 16,828 (68%) receiving typical, and 5681 (23%) exposed to both. Among admissions with dementia, exposure occurred in 23,179 (27%), with 17,068 (74%) receiving atypical, 10,108 (44%) receiving typical, and 3997 (17%) exposed to both.
Use of Specific Drugs and Potentially Excessive Dosing
Table 2 demonstrates the most commonly used antipsychotic medications and the rates of potentially excessive dosing. Quetiapine and olanzapine were the most commonly used atypical antipsychotics, and haloperidol represented the majority of typical antipsychotic use. Among admissions with antipsychotic exposure, 47% received at least 1 potentially excessive daily dose, 18% of those with atypical exposure and 79% of those with typical exposure. Among admissions aged 65 years and up (n = 1,291,375), the prevalence of potentially excessive dosing was almost identical; 46% received at least 1 daily dose in excess of the recommended daily dose, 11% of those with atypical exposure and 79% of those with typical exposure.
Agent |
Overall Prevalence,N = 2,695,081 |
% of Exposed With Potentially Excessive Dosing* | ||
---|---|---|---|---|
Within 100% of Recommended DD* | 101% to 150% of Recommended DD* | >150% of Recommended DD* | ||
| ||||
Any antipsychotic | 6.0 | 52.9 | 20.2 | 26.9 |
Atypical | 3.8 | 82.0 | 5.4 | 12.6 |
Quetiapine (200) | 1.8 | 81.7 | 5.7 | 12.6 |
Olanzapine (10) | 0.6 | 73.7 | 7.3 | 19.0 |
Risperidone (2) | 0.9 | 79.2 | 6.8 | 14.0 |
Other | 0.7 | 98.3 | 0.1 | 1.6 |
Typical | 2.9 | 21.1 | 37.0 | 41.9 |
Haloperidol (4) | 2.5 | 13.2 | 41.3 | 45.5 |
Chlorpromazine (75) | 0.3 | 76.0 | 9.8 | 14.2 |
Other | 0.4 | 89.1 | 2.9 | 8.0 |
Characteristics Associated With Antipsychotic Use
Among the patient and hospital characteristics included in our analysis, the 5 characteristics most strongly associated with antipsychotic exposure after adjustment were (Table 3): delirium (relative risk [RR]: 2.93, 95% confidence interval [CI]: 2.88‐2.98); dementia (RR: 2.78, 95% CI: 2.72‐2.83); insurance status, with higher risk among patients with traditional Medicare (RR: 2.09, 95% CI: 2.04‐2.13), Medicare managed (RR: 1.98, 95% CI: 1.93‐2.03), Medicaid (RR: 1.84, 95% CI: 1.80‐1.88), and self‐pay/other (RR: 1.26, 95% CI: 1.23‐1.29) compared to private (commercial) insurance; use of mechanical ventilation (RR: 1.84, 95% CI: 1.81‐1.87); and any ICU stay (RR: 1.53, 95% CI: 1.51‐1.55).
Unadjusted RR of Receiving Any Antipsychotic [95% CI] | Adjusted RR of Receiving Any Antipsychotic [95% CI]* | |
---|---|---|
| ||
Age group, y, % | ||
<65 | Reference | Reference |
6574 | 1.12 [1.10,1.14] | 0.74 [0.72, 0.75] |
75+ | 1.90 [1.88,1.92] | 1.03 [1.01, 1.05] |
Gender | ||
Female | Reference | Reference |
Male | 1.19 [1.18,1.20] | 1.27 [1.26, 1.28] |
Race | ||
White | Reference | Reference |
Black | 0.91 [0.90,0.92] | 0.85 [0.83, 0.86] |
Hispanic | 0.80 [0.78,0.82] | 0.79 [0.76, 0.81] |
Other | 0.99 [0.98,1.00] | 0.96 [0.95, 0.98] |
Marital status | ||
Married | Reference | Reference |
Single | 1.57 [1.55,1.59] | 1.43 [1.42, 1.45] |
Unknown/other | 1.41 [1.39,1.43] | 1.27 [1.24, 1.29] |
Primary insurance | ||
Private (commercial) | Reference | Reference |
Medicaid | 2.13 [2.09,2.17] | 1.84 [1.80, 1.88] |
Medicare managed | 2.35 [2.31,2.39] | 1.98 [1.93, 2.03] |
Medicare traditional | 2.65 [2.61,2.69] | 2.09 [2.04, 2.13] |
Self‐pay or other | 1.41 [1.38,1.44] | 1.26 [1.23, 1.29] |
Admitting department | ||
Surgical | Reference | Reference |
Nonsurgical | 1.06 [1.05,1.07] | 1.05 [1.03, 1.06] |
Any ICU stay | 2.05 [2.03,2.07] | 1.53 [1.51, 1.55] |
Mechanical ventilation | 3.22 [3.18,3.26] | 1.84 [1.81, 1.87] |
Diagnoses | ||
Delirium | 5.48 [5.42, 5.45] | 2.93 [2.88, 2.98] |
Dementia | 5.21 [5.15,5.27] | 2.78 [2.72, 2.83] |
Insomnia | 1.72 [1.67,1.78] | 1.51 [1.45, 1.57] |
No. of beds | ||
200 | Reference | Reference |
201300 | 1.01 [0.99,1.03] | 0.96 [0.94, 0.98] |
301500 | 0.98 [0.97,1.00] | 0.93 [0.91, 0.95] |
500+ | 0.97 [0.96,0.98] | 0.91 [0.90, 0.93] |
Population served | ||
Urban | Reference | Reference |
Rural | 0.96 [0.95,0.98] | 0.91 [0.89, 0.93] |
Teaching status | ||
Teaching | Reference | Reference |
Nonteaching | 1.03 [1.02,1.04] | 0.98 [0.97, 1.00] |
US Census region | ||
West | Reference | Reference |
Northeast | 1.03 [1.01,1.05] | 1.04 [1.02, 1.06] |
Midwest | 0.95 [0.94,0.97] | 0.93 [0.91, 0.94] |
South | 1.02 [1.01,1.03] | 1.07 [1.05, 1.09] |
Hospital Variation in Antipsychotic Use
Figure 1 demonstrates the antipsychotic prescribing rate at each hospital in our cohort, and the corresponding quintiles. Patients admitted to hospitals in the highest prescribing quintile were more than twice as likely to be exposed to antipsychotics compared to patients admitted to hospitals in the lowest prescribing quintile, even after adjustment for patient characteristics and comorbidities (Table 4). This relationship was similar across subgroups of admissions with delirium and dementia (see Supporting Information, Appendix, in the online version of this article for the distribution of hospital antipsychotic prescribing rates before and after adjustment for patient characteristics).
Admissions, No. (% of Total) | Unadjusted RR of Exposure [95% CI] | Adjusted RR of exposure [95% CI]* | |
---|---|---|---|
| |||
Overall | |||
Q1 | 431,017 (16%) | Reference | Reference |
Q2 | 630,486 (23%) | 1.67 [1.63, 1.71] | 1.59 [1.55, 1.62] |
Q3 | 548,337 (20%) | 1.93 [1.88, 1.97] | 1.84 [1.80, 1.88] |
Q4 | 639,027 (24%) | 2.16 [2.12, 2.21] | 2.07 [2.03, 2.12] |
Q5 | 446,214 (17%) | 2.83 [2.77, 2.89] | 2.56 [2.50, 2.61] |
Delirium | |||
Q1 | 12,878 (15%) | Reference | Reference |
Q2 | 20,588 (24%) | 1.58 [1.51, 1.65] | 1.58 [1.51, 1.65] |
Q3 | 17,402 (20%) | 1.71 [1.64, 1.80] | 1.73 [1.65, 1.82] |
Q4 | 20,943 (24%) | 2.01 [1.92, 2.10] | 1.99 [1.91, 2.08] |
Q5 | 14,883 (17%) | 2.15 [2.05, 2.25] | 2.16 [2.07, 2.26] |
Dementia | |||
Q1 | 28,290 (15%) | Reference | Reference |
Q2 | 42,018 (22%) | 1.43 [1.36, 1.50] | 1.40 [1.34, 1.47] |
Q3 | 38,593 (21%) | 1.61 [1.53, 1.69] | 1.59 [1.51, 1.66] |
Q4 | 44,638 (24%) | 1.69 [1.62, 1.77] | 1.69 [1.61, 1.77] |
Q5 | 34,442 (18%) | 1.92 [1.83, 2.01] | 1.90 [1.81, 1.99] |
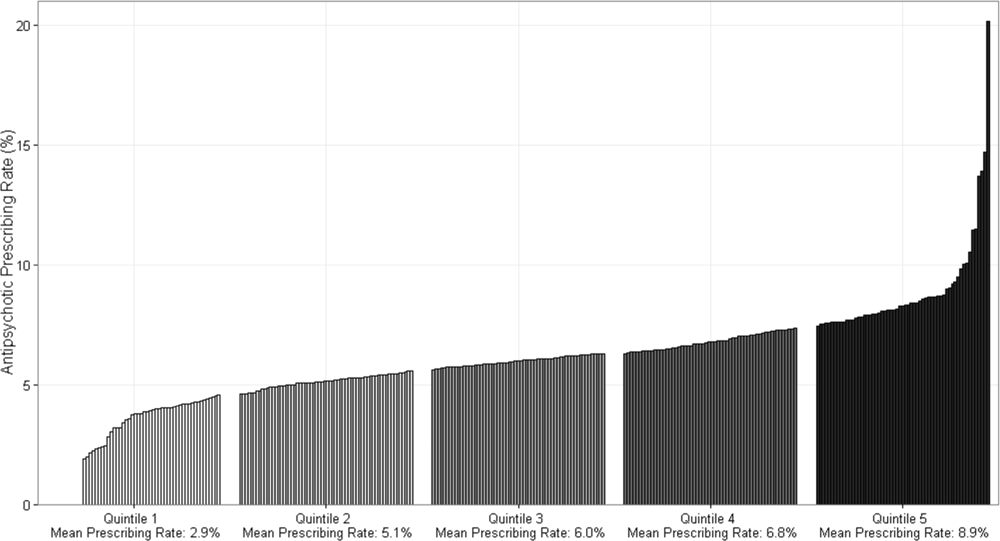
DISCUSSION
In this cohort of nonpsychiatric admissions to 300 US hospitals, antipsychotic medications were used in 6% of admissions, with atypical antipsychotics representing the majority of use. Potentially excessive daily doses based on CMS recommendations for long‐term care facilities occurred in almost half of admissions with any antipsychotic exposure, and in 87% of admissions with haloperidol exposure specifically. We found variation in hospital use of antipsychotics that was not fully accounted for by measured patient characteristics, and which persisted among subgroups of admissions with delirium and/or dementia. Although unmeasured patient characteristics or different billing practices between hospitals are potential explanations, our findings also raise the possibility of different hospital antipsychotic prescribing cultures. These findings provide new information regarding the scope of prescribing in US hospitals, and draw attention to the need for additional studies to better define what constitutes appropriate use of antipsychotics in the hospital setting.
A recent single‐center study at a large academic medical center found an overall antipsychotic exposure rate of 9% of nonpsychiatric admissions.[12] Our finding that 6% of admissions in this multicenter cohort were exposed to antipsychotics is slightly lower, but similar to the previous estimate. Assuming 37 million discharges from US hospitals each year,[13] our study suggests that more than 2 million hospitalized patients receive antipsychotics annually. With around 1.4 million residents in nursing homes on any given day,[14] and an exposure rate of 25% to 30% in that setting,[15, 16, 17] our study suggests that the number of patients exposed in the hospital setting is greater than the number exposed in the nursing home setting, the site of care for which prescribing regulations have been focused thus far.
Because our dataset does not contain preadmission medications, we were unable to specifically investigate new initiation. In the prior single‐center study, approximately 55% of overall use in the hospital setting was new initiation,[12] which would suggest that antipsychotics are newly initiated in around 1 million admissions each year in the hospital. Although we are unable to determine reason for use in our analysis, delirium was a strong predictor of antipsychotic use in our multivariable model, and prior studies have demonstrated delirium to be the most common reason for antipsychotic initiation in hospitalized patients,[12, 18] an indication for which efficacy/effectiveness data are lacking. A recent systematic review of antipsychotics for the treatment of delirium in older adults concluded that because of severe methodological limitations, the small number of existing studies on this topic do not support the use of antipsychotics in the treatment of delirium in older hospitalized adults.[19] Our results further highlight the need for randomized placebo‐controlled trials of antipsychotics in treatment of delirium.
We found variation in antipsychotic use between hospitals that was not fully explained by patient characteristics. Insufficient data to inform clinical decisions surrounding management of agitated delirium/dementia and lack of clear criteria by which to judge appropriateness of antipsychotic use may contribute to this variation. Some variation may relate to resource allocation at different hospitals, and the feasibility of implementing nonpharmacologic management options across settings. Our results collectively highlight the need for studies evaluating the efficacy/effectiveness of antipsychotics in the treatment of delirium and drivers of physician decision‐making in this realm, as well as the need for greater hospital investment in nonpharmacologic delirium‐prevention programs, which have been shown to be effective in prevention of delirium in hospitalized patients.[20]
We observed high levels of potentially excessive daily dosing using cutoffs applied in the long‐term care setting. The majority of the potentially excessive doses were in the setting of typical antipsychotic use, and haloperidol specifically, where doses exceeded 4 mg on at least 1 day in 87% of exposed admissions. Of note, the threshold for haloperidol dosage above which justification is required was decreased from 4 to 2 mg per day in the 2015 update to the CMS guidelines.[21] For the present analysis, we used the guidelines that were contemporaneous to our cohort; we are unable to determine current rates of potentially inappropriate dosages in the present analysis, but given the high prevalence in 2009 to 2010, and the lowering of the dosage threshold since then, it is unlikely that any decrease in use would be enough to substantially reduce the estimate. Whether these high dosages are actually inappropriate in the hospital setting is not established, and we were not able to review medical records to determine whether justification for use of such doses was documented.[22, 23] It is possible that hospitalized patients with altered pharmacodynamics and greater severity of illness could require larger doses of these medications; however, this is an area in need of further investigation, and current critical care guidelines note the lack of sufficient data upon which to justify use of haloperidol in the prevention or treatment of delirium in ICU patients.[24, 25]
The dosages in use are concerning given that the risk of extrapyramidal side effects increases with increasing dose, and prior studies have demonstrated an association between increased dose of antipsychotics and increased risk of other adverse events, including hip fracture and sudden cardiac death.[22, 23] Further, despite these known risks, studies have demonstrated failure to follow recommendations to mitigate risk,[26] such as electrocardiogram monitoring in individuals receiving intravenous haloperidol.[27] Our results suggest that physicians are similarly not following recommendations to use lower doses of haloperidol when treating older patients, given the almost identical incidence of potentially excessive dosing among admissions of patients aged 65 years and older in our cohort.[25] Clinical decision support prompts have been effective at increasing appropriate use of antipsychotic medications in several single‐center analyses,[28, 29, 30] and widespread implementation of such support with a focus on haloperidol dosing should be considered on the basis of our results.
The patient characteristics associated with antipsychotic use in this large, nationally representative analysis are consistent with those identified in prior single‐center analyses.[12, 18] Both prior analyses identified delirium as the most common reason for antipsychotic use, and dementia, intensive care unit stay, and mechanical ventilation were also previously identified as strong predictors of use that we believe hold face validity for the practicing hospitalist. On the other hand, some of the factors associated with antipsychotic use in our model cannot be readily explained, such as insurance status and race, and may be serving as proxies for other variables not included in our analysis. That nonwhite patients are less likely than white patients to receive antipsychotic medications in the hospital has been previously demonstrated,[12] and further investigation to understand this disparity is warranted.
Our study has several additional limitations. First, because our study is observational, the possibility of residual confounding exists, and we cannot rule out that there are other patient factors driving the hospital variation in antipsychotic use that we observed. Second, because guidelines do not exist for antipsychotic dosing in hospitalized patients, we could only comment on potentially excessive dosing, extrapolating from guidelines in the long‐term care setting. Whether such doses are actually excessive in hospitalized patients is not defined. Third, although Premier performs quality checks on charge and ICD‐9‐CM coding data submitted by participating hospitals, the validity of administrative data is uncertain. For example, the use of administrative data to identify delirium diagnoses is likely to have resulted in underestimation of delirium incidence among our different exposure groups. Delirium is likely to be coded more often in the setting of more severe or hyperactive cases, when antipsychotics are more likely to be utilized. This could result in an overestimation of the association between delirium and antipsychotic use. Additionally, differences in coding practices between hospitals for any of the variables in our models could explain some of the variation in antipsychotic prescribing that we observed. Finally, because we were unable to differentiate between new initiation and continuation of a preadmission antipsychotic, some of the variation that we observed is likely to reflect differences in outpatient antipsychotic prescribing practices.
In conclusion, in this large cohort of nonpsychiatric admissions to 300 US hospitals, we found that antipsychotic medication exposure was common, often at high daily doses. Delirium and dementia were the strongest predictors of use among the patient and hospital characteristics examined. The variation in antipsychotic prescribing that we observed was not fully accounted for by measured patient characteristics, and raises the possibility of differing hospital prescribing cultures. Our results draw attention to the need for additional research to better define what constitutes appropriate use of these potentially harmful medications in the hospital setting.
Disclosures: Dr. Herzig had full access to all of the data in the study and takes responsibility for the integrity of the data and the accuracy of the data analysis. Study concept and design: Drs. Herzig, Rothberg, Gurwitz, and Marcantonio. Acquisition of data: Dr. Herzig. Analysis of data: Mr. Guess. Interpretation of data: Drs. Herzig, Rothberg, Gurwitz, Marcantonio, and Mr. Guess. Drafting of the manuscript: Dr. Herzig. Critical revision of the manuscript for important intellectual content: Drs. Rothberg, Gurwitz, Marcantonio, and Mr. Guess. Dr. Herzig was funded by grant number K23AG042459 from the National Institute on Aging. Dr. Marcantonio was funded by grant number K24AG035075 from the National Institute on Aging. The funding organizations had no involvement in any aspect of the study, including design and conduct of the study; collection, management, analysis, and interpretation of the data; and preparation, review, or approval of the manuscript. The authors report no conflicts of interest.
- Variation in nursing home antipsychotic prescribing rates. Arch Intern Med. 2007;167(7):676–683. , , , et al.
- Precipitating factors for delirium in hospitalized elderly persons. Predictive model and interrelationship with baseline vulnerability. JAMA. 1996;275(11):852–857. , .
- A predictive model for delirium in hospitalized elderly medical patients based on admission characteristics. Ann Intern Med. 1993;119(6):474–481. , , , , .
- Delirium in hospitalized older persons: outcomes and predictors. J Am Geriatr Soc. 1994;42(8):809–815. , , , , , .
- Small‐area variations in the use of common surgical procedures: an international comparison of New England, England, and Norway. N Engl J Med. 1982;307(21):1310–1314. , , , .
- Premier Research Services. Available at: https://www.premierinc.com/transforming‐healthcare/healthcare‐performance‐improvement/premier‐research‐services. Accessed March 15, 2016.
- U.S. Food and Drug Administration. Atypical antipsychotic drugs information. Available at: http://www.fda.gov/drugs/drugsafety/postmarketdrugsafetyinformationforpatientsandproviders/ucm094303.htm. Accessed November 1, 2015.
- U.S. Food and Drug Administration. Information on conventional antipsychotics. Available at: http://www.fda.gov/drugs/drugsafety/postmarketdrugsafetyinformationforpatientsandproviders/ucm107211. htm. Accessed November 1, 2015.
- Centers for Medicare and Medicaid Services. State Operations Manual. Appendix PP: guidance to surveyors for long‐term care facilities. Available at: https://www.cms.gov/Medicare/Provider‐Enrollment‐and‐Certification/GuidanceforLawsAndRegulations/Downloads/som107 ap_pp_guidelines_ltcf.pdf. Revised October 14, 2005. Accessed March 15, 2016.
- A modified Poisson regression approach to prospective studies with binary data. Am J Epidemiol. 2004;159(7):702–706. .
- Healthcare Cost and Utilization Project. Comorbidity software, version 3.7. Agency for Healthcare Research and Quality, Rockville, MD. Available at: http://www.hcup‐us.ahrq.gov/toolssoftware/comorbidity/comorbidity.jsp. Accessed March 15, 2016.
- Antipsychotic use in hospitalized adults: rates, indications, and predictors. J Am Geriatr Soc. 2016;64(2):299–305. , , , et al.
- Overview of hospital stays in the United States, 2012. HCUP Statistical Brief #180. Agency for Healthcare Research and Quality, Rockville, MD. Available at: http://www.hcup‐us.ahrq.gov/reports/statbriefs/sb180‐Hospitalizations‐United‐States‐2012.pdf. Published October 2014. Accessed June 29, 2015. , .
- Long‐term care services in the United States: 2013 overview. Vital Health Stat 3. 2013;(37):1–107. Available at: http://www.cdc.gov/nchs/data/nsltcp/long_term_care_services_2013.pdf. Accessed March 16, 2016. , , , .
- The quality of antipsychotic drug prescribing in nursing homes. Arch Intern Med. 2005;165(11):1280–1285. , , , et al.
- Unexplained variation across US nursing homes in antipsychotic prescribing rates. Arch Intern Med. 2010;170(1):89–95. , , , , , .
- Broadened use of atypical antipsychotics: safety, effectiveness, and policy challenges. Health Aff (Millwood). 2009;28(5):w770–w781. , , , , .
- From hospital to community: use of antipsychotics in hospitalized elders. J Hosp Med. 2014;9(12):802–804. , , , , , .
- Antipsychotics in the treatment of delirium in older hospitalized adults: a systematic review. J Am Geriatr Soc. 2011;59(suppl 2):S269–S276. , , .
- Effectiveness of multicomponent nonpharmacological delirium interventions: a meta‐analysis. JAMA Intern Med. 2015;175(4):512–520. , , , et al.
- Centers for Medicare and Medicaid Services. State operations manual, appendix PP: guidance to surveyors for long‐term care facilities. Available at: https://www.cms.gov/Regulations‐and‐Guidance/Guidance/Manuals/downloads/som107ap_pp_guidelines_ltcf.pdf. Revised October 9, 2015. Accessed February 22, 2016.
- Psychotropic drug use and the risk of hip fracture. N Engl J Med. 1987;316(7):363–369. , , , , .
- Atypical antipsychotic drugs and the risk of sudden cardiac death. N Engl J Med. 2009;360(3):225–235. , , , , .
- Clinical practice guidelines for the management of pain, agitation, and delirium in adult patients in the intensive care unit. Crit Care Med. 2013;41(1):263–306. , , , et al.
- Haloperidol overdosing in the treatment of agitated hospitalized older people with delirium: a retrospective chart review from a community teaching hospital. Drugs Aging. 2013;30(8):639–644. , , , , .
- Unsafe use of intravenous haloperidol: evaluation of recommendation‐concordant care in hospitalized elderly adults. J Am Geriatr Soc. 2013;61(1):160–161. , , , .
- U.S. Food and Drug Administration. HALDOL brand of haloperidol injection. Available at: http://www.accessdata.fda.gov/drugsatfda_docs/label/2008/015923s082,018701s057lbl.pdf. Accessed February 23, 2016.
- Preventing potentially inappropriate medication use in hospitalized older patients with a computerized provider order entry warning system. Arch Intern Med. 2010;170(15):1331–1336. , , , .
- A standardized, bundled approach to providing geriatric‐focused acute care. J Am Geriatr Soc. 2014;62(5):936–942. , , , et al.
- Don't fuel the fire: decreasing intravenous haloperidol use in high risk patients via a customized electronic alert. J Am Med Inform Assoc. 2014;21(6):1109–1112. , , , .
Antipsychotic medications are frequently used off label for management of behavioral symptoms associated with delirium and/or dementia. Despite regulations designed to curb inappropriate prescribing of these medications in nursing homes, substantial levels of use and variation in use have been observed in this setting.[1] Although antipsychotic medications are also frequently used in the hospital, the scope and variation in use have not been adequately investigated. Given the lack of oversight for medication prescribing in the hospital setting and the frequency of delirium, occurring in 15% to 26% of hospitalized older adults,[2, 3, 4] off‐label use of antipsychotic medications and variation in use could be substantial.
Because variation in practice is known to increase in the setting of controversy or lack of clarity regarding appropriate management,[5] large degrees of variation can draw attention to priority areas for clinical effectiveness studies, and the need for guidelines, clinical decision support, or regulatory oversight. In the absence of clear guidelines for the use of antipsychotic medication in nonpsychiatric hospitalized patients, we hypothesized that significant variation in use would persist after controlling for patient characteristics. Using a large, nationally representative cohort of admissions to 300 hospitals from July 2009 to June 2010, we sought to investigate prescribing patterns and hospital variation in use of antipsychotic medications in nonpsychiatric admissions to US hospitals.
METHODS
Setting and Data Collection
We conducted a retrospective cohort study using data from 300 US, nonfederal, acute care facilities contributing to the database maintained by Premier (Premier Healthcare Solutions, Inc., Charlotte, NC). This nationally representative database, created to measure healthcare utilization and quality of care, is drawn from voluntarily participating hospitals and contains data on approximately 1 in every 4 discharges nationwide.[6] Participating hospitals are similar in geographic distribution and urban/rural status to hospitals nationwide, although large, nonteaching hospitals are slightly over‐represented. The study was approved by the institutional review board at Beth Israel Deaconess Medical Center.
Inclusion and Exclusion Criteria
We studied a cohort of all adult (18 years) nonpsychiatric admissions to participating hospitals from July 1, 2009 through June 30, 2010. We excluded patients admitted to a psychiatry service or with any discharge diagnosis of a psychotic disorder (defined by the Elixhauser comorbidity Psychoses: 295.00‐298.9, 299.10‐299.11), because we were interested in use of antipsychotics for conditions other than primary psychiatric disorders. We also excluded patients with a charge for labor and delivery owing to the nonrepresentativeness of this patient population for the general hospitalized patient. We excluded admissions with unknown gender, and admissions with a length of stay greater than 365 days, as these admissions are not representative of the typical admission to an acute care hospital. We also excluded hospitals contributing less than 100 admissions owing to lack of precision in corresponding hospital prescribing rates.
Antipsychotic Medication Utilization
In‐hospital antipsychotic use was ascertained from pharmacy charges, reflecting each medication dispensed during the hospitalization. We categorized antipsychotic medications as typical (haloperidol, loxapine, thioridazine, molindone, thiothixine, pimozide, fluphenazine, trifluoperazine, chlorpromazine, and perphenazine) and atypical (aripiprazole, asenapine, clozapine, iloperidone, lurasidone, olanzapine, paliperidone, quetiapine, risperidone, and ziprasidone) based on classification by the Food and Drug Administration.[7, 8] We excluded prochlorperazine (Compazine) from our typical antipsychotic definition, as this medication is almost exclusively used as an antiemetic.
In the absence of guidelines for use of antipsychotic agents in hospitalized patients, we used the Centers for Medicare and Medicaid Services (CMS) guidelines for long‐term care facilities to define measures of potentially excessive dosing in the hospital setting.[9] These guidelines define the daily dosage levels of antipsychotics above which the medical necessity of the higher dose should be explained in the medical record. We defined any daily dosage above these specified levels as a potentially excessive daily dose.
Characteristics Associated With Use
We investigated the association between antipsychotic use and patient and hospital characteristics, selected based on clinical grounds. Patient characteristics included: (1) demographic variables such as age (<65, 6574, 75+ years), gender, self‐reported race, marital status, and primary insurance; (2) admission characteristic variables, including admitting department (surgical vs nonsurgical, defined by a surgical attending of record and presence of operating room charges), whether the patient spent any time in the intensive care unit (ICU), and whether they received mechanical ventilation; and (3) potential indications for use, including delirium (included delirium superimposed upon dementia), dementia (without delirium), and insomnia (see Supporting Information, Appendix, in the online version of this article for International Classification of Diseases, Ninth Revision, Clinical Modification [ICD‐9‐CM] codes). Hospital characteristics included number of beds, urban versus rural status, teaching status, and US Census region.
Statistical Analysis
We report the proportion of admissions with in‐hospital use of any antipsychotic, and the number of days of exposure, overall and stratified by typical and atypical.
We determined potentially excessive dosing by taking the sum of the doses for a specific antipsychotic charged on a given day and comparing it to the CMS guidelines for long‐term care settings described above. We report the percentage of exposed admissions with at least 1 day of potentially excessive dosing.
All multivariable models below were operationalized as generalized estimating equations with a Poisson error term, log link, robust variance estimator,[10] and an exchangeable correlation structure to account for repeated admissions of the same patient.
We investigated patient and hospital characteristics associated with use of any antipsychotic medication using a multivariable model that simultaneously included all patient and hospital characteristics in Table 1 as independent variables.
% of Cohort | Any Exposure, % | Typical Exposure, % | Atypical Exposure, % | |
---|---|---|---|---|
| ||||
Patient characteristics | ||||
Age group, y | ||||
<65 | 52.1 | 4.6 | 2.0 | 3.1 |
6574 | 18.5 | 5.2 | 2.7 | 3.1 |
75+ | 29.4 | 8.8 | 4.6 | 5.4 |
Gender | ||||
Male | 43.8 | 6.6 | 3.6 | 3.8 |
Female | 56.2 | 5.5 | 2.3 | 3.8 |
Race | ||||
White | 64.6 | 6.1 | 2.9 | 4.0 |
Black | 13.5 | 5.5 | 2.8 | 3.3 |
Hispanic | 5.0 | 4.9 | 2.2 | 3.2 |
Other | 19.9 | 6.1 | 3.1 | 3.7 |
Marital Status | ||||
Married | 42.5 | 4.6 | 2.4 | 2.7 |
Single | 46.7 | 7.2 | 3.2 | 4.7 |
Unknown/other | 10.8 | 6.4 | 3.1 | 4.1 |
Primary insurance | ||||
Private (commercial) | 28.8 | 3.0 | 1.5 | 1.8 |
Medicaid | 10.3 | 6.4 | 2.4 | 4.6 |
Medicare managed | 10.6 | 7.1 | 4.1 | 4.0 |
Medicare traditional | 40.9 | 8.0 | 3.7 | 5.3 |
Self‐pay or other | 9.4 | 4.3 | 2.5 | 2.2 |
Admitting department | ||||
Surgical | 60.6 | 5.8 | 3.1 | 3.4 |
Nonsurgical | 39.4 | 6.2 | 2.4 | 4.4 |
Any ICU stay | 16.6 | 10.4 | 7.2 | 4.9 |
Mechanical ventilation | 4.7 | 17.4 | 12.9 | 7.9 |
Diagnoses | ||||
Delirium | 3.2 | 28.6 | 19.4 | 15.7 |
Dementia | 3.1 | 27.4 | 12.0 | 20.2 |
Insomnia | 1.3 | 10.2 | 3.9 | 7.5 |
Discharge disposition | ||||
Home | 77.9 | 3.8 | 1.6 | 2.5 |
SNF/Rehab | 15.5 | 13.7 | 6.8 | 9.0 |
Hospice | 1.7 | 16.0 | 10.3 | 8.1 |
Other | 4.9 | 11.6 | 7.6 | 5.7 |
Hospital characteristics, % | ||||
No. of beds | ||||
200 | 14.1 | 6.1 | 2.8 | 3.8 |
201300 | 18.6 | 6.1 | 2.9 | 3.9 |
301500 | 37.7 | 5.9 | 2.9 | 3.7 |
500+ | 29.7 | 5.9 | 2.8 | 3.8 |
Population served | ||||
Urban | 89.4 | 6.0 | 2.9 | 3.8 |
Rural | 10.6 | 5.8 | 2.4 | 3.9 |
Teaching status | ||||
Teaching | 39.2 | 5.8 | 2.9 | 3.7 |
Nonteaching | 60.8 | 6.0 | 2.8 | 3.9 |
US Census region | ||||
West | 16.9 | 5.9 | 3.2 | 3.5 |
Northeast | 20.1 | 6.1 | 2.9 | 3.9 |
Midwest | 21.9 | 5.7 | 2.5 | 3.8 |
South | 41.0 | 6.1 | 2.9 | 3.9 |
To determine hospital variation in antipsychotic use, we first determined the proportion of admissions at each hospital with at least 1 charge for antipsychotic medication. We then divided hospitals into quintiles based on their facility‐level antipsychotic prescribing rates and assigned all admissions to their corresponding hospital quintile. We then used a multivariable model to measure the adjusted association between prescribing quintile and patient‐level receipt of antipsychotic medication, controlling for all patient characteristics listed in Table 1 (except discharge disposition), and comorbidities using the Healthcare Cost and Utilization Project Comorbidity Software version 3.7 (Agency for Healthcare Research and Quality, Rockville, MD).[11] We used the lowest prescribing quintile as the reference group. We also report in the Supporting Information, Appendix, in the online version of this article, the distribution of prescribing rates for the hospitals in our cohort before and after adjustment for patient characteristics. For both approaches, we conducted stratified analyses in admissions with delirium and dementia.
All analyses were carried out using SAS software (SAS Institute Inc., Cary, NC).
RESULTS
Admission Characteristics
There were 3,190,934 admissions aged 18 years and over to 300 hospitals from July 1, 2009 to June 30, 2010. After excluding admissions with unknown gender (n = 17), length of stay greater than 365 days (n = 25), charges for labor and delivery (n = 323,111) or a psychiatric attending of record or psychiatric comorbidity (n = 172,669), and admissions to hospitals with fewer than 100 admissions (n = 31), our cohort included 2,695,081 admissions. The median age was 63 years (25th, 75th percentile 48, 77 years), and 1,514,986 (56%) were women. Table 1 shows the overall admission characteristics of the cohort and the percent exposed to antipsychotics among each patient and hospital characteristic.
Antipsychotic Use
There were 160,773 (6%) admissions with antipsychotic exposure. Among exposed admissions, 102,148 (64%) received atypical and 76,979 (48%) received typical antipsychotics, with 18,354 (11%) exposed to both. The median (25th, 75th percentile) length of stay among exposed was 5 days (3, 9 days), and the median (25th, 75th percentile) number of days of exposure was 3 (1, 5 days) overall, 3 days (2, 6 days) for atypical and 2 days (1, 3 days) for typical exposure.
Among admissions aged 65 to 74 years, 25,855 (5%) were exposed. Among admissions aged 75 years or older, 69,792 (9%) were exposed. Among admissions with delirium, exposure occurred in 24,787 (29%), with 13,640 (55%) receiving atypical, 16,828 (68%) receiving typical, and 5681 (23%) exposed to both. Among admissions with dementia, exposure occurred in 23,179 (27%), with 17,068 (74%) receiving atypical, 10,108 (44%) receiving typical, and 3997 (17%) exposed to both.
Use of Specific Drugs and Potentially Excessive Dosing
Table 2 demonstrates the most commonly used antipsychotic medications and the rates of potentially excessive dosing. Quetiapine and olanzapine were the most commonly used atypical antipsychotics, and haloperidol represented the majority of typical antipsychotic use. Among admissions with antipsychotic exposure, 47% received at least 1 potentially excessive daily dose, 18% of those with atypical exposure and 79% of those with typical exposure. Among admissions aged 65 years and up (n = 1,291,375), the prevalence of potentially excessive dosing was almost identical; 46% received at least 1 daily dose in excess of the recommended daily dose, 11% of those with atypical exposure and 79% of those with typical exposure.
Agent |
Overall Prevalence,N = 2,695,081 |
% of Exposed With Potentially Excessive Dosing* | ||
---|---|---|---|---|
Within 100% of Recommended DD* | 101% to 150% of Recommended DD* | >150% of Recommended DD* | ||
| ||||
Any antipsychotic | 6.0 | 52.9 | 20.2 | 26.9 |
Atypical | 3.8 | 82.0 | 5.4 | 12.6 |
Quetiapine (200) | 1.8 | 81.7 | 5.7 | 12.6 |
Olanzapine (10) | 0.6 | 73.7 | 7.3 | 19.0 |
Risperidone (2) | 0.9 | 79.2 | 6.8 | 14.0 |
Other | 0.7 | 98.3 | 0.1 | 1.6 |
Typical | 2.9 | 21.1 | 37.0 | 41.9 |
Haloperidol (4) | 2.5 | 13.2 | 41.3 | 45.5 |
Chlorpromazine (75) | 0.3 | 76.0 | 9.8 | 14.2 |
Other | 0.4 | 89.1 | 2.9 | 8.0 |
Characteristics Associated With Antipsychotic Use
Among the patient and hospital characteristics included in our analysis, the 5 characteristics most strongly associated with antipsychotic exposure after adjustment were (Table 3): delirium (relative risk [RR]: 2.93, 95% confidence interval [CI]: 2.88‐2.98); dementia (RR: 2.78, 95% CI: 2.72‐2.83); insurance status, with higher risk among patients with traditional Medicare (RR: 2.09, 95% CI: 2.04‐2.13), Medicare managed (RR: 1.98, 95% CI: 1.93‐2.03), Medicaid (RR: 1.84, 95% CI: 1.80‐1.88), and self‐pay/other (RR: 1.26, 95% CI: 1.23‐1.29) compared to private (commercial) insurance; use of mechanical ventilation (RR: 1.84, 95% CI: 1.81‐1.87); and any ICU stay (RR: 1.53, 95% CI: 1.51‐1.55).
Unadjusted RR of Receiving Any Antipsychotic [95% CI] | Adjusted RR of Receiving Any Antipsychotic [95% CI]* | |
---|---|---|
| ||
Age group, y, % | ||
<65 | Reference | Reference |
6574 | 1.12 [1.10,1.14] | 0.74 [0.72, 0.75] |
75+ | 1.90 [1.88,1.92] | 1.03 [1.01, 1.05] |
Gender | ||
Female | Reference | Reference |
Male | 1.19 [1.18,1.20] | 1.27 [1.26, 1.28] |
Race | ||
White | Reference | Reference |
Black | 0.91 [0.90,0.92] | 0.85 [0.83, 0.86] |
Hispanic | 0.80 [0.78,0.82] | 0.79 [0.76, 0.81] |
Other | 0.99 [0.98,1.00] | 0.96 [0.95, 0.98] |
Marital status | ||
Married | Reference | Reference |
Single | 1.57 [1.55,1.59] | 1.43 [1.42, 1.45] |
Unknown/other | 1.41 [1.39,1.43] | 1.27 [1.24, 1.29] |
Primary insurance | ||
Private (commercial) | Reference | Reference |
Medicaid | 2.13 [2.09,2.17] | 1.84 [1.80, 1.88] |
Medicare managed | 2.35 [2.31,2.39] | 1.98 [1.93, 2.03] |
Medicare traditional | 2.65 [2.61,2.69] | 2.09 [2.04, 2.13] |
Self‐pay or other | 1.41 [1.38,1.44] | 1.26 [1.23, 1.29] |
Admitting department | ||
Surgical | Reference | Reference |
Nonsurgical | 1.06 [1.05,1.07] | 1.05 [1.03, 1.06] |
Any ICU stay | 2.05 [2.03,2.07] | 1.53 [1.51, 1.55] |
Mechanical ventilation | 3.22 [3.18,3.26] | 1.84 [1.81, 1.87] |
Diagnoses | ||
Delirium | 5.48 [5.42, 5.45] | 2.93 [2.88, 2.98] |
Dementia | 5.21 [5.15,5.27] | 2.78 [2.72, 2.83] |
Insomnia | 1.72 [1.67,1.78] | 1.51 [1.45, 1.57] |
No. of beds | ||
200 | Reference | Reference |
201300 | 1.01 [0.99,1.03] | 0.96 [0.94, 0.98] |
301500 | 0.98 [0.97,1.00] | 0.93 [0.91, 0.95] |
500+ | 0.97 [0.96,0.98] | 0.91 [0.90, 0.93] |
Population served | ||
Urban | Reference | Reference |
Rural | 0.96 [0.95,0.98] | 0.91 [0.89, 0.93] |
Teaching status | ||
Teaching | Reference | Reference |
Nonteaching | 1.03 [1.02,1.04] | 0.98 [0.97, 1.00] |
US Census region | ||
West | Reference | Reference |
Northeast | 1.03 [1.01,1.05] | 1.04 [1.02, 1.06] |
Midwest | 0.95 [0.94,0.97] | 0.93 [0.91, 0.94] |
South | 1.02 [1.01,1.03] | 1.07 [1.05, 1.09] |
Hospital Variation in Antipsychotic Use
Figure 1 demonstrates the antipsychotic prescribing rate at each hospital in our cohort, and the corresponding quintiles. Patients admitted to hospitals in the highest prescribing quintile were more than twice as likely to be exposed to antipsychotics compared to patients admitted to hospitals in the lowest prescribing quintile, even after adjustment for patient characteristics and comorbidities (Table 4). This relationship was similar across subgroups of admissions with delirium and dementia (see Supporting Information, Appendix, in the online version of this article for the distribution of hospital antipsychotic prescribing rates before and after adjustment for patient characteristics).
Admissions, No. (% of Total) | Unadjusted RR of Exposure [95% CI] | Adjusted RR of exposure [95% CI]* | |
---|---|---|---|
| |||
Overall | |||
Q1 | 431,017 (16%) | Reference | Reference |
Q2 | 630,486 (23%) | 1.67 [1.63, 1.71] | 1.59 [1.55, 1.62] |
Q3 | 548,337 (20%) | 1.93 [1.88, 1.97] | 1.84 [1.80, 1.88] |
Q4 | 639,027 (24%) | 2.16 [2.12, 2.21] | 2.07 [2.03, 2.12] |
Q5 | 446,214 (17%) | 2.83 [2.77, 2.89] | 2.56 [2.50, 2.61] |
Delirium | |||
Q1 | 12,878 (15%) | Reference | Reference |
Q2 | 20,588 (24%) | 1.58 [1.51, 1.65] | 1.58 [1.51, 1.65] |
Q3 | 17,402 (20%) | 1.71 [1.64, 1.80] | 1.73 [1.65, 1.82] |
Q4 | 20,943 (24%) | 2.01 [1.92, 2.10] | 1.99 [1.91, 2.08] |
Q5 | 14,883 (17%) | 2.15 [2.05, 2.25] | 2.16 [2.07, 2.26] |
Dementia | |||
Q1 | 28,290 (15%) | Reference | Reference |
Q2 | 42,018 (22%) | 1.43 [1.36, 1.50] | 1.40 [1.34, 1.47] |
Q3 | 38,593 (21%) | 1.61 [1.53, 1.69] | 1.59 [1.51, 1.66] |
Q4 | 44,638 (24%) | 1.69 [1.62, 1.77] | 1.69 [1.61, 1.77] |
Q5 | 34,442 (18%) | 1.92 [1.83, 2.01] | 1.90 [1.81, 1.99] |
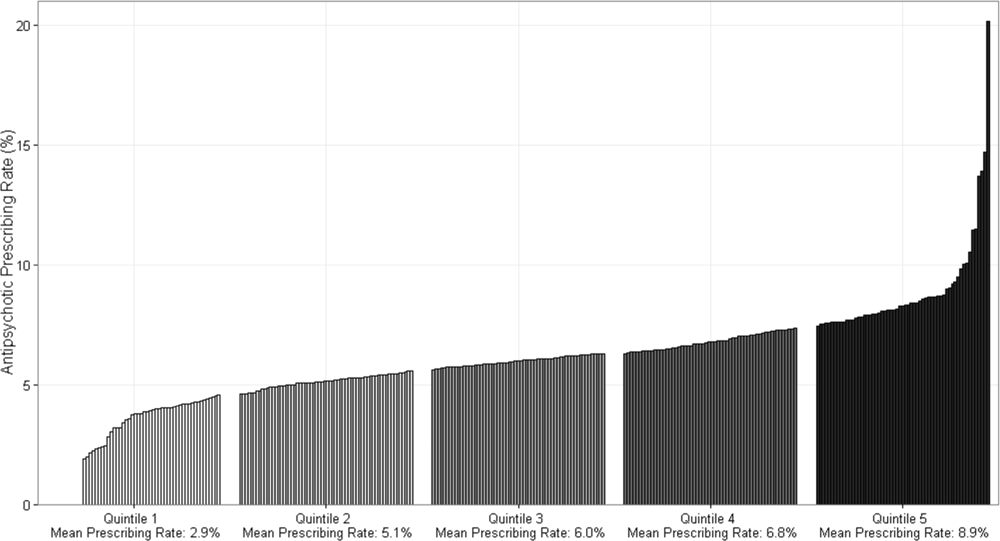
DISCUSSION
In this cohort of nonpsychiatric admissions to 300 US hospitals, antipsychotic medications were used in 6% of admissions, with atypical antipsychotics representing the majority of use. Potentially excessive daily doses based on CMS recommendations for long‐term care facilities occurred in almost half of admissions with any antipsychotic exposure, and in 87% of admissions with haloperidol exposure specifically. We found variation in hospital use of antipsychotics that was not fully accounted for by measured patient characteristics, and which persisted among subgroups of admissions with delirium and/or dementia. Although unmeasured patient characteristics or different billing practices between hospitals are potential explanations, our findings also raise the possibility of different hospital antipsychotic prescribing cultures. These findings provide new information regarding the scope of prescribing in US hospitals, and draw attention to the need for additional studies to better define what constitutes appropriate use of antipsychotics in the hospital setting.
A recent single‐center study at a large academic medical center found an overall antipsychotic exposure rate of 9% of nonpsychiatric admissions.[12] Our finding that 6% of admissions in this multicenter cohort were exposed to antipsychotics is slightly lower, but similar to the previous estimate. Assuming 37 million discharges from US hospitals each year,[13] our study suggests that more than 2 million hospitalized patients receive antipsychotics annually. With around 1.4 million residents in nursing homes on any given day,[14] and an exposure rate of 25% to 30% in that setting,[15, 16, 17] our study suggests that the number of patients exposed in the hospital setting is greater than the number exposed in the nursing home setting, the site of care for which prescribing regulations have been focused thus far.
Because our dataset does not contain preadmission medications, we were unable to specifically investigate new initiation. In the prior single‐center study, approximately 55% of overall use in the hospital setting was new initiation,[12] which would suggest that antipsychotics are newly initiated in around 1 million admissions each year in the hospital. Although we are unable to determine reason for use in our analysis, delirium was a strong predictor of antipsychotic use in our multivariable model, and prior studies have demonstrated delirium to be the most common reason for antipsychotic initiation in hospitalized patients,[12, 18] an indication for which efficacy/effectiveness data are lacking. A recent systematic review of antipsychotics for the treatment of delirium in older adults concluded that because of severe methodological limitations, the small number of existing studies on this topic do not support the use of antipsychotics in the treatment of delirium in older hospitalized adults.[19] Our results further highlight the need for randomized placebo‐controlled trials of antipsychotics in treatment of delirium.
We found variation in antipsychotic use between hospitals that was not fully explained by patient characteristics. Insufficient data to inform clinical decisions surrounding management of agitated delirium/dementia and lack of clear criteria by which to judge appropriateness of antipsychotic use may contribute to this variation. Some variation may relate to resource allocation at different hospitals, and the feasibility of implementing nonpharmacologic management options across settings. Our results collectively highlight the need for studies evaluating the efficacy/effectiveness of antipsychotics in the treatment of delirium and drivers of physician decision‐making in this realm, as well as the need for greater hospital investment in nonpharmacologic delirium‐prevention programs, which have been shown to be effective in prevention of delirium in hospitalized patients.[20]
We observed high levels of potentially excessive daily dosing using cutoffs applied in the long‐term care setting. The majority of the potentially excessive doses were in the setting of typical antipsychotic use, and haloperidol specifically, where doses exceeded 4 mg on at least 1 day in 87% of exposed admissions. Of note, the threshold for haloperidol dosage above which justification is required was decreased from 4 to 2 mg per day in the 2015 update to the CMS guidelines.[21] For the present analysis, we used the guidelines that were contemporaneous to our cohort; we are unable to determine current rates of potentially inappropriate dosages in the present analysis, but given the high prevalence in 2009 to 2010, and the lowering of the dosage threshold since then, it is unlikely that any decrease in use would be enough to substantially reduce the estimate. Whether these high dosages are actually inappropriate in the hospital setting is not established, and we were not able to review medical records to determine whether justification for use of such doses was documented.[22, 23] It is possible that hospitalized patients with altered pharmacodynamics and greater severity of illness could require larger doses of these medications; however, this is an area in need of further investigation, and current critical care guidelines note the lack of sufficient data upon which to justify use of haloperidol in the prevention or treatment of delirium in ICU patients.[24, 25]
The dosages in use are concerning given that the risk of extrapyramidal side effects increases with increasing dose, and prior studies have demonstrated an association between increased dose of antipsychotics and increased risk of other adverse events, including hip fracture and sudden cardiac death.[22, 23] Further, despite these known risks, studies have demonstrated failure to follow recommendations to mitigate risk,[26] such as electrocardiogram monitoring in individuals receiving intravenous haloperidol.[27] Our results suggest that physicians are similarly not following recommendations to use lower doses of haloperidol when treating older patients, given the almost identical incidence of potentially excessive dosing among admissions of patients aged 65 years and older in our cohort.[25] Clinical decision support prompts have been effective at increasing appropriate use of antipsychotic medications in several single‐center analyses,[28, 29, 30] and widespread implementation of such support with a focus on haloperidol dosing should be considered on the basis of our results.
The patient characteristics associated with antipsychotic use in this large, nationally representative analysis are consistent with those identified in prior single‐center analyses.[12, 18] Both prior analyses identified delirium as the most common reason for antipsychotic use, and dementia, intensive care unit stay, and mechanical ventilation were also previously identified as strong predictors of use that we believe hold face validity for the practicing hospitalist. On the other hand, some of the factors associated with antipsychotic use in our model cannot be readily explained, such as insurance status and race, and may be serving as proxies for other variables not included in our analysis. That nonwhite patients are less likely than white patients to receive antipsychotic medications in the hospital has been previously demonstrated,[12] and further investigation to understand this disparity is warranted.
Our study has several additional limitations. First, because our study is observational, the possibility of residual confounding exists, and we cannot rule out that there are other patient factors driving the hospital variation in antipsychotic use that we observed. Second, because guidelines do not exist for antipsychotic dosing in hospitalized patients, we could only comment on potentially excessive dosing, extrapolating from guidelines in the long‐term care setting. Whether such doses are actually excessive in hospitalized patients is not defined. Third, although Premier performs quality checks on charge and ICD‐9‐CM coding data submitted by participating hospitals, the validity of administrative data is uncertain. For example, the use of administrative data to identify delirium diagnoses is likely to have resulted in underestimation of delirium incidence among our different exposure groups. Delirium is likely to be coded more often in the setting of more severe or hyperactive cases, when antipsychotics are more likely to be utilized. This could result in an overestimation of the association between delirium and antipsychotic use. Additionally, differences in coding practices between hospitals for any of the variables in our models could explain some of the variation in antipsychotic prescribing that we observed. Finally, because we were unable to differentiate between new initiation and continuation of a preadmission antipsychotic, some of the variation that we observed is likely to reflect differences in outpatient antipsychotic prescribing practices.
In conclusion, in this large cohort of nonpsychiatric admissions to 300 US hospitals, we found that antipsychotic medication exposure was common, often at high daily doses. Delirium and dementia were the strongest predictors of use among the patient and hospital characteristics examined. The variation in antipsychotic prescribing that we observed was not fully accounted for by measured patient characteristics, and raises the possibility of differing hospital prescribing cultures. Our results draw attention to the need for additional research to better define what constitutes appropriate use of these potentially harmful medications in the hospital setting.
Disclosures: Dr. Herzig had full access to all of the data in the study and takes responsibility for the integrity of the data and the accuracy of the data analysis. Study concept and design: Drs. Herzig, Rothberg, Gurwitz, and Marcantonio. Acquisition of data: Dr. Herzig. Analysis of data: Mr. Guess. Interpretation of data: Drs. Herzig, Rothberg, Gurwitz, Marcantonio, and Mr. Guess. Drafting of the manuscript: Dr. Herzig. Critical revision of the manuscript for important intellectual content: Drs. Rothberg, Gurwitz, Marcantonio, and Mr. Guess. Dr. Herzig was funded by grant number K23AG042459 from the National Institute on Aging. Dr. Marcantonio was funded by grant number K24AG035075 from the National Institute on Aging. The funding organizations had no involvement in any aspect of the study, including design and conduct of the study; collection, management, analysis, and interpretation of the data; and preparation, review, or approval of the manuscript. The authors report no conflicts of interest.
Antipsychotic medications are frequently used off label for management of behavioral symptoms associated with delirium and/or dementia. Despite regulations designed to curb inappropriate prescribing of these medications in nursing homes, substantial levels of use and variation in use have been observed in this setting.[1] Although antipsychotic medications are also frequently used in the hospital, the scope and variation in use have not been adequately investigated. Given the lack of oversight for medication prescribing in the hospital setting and the frequency of delirium, occurring in 15% to 26% of hospitalized older adults,[2, 3, 4] off‐label use of antipsychotic medications and variation in use could be substantial.
Because variation in practice is known to increase in the setting of controversy or lack of clarity regarding appropriate management,[5] large degrees of variation can draw attention to priority areas for clinical effectiveness studies, and the need for guidelines, clinical decision support, or regulatory oversight. In the absence of clear guidelines for the use of antipsychotic medication in nonpsychiatric hospitalized patients, we hypothesized that significant variation in use would persist after controlling for patient characteristics. Using a large, nationally representative cohort of admissions to 300 hospitals from July 2009 to June 2010, we sought to investigate prescribing patterns and hospital variation in use of antipsychotic medications in nonpsychiatric admissions to US hospitals.
METHODS
Setting and Data Collection
We conducted a retrospective cohort study using data from 300 US, nonfederal, acute care facilities contributing to the database maintained by Premier (Premier Healthcare Solutions, Inc., Charlotte, NC). This nationally representative database, created to measure healthcare utilization and quality of care, is drawn from voluntarily participating hospitals and contains data on approximately 1 in every 4 discharges nationwide.[6] Participating hospitals are similar in geographic distribution and urban/rural status to hospitals nationwide, although large, nonteaching hospitals are slightly over‐represented. The study was approved by the institutional review board at Beth Israel Deaconess Medical Center.
Inclusion and Exclusion Criteria
We studied a cohort of all adult (18 years) nonpsychiatric admissions to participating hospitals from July 1, 2009 through June 30, 2010. We excluded patients admitted to a psychiatry service or with any discharge diagnosis of a psychotic disorder (defined by the Elixhauser comorbidity Psychoses: 295.00‐298.9, 299.10‐299.11), because we were interested in use of antipsychotics for conditions other than primary psychiatric disorders. We also excluded patients with a charge for labor and delivery owing to the nonrepresentativeness of this patient population for the general hospitalized patient. We excluded admissions with unknown gender, and admissions with a length of stay greater than 365 days, as these admissions are not representative of the typical admission to an acute care hospital. We also excluded hospitals contributing less than 100 admissions owing to lack of precision in corresponding hospital prescribing rates.
Antipsychotic Medication Utilization
In‐hospital antipsychotic use was ascertained from pharmacy charges, reflecting each medication dispensed during the hospitalization. We categorized antipsychotic medications as typical (haloperidol, loxapine, thioridazine, molindone, thiothixine, pimozide, fluphenazine, trifluoperazine, chlorpromazine, and perphenazine) and atypical (aripiprazole, asenapine, clozapine, iloperidone, lurasidone, olanzapine, paliperidone, quetiapine, risperidone, and ziprasidone) based on classification by the Food and Drug Administration.[7, 8] We excluded prochlorperazine (Compazine) from our typical antipsychotic definition, as this medication is almost exclusively used as an antiemetic.
In the absence of guidelines for use of antipsychotic agents in hospitalized patients, we used the Centers for Medicare and Medicaid Services (CMS) guidelines for long‐term care facilities to define measures of potentially excessive dosing in the hospital setting.[9] These guidelines define the daily dosage levels of antipsychotics above which the medical necessity of the higher dose should be explained in the medical record. We defined any daily dosage above these specified levels as a potentially excessive daily dose.
Characteristics Associated With Use
We investigated the association between antipsychotic use and patient and hospital characteristics, selected based on clinical grounds. Patient characteristics included: (1) demographic variables such as age (<65, 6574, 75+ years), gender, self‐reported race, marital status, and primary insurance; (2) admission characteristic variables, including admitting department (surgical vs nonsurgical, defined by a surgical attending of record and presence of operating room charges), whether the patient spent any time in the intensive care unit (ICU), and whether they received mechanical ventilation; and (3) potential indications for use, including delirium (included delirium superimposed upon dementia), dementia (without delirium), and insomnia (see Supporting Information, Appendix, in the online version of this article for International Classification of Diseases, Ninth Revision, Clinical Modification [ICD‐9‐CM] codes). Hospital characteristics included number of beds, urban versus rural status, teaching status, and US Census region.
Statistical Analysis
We report the proportion of admissions with in‐hospital use of any antipsychotic, and the number of days of exposure, overall and stratified by typical and atypical.
We determined potentially excessive dosing by taking the sum of the doses for a specific antipsychotic charged on a given day and comparing it to the CMS guidelines for long‐term care settings described above. We report the percentage of exposed admissions with at least 1 day of potentially excessive dosing.
All multivariable models below were operationalized as generalized estimating equations with a Poisson error term, log link, robust variance estimator,[10] and an exchangeable correlation structure to account for repeated admissions of the same patient.
We investigated patient and hospital characteristics associated with use of any antipsychotic medication using a multivariable model that simultaneously included all patient and hospital characteristics in Table 1 as independent variables.
% of Cohort | Any Exposure, % | Typical Exposure, % | Atypical Exposure, % | |
---|---|---|---|---|
| ||||
Patient characteristics | ||||
Age group, y | ||||
<65 | 52.1 | 4.6 | 2.0 | 3.1 |
6574 | 18.5 | 5.2 | 2.7 | 3.1 |
75+ | 29.4 | 8.8 | 4.6 | 5.4 |
Gender | ||||
Male | 43.8 | 6.6 | 3.6 | 3.8 |
Female | 56.2 | 5.5 | 2.3 | 3.8 |
Race | ||||
White | 64.6 | 6.1 | 2.9 | 4.0 |
Black | 13.5 | 5.5 | 2.8 | 3.3 |
Hispanic | 5.0 | 4.9 | 2.2 | 3.2 |
Other | 19.9 | 6.1 | 3.1 | 3.7 |
Marital Status | ||||
Married | 42.5 | 4.6 | 2.4 | 2.7 |
Single | 46.7 | 7.2 | 3.2 | 4.7 |
Unknown/other | 10.8 | 6.4 | 3.1 | 4.1 |
Primary insurance | ||||
Private (commercial) | 28.8 | 3.0 | 1.5 | 1.8 |
Medicaid | 10.3 | 6.4 | 2.4 | 4.6 |
Medicare managed | 10.6 | 7.1 | 4.1 | 4.0 |
Medicare traditional | 40.9 | 8.0 | 3.7 | 5.3 |
Self‐pay or other | 9.4 | 4.3 | 2.5 | 2.2 |
Admitting department | ||||
Surgical | 60.6 | 5.8 | 3.1 | 3.4 |
Nonsurgical | 39.4 | 6.2 | 2.4 | 4.4 |
Any ICU stay | 16.6 | 10.4 | 7.2 | 4.9 |
Mechanical ventilation | 4.7 | 17.4 | 12.9 | 7.9 |
Diagnoses | ||||
Delirium | 3.2 | 28.6 | 19.4 | 15.7 |
Dementia | 3.1 | 27.4 | 12.0 | 20.2 |
Insomnia | 1.3 | 10.2 | 3.9 | 7.5 |
Discharge disposition | ||||
Home | 77.9 | 3.8 | 1.6 | 2.5 |
SNF/Rehab | 15.5 | 13.7 | 6.8 | 9.0 |
Hospice | 1.7 | 16.0 | 10.3 | 8.1 |
Other | 4.9 | 11.6 | 7.6 | 5.7 |
Hospital characteristics, % | ||||
No. of beds | ||||
200 | 14.1 | 6.1 | 2.8 | 3.8 |
201300 | 18.6 | 6.1 | 2.9 | 3.9 |
301500 | 37.7 | 5.9 | 2.9 | 3.7 |
500+ | 29.7 | 5.9 | 2.8 | 3.8 |
Population served | ||||
Urban | 89.4 | 6.0 | 2.9 | 3.8 |
Rural | 10.6 | 5.8 | 2.4 | 3.9 |
Teaching status | ||||
Teaching | 39.2 | 5.8 | 2.9 | 3.7 |
Nonteaching | 60.8 | 6.0 | 2.8 | 3.9 |
US Census region | ||||
West | 16.9 | 5.9 | 3.2 | 3.5 |
Northeast | 20.1 | 6.1 | 2.9 | 3.9 |
Midwest | 21.9 | 5.7 | 2.5 | 3.8 |
South | 41.0 | 6.1 | 2.9 | 3.9 |
To determine hospital variation in antipsychotic use, we first determined the proportion of admissions at each hospital with at least 1 charge for antipsychotic medication. We then divided hospitals into quintiles based on their facility‐level antipsychotic prescribing rates and assigned all admissions to their corresponding hospital quintile. We then used a multivariable model to measure the adjusted association between prescribing quintile and patient‐level receipt of antipsychotic medication, controlling for all patient characteristics listed in Table 1 (except discharge disposition), and comorbidities using the Healthcare Cost and Utilization Project Comorbidity Software version 3.7 (Agency for Healthcare Research and Quality, Rockville, MD).[11] We used the lowest prescribing quintile as the reference group. We also report in the Supporting Information, Appendix, in the online version of this article, the distribution of prescribing rates for the hospitals in our cohort before and after adjustment for patient characteristics. For both approaches, we conducted stratified analyses in admissions with delirium and dementia.
All analyses were carried out using SAS software (SAS Institute Inc., Cary, NC).
RESULTS
Admission Characteristics
There were 3,190,934 admissions aged 18 years and over to 300 hospitals from July 1, 2009 to June 30, 2010. After excluding admissions with unknown gender (n = 17), length of stay greater than 365 days (n = 25), charges for labor and delivery (n = 323,111) or a psychiatric attending of record or psychiatric comorbidity (n = 172,669), and admissions to hospitals with fewer than 100 admissions (n = 31), our cohort included 2,695,081 admissions. The median age was 63 years (25th, 75th percentile 48, 77 years), and 1,514,986 (56%) were women. Table 1 shows the overall admission characteristics of the cohort and the percent exposed to antipsychotics among each patient and hospital characteristic.
Antipsychotic Use
There were 160,773 (6%) admissions with antipsychotic exposure. Among exposed admissions, 102,148 (64%) received atypical and 76,979 (48%) received typical antipsychotics, with 18,354 (11%) exposed to both. The median (25th, 75th percentile) length of stay among exposed was 5 days (3, 9 days), and the median (25th, 75th percentile) number of days of exposure was 3 (1, 5 days) overall, 3 days (2, 6 days) for atypical and 2 days (1, 3 days) for typical exposure.
Among admissions aged 65 to 74 years, 25,855 (5%) were exposed. Among admissions aged 75 years or older, 69,792 (9%) were exposed. Among admissions with delirium, exposure occurred in 24,787 (29%), with 13,640 (55%) receiving atypical, 16,828 (68%) receiving typical, and 5681 (23%) exposed to both. Among admissions with dementia, exposure occurred in 23,179 (27%), with 17,068 (74%) receiving atypical, 10,108 (44%) receiving typical, and 3997 (17%) exposed to both.
Use of Specific Drugs and Potentially Excessive Dosing
Table 2 demonstrates the most commonly used antipsychotic medications and the rates of potentially excessive dosing. Quetiapine and olanzapine were the most commonly used atypical antipsychotics, and haloperidol represented the majority of typical antipsychotic use. Among admissions with antipsychotic exposure, 47% received at least 1 potentially excessive daily dose, 18% of those with atypical exposure and 79% of those with typical exposure. Among admissions aged 65 years and up (n = 1,291,375), the prevalence of potentially excessive dosing was almost identical; 46% received at least 1 daily dose in excess of the recommended daily dose, 11% of those with atypical exposure and 79% of those with typical exposure.
Agent |
Overall Prevalence,N = 2,695,081 |
% of Exposed With Potentially Excessive Dosing* | ||
---|---|---|---|---|
Within 100% of Recommended DD* | 101% to 150% of Recommended DD* | >150% of Recommended DD* | ||
| ||||
Any antipsychotic | 6.0 | 52.9 | 20.2 | 26.9 |
Atypical | 3.8 | 82.0 | 5.4 | 12.6 |
Quetiapine (200) | 1.8 | 81.7 | 5.7 | 12.6 |
Olanzapine (10) | 0.6 | 73.7 | 7.3 | 19.0 |
Risperidone (2) | 0.9 | 79.2 | 6.8 | 14.0 |
Other | 0.7 | 98.3 | 0.1 | 1.6 |
Typical | 2.9 | 21.1 | 37.0 | 41.9 |
Haloperidol (4) | 2.5 | 13.2 | 41.3 | 45.5 |
Chlorpromazine (75) | 0.3 | 76.0 | 9.8 | 14.2 |
Other | 0.4 | 89.1 | 2.9 | 8.0 |
Characteristics Associated With Antipsychotic Use
Among the patient and hospital characteristics included in our analysis, the 5 characteristics most strongly associated with antipsychotic exposure after adjustment were (Table 3): delirium (relative risk [RR]: 2.93, 95% confidence interval [CI]: 2.88‐2.98); dementia (RR: 2.78, 95% CI: 2.72‐2.83); insurance status, with higher risk among patients with traditional Medicare (RR: 2.09, 95% CI: 2.04‐2.13), Medicare managed (RR: 1.98, 95% CI: 1.93‐2.03), Medicaid (RR: 1.84, 95% CI: 1.80‐1.88), and self‐pay/other (RR: 1.26, 95% CI: 1.23‐1.29) compared to private (commercial) insurance; use of mechanical ventilation (RR: 1.84, 95% CI: 1.81‐1.87); and any ICU stay (RR: 1.53, 95% CI: 1.51‐1.55).
Unadjusted RR of Receiving Any Antipsychotic [95% CI] | Adjusted RR of Receiving Any Antipsychotic [95% CI]* | |
---|---|---|
| ||
Age group, y, % | ||
<65 | Reference | Reference |
6574 | 1.12 [1.10,1.14] | 0.74 [0.72, 0.75] |
75+ | 1.90 [1.88,1.92] | 1.03 [1.01, 1.05] |
Gender | ||
Female | Reference | Reference |
Male | 1.19 [1.18,1.20] | 1.27 [1.26, 1.28] |
Race | ||
White | Reference | Reference |
Black | 0.91 [0.90,0.92] | 0.85 [0.83, 0.86] |
Hispanic | 0.80 [0.78,0.82] | 0.79 [0.76, 0.81] |
Other | 0.99 [0.98,1.00] | 0.96 [0.95, 0.98] |
Marital status | ||
Married | Reference | Reference |
Single | 1.57 [1.55,1.59] | 1.43 [1.42, 1.45] |
Unknown/other | 1.41 [1.39,1.43] | 1.27 [1.24, 1.29] |
Primary insurance | ||
Private (commercial) | Reference | Reference |
Medicaid | 2.13 [2.09,2.17] | 1.84 [1.80, 1.88] |
Medicare managed | 2.35 [2.31,2.39] | 1.98 [1.93, 2.03] |
Medicare traditional | 2.65 [2.61,2.69] | 2.09 [2.04, 2.13] |
Self‐pay or other | 1.41 [1.38,1.44] | 1.26 [1.23, 1.29] |
Admitting department | ||
Surgical | Reference | Reference |
Nonsurgical | 1.06 [1.05,1.07] | 1.05 [1.03, 1.06] |
Any ICU stay | 2.05 [2.03,2.07] | 1.53 [1.51, 1.55] |
Mechanical ventilation | 3.22 [3.18,3.26] | 1.84 [1.81, 1.87] |
Diagnoses | ||
Delirium | 5.48 [5.42, 5.45] | 2.93 [2.88, 2.98] |
Dementia | 5.21 [5.15,5.27] | 2.78 [2.72, 2.83] |
Insomnia | 1.72 [1.67,1.78] | 1.51 [1.45, 1.57] |
No. of beds | ||
200 | Reference | Reference |
201300 | 1.01 [0.99,1.03] | 0.96 [0.94, 0.98] |
301500 | 0.98 [0.97,1.00] | 0.93 [0.91, 0.95] |
500+ | 0.97 [0.96,0.98] | 0.91 [0.90, 0.93] |
Population served | ||
Urban | Reference | Reference |
Rural | 0.96 [0.95,0.98] | 0.91 [0.89, 0.93] |
Teaching status | ||
Teaching | Reference | Reference |
Nonteaching | 1.03 [1.02,1.04] | 0.98 [0.97, 1.00] |
US Census region | ||
West | Reference | Reference |
Northeast | 1.03 [1.01,1.05] | 1.04 [1.02, 1.06] |
Midwest | 0.95 [0.94,0.97] | 0.93 [0.91, 0.94] |
South | 1.02 [1.01,1.03] | 1.07 [1.05, 1.09] |
Hospital Variation in Antipsychotic Use
Figure 1 demonstrates the antipsychotic prescribing rate at each hospital in our cohort, and the corresponding quintiles. Patients admitted to hospitals in the highest prescribing quintile were more than twice as likely to be exposed to antipsychotics compared to patients admitted to hospitals in the lowest prescribing quintile, even after adjustment for patient characteristics and comorbidities (Table 4). This relationship was similar across subgroups of admissions with delirium and dementia (see Supporting Information, Appendix, in the online version of this article for the distribution of hospital antipsychotic prescribing rates before and after adjustment for patient characteristics).
Admissions, No. (% of Total) | Unadjusted RR of Exposure [95% CI] | Adjusted RR of exposure [95% CI]* | |
---|---|---|---|
| |||
Overall | |||
Q1 | 431,017 (16%) | Reference | Reference |
Q2 | 630,486 (23%) | 1.67 [1.63, 1.71] | 1.59 [1.55, 1.62] |
Q3 | 548,337 (20%) | 1.93 [1.88, 1.97] | 1.84 [1.80, 1.88] |
Q4 | 639,027 (24%) | 2.16 [2.12, 2.21] | 2.07 [2.03, 2.12] |
Q5 | 446,214 (17%) | 2.83 [2.77, 2.89] | 2.56 [2.50, 2.61] |
Delirium | |||
Q1 | 12,878 (15%) | Reference | Reference |
Q2 | 20,588 (24%) | 1.58 [1.51, 1.65] | 1.58 [1.51, 1.65] |
Q3 | 17,402 (20%) | 1.71 [1.64, 1.80] | 1.73 [1.65, 1.82] |
Q4 | 20,943 (24%) | 2.01 [1.92, 2.10] | 1.99 [1.91, 2.08] |
Q5 | 14,883 (17%) | 2.15 [2.05, 2.25] | 2.16 [2.07, 2.26] |
Dementia | |||
Q1 | 28,290 (15%) | Reference | Reference |
Q2 | 42,018 (22%) | 1.43 [1.36, 1.50] | 1.40 [1.34, 1.47] |
Q3 | 38,593 (21%) | 1.61 [1.53, 1.69] | 1.59 [1.51, 1.66] |
Q4 | 44,638 (24%) | 1.69 [1.62, 1.77] | 1.69 [1.61, 1.77] |
Q5 | 34,442 (18%) | 1.92 [1.83, 2.01] | 1.90 [1.81, 1.99] |
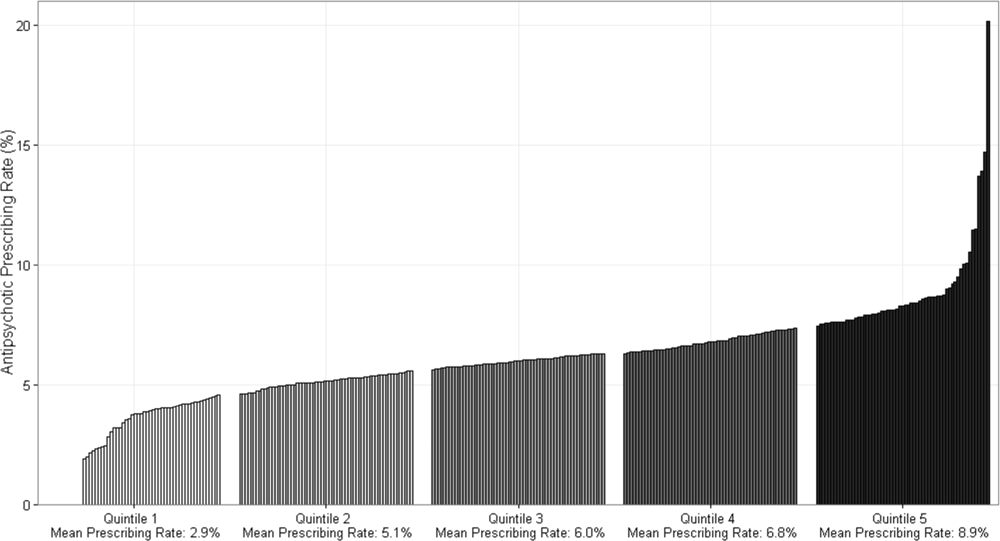
DISCUSSION
In this cohort of nonpsychiatric admissions to 300 US hospitals, antipsychotic medications were used in 6% of admissions, with atypical antipsychotics representing the majority of use. Potentially excessive daily doses based on CMS recommendations for long‐term care facilities occurred in almost half of admissions with any antipsychotic exposure, and in 87% of admissions with haloperidol exposure specifically. We found variation in hospital use of antipsychotics that was not fully accounted for by measured patient characteristics, and which persisted among subgroups of admissions with delirium and/or dementia. Although unmeasured patient characteristics or different billing practices between hospitals are potential explanations, our findings also raise the possibility of different hospital antipsychotic prescribing cultures. These findings provide new information regarding the scope of prescribing in US hospitals, and draw attention to the need for additional studies to better define what constitutes appropriate use of antipsychotics in the hospital setting.
A recent single‐center study at a large academic medical center found an overall antipsychotic exposure rate of 9% of nonpsychiatric admissions.[12] Our finding that 6% of admissions in this multicenter cohort were exposed to antipsychotics is slightly lower, but similar to the previous estimate. Assuming 37 million discharges from US hospitals each year,[13] our study suggests that more than 2 million hospitalized patients receive antipsychotics annually. With around 1.4 million residents in nursing homes on any given day,[14] and an exposure rate of 25% to 30% in that setting,[15, 16, 17] our study suggests that the number of patients exposed in the hospital setting is greater than the number exposed in the nursing home setting, the site of care for which prescribing regulations have been focused thus far.
Because our dataset does not contain preadmission medications, we were unable to specifically investigate new initiation. In the prior single‐center study, approximately 55% of overall use in the hospital setting was new initiation,[12] which would suggest that antipsychotics are newly initiated in around 1 million admissions each year in the hospital. Although we are unable to determine reason for use in our analysis, delirium was a strong predictor of antipsychotic use in our multivariable model, and prior studies have demonstrated delirium to be the most common reason for antipsychotic initiation in hospitalized patients,[12, 18] an indication for which efficacy/effectiveness data are lacking. A recent systematic review of antipsychotics for the treatment of delirium in older adults concluded that because of severe methodological limitations, the small number of existing studies on this topic do not support the use of antipsychotics in the treatment of delirium in older hospitalized adults.[19] Our results further highlight the need for randomized placebo‐controlled trials of antipsychotics in treatment of delirium.
We found variation in antipsychotic use between hospitals that was not fully explained by patient characteristics. Insufficient data to inform clinical decisions surrounding management of agitated delirium/dementia and lack of clear criteria by which to judge appropriateness of antipsychotic use may contribute to this variation. Some variation may relate to resource allocation at different hospitals, and the feasibility of implementing nonpharmacologic management options across settings. Our results collectively highlight the need for studies evaluating the efficacy/effectiveness of antipsychotics in the treatment of delirium and drivers of physician decision‐making in this realm, as well as the need for greater hospital investment in nonpharmacologic delirium‐prevention programs, which have been shown to be effective in prevention of delirium in hospitalized patients.[20]
We observed high levels of potentially excessive daily dosing using cutoffs applied in the long‐term care setting. The majority of the potentially excessive doses were in the setting of typical antipsychotic use, and haloperidol specifically, where doses exceeded 4 mg on at least 1 day in 87% of exposed admissions. Of note, the threshold for haloperidol dosage above which justification is required was decreased from 4 to 2 mg per day in the 2015 update to the CMS guidelines.[21] For the present analysis, we used the guidelines that were contemporaneous to our cohort; we are unable to determine current rates of potentially inappropriate dosages in the present analysis, but given the high prevalence in 2009 to 2010, and the lowering of the dosage threshold since then, it is unlikely that any decrease in use would be enough to substantially reduce the estimate. Whether these high dosages are actually inappropriate in the hospital setting is not established, and we were not able to review medical records to determine whether justification for use of such doses was documented.[22, 23] It is possible that hospitalized patients with altered pharmacodynamics and greater severity of illness could require larger doses of these medications; however, this is an area in need of further investigation, and current critical care guidelines note the lack of sufficient data upon which to justify use of haloperidol in the prevention or treatment of delirium in ICU patients.[24, 25]
The dosages in use are concerning given that the risk of extrapyramidal side effects increases with increasing dose, and prior studies have demonstrated an association between increased dose of antipsychotics and increased risk of other adverse events, including hip fracture and sudden cardiac death.[22, 23] Further, despite these known risks, studies have demonstrated failure to follow recommendations to mitigate risk,[26] such as electrocardiogram monitoring in individuals receiving intravenous haloperidol.[27] Our results suggest that physicians are similarly not following recommendations to use lower doses of haloperidol when treating older patients, given the almost identical incidence of potentially excessive dosing among admissions of patients aged 65 years and older in our cohort.[25] Clinical decision support prompts have been effective at increasing appropriate use of antipsychotic medications in several single‐center analyses,[28, 29, 30] and widespread implementation of such support with a focus on haloperidol dosing should be considered on the basis of our results.
The patient characteristics associated with antipsychotic use in this large, nationally representative analysis are consistent with those identified in prior single‐center analyses.[12, 18] Both prior analyses identified delirium as the most common reason for antipsychotic use, and dementia, intensive care unit stay, and mechanical ventilation were also previously identified as strong predictors of use that we believe hold face validity for the practicing hospitalist. On the other hand, some of the factors associated with antipsychotic use in our model cannot be readily explained, such as insurance status and race, and may be serving as proxies for other variables not included in our analysis. That nonwhite patients are less likely than white patients to receive antipsychotic medications in the hospital has been previously demonstrated,[12] and further investigation to understand this disparity is warranted.
Our study has several additional limitations. First, because our study is observational, the possibility of residual confounding exists, and we cannot rule out that there are other patient factors driving the hospital variation in antipsychotic use that we observed. Second, because guidelines do not exist for antipsychotic dosing in hospitalized patients, we could only comment on potentially excessive dosing, extrapolating from guidelines in the long‐term care setting. Whether such doses are actually excessive in hospitalized patients is not defined. Third, although Premier performs quality checks on charge and ICD‐9‐CM coding data submitted by participating hospitals, the validity of administrative data is uncertain. For example, the use of administrative data to identify delirium diagnoses is likely to have resulted in underestimation of delirium incidence among our different exposure groups. Delirium is likely to be coded more often in the setting of more severe or hyperactive cases, when antipsychotics are more likely to be utilized. This could result in an overestimation of the association between delirium and antipsychotic use. Additionally, differences in coding practices between hospitals for any of the variables in our models could explain some of the variation in antipsychotic prescribing that we observed. Finally, because we were unable to differentiate between new initiation and continuation of a preadmission antipsychotic, some of the variation that we observed is likely to reflect differences in outpatient antipsychotic prescribing practices.
In conclusion, in this large cohort of nonpsychiatric admissions to 300 US hospitals, we found that antipsychotic medication exposure was common, often at high daily doses. Delirium and dementia were the strongest predictors of use among the patient and hospital characteristics examined. The variation in antipsychotic prescribing that we observed was not fully accounted for by measured patient characteristics, and raises the possibility of differing hospital prescribing cultures. Our results draw attention to the need for additional research to better define what constitutes appropriate use of these potentially harmful medications in the hospital setting.
Disclosures: Dr. Herzig had full access to all of the data in the study and takes responsibility for the integrity of the data and the accuracy of the data analysis. Study concept and design: Drs. Herzig, Rothberg, Gurwitz, and Marcantonio. Acquisition of data: Dr. Herzig. Analysis of data: Mr. Guess. Interpretation of data: Drs. Herzig, Rothberg, Gurwitz, Marcantonio, and Mr. Guess. Drafting of the manuscript: Dr. Herzig. Critical revision of the manuscript for important intellectual content: Drs. Rothberg, Gurwitz, Marcantonio, and Mr. Guess. Dr. Herzig was funded by grant number K23AG042459 from the National Institute on Aging. Dr. Marcantonio was funded by grant number K24AG035075 from the National Institute on Aging. The funding organizations had no involvement in any aspect of the study, including design and conduct of the study; collection, management, analysis, and interpretation of the data; and preparation, review, or approval of the manuscript. The authors report no conflicts of interest.
- Variation in nursing home antipsychotic prescribing rates. Arch Intern Med. 2007;167(7):676–683. , , , et al.
- Precipitating factors for delirium in hospitalized elderly persons. Predictive model and interrelationship with baseline vulnerability. JAMA. 1996;275(11):852–857. , .
- A predictive model for delirium in hospitalized elderly medical patients based on admission characteristics. Ann Intern Med. 1993;119(6):474–481. , , , , .
- Delirium in hospitalized older persons: outcomes and predictors. J Am Geriatr Soc. 1994;42(8):809–815. , , , , , .
- Small‐area variations in the use of common surgical procedures: an international comparison of New England, England, and Norway. N Engl J Med. 1982;307(21):1310–1314. , , , .
- Premier Research Services. Available at: https://www.premierinc.com/transforming‐healthcare/healthcare‐performance‐improvement/premier‐research‐services. Accessed March 15, 2016.
- U.S. Food and Drug Administration. Atypical antipsychotic drugs information. Available at: http://www.fda.gov/drugs/drugsafety/postmarketdrugsafetyinformationforpatientsandproviders/ucm094303.htm. Accessed November 1, 2015.
- U.S. Food and Drug Administration. Information on conventional antipsychotics. Available at: http://www.fda.gov/drugs/drugsafety/postmarketdrugsafetyinformationforpatientsandproviders/ucm107211. htm. Accessed November 1, 2015.
- Centers for Medicare and Medicaid Services. State Operations Manual. Appendix PP: guidance to surveyors for long‐term care facilities. Available at: https://www.cms.gov/Medicare/Provider‐Enrollment‐and‐Certification/GuidanceforLawsAndRegulations/Downloads/som107 ap_pp_guidelines_ltcf.pdf. Revised October 14, 2005. Accessed March 15, 2016.
- A modified Poisson regression approach to prospective studies with binary data. Am J Epidemiol. 2004;159(7):702–706. .
- Healthcare Cost and Utilization Project. Comorbidity software, version 3.7. Agency for Healthcare Research and Quality, Rockville, MD. Available at: http://www.hcup‐us.ahrq.gov/toolssoftware/comorbidity/comorbidity.jsp. Accessed March 15, 2016.
- Antipsychotic use in hospitalized adults: rates, indications, and predictors. J Am Geriatr Soc. 2016;64(2):299–305. , , , et al.
- Overview of hospital stays in the United States, 2012. HCUP Statistical Brief #180. Agency for Healthcare Research and Quality, Rockville, MD. Available at: http://www.hcup‐us.ahrq.gov/reports/statbriefs/sb180‐Hospitalizations‐United‐States‐2012.pdf. Published October 2014. Accessed June 29, 2015. , .
- Long‐term care services in the United States: 2013 overview. Vital Health Stat 3. 2013;(37):1–107. Available at: http://www.cdc.gov/nchs/data/nsltcp/long_term_care_services_2013.pdf. Accessed March 16, 2016. , , , .
- The quality of antipsychotic drug prescribing in nursing homes. Arch Intern Med. 2005;165(11):1280–1285. , , , et al.
- Unexplained variation across US nursing homes in antipsychotic prescribing rates. Arch Intern Med. 2010;170(1):89–95. , , , , , .
- Broadened use of atypical antipsychotics: safety, effectiveness, and policy challenges. Health Aff (Millwood). 2009;28(5):w770–w781. , , , , .
- From hospital to community: use of antipsychotics in hospitalized elders. J Hosp Med. 2014;9(12):802–804. , , , , , .
- Antipsychotics in the treatment of delirium in older hospitalized adults: a systematic review. J Am Geriatr Soc. 2011;59(suppl 2):S269–S276. , , .
- Effectiveness of multicomponent nonpharmacological delirium interventions: a meta‐analysis. JAMA Intern Med. 2015;175(4):512–520. , , , et al.
- Centers for Medicare and Medicaid Services. State operations manual, appendix PP: guidance to surveyors for long‐term care facilities. Available at: https://www.cms.gov/Regulations‐and‐Guidance/Guidance/Manuals/downloads/som107ap_pp_guidelines_ltcf.pdf. Revised October 9, 2015. Accessed February 22, 2016.
- Psychotropic drug use and the risk of hip fracture. N Engl J Med. 1987;316(7):363–369. , , , , .
- Atypical antipsychotic drugs and the risk of sudden cardiac death. N Engl J Med. 2009;360(3):225–235. , , , , .
- Clinical practice guidelines for the management of pain, agitation, and delirium in adult patients in the intensive care unit. Crit Care Med. 2013;41(1):263–306. , , , et al.
- Haloperidol overdosing in the treatment of agitated hospitalized older people with delirium: a retrospective chart review from a community teaching hospital. Drugs Aging. 2013;30(8):639–644. , , , , .
- Unsafe use of intravenous haloperidol: evaluation of recommendation‐concordant care in hospitalized elderly adults. J Am Geriatr Soc. 2013;61(1):160–161. , , , .
- U.S. Food and Drug Administration. HALDOL brand of haloperidol injection. Available at: http://www.accessdata.fda.gov/drugsatfda_docs/label/2008/015923s082,018701s057lbl.pdf. Accessed February 23, 2016.
- Preventing potentially inappropriate medication use in hospitalized older patients with a computerized provider order entry warning system. Arch Intern Med. 2010;170(15):1331–1336. , , , .
- A standardized, bundled approach to providing geriatric‐focused acute care. J Am Geriatr Soc. 2014;62(5):936–942. , , , et al.
- Don't fuel the fire: decreasing intravenous haloperidol use in high risk patients via a customized electronic alert. J Am Med Inform Assoc. 2014;21(6):1109–1112. , , , .
- Variation in nursing home antipsychotic prescribing rates. Arch Intern Med. 2007;167(7):676–683. , , , et al.
- Precipitating factors for delirium in hospitalized elderly persons. Predictive model and interrelationship with baseline vulnerability. JAMA. 1996;275(11):852–857. , .
- A predictive model for delirium in hospitalized elderly medical patients based on admission characteristics. Ann Intern Med. 1993;119(6):474–481. , , , , .
- Delirium in hospitalized older persons: outcomes and predictors. J Am Geriatr Soc. 1994;42(8):809–815. , , , , , .
- Small‐area variations in the use of common surgical procedures: an international comparison of New England, England, and Norway. N Engl J Med. 1982;307(21):1310–1314. , , , .
- Premier Research Services. Available at: https://www.premierinc.com/transforming‐healthcare/healthcare‐performance‐improvement/premier‐research‐services. Accessed March 15, 2016.
- U.S. Food and Drug Administration. Atypical antipsychotic drugs information. Available at: http://www.fda.gov/drugs/drugsafety/postmarketdrugsafetyinformationforpatientsandproviders/ucm094303.htm. Accessed November 1, 2015.
- U.S. Food and Drug Administration. Information on conventional antipsychotics. Available at: http://www.fda.gov/drugs/drugsafety/postmarketdrugsafetyinformationforpatientsandproviders/ucm107211. htm. Accessed November 1, 2015.
- Centers for Medicare and Medicaid Services. State Operations Manual. Appendix PP: guidance to surveyors for long‐term care facilities. Available at: https://www.cms.gov/Medicare/Provider‐Enrollment‐and‐Certification/GuidanceforLawsAndRegulations/Downloads/som107 ap_pp_guidelines_ltcf.pdf. Revised October 14, 2005. Accessed March 15, 2016.
- A modified Poisson regression approach to prospective studies with binary data. Am J Epidemiol. 2004;159(7):702–706. .
- Healthcare Cost and Utilization Project. Comorbidity software, version 3.7. Agency for Healthcare Research and Quality, Rockville, MD. Available at: http://www.hcup‐us.ahrq.gov/toolssoftware/comorbidity/comorbidity.jsp. Accessed March 15, 2016.
- Antipsychotic use in hospitalized adults: rates, indications, and predictors. J Am Geriatr Soc. 2016;64(2):299–305. , , , et al.
- Overview of hospital stays in the United States, 2012. HCUP Statistical Brief #180. Agency for Healthcare Research and Quality, Rockville, MD. Available at: http://www.hcup‐us.ahrq.gov/reports/statbriefs/sb180‐Hospitalizations‐United‐States‐2012.pdf. Published October 2014. Accessed June 29, 2015. , .
- Long‐term care services in the United States: 2013 overview. Vital Health Stat 3. 2013;(37):1–107. Available at: http://www.cdc.gov/nchs/data/nsltcp/long_term_care_services_2013.pdf. Accessed March 16, 2016. , , , .
- The quality of antipsychotic drug prescribing in nursing homes. Arch Intern Med. 2005;165(11):1280–1285. , , , et al.
- Unexplained variation across US nursing homes in antipsychotic prescribing rates. Arch Intern Med. 2010;170(1):89–95. , , , , , .
- Broadened use of atypical antipsychotics: safety, effectiveness, and policy challenges. Health Aff (Millwood). 2009;28(5):w770–w781. , , , , .
- From hospital to community: use of antipsychotics in hospitalized elders. J Hosp Med. 2014;9(12):802–804. , , , , , .
- Antipsychotics in the treatment of delirium in older hospitalized adults: a systematic review. J Am Geriatr Soc. 2011;59(suppl 2):S269–S276. , , .
- Effectiveness of multicomponent nonpharmacological delirium interventions: a meta‐analysis. JAMA Intern Med. 2015;175(4):512–520. , , , et al.
- Centers for Medicare and Medicaid Services. State operations manual, appendix PP: guidance to surveyors for long‐term care facilities. Available at: https://www.cms.gov/Regulations‐and‐Guidance/Guidance/Manuals/downloads/som107ap_pp_guidelines_ltcf.pdf. Revised October 9, 2015. Accessed February 22, 2016.
- Psychotropic drug use and the risk of hip fracture. N Engl J Med. 1987;316(7):363–369. , , , , .
- Atypical antipsychotic drugs and the risk of sudden cardiac death. N Engl J Med. 2009;360(3):225–235. , , , , .
- Clinical practice guidelines for the management of pain, agitation, and delirium in adult patients in the intensive care unit. Crit Care Med. 2013;41(1):263–306. , , , et al.
- Haloperidol overdosing in the treatment of agitated hospitalized older people with delirium: a retrospective chart review from a community teaching hospital. Drugs Aging. 2013;30(8):639–644. , , , , .
- Unsafe use of intravenous haloperidol: evaluation of recommendation‐concordant care in hospitalized elderly adults. J Am Geriatr Soc. 2013;61(1):160–161. , , , .
- U.S. Food and Drug Administration. HALDOL brand of haloperidol injection. Available at: http://www.accessdata.fda.gov/drugsatfda_docs/label/2008/015923s082,018701s057lbl.pdf. Accessed February 23, 2016.
- Preventing potentially inappropriate medication use in hospitalized older patients with a computerized provider order entry warning system. Arch Intern Med. 2010;170(15):1331–1336. , , , .
- A standardized, bundled approach to providing geriatric‐focused acute care. J Am Geriatr Soc. 2014;62(5):936–942. , , , et al.
- Don't fuel the fire: decreasing intravenous haloperidol use in high risk patients via a customized electronic alert. J Am Med Inform Assoc. 2014;21(6):1109–1112. , , , .
Reducing Inappropriate Acid Suppressives
Prior studies have found that up to 70% of acid‐suppressive medication (ASM) use in the hospital is not indicated, most commonly for stress ulcer prophylaxis in patients outside of the intensive care unit (ICU).[1, 2, 3, 4, 5, 6, 7] Accordingly, reducing inappropriate use of ASM for stress ulcer prophylaxis in hospitalized patients is 1 of the 5 opportunities for improved healthcare value identified by the Society of Hospital Medicine as part of the American Board of Internal Medicine's Choosing Wisely campaign.[8]
We designed and tested a computerized clinical decision support (CDS) intervention with the goal of reducing use of ASM for stress ulcer prophylaxis in hospitalized patients outside the ICU at an academic medical center.
METHODS
Study Design
We conducted a quasiexperimental study using an interrupted time series to analyze data collected prospectively during clinical care before and after implementation of our intervention. The study was deemed a quality improvement initiative by the Beth Israel Deaconess Medical Center Committee on Clinical Investigations/Institutional Review Board.
Patients and Setting
All admissions >18 years of age to a 649‐bed academic medical center in Boston, Massachusetts from September 12, 2011 through July 3, 2012 were included. The medical center consists of an East and West Campus, located across the street from each other. Care for both critically ill and noncritically ill medical and surgical patients occurs on both campuses. Differences include greater proportions of patients with gastrointestinal and oncologic conditions on the East Campus, and renal and cardiac conditions on the West Campus. Additionally, labor and delivery occurs exclusively on the East Campus, and the density of ICU beds is greater on the West Campus. Both campuses utilize a computer‐based provider order entry (POE) system.
Intervention
Our study was implemented in 2 phases (Figure 1).
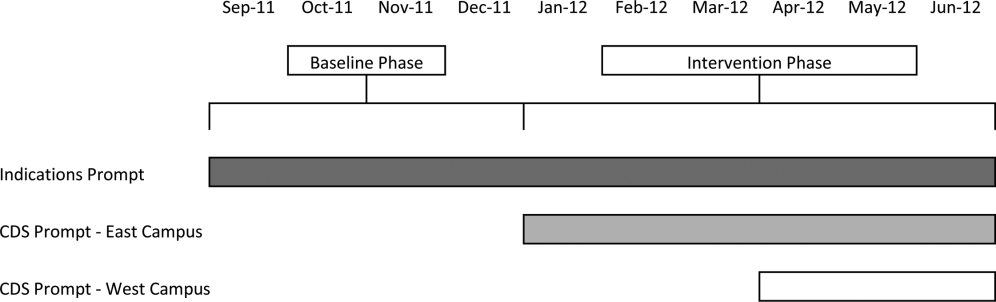
Baseline Phase
The purpose of the first phase was to obtain baseline data on ASM use prior to implementing our CDS tool designed to influence prescribing. During this baseline phase, a computerized prompt was activated through our POE system whenever a clinician initiated an order for ASM (histamine 2 receptor antagonists or proton pump inhibitors), asking the clinician to select the reason/reasons for the order based on the following predefined response options: (1) active/recent upper gastrointestinal bleed, (2) continuing preadmission medication, (3) Helicobacter pylori treatment, (4) prophylaxis in patient on medications that increase bleeding risk, (5) stress ulcer prophylaxis, (6) suspected/known peptic ulcer disease, gastritis, esophagitis, gastroesophageal reflux disease, and (7) other, with a free‐text box to input the indication. This indications prompt was rolled out to the entire medical center on September 12, 2011 and remained active for the duration of the study period.
Intervention Phase
In the second phase of the study, if a clinician selected stress ulcer prophylaxis as the only indication for ordering ASM, a CDS prompt alerted the clinician that Stress ulcer prophylaxis is not recommended for patients outside of the intensive care unit (ASHP Therapeutic Guidelines on Stress Ulcer Prophylaxis. Am J Health‐Syst Pharm. 1999, 56:347‐79). The clinician could then select either, For use in ICUOrder Medication, Choose Other Indication, or Cancel Order. This CDS prompt was rolled out in a staggered manner to the East Campus on January 3, 2012, followed by the West Campus on April 3, 2012.
Outcomes
The primary outcome was the rate of ASM use with stress ulcer prophylaxis selected as the only indication in a patient located outside of the ICU. We confirmed patient location in the 24 hours after the order was placed. Secondary outcomes were rates of overall ASM use, defined via pharmacy charges, and rates of use on discharge.
Statistical Analysis
To assure stable measurement of trends, we studied at least 3 months before and after the intervention on each campus. We used the Fisher exact test to compare the rates of our primary and secondary outcomes before and after the intervention, stratified by campus. For our primary outcomeat least 1 ASM order with stress ulcer prophylaxis selected as the only indication during hospitalizationwe developed a logistic regression model with a generalized estimating equation and exchangeable working correlation structure to control for admission characteristics (Table 1) and repeated admissions. Using a term for the interaction between time and the intervention, this model allowed us to assess changes in level and trend for the odds of a patient receiving at least 1 ASM order with stress ulcer prophylaxis as the only indication before, compared to after the intervention, stratified by campus. We used a 2‐sided type I error of <0.05 to indicate statistical significance.
Study Phase | Campus | |||
---|---|---|---|---|
East | West | |||
Baseline, n=3,747 | Intervention, n=6,191 | Baseline, n=11,177 | Intervention, n=5,285 | |
| ||||
Age, y, mean (SD) | 48.1 (18.5) | 47.7 (18.2) | 61.0 (18.0) | 60.3 (18.1) |
Gender, no. (%) | ||||
Female | 2744 (73.2%) | 4542 (73.4%) | 5551 (49.7%) | 2653 (50.2%) |
Male | 1003 (26.8%) | 1649 (26.6%) | 5626 (50.3%) | 2632 (49.8%) |
Race, no. (%) | ||||
Asian | 281 (7.5%) | 516 (8.3%) | 302 (2.7%) | 156 (3%) |
Black | 424 (11.3%) | 667 (10.8%) | 1426 (12.8%) | 685 (13%) |
Hispanic | 224 (6%) | 380 (6.1%) | 619 (5.5%) | 282 (5.3%) |
Other | 378 (10.1%) | 738 (11.9%) | 776 (6.9%) | 396 (7.5%) |
White | 2440 (65.1%) | 3890 (62.8%) | 8054 (72%) | 3766 (71.3%) |
Charlson score, mean (SD) | 0.8 (1.1) | 0.7 (1.1) | 1.5 (1.4) | 1.4 (1.4) |
Gastrointestinal bleeding, no. (%)* | 49 (1.3%) | 99 (1.6%) | 385 (3.4%) | 149 (2.8%) |
Other medication exposures, no. (%) | ||||
Therapeutic anticoagulant | 218 (5.8%) | 409 (6.6%) | 2242 (20.1%) | 1022 (19.3%) |
Prophylactic anticoagulant | 1081 (28.8%) | 1682 (27.2%) | 5999 (53.7%) | 2892 (54.7%) |
NSAID | 1899 (50.7%) | 3141 (50.7%) | 1248 (11.2%) | 575 (10.9%) |
Antiplatelet | 313 (8.4%) | 585 (9.4%) | 4543 (40.6%) | 2071 (39.2%) |
Admitting department, no. (%) | ||||
Surgery | 2507 (66.9%) | 4146 (67%) | 3255 (29.1%) | 1578 (29.9%) |
Nonsurgery | 1240 (33.1%) | 2045 (33%) | 7922 (70.9%) | 3707 (70.1%) |
Any ICU Stay, no. (%) | 217 (5.8%) | 383 (6.2%) | 2786 (24.9%) | 1252 (23.7%) |
RESULTS
There were 26,400 adult admissions during the study period, and 22,330 discrete orders for ASM. Overall, 12,056 (46%) admissions had at least 1 charge for ASM. Admission characteristics were similar before and after the intervention on each campus (Table 1).
Table 2 shows the indications chosen each time ASM was ordered, stratified by campus and study phase. Although selection of stress ulcer prophylaxis decreased on both campuses during the intervention phase, selection of continuing preadmission medication increased.
Study Phase | Campus | |||
---|---|---|---|---|
East | West | |||
Baseline, n=2,062 | Intervention, n=3,243 | Baseline, n=12,038 | Intervention, n=4,987 | |
| ||||
Indication* | ||||
Continuing preadmission medication | 910 (44.1%) | 1695 (52.3%) | 5597 (46.5%) | 2802 (56.2%) |
PUD, gastritis, esophagitis, GERD | 440 (21.3%) | 797 (24.6%) | 1303 (10.8%) | 582 (11.7%) |
Stress ulcer prophylaxis | 298 (14.4%) | 100 (3.1%) | 2659 (22.1%) | 681 (13.7%) |
Prophylaxis in patient on medications that increase bleeding risk | 226 (11.0%) | 259 (8.0%) | 965 (8.0%) | 411 (8.2%) |
Active/recent gastrointestinal bleed | 154 (7.5%) | 321 (9.9%) | 1450 (12.0%) | 515 (10.3) |
Helicobacter pylori treatment | 6 (0.2%) | 2 (0.1%) | 43 (0.4%) | 21 (0.4%) |
Other | 111 (5.4%) | 156 (4.8%) | 384 (3.2%) | 186 (3.7%) |
Table 3 shows the unadjusted comparison of outcomes between baseline and intervention phases on each campus. Use of ASM with stress ulcer prophylaxis as the only indication decreased during the intervention phase on both campuses. There was a nonsignificant reduction in overall rates of use on both campuses, and use on discharge was unchanged. Figure 2 demonstrates the unadjusted and modeled monthly rates of admissions with at least 1 ASM order with stress ulcer prophylaxis selected as the only indication, stratified by campus. After adjusting for the admission characteristics in Table 1, during the intervention phase on both campuses there was a significant immediate reduction in the odds of receiving an ASM with stress ulcer prophylaxis selected as the only indication (East Campus odds ratio [OR]: 0.36, 95% confidence interval [CI]: 0.180.71; West Campus OR: 0.41, 95% CI: 0.280.60), and a significant change in trend compared to the baseline phase (East Campus 1.5% daily decrease in odds of receiving ASM solely for stress ulcer prophylaxis, P=0.002; West Campus 0.9% daily decrease in odds of receiving ASM solely for stress ulcer prophylaxis, P=0.02).
Study Phase | Campus | |||||
---|---|---|---|---|---|---|
East | West | |||||
Baseline, n=3,747 | Intervention, n=6,191 | P Value* | Baseline, n=11,177 | Intervention, n=5,285 | P Value* | |
| ||||||
Outcome | ||||||
Any inappropriate acid‐suppressive exposure | 4.0% | 0.6% | <0.001 | 7.7% | 2.2% | <0.001 |
Any acid‐suppressive exposure | 33.1% | 31.8% | 0.16 | 54.5% | 52.9% | 0.05 |
Discharged on acid‐suppressive medication | 18.9% | 19.6% | 0.40 | 34.7% | 34.7% | 0.95 |
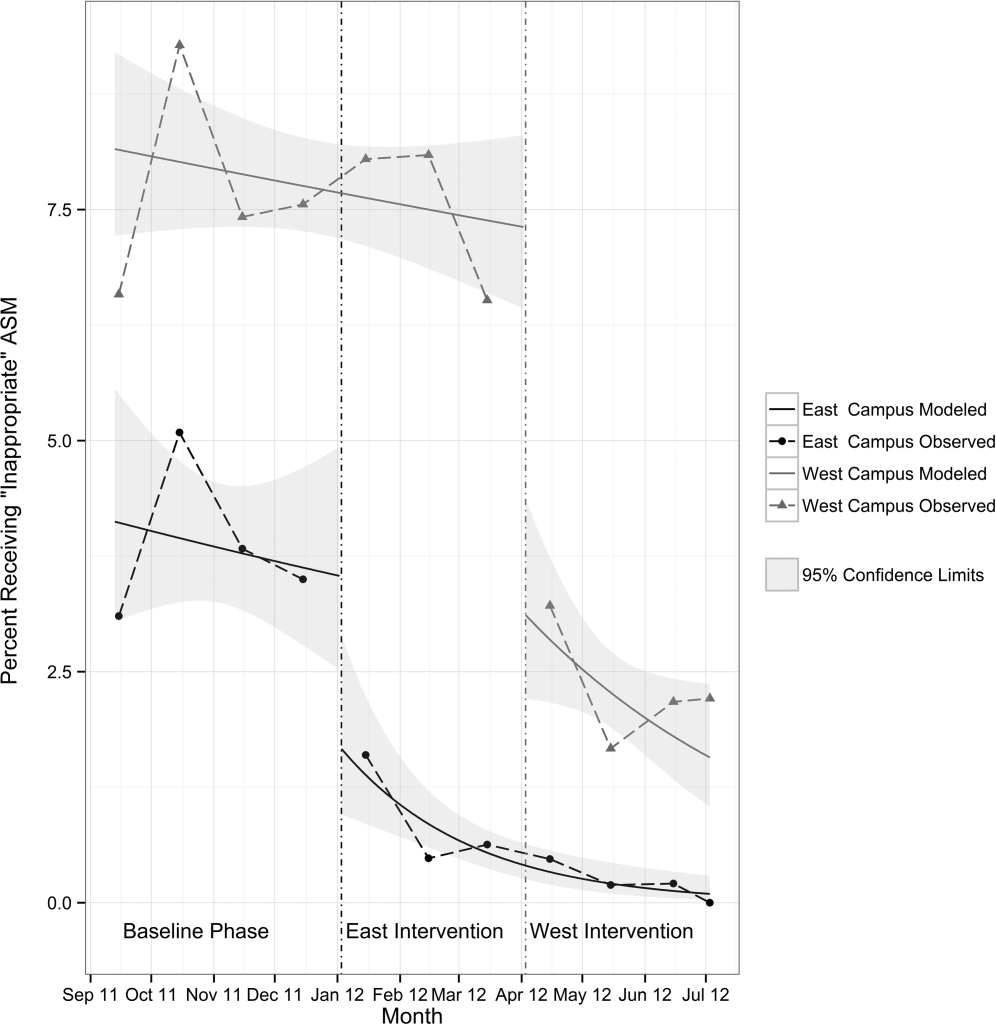
DISCUSSION
In this single‐center study, we found that a computerized CDS intervention resulted in a significant reduction in use of ASM for the sole purpose of stress ulcer prophylaxis in patients outside the ICU, a nonsignificant reduction in overall use, and no change in use on discharge. We found low rates of use for the isolated purpose of stress ulcer prophylaxis even before the intervention, and continuing preadmission medication was the most commonly selected indication throughout the study.
Although overall rates of ASM use declined after the intervention, the change was not statistically significant, and was not of the same magnitude as the decline in rates of use for the purpose of stress ulcer prophylaxis. This suggests that our intervention, in part, led to substitution of 1 indication for another. The indication that increased the most after rollout on both campuses was continuing preadmission medication. There are at least 2 possibilities for this finding: (1) the intervention prompted physicians to more accurately record the indication, or (2) physicians falsified the indication in order to execute the order. To explore these possibilities, we reviewed the charts of a random sample of 100 admissions during each of the baseline and intervention phases where continuing preadmission medication was selected as an indication for an ASM order. We found that 6/100 orders in the baseline phase and 7/100 orders in the intervention phase incorrectly indicated that the patient was on ASM prior to admission (P=0.77). This suggests that scenario 1 above is the more likely explanation for the increased use of this indication, and that the intervention, in part, simply unmasked the true rate of use at our medical center for the isolated purpose of stress ulcer prophylaxis.
These findings have implications for others attempting to use computerized CDS to better understand physician prescribing. They suggest that information collected through computer‐based interaction with clinicians at the point of care may not always be accurate or complete. As institutions increasingly use similar interventions to drive behavior, information obtained from such interaction should be validated, and when possible, patient outcomes should be measured.
Our findings suggest that rates of ASM use for the purpose of stress ulcer prophylaxis in the hospital may have declined over the last decade. Studies demonstrating that up to 70% of inpatient use of ASM was inappropriate were conducted 5 to 10 years ago.[1, 2, 3, 4, 5] Since then, studies have demonstrated risk of nosocomial infections in patients on ASM.[9, 10, 11] It is possible that the low rate of use for stress ulcer prophylaxis in our study is attributable to awareness of the risks of these medications, and limited our ability to detect differences in overall use. It is also possible, however, that a portion of the admissions with continuation of preadmission medication as the indication were started on these medications during a prior hospitalization. Thus, some portion of preadmission use is likely to represent failed medication reconciliation during a prior discharge. In this context, hospitalization may serve as an opportunity to evaluate the indication for ASM use even when these medications show up as preadmission medications.
There are additional limitations. First, the single‐center nature limits generalizability. Second, the first phase of our study, designed to obtain baseline data on ASM use, may have led to changes in prescribing prior to implementation of our CDS tool. Additionally, we did not validate the accuracy of each of the chosen indications, or the site of initial prescription in the case of preadmission exposure. Last, our study was not powered to investigate changes in rates of nosocomial gastrointestinal bleeding or nosocomial pneumonia owing to the infrequent nature of these complications.
In conclusion, we designed a simple computerized CDS intervention that was associated with a reduction in ASM use for stress ulcer prophylaxis in patients outside the ICU, a nonsignificant reduction in overall use, and no change in use on discharge. The majority of inpatient use represented continuation of preadmission medication, suggesting that interventions to improve the appropriateness of ASM prescribing should span the continuum of care. Future studies should investigate whether it is worthwhile and appropriate to reevaluate continued use of preadmission ASM during an inpatient stay.
Acknowledgements
The authors acknowledge Joshua Guthermann, MBA, and Jane Hui Chen Lim, MBA, for their assistance in the early phases of data analysis, and Long H. Ngo, PhD, for his statistical consultation.
Disclosures: Dr. Herzig was funded by a Young Clinician Research Award from the Center for Integration of Medicine and Innovative Technology, a nonprofit consortium of Boston teaching hospitals and universities, and grant number K23AG042459 from the National Institute on Aging. Dr. Marcantonio was funded by grant number K24AG035075 from the National Institute on Aging. The funding organizations had no involvement in any aspect of the study, including design, conduct, and reporting of the study. Dr. Herzig had full access to all of the data in the study and takes responsibility for the integrity of the data and the accuracy of the data analysis. Drs. Herzig and Marcantonio were responsible for the study concept and design. Drs. Herzig, Feinbloom, Howell, and Ms. Adra and Mr. Afonso were responsible for the acquisition of data. Drs. Herzig, Howell, Marcantonio, and Mr. Guess were responsible for the analysis and interpretation of the data. Dr. Herzig drafted the manuscript. All of the authors participated in the critical revision of the manuscript for important intellectual content. Drs. Herzig and Marcantonio were responsible for study supervision. The authors report no conflicts of interest.
- Stress ulcer prophylaxis in hospitalized patients not in intensive care units. Am J Health Syst Pharm. 2007;64(13):1396–1400. , .
- Magnitude and economic impact of inappropriate use of stress ulcer prophylaxis in non‐ICU hospitalized patients. Am J Gastroenterol. 2006;101(10):2200–2205. , .
- Stress‐ulcer prophylaxis for general medical patients: a review of the evidence. J Hosp Med. 2007;2(2):86–92. , .
- Hospital use of acid‐suppressive medications and its fall‐out on prescribing in general practice: a 1‐month survey. Aliment Pharmacol Ther. 2003;17(12):1503–1506. , , , et al.
- Inadequate use of acid‐suppressive therapy in hospitalized patients and its implications for general practice. Dig Dis Sci. 2005;50(12):2307–2311. , , , , , .
- Brief report: reducing inappropriate usage of stress ulcer prophylaxis among internal medicine residents. A practice‐based educational intervention. J Gen Intern Med. 2006;21(5):498–500. , .
- Inappropriate continuation of stress ulcer prophylactic therapy after discharge. Ann Pharmacother. 2007;41(10):1611–1616. , , .
- Choosing wisely in adult hospital medicine: five opportunities for improved healthcare value. J Hosp Med. 2013;8(9):486–492. , , , et al.
- Risk of Clostridium difficile diarrhea among hospital inpatients prescribed proton pump inhibitors: cohort and case‐control studies. CMAJ. 2004;171(1):33–38. , , , , .
- Iatrogenic gastric acid suppression and the risk of nosocomial Clostridium difficile infection. Arch Intern Med. 2010;170(9):784–790. , , , et al.
- Acid‐suppressive medication use and the risk for hospital‐acquired pneumonia. JAMA. 2009;301(20):2120–2128. , , , .
- Healthcare Cost and Utilization Project. Clinical classifications software (CCS) for ICD‐9‐CM. December 2009. Agency for Healthcare Research and Quality, Rockville, MD. Available at: www.hcup‐us.ahrq.gov/toolssoftware/ccs/ccs.jsp. Accessed June 18, 2014.
Prior studies have found that up to 70% of acid‐suppressive medication (ASM) use in the hospital is not indicated, most commonly for stress ulcer prophylaxis in patients outside of the intensive care unit (ICU).[1, 2, 3, 4, 5, 6, 7] Accordingly, reducing inappropriate use of ASM for stress ulcer prophylaxis in hospitalized patients is 1 of the 5 opportunities for improved healthcare value identified by the Society of Hospital Medicine as part of the American Board of Internal Medicine's Choosing Wisely campaign.[8]
We designed and tested a computerized clinical decision support (CDS) intervention with the goal of reducing use of ASM for stress ulcer prophylaxis in hospitalized patients outside the ICU at an academic medical center.
METHODS
Study Design
We conducted a quasiexperimental study using an interrupted time series to analyze data collected prospectively during clinical care before and after implementation of our intervention. The study was deemed a quality improvement initiative by the Beth Israel Deaconess Medical Center Committee on Clinical Investigations/Institutional Review Board.
Patients and Setting
All admissions >18 years of age to a 649‐bed academic medical center in Boston, Massachusetts from September 12, 2011 through July 3, 2012 were included. The medical center consists of an East and West Campus, located across the street from each other. Care for both critically ill and noncritically ill medical and surgical patients occurs on both campuses. Differences include greater proportions of patients with gastrointestinal and oncologic conditions on the East Campus, and renal and cardiac conditions on the West Campus. Additionally, labor and delivery occurs exclusively on the East Campus, and the density of ICU beds is greater on the West Campus. Both campuses utilize a computer‐based provider order entry (POE) system.
Intervention
Our study was implemented in 2 phases (Figure 1).
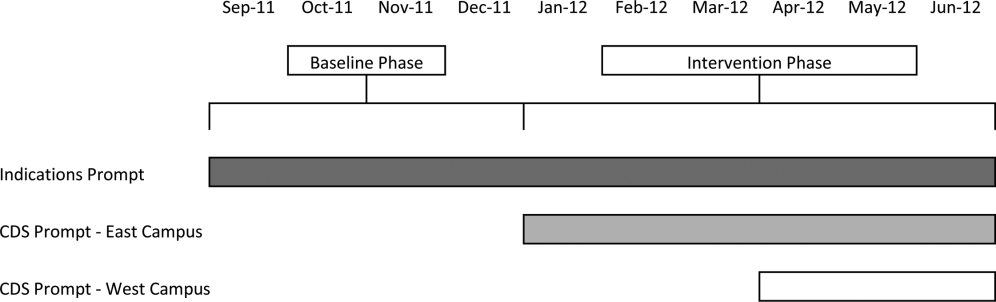
Baseline Phase
The purpose of the first phase was to obtain baseline data on ASM use prior to implementing our CDS tool designed to influence prescribing. During this baseline phase, a computerized prompt was activated through our POE system whenever a clinician initiated an order for ASM (histamine 2 receptor antagonists or proton pump inhibitors), asking the clinician to select the reason/reasons for the order based on the following predefined response options: (1) active/recent upper gastrointestinal bleed, (2) continuing preadmission medication, (3) Helicobacter pylori treatment, (4) prophylaxis in patient on medications that increase bleeding risk, (5) stress ulcer prophylaxis, (6) suspected/known peptic ulcer disease, gastritis, esophagitis, gastroesophageal reflux disease, and (7) other, with a free‐text box to input the indication. This indications prompt was rolled out to the entire medical center on September 12, 2011 and remained active for the duration of the study period.
Intervention Phase
In the second phase of the study, if a clinician selected stress ulcer prophylaxis as the only indication for ordering ASM, a CDS prompt alerted the clinician that Stress ulcer prophylaxis is not recommended for patients outside of the intensive care unit (ASHP Therapeutic Guidelines on Stress Ulcer Prophylaxis. Am J Health‐Syst Pharm. 1999, 56:347‐79). The clinician could then select either, For use in ICUOrder Medication, Choose Other Indication, or Cancel Order. This CDS prompt was rolled out in a staggered manner to the East Campus on January 3, 2012, followed by the West Campus on April 3, 2012.
Outcomes
The primary outcome was the rate of ASM use with stress ulcer prophylaxis selected as the only indication in a patient located outside of the ICU. We confirmed patient location in the 24 hours after the order was placed. Secondary outcomes were rates of overall ASM use, defined via pharmacy charges, and rates of use on discharge.
Statistical Analysis
To assure stable measurement of trends, we studied at least 3 months before and after the intervention on each campus. We used the Fisher exact test to compare the rates of our primary and secondary outcomes before and after the intervention, stratified by campus. For our primary outcomeat least 1 ASM order with stress ulcer prophylaxis selected as the only indication during hospitalizationwe developed a logistic regression model with a generalized estimating equation and exchangeable working correlation structure to control for admission characteristics (Table 1) and repeated admissions. Using a term for the interaction between time and the intervention, this model allowed us to assess changes in level and trend for the odds of a patient receiving at least 1 ASM order with stress ulcer prophylaxis as the only indication before, compared to after the intervention, stratified by campus. We used a 2‐sided type I error of <0.05 to indicate statistical significance.
Study Phase | Campus | |||
---|---|---|---|---|
East | West | |||
Baseline, n=3,747 | Intervention, n=6,191 | Baseline, n=11,177 | Intervention, n=5,285 | |
| ||||
Age, y, mean (SD) | 48.1 (18.5) | 47.7 (18.2) | 61.0 (18.0) | 60.3 (18.1) |
Gender, no. (%) | ||||
Female | 2744 (73.2%) | 4542 (73.4%) | 5551 (49.7%) | 2653 (50.2%) |
Male | 1003 (26.8%) | 1649 (26.6%) | 5626 (50.3%) | 2632 (49.8%) |
Race, no. (%) | ||||
Asian | 281 (7.5%) | 516 (8.3%) | 302 (2.7%) | 156 (3%) |
Black | 424 (11.3%) | 667 (10.8%) | 1426 (12.8%) | 685 (13%) |
Hispanic | 224 (6%) | 380 (6.1%) | 619 (5.5%) | 282 (5.3%) |
Other | 378 (10.1%) | 738 (11.9%) | 776 (6.9%) | 396 (7.5%) |
White | 2440 (65.1%) | 3890 (62.8%) | 8054 (72%) | 3766 (71.3%) |
Charlson score, mean (SD) | 0.8 (1.1) | 0.7 (1.1) | 1.5 (1.4) | 1.4 (1.4) |
Gastrointestinal bleeding, no. (%)* | 49 (1.3%) | 99 (1.6%) | 385 (3.4%) | 149 (2.8%) |
Other medication exposures, no. (%) | ||||
Therapeutic anticoagulant | 218 (5.8%) | 409 (6.6%) | 2242 (20.1%) | 1022 (19.3%) |
Prophylactic anticoagulant | 1081 (28.8%) | 1682 (27.2%) | 5999 (53.7%) | 2892 (54.7%) |
NSAID | 1899 (50.7%) | 3141 (50.7%) | 1248 (11.2%) | 575 (10.9%) |
Antiplatelet | 313 (8.4%) | 585 (9.4%) | 4543 (40.6%) | 2071 (39.2%) |
Admitting department, no. (%) | ||||
Surgery | 2507 (66.9%) | 4146 (67%) | 3255 (29.1%) | 1578 (29.9%) |
Nonsurgery | 1240 (33.1%) | 2045 (33%) | 7922 (70.9%) | 3707 (70.1%) |
Any ICU Stay, no. (%) | 217 (5.8%) | 383 (6.2%) | 2786 (24.9%) | 1252 (23.7%) |
RESULTS
There were 26,400 adult admissions during the study period, and 22,330 discrete orders for ASM. Overall, 12,056 (46%) admissions had at least 1 charge for ASM. Admission characteristics were similar before and after the intervention on each campus (Table 1).
Table 2 shows the indications chosen each time ASM was ordered, stratified by campus and study phase. Although selection of stress ulcer prophylaxis decreased on both campuses during the intervention phase, selection of continuing preadmission medication increased.
Study Phase | Campus | |||
---|---|---|---|---|
East | West | |||
Baseline, n=2,062 | Intervention, n=3,243 | Baseline, n=12,038 | Intervention, n=4,987 | |
| ||||
Indication* | ||||
Continuing preadmission medication | 910 (44.1%) | 1695 (52.3%) | 5597 (46.5%) | 2802 (56.2%) |
PUD, gastritis, esophagitis, GERD | 440 (21.3%) | 797 (24.6%) | 1303 (10.8%) | 582 (11.7%) |
Stress ulcer prophylaxis | 298 (14.4%) | 100 (3.1%) | 2659 (22.1%) | 681 (13.7%) |
Prophylaxis in patient on medications that increase bleeding risk | 226 (11.0%) | 259 (8.0%) | 965 (8.0%) | 411 (8.2%) |
Active/recent gastrointestinal bleed | 154 (7.5%) | 321 (9.9%) | 1450 (12.0%) | 515 (10.3) |
Helicobacter pylori treatment | 6 (0.2%) | 2 (0.1%) | 43 (0.4%) | 21 (0.4%) |
Other | 111 (5.4%) | 156 (4.8%) | 384 (3.2%) | 186 (3.7%) |
Table 3 shows the unadjusted comparison of outcomes between baseline and intervention phases on each campus. Use of ASM with stress ulcer prophylaxis as the only indication decreased during the intervention phase on both campuses. There was a nonsignificant reduction in overall rates of use on both campuses, and use on discharge was unchanged. Figure 2 demonstrates the unadjusted and modeled monthly rates of admissions with at least 1 ASM order with stress ulcer prophylaxis selected as the only indication, stratified by campus. After adjusting for the admission characteristics in Table 1, during the intervention phase on both campuses there was a significant immediate reduction in the odds of receiving an ASM with stress ulcer prophylaxis selected as the only indication (East Campus odds ratio [OR]: 0.36, 95% confidence interval [CI]: 0.180.71; West Campus OR: 0.41, 95% CI: 0.280.60), and a significant change in trend compared to the baseline phase (East Campus 1.5% daily decrease in odds of receiving ASM solely for stress ulcer prophylaxis, P=0.002; West Campus 0.9% daily decrease in odds of receiving ASM solely for stress ulcer prophylaxis, P=0.02).
Study Phase | Campus | |||||
---|---|---|---|---|---|---|
East | West | |||||
Baseline, n=3,747 | Intervention, n=6,191 | P Value* | Baseline, n=11,177 | Intervention, n=5,285 | P Value* | |
| ||||||
Outcome | ||||||
Any inappropriate acid‐suppressive exposure | 4.0% | 0.6% | <0.001 | 7.7% | 2.2% | <0.001 |
Any acid‐suppressive exposure | 33.1% | 31.8% | 0.16 | 54.5% | 52.9% | 0.05 |
Discharged on acid‐suppressive medication | 18.9% | 19.6% | 0.40 | 34.7% | 34.7% | 0.95 |
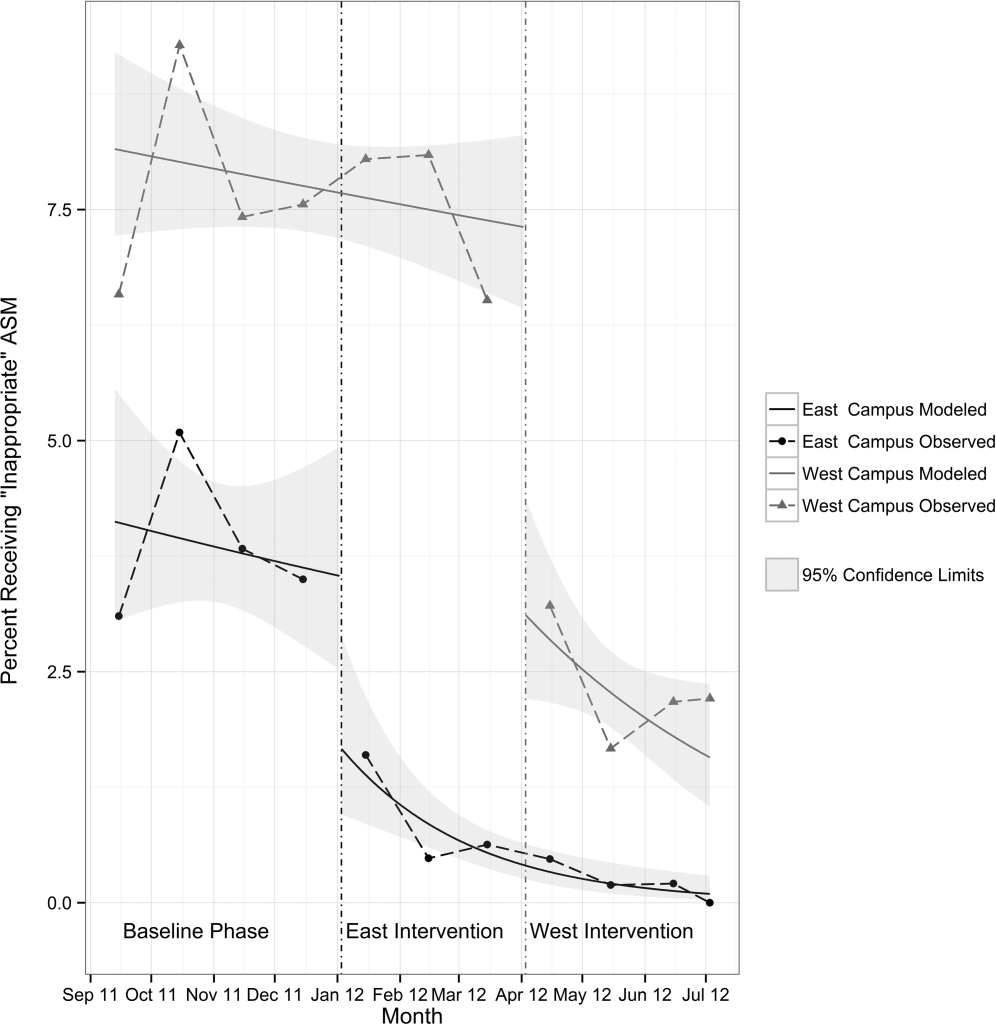
DISCUSSION
In this single‐center study, we found that a computerized CDS intervention resulted in a significant reduction in use of ASM for the sole purpose of stress ulcer prophylaxis in patients outside the ICU, a nonsignificant reduction in overall use, and no change in use on discharge. We found low rates of use for the isolated purpose of stress ulcer prophylaxis even before the intervention, and continuing preadmission medication was the most commonly selected indication throughout the study.
Although overall rates of ASM use declined after the intervention, the change was not statistically significant, and was not of the same magnitude as the decline in rates of use for the purpose of stress ulcer prophylaxis. This suggests that our intervention, in part, led to substitution of 1 indication for another. The indication that increased the most after rollout on both campuses was continuing preadmission medication. There are at least 2 possibilities for this finding: (1) the intervention prompted physicians to more accurately record the indication, or (2) physicians falsified the indication in order to execute the order. To explore these possibilities, we reviewed the charts of a random sample of 100 admissions during each of the baseline and intervention phases where continuing preadmission medication was selected as an indication for an ASM order. We found that 6/100 orders in the baseline phase and 7/100 orders in the intervention phase incorrectly indicated that the patient was on ASM prior to admission (P=0.77). This suggests that scenario 1 above is the more likely explanation for the increased use of this indication, and that the intervention, in part, simply unmasked the true rate of use at our medical center for the isolated purpose of stress ulcer prophylaxis.
These findings have implications for others attempting to use computerized CDS to better understand physician prescribing. They suggest that information collected through computer‐based interaction with clinicians at the point of care may not always be accurate or complete. As institutions increasingly use similar interventions to drive behavior, information obtained from such interaction should be validated, and when possible, patient outcomes should be measured.
Our findings suggest that rates of ASM use for the purpose of stress ulcer prophylaxis in the hospital may have declined over the last decade. Studies demonstrating that up to 70% of inpatient use of ASM was inappropriate were conducted 5 to 10 years ago.[1, 2, 3, 4, 5] Since then, studies have demonstrated risk of nosocomial infections in patients on ASM.[9, 10, 11] It is possible that the low rate of use for stress ulcer prophylaxis in our study is attributable to awareness of the risks of these medications, and limited our ability to detect differences in overall use. It is also possible, however, that a portion of the admissions with continuation of preadmission medication as the indication were started on these medications during a prior hospitalization. Thus, some portion of preadmission use is likely to represent failed medication reconciliation during a prior discharge. In this context, hospitalization may serve as an opportunity to evaluate the indication for ASM use even when these medications show up as preadmission medications.
There are additional limitations. First, the single‐center nature limits generalizability. Second, the first phase of our study, designed to obtain baseline data on ASM use, may have led to changes in prescribing prior to implementation of our CDS tool. Additionally, we did not validate the accuracy of each of the chosen indications, or the site of initial prescription in the case of preadmission exposure. Last, our study was not powered to investigate changes in rates of nosocomial gastrointestinal bleeding or nosocomial pneumonia owing to the infrequent nature of these complications.
In conclusion, we designed a simple computerized CDS intervention that was associated with a reduction in ASM use for stress ulcer prophylaxis in patients outside the ICU, a nonsignificant reduction in overall use, and no change in use on discharge. The majority of inpatient use represented continuation of preadmission medication, suggesting that interventions to improve the appropriateness of ASM prescribing should span the continuum of care. Future studies should investigate whether it is worthwhile and appropriate to reevaluate continued use of preadmission ASM during an inpatient stay.
Acknowledgements
The authors acknowledge Joshua Guthermann, MBA, and Jane Hui Chen Lim, MBA, for their assistance in the early phases of data analysis, and Long H. Ngo, PhD, for his statistical consultation.
Disclosures: Dr. Herzig was funded by a Young Clinician Research Award from the Center for Integration of Medicine and Innovative Technology, a nonprofit consortium of Boston teaching hospitals and universities, and grant number K23AG042459 from the National Institute on Aging. Dr. Marcantonio was funded by grant number K24AG035075 from the National Institute on Aging. The funding organizations had no involvement in any aspect of the study, including design, conduct, and reporting of the study. Dr. Herzig had full access to all of the data in the study and takes responsibility for the integrity of the data and the accuracy of the data analysis. Drs. Herzig and Marcantonio were responsible for the study concept and design. Drs. Herzig, Feinbloom, Howell, and Ms. Adra and Mr. Afonso were responsible for the acquisition of data. Drs. Herzig, Howell, Marcantonio, and Mr. Guess were responsible for the analysis and interpretation of the data. Dr. Herzig drafted the manuscript. All of the authors participated in the critical revision of the manuscript for important intellectual content. Drs. Herzig and Marcantonio were responsible for study supervision. The authors report no conflicts of interest.
Prior studies have found that up to 70% of acid‐suppressive medication (ASM) use in the hospital is not indicated, most commonly for stress ulcer prophylaxis in patients outside of the intensive care unit (ICU).[1, 2, 3, 4, 5, 6, 7] Accordingly, reducing inappropriate use of ASM for stress ulcer prophylaxis in hospitalized patients is 1 of the 5 opportunities for improved healthcare value identified by the Society of Hospital Medicine as part of the American Board of Internal Medicine's Choosing Wisely campaign.[8]
We designed and tested a computerized clinical decision support (CDS) intervention with the goal of reducing use of ASM for stress ulcer prophylaxis in hospitalized patients outside the ICU at an academic medical center.
METHODS
Study Design
We conducted a quasiexperimental study using an interrupted time series to analyze data collected prospectively during clinical care before and after implementation of our intervention. The study was deemed a quality improvement initiative by the Beth Israel Deaconess Medical Center Committee on Clinical Investigations/Institutional Review Board.
Patients and Setting
All admissions >18 years of age to a 649‐bed academic medical center in Boston, Massachusetts from September 12, 2011 through July 3, 2012 were included. The medical center consists of an East and West Campus, located across the street from each other. Care for both critically ill and noncritically ill medical and surgical patients occurs on both campuses. Differences include greater proportions of patients with gastrointestinal and oncologic conditions on the East Campus, and renal and cardiac conditions on the West Campus. Additionally, labor and delivery occurs exclusively on the East Campus, and the density of ICU beds is greater on the West Campus. Both campuses utilize a computer‐based provider order entry (POE) system.
Intervention
Our study was implemented in 2 phases (Figure 1).
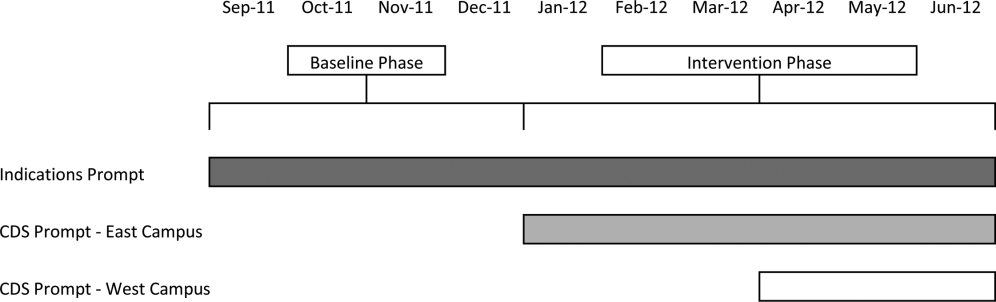
Baseline Phase
The purpose of the first phase was to obtain baseline data on ASM use prior to implementing our CDS tool designed to influence prescribing. During this baseline phase, a computerized prompt was activated through our POE system whenever a clinician initiated an order for ASM (histamine 2 receptor antagonists or proton pump inhibitors), asking the clinician to select the reason/reasons for the order based on the following predefined response options: (1) active/recent upper gastrointestinal bleed, (2) continuing preadmission medication, (3) Helicobacter pylori treatment, (4) prophylaxis in patient on medications that increase bleeding risk, (5) stress ulcer prophylaxis, (6) suspected/known peptic ulcer disease, gastritis, esophagitis, gastroesophageal reflux disease, and (7) other, with a free‐text box to input the indication. This indications prompt was rolled out to the entire medical center on September 12, 2011 and remained active for the duration of the study period.
Intervention Phase
In the second phase of the study, if a clinician selected stress ulcer prophylaxis as the only indication for ordering ASM, a CDS prompt alerted the clinician that Stress ulcer prophylaxis is not recommended for patients outside of the intensive care unit (ASHP Therapeutic Guidelines on Stress Ulcer Prophylaxis. Am J Health‐Syst Pharm. 1999, 56:347‐79). The clinician could then select either, For use in ICUOrder Medication, Choose Other Indication, or Cancel Order. This CDS prompt was rolled out in a staggered manner to the East Campus on January 3, 2012, followed by the West Campus on April 3, 2012.
Outcomes
The primary outcome was the rate of ASM use with stress ulcer prophylaxis selected as the only indication in a patient located outside of the ICU. We confirmed patient location in the 24 hours after the order was placed. Secondary outcomes were rates of overall ASM use, defined via pharmacy charges, and rates of use on discharge.
Statistical Analysis
To assure stable measurement of trends, we studied at least 3 months before and after the intervention on each campus. We used the Fisher exact test to compare the rates of our primary and secondary outcomes before and after the intervention, stratified by campus. For our primary outcomeat least 1 ASM order with stress ulcer prophylaxis selected as the only indication during hospitalizationwe developed a logistic regression model with a generalized estimating equation and exchangeable working correlation structure to control for admission characteristics (Table 1) and repeated admissions. Using a term for the interaction between time and the intervention, this model allowed us to assess changes in level and trend for the odds of a patient receiving at least 1 ASM order with stress ulcer prophylaxis as the only indication before, compared to after the intervention, stratified by campus. We used a 2‐sided type I error of <0.05 to indicate statistical significance.
Study Phase | Campus | |||
---|---|---|---|---|
East | West | |||
Baseline, n=3,747 | Intervention, n=6,191 | Baseline, n=11,177 | Intervention, n=5,285 | |
| ||||
Age, y, mean (SD) | 48.1 (18.5) | 47.7 (18.2) | 61.0 (18.0) | 60.3 (18.1) |
Gender, no. (%) | ||||
Female | 2744 (73.2%) | 4542 (73.4%) | 5551 (49.7%) | 2653 (50.2%) |
Male | 1003 (26.8%) | 1649 (26.6%) | 5626 (50.3%) | 2632 (49.8%) |
Race, no. (%) | ||||
Asian | 281 (7.5%) | 516 (8.3%) | 302 (2.7%) | 156 (3%) |
Black | 424 (11.3%) | 667 (10.8%) | 1426 (12.8%) | 685 (13%) |
Hispanic | 224 (6%) | 380 (6.1%) | 619 (5.5%) | 282 (5.3%) |
Other | 378 (10.1%) | 738 (11.9%) | 776 (6.9%) | 396 (7.5%) |
White | 2440 (65.1%) | 3890 (62.8%) | 8054 (72%) | 3766 (71.3%) |
Charlson score, mean (SD) | 0.8 (1.1) | 0.7 (1.1) | 1.5 (1.4) | 1.4 (1.4) |
Gastrointestinal bleeding, no. (%)* | 49 (1.3%) | 99 (1.6%) | 385 (3.4%) | 149 (2.8%) |
Other medication exposures, no. (%) | ||||
Therapeutic anticoagulant | 218 (5.8%) | 409 (6.6%) | 2242 (20.1%) | 1022 (19.3%) |
Prophylactic anticoagulant | 1081 (28.8%) | 1682 (27.2%) | 5999 (53.7%) | 2892 (54.7%) |
NSAID | 1899 (50.7%) | 3141 (50.7%) | 1248 (11.2%) | 575 (10.9%) |
Antiplatelet | 313 (8.4%) | 585 (9.4%) | 4543 (40.6%) | 2071 (39.2%) |
Admitting department, no. (%) | ||||
Surgery | 2507 (66.9%) | 4146 (67%) | 3255 (29.1%) | 1578 (29.9%) |
Nonsurgery | 1240 (33.1%) | 2045 (33%) | 7922 (70.9%) | 3707 (70.1%) |
Any ICU Stay, no. (%) | 217 (5.8%) | 383 (6.2%) | 2786 (24.9%) | 1252 (23.7%) |
RESULTS
There were 26,400 adult admissions during the study period, and 22,330 discrete orders for ASM. Overall, 12,056 (46%) admissions had at least 1 charge for ASM. Admission characteristics were similar before and after the intervention on each campus (Table 1).
Table 2 shows the indications chosen each time ASM was ordered, stratified by campus and study phase. Although selection of stress ulcer prophylaxis decreased on both campuses during the intervention phase, selection of continuing preadmission medication increased.
Study Phase | Campus | |||
---|---|---|---|---|
East | West | |||
Baseline, n=2,062 | Intervention, n=3,243 | Baseline, n=12,038 | Intervention, n=4,987 | |
| ||||
Indication* | ||||
Continuing preadmission medication | 910 (44.1%) | 1695 (52.3%) | 5597 (46.5%) | 2802 (56.2%) |
PUD, gastritis, esophagitis, GERD | 440 (21.3%) | 797 (24.6%) | 1303 (10.8%) | 582 (11.7%) |
Stress ulcer prophylaxis | 298 (14.4%) | 100 (3.1%) | 2659 (22.1%) | 681 (13.7%) |
Prophylaxis in patient on medications that increase bleeding risk | 226 (11.0%) | 259 (8.0%) | 965 (8.0%) | 411 (8.2%) |
Active/recent gastrointestinal bleed | 154 (7.5%) | 321 (9.9%) | 1450 (12.0%) | 515 (10.3) |
Helicobacter pylori treatment | 6 (0.2%) | 2 (0.1%) | 43 (0.4%) | 21 (0.4%) |
Other | 111 (5.4%) | 156 (4.8%) | 384 (3.2%) | 186 (3.7%) |
Table 3 shows the unadjusted comparison of outcomes between baseline and intervention phases on each campus. Use of ASM with stress ulcer prophylaxis as the only indication decreased during the intervention phase on both campuses. There was a nonsignificant reduction in overall rates of use on both campuses, and use on discharge was unchanged. Figure 2 demonstrates the unadjusted and modeled monthly rates of admissions with at least 1 ASM order with stress ulcer prophylaxis selected as the only indication, stratified by campus. After adjusting for the admission characteristics in Table 1, during the intervention phase on both campuses there was a significant immediate reduction in the odds of receiving an ASM with stress ulcer prophylaxis selected as the only indication (East Campus odds ratio [OR]: 0.36, 95% confidence interval [CI]: 0.180.71; West Campus OR: 0.41, 95% CI: 0.280.60), and a significant change in trend compared to the baseline phase (East Campus 1.5% daily decrease in odds of receiving ASM solely for stress ulcer prophylaxis, P=0.002; West Campus 0.9% daily decrease in odds of receiving ASM solely for stress ulcer prophylaxis, P=0.02).
Study Phase | Campus | |||||
---|---|---|---|---|---|---|
East | West | |||||
Baseline, n=3,747 | Intervention, n=6,191 | P Value* | Baseline, n=11,177 | Intervention, n=5,285 | P Value* | |
| ||||||
Outcome | ||||||
Any inappropriate acid‐suppressive exposure | 4.0% | 0.6% | <0.001 | 7.7% | 2.2% | <0.001 |
Any acid‐suppressive exposure | 33.1% | 31.8% | 0.16 | 54.5% | 52.9% | 0.05 |
Discharged on acid‐suppressive medication | 18.9% | 19.6% | 0.40 | 34.7% | 34.7% | 0.95 |
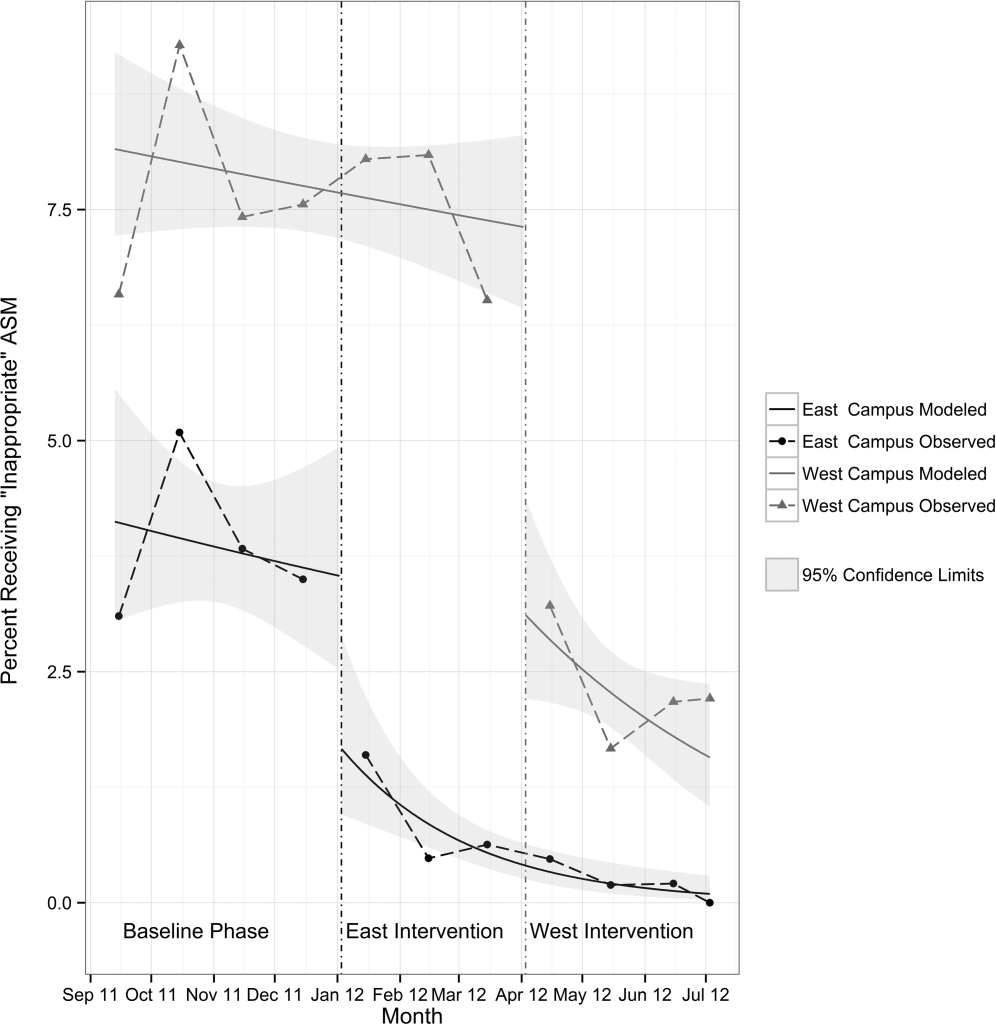
DISCUSSION
In this single‐center study, we found that a computerized CDS intervention resulted in a significant reduction in use of ASM for the sole purpose of stress ulcer prophylaxis in patients outside the ICU, a nonsignificant reduction in overall use, and no change in use on discharge. We found low rates of use for the isolated purpose of stress ulcer prophylaxis even before the intervention, and continuing preadmission medication was the most commonly selected indication throughout the study.
Although overall rates of ASM use declined after the intervention, the change was not statistically significant, and was not of the same magnitude as the decline in rates of use for the purpose of stress ulcer prophylaxis. This suggests that our intervention, in part, led to substitution of 1 indication for another. The indication that increased the most after rollout on both campuses was continuing preadmission medication. There are at least 2 possibilities for this finding: (1) the intervention prompted physicians to more accurately record the indication, or (2) physicians falsified the indication in order to execute the order. To explore these possibilities, we reviewed the charts of a random sample of 100 admissions during each of the baseline and intervention phases where continuing preadmission medication was selected as an indication for an ASM order. We found that 6/100 orders in the baseline phase and 7/100 orders in the intervention phase incorrectly indicated that the patient was on ASM prior to admission (P=0.77). This suggests that scenario 1 above is the more likely explanation for the increased use of this indication, and that the intervention, in part, simply unmasked the true rate of use at our medical center for the isolated purpose of stress ulcer prophylaxis.
These findings have implications for others attempting to use computerized CDS to better understand physician prescribing. They suggest that information collected through computer‐based interaction with clinicians at the point of care may not always be accurate or complete. As institutions increasingly use similar interventions to drive behavior, information obtained from such interaction should be validated, and when possible, patient outcomes should be measured.
Our findings suggest that rates of ASM use for the purpose of stress ulcer prophylaxis in the hospital may have declined over the last decade. Studies demonstrating that up to 70% of inpatient use of ASM was inappropriate were conducted 5 to 10 years ago.[1, 2, 3, 4, 5] Since then, studies have demonstrated risk of nosocomial infections in patients on ASM.[9, 10, 11] It is possible that the low rate of use for stress ulcer prophylaxis in our study is attributable to awareness of the risks of these medications, and limited our ability to detect differences in overall use. It is also possible, however, that a portion of the admissions with continuation of preadmission medication as the indication were started on these medications during a prior hospitalization. Thus, some portion of preadmission use is likely to represent failed medication reconciliation during a prior discharge. In this context, hospitalization may serve as an opportunity to evaluate the indication for ASM use even when these medications show up as preadmission medications.
There are additional limitations. First, the single‐center nature limits generalizability. Second, the first phase of our study, designed to obtain baseline data on ASM use, may have led to changes in prescribing prior to implementation of our CDS tool. Additionally, we did not validate the accuracy of each of the chosen indications, or the site of initial prescription in the case of preadmission exposure. Last, our study was not powered to investigate changes in rates of nosocomial gastrointestinal bleeding or nosocomial pneumonia owing to the infrequent nature of these complications.
In conclusion, we designed a simple computerized CDS intervention that was associated with a reduction in ASM use for stress ulcer prophylaxis in patients outside the ICU, a nonsignificant reduction in overall use, and no change in use on discharge. The majority of inpatient use represented continuation of preadmission medication, suggesting that interventions to improve the appropriateness of ASM prescribing should span the continuum of care. Future studies should investigate whether it is worthwhile and appropriate to reevaluate continued use of preadmission ASM during an inpatient stay.
Acknowledgements
The authors acknowledge Joshua Guthermann, MBA, and Jane Hui Chen Lim, MBA, for their assistance in the early phases of data analysis, and Long H. Ngo, PhD, for his statistical consultation.
Disclosures: Dr. Herzig was funded by a Young Clinician Research Award from the Center for Integration of Medicine and Innovative Technology, a nonprofit consortium of Boston teaching hospitals and universities, and grant number K23AG042459 from the National Institute on Aging. Dr. Marcantonio was funded by grant number K24AG035075 from the National Institute on Aging. The funding organizations had no involvement in any aspect of the study, including design, conduct, and reporting of the study. Dr. Herzig had full access to all of the data in the study and takes responsibility for the integrity of the data and the accuracy of the data analysis. Drs. Herzig and Marcantonio were responsible for the study concept and design. Drs. Herzig, Feinbloom, Howell, and Ms. Adra and Mr. Afonso were responsible for the acquisition of data. Drs. Herzig, Howell, Marcantonio, and Mr. Guess were responsible for the analysis and interpretation of the data. Dr. Herzig drafted the manuscript. All of the authors participated in the critical revision of the manuscript for important intellectual content. Drs. Herzig and Marcantonio were responsible for study supervision. The authors report no conflicts of interest.
- Stress ulcer prophylaxis in hospitalized patients not in intensive care units. Am J Health Syst Pharm. 2007;64(13):1396–1400. , .
- Magnitude and economic impact of inappropriate use of stress ulcer prophylaxis in non‐ICU hospitalized patients. Am J Gastroenterol. 2006;101(10):2200–2205. , .
- Stress‐ulcer prophylaxis for general medical patients: a review of the evidence. J Hosp Med. 2007;2(2):86–92. , .
- Hospital use of acid‐suppressive medications and its fall‐out on prescribing in general practice: a 1‐month survey. Aliment Pharmacol Ther. 2003;17(12):1503–1506. , , , et al.
- Inadequate use of acid‐suppressive therapy in hospitalized patients and its implications for general practice. Dig Dis Sci. 2005;50(12):2307–2311. , , , , , .
- Brief report: reducing inappropriate usage of stress ulcer prophylaxis among internal medicine residents. A practice‐based educational intervention. J Gen Intern Med. 2006;21(5):498–500. , .
- Inappropriate continuation of stress ulcer prophylactic therapy after discharge. Ann Pharmacother. 2007;41(10):1611–1616. , , .
- Choosing wisely in adult hospital medicine: five opportunities for improved healthcare value. J Hosp Med. 2013;8(9):486–492. , , , et al.
- Risk of Clostridium difficile diarrhea among hospital inpatients prescribed proton pump inhibitors: cohort and case‐control studies. CMAJ. 2004;171(1):33–38. , , , , .
- Iatrogenic gastric acid suppression and the risk of nosocomial Clostridium difficile infection. Arch Intern Med. 2010;170(9):784–790. , , , et al.
- Acid‐suppressive medication use and the risk for hospital‐acquired pneumonia. JAMA. 2009;301(20):2120–2128. , , , .
- Healthcare Cost and Utilization Project. Clinical classifications software (CCS) for ICD‐9‐CM. December 2009. Agency for Healthcare Research and Quality, Rockville, MD. Available at: www.hcup‐us.ahrq.gov/toolssoftware/ccs/ccs.jsp. Accessed June 18, 2014.
- Stress ulcer prophylaxis in hospitalized patients not in intensive care units. Am J Health Syst Pharm. 2007;64(13):1396–1400. , .
- Magnitude and economic impact of inappropriate use of stress ulcer prophylaxis in non‐ICU hospitalized patients. Am J Gastroenterol. 2006;101(10):2200–2205. , .
- Stress‐ulcer prophylaxis for general medical patients: a review of the evidence. J Hosp Med. 2007;2(2):86–92. , .
- Hospital use of acid‐suppressive medications and its fall‐out on prescribing in general practice: a 1‐month survey. Aliment Pharmacol Ther. 2003;17(12):1503–1506. , , , et al.
- Inadequate use of acid‐suppressive therapy in hospitalized patients and its implications for general practice. Dig Dis Sci. 2005;50(12):2307–2311. , , , , , .
- Brief report: reducing inappropriate usage of stress ulcer prophylaxis among internal medicine residents. A practice‐based educational intervention. J Gen Intern Med. 2006;21(5):498–500. , .
- Inappropriate continuation of stress ulcer prophylactic therapy after discharge. Ann Pharmacother. 2007;41(10):1611–1616. , , .
- Choosing wisely in adult hospital medicine: five opportunities for improved healthcare value. J Hosp Med. 2013;8(9):486–492. , , , et al.
- Risk of Clostridium difficile diarrhea among hospital inpatients prescribed proton pump inhibitors: cohort and case‐control studies. CMAJ. 2004;171(1):33–38. , , , , .
- Iatrogenic gastric acid suppression and the risk of nosocomial Clostridium difficile infection. Arch Intern Med. 2010;170(9):784–790. , , , et al.
- Acid‐suppressive medication use and the risk for hospital‐acquired pneumonia. JAMA. 2009;301(20):2120–2128. , , , .
- Healthcare Cost and Utilization Project. Clinical classifications software (CCS) for ICD‐9‐CM. December 2009. Agency for Healthcare Research and Quality, Rockville, MD. Available at: www.hcup‐us.ahrq.gov/toolssoftware/ccs/ccs.jsp. Accessed June 18, 2014.