User login
EHR Enhancements for Physician Assignment
Preserving continuity of care and assuring patient safety are core values of the hospitalist movement.1 Communicating clinical information to the patient, the primary care provider (PCP), and hospital‐based providers has been recognized in the hospitalist literature as an important domain of quality assurance.29 Correctly identifying a patient's inpatient physician during admission and later in the hospital course is essential to achieve these goals. This initial step has not been specifically addressed in the literature, though the complexity of this process can create significant challenges for larger hospitalist programs.
Rochester General Hospital (RGH) is a 528‐bed community teaching hospital. Our hospitalist program evolved from the general medicine unit (GMU) faculty that traditionally cared for most unreferred patients; ie, those whose PCPs had no admitting privileges. In 2004, we began covering patients of privilege‐holder partnering PCPs. Within 3 years, more than 120 privileged PCPs decided to refer all their admissions to us, though unreferred cases still make up the majority of our 10,000 annual admissions. GMU hospitalists either round as teaching attendings or attend patients on nonteaching services. Many PCPs continue to admit their own patients.
As our program began to work with numerous partnering PCPs, it became difficult to decide which patients the hospitalist team should be called to admit, and which should be admitted to the PCP. Having the emergency department (ED) providers page the PCPs or their call partners of the PCPs for attending service decisions proved infeasible and unreliable. Additionally, similar challenges emerged later in the hospitalization when patients were transferred from one service, floor, or provider to another, especially from the intensive care unit (ICU) to the medical service.
Errors regarding admissions to the hospitalist service can be classified as:
Type‐I errors: The PCP provides inpatient care but the patient is erroneously admitted to a hospitalist, creating discontinuity of care and dissatisfaction.
Type‐II errors: The PCP refers to the hospitalists but is erroneously identified as the inpatient attending physician. As the hospitalist team is not notified about these cases, admitted patients may go without physician services for a period of time.
Methods
RGH has a widely used, clinically focused EHR system that does not offer full CPOE functionality. The EHR includes a database of physicians with admitting privileges, so we decided to store information on the system regarding PCPs' hospitalist coverage. Initially, we simply uploaded a list of our partnering PCPs. However, looking up information from files proved too cumbersome for busy ED providers and hospitalists. Additionally, this solution lacked a feedback loop to alert for errors.
Therefore, we designed a system with 3 main functional elements to identify and display each patient's:
candidacy for hospitalist coverage;
actual hospitalist coverage; and
mismatches between the above statuses.
These steps are explained below, followed by a description of the system's actual utilization. In addition to the text, we demonstrate the concept of and provide detailed information on our system in Figure 1 and Table 1.
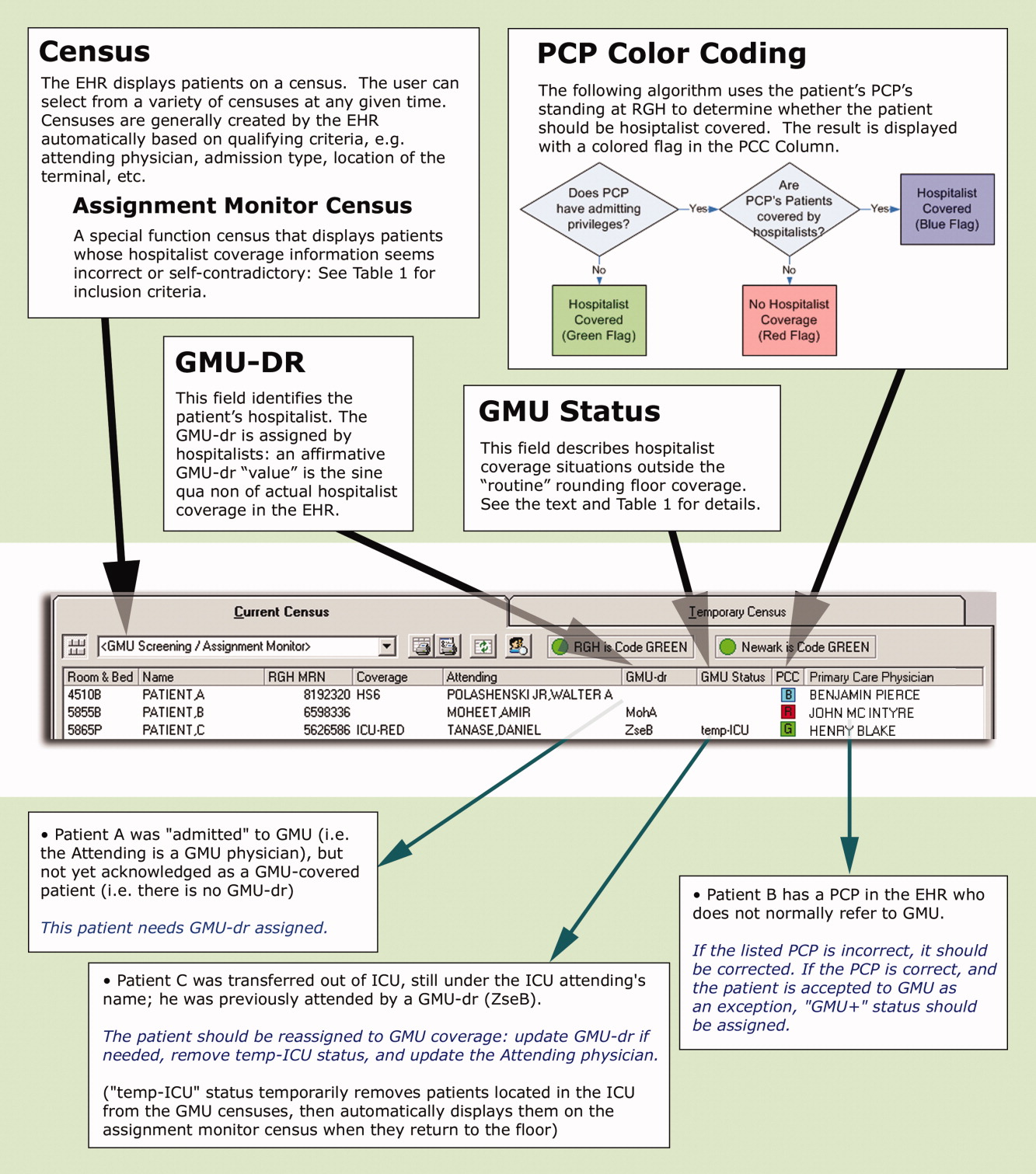
Algorithms |
This special census includes patients who meet any of the following criteria: |
Attending physician is a GMU‐MD and GMU‐dr is {blank} |
Attending physician is a GMU‐MD and GMU‐status is GMU‐cons, temp‐ICU, or non‐GMU |
Attending physician is not a GMU‐MD and GMU‐dr is not {blank} and GMU‐status is not GMU‐cons, non‐GMU, or temp‐ICU |
Attending physician is an ICU‐MD and patient's location is not ED or ICU and GMU‐status is not non‐GMU |
Patient's status is INT (internal medicine in‐bed) and PCP has no privilege or is a GMU‐PCPCP and GMU‐dr is {blank} and Attending physician is not an ICU‐MD and GMU‐status is not temp‐ICU or non‐GMU |
PCP has admission privilege and PCP is not a GMU‐PCPCP and GMU‐dr is not {blank} and GMU‐status is not non‐GMU or GMU+ |
GMU‐status is temp‐ICU and patient's location is not ED or ICU |
GMU‐dr is {blank} and GMU‐status is GMU‐cons, GMU‐ALC or GMU+ |
GMU‐dr is ‐TBA, or ‐TRD and present time is between 8 AMand 4 PM |
Definitions |
Attending physician and PCP refers to the physicians assigned as attending and PCP, respectively in the core EHR system. |
Physician categories are defined by listing all belonging members on centrally maintained, up‐to‐date databases: |
GMU‐PCPCP: partnering community PCP physicians who refer their patients to the GMU hospitalist service |
GMU‐MD: a GMU hospitalist attending physician |
ICU‐MD: a critical care physician who attends in ICU, and automatically transfers most patients to hospitalists upon discharge from ICU |
GMU‐dr signals acceptance to GMU hospitalist service, and names the hospitalist in the EHR. Temporary assignments (TBA or TRD) are used when the patient is accepted to the hospitalist program, but the rounding physician is not yet known (eg, a patient seen by the night hospitalist in the ED, or waiting reassignment from a weekend). Options include: |
A physician's acronym (from last name and first initial) identifies the rounding hospitalist |
TBA = to be assigned: accepted to GMU but not yet assigned to a rounding attending (used for new patients during evening and night hours while under the care of the on call team) |
TRD = to redistribute from an attending's care who is leaving service (used for patients whose follow‐up coverage is distributed in the next morning by the call team) |
A blank field means no hospitalist service for the patient |
GMU‐status signals irregular rounding relationship with the patient. The following options are used: |
Non‐GMU: patient will not be covered by GMU hospitalists (eg, signing off consult, admission to subspecialist service) |
Temp‐ICU: patient will not be covered by GMU while in ICU (will automatically accept to GMU upon transfer to floor) |
GMU‐cons: patient is on consultation service (GMU is not the attending service) |
GMU‐ALC: patient is on alternative level of care (skilled nursing needs: no daily rounding by GMU hospitalist) |
GMU+: patient is covered by GMU (despite the PCP not normally referring to GMU) |
First, to identify a patient's candidacy for hospitalist coverage, we created a PCP color coding algorithm, based on whether the PCP has admitting privileges at RGH and/or has arranged hospitalist coverage for her/his patients. Whether or not hospitalist coverage is expected for a given patient's PCP is displayed on the EHR.
Second, a data field called GMU‐dr was created to describe whether the patient is actually assigned to a hospitalist. The GMU on‐call physician assigns a GMU‐dr to all appropriate patients (ie, updates the field's value). This step acknowledges hospitalist coverage for a given patient and also identifies the hospitalist physician. It simultaneously adds the patient to the appropriate rounding censuses. The GMU‐dr data field is updated every time the rounding physician changes (eg, for weekend coverage).
Third, the EHR's assignment monitor algorithm compares the expected and actual hospitalist coverage (based on PCP color coding, GMU‐dr, admission type, location, time of day, etc.) and displays mismatches on the assignment monitor census. We created this special‐function census to include patients with the more dangerous type‐II coverage errors that would not show up on our rounding censuses.
The assignment monitor census is regularly reviewed by the call physicians and should be cleared of patients. Unaccounted patients showing up on this census are handled with urgency equal to that of an unseen admission. Most patients on the assignment monitor census have missing or incorrect information: correcting that information removes the patient from this alert list. However, some patients with correct information are captured due to an unusual relationship with the hospitalist program; eg, consultation or individual exceptions from coverage arrangements. The GMU‐status field was created to explain such situations and remove these patients from the alert list.
Results
According to a survey that was distributed to the 19 eligible hospitalists and returned by 17 (89%), this system greatly improved our admission and patient distribution process, with the following results:
PCP color coding prevents type‐I and type‐II coverage identification errors more than once a week according to 94.1% and 88.2% of hospitalists, respectively. Ninety‐four percent agreed (absolutely or strongly) that color coding is a convenient tool to identify PCP referral status.
The assignment monitor identifies patients more than once a month that would have been lost in the preintervention era, according to 94.1% of the hospitalists. All hospitalists surveyed believed that this alert list had several times prevented potentially life‐threatening complications, and that the system is more useful than burdensome.
The GMU‐dr data field correctly identifies the hospitalist while the attending is misassigned in the core EHR system more than once a week, according to 93.7% of surveyed hospitalists (80% of these stated it happens daily). None believed the system would provide incorrect information with that frequency. Every hospitalist agreed that the GMU‐dr and GMU‐status tools are efficient methods for distributing patients, keeping track of attending designations, and maintaining a unit census and personal rounding censuses: cumulatively 85.7% absolutely agreed, while 12.7% strongly agreed.
We also assessed how promptly the call team responded to the assignment monitor alerts by correcting the information in the system (the census is recorded every 4 hours). Due to the intensity of the ED call, physicians often just scan this alert list (and take clinical action on the captured patients as needed) without updating the EHR fields until the end of their shifts. Measuring the speed of clearing patients from the census may grossly underestimate the actual usage of the list, though this data is useful to access a worst‐case scenario.
During the first week of September 2007 we cared for 270 patients: 52 were captured on the assignment monitor before a GMU‐dr was assigned or the patient was deemed non‐GMU. No patient was recaptured on the list more than 8 hours after the initial appearance.
Discussion
Since we enhanced a widely‐used program, our intervention required minimal training. As our innovation was designed and underwent trial by hospitalists, immediate feedback assured user‐friendly implementation, good acceptance, and improved workflow.
Color coding eliminated the time‐intensive and error‐prone lookup of coverage arrangements.
Updating GMU‐dr and GMU‐status in the EHR takes very little time and provides immediate benefits (eg, clearly defined rounding censuses). This task has been integrated into our signout tool, making these functions even more intuitive.
Using the assignment monitor census is fundamental for patient safety, but correcting EHR information creates some additional work for busy call physicians. Implementing this step required active change‐management: writing policies, designing metrics, and considering incentives. The call physicians (3 shifts per day) are officially responsible for patient distribution, including clearing the screening census before passing the call pager to the next shift.
We identified some potential limitations, though these issues generally apply to any information technology (IT) implementation: Setting up the program requires an adaptable EHR and close collaboration with the IT department. The system's accuracy depends on correctly identifying the patient's PCP in the EHR. Maintaining and coordinating the data regarding PCP privileges and hospitalist coverage requires a central database has been created.
Meanwhile, we found these tools very useful in solving problems beyond patient distribution:
PCP color coding can export information to other applications about hospitalist coverage and assist ED and nonmedical services to contact the proper medical service for admissions and consults.
GMU‐dr and GMU‐status can be used to create personal rounding censuses, provide billing lists to third‐party applications, and support proprietary applications, thus assisting patient distribution decisions and guiding hospital staff to call the patient's correct provider 24/7.
Special function censuses (defined by algorithms) are now used as alert lists for different patient issues (eg, observational patients staying beyond their allotted time) and by nonhospitalist services.
We presented hospitalist‐specific EHR concepts (patient coverage algorithms, special‐function censuses, and patient tracking by provider‐entered information) and their specific applications in our hospital. We believe our tools can be implemented in various locations and EHRs. Though the challenges may differ from setting to setting (eg, patient distribution between coexistent hospitalist programs, or responding to limitations of resident duty hours), these solutions are highly adaptable and have the potential of providing additional benefits beyond hospitalist coverage issues.
Acknowledgements
The authors thank Andrew Hakes for his invaluable assistance in graphic design; the authors also thank their information technology (IT) engineers and staff members, who programmed and are maintaining these functions in their EHR system, for their enthusiastic collaboration and for their assistance with the manuscript: Rich Prideaux, Robert Cawlfield, Kim Johnston, Brenda Ventrillo, and Katura Gardner.
- The core competencies in hospital medicine.J Hosp Med.2006;1(Suppl 1):84–95. , , , , .
- Continuity of care and patient outcomes after hospital discharge.J Gen Intern Med.2004;19(6):624–631. , , , .
- The “continuity visit” and the hospitalist model of care.Am J Med.2001;111(9B):40S–42S. , .
- Deficits in communication transfer between hospital‐based and primary care physicians: implications for patient safety and continuity of care.JAMA.2007;297:831–841. , , , , , .
- Passing the clinical baton: 6 principles to guide the hospitalist.Am J Med.2001;111(9B):36S–39S. , , .
- The importance of postdischarge telephone follow‐up for hospitalists: a view from the trenches.Am J Med.2001;111(9B):43S–44S. .
- The language of quality improvement: therapy classes.J Hosp Med.2006;1:327–330. .
- Executing high‐quality care transitions: a call to do it right.J Hosp Med.2007;2:287–290. , .
- Promoting effective transitions of care at hospital discharge: a review of key issues for hospitalists.J Hosp Med.2007;2:314–323. , , , .
Preserving continuity of care and assuring patient safety are core values of the hospitalist movement.1 Communicating clinical information to the patient, the primary care provider (PCP), and hospital‐based providers has been recognized in the hospitalist literature as an important domain of quality assurance.29 Correctly identifying a patient's inpatient physician during admission and later in the hospital course is essential to achieve these goals. This initial step has not been specifically addressed in the literature, though the complexity of this process can create significant challenges for larger hospitalist programs.
Rochester General Hospital (RGH) is a 528‐bed community teaching hospital. Our hospitalist program evolved from the general medicine unit (GMU) faculty that traditionally cared for most unreferred patients; ie, those whose PCPs had no admitting privileges. In 2004, we began covering patients of privilege‐holder partnering PCPs. Within 3 years, more than 120 privileged PCPs decided to refer all their admissions to us, though unreferred cases still make up the majority of our 10,000 annual admissions. GMU hospitalists either round as teaching attendings or attend patients on nonteaching services. Many PCPs continue to admit their own patients.
As our program began to work with numerous partnering PCPs, it became difficult to decide which patients the hospitalist team should be called to admit, and which should be admitted to the PCP. Having the emergency department (ED) providers page the PCPs or their call partners of the PCPs for attending service decisions proved infeasible and unreliable. Additionally, similar challenges emerged later in the hospitalization when patients were transferred from one service, floor, or provider to another, especially from the intensive care unit (ICU) to the medical service.
Errors regarding admissions to the hospitalist service can be classified as:
Type‐I errors: The PCP provides inpatient care but the patient is erroneously admitted to a hospitalist, creating discontinuity of care and dissatisfaction.
Type‐II errors: The PCP refers to the hospitalists but is erroneously identified as the inpatient attending physician. As the hospitalist team is not notified about these cases, admitted patients may go without physician services for a period of time.
Methods
RGH has a widely used, clinically focused EHR system that does not offer full CPOE functionality. The EHR includes a database of physicians with admitting privileges, so we decided to store information on the system regarding PCPs' hospitalist coverage. Initially, we simply uploaded a list of our partnering PCPs. However, looking up information from files proved too cumbersome for busy ED providers and hospitalists. Additionally, this solution lacked a feedback loop to alert for errors.
Therefore, we designed a system with 3 main functional elements to identify and display each patient's:
candidacy for hospitalist coverage;
actual hospitalist coverage; and
mismatches between the above statuses.
These steps are explained below, followed by a description of the system's actual utilization. In addition to the text, we demonstrate the concept of and provide detailed information on our system in Figure 1 and Table 1.
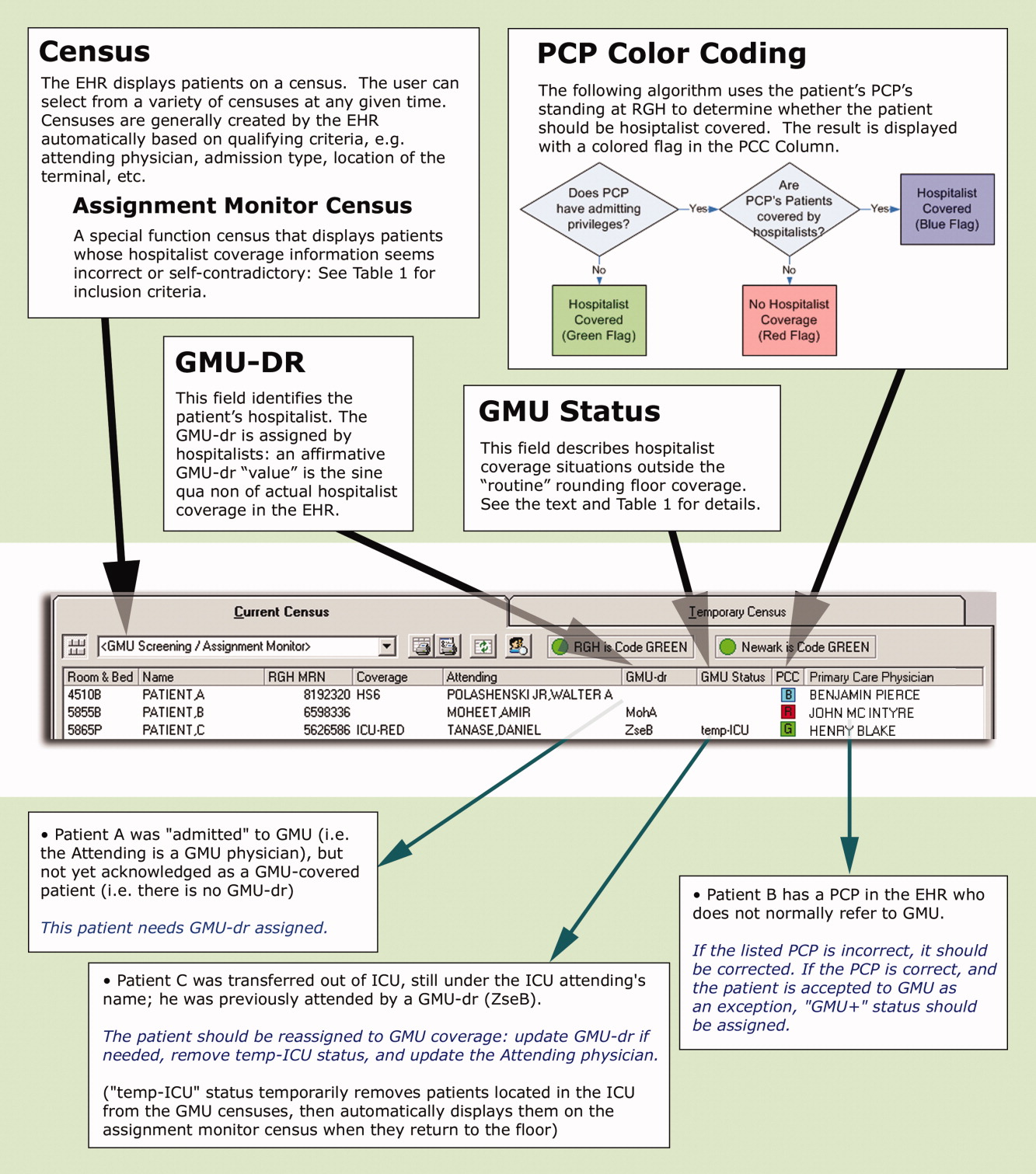
Algorithms |
This special census includes patients who meet any of the following criteria: |
Attending physician is a GMU‐MD and GMU‐dr is {blank} |
Attending physician is a GMU‐MD and GMU‐status is GMU‐cons, temp‐ICU, or non‐GMU |
Attending physician is not a GMU‐MD and GMU‐dr is not {blank} and GMU‐status is not GMU‐cons, non‐GMU, or temp‐ICU |
Attending physician is an ICU‐MD and patient's location is not ED or ICU and GMU‐status is not non‐GMU |
Patient's status is INT (internal medicine in‐bed) and PCP has no privilege or is a GMU‐PCPCP and GMU‐dr is {blank} and Attending physician is not an ICU‐MD and GMU‐status is not temp‐ICU or non‐GMU |
PCP has admission privilege and PCP is not a GMU‐PCPCP and GMU‐dr is not {blank} and GMU‐status is not non‐GMU or GMU+ |
GMU‐status is temp‐ICU and patient's location is not ED or ICU |
GMU‐dr is {blank} and GMU‐status is GMU‐cons, GMU‐ALC or GMU+ |
GMU‐dr is ‐TBA, or ‐TRD and present time is between 8 AMand 4 PM |
Definitions |
Attending physician and PCP refers to the physicians assigned as attending and PCP, respectively in the core EHR system. |
Physician categories are defined by listing all belonging members on centrally maintained, up‐to‐date databases: |
GMU‐PCPCP: partnering community PCP physicians who refer their patients to the GMU hospitalist service |
GMU‐MD: a GMU hospitalist attending physician |
ICU‐MD: a critical care physician who attends in ICU, and automatically transfers most patients to hospitalists upon discharge from ICU |
GMU‐dr signals acceptance to GMU hospitalist service, and names the hospitalist in the EHR. Temporary assignments (TBA or TRD) are used when the patient is accepted to the hospitalist program, but the rounding physician is not yet known (eg, a patient seen by the night hospitalist in the ED, or waiting reassignment from a weekend). Options include: |
A physician's acronym (from last name and first initial) identifies the rounding hospitalist |
TBA = to be assigned: accepted to GMU but not yet assigned to a rounding attending (used for new patients during evening and night hours while under the care of the on call team) |
TRD = to redistribute from an attending's care who is leaving service (used for patients whose follow‐up coverage is distributed in the next morning by the call team) |
A blank field means no hospitalist service for the patient |
GMU‐status signals irregular rounding relationship with the patient. The following options are used: |
Non‐GMU: patient will not be covered by GMU hospitalists (eg, signing off consult, admission to subspecialist service) |
Temp‐ICU: patient will not be covered by GMU while in ICU (will automatically accept to GMU upon transfer to floor) |
GMU‐cons: patient is on consultation service (GMU is not the attending service) |
GMU‐ALC: patient is on alternative level of care (skilled nursing needs: no daily rounding by GMU hospitalist) |
GMU+: patient is covered by GMU (despite the PCP not normally referring to GMU) |
First, to identify a patient's candidacy for hospitalist coverage, we created a PCP color coding algorithm, based on whether the PCP has admitting privileges at RGH and/or has arranged hospitalist coverage for her/his patients. Whether or not hospitalist coverage is expected for a given patient's PCP is displayed on the EHR.
Second, a data field called GMU‐dr was created to describe whether the patient is actually assigned to a hospitalist. The GMU on‐call physician assigns a GMU‐dr to all appropriate patients (ie, updates the field's value). This step acknowledges hospitalist coverage for a given patient and also identifies the hospitalist physician. It simultaneously adds the patient to the appropriate rounding censuses. The GMU‐dr data field is updated every time the rounding physician changes (eg, for weekend coverage).
Third, the EHR's assignment monitor algorithm compares the expected and actual hospitalist coverage (based on PCP color coding, GMU‐dr, admission type, location, time of day, etc.) and displays mismatches on the assignment monitor census. We created this special‐function census to include patients with the more dangerous type‐II coverage errors that would not show up on our rounding censuses.
The assignment monitor census is regularly reviewed by the call physicians and should be cleared of patients. Unaccounted patients showing up on this census are handled with urgency equal to that of an unseen admission. Most patients on the assignment monitor census have missing or incorrect information: correcting that information removes the patient from this alert list. However, some patients with correct information are captured due to an unusual relationship with the hospitalist program; eg, consultation or individual exceptions from coverage arrangements. The GMU‐status field was created to explain such situations and remove these patients from the alert list.
Results
According to a survey that was distributed to the 19 eligible hospitalists and returned by 17 (89%), this system greatly improved our admission and patient distribution process, with the following results:
PCP color coding prevents type‐I and type‐II coverage identification errors more than once a week according to 94.1% and 88.2% of hospitalists, respectively. Ninety‐four percent agreed (absolutely or strongly) that color coding is a convenient tool to identify PCP referral status.
The assignment monitor identifies patients more than once a month that would have been lost in the preintervention era, according to 94.1% of the hospitalists. All hospitalists surveyed believed that this alert list had several times prevented potentially life‐threatening complications, and that the system is more useful than burdensome.
The GMU‐dr data field correctly identifies the hospitalist while the attending is misassigned in the core EHR system more than once a week, according to 93.7% of surveyed hospitalists (80% of these stated it happens daily). None believed the system would provide incorrect information with that frequency. Every hospitalist agreed that the GMU‐dr and GMU‐status tools are efficient methods for distributing patients, keeping track of attending designations, and maintaining a unit census and personal rounding censuses: cumulatively 85.7% absolutely agreed, while 12.7% strongly agreed.
We also assessed how promptly the call team responded to the assignment monitor alerts by correcting the information in the system (the census is recorded every 4 hours). Due to the intensity of the ED call, physicians often just scan this alert list (and take clinical action on the captured patients as needed) without updating the EHR fields until the end of their shifts. Measuring the speed of clearing patients from the census may grossly underestimate the actual usage of the list, though this data is useful to access a worst‐case scenario.
During the first week of September 2007 we cared for 270 patients: 52 were captured on the assignment monitor before a GMU‐dr was assigned or the patient was deemed non‐GMU. No patient was recaptured on the list more than 8 hours after the initial appearance.
Discussion
Since we enhanced a widely‐used program, our intervention required minimal training. As our innovation was designed and underwent trial by hospitalists, immediate feedback assured user‐friendly implementation, good acceptance, and improved workflow.
Color coding eliminated the time‐intensive and error‐prone lookup of coverage arrangements.
Updating GMU‐dr and GMU‐status in the EHR takes very little time and provides immediate benefits (eg, clearly defined rounding censuses). This task has been integrated into our signout tool, making these functions even more intuitive.
Using the assignment monitor census is fundamental for patient safety, but correcting EHR information creates some additional work for busy call physicians. Implementing this step required active change‐management: writing policies, designing metrics, and considering incentives. The call physicians (3 shifts per day) are officially responsible for patient distribution, including clearing the screening census before passing the call pager to the next shift.
We identified some potential limitations, though these issues generally apply to any information technology (IT) implementation: Setting up the program requires an adaptable EHR and close collaboration with the IT department. The system's accuracy depends on correctly identifying the patient's PCP in the EHR. Maintaining and coordinating the data regarding PCP privileges and hospitalist coverage requires a central database has been created.
Meanwhile, we found these tools very useful in solving problems beyond patient distribution:
PCP color coding can export information to other applications about hospitalist coverage and assist ED and nonmedical services to contact the proper medical service for admissions and consults.
GMU‐dr and GMU‐status can be used to create personal rounding censuses, provide billing lists to third‐party applications, and support proprietary applications, thus assisting patient distribution decisions and guiding hospital staff to call the patient's correct provider 24/7.
Special function censuses (defined by algorithms) are now used as alert lists for different patient issues (eg, observational patients staying beyond their allotted time) and by nonhospitalist services.
We presented hospitalist‐specific EHR concepts (patient coverage algorithms, special‐function censuses, and patient tracking by provider‐entered information) and their specific applications in our hospital. We believe our tools can be implemented in various locations and EHRs. Though the challenges may differ from setting to setting (eg, patient distribution between coexistent hospitalist programs, or responding to limitations of resident duty hours), these solutions are highly adaptable and have the potential of providing additional benefits beyond hospitalist coverage issues.
Acknowledgements
The authors thank Andrew Hakes for his invaluable assistance in graphic design; the authors also thank their information technology (IT) engineers and staff members, who programmed and are maintaining these functions in their EHR system, for their enthusiastic collaboration and for their assistance with the manuscript: Rich Prideaux, Robert Cawlfield, Kim Johnston, Brenda Ventrillo, and Katura Gardner.
Preserving continuity of care and assuring patient safety are core values of the hospitalist movement.1 Communicating clinical information to the patient, the primary care provider (PCP), and hospital‐based providers has been recognized in the hospitalist literature as an important domain of quality assurance.29 Correctly identifying a patient's inpatient physician during admission and later in the hospital course is essential to achieve these goals. This initial step has not been specifically addressed in the literature, though the complexity of this process can create significant challenges for larger hospitalist programs.
Rochester General Hospital (RGH) is a 528‐bed community teaching hospital. Our hospitalist program evolved from the general medicine unit (GMU) faculty that traditionally cared for most unreferred patients; ie, those whose PCPs had no admitting privileges. In 2004, we began covering patients of privilege‐holder partnering PCPs. Within 3 years, more than 120 privileged PCPs decided to refer all their admissions to us, though unreferred cases still make up the majority of our 10,000 annual admissions. GMU hospitalists either round as teaching attendings or attend patients on nonteaching services. Many PCPs continue to admit their own patients.
As our program began to work with numerous partnering PCPs, it became difficult to decide which patients the hospitalist team should be called to admit, and which should be admitted to the PCP. Having the emergency department (ED) providers page the PCPs or their call partners of the PCPs for attending service decisions proved infeasible and unreliable. Additionally, similar challenges emerged later in the hospitalization when patients were transferred from one service, floor, or provider to another, especially from the intensive care unit (ICU) to the medical service.
Errors regarding admissions to the hospitalist service can be classified as:
Type‐I errors: The PCP provides inpatient care but the patient is erroneously admitted to a hospitalist, creating discontinuity of care and dissatisfaction.
Type‐II errors: The PCP refers to the hospitalists but is erroneously identified as the inpatient attending physician. As the hospitalist team is not notified about these cases, admitted patients may go without physician services for a period of time.
Methods
RGH has a widely used, clinically focused EHR system that does not offer full CPOE functionality. The EHR includes a database of physicians with admitting privileges, so we decided to store information on the system regarding PCPs' hospitalist coverage. Initially, we simply uploaded a list of our partnering PCPs. However, looking up information from files proved too cumbersome for busy ED providers and hospitalists. Additionally, this solution lacked a feedback loop to alert for errors.
Therefore, we designed a system with 3 main functional elements to identify and display each patient's:
candidacy for hospitalist coverage;
actual hospitalist coverage; and
mismatches between the above statuses.
These steps are explained below, followed by a description of the system's actual utilization. In addition to the text, we demonstrate the concept of and provide detailed information on our system in Figure 1 and Table 1.
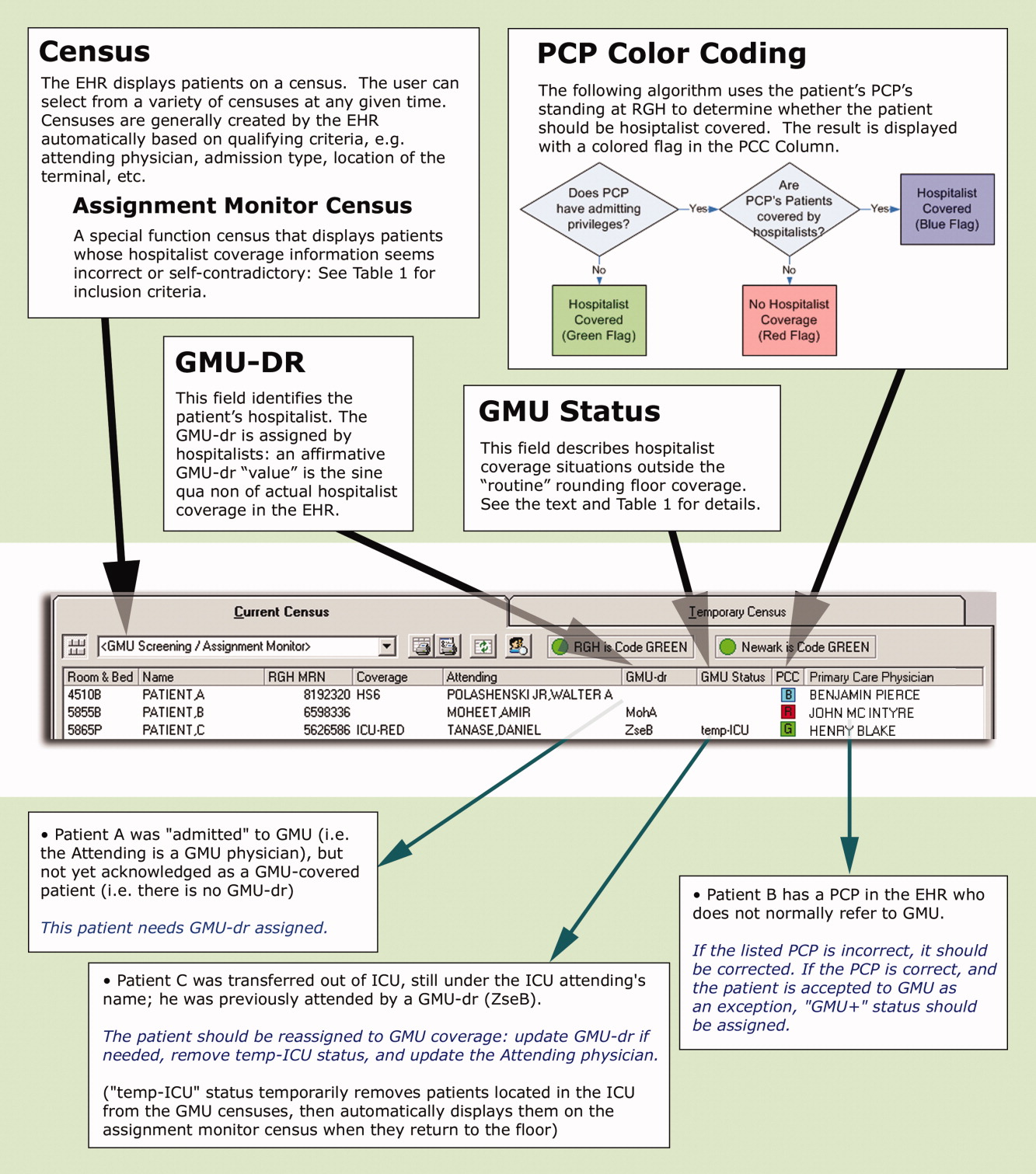
Algorithms |
This special census includes patients who meet any of the following criteria: |
Attending physician is a GMU‐MD and GMU‐dr is {blank} |
Attending physician is a GMU‐MD and GMU‐status is GMU‐cons, temp‐ICU, or non‐GMU |
Attending physician is not a GMU‐MD and GMU‐dr is not {blank} and GMU‐status is not GMU‐cons, non‐GMU, or temp‐ICU |
Attending physician is an ICU‐MD and patient's location is not ED or ICU and GMU‐status is not non‐GMU |
Patient's status is INT (internal medicine in‐bed) and PCP has no privilege or is a GMU‐PCPCP and GMU‐dr is {blank} and Attending physician is not an ICU‐MD and GMU‐status is not temp‐ICU or non‐GMU |
PCP has admission privilege and PCP is not a GMU‐PCPCP and GMU‐dr is not {blank} and GMU‐status is not non‐GMU or GMU+ |
GMU‐status is temp‐ICU and patient's location is not ED or ICU |
GMU‐dr is {blank} and GMU‐status is GMU‐cons, GMU‐ALC or GMU+ |
GMU‐dr is ‐TBA, or ‐TRD and present time is between 8 AMand 4 PM |
Definitions |
Attending physician and PCP refers to the physicians assigned as attending and PCP, respectively in the core EHR system. |
Physician categories are defined by listing all belonging members on centrally maintained, up‐to‐date databases: |
GMU‐PCPCP: partnering community PCP physicians who refer their patients to the GMU hospitalist service |
GMU‐MD: a GMU hospitalist attending physician |
ICU‐MD: a critical care physician who attends in ICU, and automatically transfers most patients to hospitalists upon discharge from ICU |
GMU‐dr signals acceptance to GMU hospitalist service, and names the hospitalist in the EHR. Temporary assignments (TBA or TRD) are used when the patient is accepted to the hospitalist program, but the rounding physician is not yet known (eg, a patient seen by the night hospitalist in the ED, or waiting reassignment from a weekend). Options include: |
A physician's acronym (from last name and first initial) identifies the rounding hospitalist |
TBA = to be assigned: accepted to GMU but not yet assigned to a rounding attending (used for new patients during evening and night hours while under the care of the on call team) |
TRD = to redistribute from an attending's care who is leaving service (used for patients whose follow‐up coverage is distributed in the next morning by the call team) |
A blank field means no hospitalist service for the patient |
GMU‐status signals irregular rounding relationship with the patient. The following options are used: |
Non‐GMU: patient will not be covered by GMU hospitalists (eg, signing off consult, admission to subspecialist service) |
Temp‐ICU: patient will not be covered by GMU while in ICU (will automatically accept to GMU upon transfer to floor) |
GMU‐cons: patient is on consultation service (GMU is not the attending service) |
GMU‐ALC: patient is on alternative level of care (skilled nursing needs: no daily rounding by GMU hospitalist) |
GMU+: patient is covered by GMU (despite the PCP not normally referring to GMU) |
First, to identify a patient's candidacy for hospitalist coverage, we created a PCP color coding algorithm, based on whether the PCP has admitting privileges at RGH and/or has arranged hospitalist coverage for her/his patients. Whether or not hospitalist coverage is expected for a given patient's PCP is displayed on the EHR.
Second, a data field called GMU‐dr was created to describe whether the patient is actually assigned to a hospitalist. The GMU on‐call physician assigns a GMU‐dr to all appropriate patients (ie, updates the field's value). This step acknowledges hospitalist coverage for a given patient and also identifies the hospitalist physician. It simultaneously adds the patient to the appropriate rounding censuses. The GMU‐dr data field is updated every time the rounding physician changes (eg, for weekend coverage).
Third, the EHR's assignment monitor algorithm compares the expected and actual hospitalist coverage (based on PCP color coding, GMU‐dr, admission type, location, time of day, etc.) and displays mismatches on the assignment monitor census. We created this special‐function census to include patients with the more dangerous type‐II coverage errors that would not show up on our rounding censuses.
The assignment monitor census is regularly reviewed by the call physicians and should be cleared of patients. Unaccounted patients showing up on this census are handled with urgency equal to that of an unseen admission. Most patients on the assignment monitor census have missing or incorrect information: correcting that information removes the patient from this alert list. However, some patients with correct information are captured due to an unusual relationship with the hospitalist program; eg, consultation or individual exceptions from coverage arrangements. The GMU‐status field was created to explain such situations and remove these patients from the alert list.
Results
According to a survey that was distributed to the 19 eligible hospitalists and returned by 17 (89%), this system greatly improved our admission and patient distribution process, with the following results:
PCP color coding prevents type‐I and type‐II coverage identification errors more than once a week according to 94.1% and 88.2% of hospitalists, respectively. Ninety‐four percent agreed (absolutely or strongly) that color coding is a convenient tool to identify PCP referral status.
The assignment monitor identifies patients more than once a month that would have been lost in the preintervention era, according to 94.1% of the hospitalists. All hospitalists surveyed believed that this alert list had several times prevented potentially life‐threatening complications, and that the system is more useful than burdensome.
The GMU‐dr data field correctly identifies the hospitalist while the attending is misassigned in the core EHR system more than once a week, according to 93.7% of surveyed hospitalists (80% of these stated it happens daily). None believed the system would provide incorrect information with that frequency. Every hospitalist agreed that the GMU‐dr and GMU‐status tools are efficient methods for distributing patients, keeping track of attending designations, and maintaining a unit census and personal rounding censuses: cumulatively 85.7% absolutely agreed, while 12.7% strongly agreed.
We also assessed how promptly the call team responded to the assignment monitor alerts by correcting the information in the system (the census is recorded every 4 hours). Due to the intensity of the ED call, physicians often just scan this alert list (and take clinical action on the captured patients as needed) without updating the EHR fields until the end of their shifts. Measuring the speed of clearing patients from the census may grossly underestimate the actual usage of the list, though this data is useful to access a worst‐case scenario.
During the first week of September 2007 we cared for 270 patients: 52 were captured on the assignment monitor before a GMU‐dr was assigned or the patient was deemed non‐GMU. No patient was recaptured on the list more than 8 hours after the initial appearance.
Discussion
Since we enhanced a widely‐used program, our intervention required minimal training. As our innovation was designed and underwent trial by hospitalists, immediate feedback assured user‐friendly implementation, good acceptance, and improved workflow.
Color coding eliminated the time‐intensive and error‐prone lookup of coverage arrangements.
Updating GMU‐dr and GMU‐status in the EHR takes very little time and provides immediate benefits (eg, clearly defined rounding censuses). This task has been integrated into our signout tool, making these functions even more intuitive.
Using the assignment monitor census is fundamental for patient safety, but correcting EHR information creates some additional work for busy call physicians. Implementing this step required active change‐management: writing policies, designing metrics, and considering incentives. The call physicians (3 shifts per day) are officially responsible for patient distribution, including clearing the screening census before passing the call pager to the next shift.
We identified some potential limitations, though these issues generally apply to any information technology (IT) implementation: Setting up the program requires an adaptable EHR and close collaboration with the IT department. The system's accuracy depends on correctly identifying the patient's PCP in the EHR. Maintaining and coordinating the data regarding PCP privileges and hospitalist coverage requires a central database has been created.
Meanwhile, we found these tools very useful in solving problems beyond patient distribution:
PCP color coding can export information to other applications about hospitalist coverage and assist ED and nonmedical services to contact the proper medical service for admissions and consults.
GMU‐dr and GMU‐status can be used to create personal rounding censuses, provide billing lists to third‐party applications, and support proprietary applications, thus assisting patient distribution decisions and guiding hospital staff to call the patient's correct provider 24/7.
Special function censuses (defined by algorithms) are now used as alert lists for different patient issues (eg, observational patients staying beyond their allotted time) and by nonhospitalist services.
We presented hospitalist‐specific EHR concepts (patient coverage algorithms, special‐function censuses, and patient tracking by provider‐entered information) and their specific applications in our hospital. We believe our tools can be implemented in various locations and EHRs. Though the challenges may differ from setting to setting (eg, patient distribution between coexistent hospitalist programs, or responding to limitations of resident duty hours), these solutions are highly adaptable and have the potential of providing additional benefits beyond hospitalist coverage issues.
Acknowledgements
The authors thank Andrew Hakes for his invaluable assistance in graphic design; the authors also thank their information technology (IT) engineers and staff members, who programmed and are maintaining these functions in their EHR system, for their enthusiastic collaboration and for their assistance with the manuscript: Rich Prideaux, Robert Cawlfield, Kim Johnston, Brenda Ventrillo, and Katura Gardner.
- The core competencies in hospital medicine.J Hosp Med.2006;1(Suppl 1):84–95. , , , , .
- Continuity of care and patient outcomes after hospital discharge.J Gen Intern Med.2004;19(6):624–631. , , , .
- The “continuity visit” and the hospitalist model of care.Am J Med.2001;111(9B):40S–42S. , .
- Deficits in communication transfer between hospital‐based and primary care physicians: implications for patient safety and continuity of care.JAMA.2007;297:831–841. , , , , , .
- Passing the clinical baton: 6 principles to guide the hospitalist.Am J Med.2001;111(9B):36S–39S. , , .
- The importance of postdischarge telephone follow‐up for hospitalists: a view from the trenches.Am J Med.2001;111(9B):43S–44S. .
- The language of quality improvement: therapy classes.J Hosp Med.2006;1:327–330. .
- Executing high‐quality care transitions: a call to do it right.J Hosp Med.2007;2:287–290. , .
- Promoting effective transitions of care at hospital discharge: a review of key issues for hospitalists.J Hosp Med.2007;2:314–323. , , , .
- The core competencies in hospital medicine.J Hosp Med.2006;1(Suppl 1):84–95. , , , , .
- Continuity of care and patient outcomes after hospital discharge.J Gen Intern Med.2004;19(6):624–631. , , , .
- The “continuity visit” and the hospitalist model of care.Am J Med.2001;111(9B):40S–42S. , .
- Deficits in communication transfer between hospital‐based and primary care physicians: implications for patient safety and continuity of care.JAMA.2007;297:831–841. , , , , , .
- Passing the clinical baton: 6 principles to guide the hospitalist.Am J Med.2001;111(9B):36S–39S. , , .
- The importance of postdischarge telephone follow‐up for hospitalists: a view from the trenches.Am J Med.2001;111(9B):43S–44S. .
- The language of quality improvement: therapy classes.J Hosp Med.2006;1:327–330. .
- Executing high‐quality care transitions: a call to do it right.J Hosp Med.2007;2:287–290. , .
- Promoting effective transitions of care at hospital discharge: a review of key issues for hospitalists.J Hosp Med.2007;2:314–323. , , , .
Copyright © 2009 Society of Hospital Medicine
In the Literature
Hematocrit and Perioperative Mortality
Wu WC, Schifftner TL, Henderson WG, et al. Preoperative hematocrit levels and postoperative outcomes in older patients undergoing noncardiac surgery. JAMA. 2007 Jun 13;297(22):2481-2488.
Several studies have outlined the risk of preoperative anemia prior to noncardiac surgery in elderly patients. These studies have not linked anemia to risk of death unless cardiac disease is present.
Anemia management remains a challenge for many hospitals and is the most important predictor of the need for blood transfusion. Transfusion increases morbidity and mortality in the perioperative setting. At the same time, little is known about the risks of polycythemia in this setting.
This retrospective cohort study used the Veterans’ Affairs National Surgical Quality Improvement Program database of 310,311 veterans 65 or older from 132 VA hospitals. It explores the relationship between abnormal levels of hematocrit and adverse events among elderly surgical patients.
The data suggest an incremental relationship between positive and negative deviation of hematocrit levels with 30-day postoperative mortality in patients 65 and older. Specifically, the study found a 1.6% increase (95% confidence interval, 1.1%-2.2%) in 30-day mortality for every percentage point of increase or decrease in hematocrit from the normal range.
Because this is an observational study of anemia and adverse events, no causal relationship can be established from this data. Hospitalists involved in perioperative care should be careful about drawing conclusions from this study alone and should not necessarily plan interventions to treat abnormal levels of hematocrit without carefully considering the risks and benefits of intervention.
Prognostic Utility of Pre-operative BNP
Feringa HH, Schouten O, Dunkelgrun M, et al. Plasma N-terminal pro-B-type natriuretic peptide as long term prognostic marker after major vascular surgery. Heart. 2007 Feb;93(2):226-231.
Traditional stratification of patients at high risk for cardiac complications and undergoing noncardiac surgery has included clinical risk index scoring and pre-operative stress testing. It is unclear if cardiac biomarkers can be used in conjunction with these measures to improve the identification of patients at risk.
Feringa and colleagues addressed this question by looking prospectively at 335 patients undergoing major vascular surgery over a two-year period. The mean age of patients was 62.2 years; 46% of patients underwent abdominal aortic aneurysm repair, and the remaining 54% received lower-extremity revascularization.
Patients had cardiac risk scores calculated based on the Revised Cardiac Risk Index (RCRI), and all patients had dobutamine stress echocardiogram (DSE) to assess for stress-induced ischemia. N-terminal pro-B-type natriuretic peptide (BNP) was measured at a mean of 12 days before surgery. Patients were followed for all-cause mortality and post-op death for a mean follow-up time of 14 months.
The authors found that NT-pro BNP performed better than the RCRI and DSE for predicting six-month mortality and cardiac events. An NT-pro BNP cut-off level of 319 ng/l was identified as optimal for predicting six-month mortality and cardiac events with 69% sensitivity and 70% specificity for mortality. Patients with levels 319 mg/l had a lower survival during the follow up period (p<0.0001).
Based on this prospective study, it appears that a preoperative elevated NT-Pro BNP is associated with long-term mortality and morbidity and could be used as an additional risk-stratification tool along with clinical risk scoring and stress testing.
Utility of Combination Medications in COPD
Aaron SD, Vandemheen KL, Fergusson D, et al. Tiotropium in combination with placebo, salmeterol, or fluticasone-salmeterol for treatment of chronic obstructive pulmonary disease. Ann Intern Med. 2007 Feb 19;146:545-555
The appropriateness of multiple long-acting inhaled medications in treating chronic obstructive pulmonary disease (COPD) is poorly studied. This study evaluated whether combining tiotropium with fluticasone-salmeterol or with salmeterol alone improves clinical outcomes in adult patients with moderate to severe COPD, as compared with tiotropium plus placebo.
This randomized, double-blind, placebo-controlled trial was set in academic and community medical centers in Canada. Researchers monitored 449 patients in the three parallel treatment groups for COPD exacerbations for 52 weeks. Analysis was done on an intention-to-treat basis. The rate of COPD exacerbations within the follow-up period (the primary outcome) was not significantly different among the three treatment groups. However, secondary outcomes, such as rates for hospitalization for COPD exacerbations, all-cause hospitalizations, health-related quality of life and lung function were significantly improved in the group receiving tiotropium and fluticasone-salmeterol.
A notable limitation was that more subjects stopped taking the study medications in the tiotropium-placebo and the tiotropium-salmeterol group. Many crossed over to treatments with inhaled corticosteroids or beta-agonists.
The results are in contrast to current guidelines, which recommend adding inhaled steroids to reduce exacerbations in moderate to severe COPD. Whether these results are due to differing statistical analysis among studies remains unclear. The authors postulate that reduction in secondary outcomes may be related to fluticasone reducing the severity of exacerbations rather than the actual number.
COPD exacerbations are among the most common diagnoses encountered by hospitalists. Most patients are treated with multiple inhaled medications to optimize their pulmonary status. Polypharmacy and the added financial burdens on the patient (particularly the elderly) are important considerations when deciding discharge medications, and the evidence of efficacy for combination inhaled medications had not been assessed as a clinical outcome prior to this study.
Benefits of Rapid Response Teams
Winters BD, Pham JC, Hunt EA et al. Rapid response systems: a systematic review. Crit Care Med. 2007 May;35(5):1238-1243.
Although the Institute for Healthcare Improvement has endorsed rapid response teams, and many hospitalist groups are involved with such systems, quality research is lacking.
Following up on the 2006 “First Consensus Conference on Medical Emergency Teams,” this meta-analysis sought to evaluate current literature to identify the effect of rapid response systems (RRS) on rates of hospital mortality and cardiac arrest.
The authors included randomized trials and observational studies in their analysis. Only eight studies met their inclusion criteria (six observational studies, one multicenter randomized trial, and one single-center randomized trial).
The pooled results did not demonstrate a statistically significant benefit of rapid-response systems in rates of hospital mortality. When rates of in-hospital cardiac arrest were analyzed, there was a weak finding in support of RRS, with the relative risk of 0.70 (confidence interval 0.56-0.92) in favor of RRSs. But the confidence interval was wide, and there was substantial heterogeneity among the included studies.
The authors conclude that “it seems premature to declare RRS as the standard of care,” and that data are lacking to justify any particular implementation scheme or composition of RRS or to support the cost-effectiveness of RRS.
Finally, they recognized the need for larger, better-designed randomized trials. However, in an accompanying editorial, Michael DeVita, MD—a pioneer in the development of RRS—rejects the use of techniques of evidence-based medicine such as multicenter trials and meta-analysis in assessing the utility of RRS. Dr. DeVita essentially says that changing the systems and culture of care within the hospital to accommodate patients with unmet critical needs must be effective in improving outcomes.
This meta-analysis is hindered by the suboptimal quality and homogeneity of studies available for assessment. Hospitalists should be aware of the limitations of the data and literature, as well as the empirical arguments raised by Dr. DeVita, when considering involvement in or designing RRS. TH
CLASSIC LIT
Perioperative Statins
Kapoor AS, Kanji H, Buckingham J, et al. Strength of evidence for perioperative use of statins to reduce cardiovascular risk: systematic review of controlled studies. BMJ. 2006 Nov 6;333(7579):1149.
Recent literature and randomized trials have claimed statins decrease morbidity and mortality from cardiovascular events in patients with or at high risk of coronary artery disease. This meta-analysis sought to determine the strength of evidence leading to the recommendations that perioperative statins be used to reduce perioperative cardiovascular events.
The literature search and exclusion criteria identified 18 studies. Two were randomized controlled trials (n=177), 15 were cohort studies (n=799,632), and one was a case-control study (n=480). Of these, 12 studies enrolled patients undergoing noncardiac vascular surgery, four enrolled patients undergoing coronary bypass surgery, and two enrolled patients undergoing various surgical procedures. The 16 nonrandomized studies were rated good. The two randomized trials were rated five and two out of five using the Jadad quality scores.
The results showed that in the randomized trials the summary odds ratio (OR) for death or acute coronary syndrome during the perioperative period with statin use was 0.26 (95% confidence interval 0.07 to 0.99), but this was based on only 13 events in 177 patients and cannot be considered conclusive. In the cohort studies, the OR was 0.70 (95% confidence interval 0.57 to 0.87). Although the pooled cohort data provided a statistically significant result, these cannot be considered conclusive because the statins were not randomly allocated and the results from retrospective studies were more impressive (OR 0.65, 95% confidence interval 0.50 to 0.84) than those in the prospective cohorts (OR 0.91, 95% confidence interval 0.65 to 1.27) and dose, duration, and safety of statin use were not reported.
Limitations of this meta-analysis include that none of the studies reported patient compliance or doses of statins or cholesterol levels before and after surgery, and few reported the duration of therapy before surgery or the which statin was used. Thus, the authors were unable to demonstrate a dose-response association. They were also unable to ascertain if the benefits seen with statins in the observational studies were exaggerated owing to inclusion of patients in the nonstatin group who had their statins stopped prior to surgery, because acute statin withdrawal may be associated with cardiac events.
The authors concluded that although their meta-analysis—which included data from more than 800,000 patients—suggests considerable benefits from perioperative statin use, the evidence from the randomized trials is not definitive. They advocate only that statins be started preoperatively in eligible patients (e.g., patients with coronary artery disease, multiple cardiac risk factors, elevated LDL) who would warrant statin therapy for medical reasons independent of the proposed operation.
Electronic Alerts to Prevent Hospital-acquired VTE
Kucher N, Koo S, Quiroz R, et al. Electronic alerts to prevent venous thromboembolism among hospitalized patients. N Engl J Med. 2005 Mar 10;352(10):969-977
Surveys conducted in North America and Europe have shown that prophylaxis against deep venous thrombosis (DVT) has been consistently underused in hospitalized patients despite consensus guidelines. Studies involving continuing medical education and computerized electronic alerts have shown that physician use of prophylaxis improves when such processes are in place, but have not demonstrated that they can reduce the rate of DVT.
A computer program was developed to identify consecutive hospitalized patients at increased risk for DVT. The program used eight common risk factors to determine each patient’s risk profile for DVT and each risk factor was assigned a score. A cumulative score of four or higher was used to determine patients at high risk for DVT. The computer alert program was screened daily to identify patients whose score increased to four or higher after admission into the hospital. If the cumulative risk score was at least four, the computer program reviewed the current electronic orders and active medications for the use of DVT prophylaxis.
In the study, 2,506 consecutive adult patients were identified as high risk for DVT. Further,1,255 were randomized to the intervention group—in which the responsible physician received one electronic alert about the risk of DVT—and 1,251 patients were randomized to the control group in which no alert was issued. The 120 physicians involved took care of patients in the intervention and control groups. Physicians responsible for the control group were not aware that patients were being followed for clinical events. When physicians received alerts, they had to acknowledge them and could either withhold prophylaxis or order it on the same computer screen.
Patients were followed for 90 days after the index hospitalization. The primary end point was clinically apparent DVT or pulmonary embolism (PE). Safety end points included mortality at 30 days, and the rate of hemorrhagic events at 90 days.
The results showed that prophylactic measures were ordered for 421 of the 1,255 patients in the intervention group (33.5%) and 182 of the 1,251 patients in the control group (14.5%, p <0.001). There were higher rates of both mechanical (10% versus 1.5%, p<0.001) and pharmacological (23.6% versus 13.0%, p<0.001) prophylaxis in the intervention group. The primary end point of DVT or PE at 90 days occurred in 61 patients in the intervention group (4.9%) as compared with 103 patients in the control group (8.2%).
The computer alert reduced the risk of events at 90 days by 41% (HR 0.59; 95% CI 0.43 to 0.81; P=0.001). Of the patients who received prophylaxis 5.1% had DVT or PE compared with 7.0% of those who did not. In the intervention group, DVT or PE occurred in 20 of 421 (4.8%) patients who received prophylaxis compared with 41 of 834 (4.9%) who did not receive any. In the control group, the same numbers were 11 of 182 (6.0%) and 91 of 1,069 (8.5%).
Some of this benefit might be attributed to the additional preventive measures such as physiotherapy and early ambulation in patients assigned to the intervention group. Diagnostic bias also could have played into the results. Not all patients were screened for VTE, and it is likely that symptomatic patients without prophylaxis were screened more frequently than symptomatic patients with prophylaxis. Because physicians took care of both the control and intervention group, alerts received by physicians in the control group could have influenced their decision in the control group as well.
The authors concluded that instituting computer alerts markedly reduced the rates of DVT or PE in hospitalized patients.
Hematocrit and Perioperative Mortality
Wu WC, Schifftner TL, Henderson WG, et al. Preoperative hematocrit levels and postoperative outcomes in older patients undergoing noncardiac surgery. JAMA. 2007 Jun 13;297(22):2481-2488.
Several studies have outlined the risk of preoperative anemia prior to noncardiac surgery in elderly patients. These studies have not linked anemia to risk of death unless cardiac disease is present.
Anemia management remains a challenge for many hospitals and is the most important predictor of the need for blood transfusion. Transfusion increases morbidity and mortality in the perioperative setting. At the same time, little is known about the risks of polycythemia in this setting.
This retrospective cohort study used the Veterans’ Affairs National Surgical Quality Improvement Program database of 310,311 veterans 65 or older from 132 VA hospitals. It explores the relationship between abnormal levels of hematocrit and adverse events among elderly surgical patients.
The data suggest an incremental relationship between positive and negative deviation of hematocrit levels with 30-day postoperative mortality in patients 65 and older. Specifically, the study found a 1.6% increase (95% confidence interval, 1.1%-2.2%) in 30-day mortality for every percentage point of increase or decrease in hematocrit from the normal range.
Because this is an observational study of anemia and adverse events, no causal relationship can be established from this data. Hospitalists involved in perioperative care should be careful about drawing conclusions from this study alone and should not necessarily plan interventions to treat abnormal levels of hematocrit without carefully considering the risks and benefits of intervention.
Prognostic Utility of Pre-operative BNP
Feringa HH, Schouten O, Dunkelgrun M, et al. Plasma N-terminal pro-B-type natriuretic peptide as long term prognostic marker after major vascular surgery. Heart. 2007 Feb;93(2):226-231.
Traditional stratification of patients at high risk for cardiac complications and undergoing noncardiac surgery has included clinical risk index scoring and pre-operative stress testing. It is unclear if cardiac biomarkers can be used in conjunction with these measures to improve the identification of patients at risk.
Feringa and colleagues addressed this question by looking prospectively at 335 patients undergoing major vascular surgery over a two-year period. The mean age of patients was 62.2 years; 46% of patients underwent abdominal aortic aneurysm repair, and the remaining 54% received lower-extremity revascularization.
Patients had cardiac risk scores calculated based on the Revised Cardiac Risk Index (RCRI), and all patients had dobutamine stress echocardiogram (DSE) to assess for stress-induced ischemia. N-terminal pro-B-type natriuretic peptide (BNP) was measured at a mean of 12 days before surgery. Patients were followed for all-cause mortality and post-op death for a mean follow-up time of 14 months.
The authors found that NT-pro BNP performed better than the RCRI and DSE for predicting six-month mortality and cardiac events. An NT-pro BNP cut-off level of 319 ng/l was identified as optimal for predicting six-month mortality and cardiac events with 69% sensitivity and 70% specificity for mortality. Patients with levels 319 mg/l had a lower survival during the follow up period (p<0.0001).
Based on this prospective study, it appears that a preoperative elevated NT-Pro BNP is associated with long-term mortality and morbidity and could be used as an additional risk-stratification tool along with clinical risk scoring and stress testing.
Utility of Combination Medications in COPD
Aaron SD, Vandemheen KL, Fergusson D, et al. Tiotropium in combination with placebo, salmeterol, or fluticasone-salmeterol for treatment of chronic obstructive pulmonary disease. Ann Intern Med. 2007 Feb 19;146:545-555
The appropriateness of multiple long-acting inhaled medications in treating chronic obstructive pulmonary disease (COPD) is poorly studied. This study evaluated whether combining tiotropium with fluticasone-salmeterol or with salmeterol alone improves clinical outcomes in adult patients with moderate to severe COPD, as compared with tiotropium plus placebo.
This randomized, double-blind, placebo-controlled trial was set in academic and community medical centers in Canada. Researchers monitored 449 patients in the three parallel treatment groups for COPD exacerbations for 52 weeks. Analysis was done on an intention-to-treat basis. The rate of COPD exacerbations within the follow-up period (the primary outcome) was not significantly different among the three treatment groups. However, secondary outcomes, such as rates for hospitalization for COPD exacerbations, all-cause hospitalizations, health-related quality of life and lung function were significantly improved in the group receiving tiotropium and fluticasone-salmeterol.
A notable limitation was that more subjects stopped taking the study medications in the tiotropium-placebo and the tiotropium-salmeterol group. Many crossed over to treatments with inhaled corticosteroids or beta-agonists.
The results are in contrast to current guidelines, which recommend adding inhaled steroids to reduce exacerbations in moderate to severe COPD. Whether these results are due to differing statistical analysis among studies remains unclear. The authors postulate that reduction in secondary outcomes may be related to fluticasone reducing the severity of exacerbations rather than the actual number.
COPD exacerbations are among the most common diagnoses encountered by hospitalists. Most patients are treated with multiple inhaled medications to optimize their pulmonary status. Polypharmacy and the added financial burdens on the patient (particularly the elderly) are important considerations when deciding discharge medications, and the evidence of efficacy for combination inhaled medications had not been assessed as a clinical outcome prior to this study.
Benefits of Rapid Response Teams
Winters BD, Pham JC, Hunt EA et al. Rapid response systems: a systematic review. Crit Care Med. 2007 May;35(5):1238-1243.
Although the Institute for Healthcare Improvement has endorsed rapid response teams, and many hospitalist groups are involved with such systems, quality research is lacking.
Following up on the 2006 “First Consensus Conference on Medical Emergency Teams,” this meta-analysis sought to evaluate current literature to identify the effect of rapid response systems (RRS) on rates of hospital mortality and cardiac arrest.
The authors included randomized trials and observational studies in their analysis. Only eight studies met their inclusion criteria (six observational studies, one multicenter randomized trial, and one single-center randomized trial).
The pooled results did not demonstrate a statistically significant benefit of rapid-response systems in rates of hospital mortality. When rates of in-hospital cardiac arrest were analyzed, there was a weak finding in support of RRS, with the relative risk of 0.70 (confidence interval 0.56-0.92) in favor of RRSs. But the confidence interval was wide, and there was substantial heterogeneity among the included studies.
The authors conclude that “it seems premature to declare RRS as the standard of care,” and that data are lacking to justify any particular implementation scheme or composition of RRS or to support the cost-effectiveness of RRS.
Finally, they recognized the need for larger, better-designed randomized trials. However, in an accompanying editorial, Michael DeVita, MD—a pioneer in the development of RRS—rejects the use of techniques of evidence-based medicine such as multicenter trials and meta-analysis in assessing the utility of RRS. Dr. DeVita essentially says that changing the systems and culture of care within the hospital to accommodate patients with unmet critical needs must be effective in improving outcomes.
This meta-analysis is hindered by the suboptimal quality and homogeneity of studies available for assessment. Hospitalists should be aware of the limitations of the data and literature, as well as the empirical arguments raised by Dr. DeVita, when considering involvement in or designing RRS. TH
CLASSIC LIT
Perioperative Statins
Kapoor AS, Kanji H, Buckingham J, et al. Strength of evidence for perioperative use of statins to reduce cardiovascular risk: systematic review of controlled studies. BMJ. 2006 Nov 6;333(7579):1149.
Recent literature and randomized trials have claimed statins decrease morbidity and mortality from cardiovascular events in patients with or at high risk of coronary artery disease. This meta-analysis sought to determine the strength of evidence leading to the recommendations that perioperative statins be used to reduce perioperative cardiovascular events.
The literature search and exclusion criteria identified 18 studies. Two were randomized controlled trials (n=177), 15 were cohort studies (n=799,632), and one was a case-control study (n=480). Of these, 12 studies enrolled patients undergoing noncardiac vascular surgery, four enrolled patients undergoing coronary bypass surgery, and two enrolled patients undergoing various surgical procedures. The 16 nonrandomized studies were rated good. The two randomized trials were rated five and two out of five using the Jadad quality scores.
The results showed that in the randomized trials the summary odds ratio (OR) for death or acute coronary syndrome during the perioperative period with statin use was 0.26 (95% confidence interval 0.07 to 0.99), but this was based on only 13 events in 177 patients and cannot be considered conclusive. In the cohort studies, the OR was 0.70 (95% confidence interval 0.57 to 0.87). Although the pooled cohort data provided a statistically significant result, these cannot be considered conclusive because the statins were not randomly allocated and the results from retrospective studies were more impressive (OR 0.65, 95% confidence interval 0.50 to 0.84) than those in the prospective cohorts (OR 0.91, 95% confidence interval 0.65 to 1.27) and dose, duration, and safety of statin use were not reported.
Limitations of this meta-analysis include that none of the studies reported patient compliance or doses of statins or cholesterol levels before and after surgery, and few reported the duration of therapy before surgery or the which statin was used. Thus, the authors were unable to demonstrate a dose-response association. They were also unable to ascertain if the benefits seen with statins in the observational studies were exaggerated owing to inclusion of patients in the nonstatin group who had their statins stopped prior to surgery, because acute statin withdrawal may be associated with cardiac events.
The authors concluded that although their meta-analysis—which included data from more than 800,000 patients—suggests considerable benefits from perioperative statin use, the evidence from the randomized trials is not definitive. They advocate only that statins be started preoperatively in eligible patients (e.g., patients with coronary artery disease, multiple cardiac risk factors, elevated LDL) who would warrant statin therapy for medical reasons independent of the proposed operation.
Electronic Alerts to Prevent Hospital-acquired VTE
Kucher N, Koo S, Quiroz R, et al. Electronic alerts to prevent venous thromboembolism among hospitalized patients. N Engl J Med. 2005 Mar 10;352(10):969-977
Surveys conducted in North America and Europe have shown that prophylaxis against deep venous thrombosis (DVT) has been consistently underused in hospitalized patients despite consensus guidelines. Studies involving continuing medical education and computerized electronic alerts have shown that physician use of prophylaxis improves when such processes are in place, but have not demonstrated that they can reduce the rate of DVT.
A computer program was developed to identify consecutive hospitalized patients at increased risk for DVT. The program used eight common risk factors to determine each patient’s risk profile for DVT and each risk factor was assigned a score. A cumulative score of four or higher was used to determine patients at high risk for DVT. The computer alert program was screened daily to identify patients whose score increased to four or higher after admission into the hospital. If the cumulative risk score was at least four, the computer program reviewed the current electronic orders and active medications for the use of DVT prophylaxis.
In the study, 2,506 consecutive adult patients were identified as high risk for DVT. Further,1,255 were randomized to the intervention group—in which the responsible physician received one electronic alert about the risk of DVT—and 1,251 patients were randomized to the control group in which no alert was issued. The 120 physicians involved took care of patients in the intervention and control groups. Physicians responsible for the control group were not aware that patients were being followed for clinical events. When physicians received alerts, they had to acknowledge them and could either withhold prophylaxis or order it on the same computer screen.
Patients were followed for 90 days after the index hospitalization. The primary end point was clinically apparent DVT or pulmonary embolism (PE). Safety end points included mortality at 30 days, and the rate of hemorrhagic events at 90 days.
The results showed that prophylactic measures were ordered for 421 of the 1,255 patients in the intervention group (33.5%) and 182 of the 1,251 patients in the control group (14.5%, p <0.001). There were higher rates of both mechanical (10% versus 1.5%, p<0.001) and pharmacological (23.6% versus 13.0%, p<0.001) prophylaxis in the intervention group. The primary end point of DVT or PE at 90 days occurred in 61 patients in the intervention group (4.9%) as compared with 103 patients in the control group (8.2%).
The computer alert reduced the risk of events at 90 days by 41% (HR 0.59; 95% CI 0.43 to 0.81; P=0.001). Of the patients who received prophylaxis 5.1% had DVT or PE compared with 7.0% of those who did not. In the intervention group, DVT or PE occurred in 20 of 421 (4.8%) patients who received prophylaxis compared with 41 of 834 (4.9%) who did not receive any. In the control group, the same numbers were 11 of 182 (6.0%) and 91 of 1,069 (8.5%).
Some of this benefit might be attributed to the additional preventive measures such as physiotherapy and early ambulation in patients assigned to the intervention group. Diagnostic bias also could have played into the results. Not all patients were screened for VTE, and it is likely that symptomatic patients without prophylaxis were screened more frequently than symptomatic patients with prophylaxis. Because physicians took care of both the control and intervention group, alerts received by physicians in the control group could have influenced their decision in the control group as well.
The authors concluded that instituting computer alerts markedly reduced the rates of DVT or PE in hospitalized patients.
Hematocrit and Perioperative Mortality
Wu WC, Schifftner TL, Henderson WG, et al. Preoperative hematocrit levels and postoperative outcomes in older patients undergoing noncardiac surgery. JAMA. 2007 Jun 13;297(22):2481-2488.
Several studies have outlined the risk of preoperative anemia prior to noncardiac surgery in elderly patients. These studies have not linked anemia to risk of death unless cardiac disease is present.
Anemia management remains a challenge for many hospitals and is the most important predictor of the need for blood transfusion. Transfusion increases morbidity and mortality in the perioperative setting. At the same time, little is known about the risks of polycythemia in this setting.
This retrospective cohort study used the Veterans’ Affairs National Surgical Quality Improvement Program database of 310,311 veterans 65 or older from 132 VA hospitals. It explores the relationship between abnormal levels of hematocrit and adverse events among elderly surgical patients.
The data suggest an incremental relationship between positive and negative deviation of hematocrit levels with 30-day postoperative mortality in patients 65 and older. Specifically, the study found a 1.6% increase (95% confidence interval, 1.1%-2.2%) in 30-day mortality for every percentage point of increase or decrease in hematocrit from the normal range.
Because this is an observational study of anemia and adverse events, no causal relationship can be established from this data. Hospitalists involved in perioperative care should be careful about drawing conclusions from this study alone and should not necessarily plan interventions to treat abnormal levels of hematocrit without carefully considering the risks and benefits of intervention.
Prognostic Utility of Pre-operative BNP
Feringa HH, Schouten O, Dunkelgrun M, et al. Plasma N-terminal pro-B-type natriuretic peptide as long term prognostic marker after major vascular surgery. Heart. 2007 Feb;93(2):226-231.
Traditional stratification of patients at high risk for cardiac complications and undergoing noncardiac surgery has included clinical risk index scoring and pre-operative stress testing. It is unclear if cardiac biomarkers can be used in conjunction with these measures to improve the identification of patients at risk.
Feringa and colleagues addressed this question by looking prospectively at 335 patients undergoing major vascular surgery over a two-year period. The mean age of patients was 62.2 years; 46% of patients underwent abdominal aortic aneurysm repair, and the remaining 54% received lower-extremity revascularization.
Patients had cardiac risk scores calculated based on the Revised Cardiac Risk Index (RCRI), and all patients had dobutamine stress echocardiogram (DSE) to assess for stress-induced ischemia. N-terminal pro-B-type natriuretic peptide (BNP) was measured at a mean of 12 days before surgery. Patients were followed for all-cause mortality and post-op death for a mean follow-up time of 14 months.
The authors found that NT-pro BNP performed better than the RCRI and DSE for predicting six-month mortality and cardiac events. An NT-pro BNP cut-off level of 319 ng/l was identified as optimal for predicting six-month mortality and cardiac events with 69% sensitivity and 70% specificity for mortality. Patients with levels 319 mg/l had a lower survival during the follow up period (p<0.0001).
Based on this prospective study, it appears that a preoperative elevated NT-Pro BNP is associated with long-term mortality and morbidity and could be used as an additional risk-stratification tool along with clinical risk scoring and stress testing.
Utility of Combination Medications in COPD
Aaron SD, Vandemheen KL, Fergusson D, et al. Tiotropium in combination with placebo, salmeterol, or fluticasone-salmeterol for treatment of chronic obstructive pulmonary disease. Ann Intern Med. 2007 Feb 19;146:545-555
The appropriateness of multiple long-acting inhaled medications in treating chronic obstructive pulmonary disease (COPD) is poorly studied. This study evaluated whether combining tiotropium with fluticasone-salmeterol or with salmeterol alone improves clinical outcomes in adult patients with moderate to severe COPD, as compared with tiotropium plus placebo.
This randomized, double-blind, placebo-controlled trial was set in academic and community medical centers in Canada. Researchers monitored 449 patients in the three parallel treatment groups for COPD exacerbations for 52 weeks. Analysis was done on an intention-to-treat basis. The rate of COPD exacerbations within the follow-up period (the primary outcome) was not significantly different among the three treatment groups. However, secondary outcomes, such as rates for hospitalization for COPD exacerbations, all-cause hospitalizations, health-related quality of life and lung function were significantly improved in the group receiving tiotropium and fluticasone-salmeterol.
A notable limitation was that more subjects stopped taking the study medications in the tiotropium-placebo and the tiotropium-salmeterol group. Many crossed over to treatments with inhaled corticosteroids or beta-agonists.
The results are in contrast to current guidelines, which recommend adding inhaled steroids to reduce exacerbations in moderate to severe COPD. Whether these results are due to differing statistical analysis among studies remains unclear. The authors postulate that reduction in secondary outcomes may be related to fluticasone reducing the severity of exacerbations rather than the actual number.
COPD exacerbations are among the most common diagnoses encountered by hospitalists. Most patients are treated with multiple inhaled medications to optimize their pulmonary status. Polypharmacy and the added financial burdens on the patient (particularly the elderly) are important considerations when deciding discharge medications, and the evidence of efficacy for combination inhaled medications had not been assessed as a clinical outcome prior to this study.
Benefits of Rapid Response Teams
Winters BD, Pham JC, Hunt EA et al. Rapid response systems: a systematic review. Crit Care Med. 2007 May;35(5):1238-1243.
Although the Institute for Healthcare Improvement has endorsed rapid response teams, and many hospitalist groups are involved with such systems, quality research is lacking.
Following up on the 2006 “First Consensus Conference on Medical Emergency Teams,” this meta-analysis sought to evaluate current literature to identify the effect of rapid response systems (RRS) on rates of hospital mortality and cardiac arrest.
The authors included randomized trials and observational studies in their analysis. Only eight studies met their inclusion criteria (six observational studies, one multicenter randomized trial, and one single-center randomized trial).
The pooled results did not demonstrate a statistically significant benefit of rapid-response systems in rates of hospital mortality. When rates of in-hospital cardiac arrest were analyzed, there was a weak finding in support of RRS, with the relative risk of 0.70 (confidence interval 0.56-0.92) in favor of RRSs. But the confidence interval was wide, and there was substantial heterogeneity among the included studies.
The authors conclude that “it seems premature to declare RRS as the standard of care,” and that data are lacking to justify any particular implementation scheme or composition of RRS or to support the cost-effectiveness of RRS.
Finally, they recognized the need for larger, better-designed randomized trials. However, in an accompanying editorial, Michael DeVita, MD—a pioneer in the development of RRS—rejects the use of techniques of evidence-based medicine such as multicenter trials and meta-analysis in assessing the utility of RRS. Dr. DeVita essentially says that changing the systems and culture of care within the hospital to accommodate patients with unmet critical needs must be effective in improving outcomes.
This meta-analysis is hindered by the suboptimal quality and homogeneity of studies available for assessment. Hospitalists should be aware of the limitations of the data and literature, as well as the empirical arguments raised by Dr. DeVita, when considering involvement in or designing RRS. TH
CLASSIC LIT
Perioperative Statins
Kapoor AS, Kanji H, Buckingham J, et al. Strength of evidence for perioperative use of statins to reduce cardiovascular risk: systematic review of controlled studies. BMJ. 2006 Nov 6;333(7579):1149.
Recent literature and randomized trials have claimed statins decrease morbidity and mortality from cardiovascular events in patients with or at high risk of coronary artery disease. This meta-analysis sought to determine the strength of evidence leading to the recommendations that perioperative statins be used to reduce perioperative cardiovascular events.
The literature search and exclusion criteria identified 18 studies. Two were randomized controlled trials (n=177), 15 were cohort studies (n=799,632), and one was a case-control study (n=480). Of these, 12 studies enrolled patients undergoing noncardiac vascular surgery, four enrolled patients undergoing coronary bypass surgery, and two enrolled patients undergoing various surgical procedures. The 16 nonrandomized studies were rated good. The two randomized trials were rated five and two out of five using the Jadad quality scores.
The results showed that in the randomized trials the summary odds ratio (OR) for death or acute coronary syndrome during the perioperative period with statin use was 0.26 (95% confidence interval 0.07 to 0.99), but this was based on only 13 events in 177 patients and cannot be considered conclusive. In the cohort studies, the OR was 0.70 (95% confidence interval 0.57 to 0.87). Although the pooled cohort data provided a statistically significant result, these cannot be considered conclusive because the statins were not randomly allocated and the results from retrospective studies were more impressive (OR 0.65, 95% confidence interval 0.50 to 0.84) than those in the prospective cohorts (OR 0.91, 95% confidence interval 0.65 to 1.27) and dose, duration, and safety of statin use were not reported.
Limitations of this meta-analysis include that none of the studies reported patient compliance or doses of statins or cholesterol levels before and after surgery, and few reported the duration of therapy before surgery or the which statin was used. Thus, the authors were unable to demonstrate a dose-response association. They were also unable to ascertain if the benefits seen with statins in the observational studies were exaggerated owing to inclusion of patients in the nonstatin group who had their statins stopped prior to surgery, because acute statin withdrawal may be associated with cardiac events.
The authors concluded that although their meta-analysis—which included data from more than 800,000 patients—suggests considerable benefits from perioperative statin use, the evidence from the randomized trials is not definitive. They advocate only that statins be started preoperatively in eligible patients (e.g., patients with coronary artery disease, multiple cardiac risk factors, elevated LDL) who would warrant statin therapy for medical reasons independent of the proposed operation.
Electronic Alerts to Prevent Hospital-acquired VTE
Kucher N, Koo S, Quiroz R, et al. Electronic alerts to prevent venous thromboembolism among hospitalized patients. N Engl J Med. 2005 Mar 10;352(10):969-977
Surveys conducted in North America and Europe have shown that prophylaxis against deep venous thrombosis (DVT) has been consistently underused in hospitalized patients despite consensus guidelines. Studies involving continuing medical education and computerized electronic alerts have shown that physician use of prophylaxis improves when such processes are in place, but have not demonstrated that they can reduce the rate of DVT.
A computer program was developed to identify consecutive hospitalized patients at increased risk for DVT. The program used eight common risk factors to determine each patient’s risk profile for DVT and each risk factor was assigned a score. A cumulative score of four or higher was used to determine patients at high risk for DVT. The computer alert program was screened daily to identify patients whose score increased to four or higher after admission into the hospital. If the cumulative risk score was at least four, the computer program reviewed the current electronic orders and active medications for the use of DVT prophylaxis.
In the study, 2,506 consecutive adult patients were identified as high risk for DVT. Further,1,255 were randomized to the intervention group—in which the responsible physician received one electronic alert about the risk of DVT—and 1,251 patients were randomized to the control group in which no alert was issued. The 120 physicians involved took care of patients in the intervention and control groups. Physicians responsible for the control group were not aware that patients were being followed for clinical events. When physicians received alerts, they had to acknowledge them and could either withhold prophylaxis or order it on the same computer screen.
Patients were followed for 90 days after the index hospitalization. The primary end point was clinically apparent DVT or pulmonary embolism (PE). Safety end points included mortality at 30 days, and the rate of hemorrhagic events at 90 days.
The results showed that prophylactic measures were ordered for 421 of the 1,255 patients in the intervention group (33.5%) and 182 of the 1,251 patients in the control group (14.5%, p <0.001). There were higher rates of both mechanical (10% versus 1.5%, p<0.001) and pharmacological (23.6% versus 13.0%, p<0.001) prophylaxis in the intervention group. The primary end point of DVT or PE at 90 days occurred in 61 patients in the intervention group (4.9%) as compared with 103 patients in the control group (8.2%).
The computer alert reduced the risk of events at 90 days by 41% (HR 0.59; 95% CI 0.43 to 0.81; P=0.001). Of the patients who received prophylaxis 5.1% had DVT or PE compared with 7.0% of those who did not. In the intervention group, DVT or PE occurred in 20 of 421 (4.8%) patients who received prophylaxis compared with 41 of 834 (4.9%) who did not receive any. In the control group, the same numbers were 11 of 182 (6.0%) and 91 of 1,069 (8.5%).
Some of this benefit might be attributed to the additional preventive measures such as physiotherapy and early ambulation in patients assigned to the intervention group. Diagnostic bias also could have played into the results. Not all patients were screened for VTE, and it is likely that symptomatic patients without prophylaxis were screened more frequently than symptomatic patients with prophylaxis. Because physicians took care of both the control and intervention group, alerts received by physicians in the control group could have influenced their decision in the control group as well.
The authors concluded that instituting computer alerts markedly reduced the rates of DVT or PE in hospitalized patients.