User login
Barriers and Facilitators to Guideline-Adherent Pulse Oximetry Use in Bronchiolitis
Continuous pulse oximetry monitoring (cSpO2) in children with bronchiolitis is associated with increased rates of hospital admission, longer lengths of stay, more frequent treatment with supplemental oxygen, alarm fatigue, and higher hospital cost. There is no evidence that it improves clinical outcomes.1-7 The safety of reducing cSpO2 for stable bronchiolitis patients (ie, those who are clinically well and not requiring supplemental oxygen) has been assessed in quality improvement initiatives8-10 and a randomized controlled trial.2 These studies showed no increase in intensive care unit transfers, codes, or readmissions associated with reduced cSpO2. Current national guidelines from the American Academy of Pediatrics5 and the Society of Hospital Medicine Choosing Wisely in Pediatric Hospital Medicine workgroup4 support limiting monitoring of children with bronchiolitis. Despite this, the practice of cSpO2 in stable bronchiolitis patients off supplemental oxygen remains widespread.11,12
Deimplementation, defined as reducing or stopping low-value or ineffective healthcare practices,13,14 is a discrete focus area within implementation science. Deimplementation research involves the reduction of unnecessary and overused services for which there is potential for harm or no benefit.15,16 In pediatrics, there are a number of potential targets for deimplementation,4,17-20 including cSpO2 for stable infants with bronchiolitis, but efforts to reduce low-value practices have met limited success to date. 21,22
Implementation science offers rigorous methods for advancing the development and evaluation of strategies for deimplementation.23 In particular, implementation science frameworks can facilitate our understanding of relevant contextual factors that may hinder or help efforts to deimplement low-value practices. To develop broadly applicable strategies to reduce monitoring overuse, it is important to understand the barriers, facilitators, and contextual factors (eg, clinical, political, interpersonal) that contribute to guideline-discordant cSpO2 in hospitalized bronchiolitis patients. Further, the process by which one can develop a rigorous understanding of these factors and how they may impact deimplementation efforts could generalize to other scenarios in pediatrics where overuse remains an issue.
The goal of this study was to use semistructured interviews, informed by an established implementation science framework, specifically the Consolidated Framework for Implementation Research (CFIR),24 to (1) identify barriers and facilitators to deimplementing unnecessary cSpO2, and (2) develop strategies to deimplement cSpO2 in a multicenter cohort of hospital-based clinician and administrative stakeholders.
METHODS
Study Setting
This multicenter qualitative study using semistructured interviews took place within the Eliminating Monitor Overuse (EMO) SpO2 study. The EMO SpO2 study established rates of cSpO2 in bronchiolitis patients not receiving supplemental oxygen or not receiving room air flow at 56 hospitals across the United States and in Canada from December 1, 2018, through March 31, 2019.12 The study identified hospital-level risk-adjusted cSpO2 rates ranging from 6% to 82%. A description of the EMO SpO2 study methods25 and its findings12 have been published elsewhere.
Participants
We approached EMO study site principal investigators at 12 hospitals: the two highest- and two lowest-use hospitals within three hospital types (ie, freestanding children’s hospitals, children’s hospitals within large general hospitals, and community hospitals). We collaborated with the participating site principal investigators (n = 12), who were primarily hospitalist physicians in leadership roles, to recruit a purposive sample of additional stakeholders including bedside nurses (n = 12), hospitalist physicians (n = 15), respiratory therapists (n = 9), and hospital administrators (n = 8) to participate in semistructured interviews. Interviews were conducted until we achieved thematic saturation within each stakeholder group and within the high and low performing strata (total 56 interviews). Participants were asked to self-report basic demographic information (see Appendix, interview guide) as required by the study funder and to allow us to comment on the representativeness of the participant group.
Procedure
The interview guide was informed by the CFIR, a comprehensive framework detailing contextual factors that require consideration when planning for the implementation of a health service intervention. Table 1 details the CFIR domains with study-related examples. The interview guide (Appendix) provided limited clinical context apart from the age, diagnosis, and oxygen requirement for the population of interest to promote a broad array of responses and to avoid anchoring on specific clinical scenarios. Interviews were conducted by master’s degree or doctoral-level research coordinators with qualitative interviewing experience and supervised by a medical anthropologist and qualitative methods expert (F.K.B.). Prior to engaging in audio recorded phone interviews, the interviewer explained the risks and benefits of participating. Participants were compensated $50. Audio recordings were transcribed, deidentified, and uploaded to NVivo 12 Plus (QSR International) for data management.
The Institutional Review Boards of Children’s Hospital of Philadelphia, Pennsylvania, and the University of Pennsylvania in Philadelphia determined that the study met eligibility criteria for IRB exemption.
Data Analysis
Using an integrated approach to codebook development,26 a priori codes were developed using constructs from the CFIR. Additional codes were added by the research team following a close reading of the first five transcripts.27,28 Each code was defined, including decision rules for its application. Two research coordinators independently coded each transcript. Using the intercoder reliability function within NVivo, the coders established strong interrater reliability accordance scores (
RESULTS
Barriers and facilitators to deimplementation were identified in multiple domains of the CFIR: outer setting, inner setting, characteristics of the individuals, and intervention characteristics (Table 1). Participants also suggested strategies to facilitate deimplementation in response to some identified barriers. See Table 2 for participant demographics and Table 3 for illustrative participant quotations.
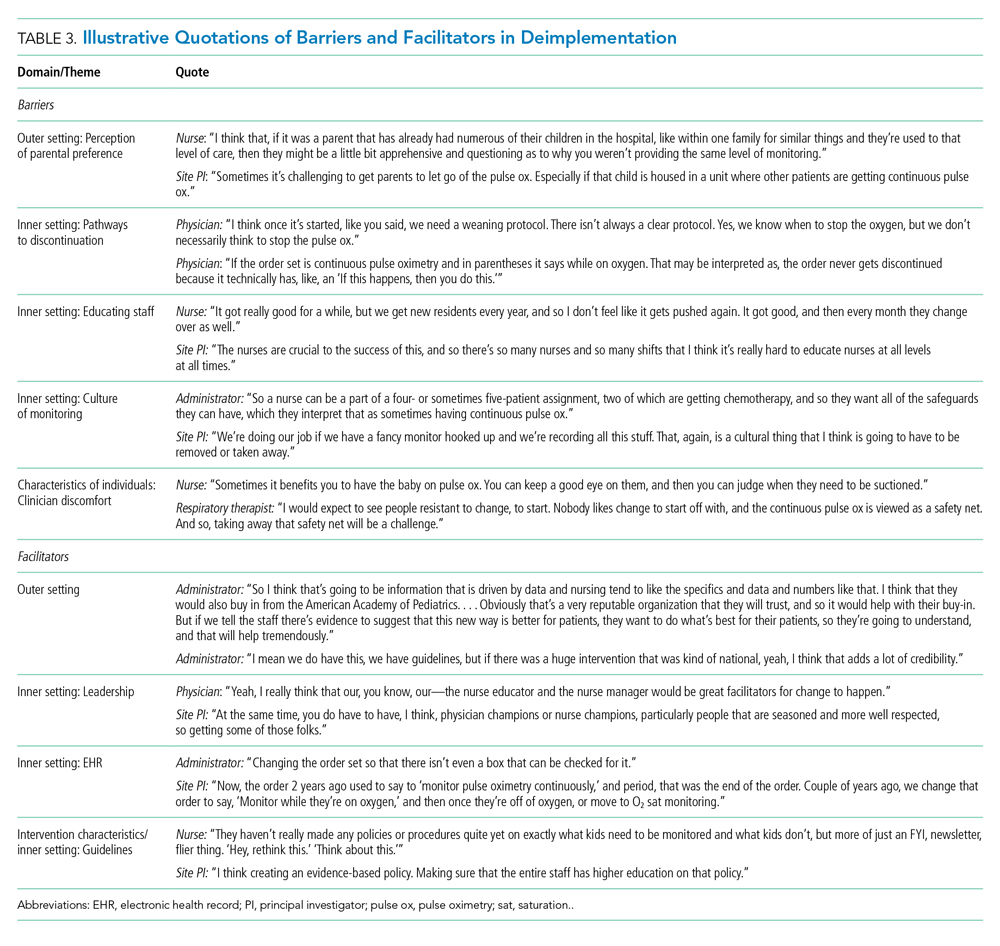
Barriers
Outer Setting: Clinician Perceptions of Parental Discomfort With Discontinuing Monitoring
Participants mentioned parental preferences as a barrier to discontinuing cSpO2, noting that parents seem to take comfort in watching the numbers on the monitor screen and are reluctant to have it withdrawn. Clinicians noted that parents sometimes put the monitor back on their child after a clinician removed it or have expressed concern that their unmonitored child was not receiving the same level of care as other patients who were being monitored. In these scenarios, clinicians reported they have found it helpful to educate caregivers about when cSpO2 is and is not appropriate.
Inner Setting: Unclear or Nonexistent Guideline to Discontinue cSpO2
Guidelines to discontinue cSpO2 reportedly did not exist at all institutions. If a guideline did exist, lack of clarity or conflicting guidelines about when to use oxygen presented a barrier. Participants suggested that a clear guideline or additional oversight to ensure all clinicians are informed of the procedure for discontinuing cSpO2 may help prevent miscommunication. Participants noted that their electronic health record (EHR) order sets commonly included cSpO2 orders and that removing that option would facilitate deimplementation.
Inner Setting: Difficulty Educating All Staff
Participants noted difficulty with incorporating education about discontinuing cSpO2 to all clinicians, particularly to those who are nightshift only or to rotating staff or trainees. This created barriers for frequent re-education because these staff are not familiar with the policies and procedures of the unit, which is crucial to developing a culture that supports the deimplementation of cSpO2. Participants suggested that recurring education about procedures for discontinuing cSpO2 should target trainees, new nurses, and overnight nurses. This would help to ensure that the guideline is uniformly followed.
Inner Setting: Culture of High cSpO2 Use
Participants from high-use sites discussed a culture driven by readily available monitoring features or an expectation that monitoring indicates higher-quality care. Participants from low-use sites discussed increased cSpO2 driven by clinicians who were accustomed to caring for higher-acuity patients, for whom continuous monitoring is likely appropriate, and were simultaneously caring for stable bronchiolitis patients.
Some suggested that visual cues would be useful to clinicians to sustain awareness about a cSpO2 deimplementation guideline. It was also suggested that audit and feedback techniques like posting unit deimplementation statistics and creating a competition among units by posting unit performance could facilitate deimplementation. Additionally, some noted that visual aids in common spaces would be useful to remind clinicians and to engage caregivers about discontinuing cSpO2.
Characteristics of Individuals: Clinician Discomfort Discontinuing cSpO2
One frequently cited barrier across participants is that cSpO2 provides “peace of mind” to alert clinicians to patients with low oxygen saturations that might otherwise be missed. Participants identified that clinician discomfort with reducing cSpO2 may be driven by inexperienced clinicians less familiar with the bronchiolitis disease process, such as trainees, new nurses, or rotating clinicians unaccustomed to pediatric care. Trainees and new nurses were perceived as being more likely to work at night when there are fewer clinicians to provide patient care. Additionally, participants perceived that night shift clinicians favored cSpO2 because they could measure vital signs without waking patients and families.
Clinicians discussed that discontinuing cSpO2 would require alternative methods for assessing patient status, particularly for night shift nurses. Participants suggested strategies including changes to pulse oximetry assessment procedures to include more frequent “spot checks,” incorporation of assessments during sleep events (eg, naps) to ensure the patient does not experience desaturations during sleep, and training nurses to become more comfortable with suctioning patients. Suggestions also included education on the typical features of transient oxygen desaturations in otherwise stable patients with bronchiolitis2 to bolster clinical confidence for clinicians unfamiliar with caring for bronchiolitis patients. Participants perceived that education about appropriate vs inappropriate use may help to empower clinicians to employ cSpO2 appropriately.
Facilitators
Outer Setting: Standards and Evidence From Research, Professional Organizations, and Leaders in the Field
Many participants expressed the importance of consistent guidelines that are advocated by thought leaders in the field, supported by robust evidence, and consistent with approaches at peer hospitals. The more authoritative support a guideline has, the more comfortable people are adopting it and taking it seriously. Additionally, consistent education about guidelines was desired. Participants noted that all clinicians should be receiving education related to the American Academy of Pediatrics (AAP) Bronchiolitis and Choosing Wisely® guidelines, ranging from a one-time update to annually. Continual updates and re-education sessions for clinicians who shared evidence about how cSpO2 deimplementation could improve the quality of patient care by shortening hospital length of stay and lowering cost were suggested strategies.
Inner Setting: Leadership
Participants noted that successful deimplementation depends upon the presence of a champion or educator who will be able to lead the institutional charge in making practice change. This is typically an individual who is trusted at the institution, experienced in their field, or already doing implementation work. This could be either a single individual (champion) or a team. The most commonly noted clinician roles to engage in a leadership role or team were physicians and nurses.
Participants noted that a change in related clinical care pathways or EHR order sets would require cooperation from multiple clinical disciplines, administrators, and information technology leaders and explained that messaging and education about the value of the change would facilitate buy-in from those clinicians.
Inner Setting: EHR Support for Guidelines
Participants often endorsed the use of an order set within the EHR that supports guidelines and includes reminders to decrease cSpO2. These reminders could come up when supplemental oxygen is discontinued or occur regularly throughout the patient’s stay to prompt the clinician to consider discontinuing cSpO2.
Intervention Characteristics/Inner Setting: Clear Bronchiolitis Guidelines
The presence of a well-articulated hospital policy that delineates the appropriate and inappropriate use of cSpO2 in bronchiolitis was mentioned as another facilitator of deimplementation.
DISCUSSION
Results of this qualitative study of stakeholders across hospitals with high and low cSpO2 use illustrated the complexities involved with deimplementation of cSpO2 in pediatric patients hospitalized with bronchiolitis. We identified numerous barriers spanning the CFIR constructs, including unclear or absent guidelines for stopping cSpO2, clinician knowledge and comfort with bronchiolitis disease features, and unit culture. This suggests that multicomponent strategies that target various domains and a variety of stakeholders are needed to deimplement cSpO2 use for stable bronchiolitis patients. Participants also identified facilitators, including clear cSpO2 guidelines, supportive leaders and champions, and EHR modifications, that provide insight into strategies that may help sites reduce their use of cSpO2. Additionally, participants also provided concrete, actionable suggestions for ways to reduce unnecessary monitoring that will be useful in informing promising deimplementation strategies for subsequent trials.
The importance of having specific and well-known guidelines from trusted sources, such as the AAP, about cSpO2 and bronchiolitis treatment that are thoughtfully integrated in the EHR came through in multiple themes of our analysis. Prior studies on the effect of guidelines on clinical practice have suggested that rigorously designed guidelines can positively impact practice.29 Participants also noted that cSpO2 guidelines should be authoritative and that knowledge of guideline adoption by peer institutions was a facilitator of adoption. Usability issues negatively impact clinicians’ ability to follow guidelines.30 Further, prior studies have demonstrated that EHR integration of guidelines can change practice.31-33 Based on our findings, incorporating clear guidelines into commonly used formats, such as EHR order sets, could be an important deimplementation tool for cSpO2 in stable bronchiolitis patients.
Education about and awareness of cSpO2 guidelines was described as an important facilitator for appropriate cSpO2 use and was suggested as a potential deimplementation strategy. Participants noted that educational need may vary by stakeholder group. For example, education may facilitate obtaining buy-in from hospital leaders, which is necessary to support changes to the EHR. Education incorporating information on the typical features of bronchiolitis and examples of appropriate and inappropriate cSpO2 use was suggested for clinical team members. The limitations of education as a stand-alone deimplementation strategy were also noted, and participants highlighted challenges such as time needed for education and the need for ongoing education for rotating trainees. Inner and outer setting barriers, such as a perceived “culture of high pulse oximetryuse” and patient and family expectations, could also make education less effective as a stand-alone strategy. That—coupled with evidence that education and training alone are generally insufficient for producing reliable, sustained behavior change34,35—suggests that a multifaceted approach will be important.
Our respondents consider parental perceptions and preference in their practice, which provides nuance to recent studies suggesting that parents prefer continuous monitors when their child is hospitalized with bronchiolitis. Chi et al described the impact of a brief educational intervention on parental preferences for monitoring children hospitalized for bronchiolitis.36 This work suggests that educational interventions aimed at families should be considered in future (de)implementation studies because they may indirectly impact clinician behavior. Future studies should directly assess parental discomfort with discontinuing monitoring. Participants highlighted the link between knowledge and confidence in caring for typical bronchiolitis patients and monitoring practice, perceiving that less experienced clinicians are more likely to rely on cSpO2. Participants at high-use sites emphasized the expectation that monitoring should occur during hospitalizations. This reflection is particularly pertinent for bronchiolitis, a disease characterized by frequent, self-resolving desaturations even after hospital discharge.3 This may reinforce a perceived need to capture and react to these desaturation events even though they are expected in bronchiolitis and can occur in healthy infants.37 Some participants suggested that continuous monitoring be replaced with “nap tests” (ie, assessment for desaturations during a nap prior to discharge); however, like cSpO2 in stable infants with bronchiolitis, this is another low-value practice. Otherwise healthy infants with mild to moderate disease are unlikely to subsequently worsen after showing signs of clinical improvement.38 Nap tests are likely to lead to infants who are clinically improving being placed unnecessarily back on oxygen in reaction to the transient desaturations. Participants’ perception about the importance of cSpO2 in bronchiolitis management, despite evidence suggesting it is a low-value practice, underscores the importance of not simply telling clinicians to stop cSpO2. Employing strategies that replace continuous monitoring with another acceptable and feasible alternative (eg, regular clinician assessments including intermittent pulse oximetry checks) should be considered when planning for deimplementation.39
Previous studies indicate that continuous monitoring can affect clinician decision-making, independent of other factors,6,40 despite limited evidence that continuous monitors improve patient outcomes.1-7 Studies have demonstrated noticeable increase in admissions based purely on pulse oximetry values,40 with no evidence that this type of admission changes outcomes for bronchiolitis patients.6 One previous, single-center study identified inexperience as a potential driver for monitor use,41 and studies in adult populations have suggested that clinicians overestimate the value that continuous monitoring contributes to patient care,42,43 which promotes guideline-discordant use. Our study provides novel insight into the issue of monitoring in bronchiolitis. Our results suggest that there is a need to shift organizational cultures around monitoring (which likely vary based on a range of factors) and that educational strategies addressing typical disease course, especially desaturations, in bronchiolitis will be an essential component in any deimplementation effort.
This study is strengthened by its sample of diverse stakeholder groups from multiple US health systems. Additionally, we interviewed individuals at sites with high cSpO2 rates and at sites with low rates, as well as from community hospitals, children’s hospitals within general hospitals, and freestanding children’s hospitals, which allows us to understand barriers high-use sites encounter and facilitators of lower cSpO2 rates at low-use sites. We also employed an interview approach informed by an established implementation science framework. Nonetheless, several limitations exist. First, participants at low-use sites did not necessarily have direct experience with a previous deimplementation effort to reduce cSpO2. Additionally, participants were predominantly White and female; more diverse perspectives would strengthen confidence in the generalizability of our findings. While thematic saturation was achieved within each stakeholder group and within the high- and low-use strata, we interviewed fewer administrators and respiratory therapists relative to other stakeholder groups. Nevertheless, our conclusions were validated by our interdisciplinary stakeholder panel. As noted by participants, family preferences may influence clinician practice, and parents were not interviewed for this study. The information gleaned from the present study will inform the development of strategies to deimplement unnecessary cSpO2 in pediatric hospitals, which we aim to rigorously evaluate in a future trial.
CONCLUSION
We identified barriers and facilitators to deimplementation of cSpO2 for stable patients with bronchiolitis across children’s hospitals with high and low utilization of cSpO2. These themes map to multiple CFIR domains and, along with participant-suggested strategies, can directly inform an approach to cSpO2 deimplementation in a range of inpatient settings. Based on these data, future deimplementation efforts should focus on clear protocols for use and discontinuation of cSpO2, EHR changes, and regular bronchiolitis education for hospital staff that emphasizes reducing unnecessary cSpO2 utilization.
ACKNOWLEDGMENTS
We acknowledge the NHLBI scientists who contributed their expertise to this project as part of the U01 Cooperative Agreement funding mechanism as federal employees conducting their official job duties: Lora Reineck, MD, MS, Karen Bienstock, MS, and Cheryl Boyce, PhD. We thank the Executive Council of the Pediatric Research in Inpatient Settings (PRIS) Network for their contributions to the early scientific development of this project. The Network assessed a Collaborative Support Fee for access to the hospitals and support of this project. We thank the PRIS Network collaborators for their major contributions to data collection measuring utilization to identify the hospitals we subsequently chose for this project. We thank Claire Bocage and the Mixed Methods Research Lab for major help in data management and data analysis.
1. Cunningham S, Rodriguez A, Adams T, et al; Bronchiolitis of Infancy Discharge Study (BIDS) group. Oxygen saturation targets in infants with bronchiolitis (BIDS): a double-blind, randomised, equivalence trial. Lancet. 2015;386(9998):1041-1048. https://doi.org/10.1016/s0140-6736(15)00163-4
2. McCulloh R, Koster M, Ralston S, et al. Use of intermittent vs continuous pulse oximetry for nonhypoxemic infants and young children hospitalized for bronchiolitis: a randomized clinical trial. JAMA Pediatr. 2015;169(10):898-904. https://doi.org/10.1001/jamapediatrics.2015.1746
3. Principi T, Coates AL, Parkin PC, Stephens D, DaSilva Z, Schuh S. Effect of oxygen desaturations on subsequent medical visits in infants discharged from the emergency department with bronchiolitis. JAMA Pediatr. 2016;170(6):602-608. https://doi.org/10.1001/jamapediatrics.2016.0114
4. Quinonez RA, Garber MD, Schroeder AR, et al. Choosing wisely in pediatric hospital medicine: five opportunities for improved healthcare value. J Hosp Med. 2013;8(9):479-485. Quinonez RA, Garber MD, Schroeder AR, et al. Choosing wisely in pediatric hospital medicine: five opportunities for improved healthcare value. J Hosp Med. 2013;8(9):479-485. https://doi.org/10.1002/jhm.2064
5. Ralston SL, Lieberthal AS, Meissner HC, et al. Clinical practice guideline: the diagnosis, management, and prevention of bronchiolitis. Pediatrics. 2014;134(5):e1474-e1502. https://doi.org/10.1542/peds.2014-2742
6. Schuh S, Freedman S, Coates A, et al. Effect of oximetry on hospitalization in bronchiolitis: a randomized clinical trial. JAMA. 2014;312(7):712-718. https://doi.org/10.1001/jama.2014.8637
7. Schuh S, Kwong JC, Holder L, Graves E, Macdonald EM, Finkelstein Y. Predictors of critical care and mortality in bronchiolitis after emergency department discharge. J Pediatr. 2018;199:217-222 e211. https://doi.org/10.1016/j.jpeds.2018.04.010
8. Schondelmeyer AC, Simmons JM, Statile AM, et al. Using quality improvement to reduce continuous pulse oximetry use in children with wheezing. Pediatrics. 2015;135(4):e1044-e1051. https://doi.org/10.1542/peds.2014-2295
9. Mittal S, Marlowe L, Blakeslee S, et al. Successful use of quality improvement methodology to reduce inpatient length of stay in bronchiolitis through judicious use of intermittent pulse oximetry. Hosp Pediatr. 2019;9(2):73-78. https://doi.org/10.1542/hpeds.2018-0023
10. Heneghan M, Hart J, Dewan M, et al. No Cause for Alarm: Decreasing inappropriate pulse oximetry use in bronchiolitis. Hosp Pediatr. 2018;8(2):109-111. https://doi.org/10.1542/hpeds.2017-0126
11. Ralston S, Garber M, Narang S, et al. Decreasing unnecessary utilization in acute bronchiolitis care: results from the value in inpatient pediatrics network. J Hosp Med. 2013;8(1):25-30. https://doi.org/10.1002/jhm.1982
12. Bonafide CP, Xiao R, Brady PW, et al. Prevalence of continuous pulse oximetry monitoring in hospitalized children with bronchiolitis not requiring supplemental oxygen. JAMA. 2020;323(15):1467-1477. https://doi.org/10.1001/jama.2020.2998
13. van Bodegom-Vos L, Davidoff F, Marang-van de Mheen PJ. Implementation and de-implementation: two sides of the same coin? BMJ Qual Saf. 2017;26(6):495-501. https://doi.org/10.1136/bmjqs-2016-005473
14. McKay VR, Morshed AB, Brownson RC, Proctor EK, Prusaczyk B. Letting go: conceptualizing intervention de-implementation in public health and social service settings. Am J Community Psychol. 2018;62(1-2):189-202. https://doi.org/10.1002/ajcp.12258
15. Brownlee S, Chalkidou K, Doust J, et al. Evidence for overuse of medical services around the world. Lancet. 2017;390(10090):156-168. https://doi.org/10.1016/s0140-6736(16)32585-5
16. Chassin MR, Galvin RW. The urgent need to improve health care quality. Institute of Medicine National roundtable on health care quality. JAMA. 1998;280(11):1000-1005. https://doi.org/10.1001/jama.280.11.1000
17. Coon ER, Young PC, Quinonez RA, Morgan DJ, Dhruva SS, Schroeder AR. 2017 update on pediatric medical overuse: a review. JAMA Pediatr. 2018;172(5):482-486. https://doi.org/10.1001/jamapediatrics.2017.5752
18. Schuh S, Babl FE, Dalziel SR, et al; Pediatric Emergency Research Networks (PERN). Practice variation in acute bronchiolitis: a Pediatric Emergency Research Networks study. Pediatrics. 2017;140(6):e20170842. https://doi.org/10.1542/peds.2017-0842
19. Lewis-de Los Angeles WW, Thurm C, Hersh AL, et al. Trends in intravenous antibiotic duration for urinary tract infections in young infants. Pediatrics. 2017;140(6):e20171021. https://doi.org/10.1542/peds.2017-1021
20. Parikh K, Hall M, Mittal V, et al. Establishing benchmarks for the hospitalized care of children with asthma, bronchiolitis, and pneumonia. Pediatrics. 2014;134(3):555-562. https://doi.org/10.1542/peds.2014-1052
21. Ralston SL, Garber MD, Rice-Conboy E, et al; Value in Inpatient Pediatrics Network Quality Collaborative for Improving Hospital Compliance with AAP Bronchiolitis Guideline (BQIP). A multicenter collaborative to reduce unnecessary care in inpatient bronchiolitis. Pediatrics. 2016;137(1):e20150851. https://doi.org/10.1542/peds.2015-0851
22. Reyes MA, Etinger V, Hall M, et al. Impact of the Choosing Wisely((R)) Campaign recommendations for hospitalized children on clinical practice: trends from 2008 to 2017. J Hosp Med. 2020;15(2):68-74. https://doi.org/10.12788/jhm.3291
23. Norton WE, Chambers DA. Unpacking the complexities of de-implementing inappropriate health interventions. Implement Sci. 2020;15(1):2. https://doi.org/10.1186/s13012-019-0960-9
24. Damschroder LJ, Aron DC, Keith RE, Kirsh SR, Alexander JA, Lowery JC. Fostering implementation of health services research findings into practice: a consolidated framework for advancing implementation science. Implement Sci. 2009;4:50. https://doi.org/10.1186/1748-5908-4-50
25. Rasooly IR, Beidas RS, Wolk CB, et al. Measuring overuse of continuous pulse oximetry in bronchiolitis and developing strategies for large-scale deimplementation: study protocol for a feasibility trial. Pilot Feasibility Stud. 2019;5:68. https://doi.org/10.1186/s40814-019-0453-2
26. Bradley EH, Curry LA, Devers KJ. Qualitative data analysis for health services research: developing taxonomy, themes, and theory. Health Serv Res. 2007;42(4):1758-1772. https://doi.org/10.1111/j.1475-6773.2006.00684.x
27. Glaser BG, Strauss AL. The Discovery of Grounded Theory: Strategies for Qualitative Research. Aldine Pub. Co.; 1967.
28. Charmaz K. Grounded Theory: Objectivist and Constructivist Methods. In: Denzin NK, Lincoln Y, eds. Handbook of Qualitative Research. 2nd ed. Sage Publications; 2000:509-535.
29. Grimshaw JM, Russell IT. Effect of clinical guidelines on medical practice: a systematic review of rigorous evaluations. Lancet. 1993;342(8883):1317-1322. https://doi.org/10.1016/0140-6736(93)92244-n
30. Cabana MD, Rand CS, Powe NR, et al. Why don’t physicians follow clinical practice guidelines? a framework for improvement. JAMA. 1999;282(15):1458-1465. https://doi.org/10.1001/jama.282.15.1458
31. Dressler R, Dryer MM, Coletti C, Mahoney D, Doorey AJ. Altering overuse of cardiac telemetry in non-intensive care unit settings by hardwiring the use of American Heart Association guidelines. JAMA Intern Med. 2014;174(11):1852-1854. https://doi.org/10.1001/jamainternmed.2014.4491
32. Forrest CB, Fiks AG, Bailey LC, et al. Improving adherence to otitis media guidelines with clinical decision support and physician feedback. Pediatrics. 2013;131(4):e1071-e1081. https://doi.org/10.1542/peds.2012-1988
33. Fiks AG, Grundmeier RW, Mayne S, et al. Effectiveness of decision support for families, clinicians, or both on HPV vaccine receipt. Pediatrics. 2013;131(6):1114-1124. https://doi.org/10.1542/peds.2012-3122
34. Nolan T, Resar R, Griffin F, Gordon AB. Improving the Reliability of Health Care. Institute for Healthcare Improvement; 2004. http://www.ihi.org/resources/Pages/IHIWhitePapers/ImprovingtheReliabilityofHealthCare.aspx
35. Beidas RS, Kendall PC. Training Therapists in evidence-based practice: a critical review of studies from a systems-contextual perspective. Clin Psychol (New York). 2010;17(1):1-30. https://doi.org/10.1111/j.1468-2850.2009.01187.x
36. Chi KW, Coon ER, Destino L, Schroeder AR. Parental perspectives on continuous pulse oximetry use in bronchiolitis hospitalizations. Pediatrics. 2020;146(2):e20200130. https://doi.org/10.1542/peds.2020-0130
37. Hunt CE, Corwin MJ, Lister G, et al. Longitudinal assessment of hemoglobin oxygen saturation in healthy infants during the first 6 months of age. Collaborative Home Infant Monitoring Evaluation (CHIME) Study Group. J Pediatr. 1999;135(5):580-586. https://doi.org/10.1016/s0022-3476(99)70056-9
38. Mansbach JM, Clark S, Piedra PA, et al; MARC-30 Investigators. Hospital course and discharge criteria for children hospitalized with bronchiolitis. J Hosp Med. 2015;10(4):205-211. https://doi.org/10.1002/jhm.2318
39. Burton C, Williams L, Bucknall T, et al. Understanding how and why de-implementation works in health and care: research protocol for a realist synthesis of evidence. Syst Rev. 2019;8(1):194. https://doi.org/10.1186/s13643-019-1111-840. Mallory MD, Shay DK, Garrett J, Bordley WC. Bronchiolitis management preferences and the influence of pulse oximetry and respiratory rate on the decision to admit. Pediatrics. 2003;111(1):e45-51. https://doi.org/10.1542/peds.111.1.e45.
41. Schondelmeyer AC, Jenkins AM, Allison B, et al. Factors influencing use of continuous physiologic monitors for hospitalized pediatric patients. Hosp Pediatr. 2019;9(6):423-428. https://doi.org/10.1542/hpeds.2019-0007
42. Najafi N, Auerbach A. Use and outcomes of telemetry monitoring on a medicine service. Arch Intern Med. 2012;172(17):1349-1350. https://doi.org/10.1001/archinternmed.2012.3163
43. Estrada CA, Rosman HS, Prasad NK, et al. Role of telemetry monitoring in the non-intensive care unit. Am J Cardiol. 1995;76(12):960-965. https://doi.org/10.1016/s0002-9149(99)80270-7
Continuous pulse oximetry monitoring (cSpO2) in children with bronchiolitis is associated with increased rates of hospital admission, longer lengths of stay, more frequent treatment with supplemental oxygen, alarm fatigue, and higher hospital cost. There is no evidence that it improves clinical outcomes.1-7 The safety of reducing cSpO2 for stable bronchiolitis patients (ie, those who are clinically well and not requiring supplemental oxygen) has been assessed in quality improvement initiatives8-10 and a randomized controlled trial.2 These studies showed no increase in intensive care unit transfers, codes, or readmissions associated with reduced cSpO2. Current national guidelines from the American Academy of Pediatrics5 and the Society of Hospital Medicine Choosing Wisely in Pediatric Hospital Medicine workgroup4 support limiting monitoring of children with bronchiolitis. Despite this, the practice of cSpO2 in stable bronchiolitis patients off supplemental oxygen remains widespread.11,12
Deimplementation, defined as reducing or stopping low-value or ineffective healthcare practices,13,14 is a discrete focus area within implementation science. Deimplementation research involves the reduction of unnecessary and overused services for which there is potential for harm or no benefit.15,16 In pediatrics, there are a number of potential targets for deimplementation,4,17-20 including cSpO2 for stable infants with bronchiolitis, but efforts to reduce low-value practices have met limited success to date. 21,22
Implementation science offers rigorous methods for advancing the development and evaluation of strategies for deimplementation.23 In particular, implementation science frameworks can facilitate our understanding of relevant contextual factors that may hinder or help efforts to deimplement low-value practices. To develop broadly applicable strategies to reduce monitoring overuse, it is important to understand the barriers, facilitators, and contextual factors (eg, clinical, political, interpersonal) that contribute to guideline-discordant cSpO2 in hospitalized bronchiolitis patients. Further, the process by which one can develop a rigorous understanding of these factors and how they may impact deimplementation efforts could generalize to other scenarios in pediatrics where overuse remains an issue.
The goal of this study was to use semistructured interviews, informed by an established implementation science framework, specifically the Consolidated Framework for Implementation Research (CFIR),24 to (1) identify barriers and facilitators to deimplementing unnecessary cSpO2, and (2) develop strategies to deimplement cSpO2 in a multicenter cohort of hospital-based clinician and administrative stakeholders.
METHODS
Study Setting
This multicenter qualitative study using semistructured interviews took place within the Eliminating Monitor Overuse (EMO) SpO2 study. The EMO SpO2 study established rates of cSpO2 in bronchiolitis patients not receiving supplemental oxygen or not receiving room air flow at 56 hospitals across the United States and in Canada from December 1, 2018, through March 31, 2019.12 The study identified hospital-level risk-adjusted cSpO2 rates ranging from 6% to 82%. A description of the EMO SpO2 study methods25 and its findings12 have been published elsewhere.
Participants
We approached EMO study site principal investigators at 12 hospitals: the two highest- and two lowest-use hospitals within three hospital types (ie, freestanding children’s hospitals, children’s hospitals within large general hospitals, and community hospitals). We collaborated with the participating site principal investigators (n = 12), who were primarily hospitalist physicians in leadership roles, to recruit a purposive sample of additional stakeholders including bedside nurses (n = 12), hospitalist physicians (n = 15), respiratory therapists (n = 9), and hospital administrators (n = 8) to participate in semistructured interviews. Interviews were conducted until we achieved thematic saturation within each stakeholder group and within the high and low performing strata (total 56 interviews). Participants were asked to self-report basic demographic information (see Appendix, interview guide) as required by the study funder and to allow us to comment on the representativeness of the participant group.
Procedure
The interview guide was informed by the CFIR, a comprehensive framework detailing contextual factors that require consideration when planning for the implementation of a health service intervention. Table 1 details the CFIR domains with study-related examples. The interview guide (Appendix) provided limited clinical context apart from the age, diagnosis, and oxygen requirement for the population of interest to promote a broad array of responses and to avoid anchoring on specific clinical scenarios. Interviews were conducted by master’s degree or doctoral-level research coordinators with qualitative interviewing experience and supervised by a medical anthropologist and qualitative methods expert (F.K.B.). Prior to engaging in audio recorded phone interviews, the interviewer explained the risks and benefits of participating. Participants were compensated $50. Audio recordings were transcribed, deidentified, and uploaded to NVivo 12 Plus (QSR International) for data management.
The Institutional Review Boards of Children’s Hospital of Philadelphia, Pennsylvania, and the University of Pennsylvania in Philadelphia determined that the study met eligibility criteria for IRB exemption.
Data Analysis
Using an integrated approach to codebook development,26 a priori codes were developed using constructs from the CFIR. Additional codes were added by the research team following a close reading of the first five transcripts.27,28 Each code was defined, including decision rules for its application. Two research coordinators independently coded each transcript. Using the intercoder reliability function within NVivo, the coders established strong interrater reliability accordance scores (
RESULTS
Barriers and facilitators to deimplementation were identified in multiple domains of the CFIR: outer setting, inner setting, characteristics of the individuals, and intervention characteristics (Table 1). Participants also suggested strategies to facilitate deimplementation in response to some identified barriers. See Table 2 for participant demographics and Table 3 for illustrative participant quotations.
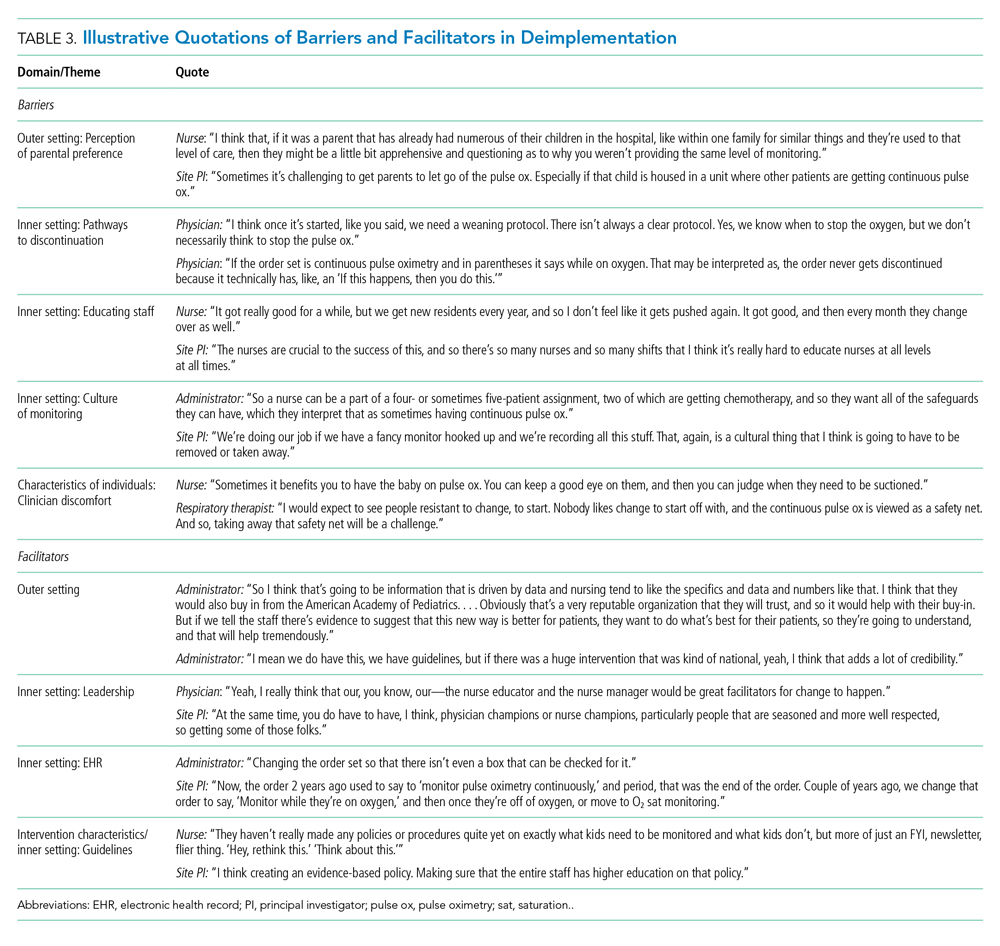
Barriers
Outer Setting: Clinician Perceptions of Parental Discomfort With Discontinuing Monitoring
Participants mentioned parental preferences as a barrier to discontinuing cSpO2, noting that parents seem to take comfort in watching the numbers on the monitor screen and are reluctant to have it withdrawn. Clinicians noted that parents sometimes put the monitor back on their child after a clinician removed it or have expressed concern that their unmonitored child was not receiving the same level of care as other patients who were being monitored. In these scenarios, clinicians reported they have found it helpful to educate caregivers about when cSpO2 is and is not appropriate.
Inner Setting: Unclear or Nonexistent Guideline to Discontinue cSpO2
Guidelines to discontinue cSpO2 reportedly did not exist at all institutions. If a guideline did exist, lack of clarity or conflicting guidelines about when to use oxygen presented a barrier. Participants suggested that a clear guideline or additional oversight to ensure all clinicians are informed of the procedure for discontinuing cSpO2 may help prevent miscommunication. Participants noted that their electronic health record (EHR) order sets commonly included cSpO2 orders and that removing that option would facilitate deimplementation.
Inner Setting: Difficulty Educating All Staff
Participants noted difficulty with incorporating education about discontinuing cSpO2 to all clinicians, particularly to those who are nightshift only or to rotating staff or trainees. This created barriers for frequent re-education because these staff are not familiar with the policies and procedures of the unit, which is crucial to developing a culture that supports the deimplementation of cSpO2. Participants suggested that recurring education about procedures for discontinuing cSpO2 should target trainees, new nurses, and overnight nurses. This would help to ensure that the guideline is uniformly followed.
Inner Setting: Culture of High cSpO2 Use
Participants from high-use sites discussed a culture driven by readily available monitoring features or an expectation that monitoring indicates higher-quality care. Participants from low-use sites discussed increased cSpO2 driven by clinicians who were accustomed to caring for higher-acuity patients, for whom continuous monitoring is likely appropriate, and were simultaneously caring for stable bronchiolitis patients.
Some suggested that visual cues would be useful to clinicians to sustain awareness about a cSpO2 deimplementation guideline. It was also suggested that audit and feedback techniques like posting unit deimplementation statistics and creating a competition among units by posting unit performance could facilitate deimplementation. Additionally, some noted that visual aids in common spaces would be useful to remind clinicians and to engage caregivers about discontinuing cSpO2.
Characteristics of Individuals: Clinician Discomfort Discontinuing cSpO2
One frequently cited barrier across participants is that cSpO2 provides “peace of mind” to alert clinicians to patients with low oxygen saturations that might otherwise be missed. Participants identified that clinician discomfort with reducing cSpO2 may be driven by inexperienced clinicians less familiar with the bronchiolitis disease process, such as trainees, new nurses, or rotating clinicians unaccustomed to pediatric care. Trainees and new nurses were perceived as being more likely to work at night when there are fewer clinicians to provide patient care. Additionally, participants perceived that night shift clinicians favored cSpO2 because they could measure vital signs without waking patients and families.
Clinicians discussed that discontinuing cSpO2 would require alternative methods for assessing patient status, particularly for night shift nurses. Participants suggested strategies including changes to pulse oximetry assessment procedures to include more frequent “spot checks,” incorporation of assessments during sleep events (eg, naps) to ensure the patient does not experience desaturations during sleep, and training nurses to become more comfortable with suctioning patients. Suggestions also included education on the typical features of transient oxygen desaturations in otherwise stable patients with bronchiolitis2 to bolster clinical confidence for clinicians unfamiliar with caring for bronchiolitis patients. Participants perceived that education about appropriate vs inappropriate use may help to empower clinicians to employ cSpO2 appropriately.
Facilitators
Outer Setting: Standards and Evidence From Research, Professional Organizations, and Leaders in the Field
Many participants expressed the importance of consistent guidelines that are advocated by thought leaders in the field, supported by robust evidence, and consistent with approaches at peer hospitals. The more authoritative support a guideline has, the more comfortable people are adopting it and taking it seriously. Additionally, consistent education about guidelines was desired. Participants noted that all clinicians should be receiving education related to the American Academy of Pediatrics (AAP) Bronchiolitis and Choosing Wisely® guidelines, ranging from a one-time update to annually. Continual updates and re-education sessions for clinicians who shared evidence about how cSpO2 deimplementation could improve the quality of patient care by shortening hospital length of stay and lowering cost were suggested strategies.
Inner Setting: Leadership
Participants noted that successful deimplementation depends upon the presence of a champion or educator who will be able to lead the institutional charge in making practice change. This is typically an individual who is trusted at the institution, experienced in their field, or already doing implementation work. This could be either a single individual (champion) or a team. The most commonly noted clinician roles to engage in a leadership role or team were physicians and nurses.
Participants noted that a change in related clinical care pathways or EHR order sets would require cooperation from multiple clinical disciplines, administrators, and information technology leaders and explained that messaging and education about the value of the change would facilitate buy-in from those clinicians.
Inner Setting: EHR Support for Guidelines
Participants often endorsed the use of an order set within the EHR that supports guidelines and includes reminders to decrease cSpO2. These reminders could come up when supplemental oxygen is discontinued or occur regularly throughout the patient’s stay to prompt the clinician to consider discontinuing cSpO2.
Intervention Characteristics/Inner Setting: Clear Bronchiolitis Guidelines
The presence of a well-articulated hospital policy that delineates the appropriate and inappropriate use of cSpO2 in bronchiolitis was mentioned as another facilitator of deimplementation.
DISCUSSION
Results of this qualitative study of stakeholders across hospitals with high and low cSpO2 use illustrated the complexities involved with deimplementation of cSpO2 in pediatric patients hospitalized with bronchiolitis. We identified numerous barriers spanning the CFIR constructs, including unclear or absent guidelines for stopping cSpO2, clinician knowledge and comfort with bronchiolitis disease features, and unit culture. This suggests that multicomponent strategies that target various domains and a variety of stakeholders are needed to deimplement cSpO2 use for stable bronchiolitis patients. Participants also identified facilitators, including clear cSpO2 guidelines, supportive leaders and champions, and EHR modifications, that provide insight into strategies that may help sites reduce their use of cSpO2. Additionally, participants also provided concrete, actionable suggestions for ways to reduce unnecessary monitoring that will be useful in informing promising deimplementation strategies for subsequent trials.
The importance of having specific and well-known guidelines from trusted sources, such as the AAP, about cSpO2 and bronchiolitis treatment that are thoughtfully integrated in the EHR came through in multiple themes of our analysis. Prior studies on the effect of guidelines on clinical practice have suggested that rigorously designed guidelines can positively impact practice.29 Participants also noted that cSpO2 guidelines should be authoritative and that knowledge of guideline adoption by peer institutions was a facilitator of adoption. Usability issues negatively impact clinicians’ ability to follow guidelines.30 Further, prior studies have demonstrated that EHR integration of guidelines can change practice.31-33 Based on our findings, incorporating clear guidelines into commonly used formats, such as EHR order sets, could be an important deimplementation tool for cSpO2 in stable bronchiolitis patients.
Education about and awareness of cSpO2 guidelines was described as an important facilitator for appropriate cSpO2 use and was suggested as a potential deimplementation strategy. Participants noted that educational need may vary by stakeholder group. For example, education may facilitate obtaining buy-in from hospital leaders, which is necessary to support changes to the EHR. Education incorporating information on the typical features of bronchiolitis and examples of appropriate and inappropriate cSpO2 use was suggested for clinical team members. The limitations of education as a stand-alone deimplementation strategy were also noted, and participants highlighted challenges such as time needed for education and the need for ongoing education for rotating trainees. Inner and outer setting barriers, such as a perceived “culture of high pulse oximetryuse” and patient and family expectations, could also make education less effective as a stand-alone strategy. That—coupled with evidence that education and training alone are generally insufficient for producing reliable, sustained behavior change34,35—suggests that a multifaceted approach will be important.
Our respondents consider parental perceptions and preference in their practice, which provides nuance to recent studies suggesting that parents prefer continuous monitors when their child is hospitalized with bronchiolitis. Chi et al described the impact of a brief educational intervention on parental preferences for monitoring children hospitalized for bronchiolitis.36 This work suggests that educational interventions aimed at families should be considered in future (de)implementation studies because they may indirectly impact clinician behavior. Future studies should directly assess parental discomfort with discontinuing monitoring. Participants highlighted the link between knowledge and confidence in caring for typical bronchiolitis patients and monitoring practice, perceiving that less experienced clinicians are more likely to rely on cSpO2. Participants at high-use sites emphasized the expectation that monitoring should occur during hospitalizations. This reflection is particularly pertinent for bronchiolitis, a disease characterized by frequent, self-resolving desaturations even after hospital discharge.3 This may reinforce a perceived need to capture and react to these desaturation events even though they are expected in bronchiolitis and can occur in healthy infants.37 Some participants suggested that continuous monitoring be replaced with “nap tests” (ie, assessment for desaturations during a nap prior to discharge); however, like cSpO2 in stable infants with bronchiolitis, this is another low-value practice. Otherwise healthy infants with mild to moderate disease are unlikely to subsequently worsen after showing signs of clinical improvement.38 Nap tests are likely to lead to infants who are clinically improving being placed unnecessarily back on oxygen in reaction to the transient desaturations. Participants’ perception about the importance of cSpO2 in bronchiolitis management, despite evidence suggesting it is a low-value practice, underscores the importance of not simply telling clinicians to stop cSpO2. Employing strategies that replace continuous monitoring with another acceptable and feasible alternative (eg, regular clinician assessments including intermittent pulse oximetry checks) should be considered when planning for deimplementation.39
Previous studies indicate that continuous monitoring can affect clinician decision-making, independent of other factors,6,40 despite limited evidence that continuous monitors improve patient outcomes.1-7 Studies have demonstrated noticeable increase in admissions based purely on pulse oximetry values,40 with no evidence that this type of admission changes outcomes for bronchiolitis patients.6 One previous, single-center study identified inexperience as a potential driver for monitor use,41 and studies in adult populations have suggested that clinicians overestimate the value that continuous monitoring contributes to patient care,42,43 which promotes guideline-discordant use. Our study provides novel insight into the issue of monitoring in bronchiolitis. Our results suggest that there is a need to shift organizational cultures around monitoring (which likely vary based on a range of factors) and that educational strategies addressing typical disease course, especially desaturations, in bronchiolitis will be an essential component in any deimplementation effort.
This study is strengthened by its sample of diverse stakeholder groups from multiple US health systems. Additionally, we interviewed individuals at sites with high cSpO2 rates and at sites with low rates, as well as from community hospitals, children’s hospitals within general hospitals, and freestanding children’s hospitals, which allows us to understand barriers high-use sites encounter and facilitators of lower cSpO2 rates at low-use sites. We also employed an interview approach informed by an established implementation science framework. Nonetheless, several limitations exist. First, participants at low-use sites did not necessarily have direct experience with a previous deimplementation effort to reduce cSpO2. Additionally, participants were predominantly White and female; more diverse perspectives would strengthen confidence in the generalizability of our findings. While thematic saturation was achieved within each stakeholder group and within the high- and low-use strata, we interviewed fewer administrators and respiratory therapists relative to other stakeholder groups. Nevertheless, our conclusions were validated by our interdisciplinary stakeholder panel. As noted by participants, family preferences may influence clinician practice, and parents were not interviewed for this study. The information gleaned from the present study will inform the development of strategies to deimplement unnecessary cSpO2 in pediatric hospitals, which we aim to rigorously evaluate in a future trial.
CONCLUSION
We identified barriers and facilitators to deimplementation of cSpO2 for stable patients with bronchiolitis across children’s hospitals with high and low utilization of cSpO2. These themes map to multiple CFIR domains and, along with participant-suggested strategies, can directly inform an approach to cSpO2 deimplementation in a range of inpatient settings. Based on these data, future deimplementation efforts should focus on clear protocols for use and discontinuation of cSpO2, EHR changes, and regular bronchiolitis education for hospital staff that emphasizes reducing unnecessary cSpO2 utilization.
ACKNOWLEDGMENTS
We acknowledge the NHLBI scientists who contributed their expertise to this project as part of the U01 Cooperative Agreement funding mechanism as federal employees conducting their official job duties: Lora Reineck, MD, MS, Karen Bienstock, MS, and Cheryl Boyce, PhD. We thank the Executive Council of the Pediatric Research in Inpatient Settings (PRIS) Network for their contributions to the early scientific development of this project. The Network assessed a Collaborative Support Fee for access to the hospitals and support of this project. We thank the PRIS Network collaborators for their major contributions to data collection measuring utilization to identify the hospitals we subsequently chose for this project. We thank Claire Bocage and the Mixed Methods Research Lab for major help in data management and data analysis.
Continuous pulse oximetry monitoring (cSpO2) in children with bronchiolitis is associated with increased rates of hospital admission, longer lengths of stay, more frequent treatment with supplemental oxygen, alarm fatigue, and higher hospital cost. There is no evidence that it improves clinical outcomes.1-7 The safety of reducing cSpO2 for stable bronchiolitis patients (ie, those who are clinically well and not requiring supplemental oxygen) has been assessed in quality improvement initiatives8-10 and a randomized controlled trial.2 These studies showed no increase in intensive care unit transfers, codes, or readmissions associated with reduced cSpO2. Current national guidelines from the American Academy of Pediatrics5 and the Society of Hospital Medicine Choosing Wisely in Pediatric Hospital Medicine workgroup4 support limiting monitoring of children with bronchiolitis. Despite this, the practice of cSpO2 in stable bronchiolitis patients off supplemental oxygen remains widespread.11,12
Deimplementation, defined as reducing or stopping low-value or ineffective healthcare practices,13,14 is a discrete focus area within implementation science. Deimplementation research involves the reduction of unnecessary and overused services for which there is potential for harm or no benefit.15,16 In pediatrics, there are a number of potential targets for deimplementation,4,17-20 including cSpO2 for stable infants with bronchiolitis, but efforts to reduce low-value practices have met limited success to date. 21,22
Implementation science offers rigorous methods for advancing the development and evaluation of strategies for deimplementation.23 In particular, implementation science frameworks can facilitate our understanding of relevant contextual factors that may hinder or help efforts to deimplement low-value practices. To develop broadly applicable strategies to reduce monitoring overuse, it is important to understand the barriers, facilitators, and contextual factors (eg, clinical, political, interpersonal) that contribute to guideline-discordant cSpO2 in hospitalized bronchiolitis patients. Further, the process by which one can develop a rigorous understanding of these factors and how they may impact deimplementation efforts could generalize to other scenarios in pediatrics where overuse remains an issue.
The goal of this study was to use semistructured interviews, informed by an established implementation science framework, specifically the Consolidated Framework for Implementation Research (CFIR),24 to (1) identify barriers and facilitators to deimplementing unnecessary cSpO2, and (2) develop strategies to deimplement cSpO2 in a multicenter cohort of hospital-based clinician and administrative stakeholders.
METHODS
Study Setting
This multicenter qualitative study using semistructured interviews took place within the Eliminating Monitor Overuse (EMO) SpO2 study. The EMO SpO2 study established rates of cSpO2 in bronchiolitis patients not receiving supplemental oxygen or not receiving room air flow at 56 hospitals across the United States and in Canada from December 1, 2018, through March 31, 2019.12 The study identified hospital-level risk-adjusted cSpO2 rates ranging from 6% to 82%. A description of the EMO SpO2 study methods25 and its findings12 have been published elsewhere.
Participants
We approached EMO study site principal investigators at 12 hospitals: the two highest- and two lowest-use hospitals within three hospital types (ie, freestanding children’s hospitals, children’s hospitals within large general hospitals, and community hospitals). We collaborated with the participating site principal investigators (n = 12), who were primarily hospitalist physicians in leadership roles, to recruit a purposive sample of additional stakeholders including bedside nurses (n = 12), hospitalist physicians (n = 15), respiratory therapists (n = 9), and hospital administrators (n = 8) to participate in semistructured interviews. Interviews were conducted until we achieved thematic saturation within each stakeholder group and within the high and low performing strata (total 56 interviews). Participants were asked to self-report basic demographic information (see Appendix, interview guide) as required by the study funder and to allow us to comment on the representativeness of the participant group.
Procedure
The interview guide was informed by the CFIR, a comprehensive framework detailing contextual factors that require consideration when planning for the implementation of a health service intervention. Table 1 details the CFIR domains with study-related examples. The interview guide (Appendix) provided limited clinical context apart from the age, diagnosis, and oxygen requirement for the population of interest to promote a broad array of responses and to avoid anchoring on specific clinical scenarios. Interviews were conducted by master’s degree or doctoral-level research coordinators with qualitative interviewing experience and supervised by a medical anthropologist and qualitative methods expert (F.K.B.). Prior to engaging in audio recorded phone interviews, the interviewer explained the risks and benefits of participating. Participants were compensated $50. Audio recordings were transcribed, deidentified, and uploaded to NVivo 12 Plus (QSR International) for data management.
The Institutional Review Boards of Children’s Hospital of Philadelphia, Pennsylvania, and the University of Pennsylvania in Philadelphia determined that the study met eligibility criteria for IRB exemption.
Data Analysis
Using an integrated approach to codebook development,26 a priori codes were developed using constructs from the CFIR. Additional codes were added by the research team following a close reading of the first five transcripts.27,28 Each code was defined, including decision rules for its application. Two research coordinators independently coded each transcript. Using the intercoder reliability function within NVivo, the coders established strong interrater reliability accordance scores (
RESULTS
Barriers and facilitators to deimplementation were identified in multiple domains of the CFIR: outer setting, inner setting, characteristics of the individuals, and intervention characteristics (Table 1). Participants also suggested strategies to facilitate deimplementation in response to some identified barriers. See Table 2 for participant demographics and Table 3 for illustrative participant quotations.
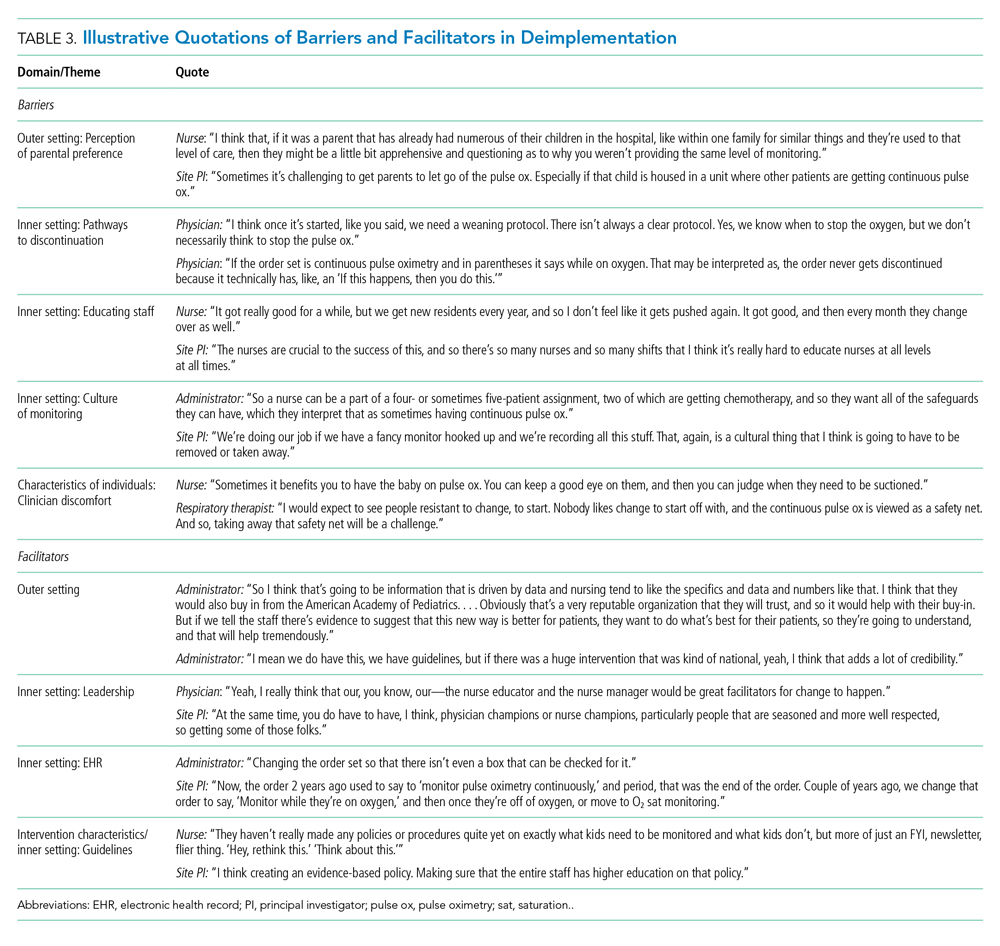
Barriers
Outer Setting: Clinician Perceptions of Parental Discomfort With Discontinuing Monitoring
Participants mentioned parental preferences as a barrier to discontinuing cSpO2, noting that parents seem to take comfort in watching the numbers on the monitor screen and are reluctant to have it withdrawn. Clinicians noted that parents sometimes put the monitor back on their child after a clinician removed it or have expressed concern that their unmonitored child was not receiving the same level of care as other patients who were being monitored. In these scenarios, clinicians reported they have found it helpful to educate caregivers about when cSpO2 is and is not appropriate.
Inner Setting: Unclear or Nonexistent Guideline to Discontinue cSpO2
Guidelines to discontinue cSpO2 reportedly did not exist at all institutions. If a guideline did exist, lack of clarity or conflicting guidelines about when to use oxygen presented a barrier. Participants suggested that a clear guideline or additional oversight to ensure all clinicians are informed of the procedure for discontinuing cSpO2 may help prevent miscommunication. Participants noted that their electronic health record (EHR) order sets commonly included cSpO2 orders and that removing that option would facilitate deimplementation.
Inner Setting: Difficulty Educating All Staff
Participants noted difficulty with incorporating education about discontinuing cSpO2 to all clinicians, particularly to those who are nightshift only or to rotating staff or trainees. This created barriers for frequent re-education because these staff are not familiar with the policies and procedures of the unit, which is crucial to developing a culture that supports the deimplementation of cSpO2. Participants suggested that recurring education about procedures for discontinuing cSpO2 should target trainees, new nurses, and overnight nurses. This would help to ensure that the guideline is uniformly followed.
Inner Setting: Culture of High cSpO2 Use
Participants from high-use sites discussed a culture driven by readily available monitoring features or an expectation that monitoring indicates higher-quality care. Participants from low-use sites discussed increased cSpO2 driven by clinicians who were accustomed to caring for higher-acuity patients, for whom continuous monitoring is likely appropriate, and were simultaneously caring for stable bronchiolitis patients.
Some suggested that visual cues would be useful to clinicians to sustain awareness about a cSpO2 deimplementation guideline. It was also suggested that audit and feedback techniques like posting unit deimplementation statistics and creating a competition among units by posting unit performance could facilitate deimplementation. Additionally, some noted that visual aids in common spaces would be useful to remind clinicians and to engage caregivers about discontinuing cSpO2.
Characteristics of Individuals: Clinician Discomfort Discontinuing cSpO2
One frequently cited barrier across participants is that cSpO2 provides “peace of mind” to alert clinicians to patients with low oxygen saturations that might otherwise be missed. Participants identified that clinician discomfort with reducing cSpO2 may be driven by inexperienced clinicians less familiar with the bronchiolitis disease process, such as trainees, new nurses, or rotating clinicians unaccustomed to pediatric care. Trainees and new nurses were perceived as being more likely to work at night when there are fewer clinicians to provide patient care. Additionally, participants perceived that night shift clinicians favored cSpO2 because they could measure vital signs without waking patients and families.
Clinicians discussed that discontinuing cSpO2 would require alternative methods for assessing patient status, particularly for night shift nurses. Participants suggested strategies including changes to pulse oximetry assessment procedures to include more frequent “spot checks,” incorporation of assessments during sleep events (eg, naps) to ensure the patient does not experience desaturations during sleep, and training nurses to become more comfortable with suctioning patients. Suggestions also included education on the typical features of transient oxygen desaturations in otherwise stable patients with bronchiolitis2 to bolster clinical confidence for clinicians unfamiliar with caring for bronchiolitis patients. Participants perceived that education about appropriate vs inappropriate use may help to empower clinicians to employ cSpO2 appropriately.
Facilitators
Outer Setting: Standards and Evidence From Research, Professional Organizations, and Leaders in the Field
Many participants expressed the importance of consistent guidelines that are advocated by thought leaders in the field, supported by robust evidence, and consistent with approaches at peer hospitals. The more authoritative support a guideline has, the more comfortable people are adopting it and taking it seriously. Additionally, consistent education about guidelines was desired. Participants noted that all clinicians should be receiving education related to the American Academy of Pediatrics (AAP) Bronchiolitis and Choosing Wisely® guidelines, ranging from a one-time update to annually. Continual updates and re-education sessions for clinicians who shared evidence about how cSpO2 deimplementation could improve the quality of patient care by shortening hospital length of stay and lowering cost were suggested strategies.
Inner Setting: Leadership
Participants noted that successful deimplementation depends upon the presence of a champion or educator who will be able to lead the institutional charge in making practice change. This is typically an individual who is trusted at the institution, experienced in their field, or already doing implementation work. This could be either a single individual (champion) or a team. The most commonly noted clinician roles to engage in a leadership role or team were physicians and nurses.
Participants noted that a change in related clinical care pathways or EHR order sets would require cooperation from multiple clinical disciplines, administrators, and information technology leaders and explained that messaging and education about the value of the change would facilitate buy-in from those clinicians.
Inner Setting: EHR Support for Guidelines
Participants often endorsed the use of an order set within the EHR that supports guidelines and includes reminders to decrease cSpO2. These reminders could come up when supplemental oxygen is discontinued or occur regularly throughout the patient’s stay to prompt the clinician to consider discontinuing cSpO2.
Intervention Characteristics/Inner Setting: Clear Bronchiolitis Guidelines
The presence of a well-articulated hospital policy that delineates the appropriate and inappropriate use of cSpO2 in bronchiolitis was mentioned as another facilitator of deimplementation.
DISCUSSION
Results of this qualitative study of stakeholders across hospitals with high and low cSpO2 use illustrated the complexities involved with deimplementation of cSpO2 in pediatric patients hospitalized with bronchiolitis. We identified numerous barriers spanning the CFIR constructs, including unclear or absent guidelines for stopping cSpO2, clinician knowledge and comfort with bronchiolitis disease features, and unit culture. This suggests that multicomponent strategies that target various domains and a variety of stakeholders are needed to deimplement cSpO2 use for stable bronchiolitis patients. Participants also identified facilitators, including clear cSpO2 guidelines, supportive leaders and champions, and EHR modifications, that provide insight into strategies that may help sites reduce their use of cSpO2. Additionally, participants also provided concrete, actionable suggestions for ways to reduce unnecessary monitoring that will be useful in informing promising deimplementation strategies for subsequent trials.
The importance of having specific and well-known guidelines from trusted sources, such as the AAP, about cSpO2 and bronchiolitis treatment that are thoughtfully integrated in the EHR came through in multiple themes of our analysis. Prior studies on the effect of guidelines on clinical practice have suggested that rigorously designed guidelines can positively impact practice.29 Participants also noted that cSpO2 guidelines should be authoritative and that knowledge of guideline adoption by peer institutions was a facilitator of adoption. Usability issues negatively impact clinicians’ ability to follow guidelines.30 Further, prior studies have demonstrated that EHR integration of guidelines can change practice.31-33 Based on our findings, incorporating clear guidelines into commonly used formats, such as EHR order sets, could be an important deimplementation tool for cSpO2 in stable bronchiolitis patients.
Education about and awareness of cSpO2 guidelines was described as an important facilitator for appropriate cSpO2 use and was suggested as a potential deimplementation strategy. Participants noted that educational need may vary by stakeholder group. For example, education may facilitate obtaining buy-in from hospital leaders, which is necessary to support changes to the EHR. Education incorporating information on the typical features of bronchiolitis and examples of appropriate and inappropriate cSpO2 use was suggested for clinical team members. The limitations of education as a stand-alone deimplementation strategy were also noted, and participants highlighted challenges such as time needed for education and the need for ongoing education for rotating trainees. Inner and outer setting barriers, such as a perceived “culture of high pulse oximetryuse” and patient and family expectations, could also make education less effective as a stand-alone strategy. That—coupled with evidence that education and training alone are generally insufficient for producing reliable, sustained behavior change34,35—suggests that a multifaceted approach will be important.
Our respondents consider parental perceptions and preference in their practice, which provides nuance to recent studies suggesting that parents prefer continuous monitors when their child is hospitalized with bronchiolitis. Chi et al described the impact of a brief educational intervention on parental preferences for monitoring children hospitalized for bronchiolitis.36 This work suggests that educational interventions aimed at families should be considered in future (de)implementation studies because they may indirectly impact clinician behavior. Future studies should directly assess parental discomfort with discontinuing monitoring. Participants highlighted the link between knowledge and confidence in caring for typical bronchiolitis patients and monitoring practice, perceiving that less experienced clinicians are more likely to rely on cSpO2. Participants at high-use sites emphasized the expectation that monitoring should occur during hospitalizations. This reflection is particularly pertinent for bronchiolitis, a disease characterized by frequent, self-resolving desaturations even after hospital discharge.3 This may reinforce a perceived need to capture and react to these desaturation events even though they are expected in bronchiolitis and can occur in healthy infants.37 Some participants suggested that continuous monitoring be replaced with “nap tests” (ie, assessment for desaturations during a nap prior to discharge); however, like cSpO2 in stable infants with bronchiolitis, this is another low-value practice. Otherwise healthy infants with mild to moderate disease are unlikely to subsequently worsen after showing signs of clinical improvement.38 Nap tests are likely to lead to infants who are clinically improving being placed unnecessarily back on oxygen in reaction to the transient desaturations. Participants’ perception about the importance of cSpO2 in bronchiolitis management, despite evidence suggesting it is a low-value practice, underscores the importance of not simply telling clinicians to stop cSpO2. Employing strategies that replace continuous monitoring with another acceptable and feasible alternative (eg, regular clinician assessments including intermittent pulse oximetry checks) should be considered when planning for deimplementation.39
Previous studies indicate that continuous monitoring can affect clinician decision-making, independent of other factors,6,40 despite limited evidence that continuous monitors improve patient outcomes.1-7 Studies have demonstrated noticeable increase in admissions based purely on pulse oximetry values,40 with no evidence that this type of admission changes outcomes for bronchiolitis patients.6 One previous, single-center study identified inexperience as a potential driver for monitor use,41 and studies in adult populations have suggested that clinicians overestimate the value that continuous monitoring contributes to patient care,42,43 which promotes guideline-discordant use. Our study provides novel insight into the issue of monitoring in bronchiolitis. Our results suggest that there is a need to shift organizational cultures around monitoring (which likely vary based on a range of factors) and that educational strategies addressing typical disease course, especially desaturations, in bronchiolitis will be an essential component in any deimplementation effort.
This study is strengthened by its sample of diverse stakeholder groups from multiple US health systems. Additionally, we interviewed individuals at sites with high cSpO2 rates and at sites with low rates, as well as from community hospitals, children’s hospitals within general hospitals, and freestanding children’s hospitals, which allows us to understand barriers high-use sites encounter and facilitators of lower cSpO2 rates at low-use sites. We also employed an interview approach informed by an established implementation science framework. Nonetheless, several limitations exist. First, participants at low-use sites did not necessarily have direct experience with a previous deimplementation effort to reduce cSpO2. Additionally, participants were predominantly White and female; more diverse perspectives would strengthen confidence in the generalizability of our findings. While thematic saturation was achieved within each stakeholder group and within the high- and low-use strata, we interviewed fewer administrators and respiratory therapists relative to other stakeholder groups. Nevertheless, our conclusions were validated by our interdisciplinary stakeholder panel. As noted by participants, family preferences may influence clinician practice, and parents were not interviewed for this study. The information gleaned from the present study will inform the development of strategies to deimplement unnecessary cSpO2 in pediatric hospitals, which we aim to rigorously evaluate in a future trial.
CONCLUSION
We identified barriers and facilitators to deimplementation of cSpO2 for stable patients with bronchiolitis across children’s hospitals with high and low utilization of cSpO2. These themes map to multiple CFIR domains and, along with participant-suggested strategies, can directly inform an approach to cSpO2 deimplementation in a range of inpatient settings. Based on these data, future deimplementation efforts should focus on clear protocols for use and discontinuation of cSpO2, EHR changes, and regular bronchiolitis education for hospital staff that emphasizes reducing unnecessary cSpO2 utilization.
ACKNOWLEDGMENTS
We acknowledge the NHLBI scientists who contributed their expertise to this project as part of the U01 Cooperative Agreement funding mechanism as federal employees conducting their official job duties: Lora Reineck, MD, MS, Karen Bienstock, MS, and Cheryl Boyce, PhD. We thank the Executive Council of the Pediatric Research in Inpatient Settings (PRIS) Network for their contributions to the early scientific development of this project. The Network assessed a Collaborative Support Fee for access to the hospitals and support of this project. We thank the PRIS Network collaborators for their major contributions to data collection measuring utilization to identify the hospitals we subsequently chose for this project. We thank Claire Bocage and the Mixed Methods Research Lab for major help in data management and data analysis.
1. Cunningham S, Rodriguez A, Adams T, et al; Bronchiolitis of Infancy Discharge Study (BIDS) group. Oxygen saturation targets in infants with bronchiolitis (BIDS): a double-blind, randomised, equivalence trial. Lancet. 2015;386(9998):1041-1048. https://doi.org/10.1016/s0140-6736(15)00163-4
2. McCulloh R, Koster M, Ralston S, et al. Use of intermittent vs continuous pulse oximetry for nonhypoxemic infants and young children hospitalized for bronchiolitis: a randomized clinical trial. JAMA Pediatr. 2015;169(10):898-904. https://doi.org/10.1001/jamapediatrics.2015.1746
3. Principi T, Coates AL, Parkin PC, Stephens D, DaSilva Z, Schuh S. Effect of oxygen desaturations on subsequent medical visits in infants discharged from the emergency department with bronchiolitis. JAMA Pediatr. 2016;170(6):602-608. https://doi.org/10.1001/jamapediatrics.2016.0114
4. Quinonez RA, Garber MD, Schroeder AR, et al. Choosing wisely in pediatric hospital medicine: five opportunities for improved healthcare value. J Hosp Med. 2013;8(9):479-485. Quinonez RA, Garber MD, Schroeder AR, et al. Choosing wisely in pediatric hospital medicine: five opportunities for improved healthcare value. J Hosp Med. 2013;8(9):479-485. https://doi.org/10.1002/jhm.2064
5. Ralston SL, Lieberthal AS, Meissner HC, et al. Clinical practice guideline: the diagnosis, management, and prevention of bronchiolitis. Pediatrics. 2014;134(5):e1474-e1502. https://doi.org/10.1542/peds.2014-2742
6. Schuh S, Freedman S, Coates A, et al. Effect of oximetry on hospitalization in bronchiolitis: a randomized clinical trial. JAMA. 2014;312(7):712-718. https://doi.org/10.1001/jama.2014.8637
7. Schuh S, Kwong JC, Holder L, Graves E, Macdonald EM, Finkelstein Y. Predictors of critical care and mortality in bronchiolitis after emergency department discharge. J Pediatr. 2018;199:217-222 e211. https://doi.org/10.1016/j.jpeds.2018.04.010
8. Schondelmeyer AC, Simmons JM, Statile AM, et al. Using quality improvement to reduce continuous pulse oximetry use in children with wheezing. Pediatrics. 2015;135(4):e1044-e1051. https://doi.org/10.1542/peds.2014-2295
9. Mittal S, Marlowe L, Blakeslee S, et al. Successful use of quality improvement methodology to reduce inpatient length of stay in bronchiolitis through judicious use of intermittent pulse oximetry. Hosp Pediatr. 2019;9(2):73-78. https://doi.org/10.1542/hpeds.2018-0023
10. Heneghan M, Hart J, Dewan M, et al. No Cause for Alarm: Decreasing inappropriate pulse oximetry use in bronchiolitis. Hosp Pediatr. 2018;8(2):109-111. https://doi.org/10.1542/hpeds.2017-0126
11. Ralston S, Garber M, Narang S, et al. Decreasing unnecessary utilization in acute bronchiolitis care: results from the value in inpatient pediatrics network. J Hosp Med. 2013;8(1):25-30. https://doi.org/10.1002/jhm.1982
12. Bonafide CP, Xiao R, Brady PW, et al. Prevalence of continuous pulse oximetry monitoring in hospitalized children with bronchiolitis not requiring supplemental oxygen. JAMA. 2020;323(15):1467-1477. https://doi.org/10.1001/jama.2020.2998
13. van Bodegom-Vos L, Davidoff F, Marang-van de Mheen PJ. Implementation and de-implementation: two sides of the same coin? BMJ Qual Saf. 2017;26(6):495-501. https://doi.org/10.1136/bmjqs-2016-005473
14. McKay VR, Morshed AB, Brownson RC, Proctor EK, Prusaczyk B. Letting go: conceptualizing intervention de-implementation in public health and social service settings. Am J Community Psychol. 2018;62(1-2):189-202. https://doi.org/10.1002/ajcp.12258
15. Brownlee S, Chalkidou K, Doust J, et al. Evidence for overuse of medical services around the world. Lancet. 2017;390(10090):156-168. https://doi.org/10.1016/s0140-6736(16)32585-5
16. Chassin MR, Galvin RW. The urgent need to improve health care quality. Institute of Medicine National roundtable on health care quality. JAMA. 1998;280(11):1000-1005. https://doi.org/10.1001/jama.280.11.1000
17. Coon ER, Young PC, Quinonez RA, Morgan DJ, Dhruva SS, Schroeder AR. 2017 update on pediatric medical overuse: a review. JAMA Pediatr. 2018;172(5):482-486. https://doi.org/10.1001/jamapediatrics.2017.5752
18. Schuh S, Babl FE, Dalziel SR, et al; Pediatric Emergency Research Networks (PERN). Practice variation in acute bronchiolitis: a Pediatric Emergency Research Networks study. Pediatrics. 2017;140(6):e20170842. https://doi.org/10.1542/peds.2017-0842
19. Lewis-de Los Angeles WW, Thurm C, Hersh AL, et al. Trends in intravenous antibiotic duration for urinary tract infections in young infants. Pediatrics. 2017;140(6):e20171021. https://doi.org/10.1542/peds.2017-1021
20. Parikh K, Hall M, Mittal V, et al. Establishing benchmarks for the hospitalized care of children with asthma, bronchiolitis, and pneumonia. Pediatrics. 2014;134(3):555-562. https://doi.org/10.1542/peds.2014-1052
21. Ralston SL, Garber MD, Rice-Conboy E, et al; Value in Inpatient Pediatrics Network Quality Collaborative for Improving Hospital Compliance with AAP Bronchiolitis Guideline (BQIP). A multicenter collaborative to reduce unnecessary care in inpatient bronchiolitis. Pediatrics. 2016;137(1):e20150851. https://doi.org/10.1542/peds.2015-0851
22. Reyes MA, Etinger V, Hall M, et al. Impact of the Choosing Wisely((R)) Campaign recommendations for hospitalized children on clinical practice: trends from 2008 to 2017. J Hosp Med. 2020;15(2):68-74. https://doi.org/10.12788/jhm.3291
23. Norton WE, Chambers DA. Unpacking the complexities of de-implementing inappropriate health interventions. Implement Sci. 2020;15(1):2. https://doi.org/10.1186/s13012-019-0960-9
24. Damschroder LJ, Aron DC, Keith RE, Kirsh SR, Alexander JA, Lowery JC. Fostering implementation of health services research findings into practice: a consolidated framework for advancing implementation science. Implement Sci. 2009;4:50. https://doi.org/10.1186/1748-5908-4-50
25. Rasooly IR, Beidas RS, Wolk CB, et al. Measuring overuse of continuous pulse oximetry in bronchiolitis and developing strategies for large-scale deimplementation: study protocol for a feasibility trial. Pilot Feasibility Stud. 2019;5:68. https://doi.org/10.1186/s40814-019-0453-2
26. Bradley EH, Curry LA, Devers KJ. Qualitative data analysis for health services research: developing taxonomy, themes, and theory. Health Serv Res. 2007;42(4):1758-1772. https://doi.org/10.1111/j.1475-6773.2006.00684.x
27. Glaser BG, Strauss AL. The Discovery of Grounded Theory: Strategies for Qualitative Research. Aldine Pub. Co.; 1967.
28. Charmaz K. Grounded Theory: Objectivist and Constructivist Methods. In: Denzin NK, Lincoln Y, eds. Handbook of Qualitative Research. 2nd ed. Sage Publications; 2000:509-535.
29. Grimshaw JM, Russell IT. Effect of clinical guidelines on medical practice: a systematic review of rigorous evaluations. Lancet. 1993;342(8883):1317-1322. https://doi.org/10.1016/0140-6736(93)92244-n
30. Cabana MD, Rand CS, Powe NR, et al. Why don’t physicians follow clinical practice guidelines? a framework for improvement. JAMA. 1999;282(15):1458-1465. https://doi.org/10.1001/jama.282.15.1458
31. Dressler R, Dryer MM, Coletti C, Mahoney D, Doorey AJ. Altering overuse of cardiac telemetry in non-intensive care unit settings by hardwiring the use of American Heart Association guidelines. JAMA Intern Med. 2014;174(11):1852-1854. https://doi.org/10.1001/jamainternmed.2014.4491
32. Forrest CB, Fiks AG, Bailey LC, et al. Improving adherence to otitis media guidelines with clinical decision support and physician feedback. Pediatrics. 2013;131(4):e1071-e1081. https://doi.org/10.1542/peds.2012-1988
33. Fiks AG, Grundmeier RW, Mayne S, et al. Effectiveness of decision support for families, clinicians, or both on HPV vaccine receipt. Pediatrics. 2013;131(6):1114-1124. https://doi.org/10.1542/peds.2012-3122
34. Nolan T, Resar R, Griffin F, Gordon AB. Improving the Reliability of Health Care. Institute for Healthcare Improvement; 2004. http://www.ihi.org/resources/Pages/IHIWhitePapers/ImprovingtheReliabilityofHealthCare.aspx
35. Beidas RS, Kendall PC. Training Therapists in evidence-based practice: a critical review of studies from a systems-contextual perspective. Clin Psychol (New York). 2010;17(1):1-30. https://doi.org/10.1111/j.1468-2850.2009.01187.x
36. Chi KW, Coon ER, Destino L, Schroeder AR. Parental perspectives on continuous pulse oximetry use in bronchiolitis hospitalizations. Pediatrics. 2020;146(2):e20200130. https://doi.org/10.1542/peds.2020-0130
37. Hunt CE, Corwin MJ, Lister G, et al. Longitudinal assessment of hemoglobin oxygen saturation in healthy infants during the first 6 months of age. Collaborative Home Infant Monitoring Evaluation (CHIME) Study Group. J Pediatr. 1999;135(5):580-586. https://doi.org/10.1016/s0022-3476(99)70056-9
38. Mansbach JM, Clark S, Piedra PA, et al; MARC-30 Investigators. Hospital course and discharge criteria for children hospitalized with bronchiolitis. J Hosp Med. 2015;10(4):205-211. https://doi.org/10.1002/jhm.2318
39. Burton C, Williams L, Bucknall T, et al. Understanding how and why de-implementation works in health and care: research protocol for a realist synthesis of evidence. Syst Rev. 2019;8(1):194. https://doi.org/10.1186/s13643-019-1111-840. Mallory MD, Shay DK, Garrett J, Bordley WC. Bronchiolitis management preferences and the influence of pulse oximetry and respiratory rate on the decision to admit. Pediatrics. 2003;111(1):e45-51. https://doi.org/10.1542/peds.111.1.e45.
41. Schondelmeyer AC, Jenkins AM, Allison B, et al. Factors influencing use of continuous physiologic monitors for hospitalized pediatric patients. Hosp Pediatr. 2019;9(6):423-428. https://doi.org/10.1542/hpeds.2019-0007
42. Najafi N, Auerbach A. Use and outcomes of telemetry monitoring on a medicine service. Arch Intern Med. 2012;172(17):1349-1350. https://doi.org/10.1001/archinternmed.2012.3163
43. Estrada CA, Rosman HS, Prasad NK, et al. Role of telemetry monitoring in the non-intensive care unit. Am J Cardiol. 1995;76(12):960-965. https://doi.org/10.1016/s0002-9149(99)80270-7
1. Cunningham S, Rodriguez A, Adams T, et al; Bronchiolitis of Infancy Discharge Study (BIDS) group. Oxygen saturation targets in infants with bronchiolitis (BIDS): a double-blind, randomised, equivalence trial. Lancet. 2015;386(9998):1041-1048. https://doi.org/10.1016/s0140-6736(15)00163-4
2. McCulloh R, Koster M, Ralston S, et al. Use of intermittent vs continuous pulse oximetry for nonhypoxemic infants and young children hospitalized for bronchiolitis: a randomized clinical trial. JAMA Pediatr. 2015;169(10):898-904. https://doi.org/10.1001/jamapediatrics.2015.1746
3. Principi T, Coates AL, Parkin PC, Stephens D, DaSilva Z, Schuh S. Effect of oxygen desaturations on subsequent medical visits in infants discharged from the emergency department with bronchiolitis. JAMA Pediatr. 2016;170(6):602-608. https://doi.org/10.1001/jamapediatrics.2016.0114
4. Quinonez RA, Garber MD, Schroeder AR, et al. Choosing wisely in pediatric hospital medicine: five opportunities for improved healthcare value. J Hosp Med. 2013;8(9):479-485. Quinonez RA, Garber MD, Schroeder AR, et al. Choosing wisely in pediatric hospital medicine: five opportunities for improved healthcare value. J Hosp Med. 2013;8(9):479-485. https://doi.org/10.1002/jhm.2064
5. Ralston SL, Lieberthal AS, Meissner HC, et al. Clinical practice guideline: the diagnosis, management, and prevention of bronchiolitis. Pediatrics. 2014;134(5):e1474-e1502. https://doi.org/10.1542/peds.2014-2742
6. Schuh S, Freedman S, Coates A, et al. Effect of oximetry on hospitalization in bronchiolitis: a randomized clinical trial. JAMA. 2014;312(7):712-718. https://doi.org/10.1001/jama.2014.8637
7. Schuh S, Kwong JC, Holder L, Graves E, Macdonald EM, Finkelstein Y. Predictors of critical care and mortality in bronchiolitis after emergency department discharge. J Pediatr. 2018;199:217-222 e211. https://doi.org/10.1016/j.jpeds.2018.04.010
8. Schondelmeyer AC, Simmons JM, Statile AM, et al. Using quality improvement to reduce continuous pulse oximetry use in children with wheezing. Pediatrics. 2015;135(4):e1044-e1051. https://doi.org/10.1542/peds.2014-2295
9. Mittal S, Marlowe L, Blakeslee S, et al. Successful use of quality improvement methodology to reduce inpatient length of stay in bronchiolitis through judicious use of intermittent pulse oximetry. Hosp Pediatr. 2019;9(2):73-78. https://doi.org/10.1542/hpeds.2018-0023
10. Heneghan M, Hart J, Dewan M, et al. No Cause for Alarm: Decreasing inappropriate pulse oximetry use in bronchiolitis. Hosp Pediatr. 2018;8(2):109-111. https://doi.org/10.1542/hpeds.2017-0126
11. Ralston S, Garber M, Narang S, et al. Decreasing unnecessary utilization in acute bronchiolitis care: results from the value in inpatient pediatrics network. J Hosp Med. 2013;8(1):25-30. https://doi.org/10.1002/jhm.1982
12. Bonafide CP, Xiao R, Brady PW, et al. Prevalence of continuous pulse oximetry monitoring in hospitalized children with bronchiolitis not requiring supplemental oxygen. JAMA. 2020;323(15):1467-1477. https://doi.org/10.1001/jama.2020.2998
13. van Bodegom-Vos L, Davidoff F, Marang-van de Mheen PJ. Implementation and de-implementation: two sides of the same coin? BMJ Qual Saf. 2017;26(6):495-501. https://doi.org/10.1136/bmjqs-2016-005473
14. McKay VR, Morshed AB, Brownson RC, Proctor EK, Prusaczyk B. Letting go: conceptualizing intervention de-implementation in public health and social service settings. Am J Community Psychol. 2018;62(1-2):189-202. https://doi.org/10.1002/ajcp.12258
15. Brownlee S, Chalkidou K, Doust J, et al. Evidence for overuse of medical services around the world. Lancet. 2017;390(10090):156-168. https://doi.org/10.1016/s0140-6736(16)32585-5
16. Chassin MR, Galvin RW. The urgent need to improve health care quality. Institute of Medicine National roundtable on health care quality. JAMA. 1998;280(11):1000-1005. https://doi.org/10.1001/jama.280.11.1000
17. Coon ER, Young PC, Quinonez RA, Morgan DJ, Dhruva SS, Schroeder AR. 2017 update on pediatric medical overuse: a review. JAMA Pediatr. 2018;172(5):482-486. https://doi.org/10.1001/jamapediatrics.2017.5752
18. Schuh S, Babl FE, Dalziel SR, et al; Pediatric Emergency Research Networks (PERN). Practice variation in acute bronchiolitis: a Pediatric Emergency Research Networks study. Pediatrics. 2017;140(6):e20170842. https://doi.org/10.1542/peds.2017-0842
19. Lewis-de Los Angeles WW, Thurm C, Hersh AL, et al. Trends in intravenous antibiotic duration for urinary tract infections in young infants. Pediatrics. 2017;140(6):e20171021. https://doi.org/10.1542/peds.2017-1021
20. Parikh K, Hall M, Mittal V, et al. Establishing benchmarks for the hospitalized care of children with asthma, bronchiolitis, and pneumonia. Pediatrics. 2014;134(3):555-562. https://doi.org/10.1542/peds.2014-1052
21. Ralston SL, Garber MD, Rice-Conboy E, et al; Value in Inpatient Pediatrics Network Quality Collaborative for Improving Hospital Compliance with AAP Bronchiolitis Guideline (BQIP). A multicenter collaborative to reduce unnecessary care in inpatient bronchiolitis. Pediatrics. 2016;137(1):e20150851. https://doi.org/10.1542/peds.2015-0851
22. Reyes MA, Etinger V, Hall M, et al. Impact of the Choosing Wisely((R)) Campaign recommendations for hospitalized children on clinical practice: trends from 2008 to 2017. J Hosp Med. 2020;15(2):68-74. https://doi.org/10.12788/jhm.3291
23. Norton WE, Chambers DA. Unpacking the complexities of de-implementing inappropriate health interventions. Implement Sci. 2020;15(1):2. https://doi.org/10.1186/s13012-019-0960-9
24. Damschroder LJ, Aron DC, Keith RE, Kirsh SR, Alexander JA, Lowery JC. Fostering implementation of health services research findings into practice: a consolidated framework for advancing implementation science. Implement Sci. 2009;4:50. https://doi.org/10.1186/1748-5908-4-50
25. Rasooly IR, Beidas RS, Wolk CB, et al. Measuring overuse of continuous pulse oximetry in bronchiolitis and developing strategies for large-scale deimplementation: study protocol for a feasibility trial. Pilot Feasibility Stud. 2019;5:68. https://doi.org/10.1186/s40814-019-0453-2
26. Bradley EH, Curry LA, Devers KJ. Qualitative data analysis for health services research: developing taxonomy, themes, and theory. Health Serv Res. 2007;42(4):1758-1772. https://doi.org/10.1111/j.1475-6773.2006.00684.x
27. Glaser BG, Strauss AL. The Discovery of Grounded Theory: Strategies for Qualitative Research. Aldine Pub. Co.; 1967.
28. Charmaz K. Grounded Theory: Objectivist and Constructivist Methods. In: Denzin NK, Lincoln Y, eds. Handbook of Qualitative Research. 2nd ed. Sage Publications; 2000:509-535.
29. Grimshaw JM, Russell IT. Effect of clinical guidelines on medical practice: a systematic review of rigorous evaluations. Lancet. 1993;342(8883):1317-1322. https://doi.org/10.1016/0140-6736(93)92244-n
30. Cabana MD, Rand CS, Powe NR, et al. Why don’t physicians follow clinical practice guidelines? a framework for improvement. JAMA. 1999;282(15):1458-1465. https://doi.org/10.1001/jama.282.15.1458
31. Dressler R, Dryer MM, Coletti C, Mahoney D, Doorey AJ. Altering overuse of cardiac telemetry in non-intensive care unit settings by hardwiring the use of American Heart Association guidelines. JAMA Intern Med. 2014;174(11):1852-1854. https://doi.org/10.1001/jamainternmed.2014.4491
32. Forrest CB, Fiks AG, Bailey LC, et al. Improving adherence to otitis media guidelines with clinical decision support and physician feedback. Pediatrics. 2013;131(4):e1071-e1081. https://doi.org/10.1542/peds.2012-1988
33. Fiks AG, Grundmeier RW, Mayne S, et al. Effectiveness of decision support for families, clinicians, or both on HPV vaccine receipt. Pediatrics. 2013;131(6):1114-1124. https://doi.org/10.1542/peds.2012-3122
34. Nolan T, Resar R, Griffin F, Gordon AB. Improving the Reliability of Health Care. Institute for Healthcare Improvement; 2004. http://www.ihi.org/resources/Pages/IHIWhitePapers/ImprovingtheReliabilityofHealthCare.aspx
35. Beidas RS, Kendall PC. Training Therapists in evidence-based practice: a critical review of studies from a systems-contextual perspective. Clin Psychol (New York). 2010;17(1):1-30. https://doi.org/10.1111/j.1468-2850.2009.01187.x
36. Chi KW, Coon ER, Destino L, Schroeder AR. Parental perspectives on continuous pulse oximetry use in bronchiolitis hospitalizations. Pediatrics. 2020;146(2):e20200130. https://doi.org/10.1542/peds.2020-0130
37. Hunt CE, Corwin MJ, Lister G, et al. Longitudinal assessment of hemoglobin oxygen saturation in healthy infants during the first 6 months of age. Collaborative Home Infant Monitoring Evaluation (CHIME) Study Group. J Pediatr. 1999;135(5):580-586. https://doi.org/10.1016/s0022-3476(99)70056-9
38. Mansbach JM, Clark S, Piedra PA, et al; MARC-30 Investigators. Hospital course and discharge criteria for children hospitalized with bronchiolitis. J Hosp Med. 2015;10(4):205-211. https://doi.org/10.1002/jhm.2318
39. Burton C, Williams L, Bucknall T, et al. Understanding how and why de-implementation works in health and care: research protocol for a realist synthesis of evidence. Syst Rev. 2019;8(1):194. https://doi.org/10.1186/s13643-019-1111-840. Mallory MD, Shay DK, Garrett J, Bordley WC. Bronchiolitis management preferences and the influence of pulse oximetry and respiratory rate on the decision to admit. Pediatrics. 2003;111(1):e45-51. https://doi.org/10.1542/peds.111.1.e45.
41. Schondelmeyer AC, Jenkins AM, Allison B, et al. Factors influencing use of continuous physiologic monitors for hospitalized pediatric patients. Hosp Pediatr. 2019;9(6):423-428. https://doi.org/10.1542/hpeds.2019-0007
42. Najafi N, Auerbach A. Use and outcomes of telemetry monitoring on a medicine service. Arch Intern Med. 2012;172(17):1349-1350. https://doi.org/10.1001/archinternmed.2012.3163
43. Estrada CA, Rosman HS, Prasad NK, et al. Role of telemetry monitoring in the non-intensive care unit. Am J Cardiol. 1995;76(12):960-965. https://doi.org/10.1016/s0002-9149(99)80270-7
© 2021 Society of Hospital Medicine
Email: amanda.schondelmeyer@cchmc.org; Telephone: 513-803-9158; Twitter: @SchondelmeyerMD.
Comparing Two Proximal Measures of Unrecognized Clinical Deterioration in Children
Unrecognized in-hospital clinical deterioration can lead to substantial morbidity and mortality.1 As a result, hospitals have implemented systems to identify and mitigate this form of potentially preventable harm.2-4 Cardiopulmonary arrest rates are useful metrics to evaluate the effectiveness of systems designed to identify and respond to deteriorating adult patients.5 Pediatric arrests outside of the intensive care unit (ICU) are rare; therefore, the identification of valid and more frequent proximal measures of deterioration is critical to the assessment of current systems and to guide future improvement efforts.6
Bonafide et al developed and validated the critical deterioration event (CDE) metric, demonstrating that children who were transferred to the ICU and who received noninvasive ventilation, intubation, or vasopressor initiation within 12 hours of transfer had an over 13-fold increased risk of in-hospital mortality.7 Implementation of a rapid response system was subsequently associated with a decrease in the trajectory of CDEs.2 At Cincinnati Children’s Hospital Medical Center (CCHMC), an additional proximal outcome measure was developed for unrecognized clinical deterioration: emergency transfers (ETs).8,9 An event meets criteria for an ET when the patient undergoes intubation, inotropic support, or three or more fluid boluses in the first hour after arrival or prior to ICU transfer.9 Recently, ETs were associated with an increased in-hospital mortality, ICU length of stay, and post-transfer hospital length of stay when compared with nonemergent transfers.10,11
While both CDEs and ETs were associated with adverse outcomes in children and may be modifiable through better rapid response systems, researchers have not previously compared the extent to which CDEs and ETs capture similar versus distinct events. Furthermore, the ability of focused situation awareness interventions to identify high-risk patients has not previously been assessed. Situation awareness is defined as the perception of elements in the environment, the comprehension of their meaning, and the projection of their status in the near future.12 Clinically, improved situation awareness can lead to earlier recognition of deterioration and a reduction in failure to rescue events.9 The objectives of this study were to (1) describe CDEs and ETs and assess for similarities, differences, and trends, and (2) evaluate the utility of situation awareness interventions to detect patients who experience these events.
METHODS
Setting and Inclusion Criteria
We conducted a retrospective cross-sectional study at CCHMC, a free-standing tertiary care children’s hospital. We included all patients cared for outside of the ICU during their hospitalization from January 2016 to July 2018. Transfer to the ICU included the pediatric and the cardiac ICUs.
Study Definitions
CDEs were events in which a patient received noninvasive ventilation, intubation, or vasopressor initiation within 12 hours of ICU transfer (Figure).7 ETs were events in which a patient underwent intubation, inotropes, or three or more fluid boluses in the first hour after arrival or before transfer (Figure).9 We examined two distinct situation awareness interventions: watcher identification and the pediatric early warning score (PEWS). A watcher is a situation awareness concern based on clinician perception, or “gut feeling,” that the patient is at high risk for deterioration.9,13 When clinicians designate a patient as a watcher in the electronic medical record, they establish an action plan, reassessment timeline, and objective criteria for activation of the rapid response team to assess the patient. Watcher patients are discussed at institution-wide safety huddles three times daily. The PEWS is a reproducible assessment of the patient’s status based on physiologic parameters, including behavior, cardiovascular, and respiratory assessments.3,4 At CCHMC, a Monaghan PEWS score is calculated with each assessment of vital signs.14 The bedside nurse calls the physician or advanced practice provider to assess the patient for a score of 4 or greater.
Event Identification and Classification
Two trained research nurses (C.F. and D.H.) manually reviewed all ICU transfers during the study period to determine if CDE criteria were met. Events meeting CDE criteria were classified as respiratory (requiring noninvasive or invasive ventilation), cardiac (requiring inotropes), or cardiopulmonary resuscitation (CPR) in which cardiac and respiratory interventions were initiated simultaneously. Additional information obtained included the time the patient met CDE criteria relative to the time of ICU transfer, watcher identification prior to the event, and the highest PEWS documented within 12 hours of the event. A physician (T.S.) performed manual chart review of each CDE as an additional validation step. ETs during the study period were obtained from an existing institutional database. ICU transfers meeting ET criteria are entered into this database in nearly real time by the inpatient nurse manager; this nurse attends all rapid response team calls and is aware of the disposition for each event. A physician (T.S.) performed manual chart review of each ET to determine event classification by intervention type, watcher identification, and the highest PEWS documented within 12 hours of the event. All CDEs and ETs were cross-referenced to determine overlap.
Outcome Measures and Statistical Analysis
The primary outcomes were CDEs and ETs, calculated as absolute counts and number of events per 10,000 non-ICU patient days. Events were classified by (1) category of intervention, (2) watcher identification prior to the event, and (3) PEWS of 4 or greater documented in the 12 hours prior to the event.
RESULTS
Incidence and Overlap of CDEs and ETs
There were 1,828 ICU transfers during the study period, of which 365 (20%) met criteria for a CDE, ET, or both. Among events captured, 359 (98.4%) met criteria for a CDE, occurring at a rate of 16.7 per 10,000 non-ICU patient days, and 88 (24.1%) met criteria for an ET, occurring at a rate of 4.1 per 10,000 non-ICU patient days (Table). Of the 88 ETs, 82 also met criteria for a CDE.
Timing and Categorization of CDEs and ETs
Despite the 12-hour time horizon, most CDEs (62.1%) met criteria within 1 hour of ICU transfer, and 79.9% met criteria within 3 hours (Figure). Respiratory events were most common for both CDEs (80.5%) and ETs (47.7%) (Table). Of respiratory CDEs, 67.4% required noninvasive ventilation, and 32.5% required invasive ventilation. Fluid or inotrope support were responsible for 11.7% of CDEs and nearly one-third of ETs; of note, the CDE definition does not include fluid boluses. Less than 10% of CDEs were characterized by CPR, whereas this accounted for 22.7% of ETs.
Identification of Events by Situation Awareness Interventions
The Table depicts the identification of events by watcher status and PEWS. All events were included for watcher identification, and events with a documented score in the 12 hours prior to transfer were included for PEWS. While half or less of the events were captured by watcher or PEWS separately, over 85% of events were captured by either one or both of the situation awareness interventions. The situation awareness interventions identified CDEs and ETs similarly.
DISCUSSION
This study is the first to classify and compare two proximal measures of clinical deterioration in children. Given that children with escalating respiratory symptoms are often treated successfully outside of the ICU, the findings that most events are respiratory in nature and occur within 1 hour of transfer are not unexpected. The analysis of situation awareness interventions suggests that neither watcher identification nor PEWS is independently sufficient to predict future deterioration. These findings support the necessity of both a clinician “gut feeling” and objective vital sign and physical exam findings to indicate a patient’s clinical status.9 Initiatives to improve the early recognition and mitigation of patient deterioration should focus on both tools to initiate an escalation of care, and work to understand gaps in these identification systems, which currently miss approximately 15% of acutely deteriorating patients. Although most patients had watcher identification or elevated PEWS prior to the event, they still required emergent life-sustaining care, which suggests that opportunities exist to improve mitigation and escalation pathways as a critical prevention effort.7,10
It is likely that CDEs and ETs are important outcome metrics in the evaluation of pediatric escalation systems, including rapid response systems.15 ETs are less common and more specific for unrecognized deterioration, which makes them a more feasible early metric for assessment. CDEs, which are likely more sensitive, may be useful in settings in which deterioration is rare or a more common outcome enhances power to detect the effect of interventions.10
This study has limitations and lends itself to future work. While CDEs and ETs are more common than cardiopulmonary arrest, they remain relatively uncommon. This was a single-site study at a large, tertiary care, free-standing children’s hospital, so generalizability to centers with different characteristics and patient populations may be limited. Future work should focus on comparing patient-level outcomes of CDEs and ETs, including length of stay and mortality. The determination of specific diagnoses and conditions associated with CDEs and ETs may inform targeted preventive improvement science interventions.
CONCLUSION
CDEs were roughly fourfold more common than ETs, with most CDEs occurring within 1 hour of ICU transfer. Most patients were identified by either watcher status or elevated PEWS, suggesting that these tools, when utilized as complementary situation awareness interventions, are important for identifying patients at risk for deterioration. Opportunities exist for improved escalation plans for patients identified as high-risk to prevent the need for emergent life-sustaining intervention.
1. Buist M, Bernard S, Nguyen TV, Moore G, Anderson J. Association between clinically abnormal observations and subsequent in-hospital mortality: a prospective study. Resuscitation. 2004;62(2):137-141. https://doi.org/10.1016/j.resuscitation.2004.03.005
2. Bonafide CP, Localio AR, Roberts KE, Nadkarni VM, Weirich CM, Keren R. Impact of rapid response system implementation on critical deterioration events in children. JAMA Pediatr. 2014;168(1):25-33. https://doi.org/10.1001/jamapediatrics.2013.3266
3. Duncan H, Hutchison J, Parshuram CS. The Pediatric Early Warning System score: a severity of illness score to predict urgent medical need in hospitalized children. J Crit Care. 2006;21(3):271-278. https://doi.org/10.1016/j.jcrc.2006.06.007
4. Sefton G, McGrath C, Tume L, Lane S, Lisboa PJ, Carrol ED. What impact did a Paediatric Early Warning system have on emergency admissions to the paediatric intensive care unit? an observational cohort study. Intensive Crit Care Nurs. 2015;31(2):91-99. https://doi.org/10.1016/j.iccn.2014.01.001
5. Schein RM, Hazday N, Pena M, Ruben BH, Sprung CL. Clinical antecedents to in-hospital cardiopulmonary arrest. Chest. 1990;98(6):1388-1392. https://doi.org/10.1378/chest.98.6.1388
6. Feudtner C, Berry JG, Parry G, et al. Statistical uncertainty of mortality rates and rankings for children’s hospitals. Pediatrics. 2011;128(4):e966-e972. https://doi.org/10.1542/peds.2010-3074
7. Bonafide CP, Roberts KE, Priestley MA, et al. Development of a pragmatic measure for evaluating and optimizing rapid response systems. Pediatrics. 2012;129(4):e874-e881. https://doi.org/10.1542/peds.2011-2784
8. Brady PW, Goldenhar LM. A qualitative study examining the influences on situation awareness and the identification, mitigation and escalation of recognised patient risk. BMJ Qual Saf. 2014;23(2):153-161. https://doi.org/10.1136/bmjqs-2012-001747
9. Brady PW, Muething S, Kotagal U, et al. Improving situation awareness to reduce unrecognized clinical deterioration and serious safety events. Pediatrics. 2013;131(1):e298-e308. https://doi.org/10.1542/peds.2012-1364
10. Hussain FS, Sosa T, Ambroggio L, Gallagher R, Brady PW. Emergency transfers: an important predictor of adverse outcomes in hospitalized children. J Hosp Med. 2019;14(8):482-485. https://doi.org/10.12788/jhm.3219
11. Aoki Y, Inata Y, Hatachi T, Shimizu Y, Takeuchi M. Outcomes of ‘unrecognised situation awareness failures events’ in intensive care unit transfer of children in a Japanese children’s hospital. J Paediatr Child Health. 2019;55(2):213-215. https://doi.org/10.1111/jpc.14185
12. Endsley MR. Toward a theory of situation awareness in dynamic systems. Human Factors. 1995;37(1):32-64. https://doi.org/10.1518/001872095779049543
13. McClain Smith M, Chumpia M, Wargo L, Nicol J, Bugnitz M. Watcher initiative associated with decrease in failure to rescue events in pediatric population. Hosp Pediatr. 2017;7(12):710-715. https://doi.org/10.1542/hpeds.2017-0042
14. Monaghan A. Detecting and managing deterioration in children. Paediatr Nurs. 2005;17(1):32-35. https://doi.org/10.7748/paed2005.02.17.1.32.c964
15. Subbe CP, Bannard-Smith J, Bunch J, et al. Quality metrics for the evaluation of Rapid Response Systems: proceedings from the third international consensus conference on Rapid Response Systems. Resuscitation. 2019;141:1-12. https://doi.org/10.1016/j.resuscitation.2019.05.012
Unrecognized in-hospital clinical deterioration can lead to substantial morbidity and mortality.1 As a result, hospitals have implemented systems to identify and mitigate this form of potentially preventable harm.2-4 Cardiopulmonary arrest rates are useful metrics to evaluate the effectiveness of systems designed to identify and respond to deteriorating adult patients.5 Pediatric arrests outside of the intensive care unit (ICU) are rare; therefore, the identification of valid and more frequent proximal measures of deterioration is critical to the assessment of current systems and to guide future improvement efforts.6
Bonafide et al developed and validated the critical deterioration event (CDE) metric, demonstrating that children who were transferred to the ICU and who received noninvasive ventilation, intubation, or vasopressor initiation within 12 hours of transfer had an over 13-fold increased risk of in-hospital mortality.7 Implementation of a rapid response system was subsequently associated with a decrease in the trajectory of CDEs.2 At Cincinnati Children’s Hospital Medical Center (CCHMC), an additional proximal outcome measure was developed for unrecognized clinical deterioration: emergency transfers (ETs).8,9 An event meets criteria for an ET when the patient undergoes intubation, inotropic support, or three or more fluid boluses in the first hour after arrival or prior to ICU transfer.9 Recently, ETs were associated with an increased in-hospital mortality, ICU length of stay, and post-transfer hospital length of stay when compared with nonemergent transfers.10,11
While both CDEs and ETs were associated with adverse outcomes in children and may be modifiable through better rapid response systems, researchers have not previously compared the extent to which CDEs and ETs capture similar versus distinct events. Furthermore, the ability of focused situation awareness interventions to identify high-risk patients has not previously been assessed. Situation awareness is defined as the perception of elements in the environment, the comprehension of their meaning, and the projection of their status in the near future.12 Clinically, improved situation awareness can lead to earlier recognition of deterioration and a reduction in failure to rescue events.9 The objectives of this study were to (1) describe CDEs and ETs and assess for similarities, differences, and trends, and (2) evaluate the utility of situation awareness interventions to detect patients who experience these events.
METHODS
Setting and Inclusion Criteria
We conducted a retrospective cross-sectional study at CCHMC, a free-standing tertiary care children’s hospital. We included all patients cared for outside of the ICU during their hospitalization from January 2016 to July 2018. Transfer to the ICU included the pediatric and the cardiac ICUs.
Study Definitions
CDEs were events in which a patient received noninvasive ventilation, intubation, or vasopressor initiation within 12 hours of ICU transfer (Figure).7 ETs were events in which a patient underwent intubation, inotropes, or three or more fluid boluses in the first hour after arrival or before transfer (Figure).9 We examined two distinct situation awareness interventions: watcher identification and the pediatric early warning score (PEWS). A watcher is a situation awareness concern based on clinician perception, or “gut feeling,” that the patient is at high risk for deterioration.9,13 When clinicians designate a patient as a watcher in the electronic medical record, they establish an action plan, reassessment timeline, and objective criteria for activation of the rapid response team to assess the patient. Watcher patients are discussed at institution-wide safety huddles three times daily. The PEWS is a reproducible assessment of the patient’s status based on physiologic parameters, including behavior, cardiovascular, and respiratory assessments.3,4 At CCHMC, a Monaghan PEWS score is calculated with each assessment of vital signs.14 The bedside nurse calls the physician or advanced practice provider to assess the patient for a score of 4 or greater.
Event Identification and Classification
Two trained research nurses (C.F. and D.H.) manually reviewed all ICU transfers during the study period to determine if CDE criteria were met. Events meeting CDE criteria were classified as respiratory (requiring noninvasive or invasive ventilation), cardiac (requiring inotropes), or cardiopulmonary resuscitation (CPR) in which cardiac and respiratory interventions were initiated simultaneously. Additional information obtained included the time the patient met CDE criteria relative to the time of ICU transfer, watcher identification prior to the event, and the highest PEWS documented within 12 hours of the event. A physician (T.S.) performed manual chart review of each CDE as an additional validation step. ETs during the study period were obtained from an existing institutional database. ICU transfers meeting ET criteria are entered into this database in nearly real time by the inpatient nurse manager; this nurse attends all rapid response team calls and is aware of the disposition for each event. A physician (T.S.) performed manual chart review of each ET to determine event classification by intervention type, watcher identification, and the highest PEWS documented within 12 hours of the event. All CDEs and ETs were cross-referenced to determine overlap.
Outcome Measures and Statistical Analysis
The primary outcomes were CDEs and ETs, calculated as absolute counts and number of events per 10,000 non-ICU patient days. Events were classified by (1) category of intervention, (2) watcher identification prior to the event, and (3) PEWS of 4 or greater documented in the 12 hours prior to the event.
RESULTS
Incidence and Overlap of CDEs and ETs
There were 1,828 ICU transfers during the study period, of which 365 (20%) met criteria for a CDE, ET, or both. Among events captured, 359 (98.4%) met criteria for a CDE, occurring at a rate of 16.7 per 10,000 non-ICU patient days, and 88 (24.1%) met criteria for an ET, occurring at a rate of 4.1 per 10,000 non-ICU patient days (Table). Of the 88 ETs, 82 also met criteria for a CDE.
Timing and Categorization of CDEs and ETs
Despite the 12-hour time horizon, most CDEs (62.1%) met criteria within 1 hour of ICU transfer, and 79.9% met criteria within 3 hours (Figure). Respiratory events were most common for both CDEs (80.5%) and ETs (47.7%) (Table). Of respiratory CDEs, 67.4% required noninvasive ventilation, and 32.5% required invasive ventilation. Fluid or inotrope support were responsible for 11.7% of CDEs and nearly one-third of ETs; of note, the CDE definition does not include fluid boluses. Less than 10% of CDEs were characterized by CPR, whereas this accounted for 22.7% of ETs.
Identification of Events by Situation Awareness Interventions
The Table depicts the identification of events by watcher status and PEWS. All events were included for watcher identification, and events with a documented score in the 12 hours prior to transfer were included for PEWS. While half or less of the events were captured by watcher or PEWS separately, over 85% of events were captured by either one or both of the situation awareness interventions. The situation awareness interventions identified CDEs and ETs similarly.
DISCUSSION
This study is the first to classify and compare two proximal measures of clinical deterioration in children. Given that children with escalating respiratory symptoms are often treated successfully outside of the ICU, the findings that most events are respiratory in nature and occur within 1 hour of transfer are not unexpected. The analysis of situation awareness interventions suggests that neither watcher identification nor PEWS is independently sufficient to predict future deterioration. These findings support the necessity of both a clinician “gut feeling” and objective vital sign and physical exam findings to indicate a patient’s clinical status.9 Initiatives to improve the early recognition and mitigation of patient deterioration should focus on both tools to initiate an escalation of care, and work to understand gaps in these identification systems, which currently miss approximately 15% of acutely deteriorating patients. Although most patients had watcher identification or elevated PEWS prior to the event, they still required emergent life-sustaining care, which suggests that opportunities exist to improve mitigation and escalation pathways as a critical prevention effort.7,10
It is likely that CDEs and ETs are important outcome metrics in the evaluation of pediatric escalation systems, including rapid response systems.15 ETs are less common and more specific for unrecognized deterioration, which makes them a more feasible early metric for assessment. CDEs, which are likely more sensitive, may be useful in settings in which deterioration is rare or a more common outcome enhances power to detect the effect of interventions.10
This study has limitations and lends itself to future work. While CDEs and ETs are more common than cardiopulmonary arrest, they remain relatively uncommon. This was a single-site study at a large, tertiary care, free-standing children’s hospital, so generalizability to centers with different characteristics and patient populations may be limited. Future work should focus on comparing patient-level outcomes of CDEs and ETs, including length of stay and mortality. The determination of specific diagnoses and conditions associated with CDEs and ETs may inform targeted preventive improvement science interventions.
CONCLUSION
CDEs were roughly fourfold more common than ETs, with most CDEs occurring within 1 hour of ICU transfer. Most patients were identified by either watcher status or elevated PEWS, suggesting that these tools, when utilized as complementary situation awareness interventions, are important for identifying patients at risk for deterioration. Opportunities exist for improved escalation plans for patients identified as high-risk to prevent the need for emergent life-sustaining intervention.
Unrecognized in-hospital clinical deterioration can lead to substantial morbidity and mortality.1 As a result, hospitals have implemented systems to identify and mitigate this form of potentially preventable harm.2-4 Cardiopulmonary arrest rates are useful metrics to evaluate the effectiveness of systems designed to identify and respond to deteriorating adult patients.5 Pediatric arrests outside of the intensive care unit (ICU) are rare; therefore, the identification of valid and more frequent proximal measures of deterioration is critical to the assessment of current systems and to guide future improvement efforts.6
Bonafide et al developed and validated the critical deterioration event (CDE) metric, demonstrating that children who were transferred to the ICU and who received noninvasive ventilation, intubation, or vasopressor initiation within 12 hours of transfer had an over 13-fold increased risk of in-hospital mortality.7 Implementation of a rapid response system was subsequently associated with a decrease in the trajectory of CDEs.2 At Cincinnati Children’s Hospital Medical Center (CCHMC), an additional proximal outcome measure was developed for unrecognized clinical deterioration: emergency transfers (ETs).8,9 An event meets criteria for an ET when the patient undergoes intubation, inotropic support, or three or more fluid boluses in the first hour after arrival or prior to ICU transfer.9 Recently, ETs were associated with an increased in-hospital mortality, ICU length of stay, and post-transfer hospital length of stay when compared with nonemergent transfers.10,11
While both CDEs and ETs were associated with adverse outcomes in children and may be modifiable through better rapid response systems, researchers have not previously compared the extent to which CDEs and ETs capture similar versus distinct events. Furthermore, the ability of focused situation awareness interventions to identify high-risk patients has not previously been assessed. Situation awareness is defined as the perception of elements in the environment, the comprehension of their meaning, and the projection of their status in the near future.12 Clinically, improved situation awareness can lead to earlier recognition of deterioration and a reduction in failure to rescue events.9 The objectives of this study were to (1) describe CDEs and ETs and assess for similarities, differences, and trends, and (2) evaluate the utility of situation awareness interventions to detect patients who experience these events.
METHODS
Setting and Inclusion Criteria
We conducted a retrospective cross-sectional study at CCHMC, a free-standing tertiary care children’s hospital. We included all patients cared for outside of the ICU during their hospitalization from January 2016 to July 2018. Transfer to the ICU included the pediatric and the cardiac ICUs.
Study Definitions
CDEs were events in which a patient received noninvasive ventilation, intubation, or vasopressor initiation within 12 hours of ICU transfer (Figure).7 ETs were events in which a patient underwent intubation, inotropes, or three or more fluid boluses in the first hour after arrival or before transfer (Figure).9 We examined two distinct situation awareness interventions: watcher identification and the pediatric early warning score (PEWS). A watcher is a situation awareness concern based on clinician perception, or “gut feeling,” that the patient is at high risk for deterioration.9,13 When clinicians designate a patient as a watcher in the electronic medical record, they establish an action plan, reassessment timeline, and objective criteria for activation of the rapid response team to assess the patient. Watcher patients are discussed at institution-wide safety huddles three times daily. The PEWS is a reproducible assessment of the patient’s status based on physiologic parameters, including behavior, cardiovascular, and respiratory assessments.3,4 At CCHMC, a Monaghan PEWS score is calculated with each assessment of vital signs.14 The bedside nurse calls the physician or advanced practice provider to assess the patient for a score of 4 or greater.
Event Identification and Classification
Two trained research nurses (C.F. and D.H.) manually reviewed all ICU transfers during the study period to determine if CDE criteria were met. Events meeting CDE criteria were classified as respiratory (requiring noninvasive or invasive ventilation), cardiac (requiring inotropes), or cardiopulmonary resuscitation (CPR) in which cardiac and respiratory interventions were initiated simultaneously. Additional information obtained included the time the patient met CDE criteria relative to the time of ICU transfer, watcher identification prior to the event, and the highest PEWS documented within 12 hours of the event. A physician (T.S.) performed manual chart review of each CDE as an additional validation step. ETs during the study period were obtained from an existing institutional database. ICU transfers meeting ET criteria are entered into this database in nearly real time by the inpatient nurse manager; this nurse attends all rapid response team calls and is aware of the disposition for each event. A physician (T.S.) performed manual chart review of each ET to determine event classification by intervention type, watcher identification, and the highest PEWS documented within 12 hours of the event. All CDEs and ETs were cross-referenced to determine overlap.
Outcome Measures and Statistical Analysis
The primary outcomes were CDEs and ETs, calculated as absolute counts and number of events per 10,000 non-ICU patient days. Events were classified by (1) category of intervention, (2) watcher identification prior to the event, and (3) PEWS of 4 or greater documented in the 12 hours prior to the event.
RESULTS
Incidence and Overlap of CDEs and ETs
There were 1,828 ICU transfers during the study period, of which 365 (20%) met criteria for a CDE, ET, or both. Among events captured, 359 (98.4%) met criteria for a CDE, occurring at a rate of 16.7 per 10,000 non-ICU patient days, and 88 (24.1%) met criteria for an ET, occurring at a rate of 4.1 per 10,000 non-ICU patient days (Table). Of the 88 ETs, 82 also met criteria for a CDE.
Timing and Categorization of CDEs and ETs
Despite the 12-hour time horizon, most CDEs (62.1%) met criteria within 1 hour of ICU transfer, and 79.9% met criteria within 3 hours (Figure). Respiratory events were most common for both CDEs (80.5%) and ETs (47.7%) (Table). Of respiratory CDEs, 67.4% required noninvasive ventilation, and 32.5% required invasive ventilation. Fluid or inotrope support were responsible for 11.7% of CDEs and nearly one-third of ETs; of note, the CDE definition does not include fluid boluses. Less than 10% of CDEs were characterized by CPR, whereas this accounted for 22.7% of ETs.
Identification of Events by Situation Awareness Interventions
The Table depicts the identification of events by watcher status and PEWS. All events were included for watcher identification, and events with a documented score in the 12 hours prior to transfer were included for PEWS. While half or less of the events were captured by watcher or PEWS separately, over 85% of events were captured by either one or both of the situation awareness interventions. The situation awareness interventions identified CDEs and ETs similarly.
DISCUSSION
This study is the first to classify and compare two proximal measures of clinical deterioration in children. Given that children with escalating respiratory symptoms are often treated successfully outside of the ICU, the findings that most events are respiratory in nature and occur within 1 hour of transfer are not unexpected. The analysis of situation awareness interventions suggests that neither watcher identification nor PEWS is independently sufficient to predict future deterioration. These findings support the necessity of both a clinician “gut feeling” and objective vital sign and physical exam findings to indicate a patient’s clinical status.9 Initiatives to improve the early recognition and mitigation of patient deterioration should focus on both tools to initiate an escalation of care, and work to understand gaps in these identification systems, which currently miss approximately 15% of acutely deteriorating patients. Although most patients had watcher identification or elevated PEWS prior to the event, they still required emergent life-sustaining care, which suggests that opportunities exist to improve mitigation and escalation pathways as a critical prevention effort.7,10
It is likely that CDEs and ETs are important outcome metrics in the evaluation of pediatric escalation systems, including rapid response systems.15 ETs are less common and more specific for unrecognized deterioration, which makes them a more feasible early metric for assessment. CDEs, which are likely more sensitive, may be useful in settings in which deterioration is rare or a more common outcome enhances power to detect the effect of interventions.10
This study has limitations and lends itself to future work. While CDEs and ETs are more common than cardiopulmonary arrest, they remain relatively uncommon. This was a single-site study at a large, tertiary care, free-standing children’s hospital, so generalizability to centers with different characteristics and patient populations may be limited. Future work should focus on comparing patient-level outcomes of CDEs and ETs, including length of stay and mortality. The determination of specific diagnoses and conditions associated with CDEs and ETs may inform targeted preventive improvement science interventions.
CONCLUSION
CDEs were roughly fourfold more common than ETs, with most CDEs occurring within 1 hour of ICU transfer. Most patients were identified by either watcher status or elevated PEWS, suggesting that these tools, when utilized as complementary situation awareness interventions, are important for identifying patients at risk for deterioration. Opportunities exist for improved escalation plans for patients identified as high-risk to prevent the need for emergent life-sustaining intervention.
1. Buist M, Bernard S, Nguyen TV, Moore G, Anderson J. Association between clinically abnormal observations and subsequent in-hospital mortality: a prospective study. Resuscitation. 2004;62(2):137-141. https://doi.org/10.1016/j.resuscitation.2004.03.005
2. Bonafide CP, Localio AR, Roberts KE, Nadkarni VM, Weirich CM, Keren R. Impact of rapid response system implementation on critical deterioration events in children. JAMA Pediatr. 2014;168(1):25-33. https://doi.org/10.1001/jamapediatrics.2013.3266
3. Duncan H, Hutchison J, Parshuram CS. The Pediatric Early Warning System score: a severity of illness score to predict urgent medical need in hospitalized children. J Crit Care. 2006;21(3):271-278. https://doi.org/10.1016/j.jcrc.2006.06.007
4. Sefton G, McGrath C, Tume L, Lane S, Lisboa PJ, Carrol ED. What impact did a Paediatric Early Warning system have on emergency admissions to the paediatric intensive care unit? an observational cohort study. Intensive Crit Care Nurs. 2015;31(2):91-99. https://doi.org/10.1016/j.iccn.2014.01.001
5. Schein RM, Hazday N, Pena M, Ruben BH, Sprung CL. Clinical antecedents to in-hospital cardiopulmonary arrest. Chest. 1990;98(6):1388-1392. https://doi.org/10.1378/chest.98.6.1388
6. Feudtner C, Berry JG, Parry G, et al. Statistical uncertainty of mortality rates and rankings for children’s hospitals. Pediatrics. 2011;128(4):e966-e972. https://doi.org/10.1542/peds.2010-3074
7. Bonafide CP, Roberts KE, Priestley MA, et al. Development of a pragmatic measure for evaluating and optimizing rapid response systems. Pediatrics. 2012;129(4):e874-e881. https://doi.org/10.1542/peds.2011-2784
8. Brady PW, Goldenhar LM. A qualitative study examining the influences on situation awareness and the identification, mitigation and escalation of recognised patient risk. BMJ Qual Saf. 2014;23(2):153-161. https://doi.org/10.1136/bmjqs-2012-001747
9. Brady PW, Muething S, Kotagal U, et al. Improving situation awareness to reduce unrecognized clinical deterioration and serious safety events. Pediatrics. 2013;131(1):e298-e308. https://doi.org/10.1542/peds.2012-1364
10. Hussain FS, Sosa T, Ambroggio L, Gallagher R, Brady PW. Emergency transfers: an important predictor of adverse outcomes in hospitalized children. J Hosp Med. 2019;14(8):482-485. https://doi.org/10.12788/jhm.3219
11. Aoki Y, Inata Y, Hatachi T, Shimizu Y, Takeuchi M. Outcomes of ‘unrecognised situation awareness failures events’ in intensive care unit transfer of children in a Japanese children’s hospital. J Paediatr Child Health. 2019;55(2):213-215. https://doi.org/10.1111/jpc.14185
12. Endsley MR. Toward a theory of situation awareness in dynamic systems. Human Factors. 1995;37(1):32-64. https://doi.org/10.1518/001872095779049543
13. McClain Smith M, Chumpia M, Wargo L, Nicol J, Bugnitz M. Watcher initiative associated with decrease in failure to rescue events in pediatric population. Hosp Pediatr. 2017;7(12):710-715. https://doi.org/10.1542/hpeds.2017-0042
14. Monaghan A. Detecting and managing deterioration in children. Paediatr Nurs. 2005;17(1):32-35. https://doi.org/10.7748/paed2005.02.17.1.32.c964
15. Subbe CP, Bannard-Smith J, Bunch J, et al. Quality metrics for the evaluation of Rapid Response Systems: proceedings from the third international consensus conference on Rapid Response Systems. Resuscitation. 2019;141:1-12. https://doi.org/10.1016/j.resuscitation.2019.05.012
1. Buist M, Bernard S, Nguyen TV, Moore G, Anderson J. Association between clinically abnormal observations and subsequent in-hospital mortality: a prospective study. Resuscitation. 2004;62(2):137-141. https://doi.org/10.1016/j.resuscitation.2004.03.005
2. Bonafide CP, Localio AR, Roberts KE, Nadkarni VM, Weirich CM, Keren R. Impact of rapid response system implementation on critical deterioration events in children. JAMA Pediatr. 2014;168(1):25-33. https://doi.org/10.1001/jamapediatrics.2013.3266
3. Duncan H, Hutchison J, Parshuram CS. The Pediatric Early Warning System score: a severity of illness score to predict urgent medical need in hospitalized children. J Crit Care. 2006;21(3):271-278. https://doi.org/10.1016/j.jcrc.2006.06.007
4. Sefton G, McGrath C, Tume L, Lane S, Lisboa PJ, Carrol ED. What impact did a Paediatric Early Warning system have on emergency admissions to the paediatric intensive care unit? an observational cohort study. Intensive Crit Care Nurs. 2015;31(2):91-99. https://doi.org/10.1016/j.iccn.2014.01.001
5. Schein RM, Hazday N, Pena M, Ruben BH, Sprung CL. Clinical antecedents to in-hospital cardiopulmonary arrest. Chest. 1990;98(6):1388-1392. https://doi.org/10.1378/chest.98.6.1388
6. Feudtner C, Berry JG, Parry G, et al. Statistical uncertainty of mortality rates and rankings for children’s hospitals. Pediatrics. 2011;128(4):e966-e972. https://doi.org/10.1542/peds.2010-3074
7. Bonafide CP, Roberts KE, Priestley MA, et al. Development of a pragmatic measure for evaluating and optimizing rapid response systems. Pediatrics. 2012;129(4):e874-e881. https://doi.org/10.1542/peds.2011-2784
8. Brady PW, Goldenhar LM. A qualitative study examining the influences on situation awareness and the identification, mitigation and escalation of recognised patient risk. BMJ Qual Saf. 2014;23(2):153-161. https://doi.org/10.1136/bmjqs-2012-001747
9. Brady PW, Muething S, Kotagal U, et al. Improving situation awareness to reduce unrecognized clinical deterioration and serious safety events. Pediatrics. 2013;131(1):e298-e308. https://doi.org/10.1542/peds.2012-1364
10. Hussain FS, Sosa T, Ambroggio L, Gallagher R, Brady PW. Emergency transfers: an important predictor of adverse outcomes in hospitalized children. J Hosp Med. 2019;14(8):482-485. https://doi.org/10.12788/jhm.3219
11. Aoki Y, Inata Y, Hatachi T, Shimizu Y, Takeuchi M. Outcomes of ‘unrecognised situation awareness failures events’ in intensive care unit transfer of children in a Japanese children’s hospital. J Paediatr Child Health. 2019;55(2):213-215. https://doi.org/10.1111/jpc.14185
12. Endsley MR. Toward a theory of situation awareness in dynamic systems. Human Factors. 1995;37(1):32-64. https://doi.org/10.1518/001872095779049543
13. McClain Smith M, Chumpia M, Wargo L, Nicol J, Bugnitz M. Watcher initiative associated with decrease in failure to rescue events in pediatric population. Hosp Pediatr. 2017;7(12):710-715. https://doi.org/10.1542/hpeds.2017-0042
14. Monaghan A. Detecting and managing deterioration in children. Paediatr Nurs. 2005;17(1):32-35. https://doi.org/10.7748/paed2005.02.17.1.32.c964
15. Subbe CP, Bannard-Smith J, Bunch J, et al. Quality metrics for the evaluation of Rapid Response Systems: proceedings from the third international consensus conference on Rapid Response Systems. Resuscitation. 2019;141:1-12. https://doi.org/10.1016/j.resuscitation.2019.05.012
© 2020 Society of Hospital Medicine
Validity of Continuous Pulse Oximetry Orders for Identification of Actual Monitoring Status in Bronchiolitis
As part of improvement collaboratives that aimed to reduce overuse of continuous pulse oximetry in children hospitalized with bronchiolitis, researchers used the presence of an active order for it as a proxy for the actual use of such monitoring.1,2 With use of this proxy, investigators on a national study documented a high burden of continuous oximetry overuse (86.5% before quality improvement interventions and 45.5% after),1 but the validity of orders in representing actual monitoring practice is unknown. If the presence of an active pulse oximetry order accurately identifies infants on monitors, electronic health record data could inform epidemiologic estimates of monitoring overuse and measure the success of quality improvement and deimplementation interventions. Alternatively, if nurses commonly begin and/or discontinue pulse oximetry without updated orders, a pulse oximetry order would not be an accurate proxy, and additional data capture methods (eg, bedside observation or data capture from bedside monitors) would be needed.
Understanding the validity of orders for detection of actual use is critical because continuous pulse oximetry monitoring is considered an overused practice in pediatric acute viral bronchiolitis,3 and national guidelines recommend against its use in low-risk hospitalized children.4,5 Continuous monitoring may identify trivial, self-resolving oxygen desaturation and its use is not associated with improved outcomes.6-9 When self-resolving desaturations are treated with additional supplemental oxygen, hospital stays may be unnecessarily prolonged.10 In order to reduce unnecessary continuous pulse oximetry use, measurement of the extent of the overused practice is necessary. In this 56-hospital study,11 we aimed to determine the validity of using active continuous pulse oximetry orders instead of bedside observation of actual monitor use.
METHODS
Design
In this multicenter, repeated cross-sectional study, investigators used direct bedside observation to determine continuous pulse oximetry monitor use and then assessed whether an active continuous monitoring order was present in the electronic health record. The study took place during one bronchiolitis season, December 1, 2018, through March 31, 2019.
Setting and Patients
Investigators at 56 freestanding children’s hospitals, children’s hospitals within general hospitals, and community hospitals in the Pediatric Research in Inpatient Settings (PRIS) Network collected data on infants aged 8 weeks to 23 months who were hospitalized with bronchiolitis. As this work was a substudy of the larger Eliminating Monitor Overuse study, only infants not currently receiving supplemental oxygen were included.11 Investigators observed eligible infants outside of the intensive care unit on general hospital medicine units. We excluded infants born premature (documented prematurity of <28 weeks’ gestation or documented “premature” without a gestational age listed), as well as those with a home oxygen requirement, cyanotic congenital heart disease, pulmonary hypertension, tracheostomy, primary neuromuscular disease, immunodeficiency, or cancer.
Data Collection
Investigators used the electronic health record to identify eligible infants. Investigators entered patient rooms to confirm the infant was not on supplemental oxygen (hence confirming eligibility for the study) and determine if continuous pulse oximetry was actively in use by examining the monitor display for a pulse oximetry waveform. Investigators then confirmed if active orders for pulse oximetry were present in the patient’s chart. Per study design, site investigators aimed to observe approximately half of eligible infants during the day (10
Analysis
We excluded patients with conditional orders (eg, monitored only when certain conditions exist, such as when asleep) because of the time-varying and wide range of conditions that could be specified. Furthermore, conditional orders would not be useful as proxies to measure oximetry use because investigators would still need additional data (eg, bedside observation) to determine current monitoring status.
We calculated the sensitivity, specificity, positive predictive value (PPV), and negative predictive value (NPV) of active orders using the reference standard of direct bedside observation, as well as corresponding 95% CIs that accounted for within-hospital clustering. We calculated these test characteristics overall and as stratified across four age groups: 8 weeks to 5 months, 6 months to 11 months, 12 months to 17 months, and 18 months to 23 months. We also calculated the test characteristics for each hospital. We decided a priori that a PPV and NPV of 80% would represent a reasonable threshold to use active orders as a proxy in multicenter research. For hospital-level analyses we included only hospitals with 60 or more total observations and more than 15 observations with active orders for PPV and more than 15 observations without active orders for NPV. We used Stata (StataCorp LLC, College Station, Texas) version 15.1 for analysis.
For US sites, the Institutional Review Board (IRB) at Children’s Hospital of Philadelphia approved the study as the single reviewing IRB, and the remaining US sites established reliance agreements with the reviewing IRB. Research Ethics Boards at the Canadian sites (University of Calgary and The Hospital for Sick Children) also reviewed and approved the study. All sites granted waivers of consent, assent, parental permission, and HIPAA authorization.
RESULTS
Investigators completed 3,612 observations in 56 hospitals. This included 33 freestanding children’s hospitals, 14 hospitals within large general hospitals, and 9 community hospitals. Of 3,612 completed observations, on 631 occasions (17%) patients had conditional orders (eg, continuous monitoring only when sleeping) and were excluded from further analysis.
Most pulse oximetry–monitored infants did not have an active monitoring order (670 out of 1,309; sensitivity of 49%). Test characteristics, stratified by age group, are presented in the Table. Across all observations, the overall PPV was 77% (95% CI, 72-82), and the overall NPV was 69% (95% CI, 61-77). Variation of all test characteristics across age group was small (eg, the sensitivity ranged from 43% to 51%).
With inclusion of only those hospitals with sufficient observations, hospital-level variation in the PPV and NPV of using active orders was substantial (PPV range of 48% to 96% and NPV range of 30% to 98%). Only two hospitals had both a PPV and NPV for using monitor orders that exceeded the 80% threshold.
DISCUSSION
Active continuous pulse oximetry orders did not accurately represent actual monitoring status in this study. Monitoring orders alone frequently misrepresent true monitoring status and, as such, should be interpreted with caution in research or quality improvement activities. If more valid estimates of monitoring use and overuse are needed, potential measurement options include direct observation, as used in our study, as well as the use of more complex data streams such as the output of monitoring devices or pulse oximetry data in the electronic health record. In only two of the hospitals, using active continuous monitoring orders was a reasonable proxy for detecting actual monitor use. Monitoring orders could potentially be validly used for deimplementation efforts at those centers; other hospitals could consider targeted improvement efforts (eg, morning huddles examining the discordance between monitoring orders and monitoring status) to improve the accuracy of using continuous pulse oximetry orders.
We acknowledge several limitations of this study. Site investigators employed a convenience sampling approach, so it is possible that some investigators observed sicker or less sick infants. Although the PRIS network includes a geographically diverse group of North American hospitals, community hospitals were underrepresented in this study. Our results hence generalize more precisely to freestanding children’s hospitals than to community hospitals. We did not observe infants currently on supplemental oxygen, so we do not know to what degree using orders is valid in that context. We did not collect data on why actual monitoring status differed from monitoring orders and hence cannot quantify to what extent different factors (eg, nurse belief that monitors are a safety net or infants inadvertently left on monitors after a spot check pulse oximetry reading) contributed to this discordance. Finally, our study only examined one electronic health record variable—the presence of an active order. It may be that other variables in the health record (eg, minute-by-minute pulse oximetry values in a vital sign flowsheet) are much better proxies of actual continuous monitor use.
CONCLUSION
Using an active order for continuous pulse oximetry has poor sensitivity, PPV, and NPV for detecting true monitoring status at the bedside. Teams intending to measure the actual use of pulse oximetry should be aware of the limitations of using active orders alone as an accurate measure of pulse oximetry monitoring.
Acknowledgments
We thank the NHLBI scientists who contributed to this project as part of the U01 Cooperative Agreement funding mechanism: Lora Reineck, MD, MS, Karen Bienstock, MS, and Cheryl Boyce, PhD.
We thank the Executive Council of the PRIS Network for their contributions to the early scientific development of this project. We thank the PRIS site investigators for their major contributions to the Eliminating Monitor Overuse (EMO) Study data collection. Each listed collaborator is a group author for the PRIS Network in this manuscript. Their names can be found in the online supplemental information.
1. Ralston SL, Garber MD, Rice-Conboy E, et al. A multicenter collaborative to reduce unnecessary care in inpatient bronchiolitis. Pediatrics. 2016;137(1). https://doi.org/10.1542/peds.2015-0851
2. Mittal S, Marlowe L, Blakeslee S, et al. Successful use of quality improvement methodology to reduce inpatient length of stay in bronchiolitis through judicious use of intermittent pulse oximetry. Hosp Pediatr. 2019;9(2):73-78. https://doi.org/10.1542/hpeds.2018-0023
3. Quinonez RA, Coon ER, Schroeder AR, Moyer VA. When technology creates uncertainty: pulse oximetry and overdiagnosis of hypoxaemia in bronchiolitis. BMJ. 2017;358:j3850. https://doi.org/10.1136/bmj.j3850
4. Quinonez RA, Garber MD, Schroeder AR, et al. Choosing wisely in pediatric hospital medicine: five opportunities for improved healthcare value. J Hosp Med. 2013;8(9):479-485. https://doi.org/10.1002/jhm.2064
5. Ralston SL, Lieberthal AS, Meissner HC, et al. Clinical practice guideline: the diagnosis, management, and prevention of bronchiolitis. Pediatrics. 2014;134(5):e1474-e1502. https://doi.org/10.1542/peds.2014-2742
6. Principi T, Coates AL, Parkin PC, Stephens D, DaSilva Z, Schuh S. Effect of oxygen desaturations on subsequent medical visits in infants discharged from the emergency department with bronchiolitis. JAMA Pediatr. 2016;170(6):602-608. https://doi.org/10.1001/jamapediatrics.2016.0114
7. Cunningham S, Rodriguez A, Adams T, et al. Oxygen saturation targets in infants with bronchiolitis (BIDS): a double-blind, randomised, equivalence trial. Lancet. 2015;386(9998):1041-1048. https://doi.org/10.1016/s0140-6736(15)00163-4
8. McCulloh R, Koster M, Ralston S, et al. Use of intermittent vs continuous pulse oximetry for nonhypoxemic infants and young children hospitalized for bronchiolitis: a randomized clinical trial. JAMA Pediatr. 2015;169(10):898-904. https://doi.org/10.1001/jamapediatrics.2015.1746
9. Schuh S, Freedman S, Coates A, et al. Effect of oximetry on hospitalization in bronchiolitis: a randomized clinical trial. JAMA. 2014;312(7):712-718. https://doi.org/10.1001/jama.2014.8637
10. Schroeder AR, Marmor AK, Pantell RH, Newman TB. Impact of pulse oximetry and oxygen therapy on length of stay in bronchiolitis hospitalizations. Arch Pediatr Adolesc Med. 2004;158(6):527-530. https://doi.org/10.1001/archpedi.158.6.527
11. Rasooly IR, Beidas RS, Wolk CB, et al. Measuring overuse of continuous pulse oximetry in bronchiolitis and developing strategies for large-scale deimplementation: study protocol for a feasibility trial. Pilot Feasibility Stud. 2019;5:68. https://doi.org/10.1186/s40814-019-0453-2
As part of improvement collaboratives that aimed to reduce overuse of continuous pulse oximetry in children hospitalized with bronchiolitis, researchers used the presence of an active order for it as a proxy for the actual use of such monitoring.1,2 With use of this proxy, investigators on a national study documented a high burden of continuous oximetry overuse (86.5% before quality improvement interventions and 45.5% after),1 but the validity of orders in representing actual monitoring practice is unknown. If the presence of an active pulse oximetry order accurately identifies infants on monitors, electronic health record data could inform epidemiologic estimates of monitoring overuse and measure the success of quality improvement and deimplementation interventions. Alternatively, if nurses commonly begin and/or discontinue pulse oximetry without updated orders, a pulse oximetry order would not be an accurate proxy, and additional data capture methods (eg, bedside observation or data capture from bedside monitors) would be needed.
Understanding the validity of orders for detection of actual use is critical because continuous pulse oximetry monitoring is considered an overused practice in pediatric acute viral bronchiolitis,3 and national guidelines recommend against its use in low-risk hospitalized children.4,5 Continuous monitoring may identify trivial, self-resolving oxygen desaturation and its use is not associated with improved outcomes.6-9 When self-resolving desaturations are treated with additional supplemental oxygen, hospital stays may be unnecessarily prolonged.10 In order to reduce unnecessary continuous pulse oximetry use, measurement of the extent of the overused practice is necessary. In this 56-hospital study,11 we aimed to determine the validity of using active continuous pulse oximetry orders instead of bedside observation of actual monitor use.
METHODS
Design
In this multicenter, repeated cross-sectional study, investigators used direct bedside observation to determine continuous pulse oximetry monitor use and then assessed whether an active continuous monitoring order was present in the electronic health record. The study took place during one bronchiolitis season, December 1, 2018, through March 31, 2019.
Setting and Patients
Investigators at 56 freestanding children’s hospitals, children’s hospitals within general hospitals, and community hospitals in the Pediatric Research in Inpatient Settings (PRIS) Network collected data on infants aged 8 weeks to 23 months who were hospitalized with bronchiolitis. As this work was a substudy of the larger Eliminating Monitor Overuse study, only infants not currently receiving supplemental oxygen were included.11 Investigators observed eligible infants outside of the intensive care unit on general hospital medicine units. We excluded infants born premature (documented prematurity of <28 weeks’ gestation or documented “premature” without a gestational age listed), as well as those with a home oxygen requirement, cyanotic congenital heart disease, pulmonary hypertension, tracheostomy, primary neuromuscular disease, immunodeficiency, or cancer.
Data Collection
Investigators used the electronic health record to identify eligible infants. Investigators entered patient rooms to confirm the infant was not on supplemental oxygen (hence confirming eligibility for the study) and determine if continuous pulse oximetry was actively in use by examining the monitor display for a pulse oximetry waveform. Investigators then confirmed if active orders for pulse oximetry were present in the patient’s chart. Per study design, site investigators aimed to observe approximately half of eligible infants during the day (10
Analysis
We excluded patients with conditional orders (eg, monitored only when certain conditions exist, such as when asleep) because of the time-varying and wide range of conditions that could be specified. Furthermore, conditional orders would not be useful as proxies to measure oximetry use because investigators would still need additional data (eg, bedside observation) to determine current monitoring status.
We calculated the sensitivity, specificity, positive predictive value (PPV), and negative predictive value (NPV) of active orders using the reference standard of direct bedside observation, as well as corresponding 95% CIs that accounted for within-hospital clustering. We calculated these test characteristics overall and as stratified across four age groups: 8 weeks to 5 months, 6 months to 11 months, 12 months to 17 months, and 18 months to 23 months. We also calculated the test characteristics for each hospital. We decided a priori that a PPV and NPV of 80% would represent a reasonable threshold to use active orders as a proxy in multicenter research. For hospital-level analyses we included only hospitals with 60 or more total observations and more than 15 observations with active orders for PPV and more than 15 observations without active orders for NPV. We used Stata (StataCorp LLC, College Station, Texas) version 15.1 for analysis.
For US sites, the Institutional Review Board (IRB) at Children’s Hospital of Philadelphia approved the study as the single reviewing IRB, and the remaining US sites established reliance agreements with the reviewing IRB. Research Ethics Boards at the Canadian sites (University of Calgary and The Hospital for Sick Children) also reviewed and approved the study. All sites granted waivers of consent, assent, parental permission, and HIPAA authorization.
RESULTS
Investigators completed 3,612 observations in 56 hospitals. This included 33 freestanding children’s hospitals, 14 hospitals within large general hospitals, and 9 community hospitals. Of 3,612 completed observations, on 631 occasions (17%) patients had conditional orders (eg, continuous monitoring only when sleeping) and were excluded from further analysis.
Most pulse oximetry–monitored infants did not have an active monitoring order (670 out of 1,309; sensitivity of 49%). Test characteristics, stratified by age group, are presented in the Table. Across all observations, the overall PPV was 77% (95% CI, 72-82), and the overall NPV was 69% (95% CI, 61-77). Variation of all test characteristics across age group was small (eg, the sensitivity ranged from 43% to 51%).
With inclusion of only those hospitals with sufficient observations, hospital-level variation in the PPV and NPV of using active orders was substantial (PPV range of 48% to 96% and NPV range of 30% to 98%). Only two hospitals had both a PPV and NPV for using monitor orders that exceeded the 80% threshold.
DISCUSSION
Active continuous pulse oximetry orders did not accurately represent actual monitoring status in this study. Monitoring orders alone frequently misrepresent true monitoring status and, as such, should be interpreted with caution in research or quality improvement activities. If more valid estimates of monitoring use and overuse are needed, potential measurement options include direct observation, as used in our study, as well as the use of more complex data streams such as the output of monitoring devices or pulse oximetry data in the electronic health record. In only two of the hospitals, using active continuous monitoring orders was a reasonable proxy for detecting actual monitor use. Monitoring orders could potentially be validly used for deimplementation efforts at those centers; other hospitals could consider targeted improvement efforts (eg, morning huddles examining the discordance between monitoring orders and monitoring status) to improve the accuracy of using continuous pulse oximetry orders.
We acknowledge several limitations of this study. Site investigators employed a convenience sampling approach, so it is possible that some investigators observed sicker or less sick infants. Although the PRIS network includes a geographically diverse group of North American hospitals, community hospitals were underrepresented in this study. Our results hence generalize more precisely to freestanding children’s hospitals than to community hospitals. We did not observe infants currently on supplemental oxygen, so we do not know to what degree using orders is valid in that context. We did not collect data on why actual monitoring status differed from monitoring orders and hence cannot quantify to what extent different factors (eg, nurse belief that monitors are a safety net or infants inadvertently left on monitors after a spot check pulse oximetry reading) contributed to this discordance. Finally, our study only examined one electronic health record variable—the presence of an active order. It may be that other variables in the health record (eg, minute-by-minute pulse oximetry values in a vital sign flowsheet) are much better proxies of actual continuous monitor use.
CONCLUSION
Using an active order for continuous pulse oximetry has poor sensitivity, PPV, and NPV for detecting true monitoring status at the bedside. Teams intending to measure the actual use of pulse oximetry should be aware of the limitations of using active orders alone as an accurate measure of pulse oximetry monitoring.
Acknowledgments
We thank the NHLBI scientists who contributed to this project as part of the U01 Cooperative Agreement funding mechanism: Lora Reineck, MD, MS, Karen Bienstock, MS, and Cheryl Boyce, PhD.
We thank the Executive Council of the PRIS Network for their contributions to the early scientific development of this project. We thank the PRIS site investigators for their major contributions to the Eliminating Monitor Overuse (EMO) Study data collection. Each listed collaborator is a group author for the PRIS Network in this manuscript. Their names can be found in the online supplemental information.
As part of improvement collaboratives that aimed to reduce overuse of continuous pulse oximetry in children hospitalized with bronchiolitis, researchers used the presence of an active order for it as a proxy for the actual use of such monitoring.1,2 With use of this proxy, investigators on a national study documented a high burden of continuous oximetry overuse (86.5% before quality improvement interventions and 45.5% after),1 but the validity of orders in representing actual monitoring practice is unknown. If the presence of an active pulse oximetry order accurately identifies infants on monitors, electronic health record data could inform epidemiologic estimates of monitoring overuse and measure the success of quality improvement and deimplementation interventions. Alternatively, if nurses commonly begin and/or discontinue pulse oximetry without updated orders, a pulse oximetry order would not be an accurate proxy, and additional data capture methods (eg, bedside observation or data capture from bedside monitors) would be needed.
Understanding the validity of orders for detection of actual use is critical because continuous pulse oximetry monitoring is considered an overused practice in pediatric acute viral bronchiolitis,3 and national guidelines recommend against its use in low-risk hospitalized children.4,5 Continuous monitoring may identify trivial, self-resolving oxygen desaturation and its use is not associated with improved outcomes.6-9 When self-resolving desaturations are treated with additional supplemental oxygen, hospital stays may be unnecessarily prolonged.10 In order to reduce unnecessary continuous pulse oximetry use, measurement of the extent of the overused practice is necessary. In this 56-hospital study,11 we aimed to determine the validity of using active continuous pulse oximetry orders instead of bedside observation of actual monitor use.
METHODS
Design
In this multicenter, repeated cross-sectional study, investigators used direct bedside observation to determine continuous pulse oximetry monitor use and then assessed whether an active continuous monitoring order was present in the electronic health record. The study took place during one bronchiolitis season, December 1, 2018, through March 31, 2019.
Setting and Patients
Investigators at 56 freestanding children’s hospitals, children’s hospitals within general hospitals, and community hospitals in the Pediatric Research in Inpatient Settings (PRIS) Network collected data on infants aged 8 weeks to 23 months who were hospitalized with bronchiolitis. As this work was a substudy of the larger Eliminating Monitor Overuse study, only infants not currently receiving supplemental oxygen were included.11 Investigators observed eligible infants outside of the intensive care unit on general hospital medicine units. We excluded infants born premature (documented prematurity of <28 weeks’ gestation or documented “premature” without a gestational age listed), as well as those with a home oxygen requirement, cyanotic congenital heart disease, pulmonary hypertension, tracheostomy, primary neuromuscular disease, immunodeficiency, or cancer.
Data Collection
Investigators used the electronic health record to identify eligible infants. Investigators entered patient rooms to confirm the infant was not on supplemental oxygen (hence confirming eligibility for the study) and determine if continuous pulse oximetry was actively in use by examining the monitor display for a pulse oximetry waveform. Investigators then confirmed if active orders for pulse oximetry were present in the patient’s chart. Per study design, site investigators aimed to observe approximately half of eligible infants during the day (10
Analysis
We excluded patients with conditional orders (eg, monitored only when certain conditions exist, such as when asleep) because of the time-varying and wide range of conditions that could be specified. Furthermore, conditional orders would not be useful as proxies to measure oximetry use because investigators would still need additional data (eg, bedside observation) to determine current monitoring status.
We calculated the sensitivity, specificity, positive predictive value (PPV), and negative predictive value (NPV) of active orders using the reference standard of direct bedside observation, as well as corresponding 95% CIs that accounted for within-hospital clustering. We calculated these test characteristics overall and as stratified across four age groups: 8 weeks to 5 months, 6 months to 11 months, 12 months to 17 months, and 18 months to 23 months. We also calculated the test characteristics for each hospital. We decided a priori that a PPV and NPV of 80% would represent a reasonable threshold to use active orders as a proxy in multicenter research. For hospital-level analyses we included only hospitals with 60 or more total observations and more than 15 observations with active orders for PPV and more than 15 observations without active orders for NPV. We used Stata (StataCorp LLC, College Station, Texas) version 15.1 for analysis.
For US sites, the Institutional Review Board (IRB) at Children’s Hospital of Philadelphia approved the study as the single reviewing IRB, and the remaining US sites established reliance agreements with the reviewing IRB. Research Ethics Boards at the Canadian sites (University of Calgary and The Hospital for Sick Children) also reviewed and approved the study. All sites granted waivers of consent, assent, parental permission, and HIPAA authorization.
RESULTS
Investigators completed 3,612 observations in 56 hospitals. This included 33 freestanding children’s hospitals, 14 hospitals within large general hospitals, and 9 community hospitals. Of 3,612 completed observations, on 631 occasions (17%) patients had conditional orders (eg, continuous monitoring only when sleeping) and were excluded from further analysis.
Most pulse oximetry–monitored infants did not have an active monitoring order (670 out of 1,309; sensitivity of 49%). Test characteristics, stratified by age group, are presented in the Table. Across all observations, the overall PPV was 77% (95% CI, 72-82), and the overall NPV was 69% (95% CI, 61-77). Variation of all test characteristics across age group was small (eg, the sensitivity ranged from 43% to 51%).
With inclusion of only those hospitals with sufficient observations, hospital-level variation in the PPV and NPV of using active orders was substantial (PPV range of 48% to 96% and NPV range of 30% to 98%). Only two hospitals had both a PPV and NPV for using monitor orders that exceeded the 80% threshold.
DISCUSSION
Active continuous pulse oximetry orders did not accurately represent actual monitoring status in this study. Monitoring orders alone frequently misrepresent true monitoring status and, as such, should be interpreted with caution in research or quality improvement activities. If more valid estimates of monitoring use and overuse are needed, potential measurement options include direct observation, as used in our study, as well as the use of more complex data streams such as the output of monitoring devices or pulse oximetry data in the electronic health record. In only two of the hospitals, using active continuous monitoring orders was a reasonable proxy for detecting actual monitor use. Monitoring orders could potentially be validly used for deimplementation efforts at those centers; other hospitals could consider targeted improvement efforts (eg, morning huddles examining the discordance between monitoring orders and monitoring status) to improve the accuracy of using continuous pulse oximetry orders.
We acknowledge several limitations of this study. Site investigators employed a convenience sampling approach, so it is possible that some investigators observed sicker or less sick infants. Although the PRIS network includes a geographically diverse group of North American hospitals, community hospitals were underrepresented in this study. Our results hence generalize more precisely to freestanding children’s hospitals than to community hospitals. We did not observe infants currently on supplemental oxygen, so we do not know to what degree using orders is valid in that context. We did not collect data on why actual monitoring status differed from monitoring orders and hence cannot quantify to what extent different factors (eg, nurse belief that monitors are a safety net or infants inadvertently left on monitors after a spot check pulse oximetry reading) contributed to this discordance. Finally, our study only examined one electronic health record variable—the presence of an active order. It may be that other variables in the health record (eg, minute-by-minute pulse oximetry values in a vital sign flowsheet) are much better proxies of actual continuous monitor use.
CONCLUSION
Using an active order for continuous pulse oximetry has poor sensitivity, PPV, and NPV for detecting true monitoring status at the bedside. Teams intending to measure the actual use of pulse oximetry should be aware of the limitations of using active orders alone as an accurate measure of pulse oximetry monitoring.
Acknowledgments
We thank the NHLBI scientists who contributed to this project as part of the U01 Cooperative Agreement funding mechanism: Lora Reineck, MD, MS, Karen Bienstock, MS, and Cheryl Boyce, PhD.
We thank the Executive Council of the PRIS Network for their contributions to the early scientific development of this project. We thank the PRIS site investigators for their major contributions to the Eliminating Monitor Overuse (EMO) Study data collection. Each listed collaborator is a group author for the PRIS Network in this manuscript. Their names can be found in the online supplemental information.
1. Ralston SL, Garber MD, Rice-Conboy E, et al. A multicenter collaborative to reduce unnecessary care in inpatient bronchiolitis. Pediatrics. 2016;137(1). https://doi.org/10.1542/peds.2015-0851
2. Mittal S, Marlowe L, Blakeslee S, et al. Successful use of quality improvement methodology to reduce inpatient length of stay in bronchiolitis through judicious use of intermittent pulse oximetry. Hosp Pediatr. 2019;9(2):73-78. https://doi.org/10.1542/hpeds.2018-0023
3. Quinonez RA, Coon ER, Schroeder AR, Moyer VA. When technology creates uncertainty: pulse oximetry and overdiagnosis of hypoxaemia in bronchiolitis. BMJ. 2017;358:j3850. https://doi.org/10.1136/bmj.j3850
4. Quinonez RA, Garber MD, Schroeder AR, et al. Choosing wisely in pediatric hospital medicine: five opportunities for improved healthcare value. J Hosp Med. 2013;8(9):479-485. https://doi.org/10.1002/jhm.2064
5. Ralston SL, Lieberthal AS, Meissner HC, et al. Clinical practice guideline: the diagnosis, management, and prevention of bronchiolitis. Pediatrics. 2014;134(5):e1474-e1502. https://doi.org/10.1542/peds.2014-2742
6. Principi T, Coates AL, Parkin PC, Stephens D, DaSilva Z, Schuh S. Effect of oxygen desaturations on subsequent medical visits in infants discharged from the emergency department with bronchiolitis. JAMA Pediatr. 2016;170(6):602-608. https://doi.org/10.1001/jamapediatrics.2016.0114
7. Cunningham S, Rodriguez A, Adams T, et al. Oxygen saturation targets in infants with bronchiolitis (BIDS): a double-blind, randomised, equivalence trial. Lancet. 2015;386(9998):1041-1048. https://doi.org/10.1016/s0140-6736(15)00163-4
8. McCulloh R, Koster M, Ralston S, et al. Use of intermittent vs continuous pulse oximetry for nonhypoxemic infants and young children hospitalized for bronchiolitis: a randomized clinical trial. JAMA Pediatr. 2015;169(10):898-904. https://doi.org/10.1001/jamapediatrics.2015.1746
9. Schuh S, Freedman S, Coates A, et al. Effect of oximetry on hospitalization in bronchiolitis: a randomized clinical trial. JAMA. 2014;312(7):712-718. https://doi.org/10.1001/jama.2014.8637
10. Schroeder AR, Marmor AK, Pantell RH, Newman TB. Impact of pulse oximetry and oxygen therapy on length of stay in bronchiolitis hospitalizations. Arch Pediatr Adolesc Med. 2004;158(6):527-530. https://doi.org/10.1001/archpedi.158.6.527
11. Rasooly IR, Beidas RS, Wolk CB, et al. Measuring overuse of continuous pulse oximetry in bronchiolitis and developing strategies for large-scale deimplementation: study protocol for a feasibility trial. Pilot Feasibility Stud. 2019;5:68. https://doi.org/10.1186/s40814-019-0453-2
1. Ralston SL, Garber MD, Rice-Conboy E, et al. A multicenter collaborative to reduce unnecessary care in inpatient bronchiolitis. Pediatrics. 2016;137(1). https://doi.org/10.1542/peds.2015-0851
2. Mittal S, Marlowe L, Blakeslee S, et al. Successful use of quality improvement methodology to reduce inpatient length of stay in bronchiolitis through judicious use of intermittent pulse oximetry. Hosp Pediatr. 2019;9(2):73-78. https://doi.org/10.1542/hpeds.2018-0023
3. Quinonez RA, Coon ER, Schroeder AR, Moyer VA. When technology creates uncertainty: pulse oximetry and overdiagnosis of hypoxaemia in bronchiolitis. BMJ. 2017;358:j3850. https://doi.org/10.1136/bmj.j3850
4. Quinonez RA, Garber MD, Schroeder AR, et al. Choosing wisely in pediatric hospital medicine: five opportunities for improved healthcare value. J Hosp Med. 2013;8(9):479-485. https://doi.org/10.1002/jhm.2064
5. Ralston SL, Lieberthal AS, Meissner HC, et al. Clinical practice guideline: the diagnosis, management, and prevention of bronchiolitis. Pediatrics. 2014;134(5):e1474-e1502. https://doi.org/10.1542/peds.2014-2742
6. Principi T, Coates AL, Parkin PC, Stephens D, DaSilva Z, Schuh S. Effect of oxygen desaturations on subsequent medical visits in infants discharged from the emergency department with bronchiolitis. JAMA Pediatr. 2016;170(6):602-608. https://doi.org/10.1001/jamapediatrics.2016.0114
7. Cunningham S, Rodriguez A, Adams T, et al. Oxygen saturation targets in infants with bronchiolitis (BIDS): a double-blind, randomised, equivalence trial. Lancet. 2015;386(9998):1041-1048. https://doi.org/10.1016/s0140-6736(15)00163-4
8. McCulloh R, Koster M, Ralston S, et al. Use of intermittent vs continuous pulse oximetry for nonhypoxemic infants and young children hospitalized for bronchiolitis: a randomized clinical trial. JAMA Pediatr. 2015;169(10):898-904. https://doi.org/10.1001/jamapediatrics.2015.1746
9. Schuh S, Freedman S, Coates A, et al. Effect of oximetry on hospitalization in bronchiolitis: a randomized clinical trial. JAMA. 2014;312(7):712-718. https://doi.org/10.1001/jama.2014.8637
10. Schroeder AR, Marmor AK, Pantell RH, Newman TB. Impact of pulse oximetry and oxygen therapy on length of stay in bronchiolitis hospitalizations. Arch Pediatr Adolesc Med. 2004;158(6):527-530. https://doi.org/10.1001/archpedi.158.6.527
11. Rasooly IR, Beidas RS, Wolk CB, et al. Measuring overuse of continuous pulse oximetry in bronchiolitis and developing strategies for large-scale deimplementation: study protocol for a feasibility trial. Pilot Feasibility Stud. 2019;5:68. https://doi.org/10.1186/s40814-019-0453-2
© 2020 Society of Hospital Medicine
Counting the Ways to Count Medications: The Challenges of Defining Pediatric Polypharmacy
Polypharmacy, the practice of taking multiple medications to manage health conditions, is common for children. Many children today have a higher burden chronic illness and an increasing number of pharmaceuticals—often delivered in various doses throughout the day. Polypharmacy has been linked to a variety of pediatric and adult outcomes, including medication errors and readmission.1-3 Consequently, the Society of Hospital Medicine recognizes polypharmacy as a risk factor for readmission for adult populations.4 These adverse outcomes are related to both the human elements of polypharmacy (eg, cognitive burden, adherence) and the pharmacologic elements, including drug–drug interactions. For many children, the safety implications of polypharmacy may be more consequential due to the reliance of multiple caregivers to administer medications, which requires additional coordination to ensure that medications are administered and not duplicated. Dual administration of the same medication by both parents is the most common reason for pediatric calls to Poison Control Centers.5 Yet, there is a paucity of research in this area, with most of the pediatric literature focusing on the outpatient setting and specific populations, including epilepsy and mental health.6-8
How providers, patients, and families translate medication lists to counts of medications—and hence the burden of polypharmacy—is not clearly or consistently described. Often in studies of polypharmacy, researchers utilize medication claims data to count the number of medications a patient has filled from the pharmacy. However, in routine clinical practice, clinicians rarely have access to medication claims and thus rely on patient or family report, which may or may not match the list of medications in the patients’ medical records.
Therefore, linking polypharmacy research to the pragmatic complexities of clinical care requires greater clarity and consistent application of concepts. At hospital discharge, families receive a list of medications to take, including home medications to resume as well as newly prescribed medications. However, not all medications are equally essential to patients’ care regarding importance of administration (eg, hydrocortisone ointment versus an anticonvulsant medication). Patients, parents, and caregivers are ultimately responsible for determining which medications to prioritize and administer.
Although there is no standard numerical definition for how to identify polypharmacy, five medications is commonly considered the threshold for polypharmacy.9 A recent review of the pediatric polypharmacy literature suggested a lower threshold, with any two concurrent medications for at least a day.7 Yet, the best approach to “count” medications at hospital discharge is unclear. The simplest method is to tally the number of medications listed in the discharge summary. However, medications are sometimes listed twice due to different dosages administered at different times. Frequently, medications are prescribed on an as-needed basis; these medications could be administered routinely or very infrequently (eg, epinephrine for anaphylaxis). Over-the-counter medications are also sometimes included in discharge summaries and consideration should be given as to whether these medications count toward measures of polypharmacy. Over-the-counter medications would not be counted by a polypharmacy measure that relies on claims data if those medications are not paid by the insurer.
We sought consensus on how to count discharge medications through a series of informal interviews with hospitalists, nurses, and parents. We asked the seemingly simple question, “How many medications is this child on?” across a variety of scenarios (Figure). For panel A, all stakeholders agreed that this medication list includes two medications. All other scenarios elicited disagreement. For panel B, many people responded three medications, but others (often physicians) counted only clindamycin and therefore responded one medication.
For panel C, stakeholders were split between one (only topiramate), two (topiramate and rectal diazepam), and three medications (two different doses of topiramate, which counted as two different medications, plus rectal diazepam). Interestingly, one parent reflected that they would count panel C differently, depending on with whom they were discussing the medications. If the parent were speaking with a physician, they would consider the two different doses of topiramate as a single medication; however, if they were conveying a list of medications to a babysitter, they would consider them as two different medications. Finally, panel D also split stakeholders between counting one and two medications, with some parents expressing confusion as to why the child would be prescribed the same medication at different times.
While our informal conversations with physicians, nurses, and families should not be construed as rigorous qualitative research, we are concerned about the lack of a shared mental model about the best way to count discharge polypharmacy. In reviewing the comments that we collected, the family voice stands out—physicians do not know how a parent or a caregiver will prioritize the medications to give to their child; physicians do not know whether families will count medications as a group or as separate entities. Although providers, patients, and families share a list of medications at discharge, this list may contain items not considered as “medications” by physicians.10 Nevertheless, the medication list provided at discharge is what the family must navigate once home. One way to consider discharge polypharmacy would be to count all the medications in the discharge summary, regardless of clinicians’ perceptions of necessity or importance. Electronic health record based tools should sum medications counts. Ultimately, further research is needed to understand the cognitive and care burden discharge polypharmacy places on families as well as understand this burden’s relationship to safety and transition outcomes.
Disclosures
Dr. Auger has nothing to disclose. Dr. Shah is the Editor-in-Chief of the Journal of Hospital Medicine. Dr. Davis has nothing to disclose. Dr. Brady reports grants from Agency for Healthcare Research and Quality, outside the submitted work.
Funding
This project is supported by a grant from the Agency for Healthcare Research and Quality (1K08HS204735-01A1).
1. Winer JC, Aragona E, Fields AI, Stockwell DC. Comparison of clinical risk factors among pediatric patients with single admission, multiple admissions (without any 7-day readmissions), and 7-day readmission. Hosp Pediatr. 2016;6(3):119-125. https://doi.org/10.1542/hpeds.2015-0110.
2. Feinstein J, Dai D, Zhong W, Freedman J, Feudtner C. Potential drug-drug interactions in infant, child, and adolescent patients in children’s hospitals. Pediatrics. 2015;135(1):e99-e108. https://doi.org/10.1542/peds.2014-2015.
3. Patterson SM, Cadogan CA, Kerse N, et al. Interventions to improve the appropriate use of polypharmacy for older people. Cochrane Database Syst Rev. 2014(10):CD008165. https://doi.org/10.1002/14651858.CD008165.pub3.
4. Society of Hospital Medicine. Project BOOST: better outcomes for older adults through safe transitions—implementation guide to improve care transitions.
5. Smith MD, Spiller HA, Casavant MJ, Chounthirath T, Brophy TJ, Xiang H. Out-of-hospital medication errors among young children in the United States, 2002-2012. Pediatrics. 2014;134(5):867-876. https://doi.org/10.1542/peds.2014-0309.
6. Baker C, Feinstein JA, Ma X, et al. Variation of the prevalence of pediatric polypharmacy: a scoping review. Pharmacoepidemiol Drug Saf. 2019;28(3):275-287. https://doi.org/10.1002/pds.4719.
7. Bakaki PM, Horace A, Dawson N, et al. Defining pediatric polypharmacy: a scoping review. PLoS One. 2018;13(11):e0208047. https://doi.org/10.1371/journal.pone.0208047.
8. Horace AE, Ahmed F. Polypharmacy in pediatric patients and opportunities for pharmacists’ involvement. Integr Pharm Res Pract. 2015;4:113-126. https://doi.org/10.2147/IPRP.S64535.
9. Masnoon N, Shakib S, Kalisch-Ellett L, Caughey GE. What is polypharmacy? A systematic review of definitions. BMC Geriatr. 2017;17(1):230. https://doi.org/10.1186/s12877-017-0621-2.
10. Auger KA, Shah SS, Huang B, et al. Discharge Medical Complexity, Change in Medical Complexity and Pediatric Thirty-day Readmission. J Hosp Med. 2019;14(8):474-481. https://doi.org/10.12788/jhm.3222.
11. Martin P, Tamblyn R, Benedetti A, Ahmed S, Tannenbaum C. Effect of a pharmacist-led educational intervention on inappropriate medication prescriptions in older adults: the D-PRESCRIBE randomized clinical trial. Jama. 2018;320(18):1889-1898. https://doi.org/10.1001/jama.2018.16131.
12. Page AT, Clifford RM, Potter K, Schwartz D, Etherton-Beer CD. The feasibility and effect of deprescribing in older adults on mortality and health: a systematic review and meta-analysis. Br J Clin Pharmacol. 2016;82(3):583-623. https://doi.org/10.1111/bcp.12975.
Polypharmacy, the practice of taking multiple medications to manage health conditions, is common for children. Many children today have a higher burden chronic illness and an increasing number of pharmaceuticals—often delivered in various doses throughout the day. Polypharmacy has been linked to a variety of pediatric and adult outcomes, including medication errors and readmission.1-3 Consequently, the Society of Hospital Medicine recognizes polypharmacy as a risk factor for readmission for adult populations.4 These adverse outcomes are related to both the human elements of polypharmacy (eg, cognitive burden, adherence) and the pharmacologic elements, including drug–drug interactions. For many children, the safety implications of polypharmacy may be more consequential due to the reliance of multiple caregivers to administer medications, which requires additional coordination to ensure that medications are administered and not duplicated. Dual administration of the same medication by both parents is the most common reason for pediatric calls to Poison Control Centers.5 Yet, there is a paucity of research in this area, with most of the pediatric literature focusing on the outpatient setting and specific populations, including epilepsy and mental health.6-8
How providers, patients, and families translate medication lists to counts of medications—and hence the burden of polypharmacy—is not clearly or consistently described. Often in studies of polypharmacy, researchers utilize medication claims data to count the number of medications a patient has filled from the pharmacy. However, in routine clinical practice, clinicians rarely have access to medication claims and thus rely on patient or family report, which may or may not match the list of medications in the patients’ medical records.
Therefore, linking polypharmacy research to the pragmatic complexities of clinical care requires greater clarity and consistent application of concepts. At hospital discharge, families receive a list of medications to take, including home medications to resume as well as newly prescribed medications. However, not all medications are equally essential to patients’ care regarding importance of administration (eg, hydrocortisone ointment versus an anticonvulsant medication). Patients, parents, and caregivers are ultimately responsible for determining which medications to prioritize and administer.
Although there is no standard numerical definition for how to identify polypharmacy, five medications is commonly considered the threshold for polypharmacy.9 A recent review of the pediatric polypharmacy literature suggested a lower threshold, with any two concurrent medications for at least a day.7 Yet, the best approach to “count” medications at hospital discharge is unclear. The simplest method is to tally the number of medications listed in the discharge summary. However, medications are sometimes listed twice due to different dosages administered at different times. Frequently, medications are prescribed on an as-needed basis; these medications could be administered routinely or very infrequently (eg, epinephrine for anaphylaxis). Over-the-counter medications are also sometimes included in discharge summaries and consideration should be given as to whether these medications count toward measures of polypharmacy. Over-the-counter medications would not be counted by a polypharmacy measure that relies on claims data if those medications are not paid by the insurer.
We sought consensus on how to count discharge medications through a series of informal interviews with hospitalists, nurses, and parents. We asked the seemingly simple question, “How many medications is this child on?” across a variety of scenarios (Figure). For panel A, all stakeholders agreed that this medication list includes two medications. All other scenarios elicited disagreement. For panel B, many people responded three medications, but others (often physicians) counted only clindamycin and therefore responded one medication.
For panel C, stakeholders were split between one (only topiramate), two (topiramate and rectal diazepam), and three medications (two different doses of topiramate, which counted as two different medications, plus rectal diazepam). Interestingly, one parent reflected that they would count panel C differently, depending on with whom they were discussing the medications. If the parent were speaking with a physician, they would consider the two different doses of topiramate as a single medication; however, if they were conveying a list of medications to a babysitter, they would consider them as two different medications. Finally, panel D also split stakeholders between counting one and two medications, with some parents expressing confusion as to why the child would be prescribed the same medication at different times.
While our informal conversations with physicians, nurses, and families should not be construed as rigorous qualitative research, we are concerned about the lack of a shared mental model about the best way to count discharge polypharmacy. In reviewing the comments that we collected, the family voice stands out—physicians do not know how a parent or a caregiver will prioritize the medications to give to their child; physicians do not know whether families will count medications as a group or as separate entities. Although providers, patients, and families share a list of medications at discharge, this list may contain items not considered as “medications” by physicians.10 Nevertheless, the medication list provided at discharge is what the family must navigate once home. One way to consider discharge polypharmacy would be to count all the medications in the discharge summary, regardless of clinicians’ perceptions of necessity or importance. Electronic health record based tools should sum medications counts. Ultimately, further research is needed to understand the cognitive and care burden discharge polypharmacy places on families as well as understand this burden’s relationship to safety and transition outcomes.
Disclosures
Dr. Auger has nothing to disclose. Dr. Shah is the Editor-in-Chief of the Journal of Hospital Medicine. Dr. Davis has nothing to disclose. Dr. Brady reports grants from Agency for Healthcare Research and Quality, outside the submitted work.
Funding
This project is supported by a grant from the Agency for Healthcare Research and Quality (1K08HS204735-01A1).
Polypharmacy, the practice of taking multiple medications to manage health conditions, is common for children. Many children today have a higher burden chronic illness and an increasing number of pharmaceuticals—often delivered in various doses throughout the day. Polypharmacy has been linked to a variety of pediatric and adult outcomes, including medication errors and readmission.1-3 Consequently, the Society of Hospital Medicine recognizes polypharmacy as a risk factor for readmission for adult populations.4 These adverse outcomes are related to both the human elements of polypharmacy (eg, cognitive burden, adherence) and the pharmacologic elements, including drug–drug interactions. For many children, the safety implications of polypharmacy may be more consequential due to the reliance of multiple caregivers to administer medications, which requires additional coordination to ensure that medications are administered and not duplicated. Dual administration of the same medication by both parents is the most common reason for pediatric calls to Poison Control Centers.5 Yet, there is a paucity of research in this area, with most of the pediatric literature focusing on the outpatient setting and specific populations, including epilepsy and mental health.6-8
How providers, patients, and families translate medication lists to counts of medications—and hence the burden of polypharmacy—is not clearly or consistently described. Often in studies of polypharmacy, researchers utilize medication claims data to count the number of medications a patient has filled from the pharmacy. However, in routine clinical practice, clinicians rarely have access to medication claims and thus rely on patient or family report, which may or may not match the list of medications in the patients’ medical records.
Therefore, linking polypharmacy research to the pragmatic complexities of clinical care requires greater clarity and consistent application of concepts. At hospital discharge, families receive a list of medications to take, including home medications to resume as well as newly prescribed medications. However, not all medications are equally essential to patients’ care regarding importance of administration (eg, hydrocortisone ointment versus an anticonvulsant medication). Patients, parents, and caregivers are ultimately responsible for determining which medications to prioritize and administer.
Although there is no standard numerical definition for how to identify polypharmacy, five medications is commonly considered the threshold for polypharmacy.9 A recent review of the pediatric polypharmacy literature suggested a lower threshold, with any two concurrent medications for at least a day.7 Yet, the best approach to “count” medications at hospital discharge is unclear. The simplest method is to tally the number of medications listed in the discharge summary. However, medications are sometimes listed twice due to different dosages administered at different times. Frequently, medications are prescribed on an as-needed basis; these medications could be administered routinely or very infrequently (eg, epinephrine for anaphylaxis). Over-the-counter medications are also sometimes included in discharge summaries and consideration should be given as to whether these medications count toward measures of polypharmacy. Over-the-counter medications would not be counted by a polypharmacy measure that relies on claims data if those medications are not paid by the insurer.
We sought consensus on how to count discharge medications through a series of informal interviews with hospitalists, nurses, and parents. We asked the seemingly simple question, “How many medications is this child on?” across a variety of scenarios (Figure). For panel A, all stakeholders agreed that this medication list includes two medications. All other scenarios elicited disagreement. For panel B, many people responded three medications, but others (often physicians) counted only clindamycin and therefore responded one medication.
For panel C, stakeholders were split between one (only topiramate), two (topiramate and rectal diazepam), and three medications (two different doses of topiramate, which counted as two different medications, plus rectal diazepam). Interestingly, one parent reflected that they would count panel C differently, depending on with whom they were discussing the medications. If the parent were speaking with a physician, they would consider the two different doses of topiramate as a single medication; however, if they were conveying a list of medications to a babysitter, they would consider them as two different medications. Finally, panel D also split stakeholders between counting one and two medications, with some parents expressing confusion as to why the child would be prescribed the same medication at different times.
While our informal conversations with physicians, nurses, and families should not be construed as rigorous qualitative research, we are concerned about the lack of a shared mental model about the best way to count discharge polypharmacy. In reviewing the comments that we collected, the family voice stands out—physicians do not know how a parent or a caregiver will prioritize the medications to give to their child; physicians do not know whether families will count medications as a group or as separate entities. Although providers, patients, and families share a list of medications at discharge, this list may contain items not considered as “medications” by physicians.10 Nevertheless, the medication list provided at discharge is what the family must navigate once home. One way to consider discharge polypharmacy would be to count all the medications in the discharge summary, regardless of clinicians’ perceptions of necessity or importance. Electronic health record based tools should sum medications counts. Ultimately, further research is needed to understand the cognitive and care burden discharge polypharmacy places on families as well as understand this burden’s relationship to safety and transition outcomes.
Disclosures
Dr. Auger has nothing to disclose. Dr. Shah is the Editor-in-Chief of the Journal of Hospital Medicine. Dr. Davis has nothing to disclose. Dr. Brady reports grants from Agency for Healthcare Research and Quality, outside the submitted work.
Funding
This project is supported by a grant from the Agency for Healthcare Research and Quality (1K08HS204735-01A1).
1. Winer JC, Aragona E, Fields AI, Stockwell DC. Comparison of clinical risk factors among pediatric patients with single admission, multiple admissions (without any 7-day readmissions), and 7-day readmission. Hosp Pediatr. 2016;6(3):119-125. https://doi.org/10.1542/hpeds.2015-0110.
2. Feinstein J, Dai D, Zhong W, Freedman J, Feudtner C. Potential drug-drug interactions in infant, child, and adolescent patients in children’s hospitals. Pediatrics. 2015;135(1):e99-e108. https://doi.org/10.1542/peds.2014-2015.
3. Patterson SM, Cadogan CA, Kerse N, et al. Interventions to improve the appropriate use of polypharmacy for older people. Cochrane Database Syst Rev. 2014(10):CD008165. https://doi.org/10.1002/14651858.CD008165.pub3.
4. Society of Hospital Medicine. Project BOOST: better outcomes for older adults through safe transitions—implementation guide to improve care transitions.
5. Smith MD, Spiller HA, Casavant MJ, Chounthirath T, Brophy TJ, Xiang H. Out-of-hospital medication errors among young children in the United States, 2002-2012. Pediatrics. 2014;134(5):867-876. https://doi.org/10.1542/peds.2014-0309.
6. Baker C, Feinstein JA, Ma X, et al. Variation of the prevalence of pediatric polypharmacy: a scoping review. Pharmacoepidemiol Drug Saf. 2019;28(3):275-287. https://doi.org/10.1002/pds.4719.
7. Bakaki PM, Horace A, Dawson N, et al. Defining pediatric polypharmacy: a scoping review. PLoS One. 2018;13(11):e0208047. https://doi.org/10.1371/journal.pone.0208047.
8. Horace AE, Ahmed F. Polypharmacy in pediatric patients and opportunities for pharmacists’ involvement. Integr Pharm Res Pract. 2015;4:113-126. https://doi.org/10.2147/IPRP.S64535.
9. Masnoon N, Shakib S, Kalisch-Ellett L, Caughey GE. What is polypharmacy? A systematic review of definitions. BMC Geriatr. 2017;17(1):230. https://doi.org/10.1186/s12877-017-0621-2.
10. Auger KA, Shah SS, Huang B, et al. Discharge Medical Complexity, Change in Medical Complexity and Pediatric Thirty-day Readmission. J Hosp Med. 2019;14(8):474-481. https://doi.org/10.12788/jhm.3222.
11. Martin P, Tamblyn R, Benedetti A, Ahmed S, Tannenbaum C. Effect of a pharmacist-led educational intervention on inappropriate medication prescriptions in older adults: the D-PRESCRIBE randomized clinical trial. Jama. 2018;320(18):1889-1898. https://doi.org/10.1001/jama.2018.16131.
12. Page AT, Clifford RM, Potter K, Schwartz D, Etherton-Beer CD. The feasibility and effect of deprescribing in older adults on mortality and health: a systematic review and meta-analysis. Br J Clin Pharmacol. 2016;82(3):583-623. https://doi.org/10.1111/bcp.12975.
1. Winer JC, Aragona E, Fields AI, Stockwell DC. Comparison of clinical risk factors among pediatric patients with single admission, multiple admissions (without any 7-day readmissions), and 7-day readmission. Hosp Pediatr. 2016;6(3):119-125. https://doi.org/10.1542/hpeds.2015-0110.
2. Feinstein J, Dai D, Zhong W, Freedman J, Feudtner C. Potential drug-drug interactions in infant, child, and adolescent patients in children’s hospitals. Pediatrics. 2015;135(1):e99-e108. https://doi.org/10.1542/peds.2014-2015.
3. Patterson SM, Cadogan CA, Kerse N, et al. Interventions to improve the appropriate use of polypharmacy for older people. Cochrane Database Syst Rev. 2014(10):CD008165. https://doi.org/10.1002/14651858.CD008165.pub3.
4. Society of Hospital Medicine. Project BOOST: better outcomes for older adults through safe transitions—implementation guide to improve care transitions.
5. Smith MD, Spiller HA, Casavant MJ, Chounthirath T, Brophy TJ, Xiang H. Out-of-hospital medication errors among young children in the United States, 2002-2012. Pediatrics. 2014;134(5):867-876. https://doi.org/10.1542/peds.2014-0309.
6. Baker C, Feinstein JA, Ma X, et al. Variation of the prevalence of pediatric polypharmacy: a scoping review. Pharmacoepidemiol Drug Saf. 2019;28(3):275-287. https://doi.org/10.1002/pds.4719.
7. Bakaki PM, Horace A, Dawson N, et al. Defining pediatric polypharmacy: a scoping review. PLoS One. 2018;13(11):e0208047. https://doi.org/10.1371/journal.pone.0208047.
8. Horace AE, Ahmed F. Polypharmacy in pediatric patients and opportunities for pharmacists’ involvement. Integr Pharm Res Pract. 2015;4:113-126. https://doi.org/10.2147/IPRP.S64535.
9. Masnoon N, Shakib S, Kalisch-Ellett L, Caughey GE. What is polypharmacy? A systematic review of definitions. BMC Geriatr. 2017;17(1):230. https://doi.org/10.1186/s12877-017-0621-2.
10. Auger KA, Shah SS, Huang B, et al. Discharge Medical Complexity, Change in Medical Complexity and Pediatric Thirty-day Readmission. J Hosp Med. 2019;14(8):474-481. https://doi.org/10.12788/jhm.3222.
11. Martin P, Tamblyn R, Benedetti A, Ahmed S, Tannenbaum C. Effect of a pharmacist-led educational intervention on inappropriate medication prescriptions in older adults: the D-PRESCRIBE randomized clinical trial. Jama. 2018;320(18):1889-1898. https://doi.org/10.1001/jama.2018.16131.
12. Page AT, Clifford RM, Potter K, Schwartz D, Etherton-Beer CD. The feasibility and effect of deprescribing in older adults on mortality and health: a systematic review and meta-analysis. Br J Clin Pharmacol. 2016;82(3):583-623. https://doi.org/10.1111/bcp.12975.
© 2019 Society of Hospital Medicine
Discharge Medical Complexity, Change in Medical Complexity and Pediatric 30-day Readmission
Hospitalizations are disruptive, stressful, and costly for patients and families.1-5 Hospital readmissions subject families to the additional morbidity inherent to hospitalization and place patients at additional risk of hospital-acquired conditions or other harm.6-9 In pediatrics, hospital readmissions are common for specific conditions;10 with rates varying across institutions;10,11 and as many as one-third of unplanned pediatric readmissions are potentially preventable.12
Reducing pediatric readmissions requires a deeper understanding of the mechanisms through which readmissions occur. Medical complexity—specifically chronic conditions and use of medical technology—is associated with increased risk of readmission.13,14 Polypharmacy at discharge has also been associated with readmission.15,16 However, prior studies on polypharmacy and readmission risk examined the count of total medications and did not consider the nuances of scheduled versus as-needed medications, or the frequency of doses. These nuances may be critical to caregivers as discharge medical complexity can be overwhelming, even in diagnoses which are not traditionally considered complex.17 Finally, of potentially greater importance than medical complexity at discharge is a change in medical complexity during a hospitalization—for example, new diagnoses or new technologies that require additional education in hospital and management at home.
We sought to further understand the relationship between discharge medical complexity and readmission risk with regards to polypharmacy and home healthcare referrals at discharge. Specifically, we hypothesized that a change in medical complexity during an admission—ie, a new chronic diagnosis or new technology—would be a more prominent risk factor for readmission than discharge complexity alone. We examined these factors in the context of length of stay (LOS) since this is a marker of in-hospital severity of illness and a potentially modifiable function of time allowed for in-hospital teaching and discharge preparation.
METHODS
We conducted a retrospective, case-control study of pediatric hospitalizations at one tertiary care children’s hospital. Children <18 years were eligible for inclusion. Normal birth hospitalizations were excluded. We randomly selected one hospitalization from each child as the index visit. We identified cases, hospitalizations at C.S. Mott Children’s Hospital between 2008 and 2012 with a subsequent unplanned 30-day readmission,18 and matched them one to one with hospitalizations at the same hospital during the same period without subsequent readmission. We matched cases to controls based on the month of admission to account for seasonality of certain illnesses. We also matched on distance and direction from the hospital to the patient’s home to account for the potential to have readmissions to other institutions. We utilized both distance and direction recognizing that a family living 30 miles in one direction would be closer to an urban area with access to more facilities, as opposed to 30 miles in another direction in a rural area without additional access. We subsequently performed medical record review to abstract relevant covariates.
Primary Predictors
Medical Complexity Models (Models 1 and 2):
We evaluated three attributes of discharge medical complexity abstracted by medical record review—discharge medications, technology assistance (ie, tracheostomy, cerebral spinal fluid ventricular shunt, enteral feeding tube, central line), and the need for home healthcare after discharge. We counted discharge medications based on the number of medications listed on the discharge summary separated into scheduled or as needed.19 We also considered the number of scheduled doses to be administered in a 24-hour period (see Appendix methods for more information on counting discharge medications). For assistance by technology, we considered the presence of tracheostomy, cerebral spinal fluid ventricular shunt, enteral feeding tube, and central lines. While we describe these technologies separately, for multivariable analyses we considered the presence of any of the four types of technology.
Change in Medical Complexity Models (Models 3 and 4)
We examined two aspects of change in medical complexity—the presence of a new complex chronic condition (CCC)20 diagnosed during the hospitalization, and a new reliance on medical technology. The presence of new CCC was determined by comparing discharge diagnoses to past medical history abstracted by medical record review. A new CCC was defined as any complex chronic condition that was captured in the discharge diagnoses but was not evident in the past medical history. By definition, all CCCs coded during birth hospitalization (eg, at discharge from the neonatal intensive care unit) were assigned to “new” CCC. We calculated a kappa statistic to determine interrater reliability in determining the designation of new CCC. A sensitivity analysis examining these birth CCCs was also performed comparing no new CCC, new CCC, and new CCC after birth hospitalization. The methods appendix provides additional information on considering new CCCs. New technology, abstracted from chart review, was defined as technology placed during hospitalization that remained in place at discharge. If a child with existing technology had additional technology placed during the hospitalization (eg, a new tracheostomy in a child with a previously placed enteral feeding tube), the encounter was considered as having new technology placed.
Covariates
We created different sets of multivariable models to account for patient/hospitalization characteristics.
Statistical Analysis
A review of 600 cases and 600 controls yields 89% power to detect statistical significance for covariates with an odds ratio of 1.25 (β = 0.22) if the candidate covariate has low to moderate correlation with other covariates (<0.3). If a candidate covariate has a moderate correlation with other covariates (0.6), we have 89% power to detect an odds ratio of 1.35 (β = 0.30).21 We calculated odds of 30-days unplanned readmission using conditional logistic regression to account for matched case-control design. All the analyses were performed using STATA 13 (Stata Corp., College Station, Texas).
RESULTS
Of the 41,422 eligible index hospitalizations during the study period, 9.4% resulted in a 30-day unplanned readmission. After randomly selecting one hospitalization per child, there were 781 eligible cases. We subsequent matched all but one eligible case to a control. We randomly selected encounters for medical record review, reviewing a total of 1,212 encounters. After excluding pairs with incomplete records, we included 595 cases and 595 controls in this analysis (Figure). Patient/hospitalization characteristics are displayed in Table 1. The most frequent primary discharge diagnoses are displayed in Appendix Table 1.
Models of Medical Complexity at Discharge
Polypharmacy after discharge was common for both readmitted and nonreadmitted patients. Children who experienced unplanned readmission in 30 days were discharged with a median of four different scheduled medications (interquartile range [IQR] 2,7) which translated into a median of six (IQR 3,12) scheduled doses in a 24-hour period. In comparison, children without an unplanned readmission had a median of two different scheduled medications (IQR 1,3) with a median of three (IQR 0,7) scheduled doses in a 24-hour period. Medical technology was more common in case children (42%) than in control children (14%). Central lines and enteral tubes were the most common forms of medical technology in both cases and controls. Home health referral was common in both cases (44%) and controls (23%; Table 1).
In Model 1 (adjusting only for patient characteristics; Table 3), being discharged on two or more scheduled medications was associated with higher odds of readmission compared to being discharged without medications, with additional medications associated with even higher odds of readmission. Children with any technology had higher odds of readmission than children without medical technology. Likewise, home healthcare visits after discharge were associated with elevated odds of readmission in multivariable analyses without LOS. However, after adding LOS to the model (Model 2), home healthcare visits were no longer significantly associated with readmission.
Change in Medical Complexity Models
The adjudication of new CCCs had good reliability (Κ = 0.72). New CCCs occurred in 18% and new technologies occurred in 17% of cases. Comparatively, new CCCs occurred in 10% and new technologies in 7% of hospitalizations in control children (Table 1). In bivariate analyses, both aspects of change in medical complexity were associated with higher odds of readmission (Table 2). In multivariate analysis with patient characteristics (Model 3; Table 3), all aspects of change in complexity were associated with elevated odds of readmission. A new CCC was associated with higher odds of readmission (adjusted OR (AOR) 1.75, 95% CI: 1.11-2.75) as was new technology during admission (AOR 1.84, 95%CI: 1.09-3.10). Furthermore, the odds of readmission for medical complexity variables (polypharmacy and home healthcare need) remained largely unchanged when adding the change in medical complexity variables (ie, comparing Model 1 and Model 3). However, when accounting for LOS (Model 4), neither the acquisition of a new CCC nor the addition of new technology was associated with readmission. The most common form of new technology was central line followed by nonsurgically placed enteral tube (Appendix Table 2). Finally, in sensitivity analyses (results not detailed), separating new CCC acquired at birth and new CCCs in nonbirth hospitalizations, compared to hospitalizations with no new CCC, yielded similar results as the primary analyses.
DISCUSSION
We examined multiple attributes of polypharmacy—the number of scheduled medications, number of as-needed medications, and number of scheduled doses per 24 hours. Interestingly, only the scheduled medications (count of medication and number of doses) were associated with elevated readmission risk. As-needed medications have heterogeneity in the level of importance from critical (eg, seizure rescue) to discretionary (eg, antipyretics, creams). The burden of managing these types of medications may still be high (ie, parents must decide when to administer a critical medication); however, this burden does not translate into increased readmission risk in this population.
Not surprisingly, greater medical complexity—as defined by higher numbers of scheduled discharge medications and technology assistance—is associated with 30-day readmission risk. Our analyses do not allow us to determine how much of the increased risk is due to additional care burden and risks of polypharmacy versus the inherent increase in complexity and severity of illness for which polypharmacy is a marker. Tailoring discharge regimens to the realities of daily life, with the goal of “minimally disruptive medicine”22,23 (eg, integrating manageable discharge medication routines into school and work schedules), is not a common feature of pediatric discharge planning. For adult patients with complex medical conditions, tailoring medication regimens in a minimally disruptive way is known to improve outcomes.24 Similarly, adopting minimally disruptive techniques to integrate the polypharmacy inherent in discharge could potentially mitigate some of the readmission risks for children and adolescents.
Contrary to our hypothesis, new technologies and new diagnoses did not confer additional readmission risk when accounting for LOS and patient characteristics. One potential explanation is varying risks conveyed by different types of new technologies placed during hospitalization. Central lines, the most common form of new technology, is associated with higher odds of reutilization in unadjusted analyses. However, the second most common form of new technology, nonsurgically placed enteral feeding tube, was not. Further analyses of the differential effects of new technology should be further examined in larger datasets. Additionally, the lack of additional readmission risk from new technology may relate to additional teaching and support provided to families of patients undergoing unfamiliar procedures offsets the risks inherent of greater complexity. If so, it may be that the more intensive teaching and postdischarge support provided to families with new technology or a new diagnosis could be replicated through refresher teaching during hospitalizations, when a patient’s state of health is status quo for the family (ie, the child was admitted and discharged with the same technology and diagnoses). This notion is supported by prior work that demonstrated successful readmission reduction interventions for children with chronic conditions often rely on enhanced education or coaching.25,26
We elected to present models both with and without LOS as a confounder because it is a potentially modifiable attribute of hospitalization. Change in medical complexity aspects were significantly associated with readmission in multivariable models without LOS. However, with the addition of LOS, they were no longer significant. Thus, the readmission risk of new complexity is accounted for by the readmission risk inherent in a longer LOS. This finding prompts additional questions that merit further study: is it that LOS is a general marker for heightened complexity, or is it that a longer LOS can modify readmission risk through additional in-hospital care and time for enhanced education?
Our study has several strengths. We were able to discern true complexity at the time of discharge through medical record review. For example, if a child had a peripherally inserted central catheter placed during hospitalization, it cannot be ascertained through administrative data without medical record review if the technology was removed or in place at discharge. Likewise, medical record review allows for identification of medical technology which is not surgically implanted (eg, nasogastric feeding tubes). Given the “fog” families report as part of their in-hospital experience and its threats to education and postdischarge contingency planning,17 we felt it important to evaluate medical technology regardless of whether or not it was surgically placed. Additionally, the more detailed and nuanced understanding gained of polypharmacy burden can better inform both risk prediction models and interventions to improve the transition from hospital to home.
This study
CONCLUSION
Medical complexity at discharge is associated with pediatric readmission risk. Contrary to our hypothesis, the addition of new technologies and new CCC diagnoses are not associated with pediatric readmission, after accounting for patient and hospitalization factors including LOS. The dynamics of LOS as a risk factor for readmission for children with medical complexity are likely multifaceted and merit further investigation in a multi-institutional study.
Disclosures
The authors report no potential conflicts of interest.
Funding
This work was supported by a grant from the Agency for Healthcare Research and Quality (1K08HS204735-01A1) and a grant from the Blue Cross Blue Shield of Michigan Foundation.
1. Diaz-Caneja A, Gledhill J, Weaver T, Nadel S, Garralda E. A child’s admission to hospital: a qualitative study examining the experiences of parents. Intensive Care Med. 2005;31(9):1248-1254. https://doi.org/10.1007/s00134-005-2728-8.
2. Lapillonne A, Regnault A, Gournay V, et al. Impact on parents of bronchiolitis hospitalization of full-term, preterm and congenital heart disease infants. BMC Pediatrics. 2012;12:171. https://doi.org/10.1186/1471-2431-12-171.
3. Leader S, Jacobson P, Marcin J, Vardis R, Sorrentino M, Murray D. A method for identifying the financial burden of hospitalized infants on families. Value Health. 2002;5(1):55-59. https://doi.org/10.1046/j.1524-4733.2002.51076.x.
4. Leidy NK, Margolis MK, Marcin JP, et al. The impact of severe respiratory syncytial virus on the child, caregiver, and family during hospitalization and recovery. Pediatrics. 2005;115(6):1536-1546. https://doi.org/10.1542/peds.2004-1149.
5. Rennick JE, Johnston CC, Dougherty G, Platt R, Ritchie JA. Children’s psychological responses after critical illness and exposure to invasive technology. J Dev Behav Pediatr. 2002;23(3):133-144. PubMed
6. Brennan TA, Leape LL, Laird NM, et al. Incidence of adverse events and negligence in hospitalized patients. Results of the Harvard Medical Practice Study I. N Engl J Med. 1991;324(6):370-376. https://doi.org/10.1056/NEJM199102073240604.
7. Kohn LT, Corrigan J, Donaldson MS. To err is human: building a safer health system. Washington DC: National Academy Press; 2000.
8. Landrigan CP, Parry GJ, Bones CB, Hackbarth AD, Goldmann DA, Sharek PJ. Temporal trends in rates of patient harm resulting from medical care. N Engl J Med. 2010;363(22):2124-2134. https://doi.org/10.1056/NEJMsa1004404.
9. Magill SS, Edwards JR, Bamberg W, et al. Multistate point-prevalence survey of healthcare-associated infections. N Engl J Med. 2014;370(13):1198-1208. https://doi.org/10.1056/NEJMoa1306801.
10. Berry JG, Toomey SL, Zaslavsky AM, et al. Pediatric readmission prevalence and variability across hospitals. JAMA. 2013;309(4):372-380. https://doi.org/10.1001/jama.2012.188351.
11. Bardach NS, Vittinghoff E, Asteria-Penaloza R, et al. Measuring hospital quality using pediatric readmission and revisit rates. Pediatrics. 2013;132(3):429-436. https://doi.org/10.1542/peds.2012-3527.
12. Toomey SL, Peltz A, Loren S, et al. Potentially preventable 30-day hospital readmissions at a children’s hospital. Pediatrics. 2016;138(2):pii: e20154182. https://doi.org/10.1542/peds.2015-4182.
13. Bucholz EM, Gay JC, Hall M, Harris M, Berry JG. Timing and causes of common pediatric readmissions. J Pediatr. 2018;200:240-248. https://doi.org/10.1016/j.jpeds.2018.04.044.
14. Berry JG, Hall DE, Kuo DZ, et al. Hospital utilization and characteristics of patients experiencing recurrent readmissions within children’s hospitals. JAMA. 2011;305(7):682-690. https://doi.org/10.1001/jama.2011.122.
15. Winer JC, Aragona E, Fields AI, Stockwell DC. Comparison of clinical risk factors among pediatric patients with single admission, multiple admissions (without any 7-day readmissions), and 7-day readmission. Hosp Pediatr. 2016;6(3):119-125. https://doi.org/10.1542/hpeds.2015-0110.
16. Brittan MS, Martin S, Anderson L, Moss A, Torok MR. An electronic health record tool designed to improve pediatric hospital discharge has low predictive utility for readmissions. J Hosp Med. 2018;13(11):779-782. https://doi.org/10.12788/jhm.3043.
17. Solan LG, Beck AF, Brunswick SA, et al. The family perspective on hospital to home transitions: a qualitative study. Pediatrics. 2015;136(6):e1539-e1549. https://doi.org/10.1542/peds.2015-2098.
18. Auger KA, Mueller EL, Weinberg SH, et al. A validated method for identifying unplanned pediatric readmission. J Pediatr. 2016;170:105-112. https://doi.org/10.1016/j.jpeds.2015.11.051.
19. Auger KA, Shah SS, Davis MD, Brady PW. Counting the Ways to Count Medications: The Challenges of Defining Pediatric Polypharmacy. J Hosp Med. 2019;14(8):506-507. https://doi.org/10.12788/jhm.3213.
20. Feudtner C, Feinstein JA, Zhong W, Hall M, Dai D. Pediatric complex chronic conditions classification system version 2: updated for ICD-10 and complex medical technology dependence and transplantation. BMC Pediatrics. 2014;14:199. https://doi.org/10.1186/1471-2431-14-199.
21. Hsieh FY. Sample size tables for logistic regression. Stat Med. 1989;8(7):795-802. https://doi.org/10.1002/sim.4780080704.
22. May C, Montori VM, Mair FS. We need minimally disruptive medicine. BMJ. 2009;339:b2803. https://doi.org/10.1136/bmj.b2803.
23. Leppin AL, Montori VM, Gionfriddo MR. Minimally disruptive medicine: a pragmatically comprehensive model for delivering care to patients with multiple chronic conditions. Healthcare (Basel). 2015;3(1):50-63. https://doi.org/10.3390/healthcare3010050.
24. Serrano V, Spencer-Bonilla G, Boehmer KR, Montori VM. Minimally disruptive medicine for patients with diabetes. Curr Diab Rep. 2017;17(11):104. https://doi.org/10.1007/s11892-017-0935-7.
25. Auger KA, Kenyon CC, Feudtner C, Davis MM. Pediatric hospital discharge interventions to reduce subsequent utilization: a systematic review. J Hosp Med. 2013;9(4):251-260. https://doi.org/10.1002/jhm.2134.
26. Coller RJ, Klitzner TS, Lerner CF, et al. Complex care hospital use and postdischarge coaching: a randomized controlled trial. Pediatrics. 2018;142(2):pii: e20174278. https://doi.org/10.1542/peds.2017-4278.
27. Hain PD, Gay JC, Berutti TW, Whitney GM, Wang W, Saville BR. Preventability of early readmissions at a children’s hospital. Pediatrics. 2013;131(1):e171-e181. https://doi.org/10.1542/peds.2012-0820.
28. Auger KA, Teufel RJ, 2nd, Harris JM, 2nd, et al. Children’s hospital characteristics and readmission metrics. Pediatrics. 2017;139(2). https://doi.org/10.1542/peds.2016-1720.
29. Gay JC, Agrawal R, Auger KA, et al. Rates and impact of potentially preventable readmissions at children’s hospitals. J Pediatr. 2015;166(3):613-619 e615. https://doi.org/10.1016/j.jpeds.2014.10.052.
Hospitalizations are disruptive, stressful, and costly for patients and families.1-5 Hospital readmissions subject families to the additional morbidity inherent to hospitalization and place patients at additional risk of hospital-acquired conditions or other harm.6-9 In pediatrics, hospital readmissions are common for specific conditions;10 with rates varying across institutions;10,11 and as many as one-third of unplanned pediatric readmissions are potentially preventable.12
Reducing pediatric readmissions requires a deeper understanding of the mechanisms through which readmissions occur. Medical complexity—specifically chronic conditions and use of medical technology—is associated with increased risk of readmission.13,14 Polypharmacy at discharge has also been associated with readmission.15,16 However, prior studies on polypharmacy and readmission risk examined the count of total medications and did not consider the nuances of scheduled versus as-needed medications, or the frequency of doses. These nuances may be critical to caregivers as discharge medical complexity can be overwhelming, even in diagnoses which are not traditionally considered complex.17 Finally, of potentially greater importance than medical complexity at discharge is a change in medical complexity during a hospitalization—for example, new diagnoses or new technologies that require additional education in hospital and management at home.
We sought to further understand the relationship between discharge medical complexity and readmission risk with regards to polypharmacy and home healthcare referrals at discharge. Specifically, we hypothesized that a change in medical complexity during an admission—ie, a new chronic diagnosis or new technology—would be a more prominent risk factor for readmission than discharge complexity alone. We examined these factors in the context of length of stay (LOS) since this is a marker of in-hospital severity of illness and a potentially modifiable function of time allowed for in-hospital teaching and discharge preparation.
METHODS
We conducted a retrospective, case-control study of pediatric hospitalizations at one tertiary care children’s hospital. Children <18 years were eligible for inclusion. Normal birth hospitalizations were excluded. We randomly selected one hospitalization from each child as the index visit. We identified cases, hospitalizations at C.S. Mott Children’s Hospital between 2008 and 2012 with a subsequent unplanned 30-day readmission,18 and matched them one to one with hospitalizations at the same hospital during the same period without subsequent readmission. We matched cases to controls based on the month of admission to account for seasonality of certain illnesses. We also matched on distance and direction from the hospital to the patient’s home to account for the potential to have readmissions to other institutions. We utilized both distance and direction recognizing that a family living 30 miles in one direction would be closer to an urban area with access to more facilities, as opposed to 30 miles in another direction in a rural area without additional access. We subsequently performed medical record review to abstract relevant covariates.
Primary Predictors
Medical Complexity Models (Models 1 and 2):
We evaluated three attributes of discharge medical complexity abstracted by medical record review—discharge medications, technology assistance (ie, tracheostomy, cerebral spinal fluid ventricular shunt, enteral feeding tube, central line), and the need for home healthcare after discharge. We counted discharge medications based on the number of medications listed on the discharge summary separated into scheduled or as needed.19 We also considered the number of scheduled doses to be administered in a 24-hour period (see Appendix methods for more information on counting discharge medications). For assistance by technology, we considered the presence of tracheostomy, cerebral spinal fluid ventricular shunt, enteral feeding tube, and central lines. While we describe these technologies separately, for multivariable analyses we considered the presence of any of the four types of technology.
Change in Medical Complexity Models (Models 3 and 4)
We examined two aspects of change in medical complexity—the presence of a new complex chronic condition (CCC)20 diagnosed during the hospitalization, and a new reliance on medical technology. The presence of new CCC was determined by comparing discharge diagnoses to past medical history abstracted by medical record review. A new CCC was defined as any complex chronic condition that was captured in the discharge diagnoses but was not evident in the past medical history. By definition, all CCCs coded during birth hospitalization (eg, at discharge from the neonatal intensive care unit) were assigned to “new” CCC. We calculated a kappa statistic to determine interrater reliability in determining the designation of new CCC. A sensitivity analysis examining these birth CCCs was also performed comparing no new CCC, new CCC, and new CCC after birth hospitalization. The methods appendix provides additional information on considering new CCCs. New technology, abstracted from chart review, was defined as technology placed during hospitalization that remained in place at discharge. If a child with existing technology had additional technology placed during the hospitalization (eg, a new tracheostomy in a child with a previously placed enteral feeding tube), the encounter was considered as having new technology placed.
Covariates
We created different sets of multivariable models to account for patient/hospitalization characteristics.
Statistical Analysis
A review of 600 cases and 600 controls yields 89% power to detect statistical significance for covariates with an odds ratio of 1.25 (β = 0.22) if the candidate covariate has low to moderate correlation with other covariates (<0.3). If a candidate covariate has a moderate correlation with other covariates (0.6), we have 89% power to detect an odds ratio of 1.35 (β = 0.30).21 We calculated odds of 30-days unplanned readmission using conditional logistic regression to account for matched case-control design. All the analyses were performed using STATA 13 (Stata Corp., College Station, Texas).
RESULTS
Of the 41,422 eligible index hospitalizations during the study period, 9.4% resulted in a 30-day unplanned readmission. After randomly selecting one hospitalization per child, there were 781 eligible cases. We subsequent matched all but one eligible case to a control. We randomly selected encounters for medical record review, reviewing a total of 1,212 encounters. After excluding pairs with incomplete records, we included 595 cases and 595 controls in this analysis (Figure). Patient/hospitalization characteristics are displayed in Table 1. The most frequent primary discharge diagnoses are displayed in Appendix Table 1.
Models of Medical Complexity at Discharge
Polypharmacy after discharge was common for both readmitted and nonreadmitted patients. Children who experienced unplanned readmission in 30 days were discharged with a median of four different scheduled medications (interquartile range [IQR] 2,7) which translated into a median of six (IQR 3,12) scheduled doses in a 24-hour period. In comparison, children without an unplanned readmission had a median of two different scheduled medications (IQR 1,3) with a median of three (IQR 0,7) scheduled doses in a 24-hour period. Medical technology was more common in case children (42%) than in control children (14%). Central lines and enteral tubes were the most common forms of medical technology in both cases and controls. Home health referral was common in both cases (44%) and controls (23%; Table 1).
In Model 1 (adjusting only for patient characteristics; Table 3), being discharged on two or more scheduled medications was associated with higher odds of readmission compared to being discharged without medications, with additional medications associated with even higher odds of readmission. Children with any technology had higher odds of readmission than children without medical technology. Likewise, home healthcare visits after discharge were associated with elevated odds of readmission in multivariable analyses without LOS. However, after adding LOS to the model (Model 2), home healthcare visits were no longer significantly associated with readmission.
Change in Medical Complexity Models
The adjudication of new CCCs had good reliability (Κ = 0.72). New CCCs occurred in 18% and new technologies occurred in 17% of cases. Comparatively, new CCCs occurred in 10% and new technologies in 7% of hospitalizations in control children (Table 1). In bivariate analyses, both aspects of change in medical complexity were associated with higher odds of readmission (Table 2). In multivariate analysis with patient characteristics (Model 3; Table 3), all aspects of change in complexity were associated with elevated odds of readmission. A new CCC was associated with higher odds of readmission (adjusted OR (AOR) 1.75, 95% CI: 1.11-2.75) as was new technology during admission (AOR 1.84, 95%CI: 1.09-3.10). Furthermore, the odds of readmission for medical complexity variables (polypharmacy and home healthcare need) remained largely unchanged when adding the change in medical complexity variables (ie, comparing Model 1 and Model 3). However, when accounting for LOS (Model 4), neither the acquisition of a new CCC nor the addition of new technology was associated with readmission. The most common form of new technology was central line followed by nonsurgically placed enteral tube (Appendix Table 2). Finally, in sensitivity analyses (results not detailed), separating new CCC acquired at birth and new CCCs in nonbirth hospitalizations, compared to hospitalizations with no new CCC, yielded similar results as the primary analyses.
DISCUSSION
We examined multiple attributes of polypharmacy—the number of scheduled medications, number of as-needed medications, and number of scheduled doses per 24 hours. Interestingly, only the scheduled medications (count of medication and number of doses) were associated with elevated readmission risk. As-needed medications have heterogeneity in the level of importance from critical (eg, seizure rescue) to discretionary (eg, antipyretics, creams). The burden of managing these types of medications may still be high (ie, parents must decide when to administer a critical medication); however, this burden does not translate into increased readmission risk in this population.
Not surprisingly, greater medical complexity—as defined by higher numbers of scheduled discharge medications and technology assistance—is associated with 30-day readmission risk. Our analyses do not allow us to determine how much of the increased risk is due to additional care burden and risks of polypharmacy versus the inherent increase in complexity and severity of illness for which polypharmacy is a marker. Tailoring discharge regimens to the realities of daily life, with the goal of “minimally disruptive medicine”22,23 (eg, integrating manageable discharge medication routines into school and work schedules), is not a common feature of pediatric discharge planning. For adult patients with complex medical conditions, tailoring medication regimens in a minimally disruptive way is known to improve outcomes.24 Similarly, adopting minimally disruptive techniques to integrate the polypharmacy inherent in discharge could potentially mitigate some of the readmission risks for children and adolescents.
Contrary to our hypothesis, new technologies and new diagnoses did not confer additional readmission risk when accounting for LOS and patient characteristics. One potential explanation is varying risks conveyed by different types of new technologies placed during hospitalization. Central lines, the most common form of new technology, is associated with higher odds of reutilization in unadjusted analyses. However, the second most common form of new technology, nonsurgically placed enteral feeding tube, was not. Further analyses of the differential effects of new technology should be further examined in larger datasets. Additionally, the lack of additional readmission risk from new technology may relate to additional teaching and support provided to families of patients undergoing unfamiliar procedures offsets the risks inherent of greater complexity. If so, it may be that the more intensive teaching and postdischarge support provided to families with new technology or a new diagnosis could be replicated through refresher teaching during hospitalizations, when a patient’s state of health is status quo for the family (ie, the child was admitted and discharged with the same technology and diagnoses). This notion is supported by prior work that demonstrated successful readmission reduction interventions for children with chronic conditions often rely on enhanced education or coaching.25,26
We elected to present models both with and without LOS as a confounder because it is a potentially modifiable attribute of hospitalization. Change in medical complexity aspects were significantly associated with readmission in multivariable models without LOS. However, with the addition of LOS, they were no longer significant. Thus, the readmission risk of new complexity is accounted for by the readmission risk inherent in a longer LOS. This finding prompts additional questions that merit further study: is it that LOS is a general marker for heightened complexity, or is it that a longer LOS can modify readmission risk through additional in-hospital care and time for enhanced education?
Our study has several strengths. We were able to discern true complexity at the time of discharge through medical record review. For example, if a child had a peripherally inserted central catheter placed during hospitalization, it cannot be ascertained through administrative data without medical record review if the technology was removed or in place at discharge. Likewise, medical record review allows for identification of medical technology which is not surgically implanted (eg, nasogastric feeding tubes). Given the “fog” families report as part of their in-hospital experience and its threats to education and postdischarge contingency planning,17 we felt it important to evaluate medical technology regardless of whether or not it was surgically placed. Additionally, the more detailed and nuanced understanding gained of polypharmacy burden can better inform both risk prediction models and interventions to improve the transition from hospital to home.
This study
CONCLUSION
Medical complexity at discharge is associated with pediatric readmission risk. Contrary to our hypothesis, the addition of new technologies and new CCC diagnoses are not associated with pediatric readmission, after accounting for patient and hospitalization factors including LOS. The dynamics of LOS as a risk factor for readmission for children with medical complexity are likely multifaceted and merit further investigation in a multi-institutional study.
Disclosures
The authors report no potential conflicts of interest.
Funding
This work was supported by a grant from the Agency for Healthcare Research and Quality (1K08HS204735-01A1) and a grant from the Blue Cross Blue Shield of Michigan Foundation.
Hospitalizations are disruptive, stressful, and costly for patients and families.1-5 Hospital readmissions subject families to the additional morbidity inherent to hospitalization and place patients at additional risk of hospital-acquired conditions or other harm.6-9 In pediatrics, hospital readmissions are common for specific conditions;10 with rates varying across institutions;10,11 and as many as one-third of unplanned pediatric readmissions are potentially preventable.12
Reducing pediatric readmissions requires a deeper understanding of the mechanisms through which readmissions occur. Medical complexity—specifically chronic conditions and use of medical technology—is associated with increased risk of readmission.13,14 Polypharmacy at discharge has also been associated with readmission.15,16 However, prior studies on polypharmacy and readmission risk examined the count of total medications and did not consider the nuances of scheduled versus as-needed medications, or the frequency of doses. These nuances may be critical to caregivers as discharge medical complexity can be overwhelming, even in diagnoses which are not traditionally considered complex.17 Finally, of potentially greater importance than medical complexity at discharge is a change in medical complexity during a hospitalization—for example, new diagnoses or new technologies that require additional education in hospital and management at home.
We sought to further understand the relationship between discharge medical complexity and readmission risk with regards to polypharmacy and home healthcare referrals at discharge. Specifically, we hypothesized that a change in medical complexity during an admission—ie, a new chronic diagnosis or new technology—would be a more prominent risk factor for readmission than discharge complexity alone. We examined these factors in the context of length of stay (LOS) since this is a marker of in-hospital severity of illness and a potentially modifiable function of time allowed for in-hospital teaching and discharge preparation.
METHODS
We conducted a retrospective, case-control study of pediatric hospitalizations at one tertiary care children’s hospital. Children <18 years were eligible for inclusion. Normal birth hospitalizations were excluded. We randomly selected one hospitalization from each child as the index visit. We identified cases, hospitalizations at C.S. Mott Children’s Hospital between 2008 and 2012 with a subsequent unplanned 30-day readmission,18 and matched them one to one with hospitalizations at the same hospital during the same period without subsequent readmission. We matched cases to controls based on the month of admission to account for seasonality of certain illnesses. We also matched on distance and direction from the hospital to the patient’s home to account for the potential to have readmissions to other institutions. We utilized both distance and direction recognizing that a family living 30 miles in one direction would be closer to an urban area with access to more facilities, as opposed to 30 miles in another direction in a rural area without additional access. We subsequently performed medical record review to abstract relevant covariates.
Primary Predictors
Medical Complexity Models (Models 1 and 2):
We evaluated three attributes of discharge medical complexity abstracted by medical record review—discharge medications, technology assistance (ie, tracheostomy, cerebral spinal fluid ventricular shunt, enteral feeding tube, central line), and the need for home healthcare after discharge. We counted discharge medications based on the number of medications listed on the discharge summary separated into scheduled or as needed.19 We also considered the number of scheduled doses to be administered in a 24-hour period (see Appendix methods for more information on counting discharge medications). For assistance by technology, we considered the presence of tracheostomy, cerebral spinal fluid ventricular shunt, enteral feeding tube, and central lines. While we describe these technologies separately, for multivariable analyses we considered the presence of any of the four types of technology.
Change in Medical Complexity Models (Models 3 and 4)
We examined two aspects of change in medical complexity—the presence of a new complex chronic condition (CCC)20 diagnosed during the hospitalization, and a new reliance on medical technology. The presence of new CCC was determined by comparing discharge diagnoses to past medical history abstracted by medical record review. A new CCC was defined as any complex chronic condition that was captured in the discharge diagnoses but was not evident in the past medical history. By definition, all CCCs coded during birth hospitalization (eg, at discharge from the neonatal intensive care unit) were assigned to “new” CCC. We calculated a kappa statistic to determine interrater reliability in determining the designation of new CCC. A sensitivity analysis examining these birth CCCs was also performed comparing no new CCC, new CCC, and new CCC after birth hospitalization. The methods appendix provides additional information on considering new CCCs. New technology, abstracted from chart review, was defined as technology placed during hospitalization that remained in place at discharge. If a child with existing technology had additional technology placed during the hospitalization (eg, a new tracheostomy in a child with a previously placed enteral feeding tube), the encounter was considered as having new technology placed.
Covariates
We created different sets of multivariable models to account for patient/hospitalization characteristics.
Statistical Analysis
A review of 600 cases and 600 controls yields 89% power to detect statistical significance for covariates with an odds ratio of 1.25 (β = 0.22) if the candidate covariate has low to moderate correlation with other covariates (<0.3). If a candidate covariate has a moderate correlation with other covariates (0.6), we have 89% power to detect an odds ratio of 1.35 (β = 0.30).21 We calculated odds of 30-days unplanned readmission using conditional logistic regression to account for matched case-control design. All the analyses were performed using STATA 13 (Stata Corp., College Station, Texas).
RESULTS
Of the 41,422 eligible index hospitalizations during the study period, 9.4% resulted in a 30-day unplanned readmission. After randomly selecting one hospitalization per child, there were 781 eligible cases. We subsequent matched all but one eligible case to a control. We randomly selected encounters for medical record review, reviewing a total of 1,212 encounters. After excluding pairs with incomplete records, we included 595 cases and 595 controls in this analysis (Figure). Patient/hospitalization characteristics are displayed in Table 1. The most frequent primary discharge diagnoses are displayed in Appendix Table 1.
Models of Medical Complexity at Discharge
Polypharmacy after discharge was common for both readmitted and nonreadmitted patients. Children who experienced unplanned readmission in 30 days were discharged with a median of four different scheduled medications (interquartile range [IQR] 2,7) which translated into a median of six (IQR 3,12) scheduled doses in a 24-hour period. In comparison, children without an unplanned readmission had a median of two different scheduled medications (IQR 1,3) with a median of three (IQR 0,7) scheduled doses in a 24-hour period. Medical technology was more common in case children (42%) than in control children (14%). Central lines and enteral tubes were the most common forms of medical technology in both cases and controls. Home health referral was common in both cases (44%) and controls (23%; Table 1).
In Model 1 (adjusting only for patient characteristics; Table 3), being discharged on two or more scheduled medications was associated with higher odds of readmission compared to being discharged without medications, with additional medications associated with even higher odds of readmission. Children with any technology had higher odds of readmission than children without medical technology. Likewise, home healthcare visits after discharge were associated with elevated odds of readmission in multivariable analyses without LOS. However, after adding LOS to the model (Model 2), home healthcare visits were no longer significantly associated with readmission.
Change in Medical Complexity Models
The adjudication of new CCCs had good reliability (Κ = 0.72). New CCCs occurred in 18% and new technologies occurred in 17% of cases. Comparatively, new CCCs occurred in 10% and new technologies in 7% of hospitalizations in control children (Table 1). In bivariate analyses, both aspects of change in medical complexity were associated with higher odds of readmission (Table 2). In multivariate analysis with patient characteristics (Model 3; Table 3), all aspects of change in complexity were associated with elevated odds of readmission. A new CCC was associated with higher odds of readmission (adjusted OR (AOR) 1.75, 95% CI: 1.11-2.75) as was new technology during admission (AOR 1.84, 95%CI: 1.09-3.10). Furthermore, the odds of readmission for medical complexity variables (polypharmacy and home healthcare need) remained largely unchanged when adding the change in medical complexity variables (ie, comparing Model 1 and Model 3). However, when accounting for LOS (Model 4), neither the acquisition of a new CCC nor the addition of new technology was associated with readmission. The most common form of new technology was central line followed by nonsurgically placed enteral tube (Appendix Table 2). Finally, in sensitivity analyses (results not detailed), separating new CCC acquired at birth and new CCCs in nonbirth hospitalizations, compared to hospitalizations with no new CCC, yielded similar results as the primary analyses.
DISCUSSION
We examined multiple attributes of polypharmacy—the number of scheduled medications, number of as-needed medications, and number of scheduled doses per 24 hours. Interestingly, only the scheduled medications (count of medication and number of doses) were associated with elevated readmission risk. As-needed medications have heterogeneity in the level of importance from critical (eg, seizure rescue) to discretionary (eg, antipyretics, creams). The burden of managing these types of medications may still be high (ie, parents must decide when to administer a critical medication); however, this burden does not translate into increased readmission risk in this population.
Not surprisingly, greater medical complexity—as defined by higher numbers of scheduled discharge medications and technology assistance—is associated with 30-day readmission risk. Our analyses do not allow us to determine how much of the increased risk is due to additional care burden and risks of polypharmacy versus the inherent increase in complexity and severity of illness for which polypharmacy is a marker. Tailoring discharge regimens to the realities of daily life, with the goal of “minimally disruptive medicine”22,23 (eg, integrating manageable discharge medication routines into school and work schedules), is not a common feature of pediatric discharge planning. For adult patients with complex medical conditions, tailoring medication regimens in a minimally disruptive way is known to improve outcomes.24 Similarly, adopting minimally disruptive techniques to integrate the polypharmacy inherent in discharge could potentially mitigate some of the readmission risks for children and adolescents.
Contrary to our hypothesis, new technologies and new diagnoses did not confer additional readmission risk when accounting for LOS and patient characteristics. One potential explanation is varying risks conveyed by different types of new technologies placed during hospitalization. Central lines, the most common form of new technology, is associated with higher odds of reutilization in unadjusted analyses. However, the second most common form of new technology, nonsurgically placed enteral feeding tube, was not. Further analyses of the differential effects of new technology should be further examined in larger datasets. Additionally, the lack of additional readmission risk from new technology may relate to additional teaching and support provided to families of patients undergoing unfamiliar procedures offsets the risks inherent of greater complexity. If so, it may be that the more intensive teaching and postdischarge support provided to families with new technology or a new diagnosis could be replicated through refresher teaching during hospitalizations, when a patient’s state of health is status quo for the family (ie, the child was admitted and discharged with the same technology and diagnoses). This notion is supported by prior work that demonstrated successful readmission reduction interventions for children with chronic conditions often rely on enhanced education or coaching.25,26
We elected to present models both with and without LOS as a confounder because it is a potentially modifiable attribute of hospitalization. Change in medical complexity aspects were significantly associated with readmission in multivariable models without LOS. However, with the addition of LOS, they were no longer significant. Thus, the readmission risk of new complexity is accounted for by the readmission risk inherent in a longer LOS. This finding prompts additional questions that merit further study: is it that LOS is a general marker for heightened complexity, or is it that a longer LOS can modify readmission risk through additional in-hospital care and time for enhanced education?
Our study has several strengths. We were able to discern true complexity at the time of discharge through medical record review. For example, if a child had a peripherally inserted central catheter placed during hospitalization, it cannot be ascertained through administrative data without medical record review if the technology was removed or in place at discharge. Likewise, medical record review allows for identification of medical technology which is not surgically implanted (eg, nasogastric feeding tubes). Given the “fog” families report as part of their in-hospital experience and its threats to education and postdischarge contingency planning,17 we felt it important to evaluate medical technology regardless of whether or not it was surgically placed. Additionally, the more detailed and nuanced understanding gained of polypharmacy burden can better inform both risk prediction models and interventions to improve the transition from hospital to home.
This study
CONCLUSION
Medical complexity at discharge is associated with pediatric readmission risk. Contrary to our hypothesis, the addition of new technologies and new CCC diagnoses are not associated with pediatric readmission, after accounting for patient and hospitalization factors including LOS. The dynamics of LOS as a risk factor for readmission for children with medical complexity are likely multifaceted and merit further investigation in a multi-institutional study.
Disclosures
The authors report no potential conflicts of interest.
Funding
This work was supported by a grant from the Agency for Healthcare Research and Quality (1K08HS204735-01A1) and a grant from the Blue Cross Blue Shield of Michigan Foundation.
1. Diaz-Caneja A, Gledhill J, Weaver T, Nadel S, Garralda E. A child’s admission to hospital: a qualitative study examining the experiences of parents. Intensive Care Med. 2005;31(9):1248-1254. https://doi.org/10.1007/s00134-005-2728-8.
2. Lapillonne A, Regnault A, Gournay V, et al. Impact on parents of bronchiolitis hospitalization of full-term, preterm and congenital heart disease infants. BMC Pediatrics. 2012;12:171. https://doi.org/10.1186/1471-2431-12-171.
3. Leader S, Jacobson P, Marcin J, Vardis R, Sorrentino M, Murray D. A method for identifying the financial burden of hospitalized infants on families. Value Health. 2002;5(1):55-59. https://doi.org/10.1046/j.1524-4733.2002.51076.x.
4. Leidy NK, Margolis MK, Marcin JP, et al. The impact of severe respiratory syncytial virus on the child, caregiver, and family during hospitalization and recovery. Pediatrics. 2005;115(6):1536-1546. https://doi.org/10.1542/peds.2004-1149.
5. Rennick JE, Johnston CC, Dougherty G, Platt R, Ritchie JA. Children’s psychological responses after critical illness and exposure to invasive technology. J Dev Behav Pediatr. 2002;23(3):133-144. PubMed
6. Brennan TA, Leape LL, Laird NM, et al. Incidence of adverse events and negligence in hospitalized patients. Results of the Harvard Medical Practice Study I. N Engl J Med. 1991;324(6):370-376. https://doi.org/10.1056/NEJM199102073240604.
7. Kohn LT, Corrigan J, Donaldson MS. To err is human: building a safer health system. Washington DC: National Academy Press; 2000.
8. Landrigan CP, Parry GJ, Bones CB, Hackbarth AD, Goldmann DA, Sharek PJ. Temporal trends in rates of patient harm resulting from medical care. N Engl J Med. 2010;363(22):2124-2134. https://doi.org/10.1056/NEJMsa1004404.
9. Magill SS, Edwards JR, Bamberg W, et al. Multistate point-prevalence survey of healthcare-associated infections. N Engl J Med. 2014;370(13):1198-1208. https://doi.org/10.1056/NEJMoa1306801.
10. Berry JG, Toomey SL, Zaslavsky AM, et al. Pediatric readmission prevalence and variability across hospitals. JAMA. 2013;309(4):372-380. https://doi.org/10.1001/jama.2012.188351.
11. Bardach NS, Vittinghoff E, Asteria-Penaloza R, et al. Measuring hospital quality using pediatric readmission and revisit rates. Pediatrics. 2013;132(3):429-436. https://doi.org/10.1542/peds.2012-3527.
12. Toomey SL, Peltz A, Loren S, et al. Potentially preventable 30-day hospital readmissions at a children’s hospital. Pediatrics. 2016;138(2):pii: e20154182. https://doi.org/10.1542/peds.2015-4182.
13. Bucholz EM, Gay JC, Hall M, Harris M, Berry JG. Timing and causes of common pediatric readmissions. J Pediatr. 2018;200:240-248. https://doi.org/10.1016/j.jpeds.2018.04.044.
14. Berry JG, Hall DE, Kuo DZ, et al. Hospital utilization and characteristics of patients experiencing recurrent readmissions within children’s hospitals. JAMA. 2011;305(7):682-690. https://doi.org/10.1001/jama.2011.122.
15. Winer JC, Aragona E, Fields AI, Stockwell DC. Comparison of clinical risk factors among pediatric patients with single admission, multiple admissions (without any 7-day readmissions), and 7-day readmission. Hosp Pediatr. 2016;6(3):119-125. https://doi.org/10.1542/hpeds.2015-0110.
16. Brittan MS, Martin S, Anderson L, Moss A, Torok MR. An electronic health record tool designed to improve pediatric hospital discharge has low predictive utility for readmissions. J Hosp Med. 2018;13(11):779-782. https://doi.org/10.12788/jhm.3043.
17. Solan LG, Beck AF, Brunswick SA, et al. The family perspective on hospital to home transitions: a qualitative study. Pediatrics. 2015;136(6):e1539-e1549. https://doi.org/10.1542/peds.2015-2098.
18. Auger KA, Mueller EL, Weinberg SH, et al. A validated method for identifying unplanned pediatric readmission. J Pediatr. 2016;170:105-112. https://doi.org/10.1016/j.jpeds.2015.11.051.
19. Auger KA, Shah SS, Davis MD, Brady PW. Counting the Ways to Count Medications: The Challenges of Defining Pediatric Polypharmacy. J Hosp Med. 2019;14(8):506-507. https://doi.org/10.12788/jhm.3213.
20. Feudtner C, Feinstein JA, Zhong W, Hall M, Dai D. Pediatric complex chronic conditions classification system version 2: updated for ICD-10 and complex medical technology dependence and transplantation. BMC Pediatrics. 2014;14:199. https://doi.org/10.1186/1471-2431-14-199.
21. Hsieh FY. Sample size tables for logistic regression. Stat Med. 1989;8(7):795-802. https://doi.org/10.1002/sim.4780080704.
22. May C, Montori VM, Mair FS. We need minimally disruptive medicine. BMJ. 2009;339:b2803. https://doi.org/10.1136/bmj.b2803.
23. Leppin AL, Montori VM, Gionfriddo MR. Minimally disruptive medicine: a pragmatically comprehensive model for delivering care to patients with multiple chronic conditions. Healthcare (Basel). 2015;3(1):50-63. https://doi.org/10.3390/healthcare3010050.
24. Serrano V, Spencer-Bonilla G, Boehmer KR, Montori VM. Minimally disruptive medicine for patients with diabetes. Curr Diab Rep. 2017;17(11):104. https://doi.org/10.1007/s11892-017-0935-7.
25. Auger KA, Kenyon CC, Feudtner C, Davis MM. Pediatric hospital discharge interventions to reduce subsequent utilization: a systematic review. J Hosp Med. 2013;9(4):251-260. https://doi.org/10.1002/jhm.2134.
26. Coller RJ, Klitzner TS, Lerner CF, et al. Complex care hospital use and postdischarge coaching: a randomized controlled trial. Pediatrics. 2018;142(2):pii: e20174278. https://doi.org/10.1542/peds.2017-4278.
27. Hain PD, Gay JC, Berutti TW, Whitney GM, Wang W, Saville BR. Preventability of early readmissions at a children’s hospital. Pediatrics. 2013;131(1):e171-e181. https://doi.org/10.1542/peds.2012-0820.
28. Auger KA, Teufel RJ, 2nd, Harris JM, 2nd, et al. Children’s hospital characteristics and readmission metrics. Pediatrics. 2017;139(2). https://doi.org/10.1542/peds.2016-1720.
29. Gay JC, Agrawal R, Auger KA, et al. Rates and impact of potentially preventable readmissions at children’s hospitals. J Pediatr. 2015;166(3):613-619 e615. https://doi.org/10.1016/j.jpeds.2014.10.052.
1. Diaz-Caneja A, Gledhill J, Weaver T, Nadel S, Garralda E. A child’s admission to hospital: a qualitative study examining the experiences of parents. Intensive Care Med. 2005;31(9):1248-1254. https://doi.org/10.1007/s00134-005-2728-8.
2. Lapillonne A, Regnault A, Gournay V, et al. Impact on parents of bronchiolitis hospitalization of full-term, preterm and congenital heart disease infants. BMC Pediatrics. 2012;12:171. https://doi.org/10.1186/1471-2431-12-171.
3. Leader S, Jacobson P, Marcin J, Vardis R, Sorrentino M, Murray D. A method for identifying the financial burden of hospitalized infants on families. Value Health. 2002;5(1):55-59. https://doi.org/10.1046/j.1524-4733.2002.51076.x.
4. Leidy NK, Margolis MK, Marcin JP, et al. The impact of severe respiratory syncytial virus on the child, caregiver, and family during hospitalization and recovery. Pediatrics. 2005;115(6):1536-1546. https://doi.org/10.1542/peds.2004-1149.
5. Rennick JE, Johnston CC, Dougherty G, Platt R, Ritchie JA. Children’s psychological responses after critical illness and exposure to invasive technology. J Dev Behav Pediatr. 2002;23(3):133-144. PubMed
6. Brennan TA, Leape LL, Laird NM, et al. Incidence of adverse events and negligence in hospitalized patients. Results of the Harvard Medical Practice Study I. N Engl J Med. 1991;324(6):370-376. https://doi.org/10.1056/NEJM199102073240604.
7. Kohn LT, Corrigan J, Donaldson MS. To err is human: building a safer health system. Washington DC: National Academy Press; 2000.
8. Landrigan CP, Parry GJ, Bones CB, Hackbarth AD, Goldmann DA, Sharek PJ. Temporal trends in rates of patient harm resulting from medical care. N Engl J Med. 2010;363(22):2124-2134. https://doi.org/10.1056/NEJMsa1004404.
9. Magill SS, Edwards JR, Bamberg W, et al. Multistate point-prevalence survey of healthcare-associated infections. N Engl J Med. 2014;370(13):1198-1208. https://doi.org/10.1056/NEJMoa1306801.
10. Berry JG, Toomey SL, Zaslavsky AM, et al. Pediatric readmission prevalence and variability across hospitals. JAMA. 2013;309(4):372-380. https://doi.org/10.1001/jama.2012.188351.
11. Bardach NS, Vittinghoff E, Asteria-Penaloza R, et al. Measuring hospital quality using pediatric readmission and revisit rates. Pediatrics. 2013;132(3):429-436. https://doi.org/10.1542/peds.2012-3527.
12. Toomey SL, Peltz A, Loren S, et al. Potentially preventable 30-day hospital readmissions at a children’s hospital. Pediatrics. 2016;138(2):pii: e20154182. https://doi.org/10.1542/peds.2015-4182.
13. Bucholz EM, Gay JC, Hall M, Harris M, Berry JG. Timing and causes of common pediatric readmissions. J Pediatr. 2018;200:240-248. https://doi.org/10.1016/j.jpeds.2018.04.044.
14. Berry JG, Hall DE, Kuo DZ, et al. Hospital utilization and characteristics of patients experiencing recurrent readmissions within children’s hospitals. JAMA. 2011;305(7):682-690. https://doi.org/10.1001/jama.2011.122.
15. Winer JC, Aragona E, Fields AI, Stockwell DC. Comparison of clinical risk factors among pediatric patients with single admission, multiple admissions (without any 7-day readmissions), and 7-day readmission. Hosp Pediatr. 2016;6(3):119-125. https://doi.org/10.1542/hpeds.2015-0110.
16. Brittan MS, Martin S, Anderson L, Moss A, Torok MR. An electronic health record tool designed to improve pediatric hospital discharge has low predictive utility for readmissions. J Hosp Med. 2018;13(11):779-782. https://doi.org/10.12788/jhm.3043.
17. Solan LG, Beck AF, Brunswick SA, et al. The family perspective on hospital to home transitions: a qualitative study. Pediatrics. 2015;136(6):e1539-e1549. https://doi.org/10.1542/peds.2015-2098.
18. Auger KA, Mueller EL, Weinberg SH, et al. A validated method for identifying unplanned pediatric readmission. J Pediatr. 2016;170:105-112. https://doi.org/10.1016/j.jpeds.2015.11.051.
19. Auger KA, Shah SS, Davis MD, Brady PW. Counting the Ways to Count Medications: The Challenges of Defining Pediatric Polypharmacy. J Hosp Med. 2019;14(8):506-507. https://doi.org/10.12788/jhm.3213.
20. Feudtner C, Feinstein JA, Zhong W, Hall M, Dai D. Pediatric complex chronic conditions classification system version 2: updated for ICD-10 and complex medical technology dependence and transplantation. BMC Pediatrics. 2014;14:199. https://doi.org/10.1186/1471-2431-14-199.
21. Hsieh FY. Sample size tables for logistic regression. Stat Med. 1989;8(7):795-802. https://doi.org/10.1002/sim.4780080704.
22. May C, Montori VM, Mair FS. We need minimally disruptive medicine. BMJ. 2009;339:b2803. https://doi.org/10.1136/bmj.b2803.
23. Leppin AL, Montori VM, Gionfriddo MR. Minimally disruptive medicine: a pragmatically comprehensive model for delivering care to patients with multiple chronic conditions. Healthcare (Basel). 2015;3(1):50-63. https://doi.org/10.3390/healthcare3010050.
24. Serrano V, Spencer-Bonilla G, Boehmer KR, Montori VM. Minimally disruptive medicine for patients with diabetes. Curr Diab Rep. 2017;17(11):104. https://doi.org/10.1007/s11892-017-0935-7.
25. Auger KA, Kenyon CC, Feudtner C, Davis MM. Pediatric hospital discharge interventions to reduce subsequent utilization: a systematic review. J Hosp Med. 2013;9(4):251-260. https://doi.org/10.1002/jhm.2134.
26. Coller RJ, Klitzner TS, Lerner CF, et al. Complex care hospital use and postdischarge coaching: a randomized controlled trial. Pediatrics. 2018;142(2):pii: e20174278. https://doi.org/10.1542/peds.2017-4278.
27. Hain PD, Gay JC, Berutti TW, Whitney GM, Wang W, Saville BR. Preventability of early readmissions at a children’s hospital. Pediatrics. 2013;131(1):e171-e181. https://doi.org/10.1542/peds.2012-0820.
28. Auger KA, Teufel RJ, 2nd, Harris JM, 2nd, et al. Children’s hospital characteristics and readmission metrics. Pediatrics. 2017;139(2). https://doi.org/10.1542/peds.2016-1720.
29. Gay JC, Agrawal R, Auger KA, et al. Rates and impact of potentially preventable readmissions at children’s hospitals. J Pediatr. 2015;166(3):613-619 e615. https://doi.org/10.1016/j.jpeds.2014.10.052.
© 2019 Society of Hospital Medicine
Emergency Transfers: An Important Predictor of Adverse Outcomes in Hospitalized Children
Unrecognized in-hospital deterioration can result in tragic consequences for pediatric patients. The majority of deterioration events have antecedents such as increasingly abnormal vital signs and new concerns from nurses.1 Recent meta-analyses have shown that rapid response systems (RRSs), which include trigger mechanisms such as a pediatric early warning score (PEWS), are associated with a reduced rate of arrests and in-hospital mortality.2,3 Cardiopulmonary arrest rates are useful metrics to judge the effectiveness of the system to identify and respond to deteriorating adult patients; however, there are important challenges to their use as an outcome measure in pediatrics. Arrests, which have been relatively uncommon in pediatric patients, are now even less frequent since the adoption of a RRS in the majority of children’s hospitals.4,5 Several innovations in these systems will be context-dependent and hence best first evaluated in a single center, where arrests outside of the intensive care unit (ICU) may occur rarely. Identification of valid, more frequent proximal measures to arrests may better identify the risk factors for deterioration. This could potentially inform quality improvement efforts to mitigate clinical deterioration.
Bonafide et al. at the Children’s Hospital of Philadelphia developed and validated the critical deterioration event (CDE) metric, demonstrating that children who were transferred to the ICU and who received noninvasive ventilation, intubation, or vasopressor initiation within 12 hours of transfer had a >13-fold increased risk of in-hospital mortality.6 At Cincinnati Children’s Hospital Medical Center, an additional proximal outcome measure was developed for unrecognized clinical deterioration, now termed emergency transfers (ETs).7-9 An ET is defined as any patient transferred to the ICU where the patient received intubation, inotropes, or three or more fluid boluses in the first hour after arrival or before transfer.9 Improvement science work that aimed at increasing clinician situation awareness was associated with a reduction in ETs,8 but the association of ETs with mortality or other healthcare utilization outcomes is unknown. The objective of this study was to determine the predictive validity of an ET on in-hospital mortality, ICU length of stay (LOS), and overall hospital LOS.
METHODS
We conducted a case–control study at Cincinnati Children’s Hospital, a free-standing tertiary care children’s hospital. Our center has had an ICU-based RRS in place since 2005. In 2009, we eliminated the ICU consult such that each floor-to-ICU transfer is evaluated by the RRS. Nurses calculate a Monaghan PEWS every four hours on the majority of nursing units.
Patients of all ages cared for outside of the ICU at any point in their hospitalization from January 1, 2013, to July 31, 2017, were eligible for inclusion. There were no other exclusion criteria. The ICU included both the pediatric ICU and the cardiac ICU.
Cases
We identified all ET cases from an existing situation awareness database in which each RRS call is entered by the hospital nursing supervisor, whose role includes responding to each RRS activation. If the patient transfer meets the ET criteria, the nurse indicates this in the database. Each ET entry is later confirmed for assurance purposes by the nurse leader of the RRS committee (RG). For the purposes of this study, all records were again reviewed and validated using manual chart review in the electronic health record (Epic Systems, Verona, Wisconsin).
Controls
We identified nonemergent ICU transfers to serve as controls and matched those to ET in cases to limit the impact of confounders that may increase the likelihood of both an ET and a negative outcome such as ICU mortality. We identified up to three controls for each case from our database and matched in terms of age group (within five years of age), hospital unit before transfer, and time of year (within three months of ET). These variables were chosen to adjust for the impact of age, diversity of disease (as hospital units are generally organized by organ system of illness), and seasonality on outcomes.
Outcome Measures
Posttransfer LOS in the ICU, posttransfer hospital LOS, and in-hospital mortality were the primary outcome measures. Patient demographics, specific diagnoses, and number of medical conditions were a priori defined as covariates of interest. Data for each case and control were entered into a secure, web-based Research Electronic Data Capture (REDCap) database.
Analysis
Descriptive data were summarized using counts and percentages for categorical variables and medians and ranges for continuous variables due to nonnormal distributions. Chi-square test was used to compare in-hospital mortality between the ETs and the controls. The Wilcoxon rank-sum test was used to compare LOS between ETs and controls. All data analyses were performed using SAS version 9.4 (SAS Institute Inc., Cary, North Carolina).
RESULTS
A total of 45 ETs were identified, and 110 controls were matched. Patient demographics were similar among all cases and controls (P > .05). Patients with ETs had a median age of seven years (interquartile range: 3-18 years), and 51% of them were males. The majority of patients among our examined cases were white (68%) and non-Hispanic (93%). There was no statistical difference in insurance between the ETs and the controls. When evaluating the hospital unit before the transfer, ETs occurred most commonly in the Cardiology (22%), Hematology/Oncology (22%), and Neuroscience (16%) units.
ETs stayed longer in the ICU than non-ETs [median of 4.9 days vs 2.2 days, P = .001; Figure (A)]. Similarly, ET cases had a significantly longer posttransfer hospital LOS [median of 35 days vs 21 days, P = .001; Figure (B)]. ETs had a 22% in-hospital mortality rate, compared with 9% in-hospital mortality in the matched controls (P = .02; Table).
DISCUSSION
Children who experienced an ET had a significantly longer ICU LOS, a longer posttransfer LOS, and a higher in-hospital mortality than the matched controls who were also transferred to the ICU. Researchers and improvement science teams at multiple hospitals have demonstrated that interventions targeting improved situation awareness can reduce ETs; we have demonstrated that reducing ETs may reduce subsequent adverse outcomes.8,10
These findings provide additional support for the use of the ET metric in children’s hospitals as a proximal measure for significant clinical deterioration. We found mortality rates that were overall high for a children’s hospital (22% in ET cases and 9% among controls) compared with a national average mortality rate of 2.3% in pediatric ICUs.11 This is likely due to the study sample containing a significant proportion of children with medical complexity.
Aoki et al. recently demonstrated that ETs, compared with non-ETs, were associated with longer LOS and higher mortality in a bivariate analysis.12 In our study, we found similar results with the important addition that these findings were robust when ETs were compared with matched controls who were likely at a higher risk of poor outcomes than ICU transfers in general. In addition, we demonstrated that ETs were associated with adverse outcomes in a United States children’s hospital with a mature, long-standing RRS process. As ETs are considerably more common than cardiac and respiratory arrests, use of the ET metric in children’s hospitals may enable more rapid learning and systems improvement implementations. We also found that most of the children with ETs present from units that care for children with substantial medical complexity, including Cardiology, Hematology/Oncology, and Neurosciences. Future work should continue to examine the relationship between medical complexity and ET risk.
The ET metric is complementary to the CDE measure developed by Bonafide et al. Both metrics capture potential events of unrecognized clinical deterioration, and both offer researchers the opportunity to better understand and improve their RRSs. Both ETs and CDEs are more common than arrests, and CDEs are more common than ETs. ETs, which by definition occur in the first hour of ICU care, are likely a more specific measure of unrecognized clinical deterioration. CDEs will capture therapies that may have been started up to 12 hours after transfer and thus are possibly more sensitive to identify unrecognized clinical deterioration. However, CDEs also may encompass some patients who arrived at the ICU after prompt recognition and then had a subacute deterioration in the ICU.
The maturity of the RRS and the bandwidth of teams to collect data may inform which metric(s) are best for individual centers. As ETs are less common and likely more specific to unrecognized clinical deterioration, they might be the first tracked as a center improves its RRS through QI methods. Alternatively, CDEs may be a useful metric for centers where unrecognized clinical deterioration is less common or in research studies where this more common outcome would lead to more power to detect the effect of interventions to improve care.
Our study had several limitations. Data collection was confined to one tertiary care children’s hospital with a high burden of complex cardiac and oncology care. The results may not generalize well to children hospitalized in smaller or community hospitals or in hospitals without a mature RRS. There is also the possibility of misclassification of covariates and outcomes, but any misclassification would likely be nondifferential and bias toward the null. Matching was not possible based on exact diagnosis, and the unit is a good but imperfect proxy for diagnosis grouping. At our center, overflow of patients into the Cardiology and Hematology/Oncology units is uncommon, mitigating this partially, although residual confounding may remain. The finding that ETs are associated with adverse outcomes does not necessarily mean that these events were preventable; however, it is important and encouraging that the rate of ETs has been reduced at two centers using improvement science interventions.8,10
CONCLUSION
Patients who experienced an ET had a significantly higher likelihood of in-hospital mortality, spent more time in the ICU, and had a longer hospital LOS posttransfer than matched controls. The use of the ET metric in children’s hospitals would allow for further analysis of such patients in hopes of identifying clinical characteristics that serve as predictors of deterioration. This may facilitate better risk stratification in the clinical system as well as enable more rapid learning and systems improvements targeted toward preventing unrecognized clinical deterioration.
Disclosures
Dr. Hussain, Dr. Sosa, Dr. Ambroggio, and Mrs. Gallagher have nothing to disclose. Patrick Brady reports grants from the Agency for Healthcare Research and Quality, outside the submitted work. The authors certify that this submission is not under review by any other publication. The author team has no conflicts of interest to disclose.
Funding
Ms. Hussain was supported by the Society of Hospital Medicine’s Student Hospitalist Scholar Grant Program in 2017. Dr. Brady receives career development support from AHRQ K08-HS023827. The project described was supported by the National Center for Advancing Translational Sciences of the National Institutes of Health, under Award Number 5UL1TR001425-04. The content is solely the responsibility of the authors and does not necessarily represent the official views of the SHM, AHRQ, or NIH.
1. Schein RM, Hazday N, Pena M, Ruben BH, Sprung CL. Clinical antecedents to in-hospital cardiopulmonary arrest. Chest. 1990;98(6):1388-1392. https://doi.org/10.1378/chest.98.6.1388.
2. Maharaj R, Raffaele I, Wendon J. Rapid response systems: a systematic review and meta-analysis. Crit Care. 2015;19:254. https://doi.org/10.1186/s13054-015-0973-y.
3. Bonafide CP, Roland D, Brady PW. Rapid response systems 20 years later: new approaches, old challenges. JAMA Pediatrics. 2016;170(8):729-730. https://doi.org/10.1001/jamapediatrics.2016.0398.
4. Hayes LW, Dobyns EL, DiGiovine B, et al. A multicenter collaborative approach to reducing pediatric codes outside the ICU. Pediatrics. 2012;129(3):e785-e791. https://doi.org/10.1542/peds.2011-0227.
5. Raymond TT, Bonafide CP, Praestgaard A, et al. Pediatric medical emergency team events and outcomes: a report of 3647 events from the American Heart Association’s get with the guidelines-resuscitation registry. Hosp Pediatr. 2016;6(2):57-64. https://doi.org/10.1542/hpeds.2015-0132.
6. Bonafide CP, Roberts KE, Priestley MA, et al. Development of a pragmatic measure for evaluating and optimizing rapid response systems. Pediatrics. 2012;129(4):e874-e881. https://doi.org/10.1542/peds.2011-2784.
7. Brady PW, Goldenhar LM. A qualitative study examining the influences on situation awareness and the identification, mitigation and escalation of recognised patient risk. BMJ Qual Saf. 2014;23(2):153-161. https://doi.org/10.1136/bmjqs-2012-001747.
8. Brady PW, Muething S, Kotagal U, et al. Improving situation awareness to reduce unrecognized clinical deterioration and serious safety events. Pediatrics. 2013;131(1):e298-e308. https://doi.org/10.1542/peds.2012-1364.
9. Brady PW, Wheeler DS, Muething SE, Kotagal UR. Situation awareness: a new model for predicting and preventing patient deterioration. Hosp Pediatr. 2014;4(3):143-146. https://doi.org/10.1542/hpeds.2013-0119.
10. McClain Smith M, Chumpia M, Wargo L, Nicol J, Bugnitz M. Watcher initiative associated with decrease in failure to rescue events in pediatric population. Hosp Pediatr. 2017;7(12):710-715. https://doi.org/10.1542/hpeds.2017-0042.
11. McCrory MC, Spaeder MC, Gower EW, et al. Time of admission to the PICU and mortality. Pediatr Crit Care Med. 2017;18(10):915-923. https://doi.org/10.1097/PCC.0000000000001268.
12. Aoki Y, Inata Y, Hatachi T, Shimizu Y, Takeuchi M. Outcomes of ‘unrecognised situation awareness failures events’ in intensive care unit transfer of children in a Japanese children’s hospital. J Paediatr Child Health. 2018;55(2):213-215. https://doi.org/10.1111/jpc.14185.
Unrecognized in-hospital deterioration can result in tragic consequences for pediatric patients. The majority of deterioration events have antecedents such as increasingly abnormal vital signs and new concerns from nurses.1 Recent meta-analyses have shown that rapid response systems (RRSs), which include trigger mechanisms such as a pediatric early warning score (PEWS), are associated with a reduced rate of arrests and in-hospital mortality.2,3 Cardiopulmonary arrest rates are useful metrics to judge the effectiveness of the system to identify and respond to deteriorating adult patients; however, there are important challenges to their use as an outcome measure in pediatrics. Arrests, which have been relatively uncommon in pediatric patients, are now even less frequent since the adoption of a RRS in the majority of children’s hospitals.4,5 Several innovations in these systems will be context-dependent and hence best first evaluated in a single center, where arrests outside of the intensive care unit (ICU) may occur rarely. Identification of valid, more frequent proximal measures to arrests may better identify the risk factors for deterioration. This could potentially inform quality improvement efforts to mitigate clinical deterioration.
Bonafide et al. at the Children’s Hospital of Philadelphia developed and validated the critical deterioration event (CDE) metric, demonstrating that children who were transferred to the ICU and who received noninvasive ventilation, intubation, or vasopressor initiation within 12 hours of transfer had a >13-fold increased risk of in-hospital mortality.6 At Cincinnati Children’s Hospital Medical Center, an additional proximal outcome measure was developed for unrecognized clinical deterioration, now termed emergency transfers (ETs).7-9 An ET is defined as any patient transferred to the ICU where the patient received intubation, inotropes, or three or more fluid boluses in the first hour after arrival or before transfer.9 Improvement science work that aimed at increasing clinician situation awareness was associated with a reduction in ETs,8 but the association of ETs with mortality or other healthcare utilization outcomes is unknown. The objective of this study was to determine the predictive validity of an ET on in-hospital mortality, ICU length of stay (LOS), and overall hospital LOS.
METHODS
We conducted a case–control study at Cincinnati Children’s Hospital, a free-standing tertiary care children’s hospital. Our center has had an ICU-based RRS in place since 2005. In 2009, we eliminated the ICU consult such that each floor-to-ICU transfer is evaluated by the RRS. Nurses calculate a Monaghan PEWS every four hours on the majority of nursing units.
Patients of all ages cared for outside of the ICU at any point in their hospitalization from January 1, 2013, to July 31, 2017, were eligible for inclusion. There were no other exclusion criteria. The ICU included both the pediatric ICU and the cardiac ICU.
Cases
We identified all ET cases from an existing situation awareness database in which each RRS call is entered by the hospital nursing supervisor, whose role includes responding to each RRS activation. If the patient transfer meets the ET criteria, the nurse indicates this in the database. Each ET entry is later confirmed for assurance purposes by the nurse leader of the RRS committee (RG). For the purposes of this study, all records were again reviewed and validated using manual chart review in the electronic health record (Epic Systems, Verona, Wisconsin).
Controls
We identified nonemergent ICU transfers to serve as controls and matched those to ET in cases to limit the impact of confounders that may increase the likelihood of both an ET and a negative outcome such as ICU mortality. We identified up to three controls for each case from our database and matched in terms of age group (within five years of age), hospital unit before transfer, and time of year (within three months of ET). These variables were chosen to adjust for the impact of age, diversity of disease (as hospital units are generally organized by organ system of illness), and seasonality on outcomes.
Outcome Measures
Posttransfer LOS in the ICU, posttransfer hospital LOS, and in-hospital mortality were the primary outcome measures. Patient demographics, specific diagnoses, and number of medical conditions were a priori defined as covariates of interest. Data for each case and control were entered into a secure, web-based Research Electronic Data Capture (REDCap) database.
Analysis
Descriptive data were summarized using counts and percentages for categorical variables and medians and ranges for continuous variables due to nonnormal distributions. Chi-square test was used to compare in-hospital mortality between the ETs and the controls. The Wilcoxon rank-sum test was used to compare LOS between ETs and controls. All data analyses were performed using SAS version 9.4 (SAS Institute Inc., Cary, North Carolina).
RESULTS
A total of 45 ETs were identified, and 110 controls were matched. Patient demographics were similar among all cases and controls (P > .05). Patients with ETs had a median age of seven years (interquartile range: 3-18 years), and 51% of them were males. The majority of patients among our examined cases were white (68%) and non-Hispanic (93%). There was no statistical difference in insurance between the ETs and the controls. When evaluating the hospital unit before the transfer, ETs occurred most commonly in the Cardiology (22%), Hematology/Oncology (22%), and Neuroscience (16%) units.
ETs stayed longer in the ICU than non-ETs [median of 4.9 days vs 2.2 days, P = .001; Figure (A)]. Similarly, ET cases had a significantly longer posttransfer hospital LOS [median of 35 days vs 21 days, P = .001; Figure (B)]. ETs had a 22% in-hospital mortality rate, compared with 9% in-hospital mortality in the matched controls (P = .02; Table).
DISCUSSION
Children who experienced an ET had a significantly longer ICU LOS, a longer posttransfer LOS, and a higher in-hospital mortality than the matched controls who were also transferred to the ICU. Researchers and improvement science teams at multiple hospitals have demonstrated that interventions targeting improved situation awareness can reduce ETs; we have demonstrated that reducing ETs may reduce subsequent adverse outcomes.8,10
These findings provide additional support for the use of the ET metric in children’s hospitals as a proximal measure for significant clinical deterioration. We found mortality rates that were overall high for a children’s hospital (22% in ET cases and 9% among controls) compared with a national average mortality rate of 2.3% in pediatric ICUs.11 This is likely due to the study sample containing a significant proportion of children with medical complexity.
Aoki et al. recently demonstrated that ETs, compared with non-ETs, were associated with longer LOS and higher mortality in a bivariate analysis.12 In our study, we found similar results with the important addition that these findings were robust when ETs were compared with matched controls who were likely at a higher risk of poor outcomes than ICU transfers in general. In addition, we demonstrated that ETs were associated with adverse outcomes in a United States children’s hospital with a mature, long-standing RRS process. As ETs are considerably more common than cardiac and respiratory arrests, use of the ET metric in children’s hospitals may enable more rapid learning and systems improvement implementations. We also found that most of the children with ETs present from units that care for children with substantial medical complexity, including Cardiology, Hematology/Oncology, and Neurosciences. Future work should continue to examine the relationship between medical complexity and ET risk.
The ET metric is complementary to the CDE measure developed by Bonafide et al. Both metrics capture potential events of unrecognized clinical deterioration, and both offer researchers the opportunity to better understand and improve their RRSs. Both ETs and CDEs are more common than arrests, and CDEs are more common than ETs. ETs, which by definition occur in the first hour of ICU care, are likely a more specific measure of unrecognized clinical deterioration. CDEs will capture therapies that may have been started up to 12 hours after transfer and thus are possibly more sensitive to identify unrecognized clinical deterioration. However, CDEs also may encompass some patients who arrived at the ICU after prompt recognition and then had a subacute deterioration in the ICU.
The maturity of the RRS and the bandwidth of teams to collect data may inform which metric(s) are best for individual centers. As ETs are less common and likely more specific to unrecognized clinical deterioration, they might be the first tracked as a center improves its RRS through QI methods. Alternatively, CDEs may be a useful metric for centers where unrecognized clinical deterioration is less common or in research studies where this more common outcome would lead to more power to detect the effect of interventions to improve care.
Our study had several limitations. Data collection was confined to one tertiary care children’s hospital with a high burden of complex cardiac and oncology care. The results may not generalize well to children hospitalized in smaller or community hospitals or in hospitals without a mature RRS. There is also the possibility of misclassification of covariates and outcomes, but any misclassification would likely be nondifferential and bias toward the null. Matching was not possible based on exact diagnosis, and the unit is a good but imperfect proxy for diagnosis grouping. At our center, overflow of patients into the Cardiology and Hematology/Oncology units is uncommon, mitigating this partially, although residual confounding may remain. The finding that ETs are associated with adverse outcomes does not necessarily mean that these events were preventable; however, it is important and encouraging that the rate of ETs has been reduced at two centers using improvement science interventions.8,10
CONCLUSION
Patients who experienced an ET had a significantly higher likelihood of in-hospital mortality, spent more time in the ICU, and had a longer hospital LOS posttransfer than matched controls. The use of the ET metric in children’s hospitals would allow for further analysis of such patients in hopes of identifying clinical characteristics that serve as predictors of deterioration. This may facilitate better risk stratification in the clinical system as well as enable more rapid learning and systems improvements targeted toward preventing unrecognized clinical deterioration.
Disclosures
Dr. Hussain, Dr. Sosa, Dr. Ambroggio, and Mrs. Gallagher have nothing to disclose. Patrick Brady reports grants from the Agency for Healthcare Research and Quality, outside the submitted work. The authors certify that this submission is not under review by any other publication. The author team has no conflicts of interest to disclose.
Funding
Ms. Hussain was supported by the Society of Hospital Medicine’s Student Hospitalist Scholar Grant Program in 2017. Dr. Brady receives career development support from AHRQ K08-HS023827. The project described was supported by the National Center for Advancing Translational Sciences of the National Institutes of Health, under Award Number 5UL1TR001425-04. The content is solely the responsibility of the authors and does not necessarily represent the official views of the SHM, AHRQ, or NIH.
Unrecognized in-hospital deterioration can result in tragic consequences for pediatric patients. The majority of deterioration events have antecedents such as increasingly abnormal vital signs and new concerns from nurses.1 Recent meta-analyses have shown that rapid response systems (RRSs), which include trigger mechanisms such as a pediatric early warning score (PEWS), are associated with a reduced rate of arrests and in-hospital mortality.2,3 Cardiopulmonary arrest rates are useful metrics to judge the effectiveness of the system to identify and respond to deteriorating adult patients; however, there are important challenges to their use as an outcome measure in pediatrics. Arrests, which have been relatively uncommon in pediatric patients, are now even less frequent since the adoption of a RRS in the majority of children’s hospitals.4,5 Several innovations in these systems will be context-dependent and hence best first evaluated in a single center, where arrests outside of the intensive care unit (ICU) may occur rarely. Identification of valid, more frequent proximal measures to arrests may better identify the risk factors for deterioration. This could potentially inform quality improvement efforts to mitigate clinical deterioration.
Bonafide et al. at the Children’s Hospital of Philadelphia developed and validated the critical deterioration event (CDE) metric, demonstrating that children who were transferred to the ICU and who received noninvasive ventilation, intubation, or vasopressor initiation within 12 hours of transfer had a >13-fold increased risk of in-hospital mortality.6 At Cincinnati Children’s Hospital Medical Center, an additional proximal outcome measure was developed for unrecognized clinical deterioration, now termed emergency transfers (ETs).7-9 An ET is defined as any patient transferred to the ICU where the patient received intubation, inotropes, or three or more fluid boluses in the first hour after arrival or before transfer.9 Improvement science work that aimed at increasing clinician situation awareness was associated with a reduction in ETs,8 but the association of ETs with mortality or other healthcare utilization outcomes is unknown. The objective of this study was to determine the predictive validity of an ET on in-hospital mortality, ICU length of stay (LOS), and overall hospital LOS.
METHODS
We conducted a case–control study at Cincinnati Children’s Hospital, a free-standing tertiary care children’s hospital. Our center has had an ICU-based RRS in place since 2005. In 2009, we eliminated the ICU consult such that each floor-to-ICU transfer is evaluated by the RRS. Nurses calculate a Monaghan PEWS every four hours on the majority of nursing units.
Patients of all ages cared for outside of the ICU at any point in their hospitalization from January 1, 2013, to July 31, 2017, were eligible for inclusion. There were no other exclusion criteria. The ICU included both the pediatric ICU and the cardiac ICU.
Cases
We identified all ET cases from an existing situation awareness database in which each RRS call is entered by the hospital nursing supervisor, whose role includes responding to each RRS activation. If the patient transfer meets the ET criteria, the nurse indicates this in the database. Each ET entry is later confirmed for assurance purposes by the nurse leader of the RRS committee (RG). For the purposes of this study, all records were again reviewed and validated using manual chart review in the electronic health record (Epic Systems, Verona, Wisconsin).
Controls
We identified nonemergent ICU transfers to serve as controls and matched those to ET in cases to limit the impact of confounders that may increase the likelihood of both an ET and a negative outcome such as ICU mortality. We identified up to three controls for each case from our database and matched in terms of age group (within five years of age), hospital unit before transfer, and time of year (within three months of ET). These variables were chosen to adjust for the impact of age, diversity of disease (as hospital units are generally organized by organ system of illness), and seasonality on outcomes.
Outcome Measures
Posttransfer LOS in the ICU, posttransfer hospital LOS, and in-hospital mortality were the primary outcome measures. Patient demographics, specific diagnoses, and number of medical conditions were a priori defined as covariates of interest. Data for each case and control were entered into a secure, web-based Research Electronic Data Capture (REDCap) database.
Analysis
Descriptive data were summarized using counts and percentages for categorical variables and medians and ranges for continuous variables due to nonnormal distributions. Chi-square test was used to compare in-hospital mortality between the ETs and the controls. The Wilcoxon rank-sum test was used to compare LOS between ETs and controls. All data analyses were performed using SAS version 9.4 (SAS Institute Inc., Cary, North Carolina).
RESULTS
A total of 45 ETs were identified, and 110 controls were matched. Patient demographics were similar among all cases and controls (P > .05). Patients with ETs had a median age of seven years (interquartile range: 3-18 years), and 51% of them were males. The majority of patients among our examined cases were white (68%) and non-Hispanic (93%). There was no statistical difference in insurance between the ETs and the controls. When evaluating the hospital unit before the transfer, ETs occurred most commonly in the Cardiology (22%), Hematology/Oncology (22%), and Neuroscience (16%) units.
ETs stayed longer in the ICU than non-ETs [median of 4.9 days vs 2.2 days, P = .001; Figure (A)]. Similarly, ET cases had a significantly longer posttransfer hospital LOS [median of 35 days vs 21 days, P = .001; Figure (B)]. ETs had a 22% in-hospital mortality rate, compared with 9% in-hospital mortality in the matched controls (P = .02; Table).
DISCUSSION
Children who experienced an ET had a significantly longer ICU LOS, a longer posttransfer LOS, and a higher in-hospital mortality than the matched controls who were also transferred to the ICU. Researchers and improvement science teams at multiple hospitals have demonstrated that interventions targeting improved situation awareness can reduce ETs; we have demonstrated that reducing ETs may reduce subsequent adverse outcomes.8,10
These findings provide additional support for the use of the ET metric in children’s hospitals as a proximal measure for significant clinical deterioration. We found mortality rates that were overall high for a children’s hospital (22% in ET cases and 9% among controls) compared with a national average mortality rate of 2.3% in pediatric ICUs.11 This is likely due to the study sample containing a significant proportion of children with medical complexity.
Aoki et al. recently demonstrated that ETs, compared with non-ETs, were associated with longer LOS and higher mortality in a bivariate analysis.12 In our study, we found similar results with the important addition that these findings were robust when ETs were compared with matched controls who were likely at a higher risk of poor outcomes than ICU transfers in general. In addition, we demonstrated that ETs were associated with adverse outcomes in a United States children’s hospital with a mature, long-standing RRS process. As ETs are considerably more common than cardiac and respiratory arrests, use of the ET metric in children’s hospitals may enable more rapid learning and systems improvement implementations. We also found that most of the children with ETs present from units that care for children with substantial medical complexity, including Cardiology, Hematology/Oncology, and Neurosciences. Future work should continue to examine the relationship between medical complexity and ET risk.
The ET metric is complementary to the CDE measure developed by Bonafide et al. Both metrics capture potential events of unrecognized clinical deterioration, and both offer researchers the opportunity to better understand and improve their RRSs. Both ETs and CDEs are more common than arrests, and CDEs are more common than ETs. ETs, which by definition occur in the first hour of ICU care, are likely a more specific measure of unrecognized clinical deterioration. CDEs will capture therapies that may have been started up to 12 hours after transfer and thus are possibly more sensitive to identify unrecognized clinical deterioration. However, CDEs also may encompass some patients who arrived at the ICU after prompt recognition and then had a subacute deterioration in the ICU.
The maturity of the RRS and the bandwidth of teams to collect data may inform which metric(s) are best for individual centers. As ETs are less common and likely more specific to unrecognized clinical deterioration, they might be the first tracked as a center improves its RRS through QI methods. Alternatively, CDEs may be a useful metric for centers where unrecognized clinical deterioration is less common or in research studies where this more common outcome would lead to more power to detect the effect of interventions to improve care.
Our study had several limitations. Data collection was confined to one tertiary care children’s hospital with a high burden of complex cardiac and oncology care. The results may not generalize well to children hospitalized in smaller or community hospitals or in hospitals without a mature RRS. There is also the possibility of misclassification of covariates and outcomes, but any misclassification would likely be nondifferential and bias toward the null. Matching was not possible based on exact diagnosis, and the unit is a good but imperfect proxy for diagnosis grouping. At our center, overflow of patients into the Cardiology and Hematology/Oncology units is uncommon, mitigating this partially, although residual confounding may remain. The finding that ETs are associated with adverse outcomes does not necessarily mean that these events were preventable; however, it is important and encouraging that the rate of ETs has been reduced at two centers using improvement science interventions.8,10
CONCLUSION
Patients who experienced an ET had a significantly higher likelihood of in-hospital mortality, spent more time in the ICU, and had a longer hospital LOS posttransfer than matched controls. The use of the ET metric in children’s hospitals would allow for further analysis of such patients in hopes of identifying clinical characteristics that serve as predictors of deterioration. This may facilitate better risk stratification in the clinical system as well as enable more rapid learning and systems improvements targeted toward preventing unrecognized clinical deterioration.
Disclosures
Dr. Hussain, Dr. Sosa, Dr. Ambroggio, and Mrs. Gallagher have nothing to disclose. Patrick Brady reports grants from the Agency for Healthcare Research and Quality, outside the submitted work. The authors certify that this submission is not under review by any other publication. The author team has no conflicts of interest to disclose.
Funding
Ms. Hussain was supported by the Society of Hospital Medicine’s Student Hospitalist Scholar Grant Program in 2017. Dr. Brady receives career development support from AHRQ K08-HS023827. The project described was supported by the National Center for Advancing Translational Sciences of the National Institutes of Health, under Award Number 5UL1TR001425-04. The content is solely the responsibility of the authors and does not necessarily represent the official views of the SHM, AHRQ, or NIH.
1. Schein RM, Hazday N, Pena M, Ruben BH, Sprung CL. Clinical antecedents to in-hospital cardiopulmonary arrest. Chest. 1990;98(6):1388-1392. https://doi.org/10.1378/chest.98.6.1388.
2. Maharaj R, Raffaele I, Wendon J. Rapid response systems: a systematic review and meta-analysis. Crit Care. 2015;19:254. https://doi.org/10.1186/s13054-015-0973-y.
3. Bonafide CP, Roland D, Brady PW. Rapid response systems 20 years later: new approaches, old challenges. JAMA Pediatrics. 2016;170(8):729-730. https://doi.org/10.1001/jamapediatrics.2016.0398.
4. Hayes LW, Dobyns EL, DiGiovine B, et al. A multicenter collaborative approach to reducing pediatric codes outside the ICU. Pediatrics. 2012;129(3):e785-e791. https://doi.org/10.1542/peds.2011-0227.
5. Raymond TT, Bonafide CP, Praestgaard A, et al. Pediatric medical emergency team events and outcomes: a report of 3647 events from the American Heart Association’s get with the guidelines-resuscitation registry. Hosp Pediatr. 2016;6(2):57-64. https://doi.org/10.1542/hpeds.2015-0132.
6. Bonafide CP, Roberts KE, Priestley MA, et al. Development of a pragmatic measure for evaluating and optimizing rapid response systems. Pediatrics. 2012;129(4):e874-e881. https://doi.org/10.1542/peds.2011-2784.
7. Brady PW, Goldenhar LM. A qualitative study examining the influences on situation awareness and the identification, mitigation and escalation of recognised patient risk. BMJ Qual Saf. 2014;23(2):153-161. https://doi.org/10.1136/bmjqs-2012-001747.
8. Brady PW, Muething S, Kotagal U, et al. Improving situation awareness to reduce unrecognized clinical deterioration and serious safety events. Pediatrics. 2013;131(1):e298-e308. https://doi.org/10.1542/peds.2012-1364.
9. Brady PW, Wheeler DS, Muething SE, Kotagal UR. Situation awareness: a new model for predicting and preventing patient deterioration. Hosp Pediatr. 2014;4(3):143-146. https://doi.org/10.1542/hpeds.2013-0119.
10. McClain Smith M, Chumpia M, Wargo L, Nicol J, Bugnitz M. Watcher initiative associated with decrease in failure to rescue events in pediatric population. Hosp Pediatr. 2017;7(12):710-715. https://doi.org/10.1542/hpeds.2017-0042.
11. McCrory MC, Spaeder MC, Gower EW, et al. Time of admission to the PICU and mortality. Pediatr Crit Care Med. 2017;18(10):915-923. https://doi.org/10.1097/PCC.0000000000001268.
12. Aoki Y, Inata Y, Hatachi T, Shimizu Y, Takeuchi M. Outcomes of ‘unrecognised situation awareness failures events’ in intensive care unit transfer of children in a Japanese children’s hospital. J Paediatr Child Health. 2018;55(2):213-215. https://doi.org/10.1111/jpc.14185.
1. Schein RM, Hazday N, Pena M, Ruben BH, Sprung CL. Clinical antecedents to in-hospital cardiopulmonary arrest. Chest. 1990;98(6):1388-1392. https://doi.org/10.1378/chest.98.6.1388.
2. Maharaj R, Raffaele I, Wendon J. Rapid response systems: a systematic review and meta-analysis. Crit Care. 2015;19:254. https://doi.org/10.1186/s13054-015-0973-y.
3. Bonafide CP, Roland D, Brady PW. Rapid response systems 20 years later: new approaches, old challenges. JAMA Pediatrics. 2016;170(8):729-730. https://doi.org/10.1001/jamapediatrics.2016.0398.
4. Hayes LW, Dobyns EL, DiGiovine B, et al. A multicenter collaborative approach to reducing pediatric codes outside the ICU. Pediatrics. 2012;129(3):e785-e791. https://doi.org/10.1542/peds.2011-0227.
5. Raymond TT, Bonafide CP, Praestgaard A, et al. Pediatric medical emergency team events and outcomes: a report of 3647 events from the American Heart Association’s get with the guidelines-resuscitation registry. Hosp Pediatr. 2016;6(2):57-64. https://doi.org/10.1542/hpeds.2015-0132.
6. Bonafide CP, Roberts KE, Priestley MA, et al. Development of a pragmatic measure for evaluating and optimizing rapid response systems. Pediatrics. 2012;129(4):e874-e881. https://doi.org/10.1542/peds.2011-2784.
7. Brady PW, Goldenhar LM. A qualitative study examining the influences on situation awareness and the identification, mitigation and escalation of recognised patient risk. BMJ Qual Saf. 2014;23(2):153-161. https://doi.org/10.1136/bmjqs-2012-001747.
8. Brady PW, Muething S, Kotagal U, et al. Improving situation awareness to reduce unrecognized clinical deterioration and serious safety events. Pediatrics. 2013;131(1):e298-e308. https://doi.org/10.1542/peds.2012-1364.
9. Brady PW, Wheeler DS, Muething SE, Kotagal UR. Situation awareness: a new model for predicting and preventing patient deterioration. Hosp Pediatr. 2014;4(3):143-146. https://doi.org/10.1542/hpeds.2013-0119.
10. McClain Smith M, Chumpia M, Wargo L, Nicol J, Bugnitz M. Watcher initiative associated with decrease in failure to rescue events in pediatric population. Hosp Pediatr. 2017;7(12):710-715. https://doi.org/10.1542/hpeds.2017-0042.
11. McCrory MC, Spaeder MC, Gower EW, et al. Time of admission to the PICU and mortality. Pediatr Crit Care Med. 2017;18(10):915-923. https://doi.org/10.1097/PCC.0000000000001268.
12. Aoki Y, Inata Y, Hatachi T, Shimizu Y, Takeuchi M. Outcomes of ‘unrecognised situation awareness failures events’ in intensive care unit transfer of children in a Japanese children’s hospital. J Paediatr Child Health. 2018;55(2):213-215. https://doi.org/10.1111/jpc.14185.
© 2019 Society of Hospital Medicine