User login
Treatment Trends and Outcomes in Healthcare-Associated Pneumonia
Bacterial pneumonia remains an important cause of morbidity and mortality in the United States, and is the 8th leading cause of death with 55,227 deaths among adults annually.1 In 2005, the American Thoracic Society (ATS) and the Infectious Diseases Society of America (IDSA) collaborated to update guidelines for hospital-acquired pneumonia (HAP), ventilator-associated pneumonia, and healthcare-associated pneumonia (HCAP).2 This broad document outlines an evidence-based approach to diagnostic testing and antibiotic management based on the epidemiology and risk factors for these conditions. The guideline specifies the following criteria for HCAP: hospitalization in the past 90 days, residence in a skilled nursing facility (SNF), home infusion therapy, hemodialysis, home wound care, family members with multidrug resistant organisms (MDRO), and immunosuppressive diseases or medications, with the presumption that these patients are more likely to be harboring MDRO and should thus be treated empirically with broad-spectrum antibiotic therapy. Prior studies have shown that patients with HCAP have a more severe illness, are more likely to have MDRO, are more likely to be inadequately treated, and are at a higher risk for mortality than patients with community-acquired pneumonia (CAP).3,4
These guidelines are controversial, especially in regard to the recommendations to empirically treat broadly with 2 antibiotics targeting Pseudomonas species, whether patients with HCAP merit broader spectrum coverage than patients with CAP, and whether the criteria for defining HCAP are adequate to predict which patients are harboring MDRO. It has subsequently been proposed that HCAP is more related to CAP than to HAP, and a recent update to the guideline removed recommendations for treatment of HCAP and will be placing HCAP into the guidelines for CAP instead.5 We sought to investigate the degree of uptake of the ATS and IDSA guideline recommendations by physicians over time, and whether this led to a change in outcomes among patients who met the criteria for HCAP.
METHODS
Setting and Patients
We identified patients discharged between July 1, 2007, and November 30, 2011, from 488 US hospitals that participated in the Premier database (Premier Inc., Charlotte, North Carolina), an inpatient database developed for measuring quality and healthcare utilization. The database is frequently used for healthcare research and has been described previously.6 Member hospitals are in all regions of the US and are generally reflective of US hospitals. This database contains multiple data elements, including sociodemographic information, International Classification of Diseases, 9th Revision-Clinical Modification (ICD-9-CM) diagnosis and procedure codes, hospital and physician information, source of admission, and discharge status. It also includes a date-stamped log of all billed items and services, including diagnostic tests, medications, and other treatments. Because the data do not contain identifiable information, the institutional review board at our medical center determined that this study did not constitute human subjects research.
We included all patients aged ≥18 years with a principal diagnosis of pneumonia or with a secondary diagnosis of pneumonia paired with a principal diagnosis of respiratory failure, acute respiratory distress syndrome, respiratory arrest, sepsis, or influenza. Patients were excluded if they were transferred to or from another acute care institution, had a length of stay of 1 day or less, had cystic fibrosis, did not have a chest radiograph, or did not receive antibiotics within 48 hours of admission.
For each patient, we extracted age, gender, principal diagnosis, comorbidities, and the specialty of the attending physician. Comorbidities were identified from ICD-9-CM secondary diagnosis codes and Diagnosis Related Groups by using Healthcare Cost and Utilization Project Comorbidity Software, version 3.1, based on the work of Elixhauser (Agency for Healthcare Research and Quality, Rockville, Maryland).7 In order to ensure that patients had HCAP, we required the presence of ≥1 HCAP criteria, including hospitalization in the past 90 days, hemodialysis, admission from an SNF, or immune suppression (which was derived from either a secondary diagnosis for neutropenia, hematological malignancy, organ transplant, acquired immunodeficiency virus, or receiving immunosuppressant drugs or corticosteroids [equivalent to ≥20 mg/day of prednisone]).
Definitions of Guideline-Concordant and Discordant Antibiotic Therapy
The ATS and IDSA guidelines recommended the following antibiotic combinations for HCAP: an antipseudomonal cephalosporin or carbapenem or a beta-lactam/lactamase inhibitor, plus an antipseudomonal quinolone or aminoglycoside, plus an antibiotic with activity versus methicillin resistant Staphylococcus aureus (MRSA), such as vancomycin or linezolid. Based on these guidelines, we defined the receipt of fully guideline-concordant antibiotics as 2 recommended antibiotics for Pseudomonas species plus 1 for MRSA administered by the second day of admission. Partially guideline-concordant antibiotics were defined as 1 recommended antibiotic for Pseudomonas species plus 1 for MRSA by the second day of hospitalization. Guideline-discordant antibiotics were defined as all other combinations.
Statistical Analysis
Descriptive statistics on patient characteristics are presented as frequency, proportions for categorical factors, and median with interquartile range (IQR) for continuous variables for the full cohort and by treatment group, defined as fully or partially guideline-concordant antibiotic therapy or discordant therapy. Hospital rates of fully guideline-concordant treatment are presented overall and by hospital characteristics. The association of hospital characteristics with rates of fully guideline-concordant therapy were assessed by using 1-way analysis of variance tests.
To assess trends across hospitals for the association between the use of guideline-concordant therapy and mortality, progression to respiratory failure as measured by the late initiation of invasive mechanical ventilation (day 3 or later), and the length of stay among survivors, we divided the 4.5-year study period into 9 intervals of 6 months each; 292 hospitals that submitted data for all 9 time points were examined in this analysis. Based on the distribution of length of stay in the first time period, we created an indicator variable for extended length of stay with length of stay at or above the 75th percentile, defined as extended. For each hospital at each 6-month interval, we then computed risk-standardized guideline-concordant treatment (RS-treatment) rates and risk-standardized in-hospital outcome rates similar to methods used by the Centers for Medicare and Medicaid Services for public reporting.8 For each hospital at each time interval, we estimated a predicted rate of guideline-concordant treatment as the sum of predicted probabilities of guideline-concordant treatment from patient factors and the random intercept for the hospital in which they were admitted. We then calculated the expected rate of guideline-concordant treatment as the sum of expected probabilities of treatment received from patient factors only. RS-treatment was then calculated as the ratio of predicted to expected rates multiplied by the overall unadjusted mean treatment rate from all patients.9 We repeated the same modeling strategy to calculate risk-standardized outcome (RS-outcome) rates for each hospital across all time points. All models were adjusted for patient demographics and comorbidities. Similar models using administrative data have moderate discrimination for mortality.10
We then fit mixed-effects linear models with random hospital intercept and slope across time for the RS-treatment and outcome rates, respectively. From these models, we estimated the mean slope for RS-treatment and for RS-outcome over time. In addition, we estimated a slope or trend over time for each hospital for treatment and for outcome and evaluated the correlation between the treatment and outcome trends.
All analyses were performed using the Statistical Analysis System version 9.4 (SAS Institute Inc., Cary, NC) and STATA release 13 (StataCorp, LLC, College Station, Texas).
RESULTS
DISCUSSION
In this large, retrospective cohort study, we found that there was a substantial gap between the empiric antibiotics recommended by the ATS and IDSA guidelines and the empiric antibiotics that patients actually received. Over the study period, we saw an increased adherence to guidelines, in spite of growing evidence that HCAP risk factors do not adequately predict which patients are at risk for infection with an MDRO.11 We used this change in antibiotic prescribing behavior over time to determine if there was a clinical impact on patient outcomes and found that at the hospital level, there were no improvements in mortality, excess length of stay, or progression to respiratory failure despite a doubling in guideline-concordant antibiotic use.
At least 2 other large studies have assessed the association between guideline-concordant therapy and outcomes in HCAP.12,13 Both found that guideline-concordant therapy was associated with increased mortality, despite propensity matching. Both were conducted at the individual patient level by using administrative data, and results were likely affected by unmeasured clinical confounders, with sicker patients being more likely to receive guideline-concordant therapy. Our focus on the outcomes at the hospital level avoids this selection bias because the overall severity of illness of patients at any given hospital would not be expected to change over the study period, while physician uptake of antibiotic prescribing guidelines would be expected to increase over time. Determining the correlation between increases in guideline adherence and changes in patient outcome may offer a better assessment of the impact of guideline adherence. In this regard, our results are similar to those achieved by 1 quality improvement collaborative that was aimed at increasing guideline concordant therapy in ICUs. Despite an increase in guideline concordance from 33% to 47% of patients, they found no change in overall mortality.14
There were several limitations to our study. We did not have access to microbiologic data, so we were unable to determine which patients had MDRO infection or determine antibiotic-pathogen matching. However, the treating physicians in our study population presumably did not have access to this data at the time of treatment either because the time period we examined was within the first 48 hours of hospitalization, the interval during which cultures are incubating and the patients are being treated empirically. In addition, there may have been HCAP patients that we failed to identify, such as patients who were admitted in the past 90 days to a hospital that does not submit data to Premier. However, it is unlikely that prescribing for such patients should differ systematically from what we observed. While the database draws from 488 hospitals nationwide, it is possible that practices may be different at facilities that are not contained within the Premier database, such as Veterans Administration Hospitals. Similarly, we did not have readings for chest x-rays; hence, there could be some patients in the dataset who did not have pneumonia. However, we tried to overcome this by including only those patients with a principal diagnosis of pneumonia or sepsis with a secondary pneumonia diagnosis, a chest x-ray, and antibiotics administered within the first 48 hours of admission.
There are likely several reasons why so few HCAP patients in our study received guideline-concordant antibiotics. A lack of knowledge about the ATS and IDSA guidelines may have impacted the physicians in our study population. El-Solh et al.15 surveyed physicians about the ATS-IDSA guidelines 4 years after publication and found that only 45% were familiar with the document. We found that the rate of prescribing at least partially guideline-concordant antibiotics rose steadily over time, supporting the idea that the newness of the guidelines was 1 barrier. Additionally, prior studies have shown that many physicians may not agree with or choose to follow guidelines, with only 20% of physicians indicating that guidelines have a major impact on their clinical decision making,16 and the majority do not choose HCAP guideline-concordant antibiotics when tested.17 Alternatively, clinicians may not follow the guidelines because of a belief that the HCAP criteria do not adequately indicate patients who are at risk for MDRO. Previous studies have demonstrated the relative inability of HCAP risk factors to predict patients who harbor MDRO18 and suggest that better tools such as clinical scoring systems, which include not only the traditional HCAP risk factors but also prior exposure to antibiotics, prior culture data, and a cumulative assessment of both intrinsic and extrinsic factors, could more accurately predict MDRO and lead to a more judicious use of broad-spectrum antimicrobial agents.19-25 Indeed, these collective findings have led the authors of the recently updated guidelines to remove HCAP as a clinical entity from the hospital-acquired or ventilator-associated pneumonia guidelines and place them instead in the upcoming updated guidelines on the management of CAP.5 Of these 3 explanations, the lack of familiarity fits best with our observation that guideline-concordant therapy increased steadily over time with no evidence of reaching a plateau. Ironically, as consensus was building that HCAP is a poor marker for MDROs, routine empiric treatment with vancomycin and piperacillin-tazobactam (“vanco and zosyn”) have become routine in many hospitals. Additional studies are needed to know if this trend has stabilized or reversed.
CONCLUSIONS
In conclusion, clinicians in our large, nationally representative sample treated the majority of HCAP patients as though they had CAP. Although there was an increase in the administration of guideline-concordant therapy over time, this increase was not associated with improved outcomes. This study supports the growing consensus that HCAP criteria do not accurately predict which patients benefit from broad-spectrum antibiotics for pneumonia, and most patients fare well with antibiotics targeting common community-acquired organisms.
Disclosure
This work was supported by grant # R01HS018723 from the Agency for Healthcare Research and Quality. Dr. Lagu is also supported by the National Heart, Lung, and Blood Institute of the National Institutes of Health under award number K01HL114745. Dr. Lindenauer is supported by grant K24HL132008 from the National Heart, Lung, and Blood Institute. The funding agency had no role in the data acquisition, analysis, or manuscript preparation for this study. Drs. Haessler and Rothberg had full access to all the data in the study and take responsibility for the integrity of the data and the accuracy of the data analysis. Drs. Haessler, Lagu, Lindenauer, Skiest, Zilberberg, Higgins, and Rothberg conceived of the study and analyzed and interpreted the data. Dr. Lindenauer acquired the data. Dr. Pekow and Ms. Priya carried out the statistical analyses. Dr. Haessler drafted the manuscript. All authors critically reviewed the manuscript for accuracy and integrity. All authors certify no potential conflicts of interest. Preliminary results from this study were presented in oral and poster format at IDWeek in 2012 and 2013.
1. Kochanek KD, Murphy SL, Xu JQ, Tejada-Vera B. Deaths: Final data for 2014. National vital statistics reports; vol 65 no 4. Hyattsville, MD: National Center for Health Statistics. 2016. PubMed
2. American Thoracic Society, Infectious Diseases Society of America. Guidelines for the Management of Adults with Hospital-acquired, Ventilator-associated, and Healthcare-associated Pneumonia. Am J Respir Crit Care Med. 2005;171(4):388-416. PubMed
3. Zilberberg MD, Shorr A. Healthcare-associated pneumonia: the state of the evidence to date. Curr Opin Pulm Med. 2011;17(3):142-147. PubMed
4. Kollef MK, Shorr A, Tabak YP, Gupta V, Liu LZ, Johannes RS. Epidemiology and Outcomes of Health-care-associated pneumonia. Chest. 2005;128(6):3854-3862. PubMed
5. Kalil AC, Metersky ML, Klompas M, et al. Management of Adults With Hospital-acquired and Ventilator-associated Pneumonia: 2016 Clinical Practice Guidelines by the Infectious Diseases Society of America and the American Thoracic Society. Clin Infect Dis. 2016;63(5):575-582. PubMed
6. Lindenauer PK, Pekow PS, Lahti MC, Lee Y, Benjamin EM, Rothberg MB. Association of corticosteroid dose and route of administration with risk of treatment failure in acute exacerbation of chronic obstructive pulmonary disease. JAMA. 2010;303(23):2359-2367. PubMed
7. Elixhauser A, Steiner C, Harris DR, Coffey RM. Comorbidity measures for use with administrative data. Med Care. 1998;36(1):8-27. PubMed
8. Centers for Medicare & Medicaid Services. Frequently asked questions (FAQs): Implementation and maintenance of CMS mortality measures for AMI & HF. 2007. https://www.cms.gov/Medicare/Quality-Initiatives-Patient-Assessment-Instruments/HospitalQualityInits/downloads/HospitalMortalityAboutAMI_HF.pdf. Accessed November 1, 2016.
9. Normand SL, Shahian DM. Statistical and Clinical Aspects of Hospital Outcomes Profiling. Stat Sci. 2007;22(2):206-226.
10. Rothberg MB, Pekow PS, Priya A, et al. Using highly detailed administrative data to predict pneumonia mortality. PLoS One. 2014;9(1):e87382. PubMed
11. Jones BE, Jones MM, Huttner B, et al. Trends in antibiotic use and nosocomial pathogens in hospitalized veterans with pneumonia at 128 medical centers, 2006-2010. Clin Infect Dis. 2015;61(9):1403-1410. PubMed
12. Attridge RT, Frei CR, Restrepo MI, et al. Guideline-concordant therapy and outcomes in healthcare-associated pneumonia. Eur Respir J. 2011;38(4):878-887. PubMed
13. Rothberg MB, Zilberberg MD, Pekow PS, et al. Association of Guideline-based Antimicrobial Therapy and Outcomes in Healthcare-Associated Pneumonia. J Antimicrob Chemother. 2015;70(5):1573-1579. PubMed
14. Kett DH, Cano E, Quartin AA, et al. Improving Medicine through Pathway Assessment of Critical Therapy of Hospital-Acquired Pneumonia (IMPACT-HAP) Investigators. Implementation of guidelines for management of possible multidrug-resistant pneumonia in intensive care: an observational, multicentre cohort study. Lancet Infect Dis. 2011;11(3):181-189. PubMed
15. El-Solh AA, Alhajhusain A, Saliba RG, Drinka P. Physicians’ Attitudes Toward Guidelines for the Treatment of Hospitalized Nursing-Home -Acquired Pneumonia. J Am Med Dir Assoc. 2011;12(4):270-276. PubMed
16. Tunis S, Hayward R, Wilson M, et al. Internists’ Attitudes about Clinical Practice Guidelines. Ann Intern Med. 1994;120(11):956-963. PubMed
17. Seymann GB, Di Francesco L, Sharpe B, et al. The HCAP Gap: Differences between Self-Reported Practice Patterns and Published Guidelines for Health Care-Associated Pneumonia. Clin Infect Dis. 2009;49(12):1868-1874. PubMed
18. Chalmers JD, Rother C, Salih W, Ewig S. Healthcare associated pneumonia does not accurately identify potentially resistant pathogens: a systematic review and meta-analysis. Clin Infect Dis. 2014;58(3):330-339. PubMed
19. Shorr A, Zilberberg MD, Reichley R, et al. Validation of a Clinical Score for Assessing the Risk of Resistant Pathogens in Patients with Pneumonia Presenting to the Emergency Department. Clin Infect Dis. 2012;54(2):193-198. PubMed
20. Aliberti S, Pasquale MD, Zanaboni AM, et al. Stratifying Risk Factors for Multidrug-Resistant Pathogens in Hospitalized Patients Coming from the Community with Pneumonia. Clin Infect Dis. 2012;54(4):470-478. PubMed
21. Schreiber MP, Chan CM, Shorr AF. Resistant Pathogens in Nonnosocomial Pneumonia and Respiratory Failure: Is it Time to Refine the Definition of Health-care-Associated Pneumonia? Chest. 2010;137(6):1283-1288. PubMed
22. Madaras-Kelly KJ, Remington RE, Fan VS, Sloan KL. Predicting antibiotic resistance to community-acquired pneumonia antibiotics in culture-positive patients with healthcare-associated pneumonia. J Hosp Med. 2012;7(3):195-202. PubMed
23. Shindo Y, Ito R, Kobayashi D, et al. Risk factors for drug-resistant pathogens in community-acquired and healthcare-associated pneumonia. Am J Respir Crit Care Med. 2013;188(8):985-995. PubMed
24. Metersky ML, Frei CR, Mortensen EM. Predictors of Pseudomonas and methicillin-resistant Staphylococcus aureus in hospitalized patients with healthcare-associated pneumonia. Respirology. 2016;21(1):157-163. PubMed
25. Webb BJ, Dascomb K, Stenehjem E, Dean N. Predicting risk of drug-resistant organisms in pneumonia: moving beyond the HCAP model. Respir Med. 2015;109(1):1-10. PubMed
Bacterial pneumonia remains an important cause of morbidity and mortality in the United States, and is the 8th leading cause of death with 55,227 deaths among adults annually.1 In 2005, the American Thoracic Society (ATS) and the Infectious Diseases Society of America (IDSA) collaborated to update guidelines for hospital-acquired pneumonia (HAP), ventilator-associated pneumonia, and healthcare-associated pneumonia (HCAP).2 This broad document outlines an evidence-based approach to diagnostic testing and antibiotic management based on the epidemiology and risk factors for these conditions. The guideline specifies the following criteria for HCAP: hospitalization in the past 90 days, residence in a skilled nursing facility (SNF), home infusion therapy, hemodialysis, home wound care, family members with multidrug resistant organisms (MDRO), and immunosuppressive diseases or medications, with the presumption that these patients are more likely to be harboring MDRO and should thus be treated empirically with broad-spectrum antibiotic therapy. Prior studies have shown that patients with HCAP have a more severe illness, are more likely to have MDRO, are more likely to be inadequately treated, and are at a higher risk for mortality than patients with community-acquired pneumonia (CAP).3,4
These guidelines are controversial, especially in regard to the recommendations to empirically treat broadly with 2 antibiotics targeting Pseudomonas species, whether patients with HCAP merit broader spectrum coverage than patients with CAP, and whether the criteria for defining HCAP are adequate to predict which patients are harboring MDRO. It has subsequently been proposed that HCAP is more related to CAP than to HAP, and a recent update to the guideline removed recommendations for treatment of HCAP and will be placing HCAP into the guidelines for CAP instead.5 We sought to investigate the degree of uptake of the ATS and IDSA guideline recommendations by physicians over time, and whether this led to a change in outcomes among patients who met the criteria for HCAP.
METHODS
Setting and Patients
We identified patients discharged between July 1, 2007, and November 30, 2011, from 488 US hospitals that participated in the Premier database (Premier Inc., Charlotte, North Carolina), an inpatient database developed for measuring quality and healthcare utilization. The database is frequently used for healthcare research and has been described previously.6 Member hospitals are in all regions of the US and are generally reflective of US hospitals. This database contains multiple data elements, including sociodemographic information, International Classification of Diseases, 9th Revision-Clinical Modification (ICD-9-CM) diagnosis and procedure codes, hospital and physician information, source of admission, and discharge status. It also includes a date-stamped log of all billed items and services, including diagnostic tests, medications, and other treatments. Because the data do not contain identifiable information, the institutional review board at our medical center determined that this study did not constitute human subjects research.
We included all patients aged ≥18 years with a principal diagnosis of pneumonia or with a secondary diagnosis of pneumonia paired with a principal diagnosis of respiratory failure, acute respiratory distress syndrome, respiratory arrest, sepsis, or influenza. Patients were excluded if they were transferred to or from another acute care institution, had a length of stay of 1 day or less, had cystic fibrosis, did not have a chest radiograph, or did not receive antibiotics within 48 hours of admission.
For each patient, we extracted age, gender, principal diagnosis, comorbidities, and the specialty of the attending physician. Comorbidities were identified from ICD-9-CM secondary diagnosis codes and Diagnosis Related Groups by using Healthcare Cost and Utilization Project Comorbidity Software, version 3.1, based on the work of Elixhauser (Agency for Healthcare Research and Quality, Rockville, Maryland).7 In order to ensure that patients had HCAP, we required the presence of ≥1 HCAP criteria, including hospitalization in the past 90 days, hemodialysis, admission from an SNF, or immune suppression (which was derived from either a secondary diagnosis for neutropenia, hematological malignancy, organ transplant, acquired immunodeficiency virus, or receiving immunosuppressant drugs or corticosteroids [equivalent to ≥20 mg/day of prednisone]).
Definitions of Guideline-Concordant and Discordant Antibiotic Therapy
The ATS and IDSA guidelines recommended the following antibiotic combinations for HCAP: an antipseudomonal cephalosporin or carbapenem or a beta-lactam/lactamase inhibitor, plus an antipseudomonal quinolone or aminoglycoside, plus an antibiotic with activity versus methicillin resistant Staphylococcus aureus (MRSA), such as vancomycin or linezolid. Based on these guidelines, we defined the receipt of fully guideline-concordant antibiotics as 2 recommended antibiotics for Pseudomonas species plus 1 for MRSA administered by the second day of admission. Partially guideline-concordant antibiotics were defined as 1 recommended antibiotic for Pseudomonas species plus 1 for MRSA by the second day of hospitalization. Guideline-discordant antibiotics were defined as all other combinations.
Statistical Analysis
Descriptive statistics on patient characteristics are presented as frequency, proportions for categorical factors, and median with interquartile range (IQR) for continuous variables for the full cohort and by treatment group, defined as fully or partially guideline-concordant antibiotic therapy or discordant therapy. Hospital rates of fully guideline-concordant treatment are presented overall and by hospital characteristics. The association of hospital characteristics with rates of fully guideline-concordant therapy were assessed by using 1-way analysis of variance tests.
To assess trends across hospitals for the association between the use of guideline-concordant therapy and mortality, progression to respiratory failure as measured by the late initiation of invasive mechanical ventilation (day 3 or later), and the length of stay among survivors, we divided the 4.5-year study period into 9 intervals of 6 months each; 292 hospitals that submitted data for all 9 time points were examined in this analysis. Based on the distribution of length of stay in the first time period, we created an indicator variable for extended length of stay with length of stay at or above the 75th percentile, defined as extended. For each hospital at each 6-month interval, we then computed risk-standardized guideline-concordant treatment (RS-treatment) rates and risk-standardized in-hospital outcome rates similar to methods used by the Centers for Medicare and Medicaid Services for public reporting.8 For each hospital at each time interval, we estimated a predicted rate of guideline-concordant treatment as the sum of predicted probabilities of guideline-concordant treatment from patient factors and the random intercept for the hospital in which they were admitted. We then calculated the expected rate of guideline-concordant treatment as the sum of expected probabilities of treatment received from patient factors only. RS-treatment was then calculated as the ratio of predicted to expected rates multiplied by the overall unadjusted mean treatment rate from all patients.9 We repeated the same modeling strategy to calculate risk-standardized outcome (RS-outcome) rates for each hospital across all time points. All models were adjusted for patient demographics and comorbidities. Similar models using administrative data have moderate discrimination for mortality.10
We then fit mixed-effects linear models with random hospital intercept and slope across time for the RS-treatment and outcome rates, respectively. From these models, we estimated the mean slope for RS-treatment and for RS-outcome over time. In addition, we estimated a slope or trend over time for each hospital for treatment and for outcome and evaluated the correlation between the treatment and outcome trends.
All analyses were performed using the Statistical Analysis System version 9.4 (SAS Institute Inc., Cary, NC) and STATA release 13 (StataCorp, LLC, College Station, Texas).
RESULTS
DISCUSSION
In this large, retrospective cohort study, we found that there was a substantial gap between the empiric antibiotics recommended by the ATS and IDSA guidelines and the empiric antibiotics that patients actually received. Over the study period, we saw an increased adherence to guidelines, in spite of growing evidence that HCAP risk factors do not adequately predict which patients are at risk for infection with an MDRO.11 We used this change in antibiotic prescribing behavior over time to determine if there was a clinical impact on patient outcomes and found that at the hospital level, there were no improvements in mortality, excess length of stay, or progression to respiratory failure despite a doubling in guideline-concordant antibiotic use.
At least 2 other large studies have assessed the association between guideline-concordant therapy and outcomes in HCAP.12,13 Both found that guideline-concordant therapy was associated with increased mortality, despite propensity matching. Both were conducted at the individual patient level by using administrative data, and results were likely affected by unmeasured clinical confounders, with sicker patients being more likely to receive guideline-concordant therapy. Our focus on the outcomes at the hospital level avoids this selection bias because the overall severity of illness of patients at any given hospital would not be expected to change over the study period, while physician uptake of antibiotic prescribing guidelines would be expected to increase over time. Determining the correlation between increases in guideline adherence and changes in patient outcome may offer a better assessment of the impact of guideline adherence. In this regard, our results are similar to those achieved by 1 quality improvement collaborative that was aimed at increasing guideline concordant therapy in ICUs. Despite an increase in guideline concordance from 33% to 47% of patients, they found no change in overall mortality.14
There were several limitations to our study. We did not have access to microbiologic data, so we were unable to determine which patients had MDRO infection or determine antibiotic-pathogen matching. However, the treating physicians in our study population presumably did not have access to this data at the time of treatment either because the time period we examined was within the first 48 hours of hospitalization, the interval during which cultures are incubating and the patients are being treated empirically. In addition, there may have been HCAP patients that we failed to identify, such as patients who were admitted in the past 90 days to a hospital that does not submit data to Premier. However, it is unlikely that prescribing for such patients should differ systematically from what we observed. While the database draws from 488 hospitals nationwide, it is possible that practices may be different at facilities that are not contained within the Premier database, such as Veterans Administration Hospitals. Similarly, we did not have readings for chest x-rays; hence, there could be some patients in the dataset who did not have pneumonia. However, we tried to overcome this by including only those patients with a principal diagnosis of pneumonia or sepsis with a secondary pneumonia diagnosis, a chest x-ray, and antibiotics administered within the first 48 hours of admission.
There are likely several reasons why so few HCAP patients in our study received guideline-concordant antibiotics. A lack of knowledge about the ATS and IDSA guidelines may have impacted the physicians in our study population. El-Solh et al.15 surveyed physicians about the ATS-IDSA guidelines 4 years after publication and found that only 45% were familiar with the document. We found that the rate of prescribing at least partially guideline-concordant antibiotics rose steadily over time, supporting the idea that the newness of the guidelines was 1 barrier. Additionally, prior studies have shown that many physicians may not agree with or choose to follow guidelines, with only 20% of physicians indicating that guidelines have a major impact on their clinical decision making,16 and the majority do not choose HCAP guideline-concordant antibiotics when tested.17 Alternatively, clinicians may not follow the guidelines because of a belief that the HCAP criteria do not adequately indicate patients who are at risk for MDRO. Previous studies have demonstrated the relative inability of HCAP risk factors to predict patients who harbor MDRO18 and suggest that better tools such as clinical scoring systems, which include not only the traditional HCAP risk factors but also prior exposure to antibiotics, prior culture data, and a cumulative assessment of both intrinsic and extrinsic factors, could more accurately predict MDRO and lead to a more judicious use of broad-spectrum antimicrobial agents.19-25 Indeed, these collective findings have led the authors of the recently updated guidelines to remove HCAP as a clinical entity from the hospital-acquired or ventilator-associated pneumonia guidelines and place them instead in the upcoming updated guidelines on the management of CAP.5 Of these 3 explanations, the lack of familiarity fits best with our observation that guideline-concordant therapy increased steadily over time with no evidence of reaching a plateau. Ironically, as consensus was building that HCAP is a poor marker for MDROs, routine empiric treatment with vancomycin and piperacillin-tazobactam (“vanco and zosyn”) have become routine in many hospitals. Additional studies are needed to know if this trend has stabilized or reversed.
CONCLUSIONS
In conclusion, clinicians in our large, nationally representative sample treated the majority of HCAP patients as though they had CAP. Although there was an increase in the administration of guideline-concordant therapy over time, this increase was not associated with improved outcomes. This study supports the growing consensus that HCAP criteria do not accurately predict which patients benefit from broad-spectrum antibiotics for pneumonia, and most patients fare well with antibiotics targeting common community-acquired organisms.
Disclosure
This work was supported by grant # R01HS018723 from the Agency for Healthcare Research and Quality. Dr. Lagu is also supported by the National Heart, Lung, and Blood Institute of the National Institutes of Health under award number K01HL114745. Dr. Lindenauer is supported by grant K24HL132008 from the National Heart, Lung, and Blood Institute. The funding agency had no role in the data acquisition, analysis, or manuscript preparation for this study. Drs. Haessler and Rothberg had full access to all the data in the study and take responsibility for the integrity of the data and the accuracy of the data analysis. Drs. Haessler, Lagu, Lindenauer, Skiest, Zilberberg, Higgins, and Rothberg conceived of the study and analyzed and interpreted the data. Dr. Lindenauer acquired the data. Dr. Pekow and Ms. Priya carried out the statistical analyses. Dr. Haessler drafted the manuscript. All authors critically reviewed the manuscript for accuracy and integrity. All authors certify no potential conflicts of interest. Preliminary results from this study were presented in oral and poster format at IDWeek in 2012 and 2013.
Bacterial pneumonia remains an important cause of morbidity and mortality in the United States, and is the 8th leading cause of death with 55,227 deaths among adults annually.1 In 2005, the American Thoracic Society (ATS) and the Infectious Diseases Society of America (IDSA) collaborated to update guidelines for hospital-acquired pneumonia (HAP), ventilator-associated pneumonia, and healthcare-associated pneumonia (HCAP).2 This broad document outlines an evidence-based approach to diagnostic testing and antibiotic management based on the epidemiology and risk factors for these conditions. The guideline specifies the following criteria for HCAP: hospitalization in the past 90 days, residence in a skilled nursing facility (SNF), home infusion therapy, hemodialysis, home wound care, family members with multidrug resistant organisms (MDRO), and immunosuppressive diseases or medications, with the presumption that these patients are more likely to be harboring MDRO and should thus be treated empirically with broad-spectrum antibiotic therapy. Prior studies have shown that patients with HCAP have a more severe illness, are more likely to have MDRO, are more likely to be inadequately treated, and are at a higher risk for mortality than patients with community-acquired pneumonia (CAP).3,4
These guidelines are controversial, especially in regard to the recommendations to empirically treat broadly with 2 antibiotics targeting Pseudomonas species, whether patients with HCAP merit broader spectrum coverage than patients with CAP, and whether the criteria for defining HCAP are adequate to predict which patients are harboring MDRO. It has subsequently been proposed that HCAP is more related to CAP than to HAP, and a recent update to the guideline removed recommendations for treatment of HCAP and will be placing HCAP into the guidelines for CAP instead.5 We sought to investigate the degree of uptake of the ATS and IDSA guideline recommendations by physicians over time, and whether this led to a change in outcomes among patients who met the criteria for HCAP.
METHODS
Setting and Patients
We identified patients discharged between July 1, 2007, and November 30, 2011, from 488 US hospitals that participated in the Premier database (Premier Inc., Charlotte, North Carolina), an inpatient database developed for measuring quality and healthcare utilization. The database is frequently used for healthcare research and has been described previously.6 Member hospitals are in all regions of the US and are generally reflective of US hospitals. This database contains multiple data elements, including sociodemographic information, International Classification of Diseases, 9th Revision-Clinical Modification (ICD-9-CM) diagnosis and procedure codes, hospital and physician information, source of admission, and discharge status. It also includes a date-stamped log of all billed items and services, including diagnostic tests, medications, and other treatments. Because the data do not contain identifiable information, the institutional review board at our medical center determined that this study did not constitute human subjects research.
We included all patients aged ≥18 years with a principal diagnosis of pneumonia or with a secondary diagnosis of pneumonia paired with a principal diagnosis of respiratory failure, acute respiratory distress syndrome, respiratory arrest, sepsis, or influenza. Patients were excluded if they were transferred to or from another acute care institution, had a length of stay of 1 day or less, had cystic fibrosis, did not have a chest radiograph, or did not receive antibiotics within 48 hours of admission.
For each patient, we extracted age, gender, principal diagnosis, comorbidities, and the specialty of the attending physician. Comorbidities were identified from ICD-9-CM secondary diagnosis codes and Diagnosis Related Groups by using Healthcare Cost and Utilization Project Comorbidity Software, version 3.1, based on the work of Elixhauser (Agency for Healthcare Research and Quality, Rockville, Maryland).7 In order to ensure that patients had HCAP, we required the presence of ≥1 HCAP criteria, including hospitalization in the past 90 days, hemodialysis, admission from an SNF, or immune suppression (which was derived from either a secondary diagnosis for neutropenia, hematological malignancy, organ transplant, acquired immunodeficiency virus, or receiving immunosuppressant drugs or corticosteroids [equivalent to ≥20 mg/day of prednisone]).
Definitions of Guideline-Concordant and Discordant Antibiotic Therapy
The ATS and IDSA guidelines recommended the following antibiotic combinations for HCAP: an antipseudomonal cephalosporin or carbapenem or a beta-lactam/lactamase inhibitor, plus an antipseudomonal quinolone or aminoglycoside, plus an antibiotic with activity versus methicillin resistant Staphylococcus aureus (MRSA), such as vancomycin or linezolid. Based on these guidelines, we defined the receipt of fully guideline-concordant antibiotics as 2 recommended antibiotics for Pseudomonas species plus 1 for MRSA administered by the second day of admission. Partially guideline-concordant antibiotics were defined as 1 recommended antibiotic for Pseudomonas species plus 1 for MRSA by the second day of hospitalization. Guideline-discordant antibiotics were defined as all other combinations.
Statistical Analysis
Descriptive statistics on patient characteristics are presented as frequency, proportions for categorical factors, and median with interquartile range (IQR) for continuous variables for the full cohort and by treatment group, defined as fully or partially guideline-concordant antibiotic therapy or discordant therapy. Hospital rates of fully guideline-concordant treatment are presented overall and by hospital characteristics. The association of hospital characteristics with rates of fully guideline-concordant therapy were assessed by using 1-way analysis of variance tests.
To assess trends across hospitals for the association between the use of guideline-concordant therapy and mortality, progression to respiratory failure as measured by the late initiation of invasive mechanical ventilation (day 3 or later), and the length of stay among survivors, we divided the 4.5-year study period into 9 intervals of 6 months each; 292 hospitals that submitted data for all 9 time points were examined in this analysis. Based on the distribution of length of stay in the first time period, we created an indicator variable for extended length of stay with length of stay at or above the 75th percentile, defined as extended. For each hospital at each 6-month interval, we then computed risk-standardized guideline-concordant treatment (RS-treatment) rates and risk-standardized in-hospital outcome rates similar to methods used by the Centers for Medicare and Medicaid Services for public reporting.8 For each hospital at each time interval, we estimated a predicted rate of guideline-concordant treatment as the sum of predicted probabilities of guideline-concordant treatment from patient factors and the random intercept for the hospital in which they were admitted. We then calculated the expected rate of guideline-concordant treatment as the sum of expected probabilities of treatment received from patient factors only. RS-treatment was then calculated as the ratio of predicted to expected rates multiplied by the overall unadjusted mean treatment rate from all patients.9 We repeated the same modeling strategy to calculate risk-standardized outcome (RS-outcome) rates for each hospital across all time points. All models were adjusted for patient demographics and comorbidities. Similar models using administrative data have moderate discrimination for mortality.10
We then fit mixed-effects linear models with random hospital intercept and slope across time for the RS-treatment and outcome rates, respectively. From these models, we estimated the mean slope for RS-treatment and for RS-outcome over time. In addition, we estimated a slope or trend over time for each hospital for treatment and for outcome and evaluated the correlation between the treatment and outcome trends.
All analyses were performed using the Statistical Analysis System version 9.4 (SAS Institute Inc., Cary, NC) and STATA release 13 (StataCorp, LLC, College Station, Texas).
RESULTS
DISCUSSION
In this large, retrospective cohort study, we found that there was a substantial gap between the empiric antibiotics recommended by the ATS and IDSA guidelines and the empiric antibiotics that patients actually received. Over the study period, we saw an increased adherence to guidelines, in spite of growing evidence that HCAP risk factors do not adequately predict which patients are at risk for infection with an MDRO.11 We used this change in antibiotic prescribing behavior over time to determine if there was a clinical impact on patient outcomes and found that at the hospital level, there were no improvements in mortality, excess length of stay, or progression to respiratory failure despite a doubling in guideline-concordant antibiotic use.
At least 2 other large studies have assessed the association between guideline-concordant therapy and outcomes in HCAP.12,13 Both found that guideline-concordant therapy was associated with increased mortality, despite propensity matching. Both were conducted at the individual patient level by using administrative data, and results were likely affected by unmeasured clinical confounders, with sicker patients being more likely to receive guideline-concordant therapy. Our focus on the outcomes at the hospital level avoids this selection bias because the overall severity of illness of patients at any given hospital would not be expected to change over the study period, while physician uptake of antibiotic prescribing guidelines would be expected to increase over time. Determining the correlation between increases in guideline adherence and changes in patient outcome may offer a better assessment of the impact of guideline adherence. In this regard, our results are similar to those achieved by 1 quality improvement collaborative that was aimed at increasing guideline concordant therapy in ICUs. Despite an increase in guideline concordance from 33% to 47% of patients, they found no change in overall mortality.14
There were several limitations to our study. We did not have access to microbiologic data, so we were unable to determine which patients had MDRO infection or determine antibiotic-pathogen matching. However, the treating physicians in our study population presumably did not have access to this data at the time of treatment either because the time period we examined was within the first 48 hours of hospitalization, the interval during which cultures are incubating and the patients are being treated empirically. In addition, there may have been HCAP patients that we failed to identify, such as patients who were admitted in the past 90 days to a hospital that does not submit data to Premier. However, it is unlikely that prescribing for such patients should differ systematically from what we observed. While the database draws from 488 hospitals nationwide, it is possible that practices may be different at facilities that are not contained within the Premier database, such as Veterans Administration Hospitals. Similarly, we did not have readings for chest x-rays; hence, there could be some patients in the dataset who did not have pneumonia. However, we tried to overcome this by including only those patients with a principal diagnosis of pneumonia or sepsis with a secondary pneumonia diagnosis, a chest x-ray, and antibiotics administered within the first 48 hours of admission.
There are likely several reasons why so few HCAP patients in our study received guideline-concordant antibiotics. A lack of knowledge about the ATS and IDSA guidelines may have impacted the physicians in our study population. El-Solh et al.15 surveyed physicians about the ATS-IDSA guidelines 4 years after publication and found that only 45% were familiar with the document. We found that the rate of prescribing at least partially guideline-concordant antibiotics rose steadily over time, supporting the idea that the newness of the guidelines was 1 barrier. Additionally, prior studies have shown that many physicians may not agree with or choose to follow guidelines, with only 20% of physicians indicating that guidelines have a major impact on their clinical decision making,16 and the majority do not choose HCAP guideline-concordant antibiotics when tested.17 Alternatively, clinicians may not follow the guidelines because of a belief that the HCAP criteria do not adequately indicate patients who are at risk for MDRO. Previous studies have demonstrated the relative inability of HCAP risk factors to predict patients who harbor MDRO18 and suggest that better tools such as clinical scoring systems, which include not only the traditional HCAP risk factors but also prior exposure to antibiotics, prior culture data, and a cumulative assessment of both intrinsic and extrinsic factors, could more accurately predict MDRO and lead to a more judicious use of broad-spectrum antimicrobial agents.19-25 Indeed, these collective findings have led the authors of the recently updated guidelines to remove HCAP as a clinical entity from the hospital-acquired or ventilator-associated pneumonia guidelines and place them instead in the upcoming updated guidelines on the management of CAP.5 Of these 3 explanations, the lack of familiarity fits best with our observation that guideline-concordant therapy increased steadily over time with no evidence of reaching a plateau. Ironically, as consensus was building that HCAP is a poor marker for MDROs, routine empiric treatment with vancomycin and piperacillin-tazobactam (“vanco and zosyn”) have become routine in many hospitals. Additional studies are needed to know if this trend has stabilized or reversed.
CONCLUSIONS
In conclusion, clinicians in our large, nationally representative sample treated the majority of HCAP patients as though they had CAP. Although there was an increase in the administration of guideline-concordant therapy over time, this increase was not associated with improved outcomes. This study supports the growing consensus that HCAP criteria do not accurately predict which patients benefit from broad-spectrum antibiotics for pneumonia, and most patients fare well with antibiotics targeting common community-acquired organisms.
Disclosure
This work was supported by grant # R01HS018723 from the Agency for Healthcare Research and Quality. Dr. Lagu is also supported by the National Heart, Lung, and Blood Institute of the National Institutes of Health under award number K01HL114745. Dr. Lindenauer is supported by grant K24HL132008 from the National Heart, Lung, and Blood Institute. The funding agency had no role in the data acquisition, analysis, or manuscript preparation for this study. Drs. Haessler and Rothberg had full access to all the data in the study and take responsibility for the integrity of the data and the accuracy of the data analysis. Drs. Haessler, Lagu, Lindenauer, Skiest, Zilberberg, Higgins, and Rothberg conceived of the study and analyzed and interpreted the data. Dr. Lindenauer acquired the data. Dr. Pekow and Ms. Priya carried out the statistical analyses. Dr. Haessler drafted the manuscript. All authors critically reviewed the manuscript for accuracy and integrity. All authors certify no potential conflicts of interest. Preliminary results from this study were presented in oral and poster format at IDWeek in 2012 and 2013.
1. Kochanek KD, Murphy SL, Xu JQ, Tejada-Vera B. Deaths: Final data for 2014. National vital statistics reports; vol 65 no 4. Hyattsville, MD: National Center for Health Statistics. 2016. PubMed
2. American Thoracic Society, Infectious Diseases Society of America. Guidelines for the Management of Adults with Hospital-acquired, Ventilator-associated, and Healthcare-associated Pneumonia. Am J Respir Crit Care Med. 2005;171(4):388-416. PubMed
3. Zilberberg MD, Shorr A. Healthcare-associated pneumonia: the state of the evidence to date. Curr Opin Pulm Med. 2011;17(3):142-147. PubMed
4. Kollef MK, Shorr A, Tabak YP, Gupta V, Liu LZ, Johannes RS. Epidemiology and Outcomes of Health-care-associated pneumonia. Chest. 2005;128(6):3854-3862. PubMed
5. Kalil AC, Metersky ML, Klompas M, et al. Management of Adults With Hospital-acquired and Ventilator-associated Pneumonia: 2016 Clinical Practice Guidelines by the Infectious Diseases Society of America and the American Thoracic Society. Clin Infect Dis. 2016;63(5):575-582. PubMed
6. Lindenauer PK, Pekow PS, Lahti MC, Lee Y, Benjamin EM, Rothberg MB. Association of corticosteroid dose and route of administration with risk of treatment failure in acute exacerbation of chronic obstructive pulmonary disease. JAMA. 2010;303(23):2359-2367. PubMed
7. Elixhauser A, Steiner C, Harris DR, Coffey RM. Comorbidity measures for use with administrative data. Med Care. 1998;36(1):8-27. PubMed
8. Centers for Medicare & Medicaid Services. Frequently asked questions (FAQs): Implementation and maintenance of CMS mortality measures for AMI & HF. 2007. https://www.cms.gov/Medicare/Quality-Initiatives-Patient-Assessment-Instruments/HospitalQualityInits/downloads/HospitalMortalityAboutAMI_HF.pdf. Accessed November 1, 2016.
9. Normand SL, Shahian DM. Statistical and Clinical Aspects of Hospital Outcomes Profiling. Stat Sci. 2007;22(2):206-226.
10. Rothberg MB, Pekow PS, Priya A, et al. Using highly detailed administrative data to predict pneumonia mortality. PLoS One. 2014;9(1):e87382. PubMed
11. Jones BE, Jones MM, Huttner B, et al. Trends in antibiotic use and nosocomial pathogens in hospitalized veterans with pneumonia at 128 medical centers, 2006-2010. Clin Infect Dis. 2015;61(9):1403-1410. PubMed
12. Attridge RT, Frei CR, Restrepo MI, et al. Guideline-concordant therapy and outcomes in healthcare-associated pneumonia. Eur Respir J. 2011;38(4):878-887. PubMed
13. Rothberg MB, Zilberberg MD, Pekow PS, et al. Association of Guideline-based Antimicrobial Therapy and Outcomes in Healthcare-Associated Pneumonia. J Antimicrob Chemother. 2015;70(5):1573-1579. PubMed
14. Kett DH, Cano E, Quartin AA, et al. Improving Medicine through Pathway Assessment of Critical Therapy of Hospital-Acquired Pneumonia (IMPACT-HAP) Investigators. Implementation of guidelines for management of possible multidrug-resistant pneumonia in intensive care: an observational, multicentre cohort study. Lancet Infect Dis. 2011;11(3):181-189. PubMed
15. El-Solh AA, Alhajhusain A, Saliba RG, Drinka P. Physicians’ Attitudes Toward Guidelines for the Treatment of Hospitalized Nursing-Home -Acquired Pneumonia. J Am Med Dir Assoc. 2011;12(4):270-276. PubMed
16. Tunis S, Hayward R, Wilson M, et al. Internists’ Attitudes about Clinical Practice Guidelines. Ann Intern Med. 1994;120(11):956-963. PubMed
17. Seymann GB, Di Francesco L, Sharpe B, et al. The HCAP Gap: Differences between Self-Reported Practice Patterns and Published Guidelines for Health Care-Associated Pneumonia. Clin Infect Dis. 2009;49(12):1868-1874. PubMed
18. Chalmers JD, Rother C, Salih W, Ewig S. Healthcare associated pneumonia does not accurately identify potentially resistant pathogens: a systematic review and meta-analysis. Clin Infect Dis. 2014;58(3):330-339. PubMed
19. Shorr A, Zilberberg MD, Reichley R, et al. Validation of a Clinical Score for Assessing the Risk of Resistant Pathogens in Patients with Pneumonia Presenting to the Emergency Department. Clin Infect Dis. 2012;54(2):193-198. PubMed
20. Aliberti S, Pasquale MD, Zanaboni AM, et al. Stratifying Risk Factors for Multidrug-Resistant Pathogens in Hospitalized Patients Coming from the Community with Pneumonia. Clin Infect Dis. 2012;54(4):470-478. PubMed
21. Schreiber MP, Chan CM, Shorr AF. Resistant Pathogens in Nonnosocomial Pneumonia and Respiratory Failure: Is it Time to Refine the Definition of Health-care-Associated Pneumonia? Chest. 2010;137(6):1283-1288. PubMed
22. Madaras-Kelly KJ, Remington RE, Fan VS, Sloan KL. Predicting antibiotic resistance to community-acquired pneumonia antibiotics in culture-positive patients with healthcare-associated pneumonia. J Hosp Med. 2012;7(3):195-202. PubMed
23. Shindo Y, Ito R, Kobayashi D, et al. Risk factors for drug-resistant pathogens in community-acquired and healthcare-associated pneumonia. Am J Respir Crit Care Med. 2013;188(8):985-995. PubMed
24. Metersky ML, Frei CR, Mortensen EM. Predictors of Pseudomonas and methicillin-resistant Staphylococcus aureus in hospitalized patients with healthcare-associated pneumonia. Respirology. 2016;21(1):157-163. PubMed
25. Webb BJ, Dascomb K, Stenehjem E, Dean N. Predicting risk of drug-resistant organisms in pneumonia: moving beyond the HCAP model. Respir Med. 2015;109(1):1-10. PubMed
1. Kochanek KD, Murphy SL, Xu JQ, Tejada-Vera B. Deaths: Final data for 2014. National vital statistics reports; vol 65 no 4. Hyattsville, MD: National Center for Health Statistics. 2016. PubMed
2. American Thoracic Society, Infectious Diseases Society of America. Guidelines for the Management of Adults with Hospital-acquired, Ventilator-associated, and Healthcare-associated Pneumonia. Am J Respir Crit Care Med. 2005;171(4):388-416. PubMed
3. Zilberberg MD, Shorr A. Healthcare-associated pneumonia: the state of the evidence to date. Curr Opin Pulm Med. 2011;17(3):142-147. PubMed
4. Kollef MK, Shorr A, Tabak YP, Gupta V, Liu LZ, Johannes RS. Epidemiology and Outcomes of Health-care-associated pneumonia. Chest. 2005;128(6):3854-3862. PubMed
5. Kalil AC, Metersky ML, Klompas M, et al. Management of Adults With Hospital-acquired and Ventilator-associated Pneumonia: 2016 Clinical Practice Guidelines by the Infectious Diseases Society of America and the American Thoracic Society. Clin Infect Dis. 2016;63(5):575-582. PubMed
6. Lindenauer PK, Pekow PS, Lahti MC, Lee Y, Benjamin EM, Rothberg MB. Association of corticosteroid dose and route of administration with risk of treatment failure in acute exacerbation of chronic obstructive pulmonary disease. JAMA. 2010;303(23):2359-2367. PubMed
7. Elixhauser A, Steiner C, Harris DR, Coffey RM. Comorbidity measures for use with administrative data. Med Care. 1998;36(1):8-27. PubMed
8. Centers for Medicare & Medicaid Services. Frequently asked questions (FAQs): Implementation and maintenance of CMS mortality measures for AMI & HF. 2007. https://www.cms.gov/Medicare/Quality-Initiatives-Patient-Assessment-Instruments/HospitalQualityInits/downloads/HospitalMortalityAboutAMI_HF.pdf. Accessed November 1, 2016.
9. Normand SL, Shahian DM. Statistical and Clinical Aspects of Hospital Outcomes Profiling. Stat Sci. 2007;22(2):206-226.
10. Rothberg MB, Pekow PS, Priya A, et al. Using highly detailed administrative data to predict pneumonia mortality. PLoS One. 2014;9(1):e87382. PubMed
11. Jones BE, Jones MM, Huttner B, et al. Trends in antibiotic use and nosocomial pathogens in hospitalized veterans with pneumonia at 128 medical centers, 2006-2010. Clin Infect Dis. 2015;61(9):1403-1410. PubMed
12. Attridge RT, Frei CR, Restrepo MI, et al. Guideline-concordant therapy and outcomes in healthcare-associated pneumonia. Eur Respir J. 2011;38(4):878-887. PubMed
13. Rothberg MB, Zilberberg MD, Pekow PS, et al. Association of Guideline-based Antimicrobial Therapy and Outcomes in Healthcare-Associated Pneumonia. J Antimicrob Chemother. 2015;70(5):1573-1579. PubMed
14. Kett DH, Cano E, Quartin AA, et al. Improving Medicine through Pathway Assessment of Critical Therapy of Hospital-Acquired Pneumonia (IMPACT-HAP) Investigators. Implementation of guidelines for management of possible multidrug-resistant pneumonia in intensive care: an observational, multicentre cohort study. Lancet Infect Dis. 2011;11(3):181-189. PubMed
15. El-Solh AA, Alhajhusain A, Saliba RG, Drinka P. Physicians’ Attitudes Toward Guidelines for the Treatment of Hospitalized Nursing-Home -Acquired Pneumonia. J Am Med Dir Assoc. 2011;12(4):270-276. PubMed
16. Tunis S, Hayward R, Wilson M, et al. Internists’ Attitudes about Clinical Practice Guidelines. Ann Intern Med. 1994;120(11):956-963. PubMed
17. Seymann GB, Di Francesco L, Sharpe B, et al. The HCAP Gap: Differences between Self-Reported Practice Patterns and Published Guidelines for Health Care-Associated Pneumonia. Clin Infect Dis. 2009;49(12):1868-1874. PubMed
18. Chalmers JD, Rother C, Salih W, Ewig S. Healthcare associated pneumonia does not accurately identify potentially resistant pathogens: a systematic review and meta-analysis. Clin Infect Dis. 2014;58(3):330-339. PubMed
19. Shorr A, Zilberberg MD, Reichley R, et al. Validation of a Clinical Score for Assessing the Risk of Resistant Pathogens in Patients with Pneumonia Presenting to the Emergency Department. Clin Infect Dis. 2012;54(2):193-198. PubMed
20. Aliberti S, Pasquale MD, Zanaboni AM, et al. Stratifying Risk Factors for Multidrug-Resistant Pathogens in Hospitalized Patients Coming from the Community with Pneumonia. Clin Infect Dis. 2012;54(4):470-478. PubMed
21. Schreiber MP, Chan CM, Shorr AF. Resistant Pathogens in Nonnosocomial Pneumonia and Respiratory Failure: Is it Time to Refine the Definition of Health-care-Associated Pneumonia? Chest. 2010;137(6):1283-1288. PubMed
22. Madaras-Kelly KJ, Remington RE, Fan VS, Sloan KL. Predicting antibiotic resistance to community-acquired pneumonia antibiotics in culture-positive patients with healthcare-associated pneumonia. J Hosp Med. 2012;7(3):195-202. PubMed
23. Shindo Y, Ito R, Kobayashi D, et al. Risk factors for drug-resistant pathogens in community-acquired and healthcare-associated pneumonia. Am J Respir Crit Care Med. 2013;188(8):985-995. PubMed
24. Metersky ML, Frei CR, Mortensen EM. Predictors of Pseudomonas and methicillin-resistant Staphylococcus aureus in hospitalized patients with healthcare-associated pneumonia. Respirology. 2016;21(1):157-163. PubMed
25. Webb BJ, Dascomb K, Stenehjem E, Dean N. Predicting risk of drug-resistant organisms in pneumonia: moving beyond the HCAP model. Respir Med. 2015;109(1):1-10. PubMed
© 2017 Society of Hospital Medicine
Impact of HOCDI on Sepsis Patients
There are approximately 3 million cases of Clostridium difficile infection (CDI) per year in the United States.[1, 2, 3, 4] Of these, 10% result in a hospitalization or occur as a consequence of the exposures and treatments associated with hospitalization.[1, 2, 3, 4] Some patients with CDI experience mild diarrhea that is responsive to therapy, but other patients experience severe, life‐threatening disease that is refractory to treatment, leading to pseudomembranous colitis, toxic megacolon, and sepsis with a 60‐day mortality rate that exceeds 12%.[5, 6, 7, 8, 9, 10, 11, 12, 13, 14]
Hospital‐onset CDI (HOCDI), defined as C difficile‐associated diarrhea and related symptoms with onset more than 48 hours after admission to a healthcare facility,[15] represents a unique marriage of CDI risk factors.[5] A vulnerable patient is introduced into an environment that contains both exposure to C difficile (through other patients or healthcare workers) and treatment with antibacterial agents that may diminish normal flora. Consequently, CDI is common among hospitalized patients.[16, 17, 18] A particularly important group for understanding the burden of disease is patients who initially present to the hospital with sepsis and subsequently develop HOCDI. Sepsis patients are often critically ill and are universally treated with antibiotics.
Determining the incremental cost and mortality risk attributable to HOCDI is methodologically challenging. Because HOCDI is associated with presenting severity, the sickest patients are also the most likely to contract the disease. HOCDI is also associated with time of exposure or length of stay (LOS). Because LOS is a risk factor, comparing LOS between those with and without HOCDI will overestimate the impact if the time to diagnosis is not taken into account.[16, 17, 19, 20] We aimed to examine the impact of HOCDI in hospitalized patients with sepsis using a large, multihospital database with statistical methods that took presenting severity and time to diagnosis into account.
METHODS
Data Source and Subjects
Permission to conduct this study was obtained from the institutional review board at Baystate Medical Center. We used the Premier Healthcare Informatics database, a voluntary, fee‐supported database created to measure quality and healthcare utilization, which has been used extensively in health services research.[21, 22, 23] In addition to the elements found in hospital claims derived from the uniform billing 04 form, Premier data include an itemized, date‐stamped log of all items and services charged to the patient or their insurer, including medications, laboratory tests, and diagnostic and therapeutic services. Approximately 75% of hospitals that submit data also provide information on actual hospital costs, taken from internal cost accounting systems. The rest provide cost estimates based on Medicare cost‐to‐charge ratios. Participating hospitals are similar to the composition of acute care hospitals nationwide, although they are more commonly small‐ to midsized nonteaching facilities and are more likely to be located in the southern United States.
We included medical (nonsurgical) adult patients with sepsis who were admitted to a participating hospital between July 1, 2004 and December 31, 2010. Because we sought to focus on the care of patients who present to the hospital with sepsis, we defined sepsis as the presence of a diagnosis of sepsis plus evidence of both blood cultures and antibiotic treatment within the first 2 days of hospitalization; we used the first 2 days of hospitalization rather than just the first day because, in administrative datasets, the duration of the first hospital day includes partial days that can vary in length. We excluded patients who died or were discharged prior to day 3, because HOCDI is defined as onset after 48 hours in a healthcare facility.[15] We also excluded surviving patients who received less than 3 consecutive days of antibiotics, and patients who were transferred from or to another acute‐care facility; the latter exclusion criterion was used because we could not accurately determine the onset or subsequent course of their illness.
Identification of Patients at Risk for and Diagnosed With HOCDI
Among eligible patients with sepsis, we aimed to identify a cohort at risk for developing CDI during the hospital stay. We excluded patients: (1) with a diagnosis indicating that diarrhea was present on admission, (2) with a diagnosis of CDI that was indicated to be present on admission, (3) who were tested for CDI on the first or second hospital day, and (4) who received an antibiotic that could be consistent with treatment for CDI (oral or intravenous [IV] metronidazole or oral vancomycin) on hospital days 1 or 2.
Next, we aimed to identify sepsis patients at risk for HOCDI who developed HOCDI during their hospital stay. Among eligible patients described above, we considered a patient to have HOCDI if they had an International Classification of Diseases, Ninth Revision, Clinical Modification diagnosis of CDI (primary or secondary but not present on admission), plus evidence of testing for CDI after hospital day 2, and treatment with oral vancomycin or oral or IV metronidazole that was started after hospital day 2 and within 2 days of the C difficile test, and evidence of treatment for CDI for at least 3 days unless the patient was discharged or died.
Patient Information
We recorded patient age, gender, marital status, insurance status, race, and ethnicity. Using software provided by the Healthcare Costs and Utilization Project of the Agency for Healthcare Research and Quality, we categorized information on 30 comorbid conditions. We also created a single numerical comorbidity score based on a previously published and validated combined comorbidity score that predicts 1‐year mortality.[24] Based on a previously described algorithm,[25] we used diagnosis codes to assess the source (lung, abdomen, urinary tract, blood, other) and type of sepsis (Gram positive, Gram negative, mixed, anaerobic, fungal). Because patients can have more than 1 potential source of sepsis (eg, pneumonia and urinary tract infection) and more than 1 organism causing infection (eg, urine with Gram negative rods and blood culture with Gram positive cocci), these categories are not mutually exclusive (see Supporting Table 1 in the online version of this article). We used billing codes to identify the use of therapies, monitoring devices, and pharmacologic treatments to characterize both initial severity of illness and severity at the time of CDI diagnosis. These therapies are included in a validated sepsis mortality prediction model (designed for administrative datasets) with similar discrimination and calibration to clinical intensive care unit (ICU) risk‐adjustment models such as the mortality probability model, version III.[26, 27]
Outcomes
Our primary outcome of interest was in‐hospital mortality. Secondary outcomes included LOS and costs for survivors only and for all patients.
Statistical Methods
We calculated patient‐level summary statistics for all patients using frequencies for binary variables and medians and interquartile percentiles for continuous variables. P values <0.05 were considered statistically significant.
To account for presenting severity and time to diagnosis, we used methods that have been described elsewhere.[12, 13, 18, 20, 28] First, we identified patients who were eligible to develop HOCDI. Second, for all eligible patients, we identified a date of disease onset (index date). For patients who met criteria for HOCDI, this was the date on which the patient was tested for CDI. For eligible patients without disease, this was a date randomly assigned to any time during the hospital stay.[29] Next, we developed a nonparsimonious propensity score model that included all patient characteristics (demographics, comorbidities, sepsis source, and severity of illness on presentation and on the index date; all variables listed in Table 1 were included in the propensity model). Some of the variables for this model (eg, mechanical ventilation and vasopressors) were derived from a validated severity model.[26] We adjusted for correlation within hospital when creating the propensity score using Huber‐White robust standard error estimators clustered at the hospital level.[30] We then created matched pairs with the same LOS prior to the index date and similar propensity for developing CDI. We first matched on index date, and then, within each index‐datematched subset, matched patients with and without HOCDI by their propensity score using a 5‐to‐1 greedy match algorithm.[31] We used the differences in LOS between the cases and controls after the index date to calculate the additional attributable LOS estimates; we also separately estimated the impact on cost and LOS in a group limited to those who survived after discharge because of concerns that death could shorten LOS and reduce costs.
Before Matching | After Matching | |||||
---|---|---|---|---|---|---|
HOCDI, n=2,368, % | No CDI, n=216,547, % | P | HOCDI, n=2,368, % | No CDI, n=2,368, % | P | |
| ||||||
Age, y | 70.9 (15.1) | 68.6 (16.8) | <0.01 | 70.9 (15.1) | 69.8 (15.9) | 0.02 |
Male | 46.8 | 46.0 | 0.44 | 46.8 | 47.2 | 0.79 |
Race | ||||||
White | 61.0 | 63.3 | 61.0 | 58.1 | ||
Black | 15.6 | 14.5 | <0.01 | 15.6 | 17.0 | 0.11 |
Hispanic | 3.2 | 5.4 | 3.2 | 4.1 | ||
Other race | 20.2 | 16.8 | 20.2 | 20.9 | ||
Marital status | ||||||
Married | 31.6 | 36.3 | <0.01 | 31.6 | 32.6 | 0.74 |
Single/divorced | 52.8 | 51.1 | 52.8 | 52.0 | ||
Other/unknown | 15.7 | 12.6 | 15.7 | 14.5 | ||
Insurance status | ||||||
Medicare traditional | 63.2 | 59.5 | 63.2 | 60.3 | ||
Medicare managed | 10.6 | 10.1 | 10.6 | 10.9 | ||
Medicaid traditional | 7.6 | 6.9 | 7.6 | 8.2 | ||
Medicaid managed | 1.8 | 2.0 | <0.01 | 1.8 | 1.8 | 0.50 |
Managed care | 10.8 | 12.3 | 10.8 | 12.0 | ||
Commercial | 2.0 | 3.5 | 2.0 | 2.2 | ||
Self‐pay/other/unknown | 4.0 | 5.7 | 4.0 | 4.7 | ||
Infection source | ||||||
Respiratory | 46.5 | 37.0 | <0.01 | 46.5 | 49.6 | 0.03 |
Skin/bone | 10.1 | 8.6 | 0.01 | 10.1 | 11.2 | 0.21 |
Urinary | 52.2 | 51.3 | 0.38 | 52.2 | 50.3 | 0.18 |
Blood | 11.1 | 15.1 | <0.01 | 11.1 | 11.5 | 0.65 |
Infecting organism | ||||||
Gram negative | 35.0 | 36.6 | <0.01 | 35.0 | 33.1 | 0.18 |
Anaerobe | 1.4 | 0.7 | <0.01 | 1.4 | 1.1 | 0.24 |
Fungal | 17.5 | 7.5 | <0.01 | 17.5 | 18.3 | 0.44 |
Most common comorbid conditions | ||||||
Congestive heart failure | 35.1 | 24.6 | <0.01 | 35.1 | 37.5 | 0.06 |
Chronic lung disease | 31.6 | 27.6 | <0.01 | 31.6 | 32.1 | 0.71 |
Hypertension | 31.5 | 37.7 | <0.01 | 31.5 | 29.7 | 0.16 |
Renal Failure | 29.7 | 23.8 | <0.01 | 29.7 | 31.2 | 0.28 |
Weight Loss | 27.7 | 13.3 | <0.01 | 27.7 | 29.4 | 0.17 |
Treatments by day 2 | ||||||
ICU admission | 40.0 | 29.5 | <0.01 | 40.0 | 40.7 | 0.64 |
Use of bicarbonate | 12.2 | 7.1 | <0.01 | 12.2 | 13.6 | 0.15 |
Fresh frozen plasma | 1.4 | 1.0 | 0.03 | 1.4 | 1.1 | 0.36 |
Inotropes | 1.4 | 0.9 | 0.01 | 1.4 | 2.2 | 0.04 |
Hydrocortisone | 6.7 | 4.7 | <0.01 | 6.7 | 7.4 | 0.33 |
Thiamine | 4.2 | 3.3 | 0.01 | 4.2 | 4.1 | 0.83 |
Psychotropics (eg, haldol for delirium) | 10.0 | 9.2 | 0.21 | 10.0 | 10.8 | 0.36 |
Restraints (eg, for delirium) | 2.0 | 1.5 | 0.05 | 2.0 | 2.5 | 0.29 |
Angiotensin‐converting enzyme inhibitors | 12.1 | 13.2 | 0.12 | 12.1 | 10.9 | 0.20 |
Statins | 18.8 | 21.1 | 0.01 | 18.8 | 16.9 | 0.09 |
Drotrecogin alfa | 0.6 | 0.3 | 0.00 | 0.6 | 0.6 | 0.85 |
Foley catheter | 19.2 | 19.8 | 0.50 | 19.2 | 22.0 | 0.02 |
Diuretics | 28.5 | 25.4 | 0.01 | 28.5 | 29.6 | 0.42 |
Red blood cells | 15.5 | 10.6 | <0.01 | 15.5 | 15.8 | 0.81 |
Calcium channel blockers | 19.3 | 16.8 | 0.01 | 19.3 | 19.1 | 0.82 |
‐Blockers | 32.7 | 29.6 | 0.01 | 32.7 | 30.6 | 0.12 |
Proton pump inhibitors | 59.6 | 53.1 | <0.01 | 59.6 | 61.0 | 0.31 |
Analysis Across Clinical Subgroups
In a secondary analysis, we examined heterogeneity in the association between HOCDI and outcomes within subsets of patients defined by age, combined comorbidity score, and admission to the ICU by day 2. We created separate propensity scores using the same covariates in the primary analysis, but limited matches to within these subsets. For each group, we examined how the covariates in the HOCDI and control groups differed after matching with inference tests that took the paired nature of the data into account. All analyses were carried out using Stata/SE 11.1 (StataCorp, College Station, TX).
RESULTS
We identified 486,943 adult sepsis admissions to a Premier hospital between July 1, 2004 and December 31, 2010. After applying all exclusion criteria, we had a final sample of 218,915 admissions with sepsis (from 400 hospitals) at risk for HOCDI (Figure 1). Of these, 2368 (1.08%) met criteria for diagnosis of CDI after hospital day 2 and were matched to controls using index date and propensity score.
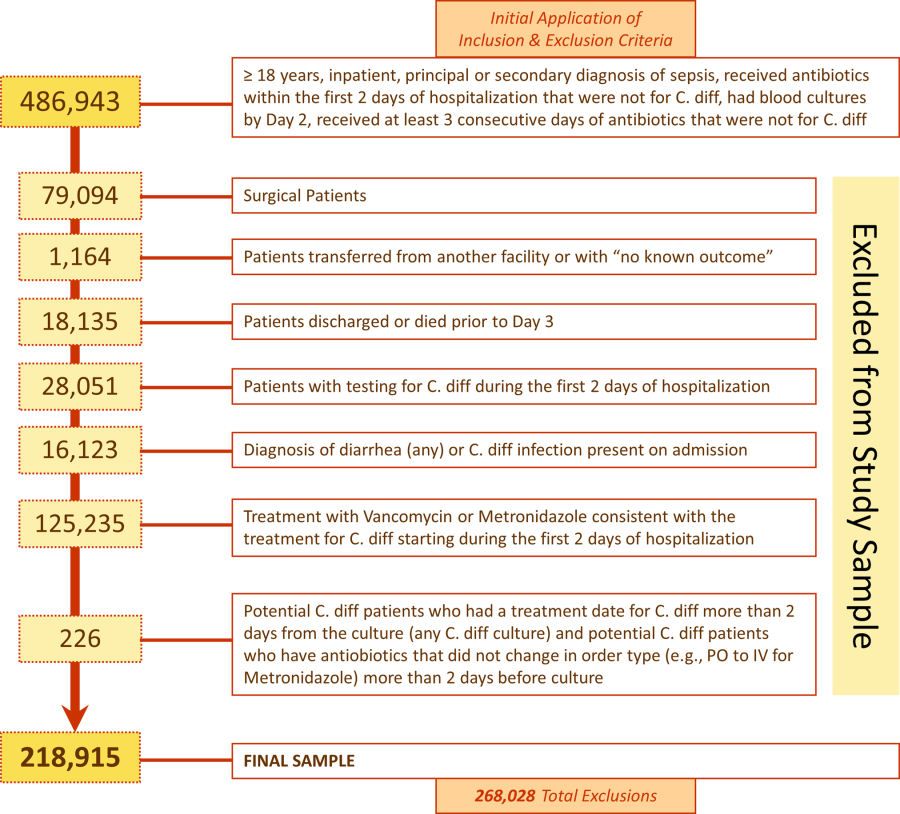
Patient and Hospital Factors
After matching, the median age was 71 years in cases and 70 years in controls (Table 1). Less than half (46%) of the population was male. Most cases (61%) and controls (58%) were white. Heart failure, hypertension, chronic lung disease, renal failure, and weight loss were the most common comorbid conditions. Our propensity model, which had a C statistic of 0.75, identified patients whose risk varied from a mean of 0.1% in the first decile to a mean of 3.8% in the tenth decile. Before matching, 40% of cases and 29% of controls were treated in the ICU by hospital day 2; after matching, 40% of both cases and controls were treated in the ICU by hospital day 2.
Distribution by LOS, Index Day, and Risk for Mortality
The unadjusted and unmatched LOS was longer for cases than controls (19 days vs 8 days, Table 2) (see Supporting Figure 1 in the online version of this article). Approximately 90% of the patients had an index day of 14 or less (Figure 2). Among patients both with and without CDI, the unadjusted mortality risk increased as the index day (and thus the total LOS) increased.
Outcome | HOCDI | No HOCDI | Difference (95% CI) | P |
---|---|---|---|---|
| ||||
Length of stay, d | ||||
Raw results | 19.2 | 8.3 | 8.4 (8.48.5) | <0.01 |
Raw results for survivors only | 18.6 | 8.0 | 10.6 (10.311.0) | <0.01 |
Matched results | 19.2 | 14.2 | 5.1(4.45.7) | <0.01 |
Matched results for survivors only | 18.6 | 13.6 | 5.1 (4.45.8) | <0.01 |
Mortality, % | ||||
Raw results | 24.0 | 10.1 | 13.9 (12.615.1), RR=2.4 (2.22.5) | <0.01 |
Matched results | 24.0 | 15.4 | 8.6 (6.410.9), RR=1.6 (1.41.8) | <0.01 |
Costs, US$ | ||||
Raw results median costs [interquartile range] | $26,187 [$15,117$46,273] | $9,988 [$6,296$17,351] | $16,190 ($15,826$16,555) | <0.01 |
Raw results for survivors only [interquartile range] | $24,038 [$14,169$41,654] | $9,429 [$6,070$15,875] | $14,620 ($14,246$14,996) | <0.01 |
Matched results [interquartile range] | $26,187 [$15,117$46,273] | $19,160 [$12,392$33,777] | $5,308 ($4,521$6,108) | |
Matched results for survivors only [interquartile range] | $24,038 [$14,169$41,654] | $17,811 [$11,614$29,298] | $4,916 ($4,088$5,768) | <0.01 |
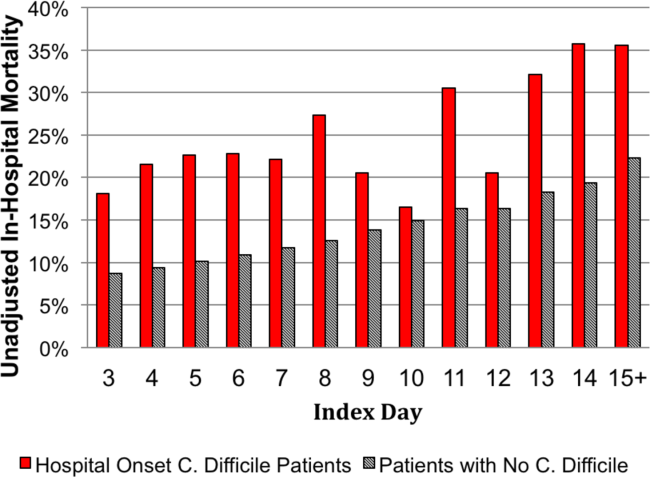
Adjusted Results
Compared to patients without disease, HOCDI patients had an increased unadjusted mortality (24% vs 10%, P<0.001). This translates into a relative risk of 2.4 (95% confidence interval [CI]: 2.2, 2.5). In the matched cohort, the difference in the mortality rates was attenuated, but still significantly higher in the HOCDI patients (24% versus 15%, P<0.001, an absolute difference of 9%; 95% CI: 6.410.8). The adjusted relative risk of mortality for HOCDI was 1.6 (95% CI: 1.41.8; Table 2). After matching, patients with CDI had a LOS of 19.2 days versus 14.2 days in matched controls (difference of 5.1 days; 95% CI: 4.45.7; P<0.001). When the LOS analysis was limited to survivors only, this difference of 5 days remained (P<0.001). In an analysis limited to survivors only, the difference in median costs between cases and controls was $4916 (95% CI: $4088$5768; P<0.001). In a secondary analysis examining heterogeneity between HOCDI and outcomes across clinical subgroups, the absolute difference in mortality and costs between cases and controls varied across demographics, comorbidity, and ICU admission, but the relative risks were similar (Figure 3) (see Supporting Figure 3 in the online version of this article).
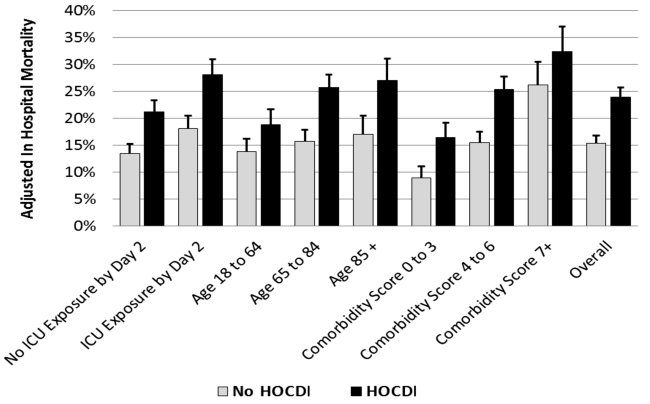
DISCUSSION
In this large cohort of patients with sepsis, we found that approximately 1 in 100 patients with sepsis developed HOCDI. Even after matching with controls based on the date of symptom onset and propensity score, patients who developed HOCDI were more than 1.6 times more likely to die in the hospital. HOCDI also added 5 days to the average hospitalization for patients with sepsis and increased median costs by approximately $5000. These findings suggest that a hospital that prevents 1 case of HOCDI per month in sepsis patients could avoid 1 death and 60 inpatient days annually, achieving an approximate yearly savings of $60,000.
Until now, the incremental cost and mortality attributable to HOCDI in sepsis patients have been poorly understood. Attributing outcomes can be methodologically challenging because patients who are at greatest risk for poor outcomes are the most likely to contract the disease and are at risk for longer periods of time. Therefore, it is necessary to take into account differences in severity of illness and time at risk between diseased and nondiseased populations and to ensure that outcomes attributed to the disease occur after disease onset.[28, 32] The majority of prior studies examining the impact of CDI on hospitalized patients have been limited by a lack of adequate matching to controls, small sample size, or failure to take into account time to infection.[16, 17, 19, 20]
A few studies have taken into account severity, time to infection, or both in estimating the impact of HOCDI. Using a time‐dependent Cox model that accounted for time to infection, Micek et al. found no difference in mortality but a longer LOS in mechanically ventilated patients (not limited to sepsis) with CDI.[33] However, their study was conducted at only 3 centers, did not take into account severity at the time of diagnosis, and did not clearly distinguish between community‐onset CDI and HOCDI. Oake et al. and Forster et al. examined the impact of CDI on patients hospitalized in a 2‐hospital health system in Canada.[12, 13] Using the baseline mortality estimate in a Cox multivariate proportional hazards regression model that accounted for the time‐varying nature of CDI, they found that HOCDI increased absolute risk of death by approximately 10%. Also, notably similar to our study were their findings that HOCDI occurred in approximately 1 in 100 patients and that the attributable median increase in LOS due to hospital‐onset CDI was 6 days. Although methodologically rigorous, these 2 small studies did not assess the impact of CDI on costs of care, were not focused on sepsis patients or even patients who received antibiotics, and also did not clearly distinguish between community‐onset CDI and HOCDI.
Our study therefore has important strengths. It is the first to examine the impact of HOCDI, including costs, on the outcomes of patients hospitalized with sepsis. The fact that we took into account both time to diagnosis and severity at the time of diagnosis (by using an index date for both cases and controls and determining severity on that date) prevented us from overestimating the impact of HOCDI on outcomes. The large differences in outcomes we observed in unadjusted and unmatched data were tempered after multivariate adjustment (eg, difference in LOS from 10.6 days to 5.1 additional days, costs from $14,620 to $4916 additional costs after adjustment). Our patient sample was derived from a large, multihospital database that contains actual hospital costs as derived from internal accounting systems. The fact that our study used data from hundreds of hospitals means that our estimates of cost, LOS, and mortality may be more generalizable than the work of Micek et al., Oake et al., and Forster et al.
This work also has important implications. First, hospital administrators, clinicians, and researchers can use our results to evaluate the cost‐effectiveness of HOCDI prevention measures (eg, hand hygiene programs, antibiotic stewardship). By quantifying the cost per case in sepsis patients, we allow administrators and researchers to compare the incremental costs of HOCDI prevention programs to the dollars and lives saved due to prevention efforts. Second, we found that our propensity model identified patients whose risk varied greatly. This suggests that an opportunity exists to identify subgroups of patients that are at highest risk. Identifying high‐risk subgroups will allow for targeted risk reduction interventions and the opportunity to reduce transmission (eg, by placing high‐risk patients in a private room). Finally, we have reaffirmed that time to diagnosis and presenting severity need to be rigorously addressed prior to making estimates of the impact of CDI burden and other hospital‐acquired conditions and injuries.
There are limitations to this study as well. We did not have access to microbiological data. However, we required a diagnosis code of CDI, evidence of testing, and treatment after the date of testing to confirm a diagnosis. We also adopted detailed exclusion criteria to ensure that CDI that was not present on admission and that controls did not have CDI. These stringent inclusion and exclusion criteria strengthened the internal validity of our estimates of disease impact. We used administrative claims data, which limited our ability to adjust for severity. However, the detailed nature of the database allowed us to use treatments, such as vasopressors and antibiotics, to identify cases; treatments were also used as a validated indicator of severity,[26] which may have helped to reduce some of this potential bias. Although our propensity model included many predictors of CDI, such as use of proton pump inhibitors and factors associated with mortality, not every confounder was completely balanced after propensity matching, although the statistical differences may have been related to our large sample size and therefore might not be clinically significant. We also may have failed to include all possible predictors of CDI in the propensity model.
In a large, diverse cohort of hospitalized patients with sepsis, we found that HOCDI lengthened hospital stay by approximately 5 days, increased risk of in‐hospital mortality by 9%, and increased hospital cost by approximately $5000 per patient. These findings highlight the importance of identifying effective prevention measures and of determining the patient populations at greatest risk for HOCDI.
Disclosures: The study was conducted with funding from the Division of Critical Care and the Center for Quality of Care Research at Baystate Medical Center. Dr. Lagu is supported by the National Heart, Lung, and Blood Institute of the National Institutes of Health under award number K01HL114745. Dr. Stefan is supported by the National Heart, Lung, and Blood Institute of the National Institutes of Health under award number K01HL114631. Drs. Lagu and Lindenauer had full access to all of the data in the study; they take responsibility for the integrity of the data and the accuracy of the data analysis. Drs. Lagu, Lindenauer, Steingrub, Higgins, Stefan, Haessler, and Rothberg conceived of the study. Dr. Lindenauer acquired the data. Drs. Lagu, Lindenauer, Rothberg, Steingrub, Nathanson, Stefan, Haessler, Higgins, and Mr. Hannon analyzed and interpreted the data. Dr. Lagu drafted the manuscript. Drs. Lagu, Lindenauer, Rothberg, Steingrub, Nathanson, Stefan, Haessler, Higgins, and Mr. Hannon critically reviewed the manuscript for important intellectual content. Dr. Nathanson carried out the statistical analyses. Dr. Nathanson, through his company OptiStatim LLC, was paid by the investigators with funding from the Department of Medicine at Baystate Medical Center to assist in conducting the statistical analyses in this study. The authors report no further conflicts of interest.
- Increasing prevalence and severity of Clostridium difficile colitis in hospitalized patients in the United States. Arch Surg. 2007;142(7):624–631; discussion 631. , , , .
- The changing epidemiology of Clostridium difficile infections. Clin Microbiol Rev. 2010;23(3):529–549. , , , et al.
- Clostridium Difficile‐Associated Disease in U.S. Hospitals, 1993–2005. HCUP Statistical Brief #50. April 2008. Agency for Healthcare Research and Quality, Rockville, MD. Available at: http://www.hcup‐us.ahrq.gov/reports/statbriefs/sb50.pdf. Accessed April 4, 2014. , .
- National point prevalence of Clostridium difficile in US health care facility inpatients, 2008. Am J Infect Control. 2009;37(4):263–270. , , , .
- A 76‐year‐old man with recurrent Clostridium difficile‐associated diarrhea: review of C. difficile infection. JAMA. 2009;301(9):954–962. .
- Recurrent Clostridium difficile disease: epidemiology and clinical characteristics. Infect Control Hosp Epidemiol. 1999;20(1):43–50. , , , , , .
- Recurrent Clostridium difficile diarrhea: characteristics of and risk factors for patients enrolled in a prospective, randomized, double‐blinded trial. Clin Infect Dis. 1997;24(3):324–333. , , , , , .
- Narrative review: the new epidemic of Clostridium difficile‐associated enteric disease. Ann Intern Med. 2006;145(10):758–764. .
- Impact of emergency colectomy on survival of patients with fulminant Clostridium difficile colitis during an epidemic caused by a hypervirulent strain. Ann Surg. 2007;245(2):267–272. , , , et al.
- Hospital‐acquired Clostridium difficile‐associated disease in the intensive care unit setting: epidemiology, clinical course and outcome. BMC Infect Dis. 2007;7:42. , , , .
- Factors associated with prolonged symptoms and severe disease due to Clostridium difficile. Age Ageing. 1999;28(2):107–113. , , , , , .
- The effect of hospital‐acquired Clostridium difficile infection on in‐hospital mortality. Arch Intern Med. 2010;170(20):1804–1810. , , , , , .
- The effect of hospital‐acquired infection with Clostridium difficile on length of stay in hospital. CMAJ. 2012;184(1):37–42. , , , , , .
- Clostridium difficile—more difficult than ever. N Engl J Med. 2008;359(18):1932–1940. , .
- Clinical practice guidelines for Clostridium difficile infection in adults: 2010 update by the society for healthcare epidemiology of America (SHEA) and the infectious diseases society of America (IDSA). Infect Control Hosp Epidemiol. 2010;31(5):431–455. , , , et al.
- Health care costs and mortality associated with nosocomial diarrhea due to Clostridium difficile. Clin Infect Dis. 2002;34(3):346–353. , , , .
- Short‐ and long‐term attributable costs of Clostridium difficile‐associated disease in nonsurgical inpatients. Clin Infect Dis. 2008;46(4):497–504. , , , , .
- Estimation of extra hospital stay attributable to nosocomial infections: heterogeneity and timing of events. J Clin Epidemiol. 2000;53(4):409–417. , , , , .
- Attributable outcomes of endemic Clostridium difficile‐associated disease in nonsurgical patients. Emerging Infect Dis. 2008;14(7):1031–1038. , , , et al.
- Excess length of stay, charges, and mortality attributable to medical injuries during hospitalization. JAMA. 2003;290(14):1868–1874. , .
- Association of corticosteroid dose and route of administration with risk of treatment failure in acute exacerbation of chronic obstructive pulmonary disease. JAMA. 2010;303(23):2359–2367. , , , , , .
- The relationship between hospital spending and mortality in patients with sepsis. Arch Intern Med. 2011;171(4):292–299. , , , , , .
- Comparative effectiveness of macrolides and quinolones for patients hospitalized with acute exacerbations of chronic obstructive pulmonary disease (AECOPD). J Hosp Med. 2010;5(5):261–267. , , , , , .
- A combined comorbidity score predicted mortality in elderly patients better than existing scores. J Clin Epidemiol. 2011;64(7):749–759. , , , , .
- Epidemiology of severe sepsis in the United States: analysis of incidence, outcome, and associated costs of care. Crit Care Med. 2001;29(7):1303–1310. , , , , , .
- Development and validation of a model that uses enhanced administrative data to predict mortality in patients with sepsis. Crit Care Med. 2011;39(11):2425–2430. , , , et al.
- Incorporating initial treatments improves performance of a mortality prediction model for patients with sepsis. Pharmacoepidemiol Drug Saf. 2012;21(suppl 2):44–52. , , , , .
- Nosocomial infection, length of stay, and time‐dependent bias. Infect Control Hosp Epidemiol. 2009;30(3):273–276. , , , .
- Length of stay and hospital costs among high‐risk patients with hospital‐origin Clostridium difficile‐associated diarrhea. J Med Econ. 2013;16(3):440–448. , , , , , .
- Rogers. Regression standard errors in clustered samples. Stata Technical Bulletin. 1993;13(13):19–23.
- Reducing bias in a propensity score matched‐pair sample using greedy matching techniques. In: Proceedings of the 26th Annual SAS Users Group International Conference; April 22–25, 2001; Long Beach, CA. Paper 214‐26. Available at: http://www2.sas.com/proceedings/sugi26/p214‐26.pdf. Accessed April 4, 2014. .
- Prolongation of length of stay and Clostridium difficile infection: a review of the methods used to examine length of stay due to healthcare associated infections. Antimicrob Resist Infect Control. 2012;1(1):14. , .
- Clostridium difficile Infection: a multicenter study of epidemiology and outcomes in mechanically ventilated patients. Crit Care Med. 2013;41(8):1968–1975. , , , et al.
There are approximately 3 million cases of Clostridium difficile infection (CDI) per year in the United States.[1, 2, 3, 4] Of these, 10% result in a hospitalization or occur as a consequence of the exposures and treatments associated with hospitalization.[1, 2, 3, 4] Some patients with CDI experience mild diarrhea that is responsive to therapy, but other patients experience severe, life‐threatening disease that is refractory to treatment, leading to pseudomembranous colitis, toxic megacolon, and sepsis with a 60‐day mortality rate that exceeds 12%.[5, 6, 7, 8, 9, 10, 11, 12, 13, 14]
Hospital‐onset CDI (HOCDI), defined as C difficile‐associated diarrhea and related symptoms with onset more than 48 hours after admission to a healthcare facility,[15] represents a unique marriage of CDI risk factors.[5] A vulnerable patient is introduced into an environment that contains both exposure to C difficile (through other patients or healthcare workers) and treatment with antibacterial agents that may diminish normal flora. Consequently, CDI is common among hospitalized patients.[16, 17, 18] A particularly important group for understanding the burden of disease is patients who initially present to the hospital with sepsis and subsequently develop HOCDI. Sepsis patients are often critically ill and are universally treated with antibiotics.
Determining the incremental cost and mortality risk attributable to HOCDI is methodologically challenging. Because HOCDI is associated with presenting severity, the sickest patients are also the most likely to contract the disease. HOCDI is also associated with time of exposure or length of stay (LOS). Because LOS is a risk factor, comparing LOS between those with and without HOCDI will overestimate the impact if the time to diagnosis is not taken into account.[16, 17, 19, 20] We aimed to examine the impact of HOCDI in hospitalized patients with sepsis using a large, multihospital database with statistical methods that took presenting severity and time to diagnosis into account.
METHODS
Data Source and Subjects
Permission to conduct this study was obtained from the institutional review board at Baystate Medical Center. We used the Premier Healthcare Informatics database, a voluntary, fee‐supported database created to measure quality and healthcare utilization, which has been used extensively in health services research.[21, 22, 23] In addition to the elements found in hospital claims derived from the uniform billing 04 form, Premier data include an itemized, date‐stamped log of all items and services charged to the patient or their insurer, including medications, laboratory tests, and diagnostic and therapeutic services. Approximately 75% of hospitals that submit data also provide information on actual hospital costs, taken from internal cost accounting systems. The rest provide cost estimates based on Medicare cost‐to‐charge ratios. Participating hospitals are similar to the composition of acute care hospitals nationwide, although they are more commonly small‐ to midsized nonteaching facilities and are more likely to be located in the southern United States.
We included medical (nonsurgical) adult patients with sepsis who were admitted to a participating hospital between July 1, 2004 and December 31, 2010. Because we sought to focus on the care of patients who present to the hospital with sepsis, we defined sepsis as the presence of a diagnosis of sepsis plus evidence of both blood cultures and antibiotic treatment within the first 2 days of hospitalization; we used the first 2 days of hospitalization rather than just the first day because, in administrative datasets, the duration of the first hospital day includes partial days that can vary in length. We excluded patients who died or were discharged prior to day 3, because HOCDI is defined as onset after 48 hours in a healthcare facility.[15] We also excluded surviving patients who received less than 3 consecutive days of antibiotics, and patients who were transferred from or to another acute‐care facility; the latter exclusion criterion was used because we could not accurately determine the onset or subsequent course of their illness.
Identification of Patients at Risk for and Diagnosed With HOCDI
Among eligible patients with sepsis, we aimed to identify a cohort at risk for developing CDI during the hospital stay. We excluded patients: (1) with a diagnosis indicating that diarrhea was present on admission, (2) with a diagnosis of CDI that was indicated to be present on admission, (3) who were tested for CDI on the first or second hospital day, and (4) who received an antibiotic that could be consistent with treatment for CDI (oral or intravenous [IV] metronidazole or oral vancomycin) on hospital days 1 or 2.
Next, we aimed to identify sepsis patients at risk for HOCDI who developed HOCDI during their hospital stay. Among eligible patients described above, we considered a patient to have HOCDI if they had an International Classification of Diseases, Ninth Revision, Clinical Modification diagnosis of CDI (primary or secondary but not present on admission), plus evidence of testing for CDI after hospital day 2, and treatment with oral vancomycin or oral or IV metronidazole that was started after hospital day 2 and within 2 days of the C difficile test, and evidence of treatment for CDI for at least 3 days unless the patient was discharged or died.
Patient Information
We recorded patient age, gender, marital status, insurance status, race, and ethnicity. Using software provided by the Healthcare Costs and Utilization Project of the Agency for Healthcare Research and Quality, we categorized information on 30 comorbid conditions. We also created a single numerical comorbidity score based on a previously published and validated combined comorbidity score that predicts 1‐year mortality.[24] Based on a previously described algorithm,[25] we used diagnosis codes to assess the source (lung, abdomen, urinary tract, blood, other) and type of sepsis (Gram positive, Gram negative, mixed, anaerobic, fungal). Because patients can have more than 1 potential source of sepsis (eg, pneumonia and urinary tract infection) and more than 1 organism causing infection (eg, urine with Gram negative rods and blood culture with Gram positive cocci), these categories are not mutually exclusive (see Supporting Table 1 in the online version of this article). We used billing codes to identify the use of therapies, monitoring devices, and pharmacologic treatments to characterize both initial severity of illness and severity at the time of CDI diagnosis. These therapies are included in a validated sepsis mortality prediction model (designed for administrative datasets) with similar discrimination and calibration to clinical intensive care unit (ICU) risk‐adjustment models such as the mortality probability model, version III.[26, 27]
Outcomes
Our primary outcome of interest was in‐hospital mortality. Secondary outcomes included LOS and costs for survivors only and for all patients.
Statistical Methods
We calculated patient‐level summary statistics for all patients using frequencies for binary variables and medians and interquartile percentiles for continuous variables. P values <0.05 were considered statistically significant.
To account for presenting severity and time to diagnosis, we used methods that have been described elsewhere.[12, 13, 18, 20, 28] First, we identified patients who were eligible to develop HOCDI. Second, for all eligible patients, we identified a date of disease onset (index date). For patients who met criteria for HOCDI, this was the date on which the patient was tested for CDI. For eligible patients without disease, this was a date randomly assigned to any time during the hospital stay.[29] Next, we developed a nonparsimonious propensity score model that included all patient characteristics (demographics, comorbidities, sepsis source, and severity of illness on presentation and on the index date; all variables listed in Table 1 were included in the propensity model). Some of the variables for this model (eg, mechanical ventilation and vasopressors) were derived from a validated severity model.[26] We adjusted for correlation within hospital when creating the propensity score using Huber‐White robust standard error estimators clustered at the hospital level.[30] We then created matched pairs with the same LOS prior to the index date and similar propensity for developing CDI. We first matched on index date, and then, within each index‐datematched subset, matched patients with and without HOCDI by their propensity score using a 5‐to‐1 greedy match algorithm.[31] We used the differences in LOS between the cases and controls after the index date to calculate the additional attributable LOS estimates; we also separately estimated the impact on cost and LOS in a group limited to those who survived after discharge because of concerns that death could shorten LOS and reduce costs.
Before Matching | After Matching | |||||
---|---|---|---|---|---|---|
HOCDI, n=2,368, % | No CDI, n=216,547, % | P | HOCDI, n=2,368, % | No CDI, n=2,368, % | P | |
| ||||||
Age, y | 70.9 (15.1) | 68.6 (16.8) | <0.01 | 70.9 (15.1) | 69.8 (15.9) | 0.02 |
Male | 46.8 | 46.0 | 0.44 | 46.8 | 47.2 | 0.79 |
Race | ||||||
White | 61.0 | 63.3 | 61.0 | 58.1 | ||
Black | 15.6 | 14.5 | <0.01 | 15.6 | 17.0 | 0.11 |
Hispanic | 3.2 | 5.4 | 3.2 | 4.1 | ||
Other race | 20.2 | 16.8 | 20.2 | 20.9 | ||
Marital status | ||||||
Married | 31.6 | 36.3 | <0.01 | 31.6 | 32.6 | 0.74 |
Single/divorced | 52.8 | 51.1 | 52.8 | 52.0 | ||
Other/unknown | 15.7 | 12.6 | 15.7 | 14.5 | ||
Insurance status | ||||||
Medicare traditional | 63.2 | 59.5 | 63.2 | 60.3 | ||
Medicare managed | 10.6 | 10.1 | 10.6 | 10.9 | ||
Medicaid traditional | 7.6 | 6.9 | 7.6 | 8.2 | ||
Medicaid managed | 1.8 | 2.0 | <0.01 | 1.8 | 1.8 | 0.50 |
Managed care | 10.8 | 12.3 | 10.8 | 12.0 | ||
Commercial | 2.0 | 3.5 | 2.0 | 2.2 | ||
Self‐pay/other/unknown | 4.0 | 5.7 | 4.0 | 4.7 | ||
Infection source | ||||||
Respiratory | 46.5 | 37.0 | <0.01 | 46.5 | 49.6 | 0.03 |
Skin/bone | 10.1 | 8.6 | 0.01 | 10.1 | 11.2 | 0.21 |
Urinary | 52.2 | 51.3 | 0.38 | 52.2 | 50.3 | 0.18 |
Blood | 11.1 | 15.1 | <0.01 | 11.1 | 11.5 | 0.65 |
Infecting organism | ||||||
Gram negative | 35.0 | 36.6 | <0.01 | 35.0 | 33.1 | 0.18 |
Anaerobe | 1.4 | 0.7 | <0.01 | 1.4 | 1.1 | 0.24 |
Fungal | 17.5 | 7.5 | <0.01 | 17.5 | 18.3 | 0.44 |
Most common comorbid conditions | ||||||
Congestive heart failure | 35.1 | 24.6 | <0.01 | 35.1 | 37.5 | 0.06 |
Chronic lung disease | 31.6 | 27.6 | <0.01 | 31.6 | 32.1 | 0.71 |
Hypertension | 31.5 | 37.7 | <0.01 | 31.5 | 29.7 | 0.16 |
Renal Failure | 29.7 | 23.8 | <0.01 | 29.7 | 31.2 | 0.28 |
Weight Loss | 27.7 | 13.3 | <0.01 | 27.7 | 29.4 | 0.17 |
Treatments by day 2 | ||||||
ICU admission | 40.0 | 29.5 | <0.01 | 40.0 | 40.7 | 0.64 |
Use of bicarbonate | 12.2 | 7.1 | <0.01 | 12.2 | 13.6 | 0.15 |
Fresh frozen plasma | 1.4 | 1.0 | 0.03 | 1.4 | 1.1 | 0.36 |
Inotropes | 1.4 | 0.9 | 0.01 | 1.4 | 2.2 | 0.04 |
Hydrocortisone | 6.7 | 4.7 | <0.01 | 6.7 | 7.4 | 0.33 |
Thiamine | 4.2 | 3.3 | 0.01 | 4.2 | 4.1 | 0.83 |
Psychotropics (eg, haldol for delirium) | 10.0 | 9.2 | 0.21 | 10.0 | 10.8 | 0.36 |
Restraints (eg, for delirium) | 2.0 | 1.5 | 0.05 | 2.0 | 2.5 | 0.29 |
Angiotensin‐converting enzyme inhibitors | 12.1 | 13.2 | 0.12 | 12.1 | 10.9 | 0.20 |
Statins | 18.8 | 21.1 | 0.01 | 18.8 | 16.9 | 0.09 |
Drotrecogin alfa | 0.6 | 0.3 | 0.00 | 0.6 | 0.6 | 0.85 |
Foley catheter | 19.2 | 19.8 | 0.50 | 19.2 | 22.0 | 0.02 |
Diuretics | 28.5 | 25.4 | 0.01 | 28.5 | 29.6 | 0.42 |
Red blood cells | 15.5 | 10.6 | <0.01 | 15.5 | 15.8 | 0.81 |
Calcium channel blockers | 19.3 | 16.8 | 0.01 | 19.3 | 19.1 | 0.82 |
‐Blockers | 32.7 | 29.6 | 0.01 | 32.7 | 30.6 | 0.12 |
Proton pump inhibitors | 59.6 | 53.1 | <0.01 | 59.6 | 61.0 | 0.31 |
Analysis Across Clinical Subgroups
In a secondary analysis, we examined heterogeneity in the association between HOCDI and outcomes within subsets of patients defined by age, combined comorbidity score, and admission to the ICU by day 2. We created separate propensity scores using the same covariates in the primary analysis, but limited matches to within these subsets. For each group, we examined how the covariates in the HOCDI and control groups differed after matching with inference tests that took the paired nature of the data into account. All analyses were carried out using Stata/SE 11.1 (StataCorp, College Station, TX).
RESULTS
We identified 486,943 adult sepsis admissions to a Premier hospital between July 1, 2004 and December 31, 2010. After applying all exclusion criteria, we had a final sample of 218,915 admissions with sepsis (from 400 hospitals) at risk for HOCDI (Figure 1). Of these, 2368 (1.08%) met criteria for diagnosis of CDI after hospital day 2 and were matched to controls using index date and propensity score.
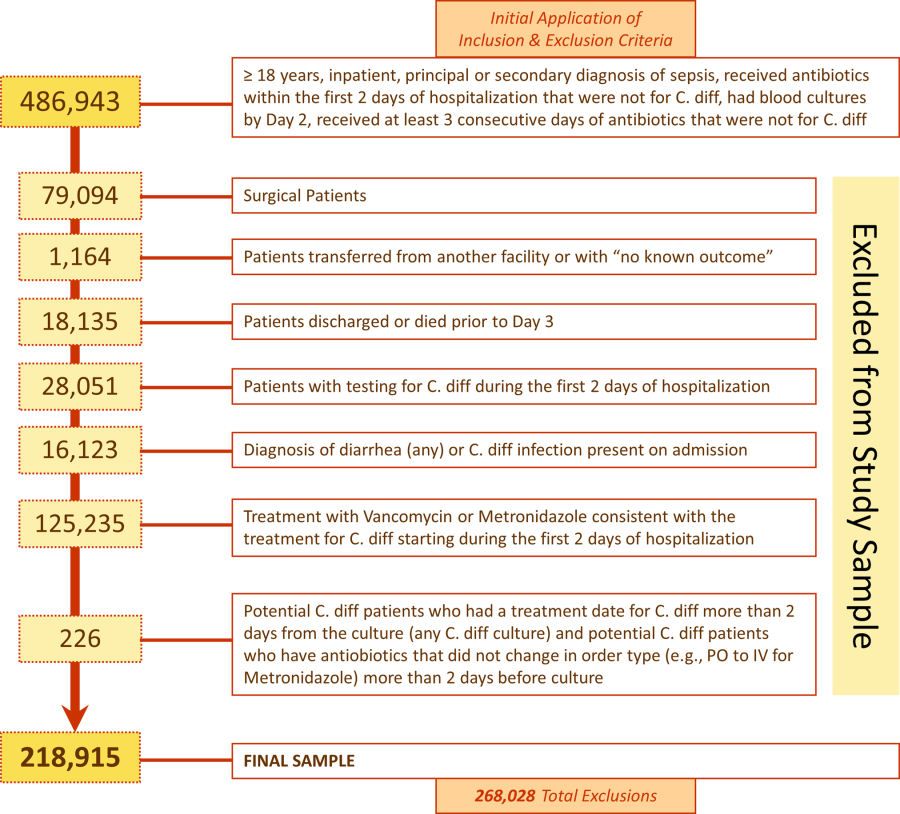
Patient and Hospital Factors
After matching, the median age was 71 years in cases and 70 years in controls (Table 1). Less than half (46%) of the population was male. Most cases (61%) and controls (58%) were white. Heart failure, hypertension, chronic lung disease, renal failure, and weight loss were the most common comorbid conditions. Our propensity model, which had a C statistic of 0.75, identified patients whose risk varied from a mean of 0.1% in the first decile to a mean of 3.8% in the tenth decile. Before matching, 40% of cases and 29% of controls were treated in the ICU by hospital day 2; after matching, 40% of both cases and controls were treated in the ICU by hospital day 2.
Distribution by LOS, Index Day, and Risk for Mortality
The unadjusted and unmatched LOS was longer for cases than controls (19 days vs 8 days, Table 2) (see Supporting Figure 1 in the online version of this article). Approximately 90% of the patients had an index day of 14 or less (Figure 2). Among patients both with and without CDI, the unadjusted mortality risk increased as the index day (and thus the total LOS) increased.
Outcome | HOCDI | No HOCDI | Difference (95% CI) | P |
---|---|---|---|---|
| ||||
Length of stay, d | ||||
Raw results | 19.2 | 8.3 | 8.4 (8.48.5) | <0.01 |
Raw results for survivors only | 18.6 | 8.0 | 10.6 (10.311.0) | <0.01 |
Matched results | 19.2 | 14.2 | 5.1(4.45.7) | <0.01 |
Matched results for survivors only | 18.6 | 13.6 | 5.1 (4.45.8) | <0.01 |
Mortality, % | ||||
Raw results | 24.0 | 10.1 | 13.9 (12.615.1), RR=2.4 (2.22.5) | <0.01 |
Matched results | 24.0 | 15.4 | 8.6 (6.410.9), RR=1.6 (1.41.8) | <0.01 |
Costs, US$ | ||||
Raw results median costs [interquartile range] | $26,187 [$15,117$46,273] | $9,988 [$6,296$17,351] | $16,190 ($15,826$16,555) | <0.01 |
Raw results for survivors only [interquartile range] | $24,038 [$14,169$41,654] | $9,429 [$6,070$15,875] | $14,620 ($14,246$14,996) | <0.01 |
Matched results [interquartile range] | $26,187 [$15,117$46,273] | $19,160 [$12,392$33,777] | $5,308 ($4,521$6,108) | |
Matched results for survivors only [interquartile range] | $24,038 [$14,169$41,654] | $17,811 [$11,614$29,298] | $4,916 ($4,088$5,768) | <0.01 |
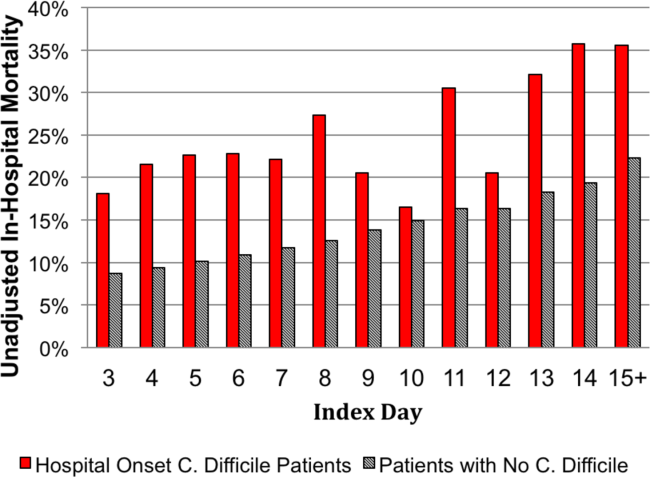
Adjusted Results
Compared to patients without disease, HOCDI patients had an increased unadjusted mortality (24% vs 10%, P<0.001). This translates into a relative risk of 2.4 (95% confidence interval [CI]: 2.2, 2.5). In the matched cohort, the difference in the mortality rates was attenuated, but still significantly higher in the HOCDI patients (24% versus 15%, P<0.001, an absolute difference of 9%; 95% CI: 6.410.8). The adjusted relative risk of mortality for HOCDI was 1.6 (95% CI: 1.41.8; Table 2). After matching, patients with CDI had a LOS of 19.2 days versus 14.2 days in matched controls (difference of 5.1 days; 95% CI: 4.45.7; P<0.001). When the LOS analysis was limited to survivors only, this difference of 5 days remained (P<0.001). In an analysis limited to survivors only, the difference in median costs between cases and controls was $4916 (95% CI: $4088$5768; P<0.001). In a secondary analysis examining heterogeneity between HOCDI and outcomes across clinical subgroups, the absolute difference in mortality and costs between cases and controls varied across demographics, comorbidity, and ICU admission, but the relative risks were similar (Figure 3) (see Supporting Figure 3 in the online version of this article).
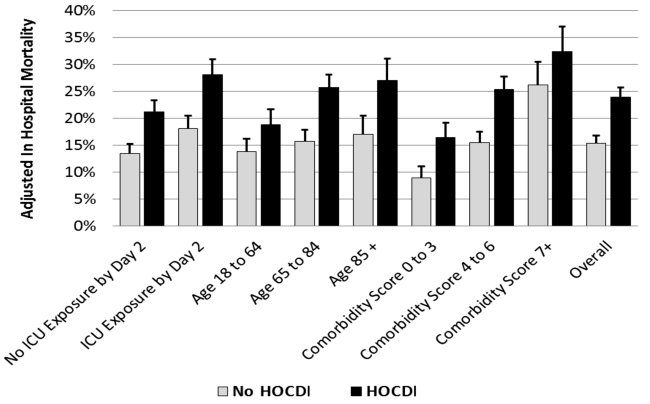
DISCUSSION
In this large cohort of patients with sepsis, we found that approximately 1 in 100 patients with sepsis developed HOCDI. Even after matching with controls based on the date of symptom onset and propensity score, patients who developed HOCDI were more than 1.6 times more likely to die in the hospital. HOCDI also added 5 days to the average hospitalization for patients with sepsis and increased median costs by approximately $5000. These findings suggest that a hospital that prevents 1 case of HOCDI per month in sepsis patients could avoid 1 death and 60 inpatient days annually, achieving an approximate yearly savings of $60,000.
Until now, the incremental cost and mortality attributable to HOCDI in sepsis patients have been poorly understood. Attributing outcomes can be methodologically challenging because patients who are at greatest risk for poor outcomes are the most likely to contract the disease and are at risk for longer periods of time. Therefore, it is necessary to take into account differences in severity of illness and time at risk between diseased and nondiseased populations and to ensure that outcomes attributed to the disease occur after disease onset.[28, 32] The majority of prior studies examining the impact of CDI on hospitalized patients have been limited by a lack of adequate matching to controls, small sample size, or failure to take into account time to infection.[16, 17, 19, 20]
A few studies have taken into account severity, time to infection, or both in estimating the impact of HOCDI. Using a time‐dependent Cox model that accounted for time to infection, Micek et al. found no difference in mortality but a longer LOS in mechanically ventilated patients (not limited to sepsis) with CDI.[33] However, their study was conducted at only 3 centers, did not take into account severity at the time of diagnosis, and did not clearly distinguish between community‐onset CDI and HOCDI. Oake et al. and Forster et al. examined the impact of CDI on patients hospitalized in a 2‐hospital health system in Canada.[12, 13] Using the baseline mortality estimate in a Cox multivariate proportional hazards regression model that accounted for the time‐varying nature of CDI, they found that HOCDI increased absolute risk of death by approximately 10%. Also, notably similar to our study were their findings that HOCDI occurred in approximately 1 in 100 patients and that the attributable median increase in LOS due to hospital‐onset CDI was 6 days. Although methodologically rigorous, these 2 small studies did not assess the impact of CDI on costs of care, were not focused on sepsis patients or even patients who received antibiotics, and also did not clearly distinguish between community‐onset CDI and HOCDI.
Our study therefore has important strengths. It is the first to examine the impact of HOCDI, including costs, on the outcomes of patients hospitalized with sepsis. The fact that we took into account both time to diagnosis and severity at the time of diagnosis (by using an index date for both cases and controls and determining severity on that date) prevented us from overestimating the impact of HOCDI on outcomes. The large differences in outcomes we observed in unadjusted and unmatched data were tempered after multivariate adjustment (eg, difference in LOS from 10.6 days to 5.1 additional days, costs from $14,620 to $4916 additional costs after adjustment). Our patient sample was derived from a large, multihospital database that contains actual hospital costs as derived from internal accounting systems. The fact that our study used data from hundreds of hospitals means that our estimates of cost, LOS, and mortality may be more generalizable than the work of Micek et al., Oake et al., and Forster et al.
This work also has important implications. First, hospital administrators, clinicians, and researchers can use our results to evaluate the cost‐effectiveness of HOCDI prevention measures (eg, hand hygiene programs, antibiotic stewardship). By quantifying the cost per case in sepsis patients, we allow administrators and researchers to compare the incremental costs of HOCDI prevention programs to the dollars and lives saved due to prevention efforts. Second, we found that our propensity model identified patients whose risk varied greatly. This suggests that an opportunity exists to identify subgroups of patients that are at highest risk. Identifying high‐risk subgroups will allow for targeted risk reduction interventions and the opportunity to reduce transmission (eg, by placing high‐risk patients in a private room). Finally, we have reaffirmed that time to diagnosis and presenting severity need to be rigorously addressed prior to making estimates of the impact of CDI burden and other hospital‐acquired conditions and injuries.
There are limitations to this study as well. We did not have access to microbiological data. However, we required a diagnosis code of CDI, evidence of testing, and treatment after the date of testing to confirm a diagnosis. We also adopted detailed exclusion criteria to ensure that CDI that was not present on admission and that controls did not have CDI. These stringent inclusion and exclusion criteria strengthened the internal validity of our estimates of disease impact. We used administrative claims data, which limited our ability to adjust for severity. However, the detailed nature of the database allowed us to use treatments, such as vasopressors and antibiotics, to identify cases; treatments were also used as a validated indicator of severity,[26] which may have helped to reduce some of this potential bias. Although our propensity model included many predictors of CDI, such as use of proton pump inhibitors and factors associated with mortality, not every confounder was completely balanced after propensity matching, although the statistical differences may have been related to our large sample size and therefore might not be clinically significant. We also may have failed to include all possible predictors of CDI in the propensity model.
In a large, diverse cohort of hospitalized patients with sepsis, we found that HOCDI lengthened hospital stay by approximately 5 days, increased risk of in‐hospital mortality by 9%, and increased hospital cost by approximately $5000 per patient. These findings highlight the importance of identifying effective prevention measures and of determining the patient populations at greatest risk for HOCDI.
Disclosures: The study was conducted with funding from the Division of Critical Care and the Center for Quality of Care Research at Baystate Medical Center. Dr. Lagu is supported by the National Heart, Lung, and Blood Institute of the National Institutes of Health under award number K01HL114745. Dr. Stefan is supported by the National Heart, Lung, and Blood Institute of the National Institutes of Health under award number K01HL114631. Drs. Lagu and Lindenauer had full access to all of the data in the study; they take responsibility for the integrity of the data and the accuracy of the data analysis. Drs. Lagu, Lindenauer, Steingrub, Higgins, Stefan, Haessler, and Rothberg conceived of the study. Dr. Lindenauer acquired the data. Drs. Lagu, Lindenauer, Rothberg, Steingrub, Nathanson, Stefan, Haessler, Higgins, and Mr. Hannon analyzed and interpreted the data. Dr. Lagu drafted the manuscript. Drs. Lagu, Lindenauer, Rothberg, Steingrub, Nathanson, Stefan, Haessler, Higgins, and Mr. Hannon critically reviewed the manuscript for important intellectual content. Dr. Nathanson carried out the statistical analyses. Dr. Nathanson, through his company OptiStatim LLC, was paid by the investigators with funding from the Department of Medicine at Baystate Medical Center to assist in conducting the statistical analyses in this study. The authors report no further conflicts of interest.
There are approximately 3 million cases of Clostridium difficile infection (CDI) per year in the United States.[1, 2, 3, 4] Of these, 10% result in a hospitalization or occur as a consequence of the exposures and treatments associated with hospitalization.[1, 2, 3, 4] Some patients with CDI experience mild diarrhea that is responsive to therapy, but other patients experience severe, life‐threatening disease that is refractory to treatment, leading to pseudomembranous colitis, toxic megacolon, and sepsis with a 60‐day mortality rate that exceeds 12%.[5, 6, 7, 8, 9, 10, 11, 12, 13, 14]
Hospital‐onset CDI (HOCDI), defined as C difficile‐associated diarrhea and related symptoms with onset more than 48 hours after admission to a healthcare facility,[15] represents a unique marriage of CDI risk factors.[5] A vulnerable patient is introduced into an environment that contains both exposure to C difficile (through other patients or healthcare workers) and treatment with antibacterial agents that may diminish normal flora. Consequently, CDI is common among hospitalized patients.[16, 17, 18] A particularly important group for understanding the burden of disease is patients who initially present to the hospital with sepsis and subsequently develop HOCDI. Sepsis patients are often critically ill and are universally treated with antibiotics.
Determining the incremental cost and mortality risk attributable to HOCDI is methodologically challenging. Because HOCDI is associated with presenting severity, the sickest patients are also the most likely to contract the disease. HOCDI is also associated with time of exposure or length of stay (LOS). Because LOS is a risk factor, comparing LOS between those with and without HOCDI will overestimate the impact if the time to diagnosis is not taken into account.[16, 17, 19, 20] We aimed to examine the impact of HOCDI in hospitalized patients with sepsis using a large, multihospital database with statistical methods that took presenting severity and time to diagnosis into account.
METHODS
Data Source and Subjects
Permission to conduct this study was obtained from the institutional review board at Baystate Medical Center. We used the Premier Healthcare Informatics database, a voluntary, fee‐supported database created to measure quality and healthcare utilization, which has been used extensively in health services research.[21, 22, 23] In addition to the elements found in hospital claims derived from the uniform billing 04 form, Premier data include an itemized, date‐stamped log of all items and services charged to the patient or their insurer, including medications, laboratory tests, and diagnostic and therapeutic services. Approximately 75% of hospitals that submit data also provide information on actual hospital costs, taken from internal cost accounting systems. The rest provide cost estimates based on Medicare cost‐to‐charge ratios. Participating hospitals are similar to the composition of acute care hospitals nationwide, although they are more commonly small‐ to midsized nonteaching facilities and are more likely to be located in the southern United States.
We included medical (nonsurgical) adult patients with sepsis who were admitted to a participating hospital between July 1, 2004 and December 31, 2010. Because we sought to focus on the care of patients who present to the hospital with sepsis, we defined sepsis as the presence of a diagnosis of sepsis plus evidence of both blood cultures and antibiotic treatment within the first 2 days of hospitalization; we used the first 2 days of hospitalization rather than just the first day because, in administrative datasets, the duration of the first hospital day includes partial days that can vary in length. We excluded patients who died or were discharged prior to day 3, because HOCDI is defined as onset after 48 hours in a healthcare facility.[15] We also excluded surviving patients who received less than 3 consecutive days of antibiotics, and patients who were transferred from or to another acute‐care facility; the latter exclusion criterion was used because we could not accurately determine the onset or subsequent course of their illness.
Identification of Patients at Risk for and Diagnosed With HOCDI
Among eligible patients with sepsis, we aimed to identify a cohort at risk for developing CDI during the hospital stay. We excluded patients: (1) with a diagnosis indicating that diarrhea was present on admission, (2) with a diagnosis of CDI that was indicated to be present on admission, (3) who were tested for CDI on the first or second hospital day, and (4) who received an antibiotic that could be consistent with treatment for CDI (oral or intravenous [IV] metronidazole or oral vancomycin) on hospital days 1 or 2.
Next, we aimed to identify sepsis patients at risk for HOCDI who developed HOCDI during their hospital stay. Among eligible patients described above, we considered a patient to have HOCDI if they had an International Classification of Diseases, Ninth Revision, Clinical Modification diagnosis of CDI (primary or secondary but not present on admission), plus evidence of testing for CDI after hospital day 2, and treatment with oral vancomycin or oral or IV metronidazole that was started after hospital day 2 and within 2 days of the C difficile test, and evidence of treatment for CDI for at least 3 days unless the patient was discharged or died.
Patient Information
We recorded patient age, gender, marital status, insurance status, race, and ethnicity. Using software provided by the Healthcare Costs and Utilization Project of the Agency for Healthcare Research and Quality, we categorized information on 30 comorbid conditions. We also created a single numerical comorbidity score based on a previously published and validated combined comorbidity score that predicts 1‐year mortality.[24] Based on a previously described algorithm,[25] we used diagnosis codes to assess the source (lung, abdomen, urinary tract, blood, other) and type of sepsis (Gram positive, Gram negative, mixed, anaerobic, fungal). Because patients can have more than 1 potential source of sepsis (eg, pneumonia and urinary tract infection) and more than 1 organism causing infection (eg, urine with Gram negative rods and blood culture with Gram positive cocci), these categories are not mutually exclusive (see Supporting Table 1 in the online version of this article). We used billing codes to identify the use of therapies, monitoring devices, and pharmacologic treatments to characterize both initial severity of illness and severity at the time of CDI diagnosis. These therapies are included in a validated sepsis mortality prediction model (designed for administrative datasets) with similar discrimination and calibration to clinical intensive care unit (ICU) risk‐adjustment models such as the mortality probability model, version III.[26, 27]
Outcomes
Our primary outcome of interest was in‐hospital mortality. Secondary outcomes included LOS and costs for survivors only and for all patients.
Statistical Methods
We calculated patient‐level summary statistics for all patients using frequencies for binary variables and medians and interquartile percentiles for continuous variables. P values <0.05 were considered statistically significant.
To account for presenting severity and time to diagnosis, we used methods that have been described elsewhere.[12, 13, 18, 20, 28] First, we identified patients who were eligible to develop HOCDI. Second, for all eligible patients, we identified a date of disease onset (index date). For patients who met criteria for HOCDI, this was the date on which the patient was tested for CDI. For eligible patients without disease, this was a date randomly assigned to any time during the hospital stay.[29] Next, we developed a nonparsimonious propensity score model that included all patient characteristics (demographics, comorbidities, sepsis source, and severity of illness on presentation and on the index date; all variables listed in Table 1 were included in the propensity model). Some of the variables for this model (eg, mechanical ventilation and vasopressors) were derived from a validated severity model.[26] We adjusted for correlation within hospital when creating the propensity score using Huber‐White robust standard error estimators clustered at the hospital level.[30] We then created matched pairs with the same LOS prior to the index date and similar propensity for developing CDI. We first matched on index date, and then, within each index‐datematched subset, matched patients with and without HOCDI by their propensity score using a 5‐to‐1 greedy match algorithm.[31] We used the differences in LOS between the cases and controls after the index date to calculate the additional attributable LOS estimates; we also separately estimated the impact on cost and LOS in a group limited to those who survived after discharge because of concerns that death could shorten LOS and reduce costs.
Before Matching | After Matching | |||||
---|---|---|---|---|---|---|
HOCDI, n=2,368, % | No CDI, n=216,547, % | P | HOCDI, n=2,368, % | No CDI, n=2,368, % | P | |
| ||||||
Age, y | 70.9 (15.1) | 68.6 (16.8) | <0.01 | 70.9 (15.1) | 69.8 (15.9) | 0.02 |
Male | 46.8 | 46.0 | 0.44 | 46.8 | 47.2 | 0.79 |
Race | ||||||
White | 61.0 | 63.3 | 61.0 | 58.1 | ||
Black | 15.6 | 14.5 | <0.01 | 15.6 | 17.0 | 0.11 |
Hispanic | 3.2 | 5.4 | 3.2 | 4.1 | ||
Other race | 20.2 | 16.8 | 20.2 | 20.9 | ||
Marital status | ||||||
Married | 31.6 | 36.3 | <0.01 | 31.6 | 32.6 | 0.74 |
Single/divorced | 52.8 | 51.1 | 52.8 | 52.0 | ||
Other/unknown | 15.7 | 12.6 | 15.7 | 14.5 | ||
Insurance status | ||||||
Medicare traditional | 63.2 | 59.5 | 63.2 | 60.3 | ||
Medicare managed | 10.6 | 10.1 | 10.6 | 10.9 | ||
Medicaid traditional | 7.6 | 6.9 | 7.6 | 8.2 | ||
Medicaid managed | 1.8 | 2.0 | <0.01 | 1.8 | 1.8 | 0.50 |
Managed care | 10.8 | 12.3 | 10.8 | 12.0 | ||
Commercial | 2.0 | 3.5 | 2.0 | 2.2 | ||
Self‐pay/other/unknown | 4.0 | 5.7 | 4.0 | 4.7 | ||
Infection source | ||||||
Respiratory | 46.5 | 37.0 | <0.01 | 46.5 | 49.6 | 0.03 |
Skin/bone | 10.1 | 8.6 | 0.01 | 10.1 | 11.2 | 0.21 |
Urinary | 52.2 | 51.3 | 0.38 | 52.2 | 50.3 | 0.18 |
Blood | 11.1 | 15.1 | <0.01 | 11.1 | 11.5 | 0.65 |
Infecting organism | ||||||
Gram negative | 35.0 | 36.6 | <0.01 | 35.0 | 33.1 | 0.18 |
Anaerobe | 1.4 | 0.7 | <0.01 | 1.4 | 1.1 | 0.24 |
Fungal | 17.5 | 7.5 | <0.01 | 17.5 | 18.3 | 0.44 |
Most common comorbid conditions | ||||||
Congestive heart failure | 35.1 | 24.6 | <0.01 | 35.1 | 37.5 | 0.06 |
Chronic lung disease | 31.6 | 27.6 | <0.01 | 31.6 | 32.1 | 0.71 |
Hypertension | 31.5 | 37.7 | <0.01 | 31.5 | 29.7 | 0.16 |
Renal Failure | 29.7 | 23.8 | <0.01 | 29.7 | 31.2 | 0.28 |
Weight Loss | 27.7 | 13.3 | <0.01 | 27.7 | 29.4 | 0.17 |
Treatments by day 2 | ||||||
ICU admission | 40.0 | 29.5 | <0.01 | 40.0 | 40.7 | 0.64 |
Use of bicarbonate | 12.2 | 7.1 | <0.01 | 12.2 | 13.6 | 0.15 |
Fresh frozen plasma | 1.4 | 1.0 | 0.03 | 1.4 | 1.1 | 0.36 |
Inotropes | 1.4 | 0.9 | 0.01 | 1.4 | 2.2 | 0.04 |
Hydrocortisone | 6.7 | 4.7 | <0.01 | 6.7 | 7.4 | 0.33 |
Thiamine | 4.2 | 3.3 | 0.01 | 4.2 | 4.1 | 0.83 |
Psychotropics (eg, haldol for delirium) | 10.0 | 9.2 | 0.21 | 10.0 | 10.8 | 0.36 |
Restraints (eg, for delirium) | 2.0 | 1.5 | 0.05 | 2.0 | 2.5 | 0.29 |
Angiotensin‐converting enzyme inhibitors | 12.1 | 13.2 | 0.12 | 12.1 | 10.9 | 0.20 |
Statins | 18.8 | 21.1 | 0.01 | 18.8 | 16.9 | 0.09 |
Drotrecogin alfa | 0.6 | 0.3 | 0.00 | 0.6 | 0.6 | 0.85 |
Foley catheter | 19.2 | 19.8 | 0.50 | 19.2 | 22.0 | 0.02 |
Diuretics | 28.5 | 25.4 | 0.01 | 28.5 | 29.6 | 0.42 |
Red blood cells | 15.5 | 10.6 | <0.01 | 15.5 | 15.8 | 0.81 |
Calcium channel blockers | 19.3 | 16.8 | 0.01 | 19.3 | 19.1 | 0.82 |
‐Blockers | 32.7 | 29.6 | 0.01 | 32.7 | 30.6 | 0.12 |
Proton pump inhibitors | 59.6 | 53.1 | <0.01 | 59.6 | 61.0 | 0.31 |
Analysis Across Clinical Subgroups
In a secondary analysis, we examined heterogeneity in the association between HOCDI and outcomes within subsets of patients defined by age, combined comorbidity score, and admission to the ICU by day 2. We created separate propensity scores using the same covariates in the primary analysis, but limited matches to within these subsets. For each group, we examined how the covariates in the HOCDI and control groups differed after matching with inference tests that took the paired nature of the data into account. All analyses were carried out using Stata/SE 11.1 (StataCorp, College Station, TX).
RESULTS
We identified 486,943 adult sepsis admissions to a Premier hospital between July 1, 2004 and December 31, 2010. After applying all exclusion criteria, we had a final sample of 218,915 admissions with sepsis (from 400 hospitals) at risk for HOCDI (Figure 1). Of these, 2368 (1.08%) met criteria for diagnosis of CDI after hospital day 2 and were matched to controls using index date and propensity score.
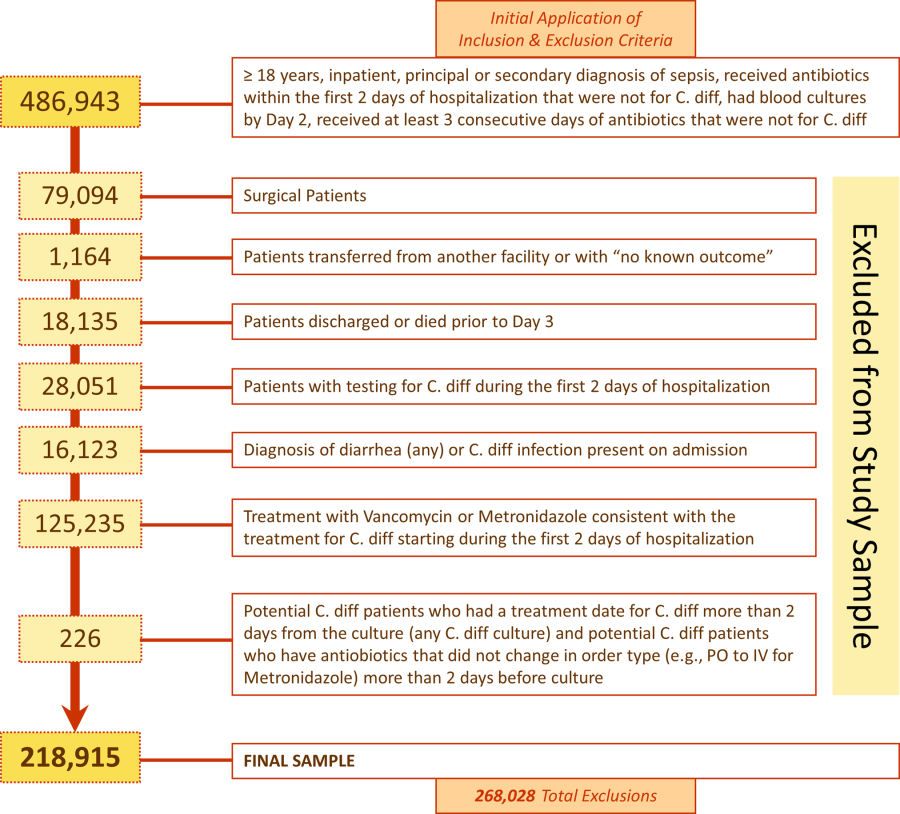
Patient and Hospital Factors
After matching, the median age was 71 years in cases and 70 years in controls (Table 1). Less than half (46%) of the population was male. Most cases (61%) and controls (58%) were white. Heart failure, hypertension, chronic lung disease, renal failure, and weight loss were the most common comorbid conditions. Our propensity model, which had a C statistic of 0.75, identified patients whose risk varied from a mean of 0.1% in the first decile to a mean of 3.8% in the tenth decile. Before matching, 40% of cases and 29% of controls were treated in the ICU by hospital day 2; after matching, 40% of both cases and controls were treated in the ICU by hospital day 2.
Distribution by LOS, Index Day, and Risk for Mortality
The unadjusted and unmatched LOS was longer for cases than controls (19 days vs 8 days, Table 2) (see Supporting Figure 1 in the online version of this article). Approximately 90% of the patients had an index day of 14 or less (Figure 2). Among patients both with and without CDI, the unadjusted mortality risk increased as the index day (and thus the total LOS) increased.
Outcome | HOCDI | No HOCDI | Difference (95% CI) | P |
---|---|---|---|---|
| ||||
Length of stay, d | ||||
Raw results | 19.2 | 8.3 | 8.4 (8.48.5) | <0.01 |
Raw results for survivors only | 18.6 | 8.0 | 10.6 (10.311.0) | <0.01 |
Matched results | 19.2 | 14.2 | 5.1(4.45.7) | <0.01 |
Matched results for survivors only | 18.6 | 13.6 | 5.1 (4.45.8) | <0.01 |
Mortality, % | ||||
Raw results | 24.0 | 10.1 | 13.9 (12.615.1), RR=2.4 (2.22.5) | <0.01 |
Matched results | 24.0 | 15.4 | 8.6 (6.410.9), RR=1.6 (1.41.8) | <0.01 |
Costs, US$ | ||||
Raw results median costs [interquartile range] | $26,187 [$15,117$46,273] | $9,988 [$6,296$17,351] | $16,190 ($15,826$16,555) | <0.01 |
Raw results for survivors only [interquartile range] | $24,038 [$14,169$41,654] | $9,429 [$6,070$15,875] | $14,620 ($14,246$14,996) | <0.01 |
Matched results [interquartile range] | $26,187 [$15,117$46,273] | $19,160 [$12,392$33,777] | $5,308 ($4,521$6,108) | |
Matched results for survivors only [interquartile range] | $24,038 [$14,169$41,654] | $17,811 [$11,614$29,298] | $4,916 ($4,088$5,768) | <0.01 |
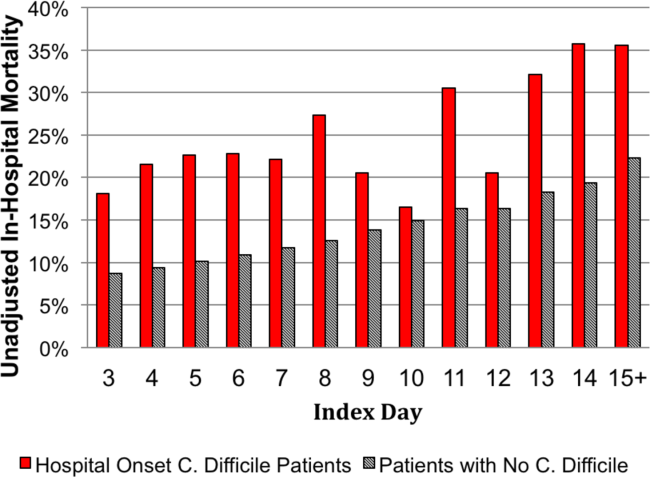
Adjusted Results
Compared to patients without disease, HOCDI patients had an increased unadjusted mortality (24% vs 10%, P<0.001). This translates into a relative risk of 2.4 (95% confidence interval [CI]: 2.2, 2.5). In the matched cohort, the difference in the mortality rates was attenuated, but still significantly higher in the HOCDI patients (24% versus 15%, P<0.001, an absolute difference of 9%; 95% CI: 6.410.8). The adjusted relative risk of mortality for HOCDI was 1.6 (95% CI: 1.41.8; Table 2). After matching, patients with CDI had a LOS of 19.2 days versus 14.2 days in matched controls (difference of 5.1 days; 95% CI: 4.45.7; P<0.001). When the LOS analysis was limited to survivors only, this difference of 5 days remained (P<0.001). In an analysis limited to survivors only, the difference in median costs between cases and controls was $4916 (95% CI: $4088$5768; P<0.001). In a secondary analysis examining heterogeneity between HOCDI and outcomes across clinical subgroups, the absolute difference in mortality and costs between cases and controls varied across demographics, comorbidity, and ICU admission, but the relative risks were similar (Figure 3) (see Supporting Figure 3 in the online version of this article).
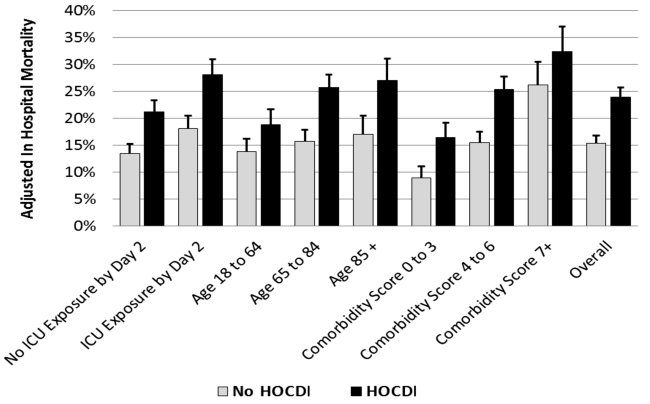
DISCUSSION
In this large cohort of patients with sepsis, we found that approximately 1 in 100 patients with sepsis developed HOCDI. Even after matching with controls based on the date of symptom onset and propensity score, patients who developed HOCDI were more than 1.6 times more likely to die in the hospital. HOCDI also added 5 days to the average hospitalization for patients with sepsis and increased median costs by approximately $5000. These findings suggest that a hospital that prevents 1 case of HOCDI per month in sepsis patients could avoid 1 death and 60 inpatient days annually, achieving an approximate yearly savings of $60,000.
Until now, the incremental cost and mortality attributable to HOCDI in sepsis patients have been poorly understood. Attributing outcomes can be methodologically challenging because patients who are at greatest risk for poor outcomes are the most likely to contract the disease and are at risk for longer periods of time. Therefore, it is necessary to take into account differences in severity of illness and time at risk between diseased and nondiseased populations and to ensure that outcomes attributed to the disease occur after disease onset.[28, 32] The majority of prior studies examining the impact of CDI on hospitalized patients have been limited by a lack of adequate matching to controls, small sample size, or failure to take into account time to infection.[16, 17, 19, 20]
A few studies have taken into account severity, time to infection, or both in estimating the impact of HOCDI. Using a time‐dependent Cox model that accounted for time to infection, Micek et al. found no difference in mortality but a longer LOS in mechanically ventilated patients (not limited to sepsis) with CDI.[33] However, their study was conducted at only 3 centers, did not take into account severity at the time of diagnosis, and did not clearly distinguish between community‐onset CDI and HOCDI. Oake et al. and Forster et al. examined the impact of CDI on patients hospitalized in a 2‐hospital health system in Canada.[12, 13] Using the baseline mortality estimate in a Cox multivariate proportional hazards regression model that accounted for the time‐varying nature of CDI, they found that HOCDI increased absolute risk of death by approximately 10%. Also, notably similar to our study were their findings that HOCDI occurred in approximately 1 in 100 patients and that the attributable median increase in LOS due to hospital‐onset CDI was 6 days. Although methodologically rigorous, these 2 small studies did not assess the impact of CDI on costs of care, were not focused on sepsis patients or even patients who received antibiotics, and also did not clearly distinguish between community‐onset CDI and HOCDI.
Our study therefore has important strengths. It is the first to examine the impact of HOCDI, including costs, on the outcomes of patients hospitalized with sepsis. The fact that we took into account both time to diagnosis and severity at the time of diagnosis (by using an index date for both cases and controls and determining severity on that date) prevented us from overestimating the impact of HOCDI on outcomes. The large differences in outcomes we observed in unadjusted and unmatched data were tempered after multivariate adjustment (eg, difference in LOS from 10.6 days to 5.1 additional days, costs from $14,620 to $4916 additional costs after adjustment). Our patient sample was derived from a large, multihospital database that contains actual hospital costs as derived from internal accounting systems. The fact that our study used data from hundreds of hospitals means that our estimates of cost, LOS, and mortality may be more generalizable than the work of Micek et al., Oake et al., and Forster et al.
This work also has important implications. First, hospital administrators, clinicians, and researchers can use our results to evaluate the cost‐effectiveness of HOCDI prevention measures (eg, hand hygiene programs, antibiotic stewardship). By quantifying the cost per case in sepsis patients, we allow administrators and researchers to compare the incremental costs of HOCDI prevention programs to the dollars and lives saved due to prevention efforts. Second, we found that our propensity model identified patients whose risk varied greatly. This suggests that an opportunity exists to identify subgroups of patients that are at highest risk. Identifying high‐risk subgroups will allow for targeted risk reduction interventions and the opportunity to reduce transmission (eg, by placing high‐risk patients in a private room). Finally, we have reaffirmed that time to diagnosis and presenting severity need to be rigorously addressed prior to making estimates of the impact of CDI burden and other hospital‐acquired conditions and injuries.
There are limitations to this study as well. We did not have access to microbiological data. However, we required a diagnosis code of CDI, evidence of testing, and treatment after the date of testing to confirm a diagnosis. We also adopted detailed exclusion criteria to ensure that CDI that was not present on admission and that controls did not have CDI. These stringent inclusion and exclusion criteria strengthened the internal validity of our estimates of disease impact. We used administrative claims data, which limited our ability to adjust for severity. However, the detailed nature of the database allowed us to use treatments, such as vasopressors and antibiotics, to identify cases; treatments were also used as a validated indicator of severity,[26] which may have helped to reduce some of this potential bias. Although our propensity model included many predictors of CDI, such as use of proton pump inhibitors and factors associated with mortality, not every confounder was completely balanced after propensity matching, although the statistical differences may have been related to our large sample size and therefore might not be clinically significant. We also may have failed to include all possible predictors of CDI in the propensity model.
In a large, diverse cohort of hospitalized patients with sepsis, we found that HOCDI lengthened hospital stay by approximately 5 days, increased risk of in‐hospital mortality by 9%, and increased hospital cost by approximately $5000 per patient. These findings highlight the importance of identifying effective prevention measures and of determining the patient populations at greatest risk for HOCDI.
Disclosures: The study was conducted with funding from the Division of Critical Care and the Center for Quality of Care Research at Baystate Medical Center. Dr. Lagu is supported by the National Heart, Lung, and Blood Institute of the National Institutes of Health under award number K01HL114745. Dr. Stefan is supported by the National Heart, Lung, and Blood Institute of the National Institutes of Health under award number K01HL114631. Drs. Lagu and Lindenauer had full access to all of the data in the study; they take responsibility for the integrity of the data and the accuracy of the data analysis. Drs. Lagu, Lindenauer, Steingrub, Higgins, Stefan, Haessler, and Rothberg conceived of the study. Dr. Lindenauer acquired the data. Drs. Lagu, Lindenauer, Rothberg, Steingrub, Nathanson, Stefan, Haessler, Higgins, and Mr. Hannon analyzed and interpreted the data. Dr. Lagu drafted the manuscript. Drs. Lagu, Lindenauer, Rothberg, Steingrub, Nathanson, Stefan, Haessler, Higgins, and Mr. Hannon critically reviewed the manuscript for important intellectual content. Dr. Nathanson carried out the statistical analyses. Dr. Nathanson, through his company OptiStatim LLC, was paid by the investigators with funding from the Department of Medicine at Baystate Medical Center to assist in conducting the statistical analyses in this study. The authors report no further conflicts of interest.
- Increasing prevalence and severity of Clostridium difficile colitis in hospitalized patients in the United States. Arch Surg. 2007;142(7):624–631; discussion 631. , , , .
- The changing epidemiology of Clostridium difficile infections. Clin Microbiol Rev. 2010;23(3):529–549. , , , et al.
- Clostridium Difficile‐Associated Disease in U.S. Hospitals, 1993–2005. HCUP Statistical Brief #50. April 2008. Agency for Healthcare Research and Quality, Rockville, MD. Available at: http://www.hcup‐us.ahrq.gov/reports/statbriefs/sb50.pdf. Accessed April 4, 2014. , .
- National point prevalence of Clostridium difficile in US health care facility inpatients, 2008. Am J Infect Control. 2009;37(4):263–270. , , , .
- A 76‐year‐old man with recurrent Clostridium difficile‐associated diarrhea: review of C. difficile infection. JAMA. 2009;301(9):954–962. .
- Recurrent Clostridium difficile disease: epidemiology and clinical characteristics. Infect Control Hosp Epidemiol. 1999;20(1):43–50. , , , , , .
- Recurrent Clostridium difficile diarrhea: characteristics of and risk factors for patients enrolled in a prospective, randomized, double‐blinded trial. Clin Infect Dis. 1997;24(3):324–333. , , , , , .
- Narrative review: the new epidemic of Clostridium difficile‐associated enteric disease. Ann Intern Med. 2006;145(10):758–764. .
- Impact of emergency colectomy on survival of patients with fulminant Clostridium difficile colitis during an epidemic caused by a hypervirulent strain. Ann Surg. 2007;245(2):267–272. , , , et al.
- Hospital‐acquired Clostridium difficile‐associated disease in the intensive care unit setting: epidemiology, clinical course and outcome. BMC Infect Dis. 2007;7:42. , , , .
- Factors associated with prolonged symptoms and severe disease due to Clostridium difficile. Age Ageing. 1999;28(2):107–113. , , , , , .
- The effect of hospital‐acquired Clostridium difficile infection on in‐hospital mortality. Arch Intern Med. 2010;170(20):1804–1810. , , , , , .
- The effect of hospital‐acquired infection with Clostridium difficile on length of stay in hospital. CMAJ. 2012;184(1):37–42. , , , , , .
- Clostridium difficile—more difficult than ever. N Engl J Med. 2008;359(18):1932–1940. , .
- Clinical practice guidelines for Clostridium difficile infection in adults: 2010 update by the society for healthcare epidemiology of America (SHEA) and the infectious diseases society of America (IDSA). Infect Control Hosp Epidemiol. 2010;31(5):431–455. , , , et al.
- Health care costs and mortality associated with nosocomial diarrhea due to Clostridium difficile. Clin Infect Dis. 2002;34(3):346–353. , , , .
- Short‐ and long‐term attributable costs of Clostridium difficile‐associated disease in nonsurgical inpatients. Clin Infect Dis. 2008;46(4):497–504. , , , , .
- Estimation of extra hospital stay attributable to nosocomial infections: heterogeneity and timing of events. J Clin Epidemiol. 2000;53(4):409–417. , , , , .
- Attributable outcomes of endemic Clostridium difficile‐associated disease in nonsurgical patients. Emerging Infect Dis. 2008;14(7):1031–1038. , , , et al.
- Excess length of stay, charges, and mortality attributable to medical injuries during hospitalization. JAMA. 2003;290(14):1868–1874. , .
- Association of corticosteroid dose and route of administration with risk of treatment failure in acute exacerbation of chronic obstructive pulmonary disease. JAMA. 2010;303(23):2359–2367. , , , , , .
- The relationship between hospital spending and mortality in patients with sepsis. Arch Intern Med. 2011;171(4):292–299. , , , , , .
- Comparative effectiveness of macrolides and quinolones for patients hospitalized with acute exacerbations of chronic obstructive pulmonary disease (AECOPD). J Hosp Med. 2010;5(5):261–267. , , , , , .
- A combined comorbidity score predicted mortality in elderly patients better than existing scores. J Clin Epidemiol. 2011;64(7):749–759. , , , , .
- Epidemiology of severe sepsis in the United States: analysis of incidence, outcome, and associated costs of care. Crit Care Med. 2001;29(7):1303–1310. , , , , , .
- Development and validation of a model that uses enhanced administrative data to predict mortality in patients with sepsis. Crit Care Med. 2011;39(11):2425–2430. , , , et al.
- Incorporating initial treatments improves performance of a mortality prediction model for patients with sepsis. Pharmacoepidemiol Drug Saf. 2012;21(suppl 2):44–52. , , , , .
- Nosocomial infection, length of stay, and time‐dependent bias. Infect Control Hosp Epidemiol. 2009;30(3):273–276. , , , .
- Length of stay and hospital costs among high‐risk patients with hospital‐origin Clostridium difficile‐associated diarrhea. J Med Econ. 2013;16(3):440–448. , , , , , .
- Rogers. Regression standard errors in clustered samples. Stata Technical Bulletin. 1993;13(13):19–23.
- Reducing bias in a propensity score matched‐pair sample using greedy matching techniques. In: Proceedings of the 26th Annual SAS Users Group International Conference; April 22–25, 2001; Long Beach, CA. Paper 214‐26. Available at: http://www2.sas.com/proceedings/sugi26/p214‐26.pdf. Accessed April 4, 2014. .
- Prolongation of length of stay and Clostridium difficile infection: a review of the methods used to examine length of stay due to healthcare associated infections. Antimicrob Resist Infect Control. 2012;1(1):14. , .
- Clostridium difficile Infection: a multicenter study of epidemiology and outcomes in mechanically ventilated patients. Crit Care Med. 2013;41(8):1968–1975. , , , et al.
- Increasing prevalence and severity of Clostridium difficile colitis in hospitalized patients in the United States. Arch Surg. 2007;142(7):624–631; discussion 631. , , , .
- The changing epidemiology of Clostridium difficile infections. Clin Microbiol Rev. 2010;23(3):529–549. , , , et al.
- Clostridium Difficile‐Associated Disease in U.S. Hospitals, 1993–2005. HCUP Statistical Brief #50. April 2008. Agency for Healthcare Research and Quality, Rockville, MD. Available at: http://www.hcup‐us.ahrq.gov/reports/statbriefs/sb50.pdf. Accessed April 4, 2014. , .
- National point prevalence of Clostridium difficile in US health care facility inpatients, 2008. Am J Infect Control. 2009;37(4):263–270. , , , .
- A 76‐year‐old man with recurrent Clostridium difficile‐associated diarrhea: review of C. difficile infection. JAMA. 2009;301(9):954–962. .
- Recurrent Clostridium difficile disease: epidemiology and clinical characteristics. Infect Control Hosp Epidemiol. 1999;20(1):43–50. , , , , , .
- Recurrent Clostridium difficile diarrhea: characteristics of and risk factors for patients enrolled in a prospective, randomized, double‐blinded trial. Clin Infect Dis. 1997;24(3):324–333. , , , , , .
- Narrative review: the new epidemic of Clostridium difficile‐associated enteric disease. Ann Intern Med. 2006;145(10):758–764. .
- Impact of emergency colectomy on survival of patients with fulminant Clostridium difficile colitis during an epidemic caused by a hypervirulent strain. Ann Surg. 2007;245(2):267–272. , , , et al.
- Hospital‐acquired Clostridium difficile‐associated disease in the intensive care unit setting: epidemiology, clinical course and outcome. BMC Infect Dis. 2007;7:42. , , , .
- Factors associated with prolonged symptoms and severe disease due to Clostridium difficile. Age Ageing. 1999;28(2):107–113. , , , , , .
- The effect of hospital‐acquired Clostridium difficile infection on in‐hospital mortality. Arch Intern Med. 2010;170(20):1804–1810. , , , , , .
- The effect of hospital‐acquired infection with Clostridium difficile on length of stay in hospital. CMAJ. 2012;184(1):37–42. , , , , , .
- Clostridium difficile—more difficult than ever. N Engl J Med. 2008;359(18):1932–1940. , .
- Clinical practice guidelines for Clostridium difficile infection in adults: 2010 update by the society for healthcare epidemiology of America (SHEA) and the infectious diseases society of America (IDSA). Infect Control Hosp Epidemiol. 2010;31(5):431–455. , , , et al.
- Health care costs and mortality associated with nosocomial diarrhea due to Clostridium difficile. Clin Infect Dis. 2002;34(3):346–353. , , , .
- Short‐ and long‐term attributable costs of Clostridium difficile‐associated disease in nonsurgical inpatients. Clin Infect Dis. 2008;46(4):497–504. , , , , .
- Estimation of extra hospital stay attributable to nosocomial infections: heterogeneity and timing of events. J Clin Epidemiol. 2000;53(4):409–417. , , , , .
- Attributable outcomes of endemic Clostridium difficile‐associated disease in nonsurgical patients. Emerging Infect Dis. 2008;14(7):1031–1038. , , , et al.
- Excess length of stay, charges, and mortality attributable to medical injuries during hospitalization. JAMA. 2003;290(14):1868–1874. , .
- Association of corticosteroid dose and route of administration with risk of treatment failure in acute exacerbation of chronic obstructive pulmonary disease. JAMA. 2010;303(23):2359–2367. , , , , , .
- The relationship between hospital spending and mortality in patients with sepsis. Arch Intern Med. 2011;171(4):292–299. , , , , , .
- Comparative effectiveness of macrolides and quinolones for patients hospitalized with acute exacerbations of chronic obstructive pulmonary disease (AECOPD). J Hosp Med. 2010;5(5):261–267. , , , , , .
- A combined comorbidity score predicted mortality in elderly patients better than existing scores. J Clin Epidemiol. 2011;64(7):749–759. , , , , .
- Epidemiology of severe sepsis in the United States: analysis of incidence, outcome, and associated costs of care. Crit Care Med. 2001;29(7):1303–1310. , , , , , .
- Development and validation of a model that uses enhanced administrative data to predict mortality in patients with sepsis. Crit Care Med. 2011;39(11):2425–2430. , , , et al.
- Incorporating initial treatments improves performance of a mortality prediction model for patients with sepsis. Pharmacoepidemiol Drug Saf. 2012;21(suppl 2):44–52. , , , , .
- Nosocomial infection, length of stay, and time‐dependent bias. Infect Control Hosp Epidemiol. 2009;30(3):273–276. , , , .
- Length of stay and hospital costs among high‐risk patients with hospital‐origin Clostridium difficile‐associated diarrhea. J Med Econ. 2013;16(3):440–448. , , , , , .
- Rogers. Regression standard errors in clustered samples. Stata Technical Bulletin. 1993;13(13):19–23.
- Reducing bias in a propensity score matched‐pair sample using greedy matching techniques. In: Proceedings of the 26th Annual SAS Users Group International Conference; April 22–25, 2001; Long Beach, CA. Paper 214‐26. Available at: http://www2.sas.com/proceedings/sugi26/p214‐26.pdf. Accessed April 4, 2014. .
- Prolongation of length of stay and Clostridium difficile infection: a review of the methods used to examine length of stay due to healthcare associated infections. Antimicrob Resist Infect Control. 2012;1(1):14. , .
- Clostridium difficile Infection: a multicenter study of epidemiology and outcomes in mechanically ventilated patients. Crit Care Med. 2013;41(8):1968–1975. , , , et al.
© 2014 Society of Hospital Medicine
Managing community-acquired pneumonia during flu season
General internists need to be able to recognize community-acquired pneumonia (CAP) so that diagnostic and therapeutic interventions can be initiated promptly. It is also important to understand the most likely and possible causes of CAP so that appropriate initial antimicrobial therapy can be chosen. Especially during flu season, influenza can present as CAP and should be included in the differential diagnosis.
When managing a patient with CAP, the internist must decide which level of care, diagnostic tests, antimicrobial agents, and follow-up plans are needed. These topics will be reviewed in this article.
TWO TERMS TO REMEMBER
- CAP refers to pneumonia acquired outside a health care facility. It can be either bacterial or viral.
- CABP (community-acquired bacterial pneumonia) refers only to those cases caused by bacterial pathogens.
NUMBERS AND TRENDS
In the United States, CAP is the number-one cause of death from infection and the sixth leading cause of death overall.1 Each year, it is responsible for about 4.2 million outpatient visits, more than 60,000 deaths, and more than $17 billion in health care expenses.2
Community-acquired bacterial pneumonia: Common, serious
In a population-based US study in 1991, the incidence of CABP requiring hospitalization was 266.8 per 100,000 people.3
Estimates of overall mortality in CABP vary depending on the severity of illness and comorbid conditions. A meta-analysis published in 1996 found the overall mortality rate to be 13.7%, with a range of 5.1% to 36.5% depending on severity.4
In hospitalized patients, mortality rates and length of hospital stay appear to be declining over time. Between 1993 and 2005, the age-adjusted mortality rate decreased from 8.9% to 4.1%, and the average length of stay decreased from 7.5 to 5.7 days, with an overall reduction in hospital cost.5
CABP is more prevalent in older people than in the general population, and it increases with age from 18.2 cases per 1,000 patient-years in patients 60 to 69 years to 52.3 cases per 1,000 patient-years in those older than 85 years.6 Risk factors for pneumonia in the elderly include heart disease, chronic lung disease, immunosuppressive drugs, alcoholism, and increasing age.7 Similar to the trend in the general population, the mortality rate in elderly CABP patients appears to be decreasing over time, possibly thanks to rising rates of pneumococcal and influenza vaccination.8
Among the general population, risk factors for developing CABP also include smoking, occupational dust exposure, history of childhood pneumonia, unemployment, and single marital status.9 The incidence of CABP does not appear to be higher among pregnant women, although it is the most frequent cause of nonobstetric death in this population.10
The use of proton pump inhibitors may be an emerging risk factor for CABP.11 Also, use of nonsteroidal anti-inflammatory drugs among patients with CABP is associated with a blunted inflammatory response as well as a higher risk of pleuropulmonary complications and a delay in presentation.12
Influenza is also common, potentially severe
Influenza is also very common and potentially severe. It can cause a spectrum of disease, from mild upper respiratory tract symptoms to severe viral pneumonia that can be life-threatening and complicated by respiratory failure and the acute respiratory distress syndrome (ARDS).
Influenza infection can also be complicated by subsequent bacterial pneumonia. However, the epidemiology of influenza infection differs from that of CABP in that influenza occurs seasonally.
In the United States, seasonal influenza causes 36,000 deaths and 200,000 hospitalizations annually.13,14 As with CABP, the risk of death from influenza increases with age: it is 16 times greater in people age 85 and older than in those ages 65 to 69.13
During yearly seasonal epidemics, those at the highest risk of hospitalization and death are at the extremes of age. Risk factors for complicated influenza include heart disease, lung disease, diabetes, renal failure, rheumatologic conditions, dementia, and neurologic disease.15,16 During the 2009 H1N1 influenza pandemic, unexpected severity was seen in previously healthy young adults as well as those with obesity, neurodegenerative disease, pregnancy, and asthma.17
PATHOGENS: TYPICAL, ATYPICAL, VIRAL
Identifying the etiologic organism in CAP is confounded by limitations in the available diagnostic tests and also by poor-quality specimens that often are contaminated with bacteria that colonize the upper airways. Given these caveats, the primary pathogens responsible for CAP broadly include typical bacterial pathogens, atypical bacterial pathogens, and viruses.
Typical bacterial pathogens include Streptococcus pneumoniae, Haemophilus influenzae, Staphylococcus aureus, Moraxella catarrhalis, and, less commonly, a variety of aerobic and anaerobic gram-negative rods including Pseudomonas aeruginosa, Acinetobacter species, and Klebsiella pneumoniae.
Atypical bacterial pathogens include Mycoplasma pneumoniae, Chlamydia pneumoniae, and Legionella species.18
Viruses implicated in adult CAP include influenza A and B, parainfluenza viruses, respiratory syncytial virus, and adenovirus.19 More recently, human metapneumovirus has been described as a cause of adult CAP.20
Clues to uncommon microbes
Specific historic features or coexisting conditions that may suggest an uncommon microbiologic diagnosis include21:
- Recent travel to the southwestern United States or Southeast Asia
- Ill contacts
- Exposure to birds, bats, rabbits, or farm animals
- Alcoholism
- Chronic obstructive pulmonary disease
- Human immunodeficiency virus infection
- Structural lung disease
- Prolonged cough with whoop or posttussive vomiting
- Aspiration
- Bioterrorism.
In cases in which one or more of these conditions exist, CAP may also be caused by other agents not listed above, including Mycobacterium tuberculosis, oral anaerobes, atypical mycobacteria, Histoplasma capsulatum, Chlamydophila psittaci, Francisella tularensis, Coxiella burnettii, Pneumocystis jiroveci, Cryptococcus, Aspergillus, Coccidioides, Hantavirus, avian influenza, Burkholderia pseudomallei, severe acute respiratory syndrome virus, Bordetella pertussis, Bacillus anthracis, and Yersinia pestis.
HOW BACTERIA INVADE THE LUNGS
The pathophysiology of CABP involves both host defense and microbial virulence factors.
The airways are most commonly exposed to microbes by microaspiration of upper airway flora, although hematogenous seeding of the lungs in a bacteremic patient or contiguous spread of infection from an adjacent site can also occur.
Mucociliary clearance and the cough reflex are important initial defenses against infection and can be inhibited by neurologic diseases and conditions that impair the mucociliary mechanism. Mucosal immune cells, including macrophages and neutrophils, recognize invading pathogens and generate an antibody response.
Regulation of the host inflammatory response to infection depends on a complex interaction between immune cells, inflammatory cytokines (eg, tumor necrosis factor alpha, interleukin 1-beta, and interleukin 6), and anti-inflammatory cytokines such as interleukin 1 receptor antagonist and soluble tumor necrosis factor receptor type I.22
The interaction and timing of the inflammatory and anti-inflammatory response are essential in manifesting an appropriate host response to infection. An inadequate inflammatory response can lead to sepsis and death, but an excessive, late anti-inflammatory response can lead to a systemic inflammatory response such as ARDS. Polymorphisms within the genes coding for these factors may explain the variation in severity of illness among patients with CABP.23
HOW INFLUENZA DOES ITS DAMAGE
There are three types of influenza virus: A, B, and C. Type A causes most human infections. The influenza A virus envelope comprises a lipid bilayer that contains the projecting glycoproteins hemagglutinin and neuraminidase. Influenza viruses are named on the basis of these proteins and are designated with an H and an N, respectively, each followed by a number referring to the subtype.
Influenza infection begins when the virus makes contact with the epithelium. Hemagglutinin binds to the host cell and allows viral entry, where it begins replication. Neuraminidase prevents viral aggregation and facilitates the release of virus from infected cells.24
Mature virions are released and spread to neighboring host cells; this process is associated with desquamation and inflammation of the airways, causing cough, rhinorrhea, and sore throat. Systemic symptoms are associated with the induction of interferon, which causes fever and myalgia.25
Recovery and immunity to influenza infection occurs through both humoral and cell-mediated immunity, with antibodies directed against the specific hemagglutinin and neuraminidase antigens of the infecting virus. Immunity wanes over time and with antigenic drift of circulating viruses, making the host susceptible to recurrent influenza infection.24
Influenza is often complicated by bacterial superinfection
The influenza virus acts synergistically with certain bacteria to increase infectivity, and this may explain why influenza is often complicated by bacterial superinfection.
Mechanisms leading to bacterial superinfection include increased binding and invasion of bacteria, increased viral replication, and modification of the host inflammatory response. Some S aureus strains produce a protease that directly activates influenza virus hemagglutinin; other bacteria can activate plasminogen to promote influenza replication. The resulting increase in proteases in host tissues promotes activation of influenza through cleavage of hemagglutinin.26
The influenza virus also causes damage to the airway epithelial layer, leading to increased exposure of the binding sites necessary for adherence of S pneumoniae.27
CLINICAL PRESENTATION OF COMMUNITY-ACQUIRED PNEUMONIA
Although CAP is common, agreement on its essential clinical signs and symptoms is surprisingly limited, due in part to heterogeneous patient presentations and in part to interobserver variability. The reader is referred to two excellent reviews on this topic.28,29
The diagnosis of CAP is made on clinical grounds, based on a combination of signs and symptoms. Symptoms of pneumonia can include cough, fever, chills, sputum production, dyspnea, and pleuritic pain. Physical findings can include tachypnea, tachycardia, hypoxemia, and consolidation or rales on auscultation. Laboratory data may show leukocytosis or elevated C-reactive protein, and radiographic studies may show evidence of a new infiltrate.21,30,31
Clinical presentation of influenza
Seasonal influenza as a cause of CAP is difficult to distinguish from bacterial causes. The clinical presentation of seasonal influenza most commonly includes fever or subjective feverishness, cough, myalgia, and weakness.32 In a recent multivariate analysis, five clinical features were shown to be predictive of pandemic H1N1 influenza pneumonia rather than CABP: age younger than 65 years, absence of confusion, white blood cell count less than 12 × 109/L, temperature higher than 38°C (100.4°F), and bilateral opacities on radiography.32,33
Complicated influenza infection can be either primary viral pneumonia or bacterial superinfection.
During the 1918 influenza pandemic, which predated the ability to isolate viruses, two clinical syndromes emerged: an ARDS associated with the rapid onset of cyanosis, delirium, and frothy blood-tinged sputum; and an acute bronchopneumonia characterized by necrosis, hemorrhage, edema, and vasculitis.34,35 The first syndrome has subsequently been shown to be associated with primary viral pneumonia, while the second is caused by bacterial superinfection. Modern reexamination of 1918 data has shown that bacterial superinfection was likely the reason for the distinctly fulminant presentation of that pandemic.36,37
The 2009 H1N1 influenza pandemic caused relatively mild disease in most patients. However, those with severe pneumonia more commonly developed ARDS from primary influenza pneumonia than from bacterial superinfection.17
A third influenza-associated infection is secondary bacterial pneumonia, which follows influenza infection and mimics the presentation of CABP. A typical patient presents with a recent history of influenza-like illness, followed 4 to 14 days later by a recurrence of fever, dyspnea, productive cough, and consolidation on chest radiographs.38 Leukocytosis with an increased number of immature neutrophil forms, prolonged duration of fever, and elevated erythrocyte sedimentation rate are more likely in patients with secondary bacterial pneumonia.39 Isolates from sputum samples commonly include S pneumoniae, S aureus, H influenzae, and other gram-negative rods.40
In recent flu seasons, methicillin-resistant S aureus (MRSA) has emerged as a cause of severe secondary pneumonia. Most of these isolates carry genes for the toxin Panton-Valentine leukocidin; the associated mortality rate is as high as 33%.41,42 Although community-acquired MRSA pneumonia has only been reported in case series, distinct clinical features that have been described include severe pneumonia with high fever, hypotension, shock, respiratory failure, leukopenia, and multilobar and cavitary infiltrates.43
WHEN TO SUSPECT INFLUENZA
The triad of fever, cough, and abrupt onset are the best predictors of influenza, but no single combination of signs and symptoms predict influenza infection with 100% certainty. Therefore, an understanding of local epidemiologic data regarding circulating influenza is essential to maintain a high index of suspicion.44
It is appropriate to suspect influenza in:
- Anyone who is epidemiologically linked to a known outbreak of influenza
- Children, adults, and health care workers who have fever and abrupt onset of respiratory symptoms
- Patients with fever plus exacerbation of underlying pulmonary disease
- Severely ill patients with fever or hypothermia, especially during influenza season.45
DIAGNOSTIC TESTING
Once the diagnosis of pulmonary infection is suggested by clinical features, the initial evaluation should include measurement of vital signs, physical examination, and radiographic imaging of the chest. Additional diagnostic measures to consider include viral testing, blood culture, sputum culture, urinary antigen testing for Legionella and for S pneumoniae, fungal culture, and mycobacterial smear and culture.
Chest radiography (with posterior-anterior and lateral films) is the study that usually demonstrates the presence of a pulmonary infiltrate. If initial chest radiographs do not show an infiltrate, imaging can be repeated after treatment is started if the patient’s clinical presentation still suggests pneumonia. Chest radiographs are of limited value in predicting the pathogen, but they help to determine the extent of pneumonia and to detect parapneumonic effusion.46
A caveat: anterior-posterior, posterior-anterior, and lateral views can miss more than 10% of effusions large enough to warrant thoracentesis, especially when there is lower-lobe consolidation.47
Blood cultures are recommended for patients admitted to the intensive care unit and for those with cavitary infiltrates, leukopenia, alcohol abuse, severe liver disease, asplenia, positive pneumococcal urinary antigen testing, or a pleural effusion.21 However, blood cultures are positive in only 3% to 14% of hospitalized patients with CABP, and the impact of a positive blood culture on management decisions in CABP appears to be quite small.48–50
For the highest yield, blood culture results should be obtained before antibiotics are given. Not only is this good practice, but obtaining blood culture results before starting antibiotics is one of the quality measures evaluated by the Center for Medicare and Medicaid Services.51
Sputum culture is considered optional for outpatients and patients with less-severe pneumonia.21 While it can provide a rapid diagnosis in certain cases, a good-quality sputum sample is obtained in only 39% to 54% of patients with CABP, yields a predominant morphotype in only 45% of cases, and provides a useful microbiologic diagnosis in only 14.4%.52,53 Fungal and mycobacterial cultures are only indicated in certain situations such as cavitary infiltrates or immunosuppression.
Urinary antigen testing for Legionella and S pneumoniae should be done in patients with more severe illness and in those for whom outpatient therapy has failed.21S pneumoniae testing has been shown to allow early diagnosis of pneumococcal pneumonia in 26% more patients than with Gram staining, but it fails to identify 22% of the rapid diagnoses initially identified by Gram staining.54 Thus, a sequential approach is reasonable, with urinary antigen testing for patients at high risk without useful results from sputum Gram staining. Also, recent data suggest that the pneumococcal urinary antigen test may allow optimization of antimicrobial therapy with good clinical outcomes.55
Endotracheal tests. If the patient is intubated, collection of endotracheal aspirates, bronchoscopy, or nonbronchoscopic bronchial lavage (sometimes called “mini-BAL”) should be performed.
Thoracentesis and pleural fluid cultures should be done if a pleural effusion is found. Empyema, large or loculated effusions, and parapneumonic effusions with a pH lower than 7.20, glucose levels less than 3.4 mmol/L (60 mg/dL), or positive results on microbial staining or culture should be drained by chest tube or surgically.56
Testing for influenza should be done if it will change the clinical management, such as the choice of antibiotic or infection control practices. Specimens should be obtained with either a nasopharyngeal swab or aspirate and tested with reverse transcriptase polymerase chain reaction, immunofluorescent staining, or rapid antigen detection, depending on local availability.45
Inflammatory biomarkers such as C-reactive protein and procalcitonin have been receiving interest as ways to predict the etiology and prognosis of CAP and to guide therapy. Several studies have shown that C-reactive protein can help distinguish between CAP and bronchitis, with higher values suggesting more severe pneumonia and pneumonia caused by S pneumoniae or L pneumophila.57 Procalcitonin may help discriminate between severe lower respiratory tract infections of bacterial and 2009 H1N1 origin, although less effectively than C-reactive protein. Low procalcitonin values, particularly when combined with low C-reactive protein levels, suggest that bacterial infection is unlikely.58
RISK STRATIFICATION AND SITE-OF-CARE DECISIONS
Following a presumptive diagnosis of CAP, it is important to decide not only what treatment the patient will receive but whether he or she should be hospitalized. If the patient is to be admitted to the hospital, the clinician must also decide if his or her condition warrants intensive care.
Severity-of-illness scores
A recent meta-analysis compared the performance characteristics of the PSI and CURB-65 scores for predicting mortality in CAP and found no significant differences in overall test performance.61
Another meta-analysis found that the PSI was more sensitive than the CURB-65 and had a low false-negative rate, and so was better at showing which patients do not need to be hospitalized. Conversely, the CURB-65 was more specific and had a higher positive predictive value, and thus was more likely to correctly classify high-risk patients.62
Other scoring systems that aid in deciding about hospital admission and level of care include the CRB-6563 (which can be used instead of the CURB-65 if laboratory values are not available), SMART-COP,64 and SCAP.,65
Guidelines on when to admit to the intensive care unit
Guidelines from the Infectious Diseases Society of America (IDSA) and the American Thoracic Society (ATS) also provide guidance on when intensive care admission is advised,21 and their criteria were recently validated.66 The guidelines advocate direct admission to the intensive care unit for patients requiring vasopressors or mechanical ventilation, and intensive care unit or high-level monitoring for patients with three of the following criteria for severe CAP21:
- Respiratory rate ≥ 30
- Pao2/Fio2 ratio ≤ 250
- Multilobar infiltrates
- Confusion or disorientation
- Uremia (blood urea nitrogen ≥ 20 mg/dL)
- Leukopenia (white blood cell count < 4.0 × 109/L)
- Thrombocytopenia (platelet count < 100 × 109/L)
- Hypothermia (core temperature < 36.0°C [96.8°F])
- Hypotension requiring aggressive fluid resuscitation.
None of these scoring systems or criteria is meant to replace clinical judgment. A recent study has suggested that an oxygen saturation of less than 92% is an appropriate threshold for hospital admission, in view of higher rates of adverse events in outpatients with saturations below this value.67
TREATMENT
Multiple studies have shown that treatment of CAP in accordance with guidelines has led to improved clinical outcomes.21,68–70
How fast must antibiotics be started?
Based on studies that showed a lower mortality rate when antibiotics were started sooner, Medicare and Medicaid adopted a quality measure calling for starting antibiotics within 4 hours in patients being admitted to the hospital.50,71 However, several subsequent studies showed that the diagnosis of pneumonia is often incorrect and that rapid administration of antibiotics could lead to misdiagnosis, overuse of antibiotics, and a higher risk of Clostridium difficile infection.72,73
The current IDSA/ATS guidelines21 recommend that the first antibiotic dose be given while the patient is still in the emergency department, but do not give a specific time within which it should be given. Medicare and Medicaid later updated their quality measure to antibiotic administration within 6 hours.
Which antibiotics should be used?
The selection of antimicrobial agent depends upon the patient’s severity of illness and comorbid conditions.
Although most studies of combination antibiotic therapy have been retrospective and observational, they suggest that a macrolide (ie, one of the “mycins”) added to a beta-lactam antibiotic is beneficial, possibly by covering atypical organisms or via anti-inflammatory action.74–76 The choice of one antibiotic over another appears to be less important, and a recent Cochrane review concluded that there was no significant difference in efficacy among five antibiotic pairs studied.77
Empiric outpatient treatment of a previously healthy patient with CAP and no risk factors for drug-resistant S pneumoniae should include either a macrolide (azithromycin [Zithromax], clarithromycin [Biaxin], or erythromycin) or doxycycline. If the patient has a chronic comorbid condition such as heart, lung, liver, or renal disease, diabetes mellitus, alcoholism, malignancy, asplenia, or immunosuppression or has received antimicrobials within the preceding 3 months, then treatment should include either a respiratory fluoroquinolone (moxifloxacin [Avelox] or levofloxacin [Levaquin]) or a beta-lactam plus a macrolide.21
Overall, published data suggest that the survival rate is about the same with fluoroquinolone monotherapy as with beta-lactam plus macrolide combination therapy, and better than with beta-lactam monotherapy.78
Selection of antibiotics for inpatient treatment of CAP is influenced by severity of illness. Inpatients who do not require intensive care should be treated with either a respiratory fluoroquinolone or combination therapy with a beta-lactam (cefotaxime [Claforan], ceftriaxone [Rocephin], ampicillin, or ertapenem [Invanz]) plus a macrolide or doxycycline.21,76,79
If a specific microbiologic diagnosis is made, then treatment can be narrowed. However in certain cases, such as invasive pneumococcal infection, combination therapy may still be superior.80,81 For patients who need intensive care, treatment should always include a beta-lactam plus either azithromycin or a respiratory fluoroquinolone.21 In certain situations, additional antibiotics may be added as well, such as agents to treat Pseudomonas, community-acquired MRSA, or both.
Switching to oral therapy; short-course therapy
In the interest of avoiding unnecessary antibiotics, numerous studies have addressed the issue of an “early switch” to oral antibiotics and “short-course” therapy for CAP. In general, once clinically stable, patients with CAP, including bacteremic S pneumoniae pneumonia, can be safely switched to oral antibiotics.82
The issue of short-course therapy is more complicated, and the appropriate length of therapy for CAP is not well established. However, 5 days of levofloxacin 750 mg was shown to be as successful as 7 to 10 days of levofloxacin 500 mg.83 In another study, in patients who improved after 3 days of intravenous therapy for CAP, there was no difference in clinical outcome between those who were changed to oral therapy for 5 more days and those who received an oral placebo.84
Most patients who achieve clinical stability in the first week do not need prolonged antibiotic therapy. However, certain conditions, such as S aureus bacteremic pneumonia, complicated pneumonia, and pneumonia due to unusual organisms, may require prolonged treatment.
Other therapies
Additional therapies studied in patients with pneumonia include early mobilization, adjunctive corticosteroids, and statin drugs.
Early mobilization was shown in one study to decrease hospital length of stay without increasing adverse effects.85
Corticosteroids are not supported as a standard of care for patients with severe CAP according to current available studies.86,87 Furthermore, a randomized, controlled trial showed that prednisolone daily for a week did not improve outcomes in hospitalized patients with CAP, and it was associated with increased late failure.88
Statin trials under way. Several observational studies have suggested that statins might be beneficial in managing sepsis through their effects on endothelial cell function, antioxidant effects, anti-inflammatory effects, and immunomodulatory effects.89 However, a recent large prospective multicenter cohort study of hospitalized patients with CAP did not find evidence of a protective effect of statins on clinically meaningful outcomes in CAP or significant differences in circulating biomarkers.90 Several randomized trials of statin therapy in patients with both ventilator-associated pneumonia and CAP are under way.
INFLUENZA TREATMENT: MOST EFFECTIVE WITHIN 48 HOURS
Treatment with antiviral drugs is most effective if started within 48 hours after symptom onset, although some patients with confirmed influenza who are either not improving or who are critically ill may still benefit from treatment started later.
Treatment should be considered in patients with laboratory-confirmed or suspected influenza who are at risk of developing complicated influenza and in otherwise healthy patients who wish to reduce the duration of illness or who have close contact with patients who are at high risk of complications.
Antiviral medications are oseltamivir (Tamiflu), zanamivir (Relenza), and the adamantines amantadine (Symmetrel) and rimantadine (Flumadine).
Due to evolving viral resistance patterns, the choice of antiviral drug depends on the strain. Seasonal H1N1 is best treated with zanamivir or an adamantine, while pandemic 2009 H1N1 and H3N2 are best treated with zanamivir or oseltamivir. When strain typing is not available, empiric therapy should be with either zanamivir monotherapy or a combination of oseltamivir plus rimantadine. Influenza B viruses are resistant to adamantines and should be treated only with either zanamivir or oseltamivir.45
FOLLOW-UP AND PREVENTION
Patients with CAP can generally be expected to improve within 3 to 7 days.91 However, it may be several weeks before they return to baseline.92
Follow-up plans may be guided by the time to clinical stability. For patients who do not achieve clinical stability until more than 72 hours after admission, more aggressive follow-up on discharge is indicated, since they are more likely to experience early readmission and death.93
Pneumococcal vaccination. Because S pneumoniae remains the most common cause of CAP, efforts should be made to vaccinate patients appropriately. The Advisory Committee on Immunization Practices (ACIP) and the US Centers for Disease Control and Prevention recommend that the pneumococcal polysaccharide vaccine (Pneumovax 23; PPSV23) be given to those over age 65. Those who were vaccinated before age 65 should receive another dose at age 65 or later if at least 5 years have passed since their previous dose. Those who receive it at or after age 65 should receive only a single dose. A second dose is recommended 5 years after the first dose for people age 19 to 64 years with functional or anatomic asplenia and for those who are immunocompromised.
Influenza vaccination for all. Of note, the ACIP updated its guidelines on influenza vaccination beginning with the 2010–2011 influenza season. It no longer advocates a risk-stratified approach. Instead, it recommends universal influenza vaccination for everybody more than 6 months old.94
Smoking cessation should be addressed. Smoking cessation is a Medicare and Medicaid quality measure and should be encouraged after an episode of CAP because quitting smoking reduces the risk of pneumococcal disease by approximately 14% each year thereafter.95
- Mortensen EM, Kapoor WN, Chang CC, Fine MJ. Assessment of mortality after long-term follow-up of patients with community-acquired pneumonia. Clin Infect Dis 2003; 37:1617–1624.
- File TM, Marrie TJ. Burden of community-acquired pneumonia in North American adults. Postgrad Med 2010; 122:130–141.
- Marston BJ, Plouffe JF, File TM, et al. Incidence of community-acquired pneumonia requiring hospitalization. Results of a population-based active surveillance study in Ohio. The Community-Based Pneumonia Incidence Study Group. Arch Intern Med 1997; 157:1709–1718.
- Fine MJ, Smith MA, Carson CA, et al. Prognosis and outcomes of patients with community-acquired pneumonia. JAMA 1996; 275:134–141.
- Ruhnke GW, Coca-Perraillon M, Kitch BT, Cutler DM. Trends in mortality and medical spending in patients hospitalized for community-acquired pneumonia: 1993–2005. Med Care 2010; 48:1111–1116.
- Jackson ML, Neuzil KM, Thompson WW, et al. The burden of community-acquired pneumonia in seniors: results of a population-based study. Clin Infect Dis 2004; 39:1642–1650.
- Koivula I, Sten M, Mäkelä PH. Risk factors for pneumonia in the elderly. Am J Med 1994; 96:313–320.
- Ruhnke GW, Coca-Perraillon M, Kitch BT, Cutler DM. Marked reduction in 30-day mortality among elderly patients with community-acquired pneumonia. Am J Med 2011; 124:171–178.
- Farr BM, Bartlett CL, Wadsworth J, Miller DL. Risk factors for community-acquired pneumonia diagnosed upon hospital admission. British Thoracic Society Pneumonia Study Group. Respir Med 2000; 94:954–963.
- Graves CR. Pneumonia in pregnancy. Clin Obstet Gynecol 2010; 53:329–336.
- Eom CS, Jeon CY, Lim JW, Cho EG, Park SM, Lee KS. Use of acid-suppressive drugs and risk of pneumonia: a systematic review and meta-analysis. CMAJ 2011; 183:310–319.
- Voiriot G, Dury S, Parrot A, Mayaud C, Fartoukh M. Nonsteroidal antiinflammatory drugs may affect the presentation and course of community-acquired pneumonia. Chest 2011; 139:387–394.
- Thompson WW, Shay DK, Weintraub E, et al. Mortality associated with influenza and respiratory syncytial virus in the United States. JAMA 2003; 289:179–186.
- Thompson WW, Shay DK, Weintraub E, et al. Influenza-associated hospitalizations in the United States. JAMA 2004; 292:1333–1340.
- Glezen WP, Decker M, Perrotta DM. Survey of underlying conditions of persons hospitalized with acute respiratory disease during influenza epidemics in Houston, 1978–1981. Am Rev Respir Dis 1987; 136:550–555.
- Izurieta HS, Thompson WW, Kramarz P, et al. Influenza and the rates of hospitalization for respiratory disease among infants and young children. N Engl J Med 2000; 342:232–239.
- Rothberg MB, Haessler SD. Complications of seasonal and pandemic influenza. Crit Care Med 2010; 38(suppl 4):e91–e97.
- Apisarnthanarak A, Mundy LM. Etiology of community-acquired pneumonia. Clin Chest Med 2005; 26:47–55.
- de Roux A, Marcos MA, Garcia E, et al. Viral community-acquired pneumonia in nonimmunocompromised adults. Chest 2004; 125:1343–1351.
- Hamelin ME, Côté S, Laforge J, et al. Human metapneumovirus infection in adults with community-acquired pneumonia and exacerbation of chronic obstructive pulmonary disease. Clin Infect Dis 2005; 41:498–502.
- Mandell LA, Wunderink RG, Anzueto A, et al; Infectious Diseases Society of America; American Thoracic Society. Infectious Diseases Society of America/American Thoracic Society consensus guidelines on the management of community-acquired pneumonia in adults. Clin Infect Dis 2007; 44(suppl 2):S27–S72.
- Kolling UK, Hansen F, Braun J, Rink L, Katus HA, Dalhoff K. Leucocyte response and anti-inflammatory cytokines in community acquired pneumonia. Thorax 2001; 56:121–125.
- Wunderink RG, Waterer GW. Community-acquired pneumonia: pathophysiology and host factors with focus on possible new approaches to management of lower respiratory tract infections. Infect Dis Clin North Am 2004; 18:743–759.
- Hilleman MR. Realities and enigmas of human viral influenza: pathogenesis, epidemiology and control. Vaccine 2002; 20:3068–3087.
- Bender BS, Small PA. Influenza: pathogenesis and host defense. Semin Respir Infect 1992; 7:38–45.
- Scheiblauer H, Reinacher M, Tashiro M, Rott R. Interactions between bacteria and influenza A virus in the development of influenza pneumonia. J Infect Dis 1992; 166:783–791.
- McCullers JA. Insights into the interaction between influenza virus and pneumococcus. Clin Microbiol Rev 2006; 19:571–582.
- Metlay JP, Kapoor WN, Fine MJ. Does this patient have community-acquired pneumonia? Diagnosing pneumonia by history and physical examination. JAMA 1997; 278:1440–1445.
- Benbassat J, Baumal R. Narrative review: should teaching of the respiratory physical examination be restricted only to signs with proven reliability and validity? J Gen Intern Med 2010; 25:865–872.
- Kolsuz M, Erginel S, Alatas O, et al. Acute phase reactants and cytokine levels in unilateral community-acquired pneumonia. Respiration 2003; 70:615–622.
- Alves DW, Kennedy MT. Community-acquired pneumonia in casualty: etiology, clinical features, diagnosis, and management (or a look at the “new” in pneumonia since 2002). Curr Opin Pulm Med 2004; 10:166–170.
- Monto AS, Gravenstein S, Elliott M, Colopy M, Schweinle J. Clinical signs and symptoms predicting influenza infection. Arch Intern Med 2000; 160:3243–3247.
- Bewick T, Myles P, Greenwood S, et al; Influenza Clinical Information Network. Clinical and laboratory features distinguishing pandemic H1N1 influenza-related pneumonia from interpandemic community-acquired pneumonia in adults. Thorax 2011; 66:247–252.
- Morens DM, Fauci AS. The 1918 influenza pandemic: insights for the 21st century. J Infect Dis 2007; 195:1018–1028.
- Starr I. Influenza in 1918: recollections of the epidemic in Philadelphia. 1976. Ann Intern Med 2006; 145:138–140.
- Morens DM, Taubenberger JK, Fauci AS. Predominant role of bacterial pneumonia as a cause of death in pandemic influenza: implications for pandemic influenza preparedness. J Infect Dis 2008; 198:962–970.
- Brundage JF, Shanks GD. Deaths from bacterial pneumonia during 1918–19 influenza pandemic. Emerg Infect Dis 2008; 14:1193–1199.
- Treanor J. Influenza virus. In: Mandell GL, Bennett JE, Dolin R, eds. Principles and Practice of Infectious Diseases. 6th ed. Philadelphia, PA: Elsevier/Churchill Livingstone; 2005:2060–2085.
- Jarstrand C, Tunevall G. The influence of bacterial superinfection on the clinical course of influenza. Studies from the influenza epidemics in Stockholm during the winters 1969–70 and 1971–72. Scand J Infect Dis 1975; 7:243–247.
- Schwarzmann SW, Adler JL, Sullivan RJ, Marine WM. Bacterial pneumonia during the Hong Kong influenza epidemic of 1968–1969. Arch Intern Med 1971; 127:1037–1041.
- Hageman JC, Uyeki TM, Francis JS, et al. Severe community-acquired pneumonia due to Staphylococcus aureus, 2003–04 influenza season. Emerg Infect Dis 2006; 12:894–899.
- Centers for Disease Control and Prevention (CDC). Severe methicillin-resistant Staphylococcus aureus community-acquired pneumonia associated with influenza—Louisiana and Georgia, December 2006–January 2007. MMWR Morb Mortal Wkly Rep 2007; 56:325–329.
- Hidron AI, Low CE, Honig EG, Blumberg HM. Emergence of community-acquired methicillin-resistant Staphylococcus aureus strain USA300 as a cause of necrotising community-onset pneumonia. Lancet Infect Dis 2009; 9:384–392.
- Call SA, Vollenweider MA, Hornung CA, Simel DL, McKinney WP. Does this patient have influenza? JAMA 2005; 293:987–997.
- Harper SA, Bradley JS, Englund JA, et al; Expert Panel of the Infectious Diseases Society of America. Seasonal influenza in adults and children—diagnosis, treatment, chemoprophylaxis, and institutional outbreak management: clinical practice guidelines of the Infectious Diseases Society of America. Clin Infect Dis 2009; 48:1003–1032.
- Boersma WG, Daniels JM, Löwenberg A, Boeve WJ, van de Jagt EJ. Reliability of radiographic findings and the relation to etiologic agents in community-acquired pneumonia. Respir Med 2006; 100:926–932.
- Brixey AG, Luo Y, Skouras V, Awdankiewicz A, Light RW. The efficacy of chest radiographs in detecting parapneumonic effusions. Respirology 2011; 16:1000–1004.
- Campbell SG, Marrie TJ, Anstey R, Dickinson G, Ackroyd-Stolarz S. The contribution of blood cultures to the clinical management of adult patients admitted to the hospital with community-acquired pneumonia: a prospective observational study. Chest 2003; 123:1142–1150.
- Waterer GW, Wunderink RG. The influence of the severity of community-acquired pneumonia on the usefulness of blood cultures. Respir Med 2001; 95:78–82.
- Houck PM, Bratzler DW, Nsa W, Ma A, Bartlett JG. Timing of antibiotic administration and outcomes for Medicare patients hospitalized with community-acquired pneumonia. Arch Intern Med 2004; 164:637–644.
- Information & Quality Healthcare. http://www.IQH.org/attachments/219_CoreMHelpBookletpg4_11_3.pdf. Accessed November 14, 2011.
- Rosón B, Carratalà J, Verdaguer R, Dorca J, Manresa F, Gudiol F. Prospective study of the usefulness of sputum Gram stain in the initial approach to community-acquired pneumonia requiring hospitalization. Clin Infect Dis 2000; 31:869–874.
- García-Vázquez E, Marcos MA, Mensa J, et al. Assessment of the usefulness of sputum culture for diagnosis of community-acquired pneumonia using the PORT predictive scoring system. Arch Intern Med 2004; 164:1807–1811.
- Rosón B, Fernández-Sabé N, Carratalà J, et al. Contribution of a urinary antigen assay (Binax NOW) to the early diagnosis of pneumococcal pneumonia. Clin Infect Dis 2004; 38:222–226.
- Sordé R, Falcó V, Lowak M, et al. Current and potential usefulness of pneumococcal urinary antigen detection in hospitalized patients with community-acquired pneumonia to guide antimicrobial therapy. Arch Intern Med 2011; 171:166–172.
- Koegelenberg CFN, Diacon AH, Bolliger CT. Parapneumonic pleural effusion and empyema. Respiration 2008; 75:241–250.
- Almirall J, Bolíbar I, Toran P, et al; Community-Acquired Pneumonia Maresme Study Group. Contribution of C-reactive protein to the diagnosis and assessment of severity of community-acquired pneumonia. Chest 2004; 125:1335–1342.
- Ingram PR, Inglis T, Moxon D, Speers D. Procalcitonin and C-reactive protein in severe 2009 H1N1 influenza infection. Intensive Care Med 2010; 36:528–532.
- Fine MJ, Auble TE, Yealy DM, et al. A prediction rule to identify low-risk patients with community-acquired pneumonia. N Engl J Med 1997; 336:243–250.
- Lim WS, van der Eerden MM, Laing R, et al. Defining community acquired pneumonia severity on presentation to hospital: an international derivation and validation study. Thorax 2003; 58:377–382.
- Chalmers JD, Singanayagam A, Akram AR, et al. Severity assessment tools for predicting mortality in hospitalised patients with community-acquired pneumonia. Systematic review and meta-analysis. Thorax 2010; 65:878–883.
- Loke YK, Kwok CS, Niruban A, Myint PK. Value of severity scales in predicting mortality from community-acquired pneumonia: systematic review and meta-analysis. Thorax 2010; 65:884–890.
- Capelastegui A, España PP, Quintana JM, et al. Validation of a predictive rule for the management of community-acquired pneumonia. Eur Respir J 2006; 27:151–157.
- Charles PG, Wolfe R, Whitby M, et al; Australian Community-Acquired Pneumonia Study Collaboration. SMART-COP: a tool for predicting the need for intensive respiratory or vasopressor support in community-acquired pneumonia. Clin Infect Dis 2008; 47:375–384.
- España PP, Capelastegui A, Gorordo I, et al. Development and validation of a clinical prediction rule for severe community-acquired pneumonia. Am J Respir Crit Care Med 2006; 174:1249–1256.
- Chalmers JD, Taylor JK, Mandal P, et al. Validation of the Infectious Diseases Society of America/American Thoracic Society minor criteria for intensive care unit admission in community-acquired pneumonia patients without major criteria or contraindications to intensive care unit care. Clin Infect Dis 2011; 53:503–511.
- Majumdar SR, Eurich DT, Gamble JM, Senthilselvan A, Marrie TJ. Oxygen saturations less than 92% are associated with major adverse events in outpatients with pneumonia: a population-based cohort study. Clin Infect Dis 2011; 52:325–331.
- Nathwani D, Rubinstein E, Barlow G, Davey P. Do guidelines for community-acquired pneumonia improve the cost-effectiveness of hospital care? Clin Infect Dis 2001; 32:728–741.
- Dean NC, Silver MP, Bateman KA, James B, Hadlock CJ, Hale D. Decreased mortality after implementation of a treatment guideline for community-acquired pneumonia. Am J Med 2001; 110:451–457.
- Capelastegui A, España PP, Quintana JM, et al. Improvement of process-of-care and outcomes after implementing a guideline for the management of community-acquired pneumonia: a controlled before-and-after design study. Clin Infect Dis 2004; 39:955–963.
- Silber SH, Garrett C, Singh R, et al. Early administration of antibiotics does not shorten time to clinical stability in patients with moderate-to-severe community-acquired pneumonia. Chest 2003; 124:1798–1804.
- Welker JA, Huston M, McCue JD. Antibiotic timing and errors in diagnosing pneumonia. Arch Intern Med 2008; 168:351–356.
- Polgreen PM, Chen YY, Cavanaugh JE, et al. An outbreak of severe Clostridium difficile-associated disease possibly related to inappropriate antimicrobial therapy for community-acquired pneumonia. Infect Control Hosp Epidemiol 2007; 28:212–214.
- Waterer GW, Somes GW, Wunderink RG. Monotherapy may be suboptimal for severe bacteremic pneumococcal pneumonia. Arch Intern Med 2001; 161:1837–1842.
- Lodise TP, Kwa A, Cosler L, Gupta R, Smith RP. Comparison of beta-lactam and macrolide combination therapy versus fluoroquinolone monotherapy in hospitalized Veterans Affairs patients with community-acquired pneumonia. Antimicrob Agents Chemother 2007; 51:3977–3982.
- Waterer GW, Rello J, Wunderink RG. Management of community-acquired pneumonia in adults. Am J Respir Crit Care Med 2011; 183:157–164.
- Bjerre LM, Verheij TJ, Kochen MM. Antibiotics for community acquired pneumonia in adult outpatients. Cochrane Database Syst Rev 2009; (4):CD002109.
- Frei CR, Labreche MJ, Attridge RT. Fluoroquinolones in community-acquired pneumonia: guide to selection and appropriate use. Drugs 2011; 71:757–770.
- Weiss K, Tillotson GS. The controversy of combination vs monotherapy in the treatment of hospitalized community-acquired pneumonia. Chest 2005; 128:940–946.
- Martínez JA, Horcajada JP, Almela M, et al. Addition of a macrolide to a beta-lactam-based empirical antibiotic regimen is associated with lower in-hospital mortality for patients with bacteremic pneumococcal pneumonia. Clin Infect Dis 2003; 36:389–395.
- Waterer GW, Somes GW, Wunderink RG. Monotherapy may be suboptimal for severe bacteremic pneumococcal pneumonia. Arch Intern Med 2001; 161:1837–1842.
- Ramirez JA, Bordon J. Early switch from intravenous to oral antibiotics in hospitalized patients with bacteremic community-acquired Streptococcus pneumoniae pneumonia. Arch Intern Med 2001; 161:848–850.
- Dunbar LM, Wunderink RG, Habib MP, et al. High-dose, short-course levofloxacin for community-acquired pneumonia: a new treatment paradigm. Clin Infect Dis 2003; 37:752–760.
- el Moussaoui R, de Borgie CA, van den Broek P, et al. Effectiveness of discontinuing antibiotic treatment after three days versus eight days in mild to moderate-severe community acquired pneumonia: randomised, double blind study. BMJ 2006; 332:1355.
- Mundy LM, Leet TL, Darst K, Schnitzler MA, Dunagan WC. Early mobilization of patients hospitalized with community-acquired pneumonia. Chest 2003; 124:883–889.
- Salluh JI, Póvoa P, Soares M, Castro-Faria-Neto HC, Bozza FA, Bozza PT. The role of corticosteroids in severe community-acquired pneumonia: a systematic review. Crit Care 2008; 12:R76.
- Mikami K, Suzuki M, Kitagawa H, et al. Efficacy of corticosteroids in the treatment of community-acquired pneumonia requiring hospitalization. Lung 2007; 185:249–255.
- Snijders D, Daniels JM, de Graaff CS, van der Werf TS, Boersma WG. Efficacy of corticosteroids in community-acquired pneumonia: a randomized double-blinded clinical trial. Am J Respir Crit Care Med 2010; 181:975–982.
- Chopra V, Flanders SA. Does statin use improve pneumonia outcomes? Chest 2009; 136:1381–1388.
- Yende S, Milbrandt EB, Kellum JA, et al. Understanding the potential role of statins in pneumonia and sepsis. Crit Care Med 2011; 39:1871–1878.
- Halm EA, Fine MJ, Marrie TJ, et al. Time to clinical stability in patients hospitalized with community-acquired pneumonia: implications for practice guidelines. JAMA 1998; 279:1452–1457.
- Marrie TJ, Lau CY, Wheeler SL, Wong CJ, Feagan BG. Predictors of symptom resolution in patients with community-acquired pneumonia. Clin Infect Dis 2000; 31:1362–1367.
- Aliberti S, Peyrani P, Filardo G, et al. Association between time to clinical stability and outcomes after discharge in hospitalized patients with community-acquired pneumonia. Chest 2011; 140:482–488.
- Fiore AE, Uyeki TM, Broder K, et al; Centers for Disease Control and Prevention (CDC). Prevention and control of influenza with vaccines: recommendations of the Advisory Committee on Immunization Practices (ACIP), 2010. MMWR Recomm Rep 2010; 59:1–62.
- Nuorti JP, Butler JC, Farley MM, et al. Cigarette smoking and invasive pneumococcal disease. Active Bacterial Core Surveillance Team. N Engl J Med 2000; 342:681–689.
General internists need to be able to recognize community-acquired pneumonia (CAP) so that diagnostic and therapeutic interventions can be initiated promptly. It is also important to understand the most likely and possible causes of CAP so that appropriate initial antimicrobial therapy can be chosen. Especially during flu season, influenza can present as CAP and should be included in the differential diagnosis.
When managing a patient with CAP, the internist must decide which level of care, diagnostic tests, antimicrobial agents, and follow-up plans are needed. These topics will be reviewed in this article.
TWO TERMS TO REMEMBER
- CAP refers to pneumonia acquired outside a health care facility. It can be either bacterial or viral.
- CABP (community-acquired bacterial pneumonia) refers only to those cases caused by bacterial pathogens.
NUMBERS AND TRENDS
In the United States, CAP is the number-one cause of death from infection and the sixth leading cause of death overall.1 Each year, it is responsible for about 4.2 million outpatient visits, more than 60,000 deaths, and more than $17 billion in health care expenses.2
Community-acquired bacterial pneumonia: Common, serious
In a population-based US study in 1991, the incidence of CABP requiring hospitalization was 266.8 per 100,000 people.3
Estimates of overall mortality in CABP vary depending on the severity of illness and comorbid conditions. A meta-analysis published in 1996 found the overall mortality rate to be 13.7%, with a range of 5.1% to 36.5% depending on severity.4
In hospitalized patients, mortality rates and length of hospital stay appear to be declining over time. Between 1993 and 2005, the age-adjusted mortality rate decreased from 8.9% to 4.1%, and the average length of stay decreased from 7.5 to 5.7 days, with an overall reduction in hospital cost.5
CABP is more prevalent in older people than in the general population, and it increases with age from 18.2 cases per 1,000 patient-years in patients 60 to 69 years to 52.3 cases per 1,000 patient-years in those older than 85 years.6 Risk factors for pneumonia in the elderly include heart disease, chronic lung disease, immunosuppressive drugs, alcoholism, and increasing age.7 Similar to the trend in the general population, the mortality rate in elderly CABP patients appears to be decreasing over time, possibly thanks to rising rates of pneumococcal and influenza vaccination.8
Among the general population, risk factors for developing CABP also include smoking, occupational dust exposure, history of childhood pneumonia, unemployment, and single marital status.9 The incidence of CABP does not appear to be higher among pregnant women, although it is the most frequent cause of nonobstetric death in this population.10
The use of proton pump inhibitors may be an emerging risk factor for CABP.11 Also, use of nonsteroidal anti-inflammatory drugs among patients with CABP is associated with a blunted inflammatory response as well as a higher risk of pleuropulmonary complications and a delay in presentation.12
Influenza is also common, potentially severe
Influenza is also very common and potentially severe. It can cause a spectrum of disease, from mild upper respiratory tract symptoms to severe viral pneumonia that can be life-threatening and complicated by respiratory failure and the acute respiratory distress syndrome (ARDS).
Influenza infection can also be complicated by subsequent bacterial pneumonia. However, the epidemiology of influenza infection differs from that of CABP in that influenza occurs seasonally.
In the United States, seasonal influenza causes 36,000 deaths and 200,000 hospitalizations annually.13,14 As with CABP, the risk of death from influenza increases with age: it is 16 times greater in people age 85 and older than in those ages 65 to 69.13
During yearly seasonal epidemics, those at the highest risk of hospitalization and death are at the extremes of age. Risk factors for complicated influenza include heart disease, lung disease, diabetes, renal failure, rheumatologic conditions, dementia, and neurologic disease.15,16 During the 2009 H1N1 influenza pandemic, unexpected severity was seen in previously healthy young adults as well as those with obesity, neurodegenerative disease, pregnancy, and asthma.17
PATHOGENS: TYPICAL, ATYPICAL, VIRAL
Identifying the etiologic organism in CAP is confounded by limitations in the available diagnostic tests and also by poor-quality specimens that often are contaminated with bacteria that colonize the upper airways. Given these caveats, the primary pathogens responsible for CAP broadly include typical bacterial pathogens, atypical bacterial pathogens, and viruses.
Typical bacterial pathogens include Streptococcus pneumoniae, Haemophilus influenzae, Staphylococcus aureus, Moraxella catarrhalis, and, less commonly, a variety of aerobic and anaerobic gram-negative rods including Pseudomonas aeruginosa, Acinetobacter species, and Klebsiella pneumoniae.
Atypical bacterial pathogens include Mycoplasma pneumoniae, Chlamydia pneumoniae, and Legionella species.18
Viruses implicated in adult CAP include influenza A and B, parainfluenza viruses, respiratory syncytial virus, and adenovirus.19 More recently, human metapneumovirus has been described as a cause of adult CAP.20
Clues to uncommon microbes
Specific historic features or coexisting conditions that may suggest an uncommon microbiologic diagnosis include21:
- Recent travel to the southwestern United States or Southeast Asia
- Ill contacts
- Exposure to birds, bats, rabbits, or farm animals
- Alcoholism
- Chronic obstructive pulmonary disease
- Human immunodeficiency virus infection
- Structural lung disease
- Prolonged cough with whoop or posttussive vomiting
- Aspiration
- Bioterrorism.
In cases in which one or more of these conditions exist, CAP may also be caused by other agents not listed above, including Mycobacterium tuberculosis, oral anaerobes, atypical mycobacteria, Histoplasma capsulatum, Chlamydophila psittaci, Francisella tularensis, Coxiella burnettii, Pneumocystis jiroveci, Cryptococcus, Aspergillus, Coccidioides, Hantavirus, avian influenza, Burkholderia pseudomallei, severe acute respiratory syndrome virus, Bordetella pertussis, Bacillus anthracis, and Yersinia pestis.
HOW BACTERIA INVADE THE LUNGS
The pathophysiology of CABP involves both host defense and microbial virulence factors.
The airways are most commonly exposed to microbes by microaspiration of upper airway flora, although hematogenous seeding of the lungs in a bacteremic patient or contiguous spread of infection from an adjacent site can also occur.
Mucociliary clearance and the cough reflex are important initial defenses against infection and can be inhibited by neurologic diseases and conditions that impair the mucociliary mechanism. Mucosal immune cells, including macrophages and neutrophils, recognize invading pathogens and generate an antibody response.
Regulation of the host inflammatory response to infection depends on a complex interaction between immune cells, inflammatory cytokines (eg, tumor necrosis factor alpha, interleukin 1-beta, and interleukin 6), and anti-inflammatory cytokines such as interleukin 1 receptor antagonist and soluble tumor necrosis factor receptor type I.22
The interaction and timing of the inflammatory and anti-inflammatory response are essential in manifesting an appropriate host response to infection. An inadequate inflammatory response can lead to sepsis and death, but an excessive, late anti-inflammatory response can lead to a systemic inflammatory response such as ARDS. Polymorphisms within the genes coding for these factors may explain the variation in severity of illness among patients with CABP.23
HOW INFLUENZA DOES ITS DAMAGE
There are three types of influenza virus: A, B, and C. Type A causes most human infections. The influenza A virus envelope comprises a lipid bilayer that contains the projecting glycoproteins hemagglutinin and neuraminidase. Influenza viruses are named on the basis of these proteins and are designated with an H and an N, respectively, each followed by a number referring to the subtype.
Influenza infection begins when the virus makes contact with the epithelium. Hemagglutinin binds to the host cell and allows viral entry, where it begins replication. Neuraminidase prevents viral aggregation and facilitates the release of virus from infected cells.24
Mature virions are released and spread to neighboring host cells; this process is associated with desquamation and inflammation of the airways, causing cough, rhinorrhea, and sore throat. Systemic symptoms are associated with the induction of interferon, which causes fever and myalgia.25
Recovery and immunity to influenza infection occurs through both humoral and cell-mediated immunity, with antibodies directed against the specific hemagglutinin and neuraminidase antigens of the infecting virus. Immunity wanes over time and with antigenic drift of circulating viruses, making the host susceptible to recurrent influenza infection.24
Influenza is often complicated by bacterial superinfection
The influenza virus acts synergistically with certain bacteria to increase infectivity, and this may explain why influenza is often complicated by bacterial superinfection.
Mechanisms leading to bacterial superinfection include increased binding and invasion of bacteria, increased viral replication, and modification of the host inflammatory response. Some S aureus strains produce a protease that directly activates influenza virus hemagglutinin; other bacteria can activate plasminogen to promote influenza replication. The resulting increase in proteases in host tissues promotes activation of influenza through cleavage of hemagglutinin.26
The influenza virus also causes damage to the airway epithelial layer, leading to increased exposure of the binding sites necessary for adherence of S pneumoniae.27
CLINICAL PRESENTATION OF COMMUNITY-ACQUIRED PNEUMONIA
Although CAP is common, agreement on its essential clinical signs and symptoms is surprisingly limited, due in part to heterogeneous patient presentations and in part to interobserver variability. The reader is referred to two excellent reviews on this topic.28,29
The diagnosis of CAP is made on clinical grounds, based on a combination of signs and symptoms. Symptoms of pneumonia can include cough, fever, chills, sputum production, dyspnea, and pleuritic pain. Physical findings can include tachypnea, tachycardia, hypoxemia, and consolidation or rales on auscultation. Laboratory data may show leukocytosis or elevated C-reactive protein, and radiographic studies may show evidence of a new infiltrate.21,30,31
Clinical presentation of influenza
Seasonal influenza as a cause of CAP is difficult to distinguish from bacterial causes. The clinical presentation of seasonal influenza most commonly includes fever or subjective feverishness, cough, myalgia, and weakness.32 In a recent multivariate analysis, five clinical features were shown to be predictive of pandemic H1N1 influenza pneumonia rather than CABP: age younger than 65 years, absence of confusion, white blood cell count less than 12 × 109/L, temperature higher than 38°C (100.4°F), and bilateral opacities on radiography.32,33
Complicated influenza infection can be either primary viral pneumonia or bacterial superinfection.
During the 1918 influenza pandemic, which predated the ability to isolate viruses, two clinical syndromes emerged: an ARDS associated with the rapid onset of cyanosis, delirium, and frothy blood-tinged sputum; and an acute bronchopneumonia characterized by necrosis, hemorrhage, edema, and vasculitis.34,35 The first syndrome has subsequently been shown to be associated with primary viral pneumonia, while the second is caused by bacterial superinfection. Modern reexamination of 1918 data has shown that bacterial superinfection was likely the reason for the distinctly fulminant presentation of that pandemic.36,37
The 2009 H1N1 influenza pandemic caused relatively mild disease in most patients. However, those with severe pneumonia more commonly developed ARDS from primary influenza pneumonia than from bacterial superinfection.17
A third influenza-associated infection is secondary bacterial pneumonia, which follows influenza infection and mimics the presentation of CABP. A typical patient presents with a recent history of influenza-like illness, followed 4 to 14 days later by a recurrence of fever, dyspnea, productive cough, and consolidation on chest radiographs.38 Leukocytosis with an increased number of immature neutrophil forms, prolonged duration of fever, and elevated erythrocyte sedimentation rate are more likely in patients with secondary bacterial pneumonia.39 Isolates from sputum samples commonly include S pneumoniae, S aureus, H influenzae, and other gram-negative rods.40
In recent flu seasons, methicillin-resistant S aureus (MRSA) has emerged as a cause of severe secondary pneumonia. Most of these isolates carry genes for the toxin Panton-Valentine leukocidin; the associated mortality rate is as high as 33%.41,42 Although community-acquired MRSA pneumonia has only been reported in case series, distinct clinical features that have been described include severe pneumonia with high fever, hypotension, shock, respiratory failure, leukopenia, and multilobar and cavitary infiltrates.43
WHEN TO SUSPECT INFLUENZA
The triad of fever, cough, and abrupt onset are the best predictors of influenza, but no single combination of signs and symptoms predict influenza infection with 100% certainty. Therefore, an understanding of local epidemiologic data regarding circulating influenza is essential to maintain a high index of suspicion.44
It is appropriate to suspect influenza in:
- Anyone who is epidemiologically linked to a known outbreak of influenza
- Children, adults, and health care workers who have fever and abrupt onset of respiratory symptoms
- Patients with fever plus exacerbation of underlying pulmonary disease
- Severely ill patients with fever or hypothermia, especially during influenza season.45
DIAGNOSTIC TESTING
Once the diagnosis of pulmonary infection is suggested by clinical features, the initial evaluation should include measurement of vital signs, physical examination, and radiographic imaging of the chest. Additional diagnostic measures to consider include viral testing, blood culture, sputum culture, urinary antigen testing for Legionella and for S pneumoniae, fungal culture, and mycobacterial smear and culture.
Chest radiography (with posterior-anterior and lateral films) is the study that usually demonstrates the presence of a pulmonary infiltrate. If initial chest radiographs do not show an infiltrate, imaging can be repeated after treatment is started if the patient’s clinical presentation still suggests pneumonia. Chest radiographs are of limited value in predicting the pathogen, but they help to determine the extent of pneumonia and to detect parapneumonic effusion.46
A caveat: anterior-posterior, posterior-anterior, and lateral views can miss more than 10% of effusions large enough to warrant thoracentesis, especially when there is lower-lobe consolidation.47
Blood cultures are recommended for patients admitted to the intensive care unit and for those with cavitary infiltrates, leukopenia, alcohol abuse, severe liver disease, asplenia, positive pneumococcal urinary antigen testing, or a pleural effusion.21 However, blood cultures are positive in only 3% to 14% of hospitalized patients with CABP, and the impact of a positive blood culture on management decisions in CABP appears to be quite small.48–50
For the highest yield, blood culture results should be obtained before antibiotics are given. Not only is this good practice, but obtaining blood culture results before starting antibiotics is one of the quality measures evaluated by the Center for Medicare and Medicaid Services.51
Sputum culture is considered optional for outpatients and patients with less-severe pneumonia.21 While it can provide a rapid diagnosis in certain cases, a good-quality sputum sample is obtained in only 39% to 54% of patients with CABP, yields a predominant morphotype in only 45% of cases, and provides a useful microbiologic diagnosis in only 14.4%.52,53 Fungal and mycobacterial cultures are only indicated in certain situations such as cavitary infiltrates or immunosuppression.
Urinary antigen testing for Legionella and S pneumoniae should be done in patients with more severe illness and in those for whom outpatient therapy has failed.21S pneumoniae testing has been shown to allow early diagnosis of pneumococcal pneumonia in 26% more patients than with Gram staining, but it fails to identify 22% of the rapid diagnoses initially identified by Gram staining.54 Thus, a sequential approach is reasonable, with urinary antigen testing for patients at high risk without useful results from sputum Gram staining. Also, recent data suggest that the pneumococcal urinary antigen test may allow optimization of antimicrobial therapy with good clinical outcomes.55
Endotracheal tests. If the patient is intubated, collection of endotracheal aspirates, bronchoscopy, or nonbronchoscopic bronchial lavage (sometimes called “mini-BAL”) should be performed.
Thoracentesis and pleural fluid cultures should be done if a pleural effusion is found. Empyema, large or loculated effusions, and parapneumonic effusions with a pH lower than 7.20, glucose levels less than 3.4 mmol/L (60 mg/dL), or positive results on microbial staining or culture should be drained by chest tube or surgically.56
Testing for influenza should be done if it will change the clinical management, such as the choice of antibiotic or infection control practices. Specimens should be obtained with either a nasopharyngeal swab or aspirate and tested with reverse transcriptase polymerase chain reaction, immunofluorescent staining, or rapid antigen detection, depending on local availability.45
Inflammatory biomarkers such as C-reactive protein and procalcitonin have been receiving interest as ways to predict the etiology and prognosis of CAP and to guide therapy. Several studies have shown that C-reactive protein can help distinguish between CAP and bronchitis, with higher values suggesting more severe pneumonia and pneumonia caused by S pneumoniae or L pneumophila.57 Procalcitonin may help discriminate between severe lower respiratory tract infections of bacterial and 2009 H1N1 origin, although less effectively than C-reactive protein. Low procalcitonin values, particularly when combined with low C-reactive protein levels, suggest that bacterial infection is unlikely.58
RISK STRATIFICATION AND SITE-OF-CARE DECISIONS
Following a presumptive diagnosis of CAP, it is important to decide not only what treatment the patient will receive but whether he or she should be hospitalized. If the patient is to be admitted to the hospital, the clinician must also decide if his or her condition warrants intensive care.
Severity-of-illness scores
A recent meta-analysis compared the performance characteristics of the PSI and CURB-65 scores for predicting mortality in CAP and found no significant differences in overall test performance.61
Another meta-analysis found that the PSI was more sensitive than the CURB-65 and had a low false-negative rate, and so was better at showing which patients do not need to be hospitalized. Conversely, the CURB-65 was more specific and had a higher positive predictive value, and thus was more likely to correctly classify high-risk patients.62
Other scoring systems that aid in deciding about hospital admission and level of care include the CRB-6563 (which can be used instead of the CURB-65 if laboratory values are not available), SMART-COP,64 and SCAP.,65
Guidelines on when to admit to the intensive care unit
Guidelines from the Infectious Diseases Society of America (IDSA) and the American Thoracic Society (ATS) also provide guidance on when intensive care admission is advised,21 and their criteria were recently validated.66 The guidelines advocate direct admission to the intensive care unit for patients requiring vasopressors or mechanical ventilation, and intensive care unit or high-level monitoring for patients with three of the following criteria for severe CAP21:
- Respiratory rate ≥ 30
- Pao2/Fio2 ratio ≤ 250
- Multilobar infiltrates
- Confusion or disorientation
- Uremia (blood urea nitrogen ≥ 20 mg/dL)
- Leukopenia (white blood cell count < 4.0 × 109/L)
- Thrombocytopenia (platelet count < 100 × 109/L)
- Hypothermia (core temperature < 36.0°C [96.8°F])
- Hypotension requiring aggressive fluid resuscitation.
None of these scoring systems or criteria is meant to replace clinical judgment. A recent study has suggested that an oxygen saturation of less than 92% is an appropriate threshold for hospital admission, in view of higher rates of adverse events in outpatients with saturations below this value.67
TREATMENT
Multiple studies have shown that treatment of CAP in accordance with guidelines has led to improved clinical outcomes.21,68–70
How fast must antibiotics be started?
Based on studies that showed a lower mortality rate when antibiotics were started sooner, Medicare and Medicaid adopted a quality measure calling for starting antibiotics within 4 hours in patients being admitted to the hospital.50,71 However, several subsequent studies showed that the diagnosis of pneumonia is often incorrect and that rapid administration of antibiotics could lead to misdiagnosis, overuse of antibiotics, and a higher risk of Clostridium difficile infection.72,73
The current IDSA/ATS guidelines21 recommend that the first antibiotic dose be given while the patient is still in the emergency department, but do not give a specific time within which it should be given. Medicare and Medicaid later updated their quality measure to antibiotic administration within 6 hours.
Which antibiotics should be used?
The selection of antimicrobial agent depends upon the patient’s severity of illness and comorbid conditions.
Although most studies of combination antibiotic therapy have been retrospective and observational, they suggest that a macrolide (ie, one of the “mycins”) added to a beta-lactam antibiotic is beneficial, possibly by covering atypical organisms or via anti-inflammatory action.74–76 The choice of one antibiotic over another appears to be less important, and a recent Cochrane review concluded that there was no significant difference in efficacy among five antibiotic pairs studied.77
Empiric outpatient treatment of a previously healthy patient with CAP and no risk factors for drug-resistant S pneumoniae should include either a macrolide (azithromycin [Zithromax], clarithromycin [Biaxin], or erythromycin) or doxycycline. If the patient has a chronic comorbid condition such as heart, lung, liver, or renal disease, diabetes mellitus, alcoholism, malignancy, asplenia, or immunosuppression or has received antimicrobials within the preceding 3 months, then treatment should include either a respiratory fluoroquinolone (moxifloxacin [Avelox] or levofloxacin [Levaquin]) or a beta-lactam plus a macrolide.21
Overall, published data suggest that the survival rate is about the same with fluoroquinolone monotherapy as with beta-lactam plus macrolide combination therapy, and better than with beta-lactam monotherapy.78
Selection of antibiotics for inpatient treatment of CAP is influenced by severity of illness. Inpatients who do not require intensive care should be treated with either a respiratory fluoroquinolone or combination therapy with a beta-lactam (cefotaxime [Claforan], ceftriaxone [Rocephin], ampicillin, or ertapenem [Invanz]) plus a macrolide or doxycycline.21,76,79
If a specific microbiologic diagnosis is made, then treatment can be narrowed. However in certain cases, such as invasive pneumococcal infection, combination therapy may still be superior.80,81 For patients who need intensive care, treatment should always include a beta-lactam plus either azithromycin or a respiratory fluoroquinolone.21 In certain situations, additional antibiotics may be added as well, such as agents to treat Pseudomonas, community-acquired MRSA, or both.
Switching to oral therapy; short-course therapy
In the interest of avoiding unnecessary antibiotics, numerous studies have addressed the issue of an “early switch” to oral antibiotics and “short-course” therapy for CAP. In general, once clinically stable, patients with CAP, including bacteremic S pneumoniae pneumonia, can be safely switched to oral antibiotics.82
The issue of short-course therapy is more complicated, and the appropriate length of therapy for CAP is not well established. However, 5 days of levofloxacin 750 mg was shown to be as successful as 7 to 10 days of levofloxacin 500 mg.83 In another study, in patients who improved after 3 days of intravenous therapy for CAP, there was no difference in clinical outcome between those who were changed to oral therapy for 5 more days and those who received an oral placebo.84
Most patients who achieve clinical stability in the first week do not need prolonged antibiotic therapy. However, certain conditions, such as S aureus bacteremic pneumonia, complicated pneumonia, and pneumonia due to unusual organisms, may require prolonged treatment.
Other therapies
Additional therapies studied in patients with pneumonia include early mobilization, adjunctive corticosteroids, and statin drugs.
Early mobilization was shown in one study to decrease hospital length of stay without increasing adverse effects.85
Corticosteroids are not supported as a standard of care for patients with severe CAP according to current available studies.86,87 Furthermore, a randomized, controlled trial showed that prednisolone daily for a week did not improve outcomes in hospitalized patients with CAP, and it was associated with increased late failure.88
Statin trials under way. Several observational studies have suggested that statins might be beneficial in managing sepsis through their effects on endothelial cell function, antioxidant effects, anti-inflammatory effects, and immunomodulatory effects.89 However, a recent large prospective multicenter cohort study of hospitalized patients with CAP did not find evidence of a protective effect of statins on clinically meaningful outcomes in CAP or significant differences in circulating biomarkers.90 Several randomized trials of statin therapy in patients with both ventilator-associated pneumonia and CAP are under way.
INFLUENZA TREATMENT: MOST EFFECTIVE WITHIN 48 HOURS
Treatment with antiviral drugs is most effective if started within 48 hours after symptom onset, although some patients with confirmed influenza who are either not improving or who are critically ill may still benefit from treatment started later.
Treatment should be considered in patients with laboratory-confirmed or suspected influenza who are at risk of developing complicated influenza and in otherwise healthy patients who wish to reduce the duration of illness or who have close contact with patients who are at high risk of complications.
Antiviral medications are oseltamivir (Tamiflu), zanamivir (Relenza), and the adamantines amantadine (Symmetrel) and rimantadine (Flumadine).
Due to evolving viral resistance patterns, the choice of antiviral drug depends on the strain. Seasonal H1N1 is best treated with zanamivir or an adamantine, while pandemic 2009 H1N1 and H3N2 are best treated with zanamivir or oseltamivir. When strain typing is not available, empiric therapy should be with either zanamivir monotherapy or a combination of oseltamivir plus rimantadine. Influenza B viruses are resistant to adamantines and should be treated only with either zanamivir or oseltamivir.45
FOLLOW-UP AND PREVENTION
Patients with CAP can generally be expected to improve within 3 to 7 days.91 However, it may be several weeks before they return to baseline.92
Follow-up plans may be guided by the time to clinical stability. For patients who do not achieve clinical stability until more than 72 hours after admission, more aggressive follow-up on discharge is indicated, since they are more likely to experience early readmission and death.93
Pneumococcal vaccination. Because S pneumoniae remains the most common cause of CAP, efforts should be made to vaccinate patients appropriately. The Advisory Committee on Immunization Practices (ACIP) and the US Centers for Disease Control and Prevention recommend that the pneumococcal polysaccharide vaccine (Pneumovax 23; PPSV23) be given to those over age 65. Those who were vaccinated before age 65 should receive another dose at age 65 or later if at least 5 years have passed since their previous dose. Those who receive it at or after age 65 should receive only a single dose. A second dose is recommended 5 years after the first dose for people age 19 to 64 years with functional or anatomic asplenia and for those who are immunocompromised.
Influenza vaccination for all. Of note, the ACIP updated its guidelines on influenza vaccination beginning with the 2010–2011 influenza season. It no longer advocates a risk-stratified approach. Instead, it recommends universal influenza vaccination for everybody more than 6 months old.94
Smoking cessation should be addressed. Smoking cessation is a Medicare and Medicaid quality measure and should be encouraged after an episode of CAP because quitting smoking reduces the risk of pneumococcal disease by approximately 14% each year thereafter.95
General internists need to be able to recognize community-acquired pneumonia (CAP) so that diagnostic and therapeutic interventions can be initiated promptly. It is also important to understand the most likely and possible causes of CAP so that appropriate initial antimicrobial therapy can be chosen. Especially during flu season, influenza can present as CAP and should be included in the differential diagnosis.
When managing a patient with CAP, the internist must decide which level of care, diagnostic tests, antimicrobial agents, and follow-up plans are needed. These topics will be reviewed in this article.
TWO TERMS TO REMEMBER
- CAP refers to pneumonia acquired outside a health care facility. It can be either bacterial or viral.
- CABP (community-acquired bacterial pneumonia) refers only to those cases caused by bacterial pathogens.
NUMBERS AND TRENDS
In the United States, CAP is the number-one cause of death from infection and the sixth leading cause of death overall.1 Each year, it is responsible for about 4.2 million outpatient visits, more than 60,000 deaths, and more than $17 billion in health care expenses.2
Community-acquired bacterial pneumonia: Common, serious
In a population-based US study in 1991, the incidence of CABP requiring hospitalization was 266.8 per 100,000 people.3
Estimates of overall mortality in CABP vary depending on the severity of illness and comorbid conditions. A meta-analysis published in 1996 found the overall mortality rate to be 13.7%, with a range of 5.1% to 36.5% depending on severity.4
In hospitalized patients, mortality rates and length of hospital stay appear to be declining over time. Between 1993 and 2005, the age-adjusted mortality rate decreased from 8.9% to 4.1%, and the average length of stay decreased from 7.5 to 5.7 days, with an overall reduction in hospital cost.5
CABP is more prevalent in older people than in the general population, and it increases with age from 18.2 cases per 1,000 patient-years in patients 60 to 69 years to 52.3 cases per 1,000 patient-years in those older than 85 years.6 Risk factors for pneumonia in the elderly include heart disease, chronic lung disease, immunosuppressive drugs, alcoholism, and increasing age.7 Similar to the trend in the general population, the mortality rate in elderly CABP patients appears to be decreasing over time, possibly thanks to rising rates of pneumococcal and influenza vaccination.8
Among the general population, risk factors for developing CABP also include smoking, occupational dust exposure, history of childhood pneumonia, unemployment, and single marital status.9 The incidence of CABP does not appear to be higher among pregnant women, although it is the most frequent cause of nonobstetric death in this population.10
The use of proton pump inhibitors may be an emerging risk factor for CABP.11 Also, use of nonsteroidal anti-inflammatory drugs among patients with CABP is associated with a blunted inflammatory response as well as a higher risk of pleuropulmonary complications and a delay in presentation.12
Influenza is also common, potentially severe
Influenza is also very common and potentially severe. It can cause a spectrum of disease, from mild upper respiratory tract symptoms to severe viral pneumonia that can be life-threatening and complicated by respiratory failure and the acute respiratory distress syndrome (ARDS).
Influenza infection can also be complicated by subsequent bacterial pneumonia. However, the epidemiology of influenza infection differs from that of CABP in that influenza occurs seasonally.
In the United States, seasonal influenza causes 36,000 deaths and 200,000 hospitalizations annually.13,14 As with CABP, the risk of death from influenza increases with age: it is 16 times greater in people age 85 and older than in those ages 65 to 69.13
During yearly seasonal epidemics, those at the highest risk of hospitalization and death are at the extremes of age. Risk factors for complicated influenza include heart disease, lung disease, diabetes, renal failure, rheumatologic conditions, dementia, and neurologic disease.15,16 During the 2009 H1N1 influenza pandemic, unexpected severity was seen in previously healthy young adults as well as those with obesity, neurodegenerative disease, pregnancy, and asthma.17
PATHOGENS: TYPICAL, ATYPICAL, VIRAL
Identifying the etiologic organism in CAP is confounded by limitations in the available diagnostic tests and also by poor-quality specimens that often are contaminated with bacteria that colonize the upper airways. Given these caveats, the primary pathogens responsible for CAP broadly include typical bacterial pathogens, atypical bacterial pathogens, and viruses.
Typical bacterial pathogens include Streptococcus pneumoniae, Haemophilus influenzae, Staphylococcus aureus, Moraxella catarrhalis, and, less commonly, a variety of aerobic and anaerobic gram-negative rods including Pseudomonas aeruginosa, Acinetobacter species, and Klebsiella pneumoniae.
Atypical bacterial pathogens include Mycoplasma pneumoniae, Chlamydia pneumoniae, and Legionella species.18
Viruses implicated in adult CAP include influenza A and B, parainfluenza viruses, respiratory syncytial virus, and adenovirus.19 More recently, human metapneumovirus has been described as a cause of adult CAP.20
Clues to uncommon microbes
Specific historic features or coexisting conditions that may suggest an uncommon microbiologic diagnosis include21:
- Recent travel to the southwestern United States or Southeast Asia
- Ill contacts
- Exposure to birds, bats, rabbits, or farm animals
- Alcoholism
- Chronic obstructive pulmonary disease
- Human immunodeficiency virus infection
- Structural lung disease
- Prolonged cough with whoop or posttussive vomiting
- Aspiration
- Bioterrorism.
In cases in which one or more of these conditions exist, CAP may also be caused by other agents not listed above, including Mycobacterium tuberculosis, oral anaerobes, atypical mycobacteria, Histoplasma capsulatum, Chlamydophila psittaci, Francisella tularensis, Coxiella burnettii, Pneumocystis jiroveci, Cryptococcus, Aspergillus, Coccidioides, Hantavirus, avian influenza, Burkholderia pseudomallei, severe acute respiratory syndrome virus, Bordetella pertussis, Bacillus anthracis, and Yersinia pestis.
HOW BACTERIA INVADE THE LUNGS
The pathophysiology of CABP involves both host defense and microbial virulence factors.
The airways are most commonly exposed to microbes by microaspiration of upper airway flora, although hematogenous seeding of the lungs in a bacteremic patient or contiguous spread of infection from an adjacent site can also occur.
Mucociliary clearance and the cough reflex are important initial defenses against infection and can be inhibited by neurologic diseases and conditions that impair the mucociliary mechanism. Mucosal immune cells, including macrophages and neutrophils, recognize invading pathogens and generate an antibody response.
Regulation of the host inflammatory response to infection depends on a complex interaction between immune cells, inflammatory cytokines (eg, tumor necrosis factor alpha, interleukin 1-beta, and interleukin 6), and anti-inflammatory cytokines such as interleukin 1 receptor antagonist and soluble tumor necrosis factor receptor type I.22
The interaction and timing of the inflammatory and anti-inflammatory response are essential in manifesting an appropriate host response to infection. An inadequate inflammatory response can lead to sepsis and death, but an excessive, late anti-inflammatory response can lead to a systemic inflammatory response such as ARDS. Polymorphisms within the genes coding for these factors may explain the variation in severity of illness among patients with CABP.23
HOW INFLUENZA DOES ITS DAMAGE
There are three types of influenza virus: A, B, and C. Type A causes most human infections. The influenza A virus envelope comprises a lipid bilayer that contains the projecting glycoproteins hemagglutinin and neuraminidase. Influenza viruses are named on the basis of these proteins and are designated with an H and an N, respectively, each followed by a number referring to the subtype.
Influenza infection begins when the virus makes contact with the epithelium. Hemagglutinin binds to the host cell and allows viral entry, where it begins replication. Neuraminidase prevents viral aggregation and facilitates the release of virus from infected cells.24
Mature virions are released and spread to neighboring host cells; this process is associated with desquamation and inflammation of the airways, causing cough, rhinorrhea, and sore throat. Systemic symptoms are associated with the induction of interferon, which causes fever and myalgia.25
Recovery and immunity to influenza infection occurs through both humoral and cell-mediated immunity, with antibodies directed against the specific hemagglutinin and neuraminidase antigens of the infecting virus. Immunity wanes over time and with antigenic drift of circulating viruses, making the host susceptible to recurrent influenza infection.24
Influenza is often complicated by bacterial superinfection
The influenza virus acts synergistically with certain bacteria to increase infectivity, and this may explain why influenza is often complicated by bacterial superinfection.
Mechanisms leading to bacterial superinfection include increased binding and invasion of bacteria, increased viral replication, and modification of the host inflammatory response. Some S aureus strains produce a protease that directly activates influenza virus hemagglutinin; other bacteria can activate plasminogen to promote influenza replication. The resulting increase in proteases in host tissues promotes activation of influenza through cleavage of hemagglutinin.26
The influenza virus also causes damage to the airway epithelial layer, leading to increased exposure of the binding sites necessary for adherence of S pneumoniae.27
CLINICAL PRESENTATION OF COMMUNITY-ACQUIRED PNEUMONIA
Although CAP is common, agreement on its essential clinical signs and symptoms is surprisingly limited, due in part to heterogeneous patient presentations and in part to interobserver variability. The reader is referred to two excellent reviews on this topic.28,29
The diagnosis of CAP is made on clinical grounds, based on a combination of signs and symptoms. Symptoms of pneumonia can include cough, fever, chills, sputum production, dyspnea, and pleuritic pain. Physical findings can include tachypnea, tachycardia, hypoxemia, and consolidation or rales on auscultation. Laboratory data may show leukocytosis or elevated C-reactive protein, and radiographic studies may show evidence of a new infiltrate.21,30,31
Clinical presentation of influenza
Seasonal influenza as a cause of CAP is difficult to distinguish from bacterial causes. The clinical presentation of seasonal influenza most commonly includes fever or subjective feverishness, cough, myalgia, and weakness.32 In a recent multivariate analysis, five clinical features were shown to be predictive of pandemic H1N1 influenza pneumonia rather than CABP: age younger than 65 years, absence of confusion, white blood cell count less than 12 × 109/L, temperature higher than 38°C (100.4°F), and bilateral opacities on radiography.32,33
Complicated influenza infection can be either primary viral pneumonia or bacterial superinfection.
During the 1918 influenza pandemic, which predated the ability to isolate viruses, two clinical syndromes emerged: an ARDS associated with the rapid onset of cyanosis, delirium, and frothy blood-tinged sputum; and an acute bronchopneumonia characterized by necrosis, hemorrhage, edema, and vasculitis.34,35 The first syndrome has subsequently been shown to be associated with primary viral pneumonia, while the second is caused by bacterial superinfection. Modern reexamination of 1918 data has shown that bacterial superinfection was likely the reason for the distinctly fulminant presentation of that pandemic.36,37
The 2009 H1N1 influenza pandemic caused relatively mild disease in most patients. However, those with severe pneumonia more commonly developed ARDS from primary influenza pneumonia than from bacterial superinfection.17
A third influenza-associated infection is secondary bacterial pneumonia, which follows influenza infection and mimics the presentation of CABP. A typical patient presents with a recent history of influenza-like illness, followed 4 to 14 days later by a recurrence of fever, dyspnea, productive cough, and consolidation on chest radiographs.38 Leukocytosis with an increased number of immature neutrophil forms, prolonged duration of fever, and elevated erythrocyte sedimentation rate are more likely in patients with secondary bacterial pneumonia.39 Isolates from sputum samples commonly include S pneumoniae, S aureus, H influenzae, and other gram-negative rods.40
In recent flu seasons, methicillin-resistant S aureus (MRSA) has emerged as a cause of severe secondary pneumonia. Most of these isolates carry genes for the toxin Panton-Valentine leukocidin; the associated mortality rate is as high as 33%.41,42 Although community-acquired MRSA pneumonia has only been reported in case series, distinct clinical features that have been described include severe pneumonia with high fever, hypotension, shock, respiratory failure, leukopenia, and multilobar and cavitary infiltrates.43
WHEN TO SUSPECT INFLUENZA
The triad of fever, cough, and abrupt onset are the best predictors of influenza, but no single combination of signs and symptoms predict influenza infection with 100% certainty. Therefore, an understanding of local epidemiologic data regarding circulating influenza is essential to maintain a high index of suspicion.44
It is appropriate to suspect influenza in:
- Anyone who is epidemiologically linked to a known outbreak of influenza
- Children, adults, and health care workers who have fever and abrupt onset of respiratory symptoms
- Patients with fever plus exacerbation of underlying pulmonary disease
- Severely ill patients with fever or hypothermia, especially during influenza season.45
DIAGNOSTIC TESTING
Once the diagnosis of pulmonary infection is suggested by clinical features, the initial evaluation should include measurement of vital signs, physical examination, and radiographic imaging of the chest. Additional diagnostic measures to consider include viral testing, blood culture, sputum culture, urinary antigen testing for Legionella and for S pneumoniae, fungal culture, and mycobacterial smear and culture.
Chest radiography (with posterior-anterior and lateral films) is the study that usually demonstrates the presence of a pulmonary infiltrate. If initial chest radiographs do not show an infiltrate, imaging can be repeated after treatment is started if the patient’s clinical presentation still suggests pneumonia. Chest radiographs are of limited value in predicting the pathogen, but they help to determine the extent of pneumonia and to detect parapneumonic effusion.46
A caveat: anterior-posterior, posterior-anterior, and lateral views can miss more than 10% of effusions large enough to warrant thoracentesis, especially when there is lower-lobe consolidation.47
Blood cultures are recommended for patients admitted to the intensive care unit and for those with cavitary infiltrates, leukopenia, alcohol abuse, severe liver disease, asplenia, positive pneumococcal urinary antigen testing, or a pleural effusion.21 However, blood cultures are positive in only 3% to 14% of hospitalized patients with CABP, and the impact of a positive blood culture on management decisions in CABP appears to be quite small.48–50
For the highest yield, blood culture results should be obtained before antibiotics are given. Not only is this good practice, but obtaining blood culture results before starting antibiotics is one of the quality measures evaluated by the Center for Medicare and Medicaid Services.51
Sputum culture is considered optional for outpatients and patients with less-severe pneumonia.21 While it can provide a rapid diagnosis in certain cases, a good-quality sputum sample is obtained in only 39% to 54% of patients with CABP, yields a predominant morphotype in only 45% of cases, and provides a useful microbiologic diagnosis in only 14.4%.52,53 Fungal and mycobacterial cultures are only indicated in certain situations such as cavitary infiltrates or immunosuppression.
Urinary antigen testing for Legionella and S pneumoniae should be done in patients with more severe illness and in those for whom outpatient therapy has failed.21S pneumoniae testing has been shown to allow early diagnosis of pneumococcal pneumonia in 26% more patients than with Gram staining, but it fails to identify 22% of the rapid diagnoses initially identified by Gram staining.54 Thus, a sequential approach is reasonable, with urinary antigen testing for patients at high risk without useful results from sputum Gram staining. Also, recent data suggest that the pneumococcal urinary antigen test may allow optimization of antimicrobial therapy with good clinical outcomes.55
Endotracheal tests. If the patient is intubated, collection of endotracheal aspirates, bronchoscopy, or nonbronchoscopic bronchial lavage (sometimes called “mini-BAL”) should be performed.
Thoracentesis and pleural fluid cultures should be done if a pleural effusion is found. Empyema, large or loculated effusions, and parapneumonic effusions with a pH lower than 7.20, glucose levels less than 3.4 mmol/L (60 mg/dL), or positive results on microbial staining or culture should be drained by chest tube or surgically.56
Testing for influenza should be done if it will change the clinical management, such as the choice of antibiotic or infection control practices. Specimens should be obtained with either a nasopharyngeal swab or aspirate and tested with reverse transcriptase polymerase chain reaction, immunofluorescent staining, or rapid antigen detection, depending on local availability.45
Inflammatory biomarkers such as C-reactive protein and procalcitonin have been receiving interest as ways to predict the etiology and prognosis of CAP and to guide therapy. Several studies have shown that C-reactive protein can help distinguish between CAP and bronchitis, with higher values suggesting more severe pneumonia and pneumonia caused by S pneumoniae or L pneumophila.57 Procalcitonin may help discriminate between severe lower respiratory tract infections of bacterial and 2009 H1N1 origin, although less effectively than C-reactive protein. Low procalcitonin values, particularly when combined with low C-reactive protein levels, suggest that bacterial infection is unlikely.58
RISK STRATIFICATION AND SITE-OF-CARE DECISIONS
Following a presumptive diagnosis of CAP, it is important to decide not only what treatment the patient will receive but whether he or she should be hospitalized. If the patient is to be admitted to the hospital, the clinician must also decide if his or her condition warrants intensive care.
Severity-of-illness scores
A recent meta-analysis compared the performance characteristics of the PSI and CURB-65 scores for predicting mortality in CAP and found no significant differences in overall test performance.61
Another meta-analysis found that the PSI was more sensitive than the CURB-65 and had a low false-negative rate, and so was better at showing which patients do not need to be hospitalized. Conversely, the CURB-65 was more specific and had a higher positive predictive value, and thus was more likely to correctly classify high-risk patients.62
Other scoring systems that aid in deciding about hospital admission and level of care include the CRB-6563 (which can be used instead of the CURB-65 if laboratory values are not available), SMART-COP,64 and SCAP.,65
Guidelines on when to admit to the intensive care unit
Guidelines from the Infectious Diseases Society of America (IDSA) and the American Thoracic Society (ATS) also provide guidance on when intensive care admission is advised,21 and their criteria were recently validated.66 The guidelines advocate direct admission to the intensive care unit for patients requiring vasopressors or mechanical ventilation, and intensive care unit or high-level monitoring for patients with three of the following criteria for severe CAP21:
- Respiratory rate ≥ 30
- Pao2/Fio2 ratio ≤ 250
- Multilobar infiltrates
- Confusion or disorientation
- Uremia (blood urea nitrogen ≥ 20 mg/dL)
- Leukopenia (white blood cell count < 4.0 × 109/L)
- Thrombocytopenia (platelet count < 100 × 109/L)
- Hypothermia (core temperature < 36.0°C [96.8°F])
- Hypotension requiring aggressive fluid resuscitation.
None of these scoring systems or criteria is meant to replace clinical judgment. A recent study has suggested that an oxygen saturation of less than 92% is an appropriate threshold for hospital admission, in view of higher rates of adverse events in outpatients with saturations below this value.67
TREATMENT
Multiple studies have shown that treatment of CAP in accordance with guidelines has led to improved clinical outcomes.21,68–70
How fast must antibiotics be started?
Based on studies that showed a lower mortality rate when antibiotics were started sooner, Medicare and Medicaid adopted a quality measure calling for starting antibiotics within 4 hours in patients being admitted to the hospital.50,71 However, several subsequent studies showed that the diagnosis of pneumonia is often incorrect and that rapid administration of antibiotics could lead to misdiagnosis, overuse of antibiotics, and a higher risk of Clostridium difficile infection.72,73
The current IDSA/ATS guidelines21 recommend that the first antibiotic dose be given while the patient is still in the emergency department, but do not give a specific time within which it should be given. Medicare and Medicaid later updated their quality measure to antibiotic administration within 6 hours.
Which antibiotics should be used?
The selection of antimicrobial agent depends upon the patient’s severity of illness and comorbid conditions.
Although most studies of combination antibiotic therapy have been retrospective and observational, they suggest that a macrolide (ie, one of the “mycins”) added to a beta-lactam antibiotic is beneficial, possibly by covering atypical organisms or via anti-inflammatory action.74–76 The choice of one antibiotic over another appears to be less important, and a recent Cochrane review concluded that there was no significant difference in efficacy among five antibiotic pairs studied.77
Empiric outpatient treatment of a previously healthy patient with CAP and no risk factors for drug-resistant S pneumoniae should include either a macrolide (azithromycin [Zithromax], clarithromycin [Biaxin], or erythromycin) or doxycycline. If the patient has a chronic comorbid condition such as heart, lung, liver, or renal disease, diabetes mellitus, alcoholism, malignancy, asplenia, or immunosuppression or has received antimicrobials within the preceding 3 months, then treatment should include either a respiratory fluoroquinolone (moxifloxacin [Avelox] or levofloxacin [Levaquin]) or a beta-lactam plus a macrolide.21
Overall, published data suggest that the survival rate is about the same with fluoroquinolone monotherapy as with beta-lactam plus macrolide combination therapy, and better than with beta-lactam monotherapy.78
Selection of antibiotics for inpatient treatment of CAP is influenced by severity of illness. Inpatients who do not require intensive care should be treated with either a respiratory fluoroquinolone or combination therapy with a beta-lactam (cefotaxime [Claforan], ceftriaxone [Rocephin], ampicillin, or ertapenem [Invanz]) plus a macrolide or doxycycline.21,76,79
If a specific microbiologic diagnosis is made, then treatment can be narrowed. However in certain cases, such as invasive pneumococcal infection, combination therapy may still be superior.80,81 For patients who need intensive care, treatment should always include a beta-lactam plus either azithromycin or a respiratory fluoroquinolone.21 In certain situations, additional antibiotics may be added as well, such as agents to treat Pseudomonas, community-acquired MRSA, or both.
Switching to oral therapy; short-course therapy
In the interest of avoiding unnecessary antibiotics, numerous studies have addressed the issue of an “early switch” to oral antibiotics and “short-course” therapy for CAP. In general, once clinically stable, patients with CAP, including bacteremic S pneumoniae pneumonia, can be safely switched to oral antibiotics.82
The issue of short-course therapy is more complicated, and the appropriate length of therapy for CAP is not well established. However, 5 days of levofloxacin 750 mg was shown to be as successful as 7 to 10 days of levofloxacin 500 mg.83 In another study, in patients who improved after 3 days of intravenous therapy for CAP, there was no difference in clinical outcome between those who were changed to oral therapy for 5 more days and those who received an oral placebo.84
Most patients who achieve clinical stability in the first week do not need prolonged antibiotic therapy. However, certain conditions, such as S aureus bacteremic pneumonia, complicated pneumonia, and pneumonia due to unusual organisms, may require prolonged treatment.
Other therapies
Additional therapies studied in patients with pneumonia include early mobilization, adjunctive corticosteroids, and statin drugs.
Early mobilization was shown in one study to decrease hospital length of stay without increasing adverse effects.85
Corticosteroids are not supported as a standard of care for patients with severe CAP according to current available studies.86,87 Furthermore, a randomized, controlled trial showed that prednisolone daily for a week did not improve outcomes in hospitalized patients with CAP, and it was associated with increased late failure.88
Statin trials under way. Several observational studies have suggested that statins might be beneficial in managing sepsis through their effects on endothelial cell function, antioxidant effects, anti-inflammatory effects, and immunomodulatory effects.89 However, a recent large prospective multicenter cohort study of hospitalized patients with CAP did not find evidence of a protective effect of statins on clinically meaningful outcomes in CAP or significant differences in circulating biomarkers.90 Several randomized trials of statin therapy in patients with both ventilator-associated pneumonia and CAP are under way.
INFLUENZA TREATMENT: MOST EFFECTIVE WITHIN 48 HOURS
Treatment with antiviral drugs is most effective if started within 48 hours after symptom onset, although some patients with confirmed influenza who are either not improving or who are critically ill may still benefit from treatment started later.
Treatment should be considered in patients with laboratory-confirmed or suspected influenza who are at risk of developing complicated influenza and in otherwise healthy patients who wish to reduce the duration of illness or who have close contact with patients who are at high risk of complications.
Antiviral medications are oseltamivir (Tamiflu), zanamivir (Relenza), and the adamantines amantadine (Symmetrel) and rimantadine (Flumadine).
Due to evolving viral resistance patterns, the choice of antiviral drug depends on the strain. Seasonal H1N1 is best treated with zanamivir or an adamantine, while pandemic 2009 H1N1 and H3N2 are best treated with zanamivir or oseltamivir. When strain typing is not available, empiric therapy should be with either zanamivir monotherapy or a combination of oseltamivir plus rimantadine. Influenza B viruses are resistant to adamantines and should be treated only with either zanamivir or oseltamivir.45
FOLLOW-UP AND PREVENTION
Patients with CAP can generally be expected to improve within 3 to 7 days.91 However, it may be several weeks before they return to baseline.92
Follow-up plans may be guided by the time to clinical stability. For patients who do not achieve clinical stability until more than 72 hours after admission, more aggressive follow-up on discharge is indicated, since they are more likely to experience early readmission and death.93
Pneumococcal vaccination. Because S pneumoniae remains the most common cause of CAP, efforts should be made to vaccinate patients appropriately. The Advisory Committee on Immunization Practices (ACIP) and the US Centers for Disease Control and Prevention recommend that the pneumococcal polysaccharide vaccine (Pneumovax 23; PPSV23) be given to those over age 65. Those who were vaccinated before age 65 should receive another dose at age 65 or later if at least 5 years have passed since their previous dose. Those who receive it at or after age 65 should receive only a single dose. A second dose is recommended 5 years after the first dose for people age 19 to 64 years with functional or anatomic asplenia and for those who are immunocompromised.
Influenza vaccination for all. Of note, the ACIP updated its guidelines on influenza vaccination beginning with the 2010–2011 influenza season. It no longer advocates a risk-stratified approach. Instead, it recommends universal influenza vaccination for everybody more than 6 months old.94
Smoking cessation should be addressed. Smoking cessation is a Medicare and Medicaid quality measure and should be encouraged after an episode of CAP because quitting smoking reduces the risk of pneumococcal disease by approximately 14% each year thereafter.95
- Mortensen EM, Kapoor WN, Chang CC, Fine MJ. Assessment of mortality after long-term follow-up of patients with community-acquired pneumonia. Clin Infect Dis 2003; 37:1617–1624.
- File TM, Marrie TJ. Burden of community-acquired pneumonia in North American adults. Postgrad Med 2010; 122:130–141.
- Marston BJ, Plouffe JF, File TM, et al. Incidence of community-acquired pneumonia requiring hospitalization. Results of a population-based active surveillance study in Ohio. The Community-Based Pneumonia Incidence Study Group. Arch Intern Med 1997; 157:1709–1718.
- Fine MJ, Smith MA, Carson CA, et al. Prognosis and outcomes of patients with community-acquired pneumonia. JAMA 1996; 275:134–141.
- Ruhnke GW, Coca-Perraillon M, Kitch BT, Cutler DM. Trends in mortality and medical spending in patients hospitalized for community-acquired pneumonia: 1993–2005. Med Care 2010; 48:1111–1116.
- Jackson ML, Neuzil KM, Thompson WW, et al. The burden of community-acquired pneumonia in seniors: results of a population-based study. Clin Infect Dis 2004; 39:1642–1650.
- Koivula I, Sten M, Mäkelä PH. Risk factors for pneumonia in the elderly. Am J Med 1994; 96:313–320.
- Ruhnke GW, Coca-Perraillon M, Kitch BT, Cutler DM. Marked reduction in 30-day mortality among elderly patients with community-acquired pneumonia. Am J Med 2011; 124:171–178.
- Farr BM, Bartlett CL, Wadsworth J, Miller DL. Risk factors for community-acquired pneumonia diagnosed upon hospital admission. British Thoracic Society Pneumonia Study Group. Respir Med 2000; 94:954–963.
- Graves CR. Pneumonia in pregnancy. Clin Obstet Gynecol 2010; 53:329–336.
- Eom CS, Jeon CY, Lim JW, Cho EG, Park SM, Lee KS. Use of acid-suppressive drugs and risk of pneumonia: a systematic review and meta-analysis. CMAJ 2011; 183:310–319.
- Voiriot G, Dury S, Parrot A, Mayaud C, Fartoukh M. Nonsteroidal antiinflammatory drugs may affect the presentation and course of community-acquired pneumonia. Chest 2011; 139:387–394.
- Thompson WW, Shay DK, Weintraub E, et al. Mortality associated with influenza and respiratory syncytial virus in the United States. JAMA 2003; 289:179–186.
- Thompson WW, Shay DK, Weintraub E, et al. Influenza-associated hospitalizations in the United States. JAMA 2004; 292:1333–1340.
- Glezen WP, Decker M, Perrotta DM. Survey of underlying conditions of persons hospitalized with acute respiratory disease during influenza epidemics in Houston, 1978–1981. Am Rev Respir Dis 1987; 136:550–555.
- Izurieta HS, Thompson WW, Kramarz P, et al. Influenza and the rates of hospitalization for respiratory disease among infants and young children. N Engl J Med 2000; 342:232–239.
- Rothberg MB, Haessler SD. Complications of seasonal and pandemic influenza. Crit Care Med 2010; 38(suppl 4):e91–e97.
- Apisarnthanarak A, Mundy LM. Etiology of community-acquired pneumonia. Clin Chest Med 2005; 26:47–55.
- de Roux A, Marcos MA, Garcia E, et al. Viral community-acquired pneumonia in nonimmunocompromised adults. Chest 2004; 125:1343–1351.
- Hamelin ME, Côté S, Laforge J, et al. Human metapneumovirus infection in adults with community-acquired pneumonia and exacerbation of chronic obstructive pulmonary disease. Clin Infect Dis 2005; 41:498–502.
- Mandell LA, Wunderink RG, Anzueto A, et al; Infectious Diseases Society of America; American Thoracic Society. Infectious Diseases Society of America/American Thoracic Society consensus guidelines on the management of community-acquired pneumonia in adults. Clin Infect Dis 2007; 44(suppl 2):S27–S72.
- Kolling UK, Hansen F, Braun J, Rink L, Katus HA, Dalhoff K. Leucocyte response and anti-inflammatory cytokines in community acquired pneumonia. Thorax 2001; 56:121–125.
- Wunderink RG, Waterer GW. Community-acquired pneumonia: pathophysiology and host factors with focus on possible new approaches to management of lower respiratory tract infections. Infect Dis Clin North Am 2004; 18:743–759.
- Hilleman MR. Realities and enigmas of human viral influenza: pathogenesis, epidemiology and control. Vaccine 2002; 20:3068–3087.
- Bender BS, Small PA. Influenza: pathogenesis and host defense. Semin Respir Infect 1992; 7:38–45.
- Scheiblauer H, Reinacher M, Tashiro M, Rott R. Interactions between bacteria and influenza A virus in the development of influenza pneumonia. J Infect Dis 1992; 166:783–791.
- McCullers JA. Insights into the interaction between influenza virus and pneumococcus. Clin Microbiol Rev 2006; 19:571–582.
- Metlay JP, Kapoor WN, Fine MJ. Does this patient have community-acquired pneumonia? Diagnosing pneumonia by history and physical examination. JAMA 1997; 278:1440–1445.
- Benbassat J, Baumal R. Narrative review: should teaching of the respiratory physical examination be restricted only to signs with proven reliability and validity? J Gen Intern Med 2010; 25:865–872.
- Kolsuz M, Erginel S, Alatas O, et al. Acute phase reactants and cytokine levels in unilateral community-acquired pneumonia. Respiration 2003; 70:615–622.
- Alves DW, Kennedy MT. Community-acquired pneumonia in casualty: etiology, clinical features, diagnosis, and management (or a look at the “new” in pneumonia since 2002). Curr Opin Pulm Med 2004; 10:166–170.
- Monto AS, Gravenstein S, Elliott M, Colopy M, Schweinle J. Clinical signs and symptoms predicting influenza infection. Arch Intern Med 2000; 160:3243–3247.
- Bewick T, Myles P, Greenwood S, et al; Influenza Clinical Information Network. Clinical and laboratory features distinguishing pandemic H1N1 influenza-related pneumonia from interpandemic community-acquired pneumonia in adults. Thorax 2011; 66:247–252.
- Morens DM, Fauci AS. The 1918 influenza pandemic: insights for the 21st century. J Infect Dis 2007; 195:1018–1028.
- Starr I. Influenza in 1918: recollections of the epidemic in Philadelphia. 1976. Ann Intern Med 2006; 145:138–140.
- Morens DM, Taubenberger JK, Fauci AS. Predominant role of bacterial pneumonia as a cause of death in pandemic influenza: implications for pandemic influenza preparedness. J Infect Dis 2008; 198:962–970.
- Brundage JF, Shanks GD. Deaths from bacterial pneumonia during 1918–19 influenza pandemic. Emerg Infect Dis 2008; 14:1193–1199.
- Treanor J. Influenza virus. In: Mandell GL, Bennett JE, Dolin R, eds. Principles and Practice of Infectious Diseases. 6th ed. Philadelphia, PA: Elsevier/Churchill Livingstone; 2005:2060–2085.
- Jarstrand C, Tunevall G. The influence of bacterial superinfection on the clinical course of influenza. Studies from the influenza epidemics in Stockholm during the winters 1969–70 and 1971–72. Scand J Infect Dis 1975; 7:243–247.
- Schwarzmann SW, Adler JL, Sullivan RJ, Marine WM. Bacterial pneumonia during the Hong Kong influenza epidemic of 1968–1969. Arch Intern Med 1971; 127:1037–1041.
- Hageman JC, Uyeki TM, Francis JS, et al. Severe community-acquired pneumonia due to Staphylococcus aureus, 2003–04 influenza season. Emerg Infect Dis 2006; 12:894–899.
- Centers for Disease Control and Prevention (CDC). Severe methicillin-resistant Staphylococcus aureus community-acquired pneumonia associated with influenza—Louisiana and Georgia, December 2006–January 2007. MMWR Morb Mortal Wkly Rep 2007; 56:325–329.
- Hidron AI, Low CE, Honig EG, Blumberg HM. Emergence of community-acquired methicillin-resistant Staphylococcus aureus strain USA300 as a cause of necrotising community-onset pneumonia. Lancet Infect Dis 2009; 9:384–392.
- Call SA, Vollenweider MA, Hornung CA, Simel DL, McKinney WP. Does this patient have influenza? JAMA 2005; 293:987–997.
- Harper SA, Bradley JS, Englund JA, et al; Expert Panel of the Infectious Diseases Society of America. Seasonal influenza in adults and children—diagnosis, treatment, chemoprophylaxis, and institutional outbreak management: clinical practice guidelines of the Infectious Diseases Society of America. Clin Infect Dis 2009; 48:1003–1032.
- Boersma WG, Daniels JM, Löwenberg A, Boeve WJ, van de Jagt EJ. Reliability of radiographic findings and the relation to etiologic agents in community-acquired pneumonia. Respir Med 2006; 100:926–932.
- Brixey AG, Luo Y, Skouras V, Awdankiewicz A, Light RW. The efficacy of chest radiographs in detecting parapneumonic effusions. Respirology 2011; 16:1000–1004.
- Campbell SG, Marrie TJ, Anstey R, Dickinson G, Ackroyd-Stolarz S. The contribution of blood cultures to the clinical management of adult patients admitted to the hospital with community-acquired pneumonia: a prospective observational study. Chest 2003; 123:1142–1150.
- Waterer GW, Wunderink RG. The influence of the severity of community-acquired pneumonia on the usefulness of blood cultures. Respir Med 2001; 95:78–82.
- Houck PM, Bratzler DW, Nsa W, Ma A, Bartlett JG. Timing of antibiotic administration and outcomes for Medicare patients hospitalized with community-acquired pneumonia. Arch Intern Med 2004; 164:637–644.
- Information & Quality Healthcare. http://www.IQH.org/attachments/219_CoreMHelpBookletpg4_11_3.pdf. Accessed November 14, 2011.
- Rosón B, Carratalà J, Verdaguer R, Dorca J, Manresa F, Gudiol F. Prospective study of the usefulness of sputum Gram stain in the initial approach to community-acquired pneumonia requiring hospitalization. Clin Infect Dis 2000; 31:869–874.
- García-Vázquez E, Marcos MA, Mensa J, et al. Assessment of the usefulness of sputum culture for diagnosis of community-acquired pneumonia using the PORT predictive scoring system. Arch Intern Med 2004; 164:1807–1811.
- Rosón B, Fernández-Sabé N, Carratalà J, et al. Contribution of a urinary antigen assay (Binax NOW) to the early diagnosis of pneumococcal pneumonia. Clin Infect Dis 2004; 38:222–226.
- Sordé R, Falcó V, Lowak M, et al. Current and potential usefulness of pneumococcal urinary antigen detection in hospitalized patients with community-acquired pneumonia to guide antimicrobial therapy. Arch Intern Med 2011; 171:166–172.
- Koegelenberg CFN, Diacon AH, Bolliger CT. Parapneumonic pleural effusion and empyema. Respiration 2008; 75:241–250.
- Almirall J, Bolíbar I, Toran P, et al; Community-Acquired Pneumonia Maresme Study Group. Contribution of C-reactive protein to the diagnosis and assessment of severity of community-acquired pneumonia. Chest 2004; 125:1335–1342.
- Ingram PR, Inglis T, Moxon D, Speers D. Procalcitonin and C-reactive protein in severe 2009 H1N1 influenza infection. Intensive Care Med 2010; 36:528–532.
- Fine MJ, Auble TE, Yealy DM, et al. A prediction rule to identify low-risk patients with community-acquired pneumonia. N Engl J Med 1997; 336:243–250.
- Lim WS, van der Eerden MM, Laing R, et al. Defining community acquired pneumonia severity on presentation to hospital: an international derivation and validation study. Thorax 2003; 58:377–382.
- Chalmers JD, Singanayagam A, Akram AR, et al. Severity assessment tools for predicting mortality in hospitalised patients with community-acquired pneumonia. Systematic review and meta-analysis. Thorax 2010; 65:878–883.
- Loke YK, Kwok CS, Niruban A, Myint PK. Value of severity scales in predicting mortality from community-acquired pneumonia: systematic review and meta-analysis. Thorax 2010; 65:884–890.
- Capelastegui A, España PP, Quintana JM, et al. Validation of a predictive rule for the management of community-acquired pneumonia. Eur Respir J 2006; 27:151–157.
- Charles PG, Wolfe R, Whitby M, et al; Australian Community-Acquired Pneumonia Study Collaboration. SMART-COP: a tool for predicting the need for intensive respiratory or vasopressor support in community-acquired pneumonia. Clin Infect Dis 2008; 47:375–384.
- España PP, Capelastegui A, Gorordo I, et al. Development and validation of a clinical prediction rule for severe community-acquired pneumonia. Am J Respir Crit Care Med 2006; 174:1249–1256.
- Chalmers JD, Taylor JK, Mandal P, et al. Validation of the Infectious Diseases Society of America/American Thoracic Society minor criteria for intensive care unit admission in community-acquired pneumonia patients without major criteria or contraindications to intensive care unit care. Clin Infect Dis 2011; 53:503–511.
- Majumdar SR, Eurich DT, Gamble JM, Senthilselvan A, Marrie TJ. Oxygen saturations less than 92% are associated with major adverse events in outpatients with pneumonia: a population-based cohort study. Clin Infect Dis 2011; 52:325–331.
- Nathwani D, Rubinstein E, Barlow G, Davey P. Do guidelines for community-acquired pneumonia improve the cost-effectiveness of hospital care? Clin Infect Dis 2001; 32:728–741.
- Dean NC, Silver MP, Bateman KA, James B, Hadlock CJ, Hale D. Decreased mortality after implementation of a treatment guideline for community-acquired pneumonia. Am J Med 2001; 110:451–457.
- Capelastegui A, España PP, Quintana JM, et al. Improvement of process-of-care and outcomes after implementing a guideline for the management of community-acquired pneumonia: a controlled before-and-after design study. Clin Infect Dis 2004; 39:955–963.
- Silber SH, Garrett C, Singh R, et al. Early administration of antibiotics does not shorten time to clinical stability in patients with moderate-to-severe community-acquired pneumonia. Chest 2003; 124:1798–1804.
- Welker JA, Huston M, McCue JD. Antibiotic timing and errors in diagnosing pneumonia. Arch Intern Med 2008; 168:351–356.
- Polgreen PM, Chen YY, Cavanaugh JE, et al. An outbreak of severe Clostridium difficile-associated disease possibly related to inappropriate antimicrobial therapy for community-acquired pneumonia. Infect Control Hosp Epidemiol 2007; 28:212–214.
- Waterer GW, Somes GW, Wunderink RG. Monotherapy may be suboptimal for severe bacteremic pneumococcal pneumonia. Arch Intern Med 2001; 161:1837–1842.
- Lodise TP, Kwa A, Cosler L, Gupta R, Smith RP. Comparison of beta-lactam and macrolide combination therapy versus fluoroquinolone monotherapy in hospitalized Veterans Affairs patients with community-acquired pneumonia. Antimicrob Agents Chemother 2007; 51:3977–3982.
- Waterer GW, Rello J, Wunderink RG. Management of community-acquired pneumonia in adults. Am J Respir Crit Care Med 2011; 183:157–164.
- Bjerre LM, Verheij TJ, Kochen MM. Antibiotics for community acquired pneumonia in adult outpatients. Cochrane Database Syst Rev 2009; (4):CD002109.
- Frei CR, Labreche MJ, Attridge RT. Fluoroquinolones in community-acquired pneumonia: guide to selection and appropriate use. Drugs 2011; 71:757–770.
- Weiss K, Tillotson GS. The controversy of combination vs monotherapy in the treatment of hospitalized community-acquired pneumonia. Chest 2005; 128:940–946.
- Martínez JA, Horcajada JP, Almela M, et al. Addition of a macrolide to a beta-lactam-based empirical antibiotic regimen is associated with lower in-hospital mortality for patients with bacteremic pneumococcal pneumonia. Clin Infect Dis 2003; 36:389–395.
- Waterer GW, Somes GW, Wunderink RG. Monotherapy may be suboptimal for severe bacteremic pneumococcal pneumonia. Arch Intern Med 2001; 161:1837–1842.
- Ramirez JA, Bordon J. Early switch from intravenous to oral antibiotics in hospitalized patients with bacteremic community-acquired Streptococcus pneumoniae pneumonia. Arch Intern Med 2001; 161:848–850.
- Dunbar LM, Wunderink RG, Habib MP, et al. High-dose, short-course levofloxacin for community-acquired pneumonia: a new treatment paradigm. Clin Infect Dis 2003; 37:752–760.
- el Moussaoui R, de Borgie CA, van den Broek P, et al. Effectiveness of discontinuing antibiotic treatment after three days versus eight days in mild to moderate-severe community acquired pneumonia: randomised, double blind study. BMJ 2006; 332:1355.
- Mundy LM, Leet TL, Darst K, Schnitzler MA, Dunagan WC. Early mobilization of patients hospitalized with community-acquired pneumonia. Chest 2003; 124:883–889.
- Salluh JI, Póvoa P, Soares M, Castro-Faria-Neto HC, Bozza FA, Bozza PT. The role of corticosteroids in severe community-acquired pneumonia: a systematic review. Crit Care 2008; 12:R76.
- Mikami K, Suzuki M, Kitagawa H, et al. Efficacy of corticosteroids in the treatment of community-acquired pneumonia requiring hospitalization. Lung 2007; 185:249–255.
- Snijders D, Daniels JM, de Graaff CS, van der Werf TS, Boersma WG. Efficacy of corticosteroids in community-acquired pneumonia: a randomized double-blinded clinical trial. Am J Respir Crit Care Med 2010; 181:975–982.
- Chopra V, Flanders SA. Does statin use improve pneumonia outcomes? Chest 2009; 136:1381–1388.
- Yende S, Milbrandt EB, Kellum JA, et al. Understanding the potential role of statins in pneumonia and sepsis. Crit Care Med 2011; 39:1871–1878.
- Halm EA, Fine MJ, Marrie TJ, et al. Time to clinical stability in patients hospitalized with community-acquired pneumonia: implications for practice guidelines. JAMA 1998; 279:1452–1457.
- Marrie TJ, Lau CY, Wheeler SL, Wong CJ, Feagan BG. Predictors of symptom resolution in patients with community-acquired pneumonia. Clin Infect Dis 2000; 31:1362–1367.
- Aliberti S, Peyrani P, Filardo G, et al. Association between time to clinical stability and outcomes after discharge in hospitalized patients with community-acquired pneumonia. Chest 2011; 140:482–488.
- Fiore AE, Uyeki TM, Broder K, et al; Centers for Disease Control and Prevention (CDC). Prevention and control of influenza with vaccines: recommendations of the Advisory Committee on Immunization Practices (ACIP), 2010. MMWR Recomm Rep 2010; 59:1–62.
- Nuorti JP, Butler JC, Farley MM, et al. Cigarette smoking and invasive pneumococcal disease. Active Bacterial Core Surveillance Team. N Engl J Med 2000; 342:681–689.
- Mortensen EM, Kapoor WN, Chang CC, Fine MJ. Assessment of mortality after long-term follow-up of patients with community-acquired pneumonia. Clin Infect Dis 2003; 37:1617–1624.
- File TM, Marrie TJ. Burden of community-acquired pneumonia in North American adults. Postgrad Med 2010; 122:130–141.
- Marston BJ, Plouffe JF, File TM, et al. Incidence of community-acquired pneumonia requiring hospitalization. Results of a population-based active surveillance study in Ohio. The Community-Based Pneumonia Incidence Study Group. Arch Intern Med 1997; 157:1709–1718.
- Fine MJ, Smith MA, Carson CA, et al. Prognosis and outcomes of patients with community-acquired pneumonia. JAMA 1996; 275:134–141.
- Ruhnke GW, Coca-Perraillon M, Kitch BT, Cutler DM. Trends in mortality and medical spending in patients hospitalized for community-acquired pneumonia: 1993–2005. Med Care 2010; 48:1111–1116.
- Jackson ML, Neuzil KM, Thompson WW, et al. The burden of community-acquired pneumonia in seniors: results of a population-based study. Clin Infect Dis 2004; 39:1642–1650.
- Koivula I, Sten M, Mäkelä PH. Risk factors for pneumonia in the elderly. Am J Med 1994; 96:313–320.
- Ruhnke GW, Coca-Perraillon M, Kitch BT, Cutler DM. Marked reduction in 30-day mortality among elderly patients with community-acquired pneumonia. Am J Med 2011; 124:171–178.
- Farr BM, Bartlett CL, Wadsworth J, Miller DL. Risk factors for community-acquired pneumonia diagnosed upon hospital admission. British Thoracic Society Pneumonia Study Group. Respir Med 2000; 94:954–963.
- Graves CR. Pneumonia in pregnancy. Clin Obstet Gynecol 2010; 53:329–336.
- Eom CS, Jeon CY, Lim JW, Cho EG, Park SM, Lee KS. Use of acid-suppressive drugs and risk of pneumonia: a systematic review and meta-analysis. CMAJ 2011; 183:310–319.
- Voiriot G, Dury S, Parrot A, Mayaud C, Fartoukh M. Nonsteroidal antiinflammatory drugs may affect the presentation and course of community-acquired pneumonia. Chest 2011; 139:387–394.
- Thompson WW, Shay DK, Weintraub E, et al. Mortality associated with influenza and respiratory syncytial virus in the United States. JAMA 2003; 289:179–186.
- Thompson WW, Shay DK, Weintraub E, et al. Influenza-associated hospitalizations in the United States. JAMA 2004; 292:1333–1340.
- Glezen WP, Decker M, Perrotta DM. Survey of underlying conditions of persons hospitalized with acute respiratory disease during influenza epidemics in Houston, 1978–1981. Am Rev Respir Dis 1987; 136:550–555.
- Izurieta HS, Thompson WW, Kramarz P, et al. Influenza and the rates of hospitalization for respiratory disease among infants and young children. N Engl J Med 2000; 342:232–239.
- Rothberg MB, Haessler SD. Complications of seasonal and pandemic influenza. Crit Care Med 2010; 38(suppl 4):e91–e97.
- Apisarnthanarak A, Mundy LM. Etiology of community-acquired pneumonia. Clin Chest Med 2005; 26:47–55.
- de Roux A, Marcos MA, Garcia E, et al. Viral community-acquired pneumonia in nonimmunocompromised adults. Chest 2004; 125:1343–1351.
- Hamelin ME, Côté S, Laforge J, et al. Human metapneumovirus infection in adults with community-acquired pneumonia and exacerbation of chronic obstructive pulmonary disease. Clin Infect Dis 2005; 41:498–502.
- Mandell LA, Wunderink RG, Anzueto A, et al; Infectious Diseases Society of America; American Thoracic Society. Infectious Diseases Society of America/American Thoracic Society consensus guidelines on the management of community-acquired pneumonia in adults. Clin Infect Dis 2007; 44(suppl 2):S27–S72.
- Kolling UK, Hansen F, Braun J, Rink L, Katus HA, Dalhoff K. Leucocyte response and anti-inflammatory cytokines in community acquired pneumonia. Thorax 2001; 56:121–125.
- Wunderink RG, Waterer GW. Community-acquired pneumonia: pathophysiology and host factors with focus on possible new approaches to management of lower respiratory tract infections. Infect Dis Clin North Am 2004; 18:743–759.
- Hilleman MR. Realities and enigmas of human viral influenza: pathogenesis, epidemiology and control. Vaccine 2002; 20:3068–3087.
- Bender BS, Small PA. Influenza: pathogenesis and host defense. Semin Respir Infect 1992; 7:38–45.
- Scheiblauer H, Reinacher M, Tashiro M, Rott R. Interactions between bacteria and influenza A virus in the development of influenza pneumonia. J Infect Dis 1992; 166:783–791.
- McCullers JA. Insights into the interaction between influenza virus and pneumococcus. Clin Microbiol Rev 2006; 19:571–582.
- Metlay JP, Kapoor WN, Fine MJ. Does this patient have community-acquired pneumonia? Diagnosing pneumonia by history and physical examination. JAMA 1997; 278:1440–1445.
- Benbassat J, Baumal R. Narrative review: should teaching of the respiratory physical examination be restricted only to signs with proven reliability and validity? J Gen Intern Med 2010; 25:865–872.
- Kolsuz M, Erginel S, Alatas O, et al. Acute phase reactants and cytokine levels in unilateral community-acquired pneumonia. Respiration 2003; 70:615–622.
- Alves DW, Kennedy MT. Community-acquired pneumonia in casualty: etiology, clinical features, diagnosis, and management (or a look at the “new” in pneumonia since 2002). Curr Opin Pulm Med 2004; 10:166–170.
- Monto AS, Gravenstein S, Elliott M, Colopy M, Schweinle J. Clinical signs and symptoms predicting influenza infection. Arch Intern Med 2000; 160:3243–3247.
- Bewick T, Myles P, Greenwood S, et al; Influenza Clinical Information Network. Clinical and laboratory features distinguishing pandemic H1N1 influenza-related pneumonia from interpandemic community-acquired pneumonia in adults. Thorax 2011; 66:247–252.
- Morens DM, Fauci AS. The 1918 influenza pandemic: insights for the 21st century. J Infect Dis 2007; 195:1018–1028.
- Starr I. Influenza in 1918: recollections of the epidemic in Philadelphia. 1976. Ann Intern Med 2006; 145:138–140.
- Morens DM, Taubenberger JK, Fauci AS. Predominant role of bacterial pneumonia as a cause of death in pandemic influenza: implications for pandemic influenza preparedness. J Infect Dis 2008; 198:962–970.
- Brundage JF, Shanks GD. Deaths from bacterial pneumonia during 1918–19 influenza pandemic. Emerg Infect Dis 2008; 14:1193–1199.
- Treanor J. Influenza virus. In: Mandell GL, Bennett JE, Dolin R, eds. Principles and Practice of Infectious Diseases. 6th ed. Philadelphia, PA: Elsevier/Churchill Livingstone; 2005:2060–2085.
- Jarstrand C, Tunevall G. The influence of bacterial superinfection on the clinical course of influenza. Studies from the influenza epidemics in Stockholm during the winters 1969–70 and 1971–72. Scand J Infect Dis 1975; 7:243–247.
- Schwarzmann SW, Adler JL, Sullivan RJ, Marine WM. Bacterial pneumonia during the Hong Kong influenza epidemic of 1968–1969. Arch Intern Med 1971; 127:1037–1041.
- Hageman JC, Uyeki TM, Francis JS, et al. Severe community-acquired pneumonia due to Staphylococcus aureus, 2003–04 influenza season. Emerg Infect Dis 2006; 12:894–899.
- Centers for Disease Control and Prevention (CDC). Severe methicillin-resistant Staphylococcus aureus community-acquired pneumonia associated with influenza—Louisiana and Georgia, December 2006–January 2007. MMWR Morb Mortal Wkly Rep 2007; 56:325–329.
- Hidron AI, Low CE, Honig EG, Blumberg HM. Emergence of community-acquired methicillin-resistant Staphylococcus aureus strain USA300 as a cause of necrotising community-onset pneumonia. Lancet Infect Dis 2009; 9:384–392.
- Call SA, Vollenweider MA, Hornung CA, Simel DL, McKinney WP. Does this patient have influenza? JAMA 2005; 293:987–997.
- Harper SA, Bradley JS, Englund JA, et al; Expert Panel of the Infectious Diseases Society of America. Seasonal influenza in adults and children—diagnosis, treatment, chemoprophylaxis, and institutional outbreak management: clinical practice guidelines of the Infectious Diseases Society of America. Clin Infect Dis 2009; 48:1003–1032.
- Boersma WG, Daniels JM, Löwenberg A, Boeve WJ, van de Jagt EJ. Reliability of radiographic findings and the relation to etiologic agents in community-acquired pneumonia. Respir Med 2006; 100:926–932.
- Brixey AG, Luo Y, Skouras V, Awdankiewicz A, Light RW. The efficacy of chest radiographs in detecting parapneumonic effusions. Respirology 2011; 16:1000–1004.
- Campbell SG, Marrie TJ, Anstey R, Dickinson G, Ackroyd-Stolarz S. The contribution of blood cultures to the clinical management of adult patients admitted to the hospital with community-acquired pneumonia: a prospective observational study. Chest 2003; 123:1142–1150.
- Waterer GW, Wunderink RG. The influence of the severity of community-acquired pneumonia on the usefulness of blood cultures. Respir Med 2001; 95:78–82.
- Houck PM, Bratzler DW, Nsa W, Ma A, Bartlett JG. Timing of antibiotic administration and outcomes for Medicare patients hospitalized with community-acquired pneumonia. Arch Intern Med 2004; 164:637–644.
- Information & Quality Healthcare. http://www.IQH.org/attachments/219_CoreMHelpBookletpg4_11_3.pdf. Accessed November 14, 2011.
- Rosón B, Carratalà J, Verdaguer R, Dorca J, Manresa F, Gudiol F. Prospective study of the usefulness of sputum Gram stain in the initial approach to community-acquired pneumonia requiring hospitalization. Clin Infect Dis 2000; 31:869–874.
- García-Vázquez E, Marcos MA, Mensa J, et al. Assessment of the usefulness of sputum culture for diagnosis of community-acquired pneumonia using the PORT predictive scoring system. Arch Intern Med 2004; 164:1807–1811.
- Rosón B, Fernández-Sabé N, Carratalà J, et al. Contribution of a urinary antigen assay (Binax NOW) to the early diagnosis of pneumococcal pneumonia. Clin Infect Dis 2004; 38:222–226.
- Sordé R, Falcó V, Lowak M, et al. Current and potential usefulness of pneumococcal urinary antigen detection in hospitalized patients with community-acquired pneumonia to guide antimicrobial therapy. Arch Intern Med 2011; 171:166–172.
- Koegelenberg CFN, Diacon AH, Bolliger CT. Parapneumonic pleural effusion and empyema. Respiration 2008; 75:241–250.
- Almirall J, Bolíbar I, Toran P, et al; Community-Acquired Pneumonia Maresme Study Group. Contribution of C-reactive protein to the diagnosis and assessment of severity of community-acquired pneumonia. Chest 2004; 125:1335–1342.
- Ingram PR, Inglis T, Moxon D, Speers D. Procalcitonin and C-reactive protein in severe 2009 H1N1 influenza infection. Intensive Care Med 2010; 36:528–532.
- Fine MJ, Auble TE, Yealy DM, et al. A prediction rule to identify low-risk patients with community-acquired pneumonia. N Engl J Med 1997; 336:243–250.
- Lim WS, van der Eerden MM, Laing R, et al. Defining community acquired pneumonia severity on presentation to hospital: an international derivation and validation study. Thorax 2003; 58:377–382.
- Chalmers JD, Singanayagam A, Akram AR, et al. Severity assessment tools for predicting mortality in hospitalised patients with community-acquired pneumonia. Systematic review and meta-analysis. Thorax 2010; 65:878–883.
- Loke YK, Kwok CS, Niruban A, Myint PK. Value of severity scales in predicting mortality from community-acquired pneumonia: systematic review and meta-analysis. Thorax 2010; 65:884–890.
- Capelastegui A, España PP, Quintana JM, et al. Validation of a predictive rule for the management of community-acquired pneumonia. Eur Respir J 2006; 27:151–157.
- Charles PG, Wolfe R, Whitby M, et al; Australian Community-Acquired Pneumonia Study Collaboration. SMART-COP: a tool for predicting the need for intensive respiratory or vasopressor support in community-acquired pneumonia. Clin Infect Dis 2008; 47:375–384.
- España PP, Capelastegui A, Gorordo I, et al. Development and validation of a clinical prediction rule for severe community-acquired pneumonia. Am J Respir Crit Care Med 2006; 174:1249–1256.
- Chalmers JD, Taylor JK, Mandal P, et al. Validation of the Infectious Diseases Society of America/American Thoracic Society minor criteria for intensive care unit admission in community-acquired pneumonia patients without major criteria or contraindications to intensive care unit care. Clin Infect Dis 2011; 53:503–511.
- Majumdar SR, Eurich DT, Gamble JM, Senthilselvan A, Marrie TJ. Oxygen saturations less than 92% are associated with major adverse events in outpatients with pneumonia: a population-based cohort study. Clin Infect Dis 2011; 52:325–331.
- Nathwani D, Rubinstein E, Barlow G, Davey P. Do guidelines for community-acquired pneumonia improve the cost-effectiveness of hospital care? Clin Infect Dis 2001; 32:728–741.
- Dean NC, Silver MP, Bateman KA, James B, Hadlock CJ, Hale D. Decreased mortality after implementation of a treatment guideline for community-acquired pneumonia. Am J Med 2001; 110:451–457.
- Capelastegui A, España PP, Quintana JM, et al. Improvement of process-of-care and outcomes after implementing a guideline for the management of community-acquired pneumonia: a controlled before-and-after design study. Clin Infect Dis 2004; 39:955–963.
- Silber SH, Garrett C, Singh R, et al. Early administration of antibiotics does not shorten time to clinical stability in patients with moderate-to-severe community-acquired pneumonia. Chest 2003; 124:1798–1804.
- Welker JA, Huston M, McCue JD. Antibiotic timing and errors in diagnosing pneumonia. Arch Intern Med 2008; 168:351–356.
- Polgreen PM, Chen YY, Cavanaugh JE, et al. An outbreak of severe Clostridium difficile-associated disease possibly related to inappropriate antimicrobial therapy for community-acquired pneumonia. Infect Control Hosp Epidemiol 2007; 28:212–214.
- Waterer GW, Somes GW, Wunderink RG. Monotherapy may be suboptimal for severe bacteremic pneumococcal pneumonia. Arch Intern Med 2001; 161:1837–1842.
- Lodise TP, Kwa A, Cosler L, Gupta R, Smith RP. Comparison of beta-lactam and macrolide combination therapy versus fluoroquinolone monotherapy in hospitalized Veterans Affairs patients with community-acquired pneumonia. Antimicrob Agents Chemother 2007; 51:3977–3982.
- Waterer GW, Rello J, Wunderink RG. Management of community-acquired pneumonia in adults. Am J Respir Crit Care Med 2011; 183:157–164.
- Bjerre LM, Verheij TJ, Kochen MM. Antibiotics for community acquired pneumonia in adult outpatients. Cochrane Database Syst Rev 2009; (4):CD002109.
- Frei CR, Labreche MJ, Attridge RT. Fluoroquinolones in community-acquired pneumonia: guide to selection and appropriate use. Drugs 2011; 71:757–770.
- Weiss K, Tillotson GS. The controversy of combination vs monotherapy in the treatment of hospitalized community-acquired pneumonia. Chest 2005; 128:940–946.
- Martínez JA, Horcajada JP, Almela M, et al. Addition of a macrolide to a beta-lactam-based empirical antibiotic regimen is associated with lower in-hospital mortality for patients with bacteremic pneumococcal pneumonia. Clin Infect Dis 2003; 36:389–395.
- Waterer GW, Somes GW, Wunderink RG. Monotherapy may be suboptimal for severe bacteremic pneumococcal pneumonia. Arch Intern Med 2001; 161:1837–1842.
- Ramirez JA, Bordon J. Early switch from intravenous to oral antibiotics in hospitalized patients with bacteremic community-acquired Streptococcus pneumoniae pneumonia. Arch Intern Med 2001; 161:848–850.
- Dunbar LM, Wunderink RG, Habib MP, et al. High-dose, short-course levofloxacin for community-acquired pneumonia: a new treatment paradigm. Clin Infect Dis 2003; 37:752–760.
- el Moussaoui R, de Borgie CA, van den Broek P, et al. Effectiveness of discontinuing antibiotic treatment after three days versus eight days in mild to moderate-severe community acquired pneumonia: randomised, double blind study. BMJ 2006; 332:1355.
- Mundy LM, Leet TL, Darst K, Schnitzler MA, Dunagan WC. Early mobilization of patients hospitalized with community-acquired pneumonia. Chest 2003; 124:883–889.
- Salluh JI, Póvoa P, Soares M, Castro-Faria-Neto HC, Bozza FA, Bozza PT. The role of corticosteroids in severe community-acquired pneumonia: a systematic review. Crit Care 2008; 12:R76.
- Mikami K, Suzuki M, Kitagawa H, et al. Efficacy of corticosteroids in the treatment of community-acquired pneumonia requiring hospitalization. Lung 2007; 185:249–255.
- Snijders D, Daniels JM, de Graaff CS, van der Werf TS, Boersma WG. Efficacy of corticosteroids in community-acquired pneumonia: a randomized double-blinded clinical trial. Am J Respir Crit Care Med 2010; 181:975–982.
- Chopra V, Flanders SA. Does statin use improve pneumonia outcomes? Chest 2009; 136:1381–1388.
- Yende S, Milbrandt EB, Kellum JA, et al. Understanding the potential role of statins in pneumonia and sepsis. Crit Care Med 2011; 39:1871–1878.
- Halm EA, Fine MJ, Marrie TJ, et al. Time to clinical stability in patients hospitalized with community-acquired pneumonia: implications for practice guidelines. JAMA 1998; 279:1452–1457.
- Marrie TJ, Lau CY, Wheeler SL, Wong CJ, Feagan BG. Predictors of symptom resolution in patients with community-acquired pneumonia. Clin Infect Dis 2000; 31:1362–1367.
- Aliberti S, Peyrani P, Filardo G, et al. Association between time to clinical stability and outcomes after discharge in hospitalized patients with community-acquired pneumonia. Chest 2011; 140:482–488.
- Fiore AE, Uyeki TM, Broder K, et al; Centers for Disease Control and Prevention (CDC). Prevention and control of influenza with vaccines: recommendations of the Advisory Committee on Immunization Practices (ACIP), 2010. MMWR Recomm Rep 2010; 59:1–62.
- Nuorti JP, Butler JC, Farley MM, et al. Cigarette smoking and invasive pneumococcal disease. Active Bacterial Core Surveillance Team. N Engl J Med 2000; 342:681–689.
KEY POINTS
- Especially during flu season, clinicians should consider influenza in patients with respiratory symptoms.
- The diagnosis of CAP is based primarily on clinical factors: a combination of signs and symptoms such as cough, fever, chills, sputum production, dyspnea, pleuritic pain, tachypnea, tachycardia, hypoxemia, consolidation or rales on auscultation, and a new infiltrate on chest imaging.
- Empiric outpatient treatment of a previously healthy patient with CABP should include either a macrolide or doxycycline. A fluoroquinolone or beta-lactam plus a macrolide should be used for patients with comorbid conditions.
- Several indices have been validated for use in deciding on inpatient vs outpatient treatment and whether a patient with pneumonia should be admitted to an intensive care unit.