User login
Achievable Benchmarks of Care for Pediatric Readmissions
Hospital readmission rates are a common metric for defining, evaluating, and benchmarking quality of care. The Centers for Medicare and Medicaid Services (CMS) publicly report hospital readmission rates for common adult conditions and reduces payments to hospitals with excessive readmissions.1 Recently, the focus on pediatric readmission rates has increased and the National Quality Forum (NQF) has endorsed at least two pediatric readmission-specific quality indicators which could be used by public and private payers in pay-for-performance programs aimed at institutions caring for children.2 While preventability of readmissions and their value as a marker of quality remains debated, their acceptance by the NQF and CMS has led public and private payers to propose readmission-related penalties for hospitals caring for children. 3-5
All-cause 30-day same-hospital readmission rates for pediatric conditions are half of the adult readmission rates, around 6% in most studies, compared to 12% in adults.6,7 The lower rates of pediatric readmissions makes it difficult to only use mean readmission rates to stratify hospitals into high- or low-performers and set target goals for improvement.8 While adult readmissions have been studied in depth, there are no consistent measures used to benchmark pediatric readmissions across hospital types.
Given the emphasis placed on readmissions, it is essential to understand patterns in pediatric readmission rates to determine optimal and achievable targets for improvement. Achievable Benchmarks of Care (ABCs) are one approach to understanding readmission rates and have an advantage over using mean or medians in performance improvement as they can stratify performance for conditions with low readmission rates and low volumes.9 When creating benchmarks, it is important that hospitals performance is evaluated among peer hospitals with similar patient populations, not just a cumulative average from all hospital types which may punish hospitals with a more complex patient case mix.10 The goal of this study was to calculate the readmission rates and the ABCs for common pediatric diagnoses by hospital type to identify priority conditions for quality improvement efforts using a previously published methodology.11-13
METHODS
Data Source
We conducted a retrospective analysis of patients less than 18 years of age in the Healthcare Utilization Project 2014 Nationwide Readmissions Database (NRD). The NRD includes public hospitals; academic medical centers; and specialty hospitals in obstetrics and gynecology, otolaryngology, orthopedics, and cancer; and pediatric, public, and academic medical hospitals. Excluded are long-term care facilities such as rehabilitation, long-term acute care, psychiatric, alcoholism, and chemical dependency hospitals. The readmissions data contains information from hospitals grouped by region, population census, and teaching status.14 Three hospital type classifications used in this study were metropolitan teaching hospitals, metropolitan nonteaching hospitals, and nonmetropolitan hospitals. These three hospital type classifications follow the reporting format in the NRD.
Study Population
Patients less than 18 years old were included if they were discharged from January 1, 2014 through November 30, 2014 and had a readmission to the index hospital within 30 days. We limited inclusion to discharges through November 30 so we could identify patients with a 30-day readmission as patient identifiers do not link across years in the NRD.
Exposure
We included 30-day, all-cause, same-hospital readmissions to the index acute care hospital, excluding labor and delivery, normal newborn care, chemotherapy, transfers, and mortalities. Intrahospital discharge and admissions within the same hospital system were not defined as a readmission, but rather as a “same-day event.”15 For example, institutions with inpatient mental health facilities, medical unit discharges and admission to the mental health unit were not identified as a readmission in this dataset.
Outcome
For each hospital type, we measured same-hospital, all-cause, 30-day readmission rates and achievable benchmark of care for the 17 most commonly readmitted pediatric discharge diagnoses. To identify the target readmission diagnoses and all-cause, 30-day readmissions based on their index hospitalizations, All-Patient Refined Diagnosis-Related Groups (APR-DRG), version 25 (3M Health Information Systems, Salt Lake City, Utah) were ordered by frequency for each hospital type. The 20 most common APR-DRGs were the same across all hospital types. The authors then evaluated these 20 APR-DRGs for clinical consistency of included diagnoses identified by the International Classification of Diseases, Ninth Revision, Clinical Modification (ICD-9-CM) codes within each APR-DRG. Three diagnosis-related groups were excluded from the analysis (major hematologic/immunologic disease except for sickle cell, other anemia and disorders of blood and blood forming organs, and other digestive system diagnoses) due to the heterogeneity of the diagnoses identified by the ICD-9-CM codes within each APR-DRG. We refer to each APR-DRG as a “diagnosis” throughout the article.
Analysis
The demographic characteristics of the patients seen at the three hospital types were summarized using frequencies and percentages. Reports were generated for patient age, gender, payer source, patient residence, median household income, patient complexity, and discharge disposition. Patient complexity was defined using complex chronic condition (CCC) and the number of chronic conditions (CCI).16,17 As previously defined in the literature, a complex chronic condition is “any medical condition that can be reasonably expected to last at least 12 months (unless death intervenes) and to involve either several different organ systems or one organ system severely enough to require specialty pediatric care and probably some period of hospitalization in a tertiary care center.”16 Whereas, the Agency for Healthcare Research and Quality’s Chronic Condition Indicator (CCI) defines single, non-CCCs (eg, allergic rhinitis).17
For each diagnosis, we calculated the mean readmission rate for hospitals in each hospital type category. We then calculated an ABC for each diagnosis in each hospital type using a four-step process.13,18 First, to control for hospitals with small sample sizes, we adjusted all readmission rates using an adjusted performance fraction ([numerator+1]/[denominator +2]), where the numerator is the number of all-cause 30-day readmissions and the denominator is the number of discharges for the selected diagnosis. Then the hospitals were ordered from lowest (best performing) to highest (worst performing) using the adjusted readmission rate. Third, the number of discharges from the best performing hospital to the worst performing hospital was summed until at least 10% of the total discharges had been accounted for. Finally, we computed the ABC as the average of these best performing hospitals. We only report ABCs for which at least three hospitals were included as best performers in the calculation.13
To evaluate hospital performance on ABCs for each diagnosis, we identified the percent of hospitals in each setting that were outliers. We defined an outlier as any hospital whose 95% confidence interval for their readmission rate for a given diagnosis did not contain the ABC for their hospital type. All the statistical analyses were performed using SAS version 9.3 (SAS Institute, Inc, Cary, North Carolina).
This project was reviewed by the Cincinnati Children’s Hospital Medical Center Institutional Review Board and determined to be nonhuman subjects research.
RESULTS
Hospital-Type Demographics
The 690,949 discharges from 1,664 hospitals were categorized into 525 metropolitan teaching (550,039 discharges, 79.6% of discharges), 552 metropolitan nonteaching (97,207 discharges, 14% of discharges), and 587 nonmetropolitan hospitals (43,703 discharges, 6.3% of discharges; Table 1). There were significant differences in the patient composition among the three hospital settings. Nonmetropolitan hospitals had a larger percentage of younger patients (aged 0-4 years, P < .001), prominence of first and second quartile median household income, and fewer medically complex patients (48.3% No CCC/No CCI versus 25.5% metropolitan teaching and 33.7% nonteaching, P < .001). Disposition home was over 96% in all three hospital types; however, the metropolitan teaching had a greater percentage of patients discharged to home health versus metropolitan nonteaching and nonmetropolitan hospitals (2.3% versus 0.5%; P < .001).
Readmission Rates
The 17 most common diagnoses based on the number of all-cause 30-day same-hospital readmissions, were categorized into two surgical, seven acute/infectious, four chronic, and four mental health diagnoses (Table 2). Readmission rates varied based on diagnosis and hospital type (Table 2). Overall, mean readmission rates were low, especially in acute respiratory tract related diseases. For chronic diseases, asthma readmissions were consistently low in all three hospital types, whereas sickle cell disease had the highest readmission rate in all three hospital types.
Achievable Benchmarks of Care by Hospital Type
The diagnoses for which ABC could be calculated across all three hospital types included appendectomy and four acute conditions (bronchiolitis, pneumonia, nonbacterial gastroenteritis, and kidney/urinary tract infections). For these conditions, metropolitan teaching hospitals had a more significant percentage of outlier hospitals compared to metropolitan nonteaching and nonmetropolitan hospitals. The percent of outlier hospitals varied by diagnosis and hospital type (Figure).
Metropolitan Teaching
The readmission ABC was calculated for all 17 diagnoses (Table 2). The ABC ranged from 0.4% in acute kidney and urinary tract infection to 7.0% in sickle cell anemia crisis. Bipolar disorder, major depressive disorders and other psychoses, and sickle cell disease (SCD) had the highest percent of outlier hospitals whose mean readmission rates confidence interval did not contain the ABC; tonsil and adenoid procedures and viral illness had the lowest.1
Metropolitan Nonteaching
The ABC was calculated for 13 of the 17 diagnoses because ABCs were not calculated when there were fewer than three best practicing hospitals. This was the case for tonsil and adenoid procedures, diabetes, seizures, and depression except for major depressive disorder (Table 2). Seven of the 13 diagnoses had an ABC of 0.0%: viral illness, infections of the upper respiratory tract, bronchiolitis, gastroenteritis, hypovolemia and electrolyte disorders, asthma, and childhood behavioral disorders. Like the findings at the metropolitan teaching hospitals, ABCs were lowest for surgical and acute conditions while bipolar disorder, major depressive disorders and other psychoses, and SCD had the highest percent of outlier hospitals with readmission rates beyond the 95% confidence interval of their hospital type’s ABC.
Nonmetropolitan
There was a sufficient number of best practicing hospitals to calculate the ABC for six of the 17 diagnoses (Table 2). For conditions where readmission ABCs could be calculated, they were low: 0.0% for appendectomy, bronchiolitis, gastroenteritis, and seizure; 0.3% for pneumonia; and 1.3% in kidney and urinary tract disorders. None of the conditions with the highest ABCs in other hospital settings (bipolar disease, sickle cell anemia crisis, and major depressive disorders and other psychoses) could be calculated in this setting. Seizure-related readmissions exhibited the most outlier hospitals yet were less than 5%.1
DISCUSSION
Among a nationally representative sample of different hospital types that deliver care to children, we report the mean readmission rates and ABCs for 30-day all-cause, same-hospital readmissions for the most commonly readmitted pediatric diagnoses based on hospital type. Previous studies have shown patient variables such as race, ethnicity, and insurance type influencing readmission rates.19,20 However, hospital type has also been associated with a higher risk of readmission due to the varying complexity of patients at different hospital types.21,22 Our analyses provide hospital-type specific national estimates of pediatric readmission ABCs for medical and surgical conditions, many less than 1%. While commonly encountered pediatric conditions like asthma and bronchiolitis had low mean readmission rates and ABCs across all hospital types, the mean rates and ABCs for SCD and mental health disorders were much higher with more hospitals performing far from the ABCs.
Diagnoses with a larger percentage of outlier hospitals may represent a national opportunity to improve care for children. Conditions such as SCD and mental illnesses have the highest percentage of hospitals whose readmission rates fall outside of the ABCs in both metropolitan teaching and metropolitan nonteaching hospitals. Hospital performance on SCD and mental health disorders may not reflect deficits in hospital quality or poor adherence to evidence-based best practices, but rather the complex interplay of factors on various levels from government policy and insurance plans, to patient and family resources, to access and availability of medical and mental health specific care. Most importantly, these diseases may represent a significant opportunity for quality improvementin hospitals across the United States.
Sickle cell disease is predominantly a disease among African-Americans, a demographic risk factor for decreased access to care and limited patient and family resources.23-26 In previous studies evaluating the disparity in readmission rates for Black children with asthma, socioeconomic variables explained 53% of the observed disparity and readmission rates were inversely related to the childhood opportunity index of the patient’s census tract and positively related with geographic social risk.27,28 Likewise, with SCD affecting a specific demographic and being a chronic disease, best practice policies need to account for the child’s medical needs and include the patient and family resources to ensure access to care and enhanced case management for chronic disease if we aim to improve performance among the outlier hospitals.
Similarly, barriers to care for children with mental illnesses in the United States need attention.29,30 While there is a paucity of data on the prevalence of mental health disorders in children, one national report estimates that one in 10 American adolescents have depression.29,31 The American Academy of Pediatrics has developed a policy statement on mental health competencies and a mental health tool-kit for primary care pediatricians; however, no such guidelines or policy statements exist for hospitalized patients with acute or chronic psychiatric conditions.32,33 Moreover, hospitals are increasingly facing “boarding” of children with acute psychiatric illness in inpatient units and emergency departments.34 The American Medical Association and the American College of Emergency Physicians have expressed concerns regarding the boarding of children with acute psychiatric illness because nonpsychiatric hospitals do not have adequate resources to evaluate, manage, and place these children who deserve appropriate facilities for further management. Coordinated case management and “bundled” discharge planning in other chronic illnesses have shown benefit in cost reduction and readmission.35-37 Evidence-based practices around pediatric readmissions in other diagnoses should be explored as possible interventions in these conditions.38
There are several limitations to this study. Our data is limited to one calendar year; therefore, admissions in January do not account for potential readmissions from December of the previous year, as patient identifiers do not link across years in the NRD. We also limited our evaluation to the conventional 30-day readmission window, but recent publications may indicate that readmission windows with different timelines could be a more accurate reflection of medically preventable readmissions versus a reflection of social determinants of health leading to readmissions.24 Newborn index admissions were not an allowable index admission; therefore, we may be underreporting readmissions in the neonatal age group. We also chose to include all-cause readmissions, a conventional method to evaluate readmission within an institution, but which may not reflect the quality of care delivered in the index admission. For example, an asthmatic discharged after an acute exacerbation readmitted for dehydration secondary to gastroenteritis may not reflect a lack of quality in asthma inpatient care. Readmissions were limited to the same hospital; therefore, this study cannot account for readmissions at other institutions, which may cause us to underestimate readmission rates. However, end-users of our findings most likely have access only to their own institution’s data. The inclusion of observation status admissions in the database varies from state to state; therefore, this percent of admissions in the database is unknown.
The use of the ABC methodology has some inherent limitations. One hospital with a significant volume diagnosis and low readmission rate within a hospital type may prohibit the reporting of an ABC if less than three hospitals composed the total of the ‘best performing’ hospitals. This was a significant limitation leading to the exclusion of many ABCs in nonmetropolitan institutions. The limitation of calculating and reporting an ABC then prohibits the calculation of outlier hospitals within a hospital type for a given diagnosis. However, when the ABCs are not available, we do provide the mean readmission rate for the diagnosis within the hospital type. While the hospital groupings by population and teaching status for ABCs provide meaningful comparisons for within each hospital setting, it should be noted that there may be vast differences among hospitals within each type (eg, tertiary children’s hospitals compared to teaching hospitals with a pediatric floor in the metropolitan teaching hospital category).39,40
As healthcare moves from a fee-for-service model to a population-health centered, value-based model, reduction in readmission rates will be more than a quality measure and will have potential financial implications.41 In the Medicare fee-for-service patients, the Hospital Readmission Reduction Program (HRRP) penalize hospitals with excess readmissions for acute myocardial infarction, heart failure, and pneumonia. The hospitals subject to penalties in the HRRP had greater reduction in readmission rates in the targeted, and even nontargeted conditions, compared with hospitals not subject to penalties.42 Similarly, we believe that our data on low readmission rates and ABCs for conditions such as asthma, bronchiolitis, and appendicitis could represent decades of quality improvement work for the most common pediatric conditions among hospitalized children. Sickle cell disease and mental health problems remain as outliers and merit further attention. To move to a true population-health model, hospitals will need to explore outlier conditions including evaluating patient-level readmission patterns across institutions. This moves readmission from a hospital quality measure to a patient-centric quality measure, and perhaps will provide value to the patient and the healthcare system alike.
CONCLUSIONS
The readmission ABCs for the most commonly readmitted pediatric diagnoses are low, regardless of the hospital setting. The highest pediatric readmission rates in SCD, bipolar disorders, and major depressive disorder were lower than the most common adult readmission diagnoses. However, mental health conditions and SCD remain as outliers for pediatric readmissions, burden hospital systems, and perhaps warrant national-level attention. The ABCs stratified by hospital type in this study facilitate comparisons and identify opportunities for population-level interventions to meaningfully improve patient care.
Disclosures
The authors have nothing to disclose.
1. Medicare. 30-day death and readmission measures data. https://www.medicare.gov/hospitalcompare/Data/30-day-measures.html. Accessed October 24, 2017.
2. National Quality Forum. Performance Measures; 2016 https://www.quality fourm.org/Measuring_Performance/Endorsed_Performance_Measures_Maintenance.aspx. Accessed October 24, 2017.
3. Auger KA, Simon TD, Cooperberg D, et al. Summary of STARNet: seamless transitions and (re)admissions network. Pediatrics. 2015;135(1):164-175. https://doi.org/10.1542/peds.2014-1887.
4. Toomey SL, Peltz A, Loren S, et al. Potentially preventable 30-day hospital readmissions at a children’s hospital. Pediatrics. 2016;138(2):e20154182-e20154182. https://doi.org/10.1542/peds.2015-4182.
5. Halfon P, Eggli Y, Prêtre-Rohrbach I, et al. Validation of the potentially avoidable hospital readmission rate as a routine indicator of the quality of hospital care. Med Care. 2006;44(11):972-981. https://doi.org/10.1097/01.mlr.0000228002.43688.c2.
6. Gay JC, Agrawal R, Auger KA, et al. Rates and impact of potentially preventable readmissions at children’s hospitals. J Pediatr. 2015;166(3):613-619. https://doi.org/10.1016/j.jpeds.2014.10.052.
7. Berry JG, Gay JC, Joynt Maddox KJ, et al. Age trends in 30 day hospital readmissions: US national retrospective analysis. BMJ. 2018;360:k497. https://doi.org/10.1136/bmj.k497.
8. Bardach NS, Vittinghoff E, Asteria-Penaloza R, et al. Measuring hospital quality using pediatric readmission and revisit rates. Pediatrics. 2013;132(3):429-436. https://doi.org/10.1542/peds.2012-3527d.
9. Berry JG, Toomey SL, Zaslavsky AM, et al. Pediatric readmission prevalence and variability across hospitals. JAMA. 2013;309(4):372-380. https://doi.org/10.1001/jama.2012.188351.
10. Gohil SK, Datta R, Cao C, et al. Impact of hospital population case-mix, including poverty, on hospital all-cause and infection-related 30-day readmission rates. Clin Infect Dis. 2015;61(8):1235-1243. https://doi.org/10.1093/cid/civ539.
11. Parikh K, Hall M, Mittal V, et al. Establishing benchmarks for the hospitalized care of children with asthma, bronchiolitis, and pneumonia. Pediatrics. 2014;134(3):555-562. https://doi.org/10.1542/peds.2014-1052.
12. Reyes M, Paulus E, Hronek C, et al. Choosing wisely campaign: report card and achievable benchmarks of care for children’s hospitals. Hosp Pediatr. 2017;7(11):633-641. https://doi.org/10.1542/hpeds.2017-0029.
13. Kiefe CI, Weissman NW, Allison JJ, et al. Identifying achievable benchmarks of care: concepts and methodology. Int J Qual Health Care. 1998;10(5):443-447. https://doi.org/10.1093/intqhc/10.5.443.
14. Agency for Healthcare Research and Quality. Nationwide Readmissions Database Availability of Data Elements. . https://www.hcup-us.ahrq.gov/partner/MOARef/HCUPdata_elements.pdf. Accessed 2018 Jun 6
15. Healthcare Cost and Utilization Project. HCUP NRD description of data elements. Agency Healthc Res Qual. https://www.hcup-us.ahrq.gov/db/vars/samedayevent/nrdnote.jsp. Accessed 2018 Jun 6; 2015.
16. Feudtner C, Feinstein JA, Zhong W, Hall M, Dai D. Pediatric complex chronic conditions classification system version 2: updated for ICD-10 and complex medical technology dependence and transplantation. BMC Pediatr. 2014;14:199. https://doi.org/10.1186/1471-2431-14-199.
17. Agency for Healthcare Research and Quality. HCUP chronic condition indicator. Healthc Cost Util Proj. https://www.hcup-us.ahrq.gov/toolssoftware/chronic/chronic.jsp. Accessed 2016 Apr 26; 2009.
18. Weissman NW, Allison JJ, Kiefe CI, et al. Achievable benchmarks of care: the ABCs of benchmarking. J Eval Clin Pract. 1999;5(3):269-281. https://doi.org/10.1046/j.1365-2753.1999.00203.x.
19. Joynt KE, Orav EJ, Jha AK. Thirty-day readmission rates for medicare beneficiaries by race and site of care. JAMA. 2011;305(7):675-681. https://doi.org/10.1001/jama.2011.123.
20. Kenyon CC, Melvin PR, Chiang VW, et al. Rehospitalization for childhood asthma: timing, variation, and opportunities for intervention. J Pediatr. 2014;164(2):300-305. https://doi.org/10.1016/j.jpeds.2013.10.003.
21. Sobota A, Graham DA, Neufeld EJ, Heeney MM. Thirty-day readmission rates following hospitalization for pediatric sickle cell crisis at freestanding children’s hospitals: risk factors and hospital variation. Pediatr Blood Cancer. 2012;58(1):61-65. https://doi.org/10.1002/pbc.23221.
22. Berry JG, Hall DE, Kuo DZ, et al. Hospital utilization and characteristics of patients experiencing recurrent readmissions within children’s hospitals. JAMA. 2011;305(7):682-690. https://doi.org/10.1001/jama.2011.122.
23. Ginde AA, Espinola JA, Camargo CA. Improved overall trends but persistent racial disparities in emergency department visits for acute asthma, 1993-2005. J Allergy Clin Immunol. 2008;122(2):313-318. https://doi.org/10.1016/j.jaci.2008.04.024.
24. Parikh K, Berry J, Hall M, et al. Racial and ethnic differences in pediatric readmissions for common chronic conditions. J Pediatr. 2017;186. https://doi.org/10.1016/j.jpeds.2017.03.046.
25. Chen BK, Hibbert J, Cheng X, Bennett K. Travel distance and sociodemographic correlates of potentially avoidable emergency department visits in California, 2006-2010: an observational study. Int J Equity Health. 2015;14(1):30. https://doi.org/10.1186/s12939-015-0158-y.
26. Ray KN, Chari AV, Engberg J, et al. Disparities in time spent seeking medical care in the United States. JAMA Intern Med. 2015;175(12):175(12):1983-1986. https://doi.org/10.1001/jamainternmed.2015.4468.
27. Beck AF, Huang B, Wheeler K, et al. The child opportunity index and disparities in pediatric asthma hospitalizations across one Ohio metropolitan area. J Pediatr. 2011-2013;190:200-206. https://doi.org/10.1016/j.jpeds.2017.08.007.
28. Beck AF, Simmons JM, Huang B, Kahn RS. Geomedicine: area-based socioeconomic measures for assessing the risk of hospital reutilization among children admitted for asthma. Am J Public Health. 2012;102(12):2308-2314. https://doi.org/10.2105/AJPH.2012.300806.
29. Avenevoli S, Swendsen J, He JP, Burstein M, Merikangas KR. Major depression in the national comorbidity survey-adolescent supplement: prevalence, correlates, and treatment. J Am Acad Child Adolesc Psychiatry. 2015;54(1):37-44.e2. https://doi.org/10.1016/j.jaac.2014.10.010.
30. Feng JY, Toomey SL, Zaslavsky AM, Nakamura MM, Schuster MA. Readmission after pediatric mental health admissions. Pediatrics. 2017;140(6):e20171571. https://doi.org/10.1542/peds.2017-1571.
31. Merikangas KR, He JP, Burstein M, et al. Lifetime prevalence of mental disorders in U.S. adolescents: results from the National comorbidity Survey Replication-Adolescent Supplement (NCS-A). J Am Acad Child Adolesc Psychiatry. 2010;49(10):980-989. https://doi.org/10.1016/j.jaac.2010.05.017.
32. Cheung AH, Zuckerbrot RA, Jensen PS, et al. Guidelines for adolescent depression in primary care (GLAD-PC): Part II. Treatment and ongoing management. Pediatrics. 2018;141(3):e20174082. https://doi.org/10.1542/peds.2017-4082.
33. Zuckerbrot RA, Cheung A, Jensen PS, et al. Guidelines for adolescent depression in primary care (GLAD-PC): Part I. Practice preparation, identification, assessment, and initial management. Pediatrics. 2018;141(3):e20174081. https://doi.org/10.1542/peds.2017-4081.
34. Dolan MA, Fein JA, Committee on Pediatric Emergency Medicine. Pediatric and adolescent mental health emergencies in the emergency Medical Services system. Pediatrics. 2011;127(5):e1356-e1366. https://doi.org/10.1542/peds.2011-0522.
35. Collaborative Healthcare Strategies. Hospital Guide to Reducing Medicaid Readmissions. Rockville, MD: 2014. https://www.ahrq.gov/sites/default/files/publications/files/medreadmissions.pdf. Accessed 2017 Oct 11.
36. Hilbert K, Payne R, Wooton S. Children’s Hospitals’ Solutions for Patient Safety. Readmissions Bundle Tools. Cincinnati, OH; 2014.
37. Nuckols TK, Keeler E, Morton S, et al. Economic evaluation of quality improvement interventions designed to prevent hospital readmission: a systematic review and meta-analysis. JAMA Intern Med. 2017;177(7):975-985. https://doi.org/10.1001/jamainternmed.2017.1136.
38. Berry JG, Blaine K, Rogers J, et al. A framework of pediatric hospital discharge care informed by legislation, research, and practice. JAMA Pediatr. 2014;168(10):955-962. https://doi.org/10.1001/jamapediatrics.2014.891.
39. Chen HF, Carlson E, Popoola T, Suzuki S. The impact of rurality on 30-day preventable readmission, illness severity, and risk of mortality for heart failure Medicare home health beneficiaries. J Rural Health. 2016;32(2):176-187. https://doi.org/10.1111/jrh.12142.
40. Khan A, Nakamura MM, Zaslavsky AM, et al. Same-hospital readmission rates as a measure of pediatric quality of care. JAMA Pediatr. 2015;169(10):905-912. https://doi.org/10.1001/jamapediatrics.2015.1129.
41. Share DA, Campbell DA, Birkmeyer N, et al. How a regional collaborative of hospitals and physicians in Michigan cut costs and improved the quality of care. Health Aff. 2011;30(4):636-645. https://doi.org/10.1377/hlthaff.2010.0526.
42. Desai NR, Ross JS, Kwon JY, et al. Association between hospital penalty status under the hospital readmission reduction program and readmission rates for target and nontarget conditions. JAMA. 2016;316(24):2647-2656. https://doi.org/10.1001/jama.2016.18533.
Hospital readmission rates are a common metric for defining, evaluating, and benchmarking quality of care. The Centers for Medicare and Medicaid Services (CMS) publicly report hospital readmission rates for common adult conditions and reduces payments to hospitals with excessive readmissions.1 Recently, the focus on pediatric readmission rates has increased and the National Quality Forum (NQF) has endorsed at least two pediatric readmission-specific quality indicators which could be used by public and private payers in pay-for-performance programs aimed at institutions caring for children.2 While preventability of readmissions and their value as a marker of quality remains debated, their acceptance by the NQF and CMS has led public and private payers to propose readmission-related penalties for hospitals caring for children. 3-5
All-cause 30-day same-hospital readmission rates for pediatric conditions are half of the adult readmission rates, around 6% in most studies, compared to 12% in adults.6,7 The lower rates of pediatric readmissions makes it difficult to only use mean readmission rates to stratify hospitals into high- or low-performers and set target goals for improvement.8 While adult readmissions have been studied in depth, there are no consistent measures used to benchmark pediatric readmissions across hospital types.
Given the emphasis placed on readmissions, it is essential to understand patterns in pediatric readmission rates to determine optimal and achievable targets for improvement. Achievable Benchmarks of Care (ABCs) are one approach to understanding readmission rates and have an advantage over using mean or medians in performance improvement as they can stratify performance for conditions with low readmission rates and low volumes.9 When creating benchmarks, it is important that hospitals performance is evaluated among peer hospitals with similar patient populations, not just a cumulative average from all hospital types which may punish hospitals with a more complex patient case mix.10 The goal of this study was to calculate the readmission rates and the ABCs for common pediatric diagnoses by hospital type to identify priority conditions for quality improvement efforts using a previously published methodology.11-13
METHODS
Data Source
We conducted a retrospective analysis of patients less than 18 years of age in the Healthcare Utilization Project 2014 Nationwide Readmissions Database (NRD). The NRD includes public hospitals; academic medical centers; and specialty hospitals in obstetrics and gynecology, otolaryngology, orthopedics, and cancer; and pediatric, public, and academic medical hospitals. Excluded are long-term care facilities such as rehabilitation, long-term acute care, psychiatric, alcoholism, and chemical dependency hospitals. The readmissions data contains information from hospitals grouped by region, population census, and teaching status.14 Three hospital type classifications used in this study were metropolitan teaching hospitals, metropolitan nonteaching hospitals, and nonmetropolitan hospitals. These three hospital type classifications follow the reporting format in the NRD.
Study Population
Patients less than 18 years old were included if they were discharged from January 1, 2014 through November 30, 2014 and had a readmission to the index hospital within 30 days. We limited inclusion to discharges through November 30 so we could identify patients with a 30-day readmission as patient identifiers do not link across years in the NRD.
Exposure
We included 30-day, all-cause, same-hospital readmissions to the index acute care hospital, excluding labor and delivery, normal newborn care, chemotherapy, transfers, and mortalities. Intrahospital discharge and admissions within the same hospital system were not defined as a readmission, but rather as a “same-day event.”15 For example, institutions with inpatient mental health facilities, medical unit discharges and admission to the mental health unit were not identified as a readmission in this dataset.
Outcome
For each hospital type, we measured same-hospital, all-cause, 30-day readmission rates and achievable benchmark of care for the 17 most commonly readmitted pediatric discharge diagnoses. To identify the target readmission diagnoses and all-cause, 30-day readmissions based on their index hospitalizations, All-Patient Refined Diagnosis-Related Groups (APR-DRG), version 25 (3M Health Information Systems, Salt Lake City, Utah) were ordered by frequency for each hospital type. The 20 most common APR-DRGs were the same across all hospital types. The authors then evaluated these 20 APR-DRGs for clinical consistency of included diagnoses identified by the International Classification of Diseases, Ninth Revision, Clinical Modification (ICD-9-CM) codes within each APR-DRG. Three diagnosis-related groups were excluded from the analysis (major hematologic/immunologic disease except for sickle cell, other anemia and disorders of blood and blood forming organs, and other digestive system diagnoses) due to the heterogeneity of the diagnoses identified by the ICD-9-CM codes within each APR-DRG. We refer to each APR-DRG as a “diagnosis” throughout the article.
Analysis
The demographic characteristics of the patients seen at the three hospital types were summarized using frequencies and percentages. Reports were generated for patient age, gender, payer source, patient residence, median household income, patient complexity, and discharge disposition. Patient complexity was defined using complex chronic condition (CCC) and the number of chronic conditions (CCI).16,17 As previously defined in the literature, a complex chronic condition is “any medical condition that can be reasonably expected to last at least 12 months (unless death intervenes) and to involve either several different organ systems or one organ system severely enough to require specialty pediatric care and probably some period of hospitalization in a tertiary care center.”16 Whereas, the Agency for Healthcare Research and Quality’s Chronic Condition Indicator (CCI) defines single, non-CCCs (eg, allergic rhinitis).17
For each diagnosis, we calculated the mean readmission rate for hospitals in each hospital type category. We then calculated an ABC for each diagnosis in each hospital type using a four-step process.13,18 First, to control for hospitals with small sample sizes, we adjusted all readmission rates using an adjusted performance fraction ([numerator+1]/[denominator +2]), where the numerator is the number of all-cause 30-day readmissions and the denominator is the number of discharges for the selected diagnosis. Then the hospitals were ordered from lowest (best performing) to highest (worst performing) using the adjusted readmission rate. Third, the number of discharges from the best performing hospital to the worst performing hospital was summed until at least 10% of the total discharges had been accounted for. Finally, we computed the ABC as the average of these best performing hospitals. We only report ABCs for which at least three hospitals were included as best performers in the calculation.13
To evaluate hospital performance on ABCs for each diagnosis, we identified the percent of hospitals in each setting that were outliers. We defined an outlier as any hospital whose 95% confidence interval for their readmission rate for a given diagnosis did not contain the ABC for their hospital type. All the statistical analyses were performed using SAS version 9.3 (SAS Institute, Inc, Cary, North Carolina).
This project was reviewed by the Cincinnati Children’s Hospital Medical Center Institutional Review Board and determined to be nonhuman subjects research.
RESULTS
Hospital-Type Demographics
The 690,949 discharges from 1,664 hospitals were categorized into 525 metropolitan teaching (550,039 discharges, 79.6% of discharges), 552 metropolitan nonteaching (97,207 discharges, 14% of discharges), and 587 nonmetropolitan hospitals (43,703 discharges, 6.3% of discharges; Table 1). There were significant differences in the patient composition among the three hospital settings. Nonmetropolitan hospitals had a larger percentage of younger patients (aged 0-4 years, P < .001), prominence of first and second quartile median household income, and fewer medically complex patients (48.3% No CCC/No CCI versus 25.5% metropolitan teaching and 33.7% nonteaching, P < .001). Disposition home was over 96% in all three hospital types; however, the metropolitan teaching had a greater percentage of patients discharged to home health versus metropolitan nonteaching and nonmetropolitan hospitals (2.3% versus 0.5%; P < .001).
Readmission Rates
The 17 most common diagnoses based on the number of all-cause 30-day same-hospital readmissions, were categorized into two surgical, seven acute/infectious, four chronic, and four mental health diagnoses (Table 2). Readmission rates varied based on diagnosis and hospital type (Table 2). Overall, mean readmission rates were low, especially in acute respiratory tract related diseases. For chronic diseases, asthma readmissions were consistently low in all three hospital types, whereas sickle cell disease had the highest readmission rate in all three hospital types.
Achievable Benchmarks of Care by Hospital Type
The diagnoses for which ABC could be calculated across all three hospital types included appendectomy and four acute conditions (bronchiolitis, pneumonia, nonbacterial gastroenteritis, and kidney/urinary tract infections). For these conditions, metropolitan teaching hospitals had a more significant percentage of outlier hospitals compared to metropolitan nonteaching and nonmetropolitan hospitals. The percent of outlier hospitals varied by diagnosis and hospital type (Figure).
Metropolitan Teaching
The readmission ABC was calculated for all 17 diagnoses (Table 2). The ABC ranged from 0.4% in acute kidney and urinary tract infection to 7.0% in sickle cell anemia crisis. Bipolar disorder, major depressive disorders and other psychoses, and sickle cell disease (SCD) had the highest percent of outlier hospitals whose mean readmission rates confidence interval did not contain the ABC; tonsil and adenoid procedures and viral illness had the lowest.1
Metropolitan Nonteaching
The ABC was calculated for 13 of the 17 diagnoses because ABCs were not calculated when there were fewer than three best practicing hospitals. This was the case for tonsil and adenoid procedures, diabetes, seizures, and depression except for major depressive disorder (Table 2). Seven of the 13 diagnoses had an ABC of 0.0%: viral illness, infections of the upper respiratory tract, bronchiolitis, gastroenteritis, hypovolemia and electrolyte disorders, asthma, and childhood behavioral disorders. Like the findings at the metropolitan teaching hospitals, ABCs were lowest for surgical and acute conditions while bipolar disorder, major depressive disorders and other psychoses, and SCD had the highest percent of outlier hospitals with readmission rates beyond the 95% confidence interval of their hospital type’s ABC.
Nonmetropolitan
There was a sufficient number of best practicing hospitals to calculate the ABC for six of the 17 diagnoses (Table 2). For conditions where readmission ABCs could be calculated, they were low: 0.0% for appendectomy, bronchiolitis, gastroenteritis, and seizure; 0.3% for pneumonia; and 1.3% in kidney and urinary tract disorders. None of the conditions with the highest ABCs in other hospital settings (bipolar disease, sickle cell anemia crisis, and major depressive disorders and other psychoses) could be calculated in this setting. Seizure-related readmissions exhibited the most outlier hospitals yet were less than 5%.1
DISCUSSION
Among a nationally representative sample of different hospital types that deliver care to children, we report the mean readmission rates and ABCs for 30-day all-cause, same-hospital readmissions for the most commonly readmitted pediatric diagnoses based on hospital type. Previous studies have shown patient variables such as race, ethnicity, and insurance type influencing readmission rates.19,20 However, hospital type has also been associated with a higher risk of readmission due to the varying complexity of patients at different hospital types.21,22 Our analyses provide hospital-type specific national estimates of pediatric readmission ABCs for medical and surgical conditions, many less than 1%. While commonly encountered pediatric conditions like asthma and bronchiolitis had low mean readmission rates and ABCs across all hospital types, the mean rates and ABCs for SCD and mental health disorders were much higher with more hospitals performing far from the ABCs.
Diagnoses with a larger percentage of outlier hospitals may represent a national opportunity to improve care for children. Conditions such as SCD and mental illnesses have the highest percentage of hospitals whose readmission rates fall outside of the ABCs in both metropolitan teaching and metropolitan nonteaching hospitals. Hospital performance on SCD and mental health disorders may not reflect deficits in hospital quality or poor adherence to evidence-based best practices, but rather the complex interplay of factors on various levels from government policy and insurance plans, to patient and family resources, to access and availability of medical and mental health specific care. Most importantly, these diseases may represent a significant opportunity for quality improvementin hospitals across the United States.
Sickle cell disease is predominantly a disease among African-Americans, a demographic risk factor for decreased access to care and limited patient and family resources.23-26 In previous studies evaluating the disparity in readmission rates for Black children with asthma, socioeconomic variables explained 53% of the observed disparity and readmission rates were inversely related to the childhood opportunity index of the patient’s census tract and positively related with geographic social risk.27,28 Likewise, with SCD affecting a specific demographic and being a chronic disease, best practice policies need to account for the child’s medical needs and include the patient and family resources to ensure access to care and enhanced case management for chronic disease if we aim to improve performance among the outlier hospitals.
Similarly, barriers to care for children with mental illnesses in the United States need attention.29,30 While there is a paucity of data on the prevalence of mental health disorders in children, one national report estimates that one in 10 American adolescents have depression.29,31 The American Academy of Pediatrics has developed a policy statement on mental health competencies and a mental health tool-kit for primary care pediatricians; however, no such guidelines or policy statements exist for hospitalized patients with acute or chronic psychiatric conditions.32,33 Moreover, hospitals are increasingly facing “boarding” of children with acute psychiatric illness in inpatient units and emergency departments.34 The American Medical Association and the American College of Emergency Physicians have expressed concerns regarding the boarding of children with acute psychiatric illness because nonpsychiatric hospitals do not have adequate resources to evaluate, manage, and place these children who deserve appropriate facilities for further management. Coordinated case management and “bundled” discharge planning in other chronic illnesses have shown benefit in cost reduction and readmission.35-37 Evidence-based practices around pediatric readmissions in other diagnoses should be explored as possible interventions in these conditions.38
There are several limitations to this study. Our data is limited to one calendar year; therefore, admissions in January do not account for potential readmissions from December of the previous year, as patient identifiers do not link across years in the NRD. We also limited our evaluation to the conventional 30-day readmission window, but recent publications may indicate that readmission windows with different timelines could be a more accurate reflection of medically preventable readmissions versus a reflection of social determinants of health leading to readmissions.24 Newborn index admissions were not an allowable index admission; therefore, we may be underreporting readmissions in the neonatal age group. We also chose to include all-cause readmissions, a conventional method to evaluate readmission within an institution, but which may not reflect the quality of care delivered in the index admission. For example, an asthmatic discharged after an acute exacerbation readmitted for dehydration secondary to gastroenteritis may not reflect a lack of quality in asthma inpatient care. Readmissions were limited to the same hospital; therefore, this study cannot account for readmissions at other institutions, which may cause us to underestimate readmission rates. However, end-users of our findings most likely have access only to their own institution’s data. The inclusion of observation status admissions in the database varies from state to state; therefore, this percent of admissions in the database is unknown.
The use of the ABC methodology has some inherent limitations. One hospital with a significant volume diagnosis and low readmission rate within a hospital type may prohibit the reporting of an ABC if less than three hospitals composed the total of the ‘best performing’ hospitals. This was a significant limitation leading to the exclusion of many ABCs in nonmetropolitan institutions. The limitation of calculating and reporting an ABC then prohibits the calculation of outlier hospitals within a hospital type for a given diagnosis. However, when the ABCs are not available, we do provide the mean readmission rate for the diagnosis within the hospital type. While the hospital groupings by population and teaching status for ABCs provide meaningful comparisons for within each hospital setting, it should be noted that there may be vast differences among hospitals within each type (eg, tertiary children’s hospitals compared to teaching hospitals with a pediatric floor in the metropolitan teaching hospital category).39,40
As healthcare moves from a fee-for-service model to a population-health centered, value-based model, reduction in readmission rates will be more than a quality measure and will have potential financial implications.41 In the Medicare fee-for-service patients, the Hospital Readmission Reduction Program (HRRP) penalize hospitals with excess readmissions for acute myocardial infarction, heart failure, and pneumonia. The hospitals subject to penalties in the HRRP had greater reduction in readmission rates in the targeted, and even nontargeted conditions, compared with hospitals not subject to penalties.42 Similarly, we believe that our data on low readmission rates and ABCs for conditions such as asthma, bronchiolitis, and appendicitis could represent decades of quality improvement work for the most common pediatric conditions among hospitalized children. Sickle cell disease and mental health problems remain as outliers and merit further attention. To move to a true population-health model, hospitals will need to explore outlier conditions including evaluating patient-level readmission patterns across institutions. This moves readmission from a hospital quality measure to a patient-centric quality measure, and perhaps will provide value to the patient and the healthcare system alike.
CONCLUSIONS
The readmission ABCs for the most commonly readmitted pediatric diagnoses are low, regardless of the hospital setting. The highest pediatric readmission rates in SCD, bipolar disorders, and major depressive disorder were lower than the most common adult readmission diagnoses. However, mental health conditions and SCD remain as outliers for pediatric readmissions, burden hospital systems, and perhaps warrant national-level attention. The ABCs stratified by hospital type in this study facilitate comparisons and identify opportunities for population-level interventions to meaningfully improve patient care.
Disclosures
The authors have nothing to disclose.
Hospital readmission rates are a common metric for defining, evaluating, and benchmarking quality of care. The Centers for Medicare and Medicaid Services (CMS) publicly report hospital readmission rates for common adult conditions and reduces payments to hospitals with excessive readmissions.1 Recently, the focus on pediatric readmission rates has increased and the National Quality Forum (NQF) has endorsed at least two pediatric readmission-specific quality indicators which could be used by public and private payers in pay-for-performance programs aimed at institutions caring for children.2 While preventability of readmissions and their value as a marker of quality remains debated, their acceptance by the NQF and CMS has led public and private payers to propose readmission-related penalties for hospitals caring for children. 3-5
All-cause 30-day same-hospital readmission rates for pediatric conditions are half of the adult readmission rates, around 6% in most studies, compared to 12% in adults.6,7 The lower rates of pediatric readmissions makes it difficult to only use mean readmission rates to stratify hospitals into high- or low-performers and set target goals for improvement.8 While adult readmissions have been studied in depth, there are no consistent measures used to benchmark pediatric readmissions across hospital types.
Given the emphasis placed on readmissions, it is essential to understand patterns in pediatric readmission rates to determine optimal and achievable targets for improvement. Achievable Benchmarks of Care (ABCs) are one approach to understanding readmission rates and have an advantage over using mean or medians in performance improvement as they can stratify performance for conditions with low readmission rates and low volumes.9 When creating benchmarks, it is important that hospitals performance is evaluated among peer hospitals with similar patient populations, not just a cumulative average from all hospital types which may punish hospitals with a more complex patient case mix.10 The goal of this study was to calculate the readmission rates and the ABCs for common pediatric diagnoses by hospital type to identify priority conditions for quality improvement efforts using a previously published methodology.11-13
METHODS
Data Source
We conducted a retrospective analysis of patients less than 18 years of age in the Healthcare Utilization Project 2014 Nationwide Readmissions Database (NRD). The NRD includes public hospitals; academic medical centers; and specialty hospitals in obstetrics and gynecology, otolaryngology, orthopedics, and cancer; and pediatric, public, and academic medical hospitals. Excluded are long-term care facilities such as rehabilitation, long-term acute care, psychiatric, alcoholism, and chemical dependency hospitals. The readmissions data contains information from hospitals grouped by region, population census, and teaching status.14 Three hospital type classifications used in this study were metropolitan teaching hospitals, metropolitan nonteaching hospitals, and nonmetropolitan hospitals. These three hospital type classifications follow the reporting format in the NRD.
Study Population
Patients less than 18 years old were included if they were discharged from January 1, 2014 through November 30, 2014 and had a readmission to the index hospital within 30 days. We limited inclusion to discharges through November 30 so we could identify patients with a 30-day readmission as patient identifiers do not link across years in the NRD.
Exposure
We included 30-day, all-cause, same-hospital readmissions to the index acute care hospital, excluding labor and delivery, normal newborn care, chemotherapy, transfers, and mortalities. Intrahospital discharge and admissions within the same hospital system were not defined as a readmission, but rather as a “same-day event.”15 For example, institutions with inpatient mental health facilities, medical unit discharges and admission to the mental health unit were not identified as a readmission in this dataset.
Outcome
For each hospital type, we measured same-hospital, all-cause, 30-day readmission rates and achievable benchmark of care for the 17 most commonly readmitted pediatric discharge diagnoses. To identify the target readmission diagnoses and all-cause, 30-day readmissions based on their index hospitalizations, All-Patient Refined Diagnosis-Related Groups (APR-DRG), version 25 (3M Health Information Systems, Salt Lake City, Utah) were ordered by frequency for each hospital type. The 20 most common APR-DRGs were the same across all hospital types. The authors then evaluated these 20 APR-DRGs for clinical consistency of included diagnoses identified by the International Classification of Diseases, Ninth Revision, Clinical Modification (ICD-9-CM) codes within each APR-DRG. Three diagnosis-related groups were excluded from the analysis (major hematologic/immunologic disease except for sickle cell, other anemia and disorders of blood and blood forming organs, and other digestive system diagnoses) due to the heterogeneity of the diagnoses identified by the ICD-9-CM codes within each APR-DRG. We refer to each APR-DRG as a “diagnosis” throughout the article.
Analysis
The demographic characteristics of the patients seen at the three hospital types were summarized using frequencies and percentages. Reports were generated for patient age, gender, payer source, patient residence, median household income, patient complexity, and discharge disposition. Patient complexity was defined using complex chronic condition (CCC) and the number of chronic conditions (CCI).16,17 As previously defined in the literature, a complex chronic condition is “any medical condition that can be reasonably expected to last at least 12 months (unless death intervenes) and to involve either several different organ systems or one organ system severely enough to require specialty pediatric care and probably some period of hospitalization in a tertiary care center.”16 Whereas, the Agency for Healthcare Research and Quality’s Chronic Condition Indicator (CCI) defines single, non-CCCs (eg, allergic rhinitis).17
For each diagnosis, we calculated the mean readmission rate for hospitals in each hospital type category. We then calculated an ABC for each diagnosis in each hospital type using a four-step process.13,18 First, to control for hospitals with small sample sizes, we adjusted all readmission rates using an adjusted performance fraction ([numerator+1]/[denominator +2]), where the numerator is the number of all-cause 30-day readmissions and the denominator is the number of discharges for the selected diagnosis. Then the hospitals were ordered from lowest (best performing) to highest (worst performing) using the adjusted readmission rate. Third, the number of discharges from the best performing hospital to the worst performing hospital was summed until at least 10% of the total discharges had been accounted for. Finally, we computed the ABC as the average of these best performing hospitals. We only report ABCs for which at least three hospitals were included as best performers in the calculation.13
To evaluate hospital performance on ABCs for each diagnosis, we identified the percent of hospitals in each setting that were outliers. We defined an outlier as any hospital whose 95% confidence interval for their readmission rate for a given diagnosis did not contain the ABC for their hospital type. All the statistical analyses were performed using SAS version 9.3 (SAS Institute, Inc, Cary, North Carolina).
This project was reviewed by the Cincinnati Children’s Hospital Medical Center Institutional Review Board and determined to be nonhuman subjects research.
RESULTS
Hospital-Type Demographics
The 690,949 discharges from 1,664 hospitals were categorized into 525 metropolitan teaching (550,039 discharges, 79.6% of discharges), 552 metropolitan nonteaching (97,207 discharges, 14% of discharges), and 587 nonmetropolitan hospitals (43,703 discharges, 6.3% of discharges; Table 1). There were significant differences in the patient composition among the three hospital settings. Nonmetropolitan hospitals had a larger percentage of younger patients (aged 0-4 years, P < .001), prominence of first and second quartile median household income, and fewer medically complex patients (48.3% No CCC/No CCI versus 25.5% metropolitan teaching and 33.7% nonteaching, P < .001). Disposition home was over 96% in all three hospital types; however, the metropolitan teaching had a greater percentage of patients discharged to home health versus metropolitan nonteaching and nonmetropolitan hospitals (2.3% versus 0.5%; P < .001).
Readmission Rates
The 17 most common diagnoses based on the number of all-cause 30-day same-hospital readmissions, were categorized into two surgical, seven acute/infectious, four chronic, and four mental health diagnoses (Table 2). Readmission rates varied based on diagnosis and hospital type (Table 2). Overall, mean readmission rates were low, especially in acute respiratory tract related diseases. For chronic diseases, asthma readmissions were consistently low in all three hospital types, whereas sickle cell disease had the highest readmission rate in all three hospital types.
Achievable Benchmarks of Care by Hospital Type
The diagnoses for which ABC could be calculated across all three hospital types included appendectomy and four acute conditions (bronchiolitis, pneumonia, nonbacterial gastroenteritis, and kidney/urinary tract infections). For these conditions, metropolitan teaching hospitals had a more significant percentage of outlier hospitals compared to metropolitan nonteaching and nonmetropolitan hospitals. The percent of outlier hospitals varied by diagnosis and hospital type (Figure).
Metropolitan Teaching
The readmission ABC was calculated for all 17 diagnoses (Table 2). The ABC ranged from 0.4% in acute kidney and urinary tract infection to 7.0% in sickle cell anemia crisis. Bipolar disorder, major depressive disorders and other psychoses, and sickle cell disease (SCD) had the highest percent of outlier hospitals whose mean readmission rates confidence interval did not contain the ABC; tonsil and adenoid procedures and viral illness had the lowest.1
Metropolitan Nonteaching
The ABC was calculated for 13 of the 17 diagnoses because ABCs were not calculated when there were fewer than three best practicing hospitals. This was the case for tonsil and adenoid procedures, diabetes, seizures, and depression except for major depressive disorder (Table 2). Seven of the 13 diagnoses had an ABC of 0.0%: viral illness, infections of the upper respiratory tract, bronchiolitis, gastroenteritis, hypovolemia and electrolyte disorders, asthma, and childhood behavioral disorders. Like the findings at the metropolitan teaching hospitals, ABCs were lowest for surgical and acute conditions while bipolar disorder, major depressive disorders and other psychoses, and SCD had the highest percent of outlier hospitals with readmission rates beyond the 95% confidence interval of their hospital type’s ABC.
Nonmetropolitan
There was a sufficient number of best practicing hospitals to calculate the ABC for six of the 17 diagnoses (Table 2). For conditions where readmission ABCs could be calculated, they were low: 0.0% for appendectomy, bronchiolitis, gastroenteritis, and seizure; 0.3% for pneumonia; and 1.3% in kidney and urinary tract disorders. None of the conditions with the highest ABCs in other hospital settings (bipolar disease, sickle cell anemia crisis, and major depressive disorders and other psychoses) could be calculated in this setting. Seizure-related readmissions exhibited the most outlier hospitals yet were less than 5%.1
DISCUSSION
Among a nationally representative sample of different hospital types that deliver care to children, we report the mean readmission rates and ABCs for 30-day all-cause, same-hospital readmissions for the most commonly readmitted pediatric diagnoses based on hospital type. Previous studies have shown patient variables such as race, ethnicity, and insurance type influencing readmission rates.19,20 However, hospital type has also been associated with a higher risk of readmission due to the varying complexity of patients at different hospital types.21,22 Our analyses provide hospital-type specific national estimates of pediatric readmission ABCs for medical and surgical conditions, many less than 1%. While commonly encountered pediatric conditions like asthma and bronchiolitis had low mean readmission rates and ABCs across all hospital types, the mean rates and ABCs for SCD and mental health disorders were much higher with more hospitals performing far from the ABCs.
Diagnoses with a larger percentage of outlier hospitals may represent a national opportunity to improve care for children. Conditions such as SCD and mental illnesses have the highest percentage of hospitals whose readmission rates fall outside of the ABCs in both metropolitan teaching and metropolitan nonteaching hospitals. Hospital performance on SCD and mental health disorders may not reflect deficits in hospital quality or poor adherence to evidence-based best practices, but rather the complex interplay of factors on various levels from government policy and insurance plans, to patient and family resources, to access and availability of medical and mental health specific care. Most importantly, these diseases may represent a significant opportunity for quality improvementin hospitals across the United States.
Sickle cell disease is predominantly a disease among African-Americans, a demographic risk factor for decreased access to care and limited patient and family resources.23-26 In previous studies evaluating the disparity in readmission rates for Black children with asthma, socioeconomic variables explained 53% of the observed disparity and readmission rates were inversely related to the childhood opportunity index of the patient’s census tract and positively related with geographic social risk.27,28 Likewise, with SCD affecting a specific demographic and being a chronic disease, best practice policies need to account for the child’s medical needs and include the patient and family resources to ensure access to care and enhanced case management for chronic disease if we aim to improve performance among the outlier hospitals.
Similarly, barriers to care for children with mental illnesses in the United States need attention.29,30 While there is a paucity of data on the prevalence of mental health disorders in children, one national report estimates that one in 10 American adolescents have depression.29,31 The American Academy of Pediatrics has developed a policy statement on mental health competencies and a mental health tool-kit for primary care pediatricians; however, no such guidelines or policy statements exist for hospitalized patients with acute or chronic psychiatric conditions.32,33 Moreover, hospitals are increasingly facing “boarding” of children with acute psychiatric illness in inpatient units and emergency departments.34 The American Medical Association and the American College of Emergency Physicians have expressed concerns regarding the boarding of children with acute psychiatric illness because nonpsychiatric hospitals do not have adequate resources to evaluate, manage, and place these children who deserve appropriate facilities for further management. Coordinated case management and “bundled” discharge planning in other chronic illnesses have shown benefit in cost reduction and readmission.35-37 Evidence-based practices around pediatric readmissions in other diagnoses should be explored as possible interventions in these conditions.38
There are several limitations to this study. Our data is limited to one calendar year; therefore, admissions in January do not account for potential readmissions from December of the previous year, as patient identifiers do not link across years in the NRD. We also limited our evaluation to the conventional 30-day readmission window, but recent publications may indicate that readmission windows with different timelines could be a more accurate reflection of medically preventable readmissions versus a reflection of social determinants of health leading to readmissions.24 Newborn index admissions were not an allowable index admission; therefore, we may be underreporting readmissions in the neonatal age group. We also chose to include all-cause readmissions, a conventional method to evaluate readmission within an institution, but which may not reflect the quality of care delivered in the index admission. For example, an asthmatic discharged after an acute exacerbation readmitted for dehydration secondary to gastroenteritis may not reflect a lack of quality in asthma inpatient care. Readmissions were limited to the same hospital; therefore, this study cannot account for readmissions at other institutions, which may cause us to underestimate readmission rates. However, end-users of our findings most likely have access only to their own institution’s data. The inclusion of observation status admissions in the database varies from state to state; therefore, this percent of admissions in the database is unknown.
The use of the ABC methodology has some inherent limitations. One hospital with a significant volume diagnosis and low readmission rate within a hospital type may prohibit the reporting of an ABC if less than three hospitals composed the total of the ‘best performing’ hospitals. This was a significant limitation leading to the exclusion of many ABCs in nonmetropolitan institutions. The limitation of calculating and reporting an ABC then prohibits the calculation of outlier hospitals within a hospital type for a given diagnosis. However, when the ABCs are not available, we do provide the mean readmission rate for the diagnosis within the hospital type. While the hospital groupings by population and teaching status for ABCs provide meaningful comparisons for within each hospital setting, it should be noted that there may be vast differences among hospitals within each type (eg, tertiary children’s hospitals compared to teaching hospitals with a pediatric floor in the metropolitan teaching hospital category).39,40
As healthcare moves from a fee-for-service model to a population-health centered, value-based model, reduction in readmission rates will be more than a quality measure and will have potential financial implications.41 In the Medicare fee-for-service patients, the Hospital Readmission Reduction Program (HRRP) penalize hospitals with excess readmissions for acute myocardial infarction, heart failure, and pneumonia. The hospitals subject to penalties in the HRRP had greater reduction in readmission rates in the targeted, and even nontargeted conditions, compared with hospitals not subject to penalties.42 Similarly, we believe that our data on low readmission rates and ABCs for conditions such as asthma, bronchiolitis, and appendicitis could represent decades of quality improvement work for the most common pediatric conditions among hospitalized children. Sickle cell disease and mental health problems remain as outliers and merit further attention. To move to a true population-health model, hospitals will need to explore outlier conditions including evaluating patient-level readmission patterns across institutions. This moves readmission from a hospital quality measure to a patient-centric quality measure, and perhaps will provide value to the patient and the healthcare system alike.
CONCLUSIONS
The readmission ABCs for the most commonly readmitted pediatric diagnoses are low, regardless of the hospital setting. The highest pediatric readmission rates in SCD, bipolar disorders, and major depressive disorder were lower than the most common adult readmission diagnoses. However, mental health conditions and SCD remain as outliers for pediatric readmissions, burden hospital systems, and perhaps warrant national-level attention. The ABCs stratified by hospital type in this study facilitate comparisons and identify opportunities for population-level interventions to meaningfully improve patient care.
Disclosures
The authors have nothing to disclose.
1. Medicare. 30-day death and readmission measures data. https://www.medicare.gov/hospitalcompare/Data/30-day-measures.html. Accessed October 24, 2017.
2. National Quality Forum. Performance Measures; 2016 https://www.quality fourm.org/Measuring_Performance/Endorsed_Performance_Measures_Maintenance.aspx. Accessed October 24, 2017.
3. Auger KA, Simon TD, Cooperberg D, et al. Summary of STARNet: seamless transitions and (re)admissions network. Pediatrics. 2015;135(1):164-175. https://doi.org/10.1542/peds.2014-1887.
4. Toomey SL, Peltz A, Loren S, et al. Potentially preventable 30-day hospital readmissions at a children’s hospital. Pediatrics. 2016;138(2):e20154182-e20154182. https://doi.org/10.1542/peds.2015-4182.
5. Halfon P, Eggli Y, Prêtre-Rohrbach I, et al. Validation of the potentially avoidable hospital readmission rate as a routine indicator of the quality of hospital care. Med Care. 2006;44(11):972-981. https://doi.org/10.1097/01.mlr.0000228002.43688.c2.
6. Gay JC, Agrawal R, Auger KA, et al. Rates and impact of potentially preventable readmissions at children’s hospitals. J Pediatr. 2015;166(3):613-619. https://doi.org/10.1016/j.jpeds.2014.10.052.
7. Berry JG, Gay JC, Joynt Maddox KJ, et al. Age trends in 30 day hospital readmissions: US national retrospective analysis. BMJ. 2018;360:k497. https://doi.org/10.1136/bmj.k497.
8. Bardach NS, Vittinghoff E, Asteria-Penaloza R, et al. Measuring hospital quality using pediatric readmission and revisit rates. Pediatrics. 2013;132(3):429-436. https://doi.org/10.1542/peds.2012-3527d.
9. Berry JG, Toomey SL, Zaslavsky AM, et al. Pediatric readmission prevalence and variability across hospitals. JAMA. 2013;309(4):372-380. https://doi.org/10.1001/jama.2012.188351.
10. Gohil SK, Datta R, Cao C, et al. Impact of hospital population case-mix, including poverty, on hospital all-cause and infection-related 30-day readmission rates. Clin Infect Dis. 2015;61(8):1235-1243. https://doi.org/10.1093/cid/civ539.
11. Parikh K, Hall M, Mittal V, et al. Establishing benchmarks for the hospitalized care of children with asthma, bronchiolitis, and pneumonia. Pediatrics. 2014;134(3):555-562. https://doi.org/10.1542/peds.2014-1052.
12. Reyes M, Paulus E, Hronek C, et al. Choosing wisely campaign: report card and achievable benchmarks of care for children’s hospitals. Hosp Pediatr. 2017;7(11):633-641. https://doi.org/10.1542/hpeds.2017-0029.
13. Kiefe CI, Weissman NW, Allison JJ, et al. Identifying achievable benchmarks of care: concepts and methodology. Int J Qual Health Care. 1998;10(5):443-447. https://doi.org/10.1093/intqhc/10.5.443.
14. Agency for Healthcare Research and Quality. Nationwide Readmissions Database Availability of Data Elements. . https://www.hcup-us.ahrq.gov/partner/MOARef/HCUPdata_elements.pdf. Accessed 2018 Jun 6
15. Healthcare Cost and Utilization Project. HCUP NRD description of data elements. Agency Healthc Res Qual. https://www.hcup-us.ahrq.gov/db/vars/samedayevent/nrdnote.jsp. Accessed 2018 Jun 6; 2015.
16. Feudtner C, Feinstein JA, Zhong W, Hall M, Dai D. Pediatric complex chronic conditions classification system version 2: updated for ICD-10 and complex medical technology dependence and transplantation. BMC Pediatr. 2014;14:199. https://doi.org/10.1186/1471-2431-14-199.
17. Agency for Healthcare Research and Quality. HCUP chronic condition indicator. Healthc Cost Util Proj. https://www.hcup-us.ahrq.gov/toolssoftware/chronic/chronic.jsp. Accessed 2016 Apr 26; 2009.
18. Weissman NW, Allison JJ, Kiefe CI, et al. Achievable benchmarks of care: the ABCs of benchmarking. J Eval Clin Pract. 1999;5(3):269-281. https://doi.org/10.1046/j.1365-2753.1999.00203.x.
19. Joynt KE, Orav EJ, Jha AK. Thirty-day readmission rates for medicare beneficiaries by race and site of care. JAMA. 2011;305(7):675-681. https://doi.org/10.1001/jama.2011.123.
20. Kenyon CC, Melvin PR, Chiang VW, et al. Rehospitalization for childhood asthma: timing, variation, and opportunities for intervention. J Pediatr. 2014;164(2):300-305. https://doi.org/10.1016/j.jpeds.2013.10.003.
21. Sobota A, Graham DA, Neufeld EJ, Heeney MM. Thirty-day readmission rates following hospitalization for pediatric sickle cell crisis at freestanding children’s hospitals: risk factors and hospital variation. Pediatr Blood Cancer. 2012;58(1):61-65. https://doi.org/10.1002/pbc.23221.
22. Berry JG, Hall DE, Kuo DZ, et al. Hospital utilization and characteristics of patients experiencing recurrent readmissions within children’s hospitals. JAMA. 2011;305(7):682-690. https://doi.org/10.1001/jama.2011.122.
23. Ginde AA, Espinola JA, Camargo CA. Improved overall trends but persistent racial disparities in emergency department visits for acute asthma, 1993-2005. J Allergy Clin Immunol. 2008;122(2):313-318. https://doi.org/10.1016/j.jaci.2008.04.024.
24. Parikh K, Berry J, Hall M, et al. Racial and ethnic differences in pediatric readmissions for common chronic conditions. J Pediatr. 2017;186. https://doi.org/10.1016/j.jpeds.2017.03.046.
25. Chen BK, Hibbert J, Cheng X, Bennett K. Travel distance and sociodemographic correlates of potentially avoidable emergency department visits in California, 2006-2010: an observational study. Int J Equity Health. 2015;14(1):30. https://doi.org/10.1186/s12939-015-0158-y.
26. Ray KN, Chari AV, Engberg J, et al. Disparities in time spent seeking medical care in the United States. JAMA Intern Med. 2015;175(12):175(12):1983-1986. https://doi.org/10.1001/jamainternmed.2015.4468.
27. Beck AF, Huang B, Wheeler K, et al. The child opportunity index and disparities in pediatric asthma hospitalizations across one Ohio metropolitan area. J Pediatr. 2011-2013;190:200-206. https://doi.org/10.1016/j.jpeds.2017.08.007.
28. Beck AF, Simmons JM, Huang B, Kahn RS. Geomedicine: area-based socioeconomic measures for assessing the risk of hospital reutilization among children admitted for asthma. Am J Public Health. 2012;102(12):2308-2314. https://doi.org/10.2105/AJPH.2012.300806.
29. Avenevoli S, Swendsen J, He JP, Burstein M, Merikangas KR. Major depression in the national comorbidity survey-adolescent supplement: prevalence, correlates, and treatment. J Am Acad Child Adolesc Psychiatry. 2015;54(1):37-44.e2. https://doi.org/10.1016/j.jaac.2014.10.010.
30. Feng JY, Toomey SL, Zaslavsky AM, Nakamura MM, Schuster MA. Readmission after pediatric mental health admissions. Pediatrics. 2017;140(6):e20171571. https://doi.org/10.1542/peds.2017-1571.
31. Merikangas KR, He JP, Burstein M, et al. Lifetime prevalence of mental disorders in U.S. adolescents: results from the National comorbidity Survey Replication-Adolescent Supplement (NCS-A). J Am Acad Child Adolesc Psychiatry. 2010;49(10):980-989. https://doi.org/10.1016/j.jaac.2010.05.017.
32. Cheung AH, Zuckerbrot RA, Jensen PS, et al. Guidelines for adolescent depression in primary care (GLAD-PC): Part II. Treatment and ongoing management. Pediatrics. 2018;141(3):e20174082. https://doi.org/10.1542/peds.2017-4082.
33. Zuckerbrot RA, Cheung A, Jensen PS, et al. Guidelines for adolescent depression in primary care (GLAD-PC): Part I. Practice preparation, identification, assessment, and initial management. Pediatrics. 2018;141(3):e20174081. https://doi.org/10.1542/peds.2017-4081.
34. Dolan MA, Fein JA, Committee on Pediatric Emergency Medicine. Pediatric and adolescent mental health emergencies in the emergency Medical Services system. Pediatrics. 2011;127(5):e1356-e1366. https://doi.org/10.1542/peds.2011-0522.
35. Collaborative Healthcare Strategies. Hospital Guide to Reducing Medicaid Readmissions. Rockville, MD: 2014. https://www.ahrq.gov/sites/default/files/publications/files/medreadmissions.pdf. Accessed 2017 Oct 11.
36. Hilbert K, Payne R, Wooton S. Children’s Hospitals’ Solutions for Patient Safety. Readmissions Bundle Tools. Cincinnati, OH; 2014.
37. Nuckols TK, Keeler E, Morton S, et al. Economic evaluation of quality improvement interventions designed to prevent hospital readmission: a systematic review and meta-analysis. JAMA Intern Med. 2017;177(7):975-985. https://doi.org/10.1001/jamainternmed.2017.1136.
38. Berry JG, Blaine K, Rogers J, et al. A framework of pediatric hospital discharge care informed by legislation, research, and practice. JAMA Pediatr. 2014;168(10):955-962. https://doi.org/10.1001/jamapediatrics.2014.891.
39. Chen HF, Carlson E, Popoola T, Suzuki S. The impact of rurality on 30-day preventable readmission, illness severity, and risk of mortality for heart failure Medicare home health beneficiaries. J Rural Health. 2016;32(2):176-187. https://doi.org/10.1111/jrh.12142.
40. Khan A, Nakamura MM, Zaslavsky AM, et al. Same-hospital readmission rates as a measure of pediatric quality of care. JAMA Pediatr. 2015;169(10):905-912. https://doi.org/10.1001/jamapediatrics.2015.1129.
41. Share DA, Campbell DA, Birkmeyer N, et al. How a regional collaborative of hospitals and physicians in Michigan cut costs and improved the quality of care. Health Aff. 2011;30(4):636-645. https://doi.org/10.1377/hlthaff.2010.0526.
42. Desai NR, Ross JS, Kwon JY, et al. Association between hospital penalty status under the hospital readmission reduction program and readmission rates for target and nontarget conditions. JAMA. 2016;316(24):2647-2656. https://doi.org/10.1001/jama.2016.18533.
1. Medicare. 30-day death and readmission measures data. https://www.medicare.gov/hospitalcompare/Data/30-day-measures.html. Accessed October 24, 2017.
2. National Quality Forum. Performance Measures; 2016 https://www.quality fourm.org/Measuring_Performance/Endorsed_Performance_Measures_Maintenance.aspx. Accessed October 24, 2017.
3. Auger KA, Simon TD, Cooperberg D, et al. Summary of STARNet: seamless transitions and (re)admissions network. Pediatrics. 2015;135(1):164-175. https://doi.org/10.1542/peds.2014-1887.
4. Toomey SL, Peltz A, Loren S, et al. Potentially preventable 30-day hospital readmissions at a children’s hospital. Pediatrics. 2016;138(2):e20154182-e20154182. https://doi.org/10.1542/peds.2015-4182.
5. Halfon P, Eggli Y, Prêtre-Rohrbach I, et al. Validation of the potentially avoidable hospital readmission rate as a routine indicator of the quality of hospital care. Med Care. 2006;44(11):972-981. https://doi.org/10.1097/01.mlr.0000228002.43688.c2.
6. Gay JC, Agrawal R, Auger KA, et al. Rates and impact of potentially preventable readmissions at children’s hospitals. J Pediatr. 2015;166(3):613-619. https://doi.org/10.1016/j.jpeds.2014.10.052.
7. Berry JG, Gay JC, Joynt Maddox KJ, et al. Age trends in 30 day hospital readmissions: US national retrospective analysis. BMJ. 2018;360:k497. https://doi.org/10.1136/bmj.k497.
8. Bardach NS, Vittinghoff E, Asteria-Penaloza R, et al. Measuring hospital quality using pediatric readmission and revisit rates. Pediatrics. 2013;132(3):429-436. https://doi.org/10.1542/peds.2012-3527d.
9. Berry JG, Toomey SL, Zaslavsky AM, et al. Pediatric readmission prevalence and variability across hospitals. JAMA. 2013;309(4):372-380. https://doi.org/10.1001/jama.2012.188351.
10. Gohil SK, Datta R, Cao C, et al. Impact of hospital population case-mix, including poverty, on hospital all-cause and infection-related 30-day readmission rates. Clin Infect Dis. 2015;61(8):1235-1243. https://doi.org/10.1093/cid/civ539.
11. Parikh K, Hall M, Mittal V, et al. Establishing benchmarks for the hospitalized care of children with asthma, bronchiolitis, and pneumonia. Pediatrics. 2014;134(3):555-562. https://doi.org/10.1542/peds.2014-1052.
12. Reyes M, Paulus E, Hronek C, et al. Choosing wisely campaign: report card and achievable benchmarks of care for children’s hospitals. Hosp Pediatr. 2017;7(11):633-641. https://doi.org/10.1542/hpeds.2017-0029.
13. Kiefe CI, Weissman NW, Allison JJ, et al. Identifying achievable benchmarks of care: concepts and methodology. Int J Qual Health Care. 1998;10(5):443-447. https://doi.org/10.1093/intqhc/10.5.443.
14. Agency for Healthcare Research and Quality. Nationwide Readmissions Database Availability of Data Elements. . https://www.hcup-us.ahrq.gov/partner/MOARef/HCUPdata_elements.pdf. Accessed 2018 Jun 6
15. Healthcare Cost and Utilization Project. HCUP NRD description of data elements. Agency Healthc Res Qual. https://www.hcup-us.ahrq.gov/db/vars/samedayevent/nrdnote.jsp. Accessed 2018 Jun 6; 2015.
16. Feudtner C, Feinstein JA, Zhong W, Hall M, Dai D. Pediatric complex chronic conditions classification system version 2: updated for ICD-10 and complex medical technology dependence and transplantation. BMC Pediatr. 2014;14:199. https://doi.org/10.1186/1471-2431-14-199.
17. Agency for Healthcare Research and Quality. HCUP chronic condition indicator. Healthc Cost Util Proj. https://www.hcup-us.ahrq.gov/toolssoftware/chronic/chronic.jsp. Accessed 2016 Apr 26; 2009.
18. Weissman NW, Allison JJ, Kiefe CI, et al. Achievable benchmarks of care: the ABCs of benchmarking. J Eval Clin Pract. 1999;5(3):269-281. https://doi.org/10.1046/j.1365-2753.1999.00203.x.
19. Joynt KE, Orav EJ, Jha AK. Thirty-day readmission rates for medicare beneficiaries by race and site of care. JAMA. 2011;305(7):675-681. https://doi.org/10.1001/jama.2011.123.
20. Kenyon CC, Melvin PR, Chiang VW, et al. Rehospitalization for childhood asthma: timing, variation, and opportunities for intervention. J Pediatr. 2014;164(2):300-305. https://doi.org/10.1016/j.jpeds.2013.10.003.
21. Sobota A, Graham DA, Neufeld EJ, Heeney MM. Thirty-day readmission rates following hospitalization for pediatric sickle cell crisis at freestanding children’s hospitals: risk factors and hospital variation. Pediatr Blood Cancer. 2012;58(1):61-65. https://doi.org/10.1002/pbc.23221.
22. Berry JG, Hall DE, Kuo DZ, et al. Hospital utilization and characteristics of patients experiencing recurrent readmissions within children’s hospitals. JAMA. 2011;305(7):682-690. https://doi.org/10.1001/jama.2011.122.
23. Ginde AA, Espinola JA, Camargo CA. Improved overall trends but persistent racial disparities in emergency department visits for acute asthma, 1993-2005. J Allergy Clin Immunol. 2008;122(2):313-318. https://doi.org/10.1016/j.jaci.2008.04.024.
24. Parikh K, Berry J, Hall M, et al. Racial and ethnic differences in pediatric readmissions for common chronic conditions. J Pediatr. 2017;186. https://doi.org/10.1016/j.jpeds.2017.03.046.
25. Chen BK, Hibbert J, Cheng X, Bennett K. Travel distance and sociodemographic correlates of potentially avoidable emergency department visits in California, 2006-2010: an observational study. Int J Equity Health. 2015;14(1):30. https://doi.org/10.1186/s12939-015-0158-y.
26. Ray KN, Chari AV, Engberg J, et al. Disparities in time spent seeking medical care in the United States. JAMA Intern Med. 2015;175(12):175(12):1983-1986. https://doi.org/10.1001/jamainternmed.2015.4468.
27. Beck AF, Huang B, Wheeler K, et al. The child opportunity index and disparities in pediatric asthma hospitalizations across one Ohio metropolitan area. J Pediatr. 2011-2013;190:200-206. https://doi.org/10.1016/j.jpeds.2017.08.007.
28. Beck AF, Simmons JM, Huang B, Kahn RS. Geomedicine: area-based socioeconomic measures for assessing the risk of hospital reutilization among children admitted for asthma. Am J Public Health. 2012;102(12):2308-2314. https://doi.org/10.2105/AJPH.2012.300806.
29. Avenevoli S, Swendsen J, He JP, Burstein M, Merikangas KR. Major depression in the national comorbidity survey-adolescent supplement: prevalence, correlates, and treatment. J Am Acad Child Adolesc Psychiatry. 2015;54(1):37-44.e2. https://doi.org/10.1016/j.jaac.2014.10.010.
30. Feng JY, Toomey SL, Zaslavsky AM, Nakamura MM, Schuster MA. Readmission after pediatric mental health admissions. Pediatrics. 2017;140(6):e20171571. https://doi.org/10.1542/peds.2017-1571.
31. Merikangas KR, He JP, Burstein M, et al. Lifetime prevalence of mental disorders in U.S. adolescents: results from the National comorbidity Survey Replication-Adolescent Supplement (NCS-A). J Am Acad Child Adolesc Psychiatry. 2010;49(10):980-989. https://doi.org/10.1016/j.jaac.2010.05.017.
32. Cheung AH, Zuckerbrot RA, Jensen PS, et al. Guidelines for adolescent depression in primary care (GLAD-PC): Part II. Treatment and ongoing management. Pediatrics. 2018;141(3):e20174082. https://doi.org/10.1542/peds.2017-4082.
33. Zuckerbrot RA, Cheung A, Jensen PS, et al. Guidelines for adolescent depression in primary care (GLAD-PC): Part I. Practice preparation, identification, assessment, and initial management. Pediatrics. 2018;141(3):e20174081. https://doi.org/10.1542/peds.2017-4081.
34. Dolan MA, Fein JA, Committee on Pediatric Emergency Medicine. Pediatric and adolescent mental health emergencies in the emergency Medical Services system. Pediatrics. 2011;127(5):e1356-e1366. https://doi.org/10.1542/peds.2011-0522.
35. Collaborative Healthcare Strategies. Hospital Guide to Reducing Medicaid Readmissions. Rockville, MD: 2014. https://www.ahrq.gov/sites/default/files/publications/files/medreadmissions.pdf. Accessed 2017 Oct 11.
36. Hilbert K, Payne R, Wooton S. Children’s Hospitals’ Solutions for Patient Safety. Readmissions Bundle Tools. Cincinnati, OH; 2014.
37. Nuckols TK, Keeler E, Morton S, et al. Economic evaluation of quality improvement interventions designed to prevent hospital readmission: a systematic review and meta-analysis. JAMA Intern Med. 2017;177(7):975-985. https://doi.org/10.1001/jamainternmed.2017.1136.
38. Berry JG, Blaine K, Rogers J, et al. A framework of pediatric hospital discharge care informed by legislation, research, and practice. JAMA Pediatr. 2014;168(10):955-962. https://doi.org/10.1001/jamapediatrics.2014.891.
39. Chen HF, Carlson E, Popoola T, Suzuki S. The impact of rurality on 30-day preventable readmission, illness severity, and risk of mortality for heart failure Medicare home health beneficiaries. J Rural Health. 2016;32(2):176-187. https://doi.org/10.1111/jrh.12142.
40. Khan A, Nakamura MM, Zaslavsky AM, et al. Same-hospital readmission rates as a measure of pediatric quality of care. JAMA Pediatr. 2015;169(10):905-912. https://doi.org/10.1001/jamapediatrics.2015.1129.
41. Share DA, Campbell DA, Birkmeyer N, et al. How a regional collaborative of hospitals and physicians in Michigan cut costs and improved the quality of care. Health Aff. 2011;30(4):636-645. https://doi.org/10.1377/hlthaff.2010.0526.
42. Desai NR, Ross JS, Kwon JY, et al. Association between hospital penalty status under the hospital readmission reduction program and readmission rates for target and nontarget conditions. JAMA. 2016;316(24):2647-2656. https://doi.org/10.1001/jama.2016.18533.
© 2019 Society of Hospital Medicine
LOS in Children With Medical Complexity
Children with medical complexity (CMC) have complex and chronic health conditions that often involve multiple organ systems and severely affect cognitive and physical functioning. Although the prevalence of CMC is low (1% of all children), they account for nearly one‐fifth of all pediatric admissions and one‐half of all hospital days and charges in the United States.[1] Over the last decade, CMC have had a particularly large and increasing impact in tertiary‐care children's hospitals.[1, 2] The Institute of Medicine has identified CMC as a priority population for a revised healthcare system.[3]
Medical homes, hospitals, health plans, states, federal agencies, and others are striving to reduce excessive hospital use in CMC because of its high cost.[4, 5, 6] Containing length of stay (LOS)an increasingly used indicator of the time sensitiveness and efficiency of hospital careis a common aim across these initiatives. CMC have longer hospitalizations than children without medical complexity. Speculated reasons for this are that CMC tend to have (1) higher severity of acute illnesses (eg, pneumonia, cellulitis), (2) prolonged recovery time in the hospital, and (3) higher risk of adverse events in the hospital. Moreover, hospital clinicians caring for CMC often find it difficult to determine discharge readiness, given that many CMC do not return to a completely healthy baseline.[7]
Little is known about long LOS in CMC, including which CMC have the highest risk of experiencing such stays and which stays might have the greatest opportunity to be shortened. Patient characteristics associated with prolonged length of stay have been studied extensively for many pediatric conditions (eg, asthma).[8, 9, 10, 11, 12, 13, 14] However, most of these studies excluded CMC. Therefore, the objectives of this study were to examine (1) the prevalence of long LOS in CMC, (2) patient characteristics associated with long LOS, and (3) hospital‐to‐hospital variation in prevalence of long LOS hospitalizations.
METHODS
Study Design and Data Source
This study is a multicenter, retrospective cohort analysis of the Pediatric Health Information System (PHIS). PHIS is an administrative database of 44 not for profit, tertiary care pediatric hospitals affiliated with the Children's Hospital Association (CHA) (Overland Park, KS). PHIS contains data regarding patient demographics, diagnoses, and procedures (with International Classification of Diseases, 9th Revision, Clinical Modification [ICD‐9‐CM] codes), All‐Patient Refined Diagnostic Related Groups version 30 (APR‐DRGs) (3M Health Information Systems, Salt Lake City, UT), and service lines that aggregate the APR‐DRGs into 38 distinct groups. Data quality and reliability are assured through CHA and participating hospitals. In accordance with the policies of the Cincinnati Children's Hospital Medical Center Institutional Review Board, this study of deidentified data was not considered human subjects research.
Study Population
Inclusion Criteria
Children discharged following an observation or inpatient admission from a hospital participating in the PHIS database between January 1, 2013 and December 31, 2014 were eligible for inclusion if they were considered medically complex. Medical complexity was defined using Clinical Risk Groups (CRGs) version 1.8, developed by 3M Health Information Systems and the National Association of Children's Hospitals and Related Institutions. CRGs were used to assign each hospitalized patient to 1 of 9 mutually exclusive chronicity groups according to the presence, type, and severity of chronic conditions.[15, 16, 17, 18] Each patient's CRG designation was based on 2 years of previous hospital encounters.
As defined in prior studies and definitional frameworks of CMC,[1] patients belonging to CRG group 6 (significant chronic disease in 2 organ systems), CRG group 7 (dominant chronic disease in 3 organ systems), and CRG group 9 (catastrophic condition) were considered medically complex.[17, 19] Patients with malignancies (CRG group 8) were not included for analysis because they are a unique population with anticipated, long hospital stays. Patients with CRG group 5, representing those with chronic conditions affecting a single body system, were also not included because most do not have attributes consistent with medical complexity.
Exclusion Criteria
We used the APR‐DRG system, which leverages ICD‐9‐CM codes to identify the health problem most responsible for the hospitalization, to refine the study cohort. We excluded hospitalizations that were classified by the APR‐DRG system as neonatal, as we did not wish to focus on LOS in the neonatal intensive care unit (ICU) or for birth admissions. Similarly, hospitalizations for chemotherapy (APR‐DRG 693) or malignancy (identified with previously used ICD‐9‐CM codes)[20] were also excluded because long LOS is anticipated. We also excluded hospitalizations for medical rehabilitation (APR‐DRG 860).
Outcome Measures
The primary outcome measure was long LOS, defined as LOS 10 days. The cut point of LOS 10 days represents the 90th percentile of LOS for all children, with and without medical complexity, hospitalized during 2013 to 2014. LOS 10 days has previously been used as a threshold of long LOS.[21] For hospitalizations involving transfer at admission from another acute care facility, LOS was measured from the date of transfer. We also assessed hospitals' cost attributable to long LOS admissions.
Patient Demographics and Clinical Characteristics
We measured demographic characteristics including age, gender, race/ethnicity, insurance type, and distance traveled (the linear distance between the centroid of the patient's home ZIP code and the centroid of the hospital's ZIP code). Clinical characteristics included CRG classification, complex chronic condition (CCC), and dependence on medical technology. CCCs are defined as any medical condition that can be reasonably expected to last at least 12 months (unless death intervenes) and to involve either several different organ systems or 1 system severely enough to require specialty pediatric care and probably some period of hospitalization in a tertiary care center.[20] Medical technology included devices used to optimize the health and functioning of the child (eg, gastrostomy, tracheostomy, cerebrospinal fluid shunt).[22]
Hospitalization Characteristics
Characteristics of the hospitalization included transfer from an outside facility, ICU admission, surgical procedure (using surgical APR‐DRGs), and discharge disposition (home, skilled nursing facility, home health services, death, other). Cost of the hospitalization was estimated in the PHIS from charges using hospital and year‐specific ratios of cost to charge.
Statistical Analysis
Continuous data (eg, distance from hospital to home residence) were described with median and interquartile ranges (IQR) because they were not normally distributed. Categorical data (eg, type of chronic condition) were described with counts and frequencies. In bivariate analyses, demographic, clinical, and hospitalization characteristics were stratified by LOS (long LOS vs LOS <10 days), and compared using 2 statistics or Wilcoxon rank sum tests as appropriate.
We modeled the likelihood of experiencing a long LOS using generalized linear mixed effects models with a random hospital intercept and discharge‐level fixed effects for age, gender, payor, CCC type, ICU utilization, transfer status, a medical/surgical admission indicator derived from the APR‐DRG, and CRG assigned to each hospitalization. To examine hospital‐to‐hospital variability, we generated hospital risk‐adjusted rates of long LOS from these models. Similar models and hospital risk‐adjusted rates were built for a post hoc correlational analysis of 30‐day all cause readmission, where hospitals' rates and percent of long LOS were compared with a Pearson correlation coefficient. Also, for our multivariable models, we performed a sensitivity analysis using an alternative definition of long LOS as 4 days (the 75th percentile of LOS for all children, with and without medical complexity, hospitalized during 20132014). All analyses were performed using SAS version 9.4 (SAS Institute, Cary, NC), and P values <0.05 were considered statistically significant.
RESULTS
Study Population
There were 954,018 hospitalizations of 217,163 CMC at 44 children's hospitals included for analysis. Forty‐seven percent of hospitalizations were for females, 49.4% for non‐Hispanic whites, and 61.1% for children with government insurance. Fifteen percent (n = 142,082) had a long LOS of 10 days. The median (IQR) LOS of hospitalizations <10 days versus 10 days were 2 (IQR, 14) and 16 days (IQR, 1226), respectively. Long LOS hospitalizations accounted for 61.1% (3.7 million) hospital days and 61.8% ($13.7 billion) of total hospitalization costs for all CMC in the cohort (Table 1).
Characteristic | Overall (n = 954,018) | Length of Stay | |
---|---|---|---|
<10 Days (n = 811,936) | 10 Days (n = 142,082) | ||
| |||
Age at admission, y, % | |||
<1 | 14.6 | 12.7 | 25.7 |
14 | 27.1 | 27.9 | 22.4 |
59 | 20.1 | 21.0 | 14.9 |
1018 | 33.6 | 34.0 | 31.7 |
18+ | 4.6 | 4.4 | 5.4 |
Gender, % | |||
Female | 47.0 | 46.9 | 47.5 |
Race/ethnicity, % | |||
Non‐Hispanic white | 49.4 | 49.4 | 49.4 |
Non‐Hispanic black | 23.1 | 23.8 | 19.3 |
Hispanic | 18.2 | 17.8 | 20.4 |
Asian | 2.0 | 1.9 | 2.3 |
Other | 7.4 | 7.1 | 8.6 |
Complex chronic condition, % | |||
Any | 79.5 | 77.3 | 91.8 |
Technology assistance | 37.1 | 34.1 | 54.2 |
Gastrointestinal | 30.0 | 27.2 | 45.9 |
Neuromuscular | 28.2 | 27.7 | 30.9 |
Cardiovascular | 16.8 | 14.5 | 29.9 |
Respiratory | 14.1 | 11.5 | 29.4 |
Congenital/genetic defect | 17.2 | 16.7 | 20.2 |
Metabolic | 9.9 | 8.9 | 15.4 |
Renal | 10.1 | 9.5 | 13.8 |
Hematology/emmmunodeficiency | 11.7 | 12.0 | 10.0 |
Neonatal | 3.8 | 3.1 | 7.7 |
Transplantation | 4.5 | 4.2 | 6.7 |
Clinical risk group, % | |||
Chronic condition in 2 systems | 68.4 | 71.2 | 53.9 |
Catastrophic chronic condition | 31.4 | 28.8 | 46.1 |
Distance from hospital to home residence in miles, median [IQR] | 16.2 [7.440.4] | 15.8 [7.338.7] | 19.1 [8.552.6] |
Transferred from outside hospital (%) | 6.5 | 5.3 | 13.6 |
Admitted for surgery, % | 23.4 | 20.7 | 38.7 |
Use of intensive care, % | 19.6 | 14.9 | 46.5 |
Discharge disposition, % | |||
Home | 91.2 | 92.9 | 81.4 |
Home healthcare | 4.5 | 3.5 | 9.9 |
Other | 2.9 | 2.6 | 4.5 |
Postacute care facility | 1.1 | 0.8 | 3.1 |
Died | 0.4 | 0.3 | 1.1 |
Payor, % | |||
Government | 61.1 | 60.6 | 63.5 |
Private | 33.2 | 33.6 | 30.9 |
Other | 5.7 | 5.7 | 5.7 |
Hospital resource use | |||
Median length of stay [IQR] | 3 [16] | 2 [14] | 16 [1226] |
Median hospital cost [IQR] | $8,144 [$4,122$18,447] | $6,689 [$3,685$12,395] | $49,207 [$29,444$95,738] |
Total hospital cost, $, billions | $22.2 | $8.5 | $13.7 |
Demographics and Clinical Characteristics of Children With and Without Long LOS
Compared with hospitalized CMC with LOS <10 days, a higher percentage of hospitalizations with LOS 10 days were CMC age <1 year (25.7% vs 12.7%, P < 0.001) and Hispanic (20.4% vs 17.8%, P < 0.001). CMC hospitalizations with a long LOS had a higher percentage of any CCC (91.8% vs 77.3%, P < 0.001); the most common CCCs were gastrointestinal (45.9%), neuromuscular (30.9%), and cardiovascular (29.9%). Hospitalizations of CMC with a long LOS had a higher percentage of a catastrophic chronic condition (46.1% vs 28.8%, P < 0.001) and technology dependence (46.1% vs 28.8%, P < 0.001) (Table 1).
Hospitalization Characteristics of Children With and Without Long LOS
Compared with hospitalizations of CMC with LOS <10 days, hospitalizations of CMC with a long LOS more often involved transfer in from another hospital at admission (13.6% vs 5.3%, P < 0.001). CMC hospital stays with a long LOS more often involved surgery (38.7% vs 20.7%, P < 0.001) and use of intensive care (46.5% vs 14.9%; P < 0.001). A higher percentage of CMC with long LOS were discharged with home health services (9.9% vs 3.5%; P < 0.001) (Table 1).
The most common admitting diagnoses and CCCs for hospitalizations of CMC with long LOS are presented in Table 2. The two most prevalent APR‐DRGs in CMC hospitalizations lasting 10 days or longer were cystic fibrosis (10.7%) and respiratory system disease with ventilator support (5.5%). The two most common chronic condition characteristics represented among long CMC hospitalizations were gastrointestinal devices (eg, gastrostomy tube) (39.7%) and heart and great vessel malformations (eg, tetralogy of Fallot) (12.8%). The 5 most common CCC subcategories, as listed in Table 2, account for nearly 100% of the patients with long LOS hospitalizations.
| |
Most common reason for admission* | |
Cystic fibrosis | 10.7% |
Respiratory system diagnosis with ventilator support 96+ hours | 5.5% |
Malfunction, reaction, and complication of cardiac or vascular device or procedure | 2.8% |
Craniotomy except for trauma | 2.6% |
Major small and large bowel procedures | 2.3% |
Most common complex chronic condition | |
Gastrointestinal devices | 39.7% |
Heart and great vessel malformations | 12.8% |
Cystic fibrosis | 12.5% |
Dysrhythmias | 11.0% |
Respiratory devices | 10.7% |
Multivariable Analysis of Characteristics Associated With Long LOS
In multivariable analysis, the highest likelihood of long LOS was experienced by children who received care in the ICU (odds ratio [OR]: 3.5, 95% confidence interval [CI]: 3.43.5), who had a respiratory CCC (OR: 2.7, 95% CI: 2.62.7), and who were transferred from another acute care hospital at admission (OR: 2.1, 95% CI: 2.0, 2.1). The likelihood of long LOS was also higher in children <1 year of age (OR: 1.2, 95% CI: 1.21.3), and Hispanic children (OR: 1.1, 95% CI 1.0‐1.10) (Table 3). Similar multivariable findings were observed in sensitivity analysis using the 75th percentile of LOS (4 days) as the model outcome.
Characteristic | Odds Ratio (95% CI) of LOS 10 Days | P Value |
---|---|---|
| ||
Use of intensive care | 3.5 (3.4‐3.5) | <0.001 |
Transfer from another acute‐care hospital | 2.1 (2.0‐2.1) | <0.001 |
Procedure/surgery | 1.8 (1.8‐1.9) | <0.001 |
Complex chronic condition | ||
Respiratory | 2.7 (2.6‐2.7) | <0.001 |
Gastrointestinal | 1.8 (1.8‐1.8) | <0.001 |
Metabolic | 1.7 (1.7‐1.7) | <0.001 |
Cardiovascular | 1.6 (1.5‐1.6) | <0.001 |
Neonatal | 1.5 (1.5‐1.5) | <0.001 |
Renal | 1.4 (1.4‐1.4) | <0.001 |
Transplant | 1.4 (1.4‐1.4) | <0.001 |
Hematology and immunodeficiency | 1.3 (1.3‐1.3) | <0.001 |
Technology assistance | 1.1 (1.1, 1.1) | <0.001 |
Neuromuscular | 0.9 (0.9‐0.9) | <0.001 |
Congenital or genetic defect | 0.8 (0.8‐0.8) | <0.001 |
Age at admission, y | ||
<1 | 1.2 (1.2‐1.3) | <0.001 |
14 | 0.5 (0.5‐0.5) | <0.001 |
59 | 0.6 (0.6‐0.6) | <0.001 |
1018 | 0.9 (0.9‐0.9) | <0.001 |
18+ | Reference | |
Male | 0.9 (0.9‐0.9) | <0.001 |
Race/ethnicity | ||
Non‐Hispanic black | 0.9 (0.9‐0.9) | <0.001 |
Hispanic | 1.1 (1.0‐1.1) | <0.001 |
Asian | 1.0 (1.0‐1.1) | 0.3 |
Other | 1.1 (1.1‐1.1) | <0.001 |
Non‐Hispanic white | Reference | |
Payor | ||
Private | 0.9 (0.8 0.9) | <0.001 |
Other | 1.0 (1.0‐1.0) | 0.4 |
Government | Reference | |
Season | ||
Spring | 1.0 (1.0 1.0) | <0.001 |
Summer | 0.9 (0.9‐0.9) | <0.001 |
Fall | 1.0 (0.9‐1.0) | <0.001 |
Winter | Reference |
Variation in the Prevalence of Long LOS Across Children's Hospitals
After controlling for demographic, clinical, and hospital characteristics associated with long LOS, there was significant (P < 0.001) variation in the prevalence of long LOS for CMC across children's hospitals in the cohort (range, 10.3%21.8%) (Figure 1). Twelve (27%) hospitals had a significantly (P < 0.001) higher prevalence of long LOS for their hospitalized CMC, compared to the mean. Eighteen (41%) had a significantly (P < 0.001) lower prevalence of long LOS for their hospitalized CMC. There was also significant variation across hospitals with respect to cost, with 49.7% to 73.7% of all hospital costs of CMC attributed to long LOS hospitalizations. Finally, there was indirect correlation with the prevalence of LOS across hospitals and the hospitals' 30‐day readmission rate ( = 0.3; P = 0.04). As the prevalence of long LOS increased, the readmission rate decreased.
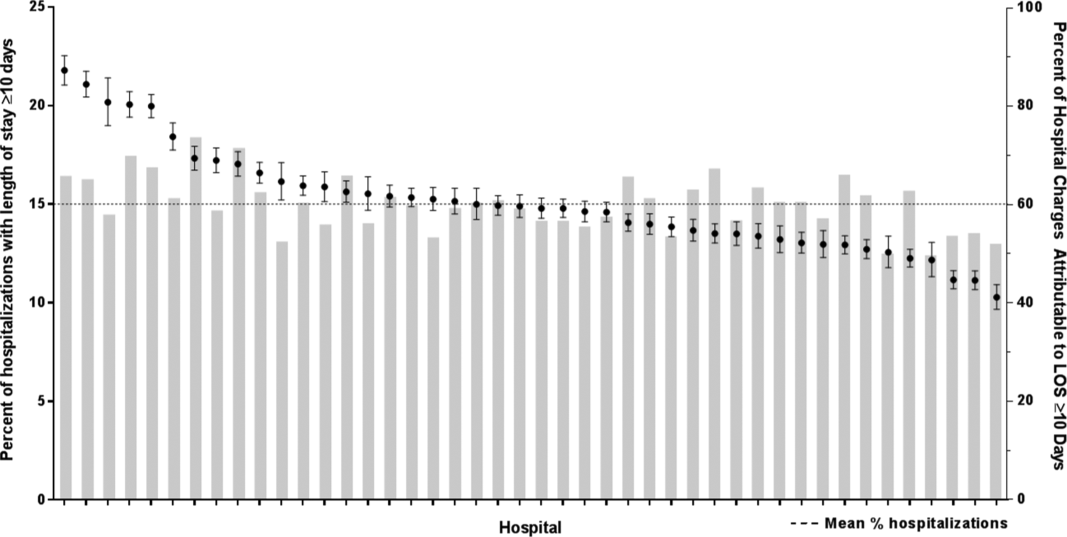
DISCUSSION
The main findings from this study suggest that a small percentage of CMC experiencing long LOS account for the majority of hospital bed days and cost of all hospitalized CMC in children's hospitals. The likelihood of long LOS varies significantly by CMC's age, race/ethnicity, and payor as well as by type and number of chronic conditions. Among CMC with long LOS, the use of gastrointestinal devices such as gastrostomy tubes, as well as congenital heart disease, were highly prevalent. In multivariable analysis, the characteristics most strongly associated with LOS 10 days were use of the ICU, respiratory complex chronic condition, and transfer from another medical facility at admission. After adjusting for these factors, there was significant variation in the prevalence of LOS 10 days for CMC across children's hospitals.
Although it is well known that CMC as a whole have a major impact on resource use in children's hospitals, this study reveals that 15% of hospitalizations of CMC account for 62% of all hospital costs of CMC. That is, a small fraction of hospitalizations of CMC is largely responsible for the significant financial impact of hospital resource use. To date, most clinical efforts and policies striving to reduce hospital use in CMC have focused on avoiding readmissions or index hospital admissions entirely, rather than improving the efficiency of hospital care after admission occurs.[23, 24, 25, 26] In the adult population, the impact of long LOS on hospital costs has been recognized, and several Medicare incentive programs have focused on in‐hospital timeliness and efficiency. As a result, LOS in Medicare beneficiaries has decreased dramatically over the past 2 decades.[27, 28, 29, 30] Optimizing the efficiency of hospital care for CMC may be an important goal to pursue, especially with precedent set in the adult literature.
Perhaps the substantial variation across hospitals in the prevalence of long LOS in CMC indicates opportunity to improve the efficiency of their inpatient care. This variation was not due to differences across hospitals' case mix of CMC. Further investigation is needed to determine how much of it is due to differences in quality of care. Clinical practice guidelines for hospital treatment of common illnesses usually exclude CMC. In our clinical experience across 9 children's hospitals, we have experienced varying approaches to setting discharge goals (ie, parameters on how healthy the child needs to be to ensure a successful hospital discharge) for CMC.[31] When the goals are absent or not clearly articulated, they can contribute to a prolonged hospitalization. Some families of CMC report significant issues when working with pediatric hospital staff to assess their child's discharge readiness.[7, 32, 33] In addition, there is significant variation across states and regions in access to and quality of post‐discharge health services (eg, home nursing, postacute care, durable medical equipment).[34, 35] In some areas, many CMC are not actively involved with their primary care physician.[5] These issues might also influence the ability of some children's hospitals to efficiently discharge CMC to a safe and supportive post‐discharge environment. Further examination of hospital outliersthose with the lowest and highest percentage of CMC hospitalizations with long LOSmay reveal opportunities to identify and spread best practices.
The demographic and clinical factors associated with long LOS in the present study, including age, ICU use, and transfer from another hospital, might help hospitals target which CMC have the greatest risk for experiencing long LOS. We found that infants age <1 year had longer LOS when compared with older children. Similar to our findings, younger‐aged children hospitalized with bronchiolitis have longer LOS.[36] Certainly, infants with medical complexity, in general, are a high‐acuity population with the potential for rapid clinical deterioration during an acute illness. Prolonged hospitalization for treatment and stabilization may be expected for many of them. Additional investigation is warranted to examine ICU use in CMC, and whether ICU admission or duration can be safely prevented or abbreviated. Opportunities to assess the quality of transfers into children's hospitals of CMC admitted to outside hospitals may be necessary. A study of pediatric burn patients reported that patients initially stabilized at a facility that was not a burn center and subsequently transferred to a burn center had a longer LOS than patients solely treated at a designated burn center.[37] Furthermore, events during transport itself may adversely impact the stability of an already fragile patient. Interventions to optimize the quality of care provided by transport teams have resulted in decreased LOS at the receiving hospital.[38]
This study's findings should be considered in the context of several limitations. Absent a gold‐standard definition of long LOS, we used the distribution of LOS across patients to inform our methods; LOS at the 90th percentile was selected as long. Although our sensitivity analysis using LOS at the 75th percentile produced similar findings, other cut points in LOS might be associated with different results. The study is not positioned to determine how much of the reported LOS was excessive, unnecessary, or preventable. The study findings may not generalize to types of hospitals not contained in PHIS (eg, nonchildren's hospitals and community hospitals). We did not focus on the impact of a new diagnosis (eg, new chronic illness) or acute in‐hospital event (eg, nosocomial infection) on prolonged LOS; future studies should investigate these clinical events with LOS.
PHIS does not contain information regarding characteristics that could influence LOS, including the children's social and familial attributes, transportation availability, home equipment needs, and local availability of postacute care facilities. Moreover, PHIS does not contain information about the hospital discharge procedures, process, or personnel across hospitals, which could influence LOS. Future studies on prolonged LOS should consider assessing this information. Because of the large sample size of hospitalizations included, the statistical power for the analyses was strong, rendering it possible that some findings that were statistically significant might have modest clinical significance (eg, relationship of Hispanic ethnicity with prolonged LOS). We could not determine why a positive correlation was not observed between hospitals' long LOS prevalence and their percentage of cost associated with long LOS; future studies should investigate the reasons for this finding.
Despite these limitations, the findings of the present study highlight the significance of long LOS in hospitalized CMC. These long hospitalizations account for a significant proportion of all hospital costs for this important population of children. The prevalence of long LOS for CMC varies considerably across children's hospitals, even after accounting for the case mix. Efforts to curtail hospital resource use and costs for CMC may benefit from focus on long LOS.
- Inpatient growth and resource use in 28 children's hospitals: a longitudinal, multi‐institutional study. JAMA Pediatr. 2013;167(2):170–177. , , , et al.
- Children with complex chronic conditions in inpatient hospital settings in the united states. Pediatrics. 2010;126(4):647–655. , , , et al.
- Meeting the health care needs of persons with disabilities. Milbank Q. 2002;80(2):381–391. , .
- Effect of an enhanced medical home on serious illness and cost of care among high‐risk children with chronic illness: a randomized clinical trial. JAMA. 2014;312(24):2640–2648. , , , et al.
- Children with medical complexity and Medicaid: spending and cost savings. Health Aff Proj Hope. 2014;33(12):2199–2206. , , , et al.
- Children's Hospital Association. CARE Award. Available at: https://www.childrenshospitals.org/Programs‐and‐Services/Quality‐Improvement‐and‐Measurement/CARE‐Award. Accessed December 18, 2015.
- Hospital readmission and parent perceptions of their child's hospital discharge. Int J Qual Health Care. 2013;25(5):573–581. , , , et al.
- Weekend matters: Friday and Saturday admissions are associated with prolonged hospitalization of children. Clin Pediatr (Phila). 2013;52(9):875–878. , , , et al.
- Attributable cost and length of stay for central line‐associated bloodstream infections. Pediatrics. 2014;133(6):e1525–e1532. , , , .
- Effect of healthcare‐acquired infection on length of hospital stay and cost. Infect Control Hosp Epidemiol. 2007;28(3):280–292. , , , et al.
- Hospital utilization and costs among children with influenza, 2003. Am J Prev Med. 2009;36(4):292–296. , , , , .
- Charges and lengths of stay attributable to adverse patient‐care events using pediatric‐specific quality indicators: a multicenter study of freestanding children's hospitals. Pediatrics. 2008;121(6):e1653–e1659. , , , .
- Variation in resource utilization for the management of uncomplicated community‐acquired pneumonia across community and children's hospitals. J Pediatr. 2014;165(3):585–591. , , , , .
- Variation and outcomes associated with direct hospital admission among children with pneumonia in the United States. JAMA Pediatr. 2014;168(9):829–836. , , , , .
- Clinical Risk Groups (CRGs): a classification system for risk‐adjusted capitation‐based payment and health care management. Med Care. 2004;42(1):81–90. , , , et al.
- Identifying children with lifelong chronic conditions for care coordination by using hospital discharge data. Acad Pediatr. 2010;10(6):417–423. , , , et al.
- Profile of medical charges for children by health status group and severity level in a Washington State Health Plan. Health Serv Res. 2004;39(1):73–89. , , , , .
- Using medical billing data to evaluate chronically ill children over time. J Ambulatory Care Manage. 2006;29(4):283–290. , , , .
- Medical complexity and pediatric emergency department and inpatient utilization. Pediatrics. 2013;131(2):e559–e565. , , , , , .
- Pediatric complex chronic conditions classification system version 2: updated for ICD‐10 and complex medical technology dependence and transplantation. BMC Pediatr. 2014;14:199. , , , , .
- Analyzing intensive care unit length of stay data: problems and possible solutions. Crit Care Med. 1997;25(9):1594–1600. .
- Hospital utilization and characteristics of patients experiencing recurrent readmissions within children's hospitals. JAMA. 2011;305(7):682–690. , , , et al.
- Hospital readmissions and repeat emergency department visits among children with medical complexity: an integrative review. J Pediatr Nurs. 2013;28(4):316–339. .
- Hospital readmission in children with complex chronic conditions discharged from subacute care. Hosp Pediatr. 2014;4(3):153–158. , , , .
- Preventing hospitalizations in children with medical complexity: a systematic review. Pediatrics. 2014;134(6):e1628–e1647. , , , et al.
- Hospital readmissions for newly discharged pediatric home mechanical ventilation patients. Pediatr Pulmonol. 2012;47(4):409–414. , , , .
- Clinical characteristics and outcomes of Medicare patients undergoing total hip arthroplasty, 1991–2008. JAMA. 2011;305(15):1560–1567. , , , et al.
- Trends in length of stay and short‐term outcomes among Medicare patients hospitalized for heart failure, 1993–2006. JAMA. 2010;303(21):2141–2147. , , , et al.
- U.S. Department of Health and Human Services. CMS Statistics 2013. Available at: https://www.cms.gov/Research‐Statistics‐Data‐and‐Systems/Statistics‐Trends‐and‐Reports/CMS‐Statistics‐Reference‐Booklet/Downloads/CMS_Stats_2013_final.pdf. Published August 2013. Accessed October 6, 2015.
- Centers for Medicare and Medicaid Services. Evaluation of the premier hospital quality incentive demonstration. Available at: https://www.cms.gov/Research‐Statistics‐Data‐and‐Systems/Statistics‐Trends‐and‐Reports/Reports/downloads/Premier_ExecSum_2010.pdf. Published March 3, 2009. Accessed September 18, 2015.
- A framework of pediatric hospital discharge care informed by legislation, research, and practice. JAMA Pediatr. 2014;168(10):955–962; quiz 965–966. , , , et al.
- Parent and provider perspectives on pediatric readmissions: what can we learn about readiness for discharge? Hosp Pediatr. 2015;5(11):559–565. , , , , .
- Preventing readmissions in children: how do we do that? Hosp Pediatr. 2015;5(11):602–604. , .
- Pediatric post‐acute hospital care: striving for identity and value. Hosp Pediatr. 2015;5(10):548–551. , , .
- Pediatric hospital discharges to home health and postacute facility care: a national study. JAMA Pediatr. 2016;170(4):326–333. , , , et al.
- Bronchiolitis: clinical characteristics associated with hospitalization and length of stay. Pediatr Emerg Care. 2012;28(2):99–103. , , , et al.
- The effect of transfers between health care facilities on costs and length of stay for pediatric burn patients. J Burn Care Res. 2015;36(1):178–183. , , , , .
- Goal‐directed resuscitative interventions during pediatric interfacility transport. Crit Care Med. 2015;43(8):1692–1698. , , , et al.
Children with medical complexity (CMC) have complex and chronic health conditions that often involve multiple organ systems and severely affect cognitive and physical functioning. Although the prevalence of CMC is low (1% of all children), they account for nearly one‐fifth of all pediatric admissions and one‐half of all hospital days and charges in the United States.[1] Over the last decade, CMC have had a particularly large and increasing impact in tertiary‐care children's hospitals.[1, 2] The Institute of Medicine has identified CMC as a priority population for a revised healthcare system.[3]
Medical homes, hospitals, health plans, states, federal agencies, and others are striving to reduce excessive hospital use in CMC because of its high cost.[4, 5, 6] Containing length of stay (LOS)an increasingly used indicator of the time sensitiveness and efficiency of hospital careis a common aim across these initiatives. CMC have longer hospitalizations than children without medical complexity. Speculated reasons for this are that CMC tend to have (1) higher severity of acute illnesses (eg, pneumonia, cellulitis), (2) prolonged recovery time in the hospital, and (3) higher risk of adverse events in the hospital. Moreover, hospital clinicians caring for CMC often find it difficult to determine discharge readiness, given that many CMC do not return to a completely healthy baseline.[7]
Little is known about long LOS in CMC, including which CMC have the highest risk of experiencing such stays and which stays might have the greatest opportunity to be shortened. Patient characteristics associated with prolonged length of stay have been studied extensively for many pediatric conditions (eg, asthma).[8, 9, 10, 11, 12, 13, 14] However, most of these studies excluded CMC. Therefore, the objectives of this study were to examine (1) the prevalence of long LOS in CMC, (2) patient characteristics associated with long LOS, and (3) hospital‐to‐hospital variation in prevalence of long LOS hospitalizations.
METHODS
Study Design and Data Source
This study is a multicenter, retrospective cohort analysis of the Pediatric Health Information System (PHIS). PHIS is an administrative database of 44 not for profit, tertiary care pediatric hospitals affiliated with the Children's Hospital Association (CHA) (Overland Park, KS). PHIS contains data regarding patient demographics, diagnoses, and procedures (with International Classification of Diseases, 9th Revision, Clinical Modification [ICD‐9‐CM] codes), All‐Patient Refined Diagnostic Related Groups version 30 (APR‐DRGs) (3M Health Information Systems, Salt Lake City, UT), and service lines that aggregate the APR‐DRGs into 38 distinct groups. Data quality and reliability are assured through CHA and participating hospitals. In accordance with the policies of the Cincinnati Children's Hospital Medical Center Institutional Review Board, this study of deidentified data was not considered human subjects research.
Study Population
Inclusion Criteria
Children discharged following an observation or inpatient admission from a hospital participating in the PHIS database between January 1, 2013 and December 31, 2014 were eligible for inclusion if they were considered medically complex. Medical complexity was defined using Clinical Risk Groups (CRGs) version 1.8, developed by 3M Health Information Systems and the National Association of Children's Hospitals and Related Institutions. CRGs were used to assign each hospitalized patient to 1 of 9 mutually exclusive chronicity groups according to the presence, type, and severity of chronic conditions.[15, 16, 17, 18] Each patient's CRG designation was based on 2 years of previous hospital encounters.
As defined in prior studies and definitional frameworks of CMC,[1] patients belonging to CRG group 6 (significant chronic disease in 2 organ systems), CRG group 7 (dominant chronic disease in 3 organ systems), and CRG group 9 (catastrophic condition) were considered medically complex.[17, 19] Patients with malignancies (CRG group 8) were not included for analysis because they are a unique population with anticipated, long hospital stays. Patients with CRG group 5, representing those with chronic conditions affecting a single body system, were also not included because most do not have attributes consistent with medical complexity.
Exclusion Criteria
We used the APR‐DRG system, which leverages ICD‐9‐CM codes to identify the health problem most responsible for the hospitalization, to refine the study cohort. We excluded hospitalizations that were classified by the APR‐DRG system as neonatal, as we did not wish to focus on LOS in the neonatal intensive care unit (ICU) or for birth admissions. Similarly, hospitalizations for chemotherapy (APR‐DRG 693) or malignancy (identified with previously used ICD‐9‐CM codes)[20] were also excluded because long LOS is anticipated. We also excluded hospitalizations for medical rehabilitation (APR‐DRG 860).
Outcome Measures
The primary outcome measure was long LOS, defined as LOS 10 days. The cut point of LOS 10 days represents the 90th percentile of LOS for all children, with and without medical complexity, hospitalized during 2013 to 2014. LOS 10 days has previously been used as a threshold of long LOS.[21] For hospitalizations involving transfer at admission from another acute care facility, LOS was measured from the date of transfer. We also assessed hospitals' cost attributable to long LOS admissions.
Patient Demographics and Clinical Characteristics
We measured demographic characteristics including age, gender, race/ethnicity, insurance type, and distance traveled (the linear distance between the centroid of the patient's home ZIP code and the centroid of the hospital's ZIP code). Clinical characteristics included CRG classification, complex chronic condition (CCC), and dependence on medical technology. CCCs are defined as any medical condition that can be reasonably expected to last at least 12 months (unless death intervenes) and to involve either several different organ systems or 1 system severely enough to require specialty pediatric care and probably some period of hospitalization in a tertiary care center.[20] Medical technology included devices used to optimize the health and functioning of the child (eg, gastrostomy, tracheostomy, cerebrospinal fluid shunt).[22]
Hospitalization Characteristics
Characteristics of the hospitalization included transfer from an outside facility, ICU admission, surgical procedure (using surgical APR‐DRGs), and discharge disposition (home, skilled nursing facility, home health services, death, other). Cost of the hospitalization was estimated in the PHIS from charges using hospital and year‐specific ratios of cost to charge.
Statistical Analysis
Continuous data (eg, distance from hospital to home residence) were described with median and interquartile ranges (IQR) because they were not normally distributed. Categorical data (eg, type of chronic condition) were described with counts and frequencies. In bivariate analyses, demographic, clinical, and hospitalization characteristics were stratified by LOS (long LOS vs LOS <10 days), and compared using 2 statistics or Wilcoxon rank sum tests as appropriate.
We modeled the likelihood of experiencing a long LOS using generalized linear mixed effects models with a random hospital intercept and discharge‐level fixed effects for age, gender, payor, CCC type, ICU utilization, transfer status, a medical/surgical admission indicator derived from the APR‐DRG, and CRG assigned to each hospitalization. To examine hospital‐to‐hospital variability, we generated hospital risk‐adjusted rates of long LOS from these models. Similar models and hospital risk‐adjusted rates were built for a post hoc correlational analysis of 30‐day all cause readmission, where hospitals' rates and percent of long LOS were compared with a Pearson correlation coefficient. Also, for our multivariable models, we performed a sensitivity analysis using an alternative definition of long LOS as 4 days (the 75th percentile of LOS for all children, with and without medical complexity, hospitalized during 20132014). All analyses were performed using SAS version 9.4 (SAS Institute, Cary, NC), and P values <0.05 were considered statistically significant.
RESULTS
Study Population
There were 954,018 hospitalizations of 217,163 CMC at 44 children's hospitals included for analysis. Forty‐seven percent of hospitalizations were for females, 49.4% for non‐Hispanic whites, and 61.1% for children with government insurance. Fifteen percent (n = 142,082) had a long LOS of 10 days. The median (IQR) LOS of hospitalizations <10 days versus 10 days were 2 (IQR, 14) and 16 days (IQR, 1226), respectively. Long LOS hospitalizations accounted for 61.1% (3.7 million) hospital days and 61.8% ($13.7 billion) of total hospitalization costs for all CMC in the cohort (Table 1).
Characteristic | Overall (n = 954,018) | Length of Stay | |
---|---|---|---|
<10 Days (n = 811,936) | 10 Days (n = 142,082) | ||
| |||
Age at admission, y, % | |||
<1 | 14.6 | 12.7 | 25.7 |
14 | 27.1 | 27.9 | 22.4 |
59 | 20.1 | 21.0 | 14.9 |
1018 | 33.6 | 34.0 | 31.7 |
18+ | 4.6 | 4.4 | 5.4 |
Gender, % | |||
Female | 47.0 | 46.9 | 47.5 |
Race/ethnicity, % | |||
Non‐Hispanic white | 49.4 | 49.4 | 49.4 |
Non‐Hispanic black | 23.1 | 23.8 | 19.3 |
Hispanic | 18.2 | 17.8 | 20.4 |
Asian | 2.0 | 1.9 | 2.3 |
Other | 7.4 | 7.1 | 8.6 |
Complex chronic condition, % | |||
Any | 79.5 | 77.3 | 91.8 |
Technology assistance | 37.1 | 34.1 | 54.2 |
Gastrointestinal | 30.0 | 27.2 | 45.9 |
Neuromuscular | 28.2 | 27.7 | 30.9 |
Cardiovascular | 16.8 | 14.5 | 29.9 |
Respiratory | 14.1 | 11.5 | 29.4 |
Congenital/genetic defect | 17.2 | 16.7 | 20.2 |
Metabolic | 9.9 | 8.9 | 15.4 |
Renal | 10.1 | 9.5 | 13.8 |
Hematology/emmmunodeficiency | 11.7 | 12.0 | 10.0 |
Neonatal | 3.8 | 3.1 | 7.7 |
Transplantation | 4.5 | 4.2 | 6.7 |
Clinical risk group, % | |||
Chronic condition in 2 systems | 68.4 | 71.2 | 53.9 |
Catastrophic chronic condition | 31.4 | 28.8 | 46.1 |
Distance from hospital to home residence in miles, median [IQR] | 16.2 [7.440.4] | 15.8 [7.338.7] | 19.1 [8.552.6] |
Transferred from outside hospital (%) | 6.5 | 5.3 | 13.6 |
Admitted for surgery, % | 23.4 | 20.7 | 38.7 |
Use of intensive care, % | 19.6 | 14.9 | 46.5 |
Discharge disposition, % | |||
Home | 91.2 | 92.9 | 81.4 |
Home healthcare | 4.5 | 3.5 | 9.9 |
Other | 2.9 | 2.6 | 4.5 |
Postacute care facility | 1.1 | 0.8 | 3.1 |
Died | 0.4 | 0.3 | 1.1 |
Payor, % | |||
Government | 61.1 | 60.6 | 63.5 |
Private | 33.2 | 33.6 | 30.9 |
Other | 5.7 | 5.7 | 5.7 |
Hospital resource use | |||
Median length of stay [IQR] | 3 [16] | 2 [14] | 16 [1226] |
Median hospital cost [IQR] | $8,144 [$4,122$18,447] | $6,689 [$3,685$12,395] | $49,207 [$29,444$95,738] |
Total hospital cost, $, billions | $22.2 | $8.5 | $13.7 |
Demographics and Clinical Characteristics of Children With and Without Long LOS
Compared with hospitalized CMC with LOS <10 days, a higher percentage of hospitalizations with LOS 10 days were CMC age <1 year (25.7% vs 12.7%, P < 0.001) and Hispanic (20.4% vs 17.8%, P < 0.001). CMC hospitalizations with a long LOS had a higher percentage of any CCC (91.8% vs 77.3%, P < 0.001); the most common CCCs were gastrointestinal (45.9%), neuromuscular (30.9%), and cardiovascular (29.9%). Hospitalizations of CMC with a long LOS had a higher percentage of a catastrophic chronic condition (46.1% vs 28.8%, P < 0.001) and technology dependence (46.1% vs 28.8%, P < 0.001) (Table 1).
Hospitalization Characteristics of Children With and Without Long LOS
Compared with hospitalizations of CMC with LOS <10 days, hospitalizations of CMC with a long LOS more often involved transfer in from another hospital at admission (13.6% vs 5.3%, P < 0.001). CMC hospital stays with a long LOS more often involved surgery (38.7% vs 20.7%, P < 0.001) and use of intensive care (46.5% vs 14.9%; P < 0.001). A higher percentage of CMC with long LOS were discharged with home health services (9.9% vs 3.5%; P < 0.001) (Table 1).
The most common admitting diagnoses and CCCs for hospitalizations of CMC with long LOS are presented in Table 2. The two most prevalent APR‐DRGs in CMC hospitalizations lasting 10 days or longer were cystic fibrosis (10.7%) and respiratory system disease with ventilator support (5.5%). The two most common chronic condition characteristics represented among long CMC hospitalizations were gastrointestinal devices (eg, gastrostomy tube) (39.7%) and heart and great vessel malformations (eg, tetralogy of Fallot) (12.8%). The 5 most common CCC subcategories, as listed in Table 2, account for nearly 100% of the patients with long LOS hospitalizations.
| |
Most common reason for admission* | |
Cystic fibrosis | 10.7% |
Respiratory system diagnosis with ventilator support 96+ hours | 5.5% |
Malfunction, reaction, and complication of cardiac or vascular device or procedure | 2.8% |
Craniotomy except for trauma | 2.6% |
Major small and large bowel procedures | 2.3% |
Most common complex chronic condition | |
Gastrointestinal devices | 39.7% |
Heart and great vessel malformations | 12.8% |
Cystic fibrosis | 12.5% |
Dysrhythmias | 11.0% |
Respiratory devices | 10.7% |
Multivariable Analysis of Characteristics Associated With Long LOS
In multivariable analysis, the highest likelihood of long LOS was experienced by children who received care in the ICU (odds ratio [OR]: 3.5, 95% confidence interval [CI]: 3.43.5), who had a respiratory CCC (OR: 2.7, 95% CI: 2.62.7), and who were transferred from another acute care hospital at admission (OR: 2.1, 95% CI: 2.0, 2.1). The likelihood of long LOS was also higher in children <1 year of age (OR: 1.2, 95% CI: 1.21.3), and Hispanic children (OR: 1.1, 95% CI 1.0‐1.10) (Table 3). Similar multivariable findings were observed in sensitivity analysis using the 75th percentile of LOS (4 days) as the model outcome.
Characteristic | Odds Ratio (95% CI) of LOS 10 Days | P Value |
---|---|---|
| ||
Use of intensive care | 3.5 (3.4‐3.5) | <0.001 |
Transfer from another acute‐care hospital | 2.1 (2.0‐2.1) | <0.001 |
Procedure/surgery | 1.8 (1.8‐1.9) | <0.001 |
Complex chronic condition | ||
Respiratory | 2.7 (2.6‐2.7) | <0.001 |
Gastrointestinal | 1.8 (1.8‐1.8) | <0.001 |
Metabolic | 1.7 (1.7‐1.7) | <0.001 |
Cardiovascular | 1.6 (1.5‐1.6) | <0.001 |
Neonatal | 1.5 (1.5‐1.5) | <0.001 |
Renal | 1.4 (1.4‐1.4) | <0.001 |
Transplant | 1.4 (1.4‐1.4) | <0.001 |
Hematology and immunodeficiency | 1.3 (1.3‐1.3) | <0.001 |
Technology assistance | 1.1 (1.1, 1.1) | <0.001 |
Neuromuscular | 0.9 (0.9‐0.9) | <0.001 |
Congenital or genetic defect | 0.8 (0.8‐0.8) | <0.001 |
Age at admission, y | ||
<1 | 1.2 (1.2‐1.3) | <0.001 |
14 | 0.5 (0.5‐0.5) | <0.001 |
59 | 0.6 (0.6‐0.6) | <0.001 |
1018 | 0.9 (0.9‐0.9) | <0.001 |
18+ | Reference | |
Male | 0.9 (0.9‐0.9) | <0.001 |
Race/ethnicity | ||
Non‐Hispanic black | 0.9 (0.9‐0.9) | <0.001 |
Hispanic | 1.1 (1.0‐1.1) | <0.001 |
Asian | 1.0 (1.0‐1.1) | 0.3 |
Other | 1.1 (1.1‐1.1) | <0.001 |
Non‐Hispanic white | Reference | |
Payor | ||
Private | 0.9 (0.8 0.9) | <0.001 |
Other | 1.0 (1.0‐1.0) | 0.4 |
Government | Reference | |
Season | ||
Spring | 1.0 (1.0 1.0) | <0.001 |
Summer | 0.9 (0.9‐0.9) | <0.001 |
Fall | 1.0 (0.9‐1.0) | <0.001 |
Winter | Reference |
Variation in the Prevalence of Long LOS Across Children's Hospitals
After controlling for demographic, clinical, and hospital characteristics associated with long LOS, there was significant (P < 0.001) variation in the prevalence of long LOS for CMC across children's hospitals in the cohort (range, 10.3%21.8%) (Figure 1). Twelve (27%) hospitals had a significantly (P < 0.001) higher prevalence of long LOS for their hospitalized CMC, compared to the mean. Eighteen (41%) had a significantly (P < 0.001) lower prevalence of long LOS for their hospitalized CMC. There was also significant variation across hospitals with respect to cost, with 49.7% to 73.7% of all hospital costs of CMC attributed to long LOS hospitalizations. Finally, there was indirect correlation with the prevalence of LOS across hospitals and the hospitals' 30‐day readmission rate ( = 0.3; P = 0.04). As the prevalence of long LOS increased, the readmission rate decreased.
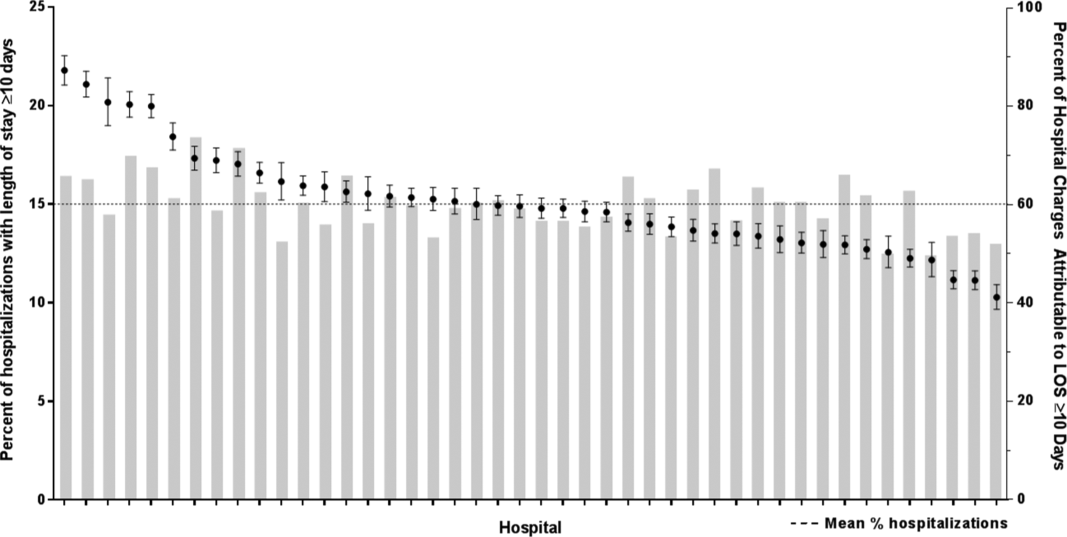
DISCUSSION
The main findings from this study suggest that a small percentage of CMC experiencing long LOS account for the majority of hospital bed days and cost of all hospitalized CMC in children's hospitals. The likelihood of long LOS varies significantly by CMC's age, race/ethnicity, and payor as well as by type and number of chronic conditions. Among CMC with long LOS, the use of gastrointestinal devices such as gastrostomy tubes, as well as congenital heart disease, were highly prevalent. In multivariable analysis, the characteristics most strongly associated with LOS 10 days were use of the ICU, respiratory complex chronic condition, and transfer from another medical facility at admission. After adjusting for these factors, there was significant variation in the prevalence of LOS 10 days for CMC across children's hospitals.
Although it is well known that CMC as a whole have a major impact on resource use in children's hospitals, this study reveals that 15% of hospitalizations of CMC account for 62% of all hospital costs of CMC. That is, a small fraction of hospitalizations of CMC is largely responsible for the significant financial impact of hospital resource use. To date, most clinical efforts and policies striving to reduce hospital use in CMC have focused on avoiding readmissions or index hospital admissions entirely, rather than improving the efficiency of hospital care after admission occurs.[23, 24, 25, 26] In the adult population, the impact of long LOS on hospital costs has been recognized, and several Medicare incentive programs have focused on in‐hospital timeliness and efficiency. As a result, LOS in Medicare beneficiaries has decreased dramatically over the past 2 decades.[27, 28, 29, 30] Optimizing the efficiency of hospital care for CMC may be an important goal to pursue, especially with precedent set in the adult literature.
Perhaps the substantial variation across hospitals in the prevalence of long LOS in CMC indicates opportunity to improve the efficiency of their inpatient care. This variation was not due to differences across hospitals' case mix of CMC. Further investigation is needed to determine how much of it is due to differences in quality of care. Clinical practice guidelines for hospital treatment of common illnesses usually exclude CMC. In our clinical experience across 9 children's hospitals, we have experienced varying approaches to setting discharge goals (ie, parameters on how healthy the child needs to be to ensure a successful hospital discharge) for CMC.[31] When the goals are absent or not clearly articulated, they can contribute to a prolonged hospitalization. Some families of CMC report significant issues when working with pediatric hospital staff to assess their child's discharge readiness.[7, 32, 33] In addition, there is significant variation across states and regions in access to and quality of post‐discharge health services (eg, home nursing, postacute care, durable medical equipment).[34, 35] In some areas, many CMC are not actively involved with their primary care physician.[5] These issues might also influence the ability of some children's hospitals to efficiently discharge CMC to a safe and supportive post‐discharge environment. Further examination of hospital outliersthose with the lowest and highest percentage of CMC hospitalizations with long LOSmay reveal opportunities to identify and spread best practices.
The demographic and clinical factors associated with long LOS in the present study, including age, ICU use, and transfer from another hospital, might help hospitals target which CMC have the greatest risk for experiencing long LOS. We found that infants age <1 year had longer LOS when compared with older children. Similar to our findings, younger‐aged children hospitalized with bronchiolitis have longer LOS.[36] Certainly, infants with medical complexity, in general, are a high‐acuity population with the potential for rapid clinical deterioration during an acute illness. Prolonged hospitalization for treatment and stabilization may be expected for many of them. Additional investigation is warranted to examine ICU use in CMC, and whether ICU admission or duration can be safely prevented or abbreviated. Opportunities to assess the quality of transfers into children's hospitals of CMC admitted to outside hospitals may be necessary. A study of pediatric burn patients reported that patients initially stabilized at a facility that was not a burn center and subsequently transferred to a burn center had a longer LOS than patients solely treated at a designated burn center.[37] Furthermore, events during transport itself may adversely impact the stability of an already fragile patient. Interventions to optimize the quality of care provided by transport teams have resulted in decreased LOS at the receiving hospital.[38]
This study's findings should be considered in the context of several limitations. Absent a gold‐standard definition of long LOS, we used the distribution of LOS across patients to inform our methods; LOS at the 90th percentile was selected as long. Although our sensitivity analysis using LOS at the 75th percentile produced similar findings, other cut points in LOS might be associated with different results. The study is not positioned to determine how much of the reported LOS was excessive, unnecessary, or preventable. The study findings may not generalize to types of hospitals not contained in PHIS (eg, nonchildren's hospitals and community hospitals). We did not focus on the impact of a new diagnosis (eg, new chronic illness) or acute in‐hospital event (eg, nosocomial infection) on prolonged LOS; future studies should investigate these clinical events with LOS.
PHIS does not contain information regarding characteristics that could influence LOS, including the children's social and familial attributes, transportation availability, home equipment needs, and local availability of postacute care facilities. Moreover, PHIS does not contain information about the hospital discharge procedures, process, or personnel across hospitals, which could influence LOS. Future studies on prolonged LOS should consider assessing this information. Because of the large sample size of hospitalizations included, the statistical power for the analyses was strong, rendering it possible that some findings that were statistically significant might have modest clinical significance (eg, relationship of Hispanic ethnicity with prolonged LOS). We could not determine why a positive correlation was not observed between hospitals' long LOS prevalence and their percentage of cost associated with long LOS; future studies should investigate the reasons for this finding.
Despite these limitations, the findings of the present study highlight the significance of long LOS in hospitalized CMC. These long hospitalizations account for a significant proportion of all hospital costs for this important population of children. The prevalence of long LOS for CMC varies considerably across children's hospitals, even after accounting for the case mix. Efforts to curtail hospital resource use and costs for CMC may benefit from focus on long LOS.
Children with medical complexity (CMC) have complex and chronic health conditions that often involve multiple organ systems and severely affect cognitive and physical functioning. Although the prevalence of CMC is low (1% of all children), they account for nearly one‐fifth of all pediatric admissions and one‐half of all hospital days and charges in the United States.[1] Over the last decade, CMC have had a particularly large and increasing impact in tertiary‐care children's hospitals.[1, 2] The Institute of Medicine has identified CMC as a priority population for a revised healthcare system.[3]
Medical homes, hospitals, health plans, states, federal agencies, and others are striving to reduce excessive hospital use in CMC because of its high cost.[4, 5, 6] Containing length of stay (LOS)an increasingly used indicator of the time sensitiveness and efficiency of hospital careis a common aim across these initiatives. CMC have longer hospitalizations than children without medical complexity. Speculated reasons for this are that CMC tend to have (1) higher severity of acute illnesses (eg, pneumonia, cellulitis), (2) prolonged recovery time in the hospital, and (3) higher risk of adverse events in the hospital. Moreover, hospital clinicians caring for CMC often find it difficult to determine discharge readiness, given that many CMC do not return to a completely healthy baseline.[7]
Little is known about long LOS in CMC, including which CMC have the highest risk of experiencing such stays and which stays might have the greatest opportunity to be shortened. Patient characteristics associated with prolonged length of stay have been studied extensively for many pediatric conditions (eg, asthma).[8, 9, 10, 11, 12, 13, 14] However, most of these studies excluded CMC. Therefore, the objectives of this study were to examine (1) the prevalence of long LOS in CMC, (2) patient characteristics associated with long LOS, and (3) hospital‐to‐hospital variation in prevalence of long LOS hospitalizations.
METHODS
Study Design and Data Source
This study is a multicenter, retrospective cohort analysis of the Pediatric Health Information System (PHIS). PHIS is an administrative database of 44 not for profit, tertiary care pediatric hospitals affiliated with the Children's Hospital Association (CHA) (Overland Park, KS). PHIS contains data regarding patient demographics, diagnoses, and procedures (with International Classification of Diseases, 9th Revision, Clinical Modification [ICD‐9‐CM] codes), All‐Patient Refined Diagnostic Related Groups version 30 (APR‐DRGs) (3M Health Information Systems, Salt Lake City, UT), and service lines that aggregate the APR‐DRGs into 38 distinct groups. Data quality and reliability are assured through CHA and participating hospitals. In accordance with the policies of the Cincinnati Children's Hospital Medical Center Institutional Review Board, this study of deidentified data was not considered human subjects research.
Study Population
Inclusion Criteria
Children discharged following an observation or inpatient admission from a hospital participating in the PHIS database between January 1, 2013 and December 31, 2014 were eligible for inclusion if they were considered medically complex. Medical complexity was defined using Clinical Risk Groups (CRGs) version 1.8, developed by 3M Health Information Systems and the National Association of Children's Hospitals and Related Institutions. CRGs were used to assign each hospitalized patient to 1 of 9 mutually exclusive chronicity groups according to the presence, type, and severity of chronic conditions.[15, 16, 17, 18] Each patient's CRG designation was based on 2 years of previous hospital encounters.
As defined in prior studies and definitional frameworks of CMC,[1] patients belonging to CRG group 6 (significant chronic disease in 2 organ systems), CRG group 7 (dominant chronic disease in 3 organ systems), and CRG group 9 (catastrophic condition) were considered medically complex.[17, 19] Patients with malignancies (CRG group 8) were not included for analysis because they are a unique population with anticipated, long hospital stays. Patients with CRG group 5, representing those with chronic conditions affecting a single body system, were also not included because most do not have attributes consistent with medical complexity.
Exclusion Criteria
We used the APR‐DRG system, which leverages ICD‐9‐CM codes to identify the health problem most responsible for the hospitalization, to refine the study cohort. We excluded hospitalizations that were classified by the APR‐DRG system as neonatal, as we did not wish to focus on LOS in the neonatal intensive care unit (ICU) or for birth admissions. Similarly, hospitalizations for chemotherapy (APR‐DRG 693) or malignancy (identified with previously used ICD‐9‐CM codes)[20] were also excluded because long LOS is anticipated. We also excluded hospitalizations for medical rehabilitation (APR‐DRG 860).
Outcome Measures
The primary outcome measure was long LOS, defined as LOS 10 days. The cut point of LOS 10 days represents the 90th percentile of LOS for all children, with and without medical complexity, hospitalized during 2013 to 2014. LOS 10 days has previously been used as a threshold of long LOS.[21] For hospitalizations involving transfer at admission from another acute care facility, LOS was measured from the date of transfer. We also assessed hospitals' cost attributable to long LOS admissions.
Patient Demographics and Clinical Characteristics
We measured demographic characteristics including age, gender, race/ethnicity, insurance type, and distance traveled (the linear distance between the centroid of the patient's home ZIP code and the centroid of the hospital's ZIP code). Clinical characteristics included CRG classification, complex chronic condition (CCC), and dependence on medical technology. CCCs are defined as any medical condition that can be reasonably expected to last at least 12 months (unless death intervenes) and to involve either several different organ systems or 1 system severely enough to require specialty pediatric care and probably some period of hospitalization in a tertiary care center.[20] Medical technology included devices used to optimize the health and functioning of the child (eg, gastrostomy, tracheostomy, cerebrospinal fluid shunt).[22]
Hospitalization Characteristics
Characteristics of the hospitalization included transfer from an outside facility, ICU admission, surgical procedure (using surgical APR‐DRGs), and discharge disposition (home, skilled nursing facility, home health services, death, other). Cost of the hospitalization was estimated in the PHIS from charges using hospital and year‐specific ratios of cost to charge.
Statistical Analysis
Continuous data (eg, distance from hospital to home residence) were described with median and interquartile ranges (IQR) because they were not normally distributed. Categorical data (eg, type of chronic condition) were described with counts and frequencies. In bivariate analyses, demographic, clinical, and hospitalization characteristics were stratified by LOS (long LOS vs LOS <10 days), and compared using 2 statistics or Wilcoxon rank sum tests as appropriate.
We modeled the likelihood of experiencing a long LOS using generalized linear mixed effects models with a random hospital intercept and discharge‐level fixed effects for age, gender, payor, CCC type, ICU utilization, transfer status, a medical/surgical admission indicator derived from the APR‐DRG, and CRG assigned to each hospitalization. To examine hospital‐to‐hospital variability, we generated hospital risk‐adjusted rates of long LOS from these models. Similar models and hospital risk‐adjusted rates were built for a post hoc correlational analysis of 30‐day all cause readmission, where hospitals' rates and percent of long LOS were compared with a Pearson correlation coefficient. Also, for our multivariable models, we performed a sensitivity analysis using an alternative definition of long LOS as 4 days (the 75th percentile of LOS for all children, with and without medical complexity, hospitalized during 20132014). All analyses were performed using SAS version 9.4 (SAS Institute, Cary, NC), and P values <0.05 were considered statistically significant.
RESULTS
Study Population
There were 954,018 hospitalizations of 217,163 CMC at 44 children's hospitals included for analysis. Forty‐seven percent of hospitalizations were for females, 49.4% for non‐Hispanic whites, and 61.1% for children with government insurance. Fifteen percent (n = 142,082) had a long LOS of 10 days. The median (IQR) LOS of hospitalizations <10 days versus 10 days were 2 (IQR, 14) and 16 days (IQR, 1226), respectively. Long LOS hospitalizations accounted for 61.1% (3.7 million) hospital days and 61.8% ($13.7 billion) of total hospitalization costs for all CMC in the cohort (Table 1).
Characteristic | Overall (n = 954,018) | Length of Stay | |
---|---|---|---|
<10 Days (n = 811,936) | 10 Days (n = 142,082) | ||
| |||
Age at admission, y, % | |||
<1 | 14.6 | 12.7 | 25.7 |
14 | 27.1 | 27.9 | 22.4 |
59 | 20.1 | 21.0 | 14.9 |
1018 | 33.6 | 34.0 | 31.7 |
18+ | 4.6 | 4.4 | 5.4 |
Gender, % | |||
Female | 47.0 | 46.9 | 47.5 |
Race/ethnicity, % | |||
Non‐Hispanic white | 49.4 | 49.4 | 49.4 |
Non‐Hispanic black | 23.1 | 23.8 | 19.3 |
Hispanic | 18.2 | 17.8 | 20.4 |
Asian | 2.0 | 1.9 | 2.3 |
Other | 7.4 | 7.1 | 8.6 |
Complex chronic condition, % | |||
Any | 79.5 | 77.3 | 91.8 |
Technology assistance | 37.1 | 34.1 | 54.2 |
Gastrointestinal | 30.0 | 27.2 | 45.9 |
Neuromuscular | 28.2 | 27.7 | 30.9 |
Cardiovascular | 16.8 | 14.5 | 29.9 |
Respiratory | 14.1 | 11.5 | 29.4 |
Congenital/genetic defect | 17.2 | 16.7 | 20.2 |
Metabolic | 9.9 | 8.9 | 15.4 |
Renal | 10.1 | 9.5 | 13.8 |
Hematology/emmmunodeficiency | 11.7 | 12.0 | 10.0 |
Neonatal | 3.8 | 3.1 | 7.7 |
Transplantation | 4.5 | 4.2 | 6.7 |
Clinical risk group, % | |||
Chronic condition in 2 systems | 68.4 | 71.2 | 53.9 |
Catastrophic chronic condition | 31.4 | 28.8 | 46.1 |
Distance from hospital to home residence in miles, median [IQR] | 16.2 [7.440.4] | 15.8 [7.338.7] | 19.1 [8.552.6] |
Transferred from outside hospital (%) | 6.5 | 5.3 | 13.6 |
Admitted for surgery, % | 23.4 | 20.7 | 38.7 |
Use of intensive care, % | 19.6 | 14.9 | 46.5 |
Discharge disposition, % | |||
Home | 91.2 | 92.9 | 81.4 |
Home healthcare | 4.5 | 3.5 | 9.9 |
Other | 2.9 | 2.6 | 4.5 |
Postacute care facility | 1.1 | 0.8 | 3.1 |
Died | 0.4 | 0.3 | 1.1 |
Payor, % | |||
Government | 61.1 | 60.6 | 63.5 |
Private | 33.2 | 33.6 | 30.9 |
Other | 5.7 | 5.7 | 5.7 |
Hospital resource use | |||
Median length of stay [IQR] | 3 [16] | 2 [14] | 16 [1226] |
Median hospital cost [IQR] | $8,144 [$4,122$18,447] | $6,689 [$3,685$12,395] | $49,207 [$29,444$95,738] |
Total hospital cost, $, billions | $22.2 | $8.5 | $13.7 |
Demographics and Clinical Characteristics of Children With and Without Long LOS
Compared with hospitalized CMC with LOS <10 days, a higher percentage of hospitalizations with LOS 10 days were CMC age <1 year (25.7% vs 12.7%, P < 0.001) and Hispanic (20.4% vs 17.8%, P < 0.001). CMC hospitalizations with a long LOS had a higher percentage of any CCC (91.8% vs 77.3%, P < 0.001); the most common CCCs were gastrointestinal (45.9%), neuromuscular (30.9%), and cardiovascular (29.9%). Hospitalizations of CMC with a long LOS had a higher percentage of a catastrophic chronic condition (46.1% vs 28.8%, P < 0.001) and technology dependence (46.1% vs 28.8%, P < 0.001) (Table 1).
Hospitalization Characteristics of Children With and Without Long LOS
Compared with hospitalizations of CMC with LOS <10 days, hospitalizations of CMC with a long LOS more often involved transfer in from another hospital at admission (13.6% vs 5.3%, P < 0.001). CMC hospital stays with a long LOS more often involved surgery (38.7% vs 20.7%, P < 0.001) and use of intensive care (46.5% vs 14.9%; P < 0.001). A higher percentage of CMC with long LOS were discharged with home health services (9.9% vs 3.5%; P < 0.001) (Table 1).
The most common admitting diagnoses and CCCs for hospitalizations of CMC with long LOS are presented in Table 2. The two most prevalent APR‐DRGs in CMC hospitalizations lasting 10 days or longer were cystic fibrosis (10.7%) and respiratory system disease with ventilator support (5.5%). The two most common chronic condition characteristics represented among long CMC hospitalizations were gastrointestinal devices (eg, gastrostomy tube) (39.7%) and heart and great vessel malformations (eg, tetralogy of Fallot) (12.8%). The 5 most common CCC subcategories, as listed in Table 2, account for nearly 100% of the patients with long LOS hospitalizations.
| |
Most common reason for admission* | |
Cystic fibrosis | 10.7% |
Respiratory system diagnosis with ventilator support 96+ hours | 5.5% |
Malfunction, reaction, and complication of cardiac or vascular device or procedure | 2.8% |
Craniotomy except for trauma | 2.6% |
Major small and large bowel procedures | 2.3% |
Most common complex chronic condition | |
Gastrointestinal devices | 39.7% |
Heart and great vessel malformations | 12.8% |
Cystic fibrosis | 12.5% |
Dysrhythmias | 11.0% |
Respiratory devices | 10.7% |
Multivariable Analysis of Characteristics Associated With Long LOS
In multivariable analysis, the highest likelihood of long LOS was experienced by children who received care in the ICU (odds ratio [OR]: 3.5, 95% confidence interval [CI]: 3.43.5), who had a respiratory CCC (OR: 2.7, 95% CI: 2.62.7), and who were transferred from another acute care hospital at admission (OR: 2.1, 95% CI: 2.0, 2.1). The likelihood of long LOS was also higher in children <1 year of age (OR: 1.2, 95% CI: 1.21.3), and Hispanic children (OR: 1.1, 95% CI 1.0‐1.10) (Table 3). Similar multivariable findings were observed in sensitivity analysis using the 75th percentile of LOS (4 days) as the model outcome.
Characteristic | Odds Ratio (95% CI) of LOS 10 Days | P Value |
---|---|---|
| ||
Use of intensive care | 3.5 (3.4‐3.5) | <0.001 |
Transfer from another acute‐care hospital | 2.1 (2.0‐2.1) | <0.001 |
Procedure/surgery | 1.8 (1.8‐1.9) | <0.001 |
Complex chronic condition | ||
Respiratory | 2.7 (2.6‐2.7) | <0.001 |
Gastrointestinal | 1.8 (1.8‐1.8) | <0.001 |
Metabolic | 1.7 (1.7‐1.7) | <0.001 |
Cardiovascular | 1.6 (1.5‐1.6) | <0.001 |
Neonatal | 1.5 (1.5‐1.5) | <0.001 |
Renal | 1.4 (1.4‐1.4) | <0.001 |
Transplant | 1.4 (1.4‐1.4) | <0.001 |
Hematology and immunodeficiency | 1.3 (1.3‐1.3) | <0.001 |
Technology assistance | 1.1 (1.1, 1.1) | <0.001 |
Neuromuscular | 0.9 (0.9‐0.9) | <0.001 |
Congenital or genetic defect | 0.8 (0.8‐0.8) | <0.001 |
Age at admission, y | ||
<1 | 1.2 (1.2‐1.3) | <0.001 |
14 | 0.5 (0.5‐0.5) | <0.001 |
59 | 0.6 (0.6‐0.6) | <0.001 |
1018 | 0.9 (0.9‐0.9) | <0.001 |
18+ | Reference | |
Male | 0.9 (0.9‐0.9) | <0.001 |
Race/ethnicity | ||
Non‐Hispanic black | 0.9 (0.9‐0.9) | <0.001 |
Hispanic | 1.1 (1.0‐1.1) | <0.001 |
Asian | 1.0 (1.0‐1.1) | 0.3 |
Other | 1.1 (1.1‐1.1) | <0.001 |
Non‐Hispanic white | Reference | |
Payor | ||
Private | 0.9 (0.8 0.9) | <0.001 |
Other | 1.0 (1.0‐1.0) | 0.4 |
Government | Reference | |
Season | ||
Spring | 1.0 (1.0 1.0) | <0.001 |
Summer | 0.9 (0.9‐0.9) | <0.001 |
Fall | 1.0 (0.9‐1.0) | <0.001 |
Winter | Reference |
Variation in the Prevalence of Long LOS Across Children's Hospitals
After controlling for demographic, clinical, and hospital characteristics associated with long LOS, there was significant (P < 0.001) variation in the prevalence of long LOS for CMC across children's hospitals in the cohort (range, 10.3%21.8%) (Figure 1). Twelve (27%) hospitals had a significantly (P < 0.001) higher prevalence of long LOS for their hospitalized CMC, compared to the mean. Eighteen (41%) had a significantly (P < 0.001) lower prevalence of long LOS for their hospitalized CMC. There was also significant variation across hospitals with respect to cost, with 49.7% to 73.7% of all hospital costs of CMC attributed to long LOS hospitalizations. Finally, there was indirect correlation with the prevalence of LOS across hospitals and the hospitals' 30‐day readmission rate ( = 0.3; P = 0.04). As the prevalence of long LOS increased, the readmission rate decreased.
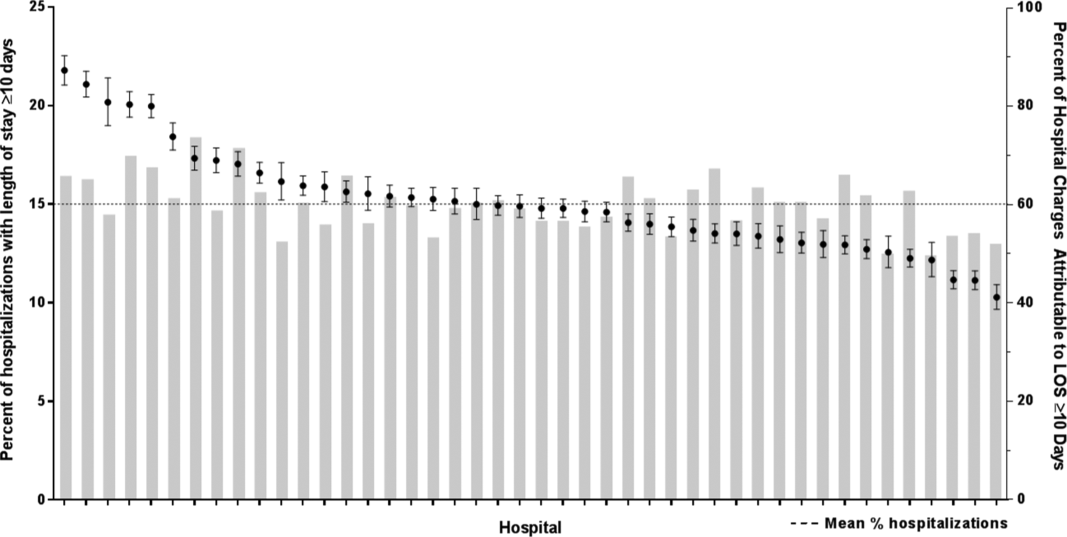
DISCUSSION
The main findings from this study suggest that a small percentage of CMC experiencing long LOS account for the majority of hospital bed days and cost of all hospitalized CMC in children's hospitals. The likelihood of long LOS varies significantly by CMC's age, race/ethnicity, and payor as well as by type and number of chronic conditions. Among CMC with long LOS, the use of gastrointestinal devices such as gastrostomy tubes, as well as congenital heart disease, were highly prevalent. In multivariable analysis, the characteristics most strongly associated with LOS 10 days were use of the ICU, respiratory complex chronic condition, and transfer from another medical facility at admission. After adjusting for these factors, there was significant variation in the prevalence of LOS 10 days for CMC across children's hospitals.
Although it is well known that CMC as a whole have a major impact on resource use in children's hospitals, this study reveals that 15% of hospitalizations of CMC account for 62% of all hospital costs of CMC. That is, a small fraction of hospitalizations of CMC is largely responsible for the significant financial impact of hospital resource use. To date, most clinical efforts and policies striving to reduce hospital use in CMC have focused on avoiding readmissions or index hospital admissions entirely, rather than improving the efficiency of hospital care after admission occurs.[23, 24, 25, 26] In the adult population, the impact of long LOS on hospital costs has been recognized, and several Medicare incentive programs have focused on in‐hospital timeliness and efficiency. As a result, LOS in Medicare beneficiaries has decreased dramatically over the past 2 decades.[27, 28, 29, 30] Optimizing the efficiency of hospital care for CMC may be an important goal to pursue, especially with precedent set in the adult literature.
Perhaps the substantial variation across hospitals in the prevalence of long LOS in CMC indicates opportunity to improve the efficiency of their inpatient care. This variation was not due to differences across hospitals' case mix of CMC. Further investigation is needed to determine how much of it is due to differences in quality of care. Clinical practice guidelines for hospital treatment of common illnesses usually exclude CMC. In our clinical experience across 9 children's hospitals, we have experienced varying approaches to setting discharge goals (ie, parameters on how healthy the child needs to be to ensure a successful hospital discharge) for CMC.[31] When the goals are absent or not clearly articulated, they can contribute to a prolonged hospitalization. Some families of CMC report significant issues when working with pediatric hospital staff to assess their child's discharge readiness.[7, 32, 33] In addition, there is significant variation across states and regions in access to and quality of post‐discharge health services (eg, home nursing, postacute care, durable medical equipment).[34, 35] In some areas, many CMC are not actively involved with their primary care physician.[5] These issues might also influence the ability of some children's hospitals to efficiently discharge CMC to a safe and supportive post‐discharge environment. Further examination of hospital outliersthose with the lowest and highest percentage of CMC hospitalizations with long LOSmay reveal opportunities to identify and spread best practices.
The demographic and clinical factors associated with long LOS in the present study, including age, ICU use, and transfer from another hospital, might help hospitals target which CMC have the greatest risk for experiencing long LOS. We found that infants age <1 year had longer LOS when compared with older children. Similar to our findings, younger‐aged children hospitalized with bronchiolitis have longer LOS.[36] Certainly, infants with medical complexity, in general, are a high‐acuity population with the potential for rapid clinical deterioration during an acute illness. Prolonged hospitalization for treatment and stabilization may be expected for many of them. Additional investigation is warranted to examine ICU use in CMC, and whether ICU admission or duration can be safely prevented or abbreviated. Opportunities to assess the quality of transfers into children's hospitals of CMC admitted to outside hospitals may be necessary. A study of pediatric burn patients reported that patients initially stabilized at a facility that was not a burn center and subsequently transferred to a burn center had a longer LOS than patients solely treated at a designated burn center.[37] Furthermore, events during transport itself may adversely impact the stability of an already fragile patient. Interventions to optimize the quality of care provided by transport teams have resulted in decreased LOS at the receiving hospital.[38]
This study's findings should be considered in the context of several limitations. Absent a gold‐standard definition of long LOS, we used the distribution of LOS across patients to inform our methods; LOS at the 90th percentile was selected as long. Although our sensitivity analysis using LOS at the 75th percentile produced similar findings, other cut points in LOS might be associated with different results. The study is not positioned to determine how much of the reported LOS was excessive, unnecessary, or preventable. The study findings may not generalize to types of hospitals not contained in PHIS (eg, nonchildren's hospitals and community hospitals). We did not focus on the impact of a new diagnosis (eg, new chronic illness) or acute in‐hospital event (eg, nosocomial infection) on prolonged LOS; future studies should investigate these clinical events with LOS.
PHIS does not contain information regarding characteristics that could influence LOS, including the children's social and familial attributes, transportation availability, home equipment needs, and local availability of postacute care facilities. Moreover, PHIS does not contain information about the hospital discharge procedures, process, or personnel across hospitals, which could influence LOS. Future studies on prolonged LOS should consider assessing this information. Because of the large sample size of hospitalizations included, the statistical power for the analyses was strong, rendering it possible that some findings that were statistically significant might have modest clinical significance (eg, relationship of Hispanic ethnicity with prolonged LOS). We could not determine why a positive correlation was not observed between hospitals' long LOS prevalence and their percentage of cost associated with long LOS; future studies should investigate the reasons for this finding.
Despite these limitations, the findings of the present study highlight the significance of long LOS in hospitalized CMC. These long hospitalizations account for a significant proportion of all hospital costs for this important population of children. The prevalence of long LOS for CMC varies considerably across children's hospitals, even after accounting for the case mix. Efforts to curtail hospital resource use and costs for CMC may benefit from focus on long LOS.
- Inpatient growth and resource use in 28 children's hospitals: a longitudinal, multi‐institutional study. JAMA Pediatr. 2013;167(2):170–177. , , , et al.
- Children with complex chronic conditions in inpatient hospital settings in the united states. Pediatrics. 2010;126(4):647–655. , , , et al.
- Meeting the health care needs of persons with disabilities. Milbank Q. 2002;80(2):381–391. , .
- Effect of an enhanced medical home on serious illness and cost of care among high‐risk children with chronic illness: a randomized clinical trial. JAMA. 2014;312(24):2640–2648. , , , et al.
- Children with medical complexity and Medicaid: spending and cost savings. Health Aff Proj Hope. 2014;33(12):2199–2206. , , , et al.
- Children's Hospital Association. CARE Award. Available at: https://www.childrenshospitals.org/Programs‐and‐Services/Quality‐Improvement‐and‐Measurement/CARE‐Award. Accessed December 18, 2015.
- Hospital readmission and parent perceptions of their child's hospital discharge. Int J Qual Health Care. 2013;25(5):573–581. , , , et al.
- Weekend matters: Friday and Saturday admissions are associated with prolonged hospitalization of children. Clin Pediatr (Phila). 2013;52(9):875–878. , , , et al.
- Attributable cost and length of stay for central line‐associated bloodstream infections. Pediatrics. 2014;133(6):e1525–e1532. , , , .
- Effect of healthcare‐acquired infection on length of hospital stay and cost. Infect Control Hosp Epidemiol. 2007;28(3):280–292. , , , et al.
- Hospital utilization and costs among children with influenza, 2003. Am J Prev Med. 2009;36(4):292–296. , , , , .
- Charges and lengths of stay attributable to adverse patient‐care events using pediatric‐specific quality indicators: a multicenter study of freestanding children's hospitals. Pediatrics. 2008;121(6):e1653–e1659. , , , .
- Variation in resource utilization for the management of uncomplicated community‐acquired pneumonia across community and children's hospitals. J Pediatr. 2014;165(3):585–591. , , , , .
- Variation and outcomes associated with direct hospital admission among children with pneumonia in the United States. JAMA Pediatr. 2014;168(9):829–836. , , , , .
- Clinical Risk Groups (CRGs): a classification system for risk‐adjusted capitation‐based payment and health care management. Med Care. 2004;42(1):81–90. , , , et al.
- Identifying children with lifelong chronic conditions for care coordination by using hospital discharge data. Acad Pediatr. 2010;10(6):417–423. , , , et al.
- Profile of medical charges for children by health status group and severity level in a Washington State Health Plan. Health Serv Res. 2004;39(1):73–89. , , , , .
- Using medical billing data to evaluate chronically ill children over time. J Ambulatory Care Manage. 2006;29(4):283–290. , , , .
- Medical complexity and pediatric emergency department and inpatient utilization. Pediatrics. 2013;131(2):e559–e565. , , , , , .
- Pediatric complex chronic conditions classification system version 2: updated for ICD‐10 and complex medical technology dependence and transplantation. BMC Pediatr. 2014;14:199. , , , , .
- Analyzing intensive care unit length of stay data: problems and possible solutions. Crit Care Med. 1997;25(9):1594–1600. .
- Hospital utilization and characteristics of patients experiencing recurrent readmissions within children's hospitals. JAMA. 2011;305(7):682–690. , , , et al.
- Hospital readmissions and repeat emergency department visits among children with medical complexity: an integrative review. J Pediatr Nurs. 2013;28(4):316–339. .
- Hospital readmission in children with complex chronic conditions discharged from subacute care. Hosp Pediatr. 2014;4(3):153–158. , , , .
- Preventing hospitalizations in children with medical complexity: a systematic review. Pediatrics. 2014;134(6):e1628–e1647. , , , et al.
- Hospital readmissions for newly discharged pediatric home mechanical ventilation patients. Pediatr Pulmonol. 2012;47(4):409–414. , , , .
- Clinical characteristics and outcomes of Medicare patients undergoing total hip arthroplasty, 1991–2008. JAMA. 2011;305(15):1560–1567. , , , et al.
- Trends in length of stay and short‐term outcomes among Medicare patients hospitalized for heart failure, 1993–2006. JAMA. 2010;303(21):2141–2147. , , , et al.
- U.S. Department of Health and Human Services. CMS Statistics 2013. Available at: https://www.cms.gov/Research‐Statistics‐Data‐and‐Systems/Statistics‐Trends‐and‐Reports/CMS‐Statistics‐Reference‐Booklet/Downloads/CMS_Stats_2013_final.pdf. Published August 2013. Accessed October 6, 2015.
- Centers for Medicare and Medicaid Services. Evaluation of the premier hospital quality incentive demonstration. Available at: https://www.cms.gov/Research‐Statistics‐Data‐and‐Systems/Statistics‐Trends‐and‐Reports/Reports/downloads/Premier_ExecSum_2010.pdf. Published March 3, 2009. Accessed September 18, 2015.
- A framework of pediatric hospital discharge care informed by legislation, research, and practice. JAMA Pediatr. 2014;168(10):955–962; quiz 965–966. , , , et al.
- Parent and provider perspectives on pediatric readmissions: what can we learn about readiness for discharge? Hosp Pediatr. 2015;5(11):559–565. , , , , .
- Preventing readmissions in children: how do we do that? Hosp Pediatr. 2015;5(11):602–604. , .
- Pediatric post‐acute hospital care: striving for identity and value. Hosp Pediatr. 2015;5(10):548–551. , , .
- Pediatric hospital discharges to home health and postacute facility care: a national study. JAMA Pediatr. 2016;170(4):326–333. , , , et al.
- Bronchiolitis: clinical characteristics associated with hospitalization and length of stay. Pediatr Emerg Care. 2012;28(2):99–103. , , , et al.
- The effect of transfers between health care facilities on costs and length of stay for pediatric burn patients. J Burn Care Res. 2015;36(1):178–183. , , , , .
- Goal‐directed resuscitative interventions during pediatric interfacility transport. Crit Care Med. 2015;43(8):1692–1698. , , , et al.
- Inpatient growth and resource use in 28 children's hospitals: a longitudinal, multi‐institutional study. JAMA Pediatr. 2013;167(2):170–177. , , , et al.
- Children with complex chronic conditions in inpatient hospital settings in the united states. Pediatrics. 2010;126(4):647–655. , , , et al.
- Meeting the health care needs of persons with disabilities. Milbank Q. 2002;80(2):381–391. , .
- Effect of an enhanced medical home on serious illness and cost of care among high‐risk children with chronic illness: a randomized clinical trial. JAMA. 2014;312(24):2640–2648. , , , et al.
- Children with medical complexity and Medicaid: spending and cost savings. Health Aff Proj Hope. 2014;33(12):2199–2206. , , , et al.
- Children's Hospital Association. CARE Award. Available at: https://www.childrenshospitals.org/Programs‐and‐Services/Quality‐Improvement‐and‐Measurement/CARE‐Award. Accessed December 18, 2015.
- Hospital readmission and parent perceptions of their child's hospital discharge. Int J Qual Health Care. 2013;25(5):573–581. , , , et al.
- Weekend matters: Friday and Saturday admissions are associated with prolonged hospitalization of children. Clin Pediatr (Phila). 2013;52(9):875–878. , , , et al.
- Attributable cost and length of stay for central line‐associated bloodstream infections. Pediatrics. 2014;133(6):e1525–e1532. , , , .
- Effect of healthcare‐acquired infection on length of hospital stay and cost. Infect Control Hosp Epidemiol. 2007;28(3):280–292. , , , et al.
- Hospital utilization and costs among children with influenza, 2003. Am J Prev Med. 2009;36(4):292–296. , , , , .
- Charges and lengths of stay attributable to adverse patient‐care events using pediatric‐specific quality indicators: a multicenter study of freestanding children's hospitals. Pediatrics. 2008;121(6):e1653–e1659. , , , .
- Variation in resource utilization for the management of uncomplicated community‐acquired pneumonia across community and children's hospitals. J Pediatr. 2014;165(3):585–591. , , , , .
- Variation and outcomes associated with direct hospital admission among children with pneumonia in the United States. JAMA Pediatr. 2014;168(9):829–836. , , , , .
- Clinical Risk Groups (CRGs): a classification system for risk‐adjusted capitation‐based payment and health care management. Med Care. 2004;42(1):81–90. , , , et al.
- Identifying children with lifelong chronic conditions for care coordination by using hospital discharge data. Acad Pediatr. 2010;10(6):417–423. , , , et al.
- Profile of medical charges for children by health status group and severity level in a Washington State Health Plan. Health Serv Res. 2004;39(1):73–89. , , , , .
- Using medical billing data to evaluate chronically ill children over time. J Ambulatory Care Manage. 2006;29(4):283–290. , , , .
- Medical complexity and pediatric emergency department and inpatient utilization. Pediatrics. 2013;131(2):e559–e565. , , , , , .
- Pediatric complex chronic conditions classification system version 2: updated for ICD‐10 and complex medical technology dependence and transplantation. BMC Pediatr. 2014;14:199. , , , , .
- Analyzing intensive care unit length of stay data: problems and possible solutions. Crit Care Med. 1997;25(9):1594–1600. .
- Hospital utilization and characteristics of patients experiencing recurrent readmissions within children's hospitals. JAMA. 2011;305(7):682–690. , , , et al.
- Hospital readmissions and repeat emergency department visits among children with medical complexity: an integrative review. J Pediatr Nurs. 2013;28(4):316–339. .
- Hospital readmission in children with complex chronic conditions discharged from subacute care. Hosp Pediatr. 2014;4(3):153–158. , , , .
- Preventing hospitalizations in children with medical complexity: a systematic review. Pediatrics. 2014;134(6):e1628–e1647. , , , et al.
- Hospital readmissions for newly discharged pediatric home mechanical ventilation patients. Pediatr Pulmonol. 2012;47(4):409–414. , , , .
- Clinical characteristics and outcomes of Medicare patients undergoing total hip arthroplasty, 1991–2008. JAMA. 2011;305(15):1560–1567. , , , et al.
- Trends in length of stay and short‐term outcomes among Medicare patients hospitalized for heart failure, 1993–2006. JAMA. 2010;303(21):2141–2147. , , , et al.
- U.S. Department of Health and Human Services. CMS Statistics 2013. Available at: https://www.cms.gov/Research‐Statistics‐Data‐and‐Systems/Statistics‐Trends‐and‐Reports/CMS‐Statistics‐Reference‐Booklet/Downloads/CMS_Stats_2013_final.pdf. Published August 2013. Accessed October 6, 2015.
- Centers for Medicare and Medicaid Services. Evaluation of the premier hospital quality incentive demonstration. Available at: https://www.cms.gov/Research‐Statistics‐Data‐and‐Systems/Statistics‐Trends‐and‐Reports/Reports/downloads/Premier_ExecSum_2010.pdf. Published March 3, 2009. Accessed September 18, 2015.
- A framework of pediatric hospital discharge care informed by legislation, research, and practice. JAMA Pediatr. 2014;168(10):955–962; quiz 965–966. , , , et al.
- Parent and provider perspectives on pediatric readmissions: what can we learn about readiness for discharge? Hosp Pediatr. 2015;5(11):559–565. , , , , .
- Preventing readmissions in children: how do we do that? Hosp Pediatr. 2015;5(11):602–604. , .
- Pediatric post‐acute hospital care: striving for identity and value. Hosp Pediatr. 2015;5(10):548–551. , , .
- Pediatric hospital discharges to home health and postacute facility care: a national study. JAMA Pediatr. 2016;170(4):326–333. , , , et al.
- Bronchiolitis: clinical characteristics associated with hospitalization and length of stay. Pediatr Emerg Care. 2012;28(2):99–103. , , , et al.
- The effect of transfers between health care facilities on costs and length of stay for pediatric burn patients. J Burn Care Res. 2015;36(1):178–183. , , , , .
- Goal‐directed resuscitative interventions during pediatric interfacility transport. Crit Care Med. 2015;43(8):1692–1698. , , , et al.