User login
Priorities and Gender Pay Gap
Hospitalists are a growing workforce numbering over 40,000 physicians, one‐third of whom are women.[1] Flexibility of work schedules and control over personal time have been the traditional selling points of the specialty.[2] Multiple studies of physician work life reveal growing physician dissatisfaction and a high prevalence of burnout.[3] To mitigate burnout risk, leaders in hospital medicine recognize the importance of creating a sustainable profession that offers both job and career satisfaction as well as work‐life balance and, importantly, fairness within the work environment.[4] Although success in some of these endeavors has been realized sporadically, sustaining work‐life balance and fairness in the specialty remains a work in progress, whereas evidence of high job attrition and pay inequities remain.[5, 6]
Pay inequity for women relative to men continues to be pervasive in medicine, including among early‐career physicians, researchers, and various specialists.[6, 7, 8, 9, 10, 11, 12, 13] The earnings gap seems to persist for physicians, even as federal efforts such as the Fair Pay Act of 2013 and the Paycheck Fairness Act of 2014 aim to end wage discrimination.[11, 14] Differences in specialty, part‐time status, and practice type do not mitigate the disparity.[8, 10, 15] Additional explanations have been proposed to explain the variability, including gender differences in negotiating skills, lack of opportunities to join networks of influence within organizations, and implicit or explicit bias and discrimination.[12, 16, 17, 18, 19, 20]
The earnings gap is also a consequence of what is commonly called the glass ceiling.[18, 19] Most agree that obstacles to fair advancement of women include absence of collaborative environments and role models who have successfully achieved work‐life balance.[17, 20, 21, 22] Somewhat surprisingly, women leaders in medicine seem to suffer greater income disparity than nonleaders; this income gap is prevalent among leaders in other elite professions as well.[7, 23] It is unknown whether women physicians' emphasis on work‐life balance, seen repeatedly in surveys, explains any of the pay disparity.[24] Little research to date has examined whether work‐life priorities of women in hospital medicine differ from men.
In this study, we sought to examine differences in job priorities between men and women hospitalists. In particular, we examine the relative prioritization of substantial pay to job satisfaction. We also examined gender differences in work patterns and earnings to explore potential sources of the persistent gender earnings gap.
METHODS
We analyzed data from the 20092010 Hospital Medicine Physician Worklife Survey, the design of which is detailed elsewhere.[4] Briefly, a 118‐item survey was administered by mail to a stratified sample of hospitalists from the Society of Hospital Medicine database and 3 large multisite hospitalist groups. A single survey item asked respondents to identify up to 4 out of 12 most important domains to their satisfaction with a hospitalist job. The domains were distilled from focus groups of nationally representative hospitalists as described previously,[4] and the survey item allowed up to one‐third of these domains to be identified as respondents' personal priorities. The list included: optimal variety of tasks, optimal workload, substantial pay, collegiality with other physicians, recognition by leaders, rewarding relationships with patients, satisfaction with nurses, optimal autonomy, control over personal time, fairness within organization, ample availability of resources to do job, and organizational climate of trust and belonging. We tabulated and ranked the frequency with which respondents selected each satisfaction domain by gender. Due to the nonstandard format of the survey item, we a priori decided to analyze only responses that were completed as instructed.
We also used demographic data including detailed work characteristics, clinical and nonclinical workload, total pretax earnings in 2009 as a hospitalist, and self‐identification as leader of their hospital medicine group. Respondent characteristics were tabulated and gender differences were tested using the t test, rank sum test, and the Fischer exact test as appropriate. We also listed the number of nonrespondents for each item. In estimating gender differences in earnings, we opted to use multiple‐imputation techniques to more conservatively account for greater variance inherent in the presence of missing data. Consistent with existing guidelines,[25] we demonstrated that item responses were not missing monotonically by visually inspecting patterns of nonresponse. We further demonstrated that data were missing at random by showing that response patterns of completed survey items did not predict whether or not a given variable response was missing using logistic regression models. We found no significant differences between respondents with complete and missing data. We verified that appropriate regression models for each variable on every other variable converged. We used Stata 13.1 (StataCorp, College Station, TX) to perform multiple imputations using chain equations (mi impute chain) to create 10 imputed tables for 7 normally distributed continuous variables using the ordinary least squares method, 3 non‐normally distributed variables using the predictive mean matching method, 2 nonordinal categorical variables using the multinomial logit method, and 1 binary variable using the logit method.[26, 27] Gender, pediatric specialty status, region of practice, and whether or not respondents prioritized substantial pay for job satisfaction were used as regular variables without missing data points.
Differences in earnings were assessed using a multivariate ordinary linear regression model applied to the imputed datasets fitted by forward selection of explanatory variables using P < 0.20 in bivariate analysis for inclusion and manual backward elimination of all statistically nonsignificant variables. We tested the significance of the women leader interaction term in the final parsimonious model. We used the usual significance threshold of P < 0.05 for inferences. Our analysis of publicly available anonymous data was exempt from IRB review.
RESULTS
Of the 816 survey respondents (response rate 25.6%), 40 either omitted the item soliciting work priorities or completed it incorrectly. Data from the remaining 776 respondents were used for the present analysis. Respondent characteristics are tabulated in Table 1. The characteristics of hospitalists by age, gender, specialty, practice model, and practice region were representative of US hospitalists from other surveys.[28]
Women | Men | P Value | No. of Missing Responses | |
---|---|---|---|---|
| ||||
No. | 263 | 513 | 0 | |
Role, n (%) | <0.01 | 0 | ||
Frontline hospitalist | 201 (76) | 337 (66) | ||
Hospitalist leader | 53 (24) | 176 (34) | ||
Age, y, mean (SD) | 42 (8) | 45 (9) | <0.01 | 67 |
Years in current job, mean (SD) | 5 (4) | 6 (5) | 0.07 | 14 |
Specialty, n (%) | <0.01 | 0 | ||
Internal medicine | 160 (61) | 369 (72) | ||
Pediatrics | 56 (21) | 57 (11) | ||
Other | 39 (15) | 47 (9) | ||
Family medicine | 8 (3) | 40 (8) | ||
Practice model, n (%) | 0.02 | 19 | ||
Hospital employed | 110 (43) | 227 (46) | ||
Multispecialty group | 44 (17) | 68 (14) | ||
University/medical school | 47 (18) | 58 (12) | ||
Multistate group | 27 (11) | 73 (15) | ||
Local hospitalist group | 22 (8) | 65 (13) | ||
Other | 7 (3) | 9 (2) | ||
Practice region, n (%) | 0.14 | 0 | ||
Southeast | 56 (21) | 151 (29) | ||
Midwest | 58 (22) | 106 (21) | ||
Northeast | 54 (21) | 96 (19) | ||
Southwest | 44 (17) | 83 (16) | ||
West | 51 (19) | 77 (15) | ||
Full‐time equivalents, n (%) | <0.01 | 42 | ||
<100% | 46 (18) | 60 (12) | ||
100% | 202 (81) | 402 (83) | ||
>100% | 2 (1) | 22 (5) | ||
Days per month doing clinical work if FTE 100%, median (IQR) | 15 (1418) | 16 (1420) | 0.12 | 11 |
Hours per day doing clinical work, median (IQR) | 11 (912) | 11 (912) | 0.67 | 30 |
Consecutive days doing clinical work, median (IQR) | 7 (57) | 7 (57) | 0.94 | 17 |
Percentage of work at night, median (IQR) | 15 (530) | 15 (525) | 0.45 | 16 |
Percentage of night work in hospital if working nights, median (IQR) | 100 (5100) | 100 (10100) | 0.12 | 8 |
Hours per month doing nonclinical work, median (IQR) | 12 (540) | 15 (540) | 0.77 | 26 |
Estimated daily billable encounters, mean (IQR) | 14 (1116) | 15 (1218) | 0.01 | 54 |
Total earnings in fiscal year 2009, median US$1,000 (IQR) | 185 (150210) | 202 (180240) | <0.01 | 56 |
Marriage/domestic partnership status, n (%) | 0.15 | 43 | ||
Married/currently in DP | 197 (80) | 421 (86) | ||
Never married/never in DP | 26 (11) | 42 (9) | ||
Divorced or separated | 18 (7) | 20 (4) | ||
Other | 4 (2) | 5 (1) | ||
Dependent children under 7 years old living in home, n (%) | 0.22 | 42 | ||
0 | 136 (55) | 265 (54) | ||
1 | 47 (19) | 92 (19) | ||
2 | 52 (21) | 87 (18) | ||
3 | 12 (5) | 43 (9) |
Several gender differences were seen in the characteristics of hospitalists and their work (Table 1). Women compared to men hospitalists were less likely to be leaders, more likely to be pediatricians, work in university settings, and practice in Western states. Women compared to men, on average, were younger by 3 years, worked fewer full‐time equivalents (FTEs), worked a greater percentage of nights, and reported fewer billable encounters per shift. They were also more likely to be divorced or separated.
Job satisfaction priorities differed for women and men hospitalists. Table 2 lists job satisfaction domains in descending order of the frequency prioritized by men. The largest proportion of women and men prioritized optimal workload. However, although substantial pay was prioritized next most frequently by men, more women prioritized collegiality and control over personal time than substantial pay.
Women, % | Rank | Men, % | Rank | |
---|---|---|---|---|
Optimal workload | 59 | 1 | 59 | 1 |
Substantial pay | 41 | 4 | 50 | 2 |
Control over personal time | 44 | 3 | 41 | 3 |
Collegiality with physicians | 47 | 2 | 38 | 4 |
Rewarding relationships with patients | 35 | 5 | 34 | 5 |
Organizational climate of trust and belonging | 27 | 7 | 33 | 6 |
Ample availability of resources to do job | 24 | 9 | 27 | 7 |
Optimal autonomy | 26 | 8 | 24 | 8 |
Fairness within organization | 15 | 10 | 23 | 9 |
Optimal variety of tasks | 29 | 6 | 22 | 10 |
Recognition by leaders | 11 | 12 | 10 | 11 |
Satisfaction with nurses | 12 | 11 | 7 | 12 |
Key differences in individual characteristics, work patterns, and indicating substantial pay as a priority were associated with self‐reported total earnings in 2009 from respondents' work as a hospitalist. As shown in Table 3, the inclusion of detailed productivity measures such as FTE, days of monthly clinical work, and estimated number of daily billable encounters yielded a model that explained 33% of variance in earnings. After adjusting for significant covariates including pediatric specialty, practice model, geography, and amount and type of clinical work, the estimated underpayment of women compared to men was $14,581. Hospitalists who prioritized substantial pay earned $10,771 more than those who did not. The female x leader interaction term testing the hypothesis that gender disparity is greater among leaders than frontline hospitalists was not statistically significant ($16,720, P = 0.087) and excluded from the final model.
Differences in Salary, 2009 US$ (95% CI) | P Value | |
---|---|---|
| ||
Women | 14,581 (23,074 to 6,089) | <0.01 |
Leader | 21,997 (13,313 to 30,682) | <0.01 |
Prioritized substantial pay | 10,771 (2,651 to 18,891) | <0.01 |
Pediatric specialty | 31,126 (43,007 to 19,244) | <0.01 |
Practice model | ||
Hospital employed | REF | |
Multispecialty group | 1,922 (13,891 to 10,047) | 0.75 |
University/medical school | 33,503 (46,336 to 20,671) | <0.01 |
Multistate group | 6,505 (72,69 to 20,279) | 0.35 |
Local hospitalist group | 9,330 (4,352 to 23,012) | 0.18 |
Other | 17,364 (45,741 to 11,012) | 0.23 |
Practice region | ||
Southeast | REF | |
Midwest | 1,225 (10,595 to 13,044) | 0.84 |
Northeast | 15,712 (28,182 to 3,242) | 0.01 |
Southwest | 722 (13,545 to 12,101) | 0.91 |
West | 5,251 (7,383 to 17,885) | 0.41 |
FTE | 1,021 (762 to 1,279) | <0.01 |
Days per month doing clinical work | 1,209 (443 to 1,975) | <0.01 |
Estimated daily billable encounters | 608 (20 to 1,196) | 0.04 |
DISCUSSION
In a national stratified sample of US hospitalists, we found gender differences in job satisfaction priorities and hospitalist work characteristics. We also confirmed the persistence of a substantial gender earnings disparity. Lower earnings among women compared to men hospitalists were present in our data after controlling for age, pediatric specialty, practice model, geography, type of clinical work, and productivity measures. The gender earnings disparity noted in 1999[6] persists, although it appears to have decreased, possibly indicating progress toward equity. We showed that women hospitalists' relative tendency not to prioritize pay explains a significant portion of the residual income gap.
Hoff examined hospitalist earnings in a large national survey of hospitalists in 1999. Our cohorts differed in age and experience (both lower in the Hoff study). An estimated $24,000 ($124,266 vs. $148,132) earnings gap between women and men was greater than our estimate of $14,581 following an interval of 10 years. Although survey items differed, both studies found that women were less interested in pay than men when considering a hospitalist job. Hoff also found that work setting and attitudes about pay and lifestyle were significantly related to earnings. We extended the previous analyses of gender differences in job satisfaction priorities, work, and demographic characteristics to explain the earnings gap and understand how it may be remedied.
When considering job satisfaction, we found that more men than women prioritized substantial pay and that prioritization of substantial pay was directly related to higher earnings. Therefore, fewer women prioritizing pay partly explains women's lower earnings. Reasons for why fewer women prioritize pay were not assessed in this study but may include factors like being part of 2‐income households and competing commitments.[29] Priorities may even be influenced by empirically observed gender differences in discussions of financial matters, governed by cultural norms. Such norms may implicitly sanction employers to offer women less pay than men for the same or similar work. Women may disadvantage themselves by negotiating less or less well than men for higher starting and promotion salaries. They may be perceived more negatively than men when they do negotiate pay, leading to unintended negative consequences such as loss of social networks, decreased likability, and even loss of job offers.[29, 30, 31, 32]
More women prioritized optimal variety of tasks (6th most prevalent among women and 10th among men). Women who highly rate optimal variety of tasks as a job satisfier may choose positions in which they teach, perform research, and participate in hospital committees and quality‐improvement work, but offer lower pay. Yet hours per month doing nonclinical work was not significantly different between men and women, nor associated with earnings differences in our earnings models. Understanding whether women self‐select into hospitalist jobs with like‐minded colleagues to achieve complementary fit or end up supplementing their skills with hospitalists with different priorities may inform strategies to reduce the gender income disparity.[5] Unlike disparities between various hospital medicine groups, systematic disparities within practices risk generating low levels of organizational fairness and burnout among employees.
Not surprisingly, productivity was positively associated with earnings but did not fully account for the gender earnings gap. Our data demonstrated that women, on average, were associated with work characteristics that expectedly generate less compensation. For example, women were younger, more often part time, academic, pediatric, less often leaders, and reported fewer billable encounters compared to men. These differences account for some of the earnings gap between men and women, but these factors were controlled for in the earnings model. In addition, our analysis may have underestimated the gap by not incorporating loss of fringe benefits from part‐time status and not comprehensively counting incentive pay associated with high productivity. Other work patterns more commonly associated with women suggest an imbalance in reimbursement. More women than men work nights that are often compensated at higher rates than daytime work, yet their average pay was less, suggesting that compensation for night work may need to be adjusted to reflect its unique burdens and responsibilities.[33]
Although the gender pay gap was not more extreme among leaders compared to frontline hospitalists in our data, the trend, nonetheless, underscores an important consideration. Whereas clinical work is paid for in mostly measurable ways, pay for leadership may be influenced by intangible factors such as reputation, negotiation, and confidence that may disadvantage women relative to men.[7, 19, 23, 34, 35, 36] Efforts to overcome implicit gender bias should be most effective when we consciously couple fair promotion of women to leadership with fair compensation commensurate with their male peers.[21]
Our data are vulnerable to nonresponse bias.[1, 4, 5] Post hoc analyses demonstrated that distributions of age, gender, practice model and region of our respondents were similar to other nationally representative cohorts of hospitalists. Consequently, we believe our data can make valid estimates about a nationally representative sample of hospitalists. However, we acknowledge several additional weaknesses of self‐reported data, including recall bias and accuracy of productivity figures, which were rounded to variable significant digits by respondents. Earnings analysis using this data was intended to be exploratory, but the findings echoed analyses using more authoritative data sources.[11] Still, we made inferences conservatively by adopting multiple imputation techniques for dealing with nonresponse surveys in adherence to established reporting guidelines.[25] We also note several limitations relevant to multiple imputations. The greater prevalence of missing data for survey items soliciting earnings and the number of billable encounters suggest they were not truly missing at random as assumed. However, we showed that missingness is unrelated to the variables under study, justifying use of the technique. The wider measures of variance derived from multiple imputations make us vulnerable to not detecting associations that may exist.
The gender earnings gap found in hospital medicine echoes the gap found in multiple medical specialties, including but not limited to pediatrics, academic medicine, gastroenterology, and plastic surgery.[7, 8, 9, 11, 12, 13, 37] Hospital medicine employment models and practice patterns have important structural differences compared to previously studied populations that could mitigate factors contributing to women physicians' lower earnings. However, despite well‐defined working hours, lack of control over the number of patient encounters per day and high prevalence of hospital‐employed practice models, the gender earnings gap persists. We showed that lower prioritization for pay may reflect the self‐selection of women into lower paying jobs. Unmeasured factors, including implicit bias and differences in negotiations, social networks and mentoring opportunities[38, 39] may also contribute to pay differences between men and women hospitalists. As hospital medicine tackles gender inequities and other disparities, strategies to assess and address fair physician compensation must be on the table.
- Society of Hospital Medicine Career Satisfaction Task F. Job characteristics, satisfaction, and burnout across hospitalist practice models. J Hosp Med. 2012;7(5):402–410. , , , , ;
- The emerging role of “hospitalists” in the American health care system. N Engl J Med. 1996;335(7):514–517. , .
- Organizational climate, stress, and error in primary care: The MEMO study. In: Henriksen K, Battles JB, Marks ES, Lewin DI, eds. Advances in Patient Safety: From Research to Implementation. Vol. 1. Research Findings. Rockville, MD; Agency for Healthcare Research and Quality; 2005. , , , et al.
- Worklife and satisfaction of hospitalists: toward flourishing careers. J Gen Intern Med. 2012;27(1):28–36. , , , , .
- Person‐job fit: an exploratory cross‐sectional analysis of hospitalists. J Hosp Med. 2013;8(2):96–101. , , , , .
- Doing the same and earning less: male and female physicians in a new medical specialty. Inquiry. 2004;41(3):301–315. .
- Compensation and advancement of women in academic medicine: Is there equity? Ann Intern Med. 2004;141(3):205–212. , , , .
- Differences in earnings between male and female physicians. N Engl J Med. 1996;334(15):960–964. .
- Gender differences in the salaries of physician researchers. JAMA. 2012;307(22):2410–2417. , , , , , .
- The work lives of women physicians results from the physician work life study. The SGIM Career Satisfaction Study Group. J Gen Intern Med. 2000;15(6):372–380. , , , , , .
- The $16,819 pay gap for newly trained physicians: the unexplained trend of men earning more than women. Health Aff (Millwood). 2011;30(2):193–201. , , , .
- Assessing gender equity in a large academic department of pediatrics. Acad Med. 2012;87(1):98–104. , , , .
- Gender disparity in the practice of gastroenterology: the first 5 years of a career. Am J Gastroenterol. 2005;100(2):259–264. , , , , , .
- H.R. 438, Fair Pay Act of 2013. 113th Congress (2013‐2014).
- Topics to ponder: part‐time practice and pay parity. Gend Med. 2010;7(4):350–356. , , , , .
- Understanding and finding mentorship: a review for junior faculty. J Palliat Med. 2010;13(11):1373–1379. , .
- Career development for women in academic medicine: Multiple interventions in a department of medicine. JAMA. 1996;276(11):898–905. , , , et al.
- Sex differences in academic advancement. Results of a national study of pediatricians. N Engl J Med. 1996;335(17):1282–1289. , , , , , .
- Promotion of women physicians in academic medicine. Glass ceiling or sticky floor? JAMA. 1995;273(13):1022–1025. , , , .
- Stories from early‐career women physicians who have left academic medicine: a qualitative study at a single institution. Acad Med. 2011;86(6):752–758. , , , , .
- Experiencing the culture of academic medicine: gender matters, a national study. J Gen Intern Med. 2013;28(2):201–207. , , , , .
- Why aren't there more women leaders in academic medicine? tHe views of clinical department chairs. Acad Med. 2001;76(5):453–465. , .
- The gender gap in executive compensation: the role of female directors and chief executive officers. Ann Am Acad Pol Soc Sci. 2012(639):258–278. .
- Keys to career satisfaction: insights from a survey of women pediatric surgeons. J Pediatr Surg. 2004;39(6):984–990. , , .
- Multiple imputation for missing data in epidemiological and clinical research: potential and pitfalls. BMJ. 2009;338:b2393. , , , et al.
- Imputation and Variance Estimation Software [computer program]. Ann Arbor, MI: Universtiy of Michigan; 2007.
- Multiple Imputation using chained equations: issues and guidance for practice. Stat Med. 2010;30(4):377–399. , , .
- State of Hospital Medicine: 2010 Report Based on 2009 Data. Englewood, CO and Philadelphia, PA: Medical Group Management Association and Society of Hospital Medicine; 2010.
- The Changing Face of Medicine: Women Doctors and the Evolution Of Health Care in America. Ithaca, NY: ILR Press/Cornell University Press; 2008. , .
- Women Don't Ask: Negotiation and the Gender Divide. Princeton, NJ: Princeton University Press; 2003. , .
- Negotiation in academic medicine: a necessary career skill. J Womens Health (Larchmt). 2007;16(2):235–244. , , , et al.
- Women and salary negotiation: the costs of self‐advocacy. Psychol Women Q. 2001;25:65–76. .
- State of Hospital Medicine: 2014 Report Based on 2013 Data. Englewood, CO and Philadelphia, PA: Medical Group Management Association and Society of Hospital Medicine; 2014.
- Interventions that affect gender bias in hiring: a systematic review. Acad Med. 2009;84(10):1440–1446. , , .
- Sociology. The gender gap in NIH grant applications. Science. 2008;322(5907):1472–1474. , .
- Organizational climate and family life: how these factors affect the status of women faculty at one medical school. Acad Med. 2009;84(1):87–94. , , , .
- Gender differences in the professional and private lives of plastic surgeons. Ann Plast Surg. 2010;64(6):775–779. , , .
- Gender and supportive co‐worker relations in the medical profession. Gend Work Organ. 2014;21(1):1–17. .
- Stuck in the out‐group: Jennifer can't grow up, Jane's invisible, and Janet's over the hill. J Womens Health (Larchmt). 2014;23(6):481–484. , .
Hospitalists are a growing workforce numbering over 40,000 physicians, one‐third of whom are women.[1] Flexibility of work schedules and control over personal time have been the traditional selling points of the specialty.[2] Multiple studies of physician work life reveal growing physician dissatisfaction and a high prevalence of burnout.[3] To mitigate burnout risk, leaders in hospital medicine recognize the importance of creating a sustainable profession that offers both job and career satisfaction as well as work‐life balance and, importantly, fairness within the work environment.[4] Although success in some of these endeavors has been realized sporadically, sustaining work‐life balance and fairness in the specialty remains a work in progress, whereas evidence of high job attrition and pay inequities remain.[5, 6]
Pay inequity for women relative to men continues to be pervasive in medicine, including among early‐career physicians, researchers, and various specialists.[6, 7, 8, 9, 10, 11, 12, 13] The earnings gap seems to persist for physicians, even as federal efforts such as the Fair Pay Act of 2013 and the Paycheck Fairness Act of 2014 aim to end wage discrimination.[11, 14] Differences in specialty, part‐time status, and practice type do not mitigate the disparity.[8, 10, 15] Additional explanations have been proposed to explain the variability, including gender differences in negotiating skills, lack of opportunities to join networks of influence within organizations, and implicit or explicit bias and discrimination.[12, 16, 17, 18, 19, 20]
The earnings gap is also a consequence of what is commonly called the glass ceiling.[18, 19] Most agree that obstacles to fair advancement of women include absence of collaborative environments and role models who have successfully achieved work‐life balance.[17, 20, 21, 22] Somewhat surprisingly, women leaders in medicine seem to suffer greater income disparity than nonleaders; this income gap is prevalent among leaders in other elite professions as well.[7, 23] It is unknown whether women physicians' emphasis on work‐life balance, seen repeatedly in surveys, explains any of the pay disparity.[24] Little research to date has examined whether work‐life priorities of women in hospital medicine differ from men.
In this study, we sought to examine differences in job priorities between men and women hospitalists. In particular, we examine the relative prioritization of substantial pay to job satisfaction. We also examined gender differences in work patterns and earnings to explore potential sources of the persistent gender earnings gap.
METHODS
We analyzed data from the 20092010 Hospital Medicine Physician Worklife Survey, the design of which is detailed elsewhere.[4] Briefly, a 118‐item survey was administered by mail to a stratified sample of hospitalists from the Society of Hospital Medicine database and 3 large multisite hospitalist groups. A single survey item asked respondents to identify up to 4 out of 12 most important domains to their satisfaction with a hospitalist job. The domains were distilled from focus groups of nationally representative hospitalists as described previously,[4] and the survey item allowed up to one‐third of these domains to be identified as respondents' personal priorities. The list included: optimal variety of tasks, optimal workload, substantial pay, collegiality with other physicians, recognition by leaders, rewarding relationships with patients, satisfaction with nurses, optimal autonomy, control over personal time, fairness within organization, ample availability of resources to do job, and organizational climate of trust and belonging. We tabulated and ranked the frequency with which respondents selected each satisfaction domain by gender. Due to the nonstandard format of the survey item, we a priori decided to analyze only responses that were completed as instructed.
We also used demographic data including detailed work characteristics, clinical and nonclinical workload, total pretax earnings in 2009 as a hospitalist, and self‐identification as leader of their hospital medicine group. Respondent characteristics were tabulated and gender differences were tested using the t test, rank sum test, and the Fischer exact test as appropriate. We also listed the number of nonrespondents for each item. In estimating gender differences in earnings, we opted to use multiple‐imputation techniques to more conservatively account for greater variance inherent in the presence of missing data. Consistent with existing guidelines,[25] we demonstrated that item responses were not missing monotonically by visually inspecting patterns of nonresponse. We further demonstrated that data were missing at random by showing that response patterns of completed survey items did not predict whether or not a given variable response was missing using logistic regression models. We found no significant differences between respondents with complete and missing data. We verified that appropriate regression models for each variable on every other variable converged. We used Stata 13.1 (StataCorp, College Station, TX) to perform multiple imputations using chain equations (mi impute chain) to create 10 imputed tables for 7 normally distributed continuous variables using the ordinary least squares method, 3 non‐normally distributed variables using the predictive mean matching method, 2 nonordinal categorical variables using the multinomial logit method, and 1 binary variable using the logit method.[26, 27] Gender, pediatric specialty status, region of practice, and whether or not respondents prioritized substantial pay for job satisfaction were used as regular variables without missing data points.
Differences in earnings were assessed using a multivariate ordinary linear regression model applied to the imputed datasets fitted by forward selection of explanatory variables using P < 0.20 in bivariate analysis for inclusion and manual backward elimination of all statistically nonsignificant variables. We tested the significance of the women leader interaction term in the final parsimonious model. We used the usual significance threshold of P < 0.05 for inferences. Our analysis of publicly available anonymous data was exempt from IRB review.
RESULTS
Of the 816 survey respondents (response rate 25.6%), 40 either omitted the item soliciting work priorities or completed it incorrectly. Data from the remaining 776 respondents were used for the present analysis. Respondent characteristics are tabulated in Table 1. The characteristics of hospitalists by age, gender, specialty, practice model, and practice region were representative of US hospitalists from other surveys.[28]
Women | Men | P Value | No. of Missing Responses | |
---|---|---|---|---|
| ||||
No. | 263 | 513 | 0 | |
Role, n (%) | <0.01 | 0 | ||
Frontline hospitalist | 201 (76) | 337 (66) | ||
Hospitalist leader | 53 (24) | 176 (34) | ||
Age, y, mean (SD) | 42 (8) | 45 (9) | <0.01 | 67 |
Years in current job, mean (SD) | 5 (4) | 6 (5) | 0.07 | 14 |
Specialty, n (%) | <0.01 | 0 | ||
Internal medicine | 160 (61) | 369 (72) | ||
Pediatrics | 56 (21) | 57 (11) | ||
Other | 39 (15) | 47 (9) | ||
Family medicine | 8 (3) | 40 (8) | ||
Practice model, n (%) | 0.02 | 19 | ||
Hospital employed | 110 (43) | 227 (46) | ||
Multispecialty group | 44 (17) | 68 (14) | ||
University/medical school | 47 (18) | 58 (12) | ||
Multistate group | 27 (11) | 73 (15) | ||
Local hospitalist group | 22 (8) | 65 (13) | ||
Other | 7 (3) | 9 (2) | ||
Practice region, n (%) | 0.14 | 0 | ||
Southeast | 56 (21) | 151 (29) | ||
Midwest | 58 (22) | 106 (21) | ||
Northeast | 54 (21) | 96 (19) | ||
Southwest | 44 (17) | 83 (16) | ||
West | 51 (19) | 77 (15) | ||
Full‐time equivalents, n (%) | <0.01 | 42 | ||
<100% | 46 (18) | 60 (12) | ||
100% | 202 (81) | 402 (83) | ||
>100% | 2 (1) | 22 (5) | ||
Days per month doing clinical work if FTE 100%, median (IQR) | 15 (1418) | 16 (1420) | 0.12 | 11 |
Hours per day doing clinical work, median (IQR) | 11 (912) | 11 (912) | 0.67 | 30 |
Consecutive days doing clinical work, median (IQR) | 7 (57) | 7 (57) | 0.94 | 17 |
Percentage of work at night, median (IQR) | 15 (530) | 15 (525) | 0.45 | 16 |
Percentage of night work in hospital if working nights, median (IQR) | 100 (5100) | 100 (10100) | 0.12 | 8 |
Hours per month doing nonclinical work, median (IQR) | 12 (540) | 15 (540) | 0.77 | 26 |
Estimated daily billable encounters, mean (IQR) | 14 (1116) | 15 (1218) | 0.01 | 54 |
Total earnings in fiscal year 2009, median US$1,000 (IQR) | 185 (150210) | 202 (180240) | <0.01 | 56 |
Marriage/domestic partnership status, n (%) | 0.15 | 43 | ||
Married/currently in DP | 197 (80) | 421 (86) | ||
Never married/never in DP | 26 (11) | 42 (9) | ||
Divorced or separated | 18 (7) | 20 (4) | ||
Other | 4 (2) | 5 (1) | ||
Dependent children under 7 years old living in home, n (%) | 0.22 | 42 | ||
0 | 136 (55) | 265 (54) | ||
1 | 47 (19) | 92 (19) | ||
2 | 52 (21) | 87 (18) | ||
3 | 12 (5) | 43 (9) |
Several gender differences were seen in the characteristics of hospitalists and their work (Table 1). Women compared to men hospitalists were less likely to be leaders, more likely to be pediatricians, work in university settings, and practice in Western states. Women compared to men, on average, were younger by 3 years, worked fewer full‐time equivalents (FTEs), worked a greater percentage of nights, and reported fewer billable encounters per shift. They were also more likely to be divorced or separated.
Job satisfaction priorities differed for women and men hospitalists. Table 2 lists job satisfaction domains in descending order of the frequency prioritized by men. The largest proportion of women and men prioritized optimal workload. However, although substantial pay was prioritized next most frequently by men, more women prioritized collegiality and control over personal time than substantial pay.
Women, % | Rank | Men, % | Rank | |
---|---|---|---|---|
Optimal workload | 59 | 1 | 59 | 1 |
Substantial pay | 41 | 4 | 50 | 2 |
Control over personal time | 44 | 3 | 41 | 3 |
Collegiality with physicians | 47 | 2 | 38 | 4 |
Rewarding relationships with patients | 35 | 5 | 34 | 5 |
Organizational climate of trust and belonging | 27 | 7 | 33 | 6 |
Ample availability of resources to do job | 24 | 9 | 27 | 7 |
Optimal autonomy | 26 | 8 | 24 | 8 |
Fairness within organization | 15 | 10 | 23 | 9 |
Optimal variety of tasks | 29 | 6 | 22 | 10 |
Recognition by leaders | 11 | 12 | 10 | 11 |
Satisfaction with nurses | 12 | 11 | 7 | 12 |
Key differences in individual characteristics, work patterns, and indicating substantial pay as a priority were associated with self‐reported total earnings in 2009 from respondents' work as a hospitalist. As shown in Table 3, the inclusion of detailed productivity measures such as FTE, days of monthly clinical work, and estimated number of daily billable encounters yielded a model that explained 33% of variance in earnings. After adjusting for significant covariates including pediatric specialty, practice model, geography, and amount and type of clinical work, the estimated underpayment of women compared to men was $14,581. Hospitalists who prioritized substantial pay earned $10,771 more than those who did not. The female x leader interaction term testing the hypothesis that gender disparity is greater among leaders than frontline hospitalists was not statistically significant ($16,720, P = 0.087) and excluded from the final model.
Differences in Salary, 2009 US$ (95% CI) | P Value | |
---|---|---|
| ||
Women | 14,581 (23,074 to 6,089) | <0.01 |
Leader | 21,997 (13,313 to 30,682) | <0.01 |
Prioritized substantial pay | 10,771 (2,651 to 18,891) | <0.01 |
Pediatric specialty | 31,126 (43,007 to 19,244) | <0.01 |
Practice model | ||
Hospital employed | REF | |
Multispecialty group | 1,922 (13,891 to 10,047) | 0.75 |
University/medical school | 33,503 (46,336 to 20,671) | <0.01 |
Multistate group | 6,505 (72,69 to 20,279) | 0.35 |
Local hospitalist group | 9,330 (4,352 to 23,012) | 0.18 |
Other | 17,364 (45,741 to 11,012) | 0.23 |
Practice region | ||
Southeast | REF | |
Midwest | 1,225 (10,595 to 13,044) | 0.84 |
Northeast | 15,712 (28,182 to 3,242) | 0.01 |
Southwest | 722 (13,545 to 12,101) | 0.91 |
West | 5,251 (7,383 to 17,885) | 0.41 |
FTE | 1,021 (762 to 1,279) | <0.01 |
Days per month doing clinical work | 1,209 (443 to 1,975) | <0.01 |
Estimated daily billable encounters | 608 (20 to 1,196) | 0.04 |
DISCUSSION
In a national stratified sample of US hospitalists, we found gender differences in job satisfaction priorities and hospitalist work characteristics. We also confirmed the persistence of a substantial gender earnings disparity. Lower earnings among women compared to men hospitalists were present in our data after controlling for age, pediatric specialty, practice model, geography, type of clinical work, and productivity measures. The gender earnings disparity noted in 1999[6] persists, although it appears to have decreased, possibly indicating progress toward equity. We showed that women hospitalists' relative tendency not to prioritize pay explains a significant portion of the residual income gap.
Hoff examined hospitalist earnings in a large national survey of hospitalists in 1999. Our cohorts differed in age and experience (both lower in the Hoff study). An estimated $24,000 ($124,266 vs. $148,132) earnings gap between women and men was greater than our estimate of $14,581 following an interval of 10 years. Although survey items differed, both studies found that women were less interested in pay than men when considering a hospitalist job. Hoff also found that work setting and attitudes about pay and lifestyle were significantly related to earnings. We extended the previous analyses of gender differences in job satisfaction priorities, work, and demographic characteristics to explain the earnings gap and understand how it may be remedied.
When considering job satisfaction, we found that more men than women prioritized substantial pay and that prioritization of substantial pay was directly related to higher earnings. Therefore, fewer women prioritizing pay partly explains women's lower earnings. Reasons for why fewer women prioritize pay were not assessed in this study but may include factors like being part of 2‐income households and competing commitments.[29] Priorities may even be influenced by empirically observed gender differences in discussions of financial matters, governed by cultural norms. Such norms may implicitly sanction employers to offer women less pay than men for the same or similar work. Women may disadvantage themselves by negotiating less or less well than men for higher starting and promotion salaries. They may be perceived more negatively than men when they do negotiate pay, leading to unintended negative consequences such as loss of social networks, decreased likability, and even loss of job offers.[29, 30, 31, 32]
More women prioritized optimal variety of tasks (6th most prevalent among women and 10th among men). Women who highly rate optimal variety of tasks as a job satisfier may choose positions in which they teach, perform research, and participate in hospital committees and quality‐improvement work, but offer lower pay. Yet hours per month doing nonclinical work was not significantly different between men and women, nor associated with earnings differences in our earnings models. Understanding whether women self‐select into hospitalist jobs with like‐minded colleagues to achieve complementary fit or end up supplementing their skills with hospitalists with different priorities may inform strategies to reduce the gender income disparity.[5] Unlike disparities between various hospital medicine groups, systematic disparities within practices risk generating low levels of organizational fairness and burnout among employees.
Not surprisingly, productivity was positively associated with earnings but did not fully account for the gender earnings gap. Our data demonstrated that women, on average, were associated with work characteristics that expectedly generate less compensation. For example, women were younger, more often part time, academic, pediatric, less often leaders, and reported fewer billable encounters compared to men. These differences account for some of the earnings gap between men and women, but these factors were controlled for in the earnings model. In addition, our analysis may have underestimated the gap by not incorporating loss of fringe benefits from part‐time status and not comprehensively counting incentive pay associated with high productivity. Other work patterns more commonly associated with women suggest an imbalance in reimbursement. More women than men work nights that are often compensated at higher rates than daytime work, yet their average pay was less, suggesting that compensation for night work may need to be adjusted to reflect its unique burdens and responsibilities.[33]
Although the gender pay gap was not more extreme among leaders compared to frontline hospitalists in our data, the trend, nonetheless, underscores an important consideration. Whereas clinical work is paid for in mostly measurable ways, pay for leadership may be influenced by intangible factors such as reputation, negotiation, and confidence that may disadvantage women relative to men.[7, 19, 23, 34, 35, 36] Efforts to overcome implicit gender bias should be most effective when we consciously couple fair promotion of women to leadership with fair compensation commensurate with their male peers.[21]
Our data are vulnerable to nonresponse bias.[1, 4, 5] Post hoc analyses demonstrated that distributions of age, gender, practice model and region of our respondents were similar to other nationally representative cohorts of hospitalists. Consequently, we believe our data can make valid estimates about a nationally representative sample of hospitalists. However, we acknowledge several additional weaknesses of self‐reported data, including recall bias and accuracy of productivity figures, which were rounded to variable significant digits by respondents. Earnings analysis using this data was intended to be exploratory, but the findings echoed analyses using more authoritative data sources.[11] Still, we made inferences conservatively by adopting multiple imputation techniques for dealing with nonresponse surveys in adherence to established reporting guidelines.[25] We also note several limitations relevant to multiple imputations. The greater prevalence of missing data for survey items soliciting earnings and the number of billable encounters suggest they were not truly missing at random as assumed. However, we showed that missingness is unrelated to the variables under study, justifying use of the technique. The wider measures of variance derived from multiple imputations make us vulnerable to not detecting associations that may exist.
The gender earnings gap found in hospital medicine echoes the gap found in multiple medical specialties, including but not limited to pediatrics, academic medicine, gastroenterology, and plastic surgery.[7, 8, 9, 11, 12, 13, 37] Hospital medicine employment models and practice patterns have important structural differences compared to previously studied populations that could mitigate factors contributing to women physicians' lower earnings. However, despite well‐defined working hours, lack of control over the number of patient encounters per day and high prevalence of hospital‐employed practice models, the gender earnings gap persists. We showed that lower prioritization for pay may reflect the self‐selection of women into lower paying jobs. Unmeasured factors, including implicit bias and differences in negotiations, social networks and mentoring opportunities[38, 39] may also contribute to pay differences between men and women hospitalists. As hospital medicine tackles gender inequities and other disparities, strategies to assess and address fair physician compensation must be on the table.
Hospitalists are a growing workforce numbering over 40,000 physicians, one‐third of whom are women.[1] Flexibility of work schedules and control over personal time have been the traditional selling points of the specialty.[2] Multiple studies of physician work life reveal growing physician dissatisfaction and a high prevalence of burnout.[3] To mitigate burnout risk, leaders in hospital medicine recognize the importance of creating a sustainable profession that offers both job and career satisfaction as well as work‐life balance and, importantly, fairness within the work environment.[4] Although success in some of these endeavors has been realized sporadically, sustaining work‐life balance and fairness in the specialty remains a work in progress, whereas evidence of high job attrition and pay inequities remain.[5, 6]
Pay inequity for women relative to men continues to be pervasive in medicine, including among early‐career physicians, researchers, and various specialists.[6, 7, 8, 9, 10, 11, 12, 13] The earnings gap seems to persist for physicians, even as federal efforts such as the Fair Pay Act of 2013 and the Paycheck Fairness Act of 2014 aim to end wage discrimination.[11, 14] Differences in specialty, part‐time status, and practice type do not mitigate the disparity.[8, 10, 15] Additional explanations have been proposed to explain the variability, including gender differences in negotiating skills, lack of opportunities to join networks of influence within organizations, and implicit or explicit bias and discrimination.[12, 16, 17, 18, 19, 20]
The earnings gap is also a consequence of what is commonly called the glass ceiling.[18, 19] Most agree that obstacles to fair advancement of women include absence of collaborative environments and role models who have successfully achieved work‐life balance.[17, 20, 21, 22] Somewhat surprisingly, women leaders in medicine seem to suffer greater income disparity than nonleaders; this income gap is prevalent among leaders in other elite professions as well.[7, 23] It is unknown whether women physicians' emphasis on work‐life balance, seen repeatedly in surveys, explains any of the pay disparity.[24] Little research to date has examined whether work‐life priorities of women in hospital medicine differ from men.
In this study, we sought to examine differences in job priorities between men and women hospitalists. In particular, we examine the relative prioritization of substantial pay to job satisfaction. We also examined gender differences in work patterns and earnings to explore potential sources of the persistent gender earnings gap.
METHODS
We analyzed data from the 20092010 Hospital Medicine Physician Worklife Survey, the design of which is detailed elsewhere.[4] Briefly, a 118‐item survey was administered by mail to a stratified sample of hospitalists from the Society of Hospital Medicine database and 3 large multisite hospitalist groups. A single survey item asked respondents to identify up to 4 out of 12 most important domains to their satisfaction with a hospitalist job. The domains were distilled from focus groups of nationally representative hospitalists as described previously,[4] and the survey item allowed up to one‐third of these domains to be identified as respondents' personal priorities. The list included: optimal variety of tasks, optimal workload, substantial pay, collegiality with other physicians, recognition by leaders, rewarding relationships with patients, satisfaction with nurses, optimal autonomy, control over personal time, fairness within organization, ample availability of resources to do job, and organizational climate of trust and belonging. We tabulated and ranked the frequency with which respondents selected each satisfaction domain by gender. Due to the nonstandard format of the survey item, we a priori decided to analyze only responses that were completed as instructed.
We also used demographic data including detailed work characteristics, clinical and nonclinical workload, total pretax earnings in 2009 as a hospitalist, and self‐identification as leader of their hospital medicine group. Respondent characteristics were tabulated and gender differences were tested using the t test, rank sum test, and the Fischer exact test as appropriate. We also listed the number of nonrespondents for each item. In estimating gender differences in earnings, we opted to use multiple‐imputation techniques to more conservatively account for greater variance inherent in the presence of missing data. Consistent with existing guidelines,[25] we demonstrated that item responses were not missing monotonically by visually inspecting patterns of nonresponse. We further demonstrated that data were missing at random by showing that response patterns of completed survey items did not predict whether or not a given variable response was missing using logistic regression models. We found no significant differences between respondents with complete and missing data. We verified that appropriate regression models for each variable on every other variable converged. We used Stata 13.1 (StataCorp, College Station, TX) to perform multiple imputations using chain equations (mi impute chain) to create 10 imputed tables for 7 normally distributed continuous variables using the ordinary least squares method, 3 non‐normally distributed variables using the predictive mean matching method, 2 nonordinal categorical variables using the multinomial logit method, and 1 binary variable using the logit method.[26, 27] Gender, pediatric specialty status, region of practice, and whether or not respondents prioritized substantial pay for job satisfaction were used as regular variables without missing data points.
Differences in earnings were assessed using a multivariate ordinary linear regression model applied to the imputed datasets fitted by forward selection of explanatory variables using P < 0.20 in bivariate analysis for inclusion and manual backward elimination of all statistically nonsignificant variables. We tested the significance of the women leader interaction term in the final parsimonious model. We used the usual significance threshold of P < 0.05 for inferences. Our analysis of publicly available anonymous data was exempt from IRB review.
RESULTS
Of the 816 survey respondents (response rate 25.6%), 40 either omitted the item soliciting work priorities or completed it incorrectly. Data from the remaining 776 respondents were used for the present analysis. Respondent characteristics are tabulated in Table 1. The characteristics of hospitalists by age, gender, specialty, practice model, and practice region were representative of US hospitalists from other surveys.[28]
Women | Men | P Value | No. of Missing Responses | |
---|---|---|---|---|
| ||||
No. | 263 | 513 | 0 | |
Role, n (%) | <0.01 | 0 | ||
Frontline hospitalist | 201 (76) | 337 (66) | ||
Hospitalist leader | 53 (24) | 176 (34) | ||
Age, y, mean (SD) | 42 (8) | 45 (9) | <0.01 | 67 |
Years in current job, mean (SD) | 5 (4) | 6 (5) | 0.07 | 14 |
Specialty, n (%) | <0.01 | 0 | ||
Internal medicine | 160 (61) | 369 (72) | ||
Pediatrics | 56 (21) | 57 (11) | ||
Other | 39 (15) | 47 (9) | ||
Family medicine | 8 (3) | 40 (8) | ||
Practice model, n (%) | 0.02 | 19 | ||
Hospital employed | 110 (43) | 227 (46) | ||
Multispecialty group | 44 (17) | 68 (14) | ||
University/medical school | 47 (18) | 58 (12) | ||
Multistate group | 27 (11) | 73 (15) | ||
Local hospitalist group | 22 (8) | 65 (13) | ||
Other | 7 (3) | 9 (2) | ||
Practice region, n (%) | 0.14 | 0 | ||
Southeast | 56 (21) | 151 (29) | ||
Midwest | 58 (22) | 106 (21) | ||
Northeast | 54 (21) | 96 (19) | ||
Southwest | 44 (17) | 83 (16) | ||
West | 51 (19) | 77 (15) | ||
Full‐time equivalents, n (%) | <0.01 | 42 | ||
<100% | 46 (18) | 60 (12) | ||
100% | 202 (81) | 402 (83) | ||
>100% | 2 (1) | 22 (5) | ||
Days per month doing clinical work if FTE 100%, median (IQR) | 15 (1418) | 16 (1420) | 0.12 | 11 |
Hours per day doing clinical work, median (IQR) | 11 (912) | 11 (912) | 0.67 | 30 |
Consecutive days doing clinical work, median (IQR) | 7 (57) | 7 (57) | 0.94 | 17 |
Percentage of work at night, median (IQR) | 15 (530) | 15 (525) | 0.45 | 16 |
Percentage of night work in hospital if working nights, median (IQR) | 100 (5100) | 100 (10100) | 0.12 | 8 |
Hours per month doing nonclinical work, median (IQR) | 12 (540) | 15 (540) | 0.77 | 26 |
Estimated daily billable encounters, mean (IQR) | 14 (1116) | 15 (1218) | 0.01 | 54 |
Total earnings in fiscal year 2009, median US$1,000 (IQR) | 185 (150210) | 202 (180240) | <0.01 | 56 |
Marriage/domestic partnership status, n (%) | 0.15 | 43 | ||
Married/currently in DP | 197 (80) | 421 (86) | ||
Never married/never in DP | 26 (11) | 42 (9) | ||
Divorced or separated | 18 (7) | 20 (4) | ||
Other | 4 (2) | 5 (1) | ||
Dependent children under 7 years old living in home, n (%) | 0.22 | 42 | ||
0 | 136 (55) | 265 (54) | ||
1 | 47 (19) | 92 (19) | ||
2 | 52 (21) | 87 (18) | ||
3 | 12 (5) | 43 (9) |
Several gender differences were seen in the characteristics of hospitalists and their work (Table 1). Women compared to men hospitalists were less likely to be leaders, more likely to be pediatricians, work in university settings, and practice in Western states. Women compared to men, on average, were younger by 3 years, worked fewer full‐time equivalents (FTEs), worked a greater percentage of nights, and reported fewer billable encounters per shift. They were also more likely to be divorced or separated.
Job satisfaction priorities differed for women and men hospitalists. Table 2 lists job satisfaction domains in descending order of the frequency prioritized by men. The largest proportion of women and men prioritized optimal workload. However, although substantial pay was prioritized next most frequently by men, more women prioritized collegiality and control over personal time than substantial pay.
Women, % | Rank | Men, % | Rank | |
---|---|---|---|---|
Optimal workload | 59 | 1 | 59 | 1 |
Substantial pay | 41 | 4 | 50 | 2 |
Control over personal time | 44 | 3 | 41 | 3 |
Collegiality with physicians | 47 | 2 | 38 | 4 |
Rewarding relationships with patients | 35 | 5 | 34 | 5 |
Organizational climate of trust and belonging | 27 | 7 | 33 | 6 |
Ample availability of resources to do job | 24 | 9 | 27 | 7 |
Optimal autonomy | 26 | 8 | 24 | 8 |
Fairness within organization | 15 | 10 | 23 | 9 |
Optimal variety of tasks | 29 | 6 | 22 | 10 |
Recognition by leaders | 11 | 12 | 10 | 11 |
Satisfaction with nurses | 12 | 11 | 7 | 12 |
Key differences in individual characteristics, work patterns, and indicating substantial pay as a priority were associated with self‐reported total earnings in 2009 from respondents' work as a hospitalist. As shown in Table 3, the inclusion of detailed productivity measures such as FTE, days of monthly clinical work, and estimated number of daily billable encounters yielded a model that explained 33% of variance in earnings. After adjusting for significant covariates including pediatric specialty, practice model, geography, and amount and type of clinical work, the estimated underpayment of women compared to men was $14,581. Hospitalists who prioritized substantial pay earned $10,771 more than those who did not. The female x leader interaction term testing the hypothesis that gender disparity is greater among leaders than frontline hospitalists was not statistically significant ($16,720, P = 0.087) and excluded from the final model.
Differences in Salary, 2009 US$ (95% CI) | P Value | |
---|---|---|
| ||
Women | 14,581 (23,074 to 6,089) | <0.01 |
Leader | 21,997 (13,313 to 30,682) | <0.01 |
Prioritized substantial pay | 10,771 (2,651 to 18,891) | <0.01 |
Pediatric specialty | 31,126 (43,007 to 19,244) | <0.01 |
Practice model | ||
Hospital employed | REF | |
Multispecialty group | 1,922 (13,891 to 10,047) | 0.75 |
University/medical school | 33,503 (46,336 to 20,671) | <0.01 |
Multistate group | 6,505 (72,69 to 20,279) | 0.35 |
Local hospitalist group | 9,330 (4,352 to 23,012) | 0.18 |
Other | 17,364 (45,741 to 11,012) | 0.23 |
Practice region | ||
Southeast | REF | |
Midwest | 1,225 (10,595 to 13,044) | 0.84 |
Northeast | 15,712 (28,182 to 3,242) | 0.01 |
Southwest | 722 (13,545 to 12,101) | 0.91 |
West | 5,251 (7,383 to 17,885) | 0.41 |
FTE | 1,021 (762 to 1,279) | <0.01 |
Days per month doing clinical work | 1,209 (443 to 1,975) | <0.01 |
Estimated daily billable encounters | 608 (20 to 1,196) | 0.04 |
DISCUSSION
In a national stratified sample of US hospitalists, we found gender differences in job satisfaction priorities and hospitalist work characteristics. We also confirmed the persistence of a substantial gender earnings disparity. Lower earnings among women compared to men hospitalists were present in our data after controlling for age, pediatric specialty, practice model, geography, type of clinical work, and productivity measures. The gender earnings disparity noted in 1999[6] persists, although it appears to have decreased, possibly indicating progress toward equity. We showed that women hospitalists' relative tendency not to prioritize pay explains a significant portion of the residual income gap.
Hoff examined hospitalist earnings in a large national survey of hospitalists in 1999. Our cohorts differed in age and experience (both lower in the Hoff study). An estimated $24,000 ($124,266 vs. $148,132) earnings gap between women and men was greater than our estimate of $14,581 following an interval of 10 years. Although survey items differed, both studies found that women were less interested in pay than men when considering a hospitalist job. Hoff also found that work setting and attitudes about pay and lifestyle were significantly related to earnings. We extended the previous analyses of gender differences in job satisfaction priorities, work, and demographic characteristics to explain the earnings gap and understand how it may be remedied.
When considering job satisfaction, we found that more men than women prioritized substantial pay and that prioritization of substantial pay was directly related to higher earnings. Therefore, fewer women prioritizing pay partly explains women's lower earnings. Reasons for why fewer women prioritize pay were not assessed in this study but may include factors like being part of 2‐income households and competing commitments.[29] Priorities may even be influenced by empirically observed gender differences in discussions of financial matters, governed by cultural norms. Such norms may implicitly sanction employers to offer women less pay than men for the same or similar work. Women may disadvantage themselves by negotiating less or less well than men for higher starting and promotion salaries. They may be perceived more negatively than men when they do negotiate pay, leading to unintended negative consequences such as loss of social networks, decreased likability, and even loss of job offers.[29, 30, 31, 32]
More women prioritized optimal variety of tasks (6th most prevalent among women and 10th among men). Women who highly rate optimal variety of tasks as a job satisfier may choose positions in which they teach, perform research, and participate in hospital committees and quality‐improvement work, but offer lower pay. Yet hours per month doing nonclinical work was not significantly different between men and women, nor associated with earnings differences in our earnings models. Understanding whether women self‐select into hospitalist jobs with like‐minded colleagues to achieve complementary fit or end up supplementing their skills with hospitalists with different priorities may inform strategies to reduce the gender income disparity.[5] Unlike disparities between various hospital medicine groups, systematic disparities within practices risk generating low levels of organizational fairness and burnout among employees.
Not surprisingly, productivity was positively associated with earnings but did not fully account for the gender earnings gap. Our data demonstrated that women, on average, were associated with work characteristics that expectedly generate less compensation. For example, women were younger, more often part time, academic, pediatric, less often leaders, and reported fewer billable encounters compared to men. These differences account for some of the earnings gap between men and women, but these factors were controlled for in the earnings model. In addition, our analysis may have underestimated the gap by not incorporating loss of fringe benefits from part‐time status and not comprehensively counting incentive pay associated with high productivity. Other work patterns more commonly associated with women suggest an imbalance in reimbursement. More women than men work nights that are often compensated at higher rates than daytime work, yet their average pay was less, suggesting that compensation for night work may need to be adjusted to reflect its unique burdens and responsibilities.[33]
Although the gender pay gap was not more extreme among leaders compared to frontline hospitalists in our data, the trend, nonetheless, underscores an important consideration. Whereas clinical work is paid for in mostly measurable ways, pay for leadership may be influenced by intangible factors such as reputation, negotiation, and confidence that may disadvantage women relative to men.[7, 19, 23, 34, 35, 36] Efforts to overcome implicit gender bias should be most effective when we consciously couple fair promotion of women to leadership with fair compensation commensurate with their male peers.[21]
Our data are vulnerable to nonresponse bias.[1, 4, 5] Post hoc analyses demonstrated that distributions of age, gender, practice model and region of our respondents were similar to other nationally representative cohorts of hospitalists. Consequently, we believe our data can make valid estimates about a nationally representative sample of hospitalists. However, we acknowledge several additional weaknesses of self‐reported data, including recall bias and accuracy of productivity figures, which were rounded to variable significant digits by respondents. Earnings analysis using this data was intended to be exploratory, but the findings echoed analyses using more authoritative data sources.[11] Still, we made inferences conservatively by adopting multiple imputation techniques for dealing with nonresponse surveys in adherence to established reporting guidelines.[25] We also note several limitations relevant to multiple imputations. The greater prevalence of missing data for survey items soliciting earnings and the number of billable encounters suggest they were not truly missing at random as assumed. However, we showed that missingness is unrelated to the variables under study, justifying use of the technique. The wider measures of variance derived from multiple imputations make us vulnerable to not detecting associations that may exist.
The gender earnings gap found in hospital medicine echoes the gap found in multiple medical specialties, including but not limited to pediatrics, academic medicine, gastroenterology, and plastic surgery.[7, 8, 9, 11, 12, 13, 37] Hospital medicine employment models and practice patterns have important structural differences compared to previously studied populations that could mitigate factors contributing to women physicians' lower earnings. However, despite well‐defined working hours, lack of control over the number of patient encounters per day and high prevalence of hospital‐employed practice models, the gender earnings gap persists. We showed that lower prioritization for pay may reflect the self‐selection of women into lower paying jobs. Unmeasured factors, including implicit bias and differences in negotiations, social networks and mentoring opportunities[38, 39] may also contribute to pay differences between men and women hospitalists. As hospital medicine tackles gender inequities and other disparities, strategies to assess and address fair physician compensation must be on the table.
- Society of Hospital Medicine Career Satisfaction Task F. Job characteristics, satisfaction, and burnout across hospitalist practice models. J Hosp Med. 2012;7(5):402–410. , , , , ;
- The emerging role of “hospitalists” in the American health care system. N Engl J Med. 1996;335(7):514–517. , .
- Organizational climate, stress, and error in primary care: The MEMO study. In: Henriksen K, Battles JB, Marks ES, Lewin DI, eds. Advances in Patient Safety: From Research to Implementation. Vol. 1. Research Findings. Rockville, MD; Agency for Healthcare Research and Quality; 2005. , , , et al.
- Worklife and satisfaction of hospitalists: toward flourishing careers. J Gen Intern Med. 2012;27(1):28–36. , , , , .
- Person‐job fit: an exploratory cross‐sectional analysis of hospitalists. J Hosp Med. 2013;8(2):96–101. , , , , .
- Doing the same and earning less: male and female physicians in a new medical specialty. Inquiry. 2004;41(3):301–315. .
- Compensation and advancement of women in academic medicine: Is there equity? Ann Intern Med. 2004;141(3):205–212. , , , .
- Differences in earnings between male and female physicians. N Engl J Med. 1996;334(15):960–964. .
- Gender differences in the salaries of physician researchers. JAMA. 2012;307(22):2410–2417. , , , , , .
- The work lives of women physicians results from the physician work life study. The SGIM Career Satisfaction Study Group. J Gen Intern Med. 2000;15(6):372–380. , , , , , .
- The $16,819 pay gap for newly trained physicians: the unexplained trend of men earning more than women. Health Aff (Millwood). 2011;30(2):193–201. , , , .
- Assessing gender equity in a large academic department of pediatrics. Acad Med. 2012;87(1):98–104. , , , .
- Gender disparity in the practice of gastroenterology: the first 5 years of a career. Am J Gastroenterol. 2005;100(2):259–264. , , , , , .
- H.R. 438, Fair Pay Act of 2013. 113th Congress (2013‐2014).
- Topics to ponder: part‐time practice and pay parity. Gend Med. 2010;7(4):350–356. , , , , .
- Understanding and finding mentorship: a review for junior faculty. J Palliat Med. 2010;13(11):1373–1379. , .
- Career development for women in academic medicine: Multiple interventions in a department of medicine. JAMA. 1996;276(11):898–905. , , , et al.
- Sex differences in academic advancement. Results of a national study of pediatricians. N Engl J Med. 1996;335(17):1282–1289. , , , , , .
- Promotion of women physicians in academic medicine. Glass ceiling or sticky floor? JAMA. 1995;273(13):1022–1025. , , , .
- Stories from early‐career women physicians who have left academic medicine: a qualitative study at a single institution. Acad Med. 2011;86(6):752–758. , , , , .
- Experiencing the culture of academic medicine: gender matters, a national study. J Gen Intern Med. 2013;28(2):201–207. , , , , .
- Why aren't there more women leaders in academic medicine? tHe views of clinical department chairs. Acad Med. 2001;76(5):453–465. , .
- The gender gap in executive compensation: the role of female directors and chief executive officers. Ann Am Acad Pol Soc Sci. 2012(639):258–278. .
- Keys to career satisfaction: insights from a survey of women pediatric surgeons. J Pediatr Surg. 2004;39(6):984–990. , , .
- Multiple imputation for missing data in epidemiological and clinical research: potential and pitfalls. BMJ. 2009;338:b2393. , , , et al.
- Imputation and Variance Estimation Software [computer program]. Ann Arbor, MI: Universtiy of Michigan; 2007.
- Multiple Imputation using chained equations: issues and guidance for practice. Stat Med. 2010;30(4):377–399. , , .
- State of Hospital Medicine: 2010 Report Based on 2009 Data. Englewood, CO and Philadelphia, PA: Medical Group Management Association and Society of Hospital Medicine; 2010.
- The Changing Face of Medicine: Women Doctors and the Evolution Of Health Care in America. Ithaca, NY: ILR Press/Cornell University Press; 2008. , .
- Women Don't Ask: Negotiation and the Gender Divide. Princeton, NJ: Princeton University Press; 2003. , .
- Negotiation in academic medicine: a necessary career skill. J Womens Health (Larchmt). 2007;16(2):235–244. , , , et al.
- Women and salary negotiation: the costs of self‐advocacy. Psychol Women Q. 2001;25:65–76. .
- State of Hospital Medicine: 2014 Report Based on 2013 Data. Englewood, CO and Philadelphia, PA: Medical Group Management Association and Society of Hospital Medicine; 2014.
- Interventions that affect gender bias in hiring: a systematic review. Acad Med. 2009;84(10):1440–1446. , , .
- Sociology. The gender gap in NIH grant applications. Science. 2008;322(5907):1472–1474. , .
- Organizational climate and family life: how these factors affect the status of women faculty at one medical school. Acad Med. 2009;84(1):87–94. , , , .
- Gender differences in the professional and private lives of plastic surgeons. Ann Plast Surg. 2010;64(6):775–779. , , .
- Gender and supportive co‐worker relations in the medical profession. Gend Work Organ. 2014;21(1):1–17. .
- Stuck in the out‐group: Jennifer can't grow up, Jane's invisible, and Janet's over the hill. J Womens Health (Larchmt). 2014;23(6):481–484. , .
- Society of Hospital Medicine Career Satisfaction Task F. Job characteristics, satisfaction, and burnout across hospitalist practice models. J Hosp Med. 2012;7(5):402–410. , , , , ;
- The emerging role of “hospitalists” in the American health care system. N Engl J Med. 1996;335(7):514–517. , .
- Organizational climate, stress, and error in primary care: The MEMO study. In: Henriksen K, Battles JB, Marks ES, Lewin DI, eds. Advances in Patient Safety: From Research to Implementation. Vol. 1. Research Findings. Rockville, MD; Agency for Healthcare Research and Quality; 2005. , , , et al.
- Worklife and satisfaction of hospitalists: toward flourishing careers. J Gen Intern Med. 2012;27(1):28–36. , , , , .
- Person‐job fit: an exploratory cross‐sectional analysis of hospitalists. J Hosp Med. 2013;8(2):96–101. , , , , .
- Doing the same and earning less: male and female physicians in a new medical specialty. Inquiry. 2004;41(3):301–315. .
- Compensation and advancement of women in academic medicine: Is there equity? Ann Intern Med. 2004;141(3):205–212. , , , .
- Differences in earnings between male and female physicians. N Engl J Med. 1996;334(15):960–964. .
- Gender differences in the salaries of physician researchers. JAMA. 2012;307(22):2410–2417. , , , , , .
- The work lives of women physicians results from the physician work life study. The SGIM Career Satisfaction Study Group. J Gen Intern Med. 2000;15(6):372–380. , , , , , .
- The $16,819 pay gap for newly trained physicians: the unexplained trend of men earning more than women. Health Aff (Millwood). 2011;30(2):193–201. , , , .
- Assessing gender equity in a large academic department of pediatrics. Acad Med. 2012;87(1):98–104. , , , .
- Gender disparity in the practice of gastroenterology: the first 5 years of a career. Am J Gastroenterol. 2005;100(2):259–264. , , , , , .
- H.R. 438, Fair Pay Act of 2013. 113th Congress (2013‐2014).
- Topics to ponder: part‐time practice and pay parity. Gend Med. 2010;7(4):350–356. , , , , .
- Understanding and finding mentorship: a review for junior faculty. J Palliat Med. 2010;13(11):1373–1379. , .
- Career development for women in academic medicine: Multiple interventions in a department of medicine. JAMA. 1996;276(11):898–905. , , , et al.
- Sex differences in academic advancement. Results of a national study of pediatricians. N Engl J Med. 1996;335(17):1282–1289. , , , , , .
- Promotion of women physicians in academic medicine. Glass ceiling or sticky floor? JAMA. 1995;273(13):1022–1025. , , , .
- Stories from early‐career women physicians who have left academic medicine: a qualitative study at a single institution. Acad Med. 2011;86(6):752–758. , , , , .
- Experiencing the culture of academic medicine: gender matters, a national study. J Gen Intern Med. 2013;28(2):201–207. , , , , .
- Why aren't there more women leaders in academic medicine? tHe views of clinical department chairs. Acad Med. 2001;76(5):453–465. , .
- The gender gap in executive compensation: the role of female directors and chief executive officers. Ann Am Acad Pol Soc Sci. 2012(639):258–278. .
- Keys to career satisfaction: insights from a survey of women pediatric surgeons. J Pediatr Surg. 2004;39(6):984–990. , , .
- Multiple imputation for missing data in epidemiological and clinical research: potential and pitfalls. BMJ. 2009;338:b2393. , , , et al.
- Imputation and Variance Estimation Software [computer program]. Ann Arbor, MI: Universtiy of Michigan; 2007.
- Multiple Imputation using chained equations: issues and guidance for practice. Stat Med. 2010;30(4):377–399. , , .
- State of Hospital Medicine: 2010 Report Based on 2009 Data. Englewood, CO and Philadelphia, PA: Medical Group Management Association and Society of Hospital Medicine; 2010.
- The Changing Face of Medicine: Women Doctors and the Evolution Of Health Care in America. Ithaca, NY: ILR Press/Cornell University Press; 2008. , .
- Women Don't Ask: Negotiation and the Gender Divide. Princeton, NJ: Princeton University Press; 2003. , .
- Negotiation in academic medicine: a necessary career skill. J Womens Health (Larchmt). 2007;16(2):235–244. , , , et al.
- Women and salary negotiation: the costs of self‐advocacy. Psychol Women Q. 2001;25:65–76. .
- State of Hospital Medicine: 2014 Report Based on 2013 Data. Englewood, CO and Philadelphia, PA: Medical Group Management Association and Society of Hospital Medicine; 2014.
- Interventions that affect gender bias in hiring: a systematic review. Acad Med. 2009;84(10):1440–1446. , , .
- Sociology. The gender gap in NIH grant applications. Science. 2008;322(5907):1472–1474. , .
- Organizational climate and family life: how these factors affect the status of women faculty at one medical school. Acad Med. 2009;84(1):87–94. , , , .
- Gender differences in the professional and private lives of plastic surgeons. Ann Plast Surg. 2010;64(6):775–779. , , .
- Gender and supportive co‐worker relations in the medical profession. Gend Work Organ. 2014;21(1):1–17. .
- Stuck in the out‐group: Jennifer can't grow up, Jane's invisible, and Janet's over the hill. J Womens Health (Larchmt). 2014;23(6):481–484. , .
© 2015 Society of Hospital Medicine
Hospitalist‐Job Fit
Person‐organization fit concerns the conditions and consequences of compatibility between people and the organizations for which they work.[1] Studies of other industries have demonstrated that person‐organization fit informs the way individuals join, perform in, and are retained by organizations.[2] Person‐job fit is a closely related subordinate concept that concerns the alignment of workers and their job in as much as workers have needs that their job supplies, or conversely, jobs have requirements that certain workers' abilities can help meet.[3] Explorations of job fit in physicians and their work have recently emerged in a few investigations published in medical journals.[4, 5, 6, 7, 8] Further expanding the understanding of fit between physicians and their employment is important, because the decline of solo practices and recent emphasis on team‐based care have led to a growing number of US physicians working in organizations.[9]
The movement of physicians into employed situations may continue if certain types of Accountable Care Organizations take root.[10] And physicians may be primed to join employer organizations based on current career priorities of individuals in American society. Surveys of medical residents entering the workforce reveal more physicians preferring the security of being employees than starting their own practices.[11] Given these trends, job fit will inform our understanding of how personal and job characteristics facilitate recruitment, performance, satisfaction, and longevity of physician employees.
BACKGROUND
Virtually all hospitalists work in organizationshospitalsand are employees of hospitals, medical schools, physician group practices, or management companies, and therefore invariably function within organizational structures and systems.[7] In spite of their rapid growth in numbers, many employers have faced difficulties recruiting and retaining enough hospitalists to fill their staffing needs. Consequently, the US hospitalist workforce today is characterized by high salaries, work load, and attrition rates.[12]
In this evolving unsaturated market, the attraction‐selection‐attrition framework[13] provides a theoretical construct that predicts that hospitalists and their employers would seek congruence of goals and values early in their relationship through a process of trial and error. This framework assumes that early interactions between workers and organizations serve as opportunities for them to understand if job fit is poor and dissociate or remain affiliated as long as job fit is mutually acceptable. Therefore, job switching on average is expected to increase job fit because workers and organizations gain a better understanding of their own goals and values and choose more wisely the next time.
Other theoretical frameworks, such as the job characteristic model,[14] suggest that over time as workers stay at the same job, they continue to maintain and improve job fit through various workplace‐ or self‐modification strategies. For example, seniority status may have privileges (eg, less undesirable call), or workers may create privileged niches through the acquisition of new skills and abilities over time. Hospitalists' tendency to diversify their work‐related activities by incorporating administrative and teaching responsibilities[15] may thus contribute to improving job fit. Additionally, as a measure of complementarity among people who work together, job fit may be influenced by the quality of relationships among hospitalists and their coworkers through their reorientation to the prevailing organizational climate[16, 17] and increasing socialization.[18] Finally, given that experiential learning is known to contribute to better hospitalist work performance,[19] job fit may affect productivity and clinical outcomes vis‐‐vis quality of work life.
To test the validity of these assumptions in a sample of hospitalists, we critically appraised the following 4 hypotheses:
- Hypothesis 1 (H1): Job attrition and reselection improves job fit among hospitalists entering the job market.
- Hypothesis 2 (H2): Better job fit is achieved through hospitalists engaging a variety of personal skills and abilities.
- Hypothesis 3 (H3): Job fit increases with hospitalists' job duration together with socialization and internalization of organizational values.
- Hypothesis 4 (H4): Job fit is correlated with hospitalists' quality of work life.
METHODS
Analysis was performed on data from the 2009 to 2010 Hospital Medicine Physician Worklife Survey. The sample frame included nonmembers and members of Society of Hospital Medicine (SHM). Details about sampling strategy, data collection, and data quality are available in previous publications.[7, 20] The 118‐item survey instrument, including 9 demographic items and 24 practice and job characteristic items, was administered by mail. Examples of information solicited through these items included respondents' practice model, the number of hospitalist jobs they have held, and the specific kinds of clinical and nonclinical activities they performed as part of their current job.
We used a reliable but broad and generic measure of self‐perceived person‐job fit.[21] The survey items of the 5‐point Likert‐type scale anchored between strongly disagree and strongly agree were: I feel that my work utilizes my full abilities, I feel competent and fully able to handle my job, my job gives me the chance to do the things I feel I do best, I feel that my job and I are well‐matched, I feel I have adequate preparation for the job I now hold. The quality of hospitalists' relationships with physician colleagues, staff, and patients as well as job satisfaction was measured using scales adapted from the Physician Worklife Study.[22] Organizational climate was measured using an adapted scale from the Minimizing Error, Maximizing Outcome study incorporating 3 items from the cohesiveness subscale, 4 items from the organizational trust subscale, and 1 item from the quality emphasis subscale that were most pertinent to hospitalists' relationship with their organizations.[23] Intent to leave practice or reduce work hours was measured using 5 items from the Multi‐Center Hospitalist Survey Project.[24] Frequency of participation in suboptimal patient care was measured by adapting 3 items from the suboptimal reported practice subscale and 2 items from the suboptimal patient care subscale developed by Shanafelt et al.[25] Stress and job burnout were assessed using validated measures.[26, 27] Detailed descriptions of the response rate calculation and imputation of missing item data are available in previous publications.[7, 20]
Mean, variance, range, and skew were used to characterize the responses to the job fit survey scale. A table of respondent characteristics was constructed. A visual representation of job fit by individual hospitalist year in current practice was created, first, by plotting a locally weighted scatterplot smoothing curve to examine the shape of the general relationship, and second, by fitting a similarly contoured functional polynomial curve with 95% confidence intervals (CI) to a plot of the mean and interquartile range of job fit for each year in current practice. Spearman partial correlations were calculated for job fit and each of the 5 items addressing likelihood of leaving practice or reducing workload adjusted for gender to control for the higher proportion of women who plan to work part time. Median (interquartile range) job fit was calculated for categories defined by the number of job changes and compared with the reference category (no job change) using the nonparametric rank sum test for comparing non‐normally distributed data. Multivariate logistic regression models were used to calculate the odds ratio (OR) of participating in each of several clinical and nonclinical hospitalist activities between respondents whose job fit score was optimal (5 on a 5‐point scale) and less than optimal controlling for covariates that influence the likelihood of participating in these activities (years in current practice, practice model, and specialty training). A Spearman correlation matrix was created to assess interscale correlations among organizational parameters (years in current practice, job fit, organizational climate, and relationship with colleagues, staff, and patients). Finally, a separate Spearman correlation matrix was created to assess the interscale correlations among individual worker parameters (job fit, suboptimal patient care, job burnout, stress, and job satisfaction). Statistical significance was defined as P value <0.05, and all analyses were performed on Stata 11.0 (StataCorp, College Station, TX). The Northwestern University institutional review board approved this study.
RESULTS
Respondents included 816 hospitalists belonging to around 700 unique organizations. The adjusted response rate from the stratified sample was 26%. Respondents and nonrespondents were similar with regard to geographic region and model of practice, but respondents were more likely to be members of the SHM than nonrespondents. Panel A of Table 1 shows the demographic characteristics of the respondents. The mean age was 44.3 years, and about one‐third were women. The average hospitalist had about 7 years of experience in the specialty and about 5 years with their current hospitalist job. The majority were trained in internal medicine or one of its subspecialties, whereas pediatricians, family physicians, and physicians with other training made up the remainder.
Panel A | Panel B | ||
---|---|---|---|
Total | Assimilation Period Hospitalists | Advancement Period Hospitalists | |
| |||
Total, n | 816 | 103 | 713 |
Female, n (%) | 284 (35) | 37 (36) | 247 (35) |
Age, mean (SD) | 44.3 (9.0) | 41.9 (9.3) | 44.7 (8.9) |
Years postresidency experience as hospitalist, mean (SD) | 6.9 (4.5) | 4.3 (3.1) | 7.2 (4.6) |
Years in current practice, mean (SD) | 5.1 (3.9) | 0.9 (0.3) | 6.7 (3.8) |
Specialty training, n (%) | |||
Internal medicine | 555 (68) | 75 (73) | 480 (67) |
Pediatrics | 117 (14) | 8 (8) | 109 (15.3) |
Family medicine | 49 (6) | 7 (7) | 42 (6) |
Other | 95 (11) | 13 (13) | 82 (12) |
Job fit was highly skewed toward optimum fit, with a mean of 4.3 on a scale of 1 to 5, with a narrow standard deviation of 0.7. The poorest job fit was reported by 0.3%, whereas optimal fit was reported by 21% of respondents. Job fit plotted against years in current practice had a logarithmic appearance typical of learning curves (Figure 1). An inflection point was visualized at around 2 years. For the purposes of this article, we refer to hospitalists' experience in the first 2 years of a job as an assimilation period, which is marked by a steep increase in job fit early when rapid learning or attrition took place. The years beyond the inflection point are characterized as an advancement period, when a more attenuated rise in job fit was experienced with time. The Spearman correlation between job fit and years in practice during the advancement period was 0.145 (n = 678, P < 0.001). Panel B of Table 1 displays the characteristics of respondents separately for the assimilation and advancement cohorts. Assimilation hospitalists in our sample had a mean age of 41.9 years and mean on‐the‐job experience of 4.3 years, reflecting that many hospitalists in the first 2 years of a job have made at least 1 job change in the past.
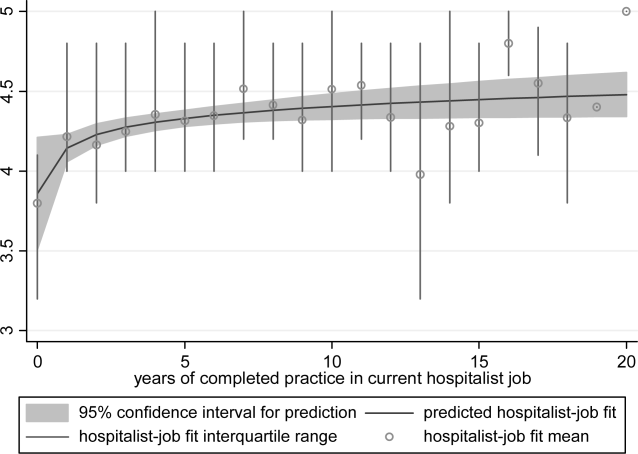
To show the effects of attrition and reselection, we first evaluated the proposition that hospitalists experience attrition (ie, intend to leave their jobs) in response to poor fit. Table 2 shows the correlations between job fit and the self‐reported intent to leave practice or reduce workload separately for the assimilation and advancement periods. For hospitalists in the assimilation period, job fit was negatively correlated with intent to leave current practice within 2 years and to leave hospital medicine within 5 years (P = 0.010 and 0.043, respectively). Hospitalists with <2 years in their current job, therefore, tended to consider attrition but not workload reduction to deal with poor job fit. On the other hand, hospitalists in the advancement period considered both attrition and workload reduction strategies in response to poor fit (all P < 0.001).
Assimilation Period Hospitalists | Advancement Period Hospitalists | |||
---|---|---|---|---|
Rho | P Value | Rho | P Value | |
Likelihood that a hospitalist will: | ||||
Leave current practice within 2 years | 0.253 | 0.010 | 0.367 | <0.001 |
Decrease total work hours within 5 years | 0.060 | 0.548 | 0.179 | <0.001 |
Decrease clinical work hours within 5 years | 0.072 | 0.469 | 0.144 | <0.001 |
Leave hospital medicine within 5 years | 0.200 | 0.043 | 0.231 | <0.001 |
Leave direct patient care within 5 years | 0.040 | 0.691 | 0.212 | <0.001 |
In Table 3, we further compared the median job fit across categories for the number of job switches. The median job fit during the assimilation period of hospitalists who had made 1 job change was slightly but statistically higher than the job fit of their counterparts who never left their first job (4.4 vs 4.0, P = 0.046). This suggests that job switching by hospitalists early in their jobs is associated with improved job fit (H1). However, the fit during the assimilation period of hospitalists who switched jobs twice or more was statistically no different from the fit of those in their first jobs, suggesting that the effect of the attrition‐reselection strategy is weak or inconsistent. The job fit for advancement period hospitalists was also different across the job change and no‐change categories. However, in the case of hospitalists later in their jobs, the median job fit was slightly but statistically lower among those who made job changes, revealing the potential drop in job fit that occurs when a hospitalist already established in his or her job starts over again in a new setting.
n | Age, Mean (95% CI), y | Hospitalist‐Job Fit, Median (IQR) | P Valuea | |
---|---|---|---|---|
| ||||
Assimilation period hospitalistsb | ||||
No job change | 29 | 42.3 (37.347.3) | 4.0 (3.84.4) | Reference |
1 job change | 39 | 40.3 (38.142.5) | 4.4 (4.04.8) | 0.046 |
2 or more job changes | 27 | 43.8 (41.046.6) | 4.4 (3.84.8) | 0.153 |
Advancement period hospitalistsc | ||||
No job change | 390 | 44.5 (43.645.5) | 4.6 (4.05.0) | Reference |
1 job change | 183 | 45.0 (43.746.3) | 4.2 (4.04.8) | 0.002 |
2 or more job changes | 99 | 44.9 (43.146.6) | 4.2 (3.84.8) | 0.002 |
We hypothesized that hospitalists who achieved high job fit within a particular job were more likely to have engaged in activities that utilize a wider spectrum of their abilities. As shown in Table 4, hospitalists in the highest quartile of job fit were associated with a general trend toward higher odds of participating in a variety of common clinical and nonclinical hospitalist activities, but only the odds ratio associated with teaching achieved statistical significance (OR: 1.53, 95% CI: 1.01‐2.31) (H2).
Participation, n/N (%) | Odds Ratio (95% CI) | P Value | |
---|---|---|---|
| |||
Administrative or committee work | 704/816 (86) | 0.73 (0.431.26) | 0.262 |
Quality improvement or patient safety initiatives | 678/816 (83) | 1.13 (0.642.00) | 0.680 |
Information technology design or implementation | 379/816 (46) | 1.18 (0.801.73) | 0.408 |
Any of the above leadership activities | 758/816 (93) | 1.31 (0.563.05) | 0.535 |
Teaching | 442/816 (54) | 1.53 (1.012.31) | 0.046 |
Research | 120/816 (15) | 1.07 (0.601.92) | 0.816 |
Any of the above academic activities | 457/816 (56) | 1.50 (0.992.27) | 0.057 |
Code team or rapid response team | 437/816 (54) | 1.13 (0.771.68) | 0.533 |
Intensive care unit | 254/816 (31) | 0.84 (0.531.35) | 0.469 |
Skilled nursing facility or long‐term acute care facility | 126/816 (15) | 1.06 (0.621.81) | 0.835 |
Outpatient general medical practice | 44/816 (5) | 1.75 (0.813.80) | 0.157 |
Any of the above clinical activities | 681/816 (79) | 1.02 (0.601.76) | 0.930 |
Socialization with peers and the gradual sharing of values within organizations are hypothesized mechanisms for increasing job fit with time. We found that the number of years in current practice was positively correlated with job fit (Spearman coefficient R = 0.149, P < 0.001), organizational climate (R = 0.128, P < 0.001), and relationship with nonphysician staff (R = 0.102, P < 0.01). The association between years in practice and relationship with physician colleagues were weaker (R = 0.079, P < 0.05). Consistent with the episodic nature of patients' encounters with hospitalists, the measure of patient relationships was not significantly associated with length of time in job. In addition, we found substantial correlations among job fit, organizational climate, and all the relational measures (all R > 0.280, P < 0.001), indicating that hospitalists increasingly share the values of their organizations over time (H3).
Finally, we also hypothesized that poor job fit is associated with poor performance and quality of work life. Strong correlations with job fit were noted for stress (R = 0.307, P < 0.001), job burnout (R = 0.360, P < 0.001), and job satisfaction (R = 0.570, P < 0.001). Job fit (R = 0.147, P < 0.001), job burnout (R = 0.236, P < 0.001), stress (R = 0.305, P < 0.001), and job satisfaction (R = 0.224, P < 0.001) were all significantly correlated with the frequency of participating in suboptimal care (H4).
DISCUSSION
In this exploratory analysis, we validated in the hospitalist workforce several assumptions about person‐job fit that have been observed in workers of other industries. We observed attrition‐reselection (ie, job switching) as a strategy used by physicians to achieve better fit early in their job tenure, whereas job modification appeared to be more effective than attrition‐reselection among physicians already established in their jobs. We provided weak but plausible evidence that physicians with optimal job fit had a tendency to participate in activities (eg, teaching) that engage a wider set of interests and abilities. We also demonstrated the growth in hospitalists sharing the values of their organization through the time‐dependent associations among organizational climate, relational measures, and job fit. Finally, we found that physicians with suboptimal job fit were more likely to report poor performance in their work compared to those indicating optimal fit.
Our previous analysis of data from the Hospital Medicine Physician Worklife Survey exposed the widely variable work characteristics of hospitalist jobs in the US market and the equally variable preferences and priorities of individual hospitalists in selecting their work setting.[7] The implication of our present study is that hospitalists achieve the high levels of observed job fit using various strategies that aid their alignment with their employment. One of these strategies involves time, but physician longevity in practice may be both a determinant and product of good job fit. Although early job attrition may be necessary for fitting the right hospitalists to the right jobs, employers may appreciate the importance of retaining experienced hospitalists not only for cost and performance considerations but also for the growth of social capital in organizations consisting of enduring individuals. As our data suggest that hospitalists grow with their jobs, physicians may experience better fit with jobs that flexibly couple their work demands with benefits that address their individual work‐life needs over time. Another implication of this study is that job fit is a useful and predictive measure of job selection, performance, and retention. In light of studies that expose the limitations of job satisfaction as a measure influenced more by workers' dispositional affect (ie, their temperament and outlook) than their compatibility with their jobs,[28] job fit may add a functional dimension to traditional employee feedback measures.
There are limitations to this analysis. The most notable is the low survey response rate. Two reasons contributed to the fairly low rate of return. First, the original sampling frame included many outdated addresses and names of individuals who did not meet inclusion criteria. Although all sampled individuals who would have been excluded from the study could not be identified, we calculated our response rate without accounting for the proportion of potential ineligibles in the denominator population [Response Rate 2 (RR2) according to standards of the American Association of Public Opinion Research].[29] Second, the response rates of physician surveys have seen a steady decline over the years.[30] Respondents to our survey may be older and more experienced than US hospitalists in general. Although concerns about bias from under‐reporting cannot be fully addressed, we believe that the study sample is adequate for this preliminary study intended to translate the evidence of observed phenomena from the nonphysician to the physician workforces. The suboptimal response characteristics (high skew and low variability) of the generic person‐job fit survey scale used in this study indicate that a reliable survey instrument specifically designed to measure physician‐job fit need to be constructed de novo and validated for any future study. Although we performed simple analyses to support our assertions, few of our subanalyses may be underpowered, contributing to overinterpretation of the data. Additional empirical work is also necessary to assess the generalizability of this study's claims in other medical and surgical specialties. Such studies would also allow measurement of the sensitivity and specificity of physicians' self‐identification of poor job fit. Finally, additional investigations of this time‐dependent construct are more appropriately performed using a longitudinal study design to overcome the limitations inherent in this cross‐sectional analysis. Our conclusions about the time‐dependent features of job fit may be explained by other characteristics such as generational and cultural differences among hospitalists with varying experience.
As the US healthcare system reorganizes to bolster accountability,[31] we anticipate increasing interdependence between physicians and their employer organizations. Ultimately, the desired accountability in healthcare is likely to be obtained if physicians function not only as passive and interchangeable employees but as active stakeholders in the achievement of each organization's goals. A methodology for assessing the alignment of physicians and their jobs will continue to be important along the way.
Disclosure
Nothing to report.
- Person‐organization fit: an integrative review of its conceptualizations, measurement, and implications. Personnel Psychol. 1996;49:1–49. .
- Consequences of individuals' fit at work: a meta‐analysis of person‐job, person‐organization, person‐group, and person‐supervisor fit. Personnel Psychol. 2005;58(2):281–342. , , .
- Person‐job fit: a conceptual integration, literature review and methodological critique. In: Cooper CL, Robertson IT, eds. International Review of Industrial and Organizational Psychology. Vol.6. New York, NY:John Wiley 1991. .
- Organizational culture, person‐culture fit, and turnover: a replication in the health care industry. J Organ Behav. 1999;20(2):175–184. .
- Organizational culture and physician satisfaction with dimensions of group practice. Health Serv Res. 2007;42(3 pt 1):1150–1176. , , , .
- Career fit and burnout among academic faculty. Arch Intern Med. 2009;169(10):990–995. , , , et al.
- Job characteristics, satisfaction, and burnout across hospitalist practice models. J Hosp Med. 2012;7(5):402–410. , , , , .
- Provider‐hospital “fit” and patient outcomes: evidence from Massachusetts cardiac surgeons, 2002–2004. Health Serv Res. 2011;46(1 pt 1):1–26. .
- The evolving primary care physician. N Engl J Med. 2012;366(20):1849–1853. .
- Hospitals' race to employ physicians—the logic behind a money‐losing proposition. N Engl J Med. 2011;364(19):1790–1793. , .
- 2011 Survey of Final‐Year Medical Residents. Irving, TX:Merritt Hawkins;2011.
- State of Hospital Medicine: 2010 Report Based on 2009 Data. Englewood, CO:Society of Hospital Medicine and the Medical Group Management Association;2010.
- The ASA framework: an update. Personnel Psychol. 1995;48(4):747–773. , , .
- Work Redesign. Reading. MA:Addison‐Wesley;1980. , .
- The expanding role of hospitalists in the United States. Swiss Med Wkly. 2006;136(37–38):591–596. , .
- Organizational socialization as a learning‐process—the role of information acquisition. Personnel Psychol. 1992;45(4):849–874. , .
- The moderating effect of tenure in person‐environment fit: a field study in educational organizations. J Occup Organ Psych. 1997;70:173–188. , .
- Matching people and organizations—selection and socialization in public accounting firms. Admin Sci Quart. 1991;36(3):459–484. .
- Effects of physician experience on costs and outcomes on an academic general medicine service: results of a trial of hospitalists. Ann Intern Med. 2002;137(11):866–874. , , , et al.
- Worklife and satisfaction of hospitalists: toward flourishing careers. J Gen Intern Med. 2012;27(1):28–36. , , , , .
- Karasek's model in the People's Republic of China: effects of job demands, control, and individual differences. Acad Manage J. 1996;39(6):1594–1618. .
- Measuring physician job satisfaction in a changing workplace and a challenging environment. SGIM Career Satisfaction Study Group. Society of General Internal Medicine. Med Care. 1999;37(11):1174–1182. , , , et al.
- Organizational climate, stress, and error in primary care: The MEMO Study. Adv Patient Saf. 2005;1:65–77. , , , et al.
- Effects of inpatient experience on outcomes and costs in a multicenter trial of academic hospitalists. J Gen Intern Med. 2005;20(suppl 1):141–142. , , , et al.
- Burnout and self‐reported patient care in an internal medicine residency program. Ann Intern Med. 2002;136(5):358–367. , , , .
- Effect of job demands and social support on worker stress—a study of VDT users. Behav Inform Technol. 1995;14(1):32–40. , .
- Validation of a single‐item measure of burnout against the Maslach Burnout Inventory among physicians. Stress Health. 2004;20(2):75–79. , , .
- Job satisfaction: a meta‐analysis of stabilities. J Organ Behav. 2001;22(5):483–504. , .
- The American Association for Public Opinion Research. Standard definitions: final dispositions of case codes and outcome rates for surveys.7th ed. Available at: http://www.aapor.org/Standard_Definitions2.htm. Accessed May 2,2012.
- Response rates and response bias for 50 surveys of pediatricians. Health Serv Res. 2005;40(1):213–226. , , , .
- Accountable care organizations: accountable for what, to whom, and how. JAMA. 2010;304(15):1715–6. , .
Person‐organization fit concerns the conditions and consequences of compatibility between people and the organizations for which they work.[1] Studies of other industries have demonstrated that person‐organization fit informs the way individuals join, perform in, and are retained by organizations.[2] Person‐job fit is a closely related subordinate concept that concerns the alignment of workers and their job in as much as workers have needs that their job supplies, or conversely, jobs have requirements that certain workers' abilities can help meet.[3] Explorations of job fit in physicians and their work have recently emerged in a few investigations published in medical journals.[4, 5, 6, 7, 8] Further expanding the understanding of fit between physicians and their employment is important, because the decline of solo practices and recent emphasis on team‐based care have led to a growing number of US physicians working in organizations.[9]
The movement of physicians into employed situations may continue if certain types of Accountable Care Organizations take root.[10] And physicians may be primed to join employer organizations based on current career priorities of individuals in American society. Surveys of medical residents entering the workforce reveal more physicians preferring the security of being employees than starting their own practices.[11] Given these trends, job fit will inform our understanding of how personal and job characteristics facilitate recruitment, performance, satisfaction, and longevity of physician employees.
BACKGROUND
Virtually all hospitalists work in organizationshospitalsand are employees of hospitals, medical schools, physician group practices, or management companies, and therefore invariably function within organizational structures and systems.[7] In spite of their rapid growth in numbers, many employers have faced difficulties recruiting and retaining enough hospitalists to fill their staffing needs. Consequently, the US hospitalist workforce today is characterized by high salaries, work load, and attrition rates.[12]
In this evolving unsaturated market, the attraction‐selection‐attrition framework[13] provides a theoretical construct that predicts that hospitalists and their employers would seek congruence of goals and values early in their relationship through a process of trial and error. This framework assumes that early interactions between workers and organizations serve as opportunities for them to understand if job fit is poor and dissociate or remain affiliated as long as job fit is mutually acceptable. Therefore, job switching on average is expected to increase job fit because workers and organizations gain a better understanding of their own goals and values and choose more wisely the next time.
Other theoretical frameworks, such as the job characteristic model,[14] suggest that over time as workers stay at the same job, they continue to maintain and improve job fit through various workplace‐ or self‐modification strategies. For example, seniority status may have privileges (eg, less undesirable call), or workers may create privileged niches through the acquisition of new skills and abilities over time. Hospitalists' tendency to diversify their work‐related activities by incorporating administrative and teaching responsibilities[15] may thus contribute to improving job fit. Additionally, as a measure of complementarity among people who work together, job fit may be influenced by the quality of relationships among hospitalists and their coworkers through their reorientation to the prevailing organizational climate[16, 17] and increasing socialization.[18] Finally, given that experiential learning is known to contribute to better hospitalist work performance,[19] job fit may affect productivity and clinical outcomes vis‐‐vis quality of work life.
To test the validity of these assumptions in a sample of hospitalists, we critically appraised the following 4 hypotheses:
- Hypothesis 1 (H1): Job attrition and reselection improves job fit among hospitalists entering the job market.
- Hypothesis 2 (H2): Better job fit is achieved through hospitalists engaging a variety of personal skills and abilities.
- Hypothesis 3 (H3): Job fit increases with hospitalists' job duration together with socialization and internalization of organizational values.
- Hypothesis 4 (H4): Job fit is correlated with hospitalists' quality of work life.
METHODS
Analysis was performed on data from the 2009 to 2010 Hospital Medicine Physician Worklife Survey. The sample frame included nonmembers and members of Society of Hospital Medicine (SHM). Details about sampling strategy, data collection, and data quality are available in previous publications.[7, 20] The 118‐item survey instrument, including 9 demographic items and 24 practice and job characteristic items, was administered by mail. Examples of information solicited through these items included respondents' practice model, the number of hospitalist jobs they have held, and the specific kinds of clinical and nonclinical activities they performed as part of their current job.
We used a reliable but broad and generic measure of self‐perceived person‐job fit.[21] The survey items of the 5‐point Likert‐type scale anchored between strongly disagree and strongly agree were: I feel that my work utilizes my full abilities, I feel competent and fully able to handle my job, my job gives me the chance to do the things I feel I do best, I feel that my job and I are well‐matched, I feel I have adequate preparation for the job I now hold. The quality of hospitalists' relationships with physician colleagues, staff, and patients as well as job satisfaction was measured using scales adapted from the Physician Worklife Study.[22] Organizational climate was measured using an adapted scale from the Minimizing Error, Maximizing Outcome study incorporating 3 items from the cohesiveness subscale, 4 items from the organizational trust subscale, and 1 item from the quality emphasis subscale that were most pertinent to hospitalists' relationship with their organizations.[23] Intent to leave practice or reduce work hours was measured using 5 items from the Multi‐Center Hospitalist Survey Project.[24] Frequency of participation in suboptimal patient care was measured by adapting 3 items from the suboptimal reported practice subscale and 2 items from the suboptimal patient care subscale developed by Shanafelt et al.[25] Stress and job burnout were assessed using validated measures.[26, 27] Detailed descriptions of the response rate calculation and imputation of missing item data are available in previous publications.[7, 20]
Mean, variance, range, and skew were used to characterize the responses to the job fit survey scale. A table of respondent characteristics was constructed. A visual representation of job fit by individual hospitalist year in current practice was created, first, by plotting a locally weighted scatterplot smoothing curve to examine the shape of the general relationship, and second, by fitting a similarly contoured functional polynomial curve with 95% confidence intervals (CI) to a plot of the mean and interquartile range of job fit for each year in current practice. Spearman partial correlations were calculated for job fit and each of the 5 items addressing likelihood of leaving practice or reducing workload adjusted for gender to control for the higher proportion of women who plan to work part time. Median (interquartile range) job fit was calculated for categories defined by the number of job changes and compared with the reference category (no job change) using the nonparametric rank sum test for comparing non‐normally distributed data. Multivariate logistic regression models were used to calculate the odds ratio (OR) of participating in each of several clinical and nonclinical hospitalist activities between respondents whose job fit score was optimal (5 on a 5‐point scale) and less than optimal controlling for covariates that influence the likelihood of participating in these activities (years in current practice, practice model, and specialty training). A Spearman correlation matrix was created to assess interscale correlations among organizational parameters (years in current practice, job fit, organizational climate, and relationship with colleagues, staff, and patients). Finally, a separate Spearman correlation matrix was created to assess the interscale correlations among individual worker parameters (job fit, suboptimal patient care, job burnout, stress, and job satisfaction). Statistical significance was defined as P value <0.05, and all analyses were performed on Stata 11.0 (StataCorp, College Station, TX). The Northwestern University institutional review board approved this study.
RESULTS
Respondents included 816 hospitalists belonging to around 700 unique organizations. The adjusted response rate from the stratified sample was 26%. Respondents and nonrespondents were similar with regard to geographic region and model of practice, but respondents were more likely to be members of the SHM than nonrespondents. Panel A of Table 1 shows the demographic characteristics of the respondents. The mean age was 44.3 years, and about one‐third were women. The average hospitalist had about 7 years of experience in the specialty and about 5 years with their current hospitalist job. The majority were trained in internal medicine or one of its subspecialties, whereas pediatricians, family physicians, and physicians with other training made up the remainder.
Panel A | Panel B | ||
---|---|---|---|
Total | Assimilation Period Hospitalists | Advancement Period Hospitalists | |
| |||
Total, n | 816 | 103 | 713 |
Female, n (%) | 284 (35) | 37 (36) | 247 (35) |
Age, mean (SD) | 44.3 (9.0) | 41.9 (9.3) | 44.7 (8.9) |
Years postresidency experience as hospitalist, mean (SD) | 6.9 (4.5) | 4.3 (3.1) | 7.2 (4.6) |
Years in current practice, mean (SD) | 5.1 (3.9) | 0.9 (0.3) | 6.7 (3.8) |
Specialty training, n (%) | |||
Internal medicine | 555 (68) | 75 (73) | 480 (67) |
Pediatrics | 117 (14) | 8 (8) | 109 (15.3) |
Family medicine | 49 (6) | 7 (7) | 42 (6) |
Other | 95 (11) | 13 (13) | 82 (12) |
Job fit was highly skewed toward optimum fit, with a mean of 4.3 on a scale of 1 to 5, with a narrow standard deviation of 0.7. The poorest job fit was reported by 0.3%, whereas optimal fit was reported by 21% of respondents. Job fit plotted against years in current practice had a logarithmic appearance typical of learning curves (Figure 1). An inflection point was visualized at around 2 years. For the purposes of this article, we refer to hospitalists' experience in the first 2 years of a job as an assimilation period, which is marked by a steep increase in job fit early when rapid learning or attrition took place. The years beyond the inflection point are characterized as an advancement period, when a more attenuated rise in job fit was experienced with time. The Spearman correlation between job fit and years in practice during the advancement period was 0.145 (n = 678, P < 0.001). Panel B of Table 1 displays the characteristics of respondents separately for the assimilation and advancement cohorts. Assimilation hospitalists in our sample had a mean age of 41.9 years and mean on‐the‐job experience of 4.3 years, reflecting that many hospitalists in the first 2 years of a job have made at least 1 job change in the past.
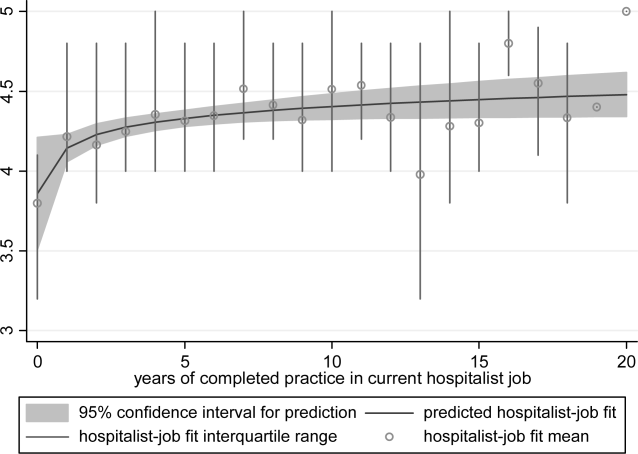
To show the effects of attrition and reselection, we first evaluated the proposition that hospitalists experience attrition (ie, intend to leave their jobs) in response to poor fit. Table 2 shows the correlations between job fit and the self‐reported intent to leave practice or reduce workload separately for the assimilation and advancement periods. For hospitalists in the assimilation period, job fit was negatively correlated with intent to leave current practice within 2 years and to leave hospital medicine within 5 years (P = 0.010 and 0.043, respectively). Hospitalists with <2 years in their current job, therefore, tended to consider attrition but not workload reduction to deal with poor job fit. On the other hand, hospitalists in the advancement period considered both attrition and workload reduction strategies in response to poor fit (all P < 0.001).
Assimilation Period Hospitalists | Advancement Period Hospitalists | |||
---|---|---|---|---|
Rho | P Value | Rho | P Value | |
Likelihood that a hospitalist will: | ||||
Leave current practice within 2 years | 0.253 | 0.010 | 0.367 | <0.001 |
Decrease total work hours within 5 years | 0.060 | 0.548 | 0.179 | <0.001 |
Decrease clinical work hours within 5 years | 0.072 | 0.469 | 0.144 | <0.001 |
Leave hospital medicine within 5 years | 0.200 | 0.043 | 0.231 | <0.001 |
Leave direct patient care within 5 years | 0.040 | 0.691 | 0.212 | <0.001 |
In Table 3, we further compared the median job fit across categories for the number of job switches. The median job fit during the assimilation period of hospitalists who had made 1 job change was slightly but statistically higher than the job fit of their counterparts who never left their first job (4.4 vs 4.0, P = 0.046). This suggests that job switching by hospitalists early in their jobs is associated with improved job fit (H1). However, the fit during the assimilation period of hospitalists who switched jobs twice or more was statistically no different from the fit of those in their first jobs, suggesting that the effect of the attrition‐reselection strategy is weak or inconsistent. The job fit for advancement period hospitalists was also different across the job change and no‐change categories. However, in the case of hospitalists later in their jobs, the median job fit was slightly but statistically lower among those who made job changes, revealing the potential drop in job fit that occurs when a hospitalist already established in his or her job starts over again in a new setting.
n | Age, Mean (95% CI), y | Hospitalist‐Job Fit, Median (IQR) | P Valuea | |
---|---|---|---|---|
| ||||
Assimilation period hospitalistsb | ||||
No job change | 29 | 42.3 (37.347.3) | 4.0 (3.84.4) | Reference |
1 job change | 39 | 40.3 (38.142.5) | 4.4 (4.04.8) | 0.046 |
2 or more job changes | 27 | 43.8 (41.046.6) | 4.4 (3.84.8) | 0.153 |
Advancement period hospitalistsc | ||||
No job change | 390 | 44.5 (43.645.5) | 4.6 (4.05.0) | Reference |
1 job change | 183 | 45.0 (43.746.3) | 4.2 (4.04.8) | 0.002 |
2 or more job changes | 99 | 44.9 (43.146.6) | 4.2 (3.84.8) | 0.002 |
We hypothesized that hospitalists who achieved high job fit within a particular job were more likely to have engaged in activities that utilize a wider spectrum of their abilities. As shown in Table 4, hospitalists in the highest quartile of job fit were associated with a general trend toward higher odds of participating in a variety of common clinical and nonclinical hospitalist activities, but only the odds ratio associated with teaching achieved statistical significance (OR: 1.53, 95% CI: 1.01‐2.31) (H2).
Participation, n/N (%) | Odds Ratio (95% CI) | P Value | |
---|---|---|---|
| |||
Administrative or committee work | 704/816 (86) | 0.73 (0.431.26) | 0.262 |
Quality improvement or patient safety initiatives | 678/816 (83) | 1.13 (0.642.00) | 0.680 |
Information technology design or implementation | 379/816 (46) | 1.18 (0.801.73) | 0.408 |
Any of the above leadership activities | 758/816 (93) | 1.31 (0.563.05) | 0.535 |
Teaching | 442/816 (54) | 1.53 (1.012.31) | 0.046 |
Research | 120/816 (15) | 1.07 (0.601.92) | 0.816 |
Any of the above academic activities | 457/816 (56) | 1.50 (0.992.27) | 0.057 |
Code team or rapid response team | 437/816 (54) | 1.13 (0.771.68) | 0.533 |
Intensive care unit | 254/816 (31) | 0.84 (0.531.35) | 0.469 |
Skilled nursing facility or long‐term acute care facility | 126/816 (15) | 1.06 (0.621.81) | 0.835 |
Outpatient general medical practice | 44/816 (5) | 1.75 (0.813.80) | 0.157 |
Any of the above clinical activities | 681/816 (79) | 1.02 (0.601.76) | 0.930 |
Socialization with peers and the gradual sharing of values within organizations are hypothesized mechanisms for increasing job fit with time. We found that the number of years in current practice was positively correlated with job fit (Spearman coefficient R = 0.149, P < 0.001), organizational climate (R = 0.128, P < 0.001), and relationship with nonphysician staff (R = 0.102, P < 0.01). The association between years in practice and relationship with physician colleagues were weaker (R = 0.079, P < 0.05). Consistent with the episodic nature of patients' encounters with hospitalists, the measure of patient relationships was not significantly associated with length of time in job. In addition, we found substantial correlations among job fit, organizational climate, and all the relational measures (all R > 0.280, P < 0.001), indicating that hospitalists increasingly share the values of their organizations over time (H3).
Finally, we also hypothesized that poor job fit is associated with poor performance and quality of work life. Strong correlations with job fit were noted for stress (R = 0.307, P < 0.001), job burnout (R = 0.360, P < 0.001), and job satisfaction (R = 0.570, P < 0.001). Job fit (R = 0.147, P < 0.001), job burnout (R = 0.236, P < 0.001), stress (R = 0.305, P < 0.001), and job satisfaction (R = 0.224, P < 0.001) were all significantly correlated with the frequency of participating in suboptimal care (H4).
DISCUSSION
In this exploratory analysis, we validated in the hospitalist workforce several assumptions about person‐job fit that have been observed in workers of other industries. We observed attrition‐reselection (ie, job switching) as a strategy used by physicians to achieve better fit early in their job tenure, whereas job modification appeared to be more effective than attrition‐reselection among physicians already established in their jobs. We provided weak but plausible evidence that physicians with optimal job fit had a tendency to participate in activities (eg, teaching) that engage a wider set of interests and abilities. We also demonstrated the growth in hospitalists sharing the values of their organization through the time‐dependent associations among organizational climate, relational measures, and job fit. Finally, we found that physicians with suboptimal job fit were more likely to report poor performance in their work compared to those indicating optimal fit.
Our previous analysis of data from the Hospital Medicine Physician Worklife Survey exposed the widely variable work characteristics of hospitalist jobs in the US market and the equally variable preferences and priorities of individual hospitalists in selecting their work setting.[7] The implication of our present study is that hospitalists achieve the high levels of observed job fit using various strategies that aid their alignment with their employment. One of these strategies involves time, but physician longevity in practice may be both a determinant and product of good job fit. Although early job attrition may be necessary for fitting the right hospitalists to the right jobs, employers may appreciate the importance of retaining experienced hospitalists not only for cost and performance considerations but also for the growth of social capital in organizations consisting of enduring individuals. As our data suggest that hospitalists grow with their jobs, physicians may experience better fit with jobs that flexibly couple their work demands with benefits that address their individual work‐life needs over time. Another implication of this study is that job fit is a useful and predictive measure of job selection, performance, and retention. In light of studies that expose the limitations of job satisfaction as a measure influenced more by workers' dispositional affect (ie, their temperament and outlook) than their compatibility with their jobs,[28] job fit may add a functional dimension to traditional employee feedback measures.
There are limitations to this analysis. The most notable is the low survey response rate. Two reasons contributed to the fairly low rate of return. First, the original sampling frame included many outdated addresses and names of individuals who did not meet inclusion criteria. Although all sampled individuals who would have been excluded from the study could not be identified, we calculated our response rate without accounting for the proportion of potential ineligibles in the denominator population [Response Rate 2 (RR2) according to standards of the American Association of Public Opinion Research].[29] Second, the response rates of physician surveys have seen a steady decline over the years.[30] Respondents to our survey may be older and more experienced than US hospitalists in general. Although concerns about bias from under‐reporting cannot be fully addressed, we believe that the study sample is adequate for this preliminary study intended to translate the evidence of observed phenomena from the nonphysician to the physician workforces. The suboptimal response characteristics (high skew and low variability) of the generic person‐job fit survey scale used in this study indicate that a reliable survey instrument specifically designed to measure physician‐job fit need to be constructed de novo and validated for any future study. Although we performed simple analyses to support our assertions, few of our subanalyses may be underpowered, contributing to overinterpretation of the data. Additional empirical work is also necessary to assess the generalizability of this study's claims in other medical and surgical specialties. Such studies would also allow measurement of the sensitivity and specificity of physicians' self‐identification of poor job fit. Finally, additional investigations of this time‐dependent construct are more appropriately performed using a longitudinal study design to overcome the limitations inherent in this cross‐sectional analysis. Our conclusions about the time‐dependent features of job fit may be explained by other characteristics such as generational and cultural differences among hospitalists with varying experience.
As the US healthcare system reorganizes to bolster accountability,[31] we anticipate increasing interdependence between physicians and their employer organizations. Ultimately, the desired accountability in healthcare is likely to be obtained if physicians function not only as passive and interchangeable employees but as active stakeholders in the achievement of each organization's goals. A methodology for assessing the alignment of physicians and their jobs will continue to be important along the way.
Disclosure
Nothing to report.
Person‐organization fit concerns the conditions and consequences of compatibility between people and the organizations for which they work.[1] Studies of other industries have demonstrated that person‐organization fit informs the way individuals join, perform in, and are retained by organizations.[2] Person‐job fit is a closely related subordinate concept that concerns the alignment of workers and their job in as much as workers have needs that their job supplies, or conversely, jobs have requirements that certain workers' abilities can help meet.[3] Explorations of job fit in physicians and their work have recently emerged in a few investigations published in medical journals.[4, 5, 6, 7, 8] Further expanding the understanding of fit between physicians and their employment is important, because the decline of solo practices and recent emphasis on team‐based care have led to a growing number of US physicians working in organizations.[9]
The movement of physicians into employed situations may continue if certain types of Accountable Care Organizations take root.[10] And physicians may be primed to join employer organizations based on current career priorities of individuals in American society. Surveys of medical residents entering the workforce reveal more physicians preferring the security of being employees than starting their own practices.[11] Given these trends, job fit will inform our understanding of how personal and job characteristics facilitate recruitment, performance, satisfaction, and longevity of physician employees.
BACKGROUND
Virtually all hospitalists work in organizationshospitalsand are employees of hospitals, medical schools, physician group practices, or management companies, and therefore invariably function within organizational structures and systems.[7] In spite of their rapid growth in numbers, many employers have faced difficulties recruiting and retaining enough hospitalists to fill their staffing needs. Consequently, the US hospitalist workforce today is characterized by high salaries, work load, and attrition rates.[12]
In this evolving unsaturated market, the attraction‐selection‐attrition framework[13] provides a theoretical construct that predicts that hospitalists and their employers would seek congruence of goals and values early in their relationship through a process of trial and error. This framework assumes that early interactions between workers and organizations serve as opportunities for them to understand if job fit is poor and dissociate or remain affiliated as long as job fit is mutually acceptable. Therefore, job switching on average is expected to increase job fit because workers and organizations gain a better understanding of their own goals and values and choose more wisely the next time.
Other theoretical frameworks, such as the job characteristic model,[14] suggest that over time as workers stay at the same job, they continue to maintain and improve job fit through various workplace‐ or self‐modification strategies. For example, seniority status may have privileges (eg, less undesirable call), or workers may create privileged niches through the acquisition of new skills and abilities over time. Hospitalists' tendency to diversify their work‐related activities by incorporating administrative and teaching responsibilities[15] may thus contribute to improving job fit. Additionally, as a measure of complementarity among people who work together, job fit may be influenced by the quality of relationships among hospitalists and their coworkers through their reorientation to the prevailing organizational climate[16, 17] and increasing socialization.[18] Finally, given that experiential learning is known to contribute to better hospitalist work performance,[19] job fit may affect productivity and clinical outcomes vis‐‐vis quality of work life.
To test the validity of these assumptions in a sample of hospitalists, we critically appraised the following 4 hypotheses:
- Hypothesis 1 (H1): Job attrition and reselection improves job fit among hospitalists entering the job market.
- Hypothesis 2 (H2): Better job fit is achieved through hospitalists engaging a variety of personal skills and abilities.
- Hypothesis 3 (H3): Job fit increases with hospitalists' job duration together with socialization and internalization of organizational values.
- Hypothesis 4 (H4): Job fit is correlated with hospitalists' quality of work life.
METHODS
Analysis was performed on data from the 2009 to 2010 Hospital Medicine Physician Worklife Survey. The sample frame included nonmembers and members of Society of Hospital Medicine (SHM). Details about sampling strategy, data collection, and data quality are available in previous publications.[7, 20] The 118‐item survey instrument, including 9 demographic items and 24 practice and job characteristic items, was administered by mail. Examples of information solicited through these items included respondents' practice model, the number of hospitalist jobs they have held, and the specific kinds of clinical and nonclinical activities they performed as part of their current job.
We used a reliable but broad and generic measure of self‐perceived person‐job fit.[21] The survey items of the 5‐point Likert‐type scale anchored between strongly disagree and strongly agree were: I feel that my work utilizes my full abilities, I feel competent and fully able to handle my job, my job gives me the chance to do the things I feel I do best, I feel that my job and I are well‐matched, I feel I have adequate preparation for the job I now hold. The quality of hospitalists' relationships with physician colleagues, staff, and patients as well as job satisfaction was measured using scales adapted from the Physician Worklife Study.[22] Organizational climate was measured using an adapted scale from the Minimizing Error, Maximizing Outcome study incorporating 3 items from the cohesiveness subscale, 4 items from the organizational trust subscale, and 1 item from the quality emphasis subscale that were most pertinent to hospitalists' relationship with their organizations.[23] Intent to leave practice or reduce work hours was measured using 5 items from the Multi‐Center Hospitalist Survey Project.[24] Frequency of participation in suboptimal patient care was measured by adapting 3 items from the suboptimal reported practice subscale and 2 items from the suboptimal patient care subscale developed by Shanafelt et al.[25] Stress and job burnout were assessed using validated measures.[26, 27] Detailed descriptions of the response rate calculation and imputation of missing item data are available in previous publications.[7, 20]
Mean, variance, range, and skew were used to characterize the responses to the job fit survey scale. A table of respondent characteristics was constructed. A visual representation of job fit by individual hospitalist year in current practice was created, first, by plotting a locally weighted scatterplot smoothing curve to examine the shape of the general relationship, and second, by fitting a similarly contoured functional polynomial curve with 95% confidence intervals (CI) to a plot of the mean and interquartile range of job fit for each year in current practice. Spearman partial correlations were calculated for job fit and each of the 5 items addressing likelihood of leaving practice or reducing workload adjusted for gender to control for the higher proportion of women who plan to work part time. Median (interquartile range) job fit was calculated for categories defined by the number of job changes and compared with the reference category (no job change) using the nonparametric rank sum test for comparing non‐normally distributed data. Multivariate logistic regression models were used to calculate the odds ratio (OR) of participating in each of several clinical and nonclinical hospitalist activities between respondents whose job fit score was optimal (5 on a 5‐point scale) and less than optimal controlling for covariates that influence the likelihood of participating in these activities (years in current practice, practice model, and specialty training). A Spearman correlation matrix was created to assess interscale correlations among organizational parameters (years in current practice, job fit, organizational climate, and relationship with colleagues, staff, and patients). Finally, a separate Spearman correlation matrix was created to assess the interscale correlations among individual worker parameters (job fit, suboptimal patient care, job burnout, stress, and job satisfaction). Statistical significance was defined as P value <0.05, and all analyses were performed on Stata 11.0 (StataCorp, College Station, TX). The Northwestern University institutional review board approved this study.
RESULTS
Respondents included 816 hospitalists belonging to around 700 unique organizations. The adjusted response rate from the stratified sample was 26%. Respondents and nonrespondents were similar with regard to geographic region and model of practice, but respondents were more likely to be members of the SHM than nonrespondents. Panel A of Table 1 shows the demographic characteristics of the respondents. The mean age was 44.3 years, and about one‐third were women. The average hospitalist had about 7 years of experience in the specialty and about 5 years with their current hospitalist job. The majority were trained in internal medicine or one of its subspecialties, whereas pediatricians, family physicians, and physicians with other training made up the remainder.
Panel A | Panel B | ||
---|---|---|---|
Total | Assimilation Period Hospitalists | Advancement Period Hospitalists | |
| |||
Total, n | 816 | 103 | 713 |
Female, n (%) | 284 (35) | 37 (36) | 247 (35) |
Age, mean (SD) | 44.3 (9.0) | 41.9 (9.3) | 44.7 (8.9) |
Years postresidency experience as hospitalist, mean (SD) | 6.9 (4.5) | 4.3 (3.1) | 7.2 (4.6) |
Years in current practice, mean (SD) | 5.1 (3.9) | 0.9 (0.3) | 6.7 (3.8) |
Specialty training, n (%) | |||
Internal medicine | 555 (68) | 75 (73) | 480 (67) |
Pediatrics | 117 (14) | 8 (8) | 109 (15.3) |
Family medicine | 49 (6) | 7 (7) | 42 (6) |
Other | 95 (11) | 13 (13) | 82 (12) |
Job fit was highly skewed toward optimum fit, with a mean of 4.3 on a scale of 1 to 5, with a narrow standard deviation of 0.7. The poorest job fit was reported by 0.3%, whereas optimal fit was reported by 21% of respondents. Job fit plotted against years in current practice had a logarithmic appearance typical of learning curves (Figure 1). An inflection point was visualized at around 2 years. For the purposes of this article, we refer to hospitalists' experience in the first 2 years of a job as an assimilation period, which is marked by a steep increase in job fit early when rapid learning or attrition took place. The years beyond the inflection point are characterized as an advancement period, when a more attenuated rise in job fit was experienced with time. The Spearman correlation between job fit and years in practice during the advancement period was 0.145 (n = 678, P < 0.001). Panel B of Table 1 displays the characteristics of respondents separately for the assimilation and advancement cohorts. Assimilation hospitalists in our sample had a mean age of 41.9 years and mean on‐the‐job experience of 4.3 years, reflecting that many hospitalists in the first 2 years of a job have made at least 1 job change in the past.
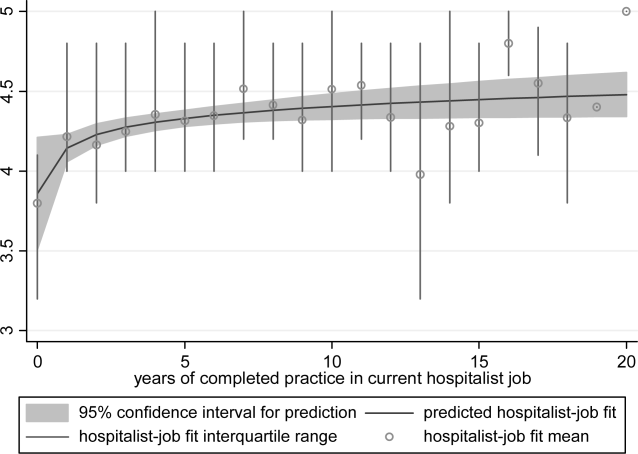
To show the effects of attrition and reselection, we first evaluated the proposition that hospitalists experience attrition (ie, intend to leave their jobs) in response to poor fit. Table 2 shows the correlations between job fit and the self‐reported intent to leave practice or reduce workload separately for the assimilation and advancement periods. For hospitalists in the assimilation period, job fit was negatively correlated with intent to leave current practice within 2 years and to leave hospital medicine within 5 years (P = 0.010 and 0.043, respectively). Hospitalists with <2 years in their current job, therefore, tended to consider attrition but not workload reduction to deal with poor job fit. On the other hand, hospitalists in the advancement period considered both attrition and workload reduction strategies in response to poor fit (all P < 0.001).
Assimilation Period Hospitalists | Advancement Period Hospitalists | |||
---|---|---|---|---|
Rho | P Value | Rho | P Value | |
Likelihood that a hospitalist will: | ||||
Leave current practice within 2 years | 0.253 | 0.010 | 0.367 | <0.001 |
Decrease total work hours within 5 years | 0.060 | 0.548 | 0.179 | <0.001 |
Decrease clinical work hours within 5 years | 0.072 | 0.469 | 0.144 | <0.001 |
Leave hospital medicine within 5 years | 0.200 | 0.043 | 0.231 | <0.001 |
Leave direct patient care within 5 years | 0.040 | 0.691 | 0.212 | <0.001 |
In Table 3, we further compared the median job fit across categories for the number of job switches. The median job fit during the assimilation period of hospitalists who had made 1 job change was slightly but statistically higher than the job fit of their counterparts who never left their first job (4.4 vs 4.0, P = 0.046). This suggests that job switching by hospitalists early in their jobs is associated with improved job fit (H1). However, the fit during the assimilation period of hospitalists who switched jobs twice or more was statistically no different from the fit of those in their first jobs, suggesting that the effect of the attrition‐reselection strategy is weak or inconsistent. The job fit for advancement period hospitalists was also different across the job change and no‐change categories. However, in the case of hospitalists later in their jobs, the median job fit was slightly but statistically lower among those who made job changes, revealing the potential drop in job fit that occurs when a hospitalist already established in his or her job starts over again in a new setting.
n | Age, Mean (95% CI), y | Hospitalist‐Job Fit, Median (IQR) | P Valuea | |
---|---|---|---|---|
| ||||
Assimilation period hospitalistsb | ||||
No job change | 29 | 42.3 (37.347.3) | 4.0 (3.84.4) | Reference |
1 job change | 39 | 40.3 (38.142.5) | 4.4 (4.04.8) | 0.046 |
2 or more job changes | 27 | 43.8 (41.046.6) | 4.4 (3.84.8) | 0.153 |
Advancement period hospitalistsc | ||||
No job change | 390 | 44.5 (43.645.5) | 4.6 (4.05.0) | Reference |
1 job change | 183 | 45.0 (43.746.3) | 4.2 (4.04.8) | 0.002 |
2 or more job changes | 99 | 44.9 (43.146.6) | 4.2 (3.84.8) | 0.002 |
We hypothesized that hospitalists who achieved high job fit within a particular job were more likely to have engaged in activities that utilize a wider spectrum of their abilities. As shown in Table 4, hospitalists in the highest quartile of job fit were associated with a general trend toward higher odds of participating in a variety of common clinical and nonclinical hospitalist activities, but only the odds ratio associated with teaching achieved statistical significance (OR: 1.53, 95% CI: 1.01‐2.31) (H2).
Participation, n/N (%) | Odds Ratio (95% CI) | P Value | |
---|---|---|---|
| |||
Administrative or committee work | 704/816 (86) | 0.73 (0.431.26) | 0.262 |
Quality improvement or patient safety initiatives | 678/816 (83) | 1.13 (0.642.00) | 0.680 |
Information technology design or implementation | 379/816 (46) | 1.18 (0.801.73) | 0.408 |
Any of the above leadership activities | 758/816 (93) | 1.31 (0.563.05) | 0.535 |
Teaching | 442/816 (54) | 1.53 (1.012.31) | 0.046 |
Research | 120/816 (15) | 1.07 (0.601.92) | 0.816 |
Any of the above academic activities | 457/816 (56) | 1.50 (0.992.27) | 0.057 |
Code team or rapid response team | 437/816 (54) | 1.13 (0.771.68) | 0.533 |
Intensive care unit | 254/816 (31) | 0.84 (0.531.35) | 0.469 |
Skilled nursing facility or long‐term acute care facility | 126/816 (15) | 1.06 (0.621.81) | 0.835 |
Outpatient general medical practice | 44/816 (5) | 1.75 (0.813.80) | 0.157 |
Any of the above clinical activities | 681/816 (79) | 1.02 (0.601.76) | 0.930 |
Socialization with peers and the gradual sharing of values within organizations are hypothesized mechanisms for increasing job fit with time. We found that the number of years in current practice was positively correlated with job fit (Spearman coefficient R = 0.149, P < 0.001), organizational climate (R = 0.128, P < 0.001), and relationship with nonphysician staff (R = 0.102, P < 0.01). The association between years in practice and relationship with physician colleagues were weaker (R = 0.079, P < 0.05). Consistent with the episodic nature of patients' encounters with hospitalists, the measure of patient relationships was not significantly associated with length of time in job. In addition, we found substantial correlations among job fit, organizational climate, and all the relational measures (all R > 0.280, P < 0.001), indicating that hospitalists increasingly share the values of their organizations over time (H3).
Finally, we also hypothesized that poor job fit is associated with poor performance and quality of work life. Strong correlations with job fit were noted for stress (R = 0.307, P < 0.001), job burnout (R = 0.360, P < 0.001), and job satisfaction (R = 0.570, P < 0.001). Job fit (R = 0.147, P < 0.001), job burnout (R = 0.236, P < 0.001), stress (R = 0.305, P < 0.001), and job satisfaction (R = 0.224, P < 0.001) were all significantly correlated with the frequency of participating in suboptimal care (H4).
DISCUSSION
In this exploratory analysis, we validated in the hospitalist workforce several assumptions about person‐job fit that have been observed in workers of other industries. We observed attrition‐reselection (ie, job switching) as a strategy used by physicians to achieve better fit early in their job tenure, whereas job modification appeared to be more effective than attrition‐reselection among physicians already established in their jobs. We provided weak but plausible evidence that physicians with optimal job fit had a tendency to participate in activities (eg, teaching) that engage a wider set of interests and abilities. We also demonstrated the growth in hospitalists sharing the values of their organization through the time‐dependent associations among organizational climate, relational measures, and job fit. Finally, we found that physicians with suboptimal job fit were more likely to report poor performance in their work compared to those indicating optimal fit.
Our previous analysis of data from the Hospital Medicine Physician Worklife Survey exposed the widely variable work characteristics of hospitalist jobs in the US market and the equally variable preferences and priorities of individual hospitalists in selecting their work setting.[7] The implication of our present study is that hospitalists achieve the high levels of observed job fit using various strategies that aid their alignment with their employment. One of these strategies involves time, but physician longevity in practice may be both a determinant and product of good job fit. Although early job attrition may be necessary for fitting the right hospitalists to the right jobs, employers may appreciate the importance of retaining experienced hospitalists not only for cost and performance considerations but also for the growth of social capital in organizations consisting of enduring individuals. As our data suggest that hospitalists grow with their jobs, physicians may experience better fit with jobs that flexibly couple their work demands with benefits that address their individual work‐life needs over time. Another implication of this study is that job fit is a useful and predictive measure of job selection, performance, and retention. In light of studies that expose the limitations of job satisfaction as a measure influenced more by workers' dispositional affect (ie, their temperament and outlook) than their compatibility with their jobs,[28] job fit may add a functional dimension to traditional employee feedback measures.
There are limitations to this analysis. The most notable is the low survey response rate. Two reasons contributed to the fairly low rate of return. First, the original sampling frame included many outdated addresses and names of individuals who did not meet inclusion criteria. Although all sampled individuals who would have been excluded from the study could not be identified, we calculated our response rate without accounting for the proportion of potential ineligibles in the denominator population [Response Rate 2 (RR2) according to standards of the American Association of Public Opinion Research].[29] Second, the response rates of physician surveys have seen a steady decline over the years.[30] Respondents to our survey may be older and more experienced than US hospitalists in general. Although concerns about bias from under‐reporting cannot be fully addressed, we believe that the study sample is adequate for this preliminary study intended to translate the evidence of observed phenomena from the nonphysician to the physician workforces. The suboptimal response characteristics (high skew and low variability) of the generic person‐job fit survey scale used in this study indicate that a reliable survey instrument specifically designed to measure physician‐job fit need to be constructed de novo and validated for any future study. Although we performed simple analyses to support our assertions, few of our subanalyses may be underpowered, contributing to overinterpretation of the data. Additional empirical work is also necessary to assess the generalizability of this study's claims in other medical and surgical specialties. Such studies would also allow measurement of the sensitivity and specificity of physicians' self‐identification of poor job fit. Finally, additional investigations of this time‐dependent construct are more appropriately performed using a longitudinal study design to overcome the limitations inherent in this cross‐sectional analysis. Our conclusions about the time‐dependent features of job fit may be explained by other characteristics such as generational and cultural differences among hospitalists with varying experience.
As the US healthcare system reorganizes to bolster accountability,[31] we anticipate increasing interdependence between physicians and their employer organizations. Ultimately, the desired accountability in healthcare is likely to be obtained if physicians function not only as passive and interchangeable employees but as active stakeholders in the achievement of each organization's goals. A methodology for assessing the alignment of physicians and their jobs will continue to be important along the way.
Disclosure
Nothing to report.
- Person‐organization fit: an integrative review of its conceptualizations, measurement, and implications. Personnel Psychol. 1996;49:1–49. .
- Consequences of individuals' fit at work: a meta‐analysis of person‐job, person‐organization, person‐group, and person‐supervisor fit. Personnel Psychol. 2005;58(2):281–342. , , .
- Person‐job fit: a conceptual integration, literature review and methodological critique. In: Cooper CL, Robertson IT, eds. International Review of Industrial and Organizational Psychology. Vol.6. New York, NY:John Wiley 1991. .
- Organizational culture, person‐culture fit, and turnover: a replication in the health care industry. J Organ Behav. 1999;20(2):175–184. .
- Organizational culture and physician satisfaction with dimensions of group practice. Health Serv Res. 2007;42(3 pt 1):1150–1176. , , , .
- Career fit and burnout among academic faculty. Arch Intern Med. 2009;169(10):990–995. , , , et al.
- Job characteristics, satisfaction, and burnout across hospitalist practice models. J Hosp Med. 2012;7(5):402–410. , , , , .
- Provider‐hospital “fit” and patient outcomes: evidence from Massachusetts cardiac surgeons, 2002–2004. Health Serv Res. 2011;46(1 pt 1):1–26. .
- The evolving primary care physician. N Engl J Med. 2012;366(20):1849–1853. .
- Hospitals' race to employ physicians—the logic behind a money‐losing proposition. N Engl J Med. 2011;364(19):1790–1793. , .
- 2011 Survey of Final‐Year Medical Residents. Irving, TX:Merritt Hawkins;2011.
- State of Hospital Medicine: 2010 Report Based on 2009 Data. Englewood, CO:Society of Hospital Medicine and the Medical Group Management Association;2010.
- The ASA framework: an update. Personnel Psychol. 1995;48(4):747–773. , , .
- Work Redesign. Reading. MA:Addison‐Wesley;1980. , .
- The expanding role of hospitalists in the United States. Swiss Med Wkly. 2006;136(37–38):591–596. , .
- Organizational socialization as a learning‐process—the role of information acquisition. Personnel Psychol. 1992;45(4):849–874. , .
- The moderating effect of tenure in person‐environment fit: a field study in educational organizations. J Occup Organ Psych. 1997;70:173–188. , .
- Matching people and organizations—selection and socialization in public accounting firms. Admin Sci Quart. 1991;36(3):459–484. .
- Effects of physician experience on costs and outcomes on an academic general medicine service: results of a trial of hospitalists. Ann Intern Med. 2002;137(11):866–874. , , , et al.
- Worklife and satisfaction of hospitalists: toward flourishing careers. J Gen Intern Med. 2012;27(1):28–36. , , , , .
- Karasek's model in the People's Republic of China: effects of job demands, control, and individual differences. Acad Manage J. 1996;39(6):1594–1618. .
- Measuring physician job satisfaction in a changing workplace and a challenging environment. SGIM Career Satisfaction Study Group. Society of General Internal Medicine. Med Care. 1999;37(11):1174–1182. , , , et al.
- Organizational climate, stress, and error in primary care: The MEMO Study. Adv Patient Saf. 2005;1:65–77. , , , et al.
- Effects of inpatient experience on outcomes and costs in a multicenter trial of academic hospitalists. J Gen Intern Med. 2005;20(suppl 1):141–142. , , , et al.
- Burnout and self‐reported patient care in an internal medicine residency program. Ann Intern Med. 2002;136(5):358–367. , , , .
- Effect of job demands and social support on worker stress—a study of VDT users. Behav Inform Technol. 1995;14(1):32–40. , .
- Validation of a single‐item measure of burnout against the Maslach Burnout Inventory among physicians. Stress Health. 2004;20(2):75–79. , , .
- Job satisfaction: a meta‐analysis of stabilities. J Organ Behav. 2001;22(5):483–504. , .
- The American Association for Public Opinion Research. Standard definitions: final dispositions of case codes and outcome rates for surveys.7th ed. Available at: http://www.aapor.org/Standard_Definitions2.htm. Accessed May 2,2012.
- Response rates and response bias for 50 surveys of pediatricians. Health Serv Res. 2005;40(1):213–226. , , , .
- Accountable care organizations: accountable for what, to whom, and how. JAMA. 2010;304(15):1715–6. , .
- Person‐organization fit: an integrative review of its conceptualizations, measurement, and implications. Personnel Psychol. 1996;49:1–49. .
- Consequences of individuals' fit at work: a meta‐analysis of person‐job, person‐organization, person‐group, and person‐supervisor fit. Personnel Psychol. 2005;58(2):281–342. , , .
- Person‐job fit: a conceptual integration, literature review and methodological critique. In: Cooper CL, Robertson IT, eds. International Review of Industrial and Organizational Psychology. Vol.6. New York, NY:John Wiley 1991. .
- Organizational culture, person‐culture fit, and turnover: a replication in the health care industry. J Organ Behav. 1999;20(2):175–184. .
- Organizational culture and physician satisfaction with dimensions of group practice. Health Serv Res. 2007;42(3 pt 1):1150–1176. , , , .
- Career fit and burnout among academic faculty. Arch Intern Med. 2009;169(10):990–995. , , , et al.
- Job characteristics, satisfaction, and burnout across hospitalist practice models. J Hosp Med. 2012;7(5):402–410. , , , , .
- Provider‐hospital “fit” and patient outcomes: evidence from Massachusetts cardiac surgeons, 2002–2004. Health Serv Res. 2011;46(1 pt 1):1–26. .
- The evolving primary care physician. N Engl J Med. 2012;366(20):1849–1853. .
- Hospitals' race to employ physicians—the logic behind a money‐losing proposition. N Engl J Med. 2011;364(19):1790–1793. , .
- 2011 Survey of Final‐Year Medical Residents. Irving, TX:Merritt Hawkins;2011.
- State of Hospital Medicine: 2010 Report Based on 2009 Data. Englewood, CO:Society of Hospital Medicine and the Medical Group Management Association;2010.
- The ASA framework: an update. Personnel Psychol. 1995;48(4):747–773. , , .
- Work Redesign. Reading. MA:Addison‐Wesley;1980. , .
- The expanding role of hospitalists in the United States. Swiss Med Wkly. 2006;136(37–38):591–596. , .
- Organizational socialization as a learning‐process—the role of information acquisition. Personnel Psychol. 1992;45(4):849–874. , .
- The moderating effect of tenure in person‐environment fit: a field study in educational organizations. J Occup Organ Psych. 1997;70:173–188. , .
- Matching people and organizations—selection and socialization in public accounting firms. Admin Sci Quart. 1991;36(3):459–484. .
- Effects of physician experience on costs and outcomes on an academic general medicine service: results of a trial of hospitalists. Ann Intern Med. 2002;137(11):866–874. , , , et al.
- Worklife and satisfaction of hospitalists: toward flourishing careers. J Gen Intern Med. 2012;27(1):28–36. , , , , .
- Karasek's model in the People's Republic of China: effects of job demands, control, and individual differences. Acad Manage J. 1996;39(6):1594–1618. .
- Measuring physician job satisfaction in a changing workplace and a challenging environment. SGIM Career Satisfaction Study Group. Society of General Internal Medicine. Med Care. 1999;37(11):1174–1182. , , , et al.
- Organizational climate, stress, and error in primary care: The MEMO Study. Adv Patient Saf. 2005;1:65–77. , , , et al.
- Effects of inpatient experience on outcomes and costs in a multicenter trial of academic hospitalists. J Gen Intern Med. 2005;20(suppl 1):141–142. , , , et al.
- Burnout and self‐reported patient care in an internal medicine residency program. Ann Intern Med. 2002;136(5):358–367. , , , .
- Effect of job demands and social support on worker stress—a study of VDT users. Behav Inform Technol. 1995;14(1):32–40. , .
- Validation of a single‐item measure of burnout against the Maslach Burnout Inventory among physicians. Stress Health. 2004;20(2):75–79. , , .
- Job satisfaction: a meta‐analysis of stabilities. J Organ Behav. 2001;22(5):483–504. , .
- The American Association for Public Opinion Research. Standard definitions: final dispositions of case codes and outcome rates for surveys.7th ed. Available at: http://www.aapor.org/Standard_Definitions2.htm. Accessed May 2,2012.
- Response rates and response bias for 50 surveys of pediatricians. Health Serv Res. 2005;40(1):213–226. , , , .
- Accountable care organizations: accountable for what, to whom, and how. JAMA. 2010;304(15):1715–6. , .
Copyright © 2012 Society of Hospital Medicine
Hospitalist Practice Models
Over the past 15 years, there has been dramatic growth in the number of hospitalist physicians in the United States and in the number of hospitals served by them.13 Hospitals are motivated to hire experienced hospitalists to staff their inpatient services,4 with goals that include obtaining cost‐savings and higher quality.59 The rapid growth of Hospital Medicine saw multiple types of hospital practice models emerge with differing job characteristics, clinical duties, workload, and compensation schemes.10 The extent of the variability of hospitalist jobs across practice models is not known.
Intensifying recruitment efforts and the concomitant increase in compensation for hospitalists over the last decade suggest that demand for hospitalists is strong and sustained.11 As a result, today's cohort of hospitalists has a wide range of choices of types of jobs, practice models, and locations. The diversity of available hospitalist jobs is characterized, for example, by setting (community hospital vs academic hospital), employer (hospital vs private practice), job duties (the amount and type of clinical work, and other administrative, teaching, or research duties), and intensity (work hours and duties to maximize income or lifestyle). How these choices relate to job satisfaction and burnout are also unknown.
The Society of Hospital Medicine (SHM) has administered surveys to hospitalist group leaders biennially since 2003.1215 These surveys, however, do not address issues related to individual hospitalist worklife, recruitment, and retention. In 2005, SHM convened a Career Satisfaction Task Force that designed and executed a national survey of hospitalists in 2009‐2010. The objective of this study is to evaluate how job characteristics vary by practice model, and the association of these characteristics and practice models with job satisfaction and burnout.
METHODS
Survey Instrument
A detailed description of the survey design, sampling strategy, data collection, and response rate calculations is described elsewhere.16 Portions of the 118‐item survey instrument assessed characteristics of the respondents' hospitalist group (12 items), details about their individual work patterns (12 items), and demographics (9 items). Work patterns were evaluated by the average number of clinical work days, consecutive days, hours per month, percentage of work assigned to night duty, and number of patient encounters. Average hours spent on nonclinical work, and the percentage of time allocated for clinical, administrative, teaching, and research activities were solicited. Additional items assessed specific clinical responsibilities, pretax earnings in FY2010, the availability of information technology capabilities, and the adequacy of available resources. Job and specialty satisfaction and 11 satisfaction domain measures were measured using validated scales.1726 Burnout symptoms were measured using a validated single‐item measure.26, 27
Sampling Strategy
We surveyed a national stratified sample of hospitalists in the US and Puerto Rico. We used the largest database of hospitalists (>24,000 names) currently available and maintained by the SHM as our sampling frame. We linked hospitalist employer information to hospital statistics from the American Hospital Association database28 to stratify the sample by number of hospital beds, geographic region, employment model, and specialty training, oversampling pediatric hospitalists due to small numbers. A respondent sample of about 700 hospitalists was calculated to be adequate to detect a 0.5 point difference in job satisfaction scores between subgroups assuming 90% power and alpha of 0.05. However, we sampled a total of 5389 addresses from the database to overcome the traditionally low physician response rates, duplicate sampling, bad addresses, and non‐hospitalists being included in the sampling frame. In addition, 2 multistate hospitalist companies (EmCare, In Compass Health) and 1 for‐profit hospital chain (HCA, Inc) financially sponsored this project with the stipulation that all of their hospitalist employees (n = 884) would be surveyed.
Data Collection
The healthcare consulting firm, Press Ganey, provided support with survey layout and administration following the modified Dillman method.29 Three rounds of coded surveys and solicitation letters from the investigators were mailed 2 weeks apart in November and December 2009. Because of low response rates to the mailed survey, an online survey was created using Survey Monkey and sent to 650 surveyees for whom e‐mail addresses were available, and administered at a kiosk for sample physicians during the SHM 2010 annual meeting.
Data Analysis
Nonresponse bias was measured by comparing characteristics between respondents of separate survey waves.30 We determined the validity of mailing addresses immediately following the survey period by mapping each address using Google, and if the address was a hospital, researching online whether or not the intended recipient was currently employed there. Practice characteristics were compared across 5 model categories distilled from the SHM & Medical Group Management Association survey: local hospitalist‐only group, multistate hospitalist group, multispecialty physician group, employer hospital, and university or medical school. Weighted proportions, means, and medians were calculated to account for oversampling of pediatric hospitalists. Differences in categorical measures were assessed using the chi‐square test and the design‐based F test for comparing weighted data. Weighted means (99% confidence intervals) and medians (interquartile ranges) were calculated. Because each parameter yielded a single outlier value across the 5 practice models, differences across weighted means were assessed using generalized linear models with the single outlier value chosen as the reference mean. Pair‐wise Wilcoxon rank sum test was used to compare median values. In these 4‐way comparisons of means and medians, significance was defined as P value of 0.0125 per Bonferroni correction. A single survey item solicited respondents to choose exactly 4 of 13 considerations most pertinent to job satisfaction. The proportion of respondents who scored 4 on a 5‐point Likert scale of the 11 satisfaction domains and 2 global measures of satisfaction, and burnout symptoms defined as 3 on a 5‐point single item measure were bar‐graphed. Chi‐square statistics were used to evaluate for differences across practice models. Statistical significance was defined by alpha less than 0.05, unless otherwise specified. All analyses were performed using STATA version 11.0 (College Station, TX). This study was approved by the Loyola University Institutional Review Board.
Survey data required cleaning prior to analysis. Missing gender information was imputed using the respondents' name. Responses to the item that asked to indicate the proportion of work dedicated to administrative responsibilities, clinical care, teaching, and research that did not add up to 100% were dropped. Two responses that indicated full‐time equivalent (FTE) of 0%, but whose respondents otherwise completed the survey implying they worked as clinical hospitalists, were replaced with values calculated from the given number of work hours relative to the median work hours in our sample. Out of range or implausible responses to the following items were dropped from analyses: the average number of billable encounters during a typical day or shift, number of shifts performing clinical activities during a typical month, pretax earnings, the year the respondent completed residency training, and the number of whole years practiced as a hospitalist. The proportion of selective item nonresponse was small and we did not, otherwise, impute missing data.
RESULTS
Response Rate
Of the 5389 originally sampled addresses, 1868 were undeliverable. Addresses were further excluded if they appeared in duplicate or were outdated. This yielded a total of 3105 eligible surveyees in the sample. As illustrated in Figure 1, 841 responded to the mailed survey and 5 responded to the Web‐based survey. After rejecting 67 non‐hospitalist respondents and 3 duplicate surveys, a total of 776 surveys were included in the final analysis. The adjusted response rate was 25.6% (776/3035). Members of SHM were more likely to return the survey than nonmembers. The adjusted response rate from hospitalists affiliated with the 3 sponsoring institutions was 6% (40/662). Because these respondents were more likely to be non‐members of SHM, we opted to analyze the responses from the sponsor hospitalists together with the sampled hospitalists. The demographics of the resulting pool of 816 respondents affiliated with over 650 unique hospitalist groups were representative of the original survey frame. We analyzed data from 794 of these who responded to the item indicating their hospitalist practice model. Demographic characteristics of responders and nonresponders to the practice model survey item were similar.
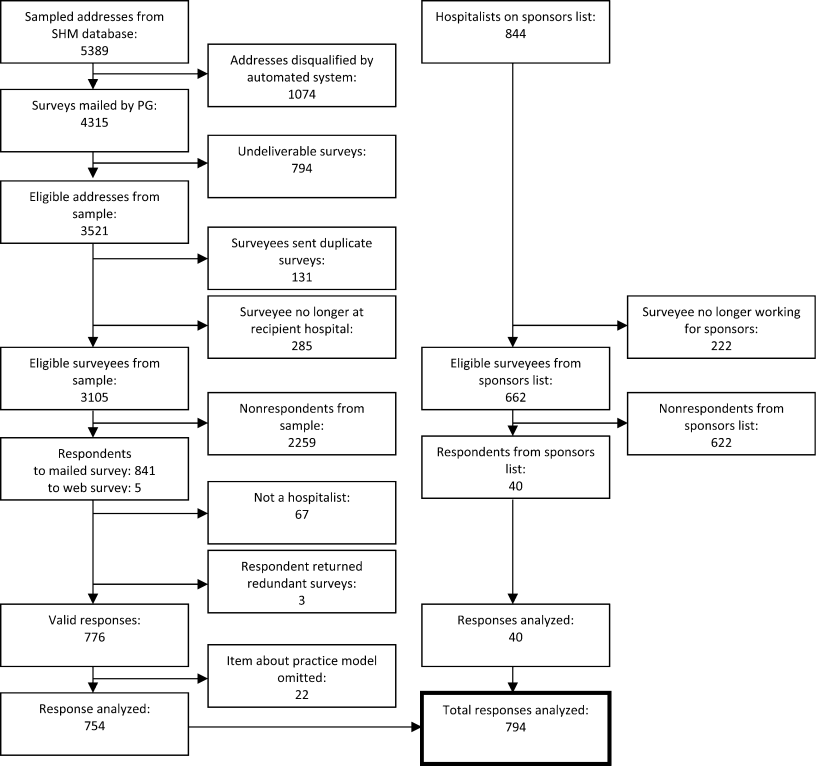
Characteristics of Hospitalists and Their Groups
Table 1 summarizes the characteristics of hospitalist respondents and their organizations by practice model. More (44%) respondents identified their practice model as directly employed by the hospital than other models, including multispecialty physician group (15%), multistate hospitalist group (14%), university or medical school (14%), local hospitalist group (12%), and other (2%). The median age of hospitalist respondents was 42 years, with 6.8 years of mean experience as a hospitalist. One third were women, 84% were married, and 46% had dependent children 6 years old or younger at home. Notably, hospitalists in multistate groups had fewer years of experience, and fewer hospitalists in local and multistate groups were married compared to hospitalists in other practice models.
Local Hospitalist‐Only Group | Multi‐State Hospitalist Group | Multispecialty Physician Group | Employer Hospital | University or Medical School | ||
---|---|---|---|---|---|---|
n = 95 | n = 111 | n = 115 | n = 348 | n = 107 | P Value | |
| ||||||
Hospitalist characteristics | ||||||
Age, weighted mean (99% CI) | 45 (42, 48) | 44 (42, 47) | 45 (43, 47) | 45 (43, 46) | 43 (40, 46) | |
Years hospitalist experience, weighted mean (99% CI) | 8 (6, 9)* | 5 (4, 6)* | 8 (7, 9) | 7 (6, 7) | 8 (6, 9) | <0.010* |
Women, weighted % | 29 | 30 | 39 | 31 | 43 | 0.118 |
Married, weighted % | 76 | 77 | 82 | 89 | 81 | 0.009 |
At least 1 dependent child younger than age 6 living in home, weighted % | 47 | 48 | 43 | 47 | 45 | 0.905 |
Pediatric specialty, n (%) | <10 | <10 | 11 (10%) | 57 (16%) | 36 (34%) | <0.001 |
Hospitalist group characteristics | ||||||
Region, weighted % | <0.001 | |||||
Northeast (AHA 1 & 2) | 13 | 10 | 16 | 27 | 13 | |
South (AHA 3 & 4) | 19 | 37 | 13 | 24 | 21 | |
Midwest (AHA 5 & 6) | 23 | 24 | 25 | 22 | 26 | |
Mountain (AHA 7 & 8) | 22 | 20 | 16 | 13 | 24 | |
West (AHA 9) | 24 | 10 | 31 | 14 | 16 | |
No. beds of primary hospital, weighted % | <0.001 | |||||
Up to 149 | 17 | 26 | 12 | 24 | 14 | |
150299 | 30 | 36 | 36 | 33 | 21 | |
300449 | 26 | 24 | 29 | 20 | 19 | |
450599 | 13 | 8 | 17 | 11 | 21 | |
600 or more | 12 | 6 | 7 | 13 | 24 | |
No. of hospital facilities served by current practice, weighted % | <0.001 | |||||
1 | 53 | 70 | 67 | 77 | 66 | |
2 | 20 | 22 | 20 | 16 | 24 | |
3 or more | 27 | 9 | 13 | 7 | 10 | |
No. of physicians in current practice, median (IQR) | 10 (5, 18) | 8 (6, 12)* | 14 (8, 25)* | 12 (6, 18) | 12 (7, 20) | <0.001*, 0.001 |
No. of non‐physician providers in current practice, median (IQR) | 0 (0, 2) | 0 (0, 2) | 0 (0, 3) | 1 (0, 2) | 0 (0, 2) | |
Available information technology capabilities, weighted % | ||||||
EHR to access physician notes | 57 | 57 | 75 | 58 | 79 | <0.001 |
EHR to access nursing documentations | 68 | 67 | 74 | 75 | 76 | 0.357 |
EHR to access laboratory or test results | 97 | 89 | 95 | 96 | 96 | 0.054 |
Electronic order entry | 30 | 19 | 53 | 38 | 56 | <0.001 |
Electronic billing | 38 | 31 | 36 | 36 | 38 | 0.818 |
Access to EHR at home or off site | 78 | 73 | 78 | 82 | 84 | 0.235 |
Access to Up‐to‐Date or other clinical guideline resources | 80 | 77 | 91 | 92 | 96 | <0.001 |
Access to schedules, calendars, or other organizational resources | 56 | 57 | 66 | 67 | 75 | 0.024 |
E‐mail, Web‐based paging, or other communication resources | 74 | 63 | 88 | 89 | 90 | <0.001 |
Several differences in respondent group characteristics by practice model were found. Respondents in multistate hospitalist groups were more likely from the South and Midwest, while respondents from multispecialty groups were likely from the West. More multistate group practices were based in smaller hospitals, while academic hospitalists tended to practice in hospitals with 600 or more beds. Respondents employed by hospitals were more likely to practice at 1 hospital facility only, while local group practices were more likely to practice at 3 or more facilities. The median number of physicians in a hospitalist group was 11 (interquartile range [IQR] 6, 19). Local and multistate groups had fewer hospitalists compared to other models. Nonphysician providers were employed by nearly half of all hospitalist practices. Although almost all groups had access to some information technology, more academic hospitalists had access to electronic order entry, electronic physician notes, electronic clinical guidelines resources and communication technology, while local and multistate groups were least likely to have access to these resources.
Work Pattern Variations
Table 2 further details hospitalist work hours by practice model. The majority of hospitalists (78%) reported their position was full‐time (FTE 1.0), while 13% reported working less than full‐time (FTE <1.0). Only 5% of local group hospitalists worked part‐time, while 20% of multispecialty group hospitalists did. An additional 9% reported FTE >1.0, indicating their work hours exceeded the definition of a full‐time physician in their practice. Among full‐time hospitalists, local group members worked a greater number of shifts per month than employees of multispecialty groups, hospitals, and academic medical centers. Academic hospitalists reported higher numbers of consecutive clinical days worked on average, but fewer night shifts compared to hospitalists employed by multistate groups, multispecialty groups, and hospitals; fewer billable encounters than hospitalists in local and multistate groups; and more nonclinical work hours than hospitalists of any other practice model. Academic hospitalists also spent more time on teaching and research than other practice models. Hospitalists spent 11%‐18% of their time on administrative and committee responsibilities, with the least amount spent by hospitalists in multistate groups and the most in academic practice.
Local Hospitalist‐Only Group | Multi‐State Hospitalist Group | Multispecialty Physician Group | Employer Hospital | University or Medical School | ||
---|---|---|---|---|---|---|
n = 95 | n = 111 | n = 115 | n = 348 | n = 107 | P Value | |
| ||||||
FTE, weighted % | 0.058 | |||||
FTE < 1.0 | 6 | 13 | 20 | 12 | 14 | |
FTE = 1.0 | 85 | 75 | 74 | 80 | 82 | |
FTE > 1.0 | 10 | 13 | 6 | 8 | 5 | |
Workload parameters, weighted mean (99% CI) | ||||||
Clinical shifts per month for FTE 1.0 | 19 (17, 20)* | 17 (16, 19) | 15 (14, 17)* | 16 (15, 16) | 15 (13, 17) | <0.001* |
Hours per clinical shift | 10 (9, 11) | 11 (10, 11)* | 10 (10, 11.0) | 11 (10, 11.0) | 10 (9, 10)* | 0.006*, 0.002 |
Consecutive days on clinical shift | 8 (6, 9) | 7 (6, 7)* | 6 (6, 7) | 7 (6, 7) | 9 (7, 10)* | 0.002*, <0.001 |
% Clinical shifts on nights | 20 (15, 25) | 23 (18, 28)* | 23 (17, 29) | 21 (17, 24) | 14 (9, 18)* | 0.001*, 0.002 |
% Night shifts spent in hospital | 61 (49, 74)* | 63 (52, 75) | 72 (62, 83) | 73 (67, 80) | 43 (29, 57)* | 0.010*, 0.003, <0.001 |
Billable encounters per clinical shift | 17 (14, 19)* | 17 (16, 18) | 14 (13, 15) | 15 (14, 16) | 13 (11, 14)* | <0.001*, 0.002 |
Hours nonclinical work per month | 23 (12, 34)* | 19 (11, 27) | 31 (20, 42) | 30 (24, 36) | 71 (55, 86)* | <0.001* |
Hours clinical and nonclinical work per month for FTE 1.0 | 202 (186, 219) | 211 (196, 226) | 184 (170, 198)* | 193 (186, 201) | 221 (203, 238)* | <0.001* |
Professional activity, weighted mean % (99% CI) | ||||||
Clinical | 84 (78, 89)* | 86 (81, 90) | 78 (72, 84) | 79 (76, 82) | 58 (51, 64)* | <0.001* |
Teaching | 2.3 (1, 5)* | 3 (1, 4) | 6 (4, 9) | 6 (5, 8) | 17 (14, 20)* | <0.001* |
Administration and Committee work | 13 (8, 19) | 11 (8, 15)* | 16 (10, 21) | 14 (12, 17) | 19 (14, 24)* | 0.001* |
Research | 0 (0, 0)* | 1 (0, 2) | 0 (0, 1) | 1 (0, 1) | 7 (3, 11)* | <0.001* |
Table 3 tabulates other work pattern characteristics. Most hospitalists indicated that their current clinical work as hospitalists involved the general medical wards (100%), medical consultations (98%), and comanagement with specialists (92%). There were wide differences in participation in comanagement (100%, local groups vs 71%, academic), intensive care unit (ICU) responsibilities (94%, multistate groups vs 27%, academic), and nursing home care (30%, local groups vs 8%, academic). Among activities that are potentially not reimbursable, academic hospitalists were less likely to participate in coordination of patient transfers and code or rapid response teams, while multistate groups were least likely to participate in quality improvement activities. In total, 99% of hospitalists reported participating in at least 1 potentially nonreimbursable clinical activity.
Local Hospitalist‐Only Group | Multi‐State Hospitalist Group | Multispecialty Physician Group | Employer Hospital | University or Medical School | ||
---|---|---|---|---|---|---|
n = 95 | n = 111 | n = 115 | n = 348 | n = 107 | P Value | |
| ||||||
Reimbursable activities, overlapping weighted % | ||||||
General medical ward | 100 | 99 | 100 | 99 | 99 | 0.809 |
Medical consultations | 99 | 99 | 100 | 98 | 95 | 0.043 |
Comanagement with specialists | 100 | 96 | 96 | 93 | 71 | <0.001 |
Preoperative evaluations | 92 | 92 | 90 | 88 | 77 | 0.002 |
Intensive care unit | 86 | 94 | 67 | 75 | 27 | <0.001 |
Skilled nursing facility or long‐term acute care facility | 30 | 19 | 12 | 16 | 8 | <0.001 |
Outpatient general medical practice | 4 | 4 | 5 | 5 | 10 | 0.241 |
Potentially nonreimbursable activities, overlapping weighted % | ||||||
Coordination of patient transfers | 92 | 94 | 95 | 93 | 82 | 0.005 |
Quality improvement or patient safety initiatives | 81 | 78 | 83 | 89 | 89 | 0.029 |
Code team or rapid response team | 56 | 57 | 53 | 62 | 37 | <0.001 |
Information technology design or implementation | 42 | 39 | 47 | 51 | 51 | 0.154 |
Admission triage for emergency department | 49 | 46 | 43 | 40 | 31 | 0.132 |
Compensation scheme, weighted % | <0.001 | |||||
Salary only | 18 | 21 | 30 | 29 | 47 | |
Salary plus performance incentive | 54 | 72 | 59 | 67 | 53 | |
Fee‐for‐service | 20 | 1 | 7 | 2 | 0 | |
Capitation | 0 | 0 | 0 | 0 | 0 | |
Other | 9 | 7 | 4 | 3 | 0 | |
Compensation links to incentives, overlapping weighted % | ||||||
No incentives | 40 | 28 | 29 | 29 | 48 | 0.003 |
Patient satisfaction | 23 | 39 | 38 | 38 | 14 | <0.001 |
Length of stay | 18 | 17 | 20 | 13 | 10 | 0.208 |
Overall cost | 8 | 11 | 9 | 5 | 6 | 0.270 |
Test utilization | 2 | 2 | 7 | 1 | 0 | <0.001 |
Clinical processes and outcomes | 26 | 34 | 44 | 43 | 24 | <0.001 |
Other | 17 | 29 | 26 | 31 | 25 | 0.087 |
Earnings, weighted mean dollars (99% CI) | 226,065 (202,891, 249,240)* | 225,613 (210,772, 240,454) | 202,617 (186,036, 219,198) | 206,087 (198,413, 213,460) | 166,478 (151,135, 181,821)* | <0.001* |
Hospitalist compensation schemes were significantly different across the practice models. Salary‐only schemes were most common among academic hospitalists (47%), while 72% of multistate groups used performance incentives in addition to salary. More local groups used fee‐for‐service compensation than other models. Incentives differed by practice model, with more multistate groups having incentives based on patient satisfaction, while more multispecialty physician groups had incentives based on clinical processes and outcomes than other models. Finally, mean earnings for academic hospitalists were significantly lower than for hospitalists of other practice models. Local and multistate group hospitalists earned more than any other practice model (all P <0.001), and $60,000 more than the lowest compensated academic hospitalists.
Components of Job Satisfaction
Hospitalists' rankings of the most important factors for job satisfaction revealed differences across models (Figure 2). Overall, hospitalists were most likely to consider optimal workload and compensation as important factors for job satisfaction from a list of 13 considerations. Local groups and academics were least likely to rank optimal workload as a top factor, and local group hospitalists were more likely to rank optimal autonomy than those of other models. Academic hospitalists had less concern for substantial pay, and more concern for the variety of tasks they perform and recognition by leaders, than other hospitalists.
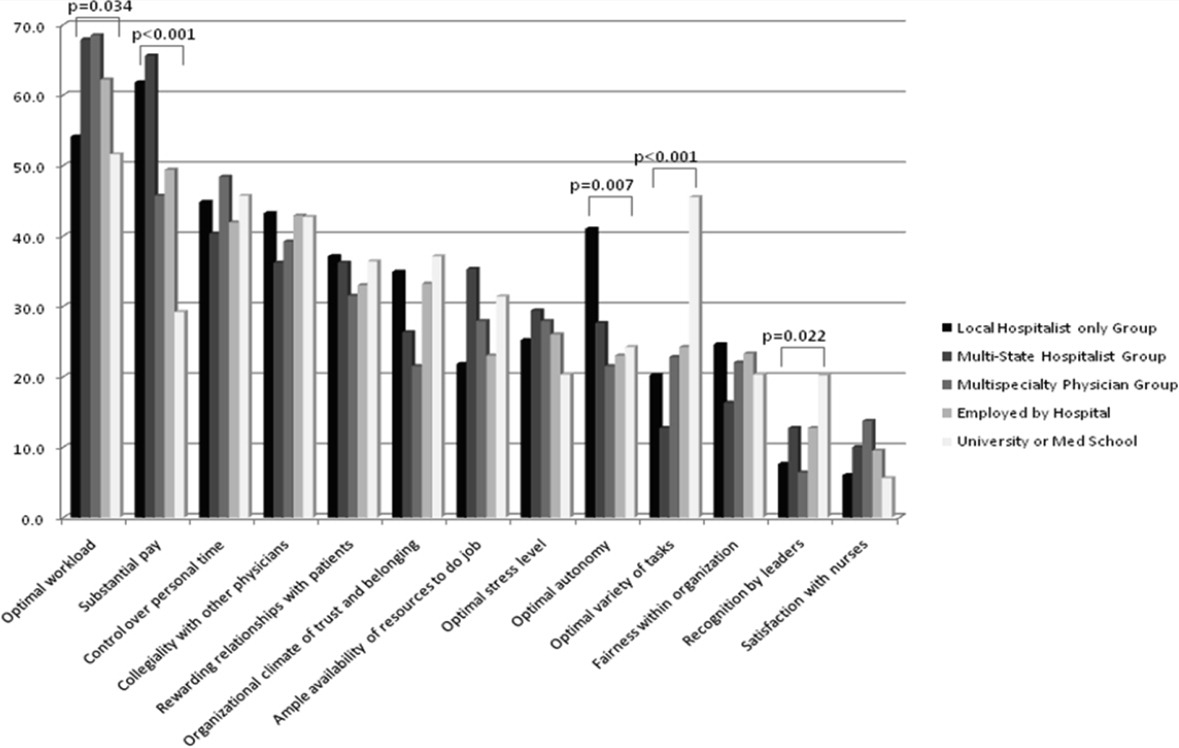
Job Satisfaction and Burnout Risk
Differences in the ratings of 4 of the 11 satisfaction and job characteristic domains were found across the practice models (Figure 3). Multispecialty group hospitalists were less satisfied with autonomy and their relationship with patients than other practice models, and along with multistate groups, reported the highest perceived workload. Organizational fairness was rated much higher by local group hospitalists than other practice models. Despite these differences in work patterns and satisfaction, there were no differences found in level of global job satisfaction, specialty satisfaction, or burnout across the practice models. Overall, 62% of respondents reported high job satisfaction (4 on a 1 to 5 scale), and 30% indicated burnout symptoms.
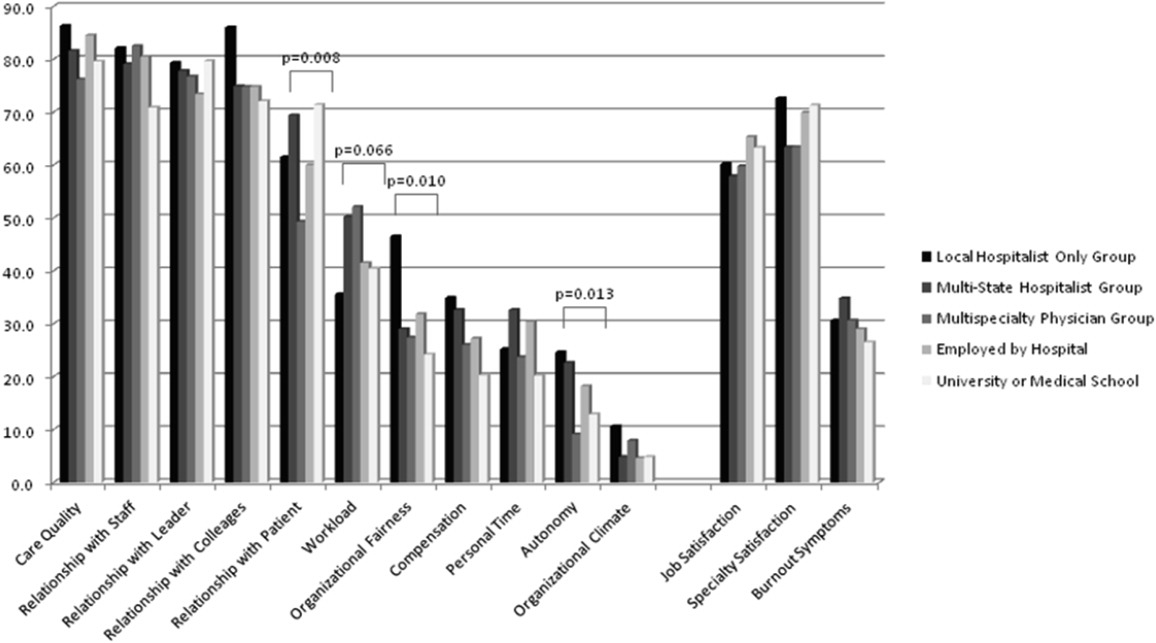
DISCUSSION
In our sample of US hospitalists, we found major differences in work patterns and compensation across hospitalist practice models, but no differences in job satisfaction, specialty satisfaction, and burnout. In particular, differences across these models included variations in hospitalist workload, hours, pay, and distribution of work activities. We found that hospitalists perform a variety of clinical and nonclinical tasks, for many of which there are not standard reimbursement mechanisms. We also found that features of a job that individual hospitalists considered most important vary by practice model.
Previous analysis of this data explored the overall state of hospitalist satisfaction.16 The present analysis offers a glimpse into hospitalists' systems‐orientation through a deeper look at their work patterns. The growth in the number of hospitalists who participate in intensive care medicine, specialty comanagement, and other work that involves close working relationships with specialist physicians confirms collaborative care as one of the dominant drivers of the hospitalist movement. At the level of indirect patient care, nearly all hospitalists contributed to work that facilitates coordination, quality, patient safety, or information technology. Understanding the integrative value of hospitalists outside of their clinical productivity may be of interest to hospital administrators.
Global satisfaction measures were similar across practice models. This finding is particularly interesting given the major differences in job characteristics seen among the practice models. This similarity in global satisfaction despite real differences in the nature of the job suggests that individuals find settings that allow them to address their individual professional goals. Our study demonstrates that, in 2010, Hospital Medicine has evolved enough to accommodate a wide variety of goals and needs.
While global satisfaction did not differ among practice types, hospitalists from various models did report differences in factors considered important to global satisfaction. While workload and pay were rated as influential across most models, the degree of importance was significantly different. In academic settings, substantial pay was not a top consideration for overall job satisfaction, whereas in local and multistate hospitalist groups, pay was a very close second in importance to optimal workload. These results may prove helpful for individual hospitalists trying to find their optimal job. For example, someone who is less concerned about workload, but wants to be paid well and have a high degree of autonomy, may find satisfaction in local hospitalist groups. However, for someone who is willing to sacrifice a higher salary for variety of activities, academic Hospital Medicine may be a better fit.
There is a concerning aspect of hospitalist job satisfaction that different practice models do not seem to solve. Control over personal time is a top consideration for many hospitalists across practice models, yet their satisfaction with personal time is low. As control over personal time is seen as a draw to the Hospital Medicine specialty, group leaders may need to evaluate their programs to ensure that schedules and workload support efforts for hospitalists to balance work and homelife commitments.
There are additional findings that are important for Hospital Medicine group leaders. Regardless of practice model, compensation and workload are often used as tools to recruit and retain hospitalists. While these tools may be effective, leaders may find more nuanced approaches to improving their hospitalists' overall satisfaction. Leaders of local hospitalist groups may find their hospitalists tolerant of heavier workloads as long as they are adequately rewarded and are given real autonomy over their work. However, leaders of academic programs may be missing the primary factor that can improve their hospitalists' satisfaction. Rather than asking for higher salaries to remain competitive, it may be more effective to advocate for time and training for their hospitalists to pursue important other activities beyond direct clinical care. Given that resources will always be limited, group leaders need to understand all of the elements that can contribute to hospitalist job satisfaction.
We point out several limitations to this study. First, our adjusted response rate of 25.6% is low for survey research, in general. As mentioned above, hospitalists are not easily identified in any available national physician database. Therefore, we deliberately designed our sampling strategy to error on the side of including ineligible surveyees to reduce systematic exclusion of practicing hospitalists. Using simple post hoc methods, we identified many nonhospitalists and bad addresses from our sample, but because these methods were exclusionary as opposed to confirmatory, we believe that a significant proportion of remaining nonrespondents may also have been ineligible for the survey. Although this does not fully address concerns about potential response bias, we believe that our sample representing a large number of hospitalist groups is adequate to make estimations about a nationally representative sample of practicing hospitalists. Second, in spite of our inclusive approach, we may still have excluded categories of practicing hospitalists. We were careful not to allow SHM members to represent all US hospitalists and included non‐members in the sampling frame, but the possibility of systematic exclusion that may alter our results remains a concern. Additionally, one of our goals was to characterize pediatric hospitalists independently from their adult‐patient counterparts. Despite oversampling of pediatricians, their sample was too small for a more detailed comparison across practice models. Also, self‐reported data about workload and compensation are subject to inaccuracies related to recall and cognitive biases. Last, this is a cross‐sectional study of hospitalist satisfaction at one point in time. Consequently, our sample may not be representative of very dissatisfied hospitalists who have already left their jobs.
The diversity found across existing practice models and the characteristics of the practices provide physicians with the opportunity to bring their unique skills and motivations to the hospitalist movement. As hospitals and other organizations seek to create, maintain, or grow hospitalist programs, the data provided here may prove useful to understand the relationship between practice characteristics and individual job satisfaction. Additionally, hospitalists looking for a job can consider these results as additional information to guide their choice of practice model and work patterns.
Acknowledgements
The authors thank Kenneth A. Rasinski for assistance with survey items refinement, and members of the SHM Career Satisfaction Task Force for their assistance in survey development.
- The status of hospital medicine groups in the United States.J Hosp Med.2006;1(2):75–80. , , , .
- Growth in the care of older patients by hospitalists in the United States.N Engl J Med.2009;360(11):1102–1112. , , , .
- The state of hospital medicine in 2008.Med Clin North Am.2008;92(2):265–273,vii. .
- Health care market trends and the evolution of hospitalist use and roles.J Gen Intern Med.2005;20(2):101–107. , , , .
- Effects of physician experience on costs and outcomes on an academic general medicine service: results of a trial of hospitalists.Ann Intern Med.2002;137(11):866–874. , , , et al.
- The Park Nicollet experience in establishing a hospitalist system.Ann Intern Med.1999;130(4 pt 2):350–354. .
- Effects of an HMO hospitalist program on inpatient utilization.Am J Manag Care.2001;7(11):1051–1057. , .
- The impact of hospitalists on the cost and quality of inpatient care in the United States: a research synthesis.Med Care Res Rev.2005;62(4):379–406. , .
- Pediatric hospitalists: a systematic review of the literature.Pediatrics.2006;117(5):1736–1744. , , , .
- The hospitalist movement 5 years later.JAMA.2002;287(4):487–494. , .
- Trends in market demand for internal medicine 1999 to 2004: an analysis of physician job advertisements.J Gen Intern Med.2006;21(10):1079–1085. , , , , , .
- SHM. 2003–2004 Survey by the Society of Hospital Medicine on Productivity and Compensation: Analysis of Results. 2004 [updated 2004]. Available at: http://www.hospitalmedicine.org/AM/Template. cfm?Section=Practice_Resources Available at: http://cme.medscape.com/viewarticle/578134. Accessed October 21,2010.
- State of Hospital Medicine: 2010 Report Based on 2009 Data.Englewood, CO and Philadelphia, PA:Medical Group Management Association and Society of Hospital Medicine;2010.
- Worklife and satisfaction of hospitalists: toward flourishing careers.J Gen Intern Med.2011, Jul 20. PMID: 21773849. , , , , .
- Worklife and satisfaction of general internists.Arch Intern Med.2002;162(6):649–656. , , , et al.
- Organizational climate, stress, and error in primary care: the MEMO study. In: Henriksen K, Battles JB, Marks ES, Lewin DI, eds.Advances in Patient Safety: From Research to Implementation. Vol 1: Research Findings.Rockville, MD:Agency for Healthcare Research and Quality;2005;1:65–77. , , , et al.
- Hospitalists and the practice of inpatient medicine: results of a survey of the National Association of Inpatient Physicians.Ann Intern Med.1999;130(4 pt 2):343–349. , , , .
- Physician attitudes toward and prevalence of the hospitalist model of care: results of a national survey.Am J Med.2000;109(8):648–653. , , , , , .
- Taking the Measure of Work: A Guide to Validated Scales for Organizational Research and Diagnosis.Thousand Oaks, CA:Sage Publications;2002. .
- Job Demands and Worker Health.Ann Arbor, MI:University of Michigan, Institute for Social Research;1980. , , , , .
- On the dimensionality of organizational justice: a construct validation of a measure.J Appl Psychol.2001;86(3):386–400. .
- Effect of job demands and social support on worker stress—a study of VDT users.Behav Inform Technol.1995;14(1):32–40. , .
- Measuring physician job satisfaction in a changing workplace and a challenging environment. SGIM Career Satisfaction Study Group. Society of General Internal Medicine.Med Care.1999;37(11):1174–1182. , , , et al.
- Working conditions in primary care: physician reactions and care quality.Ann Intern Med.2009;151(1):28–U48. , , , et al.
- Validation of a single‐item measure of burnout against the Maslach Burnout Inventory among physicians.Stress Health.2004;20(2):75–79. , , .
- American Hospital Association. AHA Hospital Statistics. 2009 [updated 2009]. Available at: http://www.ahadata.com/ahadata/html/AHAStatistics.html. Accessed April 12,2011.
- How to obtain excellent response rates when surveying physicians.Fam Pract.2009;26(1):65–68. , , , et al.
- Estimating nonresponse bias in mail surveys.J Marketing Res.1977;14(3):396–402. , .
Over the past 15 years, there has been dramatic growth in the number of hospitalist physicians in the United States and in the number of hospitals served by them.13 Hospitals are motivated to hire experienced hospitalists to staff their inpatient services,4 with goals that include obtaining cost‐savings and higher quality.59 The rapid growth of Hospital Medicine saw multiple types of hospital practice models emerge with differing job characteristics, clinical duties, workload, and compensation schemes.10 The extent of the variability of hospitalist jobs across practice models is not known.
Intensifying recruitment efforts and the concomitant increase in compensation for hospitalists over the last decade suggest that demand for hospitalists is strong and sustained.11 As a result, today's cohort of hospitalists has a wide range of choices of types of jobs, practice models, and locations. The diversity of available hospitalist jobs is characterized, for example, by setting (community hospital vs academic hospital), employer (hospital vs private practice), job duties (the amount and type of clinical work, and other administrative, teaching, or research duties), and intensity (work hours and duties to maximize income or lifestyle). How these choices relate to job satisfaction and burnout are also unknown.
The Society of Hospital Medicine (SHM) has administered surveys to hospitalist group leaders biennially since 2003.1215 These surveys, however, do not address issues related to individual hospitalist worklife, recruitment, and retention. In 2005, SHM convened a Career Satisfaction Task Force that designed and executed a national survey of hospitalists in 2009‐2010. The objective of this study is to evaluate how job characteristics vary by practice model, and the association of these characteristics and practice models with job satisfaction and burnout.
METHODS
Survey Instrument
A detailed description of the survey design, sampling strategy, data collection, and response rate calculations is described elsewhere.16 Portions of the 118‐item survey instrument assessed characteristics of the respondents' hospitalist group (12 items), details about their individual work patterns (12 items), and demographics (9 items). Work patterns were evaluated by the average number of clinical work days, consecutive days, hours per month, percentage of work assigned to night duty, and number of patient encounters. Average hours spent on nonclinical work, and the percentage of time allocated for clinical, administrative, teaching, and research activities were solicited. Additional items assessed specific clinical responsibilities, pretax earnings in FY2010, the availability of information technology capabilities, and the adequacy of available resources. Job and specialty satisfaction and 11 satisfaction domain measures were measured using validated scales.1726 Burnout symptoms were measured using a validated single‐item measure.26, 27
Sampling Strategy
We surveyed a national stratified sample of hospitalists in the US and Puerto Rico. We used the largest database of hospitalists (>24,000 names) currently available and maintained by the SHM as our sampling frame. We linked hospitalist employer information to hospital statistics from the American Hospital Association database28 to stratify the sample by number of hospital beds, geographic region, employment model, and specialty training, oversampling pediatric hospitalists due to small numbers. A respondent sample of about 700 hospitalists was calculated to be adequate to detect a 0.5 point difference in job satisfaction scores between subgroups assuming 90% power and alpha of 0.05. However, we sampled a total of 5389 addresses from the database to overcome the traditionally low physician response rates, duplicate sampling, bad addresses, and non‐hospitalists being included in the sampling frame. In addition, 2 multistate hospitalist companies (EmCare, In Compass Health) and 1 for‐profit hospital chain (HCA, Inc) financially sponsored this project with the stipulation that all of their hospitalist employees (n = 884) would be surveyed.
Data Collection
The healthcare consulting firm, Press Ganey, provided support with survey layout and administration following the modified Dillman method.29 Three rounds of coded surveys and solicitation letters from the investigators were mailed 2 weeks apart in November and December 2009. Because of low response rates to the mailed survey, an online survey was created using Survey Monkey and sent to 650 surveyees for whom e‐mail addresses were available, and administered at a kiosk for sample physicians during the SHM 2010 annual meeting.
Data Analysis
Nonresponse bias was measured by comparing characteristics between respondents of separate survey waves.30 We determined the validity of mailing addresses immediately following the survey period by mapping each address using Google, and if the address was a hospital, researching online whether or not the intended recipient was currently employed there. Practice characteristics were compared across 5 model categories distilled from the SHM & Medical Group Management Association survey: local hospitalist‐only group, multistate hospitalist group, multispecialty physician group, employer hospital, and university or medical school. Weighted proportions, means, and medians were calculated to account for oversampling of pediatric hospitalists. Differences in categorical measures were assessed using the chi‐square test and the design‐based F test for comparing weighted data. Weighted means (99% confidence intervals) and medians (interquartile ranges) were calculated. Because each parameter yielded a single outlier value across the 5 practice models, differences across weighted means were assessed using generalized linear models with the single outlier value chosen as the reference mean. Pair‐wise Wilcoxon rank sum test was used to compare median values. In these 4‐way comparisons of means and medians, significance was defined as P value of 0.0125 per Bonferroni correction. A single survey item solicited respondents to choose exactly 4 of 13 considerations most pertinent to job satisfaction. The proportion of respondents who scored 4 on a 5‐point Likert scale of the 11 satisfaction domains and 2 global measures of satisfaction, and burnout symptoms defined as 3 on a 5‐point single item measure were bar‐graphed. Chi‐square statistics were used to evaluate for differences across practice models. Statistical significance was defined by alpha less than 0.05, unless otherwise specified. All analyses were performed using STATA version 11.0 (College Station, TX). This study was approved by the Loyola University Institutional Review Board.
Survey data required cleaning prior to analysis. Missing gender information was imputed using the respondents' name. Responses to the item that asked to indicate the proportion of work dedicated to administrative responsibilities, clinical care, teaching, and research that did not add up to 100% were dropped. Two responses that indicated full‐time equivalent (FTE) of 0%, but whose respondents otherwise completed the survey implying they worked as clinical hospitalists, were replaced with values calculated from the given number of work hours relative to the median work hours in our sample. Out of range or implausible responses to the following items were dropped from analyses: the average number of billable encounters during a typical day or shift, number of shifts performing clinical activities during a typical month, pretax earnings, the year the respondent completed residency training, and the number of whole years practiced as a hospitalist. The proportion of selective item nonresponse was small and we did not, otherwise, impute missing data.
RESULTS
Response Rate
Of the 5389 originally sampled addresses, 1868 were undeliverable. Addresses were further excluded if they appeared in duplicate or were outdated. This yielded a total of 3105 eligible surveyees in the sample. As illustrated in Figure 1, 841 responded to the mailed survey and 5 responded to the Web‐based survey. After rejecting 67 non‐hospitalist respondents and 3 duplicate surveys, a total of 776 surveys were included in the final analysis. The adjusted response rate was 25.6% (776/3035). Members of SHM were more likely to return the survey than nonmembers. The adjusted response rate from hospitalists affiliated with the 3 sponsoring institutions was 6% (40/662). Because these respondents were more likely to be non‐members of SHM, we opted to analyze the responses from the sponsor hospitalists together with the sampled hospitalists. The demographics of the resulting pool of 816 respondents affiliated with over 650 unique hospitalist groups were representative of the original survey frame. We analyzed data from 794 of these who responded to the item indicating their hospitalist practice model. Demographic characteristics of responders and nonresponders to the practice model survey item were similar.
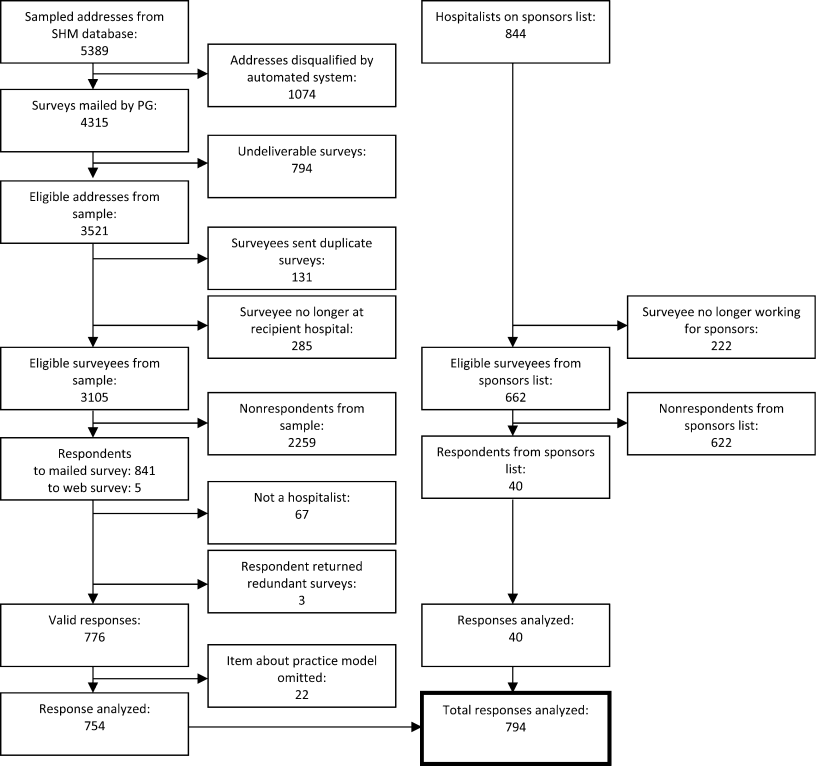
Characteristics of Hospitalists and Their Groups
Table 1 summarizes the characteristics of hospitalist respondents and their organizations by practice model. More (44%) respondents identified their practice model as directly employed by the hospital than other models, including multispecialty physician group (15%), multistate hospitalist group (14%), university or medical school (14%), local hospitalist group (12%), and other (2%). The median age of hospitalist respondents was 42 years, with 6.8 years of mean experience as a hospitalist. One third were women, 84% were married, and 46% had dependent children 6 years old or younger at home. Notably, hospitalists in multistate groups had fewer years of experience, and fewer hospitalists in local and multistate groups were married compared to hospitalists in other practice models.
Local Hospitalist‐Only Group | Multi‐State Hospitalist Group | Multispecialty Physician Group | Employer Hospital | University or Medical School | ||
---|---|---|---|---|---|---|
n = 95 | n = 111 | n = 115 | n = 348 | n = 107 | P Value | |
| ||||||
Hospitalist characteristics | ||||||
Age, weighted mean (99% CI) | 45 (42, 48) | 44 (42, 47) | 45 (43, 47) | 45 (43, 46) | 43 (40, 46) | |
Years hospitalist experience, weighted mean (99% CI) | 8 (6, 9)* | 5 (4, 6)* | 8 (7, 9) | 7 (6, 7) | 8 (6, 9) | <0.010* |
Women, weighted % | 29 | 30 | 39 | 31 | 43 | 0.118 |
Married, weighted % | 76 | 77 | 82 | 89 | 81 | 0.009 |
At least 1 dependent child younger than age 6 living in home, weighted % | 47 | 48 | 43 | 47 | 45 | 0.905 |
Pediatric specialty, n (%) | <10 | <10 | 11 (10%) | 57 (16%) | 36 (34%) | <0.001 |
Hospitalist group characteristics | ||||||
Region, weighted % | <0.001 | |||||
Northeast (AHA 1 & 2) | 13 | 10 | 16 | 27 | 13 | |
South (AHA 3 & 4) | 19 | 37 | 13 | 24 | 21 | |
Midwest (AHA 5 & 6) | 23 | 24 | 25 | 22 | 26 | |
Mountain (AHA 7 & 8) | 22 | 20 | 16 | 13 | 24 | |
West (AHA 9) | 24 | 10 | 31 | 14 | 16 | |
No. beds of primary hospital, weighted % | <0.001 | |||||
Up to 149 | 17 | 26 | 12 | 24 | 14 | |
150299 | 30 | 36 | 36 | 33 | 21 | |
300449 | 26 | 24 | 29 | 20 | 19 | |
450599 | 13 | 8 | 17 | 11 | 21 | |
600 or more | 12 | 6 | 7 | 13 | 24 | |
No. of hospital facilities served by current practice, weighted % | <0.001 | |||||
1 | 53 | 70 | 67 | 77 | 66 | |
2 | 20 | 22 | 20 | 16 | 24 | |
3 or more | 27 | 9 | 13 | 7 | 10 | |
No. of physicians in current practice, median (IQR) | 10 (5, 18) | 8 (6, 12)* | 14 (8, 25)* | 12 (6, 18) | 12 (7, 20) | <0.001*, 0.001 |
No. of non‐physician providers in current practice, median (IQR) | 0 (0, 2) | 0 (0, 2) | 0 (0, 3) | 1 (0, 2) | 0 (0, 2) | |
Available information technology capabilities, weighted % | ||||||
EHR to access physician notes | 57 | 57 | 75 | 58 | 79 | <0.001 |
EHR to access nursing documentations | 68 | 67 | 74 | 75 | 76 | 0.357 |
EHR to access laboratory or test results | 97 | 89 | 95 | 96 | 96 | 0.054 |
Electronic order entry | 30 | 19 | 53 | 38 | 56 | <0.001 |
Electronic billing | 38 | 31 | 36 | 36 | 38 | 0.818 |
Access to EHR at home or off site | 78 | 73 | 78 | 82 | 84 | 0.235 |
Access to Up‐to‐Date or other clinical guideline resources | 80 | 77 | 91 | 92 | 96 | <0.001 |
Access to schedules, calendars, or other organizational resources | 56 | 57 | 66 | 67 | 75 | 0.024 |
E‐mail, Web‐based paging, or other communication resources | 74 | 63 | 88 | 89 | 90 | <0.001 |
Several differences in respondent group characteristics by practice model were found. Respondents in multistate hospitalist groups were more likely from the South and Midwest, while respondents from multispecialty groups were likely from the West. More multistate group practices were based in smaller hospitals, while academic hospitalists tended to practice in hospitals with 600 or more beds. Respondents employed by hospitals were more likely to practice at 1 hospital facility only, while local group practices were more likely to practice at 3 or more facilities. The median number of physicians in a hospitalist group was 11 (interquartile range [IQR] 6, 19). Local and multistate groups had fewer hospitalists compared to other models. Nonphysician providers were employed by nearly half of all hospitalist practices. Although almost all groups had access to some information technology, more academic hospitalists had access to electronic order entry, electronic physician notes, electronic clinical guidelines resources and communication technology, while local and multistate groups were least likely to have access to these resources.
Work Pattern Variations
Table 2 further details hospitalist work hours by practice model. The majority of hospitalists (78%) reported their position was full‐time (FTE 1.0), while 13% reported working less than full‐time (FTE <1.0). Only 5% of local group hospitalists worked part‐time, while 20% of multispecialty group hospitalists did. An additional 9% reported FTE >1.0, indicating their work hours exceeded the definition of a full‐time physician in their practice. Among full‐time hospitalists, local group members worked a greater number of shifts per month than employees of multispecialty groups, hospitals, and academic medical centers. Academic hospitalists reported higher numbers of consecutive clinical days worked on average, but fewer night shifts compared to hospitalists employed by multistate groups, multispecialty groups, and hospitals; fewer billable encounters than hospitalists in local and multistate groups; and more nonclinical work hours than hospitalists of any other practice model. Academic hospitalists also spent more time on teaching and research than other practice models. Hospitalists spent 11%‐18% of their time on administrative and committee responsibilities, with the least amount spent by hospitalists in multistate groups and the most in academic practice.
Local Hospitalist‐Only Group | Multi‐State Hospitalist Group | Multispecialty Physician Group | Employer Hospital | University or Medical School | ||
---|---|---|---|---|---|---|
n = 95 | n = 111 | n = 115 | n = 348 | n = 107 | P Value | |
| ||||||
FTE, weighted % | 0.058 | |||||
FTE < 1.0 | 6 | 13 | 20 | 12 | 14 | |
FTE = 1.0 | 85 | 75 | 74 | 80 | 82 | |
FTE > 1.0 | 10 | 13 | 6 | 8 | 5 | |
Workload parameters, weighted mean (99% CI) | ||||||
Clinical shifts per month for FTE 1.0 | 19 (17, 20)* | 17 (16, 19) | 15 (14, 17)* | 16 (15, 16) | 15 (13, 17) | <0.001* |
Hours per clinical shift | 10 (9, 11) | 11 (10, 11)* | 10 (10, 11.0) | 11 (10, 11.0) | 10 (9, 10)* | 0.006*, 0.002 |
Consecutive days on clinical shift | 8 (6, 9) | 7 (6, 7)* | 6 (6, 7) | 7 (6, 7) | 9 (7, 10)* | 0.002*, <0.001 |
% Clinical shifts on nights | 20 (15, 25) | 23 (18, 28)* | 23 (17, 29) | 21 (17, 24) | 14 (9, 18)* | 0.001*, 0.002 |
% Night shifts spent in hospital | 61 (49, 74)* | 63 (52, 75) | 72 (62, 83) | 73 (67, 80) | 43 (29, 57)* | 0.010*, 0.003, <0.001 |
Billable encounters per clinical shift | 17 (14, 19)* | 17 (16, 18) | 14 (13, 15) | 15 (14, 16) | 13 (11, 14)* | <0.001*, 0.002 |
Hours nonclinical work per month | 23 (12, 34)* | 19 (11, 27) | 31 (20, 42) | 30 (24, 36) | 71 (55, 86)* | <0.001* |
Hours clinical and nonclinical work per month for FTE 1.0 | 202 (186, 219) | 211 (196, 226) | 184 (170, 198)* | 193 (186, 201) | 221 (203, 238)* | <0.001* |
Professional activity, weighted mean % (99% CI) | ||||||
Clinical | 84 (78, 89)* | 86 (81, 90) | 78 (72, 84) | 79 (76, 82) | 58 (51, 64)* | <0.001* |
Teaching | 2.3 (1, 5)* | 3 (1, 4) | 6 (4, 9) | 6 (5, 8) | 17 (14, 20)* | <0.001* |
Administration and Committee work | 13 (8, 19) | 11 (8, 15)* | 16 (10, 21) | 14 (12, 17) | 19 (14, 24)* | 0.001* |
Research | 0 (0, 0)* | 1 (0, 2) | 0 (0, 1) | 1 (0, 1) | 7 (3, 11)* | <0.001* |
Table 3 tabulates other work pattern characteristics. Most hospitalists indicated that their current clinical work as hospitalists involved the general medical wards (100%), medical consultations (98%), and comanagement with specialists (92%). There were wide differences in participation in comanagement (100%, local groups vs 71%, academic), intensive care unit (ICU) responsibilities (94%, multistate groups vs 27%, academic), and nursing home care (30%, local groups vs 8%, academic). Among activities that are potentially not reimbursable, academic hospitalists were less likely to participate in coordination of patient transfers and code or rapid response teams, while multistate groups were least likely to participate in quality improvement activities. In total, 99% of hospitalists reported participating in at least 1 potentially nonreimbursable clinical activity.
Local Hospitalist‐Only Group | Multi‐State Hospitalist Group | Multispecialty Physician Group | Employer Hospital | University or Medical School | ||
---|---|---|---|---|---|---|
n = 95 | n = 111 | n = 115 | n = 348 | n = 107 | P Value | |
| ||||||
Reimbursable activities, overlapping weighted % | ||||||
General medical ward | 100 | 99 | 100 | 99 | 99 | 0.809 |
Medical consultations | 99 | 99 | 100 | 98 | 95 | 0.043 |
Comanagement with specialists | 100 | 96 | 96 | 93 | 71 | <0.001 |
Preoperative evaluations | 92 | 92 | 90 | 88 | 77 | 0.002 |
Intensive care unit | 86 | 94 | 67 | 75 | 27 | <0.001 |
Skilled nursing facility or long‐term acute care facility | 30 | 19 | 12 | 16 | 8 | <0.001 |
Outpatient general medical practice | 4 | 4 | 5 | 5 | 10 | 0.241 |
Potentially nonreimbursable activities, overlapping weighted % | ||||||
Coordination of patient transfers | 92 | 94 | 95 | 93 | 82 | 0.005 |
Quality improvement or patient safety initiatives | 81 | 78 | 83 | 89 | 89 | 0.029 |
Code team or rapid response team | 56 | 57 | 53 | 62 | 37 | <0.001 |
Information technology design or implementation | 42 | 39 | 47 | 51 | 51 | 0.154 |
Admission triage for emergency department | 49 | 46 | 43 | 40 | 31 | 0.132 |
Compensation scheme, weighted % | <0.001 | |||||
Salary only | 18 | 21 | 30 | 29 | 47 | |
Salary plus performance incentive | 54 | 72 | 59 | 67 | 53 | |
Fee‐for‐service | 20 | 1 | 7 | 2 | 0 | |
Capitation | 0 | 0 | 0 | 0 | 0 | |
Other | 9 | 7 | 4 | 3 | 0 | |
Compensation links to incentives, overlapping weighted % | ||||||
No incentives | 40 | 28 | 29 | 29 | 48 | 0.003 |
Patient satisfaction | 23 | 39 | 38 | 38 | 14 | <0.001 |
Length of stay | 18 | 17 | 20 | 13 | 10 | 0.208 |
Overall cost | 8 | 11 | 9 | 5 | 6 | 0.270 |
Test utilization | 2 | 2 | 7 | 1 | 0 | <0.001 |
Clinical processes and outcomes | 26 | 34 | 44 | 43 | 24 | <0.001 |
Other | 17 | 29 | 26 | 31 | 25 | 0.087 |
Earnings, weighted mean dollars (99% CI) | 226,065 (202,891, 249,240)* | 225,613 (210,772, 240,454) | 202,617 (186,036, 219,198) | 206,087 (198,413, 213,460) | 166,478 (151,135, 181,821)* | <0.001* |
Hospitalist compensation schemes were significantly different across the practice models. Salary‐only schemes were most common among academic hospitalists (47%), while 72% of multistate groups used performance incentives in addition to salary. More local groups used fee‐for‐service compensation than other models. Incentives differed by practice model, with more multistate groups having incentives based on patient satisfaction, while more multispecialty physician groups had incentives based on clinical processes and outcomes than other models. Finally, mean earnings for academic hospitalists were significantly lower than for hospitalists of other practice models. Local and multistate group hospitalists earned more than any other practice model (all P <0.001), and $60,000 more than the lowest compensated academic hospitalists.
Components of Job Satisfaction
Hospitalists' rankings of the most important factors for job satisfaction revealed differences across models (Figure 2). Overall, hospitalists were most likely to consider optimal workload and compensation as important factors for job satisfaction from a list of 13 considerations. Local groups and academics were least likely to rank optimal workload as a top factor, and local group hospitalists were more likely to rank optimal autonomy than those of other models. Academic hospitalists had less concern for substantial pay, and more concern for the variety of tasks they perform and recognition by leaders, than other hospitalists.
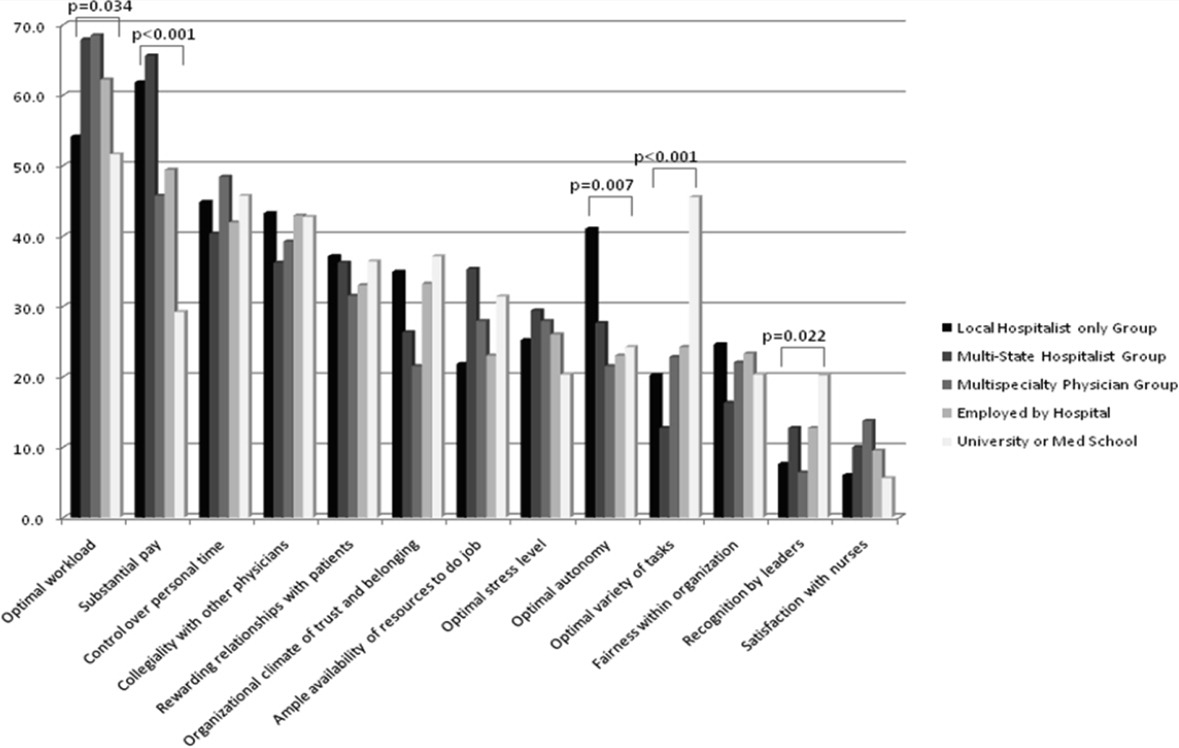
Job Satisfaction and Burnout Risk
Differences in the ratings of 4 of the 11 satisfaction and job characteristic domains were found across the practice models (Figure 3). Multispecialty group hospitalists were less satisfied with autonomy and their relationship with patients than other practice models, and along with multistate groups, reported the highest perceived workload. Organizational fairness was rated much higher by local group hospitalists than other practice models. Despite these differences in work patterns and satisfaction, there were no differences found in level of global job satisfaction, specialty satisfaction, or burnout across the practice models. Overall, 62% of respondents reported high job satisfaction (4 on a 1 to 5 scale), and 30% indicated burnout symptoms.
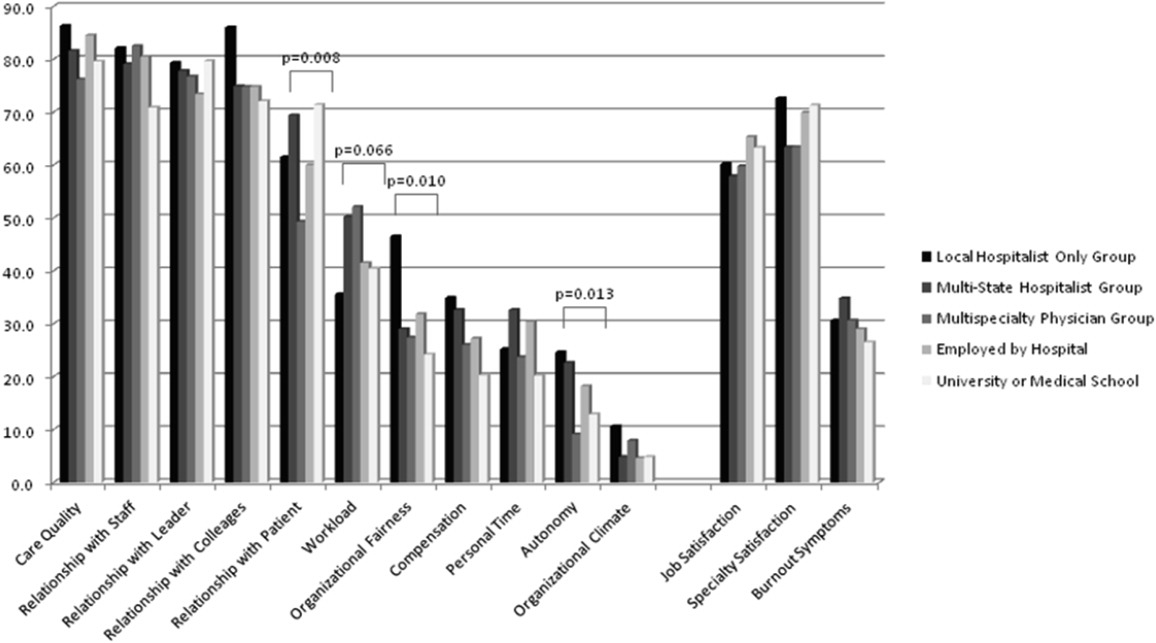
DISCUSSION
In our sample of US hospitalists, we found major differences in work patterns and compensation across hospitalist practice models, but no differences in job satisfaction, specialty satisfaction, and burnout. In particular, differences across these models included variations in hospitalist workload, hours, pay, and distribution of work activities. We found that hospitalists perform a variety of clinical and nonclinical tasks, for many of which there are not standard reimbursement mechanisms. We also found that features of a job that individual hospitalists considered most important vary by practice model.
Previous analysis of this data explored the overall state of hospitalist satisfaction.16 The present analysis offers a glimpse into hospitalists' systems‐orientation through a deeper look at their work patterns. The growth in the number of hospitalists who participate in intensive care medicine, specialty comanagement, and other work that involves close working relationships with specialist physicians confirms collaborative care as one of the dominant drivers of the hospitalist movement. At the level of indirect patient care, nearly all hospitalists contributed to work that facilitates coordination, quality, patient safety, or information technology. Understanding the integrative value of hospitalists outside of their clinical productivity may be of interest to hospital administrators.
Global satisfaction measures were similar across practice models. This finding is particularly interesting given the major differences in job characteristics seen among the practice models. This similarity in global satisfaction despite real differences in the nature of the job suggests that individuals find settings that allow them to address their individual professional goals. Our study demonstrates that, in 2010, Hospital Medicine has evolved enough to accommodate a wide variety of goals and needs.
While global satisfaction did not differ among practice types, hospitalists from various models did report differences in factors considered important to global satisfaction. While workload and pay were rated as influential across most models, the degree of importance was significantly different. In academic settings, substantial pay was not a top consideration for overall job satisfaction, whereas in local and multistate hospitalist groups, pay was a very close second in importance to optimal workload. These results may prove helpful for individual hospitalists trying to find their optimal job. For example, someone who is less concerned about workload, but wants to be paid well and have a high degree of autonomy, may find satisfaction in local hospitalist groups. However, for someone who is willing to sacrifice a higher salary for variety of activities, academic Hospital Medicine may be a better fit.
There is a concerning aspect of hospitalist job satisfaction that different practice models do not seem to solve. Control over personal time is a top consideration for many hospitalists across practice models, yet their satisfaction with personal time is low. As control over personal time is seen as a draw to the Hospital Medicine specialty, group leaders may need to evaluate their programs to ensure that schedules and workload support efforts for hospitalists to balance work and homelife commitments.
There are additional findings that are important for Hospital Medicine group leaders. Regardless of practice model, compensation and workload are often used as tools to recruit and retain hospitalists. While these tools may be effective, leaders may find more nuanced approaches to improving their hospitalists' overall satisfaction. Leaders of local hospitalist groups may find their hospitalists tolerant of heavier workloads as long as they are adequately rewarded and are given real autonomy over their work. However, leaders of academic programs may be missing the primary factor that can improve their hospitalists' satisfaction. Rather than asking for higher salaries to remain competitive, it may be more effective to advocate for time and training for their hospitalists to pursue important other activities beyond direct clinical care. Given that resources will always be limited, group leaders need to understand all of the elements that can contribute to hospitalist job satisfaction.
We point out several limitations to this study. First, our adjusted response rate of 25.6% is low for survey research, in general. As mentioned above, hospitalists are not easily identified in any available national physician database. Therefore, we deliberately designed our sampling strategy to error on the side of including ineligible surveyees to reduce systematic exclusion of practicing hospitalists. Using simple post hoc methods, we identified many nonhospitalists and bad addresses from our sample, but because these methods were exclusionary as opposed to confirmatory, we believe that a significant proportion of remaining nonrespondents may also have been ineligible for the survey. Although this does not fully address concerns about potential response bias, we believe that our sample representing a large number of hospitalist groups is adequate to make estimations about a nationally representative sample of practicing hospitalists. Second, in spite of our inclusive approach, we may still have excluded categories of practicing hospitalists. We were careful not to allow SHM members to represent all US hospitalists and included non‐members in the sampling frame, but the possibility of systematic exclusion that may alter our results remains a concern. Additionally, one of our goals was to characterize pediatric hospitalists independently from their adult‐patient counterparts. Despite oversampling of pediatricians, their sample was too small for a more detailed comparison across practice models. Also, self‐reported data about workload and compensation are subject to inaccuracies related to recall and cognitive biases. Last, this is a cross‐sectional study of hospitalist satisfaction at one point in time. Consequently, our sample may not be representative of very dissatisfied hospitalists who have already left their jobs.
The diversity found across existing practice models and the characteristics of the practices provide physicians with the opportunity to bring their unique skills and motivations to the hospitalist movement. As hospitals and other organizations seek to create, maintain, or grow hospitalist programs, the data provided here may prove useful to understand the relationship between practice characteristics and individual job satisfaction. Additionally, hospitalists looking for a job can consider these results as additional information to guide their choice of practice model and work patterns.
Acknowledgements
The authors thank Kenneth A. Rasinski for assistance with survey items refinement, and members of the SHM Career Satisfaction Task Force for their assistance in survey development.
Over the past 15 years, there has been dramatic growth in the number of hospitalist physicians in the United States and in the number of hospitals served by them.13 Hospitals are motivated to hire experienced hospitalists to staff their inpatient services,4 with goals that include obtaining cost‐savings and higher quality.59 The rapid growth of Hospital Medicine saw multiple types of hospital practice models emerge with differing job characteristics, clinical duties, workload, and compensation schemes.10 The extent of the variability of hospitalist jobs across practice models is not known.
Intensifying recruitment efforts and the concomitant increase in compensation for hospitalists over the last decade suggest that demand for hospitalists is strong and sustained.11 As a result, today's cohort of hospitalists has a wide range of choices of types of jobs, practice models, and locations. The diversity of available hospitalist jobs is characterized, for example, by setting (community hospital vs academic hospital), employer (hospital vs private practice), job duties (the amount and type of clinical work, and other administrative, teaching, or research duties), and intensity (work hours and duties to maximize income or lifestyle). How these choices relate to job satisfaction and burnout are also unknown.
The Society of Hospital Medicine (SHM) has administered surveys to hospitalist group leaders biennially since 2003.1215 These surveys, however, do not address issues related to individual hospitalist worklife, recruitment, and retention. In 2005, SHM convened a Career Satisfaction Task Force that designed and executed a national survey of hospitalists in 2009‐2010. The objective of this study is to evaluate how job characteristics vary by practice model, and the association of these characteristics and practice models with job satisfaction and burnout.
METHODS
Survey Instrument
A detailed description of the survey design, sampling strategy, data collection, and response rate calculations is described elsewhere.16 Portions of the 118‐item survey instrument assessed characteristics of the respondents' hospitalist group (12 items), details about their individual work patterns (12 items), and demographics (9 items). Work patterns were evaluated by the average number of clinical work days, consecutive days, hours per month, percentage of work assigned to night duty, and number of patient encounters. Average hours spent on nonclinical work, and the percentage of time allocated for clinical, administrative, teaching, and research activities were solicited. Additional items assessed specific clinical responsibilities, pretax earnings in FY2010, the availability of information technology capabilities, and the adequacy of available resources. Job and specialty satisfaction and 11 satisfaction domain measures were measured using validated scales.1726 Burnout symptoms were measured using a validated single‐item measure.26, 27
Sampling Strategy
We surveyed a national stratified sample of hospitalists in the US and Puerto Rico. We used the largest database of hospitalists (>24,000 names) currently available and maintained by the SHM as our sampling frame. We linked hospitalist employer information to hospital statistics from the American Hospital Association database28 to stratify the sample by number of hospital beds, geographic region, employment model, and specialty training, oversampling pediatric hospitalists due to small numbers. A respondent sample of about 700 hospitalists was calculated to be adequate to detect a 0.5 point difference in job satisfaction scores between subgroups assuming 90% power and alpha of 0.05. However, we sampled a total of 5389 addresses from the database to overcome the traditionally low physician response rates, duplicate sampling, bad addresses, and non‐hospitalists being included in the sampling frame. In addition, 2 multistate hospitalist companies (EmCare, In Compass Health) and 1 for‐profit hospital chain (HCA, Inc) financially sponsored this project with the stipulation that all of their hospitalist employees (n = 884) would be surveyed.
Data Collection
The healthcare consulting firm, Press Ganey, provided support with survey layout and administration following the modified Dillman method.29 Three rounds of coded surveys and solicitation letters from the investigators were mailed 2 weeks apart in November and December 2009. Because of low response rates to the mailed survey, an online survey was created using Survey Monkey and sent to 650 surveyees for whom e‐mail addresses were available, and administered at a kiosk for sample physicians during the SHM 2010 annual meeting.
Data Analysis
Nonresponse bias was measured by comparing characteristics between respondents of separate survey waves.30 We determined the validity of mailing addresses immediately following the survey period by mapping each address using Google, and if the address was a hospital, researching online whether or not the intended recipient was currently employed there. Practice characteristics were compared across 5 model categories distilled from the SHM & Medical Group Management Association survey: local hospitalist‐only group, multistate hospitalist group, multispecialty physician group, employer hospital, and university or medical school. Weighted proportions, means, and medians were calculated to account for oversampling of pediatric hospitalists. Differences in categorical measures were assessed using the chi‐square test and the design‐based F test for comparing weighted data. Weighted means (99% confidence intervals) and medians (interquartile ranges) were calculated. Because each parameter yielded a single outlier value across the 5 practice models, differences across weighted means were assessed using generalized linear models with the single outlier value chosen as the reference mean. Pair‐wise Wilcoxon rank sum test was used to compare median values. In these 4‐way comparisons of means and medians, significance was defined as P value of 0.0125 per Bonferroni correction. A single survey item solicited respondents to choose exactly 4 of 13 considerations most pertinent to job satisfaction. The proportion of respondents who scored 4 on a 5‐point Likert scale of the 11 satisfaction domains and 2 global measures of satisfaction, and burnout symptoms defined as 3 on a 5‐point single item measure were bar‐graphed. Chi‐square statistics were used to evaluate for differences across practice models. Statistical significance was defined by alpha less than 0.05, unless otherwise specified. All analyses were performed using STATA version 11.0 (College Station, TX). This study was approved by the Loyola University Institutional Review Board.
Survey data required cleaning prior to analysis. Missing gender information was imputed using the respondents' name. Responses to the item that asked to indicate the proportion of work dedicated to administrative responsibilities, clinical care, teaching, and research that did not add up to 100% were dropped. Two responses that indicated full‐time equivalent (FTE) of 0%, but whose respondents otherwise completed the survey implying they worked as clinical hospitalists, were replaced with values calculated from the given number of work hours relative to the median work hours in our sample. Out of range or implausible responses to the following items were dropped from analyses: the average number of billable encounters during a typical day or shift, number of shifts performing clinical activities during a typical month, pretax earnings, the year the respondent completed residency training, and the number of whole years practiced as a hospitalist. The proportion of selective item nonresponse was small and we did not, otherwise, impute missing data.
RESULTS
Response Rate
Of the 5389 originally sampled addresses, 1868 were undeliverable. Addresses were further excluded if they appeared in duplicate or were outdated. This yielded a total of 3105 eligible surveyees in the sample. As illustrated in Figure 1, 841 responded to the mailed survey and 5 responded to the Web‐based survey. After rejecting 67 non‐hospitalist respondents and 3 duplicate surveys, a total of 776 surveys were included in the final analysis. The adjusted response rate was 25.6% (776/3035). Members of SHM were more likely to return the survey than nonmembers. The adjusted response rate from hospitalists affiliated with the 3 sponsoring institutions was 6% (40/662). Because these respondents were more likely to be non‐members of SHM, we opted to analyze the responses from the sponsor hospitalists together with the sampled hospitalists. The demographics of the resulting pool of 816 respondents affiliated with over 650 unique hospitalist groups were representative of the original survey frame. We analyzed data from 794 of these who responded to the item indicating their hospitalist practice model. Demographic characteristics of responders and nonresponders to the practice model survey item were similar.
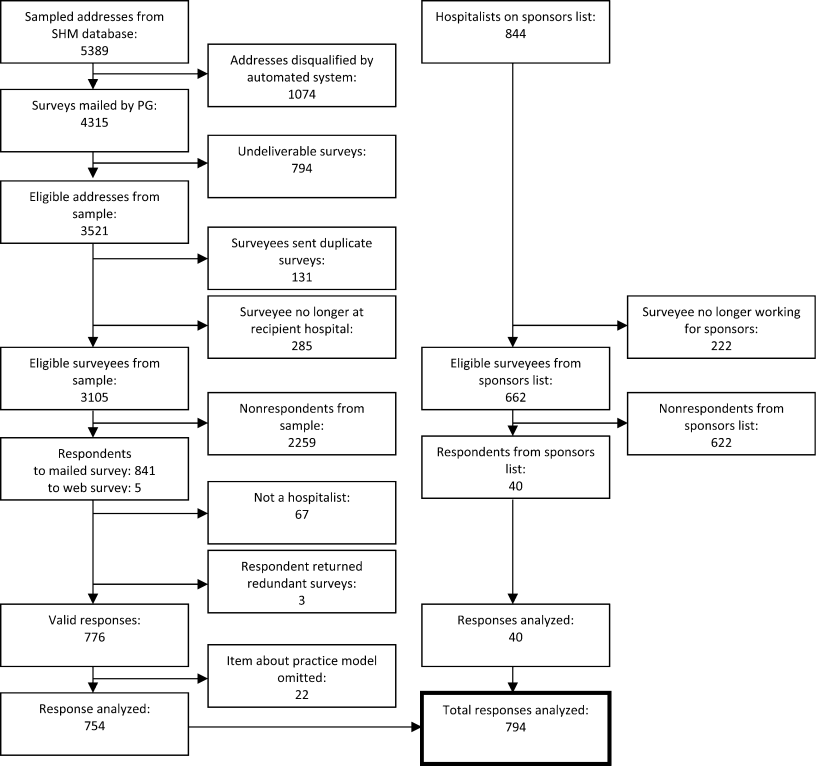
Characteristics of Hospitalists and Their Groups
Table 1 summarizes the characteristics of hospitalist respondents and their organizations by practice model. More (44%) respondents identified their practice model as directly employed by the hospital than other models, including multispecialty physician group (15%), multistate hospitalist group (14%), university or medical school (14%), local hospitalist group (12%), and other (2%). The median age of hospitalist respondents was 42 years, with 6.8 years of mean experience as a hospitalist. One third were women, 84% were married, and 46% had dependent children 6 years old or younger at home. Notably, hospitalists in multistate groups had fewer years of experience, and fewer hospitalists in local and multistate groups were married compared to hospitalists in other practice models.
Local Hospitalist‐Only Group | Multi‐State Hospitalist Group | Multispecialty Physician Group | Employer Hospital | University or Medical School | ||
---|---|---|---|---|---|---|
n = 95 | n = 111 | n = 115 | n = 348 | n = 107 | P Value | |
| ||||||
Hospitalist characteristics | ||||||
Age, weighted mean (99% CI) | 45 (42, 48) | 44 (42, 47) | 45 (43, 47) | 45 (43, 46) | 43 (40, 46) | |
Years hospitalist experience, weighted mean (99% CI) | 8 (6, 9)* | 5 (4, 6)* | 8 (7, 9) | 7 (6, 7) | 8 (6, 9) | <0.010* |
Women, weighted % | 29 | 30 | 39 | 31 | 43 | 0.118 |
Married, weighted % | 76 | 77 | 82 | 89 | 81 | 0.009 |
At least 1 dependent child younger than age 6 living in home, weighted % | 47 | 48 | 43 | 47 | 45 | 0.905 |
Pediatric specialty, n (%) | <10 | <10 | 11 (10%) | 57 (16%) | 36 (34%) | <0.001 |
Hospitalist group characteristics | ||||||
Region, weighted % | <0.001 | |||||
Northeast (AHA 1 & 2) | 13 | 10 | 16 | 27 | 13 | |
South (AHA 3 & 4) | 19 | 37 | 13 | 24 | 21 | |
Midwest (AHA 5 & 6) | 23 | 24 | 25 | 22 | 26 | |
Mountain (AHA 7 & 8) | 22 | 20 | 16 | 13 | 24 | |
West (AHA 9) | 24 | 10 | 31 | 14 | 16 | |
No. beds of primary hospital, weighted % | <0.001 | |||||
Up to 149 | 17 | 26 | 12 | 24 | 14 | |
150299 | 30 | 36 | 36 | 33 | 21 | |
300449 | 26 | 24 | 29 | 20 | 19 | |
450599 | 13 | 8 | 17 | 11 | 21 | |
600 or more | 12 | 6 | 7 | 13 | 24 | |
No. of hospital facilities served by current practice, weighted % | <0.001 | |||||
1 | 53 | 70 | 67 | 77 | 66 | |
2 | 20 | 22 | 20 | 16 | 24 | |
3 or more | 27 | 9 | 13 | 7 | 10 | |
No. of physicians in current practice, median (IQR) | 10 (5, 18) | 8 (6, 12)* | 14 (8, 25)* | 12 (6, 18) | 12 (7, 20) | <0.001*, 0.001 |
No. of non‐physician providers in current practice, median (IQR) | 0 (0, 2) | 0 (0, 2) | 0 (0, 3) | 1 (0, 2) | 0 (0, 2) | |
Available information technology capabilities, weighted % | ||||||
EHR to access physician notes | 57 | 57 | 75 | 58 | 79 | <0.001 |
EHR to access nursing documentations | 68 | 67 | 74 | 75 | 76 | 0.357 |
EHR to access laboratory or test results | 97 | 89 | 95 | 96 | 96 | 0.054 |
Electronic order entry | 30 | 19 | 53 | 38 | 56 | <0.001 |
Electronic billing | 38 | 31 | 36 | 36 | 38 | 0.818 |
Access to EHR at home or off site | 78 | 73 | 78 | 82 | 84 | 0.235 |
Access to Up‐to‐Date or other clinical guideline resources | 80 | 77 | 91 | 92 | 96 | <0.001 |
Access to schedules, calendars, or other organizational resources | 56 | 57 | 66 | 67 | 75 | 0.024 |
E‐mail, Web‐based paging, or other communication resources | 74 | 63 | 88 | 89 | 90 | <0.001 |
Several differences in respondent group characteristics by practice model were found. Respondents in multistate hospitalist groups were more likely from the South and Midwest, while respondents from multispecialty groups were likely from the West. More multistate group practices were based in smaller hospitals, while academic hospitalists tended to practice in hospitals with 600 or more beds. Respondents employed by hospitals were more likely to practice at 1 hospital facility only, while local group practices were more likely to practice at 3 or more facilities. The median number of physicians in a hospitalist group was 11 (interquartile range [IQR] 6, 19). Local and multistate groups had fewer hospitalists compared to other models. Nonphysician providers were employed by nearly half of all hospitalist practices. Although almost all groups had access to some information technology, more academic hospitalists had access to electronic order entry, electronic physician notes, electronic clinical guidelines resources and communication technology, while local and multistate groups were least likely to have access to these resources.
Work Pattern Variations
Table 2 further details hospitalist work hours by practice model. The majority of hospitalists (78%) reported their position was full‐time (FTE 1.0), while 13% reported working less than full‐time (FTE <1.0). Only 5% of local group hospitalists worked part‐time, while 20% of multispecialty group hospitalists did. An additional 9% reported FTE >1.0, indicating their work hours exceeded the definition of a full‐time physician in their practice. Among full‐time hospitalists, local group members worked a greater number of shifts per month than employees of multispecialty groups, hospitals, and academic medical centers. Academic hospitalists reported higher numbers of consecutive clinical days worked on average, but fewer night shifts compared to hospitalists employed by multistate groups, multispecialty groups, and hospitals; fewer billable encounters than hospitalists in local and multistate groups; and more nonclinical work hours than hospitalists of any other practice model. Academic hospitalists also spent more time on teaching and research than other practice models. Hospitalists spent 11%‐18% of their time on administrative and committee responsibilities, with the least amount spent by hospitalists in multistate groups and the most in academic practice.
Local Hospitalist‐Only Group | Multi‐State Hospitalist Group | Multispecialty Physician Group | Employer Hospital | University or Medical School | ||
---|---|---|---|---|---|---|
n = 95 | n = 111 | n = 115 | n = 348 | n = 107 | P Value | |
| ||||||
FTE, weighted % | 0.058 | |||||
FTE < 1.0 | 6 | 13 | 20 | 12 | 14 | |
FTE = 1.0 | 85 | 75 | 74 | 80 | 82 | |
FTE > 1.0 | 10 | 13 | 6 | 8 | 5 | |
Workload parameters, weighted mean (99% CI) | ||||||
Clinical shifts per month for FTE 1.0 | 19 (17, 20)* | 17 (16, 19) | 15 (14, 17)* | 16 (15, 16) | 15 (13, 17) | <0.001* |
Hours per clinical shift | 10 (9, 11) | 11 (10, 11)* | 10 (10, 11.0) | 11 (10, 11.0) | 10 (9, 10)* | 0.006*, 0.002 |
Consecutive days on clinical shift | 8 (6, 9) | 7 (6, 7)* | 6 (6, 7) | 7 (6, 7) | 9 (7, 10)* | 0.002*, <0.001 |
% Clinical shifts on nights | 20 (15, 25) | 23 (18, 28)* | 23 (17, 29) | 21 (17, 24) | 14 (9, 18)* | 0.001*, 0.002 |
% Night shifts spent in hospital | 61 (49, 74)* | 63 (52, 75) | 72 (62, 83) | 73 (67, 80) | 43 (29, 57)* | 0.010*, 0.003, <0.001 |
Billable encounters per clinical shift | 17 (14, 19)* | 17 (16, 18) | 14 (13, 15) | 15 (14, 16) | 13 (11, 14)* | <0.001*, 0.002 |
Hours nonclinical work per month | 23 (12, 34)* | 19 (11, 27) | 31 (20, 42) | 30 (24, 36) | 71 (55, 86)* | <0.001* |
Hours clinical and nonclinical work per month for FTE 1.0 | 202 (186, 219) | 211 (196, 226) | 184 (170, 198)* | 193 (186, 201) | 221 (203, 238)* | <0.001* |
Professional activity, weighted mean % (99% CI) | ||||||
Clinical | 84 (78, 89)* | 86 (81, 90) | 78 (72, 84) | 79 (76, 82) | 58 (51, 64)* | <0.001* |
Teaching | 2.3 (1, 5)* | 3 (1, 4) | 6 (4, 9) | 6 (5, 8) | 17 (14, 20)* | <0.001* |
Administration and Committee work | 13 (8, 19) | 11 (8, 15)* | 16 (10, 21) | 14 (12, 17) | 19 (14, 24)* | 0.001* |
Research | 0 (0, 0)* | 1 (0, 2) | 0 (0, 1) | 1 (0, 1) | 7 (3, 11)* | <0.001* |
Table 3 tabulates other work pattern characteristics. Most hospitalists indicated that their current clinical work as hospitalists involved the general medical wards (100%), medical consultations (98%), and comanagement with specialists (92%). There were wide differences in participation in comanagement (100%, local groups vs 71%, academic), intensive care unit (ICU) responsibilities (94%, multistate groups vs 27%, academic), and nursing home care (30%, local groups vs 8%, academic). Among activities that are potentially not reimbursable, academic hospitalists were less likely to participate in coordination of patient transfers and code or rapid response teams, while multistate groups were least likely to participate in quality improvement activities. In total, 99% of hospitalists reported participating in at least 1 potentially nonreimbursable clinical activity.
Local Hospitalist‐Only Group | Multi‐State Hospitalist Group | Multispecialty Physician Group | Employer Hospital | University or Medical School | ||
---|---|---|---|---|---|---|
n = 95 | n = 111 | n = 115 | n = 348 | n = 107 | P Value | |
| ||||||
Reimbursable activities, overlapping weighted % | ||||||
General medical ward | 100 | 99 | 100 | 99 | 99 | 0.809 |
Medical consultations | 99 | 99 | 100 | 98 | 95 | 0.043 |
Comanagement with specialists | 100 | 96 | 96 | 93 | 71 | <0.001 |
Preoperative evaluations | 92 | 92 | 90 | 88 | 77 | 0.002 |
Intensive care unit | 86 | 94 | 67 | 75 | 27 | <0.001 |
Skilled nursing facility or long‐term acute care facility | 30 | 19 | 12 | 16 | 8 | <0.001 |
Outpatient general medical practice | 4 | 4 | 5 | 5 | 10 | 0.241 |
Potentially nonreimbursable activities, overlapping weighted % | ||||||
Coordination of patient transfers | 92 | 94 | 95 | 93 | 82 | 0.005 |
Quality improvement or patient safety initiatives | 81 | 78 | 83 | 89 | 89 | 0.029 |
Code team or rapid response team | 56 | 57 | 53 | 62 | 37 | <0.001 |
Information technology design or implementation | 42 | 39 | 47 | 51 | 51 | 0.154 |
Admission triage for emergency department | 49 | 46 | 43 | 40 | 31 | 0.132 |
Compensation scheme, weighted % | <0.001 | |||||
Salary only | 18 | 21 | 30 | 29 | 47 | |
Salary plus performance incentive | 54 | 72 | 59 | 67 | 53 | |
Fee‐for‐service | 20 | 1 | 7 | 2 | 0 | |
Capitation | 0 | 0 | 0 | 0 | 0 | |
Other | 9 | 7 | 4 | 3 | 0 | |
Compensation links to incentives, overlapping weighted % | ||||||
No incentives | 40 | 28 | 29 | 29 | 48 | 0.003 |
Patient satisfaction | 23 | 39 | 38 | 38 | 14 | <0.001 |
Length of stay | 18 | 17 | 20 | 13 | 10 | 0.208 |
Overall cost | 8 | 11 | 9 | 5 | 6 | 0.270 |
Test utilization | 2 | 2 | 7 | 1 | 0 | <0.001 |
Clinical processes and outcomes | 26 | 34 | 44 | 43 | 24 | <0.001 |
Other | 17 | 29 | 26 | 31 | 25 | 0.087 |
Earnings, weighted mean dollars (99% CI) | 226,065 (202,891, 249,240)* | 225,613 (210,772, 240,454) | 202,617 (186,036, 219,198) | 206,087 (198,413, 213,460) | 166,478 (151,135, 181,821)* | <0.001* |
Hospitalist compensation schemes were significantly different across the practice models. Salary‐only schemes were most common among academic hospitalists (47%), while 72% of multistate groups used performance incentives in addition to salary. More local groups used fee‐for‐service compensation than other models. Incentives differed by practice model, with more multistate groups having incentives based on patient satisfaction, while more multispecialty physician groups had incentives based on clinical processes and outcomes than other models. Finally, mean earnings for academic hospitalists were significantly lower than for hospitalists of other practice models. Local and multistate group hospitalists earned more than any other practice model (all P <0.001), and $60,000 more than the lowest compensated academic hospitalists.
Components of Job Satisfaction
Hospitalists' rankings of the most important factors for job satisfaction revealed differences across models (Figure 2). Overall, hospitalists were most likely to consider optimal workload and compensation as important factors for job satisfaction from a list of 13 considerations. Local groups and academics were least likely to rank optimal workload as a top factor, and local group hospitalists were more likely to rank optimal autonomy than those of other models. Academic hospitalists had less concern for substantial pay, and more concern for the variety of tasks they perform and recognition by leaders, than other hospitalists.
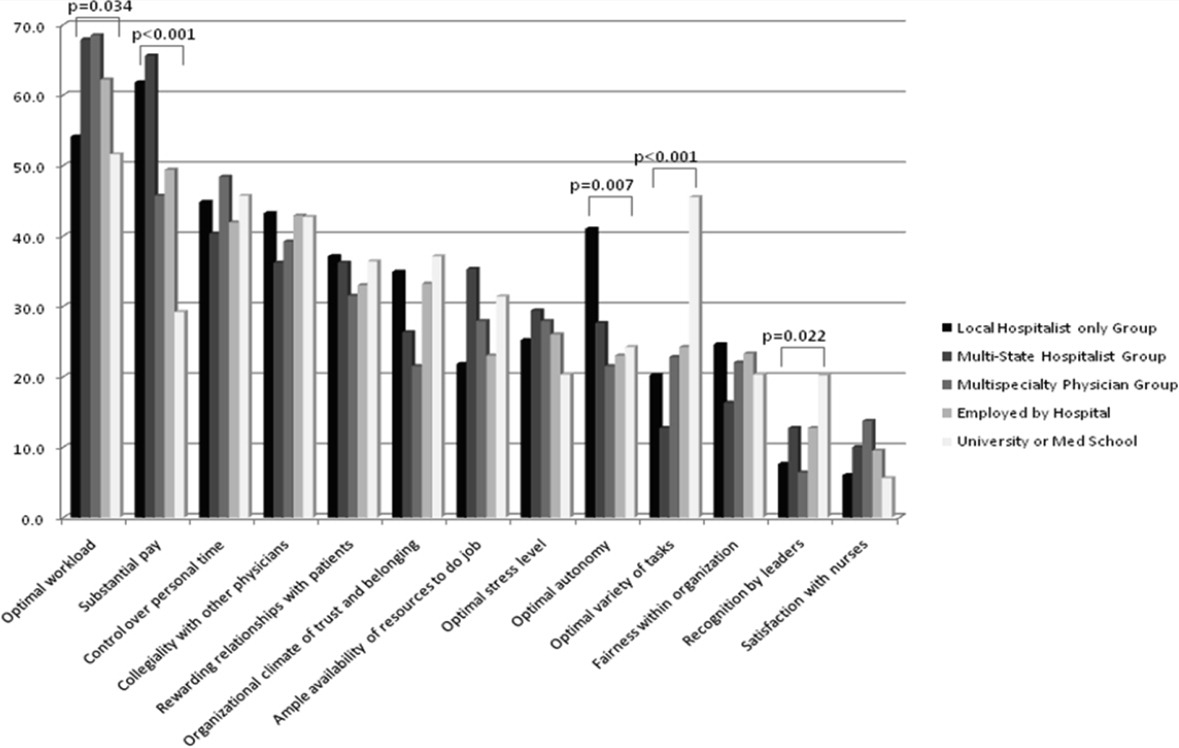
Job Satisfaction and Burnout Risk
Differences in the ratings of 4 of the 11 satisfaction and job characteristic domains were found across the practice models (Figure 3). Multispecialty group hospitalists were less satisfied with autonomy and their relationship with patients than other practice models, and along with multistate groups, reported the highest perceived workload. Organizational fairness was rated much higher by local group hospitalists than other practice models. Despite these differences in work patterns and satisfaction, there were no differences found in level of global job satisfaction, specialty satisfaction, or burnout across the practice models. Overall, 62% of respondents reported high job satisfaction (4 on a 1 to 5 scale), and 30% indicated burnout symptoms.
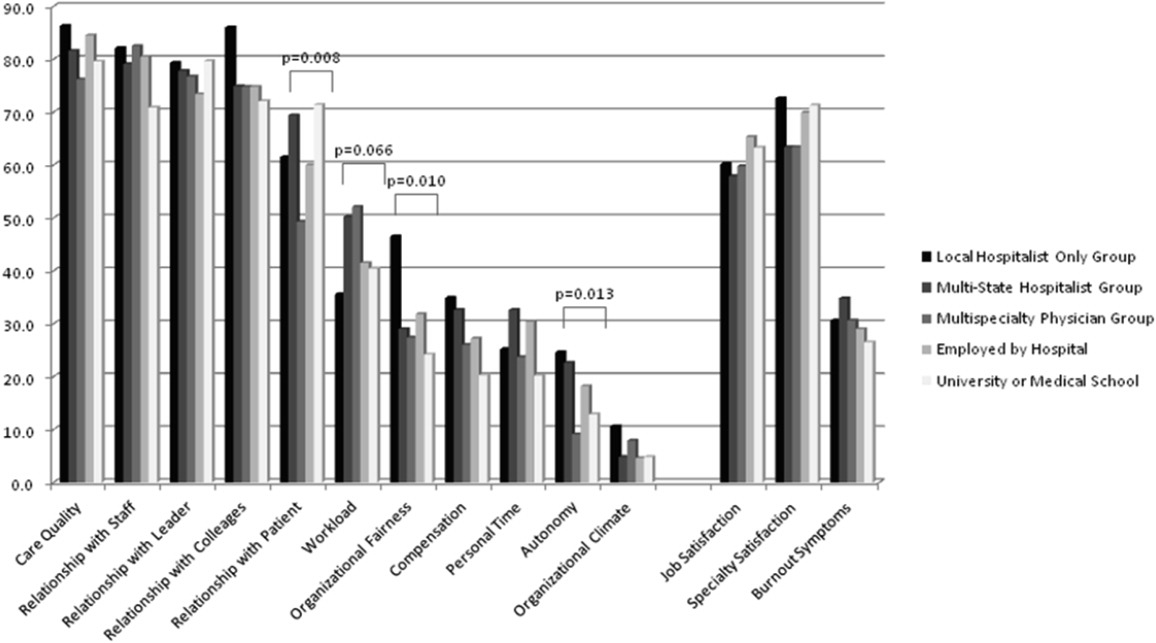
DISCUSSION
In our sample of US hospitalists, we found major differences in work patterns and compensation across hospitalist practice models, but no differences in job satisfaction, specialty satisfaction, and burnout. In particular, differences across these models included variations in hospitalist workload, hours, pay, and distribution of work activities. We found that hospitalists perform a variety of clinical and nonclinical tasks, for many of which there are not standard reimbursement mechanisms. We also found that features of a job that individual hospitalists considered most important vary by practice model.
Previous analysis of this data explored the overall state of hospitalist satisfaction.16 The present analysis offers a glimpse into hospitalists' systems‐orientation through a deeper look at their work patterns. The growth in the number of hospitalists who participate in intensive care medicine, specialty comanagement, and other work that involves close working relationships with specialist physicians confirms collaborative care as one of the dominant drivers of the hospitalist movement. At the level of indirect patient care, nearly all hospitalists contributed to work that facilitates coordination, quality, patient safety, or information technology. Understanding the integrative value of hospitalists outside of their clinical productivity may be of interest to hospital administrators.
Global satisfaction measures were similar across practice models. This finding is particularly interesting given the major differences in job characteristics seen among the practice models. This similarity in global satisfaction despite real differences in the nature of the job suggests that individuals find settings that allow them to address their individual professional goals. Our study demonstrates that, in 2010, Hospital Medicine has evolved enough to accommodate a wide variety of goals and needs.
While global satisfaction did not differ among practice types, hospitalists from various models did report differences in factors considered important to global satisfaction. While workload and pay were rated as influential across most models, the degree of importance was significantly different. In academic settings, substantial pay was not a top consideration for overall job satisfaction, whereas in local and multistate hospitalist groups, pay was a very close second in importance to optimal workload. These results may prove helpful for individual hospitalists trying to find their optimal job. For example, someone who is less concerned about workload, but wants to be paid well and have a high degree of autonomy, may find satisfaction in local hospitalist groups. However, for someone who is willing to sacrifice a higher salary for variety of activities, academic Hospital Medicine may be a better fit.
There is a concerning aspect of hospitalist job satisfaction that different practice models do not seem to solve. Control over personal time is a top consideration for many hospitalists across practice models, yet their satisfaction with personal time is low. As control over personal time is seen as a draw to the Hospital Medicine specialty, group leaders may need to evaluate their programs to ensure that schedules and workload support efforts for hospitalists to balance work and homelife commitments.
There are additional findings that are important for Hospital Medicine group leaders. Regardless of practice model, compensation and workload are often used as tools to recruit and retain hospitalists. While these tools may be effective, leaders may find more nuanced approaches to improving their hospitalists' overall satisfaction. Leaders of local hospitalist groups may find their hospitalists tolerant of heavier workloads as long as they are adequately rewarded and are given real autonomy over their work. However, leaders of academic programs may be missing the primary factor that can improve their hospitalists' satisfaction. Rather than asking for higher salaries to remain competitive, it may be more effective to advocate for time and training for their hospitalists to pursue important other activities beyond direct clinical care. Given that resources will always be limited, group leaders need to understand all of the elements that can contribute to hospitalist job satisfaction.
We point out several limitations to this study. First, our adjusted response rate of 25.6% is low for survey research, in general. As mentioned above, hospitalists are not easily identified in any available national physician database. Therefore, we deliberately designed our sampling strategy to error on the side of including ineligible surveyees to reduce systematic exclusion of practicing hospitalists. Using simple post hoc methods, we identified many nonhospitalists and bad addresses from our sample, but because these methods were exclusionary as opposed to confirmatory, we believe that a significant proportion of remaining nonrespondents may also have been ineligible for the survey. Although this does not fully address concerns about potential response bias, we believe that our sample representing a large number of hospitalist groups is adequate to make estimations about a nationally representative sample of practicing hospitalists. Second, in spite of our inclusive approach, we may still have excluded categories of practicing hospitalists. We were careful not to allow SHM members to represent all US hospitalists and included non‐members in the sampling frame, but the possibility of systematic exclusion that may alter our results remains a concern. Additionally, one of our goals was to characterize pediatric hospitalists independently from their adult‐patient counterparts. Despite oversampling of pediatricians, their sample was too small for a more detailed comparison across practice models. Also, self‐reported data about workload and compensation are subject to inaccuracies related to recall and cognitive biases. Last, this is a cross‐sectional study of hospitalist satisfaction at one point in time. Consequently, our sample may not be representative of very dissatisfied hospitalists who have already left their jobs.
The diversity found across existing practice models and the characteristics of the practices provide physicians with the opportunity to bring their unique skills and motivations to the hospitalist movement. As hospitals and other organizations seek to create, maintain, or grow hospitalist programs, the data provided here may prove useful to understand the relationship between practice characteristics and individual job satisfaction. Additionally, hospitalists looking for a job can consider these results as additional information to guide their choice of practice model and work patterns.
Acknowledgements
The authors thank Kenneth A. Rasinski for assistance with survey items refinement, and members of the SHM Career Satisfaction Task Force for their assistance in survey development.
- The status of hospital medicine groups in the United States.J Hosp Med.2006;1(2):75–80. , , , .
- Growth in the care of older patients by hospitalists in the United States.N Engl J Med.2009;360(11):1102–1112. , , , .
- The state of hospital medicine in 2008.Med Clin North Am.2008;92(2):265–273,vii. .
- Health care market trends and the evolution of hospitalist use and roles.J Gen Intern Med.2005;20(2):101–107. , , , .
- Effects of physician experience on costs and outcomes on an academic general medicine service: results of a trial of hospitalists.Ann Intern Med.2002;137(11):866–874. , , , et al.
- The Park Nicollet experience in establishing a hospitalist system.Ann Intern Med.1999;130(4 pt 2):350–354. .
- Effects of an HMO hospitalist program on inpatient utilization.Am J Manag Care.2001;7(11):1051–1057. , .
- The impact of hospitalists on the cost and quality of inpatient care in the United States: a research synthesis.Med Care Res Rev.2005;62(4):379–406. , .
- Pediatric hospitalists: a systematic review of the literature.Pediatrics.2006;117(5):1736–1744. , , , .
- The hospitalist movement 5 years later.JAMA.2002;287(4):487–494. , .
- Trends in market demand for internal medicine 1999 to 2004: an analysis of physician job advertisements.J Gen Intern Med.2006;21(10):1079–1085. , , , , , .
- SHM. 2003–2004 Survey by the Society of Hospital Medicine on Productivity and Compensation: Analysis of Results. 2004 [updated 2004]. Available at: http://www.hospitalmedicine.org/AM/Template. cfm?Section=Practice_Resources Available at: http://cme.medscape.com/viewarticle/578134. Accessed October 21,2010.
- State of Hospital Medicine: 2010 Report Based on 2009 Data.Englewood, CO and Philadelphia, PA:Medical Group Management Association and Society of Hospital Medicine;2010.
- Worklife and satisfaction of hospitalists: toward flourishing careers.J Gen Intern Med.2011, Jul 20. PMID: 21773849. , , , , .
- Worklife and satisfaction of general internists.Arch Intern Med.2002;162(6):649–656. , , , et al.
- Organizational climate, stress, and error in primary care: the MEMO study. In: Henriksen K, Battles JB, Marks ES, Lewin DI, eds.Advances in Patient Safety: From Research to Implementation. Vol 1: Research Findings.Rockville, MD:Agency for Healthcare Research and Quality;2005;1:65–77. , , , et al.
- Hospitalists and the practice of inpatient medicine: results of a survey of the National Association of Inpatient Physicians.Ann Intern Med.1999;130(4 pt 2):343–349. , , , .
- Physician attitudes toward and prevalence of the hospitalist model of care: results of a national survey.Am J Med.2000;109(8):648–653. , , , , , .
- Taking the Measure of Work: A Guide to Validated Scales for Organizational Research and Diagnosis.Thousand Oaks, CA:Sage Publications;2002. .
- Job Demands and Worker Health.Ann Arbor, MI:University of Michigan, Institute for Social Research;1980. , , , , .
- On the dimensionality of organizational justice: a construct validation of a measure.J Appl Psychol.2001;86(3):386–400. .
- Effect of job demands and social support on worker stress—a study of VDT users.Behav Inform Technol.1995;14(1):32–40. , .
- Measuring physician job satisfaction in a changing workplace and a challenging environment. SGIM Career Satisfaction Study Group. Society of General Internal Medicine.Med Care.1999;37(11):1174–1182. , , , et al.
- Working conditions in primary care: physician reactions and care quality.Ann Intern Med.2009;151(1):28–U48. , , , et al.
- Validation of a single‐item measure of burnout against the Maslach Burnout Inventory among physicians.Stress Health.2004;20(2):75–79. , , .
- American Hospital Association. AHA Hospital Statistics. 2009 [updated 2009]. Available at: http://www.ahadata.com/ahadata/html/AHAStatistics.html. Accessed April 12,2011.
- How to obtain excellent response rates when surveying physicians.Fam Pract.2009;26(1):65–68. , , , et al.
- Estimating nonresponse bias in mail surveys.J Marketing Res.1977;14(3):396–402. , .
- The status of hospital medicine groups in the United States.J Hosp Med.2006;1(2):75–80. , , , .
- Growth in the care of older patients by hospitalists in the United States.N Engl J Med.2009;360(11):1102–1112. , , , .
- The state of hospital medicine in 2008.Med Clin North Am.2008;92(2):265–273,vii. .
- Health care market trends and the evolution of hospitalist use and roles.J Gen Intern Med.2005;20(2):101–107. , , , .
- Effects of physician experience on costs and outcomes on an academic general medicine service: results of a trial of hospitalists.Ann Intern Med.2002;137(11):866–874. , , , et al.
- The Park Nicollet experience in establishing a hospitalist system.Ann Intern Med.1999;130(4 pt 2):350–354. .
- Effects of an HMO hospitalist program on inpatient utilization.Am J Manag Care.2001;7(11):1051–1057. , .
- The impact of hospitalists on the cost and quality of inpatient care in the United States: a research synthesis.Med Care Res Rev.2005;62(4):379–406. , .
- Pediatric hospitalists: a systematic review of the literature.Pediatrics.2006;117(5):1736–1744. , , , .
- The hospitalist movement 5 years later.JAMA.2002;287(4):487–494. , .
- Trends in market demand for internal medicine 1999 to 2004: an analysis of physician job advertisements.J Gen Intern Med.2006;21(10):1079–1085. , , , , , .
- SHM. 2003–2004 Survey by the Society of Hospital Medicine on Productivity and Compensation: Analysis of Results. 2004 [updated 2004]. Available at: http://www.hospitalmedicine.org/AM/Template. cfm?Section=Practice_Resources Available at: http://cme.medscape.com/viewarticle/578134. Accessed October 21,2010.
- State of Hospital Medicine: 2010 Report Based on 2009 Data.Englewood, CO and Philadelphia, PA:Medical Group Management Association and Society of Hospital Medicine;2010.
- Worklife and satisfaction of hospitalists: toward flourishing careers.J Gen Intern Med.2011, Jul 20. PMID: 21773849. , , , , .
- Worklife and satisfaction of general internists.Arch Intern Med.2002;162(6):649–656. , , , et al.
- Organizational climate, stress, and error in primary care: the MEMO study. In: Henriksen K, Battles JB, Marks ES, Lewin DI, eds.Advances in Patient Safety: From Research to Implementation. Vol 1: Research Findings.Rockville, MD:Agency for Healthcare Research and Quality;2005;1:65–77. , , , et al.
- Hospitalists and the practice of inpatient medicine: results of a survey of the National Association of Inpatient Physicians.Ann Intern Med.1999;130(4 pt 2):343–349. , , , .
- Physician attitudes toward and prevalence of the hospitalist model of care: results of a national survey.Am J Med.2000;109(8):648–653. , , , , , .
- Taking the Measure of Work: A Guide to Validated Scales for Organizational Research and Diagnosis.Thousand Oaks, CA:Sage Publications;2002. .
- Job Demands and Worker Health.Ann Arbor, MI:University of Michigan, Institute for Social Research;1980. , , , , .
- On the dimensionality of organizational justice: a construct validation of a measure.J Appl Psychol.2001;86(3):386–400. .
- Effect of job demands and social support on worker stress—a study of VDT users.Behav Inform Technol.1995;14(1):32–40. , .
- Measuring physician job satisfaction in a changing workplace and a challenging environment. SGIM Career Satisfaction Study Group. Society of General Internal Medicine.Med Care.1999;37(11):1174–1182. , , , et al.
- Working conditions in primary care: physician reactions and care quality.Ann Intern Med.2009;151(1):28–U48. , , , et al.
- Validation of a single‐item measure of burnout against the Maslach Burnout Inventory among physicians.Stress Health.2004;20(2):75–79. , , .
- American Hospital Association. AHA Hospital Statistics. 2009 [updated 2009]. Available at: http://www.ahadata.com/ahadata/html/AHAStatistics.html. Accessed April 12,2011.
- How to obtain excellent response rates when surveying physicians.Fam Pract.2009;26(1):65–68. , , , et al.
- Estimating nonresponse bias in mail surveys.J Marketing Res.1977;14(3):396–402. , .
Copyright © 2012 Society of Hospital Medicine
Hospitalist Effects on Acute IGIH Patients
Acute upper gastrointestinal hemorrhage (UGIH) is one of the most common hospital admissions for acute care. Estimates indicate that 300,000 patients (100‐150 cases per 100,000 adults) are admitted annually with an associated economic impact of $2.5 billion.15 The current standard management of UGIH requires hospital admission and esophagogastroduodenoscopy (EGD) by a gastroenterologist for diagnosis and/or treatment. This management strategy results in a high consumption of hospital resources and costs.
Simultaneously, hospitalists have dramatically changed the delivery of inpatient care in the United States and are recognized as a location‐driven subspecialty for the care of acute hospitalized patients, similar to emergency medicine. Currently there are 20,000 hospitalists, and more than one‐third of general medicine inpatients are cared for by hospitalists.6, 7
Previous studies have shown that hospitalist care offers better or comparable outcomes, with lower overall length of stay (LOS) and costs compared to traditional providers.810 However, most of these studies were performed in single institutions, had weak designs or little‐to‐no adjustment for severity of illness, or were limited to 7 specific diseases (pneumonia, congestive heart failure [CHF], chest pain, ischemic stroke, urinary tract infection, chronic obstructive lung disease [COPD], and acute myocardial infarction [AMI]).8
Furthermore, less is known about the effect of hospitalists on conditions that may be dependent upon specialist consultation for procedures and/or treatment plans. In this study, gastroenterologists performed diagnostic and/or therapeutic endoscopy work as consultants to the attending physicians in the management of acute inpatient UGIH.
To explore the effects of hospitalists on care of patients with acute UGIH, we examined data from the Multicenter Hospitalist (MCH) trial. The objectives of our study were to compare clinical outcomesin‐hospital mortality and complications (ie, recurrent bleeding, intensive care unit [ICU] transfer, decompensation, transfusion, reendoscopy, 30‐day readmission)and efficiency (LOS and costs) in hospitalized acute UGIH patients cared for by hospitalists and nonhospitalists in 6 academic centers in the United States during a 2‐year period.
Patients and Methods
Study Sites
From July 1, 2001 to June 30, 2003, the MCH trial1113 was a prospective, multicenter, observational trial of the care provided by hospitalists to patients admitted to general medical services at 6 academic medical institutions. There were 31,000 consecutive admissions to the general medical services of these participating sites: University of Chicago (Chicago, IL), University of Wisconsin Hospital (Madison, WI), University of Iowa (Iowa City, IA), University of California at San Francisco (San Francisco, CA), University of New Mexico (Albuquerque, NM), and Brigham and Women's Hospital (Boston, MA). The study was approved by the institutional review boards (IRBs) at each of the 6 participating institutions.
MCH Study Patients
Patients were eligible if they were admitted to the general medical services under the care of a hospitalist or nonhospitalist physician. Regardless of the admitting provider, each medical service was composed of rotating senior and junior resident physicians in all 6 sites. Furthermore, patients were 18 years of age or older, and were able to give consent themselves or had an appropriate proxy. Patients with mini‐mental status score of 17 (out of 22), admitted under their primary care physician or to an inpatient gastroenterology service, or transferred from another hospital, were excluded. The MCH study was designed to study the outcomes and efficiency in patients admitted for CHF, pneumonia, UGIH, and end‐of‐life care.
Acute UGIH Patients
Within the MCH‐eligible patients, we identified those with acute UGIH using the following International Classification of Diseases, 9th edition (ICD‐9) codes assigned at discharge: esophageal varices with hemorrhage (456.0, 456.20); Mallory‐Weiss syndrome (530.7); gastric ulcer with hemorrhage (531.00531.61); duodenal ulcer with hemorrhage (532.00532.61); peptic ulcer, site unspecified, with hemorrhage (533.00533.61); gastrojejunal ulcer with hemorrhage (534.00534.61); gastritis with hemorrhage (535.61); angiodysplasia of stomach/duodenum with hemorrhage (537.83); and hematemesis (578.0, 578.9). We also confirmed the diagnosis of UGIH by reviewing patient medical records for observed hematemesis, nasogastric tube aspirate with gross or hemoccult blood, or clinical history of hematemesis, melena, or hematochezia.14, 15
Data
All data were obtained from the 6 hospitals' administrative records, patient interviews, and medical chart abstractions. Dates of admission and discharge, ICD‐9 diagnosis codes, insurance type, age, race, and gender were obtained from administrative data. One‐month follow‐up telephone interviews assessed whether or not patient had any follow‐up appointment or hospital readmissions. Trained abstractors from each site performed manual chart reviews using a standard data collection sheet. The ICD‐9 code designation and chart abstraction methodology were developed prior to the initiation of the study to ensure consistent data collection and reduce bias.
The following data elements were collected: comorbidities, endoscopic findings, inpatient mortality, clinical evidence of rebleeding, endoscopic treatment or gastrointestinal (GI) surgery to control bleeding, repeat EGD, ICU transfer, decompensated comorbid illness requiring continued hospitalization, and blood transfusion (packed red cells, plasma, platelets). Clinical evidence of rebleeding was defined as either hematemesis or melena with decrease in hemoglobin of 2 g in 24 hours with or without hemodynamic compromise.14, 15 For the purpose of this study, recurrent bleeding was defined as clinical evidence of rebleeding, emergency GI surgery for control of UGIH, or repeat EGD before discharge. Furthermore, a composite endpoint termed total complications encompassed all adverse outcomes related to the UGIH hospitalization. The 30‐day readmission variable was defined using readmission identified in administrative records and a 30‐day follow‐up phone call. To guard against recall bias, self‐report data was only included for nonsite admissions.
We defined efficiency in terms of costs and LOS. Total hospital costs were measured using the TSI cost accounting system (Transition Systems, Inc., Boston, MA; now Eclipsys Corporation)16, 17 at 5 out of the 6 participating sites. TSI is a hospital cost accounting software system that integrates resource utilization and financial data already recorded in other hospital databases (such as the billing system, payroll system, and general ledger system).17 Hospital LOS was defined as the number of days from patient admission to the general medicine service until patient discharge.
Provider Specialization: Hospitalists vs. Nonhospitalists
The study was designed as a natural experiment based on a call cycle. The hospitalist‐led teams at each institution alternated in a 4‐day or 5‐day general medicine call cycle with teams led by traditional academic internal medicine attending physicians. All patients were assigned to teams according to their position in the call cycle without regard to whether the attending physician was a hospitalist or a nonhospitalist. Hospitalists are physicians whose primary professional focus is the general medical care of hospitalized patients.18, 19 As previously reported in a related MCH work,11 a hospitalist was also defined as a provider who spends at least 25% of his or her time on an academic inpatient general medicine service. Nonhospitalist physicians were most often outpatient general internal medicine faculty or subspecialists, who attended 1 month per year. Physicians were classified as hospitalists or nonhospitalists according to the designations provided by each site.
UGIH‐specific Confounders
From chart abstraction, we captured severity of illness, comorbidity, and performance of early EGD, variables that can confound analysis in UGIH. To capture severity of illness, a complete Rockall risk score was calculated for each patient. The complete Rockall uses 3 clinical variables (age, shock, and comorbidity) and 2 endoscopic variables (endoscopic diagnosis and stigmata of recent hemorrhage).5, 20 A complete Rockall score of 2 is considered low‐risk for rebleeding or death following admission.21, 22 The accepted definition of low‐risk is <5% recurrent bleeding and <1% mortality risk. A complete Rockall score of 3 to 5 is considered moderate‐risk while 6 is considered high‐risk. Comorbidity was measured using the Charlson comorbidity index.23 Performance of early endoscopy, usually defined as endoscopy performed within 24 hours from presentation, was previously shown to decrease LOS and need for surgical intervention in patients with acute UGIH.24, 25 Documented times of presentation to the emergency department and time of endoscopy performance were collected to calculate for the rate of early endoscopy in our study population.
Statistical Analysis
All statistical analyses were performed using SAS Version 9.1 for Windows (SAS Institute, Cary, NC).
Differences in baseline demographic characteristics of patients and their endoscopic findings were compared between the 2 types of providers. Univariate analyses were also performed to compare the differences in adverse outcomes, LOS, and costs between patients cared for by hospitalists and nonhospitalists. Chi‐square tests were used for categorical variables; while both Wilcoxon rank sum test and Student's t test were used in the analysis of continuous variables.
Next, we performed multivariable analyses to determine the independent association between hospitalist care and the odds of the patients having certain outcomes. However, to prevent overfitting, we only developed regression models for adverse outcomes that have at least 20% event rate.
Multivariable regression models were developed separately for LOS and costs. In contrast with the models on outcomes, analyses of LOS and costs were restricted to: (1) patients who were discharged alive; and (2) to cases with LOS and costs values within 3 standard deviations (SDs) of the mean because of the skewed nature of these data.
All models were adjusted for age, gender, race, insurance type, complete Rockall risk score, performance of early EGD, Charlson comorbidity index, and study site. Final candidate variables in the models were chosen based on stepwise selection, a method very similar to forward selection except that variables selected for the model do not necessarily remain in the model. Effects were entered into and then removed from the model in such a way that each forward selection step can be followed by 1 or more backward elimination steps. The stepwise selection was terminated if no further effect can be added to the model or if the current model was identical to the previous model. The stepwise selection model was generated using statistical criterion of alpha = 0.05 for entry and elimination from the model. Variables that can be a profound source of variation, such as study site and treating physician, were included in the model irrespective of their statistical significance.
To account for clustering of patients treated by the same physician, we used multilevel modeling with SAS PROC GLIMMIX (with random effects). For outcomes (categorical variables), we utilized models with logit‐link and binomial‐distributed errors. As for efficiency (continuous variables with skewed distribution), the multivariable analyses used a generalized linear model with log‐link and assuming gamma‐distributed errors.
Results
Patient Characteristics and Endoscopic Diagnoses
Out of 31,000 patients, the study identified a total of 566 patients (1.8%) with acute UGIH (Table 1). However, 116 patients transferred from another hospital were excluded as their initial management was provided elsewhere, giving a final study sample of 450 patients. Overall, there are 163 admitting physicians from 6 sites, with 39 (24%) classified as hospitalists and 124 (76%) as nonhospitalists. Forty‐two percent (177/450) of patients were cared for by hospitalists. Compared to nonhospitalists, patients admitted to the hospitalist service were older (62.8 vs. 57.7 years, P < 0.01) and with third‐party payor mix differences (P < 0.01). However, there were no statistical differences between patients attended by hospitalists and nonhospitalists with regard to Complete Rockall risk score, Charlson comorbidity index, performance of early endoscopy, and mean hemoglobin values on admission. Upper endoscopy was performed in all patients with distribution of the 3 most common diagnoses being similar (P > 0.05) between hospitalists and nonhospitalists: erosive disease (49.7% vs. 54.6%), peptic ulcer disease (PUD) (48% vs. 46.9%), and varices (18.6% vs. 14.7%).
Variable | Admitting Service | P | |
---|---|---|---|
Hospitalist (n = 177) | Nonhospitalist (n = 273) | ||
| |||
Age, years (meanSD) | 62.817.4 | 57.718.5 | <0.01 |
Male sex, n (%) | 104 (58.8) | 169 (61.9) | 0.50 |
Ethnicity, n (%) | 0.13 | ||
White | 83 (46.9) | 102 (37.4) | |
African‐American | 34 (19.2) | 75 (27.5) | |
Hispanic | 21 (11.9) | 40 (14.7) | |
Asian/Pacific Islander | 24 (13.6) | 29 (10.6) | |
Others/unknown | 15 (8.5) | 27 (9.9) | |
Insurance, n (%) | <0.01 | ||
Medicare | 86 (48.6) | 104 (38.1) | |
Medicaid | 15 (8.5) | 33 (12.1) | |
No payer | 18 (10.2) | 36 (13.2) | |
Private | 46 (26) | 52 (19.1) | |
Unknown | 12 (6.8) | 48 (17.5) | |
Charlson Comorbidity Index (meanSD) | 1.91.6 | 1.81.7 | 0.51 |
Complete Rockall, n (%) | 0.11 | ||
Low‐risk (0‐2) | 82 (46.3) | 103 (37.7) | |
Moderate‐risk (3‐5) | 71 (40.1) | 137 (50.2) | |
High‐risk (6) | 24 (14.6) | 33 (12.1) | |
Early endoscopy (<24 hours) | 82 (46.3) | 133 (48.7) | 0.62 |
Endoscopic diagnosis, n (%)* | |||
Erosive disease | 88 (49.7) | 149 (54.6) | 0.31 |
Peptic ulcer disease | 85 (48.0) | 128 (46.9) | 0.81 |
Varices | 33 (18.6) | 40 (14.7) | 0.26 |
Mallory‐Weiss tear | 9 (5.1) | 21 (7.7) | 0.28 |
Angiodysplasia | 9 (5.1) | 13 (4.8) | 0.88 |
GI mass | 1 (0.6) | 4 (1.5) | 0.65 |
Normal | 7 (4.0) | 8 (2.9) | 0.55 |
Admission hemoglobin values (meanSD) | 10.22.9 | 10.22.9 | 0.78 |
Clinical Outcomes
Between hospitalists and nonhospitalists, unadjusted outcomes were similar (P > 0.05) for mortality (2.3% vs. 0.4%), recurrent bleeding (11% vs. 11%), need for endoscopic therapy (24% vs. 22%), ICU‐transfer and decompensation (15% vs. 15%), as well as an overall composite measure of any complication (79% vs. 72%) (Table 2). However, the hospitalist‐led teams performed more blood transfusions (74% vs. 63%, P = 0.02) and readmission rates were higher (7.3% vs. 3.3%, P = 0.05).
Outcomes, n (%) | Admitting Service | P | |
---|---|---|---|
Hospitalist (n = 177) | Nonhospitalist (n = 273) | ||
| |||
Inpatient mortality | 4 (2.3) | 1 (0.4) | 0.08 |
Recurrent bleeding* | 20 (11.3) | 29 (10.6) | 0.88 |
Endoscopic therapy | 43 (24.3) | 60 (22.0) | 0.57 |
ICU transfers | 23 (13) | 24 (8.8) | 0.20 |
Decompensated comorbidities that required continued hospitalization | 26 (14.7) | 41 (15.0) | 0.92 |
Any transfusion | 131 (74.0) | 172 (63.0) | 0.02 |
Total complications | 139 (78.5) | 196 (71.8) | 0.11 |
30‐day all‐cause readmissions | 13 (7.3) | 9 (3.3) | 0.05 |
Efficiency | Hospitalist (n = 164) | Nonhospitalist (n = 259) | P |
LOS, days | |||
MeanSD | 4.83.5 | 4.53.0 | 0.30 |
Median (interquartile range) | 4 (36) | 4 (26) | 0.69 |
Total costs, U.S. $ | |||
MeanSD | 10,466.669191.00 | 7926.716065.00 | <0.01 |
Median (interquartile range) | 7359.00 (4,698.0012,550.00) | 6181.00 (3744.0010,344.00) | <0.01 |
Because of the low event rate of certain adverse outcomes (<20%), we were only able to perform adjusted analyses on 4 outcomes: need for endoscopic therapy (odds ratio [OR], 0.82; 95% confidence interval [CI], 0.491.37), ICU transfer and decompensation (OR, 0.82; 95% CI, 0.451.52), blood transfusion (OR, 1.30; 95% CI, 0.822.04), and any complication (OR, 1.18; 95% CI, 0.711.96). Since outcome differences disappeared after controlling for confounders, the data suggest that overall care provided by hospitalists and nonhospitalists might be equivalenteven in certain outcomes that we were unable to substantiate using multivariable methods.
Efficiency
Efficiency, as measured by LOS and costs, are presented both as means and medians in univariate analyses in Table 2. Median LOS was similar for hospitalist‐led and nonhospitalist‐led teams (4 days). Despite having similar LOS, the median costs of acute UGIH in patients cared for by hospitalists were higher ($7,359.00 vs. $6,181.00; P < 0.01).
After adjusting for demographic factors, Rockall risk score, comorbidity, early EGD, and hospital site, LOS remained similar between the 2 groups. On the other hand, the adjusted cost for UGIH patients cared for by hospitalists and nonhospitalists persisted, with hospitalist care costs $1,502.40 more than their nonhospitalist counterparts (Table 3).
Efficiency | Treatment Provider | P | |
---|---|---|---|
Hospitalist (n = 164) | Nonhospitalist (n = 259) | ||
| |||
Adjusted length of stay, days (mean SD) | 5.2 (4.95.6) | 4.7 (4.55.0) | 0.15 |
Adjusted total cost, U.S. $ (mean SD) | 9006.50 (8366.609693.60) | 7504.10 (7069.907964.20) | 0.03 |
Discussion
This is the first study that has looked at the effect of hospitalists on clinical outcomes and efficiency in patients admitted for acute UGIH, a condition highly dependent upon another specialty for procedures and management. This is also one of only a few studies on UGIH that adjusted for severity of illness (Rockall score), comorbidity, performance of early endoscopypatient‐level confounders usually unaccounted for in prior research.
We show that hospitalists and nonhospitalists caring for acute UGIH patients had overall similar unadjusted outcomes; except for blood transfusion and 30‐day readmission rates. Unfortunately, due to the small number of events for readmissions, we were unable to perform adjusted analysis for readmission. Differences between hospitalists and nonhospitalists on blood transfusion rates were not substantiated on multivariable adjustments.
As for efficiency, univariable and multivariable analyses revealed that LOS was similar between provider types while costs were greater in UGIH patients attended by hospitalists.
Reductions in resource use, particularly costs, may be achieved by increasing throughput (eg, reducing LOS) or by decreasing service intensity (eg, using fewer ancillary services and specialty consultations).26 Specifically in acute UGIH, LOS is significantly affected by performance of early EGD.27, 28 In these studies, gastroenterologist‐led teams, compared to internists and surgeons, have easier access to endoscopy, thus reducing LOS and overall costs.27, 28
Similarly, prior studies have shown that the mechanism by which hospitalists lower costs is by decreasing LOS.810, 29 There are several hypotheses on how hospitalists affect LOS. Hospitalists, by being available all day, are thought to respond quickly to acute symptoms or new test results, are more efficient in navigating the complex hospital environment, or develop greater expertise as a result of added inpatient experience.8 On the downside, although the hospitalist model reduces overall LOS and costs, they also provide higher intensity of care as reflected by greater costs when broken down per hospital day.29 Thus, the cost differential we found may represent higher intensity of care by hospitalists in their management of acute UGIH, as higher intensity care without decreasing LOS can translate to higher costs.
In addition, patients with acute UGIH are unique in several respects. In contrast to diseases like heart failure, COPD, and pneumonia, in which the admitting provider has the option to request a subspecialist consultation, all patients with acute UGIH need a gastroenterologist to perform endoscopy as part of the management. These patients are usually admitted to general medicine wards, aggressively resuscitated with intravenous fluids, with a nonurgent gastroenterology consult or EGD performed on the next available schedule.
Aside from LOS being greatly affected by performance of early EGD and/or delay in consulting gastroenterology, sicker patients require longer hospitalization and drive LOS and healthcare costs up. It was therefore crucial that we accounted for severity of illness, comorbidity, and performance of early EGD in our regression models for LOS and costs. This approach allows us to acquire a more accurate estimate on the effects of hospitalist on LOS and costs in patients admitted with acute UGIH.
Our findings suggest that the academic hospitalist model of care may not have as great of an impact on hospital efficiency in certain patient groups that require nonurgent subspecialty consultations. Future studies should focus on elucidating these relationships.
Limitations
This study has several limitations. First, clinical data were abstracted at 6 sites by different abstractors so it is possible there were variations in how data were collected. To reduce variation, a standardized abstraction form with instructions was developed and the primary investigator (PI) was available for specific questions during the abstraction process. Second, only 5 out of the 6 sites used TSI accounting systems. Although similar, interhospital costs captured by TSI may vary among sites in terms of classifying direct and indirect costs, potentially resulting in misclassification bias in our cost estimates.17 We addressed these issues by including the hospital site variable in our regression models, regardless of its significance. Third, consent rates across sites vary from 70% to 85%. It is possible that patients who refused enrollment in the MCH trial are systematically different and may introduce bias in our analysis.
Furthermore, the study was designed as a natural experiment based on a rotational call cycle between hospitalist‐led and nonhospitalist‐led teams. It is possible that the order of patient assignment might not be completely naturally random as we intended. However, the study period was for 2 years and we expect the effect of order would have averaged out in time.
There are many hospitalist models of care. In terms of generalizability, the study pertains only to academic hospitalists and may not be applicable to hospitalists practicing in community hospitals. For example, the nonhospitalist comparison group is likely different in the community and academic settings. Community nonhospitalists (traditional practitioners) are usually internists covering both inpatient and outpatient responsibilities at the same time. In contrast, academic nonhospitalists are internists or subspecialists serving as ward attendings for a limited period (usually 1 month) with considerable variation in their nonattending responsibilities (eg, research, clinic, administration). Furthermore, academic nonhospitalist providers might be a self‐selected group by their willingness to serve as a ward attending, making them more hospitalist‐like. Changes and variability of inpatient attendings may also affect our findings when compared to prior work. Finally, it is also possible that having residents at academic medical centers may attenuate the effect of hospitalists more than in community‐based models.
Conclusions/Implications
Compared to nonhospitalists, academic hospitalist care of acute UGIH patients had similar overall clinical outcomes. However, our finding of similar LOS yet higher costs for patients cared for by hospitalists support 1 proposed mechanism in which hospitalists decrease healthcare costs: providing higher intensity of care per day of hospitalization. However, in academic hospitalist models, this higher intensity hypothesis should be revisited, especially in certain patient groups in which timing and involvement of subspecialists may influence discharge decisions, affecting LOS and overall costs.
Due to inherent limitations in this observational study, future studies should focus on verifying and elucidating these relationships further. Lastly, understanding which patient groups receive the greatest potential benefit from this model will help guide both organizational efforts and quality improvement strategies.
- Bleeding peptic ulcer.N Engl J Med.1994;331(11):717–727. , .
- Epidemiology of hospitalization for acute upper gastrointestinal hemorrhage: a population‐based study.Am J Gastroenterol.1995;90(2):206–210. .
- Variation in outcome after acute upper gastrointestinal haemorrhage. the national audit of acute upper gastrointestinal haemorrhage.Lancet.1995;346(8971):346–350. , , , et al.
- Influencing the practice and outcome in acute upper gastrointestinal haemorrhage. Steering committee of the National Audit of Acute Upper Gastrointestinal Haemorrhage.Gut.1997;41(5):606–611. , , , et al.
- Risk assessment after acute upper gastrointestinal haemorrhage.Gut.1996;38(3):316–321. , , , et al.
- The potential size of the hospitalist workforce in the united states.Am J Med.1999;106(4):441–445. , , , et al.
- Society of Hospital Medicine. About SHM. Available at: http://www.hospitalmedicine.org/AM/Template.cfm?Section=General_Information357(25):2589–2600.
- Effects of physician experience on costs and outcomes on an academic general medicine service: results of a trial of hospitalists.Ann Intern Med.2002;137(11):866–874. , , , et al.
- A systematic review of outcomes and quality measures in adult patients cared for by hospitalists vs nonhospitalists.Mayo Clin Proc.2009;84(3):248–254. .
- Do hospitalists or physicians with greater inpatient HIV experience improve HIV care in the era of highly active antiretroviral therapy? Results from a multicenter trial of academic hospitalists.Clin Infect Dis.2008;46(7):1085–1092. , , , et al.
- Quality of care for decompensated heart failure: comparable performance between academic hospitalists and non‐hospitalists. J Gen Intern Med.2008;23(9):1399–1406. , , , et al.
- Factors associated with discussion of care plans and code status at the time of hospital admission: results from the Multicenter Hospitalist Study.J Hosp Med.2008;3(6):437–445. , , , et al.
- Upper gastrointestinal hemorrhage clinical guideline determining the optimal hospital length of stay.Am J Med.1996;100(3):313–322. , , , et al.
- Prospective evaluation of a clinical guideline recommending hospital length of stay in upper gastrointestinal tract hemorrhage.JAMA.1997;278(24):2151–2156. , , , et al.
- In‐hospital cost of abdominal aortic aneurysm repair in Canada and the United States.Arch Intern Med.2003;163(20):2500–2504. , , , et al.
- The use of transition cost accounting system in health services research.Cost Eff Resour Alloc.2007;5:11. , , , et al.
- Society of Hospital Medicine. Definition of a Hospitalist. Available at: http://www.hospitalmedicine.org/AM/Template.cfm?Section=General_Information335(7):514–517.
- Selection of patients for early discharge or outpatient care after acute upper gastrointestinal haemorrhage. National Audit of Acute Upper Gastrointestinal Haemorrhage.Lancet.1996;347(9009):1138–1140. , , , et al.
- Utilization of health care resources for low‐risk patients with acute, nonvariceal upper GI hemorrhage: an historical cohort study.Gastrointest Endosc.2002;55(3):321–327. , , , et al.
- Incremental value of upper endoscopy for triage of patients with acute non‐variceal upper‐GI hemorrhage.Gastrointest Endosc.2004;60(1):9–14. , .
- The Charlson comorbidity index is adapted to predict costs of chronic disease in primary care patients.J Clin Epidemiol.2008;61(12):1234–1240. , , , et al.
- The effectiveness of early endoscopy for upper gastrointestinal hemorrhage: a community‐based analysis.Med Care.1998;36(4):462–474. , , , et al.
- Early endoscopy in upper gastrointestinal hemorrhage: associations with recurrent bleeding, surgery, and length of hospital stay.Gastrointest Endosc.1999;49(2):145–152. , , , et al.
- The impact of hospitalists on the cost and quality of inpatient care in the united states: a research synthesis.Med Care Res Rev.2005;62(4):379–406. , .
- Physician specialty and variations in the cost of treating patients with acute upper gastrointestinal bleeding.Gastroenterology.1997;113(5):1443–1448. , , , et al.
- Impact of physician specialty on the cost of nonvariceal upper GI bleeding care.Am J Gastroenterol.2002;97(6):1535–1542. , , , et al.
- Associations with reduced length of stay and costs on an academic hospitalist service.Am J Manag Care.2004;10(8):561–568. , , .
Acute upper gastrointestinal hemorrhage (UGIH) is one of the most common hospital admissions for acute care. Estimates indicate that 300,000 patients (100‐150 cases per 100,000 adults) are admitted annually with an associated economic impact of $2.5 billion.15 The current standard management of UGIH requires hospital admission and esophagogastroduodenoscopy (EGD) by a gastroenterologist for diagnosis and/or treatment. This management strategy results in a high consumption of hospital resources and costs.
Simultaneously, hospitalists have dramatically changed the delivery of inpatient care in the United States and are recognized as a location‐driven subspecialty for the care of acute hospitalized patients, similar to emergency medicine. Currently there are 20,000 hospitalists, and more than one‐third of general medicine inpatients are cared for by hospitalists.6, 7
Previous studies have shown that hospitalist care offers better or comparable outcomes, with lower overall length of stay (LOS) and costs compared to traditional providers.810 However, most of these studies were performed in single institutions, had weak designs or little‐to‐no adjustment for severity of illness, or were limited to 7 specific diseases (pneumonia, congestive heart failure [CHF], chest pain, ischemic stroke, urinary tract infection, chronic obstructive lung disease [COPD], and acute myocardial infarction [AMI]).8
Furthermore, less is known about the effect of hospitalists on conditions that may be dependent upon specialist consultation for procedures and/or treatment plans. In this study, gastroenterologists performed diagnostic and/or therapeutic endoscopy work as consultants to the attending physicians in the management of acute inpatient UGIH.
To explore the effects of hospitalists on care of patients with acute UGIH, we examined data from the Multicenter Hospitalist (MCH) trial. The objectives of our study were to compare clinical outcomesin‐hospital mortality and complications (ie, recurrent bleeding, intensive care unit [ICU] transfer, decompensation, transfusion, reendoscopy, 30‐day readmission)and efficiency (LOS and costs) in hospitalized acute UGIH patients cared for by hospitalists and nonhospitalists in 6 academic centers in the United States during a 2‐year period.
Patients and Methods
Study Sites
From July 1, 2001 to June 30, 2003, the MCH trial1113 was a prospective, multicenter, observational trial of the care provided by hospitalists to patients admitted to general medical services at 6 academic medical institutions. There were 31,000 consecutive admissions to the general medical services of these participating sites: University of Chicago (Chicago, IL), University of Wisconsin Hospital (Madison, WI), University of Iowa (Iowa City, IA), University of California at San Francisco (San Francisco, CA), University of New Mexico (Albuquerque, NM), and Brigham and Women's Hospital (Boston, MA). The study was approved by the institutional review boards (IRBs) at each of the 6 participating institutions.
MCH Study Patients
Patients were eligible if they were admitted to the general medical services under the care of a hospitalist or nonhospitalist physician. Regardless of the admitting provider, each medical service was composed of rotating senior and junior resident physicians in all 6 sites. Furthermore, patients were 18 years of age or older, and were able to give consent themselves or had an appropriate proxy. Patients with mini‐mental status score of 17 (out of 22), admitted under their primary care physician or to an inpatient gastroenterology service, or transferred from another hospital, were excluded. The MCH study was designed to study the outcomes and efficiency in patients admitted for CHF, pneumonia, UGIH, and end‐of‐life care.
Acute UGIH Patients
Within the MCH‐eligible patients, we identified those with acute UGIH using the following International Classification of Diseases, 9th edition (ICD‐9) codes assigned at discharge: esophageal varices with hemorrhage (456.0, 456.20); Mallory‐Weiss syndrome (530.7); gastric ulcer with hemorrhage (531.00531.61); duodenal ulcer with hemorrhage (532.00532.61); peptic ulcer, site unspecified, with hemorrhage (533.00533.61); gastrojejunal ulcer with hemorrhage (534.00534.61); gastritis with hemorrhage (535.61); angiodysplasia of stomach/duodenum with hemorrhage (537.83); and hematemesis (578.0, 578.9). We also confirmed the diagnosis of UGIH by reviewing patient medical records for observed hematemesis, nasogastric tube aspirate with gross or hemoccult blood, or clinical history of hematemesis, melena, or hematochezia.14, 15
Data
All data were obtained from the 6 hospitals' administrative records, patient interviews, and medical chart abstractions. Dates of admission and discharge, ICD‐9 diagnosis codes, insurance type, age, race, and gender were obtained from administrative data. One‐month follow‐up telephone interviews assessed whether or not patient had any follow‐up appointment or hospital readmissions. Trained abstractors from each site performed manual chart reviews using a standard data collection sheet. The ICD‐9 code designation and chart abstraction methodology were developed prior to the initiation of the study to ensure consistent data collection and reduce bias.
The following data elements were collected: comorbidities, endoscopic findings, inpatient mortality, clinical evidence of rebleeding, endoscopic treatment or gastrointestinal (GI) surgery to control bleeding, repeat EGD, ICU transfer, decompensated comorbid illness requiring continued hospitalization, and blood transfusion (packed red cells, plasma, platelets). Clinical evidence of rebleeding was defined as either hematemesis or melena with decrease in hemoglobin of 2 g in 24 hours with or without hemodynamic compromise.14, 15 For the purpose of this study, recurrent bleeding was defined as clinical evidence of rebleeding, emergency GI surgery for control of UGIH, or repeat EGD before discharge. Furthermore, a composite endpoint termed total complications encompassed all adverse outcomes related to the UGIH hospitalization. The 30‐day readmission variable was defined using readmission identified in administrative records and a 30‐day follow‐up phone call. To guard against recall bias, self‐report data was only included for nonsite admissions.
We defined efficiency in terms of costs and LOS. Total hospital costs were measured using the TSI cost accounting system (Transition Systems, Inc., Boston, MA; now Eclipsys Corporation)16, 17 at 5 out of the 6 participating sites. TSI is a hospital cost accounting software system that integrates resource utilization and financial data already recorded in other hospital databases (such as the billing system, payroll system, and general ledger system).17 Hospital LOS was defined as the number of days from patient admission to the general medicine service until patient discharge.
Provider Specialization: Hospitalists vs. Nonhospitalists
The study was designed as a natural experiment based on a call cycle. The hospitalist‐led teams at each institution alternated in a 4‐day or 5‐day general medicine call cycle with teams led by traditional academic internal medicine attending physicians. All patients were assigned to teams according to their position in the call cycle without regard to whether the attending physician was a hospitalist or a nonhospitalist. Hospitalists are physicians whose primary professional focus is the general medical care of hospitalized patients.18, 19 As previously reported in a related MCH work,11 a hospitalist was also defined as a provider who spends at least 25% of his or her time on an academic inpatient general medicine service. Nonhospitalist physicians were most often outpatient general internal medicine faculty or subspecialists, who attended 1 month per year. Physicians were classified as hospitalists or nonhospitalists according to the designations provided by each site.
UGIH‐specific Confounders
From chart abstraction, we captured severity of illness, comorbidity, and performance of early EGD, variables that can confound analysis in UGIH. To capture severity of illness, a complete Rockall risk score was calculated for each patient. The complete Rockall uses 3 clinical variables (age, shock, and comorbidity) and 2 endoscopic variables (endoscopic diagnosis and stigmata of recent hemorrhage).5, 20 A complete Rockall score of 2 is considered low‐risk for rebleeding or death following admission.21, 22 The accepted definition of low‐risk is <5% recurrent bleeding and <1% mortality risk. A complete Rockall score of 3 to 5 is considered moderate‐risk while 6 is considered high‐risk. Comorbidity was measured using the Charlson comorbidity index.23 Performance of early endoscopy, usually defined as endoscopy performed within 24 hours from presentation, was previously shown to decrease LOS and need for surgical intervention in patients with acute UGIH.24, 25 Documented times of presentation to the emergency department and time of endoscopy performance were collected to calculate for the rate of early endoscopy in our study population.
Statistical Analysis
All statistical analyses were performed using SAS Version 9.1 for Windows (SAS Institute, Cary, NC).
Differences in baseline demographic characteristics of patients and their endoscopic findings were compared between the 2 types of providers. Univariate analyses were also performed to compare the differences in adverse outcomes, LOS, and costs between patients cared for by hospitalists and nonhospitalists. Chi‐square tests were used for categorical variables; while both Wilcoxon rank sum test and Student's t test were used in the analysis of continuous variables.
Next, we performed multivariable analyses to determine the independent association between hospitalist care and the odds of the patients having certain outcomes. However, to prevent overfitting, we only developed regression models for adverse outcomes that have at least 20% event rate.
Multivariable regression models were developed separately for LOS and costs. In contrast with the models on outcomes, analyses of LOS and costs were restricted to: (1) patients who were discharged alive; and (2) to cases with LOS and costs values within 3 standard deviations (SDs) of the mean because of the skewed nature of these data.
All models were adjusted for age, gender, race, insurance type, complete Rockall risk score, performance of early EGD, Charlson comorbidity index, and study site. Final candidate variables in the models were chosen based on stepwise selection, a method very similar to forward selection except that variables selected for the model do not necessarily remain in the model. Effects were entered into and then removed from the model in such a way that each forward selection step can be followed by 1 or more backward elimination steps. The stepwise selection was terminated if no further effect can be added to the model or if the current model was identical to the previous model. The stepwise selection model was generated using statistical criterion of alpha = 0.05 for entry and elimination from the model. Variables that can be a profound source of variation, such as study site and treating physician, were included in the model irrespective of their statistical significance.
To account for clustering of patients treated by the same physician, we used multilevel modeling with SAS PROC GLIMMIX (with random effects). For outcomes (categorical variables), we utilized models with logit‐link and binomial‐distributed errors. As for efficiency (continuous variables with skewed distribution), the multivariable analyses used a generalized linear model with log‐link and assuming gamma‐distributed errors.
Results
Patient Characteristics and Endoscopic Diagnoses
Out of 31,000 patients, the study identified a total of 566 patients (1.8%) with acute UGIH (Table 1). However, 116 patients transferred from another hospital were excluded as their initial management was provided elsewhere, giving a final study sample of 450 patients. Overall, there are 163 admitting physicians from 6 sites, with 39 (24%) classified as hospitalists and 124 (76%) as nonhospitalists. Forty‐two percent (177/450) of patients were cared for by hospitalists. Compared to nonhospitalists, patients admitted to the hospitalist service were older (62.8 vs. 57.7 years, P < 0.01) and with third‐party payor mix differences (P < 0.01). However, there were no statistical differences between patients attended by hospitalists and nonhospitalists with regard to Complete Rockall risk score, Charlson comorbidity index, performance of early endoscopy, and mean hemoglobin values on admission. Upper endoscopy was performed in all patients with distribution of the 3 most common diagnoses being similar (P > 0.05) between hospitalists and nonhospitalists: erosive disease (49.7% vs. 54.6%), peptic ulcer disease (PUD) (48% vs. 46.9%), and varices (18.6% vs. 14.7%).
Variable | Admitting Service | P | |
---|---|---|---|
Hospitalist (n = 177) | Nonhospitalist (n = 273) | ||
| |||
Age, years (meanSD) | 62.817.4 | 57.718.5 | <0.01 |
Male sex, n (%) | 104 (58.8) | 169 (61.9) | 0.50 |
Ethnicity, n (%) | 0.13 | ||
White | 83 (46.9) | 102 (37.4) | |
African‐American | 34 (19.2) | 75 (27.5) | |
Hispanic | 21 (11.9) | 40 (14.7) | |
Asian/Pacific Islander | 24 (13.6) | 29 (10.6) | |
Others/unknown | 15 (8.5) | 27 (9.9) | |
Insurance, n (%) | <0.01 | ||
Medicare | 86 (48.6) | 104 (38.1) | |
Medicaid | 15 (8.5) | 33 (12.1) | |
No payer | 18 (10.2) | 36 (13.2) | |
Private | 46 (26) | 52 (19.1) | |
Unknown | 12 (6.8) | 48 (17.5) | |
Charlson Comorbidity Index (meanSD) | 1.91.6 | 1.81.7 | 0.51 |
Complete Rockall, n (%) | 0.11 | ||
Low‐risk (0‐2) | 82 (46.3) | 103 (37.7) | |
Moderate‐risk (3‐5) | 71 (40.1) | 137 (50.2) | |
High‐risk (6) | 24 (14.6) | 33 (12.1) | |
Early endoscopy (<24 hours) | 82 (46.3) | 133 (48.7) | 0.62 |
Endoscopic diagnosis, n (%)* | |||
Erosive disease | 88 (49.7) | 149 (54.6) | 0.31 |
Peptic ulcer disease | 85 (48.0) | 128 (46.9) | 0.81 |
Varices | 33 (18.6) | 40 (14.7) | 0.26 |
Mallory‐Weiss tear | 9 (5.1) | 21 (7.7) | 0.28 |
Angiodysplasia | 9 (5.1) | 13 (4.8) | 0.88 |
GI mass | 1 (0.6) | 4 (1.5) | 0.65 |
Normal | 7 (4.0) | 8 (2.9) | 0.55 |
Admission hemoglobin values (meanSD) | 10.22.9 | 10.22.9 | 0.78 |
Clinical Outcomes
Between hospitalists and nonhospitalists, unadjusted outcomes were similar (P > 0.05) for mortality (2.3% vs. 0.4%), recurrent bleeding (11% vs. 11%), need for endoscopic therapy (24% vs. 22%), ICU‐transfer and decompensation (15% vs. 15%), as well as an overall composite measure of any complication (79% vs. 72%) (Table 2). However, the hospitalist‐led teams performed more blood transfusions (74% vs. 63%, P = 0.02) and readmission rates were higher (7.3% vs. 3.3%, P = 0.05).
Outcomes, n (%) | Admitting Service | P | |
---|---|---|---|
Hospitalist (n = 177) | Nonhospitalist (n = 273) | ||
| |||
Inpatient mortality | 4 (2.3) | 1 (0.4) | 0.08 |
Recurrent bleeding* | 20 (11.3) | 29 (10.6) | 0.88 |
Endoscopic therapy | 43 (24.3) | 60 (22.0) | 0.57 |
ICU transfers | 23 (13) | 24 (8.8) | 0.20 |
Decompensated comorbidities that required continued hospitalization | 26 (14.7) | 41 (15.0) | 0.92 |
Any transfusion | 131 (74.0) | 172 (63.0) | 0.02 |
Total complications | 139 (78.5) | 196 (71.8) | 0.11 |
30‐day all‐cause readmissions | 13 (7.3) | 9 (3.3) | 0.05 |
Efficiency | Hospitalist (n = 164) | Nonhospitalist (n = 259) | P |
LOS, days | |||
MeanSD | 4.83.5 | 4.53.0 | 0.30 |
Median (interquartile range) | 4 (36) | 4 (26) | 0.69 |
Total costs, U.S. $ | |||
MeanSD | 10,466.669191.00 | 7926.716065.00 | <0.01 |
Median (interquartile range) | 7359.00 (4,698.0012,550.00) | 6181.00 (3744.0010,344.00) | <0.01 |
Because of the low event rate of certain adverse outcomes (<20%), we were only able to perform adjusted analyses on 4 outcomes: need for endoscopic therapy (odds ratio [OR], 0.82; 95% confidence interval [CI], 0.491.37), ICU transfer and decompensation (OR, 0.82; 95% CI, 0.451.52), blood transfusion (OR, 1.30; 95% CI, 0.822.04), and any complication (OR, 1.18; 95% CI, 0.711.96). Since outcome differences disappeared after controlling for confounders, the data suggest that overall care provided by hospitalists and nonhospitalists might be equivalenteven in certain outcomes that we were unable to substantiate using multivariable methods.
Efficiency
Efficiency, as measured by LOS and costs, are presented both as means and medians in univariate analyses in Table 2. Median LOS was similar for hospitalist‐led and nonhospitalist‐led teams (4 days). Despite having similar LOS, the median costs of acute UGIH in patients cared for by hospitalists were higher ($7,359.00 vs. $6,181.00; P < 0.01).
After adjusting for demographic factors, Rockall risk score, comorbidity, early EGD, and hospital site, LOS remained similar between the 2 groups. On the other hand, the adjusted cost for UGIH patients cared for by hospitalists and nonhospitalists persisted, with hospitalist care costs $1,502.40 more than their nonhospitalist counterparts (Table 3).
Efficiency | Treatment Provider | P | |
---|---|---|---|
Hospitalist (n = 164) | Nonhospitalist (n = 259) | ||
| |||
Adjusted length of stay, days (mean SD) | 5.2 (4.95.6) | 4.7 (4.55.0) | 0.15 |
Adjusted total cost, U.S. $ (mean SD) | 9006.50 (8366.609693.60) | 7504.10 (7069.907964.20) | 0.03 |
Discussion
This is the first study that has looked at the effect of hospitalists on clinical outcomes and efficiency in patients admitted for acute UGIH, a condition highly dependent upon another specialty for procedures and management. This is also one of only a few studies on UGIH that adjusted for severity of illness (Rockall score), comorbidity, performance of early endoscopypatient‐level confounders usually unaccounted for in prior research.
We show that hospitalists and nonhospitalists caring for acute UGIH patients had overall similar unadjusted outcomes; except for blood transfusion and 30‐day readmission rates. Unfortunately, due to the small number of events for readmissions, we were unable to perform adjusted analysis for readmission. Differences between hospitalists and nonhospitalists on blood transfusion rates were not substantiated on multivariable adjustments.
As for efficiency, univariable and multivariable analyses revealed that LOS was similar between provider types while costs were greater in UGIH patients attended by hospitalists.
Reductions in resource use, particularly costs, may be achieved by increasing throughput (eg, reducing LOS) or by decreasing service intensity (eg, using fewer ancillary services and specialty consultations).26 Specifically in acute UGIH, LOS is significantly affected by performance of early EGD.27, 28 In these studies, gastroenterologist‐led teams, compared to internists and surgeons, have easier access to endoscopy, thus reducing LOS and overall costs.27, 28
Similarly, prior studies have shown that the mechanism by which hospitalists lower costs is by decreasing LOS.810, 29 There are several hypotheses on how hospitalists affect LOS. Hospitalists, by being available all day, are thought to respond quickly to acute symptoms or new test results, are more efficient in navigating the complex hospital environment, or develop greater expertise as a result of added inpatient experience.8 On the downside, although the hospitalist model reduces overall LOS and costs, they also provide higher intensity of care as reflected by greater costs when broken down per hospital day.29 Thus, the cost differential we found may represent higher intensity of care by hospitalists in their management of acute UGIH, as higher intensity care without decreasing LOS can translate to higher costs.
In addition, patients with acute UGIH are unique in several respects. In contrast to diseases like heart failure, COPD, and pneumonia, in which the admitting provider has the option to request a subspecialist consultation, all patients with acute UGIH need a gastroenterologist to perform endoscopy as part of the management. These patients are usually admitted to general medicine wards, aggressively resuscitated with intravenous fluids, with a nonurgent gastroenterology consult or EGD performed on the next available schedule.
Aside from LOS being greatly affected by performance of early EGD and/or delay in consulting gastroenterology, sicker patients require longer hospitalization and drive LOS and healthcare costs up. It was therefore crucial that we accounted for severity of illness, comorbidity, and performance of early EGD in our regression models for LOS and costs. This approach allows us to acquire a more accurate estimate on the effects of hospitalist on LOS and costs in patients admitted with acute UGIH.
Our findings suggest that the academic hospitalist model of care may not have as great of an impact on hospital efficiency in certain patient groups that require nonurgent subspecialty consultations. Future studies should focus on elucidating these relationships.
Limitations
This study has several limitations. First, clinical data were abstracted at 6 sites by different abstractors so it is possible there were variations in how data were collected. To reduce variation, a standardized abstraction form with instructions was developed and the primary investigator (PI) was available for specific questions during the abstraction process. Second, only 5 out of the 6 sites used TSI accounting systems. Although similar, interhospital costs captured by TSI may vary among sites in terms of classifying direct and indirect costs, potentially resulting in misclassification bias in our cost estimates.17 We addressed these issues by including the hospital site variable in our regression models, regardless of its significance. Third, consent rates across sites vary from 70% to 85%. It is possible that patients who refused enrollment in the MCH trial are systematically different and may introduce bias in our analysis.
Furthermore, the study was designed as a natural experiment based on a rotational call cycle between hospitalist‐led and nonhospitalist‐led teams. It is possible that the order of patient assignment might not be completely naturally random as we intended. However, the study period was for 2 years and we expect the effect of order would have averaged out in time.
There are many hospitalist models of care. In terms of generalizability, the study pertains only to academic hospitalists and may not be applicable to hospitalists practicing in community hospitals. For example, the nonhospitalist comparison group is likely different in the community and academic settings. Community nonhospitalists (traditional practitioners) are usually internists covering both inpatient and outpatient responsibilities at the same time. In contrast, academic nonhospitalists are internists or subspecialists serving as ward attendings for a limited period (usually 1 month) with considerable variation in their nonattending responsibilities (eg, research, clinic, administration). Furthermore, academic nonhospitalist providers might be a self‐selected group by their willingness to serve as a ward attending, making them more hospitalist‐like. Changes and variability of inpatient attendings may also affect our findings when compared to prior work. Finally, it is also possible that having residents at academic medical centers may attenuate the effect of hospitalists more than in community‐based models.
Conclusions/Implications
Compared to nonhospitalists, academic hospitalist care of acute UGIH patients had similar overall clinical outcomes. However, our finding of similar LOS yet higher costs for patients cared for by hospitalists support 1 proposed mechanism in which hospitalists decrease healthcare costs: providing higher intensity of care per day of hospitalization. However, in academic hospitalist models, this higher intensity hypothesis should be revisited, especially in certain patient groups in which timing and involvement of subspecialists may influence discharge decisions, affecting LOS and overall costs.
Due to inherent limitations in this observational study, future studies should focus on verifying and elucidating these relationships further. Lastly, understanding which patient groups receive the greatest potential benefit from this model will help guide both organizational efforts and quality improvement strategies.
Acute upper gastrointestinal hemorrhage (UGIH) is one of the most common hospital admissions for acute care. Estimates indicate that 300,000 patients (100‐150 cases per 100,000 adults) are admitted annually with an associated economic impact of $2.5 billion.15 The current standard management of UGIH requires hospital admission and esophagogastroduodenoscopy (EGD) by a gastroenterologist for diagnosis and/or treatment. This management strategy results in a high consumption of hospital resources and costs.
Simultaneously, hospitalists have dramatically changed the delivery of inpatient care in the United States and are recognized as a location‐driven subspecialty for the care of acute hospitalized patients, similar to emergency medicine. Currently there are 20,000 hospitalists, and more than one‐third of general medicine inpatients are cared for by hospitalists.6, 7
Previous studies have shown that hospitalist care offers better or comparable outcomes, with lower overall length of stay (LOS) and costs compared to traditional providers.810 However, most of these studies were performed in single institutions, had weak designs or little‐to‐no adjustment for severity of illness, or were limited to 7 specific diseases (pneumonia, congestive heart failure [CHF], chest pain, ischemic stroke, urinary tract infection, chronic obstructive lung disease [COPD], and acute myocardial infarction [AMI]).8
Furthermore, less is known about the effect of hospitalists on conditions that may be dependent upon specialist consultation for procedures and/or treatment plans. In this study, gastroenterologists performed diagnostic and/or therapeutic endoscopy work as consultants to the attending physicians in the management of acute inpatient UGIH.
To explore the effects of hospitalists on care of patients with acute UGIH, we examined data from the Multicenter Hospitalist (MCH) trial. The objectives of our study were to compare clinical outcomesin‐hospital mortality and complications (ie, recurrent bleeding, intensive care unit [ICU] transfer, decompensation, transfusion, reendoscopy, 30‐day readmission)and efficiency (LOS and costs) in hospitalized acute UGIH patients cared for by hospitalists and nonhospitalists in 6 academic centers in the United States during a 2‐year period.
Patients and Methods
Study Sites
From July 1, 2001 to June 30, 2003, the MCH trial1113 was a prospective, multicenter, observational trial of the care provided by hospitalists to patients admitted to general medical services at 6 academic medical institutions. There were 31,000 consecutive admissions to the general medical services of these participating sites: University of Chicago (Chicago, IL), University of Wisconsin Hospital (Madison, WI), University of Iowa (Iowa City, IA), University of California at San Francisco (San Francisco, CA), University of New Mexico (Albuquerque, NM), and Brigham and Women's Hospital (Boston, MA). The study was approved by the institutional review boards (IRBs) at each of the 6 participating institutions.
MCH Study Patients
Patients were eligible if they were admitted to the general medical services under the care of a hospitalist or nonhospitalist physician. Regardless of the admitting provider, each medical service was composed of rotating senior and junior resident physicians in all 6 sites. Furthermore, patients were 18 years of age or older, and were able to give consent themselves or had an appropriate proxy. Patients with mini‐mental status score of 17 (out of 22), admitted under their primary care physician or to an inpatient gastroenterology service, or transferred from another hospital, were excluded. The MCH study was designed to study the outcomes and efficiency in patients admitted for CHF, pneumonia, UGIH, and end‐of‐life care.
Acute UGIH Patients
Within the MCH‐eligible patients, we identified those with acute UGIH using the following International Classification of Diseases, 9th edition (ICD‐9) codes assigned at discharge: esophageal varices with hemorrhage (456.0, 456.20); Mallory‐Weiss syndrome (530.7); gastric ulcer with hemorrhage (531.00531.61); duodenal ulcer with hemorrhage (532.00532.61); peptic ulcer, site unspecified, with hemorrhage (533.00533.61); gastrojejunal ulcer with hemorrhage (534.00534.61); gastritis with hemorrhage (535.61); angiodysplasia of stomach/duodenum with hemorrhage (537.83); and hematemesis (578.0, 578.9). We also confirmed the diagnosis of UGIH by reviewing patient medical records for observed hematemesis, nasogastric tube aspirate with gross or hemoccult blood, or clinical history of hematemesis, melena, or hematochezia.14, 15
Data
All data were obtained from the 6 hospitals' administrative records, patient interviews, and medical chart abstractions. Dates of admission and discharge, ICD‐9 diagnosis codes, insurance type, age, race, and gender were obtained from administrative data. One‐month follow‐up telephone interviews assessed whether or not patient had any follow‐up appointment or hospital readmissions. Trained abstractors from each site performed manual chart reviews using a standard data collection sheet. The ICD‐9 code designation and chart abstraction methodology were developed prior to the initiation of the study to ensure consistent data collection and reduce bias.
The following data elements were collected: comorbidities, endoscopic findings, inpatient mortality, clinical evidence of rebleeding, endoscopic treatment or gastrointestinal (GI) surgery to control bleeding, repeat EGD, ICU transfer, decompensated comorbid illness requiring continued hospitalization, and blood transfusion (packed red cells, plasma, platelets). Clinical evidence of rebleeding was defined as either hematemesis or melena with decrease in hemoglobin of 2 g in 24 hours with or without hemodynamic compromise.14, 15 For the purpose of this study, recurrent bleeding was defined as clinical evidence of rebleeding, emergency GI surgery for control of UGIH, or repeat EGD before discharge. Furthermore, a composite endpoint termed total complications encompassed all adverse outcomes related to the UGIH hospitalization. The 30‐day readmission variable was defined using readmission identified in administrative records and a 30‐day follow‐up phone call. To guard against recall bias, self‐report data was only included for nonsite admissions.
We defined efficiency in terms of costs and LOS. Total hospital costs were measured using the TSI cost accounting system (Transition Systems, Inc., Boston, MA; now Eclipsys Corporation)16, 17 at 5 out of the 6 participating sites. TSI is a hospital cost accounting software system that integrates resource utilization and financial data already recorded in other hospital databases (such as the billing system, payroll system, and general ledger system).17 Hospital LOS was defined as the number of days from patient admission to the general medicine service until patient discharge.
Provider Specialization: Hospitalists vs. Nonhospitalists
The study was designed as a natural experiment based on a call cycle. The hospitalist‐led teams at each institution alternated in a 4‐day or 5‐day general medicine call cycle with teams led by traditional academic internal medicine attending physicians. All patients were assigned to teams according to their position in the call cycle without regard to whether the attending physician was a hospitalist or a nonhospitalist. Hospitalists are physicians whose primary professional focus is the general medical care of hospitalized patients.18, 19 As previously reported in a related MCH work,11 a hospitalist was also defined as a provider who spends at least 25% of his or her time on an academic inpatient general medicine service. Nonhospitalist physicians were most often outpatient general internal medicine faculty or subspecialists, who attended 1 month per year. Physicians were classified as hospitalists or nonhospitalists according to the designations provided by each site.
UGIH‐specific Confounders
From chart abstraction, we captured severity of illness, comorbidity, and performance of early EGD, variables that can confound analysis in UGIH. To capture severity of illness, a complete Rockall risk score was calculated for each patient. The complete Rockall uses 3 clinical variables (age, shock, and comorbidity) and 2 endoscopic variables (endoscopic diagnosis and stigmata of recent hemorrhage).5, 20 A complete Rockall score of 2 is considered low‐risk for rebleeding or death following admission.21, 22 The accepted definition of low‐risk is <5% recurrent bleeding and <1% mortality risk. A complete Rockall score of 3 to 5 is considered moderate‐risk while 6 is considered high‐risk. Comorbidity was measured using the Charlson comorbidity index.23 Performance of early endoscopy, usually defined as endoscopy performed within 24 hours from presentation, was previously shown to decrease LOS and need for surgical intervention in patients with acute UGIH.24, 25 Documented times of presentation to the emergency department and time of endoscopy performance were collected to calculate for the rate of early endoscopy in our study population.
Statistical Analysis
All statistical analyses were performed using SAS Version 9.1 for Windows (SAS Institute, Cary, NC).
Differences in baseline demographic characteristics of patients and their endoscopic findings were compared between the 2 types of providers. Univariate analyses were also performed to compare the differences in adverse outcomes, LOS, and costs between patients cared for by hospitalists and nonhospitalists. Chi‐square tests were used for categorical variables; while both Wilcoxon rank sum test and Student's t test were used in the analysis of continuous variables.
Next, we performed multivariable analyses to determine the independent association between hospitalist care and the odds of the patients having certain outcomes. However, to prevent overfitting, we only developed regression models for adverse outcomes that have at least 20% event rate.
Multivariable regression models were developed separately for LOS and costs. In contrast with the models on outcomes, analyses of LOS and costs were restricted to: (1) patients who were discharged alive; and (2) to cases with LOS and costs values within 3 standard deviations (SDs) of the mean because of the skewed nature of these data.
All models were adjusted for age, gender, race, insurance type, complete Rockall risk score, performance of early EGD, Charlson comorbidity index, and study site. Final candidate variables in the models were chosen based on stepwise selection, a method very similar to forward selection except that variables selected for the model do not necessarily remain in the model. Effects were entered into and then removed from the model in such a way that each forward selection step can be followed by 1 or more backward elimination steps. The stepwise selection was terminated if no further effect can be added to the model or if the current model was identical to the previous model. The stepwise selection model was generated using statistical criterion of alpha = 0.05 for entry and elimination from the model. Variables that can be a profound source of variation, such as study site and treating physician, were included in the model irrespective of their statistical significance.
To account for clustering of patients treated by the same physician, we used multilevel modeling with SAS PROC GLIMMIX (with random effects). For outcomes (categorical variables), we utilized models with logit‐link and binomial‐distributed errors. As for efficiency (continuous variables with skewed distribution), the multivariable analyses used a generalized linear model with log‐link and assuming gamma‐distributed errors.
Results
Patient Characteristics and Endoscopic Diagnoses
Out of 31,000 patients, the study identified a total of 566 patients (1.8%) with acute UGIH (Table 1). However, 116 patients transferred from another hospital were excluded as their initial management was provided elsewhere, giving a final study sample of 450 patients. Overall, there are 163 admitting physicians from 6 sites, with 39 (24%) classified as hospitalists and 124 (76%) as nonhospitalists. Forty‐two percent (177/450) of patients were cared for by hospitalists. Compared to nonhospitalists, patients admitted to the hospitalist service were older (62.8 vs. 57.7 years, P < 0.01) and with third‐party payor mix differences (P < 0.01). However, there were no statistical differences between patients attended by hospitalists and nonhospitalists with regard to Complete Rockall risk score, Charlson comorbidity index, performance of early endoscopy, and mean hemoglobin values on admission. Upper endoscopy was performed in all patients with distribution of the 3 most common diagnoses being similar (P > 0.05) between hospitalists and nonhospitalists: erosive disease (49.7% vs. 54.6%), peptic ulcer disease (PUD) (48% vs. 46.9%), and varices (18.6% vs. 14.7%).
Variable | Admitting Service | P | |
---|---|---|---|
Hospitalist (n = 177) | Nonhospitalist (n = 273) | ||
| |||
Age, years (meanSD) | 62.817.4 | 57.718.5 | <0.01 |
Male sex, n (%) | 104 (58.8) | 169 (61.9) | 0.50 |
Ethnicity, n (%) | 0.13 | ||
White | 83 (46.9) | 102 (37.4) | |
African‐American | 34 (19.2) | 75 (27.5) | |
Hispanic | 21 (11.9) | 40 (14.7) | |
Asian/Pacific Islander | 24 (13.6) | 29 (10.6) | |
Others/unknown | 15 (8.5) | 27 (9.9) | |
Insurance, n (%) | <0.01 | ||
Medicare | 86 (48.6) | 104 (38.1) | |
Medicaid | 15 (8.5) | 33 (12.1) | |
No payer | 18 (10.2) | 36 (13.2) | |
Private | 46 (26) | 52 (19.1) | |
Unknown | 12 (6.8) | 48 (17.5) | |
Charlson Comorbidity Index (meanSD) | 1.91.6 | 1.81.7 | 0.51 |
Complete Rockall, n (%) | 0.11 | ||
Low‐risk (0‐2) | 82 (46.3) | 103 (37.7) | |
Moderate‐risk (3‐5) | 71 (40.1) | 137 (50.2) | |
High‐risk (6) | 24 (14.6) | 33 (12.1) | |
Early endoscopy (<24 hours) | 82 (46.3) | 133 (48.7) | 0.62 |
Endoscopic diagnosis, n (%)* | |||
Erosive disease | 88 (49.7) | 149 (54.6) | 0.31 |
Peptic ulcer disease | 85 (48.0) | 128 (46.9) | 0.81 |
Varices | 33 (18.6) | 40 (14.7) | 0.26 |
Mallory‐Weiss tear | 9 (5.1) | 21 (7.7) | 0.28 |
Angiodysplasia | 9 (5.1) | 13 (4.8) | 0.88 |
GI mass | 1 (0.6) | 4 (1.5) | 0.65 |
Normal | 7 (4.0) | 8 (2.9) | 0.55 |
Admission hemoglobin values (meanSD) | 10.22.9 | 10.22.9 | 0.78 |
Clinical Outcomes
Between hospitalists and nonhospitalists, unadjusted outcomes were similar (P > 0.05) for mortality (2.3% vs. 0.4%), recurrent bleeding (11% vs. 11%), need for endoscopic therapy (24% vs. 22%), ICU‐transfer and decompensation (15% vs. 15%), as well as an overall composite measure of any complication (79% vs. 72%) (Table 2). However, the hospitalist‐led teams performed more blood transfusions (74% vs. 63%, P = 0.02) and readmission rates were higher (7.3% vs. 3.3%, P = 0.05).
Outcomes, n (%) | Admitting Service | P | |
---|---|---|---|
Hospitalist (n = 177) | Nonhospitalist (n = 273) | ||
| |||
Inpatient mortality | 4 (2.3) | 1 (0.4) | 0.08 |
Recurrent bleeding* | 20 (11.3) | 29 (10.6) | 0.88 |
Endoscopic therapy | 43 (24.3) | 60 (22.0) | 0.57 |
ICU transfers | 23 (13) | 24 (8.8) | 0.20 |
Decompensated comorbidities that required continued hospitalization | 26 (14.7) | 41 (15.0) | 0.92 |
Any transfusion | 131 (74.0) | 172 (63.0) | 0.02 |
Total complications | 139 (78.5) | 196 (71.8) | 0.11 |
30‐day all‐cause readmissions | 13 (7.3) | 9 (3.3) | 0.05 |
Efficiency | Hospitalist (n = 164) | Nonhospitalist (n = 259) | P |
LOS, days | |||
MeanSD | 4.83.5 | 4.53.0 | 0.30 |
Median (interquartile range) | 4 (36) | 4 (26) | 0.69 |
Total costs, U.S. $ | |||
MeanSD | 10,466.669191.00 | 7926.716065.00 | <0.01 |
Median (interquartile range) | 7359.00 (4,698.0012,550.00) | 6181.00 (3744.0010,344.00) | <0.01 |
Because of the low event rate of certain adverse outcomes (<20%), we were only able to perform adjusted analyses on 4 outcomes: need for endoscopic therapy (odds ratio [OR], 0.82; 95% confidence interval [CI], 0.491.37), ICU transfer and decompensation (OR, 0.82; 95% CI, 0.451.52), blood transfusion (OR, 1.30; 95% CI, 0.822.04), and any complication (OR, 1.18; 95% CI, 0.711.96). Since outcome differences disappeared after controlling for confounders, the data suggest that overall care provided by hospitalists and nonhospitalists might be equivalenteven in certain outcomes that we were unable to substantiate using multivariable methods.
Efficiency
Efficiency, as measured by LOS and costs, are presented both as means and medians in univariate analyses in Table 2. Median LOS was similar for hospitalist‐led and nonhospitalist‐led teams (4 days). Despite having similar LOS, the median costs of acute UGIH in patients cared for by hospitalists were higher ($7,359.00 vs. $6,181.00; P < 0.01).
After adjusting for demographic factors, Rockall risk score, comorbidity, early EGD, and hospital site, LOS remained similar between the 2 groups. On the other hand, the adjusted cost for UGIH patients cared for by hospitalists and nonhospitalists persisted, with hospitalist care costs $1,502.40 more than their nonhospitalist counterparts (Table 3).
Efficiency | Treatment Provider | P | |
---|---|---|---|
Hospitalist (n = 164) | Nonhospitalist (n = 259) | ||
| |||
Adjusted length of stay, days (mean SD) | 5.2 (4.95.6) | 4.7 (4.55.0) | 0.15 |
Adjusted total cost, U.S. $ (mean SD) | 9006.50 (8366.609693.60) | 7504.10 (7069.907964.20) | 0.03 |
Discussion
This is the first study that has looked at the effect of hospitalists on clinical outcomes and efficiency in patients admitted for acute UGIH, a condition highly dependent upon another specialty for procedures and management. This is also one of only a few studies on UGIH that adjusted for severity of illness (Rockall score), comorbidity, performance of early endoscopypatient‐level confounders usually unaccounted for in prior research.
We show that hospitalists and nonhospitalists caring for acute UGIH patients had overall similar unadjusted outcomes; except for blood transfusion and 30‐day readmission rates. Unfortunately, due to the small number of events for readmissions, we were unable to perform adjusted analysis for readmission. Differences between hospitalists and nonhospitalists on blood transfusion rates were not substantiated on multivariable adjustments.
As for efficiency, univariable and multivariable analyses revealed that LOS was similar between provider types while costs were greater in UGIH patients attended by hospitalists.
Reductions in resource use, particularly costs, may be achieved by increasing throughput (eg, reducing LOS) or by decreasing service intensity (eg, using fewer ancillary services and specialty consultations).26 Specifically in acute UGIH, LOS is significantly affected by performance of early EGD.27, 28 In these studies, gastroenterologist‐led teams, compared to internists and surgeons, have easier access to endoscopy, thus reducing LOS and overall costs.27, 28
Similarly, prior studies have shown that the mechanism by which hospitalists lower costs is by decreasing LOS.810, 29 There are several hypotheses on how hospitalists affect LOS. Hospitalists, by being available all day, are thought to respond quickly to acute symptoms or new test results, are more efficient in navigating the complex hospital environment, or develop greater expertise as a result of added inpatient experience.8 On the downside, although the hospitalist model reduces overall LOS and costs, they also provide higher intensity of care as reflected by greater costs when broken down per hospital day.29 Thus, the cost differential we found may represent higher intensity of care by hospitalists in their management of acute UGIH, as higher intensity care without decreasing LOS can translate to higher costs.
In addition, patients with acute UGIH are unique in several respects. In contrast to diseases like heart failure, COPD, and pneumonia, in which the admitting provider has the option to request a subspecialist consultation, all patients with acute UGIH need a gastroenterologist to perform endoscopy as part of the management. These patients are usually admitted to general medicine wards, aggressively resuscitated with intravenous fluids, with a nonurgent gastroenterology consult or EGD performed on the next available schedule.
Aside from LOS being greatly affected by performance of early EGD and/or delay in consulting gastroenterology, sicker patients require longer hospitalization and drive LOS and healthcare costs up. It was therefore crucial that we accounted for severity of illness, comorbidity, and performance of early EGD in our regression models for LOS and costs. This approach allows us to acquire a more accurate estimate on the effects of hospitalist on LOS and costs in patients admitted with acute UGIH.
Our findings suggest that the academic hospitalist model of care may not have as great of an impact on hospital efficiency in certain patient groups that require nonurgent subspecialty consultations. Future studies should focus on elucidating these relationships.
Limitations
This study has several limitations. First, clinical data were abstracted at 6 sites by different abstractors so it is possible there were variations in how data were collected. To reduce variation, a standardized abstraction form with instructions was developed and the primary investigator (PI) was available for specific questions during the abstraction process. Second, only 5 out of the 6 sites used TSI accounting systems. Although similar, interhospital costs captured by TSI may vary among sites in terms of classifying direct and indirect costs, potentially resulting in misclassification bias in our cost estimates.17 We addressed these issues by including the hospital site variable in our regression models, regardless of its significance. Third, consent rates across sites vary from 70% to 85%. It is possible that patients who refused enrollment in the MCH trial are systematically different and may introduce bias in our analysis.
Furthermore, the study was designed as a natural experiment based on a rotational call cycle between hospitalist‐led and nonhospitalist‐led teams. It is possible that the order of patient assignment might not be completely naturally random as we intended. However, the study period was for 2 years and we expect the effect of order would have averaged out in time.
There are many hospitalist models of care. In terms of generalizability, the study pertains only to academic hospitalists and may not be applicable to hospitalists practicing in community hospitals. For example, the nonhospitalist comparison group is likely different in the community and academic settings. Community nonhospitalists (traditional practitioners) are usually internists covering both inpatient and outpatient responsibilities at the same time. In contrast, academic nonhospitalists are internists or subspecialists serving as ward attendings for a limited period (usually 1 month) with considerable variation in their nonattending responsibilities (eg, research, clinic, administration). Furthermore, academic nonhospitalist providers might be a self‐selected group by their willingness to serve as a ward attending, making them more hospitalist‐like. Changes and variability of inpatient attendings may also affect our findings when compared to prior work. Finally, it is also possible that having residents at academic medical centers may attenuate the effect of hospitalists more than in community‐based models.
Conclusions/Implications
Compared to nonhospitalists, academic hospitalist care of acute UGIH patients had similar overall clinical outcomes. However, our finding of similar LOS yet higher costs for patients cared for by hospitalists support 1 proposed mechanism in which hospitalists decrease healthcare costs: providing higher intensity of care per day of hospitalization. However, in academic hospitalist models, this higher intensity hypothesis should be revisited, especially in certain patient groups in which timing and involvement of subspecialists may influence discharge decisions, affecting LOS and overall costs.
Due to inherent limitations in this observational study, future studies should focus on verifying and elucidating these relationships further. Lastly, understanding which patient groups receive the greatest potential benefit from this model will help guide both organizational efforts and quality improvement strategies.
- Bleeding peptic ulcer.N Engl J Med.1994;331(11):717–727. , .
- Epidemiology of hospitalization for acute upper gastrointestinal hemorrhage: a population‐based study.Am J Gastroenterol.1995;90(2):206–210. .
- Variation in outcome after acute upper gastrointestinal haemorrhage. the national audit of acute upper gastrointestinal haemorrhage.Lancet.1995;346(8971):346–350. , , , et al.
- Influencing the practice and outcome in acute upper gastrointestinal haemorrhage. Steering committee of the National Audit of Acute Upper Gastrointestinal Haemorrhage.Gut.1997;41(5):606–611. , , , et al.
- Risk assessment after acute upper gastrointestinal haemorrhage.Gut.1996;38(3):316–321. , , , et al.
- The potential size of the hospitalist workforce in the united states.Am J Med.1999;106(4):441–445. , , , et al.
- Society of Hospital Medicine. About SHM. Available at: http://www.hospitalmedicine.org/AM/Template.cfm?Section=General_Information357(25):2589–2600.
- Effects of physician experience on costs and outcomes on an academic general medicine service: results of a trial of hospitalists.Ann Intern Med.2002;137(11):866–874. , , , et al.
- A systematic review of outcomes and quality measures in adult patients cared for by hospitalists vs nonhospitalists.Mayo Clin Proc.2009;84(3):248–254. .
- Do hospitalists or physicians with greater inpatient HIV experience improve HIV care in the era of highly active antiretroviral therapy? Results from a multicenter trial of academic hospitalists.Clin Infect Dis.2008;46(7):1085–1092. , , , et al.
- Quality of care for decompensated heart failure: comparable performance between academic hospitalists and non‐hospitalists. J Gen Intern Med.2008;23(9):1399–1406. , , , et al.
- Factors associated with discussion of care plans and code status at the time of hospital admission: results from the Multicenter Hospitalist Study.J Hosp Med.2008;3(6):437–445. , , , et al.
- Upper gastrointestinal hemorrhage clinical guideline determining the optimal hospital length of stay.Am J Med.1996;100(3):313–322. , , , et al.
- Prospective evaluation of a clinical guideline recommending hospital length of stay in upper gastrointestinal tract hemorrhage.JAMA.1997;278(24):2151–2156. , , , et al.
- In‐hospital cost of abdominal aortic aneurysm repair in Canada and the United States.Arch Intern Med.2003;163(20):2500–2504. , , , et al.
- The use of transition cost accounting system in health services research.Cost Eff Resour Alloc.2007;5:11. , , , et al.
- Society of Hospital Medicine. Definition of a Hospitalist. Available at: http://www.hospitalmedicine.org/AM/Template.cfm?Section=General_Information335(7):514–517.
- Selection of patients for early discharge or outpatient care after acute upper gastrointestinal haemorrhage. National Audit of Acute Upper Gastrointestinal Haemorrhage.Lancet.1996;347(9009):1138–1140. , , , et al.
- Utilization of health care resources for low‐risk patients with acute, nonvariceal upper GI hemorrhage: an historical cohort study.Gastrointest Endosc.2002;55(3):321–327. , , , et al.
- Incremental value of upper endoscopy for triage of patients with acute non‐variceal upper‐GI hemorrhage.Gastrointest Endosc.2004;60(1):9–14. , .
- The Charlson comorbidity index is adapted to predict costs of chronic disease in primary care patients.J Clin Epidemiol.2008;61(12):1234–1240. , , , et al.
- The effectiveness of early endoscopy for upper gastrointestinal hemorrhage: a community‐based analysis.Med Care.1998;36(4):462–474. , , , et al.
- Early endoscopy in upper gastrointestinal hemorrhage: associations with recurrent bleeding, surgery, and length of hospital stay.Gastrointest Endosc.1999;49(2):145–152. , , , et al.
- The impact of hospitalists on the cost and quality of inpatient care in the united states: a research synthesis.Med Care Res Rev.2005;62(4):379–406. , .
- Physician specialty and variations in the cost of treating patients with acute upper gastrointestinal bleeding.Gastroenterology.1997;113(5):1443–1448. , , , et al.
- Impact of physician specialty on the cost of nonvariceal upper GI bleeding care.Am J Gastroenterol.2002;97(6):1535–1542. , , , et al.
- Associations with reduced length of stay and costs on an academic hospitalist service.Am J Manag Care.2004;10(8):561–568. , , .
- Bleeding peptic ulcer.N Engl J Med.1994;331(11):717–727. , .
- Epidemiology of hospitalization for acute upper gastrointestinal hemorrhage: a population‐based study.Am J Gastroenterol.1995;90(2):206–210. .
- Variation in outcome after acute upper gastrointestinal haemorrhage. the national audit of acute upper gastrointestinal haemorrhage.Lancet.1995;346(8971):346–350. , , , et al.
- Influencing the practice and outcome in acute upper gastrointestinal haemorrhage. Steering committee of the National Audit of Acute Upper Gastrointestinal Haemorrhage.Gut.1997;41(5):606–611. , , , et al.
- Risk assessment after acute upper gastrointestinal haemorrhage.Gut.1996;38(3):316–321. , , , et al.
- The potential size of the hospitalist workforce in the united states.Am J Med.1999;106(4):441–445. , , , et al.
- Society of Hospital Medicine. About SHM. Available at: http://www.hospitalmedicine.org/AM/Template.cfm?Section=General_Information357(25):2589–2600.
- Effects of physician experience on costs and outcomes on an academic general medicine service: results of a trial of hospitalists.Ann Intern Med.2002;137(11):866–874. , , , et al.
- A systematic review of outcomes and quality measures in adult patients cared for by hospitalists vs nonhospitalists.Mayo Clin Proc.2009;84(3):248–254. .
- Do hospitalists or physicians with greater inpatient HIV experience improve HIV care in the era of highly active antiretroviral therapy? Results from a multicenter trial of academic hospitalists.Clin Infect Dis.2008;46(7):1085–1092. , , , et al.
- Quality of care for decompensated heart failure: comparable performance between academic hospitalists and non‐hospitalists. J Gen Intern Med.2008;23(9):1399–1406. , , , et al.
- Factors associated with discussion of care plans and code status at the time of hospital admission: results from the Multicenter Hospitalist Study.J Hosp Med.2008;3(6):437–445. , , , et al.
- Upper gastrointestinal hemorrhage clinical guideline determining the optimal hospital length of stay.Am J Med.1996;100(3):313–322. , , , et al.
- Prospective evaluation of a clinical guideline recommending hospital length of stay in upper gastrointestinal tract hemorrhage.JAMA.1997;278(24):2151–2156. , , , et al.
- In‐hospital cost of abdominal aortic aneurysm repair in Canada and the United States.Arch Intern Med.2003;163(20):2500–2504. , , , et al.
- The use of transition cost accounting system in health services research.Cost Eff Resour Alloc.2007;5:11. , , , et al.
- Society of Hospital Medicine. Definition of a Hospitalist. Available at: http://www.hospitalmedicine.org/AM/Template.cfm?Section=General_Information335(7):514–517.
- Selection of patients for early discharge or outpatient care after acute upper gastrointestinal haemorrhage. National Audit of Acute Upper Gastrointestinal Haemorrhage.Lancet.1996;347(9009):1138–1140. , , , et al.
- Utilization of health care resources for low‐risk patients with acute, nonvariceal upper GI hemorrhage: an historical cohort study.Gastrointest Endosc.2002;55(3):321–327. , , , et al.
- Incremental value of upper endoscopy for triage of patients with acute non‐variceal upper‐GI hemorrhage.Gastrointest Endosc.2004;60(1):9–14. , .
- The Charlson comorbidity index is adapted to predict costs of chronic disease in primary care patients.J Clin Epidemiol.2008;61(12):1234–1240. , , , et al.
- The effectiveness of early endoscopy for upper gastrointestinal hemorrhage: a community‐based analysis.Med Care.1998;36(4):462–474. , , , et al.
- Early endoscopy in upper gastrointestinal hemorrhage: associations with recurrent bleeding, surgery, and length of hospital stay.Gastrointest Endosc.1999;49(2):145–152. , , , et al.
- The impact of hospitalists on the cost and quality of inpatient care in the united states: a research synthesis.Med Care Res Rev.2005;62(4):379–406. , .
- Physician specialty and variations in the cost of treating patients with acute upper gastrointestinal bleeding.Gastroenterology.1997;113(5):1443–1448. , , , et al.
- Impact of physician specialty on the cost of nonvariceal upper GI bleeding care.Am J Gastroenterol.2002;97(6):1535–1542. , , , et al.
- Associations with reduced length of stay and costs on an academic hospitalist service.Am J Manag Care.2004;10(8):561–568. , , .
Copyright © 2010 Society of Hospital Medicine