User login
Hospital Ward Adaptation During the COVID-19 Pandemic: A National Survey of Academic Medical Centers
The coronavirus disease of 2019 (COVID-19) pandemic has resulted in a surge in hospitalizations of patients with a novel, serious, and highly contagious infectious disease for which there is yet no proven treatment. Currently, much of the focus has been on intensive care unit (ICU) and ventilator capacity for the sickest of these patients who develop respiratory failure. However, most hospitalized patients are being cared for in general medical units.1 Some evidence exists to describe adaptations to capacity needs outside of medical wards,2-4 but few studies have specifically addressed the ward setting. Therefore, there is a pressing need for evidence to describe how to expand capacity and deliver medical ward–based care.
To better understand how inpatient care in the United States is adapting to the COVID-19 pandemic, we surveyed 72 sites participating in the Hospital Medicine Reengineering Network (HOMERuN), a national consortium of hospital medicine groups.5 We report results of this survey, carried out between April 3 and April 5, 2020.
METHODS
Sites and Subjects
HOMERuN is a collaborative network of hospitalists from across the United States whose primary goal is to catalyze research and share best practices across hospital medicine groups. Using surveys of Hospital Medicine leaders, targeted medical record review, and other methods, HOMERuN’s funded research interests to date have included care transitions, workforce issues, patient and family engagement, and diagnostic errors. Sites participating in HOMERuN sites are relatively large urban academic medical centers (Appendix).
Survey Development and Deployment
We designed a focused survey that aimed to provide a snapshot of evolving operational and clinical aspects of COVID-19 care (Appendix). Domains included COVID-19 testing turnaround times, personal protective equipment (PPE) stewardship,6 features of respiratory isolation units (RIUs; ie, dedicated units for patients with known or suspected COVID-19), and observed effects on clinical care. We tested the instrument to ensure feasibility and clarity internally, performed brief cognitive testing with several hospital medicine leaders in HOMERuN, then disseminated the survey by email on April 3, with two follow-up emails on 2 subsequent days. Our study was deemed non–human subjects research by the University of California, San Francisco, Committee on Human Research. Descriptive statistics were used to characterize survey responses.
RESULTS
Of 72 hospitals surveyed, 51 (71%) responded. Mean hospital bed count was 940, three were safety-net hospitals, and one was a community-based teaching center; responding and nonresponding hospitals did not differ significantly in terms of bed count (Appendix).
Health System Adaptations, Testing, and PPE Status
Nearly all responding hospitals (46 of 51; 90%) had RIUs for patients with known or suspected COVID-19 (Table 1). Nearly all hospitals took steps to keep potentially sick healthcare providers from infecting others (eg, staying home if sick or exposed). Among respondents, 32% had rapid response teams, 24% had respiratory therapy teams, and 29% had case management teams that were dedicated to COVID-19 care. Thirty-two (63%) had developed models, such as ethics or palliative care consult services, to assist with difficult resource-allocation decisions (eg, how to prioritize ventilator use if demand exceeded supply). Twenty-three (45%) had developed post-acute care monitoring programs dedicated to COVID-19 patients.
At the time of our survey, only 2 sites (4%) reported COVID-19 test time turnaround under 1 hour, and 15 (30%) reported turnaround in less than 6 hours. Of the 29 sites able to provide estimates of PPE stockpile, 14 (48%) reported a supply of 2 weeks or less. The most common approaches to PPE stewardship focused on reuse of masks and face shields if not obviously soiled, centralizing PPE distribution, and disinfecting or sterilizing masks. Ten sites (20%) were utilizing 3-D printed masks, while 10% used homemade face shields or masks.
Characteristics of COVID-19 RIUs
Forty-six hospitals (90% of all respondents) in our cohort had developed RIUs at the time of survey administration. The earliest RIU implementation date was February 10, 2020, and the most recent was launched on the day of our survey. Admission to RIUs was primarily based on clinical factors associated with known or suspected COVID-19 infection (Table 2). The number of non–critical care RIU beds among locations at that time ranged from 10 or less to more than 50. The mean number of hospitalist attendings caring for patients in the RIUs was 10.2, with a mean 4.1 advanced practice providers, 5.5 residents, and 0 medical students. The number of planned patients per attending was typically 5 to 15. Nurses and physicians typically rounded separately. Medical distancing (eg, reducing patient room entry) was accomplished most commonly by grouped timing of medication administration (76% of sites), video links to room outside of rounding times (54% of sites), the use of video or telemedicine during rounds (17%), and clustering of activities such as medication administration or phlebotomy. The most common criteria prompting discharge from the RIU were a negative COVID-19 test (59%) and hospital discharge (57%), though comments from many respondents suggested that discharge criteria were changing rapidly.
Effects of Isolation Measures on In-Room Encounters and Diagnostic Processes
More than 90% of sites reported decreases in in-room encounter frequency across all provider types whether as a result of policies in place or not. Reductions were reported among hospitalists, advanced practice providers, residents, consultants, and therapists (Table 3). Reduced room entry most often resulted from an established or developing policy, but many noted reduced room entry without formal policies in place. Nearly all sites reported moving specialty consultations to phone or video evaluations. Diagnostic error was commonly reported, with missed non–COVID-19 medical diagnoses among COVID-19 infected patients being reported by 22 sites (46%) and missed COVID-19 diagnoses in patients admitted for other reasons by 22 sites (45%).
DISCUSSION
In this study of medical wards at academic medical centers, we found that, in response to the COVID-19 pandemic, hospitals made several changes in a short period of time to adapt to the crisis. These included implementation and rapid expansion of dedicated RIUs, greatly expanded use of inpatient telehealth for patient assessments and consultation, implementation of other approaches to minimize room entry (such as grouping in-room activities), and deployment of ethics consultation services to help manage issues around potential scarcity of life-saving measures such as ventilators. We also found that availability of PPE and timely testing was limited. Finally, a large proportion of sites reported potential diagnostic problems in the assessment of both patients suspected and those not suspected of having COVID-19.
RIUs are emerging as a primary modality for caring for non-ICU COVID-19 patients, though they never involved medical students; we hope the role of students in particular will increase as new models of training emerge in response to the pandemic.7 In contrast, telemedicine evolved rapidly to hold a substantial role in RIUs, with both ward and specialty teams using video visit technology to communicate with patients. COVID-19 has been viewed as a perfect use case for outpatient telemedicine,8 and a growing number of studies are examining its outpatient use9,10; however, to date, somewhat less attention has been paid to inpatient deployment. Although our data suggest telemedicine has found a prominent place in RIUs, it remains to be seen whether it is associated with differences in patient or provider outcomes. For example, deficiencies in the physical examination, limited face-to-face contact, and lack of physical presence could all affect the patient–provider relationship, patient engagement, and the accuracy of the diagnostic process.
Our data suggest the possibility of missing non–COVID-19 diagnoses in patients suspected of COVID-19 and missing COVID-19 in those admitted for nonrespiratory reasons. The latter may be addressed as routine COVID-19 screening of admitted patients becomes commonplace. For the former, however, it is possible that physicians are “anchoring” their thinking on COVID-19 to the exclusion of other diagnoses, that physicians are not fully aware of complications unique to COVID-19 infection (such as thromboembolism), and/or that the above-mentioned limitations of telemedicine have decreased diagnostic performance.
Although PPE stockpile data were not easily available for some sites, a distressingly large number reported stockpiles of 2 weeks or less, with reuse being the most common approach to extending PPE supply. We also found it concerning that 43% of hospital leaders did not know their stockpile data; we believe this is an important question that hospital leaders need to be asking. Most sites in our study reported test turnaround times of longer than 6 hours; lack of rapid COVID-19 testing further stresses PPE stockpile and may slow patients’ transition out of the RIU or discharge to home.
Our study has several limitations, including the evolving nature of the pandemic and rapid adaptations of care systems in the pandemic’s surge phase. However, we attempted to frame our questions in ways that provided a focused snapshot of care. Furthermore, respondents may not have had exhaustive knowledge of their institution’s COVID-19 response strategies, but most were the directors of their hospitalist services, and we encouraged the respondents to confer with others to gather high-fidelity data. Finally, as a survey of large academic medical centers, our results may not apply to nonacademic centers.
Approaches to caring for non-ICU patients during the COVID-19 pandemic are rapidly evolving. Expansion of RIUs and developing the workforce to support them has been a primary focus, with rapid innovation in use of technology emerging as a critical adaptation while PPE limitations persist and needs for “medical distancing” continue to grow. Although rates of missed COVID-19 diagnoses will likely be reduced with testing and systems improvements, physicians and systems will also need to consider how to utilize emerging technology in ways that can improve clinical care and provider safety while aiding diagnostic thinking. This survey illustrates the rapid adaptations made by our hospitals in response to the pandemic; ongoing adaptation will likely be needed to optimally care for hospitalized patients with COVID-19 while the pandemic continues to evolve.
Acknowledgment
Thanks to members of the HOMERuN COVID-19 Collaborative Group: Baylor Scott & White Medical Center – Temple, Texas - Tresa McNeal MD; Beth Israel Deaconess Medical Center - Shani Herzig MD MPH, Joseph Li MD, Julius Yang MD PhD; Brigham and Women’s Hospital - Christopher Roy MD, Jeffrey Schnipper MD MPH; Cedars-Sinai Medical Center - Ed Seferian MD, ; ChristianaCare - Surekha Bhamidipati MD; Cleveland Clinic - Matthew Pappas MD MPH; Dartmouth-Hitchcock Medical Center - Jonathan Lurie MD MS; Dell Medical School at The University of Texas at Austin - Chris Moriates MD, Luci Leykum MD MBA MSc; Denver Health and Hospitals Authority - Diana Mancini MD; Emory University Hospital - Dan Hunt MD; Johns Hopkins Hospital - Daniel J Brotman MD, Zishan K Siddiqui MD, Shaker Eid MD MBA; Maine Medical Center - Daniel A Meyer MD, Robert Trowbridge MD; Massachusetts General Hospital - Melissa Mattison MD; Mayo Clinic Rochester – Caroline Burton MD, Sagar Dugani MD PhD; Medical College of Wisconsin - Sanjay Bhandari MD; Miriam Hospital - Kwame Dapaah-Afriyie MD MBA; Mount Sinai Hospital - Andrew Dunn MD; NorthShore - David Lovinger MD; Northwestern Memorial Hospital - Kevin O’Leary MD MS; Ohio State University Wexner Medical Center - Eric Schumacher DO; Oregon Health & Science University - Angela Alday MD; Penn Medicine - Ryan Greysen MD MHS MA; Rutgers- Robert Wood Johnson University Hospital - Michael Steinberg MD MPH; Stanford University School of Medicine - Neera Ahuja MD; Tulane Hospital and University Medical Center - Geraldine Ménard MD; UC San Diego Health - Ian Jenkins MD; UC Los Angeles Health - Michael Lazarus MD, Magdalena E. Ptaszny, MD; UC San Francisco Health - Bradley A Sharpe, MD, Margaret Fang MD MPH; UK HealthCare - Mark Williams MD MHM, John Romond MD; University of Chicago – David Meltzer MD PhD, Gregory Ruhnke MD; University of Colorado - Marisha Burden MD; University of Florida - Nila Radhakrishnan MD; University of Iowa Hospitals and Clinics - Kevin Glenn MD MS; University of Miami - Efren Manjarrez MD; University of Michigan - Vineet Chopra MD MSc, Valerie Vaughn MD MSc; University of Missouri-Columbia Hospital - Hasan Naqvi MD; University of Nebraska Medical Center - Chad Vokoun MD; University of North Carolina at Chapel Hill - David Hemsey MD; University of Pittsburgh Medical Center - Gena Marie Walker MD; University of Vermont Medical Center - Steven Grant MD; University of Washington Medical Center - Christopher Kim MD MBA, Andrew White MD; University of Washington-Harborview Medical Center - Maralyssa Bann MD; University of Wisconsin Hospital and Clinics - David Sterken MD, Farah Kaiksow MD MPP, Ann Sheehy MD MS, Jordan Kenik MD MPH; UW Northwest Campus - Ben Wolpaw MD; Vanderbilt University Medical Center - Sunil Kripalani MD MSc, Eduard E Vasilevskis MD, Kathleene T Wooldridge MD MPH; Wake Forest Baptist Health - Erik Summers MD; Washington University St. Louis - Michael Lin MD; Weill Cornell - Justin Choi MD; Yale New Haven Hospital - William Cushing MA, Chris Sankey MD; Zuckerberg San Francisco General Hospital - Sumant Ranji MD.
1. Institute for Health Metrics and Evaluation. COVID-19 Projections: United States of America. 2020. Accessed May 5, 2020. https://covid19.healthdata.org/united-states-of-america
2. Iserson KV. Alternative care sites: an option in disasters. West J Emerg Med. 2020;21(3):484‐489. https://doi.org/10.5811/westjem.2020.4.47552
3. Paganini M, Conti A, Weinstein E, Della Corte F, Ragazzoni L. Translating COVID-19 pandemic surge theory to practice in the emergency department: how to expand structure [online first]. Disaster Med Public Health Prep. 2020:1-10. https://doi.org/10.1017/dmp.2020.57
4. Kumaraiah D, Yip N, Ivascu N, Hill L. Innovative ICU Physician Care Models: Covid-19 Pandemic at NewYork-Presbyterian. NEJM: Catalyst. April 28, 2020. Accessed May 5, 2020. https://catalyst.nejm.org/doi/full/10.1056/CAT.20.0158
5. Auerbach AD, Patel MS, Metlay JP, et al. The Hospital Medicine Reengineering Network (HOMERuN): a learning organization focused on improving hospital care. Acad Med. 2014;89(3):415-420. https://doi.org/10.1097/acm.0000000000000139
6. Livingston E, Desai A, Berkwits M. Sourcing personal protective equipment during the COVID-19 pandemic [online first]. JAMA. 2020. https://doi.org/10.1001/jama.2020.5317
7. Bauchner H, Sharfstein J. A bold response to the COVID-19 pandemic: medical students, national service, and public health [online first]. JAMA. 2020. https://doi.org/10.1001/jama.2020.6166
8. Hollander JE, Carr BG. Virtually perfect? telemedicine for Covid-19. N Engl J Med. 2020;382(18):1679‐1681. https://doi.org/10.1056/nejmp2003539
9. Hau YS, Kim JK, Hur J, Chang MC. How about actively using telemedicine during the COVID-19 pandemic? J Med Syst. 2020;44(6):108. https://doi.org/10.1007/s10916-020-01580-z
10. Smith WR, Atala AJ, Terlecki RP, Kelly EE, Matthews CA. Implementation guide for rapid integration of an outpatient telemedicine program during the COVID-19 pandemic [online first]. J Am Coll Surg. 2020. https://doi.org/10.1016/j.jamcollsurg.2020.04.030
The coronavirus disease of 2019 (COVID-19) pandemic has resulted in a surge in hospitalizations of patients with a novel, serious, and highly contagious infectious disease for which there is yet no proven treatment. Currently, much of the focus has been on intensive care unit (ICU) and ventilator capacity for the sickest of these patients who develop respiratory failure. However, most hospitalized patients are being cared for in general medical units.1 Some evidence exists to describe adaptations to capacity needs outside of medical wards,2-4 but few studies have specifically addressed the ward setting. Therefore, there is a pressing need for evidence to describe how to expand capacity and deliver medical ward–based care.
To better understand how inpatient care in the United States is adapting to the COVID-19 pandemic, we surveyed 72 sites participating in the Hospital Medicine Reengineering Network (HOMERuN), a national consortium of hospital medicine groups.5 We report results of this survey, carried out between April 3 and April 5, 2020.
METHODS
Sites and Subjects
HOMERuN is a collaborative network of hospitalists from across the United States whose primary goal is to catalyze research and share best practices across hospital medicine groups. Using surveys of Hospital Medicine leaders, targeted medical record review, and other methods, HOMERuN’s funded research interests to date have included care transitions, workforce issues, patient and family engagement, and diagnostic errors. Sites participating in HOMERuN sites are relatively large urban academic medical centers (Appendix).
Survey Development and Deployment
We designed a focused survey that aimed to provide a snapshot of evolving operational and clinical aspects of COVID-19 care (Appendix). Domains included COVID-19 testing turnaround times, personal protective equipment (PPE) stewardship,6 features of respiratory isolation units (RIUs; ie, dedicated units for patients with known or suspected COVID-19), and observed effects on clinical care. We tested the instrument to ensure feasibility and clarity internally, performed brief cognitive testing with several hospital medicine leaders in HOMERuN, then disseminated the survey by email on April 3, with two follow-up emails on 2 subsequent days. Our study was deemed non–human subjects research by the University of California, San Francisco, Committee on Human Research. Descriptive statistics were used to characterize survey responses.
RESULTS
Of 72 hospitals surveyed, 51 (71%) responded. Mean hospital bed count was 940, three were safety-net hospitals, and one was a community-based teaching center; responding and nonresponding hospitals did not differ significantly in terms of bed count (Appendix).
Health System Adaptations, Testing, and PPE Status
Nearly all responding hospitals (46 of 51; 90%) had RIUs for patients with known or suspected COVID-19 (Table 1). Nearly all hospitals took steps to keep potentially sick healthcare providers from infecting others (eg, staying home if sick or exposed). Among respondents, 32% had rapid response teams, 24% had respiratory therapy teams, and 29% had case management teams that were dedicated to COVID-19 care. Thirty-two (63%) had developed models, such as ethics or palliative care consult services, to assist with difficult resource-allocation decisions (eg, how to prioritize ventilator use if demand exceeded supply). Twenty-three (45%) had developed post-acute care monitoring programs dedicated to COVID-19 patients.
At the time of our survey, only 2 sites (4%) reported COVID-19 test time turnaround under 1 hour, and 15 (30%) reported turnaround in less than 6 hours. Of the 29 sites able to provide estimates of PPE stockpile, 14 (48%) reported a supply of 2 weeks or less. The most common approaches to PPE stewardship focused on reuse of masks and face shields if not obviously soiled, centralizing PPE distribution, and disinfecting or sterilizing masks. Ten sites (20%) were utilizing 3-D printed masks, while 10% used homemade face shields or masks.
Characteristics of COVID-19 RIUs
Forty-six hospitals (90% of all respondents) in our cohort had developed RIUs at the time of survey administration. The earliest RIU implementation date was February 10, 2020, and the most recent was launched on the day of our survey. Admission to RIUs was primarily based on clinical factors associated with known or suspected COVID-19 infection (Table 2). The number of non–critical care RIU beds among locations at that time ranged from 10 or less to more than 50. The mean number of hospitalist attendings caring for patients in the RIUs was 10.2, with a mean 4.1 advanced practice providers, 5.5 residents, and 0 medical students. The number of planned patients per attending was typically 5 to 15. Nurses and physicians typically rounded separately. Medical distancing (eg, reducing patient room entry) was accomplished most commonly by grouped timing of medication administration (76% of sites), video links to room outside of rounding times (54% of sites), the use of video or telemedicine during rounds (17%), and clustering of activities such as medication administration or phlebotomy. The most common criteria prompting discharge from the RIU were a negative COVID-19 test (59%) and hospital discharge (57%), though comments from many respondents suggested that discharge criteria were changing rapidly.
Effects of Isolation Measures on In-Room Encounters and Diagnostic Processes
More than 90% of sites reported decreases in in-room encounter frequency across all provider types whether as a result of policies in place or not. Reductions were reported among hospitalists, advanced practice providers, residents, consultants, and therapists (Table 3). Reduced room entry most often resulted from an established or developing policy, but many noted reduced room entry without formal policies in place. Nearly all sites reported moving specialty consultations to phone or video evaluations. Diagnostic error was commonly reported, with missed non–COVID-19 medical diagnoses among COVID-19 infected patients being reported by 22 sites (46%) and missed COVID-19 diagnoses in patients admitted for other reasons by 22 sites (45%).
DISCUSSION
In this study of medical wards at academic medical centers, we found that, in response to the COVID-19 pandemic, hospitals made several changes in a short period of time to adapt to the crisis. These included implementation and rapid expansion of dedicated RIUs, greatly expanded use of inpatient telehealth for patient assessments and consultation, implementation of other approaches to minimize room entry (such as grouping in-room activities), and deployment of ethics consultation services to help manage issues around potential scarcity of life-saving measures such as ventilators. We also found that availability of PPE and timely testing was limited. Finally, a large proportion of sites reported potential diagnostic problems in the assessment of both patients suspected and those not suspected of having COVID-19.
RIUs are emerging as a primary modality for caring for non-ICU COVID-19 patients, though they never involved medical students; we hope the role of students in particular will increase as new models of training emerge in response to the pandemic.7 In contrast, telemedicine evolved rapidly to hold a substantial role in RIUs, with both ward and specialty teams using video visit technology to communicate with patients. COVID-19 has been viewed as a perfect use case for outpatient telemedicine,8 and a growing number of studies are examining its outpatient use9,10; however, to date, somewhat less attention has been paid to inpatient deployment. Although our data suggest telemedicine has found a prominent place in RIUs, it remains to be seen whether it is associated with differences in patient or provider outcomes. For example, deficiencies in the physical examination, limited face-to-face contact, and lack of physical presence could all affect the patient–provider relationship, patient engagement, and the accuracy of the diagnostic process.
Our data suggest the possibility of missing non–COVID-19 diagnoses in patients suspected of COVID-19 and missing COVID-19 in those admitted for nonrespiratory reasons. The latter may be addressed as routine COVID-19 screening of admitted patients becomes commonplace. For the former, however, it is possible that physicians are “anchoring” their thinking on COVID-19 to the exclusion of other diagnoses, that physicians are not fully aware of complications unique to COVID-19 infection (such as thromboembolism), and/or that the above-mentioned limitations of telemedicine have decreased diagnostic performance.
Although PPE stockpile data were not easily available for some sites, a distressingly large number reported stockpiles of 2 weeks or less, with reuse being the most common approach to extending PPE supply. We also found it concerning that 43% of hospital leaders did not know their stockpile data; we believe this is an important question that hospital leaders need to be asking. Most sites in our study reported test turnaround times of longer than 6 hours; lack of rapid COVID-19 testing further stresses PPE stockpile and may slow patients’ transition out of the RIU or discharge to home.
Our study has several limitations, including the evolving nature of the pandemic and rapid adaptations of care systems in the pandemic’s surge phase. However, we attempted to frame our questions in ways that provided a focused snapshot of care. Furthermore, respondents may not have had exhaustive knowledge of their institution’s COVID-19 response strategies, but most were the directors of their hospitalist services, and we encouraged the respondents to confer with others to gather high-fidelity data. Finally, as a survey of large academic medical centers, our results may not apply to nonacademic centers.
Approaches to caring for non-ICU patients during the COVID-19 pandemic are rapidly evolving. Expansion of RIUs and developing the workforce to support them has been a primary focus, with rapid innovation in use of technology emerging as a critical adaptation while PPE limitations persist and needs for “medical distancing” continue to grow. Although rates of missed COVID-19 diagnoses will likely be reduced with testing and systems improvements, physicians and systems will also need to consider how to utilize emerging technology in ways that can improve clinical care and provider safety while aiding diagnostic thinking. This survey illustrates the rapid adaptations made by our hospitals in response to the pandemic; ongoing adaptation will likely be needed to optimally care for hospitalized patients with COVID-19 while the pandemic continues to evolve.
Acknowledgment
Thanks to members of the HOMERuN COVID-19 Collaborative Group: Baylor Scott & White Medical Center – Temple, Texas - Tresa McNeal MD; Beth Israel Deaconess Medical Center - Shani Herzig MD MPH, Joseph Li MD, Julius Yang MD PhD; Brigham and Women’s Hospital - Christopher Roy MD, Jeffrey Schnipper MD MPH; Cedars-Sinai Medical Center - Ed Seferian MD, ; ChristianaCare - Surekha Bhamidipati MD; Cleveland Clinic - Matthew Pappas MD MPH; Dartmouth-Hitchcock Medical Center - Jonathan Lurie MD MS; Dell Medical School at The University of Texas at Austin - Chris Moriates MD, Luci Leykum MD MBA MSc; Denver Health and Hospitals Authority - Diana Mancini MD; Emory University Hospital - Dan Hunt MD; Johns Hopkins Hospital - Daniel J Brotman MD, Zishan K Siddiqui MD, Shaker Eid MD MBA; Maine Medical Center - Daniel A Meyer MD, Robert Trowbridge MD; Massachusetts General Hospital - Melissa Mattison MD; Mayo Clinic Rochester – Caroline Burton MD, Sagar Dugani MD PhD; Medical College of Wisconsin - Sanjay Bhandari MD; Miriam Hospital - Kwame Dapaah-Afriyie MD MBA; Mount Sinai Hospital - Andrew Dunn MD; NorthShore - David Lovinger MD; Northwestern Memorial Hospital - Kevin O’Leary MD MS; Ohio State University Wexner Medical Center - Eric Schumacher DO; Oregon Health & Science University - Angela Alday MD; Penn Medicine - Ryan Greysen MD MHS MA; Rutgers- Robert Wood Johnson University Hospital - Michael Steinberg MD MPH; Stanford University School of Medicine - Neera Ahuja MD; Tulane Hospital and University Medical Center - Geraldine Ménard MD; UC San Diego Health - Ian Jenkins MD; UC Los Angeles Health - Michael Lazarus MD, Magdalena E. Ptaszny, MD; UC San Francisco Health - Bradley A Sharpe, MD, Margaret Fang MD MPH; UK HealthCare - Mark Williams MD MHM, John Romond MD; University of Chicago – David Meltzer MD PhD, Gregory Ruhnke MD; University of Colorado - Marisha Burden MD; University of Florida - Nila Radhakrishnan MD; University of Iowa Hospitals and Clinics - Kevin Glenn MD MS; University of Miami - Efren Manjarrez MD; University of Michigan - Vineet Chopra MD MSc, Valerie Vaughn MD MSc; University of Missouri-Columbia Hospital - Hasan Naqvi MD; University of Nebraska Medical Center - Chad Vokoun MD; University of North Carolina at Chapel Hill - David Hemsey MD; University of Pittsburgh Medical Center - Gena Marie Walker MD; University of Vermont Medical Center - Steven Grant MD; University of Washington Medical Center - Christopher Kim MD MBA, Andrew White MD; University of Washington-Harborview Medical Center - Maralyssa Bann MD; University of Wisconsin Hospital and Clinics - David Sterken MD, Farah Kaiksow MD MPP, Ann Sheehy MD MS, Jordan Kenik MD MPH; UW Northwest Campus - Ben Wolpaw MD; Vanderbilt University Medical Center - Sunil Kripalani MD MSc, Eduard E Vasilevskis MD, Kathleene T Wooldridge MD MPH; Wake Forest Baptist Health - Erik Summers MD; Washington University St. Louis - Michael Lin MD; Weill Cornell - Justin Choi MD; Yale New Haven Hospital - William Cushing MA, Chris Sankey MD; Zuckerberg San Francisco General Hospital - Sumant Ranji MD.
The coronavirus disease of 2019 (COVID-19) pandemic has resulted in a surge in hospitalizations of patients with a novel, serious, and highly contagious infectious disease for which there is yet no proven treatment. Currently, much of the focus has been on intensive care unit (ICU) and ventilator capacity for the sickest of these patients who develop respiratory failure. However, most hospitalized patients are being cared for in general medical units.1 Some evidence exists to describe adaptations to capacity needs outside of medical wards,2-4 but few studies have specifically addressed the ward setting. Therefore, there is a pressing need for evidence to describe how to expand capacity and deliver medical ward–based care.
To better understand how inpatient care in the United States is adapting to the COVID-19 pandemic, we surveyed 72 sites participating in the Hospital Medicine Reengineering Network (HOMERuN), a national consortium of hospital medicine groups.5 We report results of this survey, carried out between April 3 and April 5, 2020.
METHODS
Sites and Subjects
HOMERuN is a collaborative network of hospitalists from across the United States whose primary goal is to catalyze research and share best practices across hospital medicine groups. Using surveys of Hospital Medicine leaders, targeted medical record review, and other methods, HOMERuN’s funded research interests to date have included care transitions, workforce issues, patient and family engagement, and diagnostic errors. Sites participating in HOMERuN sites are relatively large urban academic medical centers (Appendix).
Survey Development and Deployment
We designed a focused survey that aimed to provide a snapshot of evolving operational and clinical aspects of COVID-19 care (Appendix). Domains included COVID-19 testing turnaround times, personal protective equipment (PPE) stewardship,6 features of respiratory isolation units (RIUs; ie, dedicated units for patients with known or suspected COVID-19), and observed effects on clinical care. We tested the instrument to ensure feasibility and clarity internally, performed brief cognitive testing with several hospital medicine leaders in HOMERuN, then disseminated the survey by email on April 3, with two follow-up emails on 2 subsequent days. Our study was deemed non–human subjects research by the University of California, San Francisco, Committee on Human Research. Descriptive statistics were used to characterize survey responses.
RESULTS
Of 72 hospitals surveyed, 51 (71%) responded. Mean hospital bed count was 940, three were safety-net hospitals, and one was a community-based teaching center; responding and nonresponding hospitals did not differ significantly in terms of bed count (Appendix).
Health System Adaptations, Testing, and PPE Status
Nearly all responding hospitals (46 of 51; 90%) had RIUs for patients with known or suspected COVID-19 (Table 1). Nearly all hospitals took steps to keep potentially sick healthcare providers from infecting others (eg, staying home if sick or exposed). Among respondents, 32% had rapid response teams, 24% had respiratory therapy teams, and 29% had case management teams that were dedicated to COVID-19 care. Thirty-two (63%) had developed models, such as ethics or palliative care consult services, to assist with difficult resource-allocation decisions (eg, how to prioritize ventilator use if demand exceeded supply). Twenty-three (45%) had developed post-acute care monitoring programs dedicated to COVID-19 patients.
At the time of our survey, only 2 sites (4%) reported COVID-19 test time turnaround under 1 hour, and 15 (30%) reported turnaround in less than 6 hours. Of the 29 sites able to provide estimates of PPE stockpile, 14 (48%) reported a supply of 2 weeks or less. The most common approaches to PPE stewardship focused on reuse of masks and face shields if not obviously soiled, centralizing PPE distribution, and disinfecting or sterilizing masks. Ten sites (20%) were utilizing 3-D printed masks, while 10% used homemade face shields or masks.
Characteristics of COVID-19 RIUs
Forty-six hospitals (90% of all respondents) in our cohort had developed RIUs at the time of survey administration. The earliest RIU implementation date was February 10, 2020, and the most recent was launched on the day of our survey. Admission to RIUs was primarily based on clinical factors associated with known or suspected COVID-19 infection (Table 2). The number of non–critical care RIU beds among locations at that time ranged from 10 or less to more than 50. The mean number of hospitalist attendings caring for patients in the RIUs was 10.2, with a mean 4.1 advanced practice providers, 5.5 residents, and 0 medical students. The number of planned patients per attending was typically 5 to 15. Nurses and physicians typically rounded separately. Medical distancing (eg, reducing patient room entry) was accomplished most commonly by grouped timing of medication administration (76% of sites), video links to room outside of rounding times (54% of sites), the use of video or telemedicine during rounds (17%), and clustering of activities such as medication administration or phlebotomy. The most common criteria prompting discharge from the RIU were a negative COVID-19 test (59%) and hospital discharge (57%), though comments from many respondents suggested that discharge criteria were changing rapidly.
Effects of Isolation Measures on In-Room Encounters and Diagnostic Processes
More than 90% of sites reported decreases in in-room encounter frequency across all provider types whether as a result of policies in place or not. Reductions were reported among hospitalists, advanced practice providers, residents, consultants, and therapists (Table 3). Reduced room entry most often resulted from an established or developing policy, but many noted reduced room entry without formal policies in place. Nearly all sites reported moving specialty consultations to phone or video evaluations. Diagnostic error was commonly reported, with missed non–COVID-19 medical diagnoses among COVID-19 infected patients being reported by 22 sites (46%) and missed COVID-19 diagnoses in patients admitted for other reasons by 22 sites (45%).
DISCUSSION
In this study of medical wards at academic medical centers, we found that, in response to the COVID-19 pandemic, hospitals made several changes in a short period of time to adapt to the crisis. These included implementation and rapid expansion of dedicated RIUs, greatly expanded use of inpatient telehealth for patient assessments and consultation, implementation of other approaches to minimize room entry (such as grouping in-room activities), and deployment of ethics consultation services to help manage issues around potential scarcity of life-saving measures such as ventilators. We also found that availability of PPE and timely testing was limited. Finally, a large proportion of sites reported potential diagnostic problems in the assessment of both patients suspected and those not suspected of having COVID-19.
RIUs are emerging as a primary modality for caring for non-ICU COVID-19 patients, though they never involved medical students; we hope the role of students in particular will increase as new models of training emerge in response to the pandemic.7 In contrast, telemedicine evolved rapidly to hold a substantial role in RIUs, with both ward and specialty teams using video visit technology to communicate with patients. COVID-19 has been viewed as a perfect use case for outpatient telemedicine,8 and a growing number of studies are examining its outpatient use9,10; however, to date, somewhat less attention has been paid to inpatient deployment. Although our data suggest telemedicine has found a prominent place in RIUs, it remains to be seen whether it is associated with differences in patient or provider outcomes. For example, deficiencies in the physical examination, limited face-to-face contact, and lack of physical presence could all affect the patient–provider relationship, patient engagement, and the accuracy of the diagnostic process.
Our data suggest the possibility of missing non–COVID-19 diagnoses in patients suspected of COVID-19 and missing COVID-19 in those admitted for nonrespiratory reasons. The latter may be addressed as routine COVID-19 screening of admitted patients becomes commonplace. For the former, however, it is possible that physicians are “anchoring” their thinking on COVID-19 to the exclusion of other diagnoses, that physicians are not fully aware of complications unique to COVID-19 infection (such as thromboembolism), and/or that the above-mentioned limitations of telemedicine have decreased diagnostic performance.
Although PPE stockpile data were not easily available for some sites, a distressingly large number reported stockpiles of 2 weeks or less, with reuse being the most common approach to extending PPE supply. We also found it concerning that 43% of hospital leaders did not know their stockpile data; we believe this is an important question that hospital leaders need to be asking. Most sites in our study reported test turnaround times of longer than 6 hours; lack of rapid COVID-19 testing further stresses PPE stockpile and may slow patients’ transition out of the RIU or discharge to home.
Our study has several limitations, including the evolving nature of the pandemic and rapid adaptations of care systems in the pandemic’s surge phase. However, we attempted to frame our questions in ways that provided a focused snapshot of care. Furthermore, respondents may not have had exhaustive knowledge of their institution’s COVID-19 response strategies, but most were the directors of their hospitalist services, and we encouraged the respondents to confer with others to gather high-fidelity data. Finally, as a survey of large academic medical centers, our results may not apply to nonacademic centers.
Approaches to caring for non-ICU patients during the COVID-19 pandemic are rapidly evolving. Expansion of RIUs and developing the workforce to support them has been a primary focus, with rapid innovation in use of technology emerging as a critical adaptation while PPE limitations persist and needs for “medical distancing” continue to grow. Although rates of missed COVID-19 diagnoses will likely be reduced with testing and systems improvements, physicians and systems will also need to consider how to utilize emerging technology in ways that can improve clinical care and provider safety while aiding diagnostic thinking. This survey illustrates the rapid adaptations made by our hospitals in response to the pandemic; ongoing adaptation will likely be needed to optimally care for hospitalized patients with COVID-19 while the pandemic continues to evolve.
Acknowledgment
Thanks to members of the HOMERuN COVID-19 Collaborative Group: Baylor Scott & White Medical Center – Temple, Texas - Tresa McNeal MD; Beth Israel Deaconess Medical Center - Shani Herzig MD MPH, Joseph Li MD, Julius Yang MD PhD; Brigham and Women’s Hospital - Christopher Roy MD, Jeffrey Schnipper MD MPH; Cedars-Sinai Medical Center - Ed Seferian MD, ; ChristianaCare - Surekha Bhamidipati MD; Cleveland Clinic - Matthew Pappas MD MPH; Dartmouth-Hitchcock Medical Center - Jonathan Lurie MD MS; Dell Medical School at The University of Texas at Austin - Chris Moriates MD, Luci Leykum MD MBA MSc; Denver Health and Hospitals Authority - Diana Mancini MD; Emory University Hospital - Dan Hunt MD; Johns Hopkins Hospital - Daniel J Brotman MD, Zishan K Siddiqui MD, Shaker Eid MD MBA; Maine Medical Center - Daniel A Meyer MD, Robert Trowbridge MD; Massachusetts General Hospital - Melissa Mattison MD; Mayo Clinic Rochester – Caroline Burton MD, Sagar Dugani MD PhD; Medical College of Wisconsin - Sanjay Bhandari MD; Miriam Hospital - Kwame Dapaah-Afriyie MD MBA; Mount Sinai Hospital - Andrew Dunn MD; NorthShore - David Lovinger MD; Northwestern Memorial Hospital - Kevin O’Leary MD MS; Ohio State University Wexner Medical Center - Eric Schumacher DO; Oregon Health & Science University - Angela Alday MD; Penn Medicine - Ryan Greysen MD MHS MA; Rutgers- Robert Wood Johnson University Hospital - Michael Steinberg MD MPH; Stanford University School of Medicine - Neera Ahuja MD; Tulane Hospital and University Medical Center - Geraldine Ménard MD; UC San Diego Health - Ian Jenkins MD; UC Los Angeles Health - Michael Lazarus MD, Magdalena E. Ptaszny, MD; UC San Francisco Health - Bradley A Sharpe, MD, Margaret Fang MD MPH; UK HealthCare - Mark Williams MD MHM, John Romond MD; University of Chicago – David Meltzer MD PhD, Gregory Ruhnke MD; University of Colorado - Marisha Burden MD; University of Florida - Nila Radhakrishnan MD; University of Iowa Hospitals and Clinics - Kevin Glenn MD MS; University of Miami - Efren Manjarrez MD; University of Michigan - Vineet Chopra MD MSc, Valerie Vaughn MD MSc; University of Missouri-Columbia Hospital - Hasan Naqvi MD; University of Nebraska Medical Center - Chad Vokoun MD; University of North Carolina at Chapel Hill - David Hemsey MD; University of Pittsburgh Medical Center - Gena Marie Walker MD; University of Vermont Medical Center - Steven Grant MD; University of Washington Medical Center - Christopher Kim MD MBA, Andrew White MD; University of Washington-Harborview Medical Center - Maralyssa Bann MD; University of Wisconsin Hospital and Clinics - David Sterken MD, Farah Kaiksow MD MPP, Ann Sheehy MD MS, Jordan Kenik MD MPH; UW Northwest Campus - Ben Wolpaw MD; Vanderbilt University Medical Center - Sunil Kripalani MD MSc, Eduard E Vasilevskis MD, Kathleene T Wooldridge MD MPH; Wake Forest Baptist Health - Erik Summers MD; Washington University St. Louis - Michael Lin MD; Weill Cornell - Justin Choi MD; Yale New Haven Hospital - William Cushing MA, Chris Sankey MD; Zuckerberg San Francisco General Hospital - Sumant Ranji MD.
1. Institute for Health Metrics and Evaluation. COVID-19 Projections: United States of America. 2020. Accessed May 5, 2020. https://covid19.healthdata.org/united-states-of-america
2. Iserson KV. Alternative care sites: an option in disasters. West J Emerg Med. 2020;21(3):484‐489. https://doi.org/10.5811/westjem.2020.4.47552
3. Paganini M, Conti A, Weinstein E, Della Corte F, Ragazzoni L. Translating COVID-19 pandemic surge theory to practice in the emergency department: how to expand structure [online first]. Disaster Med Public Health Prep. 2020:1-10. https://doi.org/10.1017/dmp.2020.57
4. Kumaraiah D, Yip N, Ivascu N, Hill L. Innovative ICU Physician Care Models: Covid-19 Pandemic at NewYork-Presbyterian. NEJM: Catalyst. April 28, 2020. Accessed May 5, 2020. https://catalyst.nejm.org/doi/full/10.1056/CAT.20.0158
5. Auerbach AD, Patel MS, Metlay JP, et al. The Hospital Medicine Reengineering Network (HOMERuN): a learning organization focused on improving hospital care. Acad Med. 2014;89(3):415-420. https://doi.org/10.1097/acm.0000000000000139
6. Livingston E, Desai A, Berkwits M. Sourcing personal protective equipment during the COVID-19 pandemic [online first]. JAMA. 2020. https://doi.org/10.1001/jama.2020.5317
7. Bauchner H, Sharfstein J. A bold response to the COVID-19 pandemic: medical students, national service, and public health [online first]. JAMA. 2020. https://doi.org/10.1001/jama.2020.6166
8. Hollander JE, Carr BG. Virtually perfect? telemedicine for Covid-19. N Engl J Med. 2020;382(18):1679‐1681. https://doi.org/10.1056/nejmp2003539
9. Hau YS, Kim JK, Hur J, Chang MC. How about actively using telemedicine during the COVID-19 pandemic? J Med Syst. 2020;44(6):108. https://doi.org/10.1007/s10916-020-01580-z
10. Smith WR, Atala AJ, Terlecki RP, Kelly EE, Matthews CA. Implementation guide for rapid integration of an outpatient telemedicine program during the COVID-19 pandemic [online first]. J Am Coll Surg. 2020. https://doi.org/10.1016/j.jamcollsurg.2020.04.030
1. Institute for Health Metrics and Evaluation. COVID-19 Projections: United States of America. 2020. Accessed May 5, 2020. https://covid19.healthdata.org/united-states-of-america
2. Iserson KV. Alternative care sites: an option in disasters. West J Emerg Med. 2020;21(3):484‐489. https://doi.org/10.5811/westjem.2020.4.47552
3. Paganini M, Conti A, Weinstein E, Della Corte F, Ragazzoni L. Translating COVID-19 pandemic surge theory to practice in the emergency department: how to expand structure [online first]. Disaster Med Public Health Prep. 2020:1-10. https://doi.org/10.1017/dmp.2020.57
4. Kumaraiah D, Yip N, Ivascu N, Hill L. Innovative ICU Physician Care Models: Covid-19 Pandemic at NewYork-Presbyterian. NEJM: Catalyst. April 28, 2020. Accessed May 5, 2020. https://catalyst.nejm.org/doi/full/10.1056/CAT.20.0158
5. Auerbach AD, Patel MS, Metlay JP, et al. The Hospital Medicine Reengineering Network (HOMERuN): a learning organization focused on improving hospital care. Acad Med. 2014;89(3):415-420. https://doi.org/10.1097/acm.0000000000000139
6. Livingston E, Desai A, Berkwits M. Sourcing personal protective equipment during the COVID-19 pandemic [online first]. JAMA. 2020. https://doi.org/10.1001/jama.2020.5317
7. Bauchner H, Sharfstein J. A bold response to the COVID-19 pandemic: medical students, national service, and public health [online first]. JAMA. 2020. https://doi.org/10.1001/jama.2020.6166
8. Hollander JE, Carr BG. Virtually perfect? telemedicine for Covid-19. N Engl J Med. 2020;382(18):1679‐1681. https://doi.org/10.1056/nejmp2003539
9. Hau YS, Kim JK, Hur J, Chang MC. How about actively using telemedicine during the COVID-19 pandemic? J Med Syst. 2020;44(6):108. https://doi.org/10.1007/s10916-020-01580-z
10. Smith WR, Atala AJ, Terlecki RP, Kelly EE, Matthews CA. Implementation guide for rapid integration of an outpatient telemedicine program during the COVID-19 pandemic [online first]. J Am Coll Surg. 2020. https://doi.org/10.1016/j.jamcollsurg.2020.04.030
© 2020 Society of Hospital Medicine
Clinical utility of routine CBC testing in patients with community-acquired pneumonia
Avoiding repeated complete blood count (CBC) tests in the face of clinical and lab stability is a focus of the Choosing Wisely® initiatives launched by the American Board of Internal Medicine Foundation1 and endorsed by the Society of Hospital Medicine.2 However, specific scenarios in which daily morning labs can be safely avoided have not been identified. The goal of this study was to identify situations in which routine CBC testing can be avoided in patients with community-acquired pneumonia (CAP), one of the most common reasons for hospital admission.3
METHODS
This was a retrospective study of 50 patients with CAP discharged from our hospital between February 1, 2015 and May 1, 2015. We performed chart abstractions collecting daily vital signs, lab results, provider notes including assessments and plans (A&Ps), and order entry logs, as well as documentation indicating whether a lab result or clinical finding appeared to affect clinical management (eg, a new order or documentation of changing plans). Both escalations and de-escalations were included as management changes. For example, if the note stated “Persistent leukocytosis, add vancomycin,” then the clinical action of expanded antibiotic coverage would be attributed to the CBC.
We defined clinical stability based on Definition B of the Pneumonia Patient Outcomes Research Team (PORT) study criteria.4 We used descriptive statistics and likelihood ratios to characterize the utility of CBC testing in terms of producing clinical management changes. Likelihood ratios were calculated with the “test” representing a CBC being ordered or not ordered and the outcome being any change in management independent of whether it was due to the CBC.
RESULTS
Of 50 patients, 33 (66%) were female, the mean age was 75 years, the mean length of stay was 2.8 days, and the median CURB-65 score,5 an estimate of mortality in CAP used for decision-making about inpatient versus outpatient treatment, was 1 (25th to 75th interquartile range: 1, 2); no patients had a CURB score greater than 3 (Table 1). Forty-one (82%) patients met PORT clinical stability criteria prior to discharge, and 30 (75% of stable patients) had CBCs obtained.
On days after admission, 94 subsequent CBCs were obtained. Of these CBCs, 6 (6.4%) were associated with management changes indicated in documentation or orders (Table 2). In 2 of the 6 patients, management changes were likely relevant to pneumonia. In the first case, the patient had a white blood cell count (WBC) of 15.4 on the planned day of discharge but no accompanying clinical changes. Her discharge was potentially delayed pending a repeat CBC which again showed a WBC 14.7; the patient was then discharged without any additional changes in plan. In the second case, the patient experienced new-onset altered mental status on hospital day 3 and increasing O2 requirement with a rising WBC noted on hospital day 4. Repeat chest x-ray, repeat blood cultures, and an ultrasound for parapneumonic effusion were obtained, and the patient’s symptoms and signs resolved over a period of days without changes in treatment. In the 4 other cases, available documentation suggested the hemoglobin abnormalities found represented chronic or incidental illnesses, specifically iron deficiency anemia, iatrogenic anemia due to fluid resuscitation and hemodilution, previously known chronic lymphocytic leukemia, and thrombocytopenia due to acute infection. In all 6 instances, CBC values improved without treatment intervention.
Among all patients, the positive likelihood ratio of CBCs obtained after admission in terms of being followed by a change in clinical management was very poor (1.12, 95% confidence interval [CI], 0.86-1.44). For clinically unstable patients, there were 64 CBCs ordered, and the likelihood ratio was similar at 0.98 (95% CI, 0.75-1.29). The positive likelihood ratio among clinically stable patients, who had 30 CBCs ordered, was still quite weak, though confidence intervals were wider (1.23, 95% CI, 0.66-2.29).
DISCUSSION
Though small, our initial study suggests the potential opportunity for savings if Choosing Wisely® recommendations for CBC testing were implemented in patients with community-acquired pneumonia.
Our study has several limitations. Note-writing practices and ordering patterns likely varied between providers, and documentation bias may play a role in our results. However, we defined whether a CBC was associated with changes in clinical decision-making or management by incorporating a number of mutually reinforcing elements of the medical record. We recognize, however, that our approach may not capture undocumented clinical issues or other cognitive (eg, reassurance of clinical resolution) reasons why CBCs were obtained.
Even with these limitations, the likelihood of a CBC value meaningfully changing clinical management among patients with CAP appears to be quite low as evidenced by the case descriptions, particularly when obtained in stable patients by PORT criteria and on the day of discharge. Whether clinical stability as measured by PORT score can be used to target patients in whom CBC testing is unnecessary is difficult to discern from our data, as the overall utility of CBCs obtained after admission was quite low and the rate of changes in management was also low. However, even if CBCs are not particularly costly, unnecessary testing may produce harm in the form of prolonged length of stay, making even one unnecessary CBC potentially extremely expensive. More research involving larger-scale studies are needed to determine the “number needed to screen” for the daily CBC in CAP to determine if the cost savings from overtesting and treatment outweigh the potential benefit of a single CBC that changes management.
Disclosure
Nothing to report.
1. Choosing Wisely. Promoting conversations between providers and patients. Choosing Wisely. http://www.choosingwisely.org/. Accessed March 28, 2016.
2. Beresford L. The Society of Hospital Medicine’s “Choosing Wisely” Recommendations for Hospitalists. 2013. http://www.the-hospitalist.org/article/the-society-of-hospital-medicines-choosing-wisely-recommendations-for-hospitalists/. Accessed March 28, 2016.
3. File TM Jr, Marrie TJ. Burden of community-acquired pneumonia in North American adults. Postgrad Med. 2010;122(2):130-141. PubMed
4. Halm EA, Fine MJ, Marrie TJ, et al. Time to clinical stability in patients hospitalized with community-acquired pneumonia: Implications for practice guidelines. JAMA. 1998;279(18):1452-1457. PubMed
5. Lim W, van der Eerden MM, Laing R, et al. Defining community acquired pneumonia severity on presentation to hospital: an international derivation and validation study. Thorax. 2003;58(5):377-382. PubMed
Avoiding repeated complete blood count (CBC) tests in the face of clinical and lab stability is a focus of the Choosing Wisely® initiatives launched by the American Board of Internal Medicine Foundation1 and endorsed by the Society of Hospital Medicine.2 However, specific scenarios in which daily morning labs can be safely avoided have not been identified. The goal of this study was to identify situations in which routine CBC testing can be avoided in patients with community-acquired pneumonia (CAP), one of the most common reasons for hospital admission.3
METHODS
This was a retrospective study of 50 patients with CAP discharged from our hospital between February 1, 2015 and May 1, 2015. We performed chart abstractions collecting daily vital signs, lab results, provider notes including assessments and plans (A&Ps), and order entry logs, as well as documentation indicating whether a lab result or clinical finding appeared to affect clinical management (eg, a new order or documentation of changing plans). Both escalations and de-escalations were included as management changes. For example, if the note stated “Persistent leukocytosis, add vancomycin,” then the clinical action of expanded antibiotic coverage would be attributed to the CBC.
We defined clinical stability based on Definition B of the Pneumonia Patient Outcomes Research Team (PORT) study criteria.4 We used descriptive statistics and likelihood ratios to characterize the utility of CBC testing in terms of producing clinical management changes. Likelihood ratios were calculated with the “test” representing a CBC being ordered or not ordered and the outcome being any change in management independent of whether it was due to the CBC.
RESULTS
Of 50 patients, 33 (66%) were female, the mean age was 75 years, the mean length of stay was 2.8 days, and the median CURB-65 score,5 an estimate of mortality in CAP used for decision-making about inpatient versus outpatient treatment, was 1 (25th to 75th interquartile range: 1, 2); no patients had a CURB score greater than 3 (Table 1). Forty-one (82%) patients met PORT clinical stability criteria prior to discharge, and 30 (75% of stable patients) had CBCs obtained.
On days after admission, 94 subsequent CBCs were obtained. Of these CBCs, 6 (6.4%) were associated with management changes indicated in documentation or orders (Table 2). In 2 of the 6 patients, management changes were likely relevant to pneumonia. In the first case, the patient had a white blood cell count (WBC) of 15.4 on the planned day of discharge but no accompanying clinical changes. Her discharge was potentially delayed pending a repeat CBC which again showed a WBC 14.7; the patient was then discharged without any additional changes in plan. In the second case, the patient experienced new-onset altered mental status on hospital day 3 and increasing O2 requirement with a rising WBC noted on hospital day 4. Repeat chest x-ray, repeat blood cultures, and an ultrasound for parapneumonic effusion were obtained, and the patient’s symptoms and signs resolved over a period of days without changes in treatment. In the 4 other cases, available documentation suggested the hemoglobin abnormalities found represented chronic or incidental illnesses, specifically iron deficiency anemia, iatrogenic anemia due to fluid resuscitation and hemodilution, previously known chronic lymphocytic leukemia, and thrombocytopenia due to acute infection. In all 6 instances, CBC values improved without treatment intervention.
Among all patients, the positive likelihood ratio of CBCs obtained after admission in terms of being followed by a change in clinical management was very poor (1.12, 95% confidence interval [CI], 0.86-1.44). For clinically unstable patients, there were 64 CBCs ordered, and the likelihood ratio was similar at 0.98 (95% CI, 0.75-1.29). The positive likelihood ratio among clinically stable patients, who had 30 CBCs ordered, was still quite weak, though confidence intervals were wider (1.23, 95% CI, 0.66-2.29).
DISCUSSION
Though small, our initial study suggests the potential opportunity for savings if Choosing Wisely® recommendations for CBC testing were implemented in patients with community-acquired pneumonia.
Our study has several limitations. Note-writing practices and ordering patterns likely varied between providers, and documentation bias may play a role in our results. However, we defined whether a CBC was associated with changes in clinical decision-making or management by incorporating a number of mutually reinforcing elements of the medical record. We recognize, however, that our approach may not capture undocumented clinical issues or other cognitive (eg, reassurance of clinical resolution) reasons why CBCs were obtained.
Even with these limitations, the likelihood of a CBC value meaningfully changing clinical management among patients with CAP appears to be quite low as evidenced by the case descriptions, particularly when obtained in stable patients by PORT criteria and on the day of discharge. Whether clinical stability as measured by PORT score can be used to target patients in whom CBC testing is unnecessary is difficult to discern from our data, as the overall utility of CBCs obtained after admission was quite low and the rate of changes in management was also low. However, even if CBCs are not particularly costly, unnecessary testing may produce harm in the form of prolonged length of stay, making even one unnecessary CBC potentially extremely expensive. More research involving larger-scale studies are needed to determine the “number needed to screen” for the daily CBC in CAP to determine if the cost savings from overtesting and treatment outweigh the potential benefit of a single CBC that changes management.
Disclosure
Nothing to report.
Avoiding repeated complete blood count (CBC) tests in the face of clinical and lab stability is a focus of the Choosing Wisely® initiatives launched by the American Board of Internal Medicine Foundation1 and endorsed by the Society of Hospital Medicine.2 However, specific scenarios in which daily morning labs can be safely avoided have not been identified. The goal of this study was to identify situations in which routine CBC testing can be avoided in patients with community-acquired pneumonia (CAP), one of the most common reasons for hospital admission.3
METHODS
This was a retrospective study of 50 patients with CAP discharged from our hospital between February 1, 2015 and May 1, 2015. We performed chart abstractions collecting daily vital signs, lab results, provider notes including assessments and plans (A&Ps), and order entry logs, as well as documentation indicating whether a lab result or clinical finding appeared to affect clinical management (eg, a new order or documentation of changing plans). Both escalations and de-escalations were included as management changes. For example, if the note stated “Persistent leukocytosis, add vancomycin,” then the clinical action of expanded antibiotic coverage would be attributed to the CBC.
We defined clinical stability based on Definition B of the Pneumonia Patient Outcomes Research Team (PORT) study criteria.4 We used descriptive statistics and likelihood ratios to characterize the utility of CBC testing in terms of producing clinical management changes. Likelihood ratios were calculated with the “test” representing a CBC being ordered or not ordered and the outcome being any change in management independent of whether it was due to the CBC.
RESULTS
Of 50 patients, 33 (66%) were female, the mean age was 75 years, the mean length of stay was 2.8 days, and the median CURB-65 score,5 an estimate of mortality in CAP used for decision-making about inpatient versus outpatient treatment, was 1 (25th to 75th interquartile range: 1, 2); no patients had a CURB score greater than 3 (Table 1). Forty-one (82%) patients met PORT clinical stability criteria prior to discharge, and 30 (75% of stable patients) had CBCs obtained.
On days after admission, 94 subsequent CBCs were obtained. Of these CBCs, 6 (6.4%) were associated with management changes indicated in documentation or orders (Table 2). In 2 of the 6 patients, management changes were likely relevant to pneumonia. In the first case, the patient had a white blood cell count (WBC) of 15.4 on the planned day of discharge but no accompanying clinical changes. Her discharge was potentially delayed pending a repeat CBC which again showed a WBC 14.7; the patient was then discharged without any additional changes in plan. In the second case, the patient experienced new-onset altered mental status on hospital day 3 and increasing O2 requirement with a rising WBC noted on hospital day 4. Repeat chest x-ray, repeat blood cultures, and an ultrasound for parapneumonic effusion were obtained, and the patient’s symptoms and signs resolved over a period of days without changes in treatment. In the 4 other cases, available documentation suggested the hemoglobin abnormalities found represented chronic or incidental illnesses, specifically iron deficiency anemia, iatrogenic anemia due to fluid resuscitation and hemodilution, previously known chronic lymphocytic leukemia, and thrombocytopenia due to acute infection. In all 6 instances, CBC values improved without treatment intervention.
Among all patients, the positive likelihood ratio of CBCs obtained after admission in terms of being followed by a change in clinical management was very poor (1.12, 95% confidence interval [CI], 0.86-1.44). For clinically unstable patients, there were 64 CBCs ordered, and the likelihood ratio was similar at 0.98 (95% CI, 0.75-1.29). The positive likelihood ratio among clinically stable patients, who had 30 CBCs ordered, was still quite weak, though confidence intervals were wider (1.23, 95% CI, 0.66-2.29).
DISCUSSION
Though small, our initial study suggests the potential opportunity for savings if Choosing Wisely® recommendations for CBC testing were implemented in patients with community-acquired pneumonia.
Our study has several limitations. Note-writing practices and ordering patterns likely varied between providers, and documentation bias may play a role in our results. However, we defined whether a CBC was associated with changes in clinical decision-making or management by incorporating a number of mutually reinforcing elements of the medical record. We recognize, however, that our approach may not capture undocumented clinical issues or other cognitive (eg, reassurance of clinical resolution) reasons why CBCs were obtained.
Even with these limitations, the likelihood of a CBC value meaningfully changing clinical management among patients with CAP appears to be quite low as evidenced by the case descriptions, particularly when obtained in stable patients by PORT criteria and on the day of discharge. Whether clinical stability as measured by PORT score can be used to target patients in whom CBC testing is unnecessary is difficult to discern from our data, as the overall utility of CBCs obtained after admission was quite low and the rate of changes in management was also low. However, even if CBCs are not particularly costly, unnecessary testing may produce harm in the form of prolonged length of stay, making even one unnecessary CBC potentially extremely expensive. More research involving larger-scale studies are needed to determine the “number needed to screen” for the daily CBC in CAP to determine if the cost savings from overtesting and treatment outweigh the potential benefit of a single CBC that changes management.
Disclosure
Nothing to report.
1. Choosing Wisely. Promoting conversations between providers and patients. Choosing Wisely. http://www.choosingwisely.org/. Accessed March 28, 2016.
2. Beresford L. The Society of Hospital Medicine’s “Choosing Wisely” Recommendations for Hospitalists. 2013. http://www.the-hospitalist.org/article/the-society-of-hospital-medicines-choosing-wisely-recommendations-for-hospitalists/. Accessed March 28, 2016.
3. File TM Jr, Marrie TJ. Burden of community-acquired pneumonia in North American adults. Postgrad Med. 2010;122(2):130-141. PubMed
4. Halm EA, Fine MJ, Marrie TJ, et al. Time to clinical stability in patients hospitalized with community-acquired pneumonia: Implications for practice guidelines. JAMA. 1998;279(18):1452-1457. PubMed
5. Lim W, van der Eerden MM, Laing R, et al. Defining community acquired pneumonia severity on presentation to hospital: an international derivation and validation study. Thorax. 2003;58(5):377-382. PubMed
1. Choosing Wisely. Promoting conversations between providers and patients. Choosing Wisely. http://www.choosingwisely.org/. Accessed March 28, 2016.
2. Beresford L. The Society of Hospital Medicine’s “Choosing Wisely” Recommendations for Hospitalists. 2013. http://www.the-hospitalist.org/article/the-society-of-hospital-medicines-choosing-wisely-recommendations-for-hospitalists/. Accessed March 28, 2016.
3. File TM Jr, Marrie TJ. Burden of community-acquired pneumonia in North American adults. Postgrad Med. 2010;122(2):130-141. PubMed
4. Halm EA, Fine MJ, Marrie TJ, et al. Time to clinical stability in patients hospitalized with community-acquired pneumonia: Implications for practice guidelines. JAMA. 1998;279(18):1452-1457. PubMed
5. Lim W, van der Eerden MM, Laing R, et al. Defining community acquired pneumonia severity on presentation to hospital: an international derivation and validation study. Thorax. 2003;58(5):377-382. PubMed
Perceived safety and value of inpatient “very important person” services
Recent publications in the medical literature and lay press have stirred controversy regarding the use of inpatient ‘very important person’ (VIP) services.1-3 The term “VIP services” often refers to select conveniences offered in addition to the assumed basic level of care and services provided by a hospital. Examples include additional space, enhanced facilities, specific comforts, or personal support. In some instances, these amenities may only be provided to patients who have close financial, social, or professional relationships with the hospital.
How VIP patients interact with their health system to obtain VIP services has raised unique concerns. Some have speculated that the presence of a VIP patient may be disruptive to the care of non-VIP patients, while others have cautioned physicians about potential dangers to the VIP patients themselves.4-6 Despite much being written on the topics of VIP patients and services in both the lay and academic press, our literature review identified only 1 study on the topic, which cataloged the preferential treatment of VIP patients in the emergency department.6 We are unaware of any investigations of VIP-service use in the inpatient setting. Through a multisite survey of hospital medicine physicians, we assessed physician viewpoints and behavior regarding VIP services.
METHODS
The Hospital Medicine Reengineering Network (HOMERuN) is a nation-wide learning organization focused on measuring and improving the outcomes of hospitalized patients.7 We surveyed hospitalists from 8 HOMERuN hospitals (Appendix 1). The survey instrument contained 4 sections: nonidentifying respondent demographics, local use of VIP services, reported physician perceptions of VIP services, and case-based assessments (Appendix 2). Survey questions and individual cases were developed by study authors and based on real scenarios and concerns provided by front-line clinical providers. Content, length, and reliability of physician understanding were assessed by a 5-person focus group consisting of physicians not included in the survey population.
Subjects were identified via administrative rosters from each HOMERuN site. Surveys were administered via SurveyMonkey, and results were analyzed descriptively. Populations were compared via the Fisher exact test. “VIP services” were defined as conveniences provided in addition to the assumed basic level of care and services (eg, private or luxury-style rooms, access to a special menu, better views, dedicated personal care attendants, hospital liaisons). VIP patients were defined as those patients receiving VIP services. A hospital was identified as providing VIP services if 50% or more of respondents from that site reported the presence of VIP services.
RESULTS
Of 366 hospitalists contacted, 160 completed the survey (44%). Respondent characteristics and reported prevalence of VIP services are demonstrated in Table 1. In total, 78 respondents (45%) reported the presence of VIP services at their hospital. Of the 8 sites surveyed, a majority of physicians at 4 sites (50%) reported presence of VIP services.
Of respondents reporting the presence of VIP services at their hospital, a majority felt that, from a patient safety perspective, the care received by VIP patients was the same as care received by non-VIP patients (Table 2). A majority reported they had felt pressured by a VIP patient or a family member to order additional tests or treatments that the physician believed were medically unnecessary and that they would be more likely to comply with VIP patient’s requests for tests or treatments they felt were unnecessary. More than one-third (36%) felt pressured by other hospital employees or representatives to comply with VIP services patient’s requests for additional tests or treatments that the physicians believed were medically unnecessary.
When presented the case of a VIP patient with community-acquired pneumonia who is clinically stable for discharge but expressing concerns about leaving the hospital, 61 (38%) respondents reported they would not discharge this patient home: 39 of 70 (55.7%) who reported the presence of VIP services at their hospital, and 22 of 91 (24.2%) who reported the absence of VIP services (P < 0.001). Of those who reported they would not discharge this patient home, 37 (61%) reported the reason for this related to the patient’s connection to the Board of Trustees; 48 (79%) reported the reason for this related to the patient’s concerns; 9 (15%) reported the reason for this related to their own concerns regarding medical details of the patient’s case (respondents could select more than 1 reason).
When presented the case of a VIP patient with acute pulmonary embolism who is medically ready for discharge with primary care physician-approved anticoagulation and discharge plans but for whom their family requests additional consultations and inpatient hypercoagulable workup, 33 (21%) respondents reported they would order additional testing and specialist consultation: 17 of 69 (24.6%) who reported the presence of VIP services their hospital, and 16 of 91 (17.6%) who reported the absence of VIP services (P = 0.33). Of those who reported they would order additional testing and specialist consultation, 14 (42%) reported the reason for this related to the family’s financial connections to the hospital; 30 (91%) reported the reason for this related to the family’s concerns; 3 (9%) reported the reason for this related to their own concerns about the medical details of the patient’s case (respondents could select more than 1 reason).
DISCUSSION
In our study, a majority of physicians who reported the presence of VIP services at their hospital felt pressured by VIP patients or their family members to perform unnecessary testing or treatment. While this study was not designed to quantify the burden of unnecessary care for VIP patients, our results have implications for individual patients and public health, including potential effects on resource availability, the identification of clinically irrelevant incidental findings, and short- and long-term medical complications of procedures, testing and radiation exposure.
Prior publications have advocated that physicians and hospitals should not allow VIP status to influence management decisions.3,5 We found that more than one-third of physicians who reported the presence of VIP services at their hospital also reported receiving pressure from hospital representatives to provide care to VIP patients that was not medically indicated. These findings highlight an example of the tension faced by physicians who are caught between patient requests and the delivery of value-based care. This potential conflict may be amplified particularly for those patients with close financial, social, or professional ties to the hospitals (and physicians) providing their care. These results suggest the need for physicians, administrators, and patients to work together to address the potential blurring of ethical boundaries created by VIP relationships. Prevention of harm and avoidance of placing physicians in morally distressing situations are common goals for all involved parties.
Efforts to reduce unnecessary care have predominantly focused on structural and knowledge-based drivers.4,8,9 Our results highlight the presence of additional forces. A majority of physician respondents who reported the presence of VIP services at their hospital also reported that they would be more likely to comply with requests for unnecessary care for a VIP patient as compared to a non-VIP patient. Furthermore, in case-based questions about the requests of a VIP patient and their family for additional unnecessary care, a significant portion of physicians who reported they would comply with these requests listed the VIP status of the patient or family as a factor underlying this decision. Only a minority of physicians reported their decision to provide additional care was the result of their own medically-based concerns. Because these cases were hypothetical and we did not include comparator cases involving non-VIP patients, it remains uncertain whether the observed perceptions accurately reflect real-world differences in the care of VIP and non-VIP patients. Nonetheless, our findings emphasize the importance of better understanding the social drivers of overuse and physician communication strategies related to medically inappropriate tests.10,11
Demand for unnecessary testing may be driven by the mentality that “more is better.”12 Contrary to this belief, provision of unnecessary care can increase the risk of patient harm.13 Despite physician respondents reporting that VIP patients requested and/or received additional unnecessary care, a majority of respondents felt that patient safety for VIP patients was equivalent to that for non-VIP patients. As we assessed only physician perceptions of safety, which may not necessarily correlate with actual safety, further research in this area is needed.
Our study was limited by several factors. While our study population included hospitalists from 8 geographically broad hospitals, including university, safety net, and community hospitals, study responses may not be reflective of nationwide trends. Our response rate may limit our ability to generalize conclusions beyond respondents. Second, our study captured physician perceptions of behavior and safety rather than actually measuring practice and outcomes. Studies comparing physician practice patterns and outcomes between VIP and non-VIP patients would be informative. Additionally, despite our inclusive survey design process, our survey was not validated, and it is possible that our questions were not interpreted as intended. Lastly, despite the anonymous nature of our survey, physicians may have felt compelled to respond in a particular way due to conflicting professional, financial, or social factors.
Our findings provide initial insight into how care for the VIP patient may present unique challenges for physicians, hospitals, and society by systematizing care inequities, as well as potentially incentivizing low-value care practices. Whether these imbalances produce clinical harms or benefits remains worthy of future studies.
Disclosure
Nothing to report.
1. Bernstein N. Chefs, butlers, marble baths: Hospitals vie for the affluent. New York Times. January 21, 2012. http://www.nytimes.com/2012/01/22/nyregion/chefs-butlers-and-marble-baths-not-your-average-hospital-room.html. Accessed February 1, 2017.
2. Kennedy DW, Kagan SH, Abramson KB, Boberick C, Kaiser LR. Academic medicine amenities unit: developing a model to integrate academic medical care with luxury hotel services. Acad Med. 2009;84(2):185-191. PubMed
3. Alfandre D, Clever S, Farber NJ, Hughes MT, Redstone P, Lehmann LS. Caring for ‘very important patients’--ethical dilemmas and suggestions for practical management. Am J Med. 2016;129(2):143-147. PubMed
4. Bulger J, Nickel W, Messler J, et al. Choosing wisely in adult hospital medicine: five opportunities for improved healthcare value. J Hosp Med. 2013;8(9):486-492. PubMed
5. Martin A, Bostic JQ, Pruett K. The V.I.P.: hazard and promise in treating “special” patients. J Am Acad Child Adolesc Psychiatry. 2004;43(3):366-369. PubMed
6. Smally AJ, Carroll B, Carius M, Tilden F, Werdmann M. Treatment of VIPs. Ann Emerg Med. 2011;58(4):397-398. PubMed
7. Auerbach AD, Patel MS, Metlay JP, et al. The hospital medicine reengineering network (HOMERuN): a learning organization focused on improving hospital care. Acad Med. 2014;89(3):415-420. PubMed
8. Caverly TJ, Combs BP, Moriates C, Shah N, Grady D. Too much medicine happens too often: the teachable moment and a call for manuscripts from clinical trainees. JAMA Intern Med. 2014;174(1):8-9. PubMed
9. Schwartz AL, Chernew ME, Landon BE, McWilliams JM. Changes in low-value services in year 1 of the Medicare pioneer accountable care organization program. JAMA Intern Med. 2015;175(11):1815-1825. PubMed
10. Paterniti DA, Fancher TL, Cipri CS, Timmermans S, Heritage J, Kravitz RL. Getting to “no”: strategies primary care physicians use to deny patient requests. Arch Intern Med. 2010;170(4):381-388. PubMed
11. Veroff D, Marr A, Wennberg DE. Enhanced support for shared decision making reduced costs of care for patients with preference-sensitive conditions. Health Aff (Millwood). 2013;32(2):285-293. PubMed
12. Korenstein D. Patient perception of benefits and harms: the Achilles heel of high-value care. JAMA Intern Med. 2015;175(2):287-288. PubMed
13. Moynihan R, Doust J, Henry D. Preventing overdiagnosis: how to stop harming the healthy. BMJ. 2012;344:e3502. PubMed
Recent publications in the medical literature and lay press have stirred controversy regarding the use of inpatient ‘very important person’ (VIP) services.1-3 The term “VIP services” often refers to select conveniences offered in addition to the assumed basic level of care and services provided by a hospital. Examples include additional space, enhanced facilities, specific comforts, or personal support. In some instances, these amenities may only be provided to patients who have close financial, social, or professional relationships with the hospital.
How VIP patients interact with their health system to obtain VIP services has raised unique concerns. Some have speculated that the presence of a VIP patient may be disruptive to the care of non-VIP patients, while others have cautioned physicians about potential dangers to the VIP patients themselves.4-6 Despite much being written on the topics of VIP patients and services in both the lay and academic press, our literature review identified only 1 study on the topic, which cataloged the preferential treatment of VIP patients in the emergency department.6 We are unaware of any investigations of VIP-service use in the inpatient setting. Through a multisite survey of hospital medicine physicians, we assessed physician viewpoints and behavior regarding VIP services.
METHODS
The Hospital Medicine Reengineering Network (HOMERuN) is a nation-wide learning organization focused on measuring and improving the outcomes of hospitalized patients.7 We surveyed hospitalists from 8 HOMERuN hospitals (Appendix 1). The survey instrument contained 4 sections: nonidentifying respondent demographics, local use of VIP services, reported physician perceptions of VIP services, and case-based assessments (Appendix 2). Survey questions and individual cases were developed by study authors and based on real scenarios and concerns provided by front-line clinical providers. Content, length, and reliability of physician understanding were assessed by a 5-person focus group consisting of physicians not included in the survey population.
Subjects were identified via administrative rosters from each HOMERuN site. Surveys were administered via SurveyMonkey, and results were analyzed descriptively. Populations were compared via the Fisher exact test. “VIP services” were defined as conveniences provided in addition to the assumed basic level of care and services (eg, private or luxury-style rooms, access to a special menu, better views, dedicated personal care attendants, hospital liaisons). VIP patients were defined as those patients receiving VIP services. A hospital was identified as providing VIP services if 50% or more of respondents from that site reported the presence of VIP services.
RESULTS
Of 366 hospitalists contacted, 160 completed the survey (44%). Respondent characteristics and reported prevalence of VIP services are demonstrated in Table 1. In total, 78 respondents (45%) reported the presence of VIP services at their hospital. Of the 8 sites surveyed, a majority of physicians at 4 sites (50%) reported presence of VIP services.
Of respondents reporting the presence of VIP services at their hospital, a majority felt that, from a patient safety perspective, the care received by VIP patients was the same as care received by non-VIP patients (Table 2). A majority reported they had felt pressured by a VIP patient or a family member to order additional tests or treatments that the physician believed were medically unnecessary and that they would be more likely to comply with VIP patient’s requests for tests or treatments they felt were unnecessary. More than one-third (36%) felt pressured by other hospital employees or representatives to comply with VIP services patient’s requests for additional tests or treatments that the physicians believed were medically unnecessary.
When presented the case of a VIP patient with community-acquired pneumonia who is clinically stable for discharge but expressing concerns about leaving the hospital, 61 (38%) respondents reported they would not discharge this patient home: 39 of 70 (55.7%) who reported the presence of VIP services at their hospital, and 22 of 91 (24.2%) who reported the absence of VIP services (P < 0.001). Of those who reported they would not discharge this patient home, 37 (61%) reported the reason for this related to the patient’s connection to the Board of Trustees; 48 (79%) reported the reason for this related to the patient’s concerns; 9 (15%) reported the reason for this related to their own concerns regarding medical details of the patient’s case (respondents could select more than 1 reason).
When presented the case of a VIP patient with acute pulmonary embolism who is medically ready for discharge with primary care physician-approved anticoagulation and discharge plans but for whom their family requests additional consultations and inpatient hypercoagulable workup, 33 (21%) respondents reported they would order additional testing and specialist consultation: 17 of 69 (24.6%) who reported the presence of VIP services their hospital, and 16 of 91 (17.6%) who reported the absence of VIP services (P = 0.33). Of those who reported they would order additional testing and specialist consultation, 14 (42%) reported the reason for this related to the family’s financial connections to the hospital; 30 (91%) reported the reason for this related to the family’s concerns; 3 (9%) reported the reason for this related to their own concerns about the medical details of the patient’s case (respondents could select more than 1 reason).
DISCUSSION
In our study, a majority of physicians who reported the presence of VIP services at their hospital felt pressured by VIP patients or their family members to perform unnecessary testing or treatment. While this study was not designed to quantify the burden of unnecessary care for VIP patients, our results have implications for individual patients and public health, including potential effects on resource availability, the identification of clinically irrelevant incidental findings, and short- and long-term medical complications of procedures, testing and radiation exposure.
Prior publications have advocated that physicians and hospitals should not allow VIP status to influence management decisions.3,5 We found that more than one-third of physicians who reported the presence of VIP services at their hospital also reported receiving pressure from hospital representatives to provide care to VIP patients that was not medically indicated. These findings highlight an example of the tension faced by physicians who are caught between patient requests and the delivery of value-based care. This potential conflict may be amplified particularly for those patients with close financial, social, or professional ties to the hospitals (and physicians) providing their care. These results suggest the need for physicians, administrators, and patients to work together to address the potential blurring of ethical boundaries created by VIP relationships. Prevention of harm and avoidance of placing physicians in morally distressing situations are common goals for all involved parties.
Efforts to reduce unnecessary care have predominantly focused on structural and knowledge-based drivers.4,8,9 Our results highlight the presence of additional forces. A majority of physician respondents who reported the presence of VIP services at their hospital also reported that they would be more likely to comply with requests for unnecessary care for a VIP patient as compared to a non-VIP patient. Furthermore, in case-based questions about the requests of a VIP patient and their family for additional unnecessary care, a significant portion of physicians who reported they would comply with these requests listed the VIP status of the patient or family as a factor underlying this decision. Only a minority of physicians reported their decision to provide additional care was the result of their own medically-based concerns. Because these cases were hypothetical and we did not include comparator cases involving non-VIP patients, it remains uncertain whether the observed perceptions accurately reflect real-world differences in the care of VIP and non-VIP patients. Nonetheless, our findings emphasize the importance of better understanding the social drivers of overuse and physician communication strategies related to medically inappropriate tests.10,11
Demand for unnecessary testing may be driven by the mentality that “more is better.”12 Contrary to this belief, provision of unnecessary care can increase the risk of patient harm.13 Despite physician respondents reporting that VIP patients requested and/or received additional unnecessary care, a majority of respondents felt that patient safety for VIP patients was equivalent to that for non-VIP patients. As we assessed only physician perceptions of safety, which may not necessarily correlate with actual safety, further research in this area is needed.
Our study was limited by several factors. While our study population included hospitalists from 8 geographically broad hospitals, including university, safety net, and community hospitals, study responses may not be reflective of nationwide trends. Our response rate may limit our ability to generalize conclusions beyond respondents. Second, our study captured physician perceptions of behavior and safety rather than actually measuring practice and outcomes. Studies comparing physician practice patterns and outcomes between VIP and non-VIP patients would be informative. Additionally, despite our inclusive survey design process, our survey was not validated, and it is possible that our questions were not interpreted as intended. Lastly, despite the anonymous nature of our survey, physicians may have felt compelled to respond in a particular way due to conflicting professional, financial, or social factors.
Our findings provide initial insight into how care for the VIP patient may present unique challenges for physicians, hospitals, and society by systematizing care inequities, as well as potentially incentivizing low-value care practices. Whether these imbalances produce clinical harms or benefits remains worthy of future studies.
Disclosure
Nothing to report.
Recent publications in the medical literature and lay press have stirred controversy regarding the use of inpatient ‘very important person’ (VIP) services.1-3 The term “VIP services” often refers to select conveniences offered in addition to the assumed basic level of care and services provided by a hospital. Examples include additional space, enhanced facilities, specific comforts, or personal support. In some instances, these amenities may only be provided to patients who have close financial, social, or professional relationships with the hospital.
How VIP patients interact with their health system to obtain VIP services has raised unique concerns. Some have speculated that the presence of a VIP patient may be disruptive to the care of non-VIP patients, while others have cautioned physicians about potential dangers to the VIP patients themselves.4-6 Despite much being written on the topics of VIP patients and services in both the lay and academic press, our literature review identified only 1 study on the topic, which cataloged the preferential treatment of VIP patients in the emergency department.6 We are unaware of any investigations of VIP-service use in the inpatient setting. Through a multisite survey of hospital medicine physicians, we assessed physician viewpoints and behavior regarding VIP services.
METHODS
The Hospital Medicine Reengineering Network (HOMERuN) is a nation-wide learning organization focused on measuring and improving the outcomes of hospitalized patients.7 We surveyed hospitalists from 8 HOMERuN hospitals (Appendix 1). The survey instrument contained 4 sections: nonidentifying respondent demographics, local use of VIP services, reported physician perceptions of VIP services, and case-based assessments (Appendix 2). Survey questions and individual cases were developed by study authors and based on real scenarios and concerns provided by front-line clinical providers. Content, length, and reliability of physician understanding were assessed by a 5-person focus group consisting of physicians not included in the survey population.
Subjects were identified via administrative rosters from each HOMERuN site. Surveys were administered via SurveyMonkey, and results were analyzed descriptively. Populations were compared via the Fisher exact test. “VIP services” were defined as conveniences provided in addition to the assumed basic level of care and services (eg, private or luxury-style rooms, access to a special menu, better views, dedicated personal care attendants, hospital liaisons). VIP patients were defined as those patients receiving VIP services. A hospital was identified as providing VIP services if 50% or more of respondents from that site reported the presence of VIP services.
RESULTS
Of 366 hospitalists contacted, 160 completed the survey (44%). Respondent characteristics and reported prevalence of VIP services are demonstrated in Table 1. In total, 78 respondents (45%) reported the presence of VIP services at their hospital. Of the 8 sites surveyed, a majority of physicians at 4 sites (50%) reported presence of VIP services.
Of respondents reporting the presence of VIP services at their hospital, a majority felt that, from a patient safety perspective, the care received by VIP patients was the same as care received by non-VIP patients (Table 2). A majority reported they had felt pressured by a VIP patient or a family member to order additional tests or treatments that the physician believed were medically unnecessary and that they would be more likely to comply with VIP patient’s requests for tests or treatments they felt were unnecessary. More than one-third (36%) felt pressured by other hospital employees or representatives to comply with VIP services patient’s requests for additional tests or treatments that the physicians believed were medically unnecessary.
When presented the case of a VIP patient with community-acquired pneumonia who is clinically stable for discharge but expressing concerns about leaving the hospital, 61 (38%) respondents reported they would not discharge this patient home: 39 of 70 (55.7%) who reported the presence of VIP services at their hospital, and 22 of 91 (24.2%) who reported the absence of VIP services (P < 0.001). Of those who reported they would not discharge this patient home, 37 (61%) reported the reason for this related to the patient’s connection to the Board of Trustees; 48 (79%) reported the reason for this related to the patient’s concerns; 9 (15%) reported the reason for this related to their own concerns regarding medical details of the patient’s case (respondents could select more than 1 reason).
When presented the case of a VIP patient with acute pulmonary embolism who is medically ready for discharge with primary care physician-approved anticoagulation and discharge plans but for whom their family requests additional consultations and inpatient hypercoagulable workup, 33 (21%) respondents reported they would order additional testing and specialist consultation: 17 of 69 (24.6%) who reported the presence of VIP services their hospital, and 16 of 91 (17.6%) who reported the absence of VIP services (P = 0.33). Of those who reported they would order additional testing and specialist consultation, 14 (42%) reported the reason for this related to the family’s financial connections to the hospital; 30 (91%) reported the reason for this related to the family’s concerns; 3 (9%) reported the reason for this related to their own concerns about the medical details of the patient’s case (respondents could select more than 1 reason).
DISCUSSION
In our study, a majority of physicians who reported the presence of VIP services at their hospital felt pressured by VIP patients or their family members to perform unnecessary testing or treatment. While this study was not designed to quantify the burden of unnecessary care for VIP patients, our results have implications for individual patients and public health, including potential effects on resource availability, the identification of clinically irrelevant incidental findings, and short- and long-term medical complications of procedures, testing and radiation exposure.
Prior publications have advocated that physicians and hospitals should not allow VIP status to influence management decisions.3,5 We found that more than one-third of physicians who reported the presence of VIP services at their hospital also reported receiving pressure from hospital representatives to provide care to VIP patients that was not medically indicated. These findings highlight an example of the tension faced by physicians who are caught between patient requests and the delivery of value-based care. This potential conflict may be amplified particularly for those patients with close financial, social, or professional ties to the hospitals (and physicians) providing their care. These results suggest the need for physicians, administrators, and patients to work together to address the potential blurring of ethical boundaries created by VIP relationships. Prevention of harm and avoidance of placing physicians in morally distressing situations are common goals for all involved parties.
Efforts to reduce unnecessary care have predominantly focused on structural and knowledge-based drivers.4,8,9 Our results highlight the presence of additional forces. A majority of physician respondents who reported the presence of VIP services at their hospital also reported that they would be more likely to comply with requests for unnecessary care for a VIP patient as compared to a non-VIP patient. Furthermore, in case-based questions about the requests of a VIP patient and their family for additional unnecessary care, a significant portion of physicians who reported they would comply with these requests listed the VIP status of the patient or family as a factor underlying this decision. Only a minority of physicians reported their decision to provide additional care was the result of their own medically-based concerns. Because these cases were hypothetical and we did not include comparator cases involving non-VIP patients, it remains uncertain whether the observed perceptions accurately reflect real-world differences in the care of VIP and non-VIP patients. Nonetheless, our findings emphasize the importance of better understanding the social drivers of overuse and physician communication strategies related to medically inappropriate tests.10,11
Demand for unnecessary testing may be driven by the mentality that “more is better.”12 Contrary to this belief, provision of unnecessary care can increase the risk of patient harm.13 Despite physician respondents reporting that VIP patients requested and/or received additional unnecessary care, a majority of respondents felt that patient safety for VIP patients was equivalent to that for non-VIP patients. As we assessed only physician perceptions of safety, which may not necessarily correlate with actual safety, further research in this area is needed.
Our study was limited by several factors. While our study population included hospitalists from 8 geographically broad hospitals, including university, safety net, and community hospitals, study responses may not be reflective of nationwide trends. Our response rate may limit our ability to generalize conclusions beyond respondents. Second, our study captured physician perceptions of behavior and safety rather than actually measuring practice and outcomes. Studies comparing physician practice patterns and outcomes between VIP and non-VIP patients would be informative. Additionally, despite our inclusive survey design process, our survey was not validated, and it is possible that our questions were not interpreted as intended. Lastly, despite the anonymous nature of our survey, physicians may have felt compelled to respond in a particular way due to conflicting professional, financial, or social factors.
Our findings provide initial insight into how care for the VIP patient may present unique challenges for physicians, hospitals, and society by systematizing care inequities, as well as potentially incentivizing low-value care practices. Whether these imbalances produce clinical harms or benefits remains worthy of future studies.
Disclosure
Nothing to report.
1. Bernstein N. Chefs, butlers, marble baths: Hospitals vie for the affluent. New York Times. January 21, 2012. http://www.nytimes.com/2012/01/22/nyregion/chefs-butlers-and-marble-baths-not-your-average-hospital-room.html. Accessed February 1, 2017.
2. Kennedy DW, Kagan SH, Abramson KB, Boberick C, Kaiser LR. Academic medicine amenities unit: developing a model to integrate academic medical care with luxury hotel services. Acad Med. 2009;84(2):185-191. PubMed
3. Alfandre D, Clever S, Farber NJ, Hughes MT, Redstone P, Lehmann LS. Caring for ‘very important patients’--ethical dilemmas and suggestions for practical management. Am J Med. 2016;129(2):143-147. PubMed
4. Bulger J, Nickel W, Messler J, et al. Choosing wisely in adult hospital medicine: five opportunities for improved healthcare value. J Hosp Med. 2013;8(9):486-492. PubMed
5. Martin A, Bostic JQ, Pruett K. The V.I.P.: hazard and promise in treating “special” patients. J Am Acad Child Adolesc Psychiatry. 2004;43(3):366-369. PubMed
6. Smally AJ, Carroll B, Carius M, Tilden F, Werdmann M. Treatment of VIPs. Ann Emerg Med. 2011;58(4):397-398. PubMed
7. Auerbach AD, Patel MS, Metlay JP, et al. The hospital medicine reengineering network (HOMERuN): a learning organization focused on improving hospital care. Acad Med. 2014;89(3):415-420. PubMed
8. Caverly TJ, Combs BP, Moriates C, Shah N, Grady D. Too much medicine happens too often: the teachable moment and a call for manuscripts from clinical trainees. JAMA Intern Med. 2014;174(1):8-9. PubMed
9. Schwartz AL, Chernew ME, Landon BE, McWilliams JM. Changes in low-value services in year 1 of the Medicare pioneer accountable care organization program. JAMA Intern Med. 2015;175(11):1815-1825. PubMed
10. Paterniti DA, Fancher TL, Cipri CS, Timmermans S, Heritage J, Kravitz RL. Getting to “no”: strategies primary care physicians use to deny patient requests. Arch Intern Med. 2010;170(4):381-388. PubMed
11. Veroff D, Marr A, Wennberg DE. Enhanced support for shared decision making reduced costs of care for patients with preference-sensitive conditions. Health Aff (Millwood). 2013;32(2):285-293. PubMed
12. Korenstein D. Patient perception of benefits and harms: the Achilles heel of high-value care. JAMA Intern Med. 2015;175(2):287-288. PubMed
13. Moynihan R, Doust J, Henry D. Preventing overdiagnosis: how to stop harming the healthy. BMJ. 2012;344:e3502. PubMed
1. Bernstein N. Chefs, butlers, marble baths: Hospitals vie for the affluent. New York Times. January 21, 2012. http://www.nytimes.com/2012/01/22/nyregion/chefs-butlers-and-marble-baths-not-your-average-hospital-room.html. Accessed February 1, 2017.
2. Kennedy DW, Kagan SH, Abramson KB, Boberick C, Kaiser LR. Academic medicine amenities unit: developing a model to integrate academic medical care with luxury hotel services. Acad Med. 2009;84(2):185-191. PubMed
3. Alfandre D, Clever S, Farber NJ, Hughes MT, Redstone P, Lehmann LS. Caring for ‘very important patients’--ethical dilemmas and suggestions for practical management. Am J Med. 2016;129(2):143-147. PubMed
4. Bulger J, Nickel W, Messler J, et al. Choosing wisely in adult hospital medicine: five opportunities for improved healthcare value. J Hosp Med. 2013;8(9):486-492. PubMed
5. Martin A, Bostic JQ, Pruett K. The V.I.P.: hazard and promise in treating “special” patients. J Am Acad Child Adolesc Psychiatry. 2004;43(3):366-369. PubMed
6. Smally AJ, Carroll B, Carius M, Tilden F, Werdmann M. Treatment of VIPs. Ann Emerg Med. 2011;58(4):397-398. PubMed
7. Auerbach AD, Patel MS, Metlay JP, et al. The hospital medicine reengineering network (HOMERuN): a learning organization focused on improving hospital care. Acad Med. 2014;89(3):415-420. PubMed
8. Caverly TJ, Combs BP, Moriates C, Shah N, Grady D. Too much medicine happens too often: the teachable moment and a call for manuscripts from clinical trainees. JAMA Intern Med. 2014;174(1):8-9. PubMed
9. Schwartz AL, Chernew ME, Landon BE, McWilliams JM. Changes in low-value services in year 1 of the Medicare pioneer accountable care organization program. JAMA Intern Med. 2015;175(11):1815-1825. PubMed
10. Paterniti DA, Fancher TL, Cipri CS, Timmermans S, Heritage J, Kravitz RL. Getting to “no”: strategies primary care physicians use to deny patient requests. Arch Intern Med. 2010;170(4):381-388. PubMed
11. Veroff D, Marr A, Wennberg DE. Enhanced support for shared decision making reduced costs of care for patients with preference-sensitive conditions. Health Aff (Millwood). 2013;32(2):285-293. PubMed
12. Korenstein D. Patient perception of benefits and harms: the Achilles heel of high-value care. JAMA Intern Med. 2015;175(2):287-288. PubMed
13. Moynihan R, Doust J, Henry D. Preventing overdiagnosis: how to stop harming the healthy. BMJ. 2012;344:e3502. PubMed
© 2017 Society of Hospital Medicine
Association Between DCBN and LOS
Slow hospital throughputthe process whereby a patient is admitted, placed in a room, and eventually dischargedcan worsen outcomes if admitted patients are boarded in emergency rooms or postanesthesia units.[1] One potential method to improve throughput is to discharge patients earlier in the day,[2] freeing up available beds and conceivably reducing hospital length of stay (LOS).
To quantify throughput, hospitals are beginning to measure the proportion of patients discharged before noon (DCBN). One study, looking at discharges on a single medical floor in an urban academic medical center, suggested that increasing the percentage of patients discharged by noon decreased observed‐to‐expected LOS in hospitalized medicine patients,[3] and a follow‐up study demonstrated that it was associated with admissions from the emergency department occurring earlier in the day.[4] However, these studies did not adjust for changes in case mix index (CMI) and other patient‐level characteristics that may also have affected these outcomes. Concerns persist that more efforts to discharge patients by noon could inadvertently increase LOS if staff chose to keep patients overnight for an early discharge the following day.
We undertook a retrospective analysis of data from patients discharged from a large academic medical center where an institution‐wide emphasis was placed on discharging more patients by noon. Using these data, we examined the association between discharges before noon and LOS in medical and surgical inpatients.
METHODS
Site and Subjects
Our study was based at the University of California, San Francisco (UCSF) Medical Center, a 400‐bed academic hospital located in San Francisco, California. We examined adult medical and surgical discharges from July 2012 through April 2015. Patients who stayed less than 24 hours or more than 20 days were excluded. Discharges from the hospital medicine service and the following surgical services were included in the analysis: cardiac surgery, colorectal surgery, cardiothoracic surgery, general surgery, gynecologic oncology, gynecology, neurosurgery, orthopedics, otolaryngology, head and neck surgery, plastic surgery, thoracic surgery, urology, and vascular surgery. No exclusions were made based on patient status (eg, observation vs inpatient). UCSF's institutional review board approved our study.
During the time of our study, discharges before noon time became an institutional priority. To this end, rates of DCBN were tracked using retrospective data, and various units undertook efforts such as informal afternoon meetings to prompt planning for the next morning's discharges. These efforts did not differentially affect medical or surgical units or emergent or nonemergent admissions, and no financial incentives or other changes in workflow were in place to increase DCBN rates.
Data Sources
We used the cost accounting system at UCSF (Enterprise Performance System Inc. [EPSI], Chicago, IL) to collect demographic information about each patient, including age, sex, primary race, and primary ethnicity. This system was also used to collect characteristics of each hospitalization including LOS (calculated from admission date time and discharge date time), hospital service at discharge, the discharge attending, discharge disposition of the patient, and the CMI, a marker of the severity of illness of the patient during that hospitalization. EPSI was also used to collect data on the admission type of all patients, either emergent, urgent, or routine, and the insurance status of the patient during that hospitalization.
Data on time of discharge were entered by the discharging nurse or unit assistant to reflect the time the patient left the hospital. Using these data, we defined a before‐noon discharge as one taking place between 8:00 am and 12:00 pm.
Statistical Analysis
Wilcoxon rank sum test and 2 statistics were used to compare baseline characteristics of hospitalizations of patients discharged before and after noon.
We used generalized linear models to assess the association of a discharge before noon on the LOS with gamma models. We accounted for clustering of discharge attendings using generalized estimating equations with exchangeable working correlation and robust standard errors. After the initial unadjusted analyses, covariates were included in the adjusted analysis if they were associated with an LOS at P < 0.05 or reasons of face validity. These variables are shown in Table 1. Because an effort to increase the discharges before noon was started in the 2014 academic year, we added an interaction term between the date of discharge and whether a discharge occurred before noon. The interaction term was included by dividing the study period into time periods corresponding to sequential 6‐month intervals. A new variable was defined by a categorical variable that indicated in which of these time periods a discharge occurred.
Discharged Before Noon | Discharged After Noon | P Value | |
---|---|---|---|
| |||
Median LOS (IQR) | 3.4 (2.25.9) | 3.7 (2.36.3) | <0.0005 |
Median CMI (IQR) | 1.8 (1.12.4) | 1.7 (1.12.5) | 0.006 |
Service type, N (%) | |||
Hospital medicine | 1,919 (29.6) | 11,290 (35.4) | |
Surgical services | 4,565 (70.4) | 20,591 (64.6) | <0.0005 |
Discharged before noon, N (%) | 6,484 (16.9) | 31,881 (83.1) | |
Discharged on weekend, N (%) | |||
Yes | 1,543 (23.8) | 7,411 (23.3) | |
No | 4,941 (76.2) | 24,470 (76.8) | 0.34 |
Discharge disposition, N (%) | |||
Home with home health | 748 (11.5) | 5,774 (18.1) | |
Home without home health | 3,997 (61.6) | 17,862 (56.0) | |
SNF | 837 (12.9) | 3,082 (9.7) | |
Other | 902 (13.9) | 5,163 (16.2) | <0.0005 |
6‐month interval, N (%) | |||
JulyDecember 2012 | 993 (15.3) | 5,596 (17.6) | |
JanuaryJune 2013 | 980 (15.1) | 5,721 (17.9) | |
JulyDecember 2013 | 1,088 (16.8) | 5,690 (17.9) | |
JanuaryJune 2014 | 1,288 (19.9) | 5,441 (17.1) | |
JulyDecember 2014 | 1,275 (19.7) | 5,656 (17.7) | |
JanuaryApril 2015 | 860 (13.3) | 3,777 (11.9) | <0.0005 |
Age category, N (%) | |||
1864 years | 4,177 (64.4) | 20,044 (62.9) | |
65+ years | 2,307 (35.6) | 11,837 (37.1) | 0.02 |
Male, N (%) | 3,274 (50.5) | 15,596 (48.9) | |
Female, N (%) | 3,210 (49.5) | 16,284 (51.1) | 0.06 |
Race, N (%) | |||
White or Caucasian | 4,133 (63.7) | 18,798 (59.0) | |
African American | 518 (8.0) | 3,020 (9.5) | |
Asian | 703 (10.8) | 4,052 (12.7) | |
Other | 1,130 (17.4) | 6,011 (18.9) | <0.0005 |
Ethnicity, N (%) | |||
Hispanic or Latino | 691 (10.7) | 3,713 (11.7) | |
Not Hispanic or Latino | 5,597 (86.3) | 27,209 (85.4) | |
Unknown/declined | 196 (3.0) | 959 (3.0) | 0.07 |
Admission type, N (%) | |||
Elective | 3,494 (53.9) | 13,881 (43.5) | |
Emergency | 2,047 (31.6) | 12,145 (38.1) | |
Urgent | 889 (13.7) | 5,459 (17.1) | |
Other | 54 (0.8) | 396 (1.2) | <0.0005 |
Payor class, N (%) | |||
Medicare | 2,648 (40.8) | 13,808 (43.3) | |
Medi‐Cal | 1,060 (16.4) | 5,913 (18.6) | |
Commercial | 2,633 (40.6) | 11,242 (35.3) | |
Other | 143 (2.2) | 918 (2.9) | <0.0005 |
We conducted a sensitivity analysis using propensity scores. The propensity score was based on demographic and clinical variables (as listed in Table 1) that exhibited P < 0.2 in bivariate analysis between the variable and being discharged before noon. We then used the propensity score as a covariate in a generalized linear model of the LOS with a gamma distribution and with generalized estimating equations as described above.
Finally, we performed prespecified secondary subset analyses of patients admitted emergently and nonemergently.
Statistical modeling and analysis was completed using Stata version 13 (StataCorp, College Station, TX).
RESULTS
Patient Demographics and Discharge Before Noon
Our study population comprised 27,983 patients for a total of 38,365 hospitalizations with a median LOS of 3.7 days. We observed 6484 discharges before noon (16.9%) and 31,881 discharges after noon (83.1%). The characteristics of the hospitalizations are shown in Table 1.
Patients who were discharged before noon tended to be younger, white, and discharged with a disposition to home without home health. The median CMI was slightly higher in discharges before noon (1.81, P = 0.006), and elective admissions were more likely than emergent to be discharged before noon (53.9% vs 31.6%, P < 0.0005).
Multivariable Analysis
A discharge before noon was associated with a 4.3% increase in LOS (adjusted odds ratio [OR]: 1.043, 95% confidence interval [CI]: 1.003‐1.086), adjusting for CMI, the service type, discharge on the weekend, discharge disposition, age, sex, ethnicity, race, urgency of admission, payor class, and a full interaction with the date of discharge (in 6‐month intervals). In preplanned subset analyses, the association between longer LOS and DCBN was more pronounced in patients admitted emergently (adjusted OR: 1.14, 95% CI: 1.033‐1.249) and less pronounced for patients not admitted emergently (adjusted OR: 1.03, 95% CI: 0.988‐1.074), although the latter did not meet statistical significance. In patients admitted emergently, this corresponds to approximately a 12‐hour increase in LOS. The interaction term of discharge date and DCBN was significant in the model. In further subset analyses, the association between longer LOS and DCBN was more pronounced in medicine patients (adjusted OR: 1.116, 95% CI: 1.014‐1.228) than in surgical patients (adjusted OR: 1.030, 95% CI: 0.989‐1.074), although the relationship in surgical patients did not meet statistical significance.
We also undertook sensitivity analyses utilizing propensity scores as a covariate in our base multivariable models. Results from these analyses did not differ from the base models and are not presented here. Results also did not differ when comparing discharges before and after the initiation of an attending only service.
DISCUSSION AND CONCLUSION
In our retrospective study of patients discharged from an academic medical center, discharge before noon was associated with a longer LOS, with the effect more pronounced in patients admitted emergently in the hospital. Our results suggest that efforts to discharge patients earlier in the day may have varying degrees of success depending on patient characteristics. Conceivably, elective admissions recover according to predictable plans, allowing for discharges earlier in the day. In contrast, patients discharged from emergent hospitalizations may have ongoing evolution of their care plan, making plans for discharging before noon more challenging.
Our results differ from a previous study,[3] which suggested that increasing the proportion of before‐noon discharges was associated with a fall in observed‐to‐expected LOS. However, observational studies of DCBN are challenging, because the association between early discharge and LOS is potentially bidirectional. One interpretation, for example, is that patients were kept longer in order to be discharged by noon the following day, which for the subgroups of patients admitted emergently corresponded to a roughly 12‐hour increase in LOS. However, it is also plausible that patients who stayed longer also had more time to plan for an early discharge. In either scenario, the ability of managers to utilize LOS as a key metric of throughput efforts may be flawed, and suggests that alternatives (eg, number of patients waiting for beds off unit) may be a more reasonable measure of throughput. Our results have several limitations. As in any observational study, our results are vulnerable to biases from unmeasured covariates that confound the analysis. We caution that a causal relationship between a discharge before noon and LOS cannot be determined from the nature of the study. Our results are also limited in that we were unable to adjust for day‐to‐day hospital capacity and other variables that affect LOS including caregiver and transportation availability, bed capacity at receiving care facilities, and patient consent to discharge. Finally, as a single‐site study, our findings may not be applicable to nonacademic settings.
In conclusion, our observational study discerned an association between discharging patients before noon and longer LOS. We believe our findings suggest a rationale for alternate approaches to measuring an early discharge program's effectiveness, namely, that the evaluation of the success of an early discharge initiative should consider multiple evaluation metrics including the effect on emergency department wait times, intensive care unit or postanesthesia transitions, and on patient reported experiences of care transitions.
Disclosures
Andrew Auerbach, MD, is supported by a K24 grant from the National Heart, Lung, and Blood Institute: K24HL098372. The authors report no conflicts of interest.
- The effect of emergency department crowding on clinically oriented outcomes. Acad Emerg Med. 2009;16(1):1–10. , , , et al.
- Centers for Medicare 2013.
- Discharge before noon: an achievable hospital goal. J Hosp Med. 2014;9(4):210–214. , , , et al.
- Discharge before noon: effect on throughput and sustainability. J Hosp Med. 2015;10(10):664–669. , , , et al.
Slow hospital throughputthe process whereby a patient is admitted, placed in a room, and eventually dischargedcan worsen outcomes if admitted patients are boarded in emergency rooms or postanesthesia units.[1] One potential method to improve throughput is to discharge patients earlier in the day,[2] freeing up available beds and conceivably reducing hospital length of stay (LOS).
To quantify throughput, hospitals are beginning to measure the proportion of patients discharged before noon (DCBN). One study, looking at discharges on a single medical floor in an urban academic medical center, suggested that increasing the percentage of patients discharged by noon decreased observed‐to‐expected LOS in hospitalized medicine patients,[3] and a follow‐up study demonstrated that it was associated with admissions from the emergency department occurring earlier in the day.[4] However, these studies did not adjust for changes in case mix index (CMI) and other patient‐level characteristics that may also have affected these outcomes. Concerns persist that more efforts to discharge patients by noon could inadvertently increase LOS if staff chose to keep patients overnight for an early discharge the following day.
We undertook a retrospective analysis of data from patients discharged from a large academic medical center where an institution‐wide emphasis was placed on discharging more patients by noon. Using these data, we examined the association between discharges before noon and LOS in medical and surgical inpatients.
METHODS
Site and Subjects
Our study was based at the University of California, San Francisco (UCSF) Medical Center, a 400‐bed academic hospital located in San Francisco, California. We examined adult medical and surgical discharges from July 2012 through April 2015. Patients who stayed less than 24 hours or more than 20 days were excluded. Discharges from the hospital medicine service and the following surgical services were included in the analysis: cardiac surgery, colorectal surgery, cardiothoracic surgery, general surgery, gynecologic oncology, gynecology, neurosurgery, orthopedics, otolaryngology, head and neck surgery, plastic surgery, thoracic surgery, urology, and vascular surgery. No exclusions were made based on patient status (eg, observation vs inpatient). UCSF's institutional review board approved our study.
During the time of our study, discharges before noon time became an institutional priority. To this end, rates of DCBN were tracked using retrospective data, and various units undertook efforts such as informal afternoon meetings to prompt planning for the next morning's discharges. These efforts did not differentially affect medical or surgical units or emergent or nonemergent admissions, and no financial incentives or other changes in workflow were in place to increase DCBN rates.
Data Sources
We used the cost accounting system at UCSF (Enterprise Performance System Inc. [EPSI], Chicago, IL) to collect demographic information about each patient, including age, sex, primary race, and primary ethnicity. This system was also used to collect characteristics of each hospitalization including LOS (calculated from admission date time and discharge date time), hospital service at discharge, the discharge attending, discharge disposition of the patient, and the CMI, a marker of the severity of illness of the patient during that hospitalization. EPSI was also used to collect data on the admission type of all patients, either emergent, urgent, or routine, and the insurance status of the patient during that hospitalization.
Data on time of discharge were entered by the discharging nurse or unit assistant to reflect the time the patient left the hospital. Using these data, we defined a before‐noon discharge as one taking place between 8:00 am and 12:00 pm.
Statistical Analysis
Wilcoxon rank sum test and 2 statistics were used to compare baseline characteristics of hospitalizations of patients discharged before and after noon.
We used generalized linear models to assess the association of a discharge before noon on the LOS with gamma models. We accounted for clustering of discharge attendings using generalized estimating equations with exchangeable working correlation and robust standard errors. After the initial unadjusted analyses, covariates were included in the adjusted analysis if they were associated with an LOS at P < 0.05 or reasons of face validity. These variables are shown in Table 1. Because an effort to increase the discharges before noon was started in the 2014 academic year, we added an interaction term between the date of discharge and whether a discharge occurred before noon. The interaction term was included by dividing the study period into time periods corresponding to sequential 6‐month intervals. A new variable was defined by a categorical variable that indicated in which of these time periods a discharge occurred.
Discharged Before Noon | Discharged After Noon | P Value | |
---|---|---|---|
| |||
Median LOS (IQR) | 3.4 (2.25.9) | 3.7 (2.36.3) | <0.0005 |
Median CMI (IQR) | 1.8 (1.12.4) | 1.7 (1.12.5) | 0.006 |
Service type, N (%) | |||
Hospital medicine | 1,919 (29.6) | 11,290 (35.4) | |
Surgical services | 4,565 (70.4) | 20,591 (64.6) | <0.0005 |
Discharged before noon, N (%) | 6,484 (16.9) | 31,881 (83.1) | |
Discharged on weekend, N (%) | |||
Yes | 1,543 (23.8) | 7,411 (23.3) | |
No | 4,941 (76.2) | 24,470 (76.8) | 0.34 |
Discharge disposition, N (%) | |||
Home with home health | 748 (11.5) | 5,774 (18.1) | |
Home without home health | 3,997 (61.6) | 17,862 (56.0) | |
SNF | 837 (12.9) | 3,082 (9.7) | |
Other | 902 (13.9) | 5,163 (16.2) | <0.0005 |
6‐month interval, N (%) | |||
JulyDecember 2012 | 993 (15.3) | 5,596 (17.6) | |
JanuaryJune 2013 | 980 (15.1) | 5,721 (17.9) | |
JulyDecember 2013 | 1,088 (16.8) | 5,690 (17.9) | |
JanuaryJune 2014 | 1,288 (19.9) | 5,441 (17.1) | |
JulyDecember 2014 | 1,275 (19.7) | 5,656 (17.7) | |
JanuaryApril 2015 | 860 (13.3) | 3,777 (11.9) | <0.0005 |
Age category, N (%) | |||
1864 years | 4,177 (64.4) | 20,044 (62.9) | |
65+ years | 2,307 (35.6) | 11,837 (37.1) | 0.02 |
Male, N (%) | 3,274 (50.5) | 15,596 (48.9) | |
Female, N (%) | 3,210 (49.5) | 16,284 (51.1) | 0.06 |
Race, N (%) | |||
White or Caucasian | 4,133 (63.7) | 18,798 (59.0) | |
African American | 518 (8.0) | 3,020 (9.5) | |
Asian | 703 (10.8) | 4,052 (12.7) | |
Other | 1,130 (17.4) | 6,011 (18.9) | <0.0005 |
Ethnicity, N (%) | |||
Hispanic or Latino | 691 (10.7) | 3,713 (11.7) | |
Not Hispanic or Latino | 5,597 (86.3) | 27,209 (85.4) | |
Unknown/declined | 196 (3.0) | 959 (3.0) | 0.07 |
Admission type, N (%) | |||
Elective | 3,494 (53.9) | 13,881 (43.5) | |
Emergency | 2,047 (31.6) | 12,145 (38.1) | |
Urgent | 889 (13.7) | 5,459 (17.1) | |
Other | 54 (0.8) | 396 (1.2) | <0.0005 |
Payor class, N (%) | |||
Medicare | 2,648 (40.8) | 13,808 (43.3) | |
Medi‐Cal | 1,060 (16.4) | 5,913 (18.6) | |
Commercial | 2,633 (40.6) | 11,242 (35.3) | |
Other | 143 (2.2) | 918 (2.9) | <0.0005 |
We conducted a sensitivity analysis using propensity scores. The propensity score was based on demographic and clinical variables (as listed in Table 1) that exhibited P < 0.2 in bivariate analysis between the variable and being discharged before noon. We then used the propensity score as a covariate in a generalized linear model of the LOS with a gamma distribution and with generalized estimating equations as described above.
Finally, we performed prespecified secondary subset analyses of patients admitted emergently and nonemergently.
Statistical modeling and analysis was completed using Stata version 13 (StataCorp, College Station, TX).
RESULTS
Patient Demographics and Discharge Before Noon
Our study population comprised 27,983 patients for a total of 38,365 hospitalizations with a median LOS of 3.7 days. We observed 6484 discharges before noon (16.9%) and 31,881 discharges after noon (83.1%). The characteristics of the hospitalizations are shown in Table 1.
Patients who were discharged before noon tended to be younger, white, and discharged with a disposition to home without home health. The median CMI was slightly higher in discharges before noon (1.81, P = 0.006), and elective admissions were more likely than emergent to be discharged before noon (53.9% vs 31.6%, P < 0.0005).
Multivariable Analysis
A discharge before noon was associated with a 4.3% increase in LOS (adjusted odds ratio [OR]: 1.043, 95% confidence interval [CI]: 1.003‐1.086), adjusting for CMI, the service type, discharge on the weekend, discharge disposition, age, sex, ethnicity, race, urgency of admission, payor class, and a full interaction with the date of discharge (in 6‐month intervals). In preplanned subset analyses, the association between longer LOS and DCBN was more pronounced in patients admitted emergently (adjusted OR: 1.14, 95% CI: 1.033‐1.249) and less pronounced for patients not admitted emergently (adjusted OR: 1.03, 95% CI: 0.988‐1.074), although the latter did not meet statistical significance. In patients admitted emergently, this corresponds to approximately a 12‐hour increase in LOS. The interaction term of discharge date and DCBN was significant in the model. In further subset analyses, the association between longer LOS and DCBN was more pronounced in medicine patients (adjusted OR: 1.116, 95% CI: 1.014‐1.228) than in surgical patients (adjusted OR: 1.030, 95% CI: 0.989‐1.074), although the relationship in surgical patients did not meet statistical significance.
We also undertook sensitivity analyses utilizing propensity scores as a covariate in our base multivariable models. Results from these analyses did not differ from the base models and are not presented here. Results also did not differ when comparing discharges before and after the initiation of an attending only service.
DISCUSSION AND CONCLUSION
In our retrospective study of patients discharged from an academic medical center, discharge before noon was associated with a longer LOS, with the effect more pronounced in patients admitted emergently in the hospital. Our results suggest that efforts to discharge patients earlier in the day may have varying degrees of success depending on patient characteristics. Conceivably, elective admissions recover according to predictable plans, allowing for discharges earlier in the day. In contrast, patients discharged from emergent hospitalizations may have ongoing evolution of their care plan, making plans for discharging before noon more challenging.
Our results differ from a previous study,[3] which suggested that increasing the proportion of before‐noon discharges was associated with a fall in observed‐to‐expected LOS. However, observational studies of DCBN are challenging, because the association between early discharge and LOS is potentially bidirectional. One interpretation, for example, is that patients were kept longer in order to be discharged by noon the following day, which for the subgroups of patients admitted emergently corresponded to a roughly 12‐hour increase in LOS. However, it is also plausible that patients who stayed longer also had more time to plan for an early discharge. In either scenario, the ability of managers to utilize LOS as a key metric of throughput efforts may be flawed, and suggests that alternatives (eg, number of patients waiting for beds off unit) may be a more reasonable measure of throughput. Our results have several limitations. As in any observational study, our results are vulnerable to biases from unmeasured covariates that confound the analysis. We caution that a causal relationship between a discharge before noon and LOS cannot be determined from the nature of the study. Our results are also limited in that we were unable to adjust for day‐to‐day hospital capacity and other variables that affect LOS including caregiver and transportation availability, bed capacity at receiving care facilities, and patient consent to discharge. Finally, as a single‐site study, our findings may not be applicable to nonacademic settings.
In conclusion, our observational study discerned an association between discharging patients before noon and longer LOS. We believe our findings suggest a rationale for alternate approaches to measuring an early discharge program's effectiveness, namely, that the evaluation of the success of an early discharge initiative should consider multiple evaluation metrics including the effect on emergency department wait times, intensive care unit or postanesthesia transitions, and on patient reported experiences of care transitions.
Disclosures
Andrew Auerbach, MD, is supported by a K24 grant from the National Heart, Lung, and Blood Institute: K24HL098372. The authors report no conflicts of interest.
Slow hospital throughputthe process whereby a patient is admitted, placed in a room, and eventually dischargedcan worsen outcomes if admitted patients are boarded in emergency rooms or postanesthesia units.[1] One potential method to improve throughput is to discharge patients earlier in the day,[2] freeing up available beds and conceivably reducing hospital length of stay (LOS).
To quantify throughput, hospitals are beginning to measure the proportion of patients discharged before noon (DCBN). One study, looking at discharges on a single medical floor in an urban academic medical center, suggested that increasing the percentage of patients discharged by noon decreased observed‐to‐expected LOS in hospitalized medicine patients,[3] and a follow‐up study demonstrated that it was associated with admissions from the emergency department occurring earlier in the day.[4] However, these studies did not adjust for changes in case mix index (CMI) and other patient‐level characteristics that may also have affected these outcomes. Concerns persist that more efforts to discharge patients by noon could inadvertently increase LOS if staff chose to keep patients overnight for an early discharge the following day.
We undertook a retrospective analysis of data from patients discharged from a large academic medical center where an institution‐wide emphasis was placed on discharging more patients by noon. Using these data, we examined the association between discharges before noon and LOS in medical and surgical inpatients.
METHODS
Site and Subjects
Our study was based at the University of California, San Francisco (UCSF) Medical Center, a 400‐bed academic hospital located in San Francisco, California. We examined adult medical and surgical discharges from July 2012 through April 2015. Patients who stayed less than 24 hours or more than 20 days were excluded. Discharges from the hospital medicine service and the following surgical services were included in the analysis: cardiac surgery, colorectal surgery, cardiothoracic surgery, general surgery, gynecologic oncology, gynecology, neurosurgery, orthopedics, otolaryngology, head and neck surgery, plastic surgery, thoracic surgery, urology, and vascular surgery. No exclusions were made based on patient status (eg, observation vs inpatient). UCSF's institutional review board approved our study.
During the time of our study, discharges before noon time became an institutional priority. To this end, rates of DCBN were tracked using retrospective data, and various units undertook efforts such as informal afternoon meetings to prompt planning for the next morning's discharges. These efforts did not differentially affect medical or surgical units or emergent or nonemergent admissions, and no financial incentives or other changes in workflow were in place to increase DCBN rates.
Data Sources
We used the cost accounting system at UCSF (Enterprise Performance System Inc. [EPSI], Chicago, IL) to collect demographic information about each patient, including age, sex, primary race, and primary ethnicity. This system was also used to collect characteristics of each hospitalization including LOS (calculated from admission date time and discharge date time), hospital service at discharge, the discharge attending, discharge disposition of the patient, and the CMI, a marker of the severity of illness of the patient during that hospitalization. EPSI was also used to collect data on the admission type of all patients, either emergent, urgent, or routine, and the insurance status of the patient during that hospitalization.
Data on time of discharge were entered by the discharging nurse or unit assistant to reflect the time the patient left the hospital. Using these data, we defined a before‐noon discharge as one taking place between 8:00 am and 12:00 pm.
Statistical Analysis
Wilcoxon rank sum test and 2 statistics were used to compare baseline characteristics of hospitalizations of patients discharged before and after noon.
We used generalized linear models to assess the association of a discharge before noon on the LOS with gamma models. We accounted for clustering of discharge attendings using generalized estimating equations with exchangeable working correlation and robust standard errors. After the initial unadjusted analyses, covariates were included in the adjusted analysis if they were associated with an LOS at P < 0.05 or reasons of face validity. These variables are shown in Table 1. Because an effort to increase the discharges before noon was started in the 2014 academic year, we added an interaction term between the date of discharge and whether a discharge occurred before noon. The interaction term was included by dividing the study period into time periods corresponding to sequential 6‐month intervals. A new variable was defined by a categorical variable that indicated in which of these time periods a discharge occurred.
Discharged Before Noon | Discharged After Noon | P Value | |
---|---|---|---|
| |||
Median LOS (IQR) | 3.4 (2.25.9) | 3.7 (2.36.3) | <0.0005 |
Median CMI (IQR) | 1.8 (1.12.4) | 1.7 (1.12.5) | 0.006 |
Service type, N (%) | |||
Hospital medicine | 1,919 (29.6) | 11,290 (35.4) | |
Surgical services | 4,565 (70.4) | 20,591 (64.6) | <0.0005 |
Discharged before noon, N (%) | 6,484 (16.9) | 31,881 (83.1) | |
Discharged on weekend, N (%) | |||
Yes | 1,543 (23.8) | 7,411 (23.3) | |
No | 4,941 (76.2) | 24,470 (76.8) | 0.34 |
Discharge disposition, N (%) | |||
Home with home health | 748 (11.5) | 5,774 (18.1) | |
Home without home health | 3,997 (61.6) | 17,862 (56.0) | |
SNF | 837 (12.9) | 3,082 (9.7) | |
Other | 902 (13.9) | 5,163 (16.2) | <0.0005 |
6‐month interval, N (%) | |||
JulyDecember 2012 | 993 (15.3) | 5,596 (17.6) | |
JanuaryJune 2013 | 980 (15.1) | 5,721 (17.9) | |
JulyDecember 2013 | 1,088 (16.8) | 5,690 (17.9) | |
JanuaryJune 2014 | 1,288 (19.9) | 5,441 (17.1) | |
JulyDecember 2014 | 1,275 (19.7) | 5,656 (17.7) | |
JanuaryApril 2015 | 860 (13.3) | 3,777 (11.9) | <0.0005 |
Age category, N (%) | |||
1864 years | 4,177 (64.4) | 20,044 (62.9) | |
65+ years | 2,307 (35.6) | 11,837 (37.1) | 0.02 |
Male, N (%) | 3,274 (50.5) | 15,596 (48.9) | |
Female, N (%) | 3,210 (49.5) | 16,284 (51.1) | 0.06 |
Race, N (%) | |||
White or Caucasian | 4,133 (63.7) | 18,798 (59.0) | |
African American | 518 (8.0) | 3,020 (9.5) | |
Asian | 703 (10.8) | 4,052 (12.7) | |
Other | 1,130 (17.4) | 6,011 (18.9) | <0.0005 |
Ethnicity, N (%) | |||
Hispanic or Latino | 691 (10.7) | 3,713 (11.7) | |
Not Hispanic or Latino | 5,597 (86.3) | 27,209 (85.4) | |
Unknown/declined | 196 (3.0) | 959 (3.0) | 0.07 |
Admission type, N (%) | |||
Elective | 3,494 (53.9) | 13,881 (43.5) | |
Emergency | 2,047 (31.6) | 12,145 (38.1) | |
Urgent | 889 (13.7) | 5,459 (17.1) | |
Other | 54 (0.8) | 396 (1.2) | <0.0005 |
Payor class, N (%) | |||
Medicare | 2,648 (40.8) | 13,808 (43.3) | |
Medi‐Cal | 1,060 (16.4) | 5,913 (18.6) | |
Commercial | 2,633 (40.6) | 11,242 (35.3) | |
Other | 143 (2.2) | 918 (2.9) | <0.0005 |
We conducted a sensitivity analysis using propensity scores. The propensity score was based on demographic and clinical variables (as listed in Table 1) that exhibited P < 0.2 in bivariate analysis between the variable and being discharged before noon. We then used the propensity score as a covariate in a generalized linear model of the LOS with a gamma distribution and with generalized estimating equations as described above.
Finally, we performed prespecified secondary subset analyses of patients admitted emergently and nonemergently.
Statistical modeling and analysis was completed using Stata version 13 (StataCorp, College Station, TX).
RESULTS
Patient Demographics and Discharge Before Noon
Our study population comprised 27,983 patients for a total of 38,365 hospitalizations with a median LOS of 3.7 days. We observed 6484 discharges before noon (16.9%) and 31,881 discharges after noon (83.1%). The characteristics of the hospitalizations are shown in Table 1.
Patients who were discharged before noon tended to be younger, white, and discharged with a disposition to home without home health. The median CMI was slightly higher in discharges before noon (1.81, P = 0.006), and elective admissions were more likely than emergent to be discharged before noon (53.9% vs 31.6%, P < 0.0005).
Multivariable Analysis
A discharge before noon was associated with a 4.3% increase in LOS (adjusted odds ratio [OR]: 1.043, 95% confidence interval [CI]: 1.003‐1.086), adjusting for CMI, the service type, discharge on the weekend, discharge disposition, age, sex, ethnicity, race, urgency of admission, payor class, and a full interaction with the date of discharge (in 6‐month intervals). In preplanned subset analyses, the association between longer LOS and DCBN was more pronounced in patients admitted emergently (adjusted OR: 1.14, 95% CI: 1.033‐1.249) and less pronounced for patients not admitted emergently (adjusted OR: 1.03, 95% CI: 0.988‐1.074), although the latter did not meet statistical significance. In patients admitted emergently, this corresponds to approximately a 12‐hour increase in LOS. The interaction term of discharge date and DCBN was significant in the model. In further subset analyses, the association between longer LOS and DCBN was more pronounced in medicine patients (adjusted OR: 1.116, 95% CI: 1.014‐1.228) than in surgical patients (adjusted OR: 1.030, 95% CI: 0.989‐1.074), although the relationship in surgical patients did not meet statistical significance.
We also undertook sensitivity analyses utilizing propensity scores as a covariate in our base multivariable models. Results from these analyses did not differ from the base models and are not presented here. Results also did not differ when comparing discharges before and after the initiation of an attending only service.
DISCUSSION AND CONCLUSION
In our retrospective study of patients discharged from an academic medical center, discharge before noon was associated with a longer LOS, with the effect more pronounced in patients admitted emergently in the hospital. Our results suggest that efforts to discharge patients earlier in the day may have varying degrees of success depending on patient characteristics. Conceivably, elective admissions recover according to predictable plans, allowing for discharges earlier in the day. In contrast, patients discharged from emergent hospitalizations may have ongoing evolution of their care plan, making plans for discharging before noon more challenging.
Our results differ from a previous study,[3] which suggested that increasing the proportion of before‐noon discharges was associated with a fall in observed‐to‐expected LOS. However, observational studies of DCBN are challenging, because the association between early discharge and LOS is potentially bidirectional. One interpretation, for example, is that patients were kept longer in order to be discharged by noon the following day, which for the subgroups of patients admitted emergently corresponded to a roughly 12‐hour increase in LOS. However, it is also plausible that patients who stayed longer also had more time to plan for an early discharge. In either scenario, the ability of managers to utilize LOS as a key metric of throughput efforts may be flawed, and suggests that alternatives (eg, number of patients waiting for beds off unit) may be a more reasonable measure of throughput. Our results have several limitations. As in any observational study, our results are vulnerable to biases from unmeasured covariates that confound the analysis. We caution that a causal relationship between a discharge before noon and LOS cannot be determined from the nature of the study. Our results are also limited in that we were unable to adjust for day‐to‐day hospital capacity and other variables that affect LOS including caregiver and transportation availability, bed capacity at receiving care facilities, and patient consent to discharge. Finally, as a single‐site study, our findings may not be applicable to nonacademic settings.
In conclusion, our observational study discerned an association between discharging patients before noon and longer LOS. We believe our findings suggest a rationale for alternate approaches to measuring an early discharge program's effectiveness, namely, that the evaluation of the success of an early discharge initiative should consider multiple evaluation metrics including the effect on emergency department wait times, intensive care unit or postanesthesia transitions, and on patient reported experiences of care transitions.
Disclosures
Andrew Auerbach, MD, is supported by a K24 grant from the National Heart, Lung, and Blood Institute: K24HL098372. The authors report no conflicts of interest.
- The effect of emergency department crowding on clinically oriented outcomes. Acad Emerg Med. 2009;16(1):1–10. , , , et al.
- Centers for Medicare 2013.
- Discharge before noon: an achievable hospital goal. J Hosp Med. 2014;9(4):210–214. , , , et al.
- Discharge before noon: effect on throughput and sustainability. J Hosp Med. 2015;10(10):664–669. , , , et al.
- The effect of emergency department crowding on clinically oriented outcomes. Acad Emerg Med. 2009;16(1):1–10. , , , et al.
- Centers for Medicare 2013.
- Discharge before noon: an achievable hospital goal. J Hosp Med. 2014;9(4):210–214. , , , et al.
- Discharge before noon: effect on throughput and sustainability. J Hosp Med. 2015;10(10):664–669. , , , et al.
© 2015 Society of Hospital Medicine
Ordering Patterns in Shift‐Based Care
Duty‐hour restrictions were implemented by the Accreditation Council for Graduate Medical Education (ACGME) in 2003 in response to data showing that sleep deprivation was correlated with serious medical errors.[1] In 2011, the ACGME required more explicit restrictions in the number of hours worked and the maximal shift length.[2] These requirements have necessitated a transition from a traditional q4 call model for interns to one in which shifts are limited to a maximum of 16 hours.
Studies of interns working these shorter shifts have had varied results, and comprehensive reviews have failed to demonstrate consistent improvements.[3, 4, 5] Studies of shift‐length limitation initially suggested improvements in patient safety (decreased length of stay,[6, 7] cost of hospitalization,[6] medication errors,[7] serious medical errors,[8] and intensive care unit [ICU] admissions[9]) and resident quality of life.[10] However, other recent studies have reported an increased number of self‐reported medical errors[11] and either did not detect change[12] or reported perceived decreases[13] in quality of care and continuity of care.
We previously reported decreased length of stay and decreased cost of hospitalization in pediatric inpatients cared for in a day/night‐shiftbased care model.[6] An hypothesized reason for those care improvements is the restructured care model led to increased active clinical management during both day and night hours. Here we report the findings of a retrospective analysis to investigate this hypothesis.
PATIENTS AND METHODS
Study Population
We reviewed the charts of pediatric patients admitted to University of California, San Francisco Benioff Children's Hospital, a 175‐bed tertiary care facility, over a 2‐year period between September 15, 2007 and September 15, 2008 (preintervention) and September 16, 2008 and September 16, 2009 (postintervention). During this study period, our hospital was still dependent on paper orders. Admission order sets were preprinted paper forms that were unchanged for the study period. Using International Classification of Diseases, 9th Revision coding, we identified patients on the general pediatrics service with 1 of 6 common diagnosesdehydration, community‐acquired pneumonia, aspiration pneumonia, upper respiratory infection, asthma, and bronchiolitis. These diagnoses were chosen because it was hypothesized that their length of inpatient stay could be impacted by active clinical management. We excluded patients admitted to the ICU or transferred between services.
A list of medical record numbers (MRNs) corresponding to admissions for 1 of the 6 above diagnoses during the pre‐ and postintervention periods was compiled. MRNs were randomized and then sequentially reviewed until 50 admissions in each time period were obtained. After data collection was completed, we noted that 2 patients had been in the ICU for part of their hospitalization, and these were excluded, leaving 48 admissions from prior to the intervention and 50 admissions from after intervention who were examined.
Intervention
During the preintervention period, patients were cared for by interns who took call every sixth night (duty periods up to 30 hours), with cross‐coverage of patients on multiple teams. Cross‐coverage was defined as coverage of patients cared for during nonconsecutive shifts and for whom residents did not participate in attending rounds. Noncall shifts were typically 10 to 11 hours. They were supervised by senior residents who took call every fourth or fifth night and who provided similar cross‐coverage.
During the postintervention period, interns worked day and night shifts of 13 hours (1 hour overlap time between shifts for handoffs), with increased night staffing to eliminate intern‐level cross‐coverage of multiple teams and maintain interns as the primary providers. Interns covered the same team for 5 to 7 consecutive days on either the day or night shifts. Interns remained on the same teams when they switched from day shifts to night shifts to preserve continuity. There were some 24‐hour shifts for senior residents on weekends. Senior residents maintained supervisory responsibility for all patients (both hospitalist teams and a subspecialty team). They also worked 7 consecutive nights.
There were changes in the staffing ratios associated with the change to day and night teams (Table 1, Figure 1). In the preintervention period, general pediatrics patients were covered by a single hospitalist and cohorted on a single team (team A), which also covered several groups of subspecialty patients with subspecialty attendings. The team consisted of 2 interns and 1 senior resident, who shared extended (30‐hour) call in a cycle with 2 other inpatient teams. In the postintervention period, general pediatrics patients were split between 2 teams (teams D and E) and mixed with subspecialty patients. Hospitalist continued to be the attendings, and these hospitalists also covered specialty patients with subspecialists in consulting roles. The teams consisted of 3 interns on the day shift, and 1 on the night shift. There was 1 senior resident per team on day shift, and a single senior resident covering all teams at night.
Preintervention | Postintervention | |||||
---|---|---|---|---|---|---|
| ||||||
General Pediatrics | Team A | Team B | Team C | Team D | Team E | Team F |
Patient Distribution | General Pediatrics | GI/Liver | Renal | General Pediatrics | General Pediatrics | Liver |
Pulmonary | Neurology | Rheumatology | Mixed Specialty | Mixed Specialty | Renal | |
Adolescent | Endocrine | |||||
Team membersa | 2 interns (q6 call) | 4 interns (3 on day shift/1 on night shift) | ||||
1 senior resident (q5 call) | 1 senior resident | |||||
Night‐shift coveragea | 1 intern and 1 senior resident together covered all 3 teams. | 1 night intern per team (teams D/E) working 7 consecutive night shifts | ||||
1 supervising night resident covering all 3 teams | ||||||
Intern cross‐coverage of other teams | Nights/clinic afternoons | None | ||||
Length of night shift | 30 hours | 13 hours |
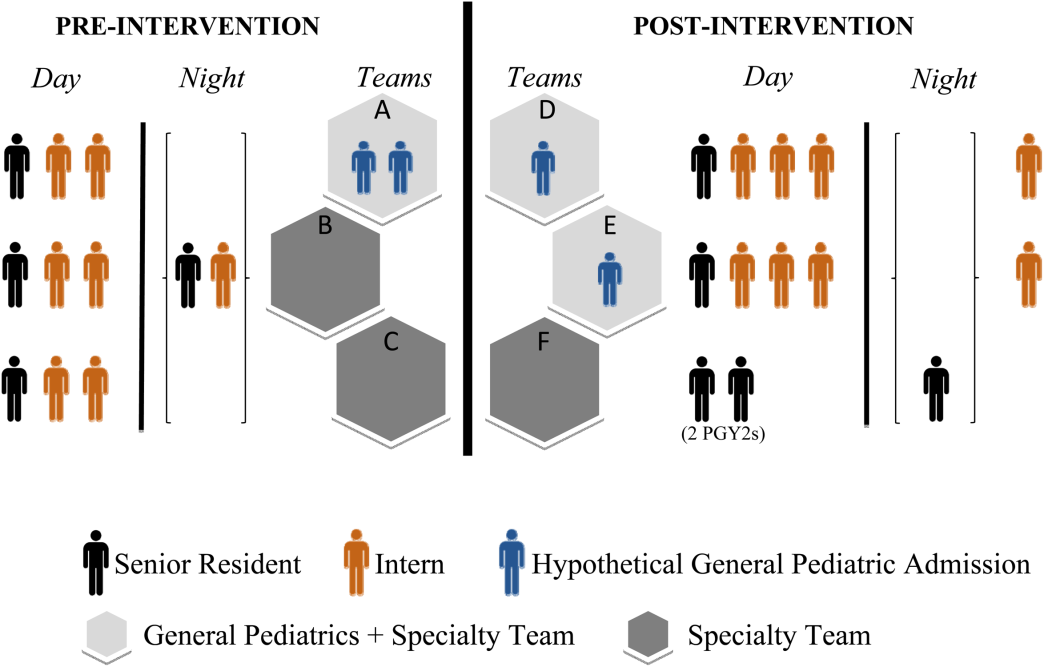
There was no change in the paper‐order system, the electronic health record, timing of the morning blood draw, use of new facilities for patient care, or protocol for emergency department admission. Concomitant with the restructuring, most subspecialty patients were consolidated onto the hospitalist service, necessitating creation of a second hospitalist team. However, patients admitted with the diagnoses identified above would have been on the hospitalist service before and after the restructuring.
Data Collection/Analysis
We reviewed specific classes of orders and categorized by type: respiratory medication, oxygen, intravenous (IV) fluids, diet, monitoring, and activity, time of day (day vs night‐shift), and whether they were an escalation or de‐escalation of care. De‐escalation of care was defined as orders that decreased patient care such as weaning a patient off nebulized albuterol or decreasing their IV fluids. Orders between 07:00 to 18:00 were considered day‐shift orders and between 18:01 and 06:59 were classified as night‐shift orders. Only orders falling into 1 of the aforementioned categories were recorded. Admission order sets were not included. Initially, charts were reviewed by both investigators together; after comparing results for 10 charts to ensure consistency of methodology and criteria, the remaining charts were reviewed by 1 of the study investigators.
To compare demographics, diagnoses, and ordering patterns, t tests and 2 (SAS version 9.2 [SAS Institute, Cary, NC], Stata version 13.1 [StataCorp, College Station, TX]) were used. Multivariate gamma models (SAS version 9.2 [SAS Institute]) that adjusted for clustering at the attending level and patient age were used to compare severity of illness before and after the intervention. This study was approved by the University of California, San Francisco Committee on Human Research.
RESULTS
We analyzed data for 48 admissions preintervention and 50 postintervention. With the exception of insurance type, there was no difference in baseline demographics, diagnoses, or severity of illness between the groups (Table 2). Within the order classes above, we identified 212 orders preintervention and 231 orders postintervention.
Preintervention,n = 48, N (%) | Postintervention, n = 50, N (%) | P Value | |
---|---|---|---|
| |||
Age, y, mean (SD) | 4.8 (4.6) | 5.5 (4.7) | 0.4474 |
Race/ethnicity | 0.1953 | ||
NH white | 12 (25.0%) | 9 (18.0%) | |
NH black | 11 (22.9%) | 7 (14.0%) | |
Hispanic | 16 (33.3%) | 13 (26.0%) | |
Asian | 6 (12.5%) | 10 (20.0%) | |
Other | 3 (6.3%) | 10 (20.0%) | |
Missing | 0 | 1 (2.0%) | |
Gender | 0.6577 | ||
Female | 19 (39.6%) | 22 (44.0%) | |
Male | 29 (60.4%) | 28 (56.0%) | |
Primary language | 0.2601 | ||
English | 38 (79.2%) | 45 (90.0%) | |
Spanish | 9 (18.8%) | 5 (10.0%) | |
Other | 1 (2.1%) | 0 | |
Insurance | 0.0118 | ||
Private | 13 (27.1%) | 26 (52.0%) | |
Medical | 35 (72.9%) | 24 (48.0%) | |
Other | 0 | 0 | |
Admit source | 0.6581 | ||
Referral | 20 (41.7%) | 18 (36.0%) | |
ED | 26 (54.2%) | 31 (62.0%) | |
Transfer | 2 (4.2%) | 1 (2.0%) | |
Severity of illness | 0.1926 | ||
Minor | 15 (31.3%) | 24 (48.0%) | |
Moderate | 23 (47.9%) | 16 (32.0%) | |
Severe | 10 (20.8%) | 10 (20.0%) | |
Extreme | 0 | 0 | |
Diagnoses | 0.562 | ||
Asthma | 21 | 19 | |
Bronchiolitis | 2 | 4 | |
Pneumonia | 17 | 19 | |
Dehydration | 6 | 7 | |
URI | 0 | 1 | |
Aspiration pneumonia | 2 | 0 |
After the intervention, there was a statistically significant increase in the average number of orders written within the first 12 hours (pre: 0.58 orders vs post: 1.12, P = 0.009) and 24 hours (pre: 1.52 vs post: 2.38, P = 0.004) following admission (Table 3), not including the admission order set. The fraction of orders written at night was not significantly different (27% at night preintervention, 33% postintervention, P = 0.149). The fraction of admissions on the day shift compared to the night shift did not change (P = 0.72). There was no difference in the ratio of de‐escalation to escalation orders written during the night (Table 2).
Preintervention, 48 Admissions | Postintervention, 50 Admissions | P Value | |
---|---|---|---|
| |||
Total no. of orders | 212 | 231 | |
Mean no. of orders per admission | 4.42 | 4.62 | |
Day shift orders, n (%) | 155 (73) | 155 (67) | 0.149 |
Night shift orders, n (%) | 57 (27) | 76 (33) | |
Mean no. of orders within first 12 hours* | 0.58 | 1.12 | 0.009 |
Mean no. of orders within first 24 hours* | 1.52 | 2.38 | 0.004 |
Night shift escalation orders (%) | 27 (47) | 33 (43) | 0.491 |
Night shift de‐escalation orders (%) | 30 (53) | 43 (57) |
DISCUSSION
In this study, we demonstrate increased patient care management early in the hospitalization, measured in this study by the mean number of orders written per patient in the first 12 and 24 hours after admission, after transition from a call schedule with extended (>16 hours) shifts to one with shorter shifts compliant with current ACGME duty‐hour restrictions and an explicit focus on greater continuity of care. We did not detect a change in the proportion of total orders written on the night shift compared to the day shift. Earlier active medical management, such as weaning nebulized albuterol or supplemental oxygen, can speed the time to discharge.[14]
Our failure to detect a significant change in the proportion or type of orders written at night may have been due to our small sample size. Anecdotally, after the intervention, medical students reported to us that they noticed a difference between our service, in which we expect night teams to advance care, and other services at our institution, in which nights are a time to focus on putting out fires. This was not something that had been reported to us prior. It is likely reflective of the overall approach to patient care taken by residents working a night shift as part of a longitudinal care team.
This study builds on previous findings that demonstrated lower costs and shorter length of stay after implementing a schedule based on day and night teams.[7] The reasons for such improvements are likely multifactorial. In our model, which was purposefully designed to create night‐team continuity and minimize cross‐coverage, it is likely that residents also felt a greater sense of responsibility for and familiarity with the patients[15] and therefore felt more comfortable advancing care. Not only were interns likely better rested, the patient‐to‐provider ratio was also lower than in the preintervention model. Increases in staffing are often necessary to eliminate cross‐coverage while maintaining safe, 24‐hour care. These findings suggest that increases in cost from additional staffing may be at least partially offset by more active patient management early in the hospitalization, which has the potential to lead to shorter hospital stays.
There are several limitations to our research. We studied a small sample, including a subset of general pediatrics diagnoses that are amenable to active management, limiting generalizability. We did not calculate a physician‐to‐patient ratio because this was not possible with the retrospective data we collected. Staffing ratios likely improved, and we consider that part of the overall improvements in staffing that may have contributed to the observed changes in ordering patterns. Although intern‐level cross‐coverage was eliminated, the senior resident continued to cover multiple teams overnight. This senior covered the same 3 teams for 7 consecutive nights. The addition of a hospitalist team, with subspecialists being placed in consultant roles, may have contributed to the increase in active management, though our study population did not include subspecialty patients. There was a difference in insurance status between the 2 groups. This was unlikely to affect resident physician practices as insurance information is not routinely discussed in the course of patient care. In the context of the ongoing debate about duty‐hour restrictions, it will be important for future studies to elucidate whether sleep or other variables are the primary contributors to this finding. Our data are derived solely from 1 inpatient service at a single academic medical center; however, we do feel there are lessons that may be applied to other settings.
CONCLUSION
A coverage system with improved nighttime resident coverage was associated with a greater number of orders written early in the hospitalization, suggesting more active management of clinical problems to advance care.
Acknowledgements
The authors thank Dr. I. Elaine Allen, John Kornak, and Dr. Derek Pappas for assistance with biostatistics, and Dr. Diana Bojorquez and Dr. Derek Pappas for assistance with review of the manuscript and creation of the figures.
Disclosures: None of the authors have financial relationships or other conflicts of interest to disclose. No external funding was secured for this study. Dr. Auerbach was supported by grant K24HL098372 during the course of this study. This project was supported by the National Center for Advancing Translational Sciences, National Institutes of Health (NIH), through University of California San FranciscoClinical and Translational Sciences Institute grant UL1 TR000004. Its contents are solely the responsibility of the authors and do not necessarily represent the official views of the NIH. Dr. Rosenbluth had access to all of the data in the study and takes responsibility for the integrity of the data and the accuracy of the data analysis.
- New requirements for resident duty hours. JAMA. 2002;288(9):1112–1114. , , .
- Accreditation Council for Graduate Medical Education. Common program requirements. 2011. Available at: http://www.acgme.org/acgmeweb/Portals/0/PDFs/Common_Program_Requirements_07012011[2].pdf. Accessed November 28, 2011.
- Patient safety, resident education and resident well‐being following implementation of the 2003 ACGME duty hour rules. J Gen Intern Med. 2011;26(8):907–919. , , .
- A systematic review of the effects of resident duty hour restrictions in surgery: impact on resident wellness, training, and patient outcomes. Ann Surg. 2014;259(6):1041–1053. , , , et al.
- Duty‐hour limits and patient care and resident outcomes: can high‐quality studies offer insight into complex relationships? Annu Rev Med. 2013;64:467–483. , , , .
- Association between adaptations to ACGME duty hour requirements, length of stay, and costs. Sleep. 2013;36(2):245–248. , , , , , .
- Effect of a change in house staff work schedule on resource utilization and patient care. Arch Intern Med. 1991;151(10):2065–2070. , , , .
- Effect of reducing interns' work hours on serious medical errors in intensive care units. N Engl J Med. 2004;351(18):1838–1848. , , , et al.
- Changes in outcomes for internal medicine inpatients after work‐hour regulations. Ann Intern Med. 2007;147(2):97–103. , , , .
- Effects of reducing or eliminating resident work shifts over 16 hours: a systematic review. Sleep. 2010;33(8):1043–1053. , , .
- Effects of the 2011 duty hour reforms on interns and their patients: a prospective longitudinal cohort study. JAMA Intern Med. 2013;173(8):657–662; discussion 663. , , , et al.
- Effect of 16‐hour duty periods on patient care and resident education. Mayo Clin Proc. 2011;86(3):192–196. , , , , .
- Effect of the 2011 vs 2003 duty hour regulation‐compliant models on sleep duration, trainee education, and continuity of patient care among internal medicine house staff: a randomized trial. JAMA Intern Med. 2013;173(8):649–655. , , , et al.
- Effectiveness of a clinical pathway for inpatient asthma management. Pediatrics. 2000;106(5):1006–1012. , , , .
- Resident perceptions of autonomy in a complex tertiary care environment improve when supervised by hospitalists. Hosp Pediatr. 2012;2(4):228–234. , , , .
Duty‐hour restrictions were implemented by the Accreditation Council for Graduate Medical Education (ACGME) in 2003 in response to data showing that sleep deprivation was correlated with serious medical errors.[1] In 2011, the ACGME required more explicit restrictions in the number of hours worked and the maximal shift length.[2] These requirements have necessitated a transition from a traditional q4 call model for interns to one in which shifts are limited to a maximum of 16 hours.
Studies of interns working these shorter shifts have had varied results, and comprehensive reviews have failed to demonstrate consistent improvements.[3, 4, 5] Studies of shift‐length limitation initially suggested improvements in patient safety (decreased length of stay,[6, 7] cost of hospitalization,[6] medication errors,[7] serious medical errors,[8] and intensive care unit [ICU] admissions[9]) and resident quality of life.[10] However, other recent studies have reported an increased number of self‐reported medical errors[11] and either did not detect change[12] or reported perceived decreases[13] in quality of care and continuity of care.
We previously reported decreased length of stay and decreased cost of hospitalization in pediatric inpatients cared for in a day/night‐shiftbased care model.[6] An hypothesized reason for those care improvements is the restructured care model led to increased active clinical management during both day and night hours. Here we report the findings of a retrospective analysis to investigate this hypothesis.
PATIENTS AND METHODS
Study Population
We reviewed the charts of pediatric patients admitted to University of California, San Francisco Benioff Children's Hospital, a 175‐bed tertiary care facility, over a 2‐year period between September 15, 2007 and September 15, 2008 (preintervention) and September 16, 2008 and September 16, 2009 (postintervention). During this study period, our hospital was still dependent on paper orders. Admission order sets were preprinted paper forms that were unchanged for the study period. Using International Classification of Diseases, 9th Revision coding, we identified patients on the general pediatrics service with 1 of 6 common diagnosesdehydration, community‐acquired pneumonia, aspiration pneumonia, upper respiratory infection, asthma, and bronchiolitis. These diagnoses were chosen because it was hypothesized that their length of inpatient stay could be impacted by active clinical management. We excluded patients admitted to the ICU or transferred between services.
A list of medical record numbers (MRNs) corresponding to admissions for 1 of the 6 above diagnoses during the pre‐ and postintervention periods was compiled. MRNs were randomized and then sequentially reviewed until 50 admissions in each time period were obtained. After data collection was completed, we noted that 2 patients had been in the ICU for part of their hospitalization, and these were excluded, leaving 48 admissions from prior to the intervention and 50 admissions from after intervention who were examined.
Intervention
During the preintervention period, patients were cared for by interns who took call every sixth night (duty periods up to 30 hours), with cross‐coverage of patients on multiple teams. Cross‐coverage was defined as coverage of patients cared for during nonconsecutive shifts and for whom residents did not participate in attending rounds. Noncall shifts were typically 10 to 11 hours. They were supervised by senior residents who took call every fourth or fifth night and who provided similar cross‐coverage.
During the postintervention period, interns worked day and night shifts of 13 hours (1 hour overlap time between shifts for handoffs), with increased night staffing to eliminate intern‐level cross‐coverage of multiple teams and maintain interns as the primary providers. Interns covered the same team for 5 to 7 consecutive days on either the day or night shifts. Interns remained on the same teams when they switched from day shifts to night shifts to preserve continuity. There were some 24‐hour shifts for senior residents on weekends. Senior residents maintained supervisory responsibility for all patients (both hospitalist teams and a subspecialty team). They also worked 7 consecutive nights.
There were changes in the staffing ratios associated with the change to day and night teams (Table 1, Figure 1). In the preintervention period, general pediatrics patients were covered by a single hospitalist and cohorted on a single team (team A), which also covered several groups of subspecialty patients with subspecialty attendings. The team consisted of 2 interns and 1 senior resident, who shared extended (30‐hour) call in a cycle with 2 other inpatient teams. In the postintervention period, general pediatrics patients were split between 2 teams (teams D and E) and mixed with subspecialty patients. Hospitalist continued to be the attendings, and these hospitalists also covered specialty patients with subspecialists in consulting roles. The teams consisted of 3 interns on the day shift, and 1 on the night shift. There was 1 senior resident per team on day shift, and a single senior resident covering all teams at night.
Preintervention | Postintervention | |||||
---|---|---|---|---|---|---|
| ||||||
General Pediatrics | Team A | Team B | Team C | Team D | Team E | Team F |
Patient Distribution | General Pediatrics | GI/Liver | Renal | General Pediatrics | General Pediatrics | Liver |
Pulmonary | Neurology | Rheumatology | Mixed Specialty | Mixed Specialty | Renal | |
Adolescent | Endocrine | |||||
Team membersa | 2 interns (q6 call) | 4 interns (3 on day shift/1 on night shift) | ||||
1 senior resident (q5 call) | 1 senior resident | |||||
Night‐shift coveragea | 1 intern and 1 senior resident together covered all 3 teams. | 1 night intern per team (teams D/E) working 7 consecutive night shifts | ||||
1 supervising night resident covering all 3 teams | ||||||
Intern cross‐coverage of other teams | Nights/clinic afternoons | None | ||||
Length of night shift | 30 hours | 13 hours |
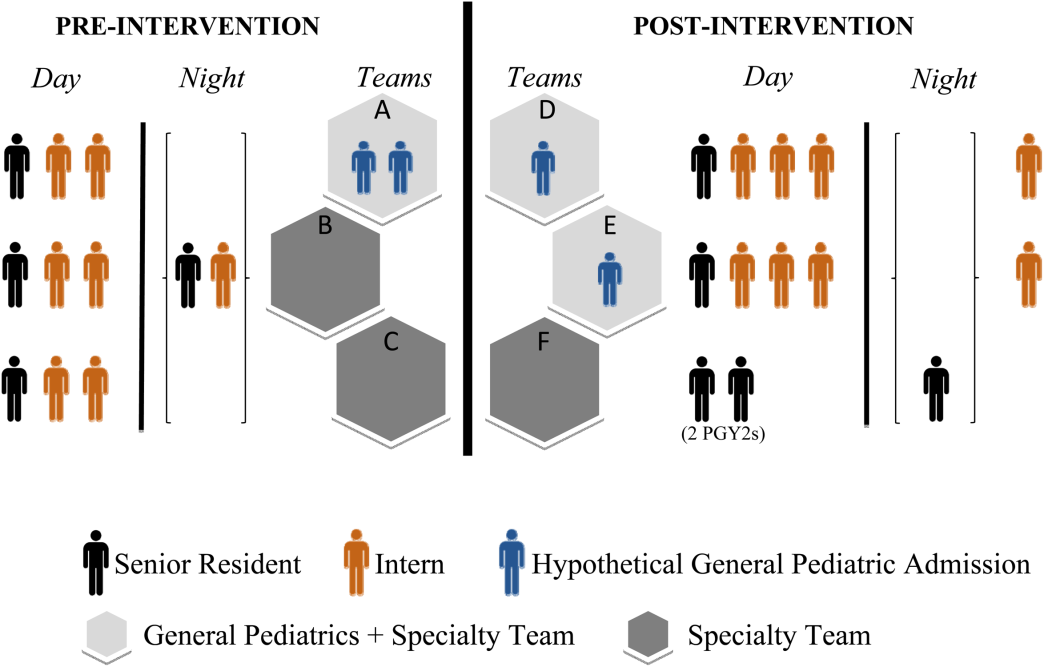
There was no change in the paper‐order system, the electronic health record, timing of the morning blood draw, use of new facilities for patient care, or protocol for emergency department admission. Concomitant with the restructuring, most subspecialty patients were consolidated onto the hospitalist service, necessitating creation of a second hospitalist team. However, patients admitted with the diagnoses identified above would have been on the hospitalist service before and after the restructuring.
Data Collection/Analysis
We reviewed specific classes of orders and categorized by type: respiratory medication, oxygen, intravenous (IV) fluids, diet, monitoring, and activity, time of day (day vs night‐shift), and whether they were an escalation or de‐escalation of care. De‐escalation of care was defined as orders that decreased patient care such as weaning a patient off nebulized albuterol or decreasing their IV fluids. Orders between 07:00 to 18:00 were considered day‐shift orders and between 18:01 and 06:59 were classified as night‐shift orders. Only orders falling into 1 of the aforementioned categories were recorded. Admission order sets were not included. Initially, charts were reviewed by both investigators together; after comparing results for 10 charts to ensure consistency of methodology and criteria, the remaining charts were reviewed by 1 of the study investigators.
To compare demographics, diagnoses, and ordering patterns, t tests and 2 (SAS version 9.2 [SAS Institute, Cary, NC], Stata version 13.1 [StataCorp, College Station, TX]) were used. Multivariate gamma models (SAS version 9.2 [SAS Institute]) that adjusted for clustering at the attending level and patient age were used to compare severity of illness before and after the intervention. This study was approved by the University of California, San Francisco Committee on Human Research.
RESULTS
We analyzed data for 48 admissions preintervention and 50 postintervention. With the exception of insurance type, there was no difference in baseline demographics, diagnoses, or severity of illness between the groups (Table 2). Within the order classes above, we identified 212 orders preintervention and 231 orders postintervention.
Preintervention,n = 48, N (%) | Postintervention, n = 50, N (%) | P Value | |
---|---|---|---|
| |||
Age, y, mean (SD) | 4.8 (4.6) | 5.5 (4.7) | 0.4474 |
Race/ethnicity | 0.1953 | ||
NH white | 12 (25.0%) | 9 (18.0%) | |
NH black | 11 (22.9%) | 7 (14.0%) | |
Hispanic | 16 (33.3%) | 13 (26.0%) | |
Asian | 6 (12.5%) | 10 (20.0%) | |
Other | 3 (6.3%) | 10 (20.0%) | |
Missing | 0 | 1 (2.0%) | |
Gender | 0.6577 | ||
Female | 19 (39.6%) | 22 (44.0%) | |
Male | 29 (60.4%) | 28 (56.0%) | |
Primary language | 0.2601 | ||
English | 38 (79.2%) | 45 (90.0%) | |
Spanish | 9 (18.8%) | 5 (10.0%) | |
Other | 1 (2.1%) | 0 | |
Insurance | 0.0118 | ||
Private | 13 (27.1%) | 26 (52.0%) | |
Medical | 35 (72.9%) | 24 (48.0%) | |
Other | 0 | 0 | |
Admit source | 0.6581 | ||
Referral | 20 (41.7%) | 18 (36.0%) | |
ED | 26 (54.2%) | 31 (62.0%) | |
Transfer | 2 (4.2%) | 1 (2.0%) | |
Severity of illness | 0.1926 | ||
Minor | 15 (31.3%) | 24 (48.0%) | |
Moderate | 23 (47.9%) | 16 (32.0%) | |
Severe | 10 (20.8%) | 10 (20.0%) | |
Extreme | 0 | 0 | |
Diagnoses | 0.562 | ||
Asthma | 21 | 19 | |
Bronchiolitis | 2 | 4 | |
Pneumonia | 17 | 19 | |
Dehydration | 6 | 7 | |
URI | 0 | 1 | |
Aspiration pneumonia | 2 | 0 |
After the intervention, there was a statistically significant increase in the average number of orders written within the first 12 hours (pre: 0.58 orders vs post: 1.12, P = 0.009) and 24 hours (pre: 1.52 vs post: 2.38, P = 0.004) following admission (Table 3), not including the admission order set. The fraction of orders written at night was not significantly different (27% at night preintervention, 33% postintervention, P = 0.149). The fraction of admissions on the day shift compared to the night shift did not change (P = 0.72). There was no difference in the ratio of de‐escalation to escalation orders written during the night (Table 2).
Preintervention, 48 Admissions | Postintervention, 50 Admissions | P Value | |
---|---|---|---|
| |||
Total no. of orders | 212 | 231 | |
Mean no. of orders per admission | 4.42 | 4.62 | |
Day shift orders, n (%) | 155 (73) | 155 (67) | 0.149 |
Night shift orders, n (%) | 57 (27) | 76 (33) | |
Mean no. of orders within first 12 hours* | 0.58 | 1.12 | 0.009 |
Mean no. of orders within first 24 hours* | 1.52 | 2.38 | 0.004 |
Night shift escalation orders (%) | 27 (47) | 33 (43) | 0.491 |
Night shift de‐escalation orders (%) | 30 (53) | 43 (57) |
DISCUSSION
In this study, we demonstrate increased patient care management early in the hospitalization, measured in this study by the mean number of orders written per patient in the first 12 and 24 hours after admission, after transition from a call schedule with extended (>16 hours) shifts to one with shorter shifts compliant with current ACGME duty‐hour restrictions and an explicit focus on greater continuity of care. We did not detect a change in the proportion of total orders written on the night shift compared to the day shift. Earlier active medical management, such as weaning nebulized albuterol or supplemental oxygen, can speed the time to discharge.[14]
Our failure to detect a significant change in the proportion or type of orders written at night may have been due to our small sample size. Anecdotally, after the intervention, medical students reported to us that they noticed a difference between our service, in which we expect night teams to advance care, and other services at our institution, in which nights are a time to focus on putting out fires. This was not something that had been reported to us prior. It is likely reflective of the overall approach to patient care taken by residents working a night shift as part of a longitudinal care team.
This study builds on previous findings that demonstrated lower costs and shorter length of stay after implementing a schedule based on day and night teams.[7] The reasons for such improvements are likely multifactorial. In our model, which was purposefully designed to create night‐team continuity and minimize cross‐coverage, it is likely that residents also felt a greater sense of responsibility for and familiarity with the patients[15] and therefore felt more comfortable advancing care. Not only were interns likely better rested, the patient‐to‐provider ratio was also lower than in the preintervention model. Increases in staffing are often necessary to eliminate cross‐coverage while maintaining safe, 24‐hour care. These findings suggest that increases in cost from additional staffing may be at least partially offset by more active patient management early in the hospitalization, which has the potential to lead to shorter hospital stays.
There are several limitations to our research. We studied a small sample, including a subset of general pediatrics diagnoses that are amenable to active management, limiting generalizability. We did not calculate a physician‐to‐patient ratio because this was not possible with the retrospective data we collected. Staffing ratios likely improved, and we consider that part of the overall improvements in staffing that may have contributed to the observed changes in ordering patterns. Although intern‐level cross‐coverage was eliminated, the senior resident continued to cover multiple teams overnight. This senior covered the same 3 teams for 7 consecutive nights. The addition of a hospitalist team, with subspecialists being placed in consultant roles, may have contributed to the increase in active management, though our study population did not include subspecialty patients. There was a difference in insurance status between the 2 groups. This was unlikely to affect resident physician practices as insurance information is not routinely discussed in the course of patient care. In the context of the ongoing debate about duty‐hour restrictions, it will be important for future studies to elucidate whether sleep or other variables are the primary contributors to this finding. Our data are derived solely from 1 inpatient service at a single academic medical center; however, we do feel there are lessons that may be applied to other settings.
CONCLUSION
A coverage system with improved nighttime resident coverage was associated with a greater number of orders written early in the hospitalization, suggesting more active management of clinical problems to advance care.
Acknowledgements
The authors thank Dr. I. Elaine Allen, John Kornak, and Dr. Derek Pappas for assistance with biostatistics, and Dr. Diana Bojorquez and Dr. Derek Pappas for assistance with review of the manuscript and creation of the figures.
Disclosures: None of the authors have financial relationships or other conflicts of interest to disclose. No external funding was secured for this study. Dr. Auerbach was supported by grant K24HL098372 during the course of this study. This project was supported by the National Center for Advancing Translational Sciences, National Institutes of Health (NIH), through University of California San FranciscoClinical and Translational Sciences Institute grant UL1 TR000004. Its contents are solely the responsibility of the authors and do not necessarily represent the official views of the NIH. Dr. Rosenbluth had access to all of the data in the study and takes responsibility for the integrity of the data and the accuracy of the data analysis.
Duty‐hour restrictions were implemented by the Accreditation Council for Graduate Medical Education (ACGME) in 2003 in response to data showing that sleep deprivation was correlated with serious medical errors.[1] In 2011, the ACGME required more explicit restrictions in the number of hours worked and the maximal shift length.[2] These requirements have necessitated a transition from a traditional q4 call model for interns to one in which shifts are limited to a maximum of 16 hours.
Studies of interns working these shorter shifts have had varied results, and comprehensive reviews have failed to demonstrate consistent improvements.[3, 4, 5] Studies of shift‐length limitation initially suggested improvements in patient safety (decreased length of stay,[6, 7] cost of hospitalization,[6] medication errors,[7] serious medical errors,[8] and intensive care unit [ICU] admissions[9]) and resident quality of life.[10] However, other recent studies have reported an increased number of self‐reported medical errors[11] and either did not detect change[12] or reported perceived decreases[13] in quality of care and continuity of care.
We previously reported decreased length of stay and decreased cost of hospitalization in pediatric inpatients cared for in a day/night‐shiftbased care model.[6] An hypothesized reason for those care improvements is the restructured care model led to increased active clinical management during both day and night hours. Here we report the findings of a retrospective analysis to investigate this hypothesis.
PATIENTS AND METHODS
Study Population
We reviewed the charts of pediatric patients admitted to University of California, San Francisco Benioff Children's Hospital, a 175‐bed tertiary care facility, over a 2‐year period between September 15, 2007 and September 15, 2008 (preintervention) and September 16, 2008 and September 16, 2009 (postintervention). During this study period, our hospital was still dependent on paper orders. Admission order sets were preprinted paper forms that were unchanged for the study period. Using International Classification of Diseases, 9th Revision coding, we identified patients on the general pediatrics service with 1 of 6 common diagnosesdehydration, community‐acquired pneumonia, aspiration pneumonia, upper respiratory infection, asthma, and bronchiolitis. These diagnoses were chosen because it was hypothesized that their length of inpatient stay could be impacted by active clinical management. We excluded patients admitted to the ICU or transferred between services.
A list of medical record numbers (MRNs) corresponding to admissions for 1 of the 6 above diagnoses during the pre‐ and postintervention periods was compiled. MRNs were randomized and then sequentially reviewed until 50 admissions in each time period were obtained. After data collection was completed, we noted that 2 patients had been in the ICU for part of their hospitalization, and these were excluded, leaving 48 admissions from prior to the intervention and 50 admissions from after intervention who were examined.
Intervention
During the preintervention period, patients were cared for by interns who took call every sixth night (duty periods up to 30 hours), with cross‐coverage of patients on multiple teams. Cross‐coverage was defined as coverage of patients cared for during nonconsecutive shifts and for whom residents did not participate in attending rounds. Noncall shifts were typically 10 to 11 hours. They were supervised by senior residents who took call every fourth or fifth night and who provided similar cross‐coverage.
During the postintervention period, interns worked day and night shifts of 13 hours (1 hour overlap time between shifts for handoffs), with increased night staffing to eliminate intern‐level cross‐coverage of multiple teams and maintain interns as the primary providers. Interns covered the same team for 5 to 7 consecutive days on either the day or night shifts. Interns remained on the same teams when they switched from day shifts to night shifts to preserve continuity. There were some 24‐hour shifts for senior residents on weekends. Senior residents maintained supervisory responsibility for all patients (both hospitalist teams and a subspecialty team). They also worked 7 consecutive nights.
There were changes in the staffing ratios associated with the change to day and night teams (Table 1, Figure 1). In the preintervention period, general pediatrics patients were covered by a single hospitalist and cohorted on a single team (team A), which also covered several groups of subspecialty patients with subspecialty attendings. The team consisted of 2 interns and 1 senior resident, who shared extended (30‐hour) call in a cycle with 2 other inpatient teams. In the postintervention period, general pediatrics patients were split between 2 teams (teams D and E) and mixed with subspecialty patients. Hospitalist continued to be the attendings, and these hospitalists also covered specialty patients with subspecialists in consulting roles. The teams consisted of 3 interns on the day shift, and 1 on the night shift. There was 1 senior resident per team on day shift, and a single senior resident covering all teams at night.
Preintervention | Postintervention | |||||
---|---|---|---|---|---|---|
| ||||||
General Pediatrics | Team A | Team B | Team C | Team D | Team E | Team F |
Patient Distribution | General Pediatrics | GI/Liver | Renal | General Pediatrics | General Pediatrics | Liver |
Pulmonary | Neurology | Rheumatology | Mixed Specialty | Mixed Specialty | Renal | |
Adolescent | Endocrine | |||||
Team membersa | 2 interns (q6 call) | 4 interns (3 on day shift/1 on night shift) | ||||
1 senior resident (q5 call) | 1 senior resident | |||||
Night‐shift coveragea | 1 intern and 1 senior resident together covered all 3 teams. | 1 night intern per team (teams D/E) working 7 consecutive night shifts | ||||
1 supervising night resident covering all 3 teams | ||||||
Intern cross‐coverage of other teams | Nights/clinic afternoons | None | ||||
Length of night shift | 30 hours | 13 hours |
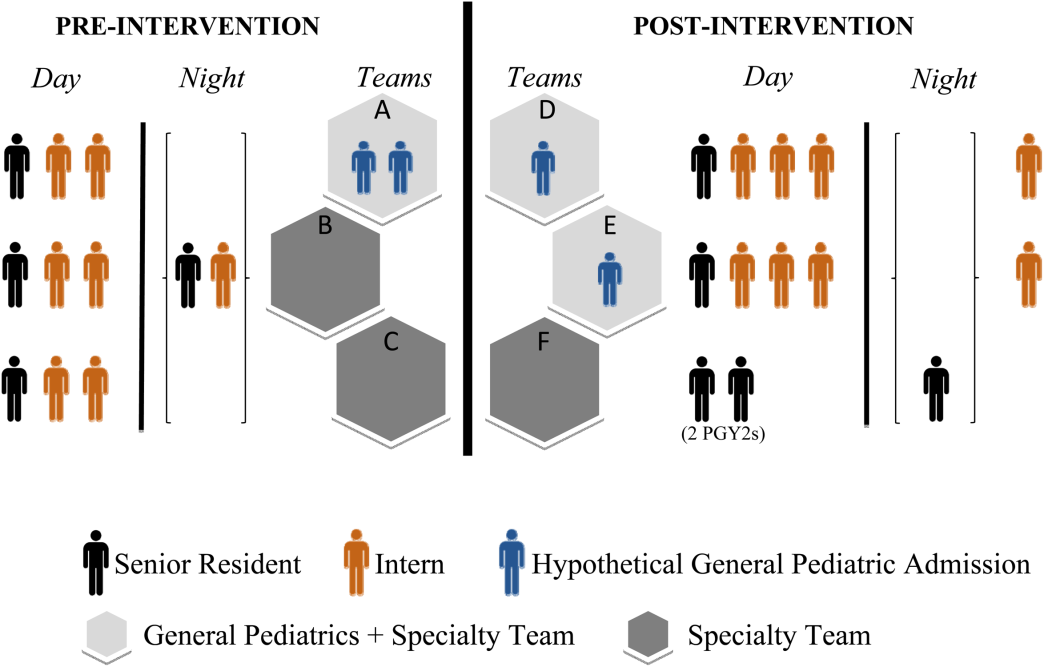
There was no change in the paper‐order system, the electronic health record, timing of the morning blood draw, use of new facilities for patient care, or protocol for emergency department admission. Concomitant with the restructuring, most subspecialty patients were consolidated onto the hospitalist service, necessitating creation of a second hospitalist team. However, patients admitted with the diagnoses identified above would have been on the hospitalist service before and after the restructuring.
Data Collection/Analysis
We reviewed specific classes of orders and categorized by type: respiratory medication, oxygen, intravenous (IV) fluids, diet, monitoring, and activity, time of day (day vs night‐shift), and whether they were an escalation or de‐escalation of care. De‐escalation of care was defined as orders that decreased patient care such as weaning a patient off nebulized albuterol or decreasing their IV fluids. Orders between 07:00 to 18:00 were considered day‐shift orders and between 18:01 and 06:59 were classified as night‐shift orders. Only orders falling into 1 of the aforementioned categories were recorded. Admission order sets were not included. Initially, charts were reviewed by both investigators together; after comparing results for 10 charts to ensure consistency of methodology and criteria, the remaining charts were reviewed by 1 of the study investigators.
To compare demographics, diagnoses, and ordering patterns, t tests and 2 (SAS version 9.2 [SAS Institute, Cary, NC], Stata version 13.1 [StataCorp, College Station, TX]) were used. Multivariate gamma models (SAS version 9.2 [SAS Institute]) that adjusted for clustering at the attending level and patient age were used to compare severity of illness before and after the intervention. This study was approved by the University of California, San Francisco Committee on Human Research.
RESULTS
We analyzed data for 48 admissions preintervention and 50 postintervention. With the exception of insurance type, there was no difference in baseline demographics, diagnoses, or severity of illness between the groups (Table 2). Within the order classes above, we identified 212 orders preintervention and 231 orders postintervention.
Preintervention,n = 48, N (%) | Postintervention, n = 50, N (%) | P Value | |
---|---|---|---|
| |||
Age, y, mean (SD) | 4.8 (4.6) | 5.5 (4.7) | 0.4474 |
Race/ethnicity | 0.1953 | ||
NH white | 12 (25.0%) | 9 (18.0%) | |
NH black | 11 (22.9%) | 7 (14.0%) | |
Hispanic | 16 (33.3%) | 13 (26.0%) | |
Asian | 6 (12.5%) | 10 (20.0%) | |
Other | 3 (6.3%) | 10 (20.0%) | |
Missing | 0 | 1 (2.0%) | |
Gender | 0.6577 | ||
Female | 19 (39.6%) | 22 (44.0%) | |
Male | 29 (60.4%) | 28 (56.0%) | |
Primary language | 0.2601 | ||
English | 38 (79.2%) | 45 (90.0%) | |
Spanish | 9 (18.8%) | 5 (10.0%) | |
Other | 1 (2.1%) | 0 | |
Insurance | 0.0118 | ||
Private | 13 (27.1%) | 26 (52.0%) | |
Medical | 35 (72.9%) | 24 (48.0%) | |
Other | 0 | 0 | |
Admit source | 0.6581 | ||
Referral | 20 (41.7%) | 18 (36.0%) | |
ED | 26 (54.2%) | 31 (62.0%) | |
Transfer | 2 (4.2%) | 1 (2.0%) | |
Severity of illness | 0.1926 | ||
Minor | 15 (31.3%) | 24 (48.0%) | |
Moderate | 23 (47.9%) | 16 (32.0%) | |
Severe | 10 (20.8%) | 10 (20.0%) | |
Extreme | 0 | 0 | |
Diagnoses | 0.562 | ||
Asthma | 21 | 19 | |
Bronchiolitis | 2 | 4 | |
Pneumonia | 17 | 19 | |
Dehydration | 6 | 7 | |
URI | 0 | 1 | |
Aspiration pneumonia | 2 | 0 |
After the intervention, there was a statistically significant increase in the average number of orders written within the first 12 hours (pre: 0.58 orders vs post: 1.12, P = 0.009) and 24 hours (pre: 1.52 vs post: 2.38, P = 0.004) following admission (Table 3), not including the admission order set. The fraction of orders written at night was not significantly different (27% at night preintervention, 33% postintervention, P = 0.149). The fraction of admissions on the day shift compared to the night shift did not change (P = 0.72). There was no difference in the ratio of de‐escalation to escalation orders written during the night (Table 2).
Preintervention, 48 Admissions | Postintervention, 50 Admissions | P Value | |
---|---|---|---|
| |||
Total no. of orders | 212 | 231 | |
Mean no. of orders per admission | 4.42 | 4.62 | |
Day shift orders, n (%) | 155 (73) | 155 (67) | 0.149 |
Night shift orders, n (%) | 57 (27) | 76 (33) | |
Mean no. of orders within first 12 hours* | 0.58 | 1.12 | 0.009 |
Mean no. of orders within first 24 hours* | 1.52 | 2.38 | 0.004 |
Night shift escalation orders (%) | 27 (47) | 33 (43) | 0.491 |
Night shift de‐escalation orders (%) | 30 (53) | 43 (57) |
DISCUSSION
In this study, we demonstrate increased patient care management early in the hospitalization, measured in this study by the mean number of orders written per patient in the first 12 and 24 hours after admission, after transition from a call schedule with extended (>16 hours) shifts to one with shorter shifts compliant with current ACGME duty‐hour restrictions and an explicit focus on greater continuity of care. We did not detect a change in the proportion of total orders written on the night shift compared to the day shift. Earlier active medical management, such as weaning nebulized albuterol or supplemental oxygen, can speed the time to discharge.[14]
Our failure to detect a significant change in the proportion or type of orders written at night may have been due to our small sample size. Anecdotally, after the intervention, medical students reported to us that they noticed a difference between our service, in which we expect night teams to advance care, and other services at our institution, in which nights are a time to focus on putting out fires. This was not something that had been reported to us prior. It is likely reflective of the overall approach to patient care taken by residents working a night shift as part of a longitudinal care team.
This study builds on previous findings that demonstrated lower costs and shorter length of stay after implementing a schedule based on day and night teams.[7] The reasons for such improvements are likely multifactorial. In our model, which was purposefully designed to create night‐team continuity and minimize cross‐coverage, it is likely that residents also felt a greater sense of responsibility for and familiarity with the patients[15] and therefore felt more comfortable advancing care. Not only were interns likely better rested, the patient‐to‐provider ratio was also lower than in the preintervention model. Increases in staffing are often necessary to eliminate cross‐coverage while maintaining safe, 24‐hour care. These findings suggest that increases in cost from additional staffing may be at least partially offset by more active patient management early in the hospitalization, which has the potential to lead to shorter hospital stays.
There are several limitations to our research. We studied a small sample, including a subset of general pediatrics diagnoses that are amenable to active management, limiting generalizability. We did not calculate a physician‐to‐patient ratio because this was not possible with the retrospective data we collected. Staffing ratios likely improved, and we consider that part of the overall improvements in staffing that may have contributed to the observed changes in ordering patterns. Although intern‐level cross‐coverage was eliminated, the senior resident continued to cover multiple teams overnight. This senior covered the same 3 teams for 7 consecutive nights. The addition of a hospitalist team, with subspecialists being placed in consultant roles, may have contributed to the increase in active management, though our study population did not include subspecialty patients. There was a difference in insurance status between the 2 groups. This was unlikely to affect resident physician practices as insurance information is not routinely discussed in the course of patient care. In the context of the ongoing debate about duty‐hour restrictions, it will be important for future studies to elucidate whether sleep or other variables are the primary contributors to this finding. Our data are derived solely from 1 inpatient service at a single academic medical center; however, we do feel there are lessons that may be applied to other settings.
CONCLUSION
A coverage system with improved nighttime resident coverage was associated with a greater number of orders written early in the hospitalization, suggesting more active management of clinical problems to advance care.
Acknowledgements
The authors thank Dr. I. Elaine Allen, John Kornak, and Dr. Derek Pappas for assistance with biostatistics, and Dr. Diana Bojorquez and Dr. Derek Pappas for assistance with review of the manuscript and creation of the figures.
Disclosures: None of the authors have financial relationships or other conflicts of interest to disclose. No external funding was secured for this study. Dr. Auerbach was supported by grant K24HL098372 during the course of this study. This project was supported by the National Center for Advancing Translational Sciences, National Institutes of Health (NIH), through University of California San FranciscoClinical and Translational Sciences Institute grant UL1 TR000004. Its contents are solely the responsibility of the authors and do not necessarily represent the official views of the NIH. Dr. Rosenbluth had access to all of the data in the study and takes responsibility for the integrity of the data and the accuracy of the data analysis.
- New requirements for resident duty hours. JAMA. 2002;288(9):1112–1114. , , .
- Accreditation Council for Graduate Medical Education. Common program requirements. 2011. Available at: http://www.acgme.org/acgmeweb/Portals/0/PDFs/Common_Program_Requirements_07012011[2].pdf. Accessed November 28, 2011.
- Patient safety, resident education and resident well‐being following implementation of the 2003 ACGME duty hour rules. J Gen Intern Med. 2011;26(8):907–919. , , .
- A systematic review of the effects of resident duty hour restrictions in surgery: impact on resident wellness, training, and patient outcomes. Ann Surg. 2014;259(6):1041–1053. , , , et al.
- Duty‐hour limits and patient care and resident outcomes: can high‐quality studies offer insight into complex relationships? Annu Rev Med. 2013;64:467–483. , , , .
- Association between adaptations to ACGME duty hour requirements, length of stay, and costs. Sleep. 2013;36(2):245–248. , , , , , .
- Effect of a change in house staff work schedule on resource utilization and patient care. Arch Intern Med. 1991;151(10):2065–2070. , , , .
- Effect of reducing interns' work hours on serious medical errors in intensive care units. N Engl J Med. 2004;351(18):1838–1848. , , , et al.
- Changes in outcomes for internal medicine inpatients after work‐hour regulations. Ann Intern Med. 2007;147(2):97–103. , , , .
- Effects of reducing or eliminating resident work shifts over 16 hours: a systematic review. Sleep. 2010;33(8):1043–1053. , , .
- Effects of the 2011 duty hour reforms on interns and their patients: a prospective longitudinal cohort study. JAMA Intern Med. 2013;173(8):657–662; discussion 663. , , , et al.
- Effect of 16‐hour duty periods on patient care and resident education. Mayo Clin Proc. 2011;86(3):192–196. , , , , .
- Effect of the 2011 vs 2003 duty hour regulation‐compliant models on sleep duration, trainee education, and continuity of patient care among internal medicine house staff: a randomized trial. JAMA Intern Med. 2013;173(8):649–655. , , , et al.
- Effectiveness of a clinical pathway for inpatient asthma management. Pediatrics. 2000;106(5):1006–1012. , , , .
- Resident perceptions of autonomy in a complex tertiary care environment improve when supervised by hospitalists. Hosp Pediatr. 2012;2(4):228–234. , , , .
- New requirements for resident duty hours. JAMA. 2002;288(9):1112–1114. , , .
- Accreditation Council for Graduate Medical Education. Common program requirements. 2011. Available at: http://www.acgme.org/acgmeweb/Portals/0/PDFs/Common_Program_Requirements_07012011[2].pdf. Accessed November 28, 2011.
- Patient safety, resident education and resident well‐being following implementation of the 2003 ACGME duty hour rules. J Gen Intern Med. 2011;26(8):907–919. , , .
- A systematic review of the effects of resident duty hour restrictions in surgery: impact on resident wellness, training, and patient outcomes. Ann Surg. 2014;259(6):1041–1053. , , , et al.
- Duty‐hour limits and patient care and resident outcomes: can high‐quality studies offer insight into complex relationships? Annu Rev Med. 2013;64:467–483. , , , .
- Association between adaptations to ACGME duty hour requirements, length of stay, and costs. Sleep. 2013;36(2):245–248. , , , , , .
- Effect of a change in house staff work schedule on resource utilization and patient care. Arch Intern Med. 1991;151(10):2065–2070. , , , .
- Effect of reducing interns' work hours on serious medical errors in intensive care units. N Engl J Med. 2004;351(18):1838–1848. , , , et al.
- Changes in outcomes for internal medicine inpatients after work‐hour regulations. Ann Intern Med. 2007;147(2):97–103. , , , .
- Effects of reducing or eliminating resident work shifts over 16 hours: a systematic review. Sleep. 2010;33(8):1043–1053. , , .
- Effects of the 2011 duty hour reforms on interns and their patients: a prospective longitudinal cohort study. JAMA Intern Med. 2013;173(8):657–662; discussion 663. , , , et al.
- Effect of 16‐hour duty periods on patient care and resident education. Mayo Clin Proc. 2011;86(3):192–196. , , , , .
- Effect of the 2011 vs 2003 duty hour regulation‐compliant models on sleep duration, trainee education, and continuity of patient care among internal medicine house staff: a randomized trial. JAMA Intern Med. 2013;173(8):649–655. , , , et al.
- Effectiveness of a clinical pathway for inpatient asthma management. Pediatrics. 2000;106(5):1006–1012. , , , .
- Resident perceptions of autonomy in a complex tertiary care environment improve when supervised by hospitalists. Hosp Pediatr. 2012;2(4):228–234. , , , .
Science and Scholarship
In 2015, the Journal of Hospital Medicine will be publishing its 10th volume, marking an important milestone for hospital medicine. The journal has survived an early period where the need for and viability of an academic hospital medicine journal was not at all clear. More to the point, the Journal of Hospital Medicine has prospered and grown.
The journal continues to evolve as hospital medicine grows and changes. When the Journal of Hospital Medicine began, there was no particular focus on readmissions, ‐blockers were important to reduce perioperative risk, and tight glucose control was thought to be a critical goal for hospitalized patients. Evidence for hospitalists' effectiveness was also changing; initial evidence supporting uniform improvements in length of stay and possible improvement in outcomes were soon tempered by larger‐scale studies.
Clearly, things have changed in medicine writ large, and hospital medicine has changed and caused things to be changed as well. We now exist in a world of value‐based purchasing, accountable care, long‐overdue focus on patient‐centered care (and research), and all in a healthcare setting that is increasingly electronic in nature. Although healthcare delivery has changed, hospital medicine has retained critical core values, including our specialty's focus on interdisciplinary care, quality improvement, and safety.
The Journal of Hospital Medicine has been active in reflecting the field of hospital medicine since the journal began. Due in no small part to the vision of the field's leaders and the exertion and vision of the Journal of Hospital Medicine's founding editor, Mark Williams, the journal is a vigorous and important contributor to academic internal medicine. It has gone from 6 issues per year to 12 issues, has rapidly expanded the number of downloads and citations, and its impact in the field of medicine continues to grow.
When reflecting on the words most often used in the Journal of Hospital Medicine's article titles (Figure 1) over the last 9 volumes, we were not surprised to see that the words hospital and hospitalists figured prominently. However, we were more pleased to see that the words patient and care were a large part of the universe of the Journal of Hospital Medicine's article titles.
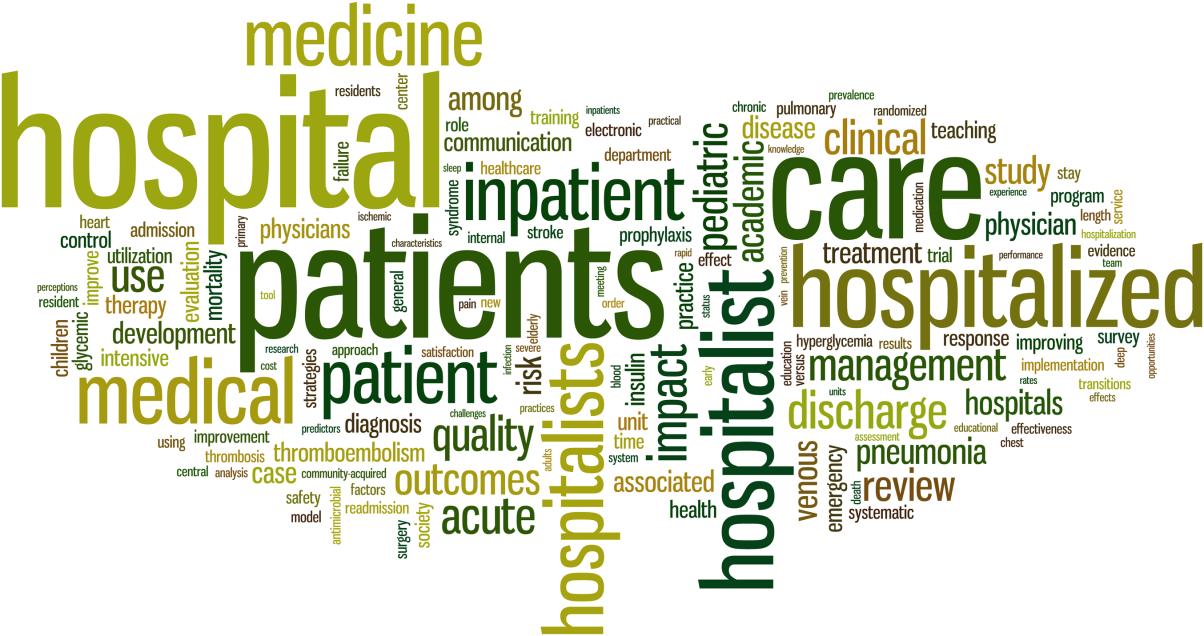
The Journal of Hospital Medicine's articles are also being read and cited more and more often. The top 10 most downloaded articles are ones that represent a wide range of topics of clinical and operational importance to the field (Table 1), and the 10 most cited articles (Table 2) represent how the scholarship being produced by the field of hospital medicine is being used to advance other scientific and policy initiatives. Only 1 article[1] was listed in both places, demonstrating the difference between what the Journal of Hospital Medicine publishes as a way to help hospitalists provide better care on a day‐to‐day basis and what the journal publishes to advance the field.
Article Title | Total Downloads |
---|---|
1. Making inpatient medication reconciliation patient centered, clinically relevant, and implementable: a consensus statement on key principles and necessary first steps[3] | 9,766 |
2. Promoting effective transitions of care at hospital discharge: a review of key issues for hospitalists[1] | 4,243 |
3. The key principles and characteristics of an effective hospital medicine group: an assessment guide for hospitals and hospitalists[4] | 3,722 |
4. Observation and inpatient status: clinical impact of the 2‐midnight rule[5] | 2,587 |
5. Measuring the modified early warning score and the Rothman Index: advantages of utilizing the electronic medical record in an early warning system[6] | 1,923 |
6. Acute coronary syndrome update for the hospitalist[7] | 1,514 |
7. Hospital performance trends on national quality measures and the association with Joint Commission accreditation[8] | 1,505 |
8. The core competencies in hospital medicine: a framework for curriculum development by the Society of Hospital Medicine[9] | 1,218 |
9. Iliac vein compression syndrome: an underdiagnosed cause of lower extremity deep venous thrombosis[10] | 1,135 |
10. Aspirin versus anticoagulation for prevention of venous thromboembolism major lower extremity orthopedic surgery: a systematic review and meta‐analysis[11] | 1,069 |
Title | Total Citations |
---|---|
1. Promoting effective transitions of care at hospital discharge: a review of key issues for hospitalists[1] | 152 |
2. Managing discontinuity in academic medical centers: strategies for a safe and effective resident sign‐out[12] | 110 |
3, Reduction of 30‐day postdischarge hospital readmission or emergency department (ED) visit rates in high‐risk elderly medical patients through delivery of a targeted care bundle[13] | 69 |
4. Mastery learning of thoracentesis skills by internal medicine residents using simulation technology and deliberate practice[14] | 61 |
5. Use of simulation‐based mastery learning to improve the quality of central venous catheter placement in a medical intensive care unit[15] | 60 |
6. Transition of care for hospitalized elderly patientsdevelopment of a discharge checklist for hospitalists[16] | 59 |
7. Hospitalist handoffs: a systematic review and task force recommendations[17] | 54 |
8. Inpatient management of diabetes and hyperglycemia among general medicine patients at a large teaching hospital[18] | 53 |
9. Transitions of care consensus policy statement: American College of Physicians, Society of General Internal Medicine, Society of Hospital Medicine, American Geriatrics Society, American College of Emergency Physicians, and Society for Academic Emergency Medicine[19] | 50 |
10. Diabetes care in hospitalized noncritically ill patients: more evidence for clinical inertia and negative therapeutic momentum[20] | 49 |
Our 10th volume will continue the work of promoting scholarship and inquiry in hospital medicine by retaining a clear focus on the science that represents the best of an intellectual agenda[2] for our field. To achieve this important goal, the Journal of Hospital Medicine and the field will need to develop durable evidence for not only how hospitalists deliver care, but also evidence for how care can be improved by providing better treatments. Stated differently, hospitalists and the Journal of Hospital Medicine will need to be focused not only on improving the healthcare system, but also on evaluating new technologies, drugs, and devices that can be used in a value‐focused health system.
In 2015, the Journal of Hospital Medicine will also be launching a new series focusing on improving healthcare value. Titled Choosing Wisely: Next Steps in Improving Healthcare Value, this series of invited reviews will cover important topics needed to frame approaches to reducing healthcare costs while improving care quality and safety. This American Board of Internal Medicine‐sponsored series will be accompanied by a parallel series titled Things We Do for No Reason. Things We Do will provide a series of case studies of tests, medications, or procedures hospitalists encounter every day. We hope these topics, along with the Society of Hospital Medicine's Choosing Wisely focus areas, will continue to outline useful opportunities to improve healthcare value at the bedside or at least frame what we expect will be a lively debate.
We are confident that the combination of highest‐quality peer review and new article offerings will only enhance our ability to share the highest‐quality research, perspectives, reviews, and clinical cases and conundrums with Journal of Hospital Medicine readers. The journal's editors and I look forward to this new year, the Journal of Hospital Medicine's 10th volume, and to many volumes of the Journal of Hospital Medicine to come.
- Promoting effective transitions of care at hospital discharge: a review of key issues for hospitalists. J Hosp Med. 2007;2(5):314–323. , , , .
- An intellectual agenda for hospitalists: lessons from bloodletting. J Hosp Med. 2013;8(7):418–419. .
- Making inpatient medication reconciliation patient centered, clinically relevant and implementable: a consensus statement on key principles and necessary first steps. J Hosp Med. 2010;5(8):477–485. , , , et al.
- The key principles and characteristics of an effective hospital medicine group: an assessment guide for hospitals and hospitalists. J Hosp Med. 2014;9(2):123–128. , , , et al.
- Observation and inpatient status: clinical impact of the 2‐midnight rule. J Hosp Med. 2014;9(4):203–209. , , , et al.
- Measuring the modified early warning score and the Rothman Index: advantages of utilizing the electronic medical record in an early warning system. J Hosp Med. 2014;9(2):116–119. , , .
- Acute coronary syndrome update for hospitalists. J Hosp Med. 2010;5(suppl 4):S15–S21. .
- Hospital performance trends on national quality measures and the association with Joint Commission accreditation. J Hosp Med. 2011;6(8):454–461. , , , , .
- The core competencies in hospital medicine: a framework for curriculum development by the society of hospital medicine. J Hosp Med. 2006;1(suppl 1):2–95.
- Iliac vein compression syndrome: an underdiagnosed cause of lower extremity deep venous thrombosis. J Hosp Med. 2010;5(7):E12–E13. , , , .
- Aspirin versus anticoagulation for prevention of venous thromboembolism major lower extremity orthopedic surgery: a systematic review and meta‐analysis. J Hosp Med. 2014;9(9):579–585. , , , , , .
- Managing discontinuity in academic medical centers: strategies for a safe and effective resident sign‐out. J Hosp Med. 2006;1(4):257–266. , , , , .
- Reduction of 30‐day postdischarge hospital readmission or emergency department (ED) visit rates in high‐risk elderly medical patients through delivery of a targeted care bundle. J Hosp Med. 2009;4(4):211–218. , , , et al.
- Mastery learning of thoracentesis skills by internal medicine residents using simulation technology and deliberate practice. J Hosp Med. 2008;3(1):48–54. , , , , .
- Use of simulation‐based mastery learning to improve the quality of central venous catheter placement in a medical intensive care unit. J Hosp Med. 2009;4(7):397–403. , , , , .
- Transition of care for hospitalized elderly patients—development of a discharge checklist for hospitalists. J Hosp Med. 2006;1(6):354–360. , , , et al.
- Hospitalist handoffs: a systematic review and task force recommendations. J Hosp Med. 2009;4(7):433–440. , , , , , .
- Inpatient management of diabetes and hyperglycemia among general medicine patients at a large teaching hospital. J Hosp Med. 2006;1(3):145–150. , , , , .
- Transitions of Care Consensus policy statement: American College of Physicians, Society of General Internal Medicine, Society of Hospital Medicine, American Geriatrics Society, American College of Emergency Physicians, and Society for Academic Emergency Medicine. J Hosp Med. 2009;4(6):364–370. , , , et al.
- Diabetes care in hospitalized noncritically ill patients: more evidence for clinical inertia and negative therapeutic momentum. J Hosp Med. 2007;2(4):203–211. , , , et al.
In 2015, the Journal of Hospital Medicine will be publishing its 10th volume, marking an important milestone for hospital medicine. The journal has survived an early period where the need for and viability of an academic hospital medicine journal was not at all clear. More to the point, the Journal of Hospital Medicine has prospered and grown.
The journal continues to evolve as hospital medicine grows and changes. When the Journal of Hospital Medicine began, there was no particular focus on readmissions, ‐blockers were important to reduce perioperative risk, and tight glucose control was thought to be a critical goal for hospitalized patients. Evidence for hospitalists' effectiveness was also changing; initial evidence supporting uniform improvements in length of stay and possible improvement in outcomes were soon tempered by larger‐scale studies.
Clearly, things have changed in medicine writ large, and hospital medicine has changed and caused things to be changed as well. We now exist in a world of value‐based purchasing, accountable care, long‐overdue focus on patient‐centered care (and research), and all in a healthcare setting that is increasingly electronic in nature. Although healthcare delivery has changed, hospital medicine has retained critical core values, including our specialty's focus on interdisciplinary care, quality improvement, and safety.
The Journal of Hospital Medicine has been active in reflecting the field of hospital medicine since the journal began. Due in no small part to the vision of the field's leaders and the exertion and vision of the Journal of Hospital Medicine's founding editor, Mark Williams, the journal is a vigorous and important contributor to academic internal medicine. It has gone from 6 issues per year to 12 issues, has rapidly expanded the number of downloads and citations, and its impact in the field of medicine continues to grow.
When reflecting on the words most often used in the Journal of Hospital Medicine's article titles (Figure 1) over the last 9 volumes, we were not surprised to see that the words hospital and hospitalists figured prominently. However, we were more pleased to see that the words patient and care were a large part of the universe of the Journal of Hospital Medicine's article titles.
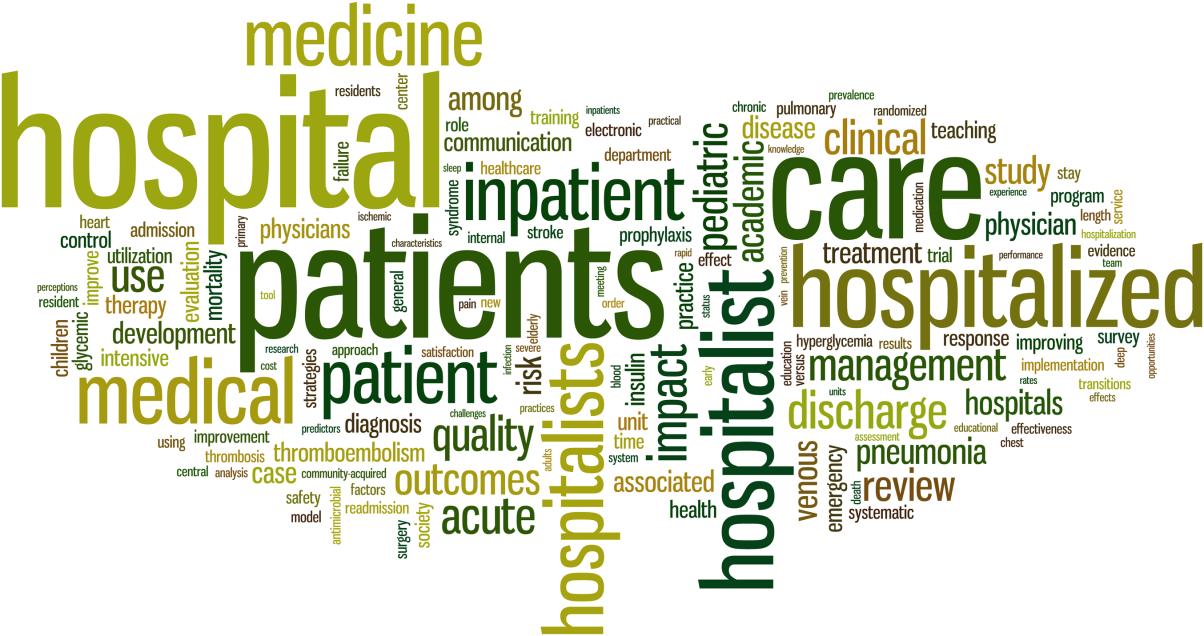
The Journal of Hospital Medicine's articles are also being read and cited more and more often. The top 10 most downloaded articles are ones that represent a wide range of topics of clinical and operational importance to the field (Table 1), and the 10 most cited articles (Table 2) represent how the scholarship being produced by the field of hospital medicine is being used to advance other scientific and policy initiatives. Only 1 article[1] was listed in both places, demonstrating the difference between what the Journal of Hospital Medicine publishes as a way to help hospitalists provide better care on a day‐to‐day basis and what the journal publishes to advance the field.
Article Title | Total Downloads |
---|---|
1. Making inpatient medication reconciliation patient centered, clinically relevant, and implementable: a consensus statement on key principles and necessary first steps[3] | 9,766 |
2. Promoting effective transitions of care at hospital discharge: a review of key issues for hospitalists[1] | 4,243 |
3. The key principles and characteristics of an effective hospital medicine group: an assessment guide for hospitals and hospitalists[4] | 3,722 |
4. Observation and inpatient status: clinical impact of the 2‐midnight rule[5] | 2,587 |
5. Measuring the modified early warning score and the Rothman Index: advantages of utilizing the electronic medical record in an early warning system[6] | 1,923 |
6. Acute coronary syndrome update for the hospitalist[7] | 1,514 |
7. Hospital performance trends on national quality measures and the association with Joint Commission accreditation[8] | 1,505 |
8. The core competencies in hospital medicine: a framework for curriculum development by the Society of Hospital Medicine[9] | 1,218 |
9. Iliac vein compression syndrome: an underdiagnosed cause of lower extremity deep venous thrombosis[10] | 1,135 |
10. Aspirin versus anticoagulation for prevention of venous thromboembolism major lower extremity orthopedic surgery: a systematic review and meta‐analysis[11] | 1,069 |
Title | Total Citations |
---|---|
1. Promoting effective transitions of care at hospital discharge: a review of key issues for hospitalists[1] | 152 |
2. Managing discontinuity in academic medical centers: strategies for a safe and effective resident sign‐out[12] | 110 |
3, Reduction of 30‐day postdischarge hospital readmission or emergency department (ED) visit rates in high‐risk elderly medical patients through delivery of a targeted care bundle[13] | 69 |
4. Mastery learning of thoracentesis skills by internal medicine residents using simulation technology and deliberate practice[14] | 61 |
5. Use of simulation‐based mastery learning to improve the quality of central venous catheter placement in a medical intensive care unit[15] | 60 |
6. Transition of care for hospitalized elderly patientsdevelopment of a discharge checklist for hospitalists[16] | 59 |
7. Hospitalist handoffs: a systematic review and task force recommendations[17] | 54 |
8. Inpatient management of diabetes and hyperglycemia among general medicine patients at a large teaching hospital[18] | 53 |
9. Transitions of care consensus policy statement: American College of Physicians, Society of General Internal Medicine, Society of Hospital Medicine, American Geriatrics Society, American College of Emergency Physicians, and Society for Academic Emergency Medicine[19] | 50 |
10. Diabetes care in hospitalized noncritically ill patients: more evidence for clinical inertia and negative therapeutic momentum[20] | 49 |
Our 10th volume will continue the work of promoting scholarship and inquiry in hospital medicine by retaining a clear focus on the science that represents the best of an intellectual agenda[2] for our field. To achieve this important goal, the Journal of Hospital Medicine and the field will need to develop durable evidence for not only how hospitalists deliver care, but also evidence for how care can be improved by providing better treatments. Stated differently, hospitalists and the Journal of Hospital Medicine will need to be focused not only on improving the healthcare system, but also on evaluating new technologies, drugs, and devices that can be used in a value‐focused health system.
In 2015, the Journal of Hospital Medicine will also be launching a new series focusing on improving healthcare value. Titled Choosing Wisely: Next Steps in Improving Healthcare Value, this series of invited reviews will cover important topics needed to frame approaches to reducing healthcare costs while improving care quality and safety. This American Board of Internal Medicine‐sponsored series will be accompanied by a parallel series titled Things We Do for No Reason. Things We Do will provide a series of case studies of tests, medications, or procedures hospitalists encounter every day. We hope these topics, along with the Society of Hospital Medicine's Choosing Wisely focus areas, will continue to outline useful opportunities to improve healthcare value at the bedside or at least frame what we expect will be a lively debate.
We are confident that the combination of highest‐quality peer review and new article offerings will only enhance our ability to share the highest‐quality research, perspectives, reviews, and clinical cases and conundrums with Journal of Hospital Medicine readers. The journal's editors and I look forward to this new year, the Journal of Hospital Medicine's 10th volume, and to many volumes of the Journal of Hospital Medicine to come.
In 2015, the Journal of Hospital Medicine will be publishing its 10th volume, marking an important milestone for hospital medicine. The journal has survived an early period where the need for and viability of an academic hospital medicine journal was not at all clear. More to the point, the Journal of Hospital Medicine has prospered and grown.
The journal continues to evolve as hospital medicine grows and changes. When the Journal of Hospital Medicine began, there was no particular focus on readmissions, ‐blockers were important to reduce perioperative risk, and tight glucose control was thought to be a critical goal for hospitalized patients. Evidence for hospitalists' effectiveness was also changing; initial evidence supporting uniform improvements in length of stay and possible improvement in outcomes were soon tempered by larger‐scale studies.
Clearly, things have changed in medicine writ large, and hospital medicine has changed and caused things to be changed as well. We now exist in a world of value‐based purchasing, accountable care, long‐overdue focus on patient‐centered care (and research), and all in a healthcare setting that is increasingly electronic in nature. Although healthcare delivery has changed, hospital medicine has retained critical core values, including our specialty's focus on interdisciplinary care, quality improvement, and safety.
The Journal of Hospital Medicine has been active in reflecting the field of hospital medicine since the journal began. Due in no small part to the vision of the field's leaders and the exertion and vision of the Journal of Hospital Medicine's founding editor, Mark Williams, the journal is a vigorous and important contributor to academic internal medicine. It has gone from 6 issues per year to 12 issues, has rapidly expanded the number of downloads and citations, and its impact in the field of medicine continues to grow.
When reflecting on the words most often used in the Journal of Hospital Medicine's article titles (Figure 1) over the last 9 volumes, we were not surprised to see that the words hospital and hospitalists figured prominently. However, we were more pleased to see that the words patient and care were a large part of the universe of the Journal of Hospital Medicine's article titles.
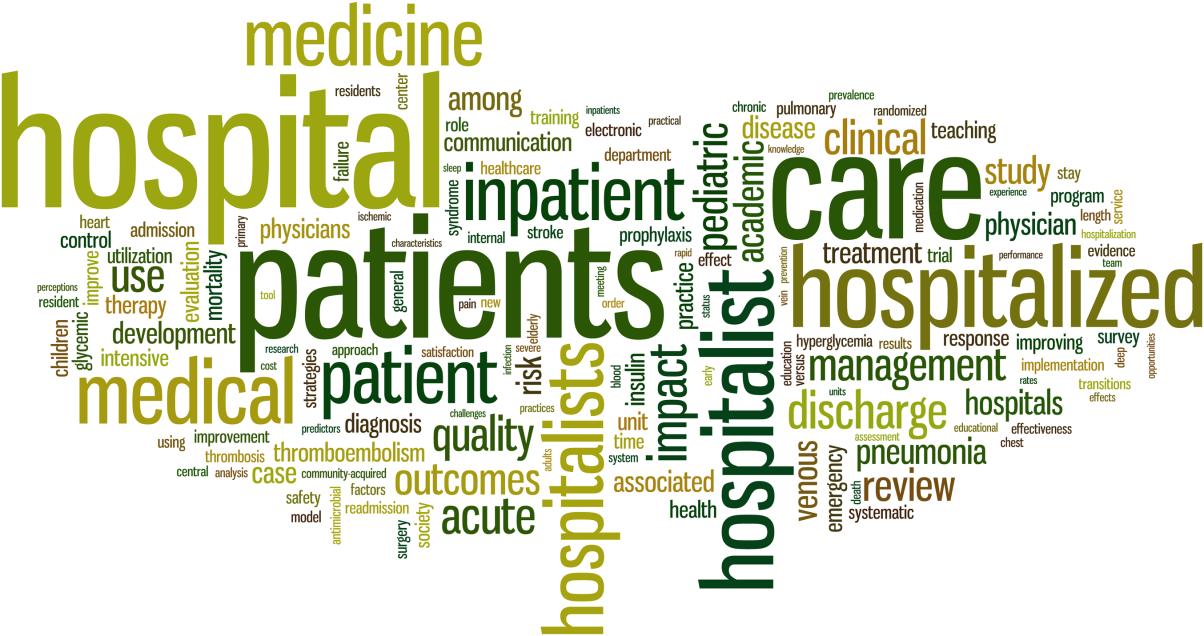
The Journal of Hospital Medicine's articles are also being read and cited more and more often. The top 10 most downloaded articles are ones that represent a wide range of topics of clinical and operational importance to the field (Table 1), and the 10 most cited articles (Table 2) represent how the scholarship being produced by the field of hospital medicine is being used to advance other scientific and policy initiatives. Only 1 article[1] was listed in both places, demonstrating the difference between what the Journal of Hospital Medicine publishes as a way to help hospitalists provide better care on a day‐to‐day basis and what the journal publishes to advance the field.
Article Title | Total Downloads |
---|---|
1. Making inpatient medication reconciliation patient centered, clinically relevant, and implementable: a consensus statement on key principles and necessary first steps[3] | 9,766 |
2. Promoting effective transitions of care at hospital discharge: a review of key issues for hospitalists[1] | 4,243 |
3. The key principles and characteristics of an effective hospital medicine group: an assessment guide for hospitals and hospitalists[4] | 3,722 |
4. Observation and inpatient status: clinical impact of the 2‐midnight rule[5] | 2,587 |
5. Measuring the modified early warning score and the Rothman Index: advantages of utilizing the electronic medical record in an early warning system[6] | 1,923 |
6. Acute coronary syndrome update for the hospitalist[7] | 1,514 |
7. Hospital performance trends on national quality measures and the association with Joint Commission accreditation[8] | 1,505 |
8. The core competencies in hospital medicine: a framework for curriculum development by the Society of Hospital Medicine[9] | 1,218 |
9. Iliac vein compression syndrome: an underdiagnosed cause of lower extremity deep venous thrombosis[10] | 1,135 |
10. Aspirin versus anticoagulation for prevention of venous thromboembolism major lower extremity orthopedic surgery: a systematic review and meta‐analysis[11] | 1,069 |
Title | Total Citations |
---|---|
1. Promoting effective transitions of care at hospital discharge: a review of key issues for hospitalists[1] | 152 |
2. Managing discontinuity in academic medical centers: strategies for a safe and effective resident sign‐out[12] | 110 |
3, Reduction of 30‐day postdischarge hospital readmission or emergency department (ED) visit rates in high‐risk elderly medical patients through delivery of a targeted care bundle[13] | 69 |
4. Mastery learning of thoracentesis skills by internal medicine residents using simulation technology and deliberate practice[14] | 61 |
5. Use of simulation‐based mastery learning to improve the quality of central venous catheter placement in a medical intensive care unit[15] | 60 |
6. Transition of care for hospitalized elderly patientsdevelopment of a discharge checklist for hospitalists[16] | 59 |
7. Hospitalist handoffs: a systematic review and task force recommendations[17] | 54 |
8. Inpatient management of diabetes and hyperglycemia among general medicine patients at a large teaching hospital[18] | 53 |
9. Transitions of care consensus policy statement: American College of Physicians, Society of General Internal Medicine, Society of Hospital Medicine, American Geriatrics Society, American College of Emergency Physicians, and Society for Academic Emergency Medicine[19] | 50 |
10. Diabetes care in hospitalized noncritically ill patients: more evidence for clinical inertia and negative therapeutic momentum[20] | 49 |
Our 10th volume will continue the work of promoting scholarship and inquiry in hospital medicine by retaining a clear focus on the science that represents the best of an intellectual agenda[2] for our field. To achieve this important goal, the Journal of Hospital Medicine and the field will need to develop durable evidence for not only how hospitalists deliver care, but also evidence for how care can be improved by providing better treatments. Stated differently, hospitalists and the Journal of Hospital Medicine will need to be focused not only on improving the healthcare system, but also on evaluating new technologies, drugs, and devices that can be used in a value‐focused health system.
In 2015, the Journal of Hospital Medicine will also be launching a new series focusing on improving healthcare value. Titled Choosing Wisely: Next Steps in Improving Healthcare Value, this series of invited reviews will cover important topics needed to frame approaches to reducing healthcare costs while improving care quality and safety. This American Board of Internal Medicine‐sponsored series will be accompanied by a parallel series titled Things We Do for No Reason. Things We Do will provide a series of case studies of tests, medications, or procedures hospitalists encounter every day. We hope these topics, along with the Society of Hospital Medicine's Choosing Wisely focus areas, will continue to outline useful opportunities to improve healthcare value at the bedside or at least frame what we expect will be a lively debate.
We are confident that the combination of highest‐quality peer review and new article offerings will only enhance our ability to share the highest‐quality research, perspectives, reviews, and clinical cases and conundrums with Journal of Hospital Medicine readers. The journal's editors and I look forward to this new year, the Journal of Hospital Medicine's 10th volume, and to many volumes of the Journal of Hospital Medicine to come.
- Promoting effective transitions of care at hospital discharge: a review of key issues for hospitalists. J Hosp Med. 2007;2(5):314–323. , , , .
- An intellectual agenda for hospitalists: lessons from bloodletting. J Hosp Med. 2013;8(7):418–419. .
- Making inpatient medication reconciliation patient centered, clinically relevant and implementable: a consensus statement on key principles and necessary first steps. J Hosp Med. 2010;5(8):477–485. , , , et al.
- The key principles and characteristics of an effective hospital medicine group: an assessment guide for hospitals and hospitalists. J Hosp Med. 2014;9(2):123–128. , , , et al.
- Observation and inpatient status: clinical impact of the 2‐midnight rule. J Hosp Med. 2014;9(4):203–209. , , , et al.
- Measuring the modified early warning score and the Rothman Index: advantages of utilizing the electronic medical record in an early warning system. J Hosp Med. 2014;9(2):116–119. , , .
- Acute coronary syndrome update for hospitalists. J Hosp Med. 2010;5(suppl 4):S15–S21. .
- Hospital performance trends on national quality measures and the association with Joint Commission accreditation. J Hosp Med. 2011;6(8):454–461. , , , , .
- The core competencies in hospital medicine: a framework for curriculum development by the society of hospital medicine. J Hosp Med. 2006;1(suppl 1):2–95.
- Iliac vein compression syndrome: an underdiagnosed cause of lower extremity deep venous thrombosis. J Hosp Med. 2010;5(7):E12–E13. , , , .
- Aspirin versus anticoagulation for prevention of venous thromboembolism major lower extremity orthopedic surgery: a systematic review and meta‐analysis. J Hosp Med. 2014;9(9):579–585. , , , , , .
- Managing discontinuity in academic medical centers: strategies for a safe and effective resident sign‐out. J Hosp Med. 2006;1(4):257–266. , , , , .
- Reduction of 30‐day postdischarge hospital readmission or emergency department (ED) visit rates in high‐risk elderly medical patients through delivery of a targeted care bundle. J Hosp Med. 2009;4(4):211–218. , , , et al.
- Mastery learning of thoracentesis skills by internal medicine residents using simulation technology and deliberate practice. J Hosp Med. 2008;3(1):48–54. , , , , .
- Use of simulation‐based mastery learning to improve the quality of central venous catheter placement in a medical intensive care unit. J Hosp Med. 2009;4(7):397–403. , , , , .
- Transition of care for hospitalized elderly patients—development of a discharge checklist for hospitalists. J Hosp Med. 2006;1(6):354–360. , , , et al.
- Hospitalist handoffs: a systematic review and task force recommendations. J Hosp Med. 2009;4(7):433–440. , , , , , .
- Inpatient management of diabetes and hyperglycemia among general medicine patients at a large teaching hospital. J Hosp Med. 2006;1(3):145–150. , , , , .
- Transitions of Care Consensus policy statement: American College of Physicians, Society of General Internal Medicine, Society of Hospital Medicine, American Geriatrics Society, American College of Emergency Physicians, and Society for Academic Emergency Medicine. J Hosp Med. 2009;4(6):364–370. , , , et al.
- Diabetes care in hospitalized noncritically ill patients: more evidence for clinical inertia and negative therapeutic momentum. J Hosp Med. 2007;2(4):203–211. , , , et al.
- Promoting effective transitions of care at hospital discharge: a review of key issues for hospitalists. J Hosp Med. 2007;2(5):314–323. , , , .
- An intellectual agenda for hospitalists: lessons from bloodletting. J Hosp Med. 2013;8(7):418–419. .
- Making inpatient medication reconciliation patient centered, clinically relevant and implementable: a consensus statement on key principles and necessary first steps. J Hosp Med. 2010;5(8):477–485. , , , et al.
- The key principles and characteristics of an effective hospital medicine group: an assessment guide for hospitals and hospitalists. J Hosp Med. 2014;9(2):123–128. , , , et al.
- Observation and inpatient status: clinical impact of the 2‐midnight rule. J Hosp Med. 2014;9(4):203–209. , , , et al.
- Measuring the modified early warning score and the Rothman Index: advantages of utilizing the electronic medical record in an early warning system. J Hosp Med. 2014;9(2):116–119. , , .
- Acute coronary syndrome update for hospitalists. J Hosp Med. 2010;5(suppl 4):S15–S21. .
- Hospital performance trends on national quality measures and the association with Joint Commission accreditation. J Hosp Med. 2011;6(8):454–461. , , , , .
- The core competencies in hospital medicine: a framework for curriculum development by the society of hospital medicine. J Hosp Med. 2006;1(suppl 1):2–95.
- Iliac vein compression syndrome: an underdiagnosed cause of lower extremity deep venous thrombosis. J Hosp Med. 2010;5(7):E12–E13. , , , .
- Aspirin versus anticoagulation for prevention of venous thromboembolism major lower extremity orthopedic surgery: a systematic review and meta‐analysis. J Hosp Med. 2014;9(9):579–585. , , , , , .
- Managing discontinuity in academic medical centers: strategies for a safe and effective resident sign‐out. J Hosp Med. 2006;1(4):257–266. , , , , .
- Reduction of 30‐day postdischarge hospital readmission or emergency department (ED) visit rates in high‐risk elderly medical patients through delivery of a targeted care bundle. J Hosp Med. 2009;4(4):211–218. , , , et al.
- Mastery learning of thoracentesis skills by internal medicine residents using simulation technology and deliberate practice. J Hosp Med. 2008;3(1):48–54. , , , , .
- Use of simulation‐based mastery learning to improve the quality of central venous catheter placement in a medical intensive care unit. J Hosp Med. 2009;4(7):397–403. , , , , .
- Transition of care for hospitalized elderly patients—development of a discharge checklist for hospitalists. J Hosp Med. 2006;1(6):354–360. , , , et al.
- Hospitalist handoffs: a systematic review and task force recommendations. J Hosp Med. 2009;4(7):433–440. , , , , , .
- Inpatient management of diabetes and hyperglycemia among general medicine patients at a large teaching hospital. J Hosp Med. 2006;1(3):145–150. , , , , .
- Transitions of Care Consensus policy statement: American College of Physicians, Society of General Internal Medicine, Society of Hospital Medicine, American Geriatrics Society, American College of Emergency Physicians, and Society for Academic Emergency Medicine. J Hosp Med. 2009;4(6):364–370. , , , et al.
- Diabetes care in hospitalized noncritically ill patients: more evidence for clinical inertia and negative therapeutic momentum. J Hosp Med. 2007;2(4):203–211. , , , et al.
Evidence Needing a Lift
In this issue of the Journal of Hospital Medicine, Hansen and colleagues provide a first, early look at the effectiveness of the BOOST intervention to reduce 30‐day readmissions among hospitalized patients.[1] BOOST[2] is 1 of a number of care transition improvement methodologies that have been applied to the problem of readmissions, each of which has evidence to support its effectiveness in its initial settings[3, 4] but has proven to be difficult to translate to other sites.[5, 6, 7]
BOOST stands in contrast with other, largely research protocol‐derived, programs in that it allows sites to tailor adoption of recommendations to local contexts and is therefore potentially more feasible to implement. Feasibility and practicality has led BOOST to be adopted in large national settings, even if it has had little evidence to support its effectiveness to date.
Given the nonstandardized and ad hoc nature of most multicenter collaboratives generally, and the flexibility of the BOOST model specifically, the BOOST authors are to be commended for undertaking any evaluation at all. Perhaps, not surprisingly, they encountered many of the problems associated with a multicenter studydropout of sites, problematic data, and limited evidence for adoption of the intervention at participating hospitals. Although these represent real‐world experiences of a quality‐improvement program, as a group they pose a number of problems that limit the study's robustness, and generate important caveats that readers should use to temper their interpretation of the authors' findings.
The first caveat relates to the substantial number of sites that either dropped out of BOOST or failed to submit data after enlisting in the collaborative. Although this may be common in quality improvement collaboratives, similar problems would not be permissible in a trial of a new drug or device. Dropout and selected ability to contribute data suggest that the ability to fully adopt BOOST may not be universal, and raises the possibility of bias, because the least successful sites may have had less interest in remaining engaged and submitting data.
The second caveat relates to how readmission rates were assessed. Because sites provided rates of readmissions at the unit level rather than the actual counts of admissions or readmissions, the authors were unable to conduct statistical analyses typically performed for these interventions, such as time series or difference‐in‐difference analyses. More importantly, one cannot discern whether their results are driven by a small absolute but large relative change in the number of readmissions at small sites. That is, large percentage changes of low statistical significance could have misleadingly affected the overall results. Conversely, we cannot identify large sites where a similar relative reduction could be statistically significant and more broadly interpreted as representing the real effectiveness of BOOST efforts.
The third caveat is in regard to the data describing the sites' performance. The effectiveness of BOOST in this analysis varied greatly among sites, with only 1 site showing a strong reduction in readmission rate, and nearly all others showing no statistical improvements. In fact, it appears that their overall results were almost entirely driven by the improvements at that 1 site.
Variable effectiveness of an intervention can be related to variable adoption or contextual factors (such as availability of personnel to implement the program). Although these authors have data on BOOST programmatic adoption, they do not have qualitative data on local barriers and facilitators to BOOST implementation, which at this stage of evaluation would be particularly valuable in understanding the results. Analyzing site‐level effectiveness is of growing relevance to multicenter quality improvement collaboratives,[8, 9] but this evaluation provides little insight into reasons for variable success across institutions.
Finally, their study design does not allow us to understand a number of key questions. How many patients were involved in the intervention? How many patients received all BOOST‐recommended interventions? Which of these interventions seemed most effective in which patients? To what degree did patient severity of illness, cognitive status, social supports, or access to primary care influence readmission risk? Such information would help frame cost‐effective deployment of BOOST or related tools.
In the end, it seems unlikely that this iteration of the BOOST program produced broad reductions in readmission rates. Having said this, the authors provide the necessary start down the road toward a fuller understanding of real‐world efforts to reduce readmissions. Stated alternately, the nuances and flaws of this study provide ample fodder for others working in the field. BOOST is in good stead with other care transition models that have not translated well from their initial research environment to real‐world practices. The question now is: Do any of these interventions actually work in clinical practice settings, and will we ever know? Even more fundamentally, how important and meaningful are these hospital‐based care transition interventions? Where is the engagement with primary care? Where are the primary care outcomes? Does BOOST truly impact outcomes other than readmission?[10]
Doing high‐quality research in the context of a rapidly evolving quality improvement program is hard. Doing it at more than 1 site is harder. BOOST's flexibility is both a great source of strength and a clear challenge to rigorous evaluation. However, when the costs of care transition programs are so high, and the potential consequences of high readmission rates are so great for patients and for hospitals, the need to address these issues with real data and better evidence is paramount. We look forward to the next phase of BOOST and to the growth and refinement of the evidence base for how to improve care coordination and transitions effectively.
- J Hosp Med. 2013;8:421–427. , , , et al. Project BOOST: effectiveness of a multihospital effort to reduce rehospitalization.
- BOOSTing the hospital discharge. J Hosp Med. 2009;4:209–210. , .
- A reengineered hospital discharge program to decrease rehospitalization: a randomized trial. Ann Intern Med. 2009;150:178–187. , , , et al.
- Comprehensive discharge planning and home follow‐up of hospitalized elders: a randomized clinical trial. JAMA. 1999;281:613–620. , , , et al.
- Effectiveness and cost of a transitional care program for heart failure: a prospective study with concurrent controls. Arch Intern Med. 2011;171:1238–1243. , , , et al.
- Hospitals question Medicare rules on readmissions. New York Times. March 29, 2013. Available at: http://www.nytimes.com/2013/03/30/business/hospitals‐question‐fairness‐of‐new‐medicare‐rules.html?pagewanted=all .
In this issue of the Journal of Hospital Medicine, Hansen and colleagues provide a first, early look at the effectiveness of the BOOST intervention to reduce 30‐day readmissions among hospitalized patients.[1] BOOST[2] is 1 of a number of care transition improvement methodologies that have been applied to the problem of readmissions, each of which has evidence to support its effectiveness in its initial settings[3, 4] but has proven to be difficult to translate to other sites.[5, 6, 7]
BOOST stands in contrast with other, largely research protocol‐derived, programs in that it allows sites to tailor adoption of recommendations to local contexts and is therefore potentially more feasible to implement. Feasibility and practicality has led BOOST to be adopted in large national settings, even if it has had little evidence to support its effectiveness to date.
Given the nonstandardized and ad hoc nature of most multicenter collaboratives generally, and the flexibility of the BOOST model specifically, the BOOST authors are to be commended for undertaking any evaluation at all. Perhaps, not surprisingly, they encountered many of the problems associated with a multicenter studydropout of sites, problematic data, and limited evidence for adoption of the intervention at participating hospitals. Although these represent real‐world experiences of a quality‐improvement program, as a group they pose a number of problems that limit the study's robustness, and generate important caveats that readers should use to temper their interpretation of the authors' findings.
The first caveat relates to the substantial number of sites that either dropped out of BOOST or failed to submit data after enlisting in the collaborative. Although this may be common in quality improvement collaboratives, similar problems would not be permissible in a trial of a new drug or device. Dropout and selected ability to contribute data suggest that the ability to fully adopt BOOST may not be universal, and raises the possibility of bias, because the least successful sites may have had less interest in remaining engaged and submitting data.
The second caveat relates to how readmission rates were assessed. Because sites provided rates of readmissions at the unit level rather than the actual counts of admissions or readmissions, the authors were unable to conduct statistical analyses typically performed for these interventions, such as time series or difference‐in‐difference analyses. More importantly, one cannot discern whether their results are driven by a small absolute but large relative change in the number of readmissions at small sites. That is, large percentage changes of low statistical significance could have misleadingly affected the overall results. Conversely, we cannot identify large sites where a similar relative reduction could be statistically significant and more broadly interpreted as representing the real effectiveness of BOOST efforts.
The third caveat is in regard to the data describing the sites' performance. The effectiveness of BOOST in this analysis varied greatly among sites, with only 1 site showing a strong reduction in readmission rate, and nearly all others showing no statistical improvements. In fact, it appears that their overall results were almost entirely driven by the improvements at that 1 site.
Variable effectiveness of an intervention can be related to variable adoption or contextual factors (such as availability of personnel to implement the program). Although these authors have data on BOOST programmatic adoption, they do not have qualitative data on local barriers and facilitators to BOOST implementation, which at this stage of evaluation would be particularly valuable in understanding the results. Analyzing site‐level effectiveness is of growing relevance to multicenter quality improvement collaboratives,[8, 9] but this evaluation provides little insight into reasons for variable success across institutions.
Finally, their study design does not allow us to understand a number of key questions. How many patients were involved in the intervention? How many patients received all BOOST‐recommended interventions? Which of these interventions seemed most effective in which patients? To what degree did patient severity of illness, cognitive status, social supports, or access to primary care influence readmission risk? Such information would help frame cost‐effective deployment of BOOST or related tools.
In the end, it seems unlikely that this iteration of the BOOST program produced broad reductions in readmission rates. Having said this, the authors provide the necessary start down the road toward a fuller understanding of real‐world efforts to reduce readmissions. Stated alternately, the nuances and flaws of this study provide ample fodder for others working in the field. BOOST is in good stead with other care transition models that have not translated well from their initial research environment to real‐world practices. The question now is: Do any of these interventions actually work in clinical practice settings, and will we ever know? Even more fundamentally, how important and meaningful are these hospital‐based care transition interventions? Where is the engagement with primary care? Where are the primary care outcomes? Does BOOST truly impact outcomes other than readmission?[10]
Doing high‐quality research in the context of a rapidly evolving quality improvement program is hard. Doing it at more than 1 site is harder. BOOST's flexibility is both a great source of strength and a clear challenge to rigorous evaluation. However, when the costs of care transition programs are so high, and the potential consequences of high readmission rates are so great for patients and for hospitals, the need to address these issues with real data and better evidence is paramount. We look forward to the next phase of BOOST and to the growth and refinement of the evidence base for how to improve care coordination and transitions effectively.
In this issue of the Journal of Hospital Medicine, Hansen and colleagues provide a first, early look at the effectiveness of the BOOST intervention to reduce 30‐day readmissions among hospitalized patients.[1] BOOST[2] is 1 of a number of care transition improvement methodologies that have been applied to the problem of readmissions, each of which has evidence to support its effectiveness in its initial settings[3, 4] but has proven to be difficult to translate to other sites.[5, 6, 7]
BOOST stands in contrast with other, largely research protocol‐derived, programs in that it allows sites to tailor adoption of recommendations to local contexts and is therefore potentially more feasible to implement. Feasibility and practicality has led BOOST to be adopted in large national settings, even if it has had little evidence to support its effectiveness to date.
Given the nonstandardized and ad hoc nature of most multicenter collaboratives generally, and the flexibility of the BOOST model specifically, the BOOST authors are to be commended for undertaking any evaluation at all. Perhaps, not surprisingly, they encountered many of the problems associated with a multicenter studydropout of sites, problematic data, and limited evidence for adoption of the intervention at participating hospitals. Although these represent real‐world experiences of a quality‐improvement program, as a group they pose a number of problems that limit the study's robustness, and generate important caveats that readers should use to temper their interpretation of the authors' findings.
The first caveat relates to the substantial number of sites that either dropped out of BOOST or failed to submit data after enlisting in the collaborative. Although this may be common in quality improvement collaboratives, similar problems would not be permissible in a trial of a new drug or device. Dropout and selected ability to contribute data suggest that the ability to fully adopt BOOST may not be universal, and raises the possibility of bias, because the least successful sites may have had less interest in remaining engaged and submitting data.
The second caveat relates to how readmission rates were assessed. Because sites provided rates of readmissions at the unit level rather than the actual counts of admissions or readmissions, the authors were unable to conduct statistical analyses typically performed for these interventions, such as time series or difference‐in‐difference analyses. More importantly, one cannot discern whether their results are driven by a small absolute but large relative change in the number of readmissions at small sites. That is, large percentage changes of low statistical significance could have misleadingly affected the overall results. Conversely, we cannot identify large sites where a similar relative reduction could be statistically significant and more broadly interpreted as representing the real effectiveness of BOOST efforts.
The third caveat is in regard to the data describing the sites' performance. The effectiveness of BOOST in this analysis varied greatly among sites, with only 1 site showing a strong reduction in readmission rate, and nearly all others showing no statistical improvements. In fact, it appears that their overall results were almost entirely driven by the improvements at that 1 site.
Variable effectiveness of an intervention can be related to variable adoption or contextual factors (such as availability of personnel to implement the program). Although these authors have data on BOOST programmatic adoption, they do not have qualitative data on local barriers and facilitators to BOOST implementation, which at this stage of evaluation would be particularly valuable in understanding the results. Analyzing site‐level effectiveness is of growing relevance to multicenter quality improvement collaboratives,[8, 9] but this evaluation provides little insight into reasons for variable success across institutions.
Finally, their study design does not allow us to understand a number of key questions. How many patients were involved in the intervention? How many patients received all BOOST‐recommended interventions? Which of these interventions seemed most effective in which patients? To what degree did patient severity of illness, cognitive status, social supports, or access to primary care influence readmission risk? Such information would help frame cost‐effective deployment of BOOST or related tools.
In the end, it seems unlikely that this iteration of the BOOST program produced broad reductions in readmission rates. Having said this, the authors provide the necessary start down the road toward a fuller understanding of real‐world efforts to reduce readmissions. Stated alternately, the nuances and flaws of this study provide ample fodder for others working in the field. BOOST is in good stead with other care transition models that have not translated well from their initial research environment to real‐world practices. The question now is: Do any of these interventions actually work in clinical practice settings, and will we ever know? Even more fundamentally, how important and meaningful are these hospital‐based care transition interventions? Where is the engagement with primary care? Where are the primary care outcomes? Does BOOST truly impact outcomes other than readmission?[10]
Doing high‐quality research in the context of a rapidly evolving quality improvement program is hard. Doing it at more than 1 site is harder. BOOST's flexibility is both a great source of strength and a clear challenge to rigorous evaluation. However, when the costs of care transition programs are so high, and the potential consequences of high readmission rates are so great for patients and for hospitals, the need to address these issues with real data and better evidence is paramount. We look forward to the next phase of BOOST and to the growth and refinement of the evidence base for how to improve care coordination and transitions effectively.
- J Hosp Med. 2013;8:421–427. , , , et al. Project BOOST: effectiveness of a multihospital effort to reduce rehospitalization.
- BOOSTing the hospital discharge. J Hosp Med. 2009;4:209–210. , .
- A reengineered hospital discharge program to decrease rehospitalization: a randomized trial. Ann Intern Med. 2009;150:178–187. , , , et al.
- Comprehensive discharge planning and home follow‐up of hospitalized elders: a randomized clinical trial. JAMA. 1999;281:613–620. , , , et al.
- Effectiveness and cost of a transitional care program for heart failure: a prospective study with concurrent controls. Arch Intern Med. 2011;171:1238–1243. , , , et al.
- Hospitals question Medicare rules on readmissions. New York Times. March 29, 2013. Available at: http://www.nytimes.com/2013/03/30/business/hospitals‐question‐fairness‐of‐new‐medicare‐rules.html?pagewanted=all .
- J Hosp Med. 2013;8:421–427. , , , et al. Project BOOST: effectiveness of a multihospital effort to reduce rehospitalization.
- BOOSTing the hospital discharge. J Hosp Med. 2009;4:209–210. , .
- A reengineered hospital discharge program to decrease rehospitalization: a randomized trial. Ann Intern Med. 2009;150:178–187. , , , et al.
- Comprehensive discharge planning and home follow‐up of hospitalized elders: a randomized clinical trial. JAMA. 1999;281:613–620. , , , et al.
- Effectiveness and cost of a transitional care program for heart failure: a prospective study with concurrent controls. Arch Intern Med. 2011;171:1238–1243. , , , et al.
- Hospitals question Medicare rules on readmissions. New York Times. March 29, 2013. Available at: http://www.nytimes.com/2013/03/30/business/hospitals‐question‐fairness‐of‐new‐medicare‐rules.html?pagewanted=all .
Redefining Readmission Risk Factors
Within Medicare recipients, an astounding one in five medical patients (19.6%) is readmitted within 30 days, accounting for $15 billion in spending.1, 2 Amidst the current healthcare system crisis, reducing these hospital readmissions has risen to the highest priority. Reducing readmissions is the newest addition to multiple quality dashboards, both institutional and national, as a measure of the care delivered during hospitalization.3 One of the most notable of these reporting entities, Hospital Compare, now publicly reports Medicare readmission rates for a few common diagnoses.4 While Medicare already withholds payment to hospitals for readmissions within 24 hours for the same diagnosis, Medicare may soon reduce payment to hospitals with the highest rates of readmission within 30 days, a powerful incentive for hospitals to intervene. Readmissions have also reached the radar of additional stakeholders, even making its way onto Obama's budget considerations, given the potential cost savings to the system overall.5
To develop systems which reduce readmissions, one must first gain understanding of the characteristics of readmissions. A few clinical risk factors (such as age, number of prior admissions, and comorbidities) have been well defined in subgroups of general medicine inpatients.612 Likewise, interventions aiming to reduce readmissions have also focused on subgroups, excluding a large portion of hospitalized patients (for example, non‐English speakers and younger patients).1320 Other data have been derived in veterans or within non‐US populations that have inherently different payer, race, ethnicity, and primary language composition, and may not be applicable outside those settings.7, 8, 10, 11, 21 Lastly, little is known regarding risk that may be associated with operational factors, such as weekend discharge or admission source. As a result, there are few data describing the clinical, operational, and demographic factors associated with readmission in a heterogeneous population of hospitalized general medicine patientsthe patient population of most generalists in the United States.
To understand the impact of a variety of risk factors in a diverse general medicine population, we evaluated the characteristics of readmitted patients in a large urban university medical center over a 2‐year period. We hypothesized that a number of clinical, operational, and sociodemographic factors would be associated with readmission.
Methods
Sites and Subjects
Our data were collected on general medicine patients during hospitalization between June 1, 2006 and May 31, 2008, at the University of California San Francisco. The University of California, San Francisco (UCSF) Medical Center is composed of Moffitt‐Long Hospital (a 400‐bed center) and UCSF‐Mount Zion Hospital (a 200‐bed facility) located in San Francisco, CA.
Medical patients at Moffitt‐Long Hospital are admitted to 1 of 8 medical teams composed of a resident, 1 to 2 interns, and 0 to 3 medical students, supervised by an attending physician who is most often a hospitalist. At Moffitt‐Long Hospital, housestaff write all orders and provide 24‐hour coverage to inpatients. Mount Zion medical patients are cared for by 1 of 2 teams and staffed by a hospitalist on each team who is responsible for all elements of care. Both services care for common inpatient diagnoses, as well as specialty‐associated diagnoses such as cancer, pneumonia, and chronic obstructive pulmonary disease (COPD). Of note, at Moffitt‐Long Hospital, those patients with primary cardiac diagnoses are cared for by a separate team composed of housestaff and students supervised by a cardiologist.
The discharge process at both sites utilizes a multidisciplinary teamincluding physicians, case managers, nurses, pharmacists, and discharge coordinatorsworking in concert. Key components include arranging follow‐up care, faxing the discharge summary to the primary care provider, and educating the patient and caregivers, especially regarding medications. While these goals are clearly delineated, significant variability exists in how these tasks are actually accomplished. The multidisciplinary approach, components of the discharge process, and lack of a systematic approach are representative of the discharge process around the country.22
Data
Data regarding patient demographics, age, comorbidities, and insurance status were collected from administrative data systems at UCSF, reflecting the patient's status at the time of index admission. These same systems were used to collect a date‐stamped log of all medications (eg, anticoagulants) for which the patient was billed during the last 48 hours of hospitalization. Specifically, data were obtained for medications previously shown to cause adverse drug events following hospital discharge.23, 24 These medication groups include corticosteroids, anticoagulants, antibiotics, narcotics, nonsteroidal anti‐inflammatory drugs (NSAIDs), cardiovascular medications, antiepileptics, anticholinergics, antidepressants, and antidiabetics. Operational factors that we hypothesized would affect readmission risk included admission source, discharge disposition, and weekday vs. weekend discharge. Case management, social work, and pharmacy services operate with limited staffing on weekends. Likewise, resident and intern physicians are more likely to be off on a weekend day than a weekday; covering attending physicians care for about half of patients during the weekend. Data were obtained from Transition Systems International (TSI, Boston, MA) administrative databases, a cost‐accounting system that collects data abstracted from patient charts upon discharge from UCSF.
Definition of Readmission Measure
Using TSI, we detected readmission to UCSF by screening for any inpatient encounters on any service (not just medicine) within the 30 days following discharge from the general medicine service at the 2 UCSF campuses. We excluded elective readmissions, such as for scheduled chemotherapy. Patients who died at the index admission were excluded from the cohort.
Adjustment Variables
Age, gender, payer status and APR risk of mortality (3M Health Information Systems, St. Paul, MN) were collected from administrative data. The All Patient Refined (APR) risk of mortality is the all patients risk of mortality score developed by 3M which divides patients into 4 subclasses of risk based on clinical problems and comorbidities.25 We used secondary diagnosis codes in administrative data to classify comorbidities using the method of Elixhauser.26
Using the log of medication charges, we grouped high‐risk medications according to the classification scheme of Forster et al.23 and Hanlon et al.24 We then created a count representing the total number of medications administered to patients within the final 48 hours of stay.
Analysis
We first described study patients and hospitals using univariable methods. Multivariable generalized estimating equations (SAS PROC GENMOD) were used to account for clustering of patients within physicians and calculate adjusted odds ratios (ORs). As there were 2 sites within UCSF Medical Center (Moffitt‐Long and Mount Zion hospitals), we included site as a fixed effect in our model. Models were constructed using manual variable selection methods with final selection being made based on whether the covariate was associated with readmission at P < 0.05. All analyses were carried out using SAS version 9.2 (SAS Institute, Inc. Cary, NC).
Results
Baseline Characteristics
During the 2‐year accrual period, 295 attending physicians admitted 6805 unique patients for a total of 10,359 admissions. Seventeen percent of these 10,359 admissions were readmitted within 30 days. The cohort of all patients had a mean age of 59.6 years 19.5 standard deviation (SD), with 52.8% women. The mean length of stay was 5.6 days 10.4 SD. Medicare was the payer source for approximately half of the admissions. The majority of admissions (90.4%) were billed for at least 1 high risk medication, with narcotics, cardiac medications, and antibiotics being the most common. Regarding disposition, 79.5% of admissions were discharged to home; 9.1% were discharged to a skilled nursing facility (SNF).
Baseline sociodemographic, operational, and clinical characteristics for patients readmitted and not readmitted are shown in Table 1. Demographic characteristics with significant differences (P < 0.05) between readmitted and nonreadmitted groups included mean age, race, payer status, and primary language other than English. Regarding operational characteristics, readmitted patients had a higher median length of stay and were more likely to be admitted through the emergency room during their index admission. Discharge to an SNF was higher in the readmitted group versus the nonreadmitted group (9.7% vs. 9.0%). Several clinical factors were more prevalent in the readmitted group: high‐risk medications, specifically steroids, narcotics, and cardiovascular medications; high‐risk medication count of 3 or greater; and comorbidities including congestive heart failure, renal disease, cancer, anemia, and depression.
Characteristic | Patients Readmitted (n = 1762 17.0%), n (%) | Patients Not Readmitted (n = 8597 83.0%), n (%) | P Value |
---|---|---|---|
| |||
Mean age (years) (SD) | 58.8 (19.3) | 59.8 (19.6) | 0.0491 |
Female | 930 (52.8) | 4548 (52.9) | 0.9260 |
Race* | |||
White | 785 (44.6) | 4166 (48.8) | <0.0001 |
Black | 442 (25.1) | 1401 (16.4) | |
Asian | 323 (18.4) | 1726 (20.2) | |
Other | 209 (11.9) | 1240 (14.5) | |
Hispanic ethnicity | 140 (8.1) | 734 (8.9) | 0.2737 |
Payer status | |||
Medicare | 905 (51.4) | 4266 (49.6) | <0.0001 |
Medicaid/Medi‐cal | 458 (26.0) | 1578 (18.4) | |
Private | 370 (21.0) | 2092 (24.3) | |
Other | 29 (1.7) | 661 (7.7) | |
Primary language other than English | 242 (17.1) | 1394 (19.5) | 0.0359 |
Median length of stay (days) (interquartile range) | 4 (2, 7) | 3 (2, 6) | <0.0001 |
Admit source | |||
Emergency room | 1506 (85.5) | 6898 (80.2) | <0.0001 |
Outside hospital | 38 (2.2) | 271 (3.2) | |
Direct admission/other (jail) | 218 (12.4) | 1428 (16.6) | |
Discharge to | |||
Home | 1461 (82.9) | 6773 (78.8) | <0.0001 |
SNF | 170 (9.7) | 774 (9.0) | |
Other | 131 (7.4) | 1050 (12.2) | |
Discharged on weekend | 381 (21.6) | 1904 (22.1) | 0.6288 |
Patient medications | |||
Any high‐risk medication | 1679 (95.3) | 7684 (89.4) | <0.0001 |
High‐risk medication count | |||
02 | 577 (32.8) | 3666 (42.6) | <0.0001 |
34 | 692 (39.3) | 2968 (34.5) | |
5 | 493 (28) | 1963 (22.8) | |
Any corticosteroids | 399 (22.6) | 1571 (18.3) | <0.0001 |
Anticoagulant | 120 (6.8) | 559 (6.5) | 0.6340 |
Any antibiotic | 904 (51.3) | 4203 (48.9) | 0.0646 |
Any narcotic | 1036 (58.8) | 4206 (48.9) | <0.0001 |
Any NSAID | 68 (3.9) | 320 (3.7) | 0.7826 |
Any cardiovascular med | 887 (50.3) | 3806 (44.3) | <0.0001 |
Any antiepileptic | 93 (5.3) | 470 (5.5) | 0.7500 |
Any anticholinergic | 47 (2.7) | 354 (4.1) | 0.0040 |
Any antidepressant | 455 (25.8) | 1863 (25.8) | 0.0001 |
Any antidiabetic | 198 (11.2) | 994 (11.6) | 0.6970 |
Elixhauser comorbidities | |||
Congestive heart failure | 219 (12.4) | 795 (9.3) | <0.0001 |
Pulmonary circulation disease | 72 (4.1) | 178 (2.1) | <0.0001 |
Peripheral vascular disease | 84 (4.8) | 331 (3.9) | 0.0737 |
Hypertension | 745 (42.3) | 3741 (43.5) | 0.3411 |
Other neurological disease | 101 (5.7) | 696 (8.1) | 0.0007 |
Chronic pulmonary disease | 317 (18.0) | 1442 (16.8) | 0.2149 |
Diabetes | 303 (17.2) | 1333 (15.5) | 0.0762 |
Renal failure | 339 (19.2) | 1286 (15.0) | <0.0001 |
Liver disease | 188 (10.7) | 774 (9.0) | 0.0281 |
Metastatic cancer | 160 (9.1) | 530 (6.2) | <0.0001 |
Solid tumor w/o metastases | 100 (5.7) | 277 (3.2) | <0.0001 |
Rheumatoid arthritis/collagen vas | 81 (4.6) | 303 (3.5) | 0.0299 |
Weight loss | 153 (8.7) | 584 (6.8) | 0.0449 |
Deficiency anemia | 522 (29.6) | 1979 (23.0) | <0.0001 |
Alcohol abuse | 101 (5.7) | 428 (5.0) | 0.1905 |
Drug abuse | 148 (8.4) | 619 (7.2) | 0.0798 |
Depression | 244 (13.9) | 963 (11.2) | 0.0016 |
APR risk of mortality | |||
1 | 451 (25.6) | 3101 (36.1) | <0.0001 |
2 | 619 (35.1) | 2797 (32.5) | |
3 | 536 (30.4) | 1907 (22.2) | |
4 | 156 (8.9) | 792 (9.2) |
Frequency of Readmission
The 30‐day readmission rate was 17.0% (1762 patients), with 49.7% (875 patients) of the readmissions occurring within 10 days of discharge. Of patients readmitted, the general medicine service was the readmitting team in 78.2%. A quarter of readmissions (26.2%) had the same primary diagnosis on initial and repeat admission.
Factors Associated With Readmission
Factors associated with readmission were categorized as sociodemographic, operational, and clinical. Factors associated with readmission with P < 0.05 and present in at least 5% of admissions are presented in Table 2. Of the sociodemographic factors, black race was significantly associated with readmission. Within the Medicare cohort, risk for readmission was similar for white vs. nonwhite race, with relative risk of 1.0 (95% confidence interval [CI], 0.86‐1.18). Medicaid as payer status was significantly associated in the unadjusted model, and in the adjusted model showed a trend toward readmission. Mean age was significantly different in the readmitted and nonreadmitted groups, but the difference was small (1.0 year). Moreover, when we evaluated age in 5‐year categories (ex. 65‐70, 71‐75, etc.), age was not associated with readmission. In the adjusted model, none of the operational factors were significantly associated with readmission, including discharge to SNF, weekend discharge, or admit source.
Covariate | Unadjusted OR (95% CI) | Adjusted OR (95% CI) |
---|---|---|
| ||
Age | 1.00 (0.991.00) | 1.00 (0.991.00) |
Race | ||
White | Referent | Referent |
Black | 1.67 (1.471.91) | 1.43 (1.241.65) |
Asian | 0.99 (0.861.14) | 0.95 (0.821.11) |
Other | 0.89 (0.761.06) | 0.84 (0.671.06) |
Payer | ||
Medicare | Referent | Referent |
Medicaid/medical | 1.37 (1.211.55) | 1.15 (0.971.36) |
Private | 0.83 (0.730.95) | 0.78 (0.650.95) |
Other | 0.21 (0.140.30) | 0.23 (0.110.45) |
Disposition | ||
To home | Referent | Referent |
SNF | 1.02 (0.851.21) | 0.98 (0.821.18) |
Other | 0.58 (0.480.70) | 0.53 (0.430.66) |
Highrisk medications | ||
Corticosteroids | 1.31 (1.161.48) | 1.24 (1.091.42) |
Narcotics | 1.49 (1.341.65) | 1.33 (1.161.53) |
Anticholinergics | 0.64 (0.470.87) | 0.66 (0.480.90) |
Comorbidities | ||
Congestive heart failure | 1.39 (1.191.63) | 1.30 (1.091.56) |
Neurological disorders | 0.69 (0.560.86) | 0.70 (0.570.87) |
Renal failure | 1.35 (1.191.55) | 1.19 (1.051.36) |
Metastatic cancer | 1.52 (1.261.83) | 1.61 (1.331.95) |
Solid tumor w/o metastasis | 1.81 (1.432.29) | 1.95 (1.542.47) |
Deficiency anemia | 1.41 (1.261.58) | 1.27 (1.131.44) |
Weight loss | 1.30 (1.081.57) | 1.26 (1.091.47) |
Of the clinical factors, high‐risk medications and 6 comorbidities were associated with readmission. High‐risk medication categories associated with readmission were steroids and narcotics; anticholinergics medications were protective. The 6 comorbidities associated with readmission were congestive heart failure, renal disease, cancer (with and without metastasis), weight loss, and iron deficiency anemia. While APR risk of mortality was associated with readmission at P < 0.05, including APR in our final model did not alter which other factors were significantly associated with readmission. When site (Moffitt‐Long vs. Mount Zion Hospitals) was added to the model, the ORs for factors associated with readmission did not change appreciably (0.01).
Discussion
In this retrospective observational study of hospitalized patients, we found that readmission was common and associated with a number of risk factors that could be easily identified early in hospitalization. Nonclinical factors associated with readmission were black race and Medicaid payer status (in the unadjusted model). Clinical factors were high risk medications including steroids and narcotics; and comorbidities including congestive heart failure, renal disease, cancer, anemia, and weight loss. In contrast, other potential riskssuch as discharge on a weekend and discharge to an SNFwere not independently associated with readmission. This cohortwith a mix of clinical scenarios, payers, age, etc.represents the inherently heterogeneous population of inpatient general medicine across the country and abroad. Hospitalists provided care for over 65% of the general medicine service, again representative of the trend in US inpatient medicine.27, 28 Lastly, our cohort did not have the benefit of a systematic and consistent discharge process with interventions focused on reducing readmissions. This gap, which is common across hospitals, highlights the utility of this data in targeting quality improvement efforts.
Reducing risks for readmission requires identification of patient populations at highest risk; in those patients, one can further identify factors which are potentially modifiable via education or patient‐engagement interventions. While in the hospital, more intensive predischarge counseling and efforts to increase mobility may be most useful if targeted early and often on those at highest risk.15, 16, 29, 30 Finally, broader‐based support in the form of better home services, more access to longitudinal care, or targeted postdischarge efforts may be required.14, 31
Though current strategies focus largely on clinical risk factors, this study shows that nonclinical factors play an equally important but underappreciated role in contributing to readmission. While prior studies have shown variable results on association of black race with readmission,2, 9, 11 none have evaluated or linked Medicaid to readmission, which just missed statistical significance in this study (OR, 1.15; 95% CI, 0.971.36). Both black race and Medicaid as payer are proxies for the underlying root cause aspects leading to readmission, such as access to longitudinal care. Following this trail to the root cause will require in‐depth qualitative evaluation that includes the patient perspective as a source of data.32 For example, risk for readmission may not stem solely from being on warfarin, but in combination with not having transportation to get an international normalized ratio (INR) checked, a suboptimal understanding of how to take the medication, or not recognizing potential side effects until too late to avoid inpatient admission.
Several of the strongest associations, and perhaps most conducive to targeted interventions, were high‐risk medications at discharge. Risk related to medications and adverse drug events following discharge have been a consistent theme in readmission literature.24, 33 Our current system, which includes mandated inpatient medicine reconciliation, does not encourage discontinuation of unnecessary medications to combat polypharmacy, address affordability of medications, provide consistent medication counseling, or focus on the highest risk medications. In fact, bundled interventions which implement pharmacists to focus on these measures have been successful in decreasing readmission,14, 16, 29 but unfortunately are not yet part of the standard of care. The challenge remains transforming a mandatory policy such as medicine reconciliation into a valuable and systematic tool in the discharge process.
Two factors were surprisingly protective against readmission: neurologic diagnosis and anticholinergic medications. This first may be explained by the presence of a separate neurology service at our institution which skews our data. For example, a patient with acute stroke, who has a 20% rate of bounce‐back to a higher level of care within 30 days of discharge,34 would be admitted to the neurology service, not general medicine, and therefore would not nr part of our cohort. Regarding anticholinergics, several factors may explain this unexpected result. First, use of anticholinergics was relatively rare in our sample (2.7% in readmitted patients, 4.1% in patients not readmitted), possibly creating a false positive result. Second, Hanlon et al.24 showed only a weak association at best between anticholinergics and postdischarge adverse drug reactions (hazard ratio, 1.11; 95% CI, 0.86‐1.43). Lastly, anticholinergics include a varied group of medications, therefore diluting possible relative risk of specific medications.
While this study allows providers to identify patients at increased risk of readmission, the identified factors do not fully account for readmission risk; we did not aim to produce a risk‐prediction rule with our study. Prior readmission studies have been unable to create a tool to predict which patients will be readmitted with much success.3537 These results underscore the complexities and variability of readmission, which often lack a clear single cause and effect relationship. Given the breadth of risk factors we identified, it seems likely that more intensive interventions will require a multidisciplinary approach, one which might be costly if applied broadly. Our study does not attempt to predict who will be readmitted and who will not, but rather provides a list of risk factors which might be used to deploy resources more efficiently.
This study had several limitations. We did not capture readmissions to outside hospitals, which account for 22% to 24% of all readmissions in prior studies, and therefore have underestimated the readmission rate in our population.2, 8 However, by limiting our data to 2 hospitals within 1 institution, we were able to include more detailed patient level data, which is not accurately available in other large databases. Also, while studies of risk factors in a managed care population (such as within Medicare, the Veterans Affairs medical centers, or countries with national integrated medical records) are able to capture all readmissions, this study is the first to evaluate readmissions risk factors in a truly heterogeneous U.S. inpatient medicine population without limitation by age or payer status. Second, we did not have access to outpatient medications lists; however use of these same medications within the last 48 hours of admission is likely a reasonable proxy for outpatient use and more conducive to potential interventions (such as medication reconciliation or patient education) that could flag patients prior to discharge. Payer data was limited to only the primary payer, so patients who were dual eligible (ie, have both Medicare and Medicaid) were categorized as Medicare. Regarding sociodemographic factors, while primary language other than English was not associated with readmission, language data was missing in 17.4% of admissions, thereby limiting our ability to evaluate this factor. Our data did not include access to outpatient or primary care, and therefore we were unable to evaluate access to postdischarge follow‐up care as a risk factor for readmission. Lastly, while this study did not include outpatient deaths, we did exclude patients who died in the hospital.
Conclusions
Readmission is common among general medicine patients, with approximately 1 in 5 patients being readmitted within 30 days. While the identified associated factors do not account for all the potential reasons for readmission, our study suggests a spectrum of risk factors which might be used to target more intensive multidisciplinary interventions. Specifically, the nonclinical factors of race and payer status merit further in depth research incorporating the patient experience to truly determine causation of readmission. Hospitalists, who are at nexus of the discharge process and uniquely invested in quality inpatient care, are ideally positioned to lead efforts to reduce readmissions. How to use our study's results to develop and implement effective interventions to reduce readmissions remains a subject for future studies.
- A path to bundled payment around a rehospitalization.: Medicare payment Advisory Commission; June2005.
- Rehospitalizations among patients in the Medicare fee‐for‐service program.N Engl J Med.2009;360(14):1418–1428. , , .
- University HealthSystem Consortium. Available at: https://www.uhc.edu. Accessed May2010.
- U.S. Department of Health 15(5):599–606.
- Development and validation of a model for predicting emergency admissions over the next year (PEONY): a UK historical cohort study.Arch Intern Med.2008;168(13):1416–1422. , , , .
- Incidence and main factors associated with early unplanned hospital readmission among French medical inpatients aged 75 and over admitted through emergency units.Age Ageing.2008;37(4):416–422. , , , et al.
- Factors associated with unplanned hospital readmission among patients 65 years of age and older in a Medicare managed care plan.Am J Med.1999;107(1):13–17. , , , , , .
- Risk factors for early unplanned hospital readmission in the elderly.J Gen Intern Med.1991;6(3):223–228. , , .
- Predicting non‐elective hospital readmissions: a multi‐site study. Department of Veterans Affairs Cooperative Study Group on Primary Care and Readmissions.J Clin Epidemiol.2000;53(11):1113–1118. , , , et al.
- Effect of gender, ethnicity, pulmonary disease, and symptom stability on rehospitalization in patients with heart failure.Am J Cardiol.2007;100(7):1139–1144. , .
- The care transitions intervention: results of a randomized controlled trial.Arch Intern Med.2006;166(17):1822–1828. , , , .
- The impact of follow‐up telephone calls to patients after hospitalization.Am J Med.2001;111(9B):26S–30S. , , , .
- A reengineered hospital discharge program to decrease rehospitalization: a randomized trial.Ann Intern Med.2009;150(3):178–187. , , , et al.
- Reduction of 30‐day postdischarge hospital readmission or emergency department (ED) visit rates in high‐risk elderly medical patients through delivery of a targeted care bundle.J Hosp Med.2009;4(4):211–218. , , , et al.
- Comprehensive discharge planning with postdischarge support for older patients with congestive heart failure: a meta‐analysis.JAMA.2004;291(11):1358–1367. , , , , , .
- Home‐based intervention in congestive heart failure: long‐term implications on readmission and survival.Circulation.2002;105(24):2861–2866. , .
- Effect of a standardized nurse case‐management telephone intervention on resource use in patients with chronic heart failure.Arch Intern Med.2002;162(6):705–712. , , , , , .
- The impact of follow‐up physician visits on emergency readmissions for patients with asthma and chronic obstructive pulmonary disease: a population‐based study.Am J Med.2002;112(2):120–125. , , , .
- Factors predicting readmission of older general medicine patients.J Gen Intern Med.1991;6(5):389–393. , .
- BOOSTing Care Transitions. Available at: http://www.hospitalmedicine.org/ResourceRoomRedesign/RR_CareTransitions/CT_Home.cfm. Accessed May2010.
- Adverse drug events occurring following hospital discharge.J Gen Intern Med.2005;20(4):317–323. , , , , .
- Incidence and predictors of all and preventable adverse drug reactions in frail elderly persons after hospital stay.J Gerontol A Biol Sci Med Sci.2006;61(5):511–515. , , , et al.
- http://www.ahrq.gov/qual/mortality/Hughes.htm. Accessed May2010. . Development of the 3M™ All Patient Refined Diagnosis Related Groups (APR DRGs). Available at:
- Volume thresholds and hospital characteristics in the United States.Health Aff (Millwood).2003;22(2):167–177. , , .
- The status of hospital medicine groups in the United States.J Hosp Med.2006;1(2):75–80. , , , .
- Growth in the care of older patients by hospitalists in the United States.N Engl J Med.2009;360(11):1102–1112. , , , .
- Role of pharmacist counseling in preventing adverse drug events after hospitalization.Arch Intern Med.2006;166(5):565–571. , , , et al.
- Effects of a multicomponent intervention on functional outcomes and process of care in hospitalized older patients: a randomized controlled trial of Acute Care for Elders (ACE) in a community hospital.J Am Geriatr Soc.2000;48(12):1572–1581. , , , et al.
- Telehome monitoring in patients with cardiac disease who are at high risk of readmission.Heart Lung.2008;37(1):36–45. , , , , , .
- Understanding rehospitalization risk: can hospital discharge be modified to reduce recurrent hospitalization?J Hosp Med.2007;2(5):297–304. , , .
- The incidence and severity of adverse events affecting patients after discharge from the hospital.Ann Intern Med.2003;138(3):161–167. , , , , .
- Bouncing back: patterns and predictors of complicated transitions 30 days after hospitalization for acute ischemic stroke.J Am Geriatr Soc.2007;55(3):365–373. , , , .
- Case finding for patients at risk of readmission to hospital: development of algorithm to identify high risk patients.BMJ.2006;333(7563):327. , , , .
- Screening elders for risk of hospital admission.J Am Geriatr Soc.1993;41(8):811–817. , , , , , .
- Using routine inpatient data to identify patients at risk of hospital readmission.BMC Health Serv Res.2009;9:96. , , , .
Within Medicare recipients, an astounding one in five medical patients (19.6%) is readmitted within 30 days, accounting for $15 billion in spending.1, 2 Amidst the current healthcare system crisis, reducing these hospital readmissions has risen to the highest priority. Reducing readmissions is the newest addition to multiple quality dashboards, both institutional and national, as a measure of the care delivered during hospitalization.3 One of the most notable of these reporting entities, Hospital Compare, now publicly reports Medicare readmission rates for a few common diagnoses.4 While Medicare already withholds payment to hospitals for readmissions within 24 hours for the same diagnosis, Medicare may soon reduce payment to hospitals with the highest rates of readmission within 30 days, a powerful incentive for hospitals to intervene. Readmissions have also reached the radar of additional stakeholders, even making its way onto Obama's budget considerations, given the potential cost savings to the system overall.5
To develop systems which reduce readmissions, one must first gain understanding of the characteristics of readmissions. A few clinical risk factors (such as age, number of prior admissions, and comorbidities) have been well defined in subgroups of general medicine inpatients.612 Likewise, interventions aiming to reduce readmissions have also focused on subgroups, excluding a large portion of hospitalized patients (for example, non‐English speakers and younger patients).1320 Other data have been derived in veterans or within non‐US populations that have inherently different payer, race, ethnicity, and primary language composition, and may not be applicable outside those settings.7, 8, 10, 11, 21 Lastly, little is known regarding risk that may be associated with operational factors, such as weekend discharge or admission source. As a result, there are few data describing the clinical, operational, and demographic factors associated with readmission in a heterogeneous population of hospitalized general medicine patientsthe patient population of most generalists in the United States.
To understand the impact of a variety of risk factors in a diverse general medicine population, we evaluated the characteristics of readmitted patients in a large urban university medical center over a 2‐year period. We hypothesized that a number of clinical, operational, and sociodemographic factors would be associated with readmission.
Methods
Sites and Subjects
Our data were collected on general medicine patients during hospitalization between June 1, 2006 and May 31, 2008, at the University of California San Francisco. The University of California, San Francisco (UCSF) Medical Center is composed of Moffitt‐Long Hospital (a 400‐bed center) and UCSF‐Mount Zion Hospital (a 200‐bed facility) located in San Francisco, CA.
Medical patients at Moffitt‐Long Hospital are admitted to 1 of 8 medical teams composed of a resident, 1 to 2 interns, and 0 to 3 medical students, supervised by an attending physician who is most often a hospitalist. At Moffitt‐Long Hospital, housestaff write all orders and provide 24‐hour coverage to inpatients. Mount Zion medical patients are cared for by 1 of 2 teams and staffed by a hospitalist on each team who is responsible for all elements of care. Both services care for common inpatient diagnoses, as well as specialty‐associated diagnoses such as cancer, pneumonia, and chronic obstructive pulmonary disease (COPD). Of note, at Moffitt‐Long Hospital, those patients with primary cardiac diagnoses are cared for by a separate team composed of housestaff and students supervised by a cardiologist.
The discharge process at both sites utilizes a multidisciplinary teamincluding physicians, case managers, nurses, pharmacists, and discharge coordinatorsworking in concert. Key components include arranging follow‐up care, faxing the discharge summary to the primary care provider, and educating the patient and caregivers, especially regarding medications. While these goals are clearly delineated, significant variability exists in how these tasks are actually accomplished. The multidisciplinary approach, components of the discharge process, and lack of a systematic approach are representative of the discharge process around the country.22
Data
Data regarding patient demographics, age, comorbidities, and insurance status were collected from administrative data systems at UCSF, reflecting the patient's status at the time of index admission. These same systems were used to collect a date‐stamped log of all medications (eg, anticoagulants) for which the patient was billed during the last 48 hours of hospitalization. Specifically, data were obtained for medications previously shown to cause adverse drug events following hospital discharge.23, 24 These medication groups include corticosteroids, anticoagulants, antibiotics, narcotics, nonsteroidal anti‐inflammatory drugs (NSAIDs), cardiovascular medications, antiepileptics, anticholinergics, antidepressants, and antidiabetics. Operational factors that we hypothesized would affect readmission risk included admission source, discharge disposition, and weekday vs. weekend discharge. Case management, social work, and pharmacy services operate with limited staffing on weekends. Likewise, resident and intern physicians are more likely to be off on a weekend day than a weekday; covering attending physicians care for about half of patients during the weekend. Data were obtained from Transition Systems International (TSI, Boston, MA) administrative databases, a cost‐accounting system that collects data abstracted from patient charts upon discharge from UCSF.
Definition of Readmission Measure
Using TSI, we detected readmission to UCSF by screening for any inpatient encounters on any service (not just medicine) within the 30 days following discharge from the general medicine service at the 2 UCSF campuses. We excluded elective readmissions, such as for scheduled chemotherapy. Patients who died at the index admission were excluded from the cohort.
Adjustment Variables
Age, gender, payer status and APR risk of mortality (3M Health Information Systems, St. Paul, MN) were collected from administrative data. The All Patient Refined (APR) risk of mortality is the all patients risk of mortality score developed by 3M which divides patients into 4 subclasses of risk based on clinical problems and comorbidities.25 We used secondary diagnosis codes in administrative data to classify comorbidities using the method of Elixhauser.26
Using the log of medication charges, we grouped high‐risk medications according to the classification scheme of Forster et al.23 and Hanlon et al.24 We then created a count representing the total number of medications administered to patients within the final 48 hours of stay.
Analysis
We first described study patients and hospitals using univariable methods. Multivariable generalized estimating equations (SAS PROC GENMOD) were used to account for clustering of patients within physicians and calculate adjusted odds ratios (ORs). As there were 2 sites within UCSF Medical Center (Moffitt‐Long and Mount Zion hospitals), we included site as a fixed effect in our model. Models were constructed using manual variable selection methods with final selection being made based on whether the covariate was associated with readmission at P < 0.05. All analyses were carried out using SAS version 9.2 (SAS Institute, Inc. Cary, NC).
Results
Baseline Characteristics
During the 2‐year accrual period, 295 attending physicians admitted 6805 unique patients for a total of 10,359 admissions. Seventeen percent of these 10,359 admissions were readmitted within 30 days. The cohort of all patients had a mean age of 59.6 years 19.5 standard deviation (SD), with 52.8% women. The mean length of stay was 5.6 days 10.4 SD. Medicare was the payer source for approximately half of the admissions. The majority of admissions (90.4%) were billed for at least 1 high risk medication, with narcotics, cardiac medications, and antibiotics being the most common. Regarding disposition, 79.5% of admissions were discharged to home; 9.1% were discharged to a skilled nursing facility (SNF).
Baseline sociodemographic, operational, and clinical characteristics for patients readmitted and not readmitted are shown in Table 1. Demographic characteristics with significant differences (P < 0.05) between readmitted and nonreadmitted groups included mean age, race, payer status, and primary language other than English. Regarding operational characteristics, readmitted patients had a higher median length of stay and were more likely to be admitted through the emergency room during their index admission. Discharge to an SNF was higher in the readmitted group versus the nonreadmitted group (9.7% vs. 9.0%). Several clinical factors were more prevalent in the readmitted group: high‐risk medications, specifically steroids, narcotics, and cardiovascular medications; high‐risk medication count of 3 or greater; and comorbidities including congestive heart failure, renal disease, cancer, anemia, and depression.
Characteristic | Patients Readmitted (n = 1762 17.0%), n (%) | Patients Not Readmitted (n = 8597 83.0%), n (%) | P Value |
---|---|---|---|
| |||
Mean age (years) (SD) | 58.8 (19.3) | 59.8 (19.6) | 0.0491 |
Female | 930 (52.8) | 4548 (52.9) | 0.9260 |
Race* | |||
White | 785 (44.6) | 4166 (48.8) | <0.0001 |
Black | 442 (25.1) | 1401 (16.4) | |
Asian | 323 (18.4) | 1726 (20.2) | |
Other | 209 (11.9) | 1240 (14.5) | |
Hispanic ethnicity | 140 (8.1) | 734 (8.9) | 0.2737 |
Payer status | |||
Medicare | 905 (51.4) | 4266 (49.6) | <0.0001 |
Medicaid/Medi‐cal | 458 (26.0) | 1578 (18.4) | |
Private | 370 (21.0) | 2092 (24.3) | |
Other | 29 (1.7) | 661 (7.7) | |
Primary language other than English | 242 (17.1) | 1394 (19.5) | 0.0359 |
Median length of stay (days) (interquartile range) | 4 (2, 7) | 3 (2, 6) | <0.0001 |
Admit source | |||
Emergency room | 1506 (85.5) | 6898 (80.2) | <0.0001 |
Outside hospital | 38 (2.2) | 271 (3.2) | |
Direct admission/other (jail) | 218 (12.4) | 1428 (16.6) | |
Discharge to | |||
Home | 1461 (82.9) | 6773 (78.8) | <0.0001 |
SNF | 170 (9.7) | 774 (9.0) | |
Other | 131 (7.4) | 1050 (12.2) | |
Discharged on weekend | 381 (21.6) | 1904 (22.1) | 0.6288 |
Patient medications | |||
Any high‐risk medication | 1679 (95.3) | 7684 (89.4) | <0.0001 |
High‐risk medication count | |||
02 | 577 (32.8) | 3666 (42.6) | <0.0001 |
34 | 692 (39.3) | 2968 (34.5) | |
5 | 493 (28) | 1963 (22.8) | |
Any corticosteroids | 399 (22.6) | 1571 (18.3) | <0.0001 |
Anticoagulant | 120 (6.8) | 559 (6.5) | 0.6340 |
Any antibiotic | 904 (51.3) | 4203 (48.9) | 0.0646 |
Any narcotic | 1036 (58.8) | 4206 (48.9) | <0.0001 |
Any NSAID | 68 (3.9) | 320 (3.7) | 0.7826 |
Any cardiovascular med | 887 (50.3) | 3806 (44.3) | <0.0001 |
Any antiepileptic | 93 (5.3) | 470 (5.5) | 0.7500 |
Any anticholinergic | 47 (2.7) | 354 (4.1) | 0.0040 |
Any antidepressant | 455 (25.8) | 1863 (25.8) | 0.0001 |
Any antidiabetic | 198 (11.2) | 994 (11.6) | 0.6970 |
Elixhauser comorbidities | |||
Congestive heart failure | 219 (12.4) | 795 (9.3) | <0.0001 |
Pulmonary circulation disease | 72 (4.1) | 178 (2.1) | <0.0001 |
Peripheral vascular disease | 84 (4.8) | 331 (3.9) | 0.0737 |
Hypertension | 745 (42.3) | 3741 (43.5) | 0.3411 |
Other neurological disease | 101 (5.7) | 696 (8.1) | 0.0007 |
Chronic pulmonary disease | 317 (18.0) | 1442 (16.8) | 0.2149 |
Diabetes | 303 (17.2) | 1333 (15.5) | 0.0762 |
Renal failure | 339 (19.2) | 1286 (15.0) | <0.0001 |
Liver disease | 188 (10.7) | 774 (9.0) | 0.0281 |
Metastatic cancer | 160 (9.1) | 530 (6.2) | <0.0001 |
Solid tumor w/o metastases | 100 (5.7) | 277 (3.2) | <0.0001 |
Rheumatoid arthritis/collagen vas | 81 (4.6) | 303 (3.5) | 0.0299 |
Weight loss | 153 (8.7) | 584 (6.8) | 0.0449 |
Deficiency anemia | 522 (29.6) | 1979 (23.0) | <0.0001 |
Alcohol abuse | 101 (5.7) | 428 (5.0) | 0.1905 |
Drug abuse | 148 (8.4) | 619 (7.2) | 0.0798 |
Depression | 244 (13.9) | 963 (11.2) | 0.0016 |
APR risk of mortality | |||
1 | 451 (25.6) | 3101 (36.1) | <0.0001 |
2 | 619 (35.1) | 2797 (32.5) | |
3 | 536 (30.4) | 1907 (22.2) | |
4 | 156 (8.9) | 792 (9.2) |
Frequency of Readmission
The 30‐day readmission rate was 17.0% (1762 patients), with 49.7% (875 patients) of the readmissions occurring within 10 days of discharge. Of patients readmitted, the general medicine service was the readmitting team in 78.2%. A quarter of readmissions (26.2%) had the same primary diagnosis on initial and repeat admission.
Factors Associated With Readmission
Factors associated with readmission were categorized as sociodemographic, operational, and clinical. Factors associated with readmission with P < 0.05 and present in at least 5% of admissions are presented in Table 2. Of the sociodemographic factors, black race was significantly associated with readmission. Within the Medicare cohort, risk for readmission was similar for white vs. nonwhite race, with relative risk of 1.0 (95% confidence interval [CI], 0.86‐1.18). Medicaid as payer status was significantly associated in the unadjusted model, and in the adjusted model showed a trend toward readmission. Mean age was significantly different in the readmitted and nonreadmitted groups, but the difference was small (1.0 year). Moreover, when we evaluated age in 5‐year categories (ex. 65‐70, 71‐75, etc.), age was not associated with readmission. In the adjusted model, none of the operational factors were significantly associated with readmission, including discharge to SNF, weekend discharge, or admit source.
Covariate | Unadjusted OR (95% CI) | Adjusted OR (95% CI) |
---|---|---|
| ||
Age | 1.00 (0.991.00) | 1.00 (0.991.00) |
Race | ||
White | Referent | Referent |
Black | 1.67 (1.471.91) | 1.43 (1.241.65) |
Asian | 0.99 (0.861.14) | 0.95 (0.821.11) |
Other | 0.89 (0.761.06) | 0.84 (0.671.06) |
Payer | ||
Medicare | Referent | Referent |
Medicaid/medical | 1.37 (1.211.55) | 1.15 (0.971.36) |
Private | 0.83 (0.730.95) | 0.78 (0.650.95) |
Other | 0.21 (0.140.30) | 0.23 (0.110.45) |
Disposition | ||
To home | Referent | Referent |
SNF | 1.02 (0.851.21) | 0.98 (0.821.18) |
Other | 0.58 (0.480.70) | 0.53 (0.430.66) |
Highrisk medications | ||
Corticosteroids | 1.31 (1.161.48) | 1.24 (1.091.42) |
Narcotics | 1.49 (1.341.65) | 1.33 (1.161.53) |
Anticholinergics | 0.64 (0.470.87) | 0.66 (0.480.90) |
Comorbidities | ||
Congestive heart failure | 1.39 (1.191.63) | 1.30 (1.091.56) |
Neurological disorders | 0.69 (0.560.86) | 0.70 (0.570.87) |
Renal failure | 1.35 (1.191.55) | 1.19 (1.051.36) |
Metastatic cancer | 1.52 (1.261.83) | 1.61 (1.331.95) |
Solid tumor w/o metastasis | 1.81 (1.432.29) | 1.95 (1.542.47) |
Deficiency anemia | 1.41 (1.261.58) | 1.27 (1.131.44) |
Weight loss | 1.30 (1.081.57) | 1.26 (1.091.47) |
Of the clinical factors, high‐risk medications and 6 comorbidities were associated with readmission. High‐risk medication categories associated with readmission were steroids and narcotics; anticholinergics medications were protective. The 6 comorbidities associated with readmission were congestive heart failure, renal disease, cancer (with and without metastasis), weight loss, and iron deficiency anemia. While APR risk of mortality was associated with readmission at P < 0.05, including APR in our final model did not alter which other factors were significantly associated with readmission. When site (Moffitt‐Long vs. Mount Zion Hospitals) was added to the model, the ORs for factors associated with readmission did not change appreciably (0.01).
Discussion
In this retrospective observational study of hospitalized patients, we found that readmission was common and associated with a number of risk factors that could be easily identified early in hospitalization. Nonclinical factors associated with readmission were black race and Medicaid payer status (in the unadjusted model). Clinical factors were high risk medications including steroids and narcotics; and comorbidities including congestive heart failure, renal disease, cancer, anemia, and weight loss. In contrast, other potential riskssuch as discharge on a weekend and discharge to an SNFwere not independently associated with readmission. This cohortwith a mix of clinical scenarios, payers, age, etc.represents the inherently heterogeneous population of inpatient general medicine across the country and abroad. Hospitalists provided care for over 65% of the general medicine service, again representative of the trend in US inpatient medicine.27, 28 Lastly, our cohort did not have the benefit of a systematic and consistent discharge process with interventions focused on reducing readmissions. This gap, which is common across hospitals, highlights the utility of this data in targeting quality improvement efforts.
Reducing risks for readmission requires identification of patient populations at highest risk; in those patients, one can further identify factors which are potentially modifiable via education or patient‐engagement interventions. While in the hospital, more intensive predischarge counseling and efforts to increase mobility may be most useful if targeted early and often on those at highest risk.15, 16, 29, 30 Finally, broader‐based support in the form of better home services, more access to longitudinal care, or targeted postdischarge efforts may be required.14, 31
Though current strategies focus largely on clinical risk factors, this study shows that nonclinical factors play an equally important but underappreciated role in contributing to readmission. While prior studies have shown variable results on association of black race with readmission,2, 9, 11 none have evaluated or linked Medicaid to readmission, which just missed statistical significance in this study (OR, 1.15; 95% CI, 0.971.36). Both black race and Medicaid as payer are proxies for the underlying root cause aspects leading to readmission, such as access to longitudinal care. Following this trail to the root cause will require in‐depth qualitative evaluation that includes the patient perspective as a source of data.32 For example, risk for readmission may not stem solely from being on warfarin, but in combination with not having transportation to get an international normalized ratio (INR) checked, a suboptimal understanding of how to take the medication, or not recognizing potential side effects until too late to avoid inpatient admission.
Several of the strongest associations, and perhaps most conducive to targeted interventions, were high‐risk medications at discharge. Risk related to medications and adverse drug events following discharge have been a consistent theme in readmission literature.24, 33 Our current system, which includes mandated inpatient medicine reconciliation, does not encourage discontinuation of unnecessary medications to combat polypharmacy, address affordability of medications, provide consistent medication counseling, or focus on the highest risk medications. In fact, bundled interventions which implement pharmacists to focus on these measures have been successful in decreasing readmission,14, 16, 29 but unfortunately are not yet part of the standard of care. The challenge remains transforming a mandatory policy such as medicine reconciliation into a valuable and systematic tool in the discharge process.
Two factors were surprisingly protective against readmission: neurologic diagnosis and anticholinergic medications. This first may be explained by the presence of a separate neurology service at our institution which skews our data. For example, a patient with acute stroke, who has a 20% rate of bounce‐back to a higher level of care within 30 days of discharge,34 would be admitted to the neurology service, not general medicine, and therefore would not nr part of our cohort. Regarding anticholinergics, several factors may explain this unexpected result. First, use of anticholinergics was relatively rare in our sample (2.7% in readmitted patients, 4.1% in patients not readmitted), possibly creating a false positive result. Second, Hanlon et al.24 showed only a weak association at best between anticholinergics and postdischarge adverse drug reactions (hazard ratio, 1.11; 95% CI, 0.86‐1.43). Lastly, anticholinergics include a varied group of medications, therefore diluting possible relative risk of specific medications.
While this study allows providers to identify patients at increased risk of readmission, the identified factors do not fully account for readmission risk; we did not aim to produce a risk‐prediction rule with our study. Prior readmission studies have been unable to create a tool to predict which patients will be readmitted with much success.3537 These results underscore the complexities and variability of readmission, which often lack a clear single cause and effect relationship. Given the breadth of risk factors we identified, it seems likely that more intensive interventions will require a multidisciplinary approach, one which might be costly if applied broadly. Our study does not attempt to predict who will be readmitted and who will not, but rather provides a list of risk factors which might be used to deploy resources more efficiently.
This study had several limitations. We did not capture readmissions to outside hospitals, which account for 22% to 24% of all readmissions in prior studies, and therefore have underestimated the readmission rate in our population.2, 8 However, by limiting our data to 2 hospitals within 1 institution, we were able to include more detailed patient level data, which is not accurately available in other large databases. Also, while studies of risk factors in a managed care population (such as within Medicare, the Veterans Affairs medical centers, or countries with national integrated medical records) are able to capture all readmissions, this study is the first to evaluate readmissions risk factors in a truly heterogeneous U.S. inpatient medicine population without limitation by age or payer status. Second, we did not have access to outpatient medications lists; however use of these same medications within the last 48 hours of admission is likely a reasonable proxy for outpatient use and more conducive to potential interventions (such as medication reconciliation or patient education) that could flag patients prior to discharge. Payer data was limited to only the primary payer, so patients who were dual eligible (ie, have both Medicare and Medicaid) were categorized as Medicare. Regarding sociodemographic factors, while primary language other than English was not associated with readmission, language data was missing in 17.4% of admissions, thereby limiting our ability to evaluate this factor. Our data did not include access to outpatient or primary care, and therefore we were unable to evaluate access to postdischarge follow‐up care as a risk factor for readmission. Lastly, while this study did not include outpatient deaths, we did exclude patients who died in the hospital.
Conclusions
Readmission is common among general medicine patients, with approximately 1 in 5 patients being readmitted within 30 days. While the identified associated factors do not account for all the potential reasons for readmission, our study suggests a spectrum of risk factors which might be used to target more intensive multidisciplinary interventions. Specifically, the nonclinical factors of race and payer status merit further in depth research incorporating the patient experience to truly determine causation of readmission. Hospitalists, who are at nexus of the discharge process and uniquely invested in quality inpatient care, are ideally positioned to lead efforts to reduce readmissions. How to use our study's results to develop and implement effective interventions to reduce readmissions remains a subject for future studies.
Within Medicare recipients, an astounding one in five medical patients (19.6%) is readmitted within 30 days, accounting for $15 billion in spending.1, 2 Amidst the current healthcare system crisis, reducing these hospital readmissions has risen to the highest priority. Reducing readmissions is the newest addition to multiple quality dashboards, both institutional and national, as a measure of the care delivered during hospitalization.3 One of the most notable of these reporting entities, Hospital Compare, now publicly reports Medicare readmission rates for a few common diagnoses.4 While Medicare already withholds payment to hospitals for readmissions within 24 hours for the same diagnosis, Medicare may soon reduce payment to hospitals with the highest rates of readmission within 30 days, a powerful incentive for hospitals to intervene. Readmissions have also reached the radar of additional stakeholders, even making its way onto Obama's budget considerations, given the potential cost savings to the system overall.5
To develop systems which reduce readmissions, one must first gain understanding of the characteristics of readmissions. A few clinical risk factors (such as age, number of prior admissions, and comorbidities) have been well defined in subgroups of general medicine inpatients.612 Likewise, interventions aiming to reduce readmissions have also focused on subgroups, excluding a large portion of hospitalized patients (for example, non‐English speakers and younger patients).1320 Other data have been derived in veterans or within non‐US populations that have inherently different payer, race, ethnicity, and primary language composition, and may not be applicable outside those settings.7, 8, 10, 11, 21 Lastly, little is known regarding risk that may be associated with operational factors, such as weekend discharge or admission source. As a result, there are few data describing the clinical, operational, and demographic factors associated with readmission in a heterogeneous population of hospitalized general medicine patientsthe patient population of most generalists in the United States.
To understand the impact of a variety of risk factors in a diverse general medicine population, we evaluated the characteristics of readmitted patients in a large urban university medical center over a 2‐year period. We hypothesized that a number of clinical, operational, and sociodemographic factors would be associated with readmission.
Methods
Sites and Subjects
Our data were collected on general medicine patients during hospitalization between June 1, 2006 and May 31, 2008, at the University of California San Francisco. The University of California, San Francisco (UCSF) Medical Center is composed of Moffitt‐Long Hospital (a 400‐bed center) and UCSF‐Mount Zion Hospital (a 200‐bed facility) located in San Francisco, CA.
Medical patients at Moffitt‐Long Hospital are admitted to 1 of 8 medical teams composed of a resident, 1 to 2 interns, and 0 to 3 medical students, supervised by an attending physician who is most often a hospitalist. At Moffitt‐Long Hospital, housestaff write all orders and provide 24‐hour coverage to inpatients. Mount Zion medical patients are cared for by 1 of 2 teams and staffed by a hospitalist on each team who is responsible for all elements of care. Both services care for common inpatient diagnoses, as well as specialty‐associated diagnoses such as cancer, pneumonia, and chronic obstructive pulmonary disease (COPD). Of note, at Moffitt‐Long Hospital, those patients with primary cardiac diagnoses are cared for by a separate team composed of housestaff and students supervised by a cardiologist.
The discharge process at both sites utilizes a multidisciplinary teamincluding physicians, case managers, nurses, pharmacists, and discharge coordinatorsworking in concert. Key components include arranging follow‐up care, faxing the discharge summary to the primary care provider, and educating the patient and caregivers, especially regarding medications. While these goals are clearly delineated, significant variability exists in how these tasks are actually accomplished. The multidisciplinary approach, components of the discharge process, and lack of a systematic approach are representative of the discharge process around the country.22
Data
Data regarding patient demographics, age, comorbidities, and insurance status were collected from administrative data systems at UCSF, reflecting the patient's status at the time of index admission. These same systems were used to collect a date‐stamped log of all medications (eg, anticoagulants) for which the patient was billed during the last 48 hours of hospitalization. Specifically, data were obtained for medications previously shown to cause adverse drug events following hospital discharge.23, 24 These medication groups include corticosteroids, anticoagulants, antibiotics, narcotics, nonsteroidal anti‐inflammatory drugs (NSAIDs), cardiovascular medications, antiepileptics, anticholinergics, antidepressants, and antidiabetics. Operational factors that we hypothesized would affect readmission risk included admission source, discharge disposition, and weekday vs. weekend discharge. Case management, social work, and pharmacy services operate with limited staffing on weekends. Likewise, resident and intern physicians are more likely to be off on a weekend day than a weekday; covering attending physicians care for about half of patients during the weekend. Data were obtained from Transition Systems International (TSI, Boston, MA) administrative databases, a cost‐accounting system that collects data abstracted from patient charts upon discharge from UCSF.
Definition of Readmission Measure
Using TSI, we detected readmission to UCSF by screening for any inpatient encounters on any service (not just medicine) within the 30 days following discharge from the general medicine service at the 2 UCSF campuses. We excluded elective readmissions, such as for scheduled chemotherapy. Patients who died at the index admission were excluded from the cohort.
Adjustment Variables
Age, gender, payer status and APR risk of mortality (3M Health Information Systems, St. Paul, MN) were collected from administrative data. The All Patient Refined (APR) risk of mortality is the all patients risk of mortality score developed by 3M which divides patients into 4 subclasses of risk based on clinical problems and comorbidities.25 We used secondary diagnosis codes in administrative data to classify comorbidities using the method of Elixhauser.26
Using the log of medication charges, we grouped high‐risk medications according to the classification scheme of Forster et al.23 and Hanlon et al.24 We then created a count representing the total number of medications administered to patients within the final 48 hours of stay.
Analysis
We first described study patients and hospitals using univariable methods. Multivariable generalized estimating equations (SAS PROC GENMOD) were used to account for clustering of patients within physicians and calculate adjusted odds ratios (ORs). As there were 2 sites within UCSF Medical Center (Moffitt‐Long and Mount Zion hospitals), we included site as a fixed effect in our model. Models were constructed using manual variable selection methods with final selection being made based on whether the covariate was associated with readmission at P < 0.05. All analyses were carried out using SAS version 9.2 (SAS Institute, Inc. Cary, NC).
Results
Baseline Characteristics
During the 2‐year accrual period, 295 attending physicians admitted 6805 unique patients for a total of 10,359 admissions. Seventeen percent of these 10,359 admissions were readmitted within 30 days. The cohort of all patients had a mean age of 59.6 years 19.5 standard deviation (SD), with 52.8% women. The mean length of stay was 5.6 days 10.4 SD. Medicare was the payer source for approximately half of the admissions. The majority of admissions (90.4%) were billed for at least 1 high risk medication, with narcotics, cardiac medications, and antibiotics being the most common. Regarding disposition, 79.5% of admissions were discharged to home; 9.1% were discharged to a skilled nursing facility (SNF).
Baseline sociodemographic, operational, and clinical characteristics for patients readmitted and not readmitted are shown in Table 1. Demographic characteristics with significant differences (P < 0.05) between readmitted and nonreadmitted groups included mean age, race, payer status, and primary language other than English. Regarding operational characteristics, readmitted patients had a higher median length of stay and were more likely to be admitted through the emergency room during their index admission. Discharge to an SNF was higher in the readmitted group versus the nonreadmitted group (9.7% vs. 9.0%). Several clinical factors were more prevalent in the readmitted group: high‐risk medications, specifically steroids, narcotics, and cardiovascular medications; high‐risk medication count of 3 or greater; and comorbidities including congestive heart failure, renal disease, cancer, anemia, and depression.
Characteristic | Patients Readmitted (n = 1762 17.0%), n (%) | Patients Not Readmitted (n = 8597 83.0%), n (%) | P Value |
---|---|---|---|
| |||
Mean age (years) (SD) | 58.8 (19.3) | 59.8 (19.6) | 0.0491 |
Female | 930 (52.8) | 4548 (52.9) | 0.9260 |
Race* | |||
White | 785 (44.6) | 4166 (48.8) | <0.0001 |
Black | 442 (25.1) | 1401 (16.4) | |
Asian | 323 (18.4) | 1726 (20.2) | |
Other | 209 (11.9) | 1240 (14.5) | |
Hispanic ethnicity | 140 (8.1) | 734 (8.9) | 0.2737 |
Payer status | |||
Medicare | 905 (51.4) | 4266 (49.6) | <0.0001 |
Medicaid/Medi‐cal | 458 (26.0) | 1578 (18.4) | |
Private | 370 (21.0) | 2092 (24.3) | |
Other | 29 (1.7) | 661 (7.7) | |
Primary language other than English | 242 (17.1) | 1394 (19.5) | 0.0359 |
Median length of stay (days) (interquartile range) | 4 (2, 7) | 3 (2, 6) | <0.0001 |
Admit source | |||
Emergency room | 1506 (85.5) | 6898 (80.2) | <0.0001 |
Outside hospital | 38 (2.2) | 271 (3.2) | |
Direct admission/other (jail) | 218 (12.4) | 1428 (16.6) | |
Discharge to | |||
Home | 1461 (82.9) | 6773 (78.8) | <0.0001 |
SNF | 170 (9.7) | 774 (9.0) | |
Other | 131 (7.4) | 1050 (12.2) | |
Discharged on weekend | 381 (21.6) | 1904 (22.1) | 0.6288 |
Patient medications | |||
Any high‐risk medication | 1679 (95.3) | 7684 (89.4) | <0.0001 |
High‐risk medication count | |||
02 | 577 (32.8) | 3666 (42.6) | <0.0001 |
34 | 692 (39.3) | 2968 (34.5) | |
5 | 493 (28) | 1963 (22.8) | |
Any corticosteroids | 399 (22.6) | 1571 (18.3) | <0.0001 |
Anticoagulant | 120 (6.8) | 559 (6.5) | 0.6340 |
Any antibiotic | 904 (51.3) | 4203 (48.9) | 0.0646 |
Any narcotic | 1036 (58.8) | 4206 (48.9) | <0.0001 |
Any NSAID | 68 (3.9) | 320 (3.7) | 0.7826 |
Any cardiovascular med | 887 (50.3) | 3806 (44.3) | <0.0001 |
Any antiepileptic | 93 (5.3) | 470 (5.5) | 0.7500 |
Any anticholinergic | 47 (2.7) | 354 (4.1) | 0.0040 |
Any antidepressant | 455 (25.8) | 1863 (25.8) | 0.0001 |
Any antidiabetic | 198 (11.2) | 994 (11.6) | 0.6970 |
Elixhauser comorbidities | |||
Congestive heart failure | 219 (12.4) | 795 (9.3) | <0.0001 |
Pulmonary circulation disease | 72 (4.1) | 178 (2.1) | <0.0001 |
Peripheral vascular disease | 84 (4.8) | 331 (3.9) | 0.0737 |
Hypertension | 745 (42.3) | 3741 (43.5) | 0.3411 |
Other neurological disease | 101 (5.7) | 696 (8.1) | 0.0007 |
Chronic pulmonary disease | 317 (18.0) | 1442 (16.8) | 0.2149 |
Diabetes | 303 (17.2) | 1333 (15.5) | 0.0762 |
Renal failure | 339 (19.2) | 1286 (15.0) | <0.0001 |
Liver disease | 188 (10.7) | 774 (9.0) | 0.0281 |
Metastatic cancer | 160 (9.1) | 530 (6.2) | <0.0001 |
Solid tumor w/o metastases | 100 (5.7) | 277 (3.2) | <0.0001 |
Rheumatoid arthritis/collagen vas | 81 (4.6) | 303 (3.5) | 0.0299 |
Weight loss | 153 (8.7) | 584 (6.8) | 0.0449 |
Deficiency anemia | 522 (29.6) | 1979 (23.0) | <0.0001 |
Alcohol abuse | 101 (5.7) | 428 (5.0) | 0.1905 |
Drug abuse | 148 (8.4) | 619 (7.2) | 0.0798 |
Depression | 244 (13.9) | 963 (11.2) | 0.0016 |
APR risk of mortality | |||
1 | 451 (25.6) | 3101 (36.1) | <0.0001 |
2 | 619 (35.1) | 2797 (32.5) | |
3 | 536 (30.4) | 1907 (22.2) | |
4 | 156 (8.9) | 792 (9.2) |
Frequency of Readmission
The 30‐day readmission rate was 17.0% (1762 patients), with 49.7% (875 patients) of the readmissions occurring within 10 days of discharge. Of patients readmitted, the general medicine service was the readmitting team in 78.2%. A quarter of readmissions (26.2%) had the same primary diagnosis on initial and repeat admission.
Factors Associated With Readmission
Factors associated with readmission were categorized as sociodemographic, operational, and clinical. Factors associated with readmission with P < 0.05 and present in at least 5% of admissions are presented in Table 2. Of the sociodemographic factors, black race was significantly associated with readmission. Within the Medicare cohort, risk for readmission was similar for white vs. nonwhite race, with relative risk of 1.0 (95% confidence interval [CI], 0.86‐1.18). Medicaid as payer status was significantly associated in the unadjusted model, and in the adjusted model showed a trend toward readmission. Mean age was significantly different in the readmitted and nonreadmitted groups, but the difference was small (1.0 year). Moreover, when we evaluated age in 5‐year categories (ex. 65‐70, 71‐75, etc.), age was not associated with readmission. In the adjusted model, none of the operational factors were significantly associated with readmission, including discharge to SNF, weekend discharge, or admit source.
Covariate | Unadjusted OR (95% CI) | Adjusted OR (95% CI) |
---|---|---|
| ||
Age | 1.00 (0.991.00) | 1.00 (0.991.00) |
Race | ||
White | Referent | Referent |
Black | 1.67 (1.471.91) | 1.43 (1.241.65) |
Asian | 0.99 (0.861.14) | 0.95 (0.821.11) |
Other | 0.89 (0.761.06) | 0.84 (0.671.06) |
Payer | ||
Medicare | Referent | Referent |
Medicaid/medical | 1.37 (1.211.55) | 1.15 (0.971.36) |
Private | 0.83 (0.730.95) | 0.78 (0.650.95) |
Other | 0.21 (0.140.30) | 0.23 (0.110.45) |
Disposition | ||
To home | Referent | Referent |
SNF | 1.02 (0.851.21) | 0.98 (0.821.18) |
Other | 0.58 (0.480.70) | 0.53 (0.430.66) |
Highrisk medications | ||
Corticosteroids | 1.31 (1.161.48) | 1.24 (1.091.42) |
Narcotics | 1.49 (1.341.65) | 1.33 (1.161.53) |
Anticholinergics | 0.64 (0.470.87) | 0.66 (0.480.90) |
Comorbidities | ||
Congestive heart failure | 1.39 (1.191.63) | 1.30 (1.091.56) |
Neurological disorders | 0.69 (0.560.86) | 0.70 (0.570.87) |
Renal failure | 1.35 (1.191.55) | 1.19 (1.051.36) |
Metastatic cancer | 1.52 (1.261.83) | 1.61 (1.331.95) |
Solid tumor w/o metastasis | 1.81 (1.432.29) | 1.95 (1.542.47) |
Deficiency anemia | 1.41 (1.261.58) | 1.27 (1.131.44) |
Weight loss | 1.30 (1.081.57) | 1.26 (1.091.47) |
Of the clinical factors, high‐risk medications and 6 comorbidities were associated with readmission. High‐risk medication categories associated with readmission were steroids and narcotics; anticholinergics medications were protective. The 6 comorbidities associated with readmission were congestive heart failure, renal disease, cancer (with and without metastasis), weight loss, and iron deficiency anemia. While APR risk of mortality was associated with readmission at P < 0.05, including APR in our final model did not alter which other factors were significantly associated with readmission. When site (Moffitt‐Long vs. Mount Zion Hospitals) was added to the model, the ORs for factors associated with readmission did not change appreciably (0.01).
Discussion
In this retrospective observational study of hospitalized patients, we found that readmission was common and associated with a number of risk factors that could be easily identified early in hospitalization. Nonclinical factors associated with readmission were black race and Medicaid payer status (in the unadjusted model). Clinical factors were high risk medications including steroids and narcotics; and comorbidities including congestive heart failure, renal disease, cancer, anemia, and weight loss. In contrast, other potential riskssuch as discharge on a weekend and discharge to an SNFwere not independently associated with readmission. This cohortwith a mix of clinical scenarios, payers, age, etc.represents the inherently heterogeneous population of inpatient general medicine across the country and abroad. Hospitalists provided care for over 65% of the general medicine service, again representative of the trend in US inpatient medicine.27, 28 Lastly, our cohort did not have the benefit of a systematic and consistent discharge process with interventions focused on reducing readmissions. This gap, which is common across hospitals, highlights the utility of this data in targeting quality improvement efforts.
Reducing risks for readmission requires identification of patient populations at highest risk; in those patients, one can further identify factors which are potentially modifiable via education or patient‐engagement interventions. While in the hospital, more intensive predischarge counseling and efforts to increase mobility may be most useful if targeted early and often on those at highest risk.15, 16, 29, 30 Finally, broader‐based support in the form of better home services, more access to longitudinal care, or targeted postdischarge efforts may be required.14, 31
Though current strategies focus largely on clinical risk factors, this study shows that nonclinical factors play an equally important but underappreciated role in contributing to readmission. While prior studies have shown variable results on association of black race with readmission,2, 9, 11 none have evaluated or linked Medicaid to readmission, which just missed statistical significance in this study (OR, 1.15; 95% CI, 0.971.36). Both black race and Medicaid as payer are proxies for the underlying root cause aspects leading to readmission, such as access to longitudinal care. Following this trail to the root cause will require in‐depth qualitative evaluation that includes the patient perspective as a source of data.32 For example, risk for readmission may not stem solely from being on warfarin, but in combination with not having transportation to get an international normalized ratio (INR) checked, a suboptimal understanding of how to take the medication, or not recognizing potential side effects until too late to avoid inpatient admission.
Several of the strongest associations, and perhaps most conducive to targeted interventions, were high‐risk medications at discharge. Risk related to medications and adverse drug events following discharge have been a consistent theme in readmission literature.24, 33 Our current system, which includes mandated inpatient medicine reconciliation, does not encourage discontinuation of unnecessary medications to combat polypharmacy, address affordability of medications, provide consistent medication counseling, or focus on the highest risk medications. In fact, bundled interventions which implement pharmacists to focus on these measures have been successful in decreasing readmission,14, 16, 29 but unfortunately are not yet part of the standard of care. The challenge remains transforming a mandatory policy such as medicine reconciliation into a valuable and systematic tool in the discharge process.
Two factors were surprisingly protective against readmission: neurologic diagnosis and anticholinergic medications. This first may be explained by the presence of a separate neurology service at our institution which skews our data. For example, a patient with acute stroke, who has a 20% rate of bounce‐back to a higher level of care within 30 days of discharge,34 would be admitted to the neurology service, not general medicine, and therefore would not nr part of our cohort. Regarding anticholinergics, several factors may explain this unexpected result. First, use of anticholinergics was relatively rare in our sample (2.7% in readmitted patients, 4.1% in patients not readmitted), possibly creating a false positive result. Second, Hanlon et al.24 showed only a weak association at best between anticholinergics and postdischarge adverse drug reactions (hazard ratio, 1.11; 95% CI, 0.86‐1.43). Lastly, anticholinergics include a varied group of medications, therefore diluting possible relative risk of specific medications.
While this study allows providers to identify patients at increased risk of readmission, the identified factors do not fully account for readmission risk; we did not aim to produce a risk‐prediction rule with our study. Prior readmission studies have been unable to create a tool to predict which patients will be readmitted with much success.3537 These results underscore the complexities and variability of readmission, which often lack a clear single cause and effect relationship. Given the breadth of risk factors we identified, it seems likely that more intensive interventions will require a multidisciplinary approach, one which might be costly if applied broadly. Our study does not attempt to predict who will be readmitted and who will not, but rather provides a list of risk factors which might be used to deploy resources more efficiently.
This study had several limitations. We did not capture readmissions to outside hospitals, which account for 22% to 24% of all readmissions in prior studies, and therefore have underestimated the readmission rate in our population.2, 8 However, by limiting our data to 2 hospitals within 1 institution, we were able to include more detailed patient level data, which is not accurately available in other large databases. Also, while studies of risk factors in a managed care population (such as within Medicare, the Veterans Affairs medical centers, or countries with national integrated medical records) are able to capture all readmissions, this study is the first to evaluate readmissions risk factors in a truly heterogeneous U.S. inpatient medicine population without limitation by age or payer status. Second, we did not have access to outpatient medications lists; however use of these same medications within the last 48 hours of admission is likely a reasonable proxy for outpatient use and more conducive to potential interventions (such as medication reconciliation or patient education) that could flag patients prior to discharge. Payer data was limited to only the primary payer, so patients who were dual eligible (ie, have both Medicare and Medicaid) were categorized as Medicare. Regarding sociodemographic factors, while primary language other than English was not associated with readmission, language data was missing in 17.4% of admissions, thereby limiting our ability to evaluate this factor. Our data did not include access to outpatient or primary care, and therefore we were unable to evaluate access to postdischarge follow‐up care as a risk factor for readmission. Lastly, while this study did not include outpatient deaths, we did exclude patients who died in the hospital.
Conclusions
Readmission is common among general medicine patients, with approximately 1 in 5 patients being readmitted within 30 days. While the identified associated factors do not account for all the potential reasons for readmission, our study suggests a spectrum of risk factors which might be used to target more intensive multidisciplinary interventions. Specifically, the nonclinical factors of race and payer status merit further in depth research incorporating the patient experience to truly determine causation of readmission. Hospitalists, who are at nexus of the discharge process and uniquely invested in quality inpatient care, are ideally positioned to lead efforts to reduce readmissions. How to use our study's results to develop and implement effective interventions to reduce readmissions remains a subject for future studies.
- A path to bundled payment around a rehospitalization.: Medicare payment Advisory Commission; June2005.
- Rehospitalizations among patients in the Medicare fee‐for‐service program.N Engl J Med.2009;360(14):1418–1428. , , .
- University HealthSystem Consortium. Available at: https://www.uhc.edu. Accessed May2010.
- U.S. Department of Health 15(5):599–606.
- Development and validation of a model for predicting emergency admissions over the next year (PEONY): a UK historical cohort study.Arch Intern Med.2008;168(13):1416–1422. , , , .
- Incidence and main factors associated with early unplanned hospital readmission among French medical inpatients aged 75 and over admitted through emergency units.Age Ageing.2008;37(4):416–422. , , , et al.
- Factors associated with unplanned hospital readmission among patients 65 years of age and older in a Medicare managed care plan.Am J Med.1999;107(1):13–17. , , , , , .
- Risk factors for early unplanned hospital readmission in the elderly.J Gen Intern Med.1991;6(3):223–228. , , .
- Predicting non‐elective hospital readmissions: a multi‐site study. Department of Veterans Affairs Cooperative Study Group on Primary Care and Readmissions.J Clin Epidemiol.2000;53(11):1113–1118. , , , et al.
- Effect of gender, ethnicity, pulmonary disease, and symptom stability on rehospitalization in patients with heart failure.Am J Cardiol.2007;100(7):1139–1144. , .
- The care transitions intervention: results of a randomized controlled trial.Arch Intern Med.2006;166(17):1822–1828. , , , .
- The impact of follow‐up telephone calls to patients after hospitalization.Am J Med.2001;111(9B):26S–30S. , , , .
- A reengineered hospital discharge program to decrease rehospitalization: a randomized trial.Ann Intern Med.2009;150(3):178–187. , , , et al.
- Reduction of 30‐day postdischarge hospital readmission or emergency department (ED) visit rates in high‐risk elderly medical patients through delivery of a targeted care bundle.J Hosp Med.2009;4(4):211–218. , , , et al.
- Comprehensive discharge planning with postdischarge support for older patients with congestive heart failure: a meta‐analysis.JAMA.2004;291(11):1358–1367. , , , , , .
- Home‐based intervention in congestive heart failure: long‐term implications on readmission and survival.Circulation.2002;105(24):2861–2866. , .
- Effect of a standardized nurse case‐management telephone intervention on resource use in patients with chronic heart failure.Arch Intern Med.2002;162(6):705–712. , , , , , .
- The impact of follow‐up physician visits on emergency readmissions for patients with asthma and chronic obstructive pulmonary disease: a population‐based study.Am J Med.2002;112(2):120–125. , , , .
- Factors predicting readmission of older general medicine patients.J Gen Intern Med.1991;6(5):389–393. , .
- BOOSTing Care Transitions. Available at: http://www.hospitalmedicine.org/ResourceRoomRedesign/RR_CareTransitions/CT_Home.cfm. Accessed May2010.
- Adverse drug events occurring following hospital discharge.J Gen Intern Med.2005;20(4):317–323. , , , , .
- Incidence and predictors of all and preventable adverse drug reactions in frail elderly persons after hospital stay.J Gerontol A Biol Sci Med Sci.2006;61(5):511–515. , , , et al.
- http://www.ahrq.gov/qual/mortality/Hughes.htm. Accessed May2010. . Development of the 3M™ All Patient Refined Diagnosis Related Groups (APR DRGs). Available at:
- Volume thresholds and hospital characteristics in the United States.Health Aff (Millwood).2003;22(2):167–177. , , .
- The status of hospital medicine groups in the United States.J Hosp Med.2006;1(2):75–80. , , , .
- Growth in the care of older patients by hospitalists in the United States.N Engl J Med.2009;360(11):1102–1112. , , , .
- Role of pharmacist counseling in preventing adverse drug events after hospitalization.Arch Intern Med.2006;166(5):565–571. , , , et al.
- Effects of a multicomponent intervention on functional outcomes and process of care in hospitalized older patients: a randomized controlled trial of Acute Care for Elders (ACE) in a community hospital.J Am Geriatr Soc.2000;48(12):1572–1581. , , , et al.
- Telehome monitoring in patients with cardiac disease who are at high risk of readmission.Heart Lung.2008;37(1):36–45. , , , , , .
- Understanding rehospitalization risk: can hospital discharge be modified to reduce recurrent hospitalization?J Hosp Med.2007;2(5):297–304. , , .
- The incidence and severity of adverse events affecting patients after discharge from the hospital.Ann Intern Med.2003;138(3):161–167. , , , , .
- Bouncing back: patterns and predictors of complicated transitions 30 days after hospitalization for acute ischemic stroke.J Am Geriatr Soc.2007;55(3):365–373. , , , .
- Case finding for patients at risk of readmission to hospital: development of algorithm to identify high risk patients.BMJ.2006;333(7563):327. , , , .
- Screening elders for risk of hospital admission.J Am Geriatr Soc.1993;41(8):811–817. , , , , , .
- Using routine inpatient data to identify patients at risk of hospital readmission.BMC Health Serv Res.2009;9:96. , , , .
- A path to bundled payment around a rehospitalization.: Medicare payment Advisory Commission; June2005.
- Rehospitalizations among patients in the Medicare fee‐for‐service program.N Engl J Med.2009;360(14):1418–1428. , , .
- University HealthSystem Consortium. Available at: https://www.uhc.edu. Accessed May2010.
- U.S. Department of Health 15(5):599–606.
- Development and validation of a model for predicting emergency admissions over the next year (PEONY): a UK historical cohort study.Arch Intern Med.2008;168(13):1416–1422. , , , .
- Incidence and main factors associated with early unplanned hospital readmission among French medical inpatients aged 75 and over admitted through emergency units.Age Ageing.2008;37(4):416–422. , , , et al.
- Factors associated with unplanned hospital readmission among patients 65 years of age and older in a Medicare managed care plan.Am J Med.1999;107(1):13–17. , , , , , .
- Risk factors for early unplanned hospital readmission in the elderly.J Gen Intern Med.1991;6(3):223–228. , , .
- Predicting non‐elective hospital readmissions: a multi‐site study. Department of Veterans Affairs Cooperative Study Group on Primary Care and Readmissions.J Clin Epidemiol.2000;53(11):1113–1118. , , , et al.
- Effect of gender, ethnicity, pulmonary disease, and symptom stability on rehospitalization in patients with heart failure.Am J Cardiol.2007;100(7):1139–1144. , .
- The care transitions intervention: results of a randomized controlled trial.Arch Intern Med.2006;166(17):1822–1828. , , , .
- The impact of follow‐up telephone calls to patients after hospitalization.Am J Med.2001;111(9B):26S–30S. , , , .
- A reengineered hospital discharge program to decrease rehospitalization: a randomized trial.Ann Intern Med.2009;150(3):178–187. , , , et al.
- Reduction of 30‐day postdischarge hospital readmission or emergency department (ED) visit rates in high‐risk elderly medical patients through delivery of a targeted care bundle.J Hosp Med.2009;4(4):211–218. , , , et al.
- Comprehensive discharge planning with postdischarge support for older patients with congestive heart failure: a meta‐analysis.JAMA.2004;291(11):1358–1367. , , , , , .
- Home‐based intervention in congestive heart failure: long‐term implications on readmission and survival.Circulation.2002;105(24):2861–2866. , .
- Effect of a standardized nurse case‐management telephone intervention on resource use in patients with chronic heart failure.Arch Intern Med.2002;162(6):705–712. , , , , , .
- The impact of follow‐up physician visits on emergency readmissions for patients with asthma and chronic obstructive pulmonary disease: a population‐based study.Am J Med.2002;112(2):120–125. , , , .
- Factors predicting readmission of older general medicine patients.J Gen Intern Med.1991;6(5):389–393. , .
- BOOSTing Care Transitions. Available at: http://www.hospitalmedicine.org/ResourceRoomRedesign/RR_CareTransitions/CT_Home.cfm. Accessed May2010.
- Adverse drug events occurring following hospital discharge.J Gen Intern Med.2005;20(4):317–323. , , , , .
- Incidence and predictors of all and preventable adverse drug reactions in frail elderly persons after hospital stay.J Gerontol A Biol Sci Med Sci.2006;61(5):511–515. , , , et al.
- http://www.ahrq.gov/qual/mortality/Hughes.htm. Accessed May2010. . Development of the 3M™ All Patient Refined Diagnosis Related Groups (APR DRGs). Available at:
- Volume thresholds and hospital characteristics in the United States.Health Aff (Millwood).2003;22(2):167–177. , , .
- The status of hospital medicine groups in the United States.J Hosp Med.2006;1(2):75–80. , , , .
- Growth in the care of older patients by hospitalists in the United States.N Engl J Med.2009;360(11):1102–1112. , , , .
- Role of pharmacist counseling in preventing adverse drug events after hospitalization.Arch Intern Med.2006;166(5):565–571. , , , et al.
- Effects of a multicomponent intervention on functional outcomes and process of care in hospitalized older patients: a randomized controlled trial of Acute Care for Elders (ACE) in a community hospital.J Am Geriatr Soc.2000;48(12):1572–1581. , , , et al.
- Telehome monitoring in patients with cardiac disease who are at high risk of readmission.Heart Lung.2008;37(1):36–45. , , , , , .
- Understanding rehospitalization risk: can hospital discharge be modified to reduce recurrent hospitalization?J Hosp Med.2007;2(5):297–304. , , .
- The incidence and severity of adverse events affecting patients after discharge from the hospital.Ann Intern Med.2003;138(3):161–167. , , , , .
- Bouncing back: patterns and predictors of complicated transitions 30 days after hospitalization for acute ischemic stroke.J Am Geriatr Soc.2007;55(3):365–373. , , , .
- Case finding for patients at risk of readmission to hospital: development of algorithm to identify high risk patients.BMJ.2006;333(7563):327. , , , .
- Screening elders for risk of hospital admission.J Am Geriatr Soc.1993;41(8):811–817. , , , , , .
- Using routine inpatient data to identify patients at risk of hospital readmission.BMC Health Serv Res.2009;9:96. , , , .
Copyright © 2010 Society of Hospital Medicine
Hospitalist Effects on Acute IGIH Patients
Acute upper gastrointestinal hemorrhage (UGIH) is one of the most common hospital admissions for acute care. Estimates indicate that 300,000 patients (100‐150 cases per 100,000 adults) are admitted annually with an associated economic impact of $2.5 billion.15 The current standard management of UGIH requires hospital admission and esophagogastroduodenoscopy (EGD) by a gastroenterologist for diagnosis and/or treatment. This management strategy results in a high consumption of hospital resources and costs.
Simultaneously, hospitalists have dramatically changed the delivery of inpatient care in the United States and are recognized as a location‐driven subspecialty for the care of acute hospitalized patients, similar to emergency medicine. Currently there are 20,000 hospitalists, and more than one‐third of general medicine inpatients are cared for by hospitalists.6, 7
Previous studies have shown that hospitalist care offers better or comparable outcomes, with lower overall length of stay (LOS) and costs compared to traditional providers.810 However, most of these studies were performed in single institutions, had weak designs or little‐to‐no adjustment for severity of illness, or were limited to 7 specific diseases (pneumonia, congestive heart failure [CHF], chest pain, ischemic stroke, urinary tract infection, chronic obstructive lung disease [COPD], and acute myocardial infarction [AMI]).8
Furthermore, less is known about the effect of hospitalists on conditions that may be dependent upon specialist consultation for procedures and/or treatment plans. In this study, gastroenterologists performed diagnostic and/or therapeutic endoscopy work as consultants to the attending physicians in the management of acute inpatient UGIH.
To explore the effects of hospitalists on care of patients with acute UGIH, we examined data from the Multicenter Hospitalist (MCH) trial. The objectives of our study were to compare clinical outcomesin‐hospital mortality and complications (ie, recurrent bleeding, intensive care unit [ICU] transfer, decompensation, transfusion, reendoscopy, 30‐day readmission)and efficiency (LOS and costs) in hospitalized acute UGIH patients cared for by hospitalists and nonhospitalists in 6 academic centers in the United States during a 2‐year period.
Patients and Methods
Study Sites
From July 1, 2001 to June 30, 2003, the MCH trial1113 was a prospective, multicenter, observational trial of the care provided by hospitalists to patients admitted to general medical services at 6 academic medical institutions. There were 31,000 consecutive admissions to the general medical services of these participating sites: University of Chicago (Chicago, IL), University of Wisconsin Hospital (Madison, WI), University of Iowa (Iowa City, IA), University of California at San Francisco (San Francisco, CA), University of New Mexico (Albuquerque, NM), and Brigham and Women's Hospital (Boston, MA). The study was approved by the institutional review boards (IRBs) at each of the 6 participating institutions.
MCH Study Patients
Patients were eligible if they were admitted to the general medical services under the care of a hospitalist or nonhospitalist physician. Regardless of the admitting provider, each medical service was composed of rotating senior and junior resident physicians in all 6 sites. Furthermore, patients were 18 years of age or older, and were able to give consent themselves or had an appropriate proxy. Patients with mini‐mental status score of 17 (out of 22), admitted under their primary care physician or to an inpatient gastroenterology service, or transferred from another hospital, were excluded. The MCH study was designed to study the outcomes and efficiency in patients admitted for CHF, pneumonia, UGIH, and end‐of‐life care.
Acute UGIH Patients
Within the MCH‐eligible patients, we identified those with acute UGIH using the following International Classification of Diseases, 9th edition (ICD‐9) codes assigned at discharge: esophageal varices with hemorrhage (456.0, 456.20); Mallory‐Weiss syndrome (530.7); gastric ulcer with hemorrhage (531.00531.61); duodenal ulcer with hemorrhage (532.00532.61); peptic ulcer, site unspecified, with hemorrhage (533.00533.61); gastrojejunal ulcer with hemorrhage (534.00534.61); gastritis with hemorrhage (535.61); angiodysplasia of stomach/duodenum with hemorrhage (537.83); and hematemesis (578.0, 578.9). We also confirmed the diagnosis of UGIH by reviewing patient medical records for observed hematemesis, nasogastric tube aspirate with gross or hemoccult blood, or clinical history of hematemesis, melena, or hematochezia.14, 15
Data
All data were obtained from the 6 hospitals' administrative records, patient interviews, and medical chart abstractions. Dates of admission and discharge, ICD‐9 diagnosis codes, insurance type, age, race, and gender were obtained from administrative data. One‐month follow‐up telephone interviews assessed whether or not patient had any follow‐up appointment or hospital readmissions. Trained abstractors from each site performed manual chart reviews using a standard data collection sheet. The ICD‐9 code designation and chart abstraction methodology were developed prior to the initiation of the study to ensure consistent data collection and reduce bias.
The following data elements were collected: comorbidities, endoscopic findings, inpatient mortality, clinical evidence of rebleeding, endoscopic treatment or gastrointestinal (GI) surgery to control bleeding, repeat EGD, ICU transfer, decompensated comorbid illness requiring continued hospitalization, and blood transfusion (packed red cells, plasma, platelets). Clinical evidence of rebleeding was defined as either hematemesis or melena with decrease in hemoglobin of 2 g in 24 hours with or without hemodynamic compromise.14, 15 For the purpose of this study, recurrent bleeding was defined as clinical evidence of rebleeding, emergency GI surgery for control of UGIH, or repeat EGD before discharge. Furthermore, a composite endpoint termed total complications encompassed all adverse outcomes related to the UGIH hospitalization. The 30‐day readmission variable was defined using readmission identified in administrative records and a 30‐day follow‐up phone call. To guard against recall bias, self‐report data was only included for nonsite admissions.
We defined efficiency in terms of costs and LOS. Total hospital costs were measured using the TSI cost accounting system (Transition Systems, Inc., Boston, MA; now Eclipsys Corporation)16, 17 at 5 out of the 6 participating sites. TSI is a hospital cost accounting software system that integrates resource utilization and financial data already recorded in other hospital databases (such as the billing system, payroll system, and general ledger system).17 Hospital LOS was defined as the number of days from patient admission to the general medicine service until patient discharge.
Provider Specialization: Hospitalists vs. Nonhospitalists
The study was designed as a natural experiment based on a call cycle. The hospitalist‐led teams at each institution alternated in a 4‐day or 5‐day general medicine call cycle with teams led by traditional academic internal medicine attending physicians. All patients were assigned to teams according to their position in the call cycle without regard to whether the attending physician was a hospitalist or a nonhospitalist. Hospitalists are physicians whose primary professional focus is the general medical care of hospitalized patients.18, 19 As previously reported in a related MCH work,11 a hospitalist was also defined as a provider who spends at least 25% of his or her time on an academic inpatient general medicine service. Nonhospitalist physicians were most often outpatient general internal medicine faculty or subspecialists, who attended 1 month per year. Physicians were classified as hospitalists or nonhospitalists according to the designations provided by each site.
UGIH‐specific Confounders
From chart abstraction, we captured severity of illness, comorbidity, and performance of early EGD, variables that can confound analysis in UGIH. To capture severity of illness, a complete Rockall risk score was calculated for each patient. The complete Rockall uses 3 clinical variables (age, shock, and comorbidity) and 2 endoscopic variables (endoscopic diagnosis and stigmata of recent hemorrhage).5, 20 A complete Rockall score of 2 is considered low‐risk for rebleeding or death following admission.21, 22 The accepted definition of low‐risk is <5% recurrent bleeding and <1% mortality risk. A complete Rockall score of 3 to 5 is considered moderate‐risk while 6 is considered high‐risk. Comorbidity was measured using the Charlson comorbidity index.23 Performance of early endoscopy, usually defined as endoscopy performed within 24 hours from presentation, was previously shown to decrease LOS and need for surgical intervention in patients with acute UGIH.24, 25 Documented times of presentation to the emergency department and time of endoscopy performance were collected to calculate for the rate of early endoscopy in our study population.
Statistical Analysis
All statistical analyses were performed using SAS Version 9.1 for Windows (SAS Institute, Cary, NC).
Differences in baseline demographic characteristics of patients and their endoscopic findings were compared between the 2 types of providers. Univariate analyses were also performed to compare the differences in adverse outcomes, LOS, and costs between patients cared for by hospitalists and nonhospitalists. Chi‐square tests were used for categorical variables; while both Wilcoxon rank sum test and Student's t test were used in the analysis of continuous variables.
Next, we performed multivariable analyses to determine the independent association between hospitalist care and the odds of the patients having certain outcomes. However, to prevent overfitting, we only developed regression models for adverse outcomes that have at least 20% event rate.
Multivariable regression models were developed separately for LOS and costs. In contrast with the models on outcomes, analyses of LOS and costs were restricted to: (1) patients who were discharged alive; and (2) to cases with LOS and costs values within 3 standard deviations (SDs) of the mean because of the skewed nature of these data.
All models were adjusted for age, gender, race, insurance type, complete Rockall risk score, performance of early EGD, Charlson comorbidity index, and study site. Final candidate variables in the models were chosen based on stepwise selection, a method very similar to forward selection except that variables selected for the model do not necessarily remain in the model. Effects were entered into and then removed from the model in such a way that each forward selection step can be followed by 1 or more backward elimination steps. The stepwise selection was terminated if no further effect can be added to the model or if the current model was identical to the previous model. The stepwise selection model was generated using statistical criterion of alpha = 0.05 for entry and elimination from the model. Variables that can be a profound source of variation, such as study site and treating physician, were included in the model irrespective of their statistical significance.
To account for clustering of patients treated by the same physician, we used multilevel modeling with SAS PROC GLIMMIX (with random effects). For outcomes (categorical variables), we utilized models with logit‐link and binomial‐distributed errors. As for efficiency (continuous variables with skewed distribution), the multivariable analyses used a generalized linear model with log‐link and assuming gamma‐distributed errors.
Results
Patient Characteristics and Endoscopic Diagnoses
Out of 31,000 patients, the study identified a total of 566 patients (1.8%) with acute UGIH (Table 1). However, 116 patients transferred from another hospital were excluded as their initial management was provided elsewhere, giving a final study sample of 450 patients. Overall, there are 163 admitting physicians from 6 sites, with 39 (24%) classified as hospitalists and 124 (76%) as nonhospitalists. Forty‐two percent (177/450) of patients were cared for by hospitalists. Compared to nonhospitalists, patients admitted to the hospitalist service were older (62.8 vs. 57.7 years, P < 0.01) and with third‐party payor mix differences (P < 0.01). However, there were no statistical differences between patients attended by hospitalists and nonhospitalists with regard to Complete Rockall risk score, Charlson comorbidity index, performance of early endoscopy, and mean hemoglobin values on admission. Upper endoscopy was performed in all patients with distribution of the 3 most common diagnoses being similar (P > 0.05) between hospitalists and nonhospitalists: erosive disease (49.7% vs. 54.6%), peptic ulcer disease (PUD) (48% vs. 46.9%), and varices (18.6% vs. 14.7%).
Variable | Admitting Service | P | |
---|---|---|---|
Hospitalist (n = 177) | Nonhospitalist (n = 273) | ||
| |||
Age, years (meanSD) | 62.817.4 | 57.718.5 | <0.01 |
Male sex, n (%) | 104 (58.8) | 169 (61.9) | 0.50 |
Ethnicity, n (%) | 0.13 | ||
White | 83 (46.9) | 102 (37.4) | |
African‐American | 34 (19.2) | 75 (27.5) | |
Hispanic | 21 (11.9) | 40 (14.7) | |
Asian/Pacific Islander | 24 (13.6) | 29 (10.6) | |
Others/unknown | 15 (8.5) | 27 (9.9) | |
Insurance, n (%) | <0.01 | ||
Medicare | 86 (48.6) | 104 (38.1) | |
Medicaid | 15 (8.5) | 33 (12.1) | |
No payer | 18 (10.2) | 36 (13.2) | |
Private | 46 (26) | 52 (19.1) | |
Unknown | 12 (6.8) | 48 (17.5) | |
Charlson Comorbidity Index (meanSD) | 1.91.6 | 1.81.7 | 0.51 |
Complete Rockall, n (%) | 0.11 | ||
Low‐risk (0‐2) | 82 (46.3) | 103 (37.7) | |
Moderate‐risk (3‐5) | 71 (40.1) | 137 (50.2) | |
High‐risk (6) | 24 (14.6) | 33 (12.1) | |
Early endoscopy (<24 hours) | 82 (46.3) | 133 (48.7) | 0.62 |
Endoscopic diagnosis, n (%)* | |||
Erosive disease | 88 (49.7) | 149 (54.6) | 0.31 |
Peptic ulcer disease | 85 (48.0) | 128 (46.9) | 0.81 |
Varices | 33 (18.6) | 40 (14.7) | 0.26 |
Mallory‐Weiss tear | 9 (5.1) | 21 (7.7) | 0.28 |
Angiodysplasia | 9 (5.1) | 13 (4.8) | 0.88 |
GI mass | 1 (0.6) | 4 (1.5) | 0.65 |
Normal | 7 (4.0) | 8 (2.9) | 0.55 |
Admission hemoglobin values (meanSD) | 10.22.9 | 10.22.9 | 0.78 |
Clinical Outcomes
Between hospitalists and nonhospitalists, unadjusted outcomes were similar (P > 0.05) for mortality (2.3% vs. 0.4%), recurrent bleeding (11% vs. 11%), need for endoscopic therapy (24% vs. 22%), ICU‐transfer and decompensation (15% vs. 15%), as well as an overall composite measure of any complication (79% vs. 72%) (Table 2). However, the hospitalist‐led teams performed more blood transfusions (74% vs. 63%, P = 0.02) and readmission rates were higher (7.3% vs. 3.3%, P = 0.05).
Outcomes, n (%) | Admitting Service | P | |
---|---|---|---|
Hospitalist (n = 177) | Nonhospitalist (n = 273) | ||
| |||
Inpatient mortality | 4 (2.3) | 1 (0.4) | 0.08 |
Recurrent bleeding* | 20 (11.3) | 29 (10.6) | 0.88 |
Endoscopic therapy | 43 (24.3) | 60 (22.0) | 0.57 |
ICU transfers | 23 (13) | 24 (8.8) | 0.20 |
Decompensated comorbidities that required continued hospitalization | 26 (14.7) | 41 (15.0) | 0.92 |
Any transfusion | 131 (74.0) | 172 (63.0) | 0.02 |
Total complications | 139 (78.5) | 196 (71.8) | 0.11 |
30‐day all‐cause readmissions | 13 (7.3) | 9 (3.3) | 0.05 |
Efficiency | Hospitalist (n = 164) | Nonhospitalist (n = 259) | P |
LOS, days | |||
MeanSD | 4.83.5 | 4.53.0 | 0.30 |
Median (interquartile range) | 4 (36) | 4 (26) | 0.69 |
Total costs, U.S. $ | |||
MeanSD | 10,466.669191.00 | 7926.716065.00 | <0.01 |
Median (interquartile range) | 7359.00 (4,698.0012,550.00) | 6181.00 (3744.0010,344.00) | <0.01 |
Because of the low event rate of certain adverse outcomes (<20%), we were only able to perform adjusted analyses on 4 outcomes: need for endoscopic therapy (odds ratio [OR], 0.82; 95% confidence interval [CI], 0.491.37), ICU transfer and decompensation (OR, 0.82; 95% CI, 0.451.52), blood transfusion (OR, 1.30; 95% CI, 0.822.04), and any complication (OR, 1.18; 95% CI, 0.711.96). Since outcome differences disappeared after controlling for confounders, the data suggest that overall care provided by hospitalists and nonhospitalists might be equivalenteven in certain outcomes that we were unable to substantiate using multivariable methods.
Efficiency
Efficiency, as measured by LOS and costs, are presented both as means and medians in univariate analyses in Table 2. Median LOS was similar for hospitalist‐led and nonhospitalist‐led teams (4 days). Despite having similar LOS, the median costs of acute UGIH in patients cared for by hospitalists were higher ($7,359.00 vs. $6,181.00; P < 0.01).
After adjusting for demographic factors, Rockall risk score, comorbidity, early EGD, and hospital site, LOS remained similar between the 2 groups. On the other hand, the adjusted cost for UGIH patients cared for by hospitalists and nonhospitalists persisted, with hospitalist care costs $1,502.40 more than their nonhospitalist counterparts (Table 3).
Efficiency | Treatment Provider | P | |
---|---|---|---|
Hospitalist (n = 164) | Nonhospitalist (n = 259) | ||
| |||
Adjusted length of stay, days (mean SD) | 5.2 (4.95.6) | 4.7 (4.55.0) | 0.15 |
Adjusted total cost, U.S. $ (mean SD) | 9006.50 (8366.609693.60) | 7504.10 (7069.907964.20) | 0.03 |
Discussion
This is the first study that has looked at the effect of hospitalists on clinical outcomes and efficiency in patients admitted for acute UGIH, a condition highly dependent upon another specialty for procedures and management. This is also one of only a few studies on UGIH that adjusted for severity of illness (Rockall score), comorbidity, performance of early endoscopypatient‐level confounders usually unaccounted for in prior research.
We show that hospitalists and nonhospitalists caring for acute UGIH patients had overall similar unadjusted outcomes; except for blood transfusion and 30‐day readmission rates. Unfortunately, due to the small number of events for readmissions, we were unable to perform adjusted analysis for readmission. Differences between hospitalists and nonhospitalists on blood transfusion rates were not substantiated on multivariable adjustments.
As for efficiency, univariable and multivariable analyses revealed that LOS was similar between provider types while costs were greater in UGIH patients attended by hospitalists.
Reductions in resource use, particularly costs, may be achieved by increasing throughput (eg, reducing LOS) or by decreasing service intensity (eg, using fewer ancillary services and specialty consultations).26 Specifically in acute UGIH, LOS is significantly affected by performance of early EGD.27, 28 In these studies, gastroenterologist‐led teams, compared to internists and surgeons, have easier access to endoscopy, thus reducing LOS and overall costs.27, 28
Similarly, prior studies have shown that the mechanism by which hospitalists lower costs is by decreasing LOS.810, 29 There are several hypotheses on how hospitalists affect LOS. Hospitalists, by being available all day, are thought to respond quickly to acute symptoms or new test results, are more efficient in navigating the complex hospital environment, or develop greater expertise as a result of added inpatient experience.8 On the downside, although the hospitalist model reduces overall LOS and costs, they also provide higher intensity of care as reflected by greater costs when broken down per hospital day.29 Thus, the cost differential we found may represent higher intensity of care by hospitalists in their management of acute UGIH, as higher intensity care without decreasing LOS can translate to higher costs.
In addition, patients with acute UGIH are unique in several respects. In contrast to diseases like heart failure, COPD, and pneumonia, in which the admitting provider has the option to request a subspecialist consultation, all patients with acute UGIH need a gastroenterologist to perform endoscopy as part of the management. These patients are usually admitted to general medicine wards, aggressively resuscitated with intravenous fluids, with a nonurgent gastroenterology consult or EGD performed on the next available schedule.
Aside from LOS being greatly affected by performance of early EGD and/or delay in consulting gastroenterology, sicker patients require longer hospitalization and drive LOS and healthcare costs up. It was therefore crucial that we accounted for severity of illness, comorbidity, and performance of early EGD in our regression models for LOS and costs. This approach allows us to acquire a more accurate estimate on the effects of hospitalist on LOS and costs in patients admitted with acute UGIH.
Our findings suggest that the academic hospitalist model of care may not have as great of an impact on hospital efficiency in certain patient groups that require nonurgent subspecialty consultations. Future studies should focus on elucidating these relationships.
Limitations
This study has several limitations. First, clinical data were abstracted at 6 sites by different abstractors so it is possible there were variations in how data were collected. To reduce variation, a standardized abstraction form with instructions was developed and the primary investigator (PI) was available for specific questions during the abstraction process. Second, only 5 out of the 6 sites used TSI accounting systems. Although similar, interhospital costs captured by TSI may vary among sites in terms of classifying direct and indirect costs, potentially resulting in misclassification bias in our cost estimates.17 We addressed these issues by including the hospital site variable in our regression models, regardless of its significance. Third, consent rates across sites vary from 70% to 85%. It is possible that patients who refused enrollment in the MCH trial are systematically different and may introduce bias in our analysis.
Furthermore, the study was designed as a natural experiment based on a rotational call cycle between hospitalist‐led and nonhospitalist‐led teams. It is possible that the order of patient assignment might not be completely naturally random as we intended. However, the study period was for 2 years and we expect the effect of order would have averaged out in time.
There are many hospitalist models of care. In terms of generalizability, the study pertains only to academic hospitalists and may not be applicable to hospitalists practicing in community hospitals. For example, the nonhospitalist comparison group is likely different in the community and academic settings. Community nonhospitalists (traditional practitioners) are usually internists covering both inpatient and outpatient responsibilities at the same time. In contrast, academic nonhospitalists are internists or subspecialists serving as ward attendings for a limited period (usually 1 month) with considerable variation in their nonattending responsibilities (eg, research, clinic, administration). Furthermore, academic nonhospitalist providers might be a self‐selected group by their willingness to serve as a ward attending, making them more hospitalist‐like. Changes and variability of inpatient attendings may also affect our findings when compared to prior work. Finally, it is also possible that having residents at academic medical centers may attenuate the effect of hospitalists more than in community‐based models.
Conclusions/Implications
Compared to nonhospitalists, academic hospitalist care of acute UGIH patients had similar overall clinical outcomes. However, our finding of similar LOS yet higher costs for patients cared for by hospitalists support 1 proposed mechanism in which hospitalists decrease healthcare costs: providing higher intensity of care per day of hospitalization. However, in academic hospitalist models, this higher intensity hypothesis should be revisited, especially in certain patient groups in which timing and involvement of subspecialists may influence discharge decisions, affecting LOS and overall costs.
Due to inherent limitations in this observational study, future studies should focus on verifying and elucidating these relationships further. Lastly, understanding which patient groups receive the greatest potential benefit from this model will help guide both organizational efforts and quality improvement strategies.
- Bleeding peptic ulcer.N Engl J Med.1994;331(11):717–727. , .
- Epidemiology of hospitalization for acute upper gastrointestinal hemorrhage: a population‐based study.Am J Gastroenterol.1995;90(2):206–210. .
- Variation in outcome after acute upper gastrointestinal haemorrhage. the national audit of acute upper gastrointestinal haemorrhage.Lancet.1995;346(8971):346–350. , , , et al.
- Influencing the practice and outcome in acute upper gastrointestinal haemorrhage. Steering committee of the National Audit of Acute Upper Gastrointestinal Haemorrhage.Gut.1997;41(5):606–611. , , , et al.
- Risk assessment after acute upper gastrointestinal haemorrhage.Gut.1996;38(3):316–321. , , , et al.
- The potential size of the hospitalist workforce in the united states.Am J Med.1999;106(4):441–445. , , , et al.
- Society of Hospital Medicine. About SHM. Available at: http://www.hospitalmedicine.org/AM/Template.cfm?Section=General_Information357(25):2589–2600.
- Effects of physician experience on costs and outcomes on an academic general medicine service: results of a trial of hospitalists.Ann Intern Med.2002;137(11):866–874. , , , et al.
- A systematic review of outcomes and quality measures in adult patients cared for by hospitalists vs nonhospitalists.Mayo Clin Proc.2009;84(3):248–254. .
- Do hospitalists or physicians with greater inpatient HIV experience improve HIV care in the era of highly active antiretroviral therapy? Results from a multicenter trial of academic hospitalists.Clin Infect Dis.2008;46(7):1085–1092. , , , et al.
- Quality of care for decompensated heart failure: comparable performance between academic hospitalists and non‐hospitalists. J Gen Intern Med.2008;23(9):1399–1406. , , , et al.
- Factors associated with discussion of care plans and code status at the time of hospital admission: results from the Multicenter Hospitalist Study.J Hosp Med.2008;3(6):437–445. , , , et al.
- Upper gastrointestinal hemorrhage clinical guideline determining the optimal hospital length of stay.Am J Med.1996;100(3):313–322. , , , et al.
- Prospective evaluation of a clinical guideline recommending hospital length of stay in upper gastrointestinal tract hemorrhage.JAMA.1997;278(24):2151–2156. , , , et al.
- In‐hospital cost of abdominal aortic aneurysm repair in Canada and the United States.Arch Intern Med.2003;163(20):2500–2504. , , , et al.
- The use of transition cost accounting system in health services research.Cost Eff Resour Alloc.2007;5:11. , , , et al.
- Society of Hospital Medicine. Definition of a Hospitalist. Available at: http://www.hospitalmedicine.org/AM/Template.cfm?Section=General_Information335(7):514–517.
- Selection of patients for early discharge or outpatient care after acute upper gastrointestinal haemorrhage. National Audit of Acute Upper Gastrointestinal Haemorrhage.Lancet.1996;347(9009):1138–1140. , , , et al.
- Utilization of health care resources for low‐risk patients with acute, nonvariceal upper GI hemorrhage: an historical cohort study.Gastrointest Endosc.2002;55(3):321–327. , , , et al.
- Incremental value of upper endoscopy for triage of patients with acute non‐variceal upper‐GI hemorrhage.Gastrointest Endosc.2004;60(1):9–14. , .
- The Charlson comorbidity index is adapted to predict costs of chronic disease in primary care patients.J Clin Epidemiol.2008;61(12):1234–1240. , , , et al.
- The effectiveness of early endoscopy for upper gastrointestinal hemorrhage: a community‐based analysis.Med Care.1998;36(4):462–474. , , , et al.
- Early endoscopy in upper gastrointestinal hemorrhage: associations with recurrent bleeding, surgery, and length of hospital stay.Gastrointest Endosc.1999;49(2):145–152. , , , et al.
- The impact of hospitalists on the cost and quality of inpatient care in the united states: a research synthesis.Med Care Res Rev.2005;62(4):379–406. , .
- Physician specialty and variations in the cost of treating patients with acute upper gastrointestinal bleeding.Gastroenterology.1997;113(5):1443–1448. , , , et al.
- Impact of physician specialty on the cost of nonvariceal upper GI bleeding care.Am J Gastroenterol.2002;97(6):1535–1542. , , , et al.
- Associations with reduced length of stay and costs on an academic hospitalist service.Am J Manag Care.2004;10(8):561–568. , , .
Acute upper gastrointestinal hemorrhage (UGIH) is one of the most common hospital admissions for acute care. Estimates indicate that 300,000 patients (100‐150 cases per 100,000 adults) are admitted annually with an associated economic impact of $2.5 billion.15 The current standard management of UGIH requires hospital admission and esophagogastroduodenoscopy (EGD) by a gastroenterologist for diagnosis and/or treatment. This management strategy results in a high consumption of hospital resources and costs.
Simultaneously, hospitalists have dramatically changed the delivery of inpatient care in the United States and are recognized as a location‐driven subspecialty for the care of acute hospitalized patients, similar to emergency medicine. Currently there are 20,000 hospitalists, and more than one‐third of general medicine inpatients are cared for by hospitalists.6, 7
Previous studies have shown that hospitalist care offers better or comparable outcomes, with lower overall length of stay (LOS) and costs compared to traditional providers.810 However, most of these studies were performed in single institutions, had weak designs or little‐to‐no adjustment for severity of illness, or were limited to 7 specific diseases (pneumonia, congestive heart failure [CHF], chest pain, ischemic stroke, urinary tract infection, chronic obstructive lung disease [COPD], and acute myocardial infarction [AMI]).8
Furthermore, less is known about the effect of hospitalists on conditions that may be dependent upon specialist consultation for procedures and/or treatment plans. In this study, gastroenterologists performed diagnostic and/or therapeutic endoscopy work as consultants to the attending physicians in the management of acute inpatient UGIH.
To explore the effects of hospitalists on care of patients with acute UGIH, we examined data from the Multicenter Hospitalist (MCH) trial. The objectives of our study were to compare clinical outcomesin‐hospital mortality and complications (ie, recurrent bleeding, intensive care unit [ICU] transfer, decompensation, transfusion, reendoscopy, 30‐day readmission)and efficiency (LOS and costs) in hospitalized acute UGIH patients cared for by hospitalists and nonhospitalists in 6 academic centers in the United States during a 2‐year period.
Patients and Methods
Study Sites
From July 1, 2001 to June 30, 2003, the MCH trial1113 was a prospective, multicenter, observational trial of the care provided by hospitalists to patients admitted to general medical services at 6 academic medical institutions. There were 31,000 consecutive admissions to the general medical services of these participating sites: University of Chicago (Chicago, IL), University of Wisconsin Hospital (Madison, WI), University of Iowa (Iowa City, IA), University of California at San Francisco (San Francisco, CA), University of New Mexico (Albuquerque, NM), and Brigham and Women's Hospital (Boston, MA). The study was approved by the institutional review boards (IRBs) at each of the 6 participating institutions.
MCH Study Patients
Patients were eligible if they were admitted to the general medical services under the care of a hospitalist or nonhospitalist physician. Regardless of the admitting provider, each medical service was composed of rotating senior and junior resident physicians in all 6 sites. Furthermore, patients were 18 years of age or older, and were able to give consent themselves or had an appropriate proxy. Patients with mini‐mental status score of 17 (out of 22), admitted under their primary care physician or to an inpatient gastroenterology service, or transferred from another hospital, were excluded. The MCH study was designed to study the outcomes and efficiency in patients admitted for CHF, pneumonia, UGIH, and end‐of‐life care.
Acute UGIH Patients
Within the MCH‐eligible patients, we identified those with acute UGIH using the following International Classification of Diseases, 9th edition (ICD‐9) codes assigned at discharge: esophageal varices with hemorrhage (456.0, 456.20); Mallory‐Weiss syndrome (530.7); gastric ulcer with hemorrhage (531.00531.61); duodenal ulcer with hemorrhage (532.00532.61); peptic ulcer, site unspecified, with hemorrhage (533.00533.61); gastrojejunal ulcer with hemorrhage (534.00534.61); gastritis with hemorrhage (535.61); angiodysplasia of stomach/duodenum with hemorrhage (537.83); and hematemesis (578.0, 578.9). We also confirmed the diagnosis of UGIH by reviewing patient medical records for observed hematemesis, nasogastric tube aspirate with gross or hemoccult blood, or clinical history of hematemesis, melena, or hematochezia.14, 15
Data
All data were obtained from the 6 hospitals' administrative records, patient interviews, and medical chart abstractions. Dates of admission and discharge, ICD‐9 diagnosis codes, insurance type, age, race, and gender were obtained from administrative data. One‐month follow‐up telephone interviews assessed whether or not patient had any follow‐up appointment or hospital readmissions. Trained abstractors from each site performed manual chart reviews using a standard data collection sheet. The ICD‐9 code designation and chart abstraction methodology were developed prior to the initiation of the study to ensure consistent data collection and reduce bias.
The following data elements were collected: comorbidities, endoscopic findings, inpatient mortality, clinical evidence of rebleeding, endoscopic treatment or gastrointestinal (GI) surgery to control bleeding, repeat EGD, ICU transfer, decompensated comorbid illness requiring continued hospitalization, and blood transfusion (packed red cells, plasma, platelets). Clinical evidence of rebleeding was defined as either hematemesis or melena with decrease in hemoglobin of 2 g in 24 hours with or without hemodynamic compromise.14, 15 For the purpose of this study, recurrent bleeding was defined as clinical evidence of rebleeding, emergency GI surgery for control of UGIH, or repeat EGD before discharge. Furthermore, a composite endpoint termed total complications encompassed all adverse outcomes related to the UGIH hospitalization. The 30‐day readmission variable was defined using readmission identified in administrative records and a 30‐day follow‐up phone call. To guard against recall bias, self‐report data was only included for nonsite admissions.
We defined efficiency in terms of costs and LOS. Total hospital costs were measured using the TSI cost accounting system (Transition Systems, Inc., Boston, MA; now Eclipsys Corporation)16, 17 at 5 out of the 6 participating sites. TSI is a hospital cost accounting software system that integrates resource utilization and financial data already recorded in other hospital databases (such as the billing system, payroll system, and general ledger system).17 Hospital LOS was defined as the number of days from patient admission to the general medicine service until patient discharge.
Provider Specialization: Hospitalists vs. Nonhospitalists
The study was designed as a natural experiment based on a call cycle. The hospitalist‐led teams at each institution alternated in a 4‐day or 5‐day general medicine call cycle with teams led by traditional academic internal medicine attending physicians. All patients were assigned to teams according to their position in the call cycle without regard to whether the attending physician was a hospitalist or a nonhospitalist. Hospitalists are physicians whose primary professional focus is the general medical care of hospitalized patients.18, 19 As previously reported in a related MCH work,11 a hospitalist was also defined as a provider who spends at least 25% of his or her time on an academic inpatient general medicine service. Nonhospitalist physicians were most often outpatient general internal medicine faculty or subspecialists, who attended 1 month per year. Physicians were classified as hospitalists or nonhospitalists according to the designations provided by each site.
UGIH‐specific Confounders
From chart abstraction, we captured severity of illness, comorbidity, and performance of early EGD, variables that can confound analysis in UGIH. To capture severity of illness, a complete Rockall risk score was calculated for each patient. The complete Rockall uses 3 clinical variables (age, shock, and comorbidity) and 2 endoscopic variables (endoscopic diagnosis and stigmata of recent hemorrhage).5, 20 A complete Rockall score of 2 is considered low‐risk for rebleeding or death following admission.21, 22 The accepted definition of low‐risk is <5% recurrent bleeding and <1% mortality risk. A complete Rockall score of 3 to 5 is considered moderate‐risk while 6 is considered high‐risk. Comorbidity was measured using the Charlson comorbidity index.23 Performance of early endoscopy, usually defined as endoscopy performed within 24 hours from presentation, was previously shown to decrease LOS and need for surgical intervention in patients with acute UGIH.24, 25 Documented times of presentation to the emergency department and time of endoscopy performance were collected to calculate for the rate of early endoscopy in our study population.
Statistical Analysis
All statistical analyses were performed using SAS Version 9.1 for Windows (SAS Institute, Cary, NC).
Differences in baseline demographic characteristics of patients and their endoscopic findings were compared between the 2 types of providers. Univariate analyses were also performed to compare the differences in adverse outcomes, LOS, and costs between patients cared for by hospitalists and nonhospitalists. Chi‐square tests were used for categorical variables; while both Wilcoxon rank sum test and Student's t test were used in the analysis of continuous variables.
Next, we performed multivariable analyses to determine the independent association between hospitalist care and the odds of the patients having certain outcomes. However, to prevent overfitting, we only developed regression models for adverse outcomes that have at least 20% event rate.
Multivariable regression models were developed separately for LOS and costs. In contrast with the models on outcomes, analyses of LOS and costs were restricted to: (1) patients who were discharged alive; and (2) to cases with LOS and costs values within 3 standard deviations (SDs) of the mean because of the skewed nature of these data.
All models were adjusted for age, gender, race, insurance type, complete Rockall risk score, performance of early EGD, Charlson comorbidity index, and study site. Final candidate variables in the models were chosen based on stepwise selection, a method very similar to forward selection except that variables selected for the model do not necessarily remain in the model. Effects were entered into and then removed from the model in such a way that each forward selection step can be followed by 1 or more backward elimination steps. The stepwise selection was terminated if no further effect can be added to the model or if the current model was identical to the previous model. The stepwise selection model was generated using statistical criterion of alpha = 0.05 for entry and elimination from the model. Variables that can be a profound source of variation, such as study site and treating physician, were included in the model irrespective of their statistical significance.
To account for clustering of patients treated by the same physician, we used multilevel modeling with SAS PROC GLIMMIX (with random effects). For outcomes (categorical variables), we utilized models with logit‐link and binomial‐distributed errors. As for efficiency (continuous variables with skewed distribution), the multivariable analyses used a generalized linear model with log‐link and assuming gamma‐distributed errors.
Results
Patient Characteristics and Endoscopic Diagnoses
Out of 31,000 patients, the study identified a total of 566 patients (1.8%) with acute UGIH (Table 1). However, 116 patients transferred from another hospital were excluded as their initial management was provided elsewhere, giving a final study sample of 450 patients. Overall, there are 163 admitting physicians from 6 sites, with 39 (24%) classified as hospitalists and 124 (76%) as nonhospitalists. Forty‐two percent (177/450) of patients were cared for by hospitalists. Compared to nonhospitalists, patients admitted to the hospitalist service were older (62.8 vs. 57.7 years, P < 0.01) and with third‐party payor mix differences (P < 0.01). However, there were no statistical differences between patients attended by hospitalists and nonhospitalists with regard to Complete Rockall risk score, Charlson comorbidity index, performance of early endoscopy, and mean hemoglobin values on admission. Upper endoscopy was performed in all patients with distribution of the 3 most common diagnoses being similar (P > 0.05) between hospitalists and nonhospitalists: erosive disease (49.7% vs. 54.6%), peptic ulcer disease (PUD) (48% vs. 46.9%), and varices (18.6% vs. 14.7%).
Variable | Admitting Service | P | |
---|---|---|---|
Hospitalist (n = 177) | Nonhospitalist (n = 273) | ||
| |||
Age, years (meanSD) | 62.817.4 | 57.718.5 | <0.01 |
Male sex, n (%) | 104 (58.8) | 169 (61.9) | 0.50 |
Ethnicity, n (%) | 0.13 | ||
White | 83 (46.9) | 102 (37.4) | |
African‐American | 34 (19.2) | 75 (27.5) | |
Hispanic | 21 (11.9) | 40 (14.7) | |
Asian/Pacific Islander | 24 (13.6) | 29 (10.6) | |
Others/unknown | 15 (8.5) | 27 (9.9) | |
Insurance, n (%) | <0.01 | ||
Medicare | 86 (48.6) | 104 (38.1) | |
Medicaid | 15 (8.5) | 33 (12.1) | |
No payer | 18 (10.2) | 36 (13.2) | |
Private | 46 (26) | 52 (19.1) | |
Unknown | 12 (6.8) | 48 (17.5) | |
Charlson Comorbidity Index (meanSD) | 1.91.6 | 1.81.7 | 0.51 |
Complete Rockall, n (%) | 0.11 | ||
Low‐risk (0‐2) | 82 (46.3) | 103 (37.7) | |
Moderate‐risk (3‐5) | 71 (40.1) | 137 (50.2) | |
High‐risk (6) | 24 (14.6) | 33 (12.1) | |
Early endoscopy (<24 hours) | 82 (46.3) | 133 (48.7) | 0.62 |
Endoscopic diagnosis, n (%)* | |||
Erosive disease | 88 (49.7) | 149 (54.6) | 0.31 |
Peptic ulcer disease | 85 (48.0) | 128 (46.9) | 0.81 |
Varices | 33 (18.6) | 40 (14.7) | 0.26 |
Mallory‐Weiss tear | 9 (5.1) | 21 (7.7) | 0.28 |
Angiodysplasia | 9 (5.1) | 13 (4.8) | 0.88 |
GI mass | 1 (0.6) | 4 (1.5) | 0.65 |
Normal | 7 (4.0) | 8 (2.9) | 0.55 |
Admission hemoglobin values (meanSD) | 10.22.9 | 10.22.9 | 0.78 |
Clinical Outcomes
Between hospitalists and nonhospitalists, unadjusted outcomes were similar (P > 0.05) for mortality (2.3% vs. 0.4%), recurrent bleeding (11% vs. 11%), need for endoscopic therapy (24% vs. 22%), ICU‐transfer and decompensation (15% vs. 15%), as well as an overall composite measure of any complication (79% vs. 72%) (Table 2). However, the hospitalist‐led teams performed more blood transfusions (74% vs. 63%, P = 0.02) and readmission rates were higher (7.3% vs. 3.3%, P = 0.05).
Outcomes, n (%) | Admitting Service | P | |
---|---|---|---|
Hospitalist (n = 177) | Nonhospitalist (n = 273) | ||
| |||
Inpatient mortality | 4 (2.3) | 1 (0.4) | 0.08 |
Recurrent bleeding* | 20 (11.3) | 29 (10.6) | 0.88 |
Endoscopic therapy | 43 (24.3) | 60 (22.0) | 0.57 |
ICU transfers | 23 (13) | 24 (8.8) | 0.20 |
Decompensated comorbidities that required continued hospitalization | 26 (14.7) | 41 (15.0) | 0.92 |
Any transfusion | 131 (74.0) | 172 (63.0) | 0.02 |
Total complications | 139 (78.5) | 196 (71.8) | 0.11 |
30‐day all‐cause readmissions | 13 (7.3) | 9 (3.3) | 0.05 |
Efficiency | Hospitalist (n = 164) | Nonhospitalist (n = 259) | P |
LOS, days | |||
MeanSD | 4.83.5 | 4.53.0 | 0.30 |
Median (interquartile range) | 4 (36) | 4 (26) | 0.69 |
Total costs, U.S. $ | |||
MeanSD | 10,466.669191.00 | 7926.716065.00 | <0.01 |
Median (interquartile range) | 7359.00 (4,698.0012,550.00) | 6181.00 (3744.0010,344.00) | <0.01 |
Because of the low event rate of certain adverse outcomes (<20%), we were only able to perform adjusted analyses on 4 outcomes: need for endoscopic therapy (odds ratio [OR], 0.82; 95% confidence interval [CI], 0.491.37), ICU transfer and decompensation (OR, 0.82; 95% CI, 0.451.52), blood transfusion (OR, 1.30; 95% CI, 0.822.04), and any complication (OR, 1.18; 95% CI, 0.711.96). Since outcome differences disappeared after controlling for confounders, the data suggest that overall care provided by hospitalists and nonhospitalists might be equivalenteven in certain outcomes that we were unable to substantiate using multivariable methods.
Efficiency
Efficiency, as measured by LOS and costs, are presented both as means and medians in univariate analyses in Table 2. Median LOS was similar for hospitalist‐led and nonhospitalist‐led teams (4 days). Despite having similar LOS, the median costs of acute UGIH in patients cared for by hospitalists were higher ($7,359.00 vs. $6,181.00; P < 0.01).
After adjusting for demographic factors, Rockall risk score, comorbidity, early EGD, and hospital site, LOS remained similar between the 2 groups. On the other hand, the adjusted cost for UGIH patients cared for by hospitalists and nonhospitalists persisted, with hospitalist care costs $1,502.40 more than their nonhospitalist counterparts (Table 3).
Efficiency | Treatment Provider | P | |
---|---|---|---|
Hospitalist (n = 164) | Nonhospitalist (n = 259) | ||
| |||
Adjusted length of stay, days (mean SD) | 5.2 (4.95.6) | 4.7 (4.55.0) | 0.15 |
Adjusted total cost, U.S. $ (mean SD) | 9006.50 (8366.609693.60) | 7504.10 (7069.907964.20) | 0.03 |
Discussion
This is the first study that has looked at the effect of hospitalists on clinical outcomes and efficiency in patients admitted for acute UGIH, a condition highly dependent upon another specialty for procedures and management. This is also one of only a few studies on UGIH that adjusted for severity of illness (Rockall score), comorbidity, performance of early endoscopypatient‐level confounders usually unaccounted for in prior research.
We show that hospitalists and nonhospitalists caring for acute UGIH patients had overall similar unadjusted outcomes; except for blood transfusion and 30‐day readmission rates. Unfortunately, due to the small number of events for readmissions, we were unable to perform adjusted analysis for readmission. Differences between hospitalists and nonhospitalists on blood transfusion rates were not substantiated on multivariable adjustments.
As for efficiency, univariable and multivariable analyses revealed that LOS was similar between provider types while costs were greater in UGIH patients attended by hospitalists.
Reductions in resource use, particularly costs, may be achieved by increasing throughput (eg, reducing LOS) or by decreasing service intensity (eg, using fewer ancillary services and specialty consultations).26 Specifically in acute UGIH, LOS is significantly affected by performance of early EGD.27, 28 In these studies, gastroenterologist‐led teams, compared to internists and surgeons, have easier access to endoscopy, thus reducing LOS and overall costs.27, 28
Similarly, prior studies have shown that the mechanism by which hospitalists lower costs is by decreasing LOS.810, 29 There are several hypotheses on how hospitalists affect LOS. Hospitalists, by being available all day, are thought to respond quickly to acute symptoms or new test results, are more efficient in navigating the complex hospital environment, or develop greater expertise as a result of added inpatient experience.8 On the downside, although the hospitalist model reduces overall LOS and costs, they also provide higher intensity of care as reflected by greater costs when broken down per hospital day.29 Thus, the cost differential we found may represent higher intensity of care by hospitalists in their management of acute UGIH, as higher intensity care without decreasing LOS can translate to higher costs.
In addition, patients with acute UGIH are unique in several respects. In contrast to diseases like heart failure, COPD, and pneumonia, in which the admitting provider has the option to request a subspecialist consultation, all patients with acute UGIH need a gastroenterologist to perform endoscopy as part of the management. These patients are usually admitted to general medicine wards, aggressively resuscitated with intravenous fluids, with a nonurgent gastroenterology consult or EGD performed on the next available schedule.
Aside from LOS being greatly affected by performance of early EGD and/or delay in consulting gastroenterology, sicker patients require longer hospitalization and drive LOS and healthcare costs up. It was therefore crucial that we accounted for severity of illness, comorbidity, and performance of early EGD in our regression models for LOS and costs. This approach allows us to acquire a more accurate estimate on the effects of hospitalist on LOS and costs in patients admitted with acute UGIH.
Our findings suggest that the academic hospitalist model of care may not have as great of an impact on hospital efficiency in certain patient groups that require nonurgent subspecialty consultations. Future studies should focus on elucidating these relationships.
Limitations
This study has several limitations. First, clinical data were abstracted at 6 sites by different abstractors so it is possible there were variations in how data were collected. To reduce variation, a standardized abstraction form with instructions was developed and the primary investigator (PI) was available for specific questions during the abstraction process. Second, only 5 out of the 6 sites used TSI accounting systems. Although similar, interhospital costs captured by TSI may vary among sites in terms of classifying direct and indirect costs, potentially resulting in misclassification bias in our cost estimates.17 We addressed these issues by including the hospital site variable in our regression models, regardless of its significance. Third, consent rates across sites vary from 70% to 85%. It is possible that patients who refused enrollment in the MCH trial are systematically different and may introduce bias in our analysis.
Furthermore, the study was designed as a natural experiment based on a rotational call cycle between hospitalist‐led and nonhospitalist‐led teams. It is possible that the order of patient assignment might not be completely naturally random as we intended. However, the study period was for 2 years and we expect the effect of order would have averaged out in time.
There are many hospitalist models of care. In terms of generalizability, the study pertains only to academic hospitalists and may not be applicable to hospitalists practicing in community hospitals. For example, the nonhospitalist comparison group is likely different in the community and academic settings. Community nonhospitalists (traditional practitioners) are usually internists covering both inpatient and outpatient responsibilities at the same time. In contrast, academic nonhospitalists are internists or subspecialists serving as ward attendings for a limited period (usually 1 month) with considerable variation in their nonattending responsibilities (eg, research, clinic, administration). Furthermore, academic nonhospitalist providers might be a self‐selected group by their willingness to serve as a ward attending, making them more hospitalist‐like. Changes and variability of inpatient attendings may also affect our findings when compared to prior work. Finally, it is also possible that having residents at academic medical centers may attenuate the effect of hospitalists more than in community‐based models.
Conclusions/Implications
Compared to nonhospitalists, academic hospitalist care of acute UGIH patients had similar overall clinical outcomes. However, our finding of similar LOS yet higher costs for patients cared for by hospitalists support 1 proposed mechanism in which hospitalists decrease healthcare costs: providing higher intensity of care per day of hospitalization. However, in academic hospitalist models, this higher intensity hypothesis should be revisited, especially in certain patient groups in which timing and involvement of subspecialists may influence discharge decisions, affecting LOS and overall costs.
Due to inherent limitations in this observational study, future studies should focus on verifying and elucidating these relationships further. Lastly, understanding which patient groups receive the greatest potential benefit from this model will help guide both organizational efforts and quality improvement strategies.
Acute upper gastrointestinal hemorrhage (UGIH) is one of the most common hospital admissions for acute care. Estimates indicate that 300,000 patients (100‐150 cases per 100,000 adults) are admitted annually with an associated economic impact of $2.5 billion.15 The current standard management of UGIH requires hospital admission and esophagogastroduodenoscopy (EGD) by a gastroenterologist for diagnosis and/or treatment. This management strategy results in a high consumption of hospital resources and costs.
Simultaneously, hospitalists have dramatically changed the delivery of inpatient care in the United States and are recognized as a location‐driven subspecialty for the care of acute hospitalized patients, similar to emergency medicine. Currently there are 20,000 hospitalists, and more than one‐third of general medicine inpatients are cared for by hospitalists.6, 7
Previous studies have shown that hospitalist care offers better or comparable outcomes, with lower overall length of stay (LOS) and costs compared to traditional providers.810 However, most of these studies were performed in single institutions, had weak designs or little‐to‐no adjustment for severity of illness, or were limited to 7 specific diseases (pneumonia, congestive heart failure [CHF], chest pain, ischemic stroke, urinary tract infection, chronic obstructive lung disease [COPD], and acute myocardial infarction [AMI]).8
Furthermore, less is known about the effect of hospitalists on conditions that may be dependent upon specialist consultation for procedures and/or treatment plans. In this study, gastroenterologists performed diagnostic and/or therapeutic endoscopy work as consultants to the attending physicians in the management of acute inpatient UGIH.
To explore the effects of hospitalists on care of patients with acute UGIH, we examined data from the Multicenter Hospitalist (MCH) trial. The objectives of our study were to compare clinical outcomesin‐hospital mortality and complications (ie, recurrent bleeding, intensive care unit [ICU] transfer, decompensation, transfusion, reendoscopy, 30‐day readmission)and efficiency (LOS and costs) in hospitalized acute UGIH patients cared for by hospitalists and nonhospitalists in 6 academic centers in the United States during a 2‐year period.
Patients and Methods
Study Sites
From July 1, 2001 to June 30, 2003, the MCH trial1113 was a prospective, multicenter, observational trial of the care provided by hospitalists to patients admitted to general medical services at 6 academic medical institutions. There were 31,000 consecutive admissions to the general medical services of these participating sites: University of Chicago (Chicago, IL), University of Wisconsin Hospital (Madison, WI), University of Iowa (Iowa City, IA), University of California at San Francisco (San Francisco, CA), University of New Mexico (Albuquerque, NM), and Brigham and Women's Hospital (Boston, MA). The study was approved by the institutional review boards (IRBs) at each of the 6 participating institutions.
MCH Study Patients
Patients were eligible if they were admitted to the general medical services under the care of a hospitalist or nonhospitalist physician. Regardless of the admitting provider, each medical service was composed of rotating senior and junior resident physicians in all 6 sites. Furthermore, patients were 18 years of age or older, and were able to give consent themselves or had an appropriate proxy. Patients with mini‐mental status score of 17 (out of 22), admitted under their primary care physician or to an inpatient gastroenterology service, or transferred from another hospital, were excluded. The MCH study was designed to study the outcomes and efficiency in patients admitted for CHF, pneumonia, UGIH, and end‐of‐life care.
Acute UGIH Patients
Within the MCH‐eligible patients, we identified those with acute UGIH using the following International Classification of Diseases, 9th edition (ICD‐9) codes assigned at discharge: esophageal varices with hemorrhage (456.0, 456.20); Mallory‐Weiss syndrome (530.7); gastric ulcer with hemorrhage (531.00531.61); duodenal ulcer with hemorrhage (532.00532.61); peptic ulcer, site unspecified, with hemorrhage (533.00533.61); gastrojejunal ulcer with hemorrhage (534.00534.61); gastritis with hemorrhage (535.61); angiodysplasia of stomach/duodenum with hemorrhage (537.83); and hematemesis (578.0, 578.9). We also confirmed the diagnosis of UGIH by reviewing patient medical records for observed hematemesis, nasogastric tube aspirate with gross or hemoccult blood, or clinical history of hematemesis, melena, or hematochezia.14, 15
Data
All data were obtained from the 6 hospitals' administrative records, patient interviews, and medical chart abstractions. Dates of admission and discharge, ICD‐9 diagnosis codes, insurance type, age, race, and gender were obtained from administrative data. One‐month follow‐up telephone interviews assessed whether or not patient had any follow‐up appointment or hospital readmissions. Trained abstractors from each site performed manual chart reviews using a standard data collection sheet. The ICD‐9 code designation and chart abstraction methodology were developed prior to the initiation of the study to ensure consistent data collection and reduce bias.
The following data elements were collected: comorbidities, endoscopic findings, inpatient mortality, clinical evidence of rebleeding, endoscopic treatment or gastrointestinal (GI) surgery to control bleeding, repeat EGD, ICU transfer, decompensated comorbid illness requiring continued hospitalization, and blood transfusion (packed red cells, plasma, platelets). Clinical evidence of rebleeding was defined as either hematemesis or melena with decrease in hemoglobin of 2 g in 24 hours with or without hemodynamic compromise.14, 15 For the purpose of this study, recurrent bleeding was defined as clinical evidence of rebleeding, emergency GI surgery for control of UGIH, or repeat EGD before discharge. Furthermore, a composite endpoint termed total complications encompassed all adverse outcomes related to the UGIH hospitalization. The 30‐day readmission variable was defined using readmission identified in administrative records and a 30‐day follow‐up phone call. To guard against recall bias, self‐report data was only included for nonsite admissions.
We defined efficiency in terms of costs and LOS. Total hospital costs were measured using the TSI cost accounting system (Transition Systems, Inc., Boston, MA; now Eclipsys Corporation)16, 17 at 5 out of the 6 participating sites. TSI is a hospital cost accounting software system that integrates resource utilization and financial data already recorded in other hospital databases (such as the billing system, payroll system, and general ledger system).17 Hospital LOS was defined as the number of days from patient admission to the general medicine service until patient discharge.
Provider Specialization: Hospitalists vs. Nonhospitalists
The study was designed as a natural experiment based on a call cycle. The hospitalist‐led teams at each institution alternated in a 4‐day or 5‐day general medicine call cycle with teams led by traditional academic internal medicine attending physicians. All patients were assigned to teams according to their position in the call cycle without regard to whether the attending physician was a hospitalist or a nonhospitalist. Hospitalists are physicians whose primary professional focus is the general medical care of hospitalized patients.18, 19 As previously reported in a related MCH work,11 a hospitalist was also defined as a provider who spends at least 25% of his or her time on an academic inpatient general medicine service. Nonhospitalist physicians were most often outpatient general internal medicine faculty or subspecialists, who attended 1 month per year. Physicians were classified as hospitalists or nonhospitalists according to the designations provided by each site.
UGIH‐specific Confounders
From chart abstraction, we captured severity of illness, comorbidity, and performance of early EGD, variables that can confound analysis in UGIH. To capture severity of illness, a complete Rockall risk score was calculated for each patient. The complete Rockall uses 3 clinical variables (age, shock, and comorbidity) and 2 endoscopic variables (endoscopic diagnosis and stigmata of recent hemorrhage).5, 20 A complete Rockall score of 2 is considered low‐risk for rebleeding or death following admission.21, 22 The accepted definition of low‐risk is <5% recurrent bleeding and <1% mortality risk. A complete Rockall score of 3 to 5 is considered moderate‐risk while 6 is considered high‐risk. Comorbidity was measured using the Charlson comorbidity index.23 Performance of early endoscopy, usually defined as endoscopy performed within 24 hours from presentation, was previously shown to decrease LOS and need for surgical intervention in patients with acute UGIH.24, 25 Documented times of presentation to the emergency department and time of endoscopy performance were collected to calculate for the rate of early endoscopy in our study population.
Statistical Analysis
All statistical analyses were performed using SAS Version 9.1 for Windows (SAS Institute, Cary, NC).
Differences in baseline demographic characteristics of patients and their endoscopic findings were compared between the 2 types of providers. Univariate analyses were also performed to compare the differences in adverse outcomes, LOS, and costs between patients cared for by hospitalists and nonhospitalists. Chi‐square tests were used for categorical variables; while both Wilcoxon rank sum test and Student's t test were used in the analysis of continuous variables.
Next, we performed multivariable analyses to determine the independent association between hospitalist care and the odds of the patients having certain outcomes. However, to prevent overfitting, we only developed regression models for adverse outcomes that have at least 20% event rate.
Multivariable regression models were developed separately for LOS and costs. In contrast with the models on outcomes, analyses of LOS and costs were restricted to: (1) patients who were discharged alive; and (2) to cases with LOS and costs values within 3 standard deviations (SDs) of the mean because of the skewed nature of these data.
All models were adjusted for age, gender, race, insurance type, complete Rockall risk score, performance of early EGD, Charlson comorbidity index, and study site. Final candidate variables in the models were chosen based on stepwise selection, a method very similar to forward selection except that variables selected for the model do not necessarily remain in the model. Effects were entered into and then removed from the model in such a way that each forward selection step can be followed by 1 or more backward elimination steps. The stepwise selection was terminated if no further effect can be added to the model or if the current model was identical to the previous model. The stepwise selection model was generated using statistical criterion of alpha = 0.05 for entry and elimination from the model. Variables that can be a profound source of variation, such as study site and treating physician, were included in the model irrespective of their statistical significance.
To account for clustering of patients treated by the same physician, we used multilevel modeling with SAS PROC GLIMMIX (with random effects). For outcomes (categorical variables), we utilized models with logit‐link and binomial‐distributed errors. As for efficiency (continuous variables with skewed distribution), the multivariable analyses used a generalized linear model with log‐link and assuming gamma‐distributed errors.
Results
Patient Characteristics and Endoscopic Diagnoses
Out of 31,000 patients, the study identified a total of 566 patients (1.8%) with acute UGIH (Table 1). However, 116 patients transferred from another hospital were excluded as their initial management was provided elsewhere, giving a final study sample of 450 patients. Overall, there are 163 admitting physicians from 6 sites, with 39 (24%) classified as hospitalists and 124 (76%) as nonhospitalists. Forty‐two percent (177/450) of patients were cared for by hospitalists. Compared to nonhospitalists, patients admitted to the hospitalist service were older (62.8 vs. 57.7 years, P < 0.01) and with third‐party payor mix differences (P < 0.01). However, there were no statistical differences between patients attended by hospitalists and nonhospitalists with regard to Complete Rockall risk score, Charlson comorbidity index, performance of early endoscopy, and mean hemoglobin values on admission. Upper endoscopy was performed in all patients with distribution of the 3 most common diagnoses being similar (P > 0.05) between hospitalists and nonhospitalists: erosive disease (49.7% vs. 54.6%), peptic ulcer disease (PUD) (48% vs. 46.9%), and varices (18.6% vs. 14.7%).
Variable | Admitting Service | P | |
---|---|---|---|
Hospitalist (n = 177) | Nonhospitalist (n = 273) | ||
| |||
Age, years (meanSD) | 62.817.4 | 57.718.5 | <0.01 |
Male sex, n (%) | 104 (58.8) | 169 (61.9) | 0.50 |
Ethnicity, n (%) | 0.13 | ||
White | 83 (46.9) | 102 (37.4) | |
African‐American | 34 (19.2) | 75 (27.5) | |
Hispanic | 21 (11.9) | 40 (14.7) | |
Asian/Pacific Islander | 24 (13.6) | 29 (10.6) | |
Others/unknown | 15 (8.5) | 27 (9.9) | |
Insurance, n (%) | <0.01 | ||
Medicare | 86 (48.6) | 104 (38.1) | |
Medicaid | 15 (8.5) | 33 (12.1) | |
No payer | 18 (10.2) | 36 (13.2) | |
Private | 46 (26) | 52 (19.1) | |
Unknown | 12 (6.8) | 48 (17.5) | |
Charlson Comorbidity Index (meanSD) | 1.91.6 | 1.81.7 | 0.51 |
Complete Rockall, n (%) | 0.11 | ||
Low‐risk (0‐2) | 82 (46.3) | 103 (37.7) | |
Moderate‐risk (3‐5) | 71 (40.1) | 137 (50.2) | |
High‐risk (6) | 24 (14.6) | 33 (12.1) | |
Early endoscopy (<24 hours) | 82 (46.3) | 133 (48.7) | 0.62 |
Endoscopic diagnosis, n (%)* | |||
Erosive disease | 88 (49.7) | 149 (54.6) | 0.31 |
Peptic ulcer disease | 85 (48.0) | 128 (46.9) | 0.81 |
Varices | 33 (18.6) | 40 (14.7) | 0.26 |
Mallory‐Weiss tear | 9 (5.1) | 21 (7.7) | 0.28 |
Angiodysplasia | 9 (5.1) | 13 (4.8) | 0.88 |
GI mass | 1 (0.6) | 4 (1.5) | 0.65 |
Normal | 7 (4.0) | 8 (2.9) | 0.55 |
Admission hemoglobin values (meanSD) | 10.22.9 | 10.22.9 | 0.78 |
Clinical Outcomes
Between hospitalists and nonhospitalists, unadjusted outcomes were similar (P > 0.05) for mortality (2.3% vs. 0.4%), recurrent bleeding (11% vs. 11%), need for endoscopic therapy (24% vs. 22%), ICU‐transfer and decompensation (15% vs. 15%), as well as an overall composite measure of any complication (79% vs. 72%) (Table 2). However, the hospitalist‐led teams performed more blood transfusions (74% vs. 63%, P = 0.02) and readmission rates were higher (7.3% vs. 3.3%, P = 0.05).
Outcomes, n (%) | Admitting Service | P | |
---|---|---|---|
Hospitalist (n = 177) | Nonhospitalist (n = 273) | ||
| |||
Inpatient mortality | 4 (2.3) | 1 (0.4) | 0.08 |
Recurrent bleeding* | 20 (11.3) | 29 (10.6) | 0.88 |
Endoscopic therapy | 43 (24.3) | 60 (22.0) | 0.57 |
ICU transfers | 23 (13) | 24 (8.8) | 0.20 |
Decompensated comorbidities that required continued hospitalization | 26 (14.7) | 41 (15.0) | 0.92 |
Any transfusion | 131 (74.0) | 172 (63.0) | 0.02 |
Total complications | 139 (78.5) | 196 (71.8) | 0.11 |
30‐day all‐cause readmissions | 13 (7.3) | 9 (3.3) | 0.05 |
Efficiency | Hospitalist (n = 164) | Nonhospitalist (n = 259) | P |
LOS, days | |||
MeanSD | 4.83.5 | 4.53.0 | 0.30 |
Median (interquartile range) | 4 (36) | 4 (26) | 0.69 |
Total costs, U.S. $ | |||
MeanSD | 10,466.669191.00 | 7926.716065.00 | <0.01 |
Median (interquartile range) | 7359.00 (4,698.0012,550.00) | 6181.00 (3744.0010,344.00) | <0.01 |
Because of the low event rate of certain adverse outcomes (<20%), we were only able to perform adjusted analyses on 4 outcomes: need for endoscopic therapy (odds ratio [OR], 0.82; 95% confidence interval [CI], 0.491.37), ICU transfer and decompensation (OR, 0.82; 95% CI, 0.451.52), blood transfusion (OR, 1.30; 95% CI, 0.822.04), and any complication (OR, 1.18; 95% CI, 0.711.96). Since outcome differences disappeared after controlling for confounders, the data suggest that overall care provided by hospitalists and nonhospitalists might be equivalenteven in certain outcomes that we were unable to substantiate using multivariable methods.
Efficiency
Efficiency, as measured by LOS and costs, are presented both as means and medians in univariate analyses in Table 2. Median LOS was similar for hospitalist‐led and nonhospitalist‐led teams (4 days). Despite having similar LOS, the median costs of acute UGIH in patients cared for by hospitalists were higher ($7,359.00 vs. $6,181.00; P < 0.01).
After adjusting for demographic factors, Rockall risk score, comorbidity, early EGD, and hospital site, LOS remained similar between the 2 groups. On the other hand, the adjusted cost for UGIH patients cared for by hospitalists and nonhospitalists persisted, with hospitalist care costs $1,502.40 more than their nonhospitalist counterparts (Table 3).
Efficiency | Treatment Provider | P | |
---|---|---|---|
Hospitalist (n = 164) | Nonhospitalist (n = 259) | ||
| |||
Adjusted length of stay, days (mean SD) | 5.2 (4.95.6) | 4.7 (4.55.0) | 0.15 |
Adjusted total cost, U.S. $ (mean SD) | 9006.50 (8366.609693.60) | 7504.10 (7069.907964.20) | 0.03 |
Discussion
This is the first study that has looked at the effect of hospitalists on clinical outcomes and efficiency in patients admitted for acute UGIH, a condition highly dependent upon another specialty for procedures and management. This is also one of only a few studies on UGIH that adjusted for severity of illness (Rockall score), comorbidity, performance of early endoscopypatient‐level confounders usually unaccounted for in prior research.
We show that hospitalists and nonhospitalists caring for acute UGIH patients had overall similar unadjusted outcomes; except for blood transfusion and 30‐day readmission rates. Unfortunately, due to the small number of events for readmissions, we were unable to perform adjusted analysis for readmission. Differences between hospitalists and nonhospitalists on blood transfusion rates were not substantiated on multivariable adjustments.
As for efficiency, univariable and multivariable analyses revealed that LOS was similar between provider types while costs were greater in UGIH patients attended by hospitalists.
Reductions in resource use, particularly costs, may be achieved by increasing throughput (eg, reducing LOS) or by decreasing service intensity (eg, using fewer ancillary services and specialty consultations).26 Specifically in acute UGIH, LOS is significantly affected by performance of early EGD.27, 28 In these studies, gastroenterologist‐led teams, compared to internists and surgeons, have easier access to endoscopy, thus reducing LOS and overall costs.27, 28
Similarly, prior studies have shown that the mechanism by which hospitalists lower costs is by decreasing LOS.810, 29 There are several hypotheses on how hospitalists affect LOS. Hospitalists, by being available all day, are thought to respond quickly to acute symptoms or new test results, are more efficient in navigating the complex hospital environment, or develop greater expertise as a result of added inpatient experience.8 On the downside, although the hospitalist model reduces overall LOS and costs, they also provide higher intensity of care as reflected by greater costs when broken down per hospital day.29 Thus, the cost differential we found may represent higher intensity of care by hospitalists in their management of acute UGIH, as higher intensity care without decreasing LOS can translate to higher costs.
In addition, patients with acute UGIH are unique in several respects. In contrast to diseases like heart failure, COPD, and pneumonia, in which the admitting provider has the option to request a subspecialist consultation, all patients with acute UGIH need a gastroenterologist to perform endoscopy as part of the management. These patients are usually admitted to general medicine wards, aggressively resuscitated with intravenous fluids, with a nonurgent gastroenterology consult or EGD performed on the next available schedule.
Aside from LOS being greatly affected by performance of early EGD and/or delay in consulting gastroenterology, sicker patients require longer hospitalization and drive LOS and healthcare costs up. It was therefore crucial that we accounted for severity of illness, comorbidity, and performance of early EGD in our regression models for LOS and costs. This approach allows us to acquire a more accurate estimate on the effects of hospitalist on LOS and costs in patients admitted with acute UGIH.
Our findings suggest that the academic hospitalist model of care may not have as great of an impact on hospital efficiency in certain patient groups that require nonurgent subspecialty consultations. Future studies should focus on elucidating these relationships.
Limitations
This study has several limitations. First, clinical data were abstracted at 6 sites by different abstractors so it is possible there were variations in how data were collected. To reduce variation, a standardized abstraction form with instructions was developed and the primary investigator (PI) was available for specific questions during the abstraction process. Second, only 5 out of the 6 sites used TSI accounting systems. Although similar, interhospital costs captured by TSI may vary among sites in terms of classifying direct and indirect costs, potentially resulting in misclassification bias in our cost estimates.17 We addressed these issues by including the hospital site variable in our regression models, regardless of its significance. Third, consent rates across sites vary from 70% to 85%. It is possible that patients who refused enrollment in the MCH trial are systematically different and may introduce bias in our analysis.
Furthermore, the study was designed as a natural experiment based on a rotational call cycle between hospitalist‐led and nonhospitalist‐led teams. It is possible that the order of patient assignment might not be completely naturally random as we intended. However, the study period was for 2 years and we expect the effect of order would have averaged out in time.
There are many hospitalist models of care. In terms of generalizability, the study pertains only to academic hospitalists and may not be applicable to hospitalists practicing in community hospitals. For example, the nonhospitalist comparison group is likely different in the community and academic settings. Community nonhospitalists (traditional practitioners) are usually internists covering both inpatient and outpatient responsibilities at the same time. In contrast, academic nonhospitalists are internists or subspecialists serving as ward attendings for a limited period (usually 1 month) with considerable variation in their nonattending responsibilities (eg, research, clinic, administration). Furthermore, academic nonhospitalist providers might be a self‐selected group by their willingness to serve as a ward attending, making them more hospitalist‐like. Changes and variability of inpatient attendings may also affect our findings when compared to prior work. Finally, it is also possible that having residents at academic medical centers may attenuate the effect of hospitalists more than in community‐based models.
Conclusions/Implications
Compared to nonhospitalists, academic hospitalist care of acute UGIH patients had similar overall clinical outcomes. However, our finding of similar LOS yet higher costs for patients cared for by hospitalists support 1 proposed mechanism in which hospitalists decrease healthcare costs: providing higher intensity of care per day of hospitalization. However, in academic hospitalist models, this higher intensity hypothesis should be revisited, especially in certain patient groups in which timing and involvement of subspecialists may influence discharge decisions, affecting LOS and overall costs.
Due to inherent limitations in this observational study, future studies should focus on verifying and elucidating these relationships further. Lastly, understanding which patient groups receive the greatest potential benefit from this model will help guide both organizational efforts and quality improvement strategies.
- Bleeding peptic ulcer.N Engl J Med.1994;331(11):717–727. , .
- Epidemiology of hospitalization for acute upper gastrointestinal hemorrhage: a population‐based study.Am J Gastroenterol.1995;90(2):206–210. .
- Variation in outcome after acute upper gastrointestinal haemorrhage. the national audit of acute upper gastrointestinal haemorrhage.Lancet.1995;346(8971):346–350. , , , et al.
- Influencing the practice and outcome in acute upper gastrointestinal haemorrhage. Steering committee of the National Audit of Acute Upper Gastrointestinal Haemorrhage.Gut.1997;41(5):606–611. , , , et al.
- Risk assessment after acute upper gastrointestinal haemorrhage.Gut.1996;38(3):316–321. , , , et al.
- The potential size of the hospitalist workforce in the united states.Am J Med.1999;106(4):441–445. , , , et al.
- Society of Hospital Medicine. About SHM. Available at: http://www.hospitalmedicine.org/AM/Template.cfm?Section=General_Information357(25):2589–2600.
- Effects of physician experience on costs and outcomes on an academic general medicine service: results of a trial of hospitalists.Ann Intern Med.2002;137(11):866–874. , , , et al.
- A systematic review of outcomes and quality measures in adult patients cared for by hospitalists vs nonhospitalists.Mayo Clin Proc.2009;84(3):248–254. .
- Do hospitalists or physicians with greater inpatient HIV experience improve HIV care in the era of highly active antiretroviral therapy? Results from a multicenter trial of academic hospitalists.Clin Infect Dis.2008;46(7):1085–1092. , , , et al.
- Quality of care for decompensated heart failure: comparable performance between academic hospitalists and non‐hospitalists. J Gen Intern Med.2008;23(9):1399–1406. , , , et al.
- Factors associated with discussion of care plans and code status at the time of hospital admission: results from the Multicenter Hospitalist Study.J Hosp Med.2008;3(6):437–445. , , , et al.
- Upper gastrointestinal hemorrhage clinical guideline determining the optimal hospital length of stay.Am J Med.1996;100(3):313–322. , , , et al.
- Prospective evaluation of a clinical guideline recommending hospital length of stay in upper gastrointestinal tract hemorrhage.JAMA.1997;278(24):2151–2156. , , , et al.
- In‐hospital cost of abdominal aortic aneurysm repair in Canada and the United States.Arch Intern Med.2003;163(20):2500–2504. , , , et al.
- The use of transition cost accounting system in health services research.Cost Eff Resour Alloc.2007;5:11. , , , et al.
- Society of Hospital Medicine. Definition of a Hospitalist. Available at: http://www.hospitalmedicine.org/AM/Template.cfm?Section=General_Information335(7):514–517.
- Selection of patients for early discharge or outpatient care after acute upper gastrointestinal haemorrhage. National Audit of Acute Upper Gastrointestinal Haemorrhage.Lancet.1996;347(9009):1138–1140. , , , et al.
- Utilization of health care resources for low‐risk patients with acute, nonvariceal upper GI hemorrhage: an historical cohort study.Gastrointest Endosc.2002;55(3):321–327. , , , et al.
- Incremental value of upper endoscopy for triage of patients with acute non‐variceal upper‐GI hemorrhage.Gastrointest Endosc.2004;60(1):9–14. , .
- The Charlson comorbidity index is adapted to predict costs of chronic disease in primary care patients.J Clin Epidemiol.2008;61(12):1234–1240. , , , et al.
- The effectiveness of early endoscopy for upper gastrointestinal hemorrhage: a community‐based analysis.Med Care.1998;36(4):462–474. , , , et al.
- Early endoscopy in upper gastrointestinal hemorrhage: associations with recurrent bleeding, surgery, and length of hospital stay.Gastrointest Endosc.1999;49(2):145–152. , , , et al.
- The impact of hospitalists on the cost and quality of inpatient care in the united states: a research synthesis.Med Care Res Rev.2005;62(4):379–406. , .
- Physician specialty and variations in the cost of treating patients with acute upper gastrointestinal bleeding.Gastroenterology.1997;113(5):1443–1448. , , , et al.
- Impact of physician specialty on the cost of nonvariceal upper GI bleeding care.Am J Gastroenterol.2002;97(6):1535–1542. , , , et al.
- Associations with reduced length of stay and costs on an academic hospitalist service.Am J Manag Care.2004;10(8):561–568. , , .
- Bleeding peptic ulcer.N Engl J Med.1994;331(11):717–727. , .
- Epidemiology of hospitalization for acute upper gastrointestinal hemorrhage: a population‐based study.Am J Gastroenterol.1995;90(2):206–210. .
- Variation in outcome after acute upper gastrointestinal haemorrhage. the national audit of acute upper gastrointestinal haemorrhage.Lancet.1995;346(8971):346–350. , , , et al.
- Influencing the practice and outcome in acute upper gastrointestinal haemorrhage. Steering committee of the National Audit of Acute Upper Gastrointestinal Haemorrhage.Gut.1997;41(5):606–611. , , , et al.
- Risk assessment after acute upper gastrointestinal haemorrhage.Gut.1996;38(3):316–321. , , , et al.
- The potential size of the hospitalist workforce in the united states.Am J Med.1999;106(4):441–445. , , , et al.
- Society of Hospital Medicine. About SHM. Available at: http://www.hospitalmedicine.org/AM/Template.cfm?Section=General_Information357(25):2589–2600.
- Effects of physician experience on costs and outcomes on an academic general medicine service: results of a trial of hospitalists.Ann Intern Med.2002;137(11):866–874. , , , et al.
- A systematic review of outcomes and quality measures in adult patients cared for by hospitalists vs nonhospitalists.Mayo Clin Proc.2009;84(3):248–254. .
- Do hospitalists or physicians with greater inpatient HIV experience improve HIV care in the era of highly active antiretroviral therapy? Results from a multicenter trial of academic hospitalists.Clin Infect Dis.2008;46(7):1085–1092. , , , et al.
- Quality of care for decompensated heart failure: comparable performance between academic hospitalists and non‐hospitalists. J Gen Intern Med.2008;23(9):1399–1406. , , , et al.
- Factors associated with discussion of care plans and code status at the time of hospital admission: results from the Multicenter Hospitalist Study.J Hosp Med.2008;3(6):437–445. , , , et al.
- Upper gastrointestinal hemorrhage clinical guideline determining the optimal hospital length of stay.Am J Med.1996;100(3):313–322. , , , et al.
- Prospective evaluation of a clinical guideline recommending hospital length of stay in upper gastrointestinal tract hemorrhage.JAMA.1997;278(24):2151–2156. , , , et al.
- In‐hospital cost of abdominal aortic aneurysm repair in Canada and the United States.Arch Intern Med.2003;163(20):2500–2504. , , , et al.
- The use of transition cost accounting system in health services research.Cost Eff Resour Alloc.2007;5:11. , , , et al.
- Society of Hospital Medicine. Definition of a Hospitalist. Available at: http://www.hospitalmedicine.org/AM/Template.cfm?Section=General_Information335(7):514–517.
- Selection of patients for early discharge or outpatient care after acute upper gastrointestinal haemorrhage. National Audit of Acute Upper Gastrointestinal Haemorrhage.Lancet.1996;347(9009):1138–1140. , , , et al.
- Utilization of health care resources for low‐risk patients with acute, nonvariceal upper GI hemorrhage: an historical cohort study.Gastrointest Endosc.2002;55(3):321–327. , , , et al.
- Incremental value of upper endoscopy for triage of patients with acute non‐variceal upper‐GI hemorrhage.Gastrointest Endosc.2004;60(1):9–14. , .
- The Charlson comorbidity index is adapted to predict costs of chronic disease in primary care patients.J Clin Epidemiol.2008;61(12):1234–1240. , , , et al.
- The effectiveness of early endoscopy for upper gastrointestinal hemorrhage: a community‐based analysis.Med Care.1998;36(4):462–474. , , , et al.
- Early endoscopy in upper gastrointestinal hemorrhage: associations with recurrent bleeding, surgery, and length of hospital stay.Gastrointest Endosc.1999;49(2):145–152. , , , et al.
- The impact of hospitalists on the cost and quality of inpatient care in the united states: a research synthesis.Med Care Res Rev.2005;62(4):379–406. , .
- Physician specialty and variations in the cost of treating patients with acute upper gastrointestinal bleeding.Gastroenterology.1997;113(5):1443–1448. , , , et al.
- Impact of physician specialty on the cost of nonvariceal upper GI bleeding care.Am J Gastroenterol.2002;97(6):1535–1542. , , , et al.
- Associations with reduced length of stay and costs on an academic hospitalist service.Am J Manag Care.2004;10(8):561–568. , , .
Copyright © 2010 Society of Hospital Medicine
Academic Hospital Medicine
The past decade has seen hospital medicine grow from fewer than 1000 hospitalists nationwide to more than 20,000.1 In fact, survey data suggest that hospital medicine is the fastest growing field of internal medicine in the history of the US, and the growth of hospital medicine has produced a net increase in the number of generalists in the US.2
Although few direct estimates exist, academic hospital medicine (AHM) is also growing rapidly.1 Fueled by potential efficiency gains, a need for increased educational oversight of teaching services, and new residency work hour limitations, many academic medical centers and teaching hospitals have developed large hospital medicine programs. Internal medicine residency graduates interested in general medicine are finding hospital medicine an increasingly popular career choice. As a result, AHM groups have many recent residency graduates with an average age that is generally younger than 40.3
Over 85% of hospitalists are generalists and should find natural alliances with the nonhospitalist side of general internal medicine by collaborating in the course of clinical care, by teaching residents and students, or by designing quality improvement or research projects. In many academic centers, hospitalists are part of the division of general internal medicine, whereas in a few centers, hospitalists either have a separate division or lie outside the internal medicine department (employed by their hospitals).
Despite sharing a common training background and generalist mindset, many new academic hospitalists face different challenges than those faced by pure outpatient‐based academic generalists. First, at many centers, the financial arrangements between the AHM group and the hospital discourage hospitalists from traditional academic pursuits and draw them into clinical, operational, or administrative duties (such as responsibility for utilization review) that, although locally valuable, may not count as academic products in themselves or may take time away from more academic activities. Close alignment between hospitals and AHM may result in hospital administrators dictating hospitalists' practice in a way that further impedes academic viability. Reductions in resident training hours and an increasing need to provide 24‐hour coverage have facilitated growth in AHM into roles beyond those of the traditional academic generalist, such as medical comanagement of surgical patients and coverage of nonteaching services.4, 5 The youth of the field may exacerbate these problems. Most academic hospitalist groups have few senior leaders, whether they are clinical‐, education‐, or research‐focused. Young faculty need senior leaders as mentors to buffer them from relentless clinical demands that would compromise their hopes for academic success.
In order to better characterize these concerns and develop a shared work plan for future activities in support of AHM, the Society of Hospital Medicine (SHM) and the Society of General Internal Medicine (SGIM) convened an AHM consensus conference, a collaborative meeting developed and attended by representatives from SHM, SGIM, the Association of Chiefs of General Internal Medicine (ACGIM), the Association of Professors of Medicine, the Association of Program Directors in Internal Medicine, and the Association of Administrators in Internal Medicine. Using a structured consensus‐building format, we identified key barriers and challenges to AHM, then developed potential solutions.
Consensus Conference Format
Consensus Conference Steering Committee
The consensus conference was developed first by the sponsoring professional societies (SGIM, SHM, and ACGIM) being asked to nominate 2 people to be part of the consensus conference steering committee. The steering committee's main functions were to identify key tasks for the consensus conference, invite consensus conference attendees, ensure adequate representation from all participating organizations, synthesize the results of the consensus conference, and work with the individual professional societies so that results from the consensus conference were acted upon in a coordinated and effective manner.
Consensus Conference Prework
The consensus conference co‐chairs convened a series of conference calls in the spring of 2007, during which the steering committee developed a series of key areas to be explored during the conference. Topic areas were selected on the basis of the group's expertise and referred to past work by AHM taskforces convened by both SGIM/ACGIM and SHM.
The steering committee then invited stakeholders from each invited society so that each professional organization would provide at least 1 representative with expertise appropriate to 1 of the key domains identified:
-
Clinical and financial issues (within which topics such as optimal job descriptions and salary structures would be explored).
-
Teaching and education mission (within which topics such as mentorship for AHM junior faculty might be discussed).
-
Research and promotable activities (within which issues related to the development of promotable activities for AHM would be discussed).
Invitees to the consensus conference were assigned to one working group, given a general description of the potential areas within their domain, and instructed to consider a number of broad questions relevant to the topic area. These questions were as follows:
-
What are the key barriers to AHM in each key domain?
-
What viewpoints or barriers are the most pressing and/or actionable?
-
What solutions could be implemented or initiated in the next 1 to 3 years?
In order to facilitate discussion, consensus conference invitees were provided copies of findings from the SGIM/ACGIM and SHM Academic Hospitalist Task Forces, preliminary results from a survey of AHM leaders, and key literature related to the field.
Consensus Conference Format
The AHM consensus conference followed a modified Delphi consensus‐building format, in which the members of each working group developed findings relevant to their area, presented these viewpoints back to the overall group for feedback, and returned to their working group to refine their initial recommendations or move on to subsequent areas.
We used Day 1 of the meeting to confirm and refine lists of key barriers and opportunities to AHM. On Day 2, we developed actionable solutions and identified barriers with no ready solution but which were felt to be worth highlighting.
Each cycle of feedback (1 on Day 1 and 2 on Day 2) was used to identify additional barriers or opportunities prespecified by the steering committee, prioritize issues/opportunities, clarify uncertainties or point them out when they existed, and identify new areas requiring consensus. Between each cycle, workflow and interim results were summarized by the co‐chairs and a professional meeting coordinator to ensure that the group felt consensus had been achieved and to identify where additional work was required.
Writing Group/Peer Review
After the consensus conference adjourned, minutes were circulated to the group and approved, whereupon a summary of the meeting was reformatted into manuscript form. The manuscript was circulated to the steering committee, consensus conference attendees, and 2 selected peer reviewers as an additional check on the external validity of the study's results.
Consensus Findings 1: Current Challenges in AHM (Table 1)
Clinical and Financial Issues in AHM
The consensus group identified misalignment of the mission of hospitals (which often provide substantial financial support for hospital medicine programs) and the mission of departments of internal medicine (or divisions of general internal medicine) in which adult hospitalists reside as a fundamental barrier in AHM. Misalignment of missions produces challenges to the development of hospitalist groups in that their primary funder, the hospital, focuses on clinical care delivery, productivity, efficiency, and, in some cases, participation in patient safety and quality improvement efforts, whereas academic departments place considerable value on education, research, grants received, dissemination of scholarly work, and the national reputation of its faculty. Further exacerbating this tension is the fact that hospitalists do not always reside within traditional academic divisions (such as divisions of general medicine) and are therefore viewed by the hospital and their peers as hospital employees more than academic faculty.
As yet, few hospital medicine programs have successfully integrated academic and clinical needs. In many AHM programs, clinical demands have trumped academic pursuits and, as a result, produced jobs that have frequent turnover. This occurs most often when hospitalists are hired by academic medical centers primarily to staff nonresident services. Hospitalists who join these academic programs expecting ample opportunity to teach and pursue scholarly work often leave when they realize these jobs differ little from those in community settings (with the exception of less pay and, in most cases, a less efficient clinical delivery system). This turnover contributes to the perception of hospitalists as transient nonacademic faculty. The participants felt that we needed to define the ideal academic hospitalist job description.
Clinical and Financial Issues | Teaching and Education Mission | Research and Promotable Activities | Cross‐Cutting Issues |
---|---|---|---|
| |||
Hospitalists' functions more often explicitly linked to hospital initiatives (clinical care, quality improvement, utilization, and throughput) | Distinguishing jobs that are predominantly clinical (C‐e) from those that are predominantly education‐focused (c‐E), which is important given the high clinical burdens | Lack of a pipeline producing hospitalist clinician investigators | Lack of leadership or negotiation skill training |
Differing political, financial, and scientific priorities between hospitalists and administrators | Further exacerbation of C‐e/c‐E distinctions by the emergence of uncovered services | Few national funders focusing on inpatient general internal medicine | Little infrastructure for academic functions |
Little guidance on the best models for each job type | Little recognition of quality improvement as a promotable/testable activity | Rapidly moving/growing field | |
Decreasing interest in general internal medicine as a career path |
Teaching and Education Mission in AHM
Traditionally, faculty in academic medical centers have had prominent roles in resident teaching services, supervising medical residents, interns, and students. Hospitalists fill these roles at some institutions and in many cases have replaced senior faculty who are no longer able (because of competing demands from clinics or labs) or willing (because of an increased need for oversight and availability) to staff the teaching service. The teaching hospitalists start at these positions straight out of residency with little experience, training, or mentoring in how to succeed as a clinician educator. The creation of nonresident hospitalist services to address residency work hour requirements has removed many hospitalists from teaching opportunities as these services often have few if any teaching opportunities. The consensus group identified the lack of teaching opportunities and a lack of any formal preparation for those who do teach as the key challenges for new hospitalist clinician educators.
Research and Promotable Activities in AHM
Numerous challenges to promotion and success in hospital medicine research were identified. Most conference attendees felt that chairs of departments of medicine do not fully understand what the roles of academic hospitalists are, how they fit into the department's mission, or what is needed to better integrate hospitalists into the research and academic activities of the department. In addition, there are few hospital medicine fellowship programs, and those that have been created focus primarily on improving teaching skills or quality improvement rather than on research or the development of academic products. Aspiring academic hospitalists could pursue research fellowship training in existing programs (ie, the Robert Wood Johnson Foundation), but few graduates currently pursue these opportunities, and federally funded fellowships (eg, the National Research Service Awards and Health Resources and Services Administration T32 awards) explicitly exclude physicians who are not focused on primary care research. The group noted that a number of Veterans Administration fellowships (such as the Quality Scholars programs) may provide avenues for the training of hospital medicinefocused researchers, but they have been underused.
For researchers who focus on hospital medicine, federal funding sources are limited for both career development awards (K‐series) and later (R‐series) grants, particularly those funding the quality and safety research that hospitalists often pursue. Agencies of the National Institutes of Health currently do not provide many opportunities for hospital‐based general internal medicine research, and thus academic hospitalist research is undervalued by many promotion committees.
Cross‐Cutting Issues
Challenges in leadership and mentorship were identified as cross‐cutting. Many AHM programs are young, and so are their leaders. As a result, hospital medicine leaders often lack the experience and skills necessary to successfully negotiate for the support that is critical for the ideal program's success. As a young field, hospital medicine lacks faculty who have succeeded in careers as hospitalists, have been promoted in tenure tracks, and can mentor and guide young faculty through the complexities of academic medicine. Absent leadership and mentoring, few hospital medicine programs will succeed in traditional academic pursuits.
Consensus Findings 2: Overcoming Challenges to the Development of AHM (Table 2)
Summit attendees spent considerable time developing and refining solutions to the challenges described previously. Addressing the challenges resulted in a diverse group of proposed products that included educating key stakeholders, designing meetings, courses, or workshops, and gathering and disseminating data. There was considerable overlap among the solutions (Table 2).
Solutions | Proposed Products | Challenge Domains Addressed* |
---|---|---|
| ||
1. Educate stakeholders | Workshops at professional society meetings (SHM, SGIM, ACGIM, APM, and APDIM) | Addresses all domains |
Publications highlighting issues | ||
2. Define the sustainable job | Data gathering and publication | Clinical/financial |
3. Quality improvement portfolio | Development and dissemination of criteria for the QI portfolio | Research/promotion |
4. Hospitalist training/mentoring | Academic hospitalist boot camp | Teaching/education |
Research/promotion | ||
Cross‐cutting | ||
5. Enhance research career pathways | Advocacy for enhanced training programs and funding sources | Research/promotion |
6. Improved relationships among general medicine societies | Society collaboration on product development | Addresses all domains |
Outreach to and Education of Stakeholders in Academic Medicine
The focus of the educational and outreach efforts suggested by the consensus group is to help leaders in academic medicine (not just AHM) and academic medical centers understand the challenges facing AHM. More importantly, efforts should reinforce the value of academic hospitalists to their hospital, department, and division. Efforts to engage these critical stakeholders to discuss and potentially address a number of the conference's proposed solutions are needed. Leaders include deans of medical schools, chairs of departments of medicine, division chiefs, and hospital administrative leadership.
Suggested outreach and educational activities included the publication of articles in key journals with the goal of increasing the visibility of AHM in professional societies as well as meetings and workshops focusing on teaching hospitalists and academic leaders methods to overcome challenges. Professional societies with a stake in AHM should better understand the challenges and position themselves to address these issues. The AHM task forces of SHM and SGIM can help give academic hospitalists a voice in having their needs addressed.
Publications
Articles have been commissioned in the following areas: descriptions of challenges and proposed solutions, best practices for nonresident hospitalist services, and metrics for the success of hospital medicine programs.
Meetings/Workshops
Meetings and workshops, sponsored by professional societies with a vested interest in AHM, were thought to be an effective way to address the needs of hospitalists, particularly those pursuing careers as clinician educators. Such workshops would provide skills in teaching and early career survival (eg, how to bill correctly) and in developing an educator's portfolio. Leadership training offerings, perhaps building on examples from SHM and ACGIM, were also thought to be valuable resources and venues that should be directed toward hospitalists, their chiefs, and relevant leaders.
Defining a Sustainable Job Description for Academic Hospitalists
The group strongly endorsed the need for transparent and readily available data aimed at developing sustainable academic hospitalist positions. For example, required information would include how academic jobs are constructed (in terms of months on service per year and the number of nights or weekends of coverage) and what successful programs and their hospitalists have found to be acceptable. Over the longer term, empiric comparisons based on key metrics are needed to not only help guide career development and retention but also facilitate negotiations for programmatic support.
The group pointed out that embedded in delineating an optimal academic hospitalist job description is the longstanding work of general medicine societies in supporting and fostering the development of clinician educators. In many ways, the pressures of academic physicians to be mostly clinician and less educator versus someone who focuses heavily on educational work is similar for hospitalists and outpatient generalists. Academic general internal medicine divisions hired many general internists in the early 1990s to expand the reach of academic medical centers and increase the outpatient base.6 Many university hospitals are now hiring hospitalists to provide the inpatient care for these patients, but residency work hour reductions have added a layer of complexity, creating the need for entirely new roles for academic generalists (such as surgical comanagement of medically complex patients).7, 8 Past experiences in refining and reinforcing education as a key function (
Development of a Quality‐Improvement Portfolio Akin to an Educator's Portfolio
Many hospitalists actively participate in administrative work related to quality improvement activities, and we should develop this additional pathway for promotable academic activities (eg, clinician administrator); however, such a pathway may not be recognized by all promotion committees. The group observed that many aspects of quality improvement are similar to those of education (eg, developing a curriculum, leading a team, evaluating a process, defining generalizability, and disseminating locally proven interventions) and as such would be amenable to the development of a quality improvement portfolio, which candidates could submit to promotion committees. Again, past work in developing the importance and value of the educator's portfolio would facilitate the development of a quality improvement portfolio, which would require endorsement from key stakeholders (eg, the Association of Professors of Medicine, SGIM, and SHM).9 Importantly, this work may also benefit many outpatient‐based generalists who are increasingly focusing their careers on quality and safety improvement.
Developing Mentoring and Training Opportunities for Newly Hired and Junior Hospitalists
We reached a strong consensus about the need to develop a retreat‐format training opportunity by which junior academic hospitalists would be able to gain training in tasks critical to early‐career success. These were envisioned as an initial 2‐ to 3‐day meeting followed by mentorship at a distance and continued collaboration within the class of attendees. Topics would include key functions in AHM, such as becoming an effective attending physician and teacher, leadership, quality improvement, the business of medicine, effective billing, and maintaining a curriculum vitae. A number of professional societies have developed leadership or mentoring retreats, and at the time of this article's preparation, both regional and national efforts were underway to develop these products.
Developing Training and Mentorship Pathways for Hospitalist Researchers
There are few funded hospitalist researchers in the midcareer phase and a small but growing number of academic hospitalists entering the field with a focus on research. Enhancing a pipeline of researchers is a critical need for the field, as cementing AHM as an equal member of the academic medical community will be predicated on the successful development of hospitalist investigators. To this end, academic hospitalist groups should be encouraged to partner with other established research units (particularly general internal medicine) to create mentoring relationships and increase collaborative activities. The emergence of the Clinical and Translational Science Awards consortium sites, with a focus on implementation and effectiveness research, may also provide local opportunities for hospitalists to partner in research important for early‐career grant submission. Furthermore, building the pipeline of academic hospitalist researchers will require a strong focus on identifying students and residents through outreach at individual sites as well as presentations at national meetings (eg, the American College of Physicians).
Two other issues were also thought to be important. First, professional societies should work to encourage funders of primary carefocused general medicine training programs (the National Research Service Awards and the Health Resources and Services Administration) to allow hospitalists to qualify for such critical research training. Second, continuing to advocate for increasing funding for implementation and effectiveness research, via either the Agency for Healthcare Research and Quality or individual agencies of the National Institutes of Health, will be key; the emergence of a medical effectiveness institute would also be a potential boon.
Improving Relationships Between the Professional Homes of Academic Generalists
Relationships between outpatient‐based general medicine and hospital medicine were rocky as the field of AHM first took shape, and some residua of initial tensions persist a decade later. These tensions persist in part because hospitalists remain underdeveloped members of the academic community, and this perhaps gives some license to aver that hospitalists are merely transient faculty in a stage between residency and fellowship hired to improve throughput.
Overcoming this perception will require more engagement between academic generalists of all types, not less. The consensus group felt strongly that there need not be a single professional home for academic hospitalists and that generalists should be willing and even encouraged to self‐identify as hospital‐ or clinic‐focused, much as they might be geriatrics‐focused, informatics‐focused, or women's healthfocused. In fact, in some academic centers, a few generalists have successfully integrated themselves into both clinic‐based and hospitalist roles. In this way, the emergence and growth of AHM should be viewed as a boon to the practice of general medicine, not a challenge.
Resources
Much of what is proposed to enhance AHM will require resources. Academic hospitals have a vested interest in supporting AHM as a way to reduce turnover in a group that is increasingly critical for hospital operations, not to mention key leadership roles. Negotiating for these resources should emphasize that hospitals benefit directly from the revenue and margin that comes from incremental hospital admissions, collect most of the federal graduate medical education dollars, and benefit from improved care processes that are a result of hospitalist quality improvement efforts.
Deans and Departments, a key audience for the conference findings, also have a clear stake in fostering a less transient, more professionally satisfied and academically successful work force, particularly when hospitalists are increasingly the key educators of medical residents. Moreover, schools have a vested interest in the academic accomplishments and national reputation of their hospitalists. The financial arrangements will be unique to each setting and institution, and it is clear that the sources to be tapped will vary from site to site, but these resources are clearly necessary for the field.
Conclusions
AHM is at a crossroads. Unparalleled growth has created a large cadre of hospitalists who are struggling to meet the clinical demands of practice and the requirements for academic promotion; this situation will likely lead to, at a minimum, worsening problems with faculty turnover, and even greater losses of talented and passionate clinicians from the field of academic General Internal Medicine.
The challenges are numerous but not insurmountable, and our process identified issues and potential solutions which address clinical, educational, and research aspects of academic hospitalists' lives. We acknowledge that our findings are most relevant to hospitalists at academic medical centers or large academically oriented community teaching hospitals rather than hospitalists at community hospitals whose work is predominantly clinical with smaller teaching roles. However, we feel the academic hospitalists we targeted are in greater need of assistance. We believe that the most important issues are unsustainable, nonacademic positions, poor job preparation and training, inadequate prioritization of academic roles, and insufficient leadership and mentoring within the field.
It is the hope of all the consensus conference attendees that efforts focusing on academic hospitalists in the short term are not viewed as effort diverted from general internal medicine; in fact, the group felt that while many of the products of the consensus conference were probably most needed by AHM in the short term, these same solutions would likely be useful to outpatient‐based generalists as well. Despite the concerns and challenges outlined, the consensus conference group was also very hopeful that, in the setting where resources and collaboration are appropriately marshaled, that AHM will flourish quickly. In doing so, academic hospitalists will become better role models for residents and students, attracting the next generation of generalists needed to provide care to an increasingly complex patient population, and further advance the mission of General Internal Medicine.
Acknowledgements
The authors thank Dr. Jeff Glasheen and Dr. Robert Wachter for their comments on an earlier version of this article. In addition, the authors thank the following conference participants: Dan Brotman, MD, Johns Hopkins University; Deborah M. DeMarco, MD, President of the Association of Program Directors in Internal Medicine; Jeff Glasheen, MD, University of Colorado; Rusty Holman, MD, President of the Society of Hospital Medicine; Martha A. Hooven, President of the Administrators of Internal Medicine; Peter Kaboli, MD, University of Iowa; David O Meltzer, MD, PhD, University of Chicago; Vikas Parekh, MD, University of Michigan; Russell Phillips, MD MPH, Harvard Medical School; Sanjay Saint, MD, MPH, University of Michigan; Barbara Schuster, MD, President of the Association of Professors of Medicine; Brad Sharpe, MD, University of California San Francisco; Jeff Wiese, MD, Tulane University; David Kushner, Facilitator of the Kushner Companies; Geri Barnes, Support Staff of the Society of Hospital Medicine; and Amy Woodward, Support Staff of the Society of General Internal Medicine.
- The status of hospital medicine groups in the United States.J Hosp Med.2006;1:75–80. , , , .
- Trends in market demand for internal medicine 1999 to 2004: an analysis of physician job advertisements.J Gen Intern Med.2006;21:1079–1085. , , , , , .
- Society of Hospital Medicine. 2006 Hospital Medicine Survey. Available at: http://www.hospitalmedicine.org/content/navigationmenu/media/mediakit/media_kit.htm. Accessed January 2009.
- Effects of work hour reduction on residents' lives: a systematic review.JAMA.2005;294:1088–1100. , , , , , .
- Hospitalists in teaching hospitals: opportunities but not without danger.J Gen Intern Med.2004;19:392–393. , .
- UCLA rewrites the script for academic networks.Med Netw Strategy Rep.1998;7:1–5.
- Systematic review: effects of resident work hours on patient safety.Ann Intern Med.2004;141:851–857. , , , , , .
- The expanding role of hospitalists in the United States.Swiss Med Wkly.2006;136:591–596. , .
- Documentation systems for educators seeking academic promotion in U.S. medical schools.Acad Med.2004;79:783–790. , , , .
The past decade has seen hospital medicine grow from fewer than 1000 hospitalists nationwide to more than 20,000.1 In fact, survey data suggest that hospital medicine is the fastest growing field of internal medicine in the history of the US, and the growth of hospital medicine has produced a net increase in the number of generalists in the US.2
Although few direct estimates exist, academic hospital medicine (AHM) is also growing rapidly.1 Fueled by potential efficiency gains, a need for increased educational oversight of teaching services, and new residency work hour limitations, many academic medical centers and teaching hospitals have developed large hospital medicine programs. Internal medicine residency graduates interested in general medicine are finding hospital medicine an increasingly popular career choice. As a result, AHM groups have many recent residency graduates with an average age that is generally younger than 40.3
Over 85% of hospitalists are generalists and should find natural alliances with the nonhospitalist side of general internal medicine by collaborating in the course of clinical care, by teaching residents and students, or by designing quality improvement or research projects. In many academic centers, hospitalists are part of the division of general internal medicine, whereas in a few centers, hospitalists either have a separate division or lie outside the internal medicine department (employed by their hospitals).
Despite sharing a common training background and generalist mindset, many new academic hospitalists face different challenges than those faced by pure outpatient‐based academic generalists. First, at many centers, the financial arrangements between the AHM group and the hospital discourage hospitalists from traditional academic pursuits and draw them into clinical, operational, or administrative duties (such as responsibility for utilization review) that, although locally valuable, may not count as academic products in themselves or may take time away from more academic activities. Close alignment between hospitals and AHM may result in hospital administrators dictating hospitalists' practice in a way that further impedes academic viability. Reductions in resident training hours and an increasing need to provide 24‐hour coverage have facilitated growth in AHM into roles beyond those of the traditional academic generalist, such as medical comanagement of surgical patients and coverage of nonteaching services.4, 5 The youth of the field may exacerbate these problems. Most academic hospitalist groups have few senior leaders, whether they are clinical‐, education‐, or research‐focused. Young faculty need senior leaders as mentors to buffer them from relentless clinical demands that would compromise their hopes for academic success.
In order to better characterize these concerns and develop a shared work plan for future activities in support of AHM, the Society of Hospital Medicine (SHM) and the Society of General Internal Medicine (SGIM) convened an AHM consensus conference, a collaborative meeting developed and attended by representatives from SHM, SGIM, the Association of Chiefs of General Internal Medicine (ACGIM), the Association of Professors of Medicine, the Association of Program Directors in Internal Medicine, and the Association of Administrators in Internal Medicine. Using a structured consensus‐building format, we identified key barriers and challenges to AHM, then developed potential solutions.
Consensus Conference Format
Consensus Conference Steering Committee
The consensus conference was developed first by the sponsoring professional societies (SGIM, SHM, and ACGIM) being asked to nominate 2 people to be part of the consensus conference steering committee. The steering committee's main functions were to identify key tasks for the consensus conference, invite consensus conference attendees, ensure adequate representation from all participating organizations, synthesize the results of the consensus conference, and work with the individual professional societies so that results from the consensus conference were acted upon in a coordinated and effective manner.
Consensus Conference Prework
The consensus conference co‐chairs convened a series of conference calls in the spring of 2007, during which the steering committee developed a series of key areas to be explored during the conference. Topic areas were selected on the basis of the group's expertise and referred to past work by AHM taskforces convened by both SGIM/ACGIM and SHM.
The steering committee then invited stakeholders from each invited society so that each professional organization would provide at least 1 representative with expertise appropriate to 1 of the key domains identified:
-
Clinical and financial issues (within which topics such as optimal job descriptions and salary structures would be explored).
-
Teaching and education mission (within which topics such as mentorship for AHM junior faculty might be discussed).
-
Research and promotable activities (within which issues related to the development of promotable activities for AHM would be discussed).
Invitees to the consensus conference were assigned to one working group, given a general description of the potential areas within their domain, and instructed to consider a number of broad questions relevant to the topic area. These questions were as follows:
-
What are the key barriers to AHM in each key domain?
-
What viewpoints or barriers are the most pressing and/or actionable?
-
What solutions could be implemented or initiated in the next 1 to 3 years?
In order to facilitate discussion, consensus conference invitees were provided copies of findings from the SGIM/ACGIM and SHM Academic Hospitalist Task Forces, preliminary results from a survey of AHM leaders, and key literature related to the field.
Consensus Conference Format
The AHM consensus conference followed a modified Delphi consensus‐building format, in which the members of each working group developed findings relevant to their area, presented these viewpoints back to the overall group for feedback, and returned to their working group to refine their initial recommendations or move on to subsequent areas.
We used Day 1 of the meeting to confirm and refine lists of key barriers and opportunities to AHM. On Day 2, we developed actionable solutions and identified barriers with no ready solution but which were felt to be worth highlighting.
Each cycle of feedback (1 on Day 1 and 2 on Day 2) was used to identify additional barriers or opportunities prespecified by the steering committee, prioritize issues/opportunities, clarify uncertainties or point them out when they existed, and identify new areas requiring consensus. Between each cycle, workflow and interim results were summarized by the co‐chairs and a professional meeting coordinator to ensure that the group felt consensus had been achieved and to identify where additional work was required.
Writing Group/Peer Review
After the consensus conference adjourned, minutes were circulated to the group and approved, whereupon a summary of the meeting was reformatted into manuscript form. The manuscript was circulated to the steering committee, consensus conference attendees, and 2 selected peer reviewers as an additional check on the external validity of the study's results.
Consensus Findings 1: Current Challenges in AHM (Table 1)
Clinical and Financial Issues in AHM
The consensus group identified misalignment of the mission of hospitals (which often provide substantial financial support for hospital medicine programs) and the mission of departments of internal medicine (or divisions of general internal medicine) in which adult hospitalists reside as a fundamental barrier in AHM. Misalignment of missions produces challenges to the development of hospitalist groups in that their primary funder, the hospital, focuses on clinical care delivery, productivity, efficiency, and, in some cases, participation in patient safety and quality improvement efforts, whereas academic departments place considerable value on education, research, grants received, dissemination of scholarly work, and the national reputation of its faculty. Further exacerbating this tension is the fact that hospitalists do not always reside within traditional academic divisions (such as divisions of general medicine) and are therefore viewed by the hospital and their peers as hospital employees more than academic faculty.
As yet, few hospital medicine programs have successfully integrated academic and clinical needs. In many AHM programs, clinical demands have trumped academic pursuits and, as a result, produced jobs that have frequent turnover. This occurs most often when hospitalists are hired by academic medical centers primarily to staff nonresident services. Hospitalists who join these academic programs expecting ample opportunity to teach and pursue scholarly work often leave when they realize these jobs differ little from those in community settings (with the exception of less pay and, in most cases, a less efficient clinical delivery system). This turnover contributes to the perception of hospitalists as transient nonacademic faculty. The participants felt that we needed to define the ideal academic hospitalist job description.
Clinical and Financial Issues | Teaching and Education Mission | Research and Promotable Activities | Cross‐Cutting Issues |
---|---|---|---|
| |||
Hospitalists' functions more often explicitly linked to hospital initiatives (clinical care, quality improvement, utilization, and throughput) | Distinguishing jobs that are predominantly clinical (C‐e) from those that are predominantly education‐focused (c‐E), which is important given the high clinical burdens | Lack of a pipeline producing hospitalist clinician investigators | Lack of leadership or negotiation skill training |
Differing political, financial, and scientific priorities between hospitalists and administrators | Further exacerbation of C‐e/c‐E distinctions by the emergence of uncovered services | Few national funders focusing on inpatient general internal medicine | Little infrastructure for academic functions |
Little guidance on the best models for each job type | Little recognition of quality improvement as a promotable/testable activity | Rapidly moving/growing field | |
Decreasing interest in general internal medicine as a career path |
Teaching and Education Mission in AHM
Traditionally, faculty in academic medical centers have had prominent roles in resident teaching services, supervising medical residents, interns, and students. Hospitalists fill these roles at some institutions and in many cases have replaced senior faculty who are no longer able (because of competing demands from clinics or labs) or willing (because of an increased need for oversight and availability) to staff the teaching service. The teaching hospitalists start at these positions straight out of residency with little experience, training, or mentoring in how to succeed as a clinician educator. The creation of nonresident hospitalist services to address residency work hour requirements has removed many hospitalists from teaching opportunities as these services often have few if any teaching opportunities. The consensus group identified the lack of teaching opportunities and a lack of any formal preparation for those who do teach as the key challenges for new hospitalist clinician educators.
Research and Promotable Activities in AHM
Numerous challenges to promotion and success in hospital medicine research were identified. Most conference attendees felt that chairs of departments of medicine do not fully understand what the roles of academic hospitalists are, how they fit into the department's mission, or what is needed to better integrate hospitalists into the research and academic activities of the department. In addition, there are few hospital medicine fellowship programs, and those that have been created focus primarily on improving teaching skills or quality improvement rather than on research or the development of academic products. Aspiring academic hospitalists could pursue research fellowship training in existing programs (ie, the Robert Wood Johnson Foundation), but few graduates currently pursue these opportunities, and federally funded fellowships (eg, the National Research Service Awards and Health Resources and Services Administration T32 awards) explicitly exclude physicians who are not focused on primary care research. The group noted that a number of Veterans Administration fellowships (such as the Quality Scholars programs) may provide avenues for the training of hospital medicinefocused researchers, but they have been underused.
For researchers who focus on hospital medicine, federal funding sources are limited for both career development awards (K‐series) and later (R‐series) grants, particularly those funding the quality and safety research that hospitalists often pursue. Agencies of the National Institutes of Health currently do not provide many opportunities for hospital‐based general internal medicine research, and thus academic hospitalist research is undervalued by many promotion committees.
Cross‐Cutting Issues
Challenges in leadership and mentorship were identified as cross‐cutting. Many AHM programs are young, and so are their leaders. As a result, hospital medicine leaders often lack the experience and skills necessary to successfully negotiate for the support that is critical for the ideal program's success. As a young field, hospital medicine lacks faculty who have succeeded in careers as hospitalists, have been promoted in tenure tracks, and can mentor and guide young faculty through the complexities of academic medicine. Absent leadership and mentoring, few hospital medicine programs will succeed in traditional academic pursuits.
Consensus Findings 2: Overcoming Challenges to the Development of AHM (Table 2)
Summit attendees spent considerable time developing and refining solutions to the challenges described previously. Addressing the challenges resulted in a diverse group of proposed products that included educating key stakeholders, designing meetings, courses, or workshops, and gathering and disseminating data. There was considerable overlap among the solutions (Table 2).
Solutions | Proposed Products | Challenge Domains Addressed* |
---|---|---|
| ||
1. Educate stakeholders | Workshops at professional society meetings (SHM, SGIM, ACGIM, APM, and APDIM) | Addresses all domains |
Publications highlighting issues | ||
2. Define the sustainable job | Data gathering and publication | Clinical/financial |
3. Quality improvement portfolio | Development and dissemination of criteria for the QI portfolio | Research/promotion |
4. Hospitalist training/mentoring | Academic hospitalist boot camp | Teaching/education |
Research/promotion | ||
Cross‐cutting | ||
5. Enhance research career pathways | Advocacy for enhanced training programs and funding sources | Research/promotion |
6. Improved relationships among general medicine societies | Society collaboration on product development | Addresses all domains |
Outreach to and Education of Stakeholders in Academic Medicine
The focus of the educational and outreach efforts suggested by the consensus group is to help leaders in academic medicine (not just AHM) and academic medical centers understand the challenges facing AHM. More importantly, efforts should reinforce the value of academic hospitalists to their hospital, department, and division. Efforts to engage these critical stakeholders to discuss and potentially address a number of the conference's proposed solutions are needed. Leaders include deans of medical schools, chairs of departments of medicine, division chiefs, and hospital administrative leadership.
Suggested outreach and educational activities included the publication of articles in key journals with the goal of increasing the visibility of AHM in professional societies as well as meetings and workshops focusing on teaching hospitalists and academic leaders methods to overcome challenges. Professional societies with a stake in AHM should better understand the challenges and position themselves to address these issues. The AHM task forces of SHM and SGIM can help give academic hospitalists a voice in having their needs addressed.
Publications
Articles have been commissioned in the following areas: descriptions of challenges and proposed solutions, best practices for nonresident hospitalist services, and metrics for the success of hospital medicine programs.
Meetings/Workshops
Meetings and workshops, sponsored by professional societies with a vested interest in AHM, were thought to be an effective way to address the needs of hospitalists, particularly those pursuing careers as clinician educators. Such workshops would provide skills in teaching and early career survival (eg, how to bill correctly) and in developing an educator's portfolio. Leadership training offerings, perhaps building on examples from SHM and ACGIM, were also thought to be valuable resources and venues that should be directed toward hospitalists, their chiefs, and relevant leaders.
Defining a Sustainable Job Description for Academic Hospitalists
The group strongly endorsed the need for transparent and readily available data aimed at developing sustainable academic hospitalist positions. For example, required information would include how academic jobs are constructed (in terms of months on service per year and the number of nights or weekends of coverage) and what successful programs and their hospitalists have found to be acceptable. Over the longer term, empiric comparisons based on key metrics are needed to not only help guide career development and retention but also facilitate negotiations for programmatic support.
The group pointed out that embedded in delineating an optimal academic hospitalist job description is the longstanding work of general medicine societies in supporting and fostering the development of clinician educators. In many ways, the pressures of academic physicians to be mostly clinician and less educator versus someone who focuses heavily on educational work is similar for hospitalists and outpatient generalists. Academic general internal medicine divisions hired many general internists in the early 1990s to expand the reach of academic medical centers and increase the outpatient base.6 Many university hospitals are now hiring hospitalists to provide the inpatient care for these patients, but residency work hour reductions have added a layer of complexity, creating the need for entirely new roles for academic generalists (such as surgical comanagement of medically complex patients).7, 8 Past experiences in refining and reinforcing education as a key function (
Development of a Quality‐Improvement Portfolio Akin to an Educator's Portfolio
Many hospitalists actively participate in administrative work related to quality improvement activities, and we should develop this additional pathway for promotable academic activities (eg, clinician administrator); however, such a pathway may not be recognized by all promotion committees. The group observed that many aspects of quality improvement are similar to those of education (eg, developing a curriculum, leading a team, evaluating a process, defining generalizability, and disseminating locally proven interventions) and as such would be amenable to the development of a quality improvement portfolio, which candidates could submit to promotion committees. Again, past work in developing the importance and value of the educator's portfolio would facilitate the development of a quality improvement portfolio, which would require endorsement from key stakeholders (eg, the Association of Professors of Medicine, SGIM, and SHM).9 Importantly, this work may also benefit many outpatient‐based generalists who are increasingly focusing their careers on quality and safety improvement.
Developing Mentoring and Training Opportunities for Newly Hired and Junior Hospitalists
We reached a strong consensus about the need to develop a retreat‐format training opportunity by which junior academic hospitalists would be able to gain training in tasks critical to early‐career success. These were envisioned as an initial 2‐ to 3‐day meeting followed by mentorship at a distance and continued collaboration within the class of attendees. Topics would include key functions in AHM, such as becoming an effective attending physician and teacher, leadership, quality improvement, the business of medicine, effective billing, and maintaining a curriculum vitae. A number of professional societies have developed leadership or mentoring retreats, and at the time of this article's preparation, both regional and national efforts were underway to develop these products.
Developing Training and Mentorship Pathways for Hospitalist Researchers
There are few funded hospitalist researchers in the midcareer phase and a small but growing number of academic hospitalists entering the field with a focus on research. Enhancing a pipeline of researchers is a critical need for the field, as cementing AHM as an equal member of the academic medical community will be predicated on the successful development of hospitalist investigators. To this end, academic hospitalist groups should be encouraged to partner with other established research units (particularly general internal medicine) to create mentoring relationships and increase collaborative activities. The emergence of the Clinical and Translational Science Awards consortium sites, with a focus on implementation and effectiveness research, may also provide local opportunities for hospitalists to partner in research important for early‐career grant submission. Furthermore, building the pipeline of academic hospitalist researchers will require a strong focus on identifying students and residents through outreach at individual sites as well as presentations at national meetings (eg, the American College of Physicians).
Two other issues were also thought to be important. First, professional societies should work to encourage funders of primary carefocused general medicine training programs (the National Research Service Awards and the Health Resources and Services Administration) to allow hospitalists to qualify for such critical research training. Second, continuing to advocate for increasing funding for implementation and effectiveness research, via either the Agency for Healthcare Research and Quality or individual agencies of the National Institutes of Health, will be key; the emergence of a medical effectiveness institute would also be a potential boon.
Improving Relationships Between the Professional Homes of Academic Generalists
Relationships between outpatient‐based general medicine and hospital medicine were rocky as the field of AHM first took shape, and some residua of initial tensions persist a decade later. These tensions persist in part because hospitalists remain underdeveloped members of the academic community, and this perhaps gives some license to aver that hospitalists are merely transient faculty in a stage between residency and fellowship hired to improve throughput.
Overcoming this perception will require more engagement between academic generalists of all types, not less. The consensus group felt strongly that there need not be a single professional home for academic hospitalists and that generalists should be willing and even encouraged to self‐identify as hospital‐ or clinic‐focused, much as they might be geriatrics‐focused, informatics‐focused, or women's healthfocused. In fact, in some academic centers, a few generalists have successfully integrated themselves into both clinic‐based and hospitalist roles. In this way, the emergence and growth of AHM should be viewed as a boon to the practice of general medicine, not a challenge.
Resources
Much of what is proposed to enhance AHM will require resources. Academic hospitals have a vested interest in supporting AHM as a way to reduce turnover in a group that is increasingly critical for hospital operations, not to mention key leadership roles. Negotiating for these resources should emphasize that hospitals benefit directly from the revenue and margin that comes from incremental hospital admissions, collect most of the federal graduate medical education dollars, and benefit from improved care processes that are a result of hospitalist quality improvement efforts.
Deans and Departments, a key audience for the conference findings, also have a clear stake in fostering a less transient, more professionally satisfied and academically successful work force, particularly when hospitalists are increasingly the key educators of medical residents. Moreover, schools have a vested interest in the academic accomplishments and national reputation of their hospitalists. The financial arrangements will be unique to each setting and institution, and it is clear that the sources to be tapped will vary from site to site, but these resources are clearly necessary for the field.
Conclusions
AHM is at a crossroads. Unparalleled growth has created a large cadre of hospitalists who are struggling to meet the clinical demands of practice and the requirements for academic promotion; this situation will likely lead to, at a minimum, worsening problems with faculty turnover, and even greater losses of talented and passionate clinicians from the field of academic General Internal Medicine.
The challenges are numerous but not insurmountable, and our process identified issues and potential solutions which address clinical, educational, and research aspects of academic hospitalists' lives. We acknowledge that our findings are most relevant to hospitalists at academic medical centers or large academically oriented community teaching hospitals rather than hospitalists at community hospitals whose work is predominantly clinical with smaller teaching roles. However, we feel the academic hospitalists we targeted are in greater need of assistance. We believe that the most important issues are unsustainable, nonacademic positions, poor job preparation and training, inadequate prioritization of academic roles, and insufficient leadership and mentoring within the field.
It is the hope of all the consensus conference attendees that efforts focusing on academic hospitalists in the short term are not viewed as effort diverted from general internal medicine; in fact, the group felt that while many of the products of the consensus conference were probably most needed by AHM in the short term, these same solutions would likely be useful to outpatient‐based generalists as well. Despite the concerns and challenges outlined, the consensus conference group was also very hopeful that, in the setting where resources and collaboration are appropriately marshaled, that AHM will flourish quickly. In doing so, academic hospitalists will become better role models for residents and students, attracting the next generation of generalists needed to provide care to an increasingly complex patient population, and further advance the mission of General Internal Medicine.
Acknowledgements
The authors thank Dr. Jeff Glasheen and Dr. Robert Wachter for their comments on an earlier version of this article. In addition, the authors thank the following conference participants: Dan Brotman, MD, Johns Hopkins University; Deborah M. DeMarco, MD, President of the Association of Program Directors in Internal Medicine; Jeff Glasheen, MD, University of Colorado; Rusty Holman, MD, President of the Society of Hospital Medicine; Martha A. Hooven, President of the Administrators of Internal Medicine; Peter Kaboli, MD, University of Iowa; David O Meltzer, MD, PhD, University of Chicago; Vikas Parekh, MD, University of Michigan; Russell Phillips, MD MPH, Harvard Medical School; Sanjay Saint, MD, MPH, University of Michigan; Barbara Schuster, MD, President of the Association of Professors of Medicine; Brad Sharpe, MD, University of California San Francisco; Jeff Wiese, MD, Tulane University; David Kushner, Facilitator of the Kushner Companies; Geri Barnes, Support Staff of the Society of Hospital Medicine; and Amy Woodward, Support Staff of the Society of General Internal Medicine.
The past decade has seen hospital medicine grow from fewer than 1000 hospitalists nationwide to more than 20,000.1 In fact, survey data suggest that hospital medicine is the fastest growing field of internal medicine in the history of the US, and the growth of hospital medicine has produced a net increase in the number of generalists in the US.2
Although few direct estimates exist, academic hospital medicine (AHM) is also growing rapidly.1 Fueled by potential efficiency gains, a need for increased educational oversight of teaching services, and new residency work hour limitations, many academic medical centers and teaching hospitals have developed large hospital medicine programs. Internal medicine residency graduates interested in general medicine are finding hospital medicine an increasingly popular career choice. As a result, AHM groups have many recent residency graduates with an average age that is generally younger than 40.3
Over 85% of hospitalists are generalists and should find natural alliances with the nonhospitalist side of general internal medicine by collaborating in the course of clinical care, by teaching residents and students, or by designing quality improvement or research projects. In many academic centers, hospitalists are part of the division of general internal medicine, whereas in a few centers, hospitalists either have a separate division or lie outside the internal medicine department (employed by their hospitals).
Despite sharing a common training background and generalist mindset, many new academic hospitalists face different challenges than those faced by pure outpatient‐based academic generalists. First, at many centers, the financial arrangements between the AHM group and the hospital discourage hospitalists from traditional academic pursuits and draw them into clinical, operational, or administrative duties (such as responsibility for utilization review) that, although locally valuable, may not count as academic products in themselves or may take time away from more academic activities. Close alignment between hospitals and AHM may result in hospital administrators dictating hospitalists' practice in a way that further impedes academic viability. Reductions in resident training hours and an increasing need to provide 24‐hour coverage have facilitated growth in AHM into roles beyond those of the traditional academic generalist, such as medical comanagement of surgical patients and coverage of nonteaching services.4, 5 The youth of the field may exacerbate these problems. Most academic hospitalist groups have few senior leaders, whether they are clinical‐, education‐, or research‐focused. Young faculty need senior leaders as mentors to buffer them from relentless clinical demands that would compromise their hopes for academic success.
In order to better characterize these concerns and develop a shared work plan for future activities in support of AHM, the Society of Hospital Medicine (SHM) and the Society of General Internal Medicine (SGIM) convened an AHM consensus conference, a collaborative meeting developed and attended by representatives from SHM, SGIM, the Association of Chiefs of General Internal Medicine (ACGIM), the Association of Professors of Medicine, the Association of Program Directors in Internal Medicine, and the Association of Administrators in Internal Medicine. Using a structured consensus‐building format, we identified key barriers and challenges to AHM, then developed potential solutions.
Consensus Conference Format
Consensus Conference Steering Committee
The consensus conference was developed first by the sponsoring professional societies (SGIM, SHM, and ACGIM) being asked to nominate 2 people to be part of the consensus conference steering committee. The steering committee's main functions were to identify key tasks for the consensus conference, invite consensus conference attendees, ensure adequate representation from all participating organizations, synthesize the results of the consensus conference, and work with the individual professional societies so that results from the consensus conference were acted upon in a coordinated and effective manner.
Consensus Conference Prework
The consensus conference co‐chairs convened a series of conference calls in the spring of 2007, during which the steering committee developed a series of key areas to be explored during the conference. Topic areas were selected on the basis of the group's expertise and referred to past work by AHM taskforces convened by both SGIM/ACGIM and SHM.
The steering committee then invited stakeholders from each invited society so that each professional organization would provide at least 1 representative with expertise appropriate to 1 of the key domains identified:
-
Clinical and financial issues (within which topics such as optimal job descriptions and salary structures would be explored).
-
Teaching and education mission (within which topics such as mentorship for AHM junior faculty might be discussed).
-
Research and promotable activities (within which issues related to the development of promotable activities for AHM would be discussed).
Invitees to the consensus conference were assigned to one working group, given a general description of the potential areas within their domain, and instructed to consider a number of broad questions relevant to the topic area. These questions were as follows:
-
What are the key barriers to AHM in each key domain?
-
What viewpoints or barriers are the most pressing and/or actionable?
-
What solutions could be implemented or initiated in the next 1 to 3 years?
In order to facilitate discussion, consensus conference invitees were provided copies of findings from the SGIM/ACGIM and SHM Academic Hospitalist Task Forces, preliminary results from a survey of AHM leaders, and key literature related to the field.
Consensus Conference Format
The AHM consensus conference followed a modified Delphi consensus‐building format, in which the members of each working group developed findings relevant to their area, presented these viewpoints back to the overall group for feedback, and returned to their working group to refine their initial recommendations or move on to subsequent areas.
We used Day 1 of the meeting to confirm and refine lists of key barriers and opportunities to AHM. On Day 2, we developed actionable solutions and identified barriers with no ready solution but which were felt to be worth highlighting.
Each cycle of feedback (1 on Day 1 and 2 on Day 2) was used to identify additional barriers or opportunities prespecified by the steering committee, prioritize issues/opportunities, clarify uncertainties or point them out when they existed, and identify new areas requiring consensus. Between each cycle, workflow and interim results were summarized by the co‐chairs and a professional meeting coordinator to ensure that the group felt consensus had been achieved and to identify where additional work was required.
Writing Group/Peer Review
After the consensus conference adjourned, minutes were circulated to the group and approved, whereupon a summary of the meeting was reformatted into manuscript form. The manuscript was circulated to the steering committee, consensus conference attendees, and 2 selected peer reviewers as an additional check on the external validity of the study's results.
Consensus Findings 1: Current Challenges in AHM (Table 1)
Clinical and Financial Issues in AHM
The consensus group identified misalignment of the mission of hospitals (which often provide substantial financial support for hospital medicine programs) and the mission of departments of internal medicine (or divisions of general internal medicine) in which adult hospitalists reside as a fundamental barrier in AHM. Misalignment of missions produces challenges to the development of hospitalist groups in that their primary funder, the hospital, focuses on clinical care delivery, productivity, efficiency, and, in some cases, participation in patient safety and quality improvement efforts, whereas academic departments place considerable value on education, research, grants received, dissemination of scholarly work, and the national reputation of its faculty. Further exacerbating this tension is the fact that hospitalists do not always reside within traditional academic divisions (such as divisions of general medicine) and are therefore viewed by the hospital and their peers as hospital employees more than academic faculty.
As yet, few hospital medicine programs have successfully integrated academic and clinical needs. In many AHM programs, clinical demands have trumped academic pursuits and, as a result, produced jobs that have frequent turnover. This occurs most often when hospitalists are hired by academic medical centers primarily to staff nonresident services. Hospitalists who join these academic programs expecting ample opportunity to teach and pursue scholarly work often leave when they realize these jobs differ little from those in community settings (with the exception of less pay and, in most cases, a less efficient clinical delivery system). This turnover contributes to the perception of hospitalists as transient nonacademic faculty. The participants felt that we needed to define the ideal academic hospitalist job description.
Clinical and Financial Issues | Teaching and Education Mission | Research and Promotable Activities | Cross‐Cutting Issues |
---|---|---|---|
| |||
Hospitalists' functions more often explicitly linked to hospital initiatives (clinical care, quality improvement, utilization, and throughput) | Distinguishing jobs that are predominantly clinical (C‐e) from those that are predominantly education‐focused (c‐E), which is important given the high clinical burdens | Lack of a pipeline producing hospitalist clinician investigators | Lack of leadership or negotiation skill training |
Differing political, financial, and scientific priorities between hospitalists and administrators | Further exacerbation of C‐e/c‐E distinctions by the emergence of uncovered services | Few national funders focusing on inpatient general internal medicine | Little infrastructure for academic functions |
Little guidance on the best models for each job type | Little recognition of quality improvement as a promotable/testable activity | Rapidly moving/growing field | |
Decreasing interest in general internal medicine as a career path |
Teaching and Education Mission in AHM
Traditionally, faculty in academic medical centers have had prominent roles in resident teaching services, supervising medical residents, interns, and students. Hospitalists fill these roles at some institutions and in many cases have replaced senior faculty who are no longer able (because of competing demands from clinics or labs) or willing (because of an increased need for oversight and availability) to staff the teaching service. The teaching hospitalists start at these positions straight out of residency with little experience, training, or mentoring in how to succeed as a clinician educator. The creation of nonresident hospitalist services to address residency work hour requirements has removed many hospitalists from teaching opportunities as these services often have few if any teaching opportunities. The consensus group identified the lack of teaching opportunities and a lack of any formal preparation for those who do teach as the key challenges for new hospitalist clinician educators.
Research and Promotable Activities in AHM
Numerous challenges to promotion and success in hospital medicine research were identified. Most conference attendees felt that chairs of departments of medicine do not fully understand what the roles of academic hospitalists are, how they fit into the department's mission, or what is needed to better integrate hospitalists into the research and academic activities of the department. In addition, there are few hospital medicine fellowship programs, and those that have been created focus primarily on improving teaching skills or quality improvement rather than on research or the development of academic products. Aspiring academic hospitalists could pursue research fellowship training in existing programs (ie, the Robert Wood Johnson Foundation), but few graduates currently pursue these opportunities, and federally funded fellowships (eg, the National Research Service Awards and Health Resources and Services Administration T32 awards) explicitly exclude physicians who are not focused on primary care research. The group noted that a number of Veterans Administration fellowships (such as the Quality Scholars programs) may provide avenues for the training of hospital medicinefocused researchers, but they have been underused.
For researchers who focus on hospital medicine, federal funding sources are limited for both career development awards (K‐series) and later (R‐series) grants, particularly those funding the quality and safety research that hospitalists often pursue. Agencies of the National Institutes of Health currently do not provide many opportunities for hospital‐based general internal medicine research, and thus academic hospitalist research is undervalued by many promotion committees.
Cross‐Cutting Issues
Challenges in leadership and mentorship were identified as cross‐cutting. Many AHM programs are young, and so are their leaders. As a result, hospital medicine leaders often lack the experience and skills necessary to successfully negotiate for the support that is critical for the ideal program's success. As a young field, hospital medicine lacks faculty who have succeeded in careers as hospitalists, have been promoted in tenure tracks, and can mentor and guide young faculty through the complexities of academic medicine. Absent leadership and mentoring, few hospital medicine programs will succeed in traditional academic pursuits.
Consensus Findings 2: Overcoming Challenges to the Development of AHM (Table 2)
Summit attendees spent considerable time developing and refining solutions to the challenges described previously. Addressing the challenges resulted in a diverse group of proposed products that included educating key stakeholders, designing meetings, courses, or workshops, and gathering and disseminating data. There was considerable overlap among the solutions (Table 2).
Solutions | Proposed Products | Challenge Domains Addressed* |
---|---|---|
| ||
1. Educate stakeholders | Workshops at professional society meetings (SHM, SGIM, ACGIM, APM, and APDIM) | Addresses all domains |
Publications highlighting issues | ||
2. Define the sustainable job | Data gathering and publication | Clinical/financial |
3. Quality improvement portfolio | Development and dissemination of criteria for the QI portfolio | Research/promotion |
4. Hospitalist training/mentoring | Academic hospitalist boot camp | Teaching/education |
Research/promotion | ||
Cross‐cutting | ||
5. Enhance research career pathways | Advocacy for enhanced training programs and funding sources | Research/promotion |
6. Improved relationships among general medicine societies | Society collaboration on product development | Addresses all domains |
Outreach to and Education of Stakeholders in Academic Medicine
The focus of the educational and outreach efforts suggested by the consensus group is to help leaders in academic medicine (not just AHM) and academic medical centers understand the challenges facing AHM. More importantly, efforts should reinforce the value of academic hospitalists to their hospital, department, and division. Efforts to engage these critical stakeholders to discuss and potentially address a number of the conference's proposed solutions are needed. Leaders include deans of medical schools, chairs of departments of medicine, division chiefs, and hospital administrative leadership.
Suggested outreach and educational activities included the publication of articles in key journals with the goal of increasing the visibility of AHM in professional societies as well as meetings and workshops focusing on teaching hospitalists and academic leaders methods to overcome challenges. Professional societies with a stake in AHM should better understand the challenges and position themselves to address these issues. The AHM task forces of SHM and SGIM can help give academic hospitalists a voice in having their needs addressed.
Publications
Articles have been commissioned in the following areas: descriptions of challenges and proposed solutions, best practices for nonresident hospitalist services, and metrics for the success of hospital medicine programs.
Meetings/Workshops
Meetings and workshops, sponsored by professional societies with a vested interest in AHM, were thought to be an effective way to address the needs of hospitalists, particularly those pursuing careers as clinician educators. Such workshops would provide skills in teaching and early career survival (eg, how to bill correctly) and in developing an educator's portfolio. Leadership training offerings, perhaps building on examples from SHM and ACGIM, were also thought to be valuable resources and venues that should be directed toward hospitalists, their chiefs, and relevant leaders.
Defining a Sustainable Job Description for Academic Hospitalists
The group strongly endorsed the need for transparent and readily available data aimed at developing sustainable academic hospitalist positions. For example, required information would include how academic jobs are constructed (in terms of months on service per year and the number of nights or weekends of coverage) and what successful programs and their hospitalists have found to be acceptable. Over the longer term, empiric comparisons based on key metrics are needed to not only help guide career development and retention but also facilitate negotiations for programmatic support.
The group pointed out that embedded in delineating an optimal academic hospitalist job description is the longstanding work of general medicine societies in supporting and fostering the development of clinician educators. In many ways, the pressures of academic physicians to be mostly clinician and less educator versus someone who focuses heavily on educational work is similar for hospitalists and outpatient generalists. Academic general internal medicine divisions hired many general internists in the early 1990s to expand the reach of academic medical centers and increase the outpatient base.6 Many university hospitals are now hiring hospitalists to provide the inpatient care for these patients, but residency work hour reductions have added a layer of complexity, creating the need for entirely new roles for academic generalists (such as surgical comanagement of medically complex patients).7, 8 Past experiences in refining and reinforcing education as a key function (
Development of a Quality‐Improvement Portfolio Akin to an Educator's Portfolio
Many hospitalists actively participate in administrative work related to quality improvement activities, and we should develop this additional pathway for promotable academic activities (eg, clinician administrator); however, such a pathway may not be recognized by all promotion committees. The group observed that many aspects of quality improvement are similar to those of education (eg, developing a curriculum, leading a team, evaluating a process, defining generalizability, and disseminating locally proven interventions) and as such would be amenable to the development of a quality improvement portfolio, which candidates could submit to promotion committees. Again, past work in developing the importance and value of the educator's portfolio would facilitate the development of a quality improvement portfolio, which would require endorsement from key stakeholders (eg, the Association of Professors of Medicine, SGIM, and SHM).9 Importantly, this work may also benefit many outpatient‐based generalists who are increasingly focusing their careers on quality and safety improvement.
Developing Mentoring and Training Opportunities for Newly Hired and Junior Hospitalists
We reached a strong consensus about the need to develop a retreat‐format training opportunity by which junior academic hospitalists would be able to gain training in tasks critical to early‐career success. These were envisioned as an initial 2‐ to 3‐day meeting followed by mentorship at a distance and continued collaboration within the class of attendees. Topics would include key functions in AHM, such as becoming an effective attending physician and teacher, leadership, quality improvement, the business of medicine, effective billing, and maintaining a curriculum vitae. A number of professional societies have developed leadership or mentoring retreats, and at the time of this article's preparation, both regional and national efforts were underway to develop these products.
Developing Training and Mentorship Pathways for Hospitalist Researchers
There are few funded hospitalist researchers in the midcareer phase and a small but growing number of academic hospitalists entering the field with a focus on research. Enhancing a pipeline of researchers is a critical need for the field, as cementing AHM as an equal member of the academic medical community will be predicated on the successful development of hospitalist investigators. To this end, academic hospitalist groups should be encouraged to partner with other established research units (particularly general internal medicine) to create mentoring relationships and increase collaborative activities. The emergence of the Clinical and Translational Science Awards consortium sites, with a focus on implementation and effectiveness research, may also provide local opportunities for hospitalists to partner in research important for early‐career grant submission. Furthermore, building the pipeline of academic hospitalist researchers will require a strong focus on identifying students and residents through outreach at individual sites as well as presentations at national meetings (eg, the American College of Physicians).
Two other issues were also thought to be important. First, professional societies should work to encourage funders of primary carefocused general medicine training programs (the National Research Service Awards and the Health Resources and Services Administration) to allow hospitalists to qualify for such critical research training. Second, continuing to advocate for increasing funding for implementation and effectiveness research, via either the Agency for Healthcare Research and Quality or individual agencies of the National Institutes of Health, will be key; the emergence of a medical effectiveness institute would also be a potential boon.
Improving Relationships Between the Professional Homes of Academic Generalists
Relationships between outpatient‐based general medicine and hospital medicine were rocky as the field of AHM first took shape, and some residua of initial tensions persist a decade later. These tensions persist in part because hospitalists remain underdeveloped members of the academic community, and this perhaps gives some license to aver that hospitalists are merely transient faculty in a stage between residency and fellowship hired to improve throughput.
Overcoming this perception will require more engagement between academic generalists of all types, not less. The consensus group felt strongly that there need not be a single professional home for academic hospitalists and that generalists should be willing and even encouraged to self‐identify as hospital‐ or clinic‐focused, much as they might be geriatrics‐focused, informatics‐focused, or women's healthfocused. In fact, in some academic centers, a few generalists have successfully integrated themselves into both clinic‐based and hospitalist roles. In this way, the emergence and growth of AHM should be viewed as a boon to the practice of general medicine, not a challenge.
Resources
Much of what is proposed to enhance AHM will require resources. Academic hospitals have a vested interest in supporting AHM as a way to reduce turnover in a group that is increasingly critical for hospital operations, not to mention key leadership roles. Negotiating for these resources should emphasize that hospitals benefit directly from the revenue and margin that comes from incremental hospital admissions, collect most of the federal graduate medical education dollars, and benefit from improved care processes that are a result of hospitalist quality improvement efforts.
Deans and Departments, a key audience for the conference findings, also have a clear stake in fostering a less transient, more professionally satisfied and academically successful work force, particularly when hospitalists are increasingly the key educators of medical residents. Moreover, schools have a vested interest in the academic accomplishments and national reputation of their hospitalists. The financial arrangements will be unique to each setting and institution, and it is clear that the sources to be tapped will vary from site to site, but these resources are clearly necessary for the field.
Conclusions
AHM is at a crossroads. Unparalleled growth has created a large cadre of hospitalists who are struggling to meet the clinical demands of practice and the requirements for academic promotion; this situation will likely lead to, at a minimum, worsening problems with faculty turnover, and even greater losses of talented and passionate clinicians from the field of academic General Internal Medicine.
The challenges are numerous but not insurmountable, and our process identified issues and potential solutions which address clinical, educational, and research aspects of academic hospitalists' lives. We acknowledge that our findings are most relevant to hospitalists at academic medical centers or large academically oriented community teaching hospitals rather than hospitalists at community hospitals whose work is predominantly clinical with smaller teaching roles. However, we feel the academic hospitalists we targeted are in greater need of assistance. We believe that the most important issues are unsustainable, nonacademic positions, poor job preparation and training, inadequate prioritization of academic roles, and insufficient leadership and mentoring within the field.
It is the hope of all the consensus conference attendees that efforts focusing on academic hospitalists in the short term are not viewed as effort diverted from general internal medicine; in fact, the group felt that while many of the products of the consensus conference were probably most needed by AHM in the short term, these same solutions would likely be useful to outpatient‐based generalists as well. Despite the concerns and challenges outlined, the consensus conference group was also very hopeful that, in the setting where resources and collaboration are appropriately marshaled, that AHM will flourish quickly. In doing so, academic hospitalists will become better role models for residents and students, attracting the next generation of generalists needed to provide care to an increasingly complex patient population, and further advance the mission of General Internal Medicine.
Acknowledgements
The authors thank Dr. Jeff Glasheen and Dr. Robert Wachter for their comments on an earlier version of this article. In addition, the authors thank the following conference participants: Dan Brotman, MD, Johns Hopkins University; Deborah M. DeMarco, MD, President of the Association of Program Directors in Internal Medicine; Jeff Glasheen, MD, University of Colorado; Rusty Holman, MD, President of the Society of Hospital Medicine; Martha A. Hooven, President of the Administrators of Internal Medicine; Peter Kaboli, MD, University of Iowa; David O Meltzer, MD, PhD, University of Chicago; Vikas Parekh, MD, University of Michigan; Russell Phillips, MD MPH, Harvard Medical School; Sanjay Saint, MD, MPH, University of Michigan; Barbara Schuster, MD, President of the Association of Professors of Medicine; Brad Sharpe, MD, University of California San Francisco; Jeff Wiese, MD, Tulane University; David Kushner, Facilitator of the Kushner Companies; Geri Barnes, Support Staff of the Society of Hospital Medicine; and Amy Woodward, Support Staff of the Society of General Internal Medicine.
- The status of hospital medicine groups in the United States.J Hosp Med.2006;1:75–80. , , , .
- Trends in market demand for internal medicine 1999 to 2004: an analysis of physician job advertisements.J Gen Intern Med.2006;21:1079–1085. , , , , , .
- Society of Hospital Medicine. 2006 Hospital Medicine Survey. Available at: http://www.hospitalmedicine.org/content/navigationmenu/media/mediakit/media_kit.htm. Accessed January 2009.
- Effects of work hour reduction on residents' lives: a systematic review.JAMA.2005;294:1088–1100. , , , , , .
- Hospitalists in teaching hospitals: opportunities but not without danger.J Gen Intern Med.2004;19:392–393. , .
- UCLA rewrites the script for academic networks.Med Netw Strategy Rep.1998;7:1–5.
- Systematic review: effects of resident work hours on patient safety.Ann Intern Med.2004;141:851–857. , , , , , .
- The expanding role of hospitalists in the United States.Swiss Med Wkly.2006;136:591–596. , .
- Documentation systems for educators seeking academic promotion in U.S. medical schools.Acad Med.2004;79:783–790. , , , .
- The status of hospital medicine groups in the United States.J Hosp Med.2006;1:75–80. , , , .
- Trends in market demand for internal medicine 1999 to 2004: an analysis of physician job advertisements.J Gen Intern Med.2006;21:1079–1085. , , , , , .
- Society of Hospital Medicine. 2006 Hospital Medicine Survey. Available at: http://www.hospitalmedicine.org/content/navigationmenu/media/mediakit/media_kit.htm. Accessed January 2009.
- Effects of work hour reduction on residents' lives: a systematic review.JAMA.2005;294:1088–1100. , , , , , .
- Hospitalists in teaching hospitals: opportunities but not without danger.J Gen Intern Med.2004;19:392–393. , .
- UCLA rewrites the script for academic networks.Med Netw Strategy Rep.1998;7:1–5.
- Systematic review: effects of resident work hours on patient safety.Ann Intern Med.2004;141:851–857. , , , , , .
- The expanding role of hospitalists in the United States.Swiss Med Wkly.2006;136:591–596. , .
- Documentation systems for educators seeking academic promotion in U.S. medical schools.Acad Med.2004;79:783–790. , , , .