User login
Advancing Diversity, Equity, and Inclusion in Hospital Medicine
Studies continue to demonstrate persistent gaps in equity for women and underrepresented minorities (URMs)1 throughout nearly all aspects of academic medicine, including rank,2-4 tenure,5 authorship,6,7 funding opportunities,8,9 awards,10 speakership,11 leadership,12,13 and salaries.2,14,15
In this article, we report DEI efforts within our division, focusing on the development of our strategic plan and specific outcomes related to compensation, recruitment, and policies.
METHODS
Our Division’s Framework to DEI—“It Takes a Village”
Our Division of Hospital Medicine (DHM), previously within the Division of General Internal Medicine, was founded in October 2017. The DHM at the University of Colorado Hospital (UCH) is composed of 100 faculty members (70 physicians and 30 advanced-practice providers; 58% women and 42% men). In 2018, we implemented a stepwise approach to critically assess DEI within our group and to build a strategic plan to address the issues.
Needs Assessment
As a new division, we sought stakeholder feedback from division members. All faculty within the division were invited to attend a meeting in which issues related to DEI were discussed. A literature review that spanned both medical and nonmedical fields was also completed. Search terms included salary equity, gender equity, diverse teams, diversity recruitment and retention, diversifying leadership, and diverse speakers. Salaries, internally funded time, and other processes, such as recruitment, promotion, and hiring for leadership positions, were evaluated during the first year we became a division.
Interventions
TThrough this work, and with stakeholder engagement, we developed a divisional strategic plan to address DEI globally. Our strategic plan included developing a DEI director role to assist with overseeing DEI efforts. We have highlighted the various methods utilized for each component (Figure 1). This work occurred from October 2017 to December 2018.
Our institutional structures
Using best practices from both medical and nonmedical fields, we developed evidence-based approaches to
Compensation: transparent and consistent approaches based upon benchmarking with a framework of equal pay for equal work and similar advanced training/academic rank. In conjunction with efforts within the School of Medicine (SOM), Department of Medicine (DOM), and the UCH, our division sought to study salaries across DHM faculty members. We had an open call for faculty to participate in a newly developed DHM Compensation Committee, with the intent of rigorously examining our compensation practices and goals. Through faculty feedback and committee work, salary equity was defined as equal pay (ie, base salary for one clinical full-time equivalent [FTE]) for equal work based on academic rank and/or years of practice/advanced training. We also compared DHM salaries to regional academic hospital medicine groups and concluded that DHM salaries were lower than local and national benchmarks. This information was used to create a two-phase approach to increasing salaries for all individuals below the American Association of Medical Colleges (AAMC) benchmarks33 for academic hospitalists. We also developed a stipend system for external roles that came with additional compensation and roles within our own division that came with additional pay (ie, nocturnist). Phase 1 focused on those whose salaries were furthest away from and below benchmark, and phase 2 targeted all remaining individuals below benchmark.
A similar review of FTEs (based on required number of shifts for a full-time hospitalist) tied to our internal DHM leadership positions was completed by the division head and director of DEI. Specifically, the mission for each of our internally funded roles, job descriptions, and responsibilities was reviewed to ensure equity in funding.
Recruitment and advancement: processes to ensure equity and diversity in recruitment, tracking, and reporting, working to eliminate/mitigate bias. In collaboration with members of the AAMC Group on Women in Medicine and Science (GWIMS) and coauthors from various institutions, we developed toolkits and checklists aimed at achieving equity and diversity within candidate pools and on major committees, including, but not limited to, search and promotion committees.32 Additionally, a checklist was developed to help recruit more diverse speakers, including women and URMs, for local, regional, and national conferences.
Policies: evidence-based approaches, tracking and reporting, standardized approaches to eliminate/mitigate bias, embracing nontraditional paths. In partnership with our departmental efforts, members of our team led data collection and reporting for salary benchmarking, leadership roles, and committee membership. This included developing surveys and reporting templates that can be used to identify disparities and inform future efforts. We worked to ensure that we have faculty representing our field at the department and SOM levels. Specifically, we made sure to nominate division members during open calls for departmental and schoolwide committees, including the promotions committee.
Our People
The faculty and staff within our division have been instrumental in moving efforts forward in the following important areas.
Leadership: develop the position of director of DEI as well as leadership structures to support and increase DEI. One of the first steps in our strategic plan was creating a director of DEI leadership role (Appendix Figure 2). The director is responsible for researching, applying, and promoting a broad scope of DEI initiatives and best practices within the DHM, DOM, and SOM (in collaboration with their leaders), including recruitment, retention, and promotion of medical students, residents, and faculty; educational program development; health disparities research; and community-engaged scholarship.
Support: develop family leave policies/develop flexible work policies. Several members of our division worked on departmental committees and served in leadership roles on staff and faculty council. Estimated costs were assessed. Through collective efforts of department leadership and division head support, the department approved parental leave to employees following the birth of an employee’s child or the placement of a child with an employee in connection with adoption or permanent foster care.
Mentorship/sponsorship: enhance faculty advancement programs/develop pipeline and trainings/collaborate with student groups and organizations/invest in all of our people. Faculty across our divisional sites have held important roles in developing pipeline programs for undergraduate students bound for health professions, as well as programs developed specifically for medical students and internal medicine residents. This includes two programs, the CU Hospitalist Scholars Program (CUHSP) and Leadership Education for Aspiring Doctors (LEAD), in which undergraduate students have the opportunity to round with hospital medicine teams, work on quality-improvement projects, and receive extensive mentorship and advising from a diverse faculty team. Additionally, our faculty advancement team within the DHM has grown and been restructured to include more defined goals and to ensure each faculty member has at least one mentor in their area of interest.
Supportive: lactation space and support/diverse space options/inclusive and diverse environments. We worked closely with hospital leadership to advocate for adequately equipped lactation spaces, including equipment such as pumps, refrigerators, and computer workstations.
Measures
Our measures focused on (1) development and implementation of our DEI strategic plan, including new policies, processes, and practices related to key components of the DEI program; and (2) assessment of specific DEI programs, including pre-post salary data disparities based on rank and pre-post disparities for protected time for similar roles.
Analysis
Through rapid PDSA cycles, we evaluated salary equity, equity in leadership allotment, and committee membership. We have developed a tracking board to track progress of the multiple projects in the strategic plan.
RESULTS
Strategic Plan Development and Tracking
From October 2017 to December 2018, we developed a robust strategic plan and stepwise approach to DEI (Figure 1 and Figure 2). The director of DEI position was developed (see Appendix Figure 2 for job description) to help oversee these efforts. Figure 3 highlights the specific efforts and the progress made on implementation (ie, high-level dashboard or “tracking board”). While outcomes are still pending in the areas of recruitment and advancement and environment, we have made measurable improvements in compensation, as outlined in the following section.
Compensation
One year after the salary-equity interventions, all of our physician faculty’s salaries were at the goal benchmark (Table), and differences in salary for those in similar years of rank were nearly eliminated. Similarly, after implementing an internally consistent approach to assigning FTE for new and established positions within the division (ie, those that fall within the purview of the division), all faculty in similar types of roles had similar amounts of protected time.
Recruitment and Advancement
Toolkits32 and committee recommendations have been incorporated into division goals, though some aspects are still in implementation phases, as division-wide implicit bias training was delayed secondary to the COVID-19 pandemic. Key goals include: (1) implicit bias training for all members of major committees; (2) aiming for a goal of at least 40% representation of women and 40% URMs on committees; (3) having a diversity expert serve on each committee in order to identify and discuss any potential bias in the search and candidate-selection processes; and (4) careful tracking of diversity metrics in regard to diversity of candidates at each step of the interview and selection process.
Surveys and reporting templates for equity on committees and leadership positions have been developed and deployed. Data dashboards for our division have been developed as well (for compensation, leadership, and committee membership). A divisional dashboard to report recruitment efforts is in progress.
We have successfully nominated several faculty members to the SOM promotions committee and departmental committees during open calls for these positions. At the division level, we have also adapted internal policies to ensure promotion occurs on time and offers alternative pathways for faculty that may primarily focus on clinical pathways. All faculty who have gone up for promotion thus far have been successfully promoted in their desired pathway.
Environment
We successfully advocated and achieved adequately equipped lactation spaces, including equipment such as pumps, refrigerators, and computer workstations. This achievement was possible because of our hospital partners. Our efforts helped us acquire sufficient space and facilities such that nursing mothers can pump and still be able to answer phones, enter orders, and document visits.
Our team members conducted environmental scans and raised concerns when the environment was not inclusive, such as conference rooms with portraits of leadership that do not show diversity. The all-male pictures were removed from one frequently used departmental conference room, which will eventually house a diverse group of pictures and achievements.
We aim to eliminate bias by offering implicit bias training for our faculty. While this is presently required for those who serve on committees, in leadership positions, or those involved in recruitment and interviewing for the DOM, our goal is to eventually provide this training to all faculty and staff in the division. We have also incorporated DEI topics into our educational conferences for faculty, including sessions on recognizing bias in medicine, how to be an upstander/ally, and the impact of race and racism on medicine.
DISCUSSION
The important findings of this work are: (1) that successes in DEI can be achieved with strategic planning and stakeholder engagement; (2) through simple modification of processes, we can improve equity in compensation and FTE allotted to leadership; (3) though it takes time, diversity recruitment can be improved using sound, sustainable, evidence-based processes; (4) this work is time-intensive and challenging, requiring ongoing efforts to improve, modify, and enhance current efforts and future successes.
We have certainly made some progress with DEI initiatives within our division and have also learned a great deal from this experience. First, change is difficult for all parties involved, including those leading change and those affected by the changes. We purposely made an effort to facilitate discussions with all of the DHM faculty and staff to ensure that everyone felt included in this work and that everyone’s voice was heard. This was exemplified by inviting all faculty members to a feedback session in which we discussed DEI within our division and areas that we wanted to improve on. Early on, we were able to define what diversity, equity, and inclusion meant to us as a division and then use these definitions to develop tangible goals for all the areas of highest importance to the group.
By increasing faculty presence on key committees, such as the promotions committee, we now have faculty members who are well versed in promotions processes. We are fortunate to have a promotions process that supports faculty advancement for faculty with diverse interests that spans from supporting highly clinical faculty, clinician educators, as well as more traditional researchers.34 By having hospitalists serve in these roles, we help to add to the diverse perspectives on these committees, including emphasizing the scholarship that is associated with quality improvement, as well as DEI efforts which can often be viewed as service as opposed to scholarship.
Clear communication and transparency were key to all of our DEI initiatives. We had monthly updates on our DEI efforts during business meetings and also held impromptu meetings (also known as flash mobs35) to answer questions and discuss concerns in real time. As with all DEI work, it is important to know where you are starting (having accurate data and a clear understanding of the data) and be able to communicate that data to the group. For example, using AAMC salary benchmarking33 as well as other benchmarks allowed us to accurately calculate variance among salaries and identify the appropriate goal salary for each of our faculty members. Likewise, by completing an in-depth inventory on the work being done by all of our faculty in leadership roles, we were able to standardize the compensation/FTE for each of these roles. Tracking these changes over time, via the use of dashboards in our case, allows for real-time measurements and accountability for all of those involved. Our end goal will be to have all of these initiatives feed into one large dashboard.
Collaborating with leadership and stakeholders in the DOM, SOM, and hospital helped to make our DEI initiatives successful. Much too often, we work in silos when it comes to DEI work. However, we tend to have similar goals and can achieve much more if we work together. Collaboration with multiple stakeholders allowed for wider dissemination and resulted in a larger impact to the campus and community at large. This has been exemplified by the committee composition guidance that has been utilized by the DOM, as well as implementation of campus-wide policies, specifically the parental leave policy, which our faculty members played an important role in creating. Likewise, it is important to look outside of our institutions and work with other hospital medicine groups around the country who are interested in promoting DEI.
We still have much work ahead of us. We are continuing to measure outcomes status postimplementation of the toolkit and checklists being used for diversity recruitment and committee composition. Additionally, we are actively working on several initiatives, including:
- Instituting implicit bias training for all of our faculty
- Partnering with national leaders and our hospital systems to develop zero-tolerance policies regarding abusive behaviors (verbal, physical, and other), racism, and sexism in the hospital and other work settings
- Development of specific recruitment strategies as a means of diversifying our healthcare workforce (of note, based on a 2020 survey of our faculty, in which there was a 70% response rate, 8.5% of our faculty identified as URMs)
- Completion of a diversity dashboard to track our progress in all of these efforts over time
- Development of a more robust pipeline to promotion and leadership for our URM faculty
This study has several strengths. Many of the plans and strategies described here can be used to guide others interested in implementing this work. Figure 2 provides a stepwise
approach to addressing DEI in hospital medicine groups and divisions. We conducted this work at a large academic medical center, and while it may not be generalizable, it does offer some ideas for others to consider in their own work to advance DEI at their institutions. There are also several limitations to this work. Eliminating salary inequities with our approach did take resources. We took advantage of already lower salaries and the need to increase salaries closer to benchmark and paired this effort with our DEI efforts to achieve salary equity. This required partnerships with the department and hospital. Efforts to advance DEI also take a lot of time and effort, and thus commitment from the division, department, and institution as a whole is key. While we have outcomes for our efforts related to salary equity, recruitment efforts should be realized over time, as currently it is too early to tell. We have highlighted the efforts that have been put in place at this time.
CONCLUSION
Using a systematic evidence-based approach with key stakeholder involvement, a division-wide DEI strategy was developed and implemented. While this work is still ongoing, short-term wins are possible, in particular around salary equity and development of policies and structures to promote DEI.
1. Underrepresented racial and ethnic groups. National Institutes of Health website. Accessed December 26, 2020. https://extramural-diversity.nih.gov/diversity-matters/underrepresented-groups
2. Ash AS, Carr PL, Goldstein R, Friedman RH. Compensation and advancement of women in academic medicine: is there equity? Ann Intern Med. 2004;141(3):205-212. https://doi.org/10.7326/0003-4819-141-3-200408030-00009
3. Jena AB, Khullar D, Ho O, Olenski AR, Blumenthal DM. Sex differences in academic rank in US medical schools in 2014. JAMA. 2015;314(11):1149-1158. https://doi.org/10.1001/jama.2015.10680
4. Fang D, Moy E, Colburn L, Hurley J. Racial and ethnic disparities in faculty promotion in academic medicine. JAMA. 2000;284(9):1085-1092. https://doi.org/10.1001/jama.284.9.1085
5. Baptiste D, Fecher AM, Dolejs SC, et al. Gender differences in academic surgery, work-life balance, and satisfaction. J Surg Res. 2017;218:99-107. https://doi.org/10.1016/j.jss.2017.05.075
6. Hart KL, Perlis RH. Trends in proportion of women as authors of medical journal articles, 2008-2018. JAMA Intern Med. 2019;179:1285-1287. https://doi.org/10.1001/jamainternmed.2019.0907
7. Thomas EG, Jayabalasingham B, Collins T, Geertzen J, Bui C, Dominici F. Gender disparities in invited commentary authorship in 2459 medical journals. JAMA Netw Open. 2019;2(10):e1913682. https://doi.org/10.1001/jamanetworkopen.2019.13682
8. Hechtman LA, Moore NP, Schulkey CE, et al. NIH funding longevity by gender. Proc Natl Acad Sci U S A. 2018;115(31):7943-7948. https://doi.org/10.1073/pnas.1800615115
9. Sege R, Nykiel-Bub L, Selk S. Sex differences in institutional support for junior biomedical researchers. JAMA. 2015;314(11):1175-1177. https://doi.org/10.1001/jama.2015.8517
10. Silver JK, Slocum CS, Bank AM, et al. Where are the women? The underrepresentation of women physicians among recognition award recipients from medical specialty societies. PM R. 2017;9(8):804-815. https://doi.org/10.1016/j.pmrj.2017.06.001
11. Ruzycki SM, Fletcher S, Earp M, Bharwani A, Lithgow KC. Trends in the proportion of female speakers at medical conferences in the United States and in Canada, 2007 to 2017. JAMA Netw Open. 2019;2(4):e192103. https://doi.org/10.1001/jamanetworkopen.2019.2103
12. Carr PL, Raj A, Kaplan SE, Terrin N, Breeze JL, Freund KM. Gender differences in academic medicine: retention, rank, and leadership comparisons from the National Faculty Survey. Acad Med. 2018;93(11):1694-1699. https://doi.org/10.1097/ACM.0000000000002146
13. Carr PL, Gunn C, Raj A, Kaplan S, Freund KM. Recruitment, promotion, and retention of women in academic medicine: how institutions are addressing gender disparities. Womens Health Issues. 2017;27(3):374-381. https://doi.org/10.1016/j.whi.2016.11.003
14. Jena AB, Olenski AR, Blumenthal DM. Sex differences in physician salary in US public medical schools. JAMA Intern Med. 2016;176(9):1294-1304. https://doi.org/10.1001/jamainternmed.2016.3284
15. Lo Sasso AT, Richards MR, Chou CF, Gerber SE. The $16,819 pay gap for newly trained physicians: the unexplained trend of men earning more than women. Health Aff (Millwood). 2011;30(2):193-201. https://doi.org/10.1377/hlthaff.2010.0597
16. Wachter RM, Goldman L. The emerging role of “hospitalists” in the American health care system. N Engl J Med. 1996;335(7):514-517. https://doi.org/10.1056/NEJM199608153350713
17. Weaver AC, Wetterneck TB, Whelan CT, Hinami K. A matter of priorities? Exploring the persistent gender pay gap in hospital medicine. J Hosp Med. 2015;10(8):486-490. https://doi.org/10.1002/jhm.2400
18. Burden M, Frank MG, Keniston A, et al. Gender disparities in leadership and scholarly productivity of academic hospitalists. J Hosp Med. 2015;10(8):481-485. https://doi.org/10.1002/jhm.2340
19. Northcutt N, Papp S, Keniston A, et al, Society of Hospital Medicine Diversity, Equity and Inclusion Special Interest Group. SPEAKers at the National Society of Hospital Medicine Meeting: a follow-up study of gender equity for conference speakers from 2015 to 2019. The SPEAK UP Study. J Hosp Med. 2020;15(4):228-231. https://doi.org/10.12788/jhm.3401
20. Shah SS, Shaughnessy EE, Spector ND. Leading by example: how medical journals can improve representation in academic medicine. J Hosp Med. 2019;14(7):393. https://doi.org/10.12788/jhm.3247
21. Shah SS, Shaughnessy EE, Spector ND. Promoting gender equity at the Journal of Hospital Medicine [editorial]. J Hosp Med. 2020;15(9):517. https://doi.org/10.12788/jhm.3522
22. Sheehy AM, Kolehmainen C, Carnes M. We specialize in change leadership: a call for hospitalists to lead the quest for workforce gender equity [editorial]. J Hosp Med. 2015;10(8):551-552. https://doi.org/10.1002/jhm.2399
23. Evans MK, Rosenbaum L, Malina D, Morrissey S, Rubin EJ. Diagnosing and treating systemic racism [editorial]. N Engl J Med. 2020;383(3):274-276. https://doi.org/10.1056/NEJMe2021693
24. Rock D, Grant H. Why diverse teams are smarter. Harvard Business Review. Published November 4, 2016. Accessed July 24, 2019. https://hbr.org/2016/11/why-diverse-teams-are-smarter
25. Johnson RL, Saha S, Arbelaez JJ, Beach MC, Cooper LA. Racial and ethnic differences in patient perceptions of bias and cultural competence in health care. J Gen Intern Med. 2004;19(2):101-110. https://doi.org/10.1111/j.1525-1497.2004.30262.x
26. Betancourt JR, Green AR, Carrillo JE, Park ER. Cultural competence and health care disparities: key perspectives and trends. Health Aff (Millwood). 2005;24(2):499-505. https://doi.org/10.1377/hlthaff.24.2.499
27. Acosta D, Ackerman-Barger K. Breaking the silence: time to talk about race and racism [comment]. Acad Med. 2017;92(3):285-288. https://doi.org/10.1097/ACM.0000000000001416
28. Cohen JJ, Gabriel BA, Terrell C. The case for diversity in the health care workforce. Health Aff (Millwood). 2002;21(5):90-102. https://doi.org/10.1377/hlthaff.21.5.90
29. Chang E, Simon M, Dong X. Integrating cultural humility into health care professional education and training. Adv Health Sci Educ Theory Pract. 2012;17(2):269-278. https://doi.org/10.1007/s10459-010-9264-1
30. Foronda C, Baptiste DL, Reinholdt MM, Ousman K. Cultural humility: a concept analysis. J Transcult Nurs. 2016;27(3):210-217. https://doi.org/10.1177/1043659615592677
31. Butkus R, Serchen J, Moyer DV, et al; Health and Public Policy Committee of the American College of Physicians. Achieving gender equity in physician compensation and career advancement: a position paper of the American College of Physicians. Ann Intern Med. 2018;168(10):721-723. https://doi.org/10.7326/M17-3438
32. Burden M, del Pino-Jones A, Shafer M, Sheth S, Rexrode K. GWIMS Equity Recruitment Toolkit. Accessed July 27, 2019. https://www.aamc.org/download/492864/data/equityinrecruitmenttoolkit.pdf
33. AAMC Faculty Salary Report. AAMC website. Accessed September 6, 2020. https://www.aamc.org/data-reports/workforce/report/aamc-faculty-salary-report
34. Promotion process. University of Colorado Anschutz Medical Campus website. Accessed September 7, 2020. https://medschool.cuanschutz.edu/faculty-affairs/for-faculty/promotion-and-tenure/promotion-process
35. Pierce RG, Diaz M, Kneeland P. Optimizing well-being, practice culture, and professional thriving in an era of turbulence. J Hosp Med. 2019;14(2):126-128. https://doi.org/10.12788/jhm.3101
Studies continue to demonstrate persistent gaps in equity for women and underrepresented minorities (URMs)1 throughout nearly all aspects of academic medicine, including rank,2-4 tenure,5 authorship,6,7 funding opportunities,8,9 awards,10 speakership,11 leadership,12,13 and salaries.2,14,15
In this article, we report DEI efforts within our division, focusing on the development of our strategic plan and specific outcomes related to compensation, recruitment, and policies.
METHODS
Our Division’s Framework to DEI—“It Takes a Village”
Our Division of Hospital Medicine (DHM), previously within the Division of General Internal Medicine, was founded in October 2017. The DHM at the University of Colorado Hospital (UCH) is composed of 100 faculty members (70 physicians and 30 advanced-practice providers; 58% women and 42% men). In 2018, we implemented a stepwise approach to critically assess DEI within our group and to build a strategic plan to address the issues.
Needs Assessment
As a new division, we sought stakeholder feedback from division members. All faculty within the division were invited to attend a meeting in which issues related to DEI were discussed. A literature review that spanned both medical and nonmedical fields was also completed. Search terms included salary equity, gender equity, diverse teams, diversity recruitment and retention, diversifying leadership, and diverse speakers. Salaries, internally funded time, and other processes, such as recruitment, promotion, and hiring for leadership positions, were evaluated during the first year we became a division.
Interventions
TThrough this work, and with stakeholder engagement, we developed a divisional strategic plan to address DEI globally. Our strategic plan included developing a DEI director role to assist with overseeing DEI efforts. We have highlighted the various methods utilized for each component (Figure 1). This work occurred from October 2017 to December 2018.
Our institutional structures
Using best practices from both medical and nonmedical fields, we developed evidence-based approaches to
Compensation: transparent and consistent approaches based upon benchmarking with a framework of equal pay for equal work and similar advanced training/academic rank. In conjunction with efforts within the School of Medicine (SOM), Department of Medicine (DOM), and the UCH, our division sought to study salaries across DHM faculty members. We had an open call for faculty to participate in a newly developed DHM Compensation Committee, with the intent of rigorously examining our compensation practices and goals. Through faculty feedback and committee work, salary equity was defined as equal pay (ie, base salary for one clinical full-time equivalent [FTE]) for equal work based on academic rank and/or years of practice/advanced training. We also compared DHM salaries to regional academic hospital medicine groups and concluded that DHM salaries were lower than local and national benchmarks. This information was used to create a two-phase approach to increasing salaries for all individuals below the American Association of Medical Colleges (AAMC) benchmarks33 for academic hospitalists. We also developed a stipend system for external roles that came with additional compensation and roles within our own division that came with additional pay (ie, nocturnist). Phase 1 focused on those whose salaries were furthest away from and below benchmark, and phase 2 targeted all remaining individuals below benchmark.
A similar review of FTEs (based on required number of shifts for a full-time hospitalist) tied to our internal DHM leadership positions was completed by the division head and director of DEI. Specifically, the mission for each of our internally funded roles, job descriptions, and responsibilities was reviewed to ensure equity in funding.
Recruitment and advancement: processes to ensure equity and diversity in recruitment, tracking, and reporting, working to eliminate/mitigate bias. In collaboration with members of the AAMC Group on Women in Medicine and Science (GWIMS) and coauthors from various institutions, we developed toolkits and checklists aimed at achieving equity and diversity within candidate pools and on major committees, including, but not limited to, search and promotion committees.32 Additionally, a checklist was developed to help recruit more diverse speakers, including women and URMs, for local, regional, and national conferences.
Policies: evidence-based approaches, tracking and reporting, standardized approaches to eliminate/mitigate bias, embracing nontraditional paths. In partnership with our departmental efforts, members of our team led data collection and reporting for salary benchmarking, leadership roles, and committee membership. This included developing surveys and reporting templates that can be used to identify disparities and inform future efforts. We worked to ensure that we have faculty representing our field at the department and SOM levels. Specifically, we made sure to nominate division members during open calls for departmental and schoolwide committees, including the promotions committee.
Our People
The faculty and staff within our division have been instrumental in moving efforts forward in the following important areas.
Leadership: develop the position of director of DEI as well as leadership structures to support and increase DEI. One of the first steps in our strategic plan was creating a director of DEI leadership role (Appendix Figure 2). The director is responsible for researching, applying, and promoting a broad scope of DEI initiatives and best practices within the DHM, DOM, and SOM (in collaboration with their leaders), including recruitment, retention, and promotion of medical students, residents, and faculty; educational program development; health disparities research; and community-engaged scholarship.
Support: develop family leave policies/develop flexible work policies. Several members of our division worked on departmental committees and served in leadership roles on staff and faculty council. Estimated costs were assessed. Through collective efforts of department leadership and division head support, the department approved parental leave to employees following the birth of an employee’s child or the placement of a child with an employee in connection with adoption or permanent foster care.
Mentorship/sponsorship: enhance faculty advancement programs/develop pipeline and trainings/collaborate with student groups and organizations/invest in all of our people. Faculty across our divisional sites have held important roles in developing pipeline programs for undergraduate students bound for health professions, as well as programs developed specifically for medical students and internal medicine residents. This includes two programs, the CU Hospitalist Scholars Program (CUHSP) and Leadership Education for Aspiring Doctors (LEAD), in which undergraduate students have the opportunity to round with hospital medicine teams, work on quality-improvement projects, and receive extensive mentorship and advising from a diverse faculty team. Additionally, our faculty advancement team within the DHM has grown and been restructured to include more defined goals and to ensure each faculty member has at least one mentor in their area of interest.
Supportive: lactation space and support/diverse space options/inclusive and diverse environments. We worked closely with hospital leadership to advocate for adequately equipped lactation spaces, including equipment such as pumps, refrigerators, and computer workstations.
Measures
Our measures focused on (1) development and implementation of our DEI strategic plan, including new policies, processes, and practices related to key components of the DEI program; and (2) assessment of specific DEI programs, including pre-post salary data disparities based on rank and pre-post disparities for protected time for similar roles.
Analysis
Through rapid PDSA cycles, we evaluated salary equity, equity in leadership allotment, and committee membership. We have developed a tracking board to track progress of the multiple projects in the strategic plan.
RESULTS
Strategic Plan Development and Tracking
From October 2017 to December 2018, we developed a robust strategic plan and stepwise approach to DEI (Figure 1 and Figure 2). The director of DEI position was developed (see Appendix Figure 2 for job description) to help oversee these efforts. Figure 3 highlights the specific efforts and the progress made on implementation (ie, high-level dashboard or “tracking board”). While outcomes are still pending in the areas of recruitment and advancement and environment, we have made measurable improvements in compensation, as outlined in the following section.
Compensation
One year after the salary-equity interventions, all of our physician faculty’s salaries were at the goal benchmark (Table), and differences in salary for those in similar years of rank were nearly eliminated. Similarly, after implementing an internally consistent approach to assigning FTE for new and established positions within the division (ie, those that fall within the purview of the division), all faculty in similar types of roles had similar amounts of protected time.
Recruitment and Advancement
Toolkits32 and committee recommendations have been incorporated into division goals, though some aspects are still in implementation phases, as division-wide implicit bias training was delayed secondary to the COVID-19 pandemic. Key goals include: (1) implicit bias training for all members of major committees; (2) aiming for a goal of at least 40% representation of women and 40% URMs on committees; (3) having a diversity expert serve on each committee in order to identify and discuss any potential bias in the search and candidate-selection processes; and (4) careful tracking of diversity metrics in regard to diversity of candidates at each step of the interview and selection process.
Surveys and reporting templates for equity on committees and leadership positions have been developed and deployed. Data dashboards for our division have been developed as well (for compensation, leadership, and committee membership). A divisional dashboard to report recruitment efforts is in progress.
We have successfully nominated several faculty members to the SOM promotions committee and departmental committees during open calls for these positions. At the division level, we have also adapted internal policies to ensure promotion occurs on time and offers alternative pathways for faculty that may primarily focus on clinical pathways. All faculty who have gone up for promotion thus far have been successfully promoted in their desired pathway.
Environment
We successfully advocated and achieved adequately equipped lactation spaces, including equipment such as pumps, refrigerators, and computer workstations. This achievement was possible because of our hospital partners. Our efforts helped us acquire sufficient space and facilities such that nursing mothers can pump and still be able to answer phones, enter orders, and document visits.
Our team members conducted environmental scans and raised concerns when the environment was not inclusive, such as conference rooms with portraits of leadership that do not show diversity. The all-male pictures were removed from one frequently used departmental conference room, which will eventually house a diverse group of pictures and achievements.
We aim to eliminate bias by offering implicit bias training for our faculty. While this is presently required for those who serve on committees, in leadership positions, or those involved in recruitment and interviewing for the DOM, our goal is to eventually provide this training to all faculty and staff in the division. We have also incorporated DEI topics into our educational conferences for faculty, including sessions on recognizing bias in medicine, how to be an upstander/ally, and the impact of race and racism on medicine.
DISCUSSION
The important findings of this work are: (1) that successes in DEI can be achieved with strategic planning and stakeholder engagement; (2) through simple modification of processes, we can improve equity in compensation and FTE allotted to leadership; (3) though it takes time, diversity recruitment can be improved using sound, sustainable, evidence-based processes; (4) this work is time-intensive and challenging, requiring ongoing efforts to improve, modify, and enhance current efforts and future successes.
We have certainly made some progress with DEI initiatives within our division and have also learned a great deal from this experience. First, change is difficult for all parties involved, including those leading change and those affected by the changes. We purposely made an effort to facilitate discussions with all of the DHM faculty and staff to ensure that everyone felt included in this work and that everyone’s voice was heard. This was exemplified by inviting all faculty members to a feedback session in which we discussed DEI within our division and areas that we wanted to improve on. Early on, we were able to define what diversity, equity, and inclusion meant to us as a division and then use these definitions to develop tangible goals for all the areas of highest importance to the group.
By increasing faculty presence on key committees, such as the promotions committee, we now have faculty members who are well versed in promotions processes. We are fortunate to have a promotions process that supports faculty advancement for faculty with diverse interests that spans from supporting highly clinical faculty, clinician educators, as well as more traditional researchers.34 By having hospitalists serve in these roles, we help to add to the diverse perspectives on these committees, including emphasizing the scholarship that is associated with quality improvement, as well as DEI efforts which can often be viewed as service as opposed to scholarship.
Clear communication and transparency were key to all of our DEI initiatives. We had monthly updates on our DEI efforts during business meetings and also held impromptu meetings (also known as flash mobs35) to answer questions and discuss concerns in real time. As with all DEI work, it is important to know where you are starting (having accurate data and a clear understanding of the data) and be able to communicate that data to the group. For example, using AAMC salary benchmarking33 as well as other benchmarks allowed us to accurately calculate variance among salaries and identify the appropriate goal salary for each of our faculty members. Likewise, by completing an in-depth inventory on the work being done by all of our faculty in leadership roles, we were able to standardize the compensation/FTE for each of these roles. Tracking these changes over time, via the use of dashboards in our case, allows for real-time measurements and accountability for all of those involved. Our end goal will be to have all of these initiatives feed into one large dashboard.
Collaborating with leadership and stakeholders in the DOM, SOM, and hospital helped to make our DEI initiatives successful. Much too often, we work in silos when it comes to DEI work. However, we tend to have similar goals and can achieve much more if we work together. Collaboration with multiple stakeholders allowed for wider dissemination and resulted in a larger impact to the campus and community at large. This has been exemplified by the committee composition guidance that has been utilized by the DOM, as well as implementation of campus-wide policies, specifically the parental leave policy, which our faculty members played an important role in creating. Likewise, it is important to look outside of our institutions and work with other hospital medicine groups around the country who are interested in promoting DEI.
We still have much work ahead of us. We are continuing to measure outcomes status postimplementation of the toolkit and checklists being used for diversity recruitment and committee composition. Additionally, we are actively working on several initiatives, including:
- Instituting implicit bias training for all of our faculty
- Partnering with national leaders and our hospital systems to develop zero-tolerance policies regarding abusive behaviors (verbal, physical, and other), racism, and sexism in the hospital and other work settings
- Development of specific recruitment strategies as a means of diversifying our healthcare workforce (of note, based on a 2020 survey of our faculty, in which there was a 70% response rate, 8.5% of our faculty identified as URMs)
- Completion of a diversity dashboard to track our progress in all of these efforts over time
- Development of a more robust pipeline to promotion and leadership for our URM faculty
This study has several strengths. Many of the plans and strategies described here can be used to guide others interested in implementing this work. Figure 2 provides a stepwise
approach to addressing DEI in hospital medicine groups and divisions. We conducted this work at a large academic medical center, and while it may not be generalizable, it does offer some ideas for others to consider in their own work to advance DEI at their institutions. There are also several limitations to this work. Eliminating salary inequities with our approach did take resources. We took advantage of already lower salaries and the need to increase salaries closer to benchmark and paired this effort with our DEI efforts to achieve salary equity. This required partnerships with the department and hospital. Efforts to advance DEI also take a lot of time and effort, and thus commitment from the division, department, and institution as a whole is key. While we have outcomes for our efforts related to salary equity, recruitment efforts should be realized over time, as currently it is too early to tell. We have highlighted the efforts that have been put in place at this time.
CONCLUSION
Using a systematic evidence-based approach with key stakeholder involvement, a division-wide DEI strategy was developed and implemented. While this work is still ongoing, short-term wins are possible, in particular around salary equity and development of policies and structures to promote DEI.
Studies continue to demonstrate persistent gaps in equity for women and underrepresented minorities (URMs)1 throughout nearly all aspects of academic medicine, including rank,2-4 tenure,5 authorship,6,7 funding opportunities,8,9 awards,10 speakership,11 leadership,12,13 and salaries.2,14,15
In this article, we report DEI efforts within our division, focusing on the development of our strategic plan and specific outcomes related to compensation, recruitment, and policies.
METHODS
Our Division’s Framework to DEI—“It Takes a Village”
Our Division of Hospital Medicine (DHM), previously within the Division of General Internal Medicine, was founded in October 2017. The DHM at the University of Colorado Hospital (UCH) is composed of 100 faculty members (70 physicians and 30 advanced-practice providers; 58% women and 42% men). In 2018, we implemented a stepwise approach to critically assess DEI within our group and to build a strategic plan to address the issues.
Needs Assessment
As a new division, we sought stakeholder feedback from division members. All faculty within the division were invited to attend a meeting in which issues related to DEI were discussed. A literature review that spanned both medical and nonmedical fields was also completed. Search terms included salary equity, gender equity, diverse teams, diversity recruitment and retention, diversifying leadership, and diverse speakers. Salaries, internally funded time, and other processes, such as recruitment, promotion, and hiring for leadership positions, were evaluated during the first year we became a division.
Interventions
TThrough this work, and with stakeholder engagement, we developed a divisional strategic plan to address DEI globally. Our strategic plan included developing a DEI director role to assist with overseeing DEI efforts. We have highlighted the various methods utilized for each component (Figure 1). This work occurred from October 2017 to December 2018.
Our institutional structures
Using best practices from both medical and nonmedical fields, we developed evidence-based approaches to
Compensation: transparent and consistent approaches based upon benchmarking with a framework of equal pay for equal work and similar advanced training/academic rank. In conjunction with efforts within the School of Medicine (SOM), Department of Medicine (DOM), and the UCH, our division sought to study salaries across DHM faculty members. We had an open call for faculty to participate in a newly developed DHM Compensation Committee, with the intent of rigorously examining our compensation practices and goals. Through faculty feedback and committee work, salary equity was defined as equal pay (ie, base salary for one clinical full-time equivalent [FTE]) for equal work based on academic rank and/or years of practice/advanced training. We also compared DHM salaries to regional academic hospital medicine groups and concluded that DHM salaries were lower than local and national benchmarks. This information was used to create a two-phase approach to increasing salaries for all individuals below the American Association of Medical Colleges (AAMC) benchmarks33 for academic hospitalists. We also developed a stipend system for external roles that came with additional compensation and roles within our own division that came with additional pay (ie, nocturnist). Phase 1 focused on those whose salaries were furthest away from and below benchmark, and phase 2 targeted all remaining individuals below benchmark.
A similar review of FTEs (based on required number of shifts for a full-time hospitalist) tied to our internal DHM leadership positions was completed by the division head and director of DEI. Specifically, the mission for each of our internally funded roles, job descriptions, and responsibilities was reviewed to ensure equity in funding.
Recruitment and advancement: processes to ensure equity and diversity in recruitment, tracking, and reporting, working to eliminate/mitigate bias. In collaboration with members of the AAMC Group on Women in Medicine and Science (GWIMS) and coauthors from various institutions, we developed toolkits and checklists aimed at achieving equity and diversity within candidate pools and on major committees, including, but not limited to, search and promotion committees.32 Additionally, a checklist was developed to help recruit more diverse speakers, including women and URMs, for local, regional, and national conferences.
Policies: evidence-based approaches, tracking and reporting, standardized approaches to eliminate/mitigate bias, embracing nontraditional paths. In partnership with our departmental efforts, members of our team led data collection and reporting for salary benchmarking, leadership roles, and committee membership. This included developing surveys and reporting templates that can be used to identify disparities and inform future efforts. We worked to ensure that we have faculty representing our field at the department and SOM levels. Specifically, we made sure to nominate division members during open calls for departmental and schoolwide committees, including the promotions committee.
Our People
The faculty and staff within our division have been instrumental in moving efforts forward in the following important areas.
Leadership: develop the position of director of DEI as well as leadership structures to support and increase DEI. One of the first steps in our strategic plan was creating a director of DEI leadership role (Appendix Figure 2). The director is responsible for researching, applying, and promoting a broad scope of DEI initiatives and best practices within the DHM, DOM, and SOM (in collaboration with their leaders), including recruitment, retention, and promotion of medical students, residents, and faculty; educational program development; health disparities research; and community-engaged scholarship.
Support: develop family leave policies/develop flexible work policies. Several members of our division worked on departmental committees and served in leadership roles on staff and faculty council. Estimated costs were assessed. Through collective efforts of department leadership and division head support, the department approved parental leave to employees following the birth of an employee’s child or the placement of a child with an employee in connection with adoption or permanent foster care.
Mentorship/sponsorship: enhance faculty advancement programs/develop pipeline and trainings/collaborate with student groups and organizations/invest in all of our people. Faculty across our divisional sites have held important roles in developing pipeline programs for undergraduate students bound for health professions, as well as programs developed specifically for medical students and internal medicine residents. This includes two programs, the CU Hospitalist Scholars Program (CUHSP) and Leadership Education for Aspiring Doctors (LEAD), in which undergraduate students have the opportunity to round with hospital medicine teams, work on quality-improvement projects, and receive extensive mentorship and advising from a diverse faculty team. Additionally, our faculty advancement team within the DHM has grown and been restructured to include more defined goals and to ensure each faculty member has at least one mentor in their area of interest.
Supportive: lactation space and support/diverse space options/inclusive and diverse environments. We worked closely with hospital leadership to advocate for adequately equipped lactation spaces, including equipment such as pumps, refrigerators, and computer workstations.
Measures
Our measures focused on (1) development and implementation of our DEI strategic plan, including new policies, processes, and practices related to key components of the DEI program; and (2) assessment of specific DEI programs, including pre-post salary data disparities based on rank and pre-post disparities for protected time for similar roles.
Analysis
Through rapid PDSA cycles, we evaluated salary equity, equity in leadership allotment, and committee membership. We have developed a tracking board to track progress of the multiple projects in the strategic plan.
RESULTS
Strategic Plan Development and Tracking
From October 2017 to December 2018, we developed a robust strategic plan and stepwise approach to DEI (Figure 1 and Figure 2). The director of DEI position was developed (see Appendix Figure 2 for job description) to help oversee these efforts. Figure 3 highlights the specific efforts and the progress made on implementation (ie, high-level dashboard or “tracking board”). While outcomes are still pending in the areas of recruitment and advancement and environment, we have made measurable improvements in compensation, as outlined in the following section.
Compensation
One year after the salary-equity interventions, all of our physician faculty’s salaries were at the goal benchmark (Table), and differences in salary for those in similar years of rank were nearly eliminated. Similarly, after implementing an internally consistent approach to assigning FTE for new and established positions within the division (ie, those that fall within the purview of the division), all faculty in similar types of roles had similar amounts of protected time.
Recruitment and Advancement
Toolkits32 and committee recommendations have been incorporated into division goals, though some aspects are still in implementation phases, as division-wide implicit bias training was delayed secondary to the COVID-19 pandemic. Key goals include: (1) implicit bias training for all members of major committees; (2) aiming for a goal of at least 40% representation of women and 40% URMs on committees; (3) having a diversity expert serve on each committee in order to identify and discuss any potential bias in the search and candidate-selection processes; and (4) careful tracking of diversity metrics in regard to diversity of candidates at each step of the interview and selection process.
Surveys and reporting templates for equity on committees and leadership positions have been developed and deployed. Data dashboards for our division have been developed as well (for compensation, leadership, and committee membership). A divisional dashboard to report recruitment efforts is in progress.
We have successfully nominated several faculty members to the SOM promotions committee and departmental committees during open calls for these positions. At the division level, we have also adapted internal policies to ensure promotion occurs on time and offers alternative pathways for faculty that may primarily focus on clinical pathways. All faculty who have gone up for promotion thus far have been successfully promoted in their desired pathway.
Environment
We successfully advocated and achieved adequately equipped lactation spaces, including equipment such as pumps, refrigerators, and computer workstations. This achievement was possible because of our hospital partners. Our efforts helped us acquire sufficient space and facilities such that nursing mothers can pump and still be able to answer phones, enter orders, and document visits.
Our team members conducted environmental scans and raised concerns when the environment was not inclusive, such as conference rooms with portraits of leadership that do not show diversity. The all-male pictures were removed from one frequently used departmental conference room, which will eventually house a diverse group of pictures and achievements.
We aim to eliminate bias by offering implicit bias training for our faculty. While this is presently required for those who serve on committees, in leadership positions, or those involved in recruitment and interviewing for the DOM, our goal is to eventually provide this training to all faculty and staff in the division. We have also incorporated DEI topics into our educational conferences for faculty, including sessions on recognizing bias in medicine, how to be an upstander/ally, and the impact of race and racism on medicine.
DISCUSSION
The important findings of this work are: (1) that successes in DEI can be achieved with strategic planning and stakeholder engagement; (2) through simple modification of processes, we can improve equity in compensation and FTE allotted to leadership; (3) though it takes time, diversity recruitment can be improved using sound, sustainable, evidence-based processes; (4) this work is time-intensive and challenging, requiring ongoing efforts to improve, modify, and enhance current efforts and future successes.
We have certainly made some progress with DEI initiatives within our division and have also learned a great deal from this experience. First, change is difficult for all parties involved, including those leading change and those affected by the changes. We purposely made an effort to facilitate discussions with all of the DHM faculty and staff to ensure that everyone felt included in this work and that everyone’s voice was heard. This was exemplified by inviting all faculty members to a feedback session in which we discussed DEI within our division and areas that we wanted to improve on. Early on, we were able to define what diversity, equity, and inclusion meant to us as a division and then use these definitions to develop tangible goals for all the areas of highest importance to the group.
By increasing faculty presence on key committees, such as the promotions committee, we now have faculty members who are well versed in promotions processes. We are fortunate to have a promotions process that supports faculty advancement for faculty with diverse interests that spans from supporting highly clinical faculty, clinician educators, as well as more traditional researchers.34 By having hospitalists serve in these roles, we help to add to the diverse perspectives on these committees, including emphasizing the scholarship that is associated with quality improvement, as well as DEI efforts which can often be viewed as service as opposed to scholarship.
Clear communication and transparency were key to all of our DEI initiatives. We had monthly updates on our DEI efforts during business meetings and also held impromptu meetings (also known as flash mobs35) to answer questions and discuss concerns in real time. As with all DEI work, it is important to know where you are starting (having accurate data and a clear understanding of the data) and be able to communicate that data to the group. For example, using AAMC salary benchmarking33 as well as other benchmarks allowed us to accurately calculate variance among salaries and identify the appropriate goal salary for each of our faculty members. Likewise, by completing an in-depth inventory on the work being done by all of our faculty in leadership roles, we were able to standardize the compensation/FTE for each of these roles. Tracking these changes over time, via the use of dashboards in our case, allows for real-time measurements and accountability for all of those involved. Our end goal will be to have all of these initiatives feed into one large dashboard.
Collaborating with leadership and stakeholders in the DOM, SOM, and hospital helped to make our DEI initiatives successful. Much too often, we work in silos when it comes to DEI work. However, we tend to have similar goals and can achieve much more if we work together. Collaboration with multiple stakeholders allowed for wider dissemination and resulted in a larger impact to the campus and community at large. This has been exemplified by the committee composition guidance that has been utilized by the DOM, as well as implementation of campus-wide policies, specifically the parental leave policy, which our faculty members played an important role in creating. Likewise, it is important to look outside of our institutions and work with other hospital medicine groups around the country who are interested in promoting DEI.
We still have much work ahead of us. We are continuing to measure outcomes status postimplementation of the toolkit and checklists being used for diversity recruitment and committee composition. Additionally, we are actively working on several initiatives, including:
- Instituting implicit bias training for all of our faculty
- Partnering with national leaders and our hospital systems to develop zero-tolerance policies regarding abusive behaviors (verbal, physical, and other), racism, and sexism in the hospital and other work settings
- Development of specific recruitment strategies as a means of diversifying our healthcare workforce (of note, based on a 2020 survey of our faculty, in which there was a 70% response rate, 8.5% of our faculty identified as URMs)
- Completion of a diversity dashboard to track our progress in all of these efforts over time
- Development of a more robust pipeline to promotion and leadership for our URM faculty
This study has several strengths. Many of the plans and strategies described here can be used to guide others interested in implementing this work. Figure 2 provides a stepwise
approach to addressing DEI in hospital medicine groups and divisions. We conducted this work at a large academic medical center, and while it may not be generalizable, it does offer some ideas for others to consider in their own work to advance DEI at their institutions. There are also several limitations to this work. Eliminating salary inequities with our approach did take resources. We took advantage of already lower salaries and the need to increase salaries closer to benchmark and paired this effort with our DEI efforts to achieve salary equity. This required partnerships with the department and hospital. Efforts to advance DEI also take a lot of time and effort, and thus commitment from the division, department, and institution as a whole is key. While we have outcomes for our efforts related to salary equity, recruitment efforts should be realized over time, as currently it is too early to tell. We have highlighted the efforts that have been put in place at this time.
CONCLUSION
Using a systematic evidence-based approach with key stakeholder involvement, a division-wide DEI strategy was developed and implemented. While this work is still ongoing, short-term wins are possible, in particular around salary equity and development of policies and structures to promote DEI.
1. Underrepresented racial and ethnic groups. National Institutes of Health website. Accessed December 26, 2020. https://extramural-diversity.nih.gov/diversity-matters/underrepresented-groups
2. Ash AS, Carr PL, Goldstein R, Friedman RH. Compensation and advancement of women in academic medicine: is there equity? Ann Intern Med. 2004;141(3):205-212. https://doi.org/10.7326/0003-4819-141-3-200408030-00009
3. Jena AB, Khullar D, Ho O, Olenski AR, Blumenthal DM. Sex differences in academic rank in US medical schools in 2014. JAMA. 2015;314(11):1149-1158. https://doi.org/10.1001/jama.2015.10680
4. Fang D, Moy E, Colburn L, Hurley J. Racial and ethnic disparities in faculty promotion in academic medicine. JAMA. 2000;284(9):1085-1092. https://doi.org/10.1001/jama.284.9.1085
5. Baptiste D, Fecher AM, Dolejs SC, et al. Gender differences in academic surgery, work-life balance, and satisfaction. J Surg Res. 2017;218:99-107. https://doi.org/10.1016/j.jss.2017.05.075
6. Hart KL, Perlis RH. Trends in proportion of women as authors of medical journal articles, 2008-2018. JAMA Intern Med. 2019;179:1285-1287. https://doi.org/10.1001/jamainternmed.2019.0907
7. Thomas EG, Jayabalasingham B, Collins T, Geertzen J, Bui C, Dominici F. Gender disparities in invited commentary authorship in 2459 medical journals. JAMA Netw Open. 2019;2(10):e1913682. https://doi.org/10.1001/jamanetworkopen.2019.13682
8. Hechtman LA, Moore NP, Schulkey CE, et al. NIH funding longevity by gender. Proc Natl Acad Sci U S A. 2018;115(31):7943-7948. https://doi.org/10.1073/pnas.1800615115
9. Sege R, Nykiel-Bub L, Selk S. Sex differences in institutional support for junior biomedical researchers. JAMA. 2015;314(11):1175-1177. https://doi.org/10.1001/jama.2015.8517
10. Silver JK, Slocum CS, Bank AM, et al. Where are the women? The underrepresentation of women physicians among recognition award recipients from medical specialty societies. PM R. 2017;9(8):804-815. https://doi.org/10.1016/j.pmrj.2017.06.001
11. Ruzycki SM, Fletcher S, Earp M, Bharwani A, Lithgow KC. Trends in the proportion of female speakers at medical conferences in the United States and in Canada, 2007 to 2017. JAMA Netw Open. 2019;2(4):e192103. https://doi.org/10.1001/jamanetworkopen.2019.2103
12. Carr PL, Raj A, Kaplan SE, Terrin N, Breeze JL, Freund KM. Gender differences in academic medicine: retention, rank, and leadership comparisons from the National Faculty Survey. Acad Med. 2018;93(11):1694-1699. https://doi.org/10.1097/ACM.0000000000002146
13. Carr PL, Gunn C, Raj A, Kaplan S, Freund KM. Recruitment, promotion, and retention of women in academic medicine: how institutions are addressing gender disparities. Womens Health Issues. 2017;27(3):374-381. https://doi.org/10.1016/j.whi.2016.11.003
14. Jena AB, Olenski AR, Blumenthal DM. Sex differences in physician salary in US public medical schools. JAMA Intern Med. 2016;176(9):1294-1304. https://doi.org/10.1001/jamainternmed.2016.3284
15. Lo Sasso AT, Richards MR, Chou CF, Gerber SE. The $16,819 pay gap for newly trained physicians: the unexplained trend of men earning more than women. Health Aff (Millwood). 2011;30(2):193-201. https://doi.org/10.1377/hlthaff.2010.0597
16. Wachter RM, Goldman L. The emerging role of “hospitalists” in the American health care system. N Engl J Med. 1996;335(7):514-517. https://doi.org/10.1056/NEJM199608153350713
17. Weaver AC, Wetterneck TB, Whelan CT, Hinami K. A matter of priorities? Exploring the persistent gender pay gap in hospital medicine. J Hosp Med. 2015;10(8):486-490. https://doi.org/10.1002/jhm.2400
18. Burden M, Frank MG, Keniston A, et al. Gender disparities in leadership and scholarly productivity of academic hospitalists. J Hosp Med. 2015;10(8):481-485. https://doi.org/10.1002/jhm.2340
19. Northcutt N, Papp S, Keniston A, et al, Society of Hospital Medicine Diversity, Equity and Inclusion Special Interest Group. SPEAKers at the National Society of Hospital Medicine Meeting: a follow-up study of gender equity for conference speakers from 2015 to 2019. The SPEAK UP Study. J Hosp Med. 2020;15(4):228-231. https://doi.org/10.12788/jhm.3401
20. Shah SS, Shaughnessy EE, Spector ND. Leading by example: how medical journals can improve representation in academic medicine. J Hosp Med. 2019;14(7):393. https://doi.org/10.12788/jhm.3247
21. Shah SS, Shaughnessy EE, Spector ND. Promoting gender equity at the Journal of Hospital Medicine [editorial]. J Hosp Med. 2020;15(9):517. https://doi.org/10.12788/jhm.3522
22. Sheehy AM, Kolehmainen C, Carnes M. We specialize in change leadership: a call for hospitalists to lead the quest for workforce gender equity [editorial]. J Hosp Med. 2015;10(8):551-552. https://doi.org/10.1002/jhm.2399
23. Evans MK, Rosenbaum L, Malina D, Morrissey S, Rubin EJ. Diagnosing and treating systemic racism [editorial]. N Engl J Med. 2020;383(3):274-276. https://doi.org/10.1056/NEJMe2021693
24. Rock D, Grant H. Why diverse teams are smarter. Harvard Business Review. Published November 4, 2016. Accessed July 24, 2019. https://hbr.org/2016/11/why-diverse-teams-are-smarter
25. Johnson RL, Saha S, Arbelaez JJ, Beach MC, Cooper LA. Racial and ethnic differences in patient perceptions of bias and cultural competence in health care. J Gen Intern Med. 2004;19(2):101-110. https://doi.org/10.1111/j.1525-1497.2004.30262.x
26. Betancourt JR, Green AR, Carrillo JE, Park ER. Cultural competence and health care disparities: key perspectives and trends. Health Aff (Millwood). 2005;24(2):499-505. https://doi.org/10.1377/hlthaff.24.2.499
27. Acosta D, Ackerman-Barger K. Breaking the silence: time to talk about race and racism [comment]. Acad Med. 2017;92(3):285-288. https://doi.org/10.1097/ACM.0000000000001416
28. Cohen JJ, Gabriel BA, Terrell C. The case for diversity in the health care workforce. Health Aff (Millwood). 2002;21(5):90-102. https://doi.org/10.1377/hlthaff.21.5.90
29. Chang E, Simon M, Dong X. Integrating cultural humility into health care professional education and training. Adv Health Sci Educ Theory Pract. 2012;17(2):269-278. https://doi.org/10.1007/s10459-010-9264-1
30. Foronda C, Baptiste DL, Reinholdt MM, Ousman K. Cultural humility: a concept analysis. J Transcult Nurs. 2016;27(3):210-217. https://doi.org/10.1177/1043659615592677
31. Butkus R, Serchen J, Moyer DV, et al; Health and Public Policy Committee of the American College of Physicians. Achieving gender equity in physician compensation and career advancement: a position paper of the American College of Physicians. Ann Intern Med. 2018;168(10):721-723. https://doi.org/10.7326/M17-3438
32. Burden M, del Pino-Jones A, Shafer M, Sheth S, Rexrode K. GWIMS Equity Recruitment Toolkit. Accessed July 27, 2019. https://www.aamc.org/download/492864/data/equityinrecruitmenttoolkit.pdf
33. AAMC Faculty Salary Report. AAMC website. Accessed September 6, 2020. https://www.aamc.org/data-reports/workforce/report/aamc-faculty-salary-report
34. Promotion process. University of Colorado Anschutz Medical Campus website. Accessed September 7, 2020. https://medschool.cuanschutz.edu/faculty-affairs/for-faculty/promotion-and-tenure/promotion-process
35. Pierce RG, Diaz M, Kneeland P. Optimizing well-being, practice culture, and professional thriving in an era of turbulence. J Hosp Med. 2019;14(2):126-128. https://doi.org/10.12788/jhm.3101
1. Underrepresented racial and ethnic groups. National Institutes of Health website. Accessed December 26, 2020. https://extramural-diversity.nih.gov/diversity-matters/underrepresented-groups
2. Ash AS, Carr PL, Goldstein R, Friedman RH. Compensation and advancement of women in academic medicine: is there equity? Ann Intern Med. 2004;141(3):205-212. https://doi.org/10.7326/0003-4819-141-3-200408030-00009
3. Jena AB, Khullar D, Ho O, Olenski AR, Blumenthal DM. Sex differences in academic rank in US medical schools in 2014. JAMA. 2015;314(11):1149-1158. https://doi.org/10.1001/jama.2015.10680
4. Fang D, Moy E, Colburn L, Hurley J. Racial and ethnic disparities in faculty promotion in academic medicine. JAMA. 2000;284(9):1085-1092. https://doi.org/10.1001/jama.284.9.1085
5. Baptiste D, Fecher AM, Dolejs SC, et al. Gender differences in academic surgery, work-life balance, and satisfaction. J Surg Res. 2017;218:99-107. https://doi.org/10.1016/j.jss.2017.05.075
6. Hart KL, Perlis RH. Trends in proportion of women as authors of medical journal articles, 2008-2018. JAMA Intern Med. 2019;179:1285-1287. https://doi.org/10.1001/jamainternmed.2019.0907
7. Thomas EG, Jayabalasingham B, Collins T, Geertzen J, Bui C, Dominici F. Gender disparities in invited commentary authorship in 2459 medical journals. JAMA Netw Open. 2019;2(10):e1913682. https://doi.org/10.1001/jamanetworkopen.2019.13682
8. Hechtman LA, Moore NP, Schulkey CE, et al. NIH funding longevity by gender. Proc Natl Acad Sci U S A. 2018;115(31):7943-7948. https://doi.org/10.1073/pnas.1800615115
9. Sege R, Nykiel-Bub L, Selk S. Sex differences in institutional support for junior biomedical researchers. JAMA. 2015;314(11):1175-1177. https://doi.org/10.1001/jama.2015.8517
10. Silver JK, Slocum CS, Bank AM, et al. Where are the women? The underrepresentation of women physicians among recognition award recipients from medical specialty societies. PM R. 2017;9(8):804-815. https://doi.org/10.1016/j.pmrj.2017.06.001
11. Ruzycki SM, Fletcher S, Earp M, Bharwani A, Lithgow KC. Trends in the proportion of female speakers at medical conferences in the United States and in Canada, 2007 to 2017. JAMA Netw Open. 2019;2(4):e192103. https://doi.org/10.1001/jamanetworkopen.2019.2103
12. Carr PL, Raj A, Kaplan SE, Terrin N, Breeze JL, Freund KM. Gender differences in academic medicine: retention, rank, and leadership comparisons from the National Faculty Survey. Acad Med. 2018;93(11):1694-1699. https://doi.org/10.1097/ACM.0000000000002146
13. Carr PL, Gunn C, Raj A, Kaplan S, Freund KM. Recruitment, promotion, and retention of women in academic medicine: how institutions are addressing gender disparities. Womens Health Issues. 2017;27(3):374-381. https://doi.org/10.1016/j.whi.2016.11.003
14. Jena AB, Olenski AR, Blumenthal DM. Sex differences in physician salary in US public medical schools. JAMA Intern Med. 2016;176(9):1294-1304. https://doi.org/10.1001/jamainternmed.2016.3284
15. Lo Sasso AT, Richards MR, Chou CF, Gerber SE. The $16,819 pay gap for newly trained physicians: the unexplained trend of men earning more than women. Health Aff (Millwood). 2011;30(2):193-201. https://doi.org/10.1377/hlthaff.2010.0597
16. Wachter RM, Goldman L. The emerging role of “hospitalists” in the American health care system. N Engl J Med. 1996;335(7):514-517. https://doi.org/10.1056/NEJM199608153350713
17. Weaver AC, Wetterneck TB, Whelan CT, Hinami K. A matter of priorities? Exploring the persistent gender pay gap in hospital medicine. J Hosp Med. 2015;10(8):486-490. https://doi.org/10.1002/jhm.2400
18. Burden M, Frank MG, Keniston A, et al. Gender disparities in leadership and scholarly productivity of academic hospitalists. J Hosp Med. 2015;10(8):481-485. https://doi.org/10.1002/jhm.2340
19. Northcutt N, Papp S, Keniston A, et al, Society of Hospital Medicine Diversity, Equity and Inclusion Special Interest Group. SPEAKers at the National Society of Hospital Medicine Meeting: a follow-up study of gender equity for conference speakers from 2015 to 2019. The SPEAK UP Study. J Hosp Med. 2020;15(4):228-231. https://doi.org/10.12788/jhm.3401
20. Shah SS, Shaughnessy EE, Spector ND. Leading by example: how medical journals can improve representation in academic medicine. J Hosp Med. 2019;14(7):393. https://doi.org/10.12788/jhm.3247
21. Shah SS, Shaughnessy EE, Spector ND. Promoting gender equity at the Journal of Hospital Medicine [editorial]. J Hosp Med. 2020;15(9):517. https://doi.org/10.12788/jhm.3522
22. Sheehy AM, Kolehmainen C, Carnes M. We specialize in change leadership: a call for hospitalists to lead the quest for workforce gender equity [editorial]. J Hosp Med. 2015;10(8):551-552. https://doi.org/10.1002/jhm.2399
23. Evans MK, Rosenbaum L, Malina D, Morrissey S, Rubin EJ. Diagnosing and treating systemic racism [editorial]. N Engl J Med. 2020;383(3):274-276. https://doi.org/10.1056/NEJMe2021693
24. Rock D, Grant H. Why diverse teams are smarter. Harvard Business Review. Published November 4, 2016. Accessed July 24, 2019. https://hbr.org/2016/11/why-diverse-teams-are-smarter
25. Johnson RL, Saha S, Arbelaez JJ, Beach MC, Cooper LA. Racial and ethnic differences in patient perceptions of bias and cultural competence in health care. J Gen Intern Med. 2004;19(2):101-110. https://doi.org/10.1111/j.1525-1497.2004.30262.x
26. Betancourt JR, Green AR, Carrillo JE, Park ER. Cultural competence and health care disparities: key perspectives and trends. Health Aff (Millwood). 2005;24(2):499-505. https://doi.org/10.1377/hlthaff.24.2.499
27. Acosta D, Ackerman-Barger K. Breaking the silence: time to talk about race and racism [comment]. Acad Med. 2017;92(3):285-288. https://doi.org/10.1097/ACM.0000000000001416
28. Cohen JJ, Gabriel BA, Terrell C. The case for diversity in the health care workforce. Health Aff (Millwood). 2002;21(5):90-102. https://doi.org/10.1377/hlthaff.21.5.90
29. Chang E, Simon M, Dong X. Integrating cultural humility into health care professional education and training. Adv Health Sci Educ Theory Pract. 2012;17(2):269-278. https://doi.org/10.1007/s10459-010-9264-1
30. Foronda C, Baptiste DL, Reinholdt MM, Ousman K. Cultural humility: a concept analysis. J Transcult Nurs. 2016;27(3):210-217. https://doi.org/10.1177/1043659615592677
31. Butkus R, Serchen J, Moyer DV, et al; Health and Public Policy Committee of the American College of Physicians. Achieving gender equity in physician compensation and career advancement: a position paper of the American College of Physicians. Ann Intern Med. 2018;168(10):721-723. https://doi.org/10.7326/M17-3438
32. Burden M, del Pino-Jones A, Shafer M, Sheth S, Rexrode K. GWIMS Equity Recruitment Toolkit. Accessed July 27, 2019. https://www.aamc.org/download/492864/data/equityinrecruitmenttoolkit.pdf
33. AAMC Faculty Salary Report. AAMC website. Accessed September 6, 2020. https://www.aamc.org/data-reports/workforce/report/aamc-faculty-salary-report
34. Promotion process. University of Colorado Anschutz Medical Campus website. Accessed September 7, 2020. https://medschool.cuanschutz.edu/faculty-affairs/for-faculty/promotion-and-tenure/promotion-process
35. Pierce RG, Diaz M, Kneeland P. Optimizing well-being, practice culture, and professional thriving in an era of turbulence. J Hosp Med. 2019;14(2):126-128. https://doi.org/10.12788/jhm.3101
© 2021 Society of Hospital Medicine
SPEAKers at the National Society of Hospital Medicine Meeting: A Follow-UP Study of Gender Equity for Conference Speakers from 2015 to 2019. The SPEAK UP Study
Persistent gender disparities exist in pay,1,2 leadership opportunities,3,4 promotion,5 and speaking opportunities.6 While the gender distribution of the hospitalist workforce may be approaching parity,3,7,8 gender differences in leadership, speakership, and authorship have already been noted in hospital medicine.3 Between 2006 and 2012, women constituted less than a third (26%) of the presenters at the national conferences of the Society of Hospital Medicine (SHM) and the Society of General Internal Medicine (SGIM).3
The SHM Annual Meeting has historically had an “open call” peer review process for workshop presenters with the goal of increasing the diversity of presenters. In 2019, this process was expanded to include didactic speakers. Our aim in this study was to assess whether these open call procedures resulted in improved representation of women speakers and how the proportion of women speakers affects the overall evaluation scores of the conference. Our hypothesis was that the introduction of an open call process for the SHM conference didactic speakers would be associated with an increased proportion of women speakers, compared with the closed call processes, without a negative impact on conference scores.
METHODS
The study is a retrospective evaluation of data collected regarding speakers at the annual SHM conference from 2015 to 2019. The SHM national conference typically has two main types of offerings: workshops and didactics. Workshop presenters from 2015 to 2019 were selected via an open call process as defined below. Didactic speakers (except for plenary speakers) were selected using the open call process for 2019 only.
We aimed to compare (1) the number and proportion of women speakers, compared with men speakers, over time and (2) the proportion of women speakers when open call processes were utilized versus that seen with closed call processes. Open call included workshops for all years and didactics for 2019; closed call included didactics for 2015 to 2018 and plenary sessions 2015 to 2019 (Table). The speaker list for the conferences was obtained from conference pamphlets or agendas available via Internet searches or obtained through attendance at the conference.
Speaker Categories and Identification Process
We determined whether each individual was a featured speaker (one whose talk was unopposed by other sessions), plenary speaker (defined as such in the conference pamphlets), whether they spoke in a group format, and whether the speaking opportunity type was a workshop or a didactic session. Numbers of featured and plenary speakers were combined because of low numbers. SHM provided deidentified conference evaluation data for each year studied. For the purposes of this study, we analyzed all speakers which included physicians, advanced practice providers, and professionals such as nurses and other interdisciplinary team members. The same speaker could be included multiple times if they had multiple speaking opportunities.
Open Call Process
We defined the “open call process” (referred to as “open call” here forward) as the process utilized by SHM that includes the following two components: (1) advertisements to members of SHM and to the medical community at large through a variety of mechanisms including emails, websites, and social media outlets and (2) an online submission process that includes names of proposed speakers and their topic and, in the case of workshops, session objectives as well as an outline of the proposed workshop. SHM committees may also submit suggestions for topics and speakers. Annual Conference Committee members then review and rate submissions on the categories of topic, organization and clarity, objectives, and speaker qualifications (with a focus on institutional, geographic, and gender diversity). Scores are assigned from 1 to 5 (with 5 being the best score) for each category and a section for comments is available. All submissions are also evaluated by the course director.
After initial committee reviews, scores with marked reviewer discrepancies are rereviewed and discussed by the committee and course director. A cutoff score is then calculated with proposals falling below the cutoff threshold omitted from further consideration. Weekly calls are then focused on subcategories (ie tracks) with emphasis on clinical and educational content. Each of the tracks have a subcommittee with track leads to curate the best content first and then focus on final speaker selection. More recently, templates are shared with the track leads that include a location to call out gender and institutional diversity. Weekly calls are held to hone the content and determine the speakers.
For the purposes of this study, when the above process was not used, the authors refer to it as “closed call.” Closed call processes do not typically involve open invitations or a peer review process. (Table)
Gender
Gender was assigned based on the speaker’s self-identification by the pronouns used in their biography submitted to the conference or on their institutional website or other websites where the speaker was referenced. Persons using she/her/hers pronouns were noted as women and persons using he/him/his were noted as men. For the purposes of this study, we conceptualized gender as binary (ie woman/man) given the limited information we had from online sources.
ANALYSIS
REDCap, a secure, Web-based application for building and managing online survey and databases, was used to collect and manage all study data.9
All analyses were performed using SAS Enterprise Guide 8.1 (SAS Institute, Inc., Cary, North Carolina) using retrospectively collected data. A Cochran-Armitage test for trend was used to evaluate the proportion of women speakers from 2015 to 2019. A chi-square test was used to assess the proportion of women speakers for open call processes versus that seen with closed call. One-way analysis of variance (ANOVA) was used to evaluate annual conference evaluation scores from 2015 to 2019. Either numbers with proportions or means with standard deviations have been reported. Bonferroni’s correction for multiple comparisons was applied, with a P < .008 considered statistically significant.
RESULTS
Between 2015 and 2019, a total of 709 workshop and didactic presentations were given by 1,261 speakers at the annual Society of Hospital Medicine Conference. Of these, 505 (40%) were women; 756 (60%) were men. There were no missing data.
From 2015 to 2019, representation of women speakers increased from 35% of all speakers to 47% of all speakers (P = .0068). Women plenary speakers increased from 23% in 2015 to 45% in 2019 (P = .0396).
The proportion of women presenters for workshops (which have utilized an open call process throughout the study period), ranged from 43% to 53% from 2015 to 2019 with no statistically significant difference in gender distribution across years (Figure).
A greater proportion of speakers selected by an open call process were women compared to when speakers were selected by a closed call process (261 (47%) vs 244 (34%); P < .0001).
Of didactics or workshops given in a group format (N = 299), 82 (27%) were given by all-men groups and 38 (13%) were given by all-women groups. Women speakers participating in all-women group talks accounted for 21% of all women speakers; whereas men speakers participating in all-men group talks account for 26% of all men speakers (P = .02). We found that all-men group speaking opportunities did decrease from 41% of group talks in 2015 to 21% of group talks in 2019 (P = .0065).
We saw an average 3% annual increase in women speakers from 2015 to 2019, an 8% increase from 2018 to 2019 for all speakers, and an 11% increase in women speakers specific to didactic sessions. Overall conference ratings increased from a mean of 4.3 ± 0.24 in 2015 to a mean of 4.6 ± 0.14 in 2019 (n = 1,202; P < .0001; Figure).
DISCUSSION
The important findings of this study are that there has been an increase in women speakers over the last 5 years at the annual Society of Hospital Medicine Conference, that women had higher representation as speakers when open call processes were followed, and that conference scores continued to improve during the time frame studied. These findings suggest that a systematic open call process helps to support equitable speaking opportunities for men and women at a national hospital medicine conference without a negative impact on conference quality.
To recruit more diverse speakers, open call and peer review processes were used in addition to deliberate efforts at ensuring diversity in speakers. We found that over time, the proportion of women with speaking opportunities increased from 2015 to 2019. Interestingly, workshops, which had open call processes in place for the duration of the study period, had almost equal numbers of men and women presenting in all years. We also found that the number of all-men speaking groups decreased between 2015 and 2019.
A single process change can impact gender equity, but the target of true equity is expected to require additional measures such as assessment of committee structures and diversity, checklists, and reporting structures (data analysis and plans when goals not achieved).10-13 For instance, the American Society for Microbiology General Meeting was able to achieve gender equity in speakers by a multifold approach including ensuring the program committee was aware of gender statistics, increasing female representation among session convener teams, and direct instruction to try to avoid all-male sessions.11
It is important to acknowledge that these processes do require valuable resources including time. SHM has historically used committee volunteers to conduct the peer review process with each committee member reviewing 20 to 30 workshop submissions and 30 to 50 didactic sessions. While open processes with peer review seem to generate improved gender equity, ensuring processes are in place during the selection process is also key.
Several recent notable efforts to enhance gender equity and to increase diversity have been proposed. One such example of a process that may further improve gender equity was proposed by editors at the Journal of Hospital Medicine to assess current representation via demographics including gender, race, and ethnicity of authors with plans to assess patterns in the coming years.14 The American College of Physicians also published a position paper on achieving gender equity with a recommendation that organizational policies and procedures should be implemented that address implicit bias.15
Our study showed that, from 2015 to 2019, conference evaluations saw a significant increase in the score concurrently with the rise in proportion of women speakers. This finding suggests that quality does not seem to be affected by this new methodology for speaker selection and in fact this methodology may actually help improve the overall quality of the conference. To our knowledge, this is one of the first studies to concurrently evaluate speaker gender equity with conference quality.
Our study offers several strengths. This study took a pragmatic approach to understanding how processes can impact gender equity, and we were able to take advantage of the evolution of the open call system (ie workshops which have been an open call process for the duration of the study versus speaking opportunities that were not).
Our study also has several limitations. First, this study is retrospective in nature and thus other processes could have contributed to the improved gender equity, such as an organization’s priorities over time. During this study period, the SHM conference saw an average 3% increase annually in women speakers and an increase of 8% from 2018 to 2019 for all speakers compared to national trends of approximately 1%,6 which suggests that the open call processes in place could be contributing to the overall increases seen. Similarly, because of the retrospective nature of the study, we cannot be certain that the improvements in conference scores were directly the result of improved gender equity, although it does suggest that the improvements in gender equity did not have an adverse impact on the scores. We also did not assess how the composition of selection committee members for the meeting could have impacted the overall composition of the speakers. Our study looked at diversity only from the perspective of gender in a binary fashion, and thus additional studies are needed to assess how to improve diversity overall. It is unclear how this new open call for speakers affects race and ethnic diversity specifically. Identifying gender for the purposes of this study was facilitated by speakers providing their own biographies and the respective pronouns used in those biographies, and thus gender was easier to ascertain than race and ethnicity, which are not as readily available. For organizations to understand their diversity, equity, and inclusion efforts, enhancing the ability to fairly track and measure diversity will be key. Lastly, understanding of the exact composition of hospitalists from both a gender and race/ethnicity perspective is lacking. Studies have suggested that, based upon those surveyed or studied, there is a fairly equal balance of men and women albeit in academic groups.3
CONCLUSIONS
An open call approach to speakers at a national hospitalist conference seems to have contributed to improvements regarding gender equity in speaking opportunities with a concurrent improvement in overall rating of the conference. The open call system is a potential mechanism that other institutions and organizations could employ to enhance their diversity efforts.
Acknowledgments
Society of Hospital Medicine Diversity, Equity, Inclusion Special Interest Group
Work Group for SPEAK UP: Marisha Burden, MD, Daniel Cabrera, MD, Amira del Pino-Jones, MD, Areeba Kara, MD, Angela Keniston, MSPH, Keshav Khanijow, MD, Flora Kisuule, MD, Chiara Mandel, Benji Mathews, MD, David Paje, MD, Stephan Papp, MD, Snehal Patel, MD, Suchita Shah Sata, MD, Dustin Smith, MD, Kevin Vuernick
1. Weaver AC, Wetterneck TB, Whelan CT, Hinami K. A matter of priorities? Exploring the persistent gender pay gap in hospital medicine. J Hosp Med. 2015;10(8):486-490. https://doi.org/10.1002/jhm.2400.
2. Jena AB, Olenski AR, Blumenthal DM. Sex differences in physician salary in US public medical schools. JAMA Intern Med. 2016;176(9):1294-1304. https://doi.org/10.1001/jamainternmed.2016.3284.
3. Burden M, Frank MG, Keniston A, et al. Gender disparities in leadership and scholarly productivity of academic hospitalists. J Hosp Med. 2015;10(8):481-485. https://doi.org/10.1002/jhm.2340.
4. Silver JK, Ghalib R, Poorman JA, et al. Analysis of gender equity in leadership of physician-focused medical specialty societies, 2008-2017. JAMA Intern Med. 2019;179(3):433-435. https://doi.org/10.1001/jamainternmed.2018.5303.
5. Jena AB, Khullar D, Ho O, Olenski AR, Blumenthal DM. Sex differences in academic rank in US medical schools in 2014. JAMA. 2015;314(11):1149-1158. https://doi.org/10.1001/jama.2015.10680.
6. Ruzycki SM, Fletcher S, Earp M, Bharwani A, Lithgow KC. Trends in the Proportion of Female Speakers at Medical Conferences in the United States and in Canada, 2007 to 2017. JAMA Netw Open. 2019;2(4):e192103. https://doi.org/10.1001/jamanetworkopen.2019.2103
7. Reid MB, Misky GJ, Harrison RA, Sharpe B, Auerbach A, Glasheen JJ. Mentorship, productivity, and promotion among academic hospitalists. J Gen Intern Med. 2012;27(1):23-27. https://doi.org/10.1007/s11606-011-1892-5.
8. Today’s Hospitalist 2018 Compensation and Career Survey Results. https://www.todayshospitalist.com/salary-survey-results/. Accessed September 28, 2019.
9. Harris PA, Taylor R, Thielke R, Payne J, Gonzalez N, Conde JG. Research electronic data capture (REDCap)--a metadata-driven methodology and workflow process for providing translational research informatics support. J Biomed Inform. 2009;42(2):377-381. https://doi.org/10.1016/j.jbi.2008.08.010.
10. Burden M, del Pino-Jones A, Shafer M, Sheth S, Rexrode K. Association of American Medical Colleagues (AAMC) Group on Women in Medicine and Science. Recruitment Toolkit: https://www.aamc.org/download/492864/data/equityinrecruitmenttoolkit.pdf. Accessed July 27, 2019.
11. Casadevall A. Achieving speaker gender equity at the american society for microbiology general meeting. MBio. 2015;6:e01146. https://doi.org/10.1128/mBio.01146-15.
12. Westring A, McDonald JM, Carr P, Grisso JA. An integrated framework for gender equity in academic medicine. Acad Med. 2016;91(8):1041-1044. https://doi.org/10.1097/ACM.0000000000001275.
13. Martin JL. Ten simple rules to achieve conference speaker gender balance. PLoS Comput Biol. 2014;10(11):e1003903. https://doi.org/10.1371/journal.pcbi.1003903.
14. Shah SS, Shaughnessy EE, Spector ND. Leading by example: how medical journals can improve representation in academic medicine. J Hosp Med. 2019;14(7):393. https://doi.org/10.12788/jhm.3247.
15. Butkus R, Serchen J, Moyer DV, et al. Achieving gender equity in physician compensation and career advancement: a position paper of the American College of Physicians. Ann Intern Med. 2018;168:721-723. https://doi.org/10.7326/M17-3438.
Persistent gender disparities exist in pay,1,2 leadership opportunities,3,4 promotion,5 and speaking opportunities.6 While the gender distribution of the hospitalist workforce may be approaching parity,3,7,8 gender differences in leadership, speakership, and authorship have already been noted in hospital medicine.3 Between 2006 and 2012, women constituted less than a third (26%) of the presenters at the national conferences of the Society of Hospital Medicine (SHM) and the Society of General Internal Medicine (SGIM).3
The SHM Annual Meeting has historically had an “open call” peer review process for workshop presenters with the goal of increasing the diversity of presenters. In 2019, this process was expanded to include didactic speakers. Our aim in this study was to assess whether these open call procedures resulted in improved representation of women speakers and how the proportion of women speakers affects the overall evaluation scores of the conference. Our hypothesis was that the introduction of an open call process for the SHM conference didactic speakers would be associated with an increased proportion of women speakers, compared with the closed call processes, without a negative impact on conference scores.
METHODS
The study is a retrospective evaluation of data collected regarding speakers at the annual SHM conference from 2015 to 2019. The SHM national conference typically has two main types of offerings: workshops and didactics. Workshop presenters from 2015 to 2019 were selected via an open call process as defined below. Didactic speakers (except for plenary speakers) were selected using the open call process for 2019 only.
We aimed to compare (1) the number and proportion of women speakers, compared with men speakers, over time and (2) the proportion of women speakers when open call processes were utilized versus that seen with closed call processes. Open call included workshops for all years and didactics for 2019; closed call included didactics for 2015 to 2018 and plenary sessions 2015 to 2019 (Table). The speaker list for the conferences was obtained from conference pamphlets or agendas available via Internet searches or obtained through attendance at the conference.
Speaker Categories and Identification Process
We determined whether each individual was a featured speaker (one whose talk was unopposed by other sessions), plenary speaker (defined as such in the conference pamphlets), whether they spoke in a group format, and whether the speaking opportunity type was a workshop or a didactic session. Numbers of featured and plenary speakers were combined because of low numbers. SHM provided deidentified conference evaluation data for each year studied. For the purposes of this study, we analyzed all speakers which included physicians, advanced practice providers, and professionals such as nurses and other interdisciplinary team members. The same speaker could be included multiple times if they had multiple speaking opportunities.
Open Call Process
We defined the “open call process” (referred to as “open call” here forward) as the process utilized by SHM that includes the following two components: (1) advertisements to members of SHM and to the medical community at large through a variety of mechanisms including emails, websites, and social media outlets and (2) an online submission process that includes names of proposed speakers and their topic and, in the case of workshops, session objectives as well as an outline of the proposed workshop. SHM committees may also submit suggestions for topics and speakers. Annual Conference Committee members then review and rate submissions on the categories of topic, organization and clarity, objectives, and speaker qualifications (with a focus on institutional, geographic, and gender diversity). Scores are assigned from 1 to 5 (with 5 being the best score) for each category and a section for comments is available. All submissions are also evaluated by the course director.
After initial committee reviews, scores with marked reviewer discrepancies are rereviewed and discussed by the committee and course director. A cutoff score is then calculated with proposals falling below the cutoff threshold omitted from further consideration. Weekly calls are then focused on subcategories (ie tracks) with emphasis on clinical and educational content. Each of the tracks have a subcommittee with track leads to curate the best content first and then focus on final speaker selection. More recently, templates are shared with the track leads that include a location to call out gender and institutional diversity. Weekly calls are held to hone the content and determine the speakers.
For the purposes of this study, when the above process was not used, the authors refer to it as “closed call.” Closed call processes do not typically involve open invitations or a peer review process. (Table)
Gender
Gender was assigned based on the speaker’s self-identification by the pronouns used in their biography submitted to the conference or on their institutional website or other websites where the speaker was referenced. Persons using she/her/hers pronouns were noted as women and persons using he/him/his were noted as men. For the purposes of this study, we conceptualized gender as binary (ie woman/man) given the limited information we had from online sources.
ANALYSIS
REDCap, a secure, Web-based application for building and managing online survey and databases, was used to collect and manage all study data.9
All analyses were performed using SAS Enterprise Guide 8.1 (SAS Institute, Inc., Cary, North Carolina) using retrospectively collected data. A Cochran-Armitage test for trend was used to evaluate the proportion of women speakers from 2015 to 2019. A chi-square test was used to assess the proportion of women speakers for open call processes versus that seen with closed call. One-way analysis of variance (ANOVA) was used to evaluate annual conference evaluation scores from 2015 to 2019. Either numbers with proportions or means with standard deviations have been reported. Bonferroni’s correction for multiple comparisons was applied, with a P < .008 considered statistically significant.
RESULTS
Between 2015 and 2019, a total of 709 workshop and didactic presentations were given by 1,261 speakers at the annual Society of Hospital Medicine Conference. Of these, 505 (40%) were women; 756 (60%) were men. There were no missing data.
From 2015 to 2019, representation of women speakers increased from 35% of all speakers to 47% of all speakers (P = .0068). Women plenary speakers increased from 23% in 2015 to 45% in 2019 (P = .0396).
The proportion of women presenters for workshops (which have utilized an open call process throughout the study period), ranged from 43% to 53% from 2015 to 2019 with no statistically significant difference in gender distribution across years (Figure).
A greater proportion of speakers selected by an open call process were women compared to when speakers were selected by a closed call process (261 (47%) vs 244 (34%); P < .0001).
Of didactics or workshops given in a group format (N = 299), 82 (27%) were given by all-men groups and 38 (13%) were given by all-women groups. Women speakers participating in all-women group talks accounted for 21% of all women speakers; whereas men speakers participating in all-men group talks account for 26% of all men speakers (P = .02). We found that all-men group speaking opportunities did decrease from 41% of group talks in 2015 to 21% of group talks in 2019 (P = .0065).
We saw an average 3% annual increase in women speakers from 2015 to 2019, an 8% increase from 2018 to 2019 for all speakers, and an 11% increase in women speakers specific to didactic sessions. Overall conference ratings increased from a mean of 4.3 ± 0.24 in 2015 to a mean of 4.6 ± 0.14 in 2019 (n = 1,202; P < .0001; Figure).
DISCUSSION
The important findings of this study are that there has been an increase in women speakers over the last 5 years at the annual Society of Hospital Medicine Conference, that women had higher representation as speakers when open call processes were followed, and that conference scores continued to improve during the time frame studied. These findings suggest that a systematic open call process helps to support equitable speaking opportunities for men and women at a national hospital medicine conference without a negative impact on conference quality.
To recruit more diverse speakers, open call and peer review processes were used in addition to deliberate efforts at ensuring diversity in speakers. We found that over time, the proportion of women with speaking opportunities increased from 2015 to 2019. Interestingly, workshops, which had open call processes in place for the duration of the study period, had almost equal numbers of men and women presenting in all years. We also found that the number of all-men speaking groups decreased between 2015 and 2019.
A single process change can impact gender equity, but the target of true equity is expected to require additional measures such as assessment of committee structures and diversity, checklists, and reporting structures (data analysis and plans when goals not achieved).10-13 For instance, the American Society for Microbiology General Meeting was able to achieve gender equity in speakers by a multifold approach including ensuring the program committee was aware of gender statistics, increasing female representation among session convener teams, and direct instruction to try to avoid all-male sessions.11
It is important to acknowledge that these processes do require valuable resources including time. SHM has historically used committee volunteers to conduct the peer review process with each committee member reviewing 20 to 30 workshop submissions and 30 to 50 didactic sessions. While open processes with peer review seem to generate improved gender equity, ensuring processes are in place during the selection process is also key.
Several recent notable efforts to enhance gender equity and to increase diversity have been proposed. One such example of a process that may further improve gender equity was proposed by editors at the Journal of Hospital Medicine to assess current representation via demographics including gender, race, and ethnicity of authors with plans to assess patterns in the coming years.14 The American College of Physicians also published a position paper on achieving gender equity with a recommendation that organizational policies and procedures should be implemented that address implicit bias.15
Our study showed that, from 2015 to 2019, conference evaluations saw a significant increase in the score concurrently with the rise in proportion of women speakers. This finding suggests that quality does not seem to be affected by this new methodology for speaker selection and in fact this methodology may actually help improve the overall quality of the conference. To our knowledge, this is one of the first studies to concurrently evaluate speaker gender equity with conference quality.
Our study offers several strengths. This study took a pragmatic approach to understanding how processes can impact gender equity, and we were able to take advantage of the evolution of the open call system (ie workshops which have been an open call process for the duration of the study versus speaking opportunities that were not).
Our study also has several limitations. First, this study is retrospective in nature and thus other processes could have contributed to the improved gender equity, such as an organization’s priorities over time. During this study period, the SHM conference saw an average 3% increase annually in women speakers and an increase of 8% from 2018 to 2019 for all speakers compared to national trends of approximately 1%,6 which suggests that the open call processes in place could be contributing to the overall increases seen. Similarly, because of the retrospective nature of the study, we cannot be certain that the improvements in conference scores were directly the result of improved gender equity, although it does suggest that the improvements in gender equity did not have an adverse impact on the scores. We also did not assess how the composition of selection committee members for the meeting could have impacted the overall composition of the speakers. Our study looked at diversity only from the perspective of gender in a binary fashion, and thus additional studies are needed to assess how to improve diversity overall. It is unclear how this new open call for speakers affects race and ethnic diversity specifically. Identifying gender for the purposes of this study was facilitated by speakers providing their own biographies and the respective pronouns used in those biographies, and thus gender was easier to ascertain than race and ethnicity, which are not as readily available. For organizations to understand their diversity, equity, and inclusion efforts, enhancing the ability to fairly track and measure diversity will be key. Lastly, understanding of the exact composition of hospitalists from both a gender and race/ethnicity perspective is lacking. Studies have suggested that, based upon those surveyed or studied, there is a fairly equal balance of men and women albeit in academic groups.3
CONCLUSIONS
An open call approach to speakers at a national hospitalist conference seems to have contributed to improvements regarding gender equity in speaking opportunities with a concurrent improvement in overall rating of the conference. The open call system is a potential mechanism that other institutions and organizations could employ to enhance their diversity efforts.
Acknowledgments
Society of Hospital Medicine Diversity, Equity, Inclusion Special Interest Group
Work Group for SPEAK UP: Marisha Burden, MD, Daniel Cabrera, MD, Amira del Pino-Jones, MD, Areeba Kara, MD, Angela Keniston, MSPH, Keshav Khanijow, MD, Flora Kisuule, MD, Chiara Mandel, Benji Mathews, MD, David Paje, MD, Stephan Papp, MD, Snehal Patel, MD, Suchita Shah Sata, MD, Dustin Smith, MD, Kevin Vuernick
Persistent gender disparities exist in pay,1,2 leadership opportunities,3,4 promotion,5 and speaking opportunities.6 While the gender distribution of the hospitalist workforce may be approaching parity,3,7,8 gender differences in leadership, speakership, and authorship have already been noted in hospital medicine.3 Between 2006 and 2012, women constituted less than a third (26%) of the presenters at the national conferences of the Society of Hospital Medicine (SHM) and the Society of General Internal Medicine (SGIM).3
The SHM Annual Meeting has historically had an “open call” peer review process for workshop presenters with the goal of increasing the diversity of presenters. In 2019, this process was expanded to include didactic speakers. Our aim in this study was to assess whether these open call procedures resulted in improved representation of women speakers and how the proportion of women speakers affects the overall evaluation scores of the conference. Our hypothesis was that the introduction of an open call process for the SHM conference didactic speakers would be associated with an increased proportion of women speakers, compared with the closed call processes, without a negative impact on conference scores.
METHODS
The study is a retrospective evaluation of data collected regarding speakers at the annual SHM conference from 2015 to 2019. The SHM national conference typically has two main types of offerings: workshops and didactics. Workshop presenters from 2015 to 2019 were selected via an open call process as defined below. Didactic speakers (except for plenary speakers) were selected using the open call process for 2019 only.
We aimed to compare (1) the number and proportion of women speakers, compared with men speakers, over time and (2) the proportion of women speakers when open call processes were utilized versus that seen with closed call processes. Open call included workshops for all years and didactics for 2019; closed call included didactics for 2015 to 2018 and plenary sessions 2015 to 2019 (Table). The speaker list for the conferences was obtained from conference pamphlets or agendas available via Internet searches or obtained through attendance at the conference.
Speaker Categories and Identification Process
We determined whether each individual was a featured speaker (one whose talk was unopposed by other sessions), plenary speaker (defined as such in the conference pamphlets), whether they spoke in a group format, and whether the speaking opportunity type was a workshop or a didactic session. Numbers of featured and plenary speakers were combined because of low numbers. SHM provided deidentified conference evaluation data for each year studied. For the purposes of this study, we analyzed all speakers which included physicians, advanced practice providers, and professionals such as nurses and other interdisciplinary team members. The same speaker could be included multiple times if they had multiple speaking opportunities.
Open Call Process
We defined the “open call process” (referred to as “open call” here forward) as the process utilized by SHM that includes the following two components: (1) advertisements to members of SHM and to the medical community at large through a variety of mechanisms including emails, websites, and social media outlets and (2) an online submission process that includes names of proposed speakers and their topic and, in the case of workshops, session objectives as well as an outline of the proposed workshop. SHM committees may also submit suggestions for topics and speakers. Annual Conference Committee members then review and rate submissions on the categories of topic, organization and clarity, objectives, and speaker qualifications (with a focus on institutional, geographic, and gender diversity). Scores are assigned from 1 to 5 (with 5 being the best score) for each category and a section for comments is available. All submissions are also evaluated by the course director.
After initial committee reviews, scores with marked reviewer discrepancies are rereviewed and discussed by the committee and course director. A cutoff score is then calculated with proposals falling below the cutoff threshold omitted from further consideration. Weekly calls are then focused on subcategories (ie tracks) with emphasis on clinical and educational content. Each of the tracks have a subcommittee with track leads to curate the best content first and then focus on final speaker selection. More recently, templates are shared with the track leads that include a location to call out gender and institutional diversity. Weekly calls are held to hone the content and determine the speakers.
For the purposes of this study, when the above process was not used, the authors refer to it as “closed call.” Closed call processes do not typically involve open invitations or a peer review process. (Table)
Gender
Gender was assigned based on the speaker’s self-identification by the pronouns used in their biography submitted to the conference or on their institutional website or other websites where the speaker was referenced. Persons using she/her/hers pronouns were noted as women and persons using he/him/his were noted as men. For the purposes of this study, we conceptualized gender as binary (ie woman/man) given the limited information we had from online sources.
ANALYSIS
REDCap, a secure, Web-based application for building and managing online survey and databases, was used to collect and manage all study data.9
All analyses were performed using SAS Enterprise Guide 8.1 (SAS Institute, Inc., Cary, North Carolina) using retrospectively collected data. A Cochran-Armitage test for trend was used to evaluate the proportion of women speakers from 2015 to 2019. A chi-square test was used to assess the proportion of women speakers for open call processes versus that seen with closed call. One-way analysis of variance (ANOVA) was used to evaluate annual conference evaluation scores from 2015 to 2019. Either numbers with proportions or means with standard deviations have been reported. Bonferroni’s correction for multiple comparisons was applied, with a P < .008 considered statistically significant.
RESULTS
Between 2015 and 2019, a total of 709 workshop and didactic presentations were given by 1,261 speakers at the annual Society of Hospital Medicine Conference. Of these, 505 (40%) were women; 756 (60%) were men. There were no missing data.
From 2015 to 2019, representation of women speakers increased from 35% of all speakers to 47% of all speakers (P = .0068). Women plenary speakers increased from 23% in 2015 to 45% in 2019 (P = .0396).
The proportion of women presenters for workshops (which have utilized an open call process throughout the study period), ranged from 43% to 53% from 2015 to 2019 with no statistically significant difference in gender distribution across years (Figure).
A greater proportion of speakers selected by an open call process were women compared to when speakers were selected by a closed call process (261 (47%) vs 244 (34%); P < .0001).
Of didactics or workshops given in a group format (N = 299), 82 (27%) were given by all-men groups and 38 (13%) were given by all-women groups. Women speakers participating in all-women group talks accounted for 21% of all women speakers; whereas men speakers participating in all-men group talks account for 26% of all men speakers (P = .02). We found that all-men group speaking opportunities did decrease from 41% of group talks in 2015 to 21% of group talks in 2019 (P = .0065).
We saw an average 3% annual increase in women speakers from 2015 to 2019, an 8% increase from 2018 to 2019 for all speakers, and an 11% increase in women speakers specific to didactic sessions. Overall conference ratings increased from a mean of 4.3 ± 0.24 in 2015 to a mean of 4.6 ± 0.14 in 2019 (n = 1,202; P < .0001; Figure).
DISCUSSION
The important findings of this study are that there has been an increase in women speakers over the last 5 years at the annual Society of Hospital Medicine Conference, that women had higher representation as speakers when open call processes were followed, and that conference scores continued to improve during the time frame studied. These findings suggest that a systematic open call process helps to support equitable speaking opportunities for men and women at a national hospital medicine conference without a negative impact on conference quality.
To recruit more diverse speakers, open call and peer review processes were used in addition to deliberate efforts at ensuring diversity in speakers. We found that over time, the proportion of women with speaking opportunities increased from 2015 to 2019. Interestingly, workshops, which had open call processes in place for the duration of the study period, had almost equal numbers of men and women presenting in all years. We also found that the number of all-men speaking groups decreased between 2015 and 2019.
A single process change can impact gender equity, but the target of true equity is expected to require additional measures such as assessment of committee structures and diversity, checklists, and reporting structures (data analysis and plans when goals not achieved).10-13 For instance, the American Society for Microbiology General Meeting was able to achieve gender equity in speakers by a multifold approach including ensuring the program committee was aware of gender statistics, increasing female representation among session convener teams, and direct instruction to try to avoid all-male sessions.11
It is important to acknowledge that these processes do require valuable resources including time. SHM has historically used committee volunteers to conduct the peer review process with each committee member reviewing 20 to 30 workshop submissions and 30 to 50 didactic sessions. While open processes with peer review seem to generate improved gender equity, ensuring processes are in place during the selection process is also key.
Several recent notable efforts to enhance gender equity and to increase diversity have been proposed. One such example of a process that may further improve gender equity was proposed by editors at the Journal of Hospital Medicine to assess current representation via demographics including gender, race, and ethnicity of authors with plans to assess patterns in the coming years.14 The American College of Physicians also published a position paper on achieving gender equity with a recommendation that organizational policies and procedures should be implemented that address implicit bias.15
Our study showed that, from 2015 to 2019, conference evaluations saw a significant increase in the score concurrently with the rise in proportion of women speakers. This finding suggests that quality does not seem to be affected by this new methodology for speaker selection and in fact this methodology may actually help improve the overall quality of the conference. To our knowledge, this is one of the first studies to concurrently evaluate speaker gender equity with conference quality.
Our study offers several strengths. This study took a pragmatic approach to understanding how processes can impact gender equity, and we were able to take advantage of the evolution of the open call system (ie workshops which have been an open call process for the duration of the study versus speaking opportunities that were not).
Our study also has several limitations. First, this study is retrospective in nature and thus other processes could have contributed to the improved gender equity, such as an organization’s priorities over time. During this study period, the SHM conference saw an average 3% increase annually in women speakers and an increase of 8% from 2018 to 2019 for all speakers compared to national trends of approximately 1%,6 which suggests that the open call processes in place could be contributing to the overall increases seen. Similarly, because of the retrospective nature of the study, we cannot be certain that the improvements in conference scores were directly the result of improved gender equity, although it does suggest that the improvements in gender equity did not have an adverse impact on the scores. We also did not assess how the composition of selection committee members for the meeting could have impacted the overall composition of the speakers. Our study looked at diversity only from the perspective of gender in a binary fashion, and thus additional studies are needed to assess how to improve diversity overall. It is unclear how this new open call for speakers affects race and ethnic diversity specifically. Identifying gender for the purposes of this study was facilitated by speakers providing their own biographies and the respective pronouns used in those biographies, and thus gender was easier to ascertain than race and ethnicity, which are not as readily available. For organizations to understand their diversity, equity, and inclusion efforts, enhancing the ability to fairly track and measure diversity will be key. Lastly, understanding of the exact composition of hospitalists from both a gender and race/ethnicity perspective is lacking. Studies have suggested that, based upon those surveyed or studied, there is a fairly equal balance of men and women albeit in academic groups.3
CONCLUSIONS
An open call approach to speakers at a national hospitalist conference seems to have contributed to improvements regarding gender equity in speaking opportunities with a concurrent improvement in overall rating of the conference. The open call system is a potential mechanism that other institutions and organizations could employ to enhance their diversity efforts.
Acknowledgments
Society of Hospital Medicine Diversity, Equity, Inclusion Special Interest Group
Work Group for SPEAK UP: Marisha Burden, MD, Daniel Cabrera, MD, Amira del Pino-Jones, MD, Areeba Kara, MD, Angela Keniston, MSPH, Keshav Khanijow, MD, Flora Kisuule, MD, Chiara Mandel, Benji Mathews, MD, David Paje, MD, Stephan Papp, MD, Snehal Patel, MD, Suchita Shah Sata, MD, Dustin Smith, MD, Kevin Vuernick
1. Weaver AC, Wetterneck TB, Whelan CT, Hinami K. A matter of priorities? Exploring the persistent gender pay gap in hospital medicine. J Hosp Med. 2015;10(8):486-490. https://doi.org/10.1002/jhm.2400.
2. Jena AB, Olenski AR, Blumenthal DM. Sex differences in physician salary in US public medical schools. JAMA Intern Med. 2016;176(9):1294-1304. https://doi.org/10.1001/jamainternmed.2016.3284.
3. Burden M, Frank MG, Keniston A, et al. Gender disparities in leadership and scholarly productivity of academic hospitalists. J Hosp Med. 2015;10(8):481-485. https://doi.org/10.1002/jhm.2340.
4. Silver JK, Ghalib R, Poorman JA, et al. Analysis of gender equity in leadership of physician-focused medical specialty societies, 2008-2017. JAMA Intern Med. 2019;179(3):433-435. https://doi.org/10.1001/jamainternmed.2018.5303.
5. Jena AB, Khullar D, Ho O, Olenski AR, Blumenthal DM. Sex differences in academic rank in US medical schools in 2014. JAMA. 2015;314(11):1149-1158. https://doi.org/10.1001/jama.2015.10680.
6. Ruzycki SM, Fletcher S, Earp M, Bharwani A, Lithgow KC. Trends in the Proportion of Female Speakers at Medical Conferences in the United States and in Canada, 2007 to 2017. JAMA Netw Open. 2019;2(4):e192103. https://doi.org/10.1001/jamanetworkopen.2019.2103
7. Reid MB, Misky GJ, Harrison RA, Sharpe B, Auerbach A, Glasheen JJ. Mentorship, productivity, and promotion among academic hospitalists. J Gen Intern Med. 2012;27(1):23-27. https://doi.org/10.1007/s11606-011-1892-5.
8. Today’s Hospitalist 2018 Compensation and Career Survey Results. https://www.todayshospitalist.com/salary-survey-results/. Accessed September 28, 2019.
9. Harris PA, Taylor R, Thielke R, Payne J, Gonzalez N, Conde JG. Research electronic data capture (REDCap)--a metadata-driven methodology and workflow process for providing translational research informatics support. J Biomed Inform. 2009;42(2):377-381. https://doi.org/10.1016/j.jbi.2008.08.010.
10. Burden M, del Pino-Jones A, Shafer M, Sheth S, Rexrode K. Association of American Medical Colleagues (AAMC) Group on Women in Medicine and Science. Recruitment Toolkit: https://www.aamc.org/download/492864/data/equityinrecruitmenttoolkit.pdf. Accessed July 27, 2019.
11. Casadevall A. Achieving speaker gender equity at the american society for microbiology general meeting. MBio. 2015;6:e01146. https://doi.org/10.1128/mBio.01146-15.
12. Westring A, McDonald JM, Carr P, Grisso JA. An integrated framework for gender equity in academic medicine. Acad Med. 2016;91(8):1041-1044. https://doi.org/10.1097/ACM.0000000000001275.
13. Martin JL. Ten simple rules to achieve conference speaker gender balance. PLoS Comput Biol. 2014;10(11):e1003903. https://doi.org/10.1371/journal.pcbi.1003903.
14. Shah SS, Shaughnessy EE, Spector ND. Leading by example: how medical journals can improve representation in academic medicine. J Hosp Med. 2019;14(7):393. https://doi.org/10.12788/jhm.3247.
15. Butkus R, Serchen J, Moyer DV, et al. Achieving gender equity in physician compensation and career advancement: a position paper of the American College of Physicians. Ann Intern Med. 2018;168:721-723. https://doi.org/10.7326/M17-3438.
1. Weaver AC, Wetterneck TB, Whelan CT, Hinami K. A matter of priorities? Exploring the persistent gender pay gap in hospital medicine. J Hosp Med. 2015;10(8):486-490. https://doi.org/10.1002/jhm.2400.
2. Jena AB, Olenski AR, Blumenthal DM. Sex differences in physician salary in US public medical schools. JAMA Intern Med. 2016;176(9):1294-1304. https://doi.org/10.1001/jamainternmed.2016.3284.
3. Burden M, Frank MG, Keniston A, et al. Gender disparities in leadership and scholarly productivity of academic hospitalists. J Hosp Med. 2015;10(8):481-485. https://doi.org/10.1002/jhm.2340.
4. Silver JK, Ghalib R, Poorman JA, et al. Analysis of gender equity in leadership of physician-focused medical specialty societies, 2008-2017. JAMA Intern Med. 2019;179(3):433-435. https://doi.org/10.1001/jamainternmed.2018.5303.
5. Jena AB, Khullar D, Ho O, Olenski AR, Blumenthal DM. Sex differences in academic rank in US medical schools in 2014. JAMA. 2015;314(11):1149-1158. https://doi.org/10.1001/jama.2015.10680.
6. Ruzycki SM, Fletcher S, Earp M, Bharwani A, Lithgow KC. Trends in the Proportion of Female Speakers at Medical Conferences in the United States and in Canada, 2007 to 2017. JAMA Netw Open. 2019;2(4):e192103. https://doi.org/10.1001/jamanetworkopen.2019.2103
7. Reid MB, Misky GJ, Harrison RA, Sharpe B, Auerbach A, Glasheen JJ. Mentorship, productivity, and promotion among academic hospitalists. J Gen Intern Med. 2012;27(1):23-27. https://doi.org/10.1007/s11606-011-1892-5.
8. Today’s Hospitalist 2018 Compensation and Career Survey Results. https://www.todayshospitalist.com/salary-survey-results/. Accessed September 28, 2019.
9. Harris PA, Taylor R, Thielke R, Payne J, Gonzalez N, Conde JG. Research electronic data capture (REDCap)--a metadata-driven methodology and workflow process for providing translational research informatics support. J Biomed Inform. 2009;42(2):377-381. https://doi.org/10.1016/j.jbi.2008.08.010.
10. Burden M, del Pino-Jones A, Shafer M, Sheth S, Rexrode K. Association of American Medical Colleagues (AAMC) Group on Women in Medicine and Science. Recruitment Toolkit: https://www.aamc.org/download/492864/data/equityinrecruitmenttoolkit.pdf. Accessed July 27, 2019.
11. Casadevall A. Achieving speaker gender equity at the american society for microbiology general meeting. MBio. 2015;6:e01146. https://doi.org/10.1128/mBio.01146-15.
12. Westring A, McDonald JM, Carr P, Grisso JA. An integrated framework for gender equity in academic medicine. Acad Med. 2016;91(8):1041-1044. https://doi.org/10.1097/ACM.0000000000001275.
13. Martin JL. Ten simple rules to achieve conference speaker gender balance. PLoS Comput Biol. 2014;10(11):e1003903. https://doi.org/10.1371/journal.pcbi.1003903.
14. Shah SS, Shaughnessy EE, Spector ND. Leading by example: how medical journals can improve representation in academic medicine. J Hosp Med. 2019;14(7):393. https://doi.org/10.12788/jhm.3247.
15. Butkus R, Serchen J, Moyer DV, et al. Achieving gender equity in physician compensation and career advancement: a position paper of the American College of Physicians. Ann Intern Med. 2018;168:721-723. https://doi.org/10.7326/M17-3438.
© 2020 Society of Hospital Medicine
Opioid Utilization and Perception of Pain Control in Hospitalized Patients: A Cross-Sectional Study of 11 Sites in 8 Countries
Since 2000, the United States has seen a marked increase in opioid prescribing1-3 and opioid-related complications, including overdoses, hospitalizations, and deaths.2,4,5 A study from 2015 showed that more than one-third of the US civilian noninstitutionalized population reported receiving an opioid prescription in the prior year, with 12.5% reporting misuse, and, of those, 16.7% reported a prescription use disorder.6 While there has been a slight decrease in opioid prescriptions in the US since 2012, rates of opioid prescribing in 2015 were three times higher than in 1999 and approximately four times higher than in Europe in 2015.3,7
Pain is commonly reported by hospitalized patients,8,9 and opioids are often a mainstay of treatment;9,10 however, treatment with opioids can have a number of adverse outcomes.2,10,11 Short-term exposure to opioids can lead to long-term use,12-16 and patients on opioids are at an increased risk for subsequent hospitalization and longer inpatient lengths of stay.5
Physician prescribing practices for opioids and patient expectations for pain control vary as a function of geographic region and culture,10,12,17,18 and pain is influenced by the cultural context in which it occurs.17,19-22 Treatment of pain may also be affected by limited access to or restrictions on selected medications, as well as by cultural biases.23 Whether these variations in the treatment of pain are reflected in patients’ satisfaction with pain control is uncertain.
We sought to compare the inpatient analgesic prescribing practices and patients’ perceptions of pain control for medical patients in four teaching hospitals in the US and in seven teaching hospitals in seven other countries.
METHODS
Study Design
We utilized a cross-sectional, observational design. The study was approved by the Institutional Review Boards at all participating sites.
Setting
The study was conducted at 11 academic hospitals in eight countries from October 8, 2013 to August 31, 2015. Sites in the US included Denver Health in Denver, Colorado; the University of Colorado Hospital in Aurora, Colorado; Hennepin Healthcare in Minneapolis, Minnesota; and Legacy Health in Portland, Oregon. Sites outside the US included McMaster University in Hamilton, Ontario, Canada; Hospital de la Santa Creu i Sant Pau, Universitat Autonòma de Barcelona in Barcelona, Spain; the University of Study of Milan and the University Ospedale “Luigi Sacco” in Milan, Italy, the National Taiwan University Hospital, in Taipei, Taiwan, the University of Ulsan College of Medicine, Asan Medical Center, in Seoul, Korea, the Imperial College, Chelsea and Westminster Hospital, in London, United Kingdom and Dunedin Hospital, Dunedin, New Zealand.
Inclusion and Exclusion Criteria
We included patients 18-89 years of age (20-89 in Taiwan because patients under 20 years of age in this country are a restricted group with respect to participating in research), admitted to an internal medicine service from the Emergency Department or Urgent Care clinic with an acute illness for a minimum of 24 hours (with time zero defined as the time care was initiated in the Emergency Department or Urgent Care Clinic), who reported pain at some time during the first 24-36 hours of their hospitalization and who provided informed consent. In the US, “admission” included both observation and inpatient status. We limited the patient population to those admitted via emergency departments and urgent care clinics in order to enroll similar patient populations across sites.
Scheduled admissions, patients transferred from an outside facility, patients admitted directly from a clinic, and those receiving care in intensive care units were excluded. We also excluded patients who were incarcerated, pregnant, those who received major surgery within the previous 14 days, those with a known diagnosis of active cancer, and those who were receiving palliative or hospice care. Patients receiving care from an investigator in the study at the time of enrollment were not eligible due to the potential conflict of interest.
Patient Screening
Primary teams were contacted to determine if any patients on their service might meet the criteria for inclusion in the study on preselected study days chosen on the basis of the research team’s availability. Identified patients were then screened to establish if they met the eligibility criteria. Patients were asked directly if they had experienced pain during their preadmission evaluation or during their hospitalization.
Data Collection
All patients were hospitalized at the time they gave consent and when data were collected. Data were collected via interviews with patients, as well as through chart review. We recorded patients’ age, gender, race, admitting diagnosis(es), length of stay, psychiatric illness, illicit drug use, whether they reported receiving opioid analgesics at the time of hospitalization, whether they were prescribed opioids and/or nonopioid analgesics during their hospitalization, the median and maximum doses of opioids prescribed and dispensed, and whether they were discharged on opioids. The question of illicit drug use was asked of all patients with the exception of those hospitalized in South Korea due to potential legal implications.
Opioid prescribing and receipt of opioids was recorded based upon current provider orders and medication administration records, respectively. Perception of and satisfaction with pain control was assessed with the American Pain Society Patient Outcome Questionnaire–Modified (APS-POQ-Modified).24,25 Versions of this survey have been validated in English as well as in other languages and cultures.26-28 Because hospitalization practices could differ across hospitals and in different countries, we compared patients’ severity of illness by using Charlson comorbidity scores. Consent forms and the APS-POQ were translated into each country’s primary language according to established processes.29 The survey was filled out by having site investigators read questions aloud and by use of a large-font visual analog scale to aid patients’ verbal responses.
Data were collected and managed using a secure, web-based application electronic data capture tool (Research Electronic Data Capture [REDCap], Nashville, Tennessee), hosted at Denver Health.30
Study Size
Preliminary data from the internal medicine units at our institution suggested that 40% of patients without cancer received opioid analgesics during their hospitalization. Assuming 90% power to detect an absolute difference in the proportion of inpatient medical patients who are receiving opioid analgesics during their hospital stay of 17%, a two-sided type 1 error rate of 0.05, six hospitals in the US, and nine hospitals from all other countries, we calculated an initial sample size of 150 patients per site. This sample size was considered feasible for enrollment in a busy inpatient clinical setting. Study end points were to either reach the goal number of patients (150 per site) or the predetermined study end date, whichever came first.
Data Analysis
We generated means with standard deviations (SDs) and medians with interquartile ranges (IQRs) for normally and nonnormally distributed continuous variables, respectively, and frequencies for categorical variables. We used linear mixed modeling for the analysis of continuous variables. For binary outcomes, our data were fitted to a generalized linear mixed model with logit as the link function and a binary distribution. For ordinal variables, specifically patient-reported satisfaction with pain control and the opinion statements, the data were fitted to a generalized linear mixed model with a cumulative logit link and a multinomial distribution. Hospital was included as a random effect in all models to account for patients cared for in the same hospital.
Country of origin, dichotomized as US or non-US, was the independent variable of interest for all models. An interaction term for exposure to opioids prior to admission and country was entered into all models to explore whether differences in the effect of country existed for patients who reported taking opioids prior to admission and those who did not.
The models for the frequency with which analgesics were given, doses of opioids given during hospitalization and at discharge, patient-reported pain score, and patient-reported satisfaction with pain control were adjusted for (1) age, (2) gender, (3) Charlson Comorbidity Index, (4) length of stay, (5) history of illicit drug use, (6) history of psychiatric illness, (7) daily dose in morphine milligram equivalents (MME) for opioids prior to admission, (8) average pain score, and (9) hospital. The patient-reported satisfaction with pain control model was also adjusted for whether or not opioids were given to the patient during their hospitalization. P < .05 was considered to indicate significance. All analyses were performed using SAS Enterprise Guide 7.1 (SAS Institute, Inc., Cary, North Carolina). We reported data on medications that were prescribed and dispensed (as opposed to just prescribed and not necessarily given). Opioids prescribed at discharge represented the total possible opioids that could be given based upon the order/prescription (eg, oxycodone 5 mg every 6 hours as needed for pain would be counted as 20 mg/24 hours maximum possible dose followed by conversion to MME).
Missing Data
When there were missing data, a query was sent to sites to verify if the data were retrievable. If retrievable, the data were then entered. Data were missing in 5% and 2% of patients who did or did not report taking an opioid prior to admission, respectively. If a variable was included in a specific statistical test, then subjects with missing data were excluded from that analysis (ie, complete case analysis).
RESULTS
We approached 1,309 eligible patients, of which 981 provided informed consent, for a response rate of 75%; 503 from the US and 478 patients from other countries (Figure). In unadjusted analyses, we found no significant differences between US and non-US patients in age (mean age 51, SD 15 vs 59, SD 19; P = .30), race, ethnicity, or Charlson comorbidity index scores (median 2, IQR 1-3 vs 3, IQR 1-4; P = .45). US patients had shorter lengths of stay (median 3 days, IQR 2-4 vs 6 days, IQR 3-11; P = .04), a more frequent history of illicit drug use (33% vs 6%; P = .003), a higher frequency of psychiatric illness (27% vs 8%; P < .0001), and more were receiving opioid analgesics prior to admission (38% vs 17%; P = .007) than those hospitalized in other countries (Table 1, Appendix 1). The primary admitting diagnoses for all patients in the study are listed in Appendix 2. Opioid prescribing practices across the individual sites are shown in Appendix 3.
Patients Taking Opioids Prior to Admission
After adjusting for relevant covariates, we found that more patients in the US were given opioids during their hospitalization and in higher doses than patients from other countries and more were prescribed opioids at discharge. Fewer patients in the US were dispensed nonopioid analgesics during their hospitalization than patients from other countries, but this difference was not significant (Table 2). Appendix 4 shows the types of nonopioid pain medications prescribed in the US and other countries.
After adjustment for relevant covariates, US patients reported greater pain severity at the time they completed their pain surveys. We found no significant difference in satisfaction with pain control between patients from the US and other countries in the models, regardless of whether we included average pain score or opioid receipt during hospitalization in the model (Table 3).
In unadjusted analyses, compared with patients hospitalized in other countries, more patients in the US stated that they would like a stronger dose of analgesic if they were still in pain, though the difference was nonsignificant, and US patients were more likely to agree with the statement that people become addicted to pain medication easily and less likely to agree with the statement that it is easier to endure pain than deal with the side effects of pain medications (Table 3).
Patients Not Taking Opioids Prior to Admission
After adjusting for relevant covariates, we found no significant difference in the proportion of US patients provided with nonopioid pain medications during their hospitalization compared with patients in other countries, but a greater percentage of US patients were given opioids during their hospitalization and at discharge and in higher doses (Table 2).
After adjusting for relevant covariates, US patients reported greater pain severity at the time they completed their pain surveys and greater pain severity in the 24-36 hours prior to completing the survey than patients from other countries, but we found no difference in patient satisfaction with pain control (Table 3). After we included the average pain score and whether or not opioids were given to the patient during their hospitalization in this model, patients in the US were more likely to report a higher level of satisfaction with pain control than patients in all other countries (P = .001).
In unadjusted analyses, compared with patients hospitalized in other countries, those in the US were less likely to agree with the statement that good patients avoid talking about pain (Table 3).
Patient Satisfaction and Opioid Receipt
Among patients cared for in the US, after controlling for the average pain score, we did not find a significant association between receiving opioids while in the hospital and satisfaction with pain control for patients who either did or did not endorse taking opioids prior to admission (P = .38 and P = .24, respectively). Among patients cared for in all other countries, after controlling for the average pain score, we found a significant association between receiving opioids while in the hospital and a lower level of satisfaction with pain control for patients who reported taking opioids prior to admission (P = .02) but not for patients who did not report taking opioids prior to admission (P = .08).
DISCUSSION
Compared with patients hospitalized in other countries, a greater percentage of those hospitalized in the US were prescribed opioid analgesics both during hospitalization and at the time of discharge, even after adjustment for pain severity. In addition, patients hospitalized in the US reported greater pain severity at the time they completed their pain surveys and in the 24 to 36 hours prior to completing the survey than patients from other countries. In this sample, satisfaction, beliefs, and expectations about pain control differed between patients in the US and other sites. Our study also suggests that opioid receipt did not lead to improved patient satisfaction with pain control.
The frequency with which we observed opioid analgesics being prescribed during hospitalization in US hospitals (79%) was higher than the 51% of patients who received opioids reported by Herzig and colleagues.10 Patients in our study had a higher prevalence of illicit drug abuse and psychiatric illness, and our study only included patients who reported pain at some point during their hospitalization. We also studied prescribing practices through analysis of provider orders and medication administration records at the time the patient was hospitalized.
While we observed that physicians in the US more frequently prescribed opioid analgesics during hospitalizations than physicians working in other countries, we also observed that patients in the US reported higher levels of pain during their hospitalization. After adjusting for a number of variables, including pain severity, however, we still found that opioids were more commonly prescribed during hospitalizations by physicians working in the US sites studied than by physicians in the non-US sites.
Opioid prescribing practices varied across the sites sampled in our study. While the US sites, Taiwan, and Korea tended to be heavier utilizers of opioids during hospitalization, there were notable differences in discharge prescribing of opioids, with the US sites more commonly prescribing opioids and higher MME for patients who did not report taking opioids prior to their hospitalization (Appendix 3). A sensitivity analysis was conducted excluding South Korea from modeling, given that patients there were not asked about illicit opioid use. There were no important changes in the magnitude or direction of the results.
Our study supports previous studies indicating that there are cultural and societal differences when it comes to the experience of pain and the expectations around pain control.17,20-22,31 Much of the focus on reducing opioid utilization has been on provider practices32 and on prescription drug monitoring programs.33 Our findings suggest that another area of focus that may be important in mitigating the opioid epidemic is patient expectations of pain control.
Our study has a number of strengths. First, we included 11 hospitals from eight different countries. Second, we believe this is the first study to assess opioid prescribing and dispensing practices during hospitalization as well as at the time of discharge. Third, patient perceptions of pain control were assessed in conjunction with analgesic prescribing and were assessed during hospitalization. Fourth, we had high response rates for patient participation in our study. Fifth, we found much larger differences in opioid prescribing than anticipated, and thus, while we did not achieve the sample size originally planned for either the number of hospitals or patients enrolled per hospital, we were sufficiently powered. This is likely secondary to the fact that the population we studied was one that specifically reported pain, resulting in the larger differences seen.
Our study also had a number of limitations. First, the prescribing practices in countries other than the US are represented by only one hospital per country and, in some countries, by limited numbers of patients. While we studied four sites in the US, we did not have a site in the Northeast, a region previously shown to have lower prescribing rates.10 Additionally, patient samples for the US sites compared with the sites in other countries varied considerably with respect to ethnicity. While some studies in US patients have shown that opioid prescribing may vary based on race/ethnicity,34 we are uncertain as to how this might impact a study that crosses multiple countries. We also had a low number of patients receiving opioids prior to hospitalization for several of the non-US countries, which reduced the power to detect differences in this subgroup. Previous research has shown that there are wide variations in prescribing practices even within countries;10,12,18 therefore, caution should be taken when generalizing our findings. Second, we assessed analgesic prescribing patterns and pain control during the first 24 to 36 hours of hospitalization and did not consider hospital days beyond this timeframe with the exception of noting what medications were prescribed at discharge. We chose this methodology in an attempt to eliminate as many differences that might exist in the duration of hospitalization across many countries. Third, investigators in the study administered the survey, and respondents may have been affected by social desirability bias in how the survey questions were answered. Because investigators were not a part of the care team of any study patients, we believe this to be unlikely. Fourth, our study was conducted from October 8, 2013 to August 31, 2015 and the opioid epidemic is dynamic. Accordingly, our data may not reflect current opioid prescribing practices or patients’ current beliefs regarding pain control. Fifth, we did not collect demographic data on the patients who did not participate and could not look for systematic differences between participants and nonparticipants. Sixth, we relied on patients to self-report whether they were taking opioids prior to hospitalization or using illicit drugs. Seventh, we found comorbid mental health conditions to be more frequent in the US population studied. Previous work has shown regional variation in mental health conditions,35,36 which could have affected our findings. To account for this, our models included psychiatric illness.
CONCLUSIONS
Our data suggest that physicians in the US may prescribe opioids more frequently during patients’ hospitalizations and at discharge than their colleagues in other countries. We also found that patient satisfaction, beliefs, and expectations about pain control differed between patients in the US and other sites. Although the small number of hospitals included in our sample coupled with the small sample size in some of the non-US countries limits the generalizability of our findings, the data suggest that reducing the opioid epidemic in the US may require addressing patients’ expectations regarding pain control in addition to providers’ inpatient analgesic prescribing patterns.
Disclosures
The authors report no conflicts of interest.
Funding
The authors report no funding source for this work.
1. Pletcher MJ, Kertesz SG, Kohn MA, Gonzales R. Trends in opioid prescribing by race/ethnicity for patients seeking care in US emergency departments. JAMA. 2008;299(1):70-78. https://doi.org/10.1001/jama.2007.64.
2. Herzig SJ. Growing concerns regarding long-term opioid use: the hospitalization hazard. J Hosp Med. 2015;10(7):469-470. https://doi.org/10.1002/jhm.2369.
3. Guy GP Jr, Zhang K, Bohm MK, et al. Vital Signs: changes in opioid prescribing in the United States, 2006–2015. MMWR Morb Mortal Wkly Rep. 2017;66(26):697-704. https://doi.org/10.15585/mmwr.mm6626a4.
4. Okie S. A flood of opioids, a rising tide of deaths. N Engl J Med. 2010;363(21):1981-1985. https://doi.org/10.1056/NEJMp1011512.
5. Liang Y, Turner BJ. National cohort study of opioid analgesic dose and risk of future hospitalization. J Hosp Med. 2015;10(7):425-431. https://doi.org/10.1002/jhm.2350.
6. Han B, Compton WM, Blanco C, et al. Prescription opioid use, misuse, and use disorders in U.S. Adults: 2015 national survey on drug use and health. Ann Intern Med. 2017;167(5):293-301. https://doi.org/10.7326/M17-0865.
7. Schuchat A, Houry D, Guy GP, Jr. New data on opioid use and prescribing in the United States. JAMA. 2017;318(5):425-426. https://doi.org/10.1001/jama.2017.8913.
8. Sawyer J, Haslam L, Robinson S, Daines P, Stilos K. Pain prevalence study in a large Canadian teaching hospital. Pain Manag Nurs. 2008;9(3):104-112. https://doi.org/10.1016/j.pmn.2008.02.001.
9. Gupta A, Daigle S, Mojica J, Hurley RW. Patient perception of pain care in hospitals in the United States. J Pain Res. 2009;2:157-164. https://doi.org/10.2147/JPR.S7903.
10. Herzig SJ, Rothberg MB, Cheung M, Ngo LH, Marcantonio ER. Opioid utilization and opioid-related adverse events in nonsurgical patients in US hospitals. J Hosp Med. 2014;9(2):73-81. https://doi.org/10.1002/jhm.2102.
11. Kanjanarat P, Winterstein AG, Johns TE, et al. Nature of preventable adverse drug events in hospitals: a literature review. Am J Health Syst Pharm. 2003;60(17):1750-1759. https://doi.org/10.1093/ajhp/60.17.1750.
12. Jena AB, Goldman D, Karaca-Mandic P. Hospital prescribing of opioids to medicare beneficiaries. JAMA Intern Med. 2016;176(7):990-997. https://doi.org/10.1001/jamainternmed.2016.2737.
13. Hooten WM, St Sauver JL, McGree ME, Jacobson DJ, Warner DO. Incidence and risk factors for progression From short-term to episodic or long-term opioid prescribing: A population-based study. Mayo Clin Proc. 2015;90(7):850-856. https://doi.org/10.1016/j.mayocp.2015.04.012.
14. Alam A, Gomes T, Zheng H, et al. Long-term analgesic use after low-risk surgery: a retrospective cohort study. Arch Intern Med. 2012;172(5):425-430. https://doi.org/10.1001/archinternmed.2011.1827.
15. Barnett ML, Olenski AR, Jena AB. Opioid-prescribing patterns of emergency physicians and risk of long-term use. N Engl J Med. 2017;376(7):663-673. https://doi.org/10.1056/NEJMsa1610524.
16. Calcaterra SL, Scarbro S, Hull ML, et al. Prediction of future chronic opioid use Among hospitalized patients. J Gen Intern Med. 2018;33(6):898-905. https://doi.org/10.1007/s11606-018-4335-8.
17. Callister LC. Cultural influences on pain perceptions and behaviors. Home Health Care Manag Pract. 2003;15(3):207-211. https://doi.org/10.1177/1084822302250687.
18. Paulozzi LJ, Mack KA, Hockenberry JM. Vital signs: Variation among states in prescribing of opioid pain relievers and benzodiazepines--United States, 2012. J Saf Res. 2014;63(26):563-568. https://doi.org/10.1016/j.jsr.2014.09.001.
19. Callister LC, Khalaf I, Semenic S, Kartchner R, Vehvilainen-Julkunen K. The pain of childbirth: perceptions of culturally diverse women. Pain Manag Nurs. 2003;4(4):145-154. https://doi.org/10.1016/S1524-9042(03)00028-6.
20. Moore R, Brødsgaard I, Mao TK, Miller ML, Dworkin SF. Perceived need for local anesthesia in tooth drilling among Anglo-Americans, Chinese, and Scandinavians. Anesth Prog. 1998;45(1):22-28.
21. Kankkunen PM, Vehviläinen-Julkunen KM, Pietilä AM, et al. A tale of two countries: comparison of the perceptions of analgesics among Finnish and American parents. Pain Manag Nurs. 2008;9(3):113-119. https://doi.org/10.1016/j.pmn.2007.12.003.
22. Hanoch Y, Katsikopoulos KV, Gummerum M, Brass EP. American and German students’ knowledge, perceptions, and behaviors with respect to over-the-counter pain relievers. Health Psychol. 2007;26(6):802-806. https://doi.org/10.1037/0278-6133.26.6.802.
23. Manjiani D, Paul DB, Kunnumpurath S, Kaye AD, Vadivelu N. Availability and utilization of opioids for pain management: global issues. Ochsner J. 2014;14(2):208-215.
24. Quality improvement guidelines for the treatment of acute pain and cancer pain. JAMA. 1995;274(23):1874-1880.
25. McNeill JA, Sherwood GD, Starck PL, Thompson CJ. Assessing clinical outcomes: patient satisfaction with pain management. J Pain Symptom Manag. 1998;16(1):29-40. https://doi.org/10.1016/S0885-3924(98)00034-7.
26. Ferrari R, Novello C, Catania G, Visentin M. Patients’ satisfaction with pain management: the Italian version of the Patient Outcome Questionnaire of the American Pain Society. Recenti Prog Med. 2010;101(7–8):283-288.
27. Malouf J, Andión O, Torrubia R, Cañellas M, Baños JE. A survey of perceptions with pain management in Spanish inpatients. J Pain Symptom Manag. 2006;32(4):361-371. https://doi.org/10.1016/j.jpainsymman.2006.05.006.
28. Gordon DB, Polomano RC, Pellino TA, et al. Revised American Pain Society Patient Outcome Questionnaire (APS-POQ-R) for quality improvement of pain management in hospitalized adults: preliminary psychometric evaluation. J Pain. 2010;11(11):1172-1186. https://doi.org/10.1016/j.jpain.2010.02.012.
29. Beaton DE, Bombardier C, Guillemin F, Ferraz MB. Guidelines for the process of cross-cultural adaptation of self-report measures. Spine (Phila Pa 1976). 2000;25(24):3186-3191. https://doi.org/10.1097/00007632-200012150-00014.
30. Harris PA, Taylor R, Thielke R, et al. Research Electronic Data Capture (REDCap)--a metadata-driven methodology and workflow process for providing translational research informatics support. J Biomed Inform. 2009;42(2):377-381. https://doi.org/10.1016/j.jbi.2008.08.010.
31. Duman F. After surgery in Germany, I wanted Vicodin, not herbal tea. New York Times. January 27, 2018. https://www.nytimes.com/2018/01/27/opinion/sunday/surgery-germany-vicodin.html. Accessed November 6, 2018.
32. Beaudoin FL, Banerjee GN, Mello MJ. State-level and system-level opioid prescribing policies: the impact on provider practices and overdose deaths, a systematic review. J Opioid Manag. 2016;12(2):109-118. https://doi.org/10.5055/jom.2016.0322.33. Bao Y, Wen K, Johnson P, et al. Assessing the impact of state policies for prescription drug monitoring programs on high-risk opioid prescriptions. Health Aff (Millwood). 2018;37(10):1596-1604. https://doi.org/10.1377/hlthaff.2018.0512.
34. Friedman J, Kim D, Schneberk T, et al. Assessment of racial/ethnic and income disparities in the prescription of opioids and other controlled medications in California. JAMA Intern Med. 2019. https://doi.org/10.1001/jamainternmed.2018.6721.
35. Steel Z, Marnane C, Iranpour C, et al. The global prevalence of common mental disorders: a systematic review and meta-analysis 1980-2013. Int J Epidemiol. 2014;43(2):476-493. https://doi.org/10.1093/ije/dyu038.
36. Simon GE, Goldberg DP, Von Korff M, Ustün TB. Understanding cross-national differences in depression prevalence. Psychol Med. 2002;32(4):585-594. https://doi.org/10.1017/S0033291702005457.
Since 2000, the United States has seen a marked increase in opioid prescribing1-3 and opioid-related complications, including overdoses, hospitalizations, and deaths.2,4,5 A study from 2015 showed that more than one-third of the US civilian noninstitutionalized population reported receiving an opioid prescription in the prior year, with 12.5% reporting misuse, and, of those, 16.7% reported a prescription use disorder.6 While there has been a slight decrease in opioid prescriptions in the US since 2012, rates of opioid prescribing in 2015 were three times higher than in 1999 and approximately four times higher than in Europe in 2015.3,7
Pain is commonly reported by hospitalized patients,8,9 and opioids are often a mainstay of treatment;9,10 however, treatment with opioids can have a number of adverse outcomes.2,10,11 Short-term exposure to opioids can lead to long-term use,12-16 and patients on opioids are at an increased risk for subsequent hospitalization and longer inpatient lengths of stay.5
Physician prescribing practices for opioids and patient expectations for pain control vary as a function of geographic region and culture,10,12,17,18 and pain is influenced by the cultural context in which it occurs.17,19-22 Treatment of pain may also be affected by limited access to or restrictions on selected medications, as well as by cultural biases.23 Whether these variations in the treatment of pain are reflected in patients’ satisfaction with pain control is uncertain.
We sought to compare the inpatient analgesic prescribing practices and patients’ perceptions of pain control for medical patients in four teaching hospitals in the US and in seven teaching hospitals in seven other countries.
METHODS
Study Design
We utilized a cross-sectional, observational design. The study was approved by the Institutional Review Boards at all participating sites.
Setting
The study was conducted at 11 academic hospitals in eight countries from October 8, 2013 to August 31, 2015. Sites in the US included Denver Health in Denver, Colorado; the University of Colorado Hospital in Aurora, Colorado; Hennepin Healthcare in Minneapolis, Minnesota; and Legacy Health in Portland, Oregon. Sites outside the US included McMaster University in Hamilton, Ontario, Canada; Hospital de la Santa Creu i Sant Pau, Universitat Autonòma de Barcelona in Barcelona, Spain; the University of Study of Milan and the University Ospedale “Luigi Sacco” in Milan, Italy, the National Taiwan University Hospital, in Taipei, Taiwan, the University of Ulsan College of Medicine, Asan Medical Center, in Seoul, Korea, the Imperial College, Chelsea and Westminster Hospital, in London, United Kingdom and Dunedin Hospital, Dunedin, New Zealand.
Inclusion and Exclusion Criteria
We included patients 18-89 years of age (20-89 in Taiwan because patients under 20 years of age in this country are a restricted group with respect to participating in research), admitted to an internal medicine service from the Emergency Department or Urgent Care clinic with an acute illness for a minimum of 24 hours (with time zero defined as the time care was initiated in the Emergency Department or Urgent Care Clinic), who reported pain at some time during the first 24-36 hours of their hospitalization and who provided informed consent. In the US, “admission” included both observation and inpatient status. We limited the patient population to those admitted via emergency departments and urgent care clinics in order to enroll similar patient populations across sites.
Scheduled admissions, patients transferred from an outside facility, patients admitted directly from a clinic, and those receiving care in intensive care units were excluded. We also excluded patients who were incarcerated, pregnant, those who received major surgery within the previous 14 days, those with a known diagnosis of active cancer, and those who were receiving palliative or hospice care. Patients receiving care from an investigator in the study at the time of enrollment were not eligible due to the potential conflict of interest.
Patient Screening
Primary teams were contacted to determine if any patients on their service might meet the criteria for inclusion in the study on preselected study days chosen on the basis of the research team’s availability. Identified patients were then screened to establish if they met the eligibility criteria. Patients were asked directly if they had experienced pain during their preadmission evaluation or during their hospitalization.
Data Collection
All patients were hospitalized at the time they gave consent and when data were collected. Data were collected via interviews with patients, as well as through chart review. We recorded patients’ age, gender, race, admitting diagnosis(es), length of stay, psychiatric illness, illicit drug use, whether they reported receiving opioid analgesics at the time of hospitalization, whether they were prescribed opioids and/or nonopioid analgesics during their hospitalization, the median and maximum doses of opioids prescribed and dispensed, and whether they were discharged on opioids. The question of illicit drug use was asked of all patients with the exception of those hospitalized in South Korea due to potential legal implications.
Opioid prescribing and receipt of opioids was recorded based upon current provider orders and medication administration records, respectively. Perception of and satisfaction with pain control was assessed with the American Pain Society Patient Outcome Questionnaire–Modified (APS-POQ-Modified).24,25 Versions of this survey have been validated in English as well as in other languages and cultures.26-28 Because hospitalization practices could differ across hospitals and in different countries, we compared patients’ severity of illness by using Charlson comorbidity scores. Consent forms and the APS-POQ were translated into each country’s primary language according to established processes.29 The survey was filled out by having site investigators read questions aloud and by use of a large-font visual analog scale to aid patients’ verbal responses.
Data were collected and managed using a secure, web-based application electronic data capture tool (Research Electronic Data Capture [REDCap], Nashville, Tennessee), hosted at Denver Health.30
Study Size
Preliminary data from the internal medicine units at our institution suggested that 40% of patients without cancer received opioid analgesics during their hospitalization. Assuming 90% power to detect an absolute difference in the proportion of inpatient medical patients who are receiving opioid analgesics during their hospital stay of 17%, a two-sided type 1 error rate of 0.05, six hospitals in the US, and nine hospitals from all other countries, we calculated an initial sample size of 150 patients per site. This sample size was considered feasible for enrollment in a busy inpatient clinical setting. Study end points were to either reach the goal number of patients (150 per site) or the predetermined study end date, whichever came first.
Data Analysis
We generated means with standard deviations (SDs) and medians with interquartile ranges (IQRs) for normally and nonnormally distributed continuous variables, respectively, and frequencies for categorical variables. We used linear mixed modeling for the analysis of continuous variables. For binary outcomes, our data were fitted to a generalized linear mixed model with logit as the link function and a binary distribution. For ordinal variables, specifically patient-reported satisfaction with pain control and the opinion statements, the data were fitted to a generalized linear mixed model with a cumulative logit link and a multinomial distribution. Hospital was included as a random effect in all models to account for patients cared for in the same hospital.
Country of origin, dichotomized as US or non-US, was the independent variable of interest for all models. An interaction term for exposure to opioids prior to admission and country was entered into all models to explore whether differences in the effect of country existed for patients who reported taking opioids prior to admission and those who did not.
The models for the frequency with which analgesics were given, doses of opioids given during hospitalization and at discharge, patient-reported pain score, and patient-reported satisfaction with pain control were adjusted for (1) age, (2) gender, (3) Charlson Comorbidity Index, (4) length of stay, (5) history of illicit drug use, (6) history of psychiatric illness, (7) daily dose in morphine milligram equivalents (MME) for opioids prior to admission, (8) average pain score, and (9) hospital. The patient-reported satisfaction with pain control model was also adjusted for whether or not opioids were given to the patient during their hospitalization. P < .05 was considered to indicate significance. All analyses were performed using SAS Enterprise Guide 7.1 (SAS Institute, Inc., Cary, North Carolina). We reported data on medications that were prescribed and dispensed (as opposed to just prescribed and not necessarily given). Opioids prescribed at discharge represented the total possible opioids that could be given based upon the order/prescription (eg, oxycodone 5 mg every 6 hours as needed for pain would be counted as 20 mg/24 hours maximum possible dose followed by conversion to MME).
Missing Data
When there were missing data, a query was sent to sites to verify if the data were retrievable. If retrievable, the data were then entered. Data were missing in 5% and 2% of patients who did or did not report taking an opioid prior to admission, respectively. If a variable was included in a specific statistical test, then subjects with missing data were excluded from that analysis (ie, complete case analysis).
RESULTS
We approached 1,309 eligible patients, of which 981 provided informed consent, for a response rate of 75%; 503 from the US and 478 patients from other countries (Figure). In unadjusted analyses, we found no significant differences between US and non-US patients in age (mean age 51, SD 15 vs 59, SD 19; P = .30), race, ethnicity, or Charlson comorbidity index scores (median 2, IQR 1-3 vs 3, IQR 1-4; P = .45). US patients had shorter lengths of stay (median 3 days, IQR 2-4 vs 6 days, IQR 3-11; P = .04), a more frequent history of illicit drug use (33% vs 6%; P = .003), a higher frequency of psychiatric illness (27% vs 8%; P < .0001), and more were receiving opioid analgesics prior to admission (38% vs 17%; P = .007) than those hospitalized in other countries (Table 1, Appendix 1). The primary admitting diagnoses for all patients in the study are listed in Appendix 2. Opioid prescribing practices across the individual sites are shown in Appendix 3.
Patients Taking Opioids Prior to Admission
After adjusting for relevant covariates, we found that more patients in the US were given opioids during their hospitalization and in higher doses than patients from other countries and more were prescribed opioids at discharge. Fewer patients in the US were dispensed nonopioid analgesics during their hospitalization than patients from other countries, but this difference was not significant (Table 2). Appendix 4 shows the types of nonopioid pain medications prescribed in the US and other countries.
After adjustment for relevant covariates, US patients reported greater pain severity at the time they completed their pain surveys. We found no significant difference in satisfaction with pain control between patients from the US and other countries in the models, regardless of whether we included average pain score or opioid receipt during hospitalization in the model (Table 3).
In unadjusted analyses, compared with patients hospitalized in other countries, more patients in the US stated that they would like a stronger dose of analgesic if they were still in pain, though the difference was nonsignificant, and US patients were more likely to agree with the statement that people become addicted to pain medication easily and less likely to agree with the statement that it is easier to endure pain than deal with the side effects of pain medications (Table 3).
Patients Not Taking Opioids Prior to Admission
After adjusting for relevant covariates, we found no significant difference in the proportion of US patients provided with nonopioid pain medications during their hospitalization compared with patients in other countries, but a greater percentage of US patients were given opioids during their hospitalization and at discharge and in higher doses (Table 2).
After adjusting for relevant covariates, US patients reported greater pain severity at the time they completed their pain surveys and greater pain severity in the 24-36 hours prior to completing the survey than patients from other countries, but we found no difference in patient satisfaction with pain control (Table 3). After we included the average pain score and whether or not opioids were given to the patient during their hospitalization in this model, patients in the US were more likely to report a higher level of satisfaction with pain control than patients in all other countries (P = .001).
In unadjusted analyses, compared with patients hospitalized in other countries, those in the US were less likely to agree with the statement that good patients avoid talking about pain (Table 3).
Patient Satisfaction and Opioid Receipt
Among patients cared for in the US, after controlling for the average pain score, we did not find a significant association between receiving opioids while in the hospital and satisfaction with pain control for patients who either did or did not endorse taking opioids prior to admission (P = .38 and P = .24, respectively). Among patients cared for in all other countries, after controlling for the average pain score, we found a significant association between receiving opioids while in the hospital and a lower level of satisfaction with pain control for patients who reported taking opioids prior to admission (P = .02) but not for patients who did not report taking opioids prior to admission (P = .08).
DISCUSSION
Compared with patients hospitalized in other countries, a greater percentage of those hospitalized in the US were prescribed opioid analgesics both during hospitalization and at the time of discharge, even after adjustment for pain severity. In addition, patients hospitalized in the US reported greater pain severity at the time they completed their pain surveys and in the 24 to 36 hours prior to completing the survey than patients from other countries. In this sample, satisfaction, beliefs, and expectations about pain control differed between patients in the US and other sites. Our study also suggests that opioid receipt did not lead to improved patient satisfaction with pain control.
The frequency with which we observed opioid analgesics being prescribed during hospitalization in US hospitals (79%) was higher than the 51% of patients who received opioids reported by Herzig and colleagues.10 Patients in our study had a higher prevalence of illicit drug abuse and psychiatric illness, and our study only included patients who reported pain at some point during their hospitalization. We also studied prescribing practices through analysis of provider orders and medication administration records at the time the patient was hospitalized.
While we observed that physicians in the US more frequently prescribed opioid analgesics during hospitalizations than physicians working in other countries, we also observed that patients in the US reported higher levels of pain during their hospitalization. After adjusting for a number of variables, including pain severity, however, we still found that opioids were more commonly prescribed during hospitalizations by physicians working in the US sites studied than by physicians in the non-US sites.
Opioid prescribing practices varied across the sites sampled in our study. While the US sites, Taiwan, and Korea tended to be heavier utilizers of opioids during hospitalization, there were notable differences in discharge prescribing of opioids, with the US sites more commonly prescribing opioids and higher MME for patients who did not report taking opioids prior to their hospitalization (Appendix 3). A sensitivity analysis was conducted excluding South Korea from modeling, given that patients there were not asked about illicit opioid use. There were no important changes in the magnitude or direction of the results.
Our study supports previous studies indicating that there are cultural and societal differences when it comes to the experience of pain and the expectations around pain control.17,20-22,31 Much of the focus on reducing opioid utilization has been on provider practices32 and on prescription drug monitoring programs.33 Our findings suggest that another area of focus that may be important in mitigating the opioid epidemic is patient expectations of pain control.
Our study has a number of strengths. First, we included 11 hospitals from eight different countries. Second, we believe this is the first study to assess opioid prescribing and dispensing practices during hospitalization as well as at the time of discharge. Third, patient perceptions of pain control were assessed in conjunction with analgesic prescribing and were assessed during hospitalization. Fourth, we had high response rates for patient participation in our study. Fifth, we found much larger differences in opioid prescribing than anticipated, and thus, while we did not achieve the sample size originally planned for either the number of hospitals or patients enrolled per hospital, we were sufficiently powered. This is likely secondary to the fact that the population we studied was one that specifically reported pain, resulting in the larger differences seen.
Our study also had a number of limitations. First, the prescribing practices in countries other than the US are represented by only one hospital per country and, in some countries, by limited numbers of patients. While we studied four sites in the US, we did not have a site in the Northeast, a region previously shown to have lower prescribing rates.10 Additionally, patient samples for the US sites compared with the sites in other countries varied considerably with respect to ethnicity. While some studies in US patients have shown that opioid prescribing may vary based on race/ethnicity,34 we are uncertain as to how this might impact a study that crosses multiple countries. We also had a low number of patients receiving opioids prior to hospitalization for several of the non-US countries, which reduced the power to detect differences in this subgroup. Previous research has shown that there are wide variations in prescribing practices even within countries;10,12,18 therefore, caution should be taken when generalizing our findings. Second, we assessed analgesic prescribing patterns and pain control during the first 24 to 36 hours of hospitalization and did not consider hospital days beyond this timeframe with the exception of noting what medications were prescribed at discharge. We chose this methodology in an attempt to eliminate as many differences that might exist in the duration of hospitalization across many countries. Third, investigators in the study administered the survey, and respondents may have been affected by social desirability bias in how the survey questions were answered. Because investigators were not a part of the care team of any study patients, we believe this to be unlikely. Fourth, our study was conducted from October 8, 2013 to August 31, 2015 and the opioid epidemic is dynamic. Accordingly, our data may not reflect current opioid prescribing practices or patients’ current beliefs regarding pain control. Fifth, we did not collect demographic data on the patients who did not participate and could not look for systematic differences between participants and nonparticipants. Sixth, we relied on patients to self-report whether they were taking opioids prior to hospitalization or using illicit drugs. Seventh, we found comorbid mental health conditions to be more frequent in the US population studied. Previous work has shown regional variation in mental health conditions,35,36 which could have affected our findings. To account for this, our models included psychiatric illness.
CONCLUSIONS
Our data suggest that physicians in the US may prescribe opioids more frequently during patients’ hospitalizations and at discharge than their colleagues in other countries. We also found that patient satisfaction, beliefs, and expectations about pain control differed between patients in the US and other sites. Although the small number of hospitals included in our sample coupled with the small sample size in some of the non-US countries limits the generalizability of our findings, the data suggest that reducing the opioid epidemic in the US may require addressing patients’ expectations regarding pain control in addition to providers’ inpatient analgesic prescribing patterns.
Disclosures
The authors report no conflicts of interest.
Funding
The authors report no funding source for this work.
Since 2000, the United States has seen a marked increase in opioid prescribing1-3 and opioid-related complications, including overdoses, hospitalizations, and deaths.2,4,5 A study from 2015 showed that more than one-third of the US civilian noninstitutionalized population reported receiving an opioid prescription in the prior year, with 12.5% reporting misuse, and, of those, 16.7% reported a prescription use disorder.6 While there has been a slight decrease in opioid prescriptions in the US since 2012, rates of opioid prescribing in 2015 were three times higher than in 1999 and approximately four times higher than in Europe in 2015.3,7
Pain is commonly reported by hospitalized patients,8,9 and opioids are often a mainstay of treatment;9,10 however, treatment with opioids can have a number of adverse outcomes.2,10,11 Short-term exposure to opioids can lead to long-term use,12-16 and patients on opioids are at an increased risk for subsequent hospitalization and longer inpatient lengths of stay.5
Physician prescribing practices for opioids and patient expectations for pain control vary as a function of geographic region and culture,10,12,17,18 and pain is influenced by the cultural context in which it occurs.17,19-22 Treatment of pain may also be affected by limited access to or restrictions on selected medications, as well as by cultural biases.23 Whether these variations in the treatment of pain are reflected in patients’ satisfaction with pain control is uncertain.
We sought to compare the inpatient analgesic prescribing practices and patients’ perceptions of pain control for medical patients in four teaching hospitals in the US and in seven teaching hospitals in seven other countries.
METHODS
Study Design
We utilized a cross-sectional, observational design. The study was approved by the Institutional Review Boards at all participating sites.
Setting
The study was conducted at 11 academic hospitals in eight countries from October 8, 2013 to August 31, 2015. Sites in the US included Denver Health in Denver, Colorado; the University of Colorado Hospital in Aurora, Colorado; Hennepin Healthcare in Minneapolis, Minnesota; and Legacy Health in Portland, Oregon. Sites outside the US included McMaster University in Hamilton, Ontario, Canada; Hospital de la Santa Creu i Sant Pau, Universitat Autonòma de Barcelona in Barcelona, Spain; the University of Study of Milan and the University Ospedale “Luigi Sacco” in Milan, Italy, the National Taiwan University Hospital, in Taipei, Taiwan, the University of Ulsan College of Medicine, Asan Medical Center, in Seoul, Korea, the Imperial College, Chelsea and Westminster Hospital, in London, United Kingdom and Dunedin Hospital, Dunedin, New Zealand.
Inclusion and Exclusion Criteria
We included patients 18-89 years of age (20-89 in Taiwan because patients under 20 years of age in this country are a restricted group with respect to participating in research), admitted to an internal medicine service from the Emergency Department or Urgent Care clinic with an acute illness for a minimum of 24 hours (with time zero defined as the time care was initiated in the Emergency Department or Urgent Care Clinic), who reported pain at some time during the first 24-36 hours of their hospitalization and who provided informed consent. In the US, “admission” included both observation and inpatient status. We limited the patient population to those admitted via emergency departments and urgent care clinics in order to enroll similar patient populations across sites.
Scheduled admissions, patients transferred from an outside facility, patients admitted directly from a clinic, and those receiving care in intensive care units were excluded. We also excluded patients who were incarcerated, pregnant, those who received major surgery within the previous 14 days, those with a known diagnosis of active cancer, and those who were receiving palliative or hospice care. Patients receiving care from an investigator in the study at the time of enrollment were not eligible due to the potential conflict of interest.
Patient Screening
Primary teams were contacted to determine if any patients on their service might meet the criteria for inclusion in the study on preselected study days chosen on the basis of the research team’s availability. Identified patients were then screened to establish if they met the eligibility criteria. Patients were asked directly if they had experienced pain during their preadmission evaluation or during their hospitalization.
Data Collection
All patients were hospitalized at the time they gave consent and when data were collected. Data were collected via interviews with patients, as well as through chart review. We recorded patients’ age, gender, race, admitting diagnosis(es), length of stay, psychiatric illness, illicit drug use, whether they reported receiving opioid analgesics at the time of hospitalization, whether they were prescribed opioids and/or nonopioid analgesics during their hospitalization, the median and maximum doses of opioids prescribed and dispensed, and whether they were discharged on opioids. The question of illicit drug use was asked of all patients with the exception of those hospitalized in South Korea due to potential legal implications.
Opioid prescribing and receipt of opioids was recorded based upon current provider orders and medication administration records, respectively. Perception of and satisfaction with pain control was assessed with the American Pain Society Patient Outcome Questionnaire–Modified (APS-POQ-Modified).24,25 Versions of this survey have been validated in English as well as in other languages and cultures.26-28 Because hospitalization practices could differ across hospitals and in different countries, we compared patients’ severity of illness by using Charlson comorbidity scores. Consent forms and the APS-POQ were translated into each country’s primary language according to established processes.29 The survey was filled out by having site investigators read questions aloud and by use of a large-font visual analog scale to aid patients’ verbal responses.
Data were collected and managed using a secure, web-based application electronic data capture tool (Research Electronic Data Capture [REDCap], Nashville, Tennessee), hosted at Denver Health.30
Study Size
Preliminary data from the internal medicine units at our institution suggested that 40% of patients without cancer received opioid analgesics during their hospitalization. Assuming 90% power to detect an absolute difference in the proportion of inpatient medical patients who are receiving opioid analgesics during their hospital stay of 17%, a two-sided type 1 error rate of 0.05, six hospitals in the US, and nine hospitals from all other countries, we calculated an initial sample size of 150 patients per site. This sample size was considered feasible for enrollment in a busy inpatient clinical setting. Study end points were to either reach the goal number of patients (150 per site) or the predetermined study end date, whichever came first.
Data Analysis
We generated means with standard deviations (SDs) and medians with interquartile ranges (IQRs) for normally and nonnormally distributed continuous variables, respectively, and frequencies for categorical variables. We used linear mixed modeling for the analysis of continuous variables. For binary outcomes, our data were fitted to a generalized linear mixed model with logit as the link function and a binary distribution. For ordinal variables, specifically patient-reported satisfaction with pain control and the opinion statements, the data were fitted to a generalized linear mixed model with a cumulative logit link and a multinomial distribution. Hospital was included as a random effect in all models to account for patients cared for in the same hospital.
Country of origin, dichotomized as US or non-US, was the independent variable of interest for all models. An interaction term for exposure to opioids prior to admission and country was entered into all models to explore whether differences in the effect of country existed for patients who reported taking opioids prior to admission and those who did not.
The models for the frequency with which analgesics were given, doses of opioids given during hospitalization and at discharge, patient-reported pain score, and patient-reported satisfaction with pain control were adjusted for (1) age, (2) gender, (3) Charlson Comorbidity Index, (4) length of stay, (5) history of illicit drug use, (6) history of psychiatric illness, (7) daily dose in morphine milligram equivalents (MME) for opioids prior to admission, (8) average pain score, and (9) hospital. The patient-reported satisfaction with pain control model was also adjusted for whether or not opioids were given to the patient during their hospitalization. P < .05 was considered to indicate significance. All analyses were performed using SAS Enterprise Guide 7.1 (SAS Institute, Inc., Cary, North Carolina). We reported data on medications that were prescribed and dispensed (as opposed to just prescribed and not necessarily given). Opioids prescribed at discharge represented the total possible opioids that could be given based upon the order/prescription (eg, oxycodone 5 mg every 6 hours as needed for pain would be counted as 20 mg/24 hours maximum possible dose followed by conversion to MME).
Missing Data
When there were missing data, a query was sent to sites to verify if the data were retrievable. If retrievable, the data were then entered. Data were missing in 5% and 2% of patients who did or did not report taking an opioid prior to admission, respectively. If a variable was included in a specific statistical test, then subjects with missing data were excluded from that analysis (ie, complete case analysis).
RESULTS
We approached 1,309 eligible patients, of which 981 provided informed consent, for a response rate of 75%; 503 from the US and 478 patients from other countries (Figure). In unadjusted analyses, we found no significant differences between US and non-US patients in age (mean age 51, SD 15 vs 59, SD 19; P = .30), race, ethnicity, or Charlson comorbidity index scores (median 2, IQR 1-3 vs 3, IQR 1-4; P = .45). US patients had shorter lengths of stay (median 3 days, IQR 2-4 vs 6 days, IQR 3-11; P = .04), a more frequent history of illicit drug use (33% vs 6%; P = .003), a higher frequency of psychiatric illness (27% vs 8%; P < .0001), and more were receiving opioid analgesics prior to admission (38% vs 17%; P = .007) than those hospitalized in other countries (Table 1, Appendix 1). The primary admitting diagnoses for all patients in the study are listed in Appendix 2. Opioid prescribing practices across the individual sites are shown in Appendix 3.
Patients Taking Opioids Prior to Admission
After adjusting for relevant covariates, we found that more patients in the US were given opioids during their hospitalization and in higher doses than patients from other countries and more were prescribed opioids at discharge. Fewer patients in the US were dispensed nonopioid analgesics during their hospitalization than patients from other countries, but this difference was not significant (Table 2). Appendix 4 shows the types of nonopioid pain medications prescribed in the US and other countries.
After adjustment for relevant covariates, US patients reported greater pain severity at the time they completed their pain surveys. We found no significant difference in satisfaction with pain control between patients from the US and other countries in the models, regardless of whether we included average pain score or opioid receipt during hospitalization in the model (Table 3).
In unadjusted analyses, compared with patients hospitalized in other countries, more patients in the US stated that they would like a stronger dose of analgesic if they were still in pain, though the difference was nonsignificant, and US patients were more likely to agree with the statement that people become addicted to pain medication easily and less likely to agree with the statement that it is easier to endure pain than deal with the side effects of pain medications (Table 3).
Patients Not Taking Opioids Prior to Admission
After adjusting for relevant covariates, we found no significant difference in the proportion of US patients provided with nonopioid pain medications during their hospitalization compared with patients in other countries, but a greater percentage of US patients were given opioids during their hospitalization and at discharge and in higher doses (Table 2).
After adjusting for relevant covariates, US patients reported greater pain severity at the time they completed their pain surveys and greater pain severity in the 24-36 hours prior to completing the survey than patients from other countries, but we found no difference in patient satisfaction with pain control (Table 3). After we included the average pain score and whether or not opioids were given to the patient during their hospitalization in this model, patients in the US were more likely to report a higher level of satisfaction with pain control than patients in all other countries (P = .001).
In unadjusted analyses, compared with patients hospitalized in other countries, those in the US were less likely to agree with the statement that good patients avoid talking about pain (Table 3).
Patient Satisfaction and Opioid Receipt
Among patients cared for in the US, after controlling for the average pain score, we did not find a significant association between receiving opioids while in the hospital and satisfaction with pain control for patients who either did or did not endorse taking opioids prior to admission (P = .38 and P = .24, respectively). Among patients cared for in all other countries, after controlling for the average pain score, we found a significant association between receiving opioids while in the hospital and a lower level of satisfaction with pain control for patients who reported taking opioids prior to admission (P = .02) but not for patients who did not report taking opioids prior to admission (P = .08).
DISCUSSION
Compared with patients hospitalized in other countries, a greater percentage of those hospitalized in the US were prescribed opioid analgesics both during hospitalization and at the time of discharge, even after adjustment for pain severity. In addition, patients hospitalized in the US reported greater pain severity at the time they completed their pain surveys and in the 24 to 36 hours prior to completing the survey than patients from other countries. In this sample, satisfaction, beliefs, and expectations about pain control differed between patients in the US and other sites. Our study also suggests that opioid receipt did not lead to improved patient satisfaction with pain control.
The frequency with which we observed opioid analgesics being prescribed during hospitalization in US hospitals (79%) was higher than the 51% of patients who received opioids reported by Herzig and colleagues.10 Patients in our study had a higher prevalence of illicit drug abuse and psychiatric illness, and our study only included patients who reported pain at some point during their hospitalization. We also studied prescribing practices through analysis of provider orders and medication administration records at the time the patient was hospitalized.
While we observed that physicians in the US more frequently prescribed opioid analgesics during hospitalizations than physicians working in other countries, we also observed that patients in the US reported higher levels of pain during their hospitalization. After adjusting for a number of variables, including pain severity, however, we still found that opioids were more commonly prescribed during hospitalizations by physicians working in the US sites studied than by physicians in the non-US sites.
Opioid prescribing practices varied across the sites sampled in our study. While the US sites, Taiwan, and Korea tended to be heavier utilizers of opioids during hospitalization, there were notable differences in discharge prescribing of opioids, with the US sites more commonly prescribing opioids and higher MME for patients who did not report taking opioids prior to their hospitalization (Appendix 3). A sensitivity analysis was conducted excluding South Korea from modeling, given that patients there were not asked about illicit opioid use. There were no important changes in the magnitude or direction of the results.
Our study supports previous studies indicating that there are cultural and societal differences when it comes to the experience of pain and the expectations around pain control.17,20-22,31 Much of the focus on reducing opioid utilization has been on provider practices32 and on prescription drug monitoring programs.33 Our findings suggest that another area of focus that may be important in mitigating the opioid epidemic is patient expectations of pain control.
Our study has a number of strengths. First, we included 11 hospitals from eight different countries. Second, we believe this is the first study to assess opioid prescribing and dispensing practices during hospitalization as well as at the time of discharge. Third, patient perceptions of pain control were assessed in conjunction with analgesic prescribing and were assessed during hospitalization. Fourth, we had high response rates for patient participation in our study. Fifth, we found much larger differences in opioid prescribing than anticipated, and thus, while we did not achieve the sample size originally planned for either the number of hospitals or patients enrolled per hospital, we were sufficiently powered. This is likely secondary to the fact that the population we studied was one that specifically reported pain, resulting in the larger differences seen.
Our study also had a number of limitations. First, the prescribing practices in countries other than the US are represented by only one hospital per country and, in some countries, by limited numbers of patients. While we studied four sites in the US, we did not have a site in the Northeast, a region previously shown to have lower prescribing rates.10 Additionally, patient samples for the US sites compared with the sites in other countries varied considerably with respect to ethnicity. While some studies in US patients have shown that opioid prescribing may vary based on race/ethnicity,34 we are uncertain as to how this might impact a study that crosses multiple countries. We also had a low number of patients receiving opioids prior to hospitalization for several of the non-US countries, which reduced the power to detect differences in this subgroup. Previous research has shown that there are wide variations in prescribing practices even within countries;10,12,18 therefore, caution should be taken when generalizing our findings. Second, we assessed analgesic prescribing patterns and pain control during the first 24 to 36 hours of hospitalization and did not consider hospital days beyond this timeframe with the exception of noting what medications were prescribed at discharge. We chose this methodology in an attempt to eliminate as many differences that might exist in the duration of hospitalization across many countries. Third, investigators in the study administered the survey, and respondents may have been affected by social desirability bias in how the survey questions were answered. Because investigators were not a part of the care team of any study patients, we believe this to be unlikely. Fourth, our study was conducted from October 8, 2013 to August 31, 2015 and the opioid epidemic is dynamic. Accordingly, our data may not reflect current opioid prescribing practices or patients’ current beliefs regarding pain control. Fifth, we did not collect demographic data on the patients who did not participate and could not look for systematic differences between participants and nonparticipants. Sixth, we relied on patients to self-report whether they were taking opioids prior to hospitalization or using illicit drugs. Seventh, we found comorbid mental health conditions to be more frequent in the US population studied. Previous work has shown regional variation in mental health conditions,35,36 which could have affected our findings. To account for this, our models included psychiatric illness.
CONCLUSIONS
Our data suggest that physicians in the US may prescribe opioids more frequently during patients’ hospitalizations and at discharge than their colleagues in other countries. We also found that patient satisfaction, beliefs, and expectations about pain control differed between patients in the US and other sites. Although the small number of hospitals included in our sample coupled with the small sample size in some of the non-US countries limits the generalizability of our findings, the data suggest that reducing the opioid epidemic in the US may require addressing patients’ expectations regarding pain control in addition to providers’ inpatient analgesic prescribing patterns.
Disclosures
The authors report no conflicts of interest.
Funding
The authors report no funding source for this work.
1. Pletcher MJ, Kertesz SG, Kohn MA, Gonzales R. Trends in opioid prescribing by race/ethnicity for patients seeking care in US emergency departments. JAMA. 2008;299(1):70-78. https://doi.org/10.1001/jama.2007.64.
2. Herzig SJ. Growing concerns regarding long-term opioid use: the hospitalization hazard. J Hosp Med. 2015;10(7):469-470. https://doi.org/10.1002/jhm.2369.
3. Guy GP Jr, Zhang K, Bohm MK, et al. Vital Signs: changes in opioid prescribing in the United States, 2006–2015. MMWR Morb Mortal Wkly Rep. 2017;66(26):697-704. https://doi.org/10.15585/mmwr.mm6626a4.
4. Okie S. A flood of opioids, a rising tide of deaths. N Engl J Med. 2010;363(21):1981-1985. https://doi.org/10.1056/NEJMp1011512.
5. Liang Y, Turner BJ. National cohort study of opioid analgesic dose and risk of future hospitalization. J Hosp Med. 2015;10(7):425-431. https://doi.org/10.1002/jhm.2350.
6. Han B, Compton WM, Blanco C, et al. Prescription opioid use, misuse, and use disorders in U.S. Adults: 2015 national survey on drug use and health. Ann Intern Med. 2017;167(5):293-301. https://doi.org/10.7326/M17-0865.
7. Schuchat A, Houry D, Guy GP, Jr. New data on opioid use and prescribing in the United States. JAMA. 2017;318(5):425-426. https://doi.org/10.1001/jama.2017.8913.
8. Sawyer J, Haslam L, Robinson S, Daines P, Stilos K. Pain prevalence study in a large Canadian teaching hospital. Pain Manag Nurs. 2008;9(3):104-112. https://doi.org/10.1016/j.pmn.2008.02.001.
9. Gupta A, Daigle S, Mojica J, Hurley RW. Patient perception of pain care in hospitals in the United States. J Pain Res. 2009;2:157-164. https://doi.org/10.2147/JPR.S7903.
10. Herzig SJ, Rothberg MB, Cheung M, Ngo LH, Marcantonio ER. Opioid utilization and opioid-related adverse events in nonsurgical patients in US hospitals. J Hosp Med. 2014;9(2):73-81. https://doi.org/10.1002/jhm.2102.
11. Kanjanarat P, Winterstein AG, Johns TE, et al. Nature of preventable adverse drug events in hospitals: a literature review. Am J Health Syst Pharm. 2003;60(17):1750-1759. https://doi.org/10.1093/ajhp/60.17.1750.
12. Jena AB, Goldman D, Karaca-Mandic P. Hospital prescribing of opioids to medicare beneficiaries. JAMA Intern Med. 2016;176(7):990-997. https://doi.org/10.1001/jamainternmed.2016.2737.
13. Hooten WM, St Sauver JL, McGree ME, Jacobson DJ, Warner DO. Incidence and risk factors for progression From short-term to episodic or long-term opioid prescribing: A population-based study. Mayo Clin Proc. 2015;90(7):850-856. https://doi.org/10.1016/j.mayocp.2015.04.012.
14. Alam A, Gomes T, Zheng H, et al. Long-term analgesic use after low-risk surgery: a retrospective cohort study. Arch Intern Med. 2012;172(5):425-430. https://doi.org/10.1001/archinternmed.2011.1827.
15. Barnett ML, Olenski AR, Jena AB. Opioid-prescribing patterns of emergency physicians and risk of long-term use. N Engl J Med. 2017;376(7):663-673. https://doi.org/10.1056/NEJMsa1610524.
16. Calcaterra SL, Scarbro S, Hull ML, et al. Prediction of future chronic opioid use Among hospitalized patients. J Gen Intern Med. 2018;33(6):898-905. https://doi.org/10.1007/s11606-018-4335-8.
17. Callister LC. Cultural influences on pain perceptions and behaviors. Home Health Care Manag Pract. 2003;15(3):207-211. https://doi.org/10.1177/1084822302250687.
18. Paulozzi LJ, Mack KA, Hockenberry JM. Vital signs: Variation among states in prescribing of opioid pain relievers and benzodiazepines--United States, 2012. J Saf Res. 2014;63(26):563-568. https://doi.org/10.1016/j.jsr.2014.09.001.
19. Callister LC, Khalaf I, Semenic S, Kartchner R, Vehvilainen-Julkunen K. The pain of childbirth: perceptions of culturally diverse women. Pain Manag Nurs. 2003;4(4):145-154. https://doi.org/10.1016/S1524-9042(03)00028-6.
20. Moore R, Brødsgaard I, Mao TK, Miller ML, Dworkin SF. Perceived need for local anesthesia in tooth drilling among Anglo-Americans, Chinese, and Scandinavians. Anesth Prog. 1998;45(1):22-28.
21. Kankkunen PM, Vehviläinen-Julkunen KM, Pietilä AM, et al. A tale of two countries: comparison of the perceptions of analgesics among Finnish and American parents. Pain Manag Nurs. 2008;9(3):113-119. https://doi.org/10.1016/j.pmn.2007.12.003.
22. Hanoch Y, Katsikopoulos KV, Gummerum M, Brass EP. American and German students’ knowledge, perceptions, and behaviors with respect to over-the-counter pain relievers. Health Psychol. 2007;26(6):802-806. https://doi.org/10.1037/0278-6133.26.6.802.
23. Manjiani D, Paul DB, Kunnumpurath S, Kaye AD, Vadivelu N. Availability and utilization of opioids for pain management: global issues. Ochsner J. 2014;14(2):208-215.
24. Quality improvement guidelines for the treatment of acute pain and cancer pain. JAMA. 1995;274(23):1874-1880.
25. McNeill JA, Sherwood GD, Starck PL, Thompson CJ. Assessing clinical outcomes: patient satisfaction with pain management. J Pain Symptom Manag. 1998;16(1):29-40. https://doi.org/10.1016/S0885-3924(98)00034-7.
26. Ferrari R, Novello C, Catania G, Visentin M. Patients’ satisfaction with pain management: the Italian version of the Patient Outcome Questionnaire of the American Pain Society. Recenti Prog Med. 2010;101(7–8):283-288.
27. Malouf J, Andión O, Torrubia R, Cañellas M, Baños JE. A survey of perceptions with pain management in Spanish inpatients. J Pain Symptom Manag. 2006;32(4):361-371. https://doi.org/10.1016/j.jpainsymman.2006.05.006.
28. Gordon DB, Polomano RC, Pellino TA, et al. Revised American Pain Society Patient Outcome Questionnaire (APS-POQ-R) for quality improvement of pain management in hospitalized adults: preliminary psychometric evaluation. J Pain. 2010;11(11):1172-1186. https://doi.org/10.1016/j.jpain.2010.02.012.
29. Beaton DE, Bombardier C, Guillemin F, Ferraz MB. Guidelines for the process of cross-cultural adaptation of self-report measures. Spine (Phila Pa 1976). 2000;25(24):3186-3191. https://doi.org/10.1097/00007632-200012150-00014.
30. Harris PA, Taylor R, Thielke R, et al. Research Electronic Data Capture (REDCap)--a metadata-driven methodology and workflow process for providing translational research informatics support. J Biomed Inform. 2009;42(2):377-381. https://doi.org/10.1016/j.jbi.2008.08.010.
31. Duman F. After surgery in Germany, I wanted Vicodin, not herbal tea. New York Times. January 27, 2018. https://www.nytimes.com/2018/01/27/opinion/sunday/surgery-germany-vicodin.html. Accessed November 6, 2018.
32. Beaudoin FL, Banerjee GN, Mello MJ. State-level and system-level opioid prescribing policies: the impact on provider practices and overdose deaths, a systematic review. J Opioid Manag. 2016;12(2):109-118. https://doi.org/10.5055/jom.2016.0322.33. Bao Y, Wen K, Johnson P, et al. Assessing the impact of state policies for prescription drug monitoring programs on high-risk opioid prescriptions. Health Aff (Millwood). 2018;37(10):1596-1604. https://doi.org/10.1377/hlthaff.2018.0512.
34. Friedman J, Kim D, Schneberk T, et al. Assessment of racial/ethnic and income disparities in the prescription of opioids and other controlled medications in California. JAMA Intern Med. 2019. https://doi.org/10.1001/jamainternmed.2018.6721.
35. Steel Z, Marnane C, Iranpour C, et al. The global prevalence of common mental disorders: a systematic review and meta-analysis 1980-2013. Int J Epidemiol. 2014;43(2):476-493. https://doi.org/10.1093/ije/dyu038.
36. Simon GE, Goldberg DP, Von Korff M, Ustün TB. Understanding cross-national differences in depression prevalence. Psychol Med. 2002;32(4):585-594. https://doi.org/10.1017/S0033291702005457.
1. Pletcher MJ, Kertesz SG, Kohn MA, Gonzales R. Trends in opioid prescribing by race/ethnicity for patients seeking care in US emergency departments. JAMA. 2008;299(1):70-78. https://doi.org/10.1001/jama.2007.64.
2. Herzig SJ. Growing concerns regarding long-term opioid use: the hospitalization hazard. J Hosp Med. 2015;10(7):469-470. https://doi.org/10.1002/jhm.2369.
3. Guy GP Jr, Zhang K, Bohm MK, et al. Vital Signs: changes in opioid prescribing in the United States, 2006–2015. MMWR Morb Mortal Wkly Rep. 2017;66(26):697-704. https://doi.org/10.15585/mmwr.mm6626a4.
4. Okie S. A flood of opioids, a rising tide of deaths. N Engl J Med. 2010;363(21):1981-1985. https://doi.org/10.1056/NEJMp1011512.
5. Liang Y, Turner BJ. National cohort study of opioid analgesic dose and risk of future hospitalization. J Hosp Med. 2015;10(7):425-431. https://doi.org/10.1002/jhm.2350.
6. Han B, Compton WM, Blanco C, et al. Prescription opioid use, misuse, and use disorders in U.S. Adults: 2015 national survey on drug use and health. Ann Intern Med. 2017;167(5):293-301. https://doi.org/10.7326/M17-0865.
7. Schuchat A, Houry D, Guy GP, Jr. New data on opioid use and prescribing in the United States. JAMA. 2017;318(5):425-426. https://doi.org/10.1001/jama.2017.8913.
8. Sawyer J, Haslam L, Robinson S, Daines P, Stilos K. Pain prevalence study in a large Canadian teaching hospital. Pain Manag Nurs. 2008;9(3):104-112. https://doi.org/10.1016/j.pmn.2008.02.001.
9. Gupta A, Daigle S, Mojica J, Hurley RW. Patient perception of pain care in hospitals in the United States. J Pain Res. 2009;2:157-164. https://doi.org/10.2147/JPR.S7903.
10. Herzig SJ, Rothberg MB, Cheung M, Ngo LH, Marcantonio ER. Opioid utilization and opioid-related adverse events in nonsurgical patients in US hospitals. J Hosp Med. 2014;9(2):73-81. https://doi.org/10.1002/jhm.2102.
11. Kanjanarat P, Winterstein AG, Johns TE, et al. Nature of preventable adverse drug events in hospitals: a literature review. Am J Health Syst Pharm. 2003;60(17):1750-1759. https://doi.org/10.1093/ajhp/60.17.1750.
12. Jena AB, Goldman D, Karaca-Mandic P. Hospital prescribing of opioids to medicare beneficiaries. JAMA Intern Med. 2016;176(7):990-997. https://doi.org/10.1001/jamainternmed.2016.2737.
13. Hooten WM, St Sauver JL, McGree ME, Jacobson DJ, Warner DO. Incidence and risk factors for progression From short-term to episodic or long-term opioid prescribing: A population-based study. Mayo Clin Proc. 2015;90(7):850-856. https://doi.org/10.1016/j.mayocp.2015.04.012.
14. Alam A, Gomes T, Zheng H, et al. Long-term analgesic use after low-risk surgery: a retrospective cohort study. Arch Intern Med. 2012;172(5):425-430. https://doi.org/10.1001/archinternmed.2011.1827.
15. Barnett ML, Olenski AR, Jena AB. Opioid-prescribing patterns of emergency physicians and risk of long-term use. N Engl J Med. 2017;376(7):663-673. https://doi.org/10.1056/NEJMsa1610524.
16. Calcaterra SL, Scarbro S, Hull ML, et al. Prediction of future chronic opioid use Among hospitalized patients. J Gen Intern Med. 2018;33(6):898-905. https://doi.org/10.1007/s11606-018-4335-8.
17. Callister LC. Cultural influences on pain perceptions and behaviors. Home Health Care Manag Pract. 2003;15(3):207-211. https://doi.org/10.1177/1084822302250687.
18. Paulozzi LJ, Mack KA, Hockenberry JM. Vital signs: Variation among states in prescribing of opioid pain relievers and benzodiazepines--United States, 2012. J Saf Res. 2014;63(26):563-568. https://doi.org/10.1016/j.jsr.2014.09.001.
19. Callister LC, Khalaf I, Semenic S, Kartchner R, Vehvilainen-Julkunen K. The pain of childbirth: perceptions of culturally diverse women. Pain Manag Nurs. 2003;4(4):145-154. https://doi.org/10.1016/S1524-9042(03)00028-6.
20. Moore R, Brødsgaard I, Mao TK, Miller ML, Dworkin SF. Perceived need for local anesthesia in tooth drilling among Anglo-Americans, Chinese, and Scandinavians. Anesth Prog. 1998;45(1):22-28.
21. Kankkunen PM, Vehviläinen-Julkunen KM, Pietilä AM, et al. A tale of two countries: comparison of the perceptions of analgesics among Finnish and American parents. Pain Manag Nurs. 2008;9(3):113-119. https://doi.org/10.1016/j.pmn.2007.12.003.
22. Hanoch Y, Katsikopoulos KV, Gummerum M, Brass EP. American and German students’ knowledge, perceptions, and behaviors with respect to over-the-counter pain relievers. Health Psychol. 2007;26(6):802-806. https://doi.org/10.1037/0278-6133.26.6.802.
23. Manjiani D, Paul DB, Kunnumpurath S, Kaye AD, Vadivelu N. Availability and utilization of opioids for pain management: global issues. Ochsner J. 2014;14(2):208-215.
24. Quality improvement guidelines for the treatment of acute pain and cancer pain. JAMA. 1995;274(23):1874-1880.
25. McNeill JA, Sherwood GD, Starck PL, Thompson CJ. Assessing clinical outcomes: patient satisfaction with pain management. J Pain Symptom Manag. 1998;16(1):29-40. https://doi.org/10.1016/S0885-3924(98)00034-7.
26. Ferrari R, Novello C, Catania G, Visentin M. Patients’ satisfaction with pain management: the Italian version of the Patient Outcome Questionnaire of the American Pain Society. Recenti Prog Med. 2010;101(7–8):283-288.
27. Malouf J, Andión O, Torrubia R, Cañellas M, Baños JE. A survey of perceptions with pain management in Spanish inpatients. J Pain Symptom Manag. 2006;32(4):361-371. https://doi.org/10.1016/j.jpainsymman.2006.05.006.
28. Gordon DB, Polomano RC, Pellino TA, et al. Revised American Pain Society Patient Outcome Questionnaire (APS-POQ-R) for quality improvement of pain management in hospitalized adults: preliminary psychometric evaluation. J Pain. 2010;11(11):1172-1186. https://doi.org/10.1016/j.jpain.2010.02.012.
29. Beaton DE, Bombardier C, Guillemin F, Ferraz MB. Guidelines for the process of cross-cultural adaptation of self-report measures. Spine (Phila Pa 1976). 2000;25(24):3186-3191. https://doi.org/10.1097/00007632-200012150-00014.
30. Harris PA, Taylor R, Thielke R, et al. Research Electronic Data Capture (REDCap)--a metadata-driven methodology and workflow process for providing translational research informatics support. J Biomed Inform. 2009;42(2):377-381. https://doi.org/10.1016/j.jbi.2008.08.010.
31. Duman F. After surgery in Germany, I wanted Vicodin, not herbal tea. New York Times. January 27, 2018. https://www.nytimes.com/2018/01/27/opinion/sunday/surgery-germany-vicodin.html. Accessed November 6, 2018.
32. Beaudoin FL, Banerjee GN, Mello MJ. State-level and system-level opioid prescribing policies: the impact on provider practices and overdose deaths, a systematic review. J Opioid Manag. 2016;12(2):109-118. https://doi.org/10.5055/jom.2016.0322.33. Bao Y, Wen K, Johnson P, et al. Assessing the impact of state policies for prescription drug monitoring programs on high-risk opioid prescriptions. Health Aff (Millwood). 2018;37(10):1596-1604. https://doi.org/10.1377/hlthaff.2018.0512.
34. Friedman J, Kim D, Schneberk T, et al. Assessment of racial/ethnic and income disparities in the prescription of opioids and other controlled medications in California. JAMA Intern Med. 2019. https://doi.org/10.1001/jamainternmed.2018.6721.
35. Steel Z, Marnane C, Iranpour C, et al. The global prevalence of common mental disorders: a systematic review and meta-analysis 1980-2013. Int J Epidemiol. 2014;43(2):476-493. https://doi.org/10.1093/ije/dyu038.
36. Simon GE, Goldberg DP, Von Korff M, Ustün TB. Understanding cross-national differences in depression prevalence. Psychol Med. 2002;32(4):585-594. https://doi.org/10.1017/S0033291702005457.
© 2019 Society of Hospital Medicine
Barriers to Early Hospital Discharge: A Cross-Sectional Study at Five Academic Hospitals
Hospital discharges frequently occur in the afternoon or evening hours.1-5 Late discharges can adversely affect patient flow throughout the hospital,3,6-9 which, in turn, can result in delays in care,10-16 more medication errors,17 increased mortality,18-20 longer lengths of stay,20-22 higher costs,23 and lower patient satisfaction.24
Various interventions have been employed in the attempts to find ways of moving discharge times to earlier in the day, including preparing the discharge paperwork and medications the previous night,25 using checklists,1,25 team huddles,2 providing real-time feedback to unit staff,1 and employing multidisciplinary teamwork.1,2,6,25,26
The purpose of this study was to identify and determine the relative frequency of barriers to writing discharge orders in the hopes of identifying issues that might be addressed by targeted interventions. We also assessed the effects of daily team census, patients being on teaching versus nonteaching services, and how daily rounds were structured at the time that the discharge orders were written.
METHODS
Study Design, Setting, and Participants
We conducted a prospective, cross-sectional survey of house-staff and attending physicians on general medicine teaching and nonteaching services from November 13, 2014, through May 31, 2016. The study was conducted at the following five hospitals: Denver Health Medical Center (DHMC) and Presbyterian/Saint Luke’s Medical Center (PSL) in Denver, Colorado; Ronald Reagan University (UCLA) and Los Angeles County/University of Southern California Medical Center (LAC+USC) in Los Angeles, California; and Harborview Medical Center (HMC) in Seattle, Washington. The study was approved by the Colorado Multi-Institutional Review Board as well as by the review boards of the other participating sites.
Data Collection
The results of the focus groups composed of attending physicians at DHMC were used to develop our initial data collection template. Additional sites joining the study provided feedback, leading to modifications (Appendix 1).
Physicians were surveyed at three different time points on study days that were selected according to the convenience of the investigators. The sampling occurred only on weekdays and was done based on the investigators’ availability. Investigators would attempt to survey as many teams as they were able to but, secondary to feasibility, not all teams could be surveyed on study days. The specific time points varied as a function of physician workflows but were standardized as much as possible to occur in the early morning, around noon, and midafternoon on weekdays. Physicians were contacted either in person or by telephone for verbal consent prior to administering the first survey. All general medicine teams were eligible. For teaching teams, the order of contact was resident, intern, and then attending based on which physician was available at the time of the survey and on which member of the team was thought to know the patients the best. For the nonteaching services, the attending physicians were contacted.
During the initial survey, the investigators assessed the provider role (ie, attending or housestaff), whether the service was a teaching or a nonteaching service, and the starting patient census on that service primarily based on interviewing the provider of record for the team and looking at team census lists. Physicians were asked about their rounding style (ie, sickest patients first, patients likely to be discharged first, room-by-room, most recently admitted patients first, patients on the team the longest, or other) and then to identify all patients they thought would be definite discharges sometime during the day of the survey. Definite discharges were defined as patients whom the provider thought were either currently ready for discharge or who had only minor barriers that, if unresolved, would not prevent same-day discharge. They were asked if the discharge order had been entered and, if not, what was preventing them from doing so, if the discharge could in their opinion have occurred the day prior and, if so, why this did not occur. We also obtained the date and time of the admission and discharge orders, the actual discharge time, as well as the length of stay either through chart review (majority of sites) or from data warehouses (Denver Health and Presbyterian St. Lukes had length of stay data retrieved from their data warehouse).
Physicians were also asked to identify all patients whom they thought might possibly be discharged that day. Possible discharges were defined as patients with barriers to discharge that, if unresolved, would prevent same-day discharge. For each of these, the physicians were asked to list whatever issues needed to be resolved prior to placing the discharge order (Appendix 1).
The second survey was administered late morning on the same day, typically between 11
The third survey was administered midafternoon, typically around 3 PM similar to the first two surveys, with the exception that the third survey did not attempt to identify new definite or possible discharges.
Sample Size
We stopped collecting data after obtaining a convenience sample of 5% of total discharges at each study site or on the study end date, which was May 31, 2016, whichever came first.
Data Analysis
Data were collected and managed using a secure, web-based application electronic data capture tool (REDCap), hosted at Denver Health. REDCap (Research Electronic Data Capture, Nashville, Tennessee) is designed to support data collection for research studies.27 Data were then analyzed using SAS Enterprise Guide 5.1 (SAS Institute, Inc., Cary, North Carolina). All data entered into REDCap were reviewed by the principal investigator to ensure that data were not missing, and when there were missing data, a query was sent to verify if the data were retrievable. If retrievable, then the data would be entered. The volume of missing data that remained is described in our results.
Continuous variables were described using means and standard deviations (SD) or medians and interquartile ranges (IQR) based on tests of normality. Differences in the time that the discharge orders were placed in the electronic medical record according to morning patient census, teaching versus nonteaching service, and rounding style were compared using the Wilcoxon rank sum test. Linear regression was used to evaluate the effect of patient census on discharge order time. P < .05 was considered as significant.
RESULTS
We conducted 1,584 patient evaluations through surveys of 254 physicians over 156 days. Given surveys coincided with the existing work we had full participation (ie, 100% participation) and no dropout during the study days. Median (IQR) survey time points were 8:30
The characteristics of the five hospitals participating in the study, the patients’ final discharge status, the types of physicians surveyed, the services on which they were working, the rounding styles employed, and the median starting daily census are summarized in Table 1. The majority of the physicians surveyed were housestaff working on teaching services, and only a small minority structured rounds such that patients ready for discharge were seen first.
Over the course of the three surveys, 949 patients were identified as being definite discharges at any time point, and the large majority of these (863, 91%) were discharged on the day of the survey. The median (IQR) time that the discharge orders were written was 11:50
During the initial morning survey, 314 patients were identified as being definite discharges for that day (representing approximately 6% of the total number of patients being cared for, or 33% of the patients identified as definite discharges throughout the day). Of these, the physicians thought that 44 (<1% of the total number of patients being cared for on the services) could have been discharged on the previous day. The most frequent reasons cited for why these patients were not discharged on the previous day were “Patient did not want to leave” (n = 15, 34%), “Too late in the day” (n = 10, 23%), and “No ride” (n = 9, 20%). The remaining 10 patients (23%) had a variety of reasons related to system or social issues (ie, shelter not available, miscommunication).
At the morning time point, the most common barriers to discharge identified were that the physicians had not finished rounding on their team of patients and that the housestaff needed to staff their patients with their attending. At noon, caring for other patients and tending to the discharge processes were most commonly cited, and in the afternoon, the most common barriers were that the physicians were in the process of completing the discharge paperwork for those patients or were discharging other patients (Table 2). When comparing barriers on teaching to nonteaching teams, a higher proportion of teaching teams were still rounding on all patients and were working on discharge paperwork at the second survey. Barriers cited by sites were similar; however, the frequency at which the barriers were mentioned varied (data not shown).
The physicians identified 1,237 patients at any time point as being possible discharges during the day of the survey and these had a mean (±SD) of 1.3 (±0.5) barriers cited for why these patients were possible rather than definite discharges. The most common were that clinical improvement was needed, one or more pending issues related to their care needed to be resolved, and/or awaiting pending test results. The need to see clinical improvement generally decreased throughout the day as did the need to staff patients with an attending physician, but barriers related to consultant recommendations or completing procedures increased (Table 3). Of the 1,237 patients ever identified as possible discharges, 594 (48%) became a definite discharge by the third call and 444 (36%) became a no discharge as their final status. As with definite discharges, barriers cited by sites were similar; however, the frequency at which the barriers were mentioned varied.
Among the 949 and 1,237 patients who were ever identified as definite or possible discharges, respectively, at any time point during the study day, 28 (3%) and 444 (36%), respectively, had their discharge status changed to no discharge, most commonly because their clinical condition either worsened or expected improvements did not occur or that barriers pertaining to social work, physical therapy, or occupational therapy were not resolved.
The median time that the discharge orders were entered into the electronic medical record was 43 minutes earlier if patients were on teams with a lower versus a higher starting census (P = .0003), 48 minutes earlier if they were seen by physicians whose rounding style was to see patients first who potentially could be discharged (P = .0026), and 58 minutes earlier if they were on nonteaching versus teaching services (P < .0001; Table 4). For every one-person increase in census, the discharge order time increased by 6 minutes (β = 5.6, SE = 1.6, P = .0003).
DISCUSSION
The important findings of this study are that (1) the large majority of issues thought to delay discharging patients identified as definite discharges were related to physicians caring for other patients on their team, (2) although 91% of patients ever identified as being definite discharges were discharged on the day of the survey, only 48% of those identified as possible discharges became definite discharges by the afternoon time point, largely because the anticipated clinical improvement did not occur or care being provided by ancillary services had not been completed, and (3) discharge orders on patients identified as definite discharges were written on average 50 minutes earlier by physicians on teams with a smaller starting patient census, on nonteaching services, or when the rounding style was to see patients ready for discharges first.
Previous research has reported that physician-perceived barriers to discharge were extrinsic to providers and even extrinsic to the hospital setting (eg, awaiting subacute nursing placement and transportation).28,29 However, many of the barriers that we identified were related directly to the providers’ workload and rounding styles and whether the patients were on teaching versus nonteaching services. We also found that delays in the ability of hospital services to complete care also contributed to delayed discharges.
Our observational data suggest that delays resulting from caring for other patients might be reduced by changing rounding styles such that patients ready for discharge are seen first and are discharged prior to seeing other patients on the team, as previously reported by Beck et al.30 Intuitively, this would seem to be a straightforward way of freeing up beds earlier in the day, but such a change will, of necessity, lead to delaying care for other patients, which, in turn, could increase their length of stays. Durvasula et al. suggested that discharges could be moved to earlier in the day by completing orders and paperwork the day prior to discharge.25 Such an approach might be effective on an Obstetrical or elective Orthopedic service on which patients predictably are hospitalized for a fixed number of days (or even hours) but may be less relevant to patients on internal medicine services where lengths of stay are less predictable. Interventions to improve discharge times have resulted in earlier discharge times in some studies,2,4 but the overall length of stay either did not decrease25 or increased31 in others. Werthheimer et al.1 did find earlier discharge times, but other interventions also occurred during the study period (eg, extending social work services to include weekends).1,32
We found that discharge times were approximately 50 minutes earlier on teams with a smaller starting census, on nonteaching compared with teaching services, or when the attending’s rounding style was to see patients ready for discharges first. Although 50 minutes may seem like a small change in discharge time, Khanna et al.33 found that when discharges occur even 1 hour earlier, hospital overcrowding is reduced. To have a lower team census would require having more teams and more providers to staff these teams, raising cost-effectiveness concerns. Moving to more nonteaching services could represent a conflict with respect to one of the missions of teaching hospitals and raises a cost-benefit issue as several teaching hospitals receive substantial funding in support of their teaching activities and housestaff would have to be replaced with more expensive providers.
Delays attributable to ancillary services indicate imbalances between demand and availability of these services. Inappropriate demand and inefficiencies could be reduced by systems redesign, but in at least some instances, additional resources will be needed to add staff, increase space, or add additional equipment.
Our study has several limitations. First, we surveyed only physicians working in university-affiliated hospitals, and three of these were public safety-net hospitals. Accordingly, our results may not be generalizable to different patient populations. Second, we surveyed only physicians, and Minichiello et al.29 found that barriers to discharge perceived by physicians were different from those of other staff. Third, our data were observational and were collected only on weekdays. Fourth, we did not differentiate interns from residents, and thus, potentially the level of training could have affected these results. Similarly, the decision for a “possible” and a “definite” discharge is likely dependent on the knowledge base of the participant, such that less experienced participants may have had differing perspectives than someone with more experience. Fifth, the sites did vary based on the infrastructure and support but also had several similarities. All sites had social work and case management involved in care, although at some sites, they were assigned according to team and at others according to geographic location. Similarly, rounding times varied. Most of the services surveyed did not utilize advanced practice providers (the exception was the nonteaching services at Denver Health, and their presence was variable). These differences in staffing models could also have affected these results.
Our study also has a number of strengths. First, we assessed the barriers at five different hospitals. Second, we collected real-time data related to specific barriers at multiple time points throughout the day, allowing us to assess the dynamic nature of identifying patients as being ready or nearly ready for discharge. Third, we assessed the perceptions of barriers to discharge from physicians working on teaching as well as nonteaching services and from physicians utilizing a variety of rounding styles. Fourth, we had a very high participation rate (100%), probably due to the fact that our study was strategically aligned with participants’ daily work activities.
In conclusion, we found two distinct categories of issues that physicians perceived as most commonly delaying writing discharge orders on their patients. The first pertained to patients thought to definitely be ready for discharge and was related to the physicians having to care for other patients on their team. The second pertained to patients identified as possibly ready for discharge and was related to the need for care to be completed by a variety of ancillary services. Addressing each of these barriers would require different interventions and a need to weigh the potential improvements that could be achieved against the increased costs and/or delays in care for other patients that may result.
Disclosures
The authors report no conflicts of interest relevant to this work.
1. Wertheimer B, Jacobs RE, Bailey M, et al. Discharge before noon: an achievable hospital goal. J Hosp Med. 2014;9(4):210-214. doi: 10.1002/jhm.2154. PubMed
2. Kane M, Weinacker A, Arthofer R, et al. A multidisciplinary initiative to increase inpatient discharges before noon. J Nurs Adm. 2016;46(12):630-635. doi: 10.1097/NNA.0000000000000418. PubMed
3. Khanna S, Sier D, Boyle J, Zeitz K. Discharge timeliness and its impact on hospital crowding and emergency department flow performance. Emerg Med Australas. 2016;28(2):164-170. doi: 10.1111/1742-6723.12543. PubMed
4. Kravet SJ, Levine RB, Rubin HR, Wright SM. Discharging patients earlier in the day: a concept worth evaluating. Health Care Manag (Frederick). 2007;26:142-146. doi: 10.1097/01.HCM.0000268617.33491.60. PubMed
5. Khanna S, Boyle J, Good N, Lind J. Impact of admission and discharge peak times on hospital overcrowding. Stud Health Technol Inform. 2011;168:82-88. doi: 10.3233/978-1-60750-791-8-82. PubMed
6. McGowan JE, Truwit JD, Cipriano P, et al. Operating room efficiency and hospital capacity: factors affecting operating room use during maximum hospital census. J Am Coll Surg. 2007;204(5):865-871; discussion 71-72. doi: 10.1016/j.jamcollsurg.2007.01.052 PubMed
7. Khanna S, Boyle J, Good N, Lind J. Early discharge and its effect on ED length of stay and access block. Stud Health Technol Inform. 2012;178:92-98. doi: 10.3233/978-1-61499-078-9-92 PubMed
8. Powell ES, Khare RK, Venkatesh AK, Van Roo BD, Adams JG, Reinhardt G. The relationship between inpatient discharge timing and emergency department boarding. J Emerg Med. 2012;42(2):186-196. doi: 10.1016/j.jemermed.2010.06.028. PubMed
9. Wertheimer B, Jacobs RE, Iturrate E, Bailey M, Hochman K. Discharge before noon: Effect on throughput and sustainability. J Hosp Med. 2015;10(10):664-669. doi: 10.1002/jhm.2412. PubMed
10. Sikka R, Mehta S, Kaucky C, Kulstad EB. ED crowding is associated with an increased time to pneumonia treatment. Am J Emerg Med. 2010;28(7):809-812. doi: 10.1016/j.ajem.2009.06.023. PubMed
11. Coil CJ, Flood JD, Belyeu BM, Young P, Kaji AH, Lewis RJ. The effect of emergency department boarding on order completion. Ann Emerg Med. 2016;67:730-736 e2. doi: 10.1016/j.annemergmed.2015.09.018. PubMed
12. Gaieski DF, Agarwal AK, Mikkelsen ME, et al. The impact of ED crowding on early interventions and mortality in patients with severe sepsis. Am J Emerg Med. 2017;35:953-960. doi: 10.1016/j.ajem.2017.01.061. PubMed
13. Pines JM, Localio AR, Hollander JE, et al. The impact of emergency department crowding measures on time to antibiotics for patients with community-acquired pneumonia. Ann Emerg Med. 2007;50(5):510-516. doi: 10.1016/j.annemergmed.2007.07.021. PubMed
14. Hwang U, Richardson L, Livote E, Harris B, Spencer N, Sean Morrison R. Emergency department crowding and decreased quality of pain care. Acad Emerg Med. 2008;15:1248-1255. doi: 10.1111/j.1553-2712.2008.00267.x. PubMed
15. Mills AM, Shofer FS, Chen EH, Hollander JE, Pines JM. The association between emergency department crowding and analgesia administration in acute abdominal pain patients. Acad Emerg Med. 2009;16:603-608. doi: 10.1111/j.1553-2712.2009.00441.x. PubMed
16. Pines JM, Shofer FS, Isserman JA, Abbuhl SB, Mills AM. The effect of emergency department crowding on analgesia in patients with back pain in two hospitals. Acad Emerg Med. 2010;17(3):276-283. doi: 10.1111/j.1553-2712.2009.00676.x. PubMed
17. Kulstad EB, Sikka R, Sweis RT, Kelley KM, Rzechula KH. ED overcrowding is associated with an increased frequency of medication errors. Am J Emerg Med. 2010;28:304-309. doi: 10.1016/j.ajem.2008.12.014. PubMed
18. Richardson DB. Increase in patient mortality at 10 days associated with emergency department overcrowding. Med J Aust. 2006;184(5):213-216. PubMed
19. Hoot NR, Aronsky D. Systematic review of emergency department crowding: causes, effects, and solutions. Ann Emerg Med. 2008;52(2):126-136. doi: 10.1016/j.annemergmed.2008.03.014. PubMed
20. Singer AJ, Thode HC, Jr., Viccellio P, Pines JM. The association between length of emergency department boarding and mortality. Acad Emerg Med. 2011;18(12):1324-1329. doi: 10.1111/j.1553-2712.2011.01236.x. PubMed
21. White BA, Biddinger PD, Chang Y, Grabowski B, Carignan S, Brown DF. Boarding inpatients in the emergency department increases discharged patient length of stay. J Emerg Med. 2013;44(1):230-235. doi: 10.1016/j.jemermed.2012.05.007. PubMed
22. Forster AJ, Stiell I, Wells G, Lee AJ, van Walraven C. The effect of hospital occupancy on emergency department length of stay and patient disposition. Acad Emerg Med. 2003;10(2):127-133. doi: 10.1197/aemj.10.2.127. PubMed
23. Foley M, Kifaieh N, Mallon WK. Financial impact of emergency department crowding. West J Emerg Med. 2011;12(2):192-197. PubMed
24. Pines JM, Iyer S, Disbot M, Hollander JE, Shofer FS, Datner EM. The effect of emergency department crowding on patient satisfaction for admitted patients. Acad Emerg Med. 2008;15(9):825-831. doi: 10.1111/j.1553-2712.2008.00200.x. PubMed
25. Durvasula R, Kayihan A, Del Bene S, et al. A multidisciplinary care pathway significantly increases the number of early morning discharges in a large academic medical center. Qual Manag Health Care. 2015;24:45-51. doi: 10.1097/QMH.0000000000000049. PubMed
26. Cho HJ, Desai N, Florendo A, et al. E-DIP: Early Discharge Project. A Model for Throughput and Early Discharge for 1-Day Admissions. BMJ Qual Improv Rep. 2016;5(1): pii: u210035.w4128. doi: 10.1136/bmjquality.u210035.w4128. PubMed
27. Harris PA, Taylor R, Thielke R, Payne J, Gonzalez N, Conde JG. Research electronic data capture (REDCap)--a metadata-driven methodology and workflow process for providing translational research informatics support. J Biomed Inform. 2009;42(2):377-381. doi: 10.1016/j.jbi.2008.08.010. PubMed
28. Patel H, Fang MC, Mourad M, et al. Hospitalist and internal medicine leaders’ perspectives of early discharge challenges at academic medical centers. J Hosp Med. 2018;13(6):388-391. doi: 10.12788/jhm.2885. PubMed
29. Minichiello TM, Auerbach AD, Wachter RM. Caregiver perceptions of the reasons for delayed hospital discharge. Eff Clin Pract. 2001;4(6):250-255. PubMed
30. Beck MJ, Okerblom D, Kumar A, Bandyopadhyay S, Scalzi LV. Lean intervention improves patient discharge times, improves emergency department throughput and reduces congestion. Hosp Pract (1995). 2016;44(5):252-259. doi: 10.1080/21548331.2016.1254559. PubMed
31. Rajkomar A, Valencia V, Novelero M, Mourad M, Auerbach A. The association between discharge before noon and length of stay in medical and surgical patients. J Hosp Med. 2016;11(12):859-861. doi: 10.1002/jhm.2529. PubMed
32. Shine D. Discharge before noon: an urban legend. Am J Med. 2015;128(5):445-446. doi: 10.1016/j.amjmed.2014.12.011. PubMed33. Khanna S, Boyle J, Good N, Lind J. Unravelling relationships: Hospital occupancy levels, discharge timing and emergency department access block. Emerg Med Australas. 2012;24(5):510-517. doi: 10.1111/j.1742-6723.2012.01587.x. PubMed
Hospital discharges frequently occur in the afternoon or evening hours.1-5 Late discharges can adversely affect patient flow throughout the hospital,3,6-9 which, in turn, can result in delays in care,10-16 more medication errors,17 increased mortality,18-20 longer lengths of stay,20-22 higher costs,23 and lower patient satisfaction.24
Various interventions have been employed in the attempts to find ways of moving discharge times to earlier in the day, including preparing the discharge paperwork and medications the previous night,25 using checklists,1,25 team huddles,2 providing real-time feedback to unit staff,1 and employing multidisciplinary teamwork.1,2,6,25,26
The purpose of this study was to identify and determine the relative frequency of barriers to writing discharge orders in the hopes of identifying issues that might be addressed by targeted interventions. We also assessed the effects of daily team census, patients being on teaching versus nonteaching services, and how daily rounds were structured at the time that the discharge orders were written.
METHODS
Study Design, Setting, and Participants
We conducted a prospective, cross-sectional survey of house-staff and attending physicians on general medicine teaching and nonteaching services from November 13, 2014, through May 31, 2016. The study was conducted at the following five hospitals: Denver Health Medical Center (DHMC) and Presbyterian/Saint Luke’s Medical Center (PSL) in Denver, Colorado; Ronald Reagan University (UCLA) and Los Angeles County/University of Southern California Medical Center (LAC+USC) in Los Angeles, California; and Harborview Medical Center (HMC) in Seattle, Washington. The study was approved by the Colorado Multi-Institutional Review Board as well as by the review boards of the other participating sites.
Data Collection
The results of the focus groups composed of attending physicians at DHMC were used to develop our initial data collection template. Additional sites joining the study provided feedback, leading to modifications (Appendix 1).
Physicians were surveyed at three different time points on study days that were selected according to the convenience of the investigators. The sampling occurred only on weekdays and was done based on the investigators’ availability. Investigators would attempt to survey as many teams as they were able to but, secondary to feasibility, not all teams could be surveyed on study days. The specific time points varied as a function of physician workflows but were standardized as much as possible to occur in the early morning, around noon, and midafternoon on weekdays. Physicians were contacted either in person or by telephone for verbal consent prior to administering the first survey. All general medicine teams were eligible. For teaching teams, the order of contact was resident, intern, and then attending based on which physician was available at the time of the survey and on which member of the team was thought to know the patients the best. For the nonteaching services, the attending physicians were contacted.
During the initial survey, the investigators assessed the provider role (ie, attending or housestaff), whether the service was a teaching or a nonteaching service, and the starting patient census on that service primarily based on interviewing the provider of record for the team and looking at team census lists. Physicians were asked about their rounding style (ie, sickest patients first, patients likely to be discharged first, room-by-room, most recently admitted patients first, patients on the team the longest, or other) and then to identify all patients they thought would be definite discharges sometime during the day of the survey. Definite discharges were defined as patients whom the provider thought were either currently ready for discharge or who had only minor barriers that, if unresolved, would not prevent same-day discharge. They were asked if the discharge order had been entered and, if not, what was preventing them from doing so, if the discharge could in their opinion have occurred the day prior and, if so, why this did not occur. We also obtained the date and time of the admission and discharge orders, the actual discharge time, as well as the length of stay either through chart review (majority of sites) or from data warehouses (Denver Health and Presbyterian St. Lukes had length of stay data retrieved from their data warehouse).
Physicians were also asked to identify all patients whom they thought might possibly be discharged that day. Possible discharges were defined as patients with barriers to discharge that, if unresolved, would prevent same-day discharge. For each of these, the physicians were asked to list whatever issues needed to be resolved prior to placing the discharge order (Appendix 1).
The second survey was administered late morning on the same day, typically between 11
The third survey was administered midafternoon, typically around 3 PM similar to the first two surveys, with the exception that the third survey did not attempt to identify new definite or possible discharges.
Sample Size
We stopped collecting data after obtaining a convenience sample of 5% of total discharges at each study site or on the study end date, which was May 31, 2016, whichever came first.
Data Analysis
Data were collected and managed using a secure, web-based application electronic data capture tool (REDCap), hosted at Denver Health. REDCap (Research Electronic Data Capture, Nashville, Tennessee) is designed to support data collection for research studies.27 Data were then analyzed using SAS Enterprise Guide 5.1 (SAS Institute, Inc., Cary, North Carolina). All data entered into REDCap were reviewed by the principal investigator to ensure that data were not missing, and when there were missing data, a query was sent to verify if the data were retrievable. If retrievable, then the data would be entered. The volume of missing data that remained is described in our results.
Continuous variables were described using means and standard deviations (SD) or medians and interquartile ranges (IQR) based on tests of normality. Differences in the time that the discharge orders were placed in the electronic medical record according to morning patient census, teaching versus nonteaching service, and rounding style were compared using the Wilcoxon rank sum test. Linear regression was used to evaluate the effect of patient census on discharge order time. P < .05 was considered as significant.
RESULTS
We conducted 1,584 patient evaluations through surveys of 254 physicians over 156 days. Given surveys coincided with the existing work we had full participation (ie, 100% participation) and no dropout during the study days. Median (IQR) survey time points were 8:30
The characteristics of the five hospitals participating in the study, the patients’ final discharge status, the types of physicians surveyed, the services on which they were working, the rounding styles employed, and the median starting daily census are summarized in Table 1. The majority of the physicians surveyed were housestaff working on teaching services, and only a small minority structured rounds such that patients ready for discharge were seen first.
Over the course of the three surveys, 949 patients were identified as being definite discharges at any time point, and the large majority of these (863, 91%) were discharged on the day of the survey. The median (IQR) time that the discharge orders were written was 11:50
During the initial morning survey, 314 patients were identified as being definite discharges for that day (representing approximately 6% of the total number of patients being cared for, or 33% of the patients identified as definite discharges throughout the day). Of these, the physicians thought that 44 (<1% of the total number of patients being cared for on the services) could have been discharged on the previous day. The most frequent reasons cited for why these patients were not discharged on the previous day were “Patient did not want to leave” (n = 15, 34%), “Too late in the day” (n = 10, 23%), and “No ride” (n = 9, 20%). The remaining 10 patients (23%) had a variety of reasons related to system or social issues (ie, shelter not available, miscommunication).
At the morning time point, the most common barriers to discharge identified were that the physicians had not finished rounding on their team of patients and that the housestaff needed to staff their patients with their attending. At noon, caring for other patients and tending to the discharge processes were most commonly cited, and in the afternoon, the most common barriers were that the physicians were in the process of completing the discharge paperwork for those patients or were discharging other patients (Table 2). When comparing barriers on teaching to nonteaching teams, a higher proportion of teaching teams were still rounding on all patients and were working on discharge paperwork at the second survey. Barriers cited by sites were similar; however, the frequency at which the barriers were mentioned varied (data not shown).
The physicians identified 1,237 patients at any time point as being possible discharges during the day of the survey and these had a mean (±SD) of 1.3 (±0.5) barriers cited for why these patients were possible rather than definite discharges. The most common were that clinical improvement was needed, one or more pending issues related to their care needed to be resolved, and/or awaiting pending test results. The need to see clinical improvement generally decreased throughout the day as did the need to staff patients with an attending physician, but barriers related to consultant recommendations or completing procedures increased (Table 3). Of the 1,237 patients ever identified as possible discharges, 594 (48%) became a definite discharge by the third call and 444 (36%) became a no discharge as their final status. As with definite discharges, barriers cited by sites were similar; however, the frequency at which the barriers were mentioned varied.
Among the 949 and 1,237 patients who were ever identified as definite or possible discharges, respectively, at any time point during the study day, 28 (3%) and 444 (36%), respectively, had their discharge status changed to no discharge, most commonly because their clinical condition either worsened or expected improvements did not occur or that barriers pertaining to social work, physical therapy, or occupational therapy were not resolved.
The median time that the discharge orders were entered into the electronic medical record was 43 minutes earlier if patients were on teams with a lower versus a higher starting census (P = .0003), 48 minutes earlier if they were seen by physicians whose rounding style was to see patients first who potentially could be discharged (P = .0026), and 58 minutes earlier if they were on nonteaching versus teaching services (P < .0001; Table 4). For every one-person increase in census, the discharge order time increased by 6 minutes (β = 5.6, SE = 1.6, P = .0003).
DISCUSSION
The important findings of this study are that (1) the large majority of issues thought to delay discharging patients identified as definite discharges were related to physicians caring for other patients on their team, (2) although 91% of patients ever identified as being definite discharges were discharged on the day of the survey, only 48% of those identified as possible discharges became definite discharges by the afternoon time point, largely because the anticipated clinical improvement did not occur or care being provided by ancillary services had not been completed, and (3) discharge orders on patients identified as definite discharges were written on average 50 minutes earlier by physicians on teams with a smaller starting patient census, on nonteaching services, or when the rounding style was to see patients ready for discharges first.
Previous research has reported that physician-perceived barriers to discharge were extrinsic to providers and even extrinsic to the hospital setting (eg, awaiting subacute nursing placement and transportation).28,29 However, many of the barriers that we identified were related directly to the providers’ workload and rounding styles and whether the patients were on teaching versus nonteaching services. We also found that delays in the ability of hospital services to complete care also contributed to delayed discharges.
Our observational data suggest that delays resulting from caring for other patients might be reduced by changing rounding styles such that patients ready for discharge are seen first and are discharged prior to seeing other patients on the team, as previously reported by Beck et al.30 Intuitively, this would seem to be a straightforward way of freeing up beds earlier in the day, but such a change will, of necessity, lead to delaying care for other patients, which, in turn, could increase their length of stays. Durvasula et al. suggested that discharges could be moved to earlier in the day by completing orders and paperwork the day prior to discharge.25 Such an approach might be effective on an Obstetrical or elective Orthopedic service on which patients predictably are hospitalized for a fixed number of days (or even hours) but may be less relevant to patients on internal medicine services where lengths of stay are less predictable. Interventions to improve discharge times have resulted in earlier discharge times in some studies,2,4 but the overall length of stay either did not decrease25 or increased31 in others. Werthheimer et al.1 did find earlier discharge times, but other interventions also occurred during the study period (eg, extending social work services to include weekends).1,32
We found that discharge times were approximately 50 minutes earlier on teams with a smaller starting census, on nonteaching compared with teaching services, or when the attending’s rounding style was to see patients ready for discharges first. Although 50 minutes may seem like a small change in discharge time, Khanna et al.33 found that when discharges occur even 1 hour earlier, hospital overcrowding is reduced. To have a lower team census would require having more teams and more providers to staff these teams, raising cost-effectiveness concerns. Moving to more nonteaching services could represent a conflict with respect to one of the missions of teaching hospitals and raises a cost-benefit issue as several teaching hospitals receive substantial funding in support of their teaching activities and housestaff would have to be replaced with more expensive providers.
Delays attributable to ancillary services indicate imbalances between demand and availability of these services. Inappropriate demand and inefficiencies could be reduced by systems redesign, but in at least some instances, additional resources will be needed to add staff, increase space, or add additional equipment.
Our study has several limitations. First, we surveyed only physicians working in university-affiliated hospitals, and three of these were public safety-net hospitals. Accordingly, our results may not be generalizable to different patient populations. Second, we surveyed only physicians, and Minichiello et al.29 found that barriers to discharge perceived by physicians were different from those of other staff. Third, our data were observational and were collected only on weekdays. Fourth, we did not differentiate interns from residents, and thus, potentially the level of training could have affected these results. Similarly, the decision for a “possible” and a “definite” discharge is likely dependent on the knowledge base of the participant, such that less experienced participants may have had differing perspectives than someone with more experience. Fifth, the sites did vary based on the infrastructure and support but also had several similarities. All sites had social work and case management involved in care, although at some sites, they were assigned according to team and at others according to geographic location. Similarly, rounding times varied. Most of the services surveyed did not utilize advanced practice providers (the exception was the nonteaching services at Denver Health, and their presence was variable). These differences in staffing models could also have affected these results.
Our study also has a number of strengths. First, we assessed the barriers at five different hospitals. Second, we collected real-time data related to specific barriers at multiple time points throughout the day, allowing us to assess the dynamic nature of identifying patients as being ready or nearly ready for discharge. Third, we assessed the perceptions of barriers to discharge from physicians working on teaching as well as nonteaching services and from physicians utilizing a variety of rounding styles. Fourth, we had a very high participation rate (100%), probably due to the fact that our study was strategically aligned with participants’ daily work activities.
In conclusion, we found two distinct categories of issues that physicians perceived as most commonly delaying writing discharge orders on their patients. The first pertained to patients thought to definitely be ready for discharge and was related to the physicians having to care for other patients on their team. The second pertained to patients identified as possibly ready for discharge and was related to the need for care to be completed by a variety of ancillary services. Addressing each of these barriers would require different interventions and a need to weigh the potential improvements that could be achieved against the increased costs and/or delays in care for other patients that may result.
Disclosures
The authors report no conflicts of interest relevant to this work.
Hospital discharges frequently occur in the afternoon or evening hours.1-5 Late discharges can adversely affect patient flow throughout the hospital,3,6-9 which, in turn, can result in delays in care,10-16 more medication errors,17 increased mortality,18-20 longer lengths of stay,20-22 higher costs,23 and lower patient satisfaction.24
Various interventions have been employed in the attempts to find ways of moving discharge times to earlier in the day, including preparing the discharge paperwork and medications the previous night,25 using checklists,1,25 team huddles,2 providing real-time feedback to unit staff,1 and employing multidisciplinary teamwork.1,2,6,25,26
The purpose of this study was to identify and determine the relative frequency of barriers to writing discharge orders in the hopes of identifying issues that might be addressed by targeted interventions. We also assessed the effects of daily team census, patients being on teaching versus nonteaching services, and how daily rounds were structured at the time that the discharge orders were written.
METHODS
Study Design, Setting, and Participants
We conducted a prospective, cross-sectional survey of house-staff and attending physicians on general medicine teaching and nonteaching services from November 13, 2014, through May 31, 2016. The study was conducted at the following five hospitals: Denver Health Medical Center (DHMC) and Presbyterian/Saint Luke’s Medical Center (PSL) in Denver, Colorado; Ronald Reagan University (UCLA) and Los Angeles County/University of Southern California Medical Center (LAC+USC) in Los Angeles, California; and Harborview Medical Center (HMC) in Seattle, Washington. The study was approved by the Colorado Multi-Institutional Review Board as well as by the review boards of the other participating sites.
Data Collection
The results of the focus groups composed of attending physicians at DHMC were used to develop our initial data collection template. Additional sites joining the study provided feedback, leading to modifications (Appendix 1).
Physicians were surveyed at three different time points on study days that were selected according to the convenience of the investigators. The sampling occurred only on weekdays and was done based on the investigators’ availability. Investigators would attempt to survey as many teams as they were able to but, secondary to feasibility, not all teams could be surveyed on study days. The specific time points varied as a function of physician workflows but were standardized as much as possible to occur in the early morning, around noon, and midafternoon on weekdays. Physicians were contacted either in person or by telephone for verbal consent prior to administering the first survey. All general medicine teams were eligible. For teaching teams, the order of contact was resident, intern, and then attending based on which physician was available at the time of the survey and on which member of the team was thought to know the patients the best. For the nonteaching services, the attending physicians were contacted.
During the initial survey, the investigators assessed the provider role (ie, attending or housestaff), whether the service was a teaching or a nonteaching service, and the starting patient census on that service primarily based on interviewing the provider of record for the team and looking at team census lists. Physicians were asked about their rounding style (ie, sickest patients first, patients likely to be discharged first, room-by-room, most recently admitted patients first, patients on the team the longest, or other) and then to identify all patients they thought would be definite discharges sometime during the day of the survey. Definite discharges were defined as patients whom the provider thought were either currently ready for discharge or who had only minor barriers that, if unresolved, would not prevent same-day discharge. They were asked if the discharge order had been entered and, if not, what was preventing them from doing so, if the discharge could in their opinion have occurred the day prior and, if so, why this did not occur. We also obtained the date and time of the admission and discharge orders, the actual discharge time, as well as the length of stay either through chart review (majority of sites) or from data warehouses (Denver Health and Presbyterian St. Lukes had length of stay data retrieved from their data warehouse).
Physicians were also asked to identify all patients whom they thought might possibly be discharged that day. Possible discharges were defined as patients with barriers to discharge that, if unresolved, would prevent same-day discharge. For each of these, the physicians were asked to list whatever issues needed to be resolved prior to placing the discharge order (Appendix 1).
The second survey was administered late morning on the same day, typically between 11
The third survey was administered midafternoon, typically around 3 PM similar to the first two surveys, with the exception that the third survey did not attempt to identify new definite or possible discharges.
Sample Size
We stopped collecting data after obtaining a convenience sample of 5% of total discharges at each study site or on the study end date, which was May 31, 2016, whichever came first.
Data Analysis
Data were collected and managed using a secure, web-based application electronic data capture tool (REDCap), hosted at Denver Health. REDCap (Research Electronic Data Capture, Nashville, Tennessee) is designed to support data collection for research studies.27 Data were then analyzed using SAS Enterprise Guide 5.1 (SAS Institute, Inc., Cary, North Carolina). All data entered into REDCap were reviewed by the principal investigator to ensure that data were not missing, and when there were missing data, a query was sent to verify if the data were retrievable. If retrievable, then the data would be entered. The volume of missing data that remained is described in our results.
Continuous variables were described using means and standard deviations (SD) or medians and interquartile ranges (IQR) based on tests of normality. Differences in the time that the discharge orders were placed in the electronic medical record according to morning patient census, teaching versus nonteaching service, and rounding style were compared using the Wilcoxon rank sum test. Linear regression was used to evaluate the effect of patient census on discharge order time. P < .05 was considered as significant.
RESULTS
We conducted 1,584 patient evaluations through surveys of 254 physicians over 156 days. Given surveys coincided with the existing work we had full participation (ie, 100% participation) and no dropout during the study days. Median (IQR) survey time points were 8:30
The characteristics of the five hospitals participating in the study, the patients’ final discharge status, the types of physicians surveyed, the services on which they were working, the rounding styles employed, and the median starting daily census are summarized in Table 1. The majority of the physicians surveyed were housestaff working on teaching services, and only a small minority structured rounds such that patients ready for discharge were seen first.
Over the course of the three surveys, 949 patients were identified as being definite discharges at any time point, and the large majority of these (863, 91%) were discharged on the day of the survey. The median (IQR) time that the discharge orders were written was 11:50
During the initial morning survey, 314 patients were identified as being definite discharges for that day (representing approximately 6% of the total number of patients being cared for, or 33% of the patients identified as definite discharges throughout the day). Of these, the physicians thought that 44 (<1% of the total number of patients being cared for on the services) could have been discharged on the previous day. The most frequent reasons cited for why these patients were not discharged on the previous day were “Patient did not want to leave” (n = 15, 34%), “Too late in the day” (n = 10, 23%), and “No ride” (n = 9, 20%). The remaining 10 patients (23%) had a variety of reasons related to system or social issues (ie, shelter not available, miscommunication).
At the morning time point, the most common barriers to discharge identified were that the physicians had not finished rounding on their team of patients and that the housestaff needed to staff their patients with their attending. At noon, caring for other patients and tending to the discharge processes were most commonly cited, and in the afternoon, the most common barriers were that the physicians were in the process of completing the discharge paperwork for those patients or were discharging other patients (Table 2). When comparing barriers on teaching to nonteaching teams, a higher proportion of teaching teams were still rounding on all patients and were working on discharge paperwork at the second survey. Barriers cited by sites were similar; however, the frequency at which the barriers were mentioned varied (data not shown).
The physicians identified 1,237 patients at any time point as being possible discharges during the day of the survey and these had a mean (±SD) of 1.3 (±0.5) barriers cited for why these patients were possible rather than definite discharges. The most common were that clinical improvement was needed, one or more pending issues related to their care needed to be resolved, and/or awaiting pending test results. The need to see clinical improvement generally decreased throughout the day as did the need to staff patients with an attending physician, but barriers related to consultant recommendations or completing procedures increased (Table 3). Of the 1,237 patients ever identified as possible discharges, 594 (48%) became a definite discharge by the third call and 444 (36%) became a no discharge as their final status. As with definite discharges, barriers cited by sites were similar; however, the frequency at which the barriers were mentioned varied.
Among the 949 and 1,237 patients who were ever identified as definite or possible discharges, respectively, at any time point during the study day, 28 (3%) and 444 (36%), respectively, had their discharge status changed to no discharge, most commonly because their clinical condition either worsened or expected improvements did not occur or that barriers pertaining to social work, physical therapy, or occupational therapy were not resolved.
The median time that the discharge orders were entered into the electronic medical record was 43 minutes earlier if patients were on teams with a lower versus a higher starting census (P = .0003), 48 minutes earlier if they were seen by physicians whose rounding style was to see patients first who potentially could be discharged (P = .0026), and 58 minutes earlier if they were on nonteaching versus teaching services (P < .0001; Table 4). For every one-person increase in census, the discharge order time increased by 6 minutes (β = 5.6, SE = 1.6, P = .0003).
DISCUSSION
The important findings of this study are that (1) the large majority of issues thought to delay discharging patients identified as definite discharges were related to physicians caring for other patients on their team, (2) although 91% of patients ever identified as being definite discharges were discharged on the day of the survey, only 48% of those identified as possible discharges became definite discharges by the afternoon time point, largely because the anticipated clinical improvement did not occur or care being provided by ancillary services had not been completed, and (3) discharge orders on patients identified as definite discharges were written on average 50 minutes earlier by physicians on teams with a smaller starting patient census, on nonteaching services, or when the rounding style was to see patients ready for discharges first.
Previous research has reported that physician-perceived barriers to discharge were extrinsic to providers and even extrinsic to the hospital setting (eg, awaiting subacute nursing placement and transportation).28,29 However, many of the barriers that we identified were related directly to the providers’ workload and rounding styles and whether the patients were on teaching versus nonteaching services. We also found that delays in the ability of hospital services to complete care also contributed to delayed discharges.
Our observational data suggest that delays resulting from caring for other patients might be reduced by changing rounding styles such that patients ready for discharge are seen first and are discharged prior to seeing other patients on the team, as previously reported by Beck et al.30 Intuitively, this would seem to be a straightforward way of freeing up beds earlier in the day, but such a change will, of necessity, lead to delaying care for other patients, which, in turn, could increase their length of stays. Durvasula et al. suggested that discharges could be moved to earlier in the day by completing orders and paperwork the day prior to discharge.25 Such an approach might be effective on an Obstetrical or elective Orthopedic service on which patients predictably are hospitalized for a fixed number of days (or even hours) but may be less relevant to patients on internal medicine services where lengths of stay are less predictable. Interventions to improve discharge times have resulted in earlier discharge times in some studies,2,4 but the overall length of stay either did not decrease25 or increased31 in others. Werthheimer et al.1 did find earlier discharge times, but other interventions also occurred during the study period (eg, extending social work services to include weekends).1,32
We found that discharge times were approximately 50 minutes earlier on teams with a smaller starting census, on nonteaching compared with teaching services, or when the attending’s rounding style was to see patients ready for discharges first. Although 50 minutes may seem like a small change in discharge time, Khanna et al.33 found that when discharges occur even 1 hour earlier, hospital overcrowding is reduced. To have a lower team census would require having more teams and more providers to staff these teams, raising cost-effectiveness concerns. Moving to more nonteaching services could represent a conflict with respect to one of the missions of teaching hospitals and raises a cost-benefit issue as several teaching hospitals receive substantial funding in support of their teaching activities and housestaff would have to be replaced with more expensive providers.
Delays attributable to ancillary services indicate imbalances between demand and availability of these services. Inappropriate demand and inefficiencies could be reduced by systems redesign, but in at least some instances, additional resources will be needed to add staff, increase space, or add additional equipment.
Our study has several limitations. First, we surveyed only physicians working in university-affiliated hospitals, and three of these were public safety-net hospitals. Accordingly, our results may not be generalizable to different patient populations. Second, we surveyed only physicians, and Minichiello et al.29 found that barriers to discharge perceived by physicians were different from those of other staff. Third, our data were observational and were collected only on weekdays. Fourth, we did not differentiate interns from residents, and thus, potentially the level of training could have affected these results. Similarly, the decision for a “possible” and a “definite” discharge is likely dependent on the knowledge base of the participant, such that less experienced participants may have had differing perspectives than someone with more experience. Fifth, the sites did vary based on the infrastructure and support but also had several similarities. All sites had social work and case management involved in care, although at some sites, they were assigned according to team and at others according to geographic location. Similarly, rounding times varied. Most of the services surveyed did not utilize advanced practice providers (the exception was the nonteaching services at Denver Health, and their presence was variable). These differences in staffing models could also have affected these results.
Our study also has a number of strengths. First, we assessed the barriers at five different hospitals. Second, we collected real-time data related to specific barriers at multiple time points throughout the day, allowing us to assess the dynamic nature of identifying patients as being ready or nearly ready for discharge. Third, we assessed the perceptions of barriers to discharge from physicians working on teaching as well as nonteaching services and from physicians utilizing a variety of rounding styles. Fourth, we had a very high participation rate (100%), probably due to the fact that our study was strategically aligned with participants’ daily work activities.
In conclusion, we found two distinct categories of issues that physicians perceived as most commonly delaying writing discharge orders on their patients. The first pertained to patients thought to definitely be ready for discharge and was related to the physicians having to care for other patients on their team. The second pertained to patients identified as possibly ready for discharge and was related to the need for care to be completed by a variety of ancillary services. Addressing each of these barriers would require different interventions and a need to weigh the potential improvements that could be achieved against the increased costs and/or delays in care for other patients that may result.
Disclosures
The authors report no conflicts of interest relevant to this work.
1. Wertheimer B, Jacobs RE, Bailey M, et al. Discharge before noon: an achievable hospital goal. J Hosp Med. 2014;9(4):210-214. doi: 10.1002/jhm.2154. PubMed
2. Kane M, Weinacker A, Arthofer R, et al. A multidisciplinary initiative to increase inpatient discharges before noon. J Nurs Adm. 2016;46(12):630-635. doi: 10.1097/NNA.0000000000000418. PubMed
3. Khanna S, Sier D, Boyle J, Zeitz K. Discharge timeliness and its impact on hospital crowding and emergency department flow performance. Emerg Med Australas. 2016;28(2):164-170. doi: 10.1111/1742-6723.12543. PubMed
4. Kravet SJ, Levine RB, Rubin HR, Wright SM. Discharging patients earlier in the day: a concept worth evaluating. Health Care Manag (Frederick). 2007;26:142-146. doi: 10.1097/01.HCM.0000268617.33491.60. PubMed
5. Khanna S, Boyle J, Good N, Lind J. Impact of admission and discharge peak times on hospital overcrowding. Stud Health Technol Inform. 2011;168:82-88. doi: 10.3233/978-1-60750-791-8-82. PubMed
6. McGowan JE, Truwit JD, Cipriano P, et al. Operating room efficiency and hospital capacity: factors affecting operating room use during maximum hospital census. J Am Coll Surg. 2007;204(5):865-871; discussion 71-72. doi: 10.1016/j.jamcollsurg.2007.01.052 PubMed
7. Khanna S, Boyle J, Good N, Lind J. Early discharge and its effect on ED length of stay and access block. Stud Health Technol Inform. 2012;178:92-98. doi: 10.3233/978-1-61499-078-9-92 PubMed
8. Powell ES, Khare RK, Venkatesh AK, Van Roo BD, Adams JG, Reinhardt G. The relationship between inpatient discharge timing and emergency department boarding. J Emerg Med. 2012;42(2):186-196. doi: 10.1016/j.jemermed.2010.06.028. PubMed
9. Wertheimer B, Jacobs RE, Iturrate E, Bailey M, Hochman K. Discharge before noon: Effect on throughput and sustainability. J Hosp Med. 2015;10(10):664-669. doi: 10.1002/jhm.2412. PubMed
10. Sikka R, Mehta S, Kaucky C, Kulstad EB. ED crowding is associated with an increased time to pneumonia treatment. Am J Emerg Med. 2010;28(7):809-812. doi: 10.1016/j.ajem.2009.06.023. PubMed
11. Coil CJ, Flood JD, Belyeu BM, Young P, Kaji AH, Lewis RJ. The effect of emergency department boarding on order completion. Ann Emerg Med. 2016;67:730-736 e2. doi: 10.1016/j.annemergmed.2015.09.018. PubMed
12. Gaieski DF, Agarwal AK, Mikkelsen ME, et al. The impact of ED crowding on early interventions and mortality in patients with severe sepsis. Am J Emerg Med. 2017;35:953-960. doi: 10.1016/j.ajem.2017.01.061. PubMed
13. Pines JM, Localio AR, Hollander JE, et al. The impact of emergency department crowding measures on time to antibiotics for patients with community-acquired pneumonia. Ann Emerg Med. 2007;50(5):510-516. doi: 10.1016/j.annemergmed.2007.07.021. PubMed
14. Hwang U, Richardson L, Livote E, Harris B, Spencer N, Sean Morrison R. Emergency department crowding and decreased quality of pain care. Acad Emerg Med. 2008;15:1248-1255. doi: 10.1111/j.1553-2712.2008.00267.x. PubMed
15. Mills AM, Shofer FS, Chen EH, Hollander JE, Pines JM. The association between emergency department crowding and analgesia administration in acute abdominal pain patients. Acad Emerg Med. 2009;16:603-608. doi: 10.1111/j.1553-2712.2009.00441.x. PubMed
16. Pines JM, Shofer FS, Isserman JA, Abbuhl SB, Mills AM. The effect of emergency department crowding on analgesia in patients with back pain in two hospitals. Acad Emerg Med. 2010;17(3):276-283. doi: 10.1111/j.1553-2712.2009.00676.x. PubMed
17. Kulstad EB, Sikka R, Sweis RT, Kelley KM, Rzechula KH. ED overcrowding is associated with an increased frequency of medication errors. Am J Emerg Med. 2010;28:304-309. doi: 10.1016/j.ajem.2008.12.014. PubMed
18. Richardson DB. Increase in patient mortality at 10 days associated with emergency department overcrowding. Med J Aust. 2006;184(5):213-216. PubMed
19. Hoot NR, Aronsky D. Systematic review of emergency department crowding: causes, effects, and solutions. Ann Emerg Med. 2008;52(2):126-136. doi: 10.1016/j.annemergmed.2008.03.014. PubMed
20. Singer AJ, Thode HC, Jr., Viccellio P, Pines JM. The association between length of emergency department boarding and mortality. Acad Emerg Med. 2011;18(12):1324-1329. doi: 10.1111/j.1553-2712.2011.01236.x. PubMed
21. White BA, Biddinger PD, Chang Y, Grabowski B, Carignan S, Brown DF. Boarding inpatients in the emergency department increases discharged patient length of stay. J Emerg Med. 2013;44(1):230-235. doi: 10.1016/j.jemermed.2012.05.007. PubMed
22. Forster AJ, Stiell I, Wells G, Lee AJ, van Walraven C. The effect of hospital occupancy on emergency department length of stay and patient disposition. Acad Emerg Med. 2003;10(2):127-133. doi: 10.1197/aemj.10.2.127. PubMed
23. Foley M, Kifaieh N, Mallon WK. Financial impact of emergency department crowding. West J Emerg Med. 2011;12(2):192-197. PubMed
24. Pines JM, Iyer S, Disbot M, Hollander JE, Shofer FS, Datner EM. The effect of emergency department crowding on patient satisfaction for admitted patients. Acad Emerg Med. 2008;15(9):825-831. doi: 10.1111/j.1553-2712.2008.00200.x. PubMed
25. Durvasula R, Kayihan A, Del Bene S, et al. A multidisciplinary care pathway significantly increases the number of early morning discharges in a large academic medical center. Qual Manag Health Care. 2015;24:45-51. doi: 10.1097/QMH.0000000000000049. PubMed
26. Cho HJ, Desai N, Florendo A, et al. E-DIP: Early Discharge Project. A Model for Throughput and Early Discharge for 1-Day Admissions. BMJ Qual Improv Rep. 2016;5(1): pii: u210035.w4128. doi: 10.1136/bmjquality.u210035.w4128. PubMed
27. Harris PA, Taylor R, Thielke R, Payne J, Gonzalez N, Conde JG. Research electronic data capture (REDCap)--a metadata-driven methodology and workflow process for providing translational research informatics support. J Biomed Inform. 2009;42(2):377-381. doi: 10.1016/j.jbi.2008.08.010. PubMed
28. Patel H, Fang MC, Mourad M, et al. Hospitalist and internal medicine leaders’ perspectives of early discharge challenges at academic medical centers. J Hosp Med. 2018;13(6):388-391. doi: 10.12788/jhm.2885. PubMed
29. Minichiello TM, Auerbach AD, Wachter RM. Caregiver perceptions of the reasons for delayed hospital discharge. Eff Clin Pract. 2001;4(6):250-255. PubMed
30. Beck MJ, Okerblom D, Kumar A, Bandyopadhyay S, Scalzi LV. Lean intervention improves patient discharge times, improves emergency department throughput and reduces congestion. Hosp Pract (1995). 2016;44(5):252-259. doi: 10.1080/21548331.2016.1254559. PubMed
31. Rajkomar A, Valencia V, Novelero M, Mourad M, Auerbach A. The association between discharge before noon and length of stay in medical and surgical patients. J Hosp Med. 2016;11(12):859-861. doi: 10.1002/jhm.2529. PubMed
32. Shine D. Discharge before noon: an urban legend. Am J Med. 2015;128(5):445-446. doi: 10.1016/j.amjmed.2014.12.011. PubMed33. Khanna S, Boyle J, Good N, Lind J. Unravelling relationships: Hospital occupancy levels, discharge timing and emergency department access block. Emerg Med Australas. 2012;24(5):510-517. doi: 10.1111/j.1742-6723.2012.01587.x. PubMed
1. Wertheimer B, Jacobs RE, Bailey M, et al. Discharge before noon: an achievable hospital goal. J Hosp Med. 2014;9(4):210-214. doi: 10.1002/jhm.2154. PubMed
2. Kane M, Weinacker A, Arthofer R, et al. A multidisciplinary initiative to increase inpatient discharges before noon. J Nurs Adm. 2016;46(12):630-635. doi: 10.1097/NNA.0000000000000418. PubMed
3. Khanna S, Sier D, Boyle J, Zeitz K. Discharge timeliness and its impact on hospital crowding and emergency department flow performance. Emerg Med Australas. 2016;28(2):164-170. doi: 10.1111/1742-6723.12543. PubMed
4. Kravet SJ, Levine RB, Rubin HR, Wright SM. Discharging patients earlier in the day: a concept worth evaluating. Health Care Manag (Frederick). 2007;26:142-146. doi: 10.1097/01.HCM.0000268617.33491.60. PubMed
5. Khanna S, Boyle J, Good N, Lind J. Impact of admission and discharge peak times on hospital overcrowding. Stud Health Technol Inform. 2011;168:82-88. doi: 10.3233/978-1-60750-791-8-82. PubMed
6. McGowan JE, Truwit JD, Cipriano P, et al. Operating room efficiency and hospital capacity: factors affecting operating room use during maximum hospital census. J Am Coll Surg. 2007;204(5):865-871; discussion 71-72. doi: 10.1016/j.jamcollsurg.2007.01.052 PubMed
7. Khanna S, Boyle J, Good N, Lind J. Early discharge and its effect on ED length of stay and access block. Stud Health Technol Inform. 2012;178:92-98. doi: 10.3233/978-1-61499-078-9-92 PubMed
8. Powell ES, Khare RK, Venkatesh AK, Van Roo BD, Adams JG, Reinhardt G. The relationship between inpatient discharge timing and emergency department boarding. J Emerg Med. 2012;42(2):186-196. doi: 10.1016/j.jemermed.2010.06.028. PubMed
9. Wertheimer B, Jacobs RE, Iturrate E, Bailey M, Hochman K. Discharge before noon: Effect on throughput and sustainability. J Hosp Med. 2015;10(10):664-669. doi: 10.1002/jhm.2412. PubMed
10. Sikka R, Mehta S, Kaucky C, Kulstad EB. ED crowding is associated with an increased time to pneumonia treatment. Am J Emerg Med. 2010;28(7):809-812. doi: 10.1016/j.ajem.2009.06.023. PubMed
11. Coil CJ, Flood JD, Belyeu BM, Young P, Kaji AH, Lewis RJ. The effect of emergency department boarding on order completion. Ann Emerg Med. 2016;67:730-736 e2. doi: 10.1016/j.annemergmed.2015.09.018. PubMed
12. Gaieski DF, Agarwal AK, Mikkelsen ME, et al. The impact of ED crowding on early interventions and mortality in patients with severe sepsis. Am J Emerg Med. 2017;35:953-960. doi: 10.1016/j.ajem.2017.01.061. PubMed
13. Pines JM, Localio AR, Hollander JE, et al. The impact of emergency department crowding measures on time to antibiotics for patients with community-acquired pneumonia. Ann Emerg Med. 2007;50(5):510-516. doi: 10.1016/j.annemergmed.2007.07.021. PubMed
14. Hwang U, Richardson L, Livote E, Harris B, Spencer N, Sean Morrison R. Emergency department crowding and decreased quality of pain care. Acad Emerg Med. 2008;15:1248-1255. doi: 10.1111/j.1553-2712.2008.00267.x. PubMed
15. Mills AM, Shofer FS, Chen EH, Hollander JE, Pines JM. The association between emergency department crowding and analgesia administration in acute abdominal pain patients. Acad Emerg Med. 2009;16:603-608. doi: 10.1111/j.1553-2712.2009.00441.x. PubMed
16. Pines JM, Shofer FS, Isserman JA, Abbuhl SB, Mills AM. The effect of emergency department crowding on analgesia in patients with back pain in two hospitals. Acad Emerg Med. 2010;17(3):276-283. doi: 10.1111/j.1553-2712.2009.00676.x. PubMed
17. Kulstad EB, Sikka R, Sweis RT, Kelley KM, Rzechula KH. ED overcrowding is associated with an increased frequency of medication errors. Am J Emerg Med. 2010;28:304-309. doi: 10.1016/j.ajem.2008.12.014. PubMed
18. Richardson DB. Increase in patient mortality at 10 days associated with emergency department overcrowding. Med J Aust. 2006;184(5):213-216. PubMed
19. Hoot NR, Aronsky D. Systematic review of emergency department crowding: causes, effects, and solutions. Ann Emerg Med. 2008;52(2):126-136. doi: 10.1016/j.annemergmed.2008.03.014. PubMed
20. Singer AJ, Thode HC, Jr., Viccellio P, Pines JM. The association between length of emergency department boarding and mortality. Acad Emerg Med. 2011;18(12):1324-1329. doi: 10.1111/j.1553-2712.2011.01236.x. PubMed
21. White BA, Biddinger PD, Chang Y, Grabowski B, Carignan S, Brown DF. Boarding inpatients in the emergency department increases discharged patient length of stay. J Emerg Med. 2013;44(1):230-235. doi: 10.1016/j.jemermed.2012.05.007. PubMed
22. Forster AJ, Stiell I, Wells G, Lee AJ, van Walraven C. The effect of hospital occupancy on emergency department length of stay and patient disposition. Acad Emerg Med. 2003;10(2):127-133. doi: 10.1197/aemj.10.2.127. PubMed
23. Foley M, Kifaieh N, Mallon WK. Financial impact of emergency department crowding. West J Emerg Med. 2011;12(2):192-197. PubMed
24. Pines JM, Iyer S, Disbot M, Hollander JE, Shofer FS, Datner EM. The effect of emergency department crowding on patient satisfaction for admitted patients. Acad Emerg Med. 2008;15(9):825-831. doi: 10.1111/j.1553-2712.2008.00200.x. PubMed
25. Durvasula R, Kayihan A, Del Bene S, et al. A multidisciplinary care pathway significantly increases the number of early morning discharges in a large academic medical center. Qual Manag Health Care. 2015;24:45-51. doi: 10.1097/QMH.0000000000000049. PubMed
26. Cho HJ, Desai N, Florendo A, et al. E-DIP: Early Discharge Project. A Model for Throughput and Early Discharge for 1-Day Admissions. BMJ Qual Improv Rep. 2016;5(1): pii: u210035.w4128. doi: 10.1136/bmjquality.u210035.w4128. PubMed
27. Harris PA, Taylor R, Thielke R, Payne J, Gonzalez N, Conde JG. Research electronic data capture (REDCap)--a metadata-driven methodology and workflow process for providing translational research informatics support. J Biomed Inform. 2009;42(2):377-381. doi: 10.1016/j.jbi.2008.08.010. PubMed
28. Patel H, Fang MC, Mourad M, et al. Hospitalist and internal medicine leaders’ perspectives of early discharge challenges at academic medical centers. J Hosp Med. 2018;13(6):388-391. doi: 10.12788/jhm.2885. PubMed
29. Minichiello TM, Auerbach AD, Wachter RM. Caregiver perceptions of the reasons for delayed hospital discharge. Eff Clin Pract. 2001;4(6):250-255. PubMed
30. Beck MJ, Okerblom D, Kumar A, Bandyopadhyay S, Scalzi LV. Lean intervention improves patient discharge times, improves emergency department throughput and reduces congestion. Hosp Pract (1995). 2016;44(5):252-259. doi: 10.1080/21548331.2016.1254559. PubMed
31. Rajkomar A, Valencia V, Novelero M, Mourad M, Auerbach A. The association between discharge before noon and length of stay in medical and surgical patients. J Hosp Med. 2016;11(12):859-861. doi: 10.1002/jhm.2529. PubMed
32. Shine D. Discharge before noon: an urban legend. Am J Med. 2015;128(5):445-446. doi: 10.1016/j.amjmed.2014.12.011. PubMed33. Khanna S, Boyle J, Good N, Lind J. Unravelling relationships: Hospital occupancy levels, discharge timing and emergency department access block. Emerg Med Australas. 2012;24(5):510-517. doi: 10.1111/j.1742-6723.2012.01587.x. PubMed
Real‐Time Patient Experience Surveys
In 2010, the Centers for Medicare and Medicaid Services implemented value‐based purchasing, a payment model that incentivizes hospitals for reaching certain quality and patient experience thresholds and penalizes those that do not, in part on the basis of patient satisfaction scores.[1] Although low patient satisfaction scores will adversely affect institutions financially, they also reflect patients' perceptions of their care. Some studies suggest that hospitals with higher patient satisfaction scores score higher overall on clinical care processes such as core measures compliance, readmission rates, lower mortality rates, and other quality‐of‐care metrics.[2, 3, 4, 5, 6, 7, 8, 9, 10, 11]
The Hospital Consumer Assessment of Healthcare Providers and Systems (HCAHPS) survey assesses patients' experience following their hospital stay.[1] The percent of top box scores (ie, response of always on a four point scale, or scores of 9 or 10 on a 10‐point scale) are utilized to compare hospitals and determine the reimbursement or penalty a hospital will receive. Although these scores are available to the public on the Hospital Compare website,[12] physicians may not know how their hospital is ranked or how they are individually perceived by their patients. Additionally, these surveys are typically conducted 48 hours to 6 weeks after patients are discharged, and the results are distributed back to the hospitals well after the time that care was provided, thereby offering providers no chance of improving patient satisfaction during a given hospital stay.
Institutions across the country are trying to improve their HCAHPS scores, but there is limited research identifying specific measures providers can implement. Some studies have suggested that utilizing etiquette‐based communication and sitting at the bedside[13, 14] may help improve patient experience with their providers, and more recently, it has been suggested that providing real‐time deidentified patient experience survey results with education and a rewards/emncentive system to residents may help as well.[15]
Surveys conducted during a patient's hospitalization can offer real‐time actionable feedback to providers. We performed a quality‐improvement project that was designed to determine if real‐time feedback to hospitalist physicians, followed by coaching, and revisits to the patients' bedside could improve the results recorded on provider‐specific patient surveys and/or patients' HCAHPS scores or percentile rankings.
METHODS
Design
This was a prospective, randomized quality‐improvement initiative that was approved by the Colorado Multiple Institutional Review Board and conducted at Denver Health, a 525‐bed university‐affiliated public safety net hospital. The initiative was conducted on both teaching and nonteaching general internal medicine services, which typically have a daily census of between 10 and 15 patients. No protocol changes occurred during the study.
Participants
Participants included all English‐ or Spanish‐speaking patients who were hospitalized on a general internal medicine service, had been admitted within the 2 days prior to enrollment, and had a hospitalist as their attending physician. Patients were excluded if they were enrolled in the study during a previous hospitalization, refused to participate, lacked capacity to participate, had hearing or speech impediments precluding regular conversation, were prisoners, if their clinical condition precluded participation, or their attending was an investigator in the project.
Intervention
Participants were prescreened by investigators by reviewing team sign‐outs to determine if patients had any exclusion criteria. Investigators attempted to survey each patient who met inclusion criteria on a daily basis between 9:00 am and 11:00 am. An investigator administered the survey to each patient verbally using scripted language. Patients were asked to rate how well their doctors were listening to them, explaining what they wanted to know, and whether the doctors were being friendly and helpful, all questions taken from a survey that was available on the US Department of Health and Human Services website (to be referred to as here forward daily survey).[16] We converted the original 5‐point Likert scale used in this survey to a 4‐point scale by removing the option of ok, leaving participants the options of poor, fair, good, or great. Patients were also asked to provide any personalized feedback they had, and these comments were recorded in writing by the investigator.
After being surveyed on day 1, patients were randomized to an intervention or control group using an automated randomization module in Research Electronic Data Capture (REDCap).[17] Patients in both groups who did not provide answers to all 3 questions that qualified as being top box (ie, great) were resurveyed on a daily basis until their responses were all top box or they were discharged, met exclusion criteria, or had been surveyed for a total of 4 consecutive days. In the pilot phase of this study, we found that if patients reported all top box scores on the initial survey their responses typically did not change over time, and the patients became frustrated if asked the same questions again when the patient felt there was not room for improvement. Accordingly, we elected to stop surveying patients when all top box responses were reported.
The attending hospitalist caring for each patient in the intervention group was given feedback about their patients' survey results (both their scores and any specific comments) on a daily basis. Feedback was provided in person by 1 of the investigators. The hospitalist also received an automatically generated electronic mail message with the survey results at 11:00 am on each study day. After informing the hospitalists of the patients' scores, the investigator provided a brief education session that included discussing Denver Health's most recent HCAHPS scores, value‐based purchasing, and the financial consequences of poor patient satisfaction scores. The investigator then coached the hospitalist on etiquette‐based communication,[18, 19] suggested that they sit down when communicating with their patients,[19, 20] and then asked the hospitalist to revisit each patient to discuss how the team could improve in any of the 3 areas where the patient did not give a top box score. These educational sessions were conducted in person and lasted a maximum of 5 minutes. An investigator followed up with each hospitalist the following day to determine whether the revisit occurred. Hospitalists caring for patients who were randomized to the control group were not given real‐time feedback or coaching and were not asked to revisit patients.
A random sample of patients surveyed for this initiative also received HCAHPS surveys 48 hours to 6 weeks following their hospital discharge, according to the standard methodology used to acquire HCAHPS data,[21] by an outside vendor contracted by Denver Health. Our vendor conducted these surveys via telephone in English or Spanish.
Outcomes
The primary outcome was the proportion of patients in each group who reported top box scores on the daily surveys. Secondary outcomes included the percent change for the scores recorded for 3 provider‐specific questions from the daily survey, the median top box HCAHPS scores for the 3 provider related questions and overall hospital rating, and the HCAHPS percentiles of top box scores for these questions.
Sample Size
The sample size for this intervention assumed that the proportion of patients whose treating physicians did not receive real‐time feedback who rated their providers as top box would be 75%, and that the effect of providing real‐time feedback would increase this proportion to 85% on the daily surveys. To have 80% power with a type 1 error of 0.05, we estimated a need to enroll 430 patients, 215 in each group.
Statistics
Data were collected and managed using a secure, Web‐based electronic data capture tool hosted at Denver Health (REDCap), which is designed to support data collection for research studies providing: (1) an intuitive interface for validated data entry, (2) audit trails for tracking data manipulation and export procedures, (3) automated export procedures for seamless data downloads to common statistical packages, and (4) procedures for importing data from external sources.[17]
A 2 test was used to compare the proportion of patients in the 2 groups who reported great scores for each question on the study survey on the first and last day. With the intent of providing a framework for understanding the effect real‐time feedback could have on patient experience, a secondary analysis of HCAHPS results was conducted using several different methods.
First, the proportion of patients in the 2 groups who reported scores of 9 or 10 for the overall hospital rating question or reported always for each doctor communication question on the HCHAPS survey was compared using a 2. Second, to allow for detection of differences in a sample with a smaller N, the median overall hospital rating scores from the HCAHPS survey reported by patients in the 2 groups who completed a survey following discharge were compared using a Wilcoxon rank sum test. Lastly, to place changes in proportion into a larger context (ie, how these changes would relate to value‐based purchasing), HCAHPS scores were converted to percentiles of national performance using the 2014 percentile rankings obtained from the external vendor that conducts the HCAHPS surveys for our hospital and compared between the intervention and control groups using a Wilcoxon rank sum test.
All comments collected from patients during their daily surveys were reviewed, and key words were abstracted from each comment. These key words were sorted and reviewed to categorize recurring key words into themes. Exemplars were then selected for each theme derived from patient comments.
RESULTS
From April 14, 2014 to September 19, 2014, we enrolled 227 patients in the control group and 228 in the intervention group (Figure 1). Patient demographics are summarized in Table 1. Of the 132 patients in the intervention group who reported anything less than top box scores for any of the 3 questions (thus prompting a revisit by their provider), 106 (80%) were revisited by their provider at least once during their hospitalization.
All Patients | HCAHPS Patients | |||
---|---|---|---|---|
Control, N = 227 | Intervention, N = 228 | Control, N = 35 | Intervention, N = 30 | |
| ||||
Age, mean SD | 55 14 | 55 15 | 55 15 | 57 16 |
Gender | ||||
Male | 126 (60) | 121 (55) | 20 (57) | 12 (40) |
Female | 85 (40) | 98 (45) | 15(43) | 18 (60) |
Race/ethnicity | ||||
Hispanic | 84 (40) | 90 (41) | 17 (49) | 12 (40) |
Black | 38 (18) | 28 (13) | 6 (17) | 7 (23) |
White | 87 (41) | 97 (44) | 12 (34) | 10 (33) |
Other | 2 (1) | 4 (2) | 0 (0) | 1 (3) |
Payer | ||||
Medicare | 65 (29) | 82 (36) | 15 (43) | 12 (40) |
Medicaid | 122 (54) | 108 (47) | 17 (49) | 14 (47) |
Commercial | 12 (5) | 15 (7) | 1 (3) | 1 (3) |
Medically indigent | 4 (2) | 7 (3) | 0 (0) | 3 (10) |
Self‐pay | 5 (2) | 4 (2) | 1 (3) | 0 (0) |
Other/unknown | 19 (8) | 12 (5) | 0 (0) | 0 (0) |
Team | ||||
Teaching | 187 (82) | 196 (86) | 27 (77) | 24 (80) |
Nonteaching | 40 (18) | 32 (14) | 8 (23) | 6 (20) |
Top 5 primary discharge diagnoses* | ||||
Septicemia | 26 (11) | 34 (15) | 3 (9) | 5 (17) |
Heart failure | 14 (6) | 13 (6) | 2 (6) | |
Acute pancreatitis | 12 (5) | 9 (4) | 3 (9) | 2 (7) |
Diabetes mellitus | 11 (5) | 8 (4) | 2 (6) | |
Alcohol withdrawal | 9 (4) | |||
Cellulitis | 7 (3) | 2 (7) | ||
Pulmonary embolism | 2 (7) | |||
Chest pain | 2 (7) | |||
Atrial fibrillation | 2 (6) | |||
Length of stay, median (IQR) | 3 (2, 5) | 3 (2, 5) | 3 (2, 5) | 3 (2, 4) |
Charlson Comorbidity Index, median (IQR) | 1 (0, 3) | 2 (0, 3) | 1 (0, 3) | 1.5 (1, 3) |
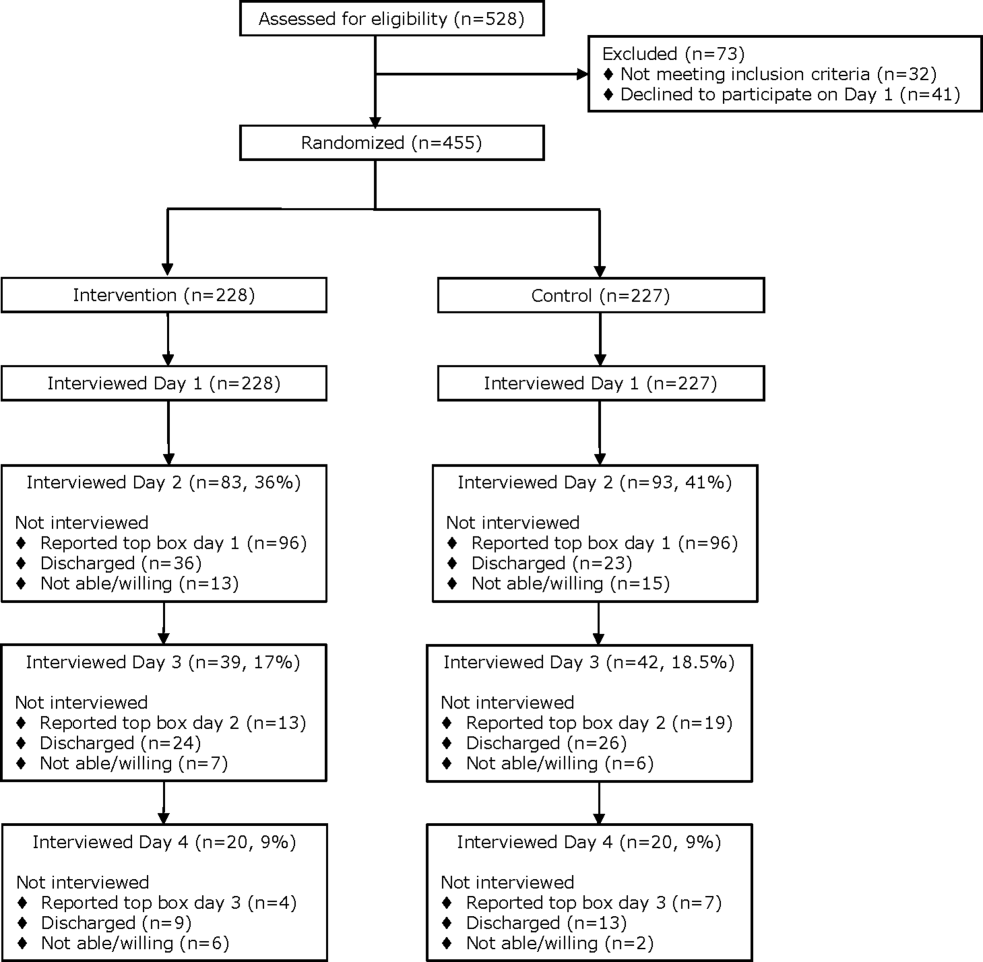
Daily Surveys
The proportion of patients in both study groups reporting top box scores tended to increase from the first day to the last day of the survey (Figure 2); however, we found no statistically significant differences between the proportion of patients who reported top box scores on first day or last day in the intervention group compared to the control group. The comments made by the patients are summarized in Supporting Table 1 in the online version of this article.
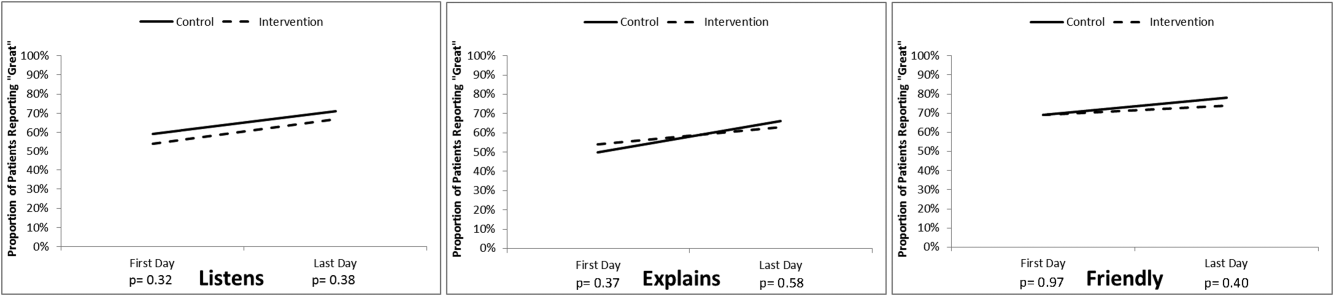
HCAHPS Scores
The proportion of top box scores from the HCAHPS surveys were higher, though not statistically significant, for all 3 provider‐specific questions and for the overall hospital rating for patients whose hospitalists received real‐time feedback (Table 2). The median [interquartile range] score for the overall hospital rating was higher for patients in the intervention group compared with those in the control group, (10 [9, 10] vs 9 [8, 10], P = 0.04]. After converting the HCAHPS scores to percentiles, we found considerably higher rankings for all 3 provider‐related questions and for the overall hospital rating in the intervention group compared to the control group (P = 0.02 for overall differences in percentiles [Table 2]).
HCAHPS Questions | Proportion Top Box* | Percentile Rank | ||
---|---|---|---|---|
Control, N = 35 | Intervention, N = 30 | Control, N = 35 | Intervention, N = 30 | |
| ||||
Overall hospital rating | 61% | 80% | 6 | 87 |
Courtesy/respect | 86% | 93% | 23 | 88 |
Clear communication | 77% | 80% | 39 | 60 |
Listening | 83% | 90% | 57 | 95 |
No adverse events occurred during the course of the study in either group.
DISCUSSION
The important findings of this study were that (1) daily patient satisfaction scores improved from first day to last day regardless of study group, (2) patients whose providers received real‐time feedback had a trend toward higher HCAHPS proportions for the 3 provider‐related questions as well as the overall rating of the hospital but were not statistically significant, (3) the percentile differences in these 3 questions as well as the overall rating of the hospital were significantly higher in the intervention group as was the median score for the overall hospital rating.
Our original sample size calculation was based upon our own preliminary data, indicating that our baseline top box scores for the daily survey was around 75%. The daily survey top box score on the first day was, however, much lower (Figure 2). Accordingly, although we did not find a significant difference in these daily scores, we were underpowered to find such a difference. Additionally, because only a small percentage of patients are selected for the HCAHPS survey, our ability to detect a difference in this secondary outcome was also limited. We felt that it was important to analyze the percentile comparisons in addition to the proportion of top box scores on the HCAHPS, because the metrics for value‐based purchasing are based upon, in part, how a hospital system compares to other systems. Finally, to improve our power to detect a difference given a small sample size, we converted the scoring system for overall hospital ranking to a continuous variable, which again was noted to be significant.
To our knowledge, this is the first randomized investigation designed to assess the effect of real‐time, patient‐specific feedback to physicians. Real‐time feedback is increasingly being incorporated into medical practice, but there is only limited information available describing how this type of feedback affects outcomes.[22, 23, 24] Banka et al.[15] found that HCAHPS scores improved as a result of real‐time feedback given to residents, but the study was not randomized, utilized a pre‐post design that resulted in there being differences between the patients studied before and after the intervention, and did not provide patient‐specific data to the residents. Tabib et al.[25] found that operating costs decreased 17% after instituting real‐time feedback to providers about these costs. Reeves et al.[26] conducted a cluster randomized trial of a patient feedback survey that was designed to improve nursing care, but the results were reviewed by the nurses several months after patients had been discharged.
The differences in median top box scores and percentile rank that we observed could have resulted from the real‐time feedback, the educational coaching, the fact that the providers revisited the majority of the patients, or a combination of all of the above. Gross et al.[27] found that longer visits lead to higher satisfaction, though others have not found this to necessarily be the case.[28, 29] Lin et al.[30] found that patient satisfaction was affected by the perceived duration of the visit as well as whether expectations on visit length were met and/or exceeded. Brown et al.[31] found that training providers in communication skills improved the providers perception of their communication skills, although patient experience scores did not improve. We feel that the results seen are more likely a combination thereof as opposed to any 1 component of the intervention.
The most commonly reported complaints or concerns in patients' undirected comments often related to communication issues. Comments on subsequent surveys suggested that patient satisfaction improved over time in the intervention group, indicating that perhaps physicians did try to improve in areas that were highlighted by the real‐time feedback, and that patients perceived the physician efforts to do so (eg, They're doing better than the last time you asked. They sat down and talked to me and listened better. They came back and explained to me about my care. They listened better. They should do this survey at the clinic. See Supporting Table 1 in the online version of this article).
Our study has several limitations. First, we did not randomize providers, and many of our providers (approximately 65%) participated in both the control group and also in the intervention group, and thus received real‐time feedback at some point during the study, which could have affected their overall practice and limited our ability to find a difference between the 2 groups. In an attempt to control for this possibility, the study was conducted on an intermittent basis during the study time frame. Furthermore, the proportion of patients who reported top box scores at the beginning of the study did not have a clear trend of change by the end of the study, suggesting that overall clinician practices with respect to patient satisfaction did not change during this short time period.
Second, only a small number of our patients were randomly selected for the HCAHPS survey, which limited our ability to detect significant differences in HCAHPS proportions. Third, the HCAHPS percentiles at our institution at that time were low. Accordingly, the improvements that we observed in patient satisfaction scores might not be reproducible at institutions with higher satisfactions scores. Fourth, time and resources were needed to obtain patient feedback to provide to providers during this study. There are, however, other ways to obtain feedback that are less resource intensive (eg, electronic feedback, the utilization of volunteers, or partnering this with manager rounding). Finally, the study was conducted at a single, university‐affiliated public teaching hospital and was a quality‐improvement initiative, and thus our results are not generalizable to other institutions.
In conclusion, real‐time feedback of patient experience to their providers, coupled with provider education, coaching, and revisits, seems to improve satisfaction of patients hospitalized on general internal medicine units who were cared for by hospitalists.
Acknowledgements
The authors thank Kate Fagan, MPH, for her excellent technical assistance.
Disclosure: Nothing to report.
- HCAHPS Fact Sheet. 2015. Available at: http://www.hcahpsonline.org/Files/HCAHPS_Fact_Sheet_June_2015.pdf. Accessed August 25, 2015.
- The relationship between commercial website ratings and traditional hospital performance measures in the USA. BMJ Qual Saf. 2013;22:194–202. , , , .
- Patients' perception of hospital care in the United States. N Engl J Med. 2008;359:1921–1931. , , , .
- The relationship between patients' perception of care and measures of hospital quality and safety. Health Serv Res. 2010;45:1024–1040. , , , .
- Relationship between quality of diabetes care and patient satisfaction. J Natl Med Assoc. 2003;95:64–70. , , , et al.
- Relationship between patient satisfaction with inpatient care and hospital readmission within 30 days. Am J Manag Care. 2011;17:41–48. , , , , .
- A systematic review of evidence on the links between patient experience and clinical safety and effectiveness. BMJ Open. 2013;3(1). , , .
- The association between satisfaction with services provided in primary care and outcomes in type 2 diabetes mellitus. Diabet Med. 2003;20:486–490. , .
- Associations between Web‐based patient ratings and objective measures of hospital quality. Arch Intern Med. 2012;172:435–436. , , , et al.
- Patient satisfaction and its relationship with clinical quality and inpatient mortality in acute myocardial infarction. Circ Cardiovasc Qual Outcomes. 2010;3:188–195. , , , et al.
- Patients' perceptions of care are associated with quality of hospital care: a survey of 4605 hospitals. Am J Med Qual. 2015;30(4):382–388. , , , , .
- Centers for Medicare 28:908–913.
- Effect of sitting vs. standing on perception of provider time at bedside: a pilot study. Patient Educ Couns. 2012;86:166–171. , , , , , .
- Improving patient satisfaction through physician education, feedback, and incentives. J Hosp Med. 2015;10:497–502. , , , et al.
- US Department of Health and Human Services. Patient satisfaction survey. Available at: http://bphc.hrsa.gov/policiesregulations/performancemeasures/patientsurvey/surveyform.html. Accessed November 15, 2013.
- Research electronic data capture (REDCap)—a metadata‐driven methodology and workflow process for providing translational research informatics support. J Biomed Inform. 2009;42:377–381. , , , , , .
- The HCAHPS Handbook. Gulf Breeze, FL: Fire Starter; 2010. .
- Etiquette‐based medicine. N Engl J Med. 2008;358:1988–1989. .
- 5 years after the Kahn's etiquette‐based medicine: a brief checklist proposal for a functional second meeting with the patient. Front Psychol. 2013;4:723. .
- Frequently Asked Questions. Hospital Value‐Based Purchasing Program. Available at: http://www.cms.gov/Medicare/Quality‐Initiatives‐Patient‐Assessment‐Instruments/hospital‐value‐based‐purchasing/Downloads/FY‐2013‐Program‐Frequently‐Asked‐Questions‐about‐Hospital‐VBP‐3‐9‐12.pdf. Accessed February 8, 2014.
- Real‐time patient survey data during routine clinical activities for rapid‐cycle quality improvement. JMIR Med Inform. 2015;3:e13. , , , .
- Mount Sinai launches real‐time patient‐feedback survey tool. Healthcare Informatics website. Available at: http://www.healthcare‐informatics.com/news‐item/mount‐sinai‐launches‐real‐time‐patient‐feedback‐survey‐tool. Accessed August 25, 2015. .
- Hospitals are finally starting to put real‐time data to use. Harvard Business Review website. Available at: https://hbr.org/2014/11/hospitals‐are‐finally‐starting‐to‐put‐real‐time‐data‐to‐use. Published November 12, 2014. Accessed August 25, 2015. , .
- Reducing operating room costs through real‐time cost information feedback: a pilot study. J Endourol. 2015;29:963–968. , , , , .
- Facilitated patient experience feedback can improve nursing care: a pilot study for a phase III cluster randomised controlled trial. BMC Health Serv Res. 2013;13:259. , , .
- Patient satisfaction with time spent with their physician. J Fam Pract. 1998;47:133–137. , , , , .
- The relationship between time spent communicating and communication outcomes on a hospital medicine service. J Gen Intern Med. 2012;27:185–189. , , , , , .
- Cognitive interview techniques reveal specific behaviors and issues that could affect patient satisfaction relative to hospitalists. J Hosp Med. 2009;4:E1–E6. , .
- Is patients' perception of time spent with the physician a determinant of ambulatory patient satisfaction? Arch Intern Med. 2001;161:1437–1442. , , , et al.
- Effect of clinician communication skills training on patient satisfaction. A randomized, controlled trial. Ann Intern Med. 1999;131:822–829. , , , .
In 2010, the Centers for Medicare and Medicaid Services implemented value‐based purchasing, a payment model that incentivizes hospitals for reaching certain quality and patient experience thresholds and penalizes those that do not, in part on the basis of patient satisfaction scores.[1] Although low patient satisfaction scores will adversely affect institutions financially, they also reflect patients' perceptions of their care. Some studies suggest that hospitals with higher patient satisfaction scores score higher overall on clinical care processes such as core measures compliance, readmission rates, lower mortality rates, and other quality‐of‐care metrics.[2, 3, 4, 5, 6, 7, 8, 9, 10, 11]
The Hospital Consumer Assessment of Healthcare Providers and Systems (HCAHPS) survey assesses patients' experience following their hospital stay.[1] The percent of top box scores (ie, response of always on a four point scale, or scores of 9 or 10 on a 10‐point scale) are utilized to compare hospitals and determine the reimbursement or penalty a hospital will receive. Although these scores are available to the public on the Hospital Compare website,[12] physicians may not know how their hospital is ranked or how they are individually perceived by their patients. Additionally, these surveys are typically conducted 48 hours to 6 weeks after patients are discharged, and the results are distributed back to the hospitals well after the time that care was provided, thereby offering providers no chance of improving patient satisfaction during a given hospital stay.
Institutions across the country are trying to improve their HCAHPS scores, but there is limited research identifying specific measures providers can implement. Some studies have suggested that utilizing etiquette‐based communication and sitting at the bedside[13, 14] may help improve patient experience with their providers, and more recently, it has been suggested that providing real‐time deidentified patient experience survey results with education and a rewards/emncentive system to residents may help as well.[15]
Surveys conducted during a patient's hospitalization can offer real‐time actionable feedback to providers. We performed a quality‐improvement project that was designed to determine if real‐time feedback to hospitalist physicians, followed by coaching, and revisits to the patients' bedside could improve the results recorded on provider‐specific patient surveys and/or patients' HCAHPS scores or percentile rankings.
METHODS
Design
This was a prospective, randomized quality‐improvement initiative that was approved by the Colorado Multiple Institutional Review Board and conducted at Denver Health, a 525‐bed university‐affiliated public safety net hospital. The initiative was conducted on both teaching and nonteaching general internal medicine services, which typically have a daily census of between 10 and 15 patients. No protocol changes occurred during the study.
Participants
Participants included all English‐ or Spanish‐speaking patients who were hospitalized on a general internal medicine service, had been admitted within the 2 days prior to enrollment, and had a hospitalist as their attending physician. Patients were excluded if they were enrolled in the study during a previous hospitalization, refused to participate, lacked capacity to participate, had hearing or speech impediments precluding regular conversation, were prisoners, if their clinical condition precluded participation, or their attending was an investigator in the project.
Intervention
Participants were prescreened by investigators by reviewing team sign‐outs to determine if patients had any exclusion criteria. Investigators attempted to survey each patient who met inclusion criteria on a daily basis between 9:00 am and 11:00 am. An investigator administered the survey to each patient verbally using scripted language. Patients were asked to rate how well their doctors were listening to them, explaining what they wanted to know, and whether the doctors were being friendly and helpful, all questions taken from a survey that was available on the US Department of Health and Human Services website (to be referred to as here forward daily survey).[16] We converted the original 5‐point Likert scale used in this survey to a 4‐point scale by removing the option of ok, leaving participants the options of poor, fair, good, or great. Patients were also asked to provide any personalized feedback they had, and these comments were recorded in writing by the investigator.
After being surveyed on day 1, patients were randomized to an intervention or control group using an automated randomization module in Research Electronic Data Capture (REDCap).[17] Patients in both groups who did not provide answers to all 3 questions that qualified as being top box (ie, great) were resurveyed on a daily basis until their responses were all top box or they were discharged, met exclusion criteria, or had been surveyed for a total of 4 consecutive days. In the pilot phase of this study, we found that if patients reported all top box scores on the initial survey their responses typically did not change over time, and the patients became frustrated if asked the same questions again when the patient felt there was not room for improvement. Accordingly, we elected to stop surveying patients when all top box responses were reported.
The attending hospitalist caring for each patient in the intervention group was given feedback about their patients' survey results (both their scores and any specific comments) on a daily basis. Feedback was provided in person by 1 of the investigators. The hospitalist also received an automatically generated electronic mail message with the survey results at 11:00 am on each study day. After informing the hospitalists of the patients' scores, the investigator provided a brief education session that included discussing Denver Health's most recent HCAHPS scores, value‐based purchasing, and the financial consequences of poor patient satisfaction scores. The investigator then coached the hospitalist on etiquette‐based communication,[18, 19] suggested that they sit down when communicating with their patients,[19, 20] and then asked the hospitalist to revisit each patient to discuss how the team could improve in any of the 3 areas where the patient did not give a top box score. These educational sessions were conducted in person and lasted a maximum of 5 minutes. An investigator followed up with each hospitalist the following day to determine whether the revisit occurred. Hospitalists caring for patients who were randomized to the control group were not given real‐time feedback or coaching and were not asked to revisit patients.
A random sample of patients surveyed for this initiative also received HCAHPS surveys 48 hours to 6 weeks following their hospital discharge, according to the standard methodology used to acquire HCAHPS data,[21] by an outside vendor contracted by Denver Health. Our vendor conducted these surveys via telephone in English or Spanish.
Outcomes
The primary outcome was the proportion of patients in each group who reported top box scores on the daily surveys. Secondary outcomes included the percent change for the scores recorded for 3 provider‐specific questions from the daily survey, the median top box HCAHPS scores for the 3 provider related questions and overall hospital rating, and the HCAHPS percentiles of top box scores for these questions.
Sample Size
The sample size for this intervention assumed that the proportion of patients whose treating physicians did not receive real‐time feedback who rated their providers as top box would be 75%, and that the effect of providing real‐time feedback would increase this proportion to 85% on the daily surveys. To have 80% power with a type 1 error of 0.05, we estimated a need to enroll 430 patients, 215 in each group.
Statistics
Data were collected and managed using a secure, Web‐based electronic data capture tool hosted at Denver Health (REDCap), which is designed to support data collection for research studies providing: (1) an intuitive interface for validated data entry, (2) audit trails for tracking data manipulation and export procedures, (3) automated export procedures for seamless data downloads to common statistical packages, and (4) procedures for importing data from external sources.[17]
A 2 test was used to compare the proportion of patients in the 2 groups who reported great scores for each question on the study survey on the first and last day. With the intent of providing a framework for understanding the effect real‐time feedback could have on patient experience, a secondary analysis of HCAHPS results was conducted using several different methods.
First, the proportion of patients in the 2 groups who reported scores of 9 or 10 for the overall hospital rating question or reported always for each doctor communication question on the HCHAPS survey was compared using a 2. Second, to allow for detection of differences in a sample with a smaller N, the median overall hospital rating scores from the HCAHPS survey reported by patients in the 2 groups who completed a survey following discharge were compared using a Wilcoxon rank sum test. Lastly, to place changes in proportion into a larger context (ie, how these changes would relate to value‐based purchasing), HCAHPS scores were converted to percentiles of national performance using the 2014 percentile rankings obtained from the external vendor that conducts the HCAHPS surveys for our hospital and compared between the intervention and control groups using a Wilcoxon rank sum test.
All comments collected from patients during their daily surveys were reviewed, and key words were abstracted from each comment. These key words were sorted and reviewed to categorize recurring key words into themes. Exemplars were then selected for each theme derived from patient comments.
RESULTS
From April 14, 2014 to September 19, 2014, we enrolled 227 patients in the control group and 228 in the intervention group (Figure 1). Patient demographics are summarized in Table 1. Of the 132 patients in the intervention group who reported anything less than top box scores for any of the 3 questions (thus prompting a revisit by their provider), 106 (80%) were revisited by their provider at least once during their hospitalization.
All Patients | HCAHPS Patients | |||
---|---|---|---|---|
Control, N = 227 | Intervention, N = 228 | Control, N = 35 | Intervention, N = 30 | |
| ||||
Age, mean SD | 55 14 | 55 15 | 55 15 | 57 16 |
Gender | ||||
Male | 126 (60) | 121 (55) | 20 (57) | 12 (40) |
Female | 85 (40) | 98 (45) | 15(43) | 18 (60) |
Race/ethnicity | ||||
Hispanic | 84 (40) | 90 (41) | 17 (49) | 12 (40) |
Black | 38 (18) | 28 (13) | 6 (17) | 7 (23) |
White | 87 (41) | 97 (44) | 12 (34) | 10 (33) |
Other | 2 (1) | 4 (2) | 0 (0) | 1 (3) |
Payer | ||||
Medicare | 65 (29) | 82 (36) | 15 (43) | 12 (40) |
Medicaid | 122 (54) | 108 (47) | 17 (49) | 14 (47) |
Commercial | 12 (5) | 15 (7) | 1 (3) | 1 (3) |
Medically indigent | 4 (2) | 7 (3) | 0 (0) | 3 (10) |
Self‐pay | 5 (2) | 4 (2) | 1 (3) | 0 (0) |
Other/unknown | 19 (8) | 12 (5) | 0 (0) | 0 (0) |
Team | ||||
Teaching | 187 (82) | 196 (86) | 27 (77) | 24 (80) |
Nonteaching | 40 (18) | 32 (14) | 8 (23) | 6 (20) |
Top 5 primary discharge diagnoses* | ||||
Septicemia | 26 (11) | 34 (15) | 3 (9) | 5 (17) |
Heart failure | 14 (6) | 13 (6) | 2 (6) | |
Acute pancreatitis | 12 (5) | 9 (4) | 3 (9) | 2 (7) |
Diabetes mellitus | 11 (5) | 8 (4) | 2 (6) | |
Alcohol withdrawal | 9 (4) | |||
Cellulitis | 7 (3) | 2 (7) | ||
Pulmonary embolism | 2 (7) | |||
Chest pain | 2 (7) | |||
Atrial fibrillation | 2 (6) | |||
Length of stay, median (IQR) | 3 (2, 5) | 3 (2, 5) | 3 (2, 5) | 3 (2, 4) |
Charlson Comorbidity Index, median (IQR) | 1 (0, 3) | 2 (0, 3) | 1 (0, 3) | 1.5 (1, 3) |
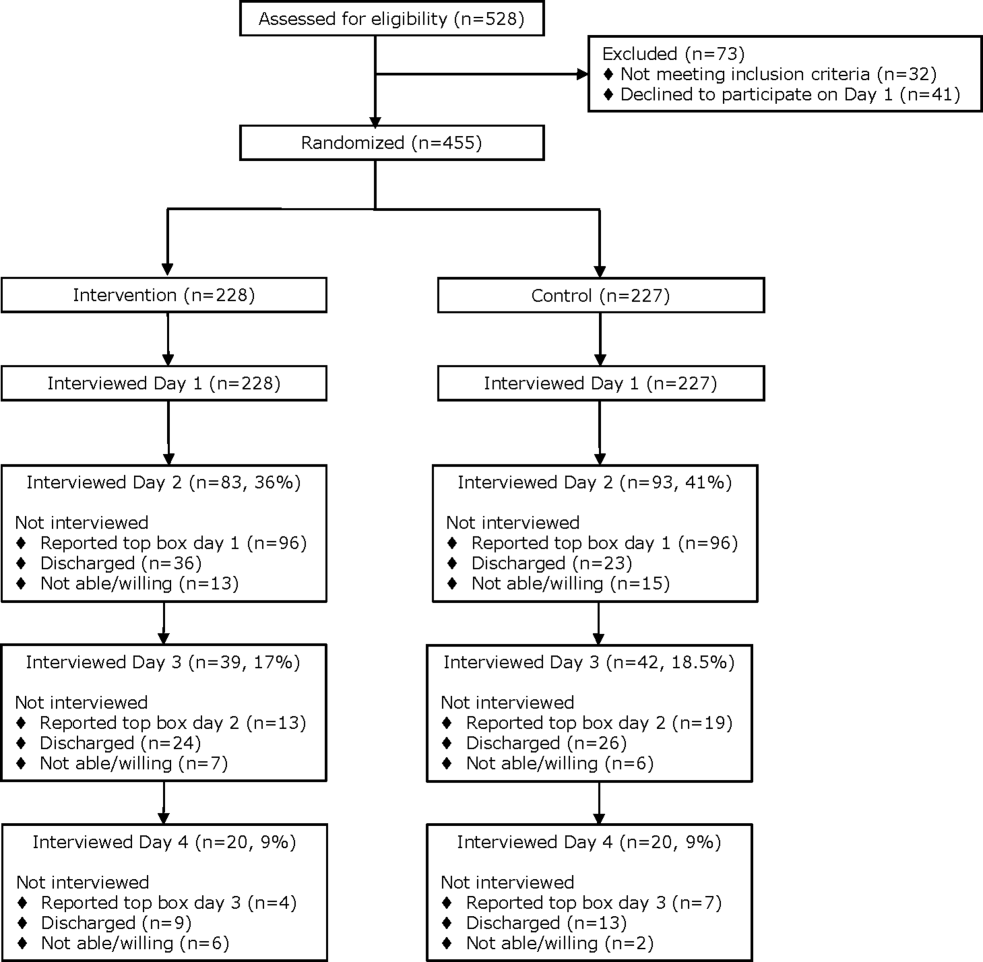
Daily Surveys
The proportion of patients in both study groups reporting top box scores tended to increase from the first day to the last day of the survey (Figure 2); however, we found no statistically significant differences between the proportion of patients who reported top box scores on first day or last day in the intervention group compared to the control group. The comments made by the patients are summarized in Supporting Table 1 in the online version of this article.
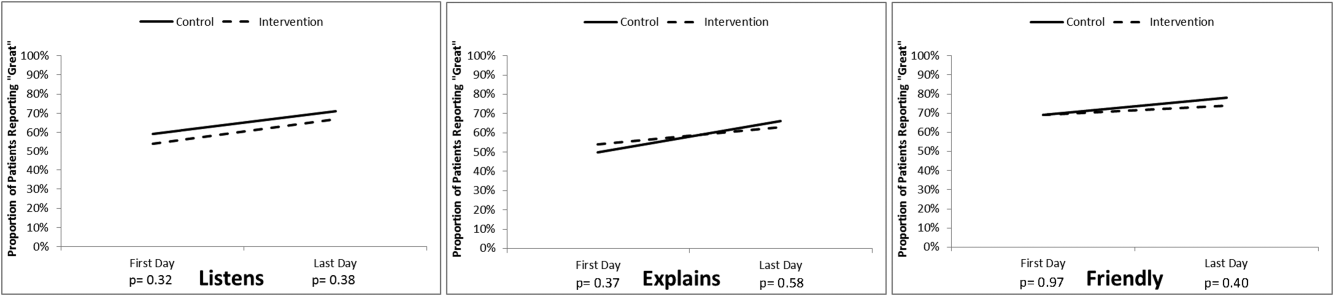
HCAHPS Scores
The proportion of top box scores from the HCAHPS surveys were higher, though not statistically significant, for all 3 provider‐specific questions and for the overall hospital rating for patients whose hospitalists received real‐time feedback (Table 2). The median [interquartile range] score for the overall hospital rating was higher for patients in the intervention group compared with those in the control group, (10 [9, 10] vs 9 [8, 10], P = 0.04]. After converting the HCAHPS scores to percentiles, we found considerably higher rankings for all 3 provider‐related questions and for the overall hospital rating in the intervention group compared to the control group (P = 0.02 for overall differences in percentiles [Table 2]).
HCAHPS Questions | Proportion Top Box* | Percentile Rank | ||
---|---|---|---|---|
Control, N = 35 | Intervention, N = 30 | Control, N = 35 | Intervention, N = 30 | |
| ||||
Overall hospital rating | 61% | 80% | 6 | 87 |
Courtesy/respect | 86% | 93% | 23 | 88 |
Clear communication | 77% | 80% | 39 | 60 |
Listening | 83% | 90% | 57 | 95 |
No adverse events occurred during the course of the study in either group.
DISCUSSION
The important findings of this study were that (1) daily patient satisfaction scores improved from first day to last day regardless of study group, (2) patients whose providers received real‐time feedback had a trend toward higher HCAHPS proportions for the 3 provider‐related questions as well as the overall rating of the hospital but were not statistically significant, (3) the percentile differences in these 3 questions as well as the overall rating of the hospital were significantly higher in the intervention group as was the median score for the overall hospital rating.
Our original sample size calculation was based upon our own preliminary data, indicating that our baseline top box scores for the daily survey was around 75%. The daily survey top box score on the first day was, however, much lower (Figure 2). Accordingly, although we did not find a significant difference in these daily scores, we were underpowered to find such a difference. Additionally, because only a small percentage of patients are selected for the HCAHPS survey, our ability to detect a difference in this secondary outcome was also limited. We felt that it was important to analyze the percentile comparisons in addition to the proportion of top box scores on the HCAHPS, because the metrics for value‐based purchasing are based upon, in part, how a hospital system compares to other systems. Finally, to improve our power to detect a difference given a small sample size, we converted the scoring system for overall hospital ranking to a continuous variable, which again was noted to be significant.
To our knowledge, this is the first randomized investigation designed to assess the effect of real‐time, patient‐specific feedback to physicians. Real‐time feedback is increasingly being incorporated into medical practice, but there is only limited information available describing how this type of feedback affects outcomes.[22, 23, 24] Banka et al.[15] found that HCAHPS scores improved as a result of real‐time feedback given to residents, but the study was not randomized, utilized a pre‐post design that resulted in there being differences between the patients studied before and after the intervention, and did not provide patient‐specific data to the residents. Tabib et al.[25] found that operating costs decreased 17% after instituting real‐time feedback to providers about these costs. Reeves et al.[26] conducted a cluster randomized trial of a patient feedback survey that was designed to improve nursing care, but the results were reviewed by the nurses several months after patients had been discharged.
The differences in median top box scores and percentile rank that we observed could have resulted from the real‐time feedback, the educational coaching, the fact that the providers revisited the majority of the patients, or a combination of all of the above. Gross et al.[27] found that longer visits lead to higher satisfaction, though others have not found this to necessarily be the case.[28, 29] Lin et al.[30] found that patient satisfaction was affected by the perceived duration of the visit as well as whether expectations on visit length were met and/or exceeded. Brown et al.[31] found that training providers in communication skills improved the providers perception of their communication skills, although patient experience scores did not improve. We feel that the results seen are more likely a combination thereof as opposed to any 1 component of the intervention.
The most commonly reported complaints or concerns in patients' undirected comments often related to communication issues. Comments on subsequent surveys suggested that patient satisfaction improved over time in the intervention group, indicating that perhaps physicians did try to improve in areas that were highlighted by the real‐time feedback, and that patients perceived the physician efforts to do so (eg, They're doing better than the last time you asked. They sat down and talked to me and listened better. They came back and explained to me about my care. They listened better. They should do this survey at the clinic. See Supporting Table 1 in the online version of this article).
Our study has several limitations. First, we did not randomize providers, and many of our providers (approximately 65%) participated in both the control group and also in the intervention group, and thus received real‐time feedback at some point during the study, which could have affected their overall practice and limited our ability to find a difference between the 2 groups. In an attempt to control for this possibility, the study was conducted on an intermittent basis during the study time frame. Furthermore, the proportion of patients who reported top box scores at the beginning of the study did not have a clear trend of change by the end of the study, suggesting that overall clinician practices with respect to patient satisfaction did not change during this short time period.
Second, only a small number of our patients were randomly selected for the HCAHPS survey, which limited our ability to detect significant differences in HCAHPS proportions. Third, the HCAHPS percentiles at our institution at that time were low. Accordingly, the improvements that we observed in patient satisfaction scores might not be reproducible at institutions with higher satisfactions scores. Fourth, time and resources were needed to obtain patient feedback to provide to providers during this study. There are, however, other ways to obtain feedback that are less resource intensive (eg, electronic feedback, the utilization of volunteers, or partnering this with manager rounding). Finally, the study was conducted at a single, university‐affiliated public teaching hospital and was a quality‐improvement initiative, and thus our results are not generalizable to other institutions.
In conclusion, real‐time feedback of patient experience to their providers, coupled with provider education, coaching, and revisits, seems to improve satisfaction of patients hospitalized on general internal medicine units who were cared for by hospitalists.
Acknowledgements
The authors thank Kate Fagan, MPH, for her excellent technical assistance.
Disclosure: Nothing to report.
In 2010, the Centers for Medicare and Medicaid Services implemented value‐based purchasing, a payment model that incentivizes hospitals for reaching certain quality and patient experience thresholds and penalizes those that do not, in part on the basis of patient satisfaction scores.[1] Although low patient satisfaction scores will adversely affect institutions financially, they also reflect patients' perceptions of their care. Some studies suggest that hospitals with higher patient satisfaction scores score higher overall on clinical care processes such as core measures compliance, readmission rates, lower mortality rates, and other quality‐of‐care metrics.[2, 3, 4, 5, 6, 7, 8, 9, 10, 11]
The Hospital Consumer Assessment of Healthcare Providers and Systems (HCAHPS) survey assesses patients' experience following their hospital stay.[1] The percent of top box scores (ie, response of always on a four point scale, or scores of 9 or 10 on a 10‐point scale) are utilized to compare hospitals and determine the reimbursement or penalty a hospital will receive. Although these scores are available to the public on the Hospital Compare website,[12] physicians may not know how their hospital is ranked or how they are individually perceived by their patients. Additionally, these surveys are typically conducted 48 hours to 6 weeks after patients are discharged, and the results are distributed back to the hospitals well after the time that care was provided, thereby offering providers no chance of improving patient satisfaction during a given hospital stay.
Institutions across the country are trying to improve their HCAHPS scores, but there is limited research identifying specific measures providers can implement. Some studies have suggested that utilizing etiquette‐based communication and sitting at the bedside[13, 14] may help improve patient experience with their providers, and more recently, it has been suggested that providing real‐time deidentified patient experience survey results with education and a rewards/emncentive system to residents may help as well.[15]
Surveys conducted during a patient's hospitalization can offer real‐time actionable feedback to providers. We performed a quality‐improvement project that was designed to determine if real‐time feedback to hospitalist physicians, followed by coaching, and revisits to the patients' bedside could improve the results recorded on provider‐specific patient surveys and/or patients' HCAHPS scores or percentile rankings.
METHODS
Design
This was a prospective, randomized quality‐improvement initiative that was approved by the Colorado Multiple Institutional Review Board and conducted at Denver Health, a 525‐bed university‐affiliated public safety net hospital. The initiative was conducted on both teaching and nonteaching general internal medicine services, which typically have a daily census of between 10 and 15 patients. No protocol changes occurred during the study.
Participants
Participants included all English‐ or Spanish‐speaking patients who were hospitalized on a general internal medicine service, had been admitted within the 2 days prior to enrollment, and had a hospitalist as their attending physician. Patients were excluded if they were enrolled in the study during a previous hospitalization, refused to participate, lacked capacity to participate, had hearing or speech impediments precluding regular conversation, were prisoners, if their clinical condition precluded participation, or their attending was an investigator in the project.
Intervention
Participants were prescreened by investigators by reviewing team sign‐outs to determine if patients had any exclusion criteria. Investigators attempted to survey each patient who met inclusion criteria on a daily basis between 9:00 am and 11:00 am. An investigator administered the survey to each patient verbally using scripted language. Patients were asked to rate how well their doctors were listening to them, explaining what they wanted to know, and whether the doctors were being friendly and helpful, all questions taken from a survey that was available on the US Department of Health and Human Services website (to be referred to as here forward daily survey).[16] We converted the original 5‐point Likert scale used in this survey to a 4‐point scale by removing the option of ok, leaving participants the options of poor, fair, good, or great. Patients were also asked to provide any personalized feedback they had, and these comments were recorded in writing by the investigator.
After being surveyed on day 1, patients were randomized to an intervention or control group using an automated randomization module in Research Electronic Data Capture (REDCap).[17] Patients in both groups who did not provide answers to all 3 questions that qualified as being top box (ie, great) were resurveyed on a daily basis until their responses were all top box or they were discharged, met exclusion criteria, or had been surveyed for a total of 4 consecutive days. In the pilot phase of this study, we found that if patients reported all top box scores on the initial survey their responses typically did not change over time, and the patients became frustrated if asked the same questions again when the patient felt there was not room for improvement. Accordingly, we elected to stop surveying patients when all top box responses were reported.
The attending hospitalist caring for each patient in the intervention group was given feedback about their patients' survey results (both their scores and any specific comments) on a daily basis. Feedback was provided in person by 1 of the investigators. The hospitalist also received an automatically generated electronic mail message with the survey results at 11:00 am on each study day. After informing the hospitalists of the patients' scores, the investigator provided a brief education session that included discussing Denver Health's most recent HCAHPS scores, value‐based purchasing, and the financial consequences of poor patient satisfaction scores. The investigator then coached the hospitalist on etiquette‐based communication,[18, 19] suggested that they sit down when communicating with their patients,[19, 20] and then asked the hospitalist to revisit each patient to discuss how the team could improve in any of the 3 areas where the patient did not give a top box score. These educational sessions were conducted in person and lasted a maximum of 5 minutes. An investigator followed up with each hospitalist the following day to determine whether the revisit occurred. Hospitalists caring for patients who were randomized to the control group were not given real‐time feedback or coaching and were not asked to revisit patients.
A random sample of patients surveyed for this initiative also received HCAHPS surveys 48 hours to 6 weeks following their hospital discharge, according to the standard methodology used to acquire HCAHPS data,[21] by an outside vendor contracted by Denver Health. Our vendor conducted these surveys via telephone in English or Spanish.
Outcomes
The primary outcome was the proportion of patients in each group who reported top box scores on the daily surveys. Secondary outcomes included the percent change for the scores recorded for 3 provider‐specific questions from the daily survey, the median top box HCAHPS scores for the 3 provider related questions and overall hospital rating, and the HCAHPS percentiles of top box scores for these questions.
Sample Size
The sample size for this intervention assumed that the proportion of patients whose treating physicians did not receive real‐time feedback who rated their providers as top box would be 75%, and that the effect of providing real‐time feedback would increase this proportion to 85% on the daily surveys. To have 80% power with a type 1 error of 0.05, we estimated a need to enroll 430 patients, 215 in each group.
Statistics
Data were collected and managed using a secure, Web‐based electronic data capture tool hosted at Denver Health (REDCap), which is designed to support data collection for research studies providing: (1) an intuitive interface for validated data entry, (2) audit trails for tracking data manipulation and export procedures, (3) automated export procedures for seamless data downloads to common statistical packages, and (4) procedures for importing data from external sources.[17]
A 2 test was used to compare the proportion of patients in the 2 groups who reported great scores for each question on the study survey on the first and last day. With the intent of providing a framework for understanding the effect real‐time feedback could have on patient experience, a secondary analysis of HCAHPS results was conducted using several different methods.
First, the proportion of patients in the 2 groups who reported scores of 9 or 10 for the overall hospital rating question or reported always for each doctor communication question on the HCHAPS survey was compared using a 2. Second, to allow for detection of differences in a sample with a smaller N, the median overall hospital rating scores from the HCAHPS survey reported by patients in the 2 groups who completed a survey following discharge were compared using a Wilcoxon rank sum test. Lastly, to place changes in proportion into a larger context (ie, how these changes would relate to value‐based purchasing), HCAHPS scores were converted to percentiles of national performance using the 2014 percentile rankings obtained from the external vendor that conducts the HCAHPS surveys for our hospital and compared between the intervention and control groups using a Wilcoxon rank sum test.
All comments collected from patients during their daily surveys were reviewed, and key words were abstracted from each comment. These key words were sorted and reviewed to categorize recurring key words into themes. Exemplars were then selected for each theme derived from patient comments.
RESULTS
From April 14, 2014 to September 19, 2014, we enrolled 227 patients in the control group and 228 in the intervention group (Figure 1). Patient demographics are summarized in Table 1. Of the 132 patients in the intervention group who reported anything less than top box scores for any of the 3 questions (thus prompting a revisit by their provider), 106 (80%) were revisited by their provider at least once during their hospitalization.
All Patients | HCAHPS Patients | |||
---|---|---|---|---|
Control, N = 227 | Intervention, N = 228 | Control, N = 35 | Intervention, N = 30 | |
| ||||
Age, mean SD | 55 14 | 55 15 | 55 15 | 57 16 |
Gender | ||||
Male | 126 (60) | 121 (55) | 20 (57) | 12 (40) |
Female | 85 (40) | 98 (45) | 15(43) | 18 (60) |
Race/ethnicity | ||||
Hispanic | 84 (40) | 90 (41) | 17 (49) | 12 (40) |
Black | 38 (18) | 28 (13) | 6 (17) | 7 (23) |
White | 87 (41) | 97 (44) | 12 (34) | 10 (33) |
Other | 2 (1) | 4 (2) | 0 (0) | 1 (3) |
Payer | ||||
Medicare | 65 (29) | 82 (36) | 15 (43) | 12 (40) |
Medicaid | 122 (54) | 108 (47) | 17 (49) | 14 (47) |
Commercial | 12 (5) | 15 (7) | 1 (3) | 1 (3) |
Medically indigent | 4 (2) | 7 (3) | 0 (0) | 3 (10) |
Self‐pay | 5 (2) | 4 (2) | 1 (3) | 0 (0) |
Other/unknown | 19 (8) | 12 (5) | 0 (0) | 0 (0) |
Team | ||||
Teaching | 187 (82) | 196 (86) | 27 (77) | 24 (80) |
Nonteaching | 40 (18) | 32 (14) | 8 (23) | 6 (20) |
Top 5 primary discharge diagnoses* | ||||
Septicemia | 26 (11) | 34 (15) | 3 (9) | 5 (17) |
Heart failure | 14 (6) | 13 (6) | 2 (6) | |
Acute pancreatitis | 12 (5) | 9 (4) | 3 (9) | 2 (7) |
Diabetes mellitus | 11 (5) | 8 (4) | 2 (6) | |
Alcohol withdrawal | 9 (4) | |||
Cellulitis | 7 (3) | 2 (7) | ||
Pulmonary embolism | 2 (7) | |||
Chest pain | 2 (7) | |||
Atrial fibrillation | 2 (6) | |||
Length of stay, median (IQR) | 3 (2, 5) | 3 (2, 5) | 3 (2, 5) | 3 (2, 4) |
Charlson Comorbidity Index, median (IQR) | 1 (0, 3) | 2 (0, 3) | 1 (0, 3) | 1.5 (1, 3) |
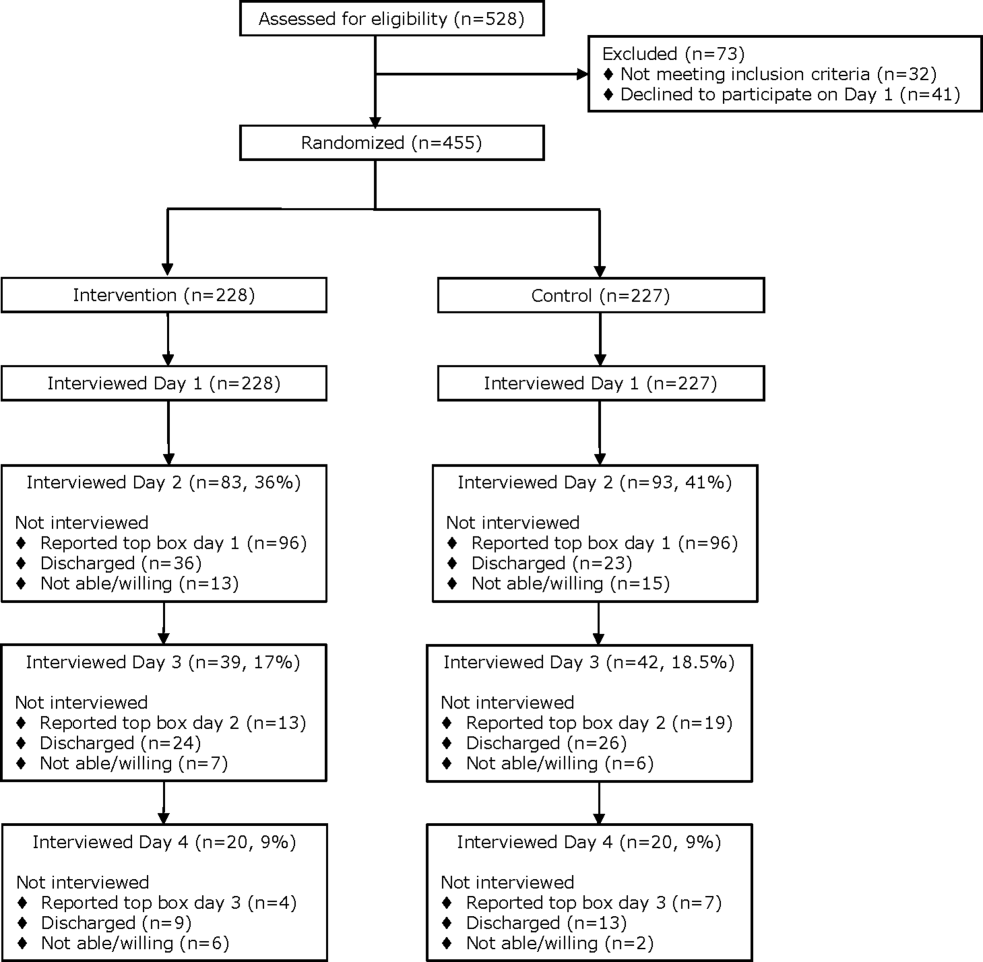
Daily Surveys
The proportion of patients in both study groups reporting top box scores tended to increase from the first day to the last day of the survey (Figure 2); however, we found no statistically significant differences between the proportion of patients who reported top box scores on first day or last day in the intervention group compared to the control group. The comments made by the patients are summarized in Supporting Table 1 in the online version of this article.
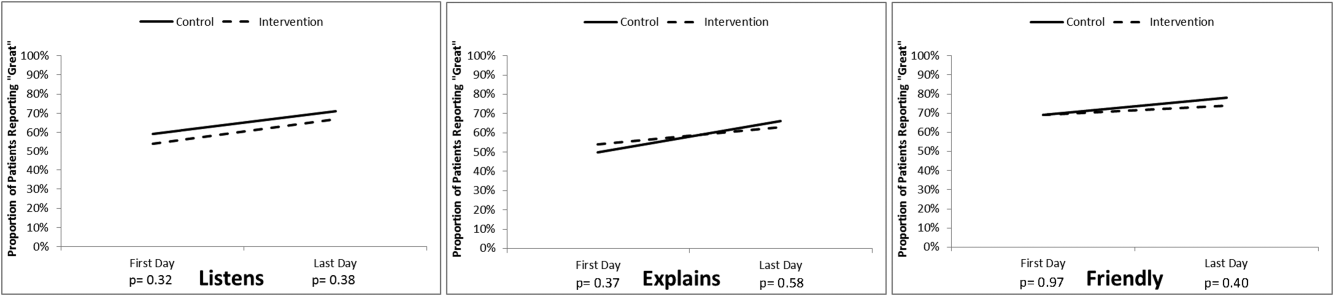
HCAHPS Scores
The proportion of top box scores from the HCAHPS surveys were higher, though not statistically significant, for all 3 provider‐specific questions and for the overall hospital rating for patients whose hospitalists received real‐time feedback (Table 2). The median [interquartile range] score for the overall hospital rating was higher for patients in the intervention group compared with those in the control group, (10 [9, 10] vs 9 [8, 10], P = 0.04]. After converting the HCAHPS scores to percentiles, we found considerably higher rankings for all 3 provider‐related questions and for the overall hospital rating in the intervention group compared to the control group (P = 0.02 for overall differences in percentiles [Table 2]).
HCAHPS Questions | Proportion Top Box* | Percentile Rank | ||
---|---|---|---|---|
Control, N = 35 | Intervention, N = 30 | Control, N = 35 | Intervention, N = 30 | |
| ||||
Overall hospital rating | 61% | 80% | 6 | 87 |
Courtesy/respect | 86% | 93% | 23 | 88 |
Clear communication | 77% | 80% | 39 | 60 |
Listening | 83% | 90% | 57 | 95 |
No adverse events occurred during the course of the study in either group.
DISCUSSION
The important findings of this study were that (1) daily patient satisfaction scores improved from first day to last day regardless of study group, (2) patients whose providers received real‐time feedback had a trend toward higher HCAHPS proportions for the 3 provider‐related questions as well as the overall rating of the hospital but were not statistically significant, (3) the percentile differences in these 3 questions as well as the overall rating of the hospital were significantly higher in the intervention group as was the median score for the overall hospital rating.
Our original sample size calculation was based upon our own preliminary data, indicating that our baseline top box scores for the daily survey was around 75%. The daily survey top box score on the first day was, however, much lower (Figure 2). Accordingly, although we did not find a significant difference in these daily scores, we were underpowered to find such a difference. Additionally, because only a small percentage of patients are selected for the HCAHPS survey, our ability to detect a difference in this secondary outcome was also limited. We felt that it was important to analyze the percentile comparisons in addition to the proportion of top box scores on the HCAHPS, because the metrics for value‐based purchasing are based upon, in part, how a hospital system compares to other systems. Finally, to improve our power to detect a difference given a small sample size, we converted the scoring system for overall hospital ranking to a continuous variable, which again was noted to be significant.
To our knowledge, this is the first randomized investigation designed to assess the effect of real‐time, patient‐specific feedback to physicians. Real‐time feedback is increasingly being incorporated into medical practice, but there is only limited information available describing how this type of feedback affects outcomes.[22, 23, 24] Banka et al.[15] found that HCAHPS scores improved as a result of real‐time feedback given to residents, but the study was not randomized, utilized a pre‐post design that resulted in there being differences between the patients studied before and after the intervention, and did not provide patient‐specific data to the residents. Tabib et al.[25] found that operating costs decreased 17% after instituting real‐time feedback to providers about these costs. Reeves et al.[26] conducted a cluster randomized trial of a patient feedback survey that was designed to improve nursing care, but the results were reviewed by the nurses several months after patients had been discharged.
The differences in median top box scores and percentile rank that we observed could have resulted from the real‐time feedback, the educational coaching, the fact that the providers revisited the majority of the patients, or a combination of all of the above. Gross et al.[27] found that longer visits lead to higher satisfaction, though others have not found this to necessarily be the case.[28, 29] Lin et al.[30] found that patient satisfaction was affected by the perceived duration of the visit as well as whether expectations on visit length were met and/or exceeded. Brown et al.[31] found that training providers in communication skills improved the providers perception of their communication skills, although patient experience scores did not improve. We feel that the results seen are more likely a combination thereof as opposed to any 1 component of the intervention.
The most commonly reported complaints or concerns in patients' undirected comments often related to communication issues. Comments on subsequent surveys suggested that patient satisfaction improved over time in the intervention group, indicating that perhaps physicians did try to improve in areas that were highlighted by the real‐time feedback, and that patients perceived the physician efforts to do so (eg, They're doing better than the last time you asked. They sat down and talked to me and listened better. They came back and explained to me about my care. They listened better. They should do this survey at the clinic. See Supporting Table 1 in the online version of this article).
Our study has several limitations. First, we did not randomize providers, and many of our providers (approximately 65%) participated in both the control group and also in the intervention group, and thus received real‐time feedback at some point during the study, which could have affected their overall practice and limited our ability to find a difference between the 2 groups. In an attempt to control for this possibility, the study was conducted on an intermittent basis during the study time frame. Furthermore, the proportion of patients who reported top box scores at the beginning of the study did not have a clear trend of change by the end of the study, suggesting that overall clinician practices with respect to patient satisfaction did not change during this short time period.
Second, only a small number of our patients were randomly selected for the HCAHPS survey, which limited our ability to detect significant differences in HCAHPS proportions. Third, the HCAHPS percentiles at our institution at that time were low. Accordingly, the improvements that we observed in patient satisfaction scores might not be reproducible at institutions with higher satisfactions scores. Fourth, time and resources were needed to obtain patient feedback to provide to providers during this study. There are, however, other ways to obtain feedback that are less resource intensive (eg, electronic feedback, the utilization of volunteers, or partnering this with manager rounding). Finally, the study was conducted at a single, university‐affiliated public teaching hospital and was a quality‐improvement initiative, and thus our results are not generalizable to other institutions.
In conclusion, real‐time feedback of patient experience to their providers, coupled with provider education, coaching, and revisits, seems to improve satisfaction of patients hospitalized on general internal medicine units who were cared for by hospitalists.
Acknowledgements
The authors thank Kate Fagan, MPH, for her excellent technical assistance.
Disclosure: Nothing to report.
- HCAHPS Fact Sheet. 2015. Available at: http://www.hcahpsonline.org/Files/HCAHPS_Fact_Sheet_June_2015.pdf. Accessed August 25, 2015.
- The relationship between commercial website ratings and traditional hospital performance measures in the USA. BMJ Qual Saf. 2013;22:194–202. , , , .
- Patients' perception of hospital care in the United States. N Engl J Med. 2008;359:1921–1931. , , , .
- The relationship between patients' perception of care and measures of hospital quality and safety. Health Serv Res. 2010;45:1024–1040. , , , .
- Relationship between quality of diabetes care and patient satisfaction. J Natl Med Assoc. 2003;95:64–70. , , , et al.
- Relationship between patient satisfaction with inpatient care and hospital readmission within 30 days. Am J Manag Care. 2011;17:41–48. , , , , .
- A systematic review of evidence on the links between patient experience and clinical safety and effectiveness. BMJ Open. 2013;3(1). , , .
- The association between satisfaction with services provided in primary care and outcomes in type 2 diabetes mellitus. Diabet Med. 2003;20:486–490. , .
- Associations between Web‐based patient ratings and objective measures of hospital quality. Arch Intern Med. 2012;172:435–436. , , , et al.
- Patient satisfaction and its relationship with clinical quality and inpatient mortality in acute myocardial infarction. Circ Cardiovasc Qual Outcomes. 2010;3:188–195. , , , et al.
- Patients' perceptions of care are associated with quality of hospital care: a survey of 4605 hospitals. Am J Med Qual. 2015;30(4):382–388. , , , , .
- Centers for Medicare 28:908–913.
- Effect of sitting vs. standing on perception of provider time at bedside: a pilot study. Patient Educ Couns. 2012;86:166–171. , , , , , .
- Improving patient satisfaction through physician education, feedback, and incentives. J Hosp Med. 2015;10:497–502. , , , et al.
- US Department of Health and Human Services. Patient satisfaction survey. Available at: http://bphc.hrsa.gov/policiesregulations/performancemeasures/patientsurvey/surveyform.html. Accessed November 15, 2013.
- Research electronic data capture (REDCap)—a metadata‐driven methodology and workflow process for providing translational research informatics support. J Biomed Inform. 2009;42:377–381. , , , , , .
- The HCAHPS Handbook. Gulf Breeze, FL: Fire Starter; 2010. .
- Etiquette‐based medicine. N Engl J Med. 2008;358:1988–1989. .
- 5 years after the Kahn's etiquette‐based medicine: a brief checklist proposal for a functional second meeting with the patient. Front Psychol. 2013;4:723. .
- Frequently Asked Questions. Hospital Value‐Based Purchasing Program. Available at: http://www.cms.gov/Medicare/Quality‐Initiatives‐Patient‐Assessment‐Instruments/hospital‐value‐based‐purchasing/Downloads/FY‐2013‐Program‐Frequently‐Asked‐Questions‐about‐Hospital‐VBP‐3‐9‐12.pdf. Accessed February 8, 2014.
- Real‐time patient survey data during routine clinical activities for rapid‐cycle quality improvement. JMIR Med Inform. 2015;3:e13. , , , .
- Mount Sinai launches real‐time patient‐feedback survey tool. Healthcare Informatics website. Available at: http://www.healthcare‐informatics.com/news‐item/mount‐sinai‐launches‐real‐time‐patient‐feedback‐survey‐tool. Accessed August 25, 2015. .
- Hospitals are finally starting to put real‐time data to use. Harvard Business Review website. Available at: https://hbr.org/2014/11/hospitals‐are‐finally‐starting‐to‐put‐real‐time‐data‐to‐use. Published November 12, 2014. Accessed August 25, 2015. , .
- Reducing operating room costs through real‐time cost information feedback: a pilot study. J Endourol. 2015;29:963–968. , , , , .
- Facilitated patient experience feedback can improve nursing care: a pilot study for a phase III cluster randomised controlled trial. BMC Health Serv Res. 2013;13:259. , , .
- Patient satisfaction with time spent with their physician. J Fam Pract. 1998;47:133–137. , , , , .
- The relationship between time spent communicating and communication outcomes on a hospital medicine service. J Gen Intern Med. 2012;27:185–189. , , , , , .
- Cognitive interview techniques reveal specific behaviors and issues that could affect patient satisfaction relative to hospitalists. J Hosp Med. 2009;4:E1–E6. , .
- Is patients' perception of time spent with the physician a determinant of ambulatory patient satisfaction? Arch Intern Med. 2001;161:1437–1442. , , , et al.
- Effect of clinician communication skills training on patient satisfaction. A randomized, controlled trial. Ann Intern Med. 1999;131:822–829. , , , .
- HCAHPS Fact Sheet. 2015. Available at: http://www.hcahpsonline.org/Files/HCAHPS_Fact_Sheet_June_2015.pdf. Accessed August 25, 2015.
- The relationship between commercial website ratings and traditional hospital performance measures in the USA. BMJ Qual Saf. 2013;22:194–202. , , , .
- Patients' perception of hospital care in the United States. N Engl J Med. 2008;359:1921–1931. , , , .
- The relationship between patients' perception of care and measures of hospital quality and safety. Health Serv Res. 2010;45:1024–1040. , , , .
- Relationship between quality of diabetes care and patient satisfaction. J Natl Med Assoc. 2003;95:64–70. , , , et al.
- Relationship between patient satisfaction with inpatient care and hospital readmission within 30 days. Am J Manag Care. 2011;17:41–48. , , , , .
- A systematic review of evidence on the links between patient experience and clinical safety and effectiveness. BMJ Open. 2013;3(1). , , .
- The association between satisfaction with services provided in primary care and outcomes in type 2 diabetes mellitus. Diabet Med. 2003;20:486–490. , .
- Associations between Web‐based patient ratings and objective measures of hospital quality. Arch Intern Med. 2012;172:435–436. , , , et al.
- Patient satisfaction and its relationship with clinical quality and inpatient mortality in acute myocardial infarction. Circ Cardiovasc Qual Outcomes. 2010;3:188–195. , , , et al.
- Patients' perceptions of care are associated with quality of hospital care: a survey of 4605 hospitals. Am J Med Qual. 2015;30(4):382–388. , , , , .
- Centers for Medicare 28:908–913.
- Effect of sitting vs. standing on perception of provider time at bedside: a pilot study. Patient Educ Couns. 2012;86:166–171. , , , , , .
- Improving patient satisfaction through physician education, feedback, and incentives. J Hosp Med. 2015;10:497–502. , , , et al.
- US Department of Health and Human Services. Patient satisfaction survey. Available at: http://bphc.hrsa.gov/policiesregulations/performancemeasures/patientsurvey/surveyform.html. Accessed November 15, 2013.
- Research electronic data capture (REDCap)—a metadata‐driven methodology and workflow process for providing translational research informatics support. J Biomed Inform. 2009;42:377–381. , , , , , .
- The HCAHPS Handbook. Gulf Breeze, FL: Fire Starter; 2010. .
- Etiquette‐based medicine. N Engl J Med. 2008;358:1988–1989. .
- 5 years after the Kahn's etiquette‐based medicine: a brief checklist proposal for a functional second meeting with the patient. Front Psychol. 2013;4:723. .
- Frequently Asked Questions. Hospital Value‐Based Purchasing Program. Available at: http://www.cms.gov/Medicare/Quality‐Initiatives‐Patient‐Assessment‐Instruments/hospital‐value‐based‐purchasing/Downloads/FY‐2013‐Program‐Frequently‐Asked‐Questions‐about‐Hospital‐VBP‐3‐9‐12.pdf. Accessed February 8, 2014.
- Real‐time patient survey data during routine clinical activities for rapid‐cycle quality improvement. JMIR Med Inform. 2015;3:e13. , , , .
- Mount Sinai launches real‐time patient‐feedback survey tool. Healthcare Informatics website. Available at: http://www.healthcare‐informatics.com/news‐item/mount‐sinai‐launches‐real‐time‐patient‐feedback‐survey‐tool. Accessed August 25, 2015. .
- Hospitals are finally starting to put real‐time data to use. Harvard Business Review website. Available at: https://hbr.org/2014/11/hospitals‐are‐finally‐starting‐to‐put‐real‐time‐data‐to‐use. Published November 12, 2014. Accessed August 25, 2015. , .
- Reducing operating room costs through real‐time cost information feedback: a pilot study. J Endourol. 2015;29:963–968. , , , , .
- Facilitated patient experience feedback can improve nursing care: a pilot study for a phase III cluster randomised controlled trial. BMC Health Serv Res. 2013;13:259. , , .
- Patient satisfaction with time spent with their physician. J Fam Pract. 1998;47:133–137. , , , , .
- The relationship between time spent communicating and communication outcomes on a hospital medicine service. J Gen Intern Med. 2012;27:185–189. , , , , , .
- Cognitive interview techniques reveal specific behaviors and issues that could affect patient satisfaction relative to hospitalists. J Hosp Med. 2009;4:E1–E6. , .
- Is patients' perception of time spent with the physician a determinant of ambulatory patient satisfaction? Arch Intern Med. 2001;161:1437–1442. , , , et al.
- Effect of clinician communication skills training on patient satisfaction. A randomized, controlled trial. Ann Intern Med. 1999;131:822–829. , , , .
© 2016 Society of Hospital Medicine
Gender Disparities for Academic Hospitalists
Gender disparities still exist for women in academic medicine.[1, 2, 3, 4, 5, 6, 7, 8, 9] The most recent data from the Association of American Medical Colleges (AAMC) show that although gender disparities are decreasing, women are still under‐represented in the assistant, associate, and full‐professor ranks as well as in leadership positions.[1]
Some studies indicate that gender differences are less evident when examining younger cohorts.[1, 10, 11, 12, 13] Hospital medicine emerged around 1996, when the term hospitalist was first coined.[14] The gender distribution of academic hospitalists is likely nearly equal,[15, 16] and they are generally younger physicians.[15, 17, 18, 19, 20] Accordingly, we questioned whether gender disparities existed in academic hospital medicine (HM) and, if so, whether these disparities were greater than those that might exist in academic general internal medicine (GIM).
METHODS
This study consisted of both prospective and retrospective observation of data collected for academic adult hospitalists and general internists who practice in the United States. It was approved by the Colorado Multiple Institutional Review Board.
Gender distribution was assessed with respect to: (1) academic HM and GIM faculty, (2) leadership (ie, division or section heads), and (3) scholarly work (ie, speaking opportunities and publications). Data were collected between October 1, 2012 and August 31, 2014.
Gender Distribution of Faculty and Division/Section Heads
All US internal medicine residency programs were identified from the list of members or affiliates of the AAMC that were fully accredited by the Liaison Committee on Medical Education[21] using the Graduate Medical Education Directory.[22] We then determined the primary training hospital(s) affiliated with each program and selected those that were considered to be university hospitals and eliminated those that did not have divisions or sections of HM or GIM. We determined the gender of the respective division/section heads on the basis of the faculty member's first name (and often from accompanying photos) as well as from information obtained via Internet searches and, if necessary, contacted the individual institutions via email or phone call(s). We also determined the number and gender of all of the HM and GIM faculty members in a random sample of 25% of these hospitals from information on their respective websites.
Gender Distribution for Scholarly Productivity
We determined the gender and specialty of all speakers at the Society of Hospital Medicine and the Society of General Internal Medicine national conferences from 2006 to 2012. A list of speakers at each conference was obtained from conference pamphlets or agendas that were available via Internet searches or obtained directly from the organization. We also determined whether each presenter was a featured speaker (defined as one whose talk was unopposed by other sessions), plenary speaker (defined as such in the conference pamphlets), or if they spoke in a group format (also as indicated in the conference pamphlets). Because of the low number of featured and plenary speakers, these data were combined. Faculty labeled as additional faculty when presenting in a group format were excluded as were speakers at precourses, those presenting abstracts, and those participating in interest group sessions.
For authorship, a PubMed search was used to identify all articles published in the Journal of Hospital Medicine (JHM) and the Journal of General Internal Medicine (JGIM) from January 1, 2006 through December 31, 2012, and the gender and specialty of all the first and last authors were determined as described above. Specialty was determined from the division, section or department affiliation indicated for each author and by Internet searches. In some instances, it was necessary to contact the authors or their departments directly to verify their specialty. When articles had only 1 author, the author was considered a first author.
Duplicate records (eg, same author, same journal) and articles without an author were excluded, as were authors who did not have an MD, DO, or MBBS degree and those who were not affiliated with an institution in the United States. All manuscripts, with the exception of errata, were analyzed together as well as in 3 subgroups: original research, editorials, and others.
A second investigator corroborated data regarding gender and specialty for all speakers and authors to strengthen data integrity. On the rare occasion when discrepancies were found, a third investigator adjudicated the results.
Definitions
Physicians were defined as being hospitalists if they were listed as a member of a division or section of HM on their publications or if Internet searches indicated that they were a hospitalist or primarily worked on inpatient medical services. Physicians were considered to be general internists if they were listed as such on their publications and their specialty could be verified in Web‐based searches. If physicians appeared to have changing roles over time, we attempted to assign their specialty based upon their role at the time the article was published or the presentation was delivered. If necessary, phone calls and/or emails were also done to determine the physician's specialty.
Analysis
REDCap, a secure, Web‐based application for building and managing online surveys and databases, was used to collect and manage all study data.[23] All analyses were performed using SAS Enterprise Guide 4.3 (SAS Institute, Inc., Cary, NC). A [2] test was used to compare proportions of male versus female physicians, and data from hospitalists versus general internists. Because we performed multiple comparisons when analyzing presentations and publications, a Bonferroni adjustment was made such that a P<0.0125 for presentations and P<0.006 (within specialty) or P<0.0125 (between specialty) for the publication analyses were considered significant. P<0.05 was considered significant for all other comparisons.
RESULTS
Gender Distribution of Faculty
Eighteen HM and 20 GIM programs from university hospitals were randomly selected for review (see Supporting Figure 1 in the online version of this article). Seven of the HM programs and 1 of the GIM programs did not have a website, did not differentiate hospitalists from other faculty, or did not list their faculty on the website and were excluded from the analysis. In the remaining 11 HM programs and 19 GIM programs, women made up 277/568 (49%) and 555/1099 (51%) of the faculty, respectively (P=0.50).
Gender Distribution of Division/Section Heads
Eighty‐six of the programs were classified as university hospitals (see Supporting Figure 1 in the online version of this article), and in these, women led 11/69 (16%) of the HM divisions or sections and 28/80 (35%) of the GIM divisions (P=0.008).
Gender Distribution for Scholarly Productivity
Speaking Opportunities
A total of 1227 presentations were given at the 2 conferences from 2006 to 2012, with 1343 of the speakers meeting inclusion criteria (see Supporting Figure 2 in the online version of this article). Hospitalists accounted for 557 of the speakers, of which 146 (26%) were women. General internists accounted for 580 of the speakers, of which 291 (50%) were women (P<0.0001) (Table 1).
Male, N (%) | Female, N (%) | |
---|---|---|
| ||
Hospitalists | ||
All presentations | 411 (74) | 146 (26)* |
Featured or plenary presentations | 49 (91) | 5 (9)* |
General internists | ||
All presentations | 289 (50) | 291 (50) |
Featured or plenary presentations | 27 (55) | 22 (45) |
Of the 117 featured or plenary speakers, 54 were hospitalists and 5 (9%) of these were women. Of the 49 who were general internists, 22 (45%) were women (P<0.0001).
Authorship
The PubMed search identified a total of 3285 articles published in the JHM and the JGIM from 2006 to 2012, and 2172 first authors and 1869 last authors met inclusion criteria (see Supporting Figure 3 in the online version of this article). Hospitalists were listed as first or last authors on 464 and 305 articles, respectively, and of these, women were first authors on 153 (33%) and last authors on 63 (21%). General internists were listed as first or last authors on 895 and 769 articles, respectively, with women as first authors on 423 (47%) and last authors on 265 (34%). Compared with general internists, fewer women hospitalists were listed as either first or last authors (both P<0.0001) (Table 2).
First Author | Last Author | |||
---|---|---|---|---|
Male, N (%) | Female, N (%) | Male, N (%) | Female, N (%) | |
| ||||
Hospitalists | ||||
All publications | 311 (67) | 153 (33)* | 242 (79) | 63 (21)* |
Original investigations/brief reports | 124 (61) | 79 (39)* | 96 (76) | 30 (24)* |
Editorials | 34 (77) | 10 (23)* | 18 (86) | 3 (14)* |
Other | 153 (71) | 64 (29)* | 128 (81) | 30 (19)* |
General internists | ||||
All publications | 472 (53) | 423 (47) | 504 (66) | 265 (34)* |
Original investigations/brief reports | 218 (46) | 261 (54) | 310 (65) | 170 (35)* |
Editorial | 98 (68) | 46 (32)* | 43 (73) | 16 (27)* |
Other | 156 (57) | 116 (43) | 151 (66) | 79 (34)* |
Fewer women hospitalists were listed as first or last authors on all article types. For original research articles written by general internists, there was a trend for more women to be listed as first authors than men (261/479, 54%), but this difference was not statistically significant.
DISCUSSION
The important findings of this study are that, despite an equal gender distribution of academic HM and GIM faculty, fewer women were HM division/section chiefs, fewer women were speakers at the 2 selected national meetings, and fewer women were first or last authors of publications in 2 selected journals in comparison with general internists.
Previous studies have found that women lag behind their male counterparts with respect to academic productivity, leadership, and promotion.[1, 5, 7] Some studies suggest, however, that gender differences are reduced when younger cohorts are examined.[1, 10, 11, 12, 13] Surveys indicate that that the mean age of hospitalists is younger than most other specialties.[15, 19, 20, 24] The mean age of academic GIM physicians is unknown, but surveys of GIM (not differentiating academic from nonacademic) suggest that it is an older cohort than that of HM.[24] Despite hospitalists being a younger cohort, we found gender disparities in all areas investigated.
Our findings with respect to gender disparities in HM division or section leadership are consistent with the annual AAMC Women in US Academic Medicine and Science Benchmarking Report that found only 22% of all permanent division or section heads were women.[1]
Gender disparities with respect to authorship of medical publications have been previously noted,[3, 6, 15, 25] but to our knowledge, this is the first study to investigate the gender of authors who were hospitalists. Although we found a higher proportion of women hospitalists who were first or last authors than was observed by Jagsi and colleagues,[3] women hospitalists were still under‐represented with respect to this measure of academic productivity. Erren et al. reviewed 6 major journals from 2010 and 2011, and found that first authorship of original research by women ranged from 23.7% to 46.7%, and for last authorship from 18.3% to 28.8%.[25] Interestingly, we found no significant gender difference for first authors who were general internists, and there was a trend toward more women general internists being first authors than men for original research, reviews, and brief reports (data not shown).
Our study did not attempt to answer the question of why gender disparities persist, but many previous studies have explored this issue.[4, 8, 12, 26, 27, 28, 29, 30, 31, 32, 33, 34, 35, 36, 37, 38, 39, 40, 41, 42] Issues raised by others include the quantity of academic work (ie, publications and grants obtained), differences in hours worked and allocation of time, lack of mentorship, family responsibilities, discrimination, differences in career motivation, and levels of institutional support, to name a few.
The under‐representation of women hospitalists in leadership, authorship, and speaking opportunities may be consistent with gender‐related differences in research productivity. Fewer publications could lead to fewer national presentations, which could lead to fewer leadership opportunities. Our findings with respect to general internists are not consistent with this idea, however, as whereas women were under‐represented in GIM leadership positions, we found no disparities with respect to the gender of first authors or speakers at national meetings for general internists. The finding that hospitalists had gender disparities with respect to first authors and national speakers but general internists did not, argues against several hypotheses (ie, that women lack mentorship, have less career motivation, fewer career building opportunities).
One notable hypothesis, and perhaps one that is often discussed in the literature, is that women shoulder the majority of family responsibilities, and this may result in women having less time for their careers. Jolly and colleagues studied physician‐researchers and noted that women were more likely than men to have spouses or domestic partners who were fully employed, spent 8.5 more hours per week on domestic activities, and were more likely to take time off during disruptions of usual child care.[33] Carr and colleagues found that women with children (compared to men with children) had fewer publications, slower self‐perceived career progress, and lower career satisfaction, but having children had little effect on faculty aspirations and goals.[2] Kaplan et al., however, found that family responsibilities do not appear to account for sex differences in academic advancement.[4] Interestingly, in a study comparing physicians from Generation X to those of the Baby Boomer age, Generation X women reported working more than their male Generation X counterparts, and both had more of a focus on worklife balance than the older generation.[12]
The nature the of 2 specialties' work environment and job requirements could have also resulted in some of the differences seen. Primary care clinical work is typically conducted Monday through Friday, and hospitalist work frequently includes some weekend, evening, night, and holiday coverage. Although these are known differences, both specialties have also been noted to offer many advantages to women and men alike, including collaborative working environments and flexible work hours.[16]
Finally, finding disparity in leadership positions in both specialties supports the possibility that those responsible for hiring could have implicit gender biases. Under‐representation in entry‐level positions is also not a likely explanation for the differences we observed, because nearly an equal number of men and women graduate from medical school, pursue residency training in internal medicine, and become either academic hospitalists or general internists at university settings.[1, 15, 24] This hypothesis could, however, explain why disparities exist with respect to senior authorship and leadership positions, as typically, these individuals have been in practice longer and the current trends of improved gender equality have not always been the case.
Our study has a number of limitations. First, we only examined publications in 2 journals and presentations at 2 national conferences, although the journals and conferences selected are considered to be the major ones in the 2 specialties. Second, using Internet searches may have resulted in inaccurate gender and specialty assignment, but previous studies have used similar methodology.[3, 43] Additionally, we also attempted to contact individuals for direct confirmation when the information we obtained was not clear and had a second investigator independently verify the gender and specialty data. Third, we utilized division/department websites when available to determine the gender of HM divisions/sections. If not recently updated, these websites may not have reflected the most current leader of the unit, but this concern would seemingly pertain to both hospitalists and general internists. Fourth, we opted to only study faculty and division/section heads at university hospitals, as typically these institutions had GIM and hospitalist groups and also typically had websites. Because we only studied faculty and leadership at university hospitals, our data are not generalizable to all hospitalist and GIM groups. Finally, we excluded pediatric hospitalists, and thus, this study is representative of adult hospitalists only. Including pediatric hospitalists was out of the scope of this project.
Our study also had a number of strengths. To our knowledge, this is the first study to provide an estimate of the gender distribution in academic HM, of hospitalists as speakers at national meetings, as first and last authors, and of HM division or section heads, and is the first to compare these results with those observed for general internists. In addition, we examined 7 years of data from 2 of the major journals and national conferences for these specialties.
In summary, despite HM being a newer field with a younger cohort of physicians, we found that gender disparities exist for women with respect to authorship, national speaking opportunities, and division or section leadership. Identifying why these gender differences exist presents an important next step.
Disclosures: Nothing to report. Marisha Burden, MD and Maria G. Frank, MD are coprincipal authors.
- Association of American Medical Colleges. Women in U.S. academic medicine and science: Statistics and benchmarking report. 2012. Available at: https://members.aamc.org/eweb/upload/Women%20in%20U%20S%20%20Academic%20Medicine%20Statistics%20and%20Benchmarking%20Report%202011-20123.pdf. Accessed September 1, 2014.
- Relation of family responsibilities and gender to the productivity and career satisfaction of medical faculty. Ann Intern Med. 1998;129:532–538. , , , et al.
- The “gender gap” in authorship of academic medical literature—a 35‐year perspective. N Engl J Med. 2006;355:281–287. , , , et al.
- Sex differences in academic advancement. Results of a national study of pediatricians. N Engl J Med. 1996;335:1282–1289. , , , , , .
- Women physicians in academic medicine: new insights from cohort studies. N Engl J Med. 2000;342:399–405. .
- Gender differences in academic productivity and leadership appointments of physicians throughout academic careers. Acad Med. 2011;86:43–47. , , , , .
- Promotion of women physicians in academic medicine. Glass ceiling or sticky floor? JAMA. 1995;273:1022–1025. , , , .
- Compensation and advancement of women in academic medicine: is there equity? Ann Intern Med. 2004;141:205–212. , , , .
- Women physicians: choosing a career in academic medicine. Acad Med. 2012;87:105–114. , , .
- The status of women at one academic medical center. Breaking through the glass ceiling. JAMA. 1990;264:1813–1817. , , , .
- Status of women in academic anesthesiology. Anesthesiology. 1986;64:496–500. , .
- The generation and gender shifts in medicine: an exploratory survey of internal medicine physicians. BMC Health Serv Res. 2006;6:55. , , .
- Pew Research Center. On pay gap, millenial women near parity—for now. December 2013. Available at: http://www.pewsocialtrends.org/files/2013/12/gender-and-work_final.pdf. Published December 11, 2013. Accessed February 5, 2015.
- The emerging role of "hospitalists" in the American health care system. N Engl J Med. 1996;335:514–517. , .
- Mentorship, productivity, and promotion among academic hospitalists. J Gen Intern Med. 2012;27:23–27. , , , , , .
- The gender factor. The Hospitalist. Available at: http://www.the‐hospitalist.org/article/the‐gender‐factor. Published March 1, 2006. Accessed September 1, 2014. .
- Association of American Medical Colleges. Analysis in brief: Supplemental information for estimating the number and characteristics of hospitalist physicians in the United States and their possible workforce implications. Available at: https://www.aamc.org/download/300686/data/aibvol12_no3-supplemental.pdf. Published August 2012. Accessed September 1, 2014.
- Survey of US academic hospitalist leaders about mentorship and academic activities in hospitalist groups. J Hosp Med. 2011;6:5–9. , , , .
- State of Hospital Medicine: 2011 Report Based on 2010 Data. Medical Group Management Association and Society of Hospital Medicine. www.mgma.com, www.hospitalmedicine.org.
- Today's Hospitalist Survey. Compensation and Career Survey Results. 2013. Available at: http://www.todayshospitalist.com/index.php?b=salary_survey_results. Accessed January 11, 2015.
- Association of American Medical Colleges. Women in U.S. Academic Medicine: Statistics and Benchmarking Report. 2009–2010. Available at: https://www.aamc.org/download/182674/data/gwims_stats_2009‐2010.pdf. Accessed September 1, 2014.
- American Medical Association. Graduate Medical Education Directory 2012–2013. Chicago, IL: American Medical Association; 2012:182–203.
- Research electronic data capture (REDCap)—a metadata‐driven methodology and workflow process for providing translational research informatics support. J Biomed Inform. 2009;42:377–381. , , , , , .
- Association of American Medical Colleges. 2012 Physician Specialty Data Book. Center for Workforce Studies. Available at: https://www.aamc.org/download/313228/data/2012physicianspecialtydatabook.pdf. Published November 2012. Accessed September 1, 2014.
- Representation of women as authors, reviewers, editors in chief, and editorial board members at 6 general medical journals in 2010 and 2011. JAMA Intern Med. 2014;174:633–635. , , , .
- Relationships of gender and career motivation to medical faculty members' production of academic publications. Acad Med. 1998;73:180–186. , , , et al.
- Faculty perceptions of gender discrimination and sexual harassment in academic medicine. Ann Intern Med. 2000;132:889–896. , , , et al.
- Attitudes of clinical faculty about career progress, career success and recognition, and commitment to academic medicine. Results of a survey. Arch Intern Med. 2000;160:2625–2629. , , , .
- A "ton of feathers": gender discrimination in academic medical careers and how to manage it. J Womens Health (Larchmt). 2003;12:1009–1018. , , , , .
- Perceived obstacles to career success for women in academic surgery. Arch Surg. 2000;135:972–977. , , .
- Career satisfaction of US women physicians: results from the Women Physicians' Health Study. Society of General Internal Medicine Career Satisfaction Study Group. Arch Intern Med. 1999;159:1417–1426. , , , .
- Doing the same and earning less: male and female physicians in a new medical specialty. Inquiry. 2004;41:301–315. .
- Gender differences in time spent on parenting and domestic responsibilities by high‐achieving young physician‐researchers. Ann Intern Med. 2014;160:344–353. , , , , , .
- Stories from early‐career women physicians who have left academic medicine: a qualitative study at a single institution. Acad Med. 2011;86:752–758. , , , , .
- The $16,819 pay gap for newly trained physicians: the unexplained trend of men earning more than women. Health Aff (Millwood). 2011;30:193–201. , , , .
- Experiencing the culture of academic medicine: gender matters, a national study. J Gen Intern Med. 2013;28:201–207. , , , , .
- Gender pay gaps in hospital medicine. The Hospitalist. Available at: http://www.the‐hospitalist.org/article/gender‐pay‐gaps‐in‐hospital‐medicine. Published February 29, 2012. Accessed September 1, 2014. .
- Mentoring in academic medicine: a systematic review. JAMA. 2006;296:1103–1115. , , .
- Inequality quantified: mind the gender gap. Nature. 2013;495:22–24. .
- Gender differences in academic advancement: patterns, causes, and potential solutions in one US College of Medicine. Acad Med. 2003;78:500–508. , , , et al.
- Why aren't there more women leaders in academic medicine? The views of clinical department chairs. Acad Med. 2001;76:453–465. , .
- Gender factors in reviewer recommendations for manuscript publication. J Appl Behav Anal. 1990;23:539–543. .
- Scientific impact of women in academic surgery. J Surg Res. 2008;148:13–16. , , , .
Gender disparities still exist for women in academic medicine.[1, 2, 3, 4, 5, 6, 7, 8, 9] The most recent data from the Association of American Medical Colleges (AAMC) show that although gender disparities are decreasing, women are still under‐represented in the assistant, associate, and full‐professor ranks as well as in leadership positions.[1]
Some studies indicate that gender differences are less evident when examining younger cohorts.[1, 10, 11, 12, 13] Hospital medicine emerged around 1996, when the term hospitalist was first coined.[14] The gender distribution of academic hospitalists is likely nearly equal,[15, 16] and they are generally younger physicians.[15, 17, 18, 19, 20] Accordingly, we questioned whether gender disparities existed in academic hospital medicine (HM) and, if so, whether these disparities were greater than those that might exist in academic general internal medicine (GIM).
METHODS
This study consisted of both prospective and retrospective observation of data collected for academic adult hospitalists and general internists who practice in the United States. It was approved by the Colorado Multiple Institutional Review Board.
Gender distribution was assessed with respect to: (1) academic HM and GIM faculty, (2) leadership (ie, division or section heads), and (3) scholarly work (ie, speaking opportunities and publications). Data were collected between October 1, 2012 and August 31, 2014.
Gender Distribution of Faculty and Division/Section Heads
All US internal medicine residency programs were identified from the list of members or affiliates of the AAMC that were fully accredited by the Liaison Committee on Medical Education[21] using the Graduate Medical Education Directory.[22] We then determined the primary training hospital(s) affiliated with each program and selected those that were considered to be university hospitals and eliminated those that did not have divisions or sections of HM or GIM. We determined the gender of the respective division/section heads on the basis of the faculty member's first name (and often from accompanying photos) as well as from information obtained via Internet searches and, if necessary, contacted the individual institutions via email or phone call(s). We also determined the number and gender of all of the HM and GIM faculty members in a random sample of 25% of these hospitals from information on their respective websites.
Gender Distribution for Scholarly Productivity
We determined the gender and specialty of all speakers at the Society of Hospital Medicine and the Society of General Internal Medicine national conferences from 2006 to 2012. A list of speakers at each conference was obtained from conference pamphlets or agendas that were available via Internet searches or obtained directly from the organization. We also determined whether each presenter was a featured speaker (defined as one whose talk was unopposed by other sessions), plenary speaker (defined as such in the conference pamphlets), or if they spoke in a group format (also as indicated in the conference pamphlets). Because of the low number of featured and plenary speakers, these data were combined. Faculty labeled as additional faculty when presenting in a group format were excluded as were speakers at precourses, those presenting abstracts, and those participating in interest group sessions.
For authorship, a PubMed search was used to identify all articles published in the Journal of Hospital Medicine (JHM) and the Journal of General Internal Medicine (JGIM) from January 1, 2006 through December 31, 2012, and the gender and specialty of all the first and last authors were determined as described above. Specialty was determined from the division, section or department affiliation indicated for each author and by Internet searches. In some instances, it was necessary to contact the authors or their departments directly to verify their specialty. When articles had only 1 author, the author was considered a first author.
Duplicate records (eg, same author, same journal) and articles without an author were excluded, as were authors who did not have an MD, DO, or MBBS degree and those who were not affiliated with an institution in the United States. All manuscripts, with the exception of errata, were analyzed together as well as in 3 subgroups: original research, editorials, and others.
A second investigator corroborated data regarding gender and specialty for all speakers and authors to strengthen data integrity. On the rare occasion when discrepancies were found, a third investigator adjudicated the results.
Definitions
Physicians were defined as being hospitalists if they were listed as a member of a division or section of HM on their publications or if Internet searches indicated that they were a hospitalist or primarily worked on inpatient medical services. Physicians were considered to be general internists if they were listed as such on their publications and their specialty could be verified in Web‐based searches. If physicians appeared to have changing roles over time, we attempted to assign their specialty based upon their role at the time the article was published or the presentation was delivered. If necessary, phone calls and/or emails were also done to determine the physician's specialty.
Analysis
REDCap, a secure, Web‐based application for building and managing online surveys and databases, was used to collect and manage all study data.[23] All analyses were performed using SAS Enterprise Guide 4.3 (SAS Institute, Inc., Cary, NC). A [2] test was used to compare proportions of male versus female physicians, and data from hospitalists versus general internists. Because we performed multiple comparisons when analyzing presentations and publications, a Bonferroni adjustment was made such that a P<0.0125 for presentations and P<0.006 (within specialty) or P<0.0125 (between specialty) for the publication analyses were considered significant. P<0.05 was considered significant for all other comparisons.
RESULTS
Gender Distribution of Faculty
Eighteen HM and 20 GIM programs from university hospitals were randomly selected for review (see Supporting Figure 1 in the online version of this article). Seven of the HM programs and 1 of the GIM programs did not have a website, did not differentiate hospitalists from other faculty, or did not list their faculty on the website and were excluded from the analysis. In the remaining 11 HM programs and 19 GIM programs, women made up 277/568 (49%) and 555/1099 (51%) of the faculty, respectively (P=0.50).
Gender Distribution of Division/Section Heads
Eighty‐six of the programs were classified as university hospitals (see Supporting Figure 1 in the online version of this article), and in these, women led 11/69 (16%) of the HM divisions or sections and 28/80 (35%) of the GIM divisions (P=0.008).
Gender Distribution for Scholarly Productivity
Speaking Opportunities
A total of 1227 presentations were given at the 2 conferences from 2006 to 2012, with 1343 of the speakers meeting inclusion criteria (see Supporting Figure 2 in the online version of this article). Hospitalists accounted for 557 of the speakers, of which 146 (26%) were women. General internists accounted for 580 of the speakers, of which 291 (50%) were women (P<0.0001) (Table 1).
Male, N (%) | Female, N (%) | |
---|---|---|
| ||
Hospitalists | ||
All presentations | 411 (74) | 146 (26)* |
Featured or plenary presentations | 49 (91) | 5 (9)* |
General internists | ||
All presentations | 289 (50) | 291 (50) |
Featured or plenary presentations | 27 (55) | 22 (45) |
Of the 117 featured or plenary speakers, 54 were hospitalists and 5 (9%) of these were women. Of the 49 who were general internists, 22 (45%) were women (P<0.0001).
Authorship
The PubMed search identified a total of 3285 articles published in the JHM and the JGIM from 2006 to 2012, and 2172 first authors and 1869 last authors met inclusion criteria (see Supporting Figure 3 in the online version of this article). Hospitalists were listed as first or last authors on 464 and 305 articles, respectively, and of these, women were first authors on 153 (33%) and last authors on 63 (21%). General internists were listed as first or last authors on 895 and 769 articles, respectively, with women as first authors on 423 (47%) and last authors on 265 (34%). Compared with general internists, fewer women hospitalists were listed as either first or last authors (both P<0.0001) (Table 2).
First Author | Last Author | |||
---|---|---|---|---|
Male, N (%) | Female, N (%) | Male, N (%) | Female, N (%) | |
| ||||
Hospitalists | ||||
All publications | 311 (67) | 153 (33)* | 242 (79) | 63 (21)* |
Original investigations/brief reports | 124 (61) | 79 (39)* | 96 (76) | 30 (24)* |
Editorials | 34 (77) | 10 (23)* | 18 (86) | 3 (14)* |
Other | 153 (71) | 64 (29)* | 128 (81) | 30 (19)* |
General internists | ||||
All publications | 472 (53) | 423 (47) | 504 (66) | 265 (34)* |
Original investigations/brief reports | 218 (46) | 261 (54) | 310 (65) | 170 (35)* |
Editorial | 98 (68) | 46 (32)* | 43 (73) | 16 (27)* |
Other | 156 (57) | 116 (43) | 151 (66) | 79 (34)* |
Fewer women hospitalists were listed as first or last authors on all article types. For original research articles written by general internists, there was a trend for more women to be listed as first authors than men (261/479, 54%), but this difference was not statistically significant.
DISCUSSION
The important findings of this study are that, despite an equal gender distribution of academic HM and GIM faculty, fewer women were HM division/section chiefs, fewer women were speakers at the 2 selected national meetings, and fewer women were first or last authors of publications in 2 selected journals in comparison with general internists.
Previous studies have found that women lag behind their male counterparts with respect to academic productivity, leadership, and promotion.[1, 5, 7] Some studies suggest, however, that gender differences are reduced when younger cohorts are examined.[1, 10, 11, 12, 13] Surveys indicate that that the mean age of hospitalists is younger than most other specialties.[15, 19, 20, 24] The mean age of academic GIM physicians is unknown, but surveys of GIM (not differentiating academic from nonacademic) suggest that it is an older cohort than that of HM.[24] Despite hospitalists being a younger cohort, we found gender disparities in all areas investigated.
Our findings with respect to gender disparities in HM division or section leadership are consistent with the annual AAMC Women in US Academic Medicine and Science Benchmarking Report that found only 22% of all permanent division or section heads were women.[1]
Gender disparities with respect to authorship of medical publications have been previously noted,[3, 6, 15, 25] but to our knowledge, this is the first study to investigate the gender of authors who were hospitalists. Although we found a higher proportion of women hospitalists who were first or last authors than was observed by Jagsi and colleagues,[3] women hospitalists were still under‐represented with respect to this measure of academic productivity. Erren et al. reviewed 6 major journals from 2010 and 2011, and found that first authorship of original research by women ranged from 23.7% to 46.7%, and for last authorship from 18.3% to 28.8%.[25] Interestingly, we found no significant gender difference for first authors who were general internists, and there was a trend toward more women general internists being first authors than men for original research, reviews, and brief reports (data not shown).
Our study did not attempt to answer the question of why gender disparities persist, but many previous studies have explored this issue.[4, 8, 12, 26, 27, 28, 29, 30, 31, 32, 33, 34, 35, 36, 37, 38, 39, 40, 41, 42] Issues raised by others include the quantity of academic work (ie, publications and grants obtained), differences in hours worked and allocation of time, lack of mentorship, family responsibilities, discrimination, differences in career motivation, and levels of institutional support, to name a few.
The under‐representation of women hospitalists in leadership, authorship, and speaking opportunities may be consistent with gender‐related differences in research productivity. Fewer publications could lead to fewer national presentations, which could lead to fewer leadership opportunities. Our findings with respect to general internists are not consistent with this idea, however, as whereas women were under‐represented in GIM leadership positions, we found no disparities with respect to the gender of first authors or speakers at national meetings for general internists. The finding that hospitalists had gender disparities with respect to first authors and national speakers but general internists did not, argues against several hypotheses (ie, that women lack mentorship, have less career motivation, fewer career building opportunities).
One notable hypothesis, and perhaps one that is often discussed in the literature, is that women shoulder the majority of family responsibilities, and this may result in women having less time for their careers. Jolly and colleagues studied physician‐researchers and noted that women were more likely than men to have spouses or domestic partners who were fully employed, spent 8.5 more hours per week on domestic activities, and were more likely to take time off during disruptions of usual child care.[33] Carr and colleagues found that women with children (compared to men with children) had fewer publications, slower self‐perceived career progress, and lower career satisfaction, but having children had little effect on faculty aspirations and goals.[2] Kaplan et al., however, found that family responsibilities do not appear to account for sex differences in academic advancement.[4] Interestingly, in a study comparing physicians from Generation X to those of the Baby Boomer age, Generation X women reported working more than their male Generation X counterparts, and both had more of a focus on worklife balance than the older generation.[12]
The nature the of 2 specialties' work environment and job requirements could have also resulted in some of the differences seen. Primary care clinical work is typically conducted Monday through Friday, and hospitalist work frequently includes some weekend, evening, night, and holiday coverage. Although these are known differences, both specialties have also been noted to offer many advantages to women and men alike, including collaborative working environments and flexible work hours.[16]
Finally, finding disparity in leadership positions in both specialties supports the possibility that those responsible for hiring could have implicit gender biases. Under‐representation in entry‐level positions is also not a likely explanation for the differences we observed, because nearly an equal number of men and women graduate from medical school, pursue residency training in internal medicine, and become either academic hospitalists or general internists at university settings.[1, 15, 24] This hypothesis could, however, explain why disparities exist with respect to senior authorship and leadership positions, as typically, these individuals have been in practice longer and the current trends of improved gender equality have not always been the case.
Our study has a number of limitations. First, we only examined publications in 2 journals and presentations at 2 national conferences, although the journals and conferences selected are considered to be the major ones in the 2 specialties. Second, using Internet searches may have resulted in inaccurate gender and specialty assignment, but previous studies have used similar methodology.[3, 43] Additionally, we also attempted to contact individuals for direct confirmation when the information we obtained was not clear and had a second investigator independently verify the gender and specialty data. Third, we utilized division/department websites when available to determine the gender of HM divisions/sections. If not recently updated, these websites may not have reflected the most current leader of the unit, but this concern would seemingly pertain to both hospitalists and general internists. Fourth, we opted to only study faculty and division/section heads at university hospitals, as typically these institutions had GIM and hospitalist groups and also typically had websites. Because we only studied faculty and leadership at university hospitals, our data are not generalizable to all hospitalist and GIM groups. Finally, we excluded pediatric hospitalists, and thus, this study is representative of adult hospitalists only. Including pediatric hospitalists was out of the scope of this project.
Our study also had a number of strengths. To our knowledge, this is the first study to provide an estimate of the gender distribution in academic HM, of hospitalists as speakers at national meetings, as first and last authors, and of HM division or section heads, and is the first to compare these results with those observed for general internists. In addition, we examined 7 years of data from 2 of the major journals and national conferences for these specialties.
In summary, despite HM being a newer field with a younger cohort of physicians, we found that gender disparities exist for women with respect to authorship, national speaking opportunities, and division or section leadership. Identifying why these gender differences exist presents an important next step.
Disclosures: Nothing to report. Marisha Burden, MD and Maria G. Frank, MD are coprincipal authors.
Gender disparities still exist for women in academic medicine.[1, 2, 3, 4, 5, 6, 7, 8, 9] The most recent data from the Association of American Medical Colleges (AAMC) show that although gender disparities are decreasing, women are still under‐represented in the assistant, associate, and full‐professor ranks as well as in leadership positions.[1]
Some studies indicate that gender differences are less evident when examining younger cohorts.[1, 10, 11, 12, 13] Hospital medicine emerged around 1996, when the term hospitalist was first coined.[14] The gender distribution of academic hospitalists is likely nearly equal,[15, 16] and they are generally younger physicians.[15, 17, 18, 19, 20] Accordingly, we questioned whether gender disparities existed in academic hospital medicine (HM) and, if so, whether these disparities were greater than those that might exist in academic general internal medicine (GIM).
METHODS
This study consisted of both prospective and retrospective observation of data collected for academic adult hospitalists and general internists who practice in the United States. It was approved by the Colorado Multiple Institutional Review Board.
Gender distribution was assessed with respect to: (1) academic HM and GIM faculty, (2) leadership (ie, division or section heads), and (3) scholarly work (ie, speaking opportunities and publications). Data were collected between October 1, 2012 and August 31, 2014.
Gender Distribution of Faculty and Division/Section Heads
All US internal medicine residency programs were identified from the list of members or affiliates of the AAMC that were fully accredited by the Liaison Committee on Medical Education[21] using the Graduate Medical Education Directory.[22] We then determined the primary training hospital(s) affiliated with each program and selected those that were considered to be university hospitals and eliminated those that did not have divisions or sections of HM or GIM. We determined the gender of the respective division/section heads on the basis of the faculty member's first name (and often from accompanying photos) as well as from information obtained via Internet searches and, if necessary, contacted the individual institutions via email or phone call(s). We also determined the number and gender of all of the HM and GIM faculty members in a random sample of 25% of these hospitals from information on their respective websites.
Gender Distribution for Scholarly Productivity
We determined the gender and specialty of all speakers at the Society of Hospital Medicine and the Society of General Internal Medicine national conferences from 2006 to 2012. A list of speakers at each conference was obtained from conference pamphlets or agendas that were available via Internet searches or obtained directly from the organization. We also determined whether each presenter was a featured speaker (defined as one whose talk was unopposed by other sessions), plenary speaker (defined as such in the conference pamphlets), or if they spoke in a group format (also as indicated in the conference pamphlets). Because of the low number of featured and plenary speakers, these data were combined. Faculty labeled as additional faculty when presenting in a group format were excluded as were speakers at precourses, those presenting abstracts, and those participating in interest group sessions.
For authorship, a PubMed search was used to identify all articles published in the Journal of Hospital Medicine (JHM) and the Journal of General Internal Medicine (JGIM) from January 1, 2006 through December 31, 2012, and the gender and specialty of all the first and last authors were determined as described above. Specialty was determined from the division, section or department affiliation indicated for each author and by Internet searches. In some instances, it was necessary to contact the authors or their departments directly to verify their specialty. When articles had only 1 author, the author was considered a first author.
Duplicate records (eg, same author, same journal) and articles without an author were excluded, as were authors who did not have an MD, DO, or MBBS degree and those who were not affiliated with an institution in the United States. All manuscripts, with the exception of errata, were analyzed together as well as in 3 subgroups: original research, editorials, and others.
A second investigator corroborated data regarding gender and specialty for all speakers and authors to strengthen data integrity. On the rare occasion when discrepancies were found, a third investigator adjudicated the results.
Definitions
Physicians were defined as being hospitalists if they were listed as a member of a division or section of HM on their publications or if Internet searches indicated that they were a hospitalist or primarily worked on inpatient medical services. Physicians were considered to be general internists if they were listed as such on their publications and their specialty could be verified in Web‐based searches. If physicians appeared to have changing roles over time, we attempted to assign their specialty based upon their role at the time the article was published or the presentation was delivered. If necessary, phone calls and/or emails were also done to determine the physician's specialty.
Analysis
REDCap, a secure, Web‐based application for building and managing online surveys and databases, was used to collect and manage all study data.[23] All analyses were performed using SAS Enterprise Guide 4.3 (SAS Institute, Inc., Cary, NC). A [2] test was used to compare proportions of male versus female physicians, and data from hospitalists versus general internists. Because we performed multiple comparisons when analyzing presentations and publications, a Bonferroni adjustment was made such that a P<0.0125 for presentations and P<0.006 (within specialty) or P<0.0125 (between specialty) for the publication analyses were considered significant. P<0.05 was considered significant for all other comparisons.
RESULTS
Gender Distribution of Faculty
Eighteen HM and 20 GIM programs from university hospitals were randomly selected for review (see Supporting Figure 1 in the online version of this article). Seven of the HM programs and 1 of the GIM programs did not have a website, did not differentiate hospitalists from other faculty, or did not list their faculty on the website and were excluded from the analysis. In the remaining 11 HM programs and 19 GIM programs, women made up 277/568 (49%) and 555/1099 (51%) of the faculty, respectively (P=0.50).
Gender Distribution of Division/Section Heads
Eighty‐six of the programs were classified as university hospitals (see Supporting Figure 1 in the online version of this article), and in these, women led 11/69 (16%) of the HM divisions or sections and 28/80 (35%) of the GIM divisions (P=0.008).
Gender Distribution for Scholarly Productivity
Speaking Opportunities
A total of 1227 presentations were given at the 2 conferences from 2006 to 2012, with 1343 of the speakers meeting inclusion criteria (see Supporting Figure 2 in the online version of this article). Hospitalists accounted for 557 of the speakers, of which 146 (26%) were women. General internists accounted for 580 of the speakers, of which 291 (50%) were women (P<0.0001) (Table 1).
Male, N (%) | Female, N (%) | |
---|---|---|
| ||
Hospitalists | ||
All presentations | 411 (74) | 146 (26)* |
Featured or plenary presentations | 49 (91) | 5 (9)* |
General internists | ||
All presentations | 289 (50) | 291 (50) |
Featured or plenary presentations | 27 (55) | 22 (45) |
Of the 117 featured or plenary speakers, 54 were hospitalists and 5 (9%) of these were women. Of the 49 who were general internists, 22 (45%) were women (P<0.0001).
Authorship
The PubMed search identified a total of 3285 articles published in the JHM and the JGIM from 2006 to 2012, and 2172 first authors and 1869 last authors met inclusion criteria (see Supporting Figure 3 in the online version of this article). Hospitalists were listed as first or last authors on 464 and 305 articles, respectively, and of these, women were first authors on 153 (33%) and last authors on 63 (21%). General internists were listed as first or last authors on 895 and 769 articles, respectively, with women as first authors on 423 (47%) and last authors on 265 (34%). Compared with general internists, fewer women hospitalists were listed as either first or last authors (both P<0.0001) (Table 2).
First Author | Last Author | |||
---|---|---|---|---|
Male, N (%) | Female, N (%) | Male, N (%) | Female, N (%) | |
| ||||
Hospitalists | ||||
All publications | 311 (67) | 153 (33)* | 242 (79) | 63 (21)* |
Original investigations/brief reports | 124 (61) | 79 (39)* | 96 (76) | 30 (24)* |
Editorials | 34 (77) | 10 (23)* | 18 (86) | 3 (14)* |
Other | 153 (71) | 64 (29)* | 128 (81) | 30 (19)* |
General internists | ||||
All publications | 472 (53) | 423 (47) | 504 (66) | 265 (34)* |
Original investigations/brief reports | 218 (46) | 261 (54) | 310 (65) | 170 (35)* |
Editorial | 98 (68) | 46 (32)* | 43 (73) | 16 (27)* |
Other | 156 (57) | 116 (43) | 151 (66) | 79 (34)* |
Fewer women hospitalists were listed as first or last authors on all article types. For original research articles written by general internists, there was a trend for more women to be listed as first authors than men (261/479, 54%), but this difference was not statistically significant.
DISCUSSION
The important findings of this study are that, despite an equal gender distribution of academic HM and GIM faculty, fewer women were HM division/section chiefs, fewer women were speakers at the 2 selected national meetings, and fewer women were first or last authors of publications in 2 selected journals in comparison with general internists.
Previous studies have found that women lag behind their male counterparts with respect to academic productivity, leadership, and promotion.[1, 5, 7] Some studies suggest, however, that gender differences are reduced when younger cohorts are examined.[1, 10, 11, 12, 13] Surveys indicate that that the mean age of hospitalists is younger than most other specialties.[15, 19, 20, 24] The mean age of academic GIM physicians is unknown, but surveys of GIM (not differentiating academic from nonacademic) suggest that it is an older cohort than that of HM.[24] Despite hospitalists being a younger cohort, we found gender disparities in all areas investigated.
Our findings with respect to gender disparities in HM division or section leadership are consistent with the annual AAMC Women in US Academic Medicine and Science Benchmarking Report that found only 22% of all permanent division or section heads were women.[1]
Gender disparities with respect to authorship of medical publications have been previously noted,[3, 6, 15, 25] but to our knowledge, this is the first study to investigate the gender of authors who were hospitalists. Although we found a higher proportion of women hospitalists who were first or last authors than was observed by Jagsi and colleagues,[3] women hospitalists were still under‐represented with respect to this measure of academic productivity. Erren et al. reviewed 6 major journals from 2010 and 2011, and found that first authorship of original research by women ranged from 23.7% to 46.7%, and for last authorship from 18.3% to 28.8%.[25] Interestingly, we found no significant gender difference for first authors who were general internists, and there was a trend toward more women general internists being first authors than men for original research, reviews, and brief reports (data not shown).
Our study did not attempt to answer the question of why gender disparities persist, but many previous studies have explored this issue.[4, 8, 12, 26, 27, 28, 29, 30, 31, 32, 33, 34, 35, 36, 37, 38, 39, 40, 41, 42] Issues raised by others include the quantity of academic work (ie, publications and grants obtained), differences in hours worked and allocation of time, lack of mentorship, family responsibilities, discrimination, differences in career motivation, and levels of institutional support, to name a few.
The under‐representation of women hospitalists in leadership, authorship, and speaking opportunities may be consistent with gender‐related differences in research productivity. Fewer publications could lead to fewer national presentations, which could lead to fewer leadership opportunities. Our findings with respect to general internists are not consistent with this idea, however, as whereas women were under‐represented in GIM leadership positions, we found no disparities with respect to the gender of first authors or speakers at national meetings for general internists. The finding that hospitalists had gender disparities with respect to first authors and national speakers but general internists did not, argues against several hypotheses (ie, that women lack mentorship, have less career motivation, fewer career building opportunities).
One notable hypothesis, and perhaps one that is often discussed in the literature, is that women shoulder the majority of family responsibilities, and this may result in women having less time for their careers. Jolly and colleagues studied physician‐researchers and noted that women were more likely than men to have spouses or domestic partners who were fully employed, spent 8.5 more hours per week on domestic activities, and were more likely to take time off during disruptions of usual child care.[33] Carr and colleagues found that women with children (compared to men with children) had fewer publications, slower self‐perceived career progress, and lower career satisfaction, but having children had little effect on faculty aspirations and goals.[2] Kaplan et al., however, found that family responsibilities do not appear to account for sex differences in academic advancement.[4] Interestingly, in a study comparing physicians from Generation X to those of the Baby Boomer age, Generation X women reported working more than their male Generation X counterparts, and both had more of a focus on worklife balance than the older generation.[12]
The nature the of 2 specialties' work environment and job requirements could have also resulted in some of the differences seen. Primary care clinical work is typically conducted Monday through Friday, and hospitalist work frequently includes some weekend, evening, night, and holiday coverage. Although these are known differences, both specialties have also been noted to offer many advantages to women and men alike, including collaborative working environments and flexible work hours.[16]
Finally, finding disparity in leadership positions in both specialties supports the possibility that those responsible for hiring could have implicit gender biases. Under‐representation in entry‐level positions is also not a likely explanation for the differences we observed, because nearly an equal number of men and women graduate from medical school, pursue residency training in internal medicine, and become either academic hospitalists or general internists at university settings.[1, 15, 24] This hypothesis could, however, explain why disparities exist with respect to senior authorship and leadership positions, as typically, these individuals have been in practice longer and the current trends of improved gender equality have not always been the case.
Our study has a number of limitations. First, we only examined publications in 2 journals and presentations at 2 national conferences, although the journals and conferences selected are considered to be the major ones in the 2 specialties. Second, using Internet searches may have resulted in inaccurate gender and specialty assignment, but previous studies have used similar methodology.[3, 43] Additionally, we also attempted to contact individuals for direct confirmation when the information we obtained was not clear and had a second investigator independently verify the gender and specialty data. Third, we utilized division/department websites when available to determine the gender of HM divisions/sections. If not recently updated, these websites may not have reflected the most current leader of the unit, but this concern would seemingly pertain to both hospitalists and general internists. Fourth, we opted to only study faculty and division/section heads at university hospitals, as typically these institutions had GIM and hospitalist groups and also typically had websites. Because we only studied faculty and leadership at university hospitals, our data are not generalizable to all hospitalist and GIM groups. Finally, we excluded pediatric hospitalists, and thus, this study is representative of adult hospitalists only. Including pediatric hospitalists was out of the scope of this project.
Our study also had a number of strengths. To our knowledge, this is the first study to provide an estimate of the gender distribution in academic HM, of hospitalists as speakers at national meetings, as first and last authors, and of HM division or section heads, and is the first to compare these results with those observed for general internists. In addition, we examined 7 years of data from 2 of the major journals and national conferences for these specialties.
In summary, despite HM being a newer field with a younger cohort of physicians, we found that gender disparities exist for women with respect to authorship, national speaking opportunities, and division or section leadership. Identifying why these gender differences exist presents an important next step.
Disclosures: Nothing to report. Marisha Burden, MD and Maria G. Frank, MD are coprincipal authors.
- Association of American Medical Colleges. Women in U.S. academic medicine and science: Statistics and benchmarking report. 2012. Available at: https://members.aamc.org/eweb/upload/Women%20in%20U%20S%20%20Academic%20Medicine%20Statistics%20and%20Benchmarking%20Report%202011-20123.pdf. Accessed September 1, 2014.
- Relation of family responsibilities and gender to the productivity and career satisfaction of medical faculty. Ann Intern Med. 1998;129:532–538. , , , et al.
- The “gender gap” in authorship of academic medical literature—a 35‐year perspective. N Engl J Med. 2006;355:281–287. , , , et al.
- Sex differences in academic advancement. Results of a national study of pediatricians. N Engl J Med. 1996;335:1282–1289. , , , , , .
- Women physicians in academic medicine: new insights from cohort studies. N Engl J Med. 2000;342:399–405. .
- Gender differences in academic productivity and leadership appointments of physicians throughout academic careers. Acad Med. 2011;86:43–47. , , , , .
- Promotion of women physicians in academic medicine. Glass ceiling or sticky floor? JAMA. 1995;273:1022–1025. , , , .
- Compensation and advancement of women in academic medicine: is there equity? Ann Intern Med. 2004;141:205–212. , , , .
- Women physicians: choosing a career in academic medicine. Acad Med. 2012;87:105–114. , , .
- The status of women at one academic medical center. Breaking through the glass ceiling. JAMA. 1990;264:1813–1817. , , , .
- Status of women in academic anesthesiology. Anesthesiology. 1986;64:496–500. , .
- The generation and gender shifts in medicine: an exploratory survey of internal medicine physicians. BMC Health Serv Res. 2006;6:55. , , .
- Pew Research Center. On pay gap, millenial women near parity—for now. December 2013. Available at: http://www.pewsocialtrends.org/files/2013/12/gender-and-work_final.pdf. Published December 11, 2013. Accessed February 5, 2015.
- The emerging role of "hospitalists" in the American health care system. N Engl J Med. 1996;335:514–517. , .
- Mentorship, productivity, and promotion among academic hospitalists. J Gen Intern Med. 2012;27:23–27. , , , , , .
- The gender factor. The Hospitalist. Available at: http://www.the‐hospitalist.org/article/the‐gender‐factor. Published March 1, 2006. Accessed September 1, 2014. .
- Association of American Medical Colleges. Analysis in brief: Supplemental information for estimating the number and characteristics of hospitalist physicians in the United States and their possible workforce implications. Available at: https://www.aamc.org/download/300686/data/aibvol12_no3-supplemental.pdf. Published August 2012. Accessed September 1, 2014.
- Survey of US academic hospitalist leaders about mentorship and academic activities in hospitalist groups. J Hosp Med. 2011;6:5–9. , , , .
- State of Hospital Medicine: 2011 Report Based on 2010 Data. Medical Group Management Association and Society of Hospital Medicine. www.mgma.com, www.hospitalmedicine.org.
- Today's Hospitalist Survey. Compensation and Career Survey Results. 2013. Available at: http://www.todayshospitalist.com/index.php?b=salary_survey_results. Accessed January 11, 2015.
- Association of American Medical Colleges. Women in U.S. Academic Medicine: Statistics and Benchmarking Report. 2009–2010. Available at: https://www.aamc.org/download/182674/data/gwims_stats_2009‐2010.pdf. Accessed September 1, 2014.
- American Medical Association. Graduate Medical Education Directory 2012–2013. Chicago, IL: American Medical Association; 2012:182–203.
- Research electronic data capture (REDCap)—a metadata‐driven methodology and workflow process for providing translational research informatics support. J Biomed Inform. 2009;42:377–381. , , , , , .
- Association of American Medical Colleges. 2012 Physician Specialty Data Book. Center for Workforce Studies. Available at: https://www.aamc.org/download/313228/data/2012physicianspecialtydatabook.pdf. Published November 2012. Accessed September 1, 2014.
- Representation of women as authors, reviewers, editors in chief, and editorial board members at 6 general medical journals in 2010 and 2011. JAMA Intern Med. 2014;174:633–635. , , , .
- Relationships of gender and career motivation to medical faculty members' production of academic publications. Acad Med. 1998;73:180–186. , , , et al.
- Faculty perceptions of gender discrimination and sexual harassment in academic medicine. Ann Intern Med. 2000;132:889–896. , , , et al.
- Attitudes of clinical faculty about career progress, career success and recognition, and commitment to academic medicine. Results of a survey. Arch Intern Med. 2000;160:2625–2629. , , , .
- A "ton of feathers": gender discrimination in academic medical careers and how to manage it. J Womens Health (Larchmt). 2003;12:1009–1018. , , , , .
- Perceived obstacles to career success for women in academic surgery. Arch Surg. 2000;135:972–977. , , .
- Career satisfaction of US women physicians: results from the Women Physicians' Health Study. Society of General Internal Medicine Career Satisfaction Study Group. Arch Intern Med. 1999;159:1417–1426. , , , .
- Doing the same and earning less: male and female physicians in a new medical specialty. Inquiry. 2004;41:301–315. .
- Gender differences in time spent on parenting and domestic responsibilities by high‐achieving young physician‐researchers. Ann Intern Med. 2014;160:344–353. , , , , , .
- Stories from early‐career women physicians who have left academic medicine: a qualitative study at a single institution. Acad Med. 2011;86:752–758. , , , , .
- The $16,819 pay gap for newly trained physicians: the unexplained trend of men earning more than women. Health Aff (Millwood). 2011;30:193–201. , , , .
- Experiencing the culture of academic medicine: gender matters, a national study. J Gen Intern Med. 2013;28:201–207. , , , , .
- Gender pay gaps in hospital medicine. The Hospitalist. Available at: http://www.the‐hospitalist.org/article/gender‐pay‐gaps‐in‐hospital‐medicine. Published February 29, 2012. Accessed September 1, 2014. .
- Mentoring in academic medicine: a systematic review. JAMA. 2006;296:1103–1115. , , .
- Inequality quantified: mind the gender gap. Nature. 2013;495:22–24. .
- Gender differences in academic advancement: patterns, causes, and potential solutions in one US College of Medicine. Acad Med. 2003;78:500–508. , , , et al.
- Why aren't there more women leaders in academic medicine? The views of clinical department chairs. Acad Med. 2001;76:453–465. , .
- Gender factors in reviewer recommendations for manuscript publication. J Appl Behav Anal. 1990;23:539–543. .
- Scientific impact of women in academic surgery. J Surg Res. 2008;148:13–16. , , , .
- Association of American Medical Colleges. Women in U.S. academic medicine and science: Statistics and benchmarking report. 2012. Available at: https://members.aamc.org/eweb/upload/Women%20in%20U%20S%20%20Academic%20Medicine%20Statistics%20and%20Benchmarking%20Report%202011-20123.pdf. Accessed September 1, 2014.
- Relation of family responsibilities and gender to the productivity and career satisfaction of medical faculty. Ann Intern Med. 1998;129:532–538. , , , et al.
- The “gender gap” in authorship of academic medical literature—a 35‐year perspective. N Engl J Med. 2006;355:281–287. , , , et al.
- Sex differences in academic advancement. Results of a national study of pediatricians. N Engl J Med. 1996;335:1282–1289. , , , , , .
- Women physicians in academic medicine: new insights from cohort studies. N Engl J Med. 2000;342:399–405. .
- Gender differences in academic productivity and leadership appointments of physicians throughout academic careers. Acad Med. 2011;86:43–47. , , , , .
- Promotion of women physicians in academic medicine. Glass ceiling or sticky floor? JAMA. 1995;273:1022–1025. , , , .
- Compensation and advancement of women in academic medicine: is there equity? Ann Intern Med. 2004;141:205–212. , , , .
- Women physicians: choosing a career in academic medicine. Acad Med. 2012;87:105–114. , , .
- The status of women at one academic medical center. Breaking through the glass ceiling. JAMA. 1990;264:1813–1817. , , , .
- Status of women in academic anesthesiology. Anesthesiology. 1986;64:496–500. , .
- The generation and gender shifts in medicine: an exploratory survey of internal medicine physicians. BMC Health Serv Res. 2006;6:55. , , .
- Pew Research Center. On pay gap, millenial women near parity—for now. December 2013. Available at: http://www.pewsocialtrends.org/files/2013/12/gender-and-work_final.pdf. Published December 11, 2013. Accessed February 5, 2015.
- The emerging role of "hospitalists" in the American health care system. N Engl J Med. 1996;335:514–517. , .
- Mentorship, productivity, and promotion among academic hospitalists. J Gen Intern Med. 2012;27:23–27. , , , , , .
- The gender factor. The Hospitalist. Available at: http://www.the‐hospitalist.org/article/the‐gender‐factor. Published March 1, 2006. Accessed September 1, 2014. .
- Association of American Medical Colleges. Analysis in brief: Supplemental information for estimating the number and characteristics of hospitalist physicians in the United States and their possible workforce implications. Available at: https://www.aamc.org/download/300686/data/aibvol12_no3-supplemental.pdf. Published August 2012. Accessed September 1, 2014.
- Survey of US academic hospitalist leaders about mentorship and academic activities in hospitalist groups. J Hosp Med. 2011;6:5–9. , , , .
- State of Hospital Medicine: 2011 Report Based on 2010 Data. Medical Group Management Association and Society of Hospital Medicine. www.mgma.com, www.hospitalmedicine.org.
- Today's Hospitalist Survey. Compensation and Career Survey Results. 2013. Available at: http://www.todayshospitalist.com/index.php?b=salary_survey_results. Accessed January 11, 2015.
- Association of American Medical Colleges. Women in U.S. Academic Medicine: Statistics and Benchmarking Report. 2009–2010. Available at: https://www.aamc.org/download/182674/data/gwims_stats_2009‐2010.pdf. Accessed September 1, 2014.
- American Medical Association. Graduate Medical Education Directory 2012–2013. Chicago, IL: American Medical Association; 2012:182–203.
- Research electronic data capture (REDCap)—a metadata‐driven methodology and workflow process for providing translational research informatics support. J Biomed Inform. 2009;42:377–381. , , , , , .
- Association of American Medical Colleges. 2012 Physician Specialty Data Book. Center for Workforce Studies. Available at: https://www.aamc.org/download/313228/data/2012physicianspecialtydatabook.pdf. Published November 2012. Accessed September 1, 2014.
- Representation of women as authors, reviewers, editors in chief, and editorial board members at 6 general medical journals in 2010 and 2011. JAMA Intern Med. 2014;174:633–635. , , , .
- Relationships of gender and career motivation to medical faculty members' production of academic publications. Acad Med. 1998;73:180–186. , , , et al.
- Faculty perceptions of gender discrimination and sexual harassment in academic medicine. Ann Intern Med. 2000;132:889–896. , , , et al.
- Attitudes of clinical faculty about career progress, career success and recognition, and commitment to academic medicine. Results of a survey. Arch Intern Med. 2000;160:2625–2629. , , , .
- A "ton of feathers": gender discrimination in academic medical careers and how to manage it. J Womens Health (Larchmt). 2003;12:1009–1018. , , , , .
- Perceived obstacles to career success for women in academic surgery. Arch Surg. 2000;135:972–977. , , .
- Career satisfaction of US women physicians: results from the Women Physicians' Health Study. Society of General Internal Medicine Career Satisfaction Study Group. Arch Intern Med. 1999;159:1417–1426. , , , .
- Doing the same and earning less: male and female physicians in a new medical specialty. Inquiry. 2004;41:301–315. .
- Gender differences in time spent on parenting and domestic responsibilities by high‐achieving young physician‐researchers. Ann Intern Med. 2014;160:344–353. , , , , , .
- Stories from early‐career women physicians who have left academic medicine: a qualitative study at a single institution. Acad Med. 2011;86:752–758. , , , , .
- The $16,819 pay gap for newly trained physicians: the unexplained trend of men earning more than women. Health Aff (Millwood). 2011;30:193–201. , , , .
- Experiencing the culture of academic medicine: gender matters, a national study. J Gen Intern Med. 2013;28:201–207. , , , , .
- Gender pay gaps in hospital medicine. The Hospitalist. Available at: http://www.the‐hospitalist.org/article/gender‐pay‐gaps‐in‐hospital‐medicine. Published February 29, 2012. Accessed September 1, 2014. .
- Mentoring in academic medicine: a systematic review. JAMA. 2006;296:1103–1115. , , .
- Inequality quantified: mind the gender gap. Nature. 2013;495:22–24. .
- Gender differences in academic advancement: patterns, causes, and potential solutions in one US College of Medicine. Acad Med. 2003;78:500–508. , , , et al.
- Why aren't there more women leaders in academic medicine? The views of clinical department chairs. Acad Med. 2001;76:453–465. , .
- Gender factors in reviewer recommendations for manuscript publication. J Appl Behav Anal. 1990;23:539–543. .
- Scientific impact of women in academic surgery. J Surg Res. 2008;148:13–16. , , , .
© 2015 Society of Hospital Medicine
Problems Identified by Advice Line Calls
The period immediately following hospital discharge is particularly hazardous for patients.[1, 2, 3, 4, 5] Problems occurring after discharge may result in high rates of rehospitalization and unscheduled visits to healthcare providers.[6, 7, 8, 9, 10] Numerous investigators have tried to identify patients who are at increased risk for rehospitalizations within 30 days of discharge, and many studies have examined whether various interventions could decrease these adverse events (summarized in Hansen et al.[11]). An increasing fraction of patients discharged by medicine and surgery services have some or all of their care supervised by hospitalists. Thus, hospitals increasingly look to hospitalists for ways to reduce rehospitalizations.
Patients discharged from our hospital are instructed to call an advice line (AL) if and when questions or concerns arise. Accordingly, we examined when these calls were made and what issues were raised, with the idea that the information collected might identify aspects of our discharge processes that needed improvement.
METHODS
Study Design
We conducted a prospective study of a cohort consisting of all unduplicated patients with a matching medical record number in our data warehouse who called our AL between September 1, 2011 and September 1, 2012, and reported being hospitalized or having surgery (inpatient or outpatient) within 30 days preceding their call. We excluded patients who were incarcerated, those who were transferred from other hospitals, those admitted for routine chemotherapy or emergent dialysis, and those discharged to a skilled nursing facility or hospice. The study involved no intervention. It was approved by the Colorado Multiple Institutional Review Board.
Setting
The study was conducted at Denver Health Medical Center, a 525‐bed, university‐affiliated, public safety‐net hospital. At the time of discharge, all patients were given paperwork that listed the telephone number of the AL and written instructions in English or Spanish telling them to call the AL or their primary care physician if they had any of a list of symptoms that was selected by their discharging physician as being relevant to that specific patient's condition(s).
The AL was established in 1997 to provide medical triage to patients of Denver Health. It operates 24 hours a day, 7 days per week, and receives approximately 100,000 calls per year. A language line service is used with nonEnglish‐speaking callers. Calls are handled by a nurse who, with the assistance of a commercial software program (E‐Centaurus; LVM Systems, Phoenix, AZ) containing clinical algorithms (Schmitt‐Thompson Clinical Content, Windsor, CO), makes a triage recommendation. Nurses rarely contact hospital or clinic physicians to assist with triage decisions.
Variables Assessed
We categorized the nature of the callers' reported problem(s) to the AL using the taxonomy summarized in the online appendix (see Supporting Appendix in the online version of this article). We then queried our data warehouse for each patient's demographic information, patient‐level comorbidities, discharging service, discharge date and diagnoses, hospital length of stay, discharge disposition, and whether they had been hospitalized or sought care in our urgent care center or emergency department within 30 days of discharge. The same variables were collected for all unduplicated patients who met the same inclusion and exclusion criteria and were discharged from Denver Health during the same time period but did not call the AL.
Statistics
Data were analyzed using SAS Enterprise Guide 4.1 (SAS Institute, Inc., Cary, NC). Because we made multiple statistical comparisons, we applied the Bonferroni correction when comparing patients calling the AL with those who did not, such that P<0.004 indicated statistical significance. A Student t test or a Wilcoxon rank sum test was used to compare continuous variables depending on results of normality tests. 2 tests were used to compare categorical variables. The intervals between hospital discharge and the call to the AL for patients discharged from medicine versus surgery services were compared using a log‐rank test, with P<0.05 indicating statistical significance.
RESULTS
During the 1‐year study period, 19,303 unique patients were discharged home with instructions regarding the use of the AL. A total of 310 patients called the AL and reported being hospitalized or having surgery within the preceding 30 days. Of these, 2 were excluded (1 who was incarcerated and 1 who was discharged to a skilled nursing facility), leaving 308 patients in the cohort. This represented 1.5% of the total number of unduplicated patients discharged during this same time period (minus the exclusions described above). The large majority of the calls (277/308, 90%) came directly from patients. The remaining 10% came from a proxy, usually a patient's family member. Compared with patients who were discharged during the same time period who did not call the AL, those who called were more likely to speak English, less likely to speak Spanish, more likely to be medically indigent, had slightly longer lengths of stays for their index hospitalization, and were more likely to be discharged from surgery than medicine services (particularly following inpatient surgery) (Table 1).
Patient Characteristics | Patients Calling Advice Line After Discharge, N=308 | Patients Not Calling Advice Line After Discharge, N=18,995 | P Valuea |
---|---|---|---|
| |||
Age, y (meanSD) | 4217 | 3921 | 0.0210 |
Gender, female, n (%) | 162 (53) | 10,655 (56) | |
Race/ethnicity, n (%) | 0.1208 | ||
Hispanic/Latino/Spanish | 129 (42) | 8,896 (47) | |
African American | 44 (14) | 2,674 (14) | |
White | 125 (41) | 6,569 (35) | |
Language, n (%) | <0.0001 | ||
English | 273 (89) | 14,236 (79) | |
Spanish | 32 (10) | 3,744 (21) | |
Payer, n (%) | |||
Medicare | 45 (15) | 3,013 (16) | |
Medicaid | 105 (34) | 7,777 (41) | 0.0152 |
Commercial | 49 (16) | 2,863 (15) | |
Medically indigentb | 93 (30) | 3,442 (18) | <0.0001 |
Self‐pay | 5 (1) | 1,070 (5) | |
Primary care provider, n (%)c | 168 (55) | 10,136 (53) | 0.6794 |
Psychiatric comorbidity, n (%) | 81 (26) | 4,528 (24) | 0.3149 |
Alcohol or substance abuse comorbidity, n (%) | 65 (21) | 3,178 (17) | 0.0417 |
Discharging service, n (%) | <0.0001 | ||
Surgery | 193 (63) | 7,247 (38) | |
Inpatient | 123 (40) | 3,425 (18) | |
Ambulatory | 70 (23) | 3,822 (20) | |
Medicine | 93 (30) | 6,038 (32) | |
Pediatric | 4 (1) | 1,315 (7) | |
Obstetric | 11 (4) | 3,333 (18) | |
Length of stay, median (IQR) | 2 (04.5) | 1 (03) | 0.0003 |
Inpatient medicine | 4 (26) | 3 (15) | 0.0020 |
Inpatient surgery | 3 (16) | 2 (14) | 0.0019 |
Charlson Comorbidity Index, median (IQR) | |||
Inpatient medicine | 1 (04) | 1 (02) | 0.0435 |
Inpatient surgery | 0 (01) | 0 (01) | 0.0240 |
The median time from hospital discharge to the call was 3 days (interquartile range [IQR], 16), but 31% and 47% of calls occurred within 24 or 48 hours of discharge, respectively. Ten percent of patients called the AL the same day of discharge (Figure 1). We found no difference in timing of the calls as a function of discharging service.
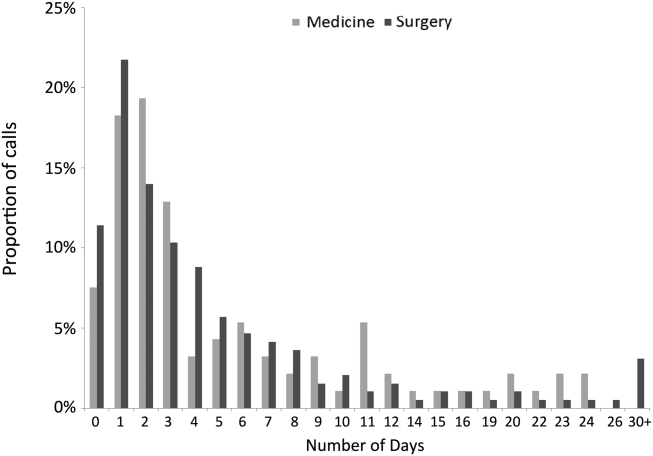
The 308 patients reported a total of 612 problems or concerns (meanstandard deviation number of complaints per caller=21), the large majority of which (71%) were symptom‐related (Table 2). The most common symptom was uncontrolled pain, reported by 33% and 40% of patients discharged from medicine and surgery services, respectively. The next most common symptoms related to the gastrointestinal system and to surgical site issues in medicine and surgery patients, respectively (data not shown).
Total Cohort, n (%) | Patients Discharged From Medicine, n (%) | Patients Discharged From Surgery, n (%) | ||||
---|---|---|---|---|---|---|
Patients | Complaints | Patients | Complaints | Patients | Complaints | |
Symptom related | 280 (91) | 433 (71) | 89 (96) | 166 (77) | 171 (89) | 234 (66) |
Discharge instructions | 65 (21) | 81 (13) | 18 (19) | 21 (10) | 43 (22) | 56 (16) |
Medication related | 65 (21) | 87 (14) | 19 (20) | 25 (11) | 39 (20) | 54 (15) |
Other | 10 (3) | 11 (2) | 4 (4) | 4 (2) | 6 (3) | 7 (2) |
Total | 612 (100) | 216 (100) | 351 (100) |
Sixty‐five patients, representing 21% of the cohort, reported 81 problems understanding or executing discharge instructions. No difference was observed between the fraction of these problems reported by patients from medicine versus surgery (19% and 22%, respectively, P=0.54).
Sixty‐five patients, again representing 21% of the cohort, reported 87 medication‐related problems, 20% from both the medicine and surgery services (P=0.99). Medicine patients more frequently reported difficulties understanding their medication instructions, whereas surgery patients more frequently reported lack of efficacy of medications, particularly with respect to pain control (data not shown).
Thirty percent of patients who called the AL were advised by the nurse to go to the emergency department immediately. Medicine patients were more likely to be triaged to the emergency department compared with surgery patients (45% vs 22%, P<0.0001).
The 30‐day readmission rates and the rates of unscheduled urgent or emergent care visits were higher for patients calling the AL compared with those who did not call (46/308, 15% vs 706/18,995, 4%, and 92/308, 30% vs 1303/18,995, 7%, respectively, both P<0.0001). Similar differences were found for patients discharged from medicine or surgery services who called the AL compared with those who did not (data not shown, both P<0.0001). The median number of days between AL call and rehospitalization was 0 (IQR, 02) and 1 (IQR, 08) for medicine and surgery patients, respectively. Ninety‐three percent of rehospitalizations were related to the index hospitalization, and 78% of patients who were readmitted had no outpatient encounter in the interim between discharge and rehospitalization.
DISCUSSION
We investigated the source and nature of patient telephone calls to an AL following a hospitalization or surgery, and our data revealed the following important findings: (1) nearly one‐half of the calls to the AL occurred within the first 48 hours following discharge; (2) the majority of the calls came from surgery patients, and a greater fraction of patients discharged from surgery services called the AL than patients discharged from medicine services; (3) the most common issues were uncontrolled pain, questions about medications, and problems understanding or executing aftercare instructions (particularly pertaining to the care of surgical wounds); and (4) patients calling the AL had higher rates of 30‐day rehospitalization and of unscheduled urgent or emergent care visits.
The utilization of our patient‐initiated call line was only 1.5%, which was on the low end of the 1% to 10% reported in the literature.[7, 12] This can be attributed to a number of issues that are specific to our system. First, the discharge instructions provided to our patients stated that they should call their primary care provider or the AL if they had questions. Accordingly, because approximately 50% of our patients had a primary care provider in our system, some may have preferentially contacted their primary care provider rather than the AL. Second, the instructions stated that the patients should call if they were experiencing the symptoms listed on the instruction sheet, so those with other problems/complaints may not have called. Third, AL personnel identified patients as being in our cohort by asking if they had been discharged or underwent a surgical procedure within 30‐days of their call. This may have resulted in the under‐reporting of patients who were hospitalized or had outpatient surgical procedures. Fourth, there may have been a number of characteristics specific to patients in our system that reduced the frequency with which they utilized the AL (eg, access to telephones or other community providers).
Most previous studies of patient‐initiated call lines have included them as part of multi‐intervention pre‐ and/or postdischarge strategies.[7, 8, 9, 10, 11, 12, 13] One prior small study compared the information reported by 37 patients who called an AL with that elicited by nurse‐initiated patient contact.[12] The most frequently reported problems in this study were medication‐related issues (43%). However, this study only included medicine patients and did not document the proportion of calls occurring at various time intervals.
The problems we identified (in both medicine and surgery patients) have previously been described,[2, 3, 4, 13, 14, 15, 16] but all of the studies reporting these problems utilized calls that were initiated by health care providers to patients at various fixed intervals following discharge (ie, 730 days). Most of these used a scripted approach seeking responses to specific questions or outcomes, and the specific timing at which the problems arose was not addressed. In contrast, we examined unsolicited concerns expressed by patients calling an AL following discharge whenever they felt sufficient urgency to address whatever problems or questions arose. We found that a large fraction of calls occurred on the day of or within the first 48 hours following discharge, much earlier than when provider‐initiated calls in the studies cited above occurred. Accordingly, our results cannot be used to compare the utility of patient‐ versus provider‐initiated calls, or to suggest that other hospitals should create an AL system. Rather, we suggest that our findings might be complementary to those reported in studies of provider‐initiated calls and only propose that by examining calls placed by patients to ALs, problems with hospital discharge processes (some of which may result in increased rates of readmission) may be discovered.
The observation that such a large fraction of calls to our AL occurred within the first 48 hours following discharge, together with the fact that many of the questions asked or concerns raised pertained to issues that should have been discussed during the discharge process (eg, pain control, care of surgical wounds), suggests that suboptimal patient education was occurring prior to discharge as was suggested by Henderson and Zernike.[17] This finding has led us to expand our patient education processes prior to discharge on both medicine and surgery services. Because our hospitalists care for approximately 90% of the patients admitted to medicine services and are increasingly involved in the care of patients on surgery services, they are integrally involved with such quality improvement initiatives.
To our knowledge this is the first study in the literature that describes both medicine and surgery patients who call an AL because of problems or questions following hospital discharge, categorizes these problems, determines when the patients called following their discharge, and identifies those who called as being at increased risk for early rehospitalizations and unscheduled urgent or emergent care visits. Given the financial penalties issued to hospitals with high 30‐day readmission rates, these patients may warrant more attention than is customarily available from telephone call lines or during routine outpatient follow‐up. The majority of patients who called our AL had Medicare, Medicaid, or a commercial insurance, and, accordingly, may have been eligible for additional services such as home visits and/or expedited follow‐up appointments.
Our study has a number of limitations. First, it is a single‐center study, so the results might not generalize to other institutions. Second, because the study was performed in a university‐affiliated, public safety‐net hospital, patient characteristics and the rates and types of postdischarge concerns that we observed might differ from those encountered in different types of hospitals and/or from those in nonteaching institutions. We would suggest, however, that the idea of using concerns raised by patients discharged from any type of hospital in calls to ALs may similarly identify problems with that specific hospital's discharge processes. Third, the information collected from the AL came from summaries provided by nurses answering the calls rather than from actual transcripts. This could have resulted in insufficient or incorrect information pertaining to some of the variables assessed in Table 2. The information presented in Table 1, however, was obtained from our data warehouse after matching medical record numbers. Fourth, we could have underestimated the number of patients who had 30‐day rehospitalizations and/or unplanned for urgent or emergent care visits if patients sought care at other hospitals. Fifth, the number of patients calling the AL was too small to allow us to do any type of robust matching or multivariable analysis. Accordingly, the differences that appeared between patients who called and those who did not (ie, English speakers, being medically indigent, the length of stay for the index hospitalization and the discharging service) could be the result of inadequate matching or interactions among the variables. Although matching or multivariate analysis might have yielded different associations between patients who called the AL versus those who did not, those who called the AL still had an increased risk of readmission and urgent or emergent visits and may still benefit from targeted interventions. Finally, the fact that only 1.5% of unique patients who were discharged called the AL could have biased our results. Because only 55% and 53% of the patients who did or did not call the AL, respectively, saw primary care physicians within our system within the 3 years prior to their index hospitalization (P=0.679), the frequency of calls to the AL that we observed could have underestimated the frequency with which patients had contact with other care providers in the community.
In summary, information collected from patient‐initiated calls to our AL identified several aspects of our discharge processes that needed improvement. We concluded that our predischarge educational processes for both medicine and surgery services needed modification, especially with respect to pain management, which problems to expect after hospitalization or surgery, and how to deal with them. The high rates of 30‐day rehospitalization and of unscheduled urgent or emergent care visits among patients calling the AL identifies them as being at increased risk for these outcomes, although the likelihood of these events may be related to factors other than just calling the AL.
- Implementation of the care transitions intervention: sustainability and lessons learned. Prof Case Manag. 2009;14(6):282–293. , , , , .
- Problems after discharge and understanding of communication with their primary care physicians among hospitalized seniors: a mixed methods study. J Hosp Med. 2010;5(7):385–391. , , , et al.
- Adverse events among medical patients after discharge from hospital. CMAJ. 2004;170(3):345–349. , , , et al.
- The incidence and severity of adverse events affecting patients after discharge from the hospital. Ann Intern Med. 2003;138(3):161–167. , , , , .
- Post‐hospitalization transitions: examining the effects of timing of primary care provider follow‐up. J Hosp Med. 2010;5(7):392–397. , , .
- Telephone follow‐up after discharge from the hospital: does it make a difference? Appl Nurs Res. 1996;9(2) 47–52. , , , .
- The effect of real‐time teleconsultations between hospital‐based nurses and patients with severe COPD discharged after an exacerbation. J Telemed Telecare. 2013;19(8):466–474. , , , et al.
- A randomized, controlled trial of an intensive community nurse‐supported discharge program in preventing hospital readmissions of older patients with chronic lung disease. J Am Geriatr Soc. 2004;52(8):1240–1246. , , , , , .
- Effects of education and support on self‐care and resource utilization in patients with heart failure. Eur Heart J. 1999;20(9):673–682. , , , et al.
- Comprehensive discharge planning and home follow‐up of hospitalized elders: a randomized clinical trial. JAMA. 1999;281(7):613–620. , , , et al.
- Interventions to reduce 30‐day rehospitalization: a systematic review. Ann Intern Med. 2011;155(8):520–528. , , , , .
- Complementary telephone strategies to improve postdischarge communication. Am J Med. 2012;125(1):28–30. , , , .
- Integrated postdischarge transitional care in a hospitalist system to improve discharge outcome: an experimental study. BMC Med. 2011;9:96. , , , , , .
- Patient experiences after hospitalizations for elective surgery. Am J Surg. 2014;207(6):855–862. , , , , , .
- Complications after discharge for surgical patients. ANZ J Surg. 2004;74(3):92–97. , , .
- Surgeons are overlooking post‐discharge complications: a prospective cohort study. World J Surg. 2014;38(5):1019–1025. , , , .
- A study of the impact of discharge information for surgical patients. J Adv Nurs. 2001;35(3):435–441. , .
The period immediately following hospital discharge is particularly hazardous for patients.[1, 2, 3, 4, 5] Problems occurring after discharge may result in high rates of rehospitalization and unscheduled visits to healthcare providers.[6, 7, 8, 9, 10] Numerous investigators have tried to identify patients who are at increased risk for rehospitalizations within 30 days of discharge, and many studies have examined whether various interventions could decrease these adverse events (summarized in Hansen et al.[11]). An increasing fraction of patients discharged by medicine and surgery services have some or all of their care supervised by hospitalists. Thus, hospitals increasingly look to hospitalists for ways to reduce rehospitalizations.
Patients discharged from our hospital are instructed to call an advice line (AL) if and when questions or concerns arise. Accordingly, we examined when these calls were made and what issues were raised, with the idea that the information collected might identify aspects of our discharge processes that needed improvement.
METHODS
Study Design
We conducted a prospective study of a cohort consisting of all unduplicated patients with a matching medical record number in our data warehouse who called our AL between September 1, 2011 and September 1, 2012, and reported being hospitalized or having surgery (inpatient or outpatient) within 30 days preceding their call. We excluded patients who were incarcerated, those who were transferred from other hospitals, those admitted for routine chemotherapy or emergent dialysis, and those discharged to a skilled nursing facility or hospice. The study involved no intervention. It was approved by the Colorado Multiple Institutional Review Board.
Setting
The study was conducted at Denver Health Medical Center, a 525‐bed, university‐affiliated, public safety‐net hospital. At the time of discharge, all patients were given paperwork that listed the telephone number of the AL and written instructions in English or Spanish telling them to call the AL or their primary care physician if they had any of a list of symptoms that was selected by their discharging physician as being relevant to that specific patient's condition(s).
The AL was established in 1997 to provide medical triage to patients of Denver Health. It operates 24 hours a day, 7 days per week, and receives approximately 100,000 calls per year. A language line service is used with nonEnglish‐speaking callers. Calls are handled by a nurse who, with the assistance of a commercial software program (E‐Centaurus; LVM Systems, Phoenix, AZ) containing clinical algorithms (Schmitt‐Thompson Clinical Content, Windsor, CO), makes a triage recommendation. Nurses rarely contact hospital or clinic physicians to assist with triage decisions.
Variables Assessed
We categorized the nature of the callers' reported problem(s) to the AL using the taxonomy summarized in the online appendix (see Supporting Appendix in the online version of this article). We then queried our data warehouse for each patient's demographic information, patient‐level comorbidities, discharging service, discharge date and diagnoses, hospital length of stay, discharge disposition, and whether they had been hospitalized or sought care in our urgent care center or emergency department within 30 days of discharge. The same variables were collected for all unduplicated patients who met the same inclusion and exclusion criteria and were discharged from Denver Health during the same time period but did not call the AL.
Statistics
Data were analyzed using SAS Enterprise Guide 4.1 (SAS Institute, Inc., Cary, NC). Because we made multiple statistical comparisons, we applied the Bonferroni correction when comparing patients calling the AL with those who did not, such that P<0.004 indicated statistical significance. A Student t test or a Wilcoxon rank sum test was used to compare continuous variables depending on results of normality tests. 2 tests were used to compare categorical variables. The intervals between hospital discharge and the call to the AL for patients discharged from medicine versus surgery services were compared using a log‐rank test, with P<0.05 indicating statistical significance.
RESULTS
During the 1‐year study period, 19,303 unique patients were discharged home with instructions regarding the use of the AL. A total of 310 patients called the AL and reported being hospitalized or having surgery within the preceding 30 days. Of these, 2 were excluded (1 who was incarcerated and 1 who was discharged to a skilled nursing facility), leaving 308 patients in the cohort. This represented 1.5% of the total number of unduplicated patients discharged during this same time period (minus the exclusions described above). The large majority of the calls (277/308, 90%) came directly from patients. The remaining 10% came from a proxy, usually a patient's family member. Compared with patients who were discharged during the same time period who did not call the AL, those who called were more likely to speak English, less likely to speak Spanish, more likely to be medically indigent, had slightly longer lengths of stays for their index hospitalization, and were more likely to be discharged from surgery than medicine services (particularly following inpatient surgery) (Table 1).
Patient Characteristics | Patients Calling Advice Line After Discharge, N=308 | Patients Not Calling Advice Line After Discharge, N=18,995 | P Valuea |
---|---|---|---|
| |||
Age, y (meanSD) | 4217 | 3921 | 0.0210 |
Gender, female, n (%) | 162 (53) | 10,655 (56) | |
Race/ethnicity, n (%) | 0.1208 | ||
Hispanic/Latino/Spanish | 129 (42) | 8,896 (47) | |
African American | 44 (14) | 2,674 (14) | |
White | 125 (41) | 6,569 (35) | |
Language, n (%) | <0.0001 | ||
English | 273 (89) | 14,236 (79) | |
Spanish | 32 (10) | 3,744 (21) | |
Payer, n (%) | |||
Medicare | 45 (15) | 3,013 (16) | |
Medicaid | 105 (34) | 7,777 (41) | 0.0152 |
Commercial | 49 (16) | 2,863 (15) | |
Medically indigentb | 93 (30) | 3,442 (18) | <0.0001 |
Self‐pay | 5 (1) | 1,070 (5) | |
Primary care provider, n (%)c | 168 (55) | 10,136 (53) | 0.6794 |
Psychiatric comorbidity, n (%) | 81 (26) | 4,528 (24) | 0.3149 |
Alcohol or substance abuse comorbidity, n (%) | 65 (21) | 3,178 (17) | 0.0417 |
Discharging service, n (%) | <0.0001 | ||
Surgery | 193 (63) | 7,247 (38) | |
Inpatient | 123 (40) | 3,425 (18) | |
Ambulatory | 70 (23) | 3,822 (20) | |
Medicine | 93 (30) | 6,038 (32) | |
Pediatric | 4 (1) | 1,315 (7) | |
Obstetric | 11 (4) | 3,333 (18) | |
Length of stay, median (IQR) | 2 (04.5) | 1 (03) | 0.0003 |
Inpatient medicine | 4 (26) | 3 (15) | 0.0020 |
Inpatient surgery | 3 (16) | 2 (14) | 0.0019 |
Charlson Comorbidity Index, median (IQR) | |||
Inpatient medicine | 1 (04) | 1 (02) | 0.0435 |
Inpatient surgery | 0 (01) | 0 (01) | 0.0240 |
The median time from hospital discharge to the call was 3 days (interquartile range [IQR], 16), but 31% and 47% of calls occurred within 24 or 48 hours of discharge, respectively. Ten percent of patients called the AL the same day of discharge (Figure 1). We found no difference in timing of the calls as a function of discharging service.
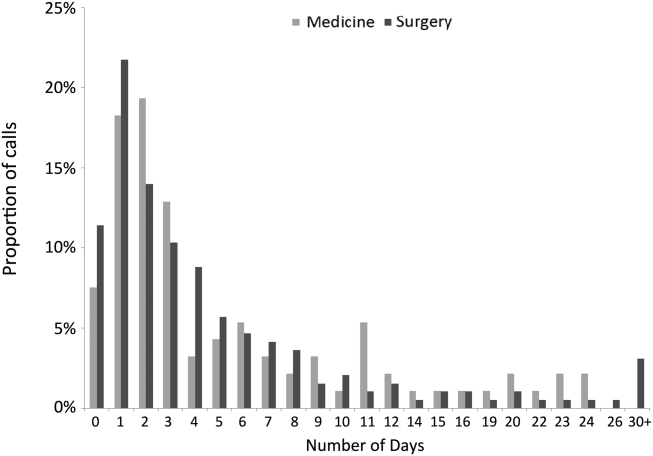
The 308 patients reported a total of 612 problems or concerns (meanstandard deviation number of complaints per caller=21), the large majority of which (71%) were symptom‐related (Table 2). The most common symptom was uncontrolled pain, reported by 33% and 40% of patients discharged from medicine and surgery services, respectively. The next most common symptoms related to the gastrointestinal system and to surgical site issues in medicine and surgery patients, respectively (data not shown).
Total Cohort, n (%) | Patients Discharged From Medicine, n (%) | Patients Discharged From Surgery, n (%) | ||||
---|---|---|---|---|---|---|
Patients | Complaints | Patients | Complaints | Patients | Complaints | |
Symptom related | 280 (91) | 433 (71) | 89 (96) | 166 (77) | 171 (89) | 234 (66) |
Discharge instructions | 65 (21) | 81 (13) | 18 (19) | 21 (10) | 43 (22) | 56 (16) |
Medication related | 65 (21) | 87 (14) | 19 (20) | 25 (11) | 39 (20) | 54 (15) |
Other | 10 (3) | 11 (2) | 4 (4) | 4 (2) | 6 (3) | 7 (2) |
Total | 612 (100) | 216 (100) | 351 (100) |
Sixty‐five patients, representing 21% of the cohort, reported 81 problems understanding or executing discharge instructions. No difference was observed between the fraction of these problems reported by patients from medicine versus surgery (19% and 22%, respectively, P=0.54).
Sixty‐five patients, again representing 21% of the cohort, reported 87 medication‐related problems, 20% from both the medicine and surgery services (P=0.99). Medicine patients more frequently reported difficulties understanding their medication instructions, whereas surgery patients more frequently reported lack of efficacy of medications, particularly with respect to pain control (data not shown).
Thirty percent of patients who called the AL were advised by the nurse to go to the emergency department immediately. Medicine patients were more likely to be triaged to the emergency department compared with surgery patients (45% vs 22%, P<0.0001).
The 30‐day readmission rates and the rates of unscheduled urgent or emergent care visits were higher for patients calling the AL compared with those who did not call (46/308, 15% vs 706/18,995, 4%, and 92/308, 30% vs 1303/18,995, 7%, respectively, both P<0.0001). Similar differences were found for patients discharged from medicine or surgery services who called the AL compared with those who did not (data not shown, both P<0.0001). The median number of days between AL call and rehospitalization was 0 (IQR, 02) and 1 (IQR, 08) for medicine and surgery patients, respectively. Ninety‐three percent of rehospitalizations were related to the index hospitalization, and 78% of patients who were readmitted had no outpatient encounter in the interim between discharge and rehospitalization.
DISCUSSION
We investigated the source and nature of patient telephone calls to an AL following a hospitalization or surgery, and our data revealed the following important findings: (1) nearly one‐half of the calls to the AL occurred within the first 48 hours following discharge; (2) the majority of the calls came from surgery patients, and a greater fraction of patients discharged from surgery services called the AL than patients discharged from medicine services; (3) the most common issues were uncontrolled pain, questions about medications, and problems understanding or executing aftercare instructions (particularly pertaining to the care of surgical wounds); and (4) patients calling the AL had higher rates of 30‐day rehospitalization and of unscheduled urgent or emergent care visits.
The utilization of our patient‐initiated call line was only 1.5%, which was on the low end of the 1% to 10% reported in the literature.[7, 12] This can be attributed to a number of issues that are specific to our system. First, the discharge instructions provided to our patients stated that they should call their primary care provider or the AL if they had questions. Accordingly, because approximately 50% of our patients had a primary care provider in our system, some may have preferentially contacted their primary care provider rather than the AL. Second, the instructions stated that the patients should call if they were experiencing the symptoms listed on the instruction sheet, so those with other problems/complaints may not have called. Third, AL personnel identified patients as being in our cohort by asking if they had been discharged or underwent a surgical procedure within 30‐days of their call. This may have resulted in the under‐reporting of patients who were hospitalized or had outpatient surgical procedures. Fourth, there may have been a number of characteristics specific to patients in our system that reduced the frequency with which they utilized the AL (eg, access to telephones or other community providers).
Most previous studies of patient‐initiated call lines have included them as part of multi‐intervention pre‐ and/or postdischarge strategies.[7, 8, 9, 10, 11, 12, 13] One prior small study compared the information reported by 37 patients who called an AL with that elicited by nurse‐initiated patient contact.[12] The most frequently reported problems in this study were medication‐related issues (43%). However, this study only included medicine patients and did not document the proportion of calls occurring at various time intervals.
The problems we identified (in both medicine and surgery patients) have previously been described,[2, 3, 4, 13, 14, 15, 16] but all of the studies reporting these problems utilized calls that were initiated by health care providers to patients at various fixed intervals following discharge (ie, 730 days). Most of these used a scripted approach seeking responses to specific questions or outcomes, and the specific timing at which the problems arose was not addressed. In contrast, we examined unsolicited concerns expressed by patients calling an AL following discharge whenever they felt sufficient urgency to address whatever problems or questions arose. We found that a large fraction of calls occurred on the day of or within the first 48 hours following discharge, much earlier than when provider‐initiated calls in the studies cited above occurred. Accordingly, our results cannot be used to compare the utility of patient‐ versus provider‐initiated calls, or to suggest that other hospitals should create an AL system. Rather, we suggest that our findings might be complementary to those reported in studies of provider‐initiated calls and only propose that by examining calls placed by patients to ALs, problems with hospital discharge processes (some of which may result in increased rates of readmission) may be discovered.
The observation that such a large fraction of calls to our AL occurred within the first 48 hours following discharge, together with the fact that many of the questions asked or concerns raised pertained to issues that should have been discussed during the discharge process (eg, pain control, care of surgical wounds), suggests that suboptimal patient education was occurring prior to discharge as was suggested by Henderson and Zernike.[17] This finding has led us to expand our patient education processes prior to discharge on both medicine and surgery services. Because our hospitalists care for approximately 90% of the patients admitted to medicine services and are increasingly involved in the care of patients on surgery services, they are integrally involved with such quality improvement initiatives.
To our knowledge this is the first study in the literature that describes both medicine and surgery patients who call an AL because of problems or questions following hospital discharge, categorizes these problems, determines when the patients called following their discharge, and identifies those who called as being at increased risk for early rehospitalizations and unscheduled urgent or emergent care visits. Given the financial penalties issued to hospitals with high 30‐day readmission rates, these patients may warrant more attention than is customarily available from telephone call lines or during routine outpatient follow‐up. The majority of patients who called our AL had Medicare, Medicaid, or a commercial insurance, and, accordingly, may have been eligible for additional services such as home visits and/or expedited follow‐up appointments.
Our study has a number of limitations. First, it is a single‐center study, so the results might not generalize to other institutions. Second, because the study was performed in a university‐affiliated, public safety‐net hospital, patient characteristics and the rates and types of postdischarge concerns that we observed might differ from those encountered in different types of hospitals and/or from those in nonteaching institutions. We would suggest, however, that the idea of using concerns raised by patients discharged from any type of hospital in calls to ALs may similarly identify problems with that specific hospital's discharge processes. Third, the information collected from the AL came from summaries provided by nurses answering the calls rather than from actual transcripts. This could have resulted in insufficient or incorrect information pertaining to some of the variables assessed in Table 2. The information presented in Table 1, however, was obtained from our data warehouse after matching medical record numbers. Fourth, we could have underestimated the number of patients who had 30‐day rehospitalizations and/or unplanned for urgent or emergent care visits if patients sought care at other hospitals. Fifth, the number of patients calling the AL was too small to allow us to do any type of robust matching or multivariable analysis. Accordingly, the differences that appeared between patients who called and those who did not (ie, English speakers, being medically indigent, the length of stay for the index hospitalization and the discharging service) could be the result of inadequate matching or interactions among the variables. Although matching or multivariate analysis might have yielded different associations between patients who called the AL versus those who did not, those who called the AL still had an increased risk of readmission and urgent or emergent visits and may still benefit from targeted interventions. Finally, the fact that only 1.5% of unique patients who were discharged called the AL could have biased our results. Because only 55% and 53% of the patients who did or did not call the AL, respectively, saw primary care physicians within our system within the 3 years prior to their index hospitalization (P=0.679), the frequency of calls to the AL that we observed could have underestimated the frequency with which patients had contact with other care providers in the community.
In summary, information collected from patient‐initiated calls to our AL identified several aspects of our discharge processes that needed improvement. We concluded that our predischarge educational processes for both medicine and surgery services needed modification, especially with respect to pain management, which problems to expect after hospitalization or surgery, and how to deal with them. The high rates of 30‐day rehospitalization and of unscheduled urgent or emergent care visits among patients calling the AL identifies them as being at increased risk for these outcomes, although the likelihood of these events may be related to factors other than just calling the AL.
The period immediately following hospital discharge is particularly hazardous for patients.[1, 2, 3, 4, 5] Problems occurring after discharge may result in high rates of rehospitalization and unscheduled visits to healthcare providers.[6, 7, 8, 9, 10] Numerous investigators have tried to identify patients who are at increased risk for rehospitalizations within 30 days of discharge, and many studies have examined whether various interventions could decrease these adverse events (summarized in Hansen et al.[11]). An increasing fraction of patients discharged by medicine and surgery services have some or all of their care supervised by hospitalists. Thus, hospitals increasingly look to hospitalists for ways to reduce rehospitalizations.
Patients discharged from our hospital are instructed to call an advice line (AL) if and when questions or concerns arise. Accordingly, we examined when these calls were made and what issues were raised, with the idea that the information collected might identify aspects of our discharge processes that needed improvement.
METHODS
Study Design
We conducted a prospective study of a cohort consisting of all unduplicated patients with a matching medical record number in our data warehouse who called our AL between September 1, 2011 and September 1, 2012, and reported being hospitalized or having surgery (inpatient or outpatient) within 30 days preceding their call. We excluded patients who were incarcerated, those who were transferred from other hospitals, those admitted for routine chemotherapy or emergent dialysis, and those discharged to a skilled nursing facility or hospice. The study involved no intervention. It was approved by the Colorado Multiple Institutional Review Board.
Setting
The study was conducted at Denver Health Medical Center, a 525‐bed, university‐affiliated, public safety‐net hospital. At the time of discharge, all patients were given paperwork that listed the telephone number of the AL and written instructions in English or Spanish telling them to call the AL or their primary care physician if they had any of a list of symptoms that was selected by their discharging physician as being relevant to that specific patient's condition(s).
The AL was established in 1997 to provide medical triage to patients of Denver Health. It operates 24 hours a day, 7 days per week, and receives approximately 100,000 calls per year. A language line service is used with nonEnglish‐speaking callers. Calls are handled by a nurse who, with the assistance of a commercial software program (E‐Centaurus; LVM Systems, Phoenix, AZ) containing clinical algorithms (Schmitt‐Thompson Clinical Content, Windsor, CO), makes a triage recommendation. Nurses rarely contact hospital or clinic physicians to assist with triage decisions.
Variables Assessed
We categorized the nature of the callers' reported problem(s) to the AL using the taxonomy summarized in the online appendix (see Supporting Appendix in the online version of this article). We then queried our data warehouse for each patient's demographic information, patient‐level comorbidities, discharging service, discharge date and diagnoses, hospital length of stay, discharge disposition, and whether they had been hospitalized or sought care in our urgent care center or emergency department within 30 days of discharge. The same variables were collected for all unduplicated patients who met the same inclusion and exclusion criteria and were discharged from Denver Health during the same time period but did not call the AL.
Statistics
Data were analyzed using SAS Enterprise Guide 4.1 (SAS Institute, Inc., Cary, NC). Because we made multiple statistical comparisons, we applied the Bonferroni correction when comparing patients calling the AL with those who did not, such that P<0.004 indicated statistical significance. A Student t test or a Wilcoxon rank sum test was used to compare continuous variables depending on results of normality tests. 2 tests were used to compare categorical variables. The intervals between hospital discharge and the call to the AL for patients discharged from medicine versus surgery services were compared using a log‐rank test, with P<0.05 indicating statistical significance.
RESULTS
During the 1‐year study period, 19,303 unique patients were discharged home with instructions regarding the use of the AL. A total of 310 patients called the AL and reported being hospitalized or having surgery within the preceding 30 days. Of these, 2 were excluded (1 who was incarcerated and 1 who was discharged to a skilled nursing facility), leaving 308 patients in the cohort. This represented 1.5% of the total number of unduplicated patients discharged during this same time period (minus the exclusions described above). The large majority of the calls (277/308, 90%) came directly from patients. The remaining 10% came from a proxy, usually a patient's family member. Compared with patients who were discharged during the same time period who did not call the AL, those who called were more likely to speak English, less likely to speak Spanish, more likely to be medically indigent, had slightly longer lengths of stays for their index hospitalization, and were more likely to be discharged from surgery than medicine services (particularly following inpatient surgery) (Table 1).
Patient Characteristics | Patients Calling Advice Line After Discharge, N=308 | Patients Not Calling Advice Line After Discharge, N=18,995 | P Valuea |
---|---|---|---|
| |||
Age, y (meanSD) | 4217 | 3921 | 0.0210 |
Gender, female, n (%) | 162 (53) | 10,655 (56) | |
Race/ethnicity, n (%) | 0.1208 | ||
Hispanic/Latino/Spanish | 129 (42) | 8,896 (47) | |
African American | 44 (14) | 2,674 (14) | |
White | 125 (41) | 6,569 (35) | |
Language, n (%) | <0.0001 | ||
English | 273 (89) | 14,236 (79) | |
Spanish | 32 (10) | 3,744 (21) | |
Payer, n (%) | |||
Medicare | 45 (15) | 3,013 (16) | |
Medicaid | 105 (34) | 7,777 (41) | 0.0152 |
Commercial | 49 (16) | 2,863 (15) | |
Medically indigentb | 93 (30) | 3,442 (18) | <0.0001 |
Self‐pay | 5 (1) | 1,070 (5) | |
Primary care provider, n (%)c | 168 (55) | 10,136 (53) | 0.6794 |
Psychiatric comorbidity, n (%) | 81 (26) | 4,528 (24) | 0.3149 |
Alcohol or substance abuse comorbidity, n (%) | 65 (21) | 3,178 (17) | 0.0417 |
Discharging service, n (%) | <0.0001 | ||
Surgery | 193 (63) | 7,247 (38) | |
Inpatient | 123 (40) | 3,425 (18) | |
Ambulatory | 70 (23) | 3,822 (20) | |
Medicine | 93 (30) | 6,038 (32) | |
Pediatric | 4 (1) | 1,315 (7) | |
Obstetric | 11 (4) | 3,333 (18) | |
Length of stay, median (IQR) | 2 (04.5) | 1 (03) | 0.0003 |
Inpatient medicine | 4 (26) | 3 (15) | 0.0020 |
Inpatient surgery | 3 (16) | 2 (14) | 0.0019 |
Charlson Comorbidity Index, median (IQR) | |||
Inpatient medicine | 1 (04) | 1 (02) | 0.0435 |
Inpatient surgery | 0 (01) | 0 (01) | 0.0240 |
The median time from hospital discharge to the call was 3 days (interquartile range [IQR], 16), but 31% and 47% of calls occurred within 24 or 48 hours of discharge, respectively. Ten percent of patients called the AL the same day of discharge (Figure 1). We found no difference in timing of the calls as a function of discharging service.
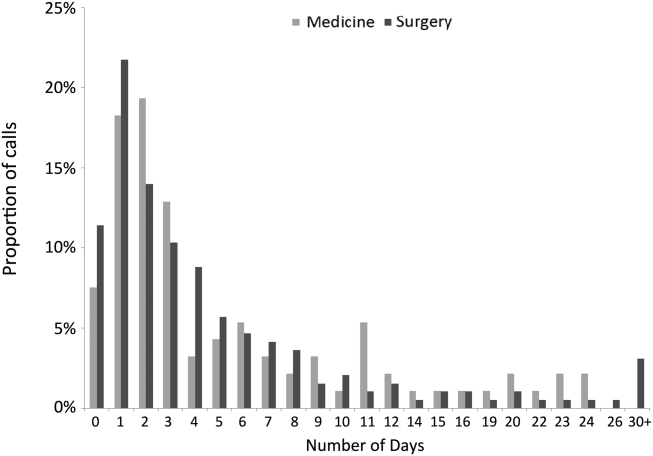
The 308 patients reported a total of 612 problems or concerns (meanstandard deviation number of complaints per caller=21), the large majority of which (71%) were symptom‐related (Table 2). The most common symptom was uncontrolled pain, reported by 33% and 40% of patients discharged from medicine and surgery services, respectively. The next most common symptoms related to the gastrointestinal system and to surgical site issues in medicine and surgery patients, respectively (data not shown).
Total Cohort, n (%) | Patients Discharged From Medicine, n (%) | Patients Discharged From Surgery, n (%) | ||||
---|---|---|---|---|---|---|
Patients | Complaints | Patients | Complaints | Patients | Complaints | |
Symptom related | 280 (91) | 433 (71) | 89 (96) | 166 (77) | 171 (89) | 234 (66) |
Discharge instructions | 65 (21) | 81 (13) | 18 (19) | 21 (10) | 43 (22) | 56 (16) |
Medication related | 65 (21) | 87 (14) | 19 (20) | 25 (11) | 39 (20) | 54 (15) |
Other | 10 (3) | 11 (2) | 4 (4) | 4 (2) | 6 (3) | 7 (2) |
Total | 612 (100) | 216 (100) | 351 (100) |
Sixty‐five patients, representing 21% of the cohort, reported 81 problems understanding or executing discharge instructions. No difference was observed between the fraction of these problems reported by patients from medicine versus surgery (19% and 22%, respectively, P=0.54).
Sixty‐five patients, again representing 21% of the cohort, reported 87 medication‐related problems, 20% from both the medicine and surgery services (P=0.99). Medicine patients more frequently reported difficulties understanding their medication instructions, whereas surgery patients more frequently reported lack of efficacy of medications, particularly with respect to pain control (data not shown).
Thirty percent of patients who called the AL were advised by the nurse to go to the emergency department immediately. Medicine patients were more likely to be triaged to the emergency department compared with surgery patients (45% vs 22%, P<0.0001).
The 30‐day readmission rates and the rates of unscheduled urgent or emergent care visits were higher for patients calling the AL compared with those who did not call (46/308, 15% vs 706/18,995, 4%, and 92/308, 30% vs 1303/18,995, 7%, respectively, both P<0.0001). Similar differences were found for patients discharged from medicine or surgery services who called the AL compared with those who did not (data not shown, both P<0.0001). The median number of days between AL call and rehospitalization was 0 (IQR, 02) and 1 (IQR, 08) for medicine and surgery patients, respectively. Ninety‐three percent of rehospitalizations were related to the index hospitalization, and 78% of patients who were readmitted had no outpatient encounter in the interim between discharge and rehospitalization.
DISCUSSION
We investigated the source and nature of patient telephone calls to an AL following a hospitalization or surgery, and our data revealed the following important findings: (1) nearly one‐half of the calls to the AL occurred within the first 48 hours following discharge; (2) the majority of the calls came from surgery patients, and a greater fraction of patients discharged from surgery services called the AL than patients discharged from medicine services; (3) the most common issues were uncontrolled pain, questions about medications, and problems understanding or executing aftercare instructions (particularly pertaining to the care of surgical wounds); and (4) patients calling the AL had higher rates of 30‐day rehospitalization and of unscheduled urgent or emergent care visits.
The utilization of our patient‐initiated call line was only 1.5%, which was on the low end of the 1% to 10% reported in the literature.[7, 12] This can be attributed to a number of issues that are specific to our system. First, the discharge instructions provided to our patients stated that they should call their primary care provider or the AL if they had questions. Accordingly, because approximately 50% of our patients had a primary care provider in our system, some may have preferentially contacted their primary care provider rather than the AL. Second, the instructions stated that the patients should call if they were experiencing the symptoms listed on the instruction sheet, so those with other problems/complaints may not have called. Third, AL personnel identified patients as being in our cohort by asking if they had been discharged or underwent a surgical procedure within 30‐days of their call. This may have resulted in the under‐reporting of patients who were hospitalized or had outpatient surgical procedures. Fourth, there may have been a number of characteristics specific to patients in our system that reduced the frequency with which they utilized the AL (eg, access to telephones or other community providers).
Most previous studies of patient‐initiated call lines have included them as part of multi‐intervention pre‐ and/or postdischarge strategies.[7, 8, 9, 10, 11, 12, 13] One prior small study compared the information reported by 37 patients who called an AL with that elicited by nurse‐initiated patient contact.[12] The most frequently reported problems in this study were medication‐related issues (43%). However, this study only included medicine patients and did not document the proportion of calls occurring at various time intervals.
The problems we identified (in both medicine and surgery patients) have previously been described,[2, 3, 4, 13, 14, 15, 16] but all of the studies reporting these problems utilized calls that were initiated by health care providers to patients at various fixed intervals following discharge (ie, 730 days). Most of these used a scripted approach seeking responses to specific questions or outcomes, and the specific timing at which the problems arose was not addressed. In contrast, we examined unsolicited concerns expressed by patients calling an AL following discharge whenever they felt sufficient urgency to address whatever problems or questions arose. We found that a large fraction of calls occurred on the day of or within the first 48 hours following discharge, much earlier than when provider‐initiated calls in the studies cited above occurred. Accordingly, our results cannot be used to compare the utility of patient‐ versus provider‐initiated calls, or to suggest that other hospitals should create an AL system. Rather, we suggest that our findings might be complementary to those reported in studies of provider‐initiated calls and only propose that by examining calls placed by patients to ALs, problems with hospital discharge processes (some of which may result in increased rates of readmission) may be discovered.
The observation that such a large fraction of calls to our AL occurred within the first 48 hours following discharge, together with the fact that many of the questions asked or concerns raised pertained to issues that should have been discussed during the discharge process (eg, pain control, care of surgical wounds), suggests that suboptimal patient education was occurring prior to discharge as was suggested by Henderson and Zernike.[17] This finding has led us to expand our patient education processes prior to discharge on both medicine and surgery services. Because our hospitalists care for approximately 90% of the patients admitted to medicine services and are increasingly involved in the care of patients on surgery services, they are integrally involved with such quality improvement initiatives.
To our knowledge this is the first study in the literature that describes both medicine and surgery patients who call an AL because of problems or questions following hospital discharge, categorizes these problems, determines when the patients called following their discharge, and identifies those who called as being at increased risk for early rehospitalizations and unscheduled urgent or emergent care visits. Given the financial penalties issued to hospitals with high 30‐day readmission rates, these patients may warrant more attention than is customarily available from telephone call lines or during routine outpatient follow‐up. The majority of patients who called our AL had Medicare, Medicaid, or a commercial insurance, and, accordingly, may have been eligible for additional services such as home visits and/or expedited follow‐up appointments.
Our study has a number of limitations. First, it is a single‐center study, so the results might not generalize to other institutions. Second, because the study was performed in a university‐affiliated, public safety‐net hospital, patient characteristics and the rates and types of postdischarge concerns that we observed might differ from those encountered in different types of hospitals and/or from those in nonteaching institutions. We would suggest, however, that the idea of using concerns raised by patients discharged from any type of hospital in calls to ALs may similarly identify problems with that specific hospital's discharge processes. Third, the information collected from the AL came from summaries provided by nurses answering the calls rather than from actual transcripts. This could have resulted in insufficient or incorrect information pertaining to some of the variables assessed in Table 2. The information presented in Table 1, however, was obtained from our data warehouse after matching medical record numbers. Fourth, we could have underestimated the number of patients who had 30‐day rehospitalizations and/or unplanned for urgent or emergent care visits if patients sought care at other hospitals. Fifth, the number of patients calling the AL was too small to allow us to do any type of robust matching or multivariable analysis. Accordingly, the differences that appeared between patients who called and those who did not (ie, English speakers, being medically indigent, the length of stay for the index hospitalization and the discharging service) could be the result of inadequate matching or interactions among the variables. Although matching or multivariate analysis might have yielded different associations between patients who called the AL versus those who did not, those who called the AL still had an increased risk of readmission and urgent or emergent visits and may still benefit from targeted interventions. Finally, the fact that only 1.5% of unique patients who were discharged called the AL could have biased our results. Because only 55% and 53% of the patients who did or did not call the AL, respectively, saw primary care physicians within our system within the 3 years prior to their index hospitalization (P=0.679), the frequency of calls to the AL that we observed could have underestimated the frequency with which patients had contact with other care providers in the community.
In summary, information collected from patient‐initiated calls to our AL identified several aspects of our discharge processes that needed improvement. We concluded that our predischarge educational processes for both medicine and surgery services needed modification, especially with respect to pain management, which problems to expect after hospitalization or surgery, and how to deal with them. The high rates of 30‐day rehospitalization and of unscheduled urgent or emergent care visits among patients calling the AL identifies them as being at increased risk for these outcomes, although the likelihood of these events may be related to factors other than just calling the AL.
- Implementation of the care transitions intervention: sustainability and lessons learned. Prof Case Manag. 2009;14(6):282–293. , , , , .
- Problems after discharge and understanding of communication with their primary care physicians among hospitalized seniors: a mixed methods study. J Hosp Med. 2010;5(7):385–391. , , , et al.
- Adverse events among medical patients after discharge from hospital. CMAJ. 2004;170(3):345–349. , , , et al.
- The incidence and severity of adverse events affecting patients after discharge from the hospital. Ann Intern Med. 2003;138(3):161–167. , , , , .
- Post‐hospitalization transitions: examining the effects of timing of primary care provider follow‐up. J Hosp Med. 2010;5(7):392–397. , , .
- Telephone follow‐up after discharge from the hospital: does it make a difference? Appl Nurs Res. 1996;9(2) 47–52. , , , .
- The effect of real‐time teleconsultations between hospital‐based nurses and patients with severe COPD discharged after an exacerbation. J Telemed Telecare. 2013;19(8):466–474. , , , et al.
- A randomized, controlled trial of an intensive community nurse‐supported discharge program in preventing hospital readmissions of older patients with chronic lung disease. J Am Geriatr Soc. 2004;52(8):1240–1246. , , , , , .
- Effects of education and support on self‐care and resource utilization in patients with heart failure. Eur Heart J. 1999;20(9):673–682. , , , et al.
- Comprehensive discharge planning and home follow‐up of hospitalized elders: a randomized clinical trial. JAMA. 1999;281(7):613–620. , , , et al.
- Interventions to reduce 30‐day rehospitalization: a systematic review. Ann Intern Med. 2011;155(8):520–528. , , , , .
- Complementary telephone strategies to improve postdischarge communication. Am J Med. 2012;125(1):28–30. , , , .
- Integrated postdischarge transitional care in a hospitalist system to improve discharge outcome: an experimental study. BMC Med. 2011;9:96. , , , , , .
- Patient experiences after hospitalizations for elective surgery. Am J Surg. 2014;207(6):855–862. , , , , , .
- Complications after discharge for surgical patients. ANZ J Surg. 2004;74(3):92–97. , , .
- Surgeons are overlooking post‐discharge complications: a prospective cohort study. World J Surg. 2014;38(5):1019–1025. , , , .
- A study of the impact of discharge information for surgical patients. J Adv Nurs. 2001;35(3):435–441. , .
- Implementation of the care transitions intervention: sustainability and lessons learned. Prof Case Manag. 2009;14(6):282–293. , , , , .
- Problems after discharge and understanding of communication with their primary care physicians among hospitalized seniors: a mixed methods study. J Hosp Med. 2010;5(7):385–391. , , , et al.
- Adverse events among medical patients after discharge from hospital. CMAJ. 2004;170(3):345–349. , , , et al.
- The incidence and severity of adverse events affecting patients after discharge from the hospital. Ann Intern Med. 2003;138(3):161–167. , , , , .
- Post‐hospitalization transitions: examining the effects of timing of primary care provider follow‐up. J Hosp Med. 2010;5(7):392–397. , , .
- Telephone follow‐up after discharge from the hospital: does it make a difference? Appl Nurs Res. 1996;9(2) 47–52. , , , .
- The effect of real‐time teleconsultations between hospital‐based nurses and patients with severe COPD discharged after an exacerbation. J Telemed Telecare. 2013;19(8):466–474. , , , et al.
- A randomized, controlled trial of an intensive community nurse‐supported discharge program in preventing hospital readmissions of older patients with chronic lung disease. J Am Geriatr Soc. 2004;52(8):1240–1246. , , , , , .
- Effects of education and support on self‐care and resource utilization in patients with heart failure. Eur Heart J. 1999;20(9):673–682. , , , et al.
- Comprehensive discharge planning and home follow‐up of hospitalized elders: a randomized clinical trial. JAMA. 1999;281(7):613–620. , , , et al.
- Interventions to reduce 30‐day rehospitalization: a systematic review. Ann Intern Med. 2011;155(8):520–528. , , , , .
- Complementary telephone strategies to improve postdischarge communication. Am J Med. 2012;125(1):28–30. , , , .
- Integrated postdischarge transitional care in a hospitalist system to improve discharge outcome: an experimental study. BMC Med. 2011;9:96. , , , , , .
- Patient experiences after hospitalizations for elective surgery. Am J Surg. 2014;207(6):855–862. , , , , , .
- Complications after discharge for surgical patients. ANZ J Surg. 2004;74(3):92–97. , , .
- Surgeons are overlooking post‐discharge complications: a prospective cohort study. World J Surg. 2014;38(5):1019–1025. , , , .
- A study of the impact of discharge information for surgical patients. J Adv Nurs. 2001;35(3):435–441. , .
© 2014 Society of Hospital Medicine
Study of Antimicrobial Scrubs
Healthcare workers' (HCWs) attire becomes contaminated with bacterial pathogens during the course of the workday,[1, 2, 3, 4, 5, 6, 7, 8, 9, 10, 11, 12] and Munoz‐Price et al.[13] recently demonstrated that finding bacterial pathogens on HCWs' white coats correlated with finding the same pathogens on their hands. Because of concern for an association between attire colonization and nosocomial infection, governmental agencies in England and Scotland banned HCWs from wearing white coats or long‐sleeve garments,[14, 15] despite evidence that such an approach does not reduce contamination.[12]
Newly developed antimicrobial textiles have been incorporated into HCW scrubs,[16, 17, 18, 19, 20] and commercial Web sites and product inserts report that these products can reduce bacterial contamination by 80.9% at 8 hours to greater than 99% under laboratory conditions depending on the product and microbe studied.[16, 17, 19] Because there are limited clinical data pertaining to the effectiveness of antimicrobial scrubs, we performed a prospective study designed to determine whether wearing these products reduced bacterial contamination of HCWs' scrubs or skin at the end of an 8‐hour workday.
METHODS
Design
The study was a prospective, unblinded, randomized, controlled trial that was approved by the Colorado Multiple Institutional Review Board and conducted at Denver Health, a university‐affiliated public safety net hospital. No protocol changes occurred during the study.
Participants
Participants included hospitalist physicians, internal medicine residents, physician assistants, nurse practitioners, and nurses who directly cared for patients hospitalized on internal medicine units between March 12, 2012 and August 28, 2012. Participants known to be pregnant or those who refused to participate in the study were excluded.
Intervention
Standard scrubs issued by the hospital were tested along with 2 different antimicrobial scrubs (scrub A and scrub B). Scrub A was made with a polyester microfiber material embedded with a proprietary antimicrobial chemical. Scrub B was a polyestercotton blend scrub that included 2 proprietary antimicrobial chemicals and silver embedded into the fabric. The standard scrub was made of a polyestercotton blend with no antimicrobial properties. All scrubs consisted of pants and a short‐sleeved shirt, with either a pocket at the left breast or lower front surface, and all were tested new prior to any washing or wear. Preliminary cultures were done on 2 scrubs in each group to assess the extent of preuse contamination. All providers were instructed not to wear white coats at any time during the day that they were wearing the scrubs. Providers were not told the type of scrub they received, but the antimicrobial scrubs had a different appearance and texture than the standard scrubs, so blinding was not possible.
Outcomes
The primary end point was the total bacterial colony count of samples obtained from the breast or lower front pocket, the sleeve cuff of the dominant hand, and the pant leg at the midthigh of the dominant leg on all scrubs after an 8‐hour workday. Secondary outcomes were the bacterial colony counts of cultures obtained from the volar surface of the wrists of the HCWs' dominant arm, and the colony counts of methicillin‐resistant Staphylococcus aureus (MRSA), vancomycin‐resistant enterococci (VRE), and resistant Gram‐negative bacteria on the 3 scrub types, all obtained after the 8‐hour workday.
Cultures were collected using a standardized RODAC imprint method[21] with BBL RODAC plates containing blood agar (Becton Dickinson, Sparks, MD). Cultures were incubated in ambient air at 35 to 37C for 18 to 22 hours. After incubation, visible colonies were counted using a dissecting microscope to a maximum of 200 colonies as recommended by the manufacturer. Colonies morphologically consistent with Staphylococcus species were subsequently tested for coagulase using a BactiStaph rapid latex agglutination test (Remel, Lenexa, KS). If positive, these colonies were subcultured to sheep blood agar (Remel) and BBL MRSA CHROMagar (Becton Dickinson) and incubated for an additional 18 to 24 hours. Characteristic growth on blood agar that also produced mauve‐colored colonies on CHROMagar was taken to indicate MRSA. Colonies morphologically suspicious for being VRE were identified and confirmed as VRE using a positive identification and susceptibility panel (Microscan; Siemens, Deerfield, IL). A negative combination panel (Microscan, Siemens) was also used to identify and confirm resistant Gram‐negative rods.
Each participant completed a survey that included questions that identified their occupation, whether they had had contact with patients who were known to be colonized or infected with MRSA, VRE, or resistant Gram‐negative rods during the testing period, and whether they experienced any adverse events that might relate to wearing the uniform.
Sample Size
We assumed that cultures taken from the sleeve of the control scrubs would have a mean ( standard deviation) colony count of 69 (67) based on data from our previous study.[12] Although the companies making the antimicrobial scrubs indicated that their respective products provided between 80.9% at 8 hours and >99% reduction in bacterial colony counts in laboratory settings, we assumed that a 70% decrease in colony count compared with standard scrubs could be clinically important. After adjusting for multiple comparisons and accounting for using nonparametric analyses with an unknown distribution, we estimated a need to recruit 35 subjects in each of 3 groups.
Randomization
The principal investigator and coinvestigators enrolled and consented participants. After obtaining consent, block randomization, stratified by occupation, occurred 1 day prior to the study using a computer‐generated table of random numbers.
Statistics
Data were collected and managed using REDCap (Research Electronic Data Capture; Vanderbilt UniversityThe Institute for Medicine and Public Health, Nashville, TN) electronic data capture tools hosted at Denver Health. REDCap is a secure Web‐based application designed to support data collection for research studies, providing: (1) an intuitive interface for validated data entry, (2) audit trails for tracking data manipulation and export procedures, (3) automated export procedures for seamless data downloads to common statistical packages, and (4) procedures for importing data from external sources.[22]
Colony counts were compared using a Kruskal‐Wallis 1‐way analysis of variance by ranks. Bonferroni's correction for multiple comparisons resulted in a P<0.01 as indicating statistical significance. Proportions were compared using [2] analysis. All data are presented as medians with interquartile range (IQR) or proportions.
RESULTS
We screened 118 HCWs for participation and randomized 109, 37 in the control and antimicrobial scrub group A, and 35 in antimicrobial scrub group B (during the course of the study we neglected to culture the pockets of 2 participants in the standard scrub group and 2 in antimicrobial scrub group A). Because our primary end point was total colony count from cultures taken from 3 sites, data from these 4 subjects could not be used, and all the data from these 4 subjects were excluded from the primary analysis; 4 additional subjects were subsequently recruited allowing us to meet our block enrollment target (Figure 1). The first and last participants were studied on March 12, 2012 and August 28, 2012, respectively. The trial ended once the defined number of participants was enrolled. The occupations of the 105 participants are summarized in Table 1.
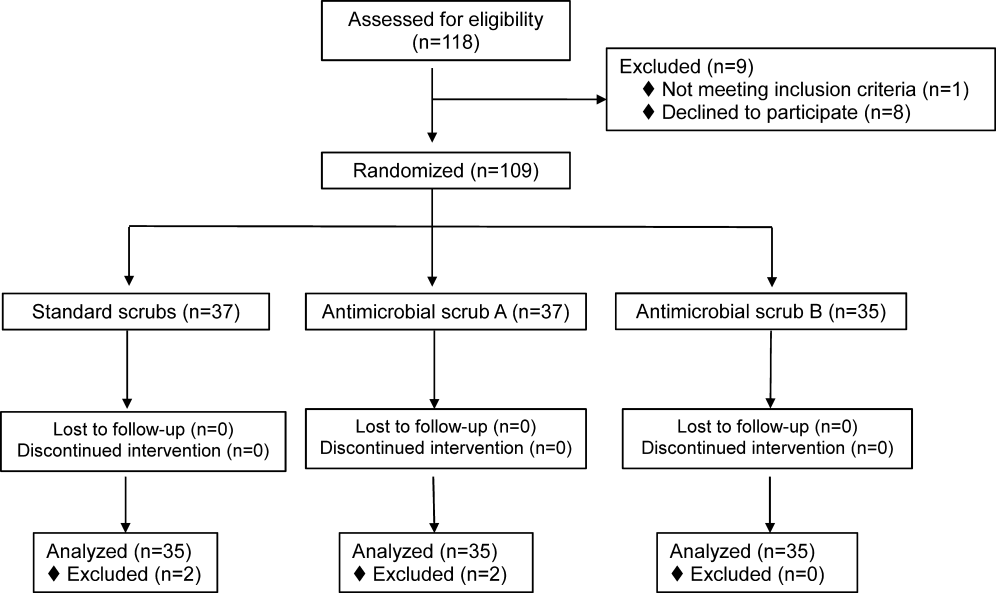
All Subjects, N=105 | Standard Scrub, n=35 | Antimicrobial Scrub A, n=35 | Antimicrobial Scrub B, n=35 | |
---|---|---|---|---|
Healthcare worker type, n (%) | ||||
Attending physician | 11 (10) | 5 (14) | 3 (9) | 3 (9) |
Intern/resident | 51 (49) | 17 (49) | 16 (46) | 18 (51) |
Midlevels | 6 (6) | 2 (6) | 2 (6) | 2 (6) |
Nurse | 37 (35) | 11 (31) | 14 (40) | 12 (34) |
Cared for colonized or infected patient with antibiotic resistant organism, n (%) | 55 (52) | 16 (46) | 20 (57) | 19 (54) |
Number of colonized or infected patients cared for, n (%) | ||||
1 | 37 (67) | 10 (63) | 13 (65) | 14 (74) |
2 | 11 (20) | 4 (25) | 6 (30) | 1 (5) |
3 or more | 6 (11) | 2 (12) | 1 (5) | 3 (16) |
Unknown | 1 (2) | 0 (0) | 0 (0) | 1 (5) |
Colony counts of all scrubs cultured prior to use never exceeded 10 colonies. The median (IQR) total colony counts from all sites on the scrubs was 99 (66182) for standard scrubs, 137 (84289) for antimicrobial scrub type A, and 138 (62274) for antimicrobial scrub type B (P=0.36). We found no significant differences between the colony counts cultured from any of the individual sites among the 3 groups, regardless of occupation (Table 2). No significant difference was observed with respect to colony counts cultured from the wrist among the 3 study groups (Table 2). Comparisons between groups were planned a priori if a difference across all groups was found. Given the nonsignificant P values across all scrub groups, no further comparisons were made.
Total (From All Sites on Scrubs) | Sleeve Cuff | Thigh | Wrist | ||
---|---|---|---|---|---|
| |||||
All subjects, N=105 | |||||
Standard scrub | 99 (66182) | 41 (2070) | 20 (944) | 32 (2161) | 16 (540) |
Antimicrobial scrub A | 137 (84289) | 65 (35117) | 33 (16124) | 41 (1586) | 23 (442) |
Antimicrobial scrub B | 138 (62274) | 41 (2299) | 21 (941) | 40 (18107) | 15 (654) |
P value | 0.36 | 0.17 | 0.07 | 0.57 | 0.92 |
Physicians and midlevels, n=68 | |||||
Standard scrub | 115.5 (72.5173.5) | 44.5 (2270.5) | 27.5 (10.538.5) | 35 (2362.5) | 24.5 (755) |
Antimicrobial scrub A | 210 (114289) | 86 (64120) | 39 (18129) | 49 (2486) | 24 (342) |
Antimicrobial scrub B | 149 (68295) | 52 (26126) | 21 (1069) | 37 (18141) | 19 (872) |
P value | 0.21 | 0.08 | 0.19 | 0.85 | 0.76 |
Nurses, n=37 | |||||
Standard scrub | 89 (31236) | 37 (1348) | 13 (552) | 28 (1342) | 9 (321) |
Antimicrobial scrub A | 105 (43256) | 45.5 (2258) | 21.5 (1654) | 38.5 (1268) | 17 (643) |
Antimicrobial scrub B | 91.5 (60174.5) | 27 (1340) | 16 (7.526) | 51 (2186.5) | 10 (3.543.5) |
P value | 0.86 | 0.39 | 0.19 | 0.49 | 0.41 |
Fifty‐five participants (52%) reported caring for patients who were known to be colonized or infected with an antibiotic‐resistant organism, 16 (46%) randomized to wear standard scrubs, and 20 (57%) and 19 (54%) randomized to wear antimicrobial scrub A or B, respectively (P=0.61). Of these, however, antibiotic‐resistant organisms were only cultured from the scrubs of 2 providers (1 with 1 colony of MRSA from the breast pocket of antimicrobial scrub A, 1 with 1 colony of MRSA cultured from the pocket of antimicrobial scrub B [P=0.55]), and from the wrist of only 1 provider (a multiresistant Gram‐negative rod who wore antimicrobial scrub B).
Adverse Events
Six subjects (5.7%) reported adverse events, all of whom were wearing antimicrobial scrubs (P=0.18). For participants wearing antimicrobial scrub A, 1 (3%) reported itchiness and 2 (6%) reported heaviness or poor breathability. For participants wearing antimicrobial scrub B, 1 (3%) reported redness, 1 (3%) reported itchiness, and 1 (3%) reported heaviness or poor breathability.
DISCUSSION
The important findings of this study are that we found no evidence indicating that either of the 2 antimicrobial scrubs tested reduced bacterial contamination or antibiotic‐resistant contamination on HCWs' scrubs or wrists compared with standard scrubs at the end of an 8‐hour workday, and that despite many HCWs being exposed to patients who were colonized or infected with antibiotic‐resistant bacteria, these organisms were only rarely cultured from their uniforms.
We found that HCWs in all 3 arms of the study had bacterial contamination on their scrubs and skin, consistent with previous studies showing that HCWs' uniforms are frequently contaminated with bacteria, including MRSA, VRE, and other pathogens.[1, 2, 3, 4, 5, 6, 7, 8, 9, 10, 11, 12] We previously found that bacterial contamination of HCWs' uniforms occurs within hours of putting on newly laundered uniforms.[12]
Literature on the effectiveness of antimicrobial HCW uniforms when tested in clinical settings is limited. Bearman and colleagues[23] recently published the results of a study of 31 subjects who wore either standard or antimicrobial scrubs, crossing over every 4 weeks for 4 months, with random culturing done weekly at the beginning and end of a work shift. Scrubs were laundered an average of 1.5 times/week, but the timing of the laundering relative to when cultures were obtained was not reported. Very few isolates of MRSA, Gram‐negative rods, or VRE were found (only 3.9%, 0.4%, and 0.05% of the 2000 samples obtained, respectively), and no differences were observed with respect to the number of HCWs who had antibiotic‐resistant organisms cultured when they were wearing standard versus antimicrobial scrubs. Those who had MRSA cultured, however, had lower mean log colony counts when they were wearing the antimicrobial scrubs. The small number of samples with positive isolates, together with differences in the extent of before‐shift contamination among groups complicates interpreting these data. The authors concluded that a prospective trial was needed. We attempted to include the scrub studied by Bearman and colleagues[23] in our study, but the company had insufficient stock available at the time we tried to purchase the product.
Gross and colleagues[24] found no difference in the mean colony counts of cultures taken from silver‐impregnated versus standard scrubs in a pilot crossover study done with 10 HCWs (although there were trends toward higher colony counts when the subjects wore antimicrobial scrubs).
Antibiotic‐resistant bacteria were only cultured from 3 participants (2.9%) in our current study, compared to 16% of those randomized to wearing white coats in our previous study and 20% of those randomized to wearing standard scrubs.[12] This difference may be explained by several recent studies reporting that rates of MRSA infections in hospitals are decreasing.[25, 26] The rate of hospital‐acquired MRSA infection or colonization at our own institution decreased 80% from 2007 to 2012. At the times of our previous and current studies, providers were expected to wear gowns and gloves when caring for patients as per standard contact precautions. Rates of infection and colonization of VRE and resistant Gram‐negative rods have remained low at our hospital, and our data are consistent with the rates reported on HCWs' uniforms in other studies.[2, 5, 10]
Only 6 of our subjects reported adverse reactions, but all were wearing antimicrobial scrubs (P=0.18). Several of the participants described that the fabrics of the 2 antimicrobial scrubs were heavier and less breathable than the standard scrubs. We believe this difference is more likely to explain the adverse reactions reported than is any type of reaction to the specific chemicals in the fabrics.
Our study has several limitations. Because it was conducted on the general internal medicine units of a single university‐affiliated public hospital, the results may not generalize to other types of institutions or other inpatient services.
As we previously described,[12] the RODAC imprint method only samples a small area of HCWs' uniforms and thus does not represent total bacterial contamination.[21] We specifically cultured areas that are known to be highly contaminated (ie, sleeve cuffs and pockets). Although imprint methods have limitations (as do other methods for culturing clothing), they have been commonly utilized in studies assessing bacterial contamination of HCW clothing.[2, 3, 5]
Although some of the bacterial load we cultured could have come from the providers themselves, previous studies have shown that 80% to 90% of the resistant bacteria cultured from HCWs' attire come from other sources.[1, 2]
Because our sample size was calculated on the basis of being able to detect a difference of 70% in total bacterial colony count, our study was not large enough to exclude a lower level of effectiveness. However, we saw no trends suggesting the antimicrobial products might have a lower level of effectiveness.
We did not observe the hand‐washing practices of the participants, and accordingly, cannot confirm that these practices were the same in each of our 3 study groups. Intermittent, surreptitious monitoring of hand‐washing practices on our internal medicine units over the last several years has found compliance with hand hygiene recommendations varying from 70% to 90%.
Although the participants in our study were not explicitly told to which scrub they were randomized, the colors, appearances, and textures of the antimicrobial fabrics were different from the standard scrubs such that blinding was impossible. Participants wearing antimicrobial scrubs could have changed their hand hygiene practices (ie, less careful hand hygiene). Lack of blinding could also have led to over‐reporting of adverse events by the subjects randomized to wear the antimicrobial scrubs.
In an effort to treat all the scrubs in the same fashion, all were tested new, prior to being washed or previously worn. Studying the scrubs prior to washing or wearing could have increased the reports of adverse effects, as the fabrics could have been stiffer and more uncomfortable than they might have been at a later stage in their use.
Our study also has some strengths. Our participants included physicians, residents, nurses, nurse practitioners, and physician assistants. Accordingly, our results should be generalizable to most HCWs. We also confirmed that the scrubs that were tested were nearly sterile prior to use.
In conclusion, we found no evidence suggesting that either of 2 antimicrobial scrubs tested decreased bacterial contamination of HCWs' scrubs or skin after an 8‐hour workday compared to standard scrubs. We also found that, although HCWs are frequently exposed to patients harboring antibiotic‐resistant bacteria, these bacteria were only rarely cultured from HCWs' scrubs or skin.
- Contamination of nurses' uniforms with Staphylococcus aureus. Lancet. 1969;2:233–235. , , , .
- Contamination of protective clothing and nurses' uniforms in an isolation ward. J Hosp Infect. 1983;4:149–157. , , .
- Microbial flora on doctors' white coats. BMJ. 1991;303:1602–1604. , , .
- Bacterial contamination of nurses' uniforms: a study. Nursing Stand. 1998;13:37–42. .
- Bacterial flora on the white coats of medical students. J Hosp Infect. 2000;45:65–68. , , .
- Bacterial contamination of uniforms. J Hosp Infect. 2001;48:238–241. , , .
- Significance of methicillin‐resistant Staphylococcus aureus (MRSA) survey in a university teaching hospital. J Infect Chemother. 2003;9:172–177. , , , et al.
- Environmental contamination makes an important contribution to hospital infection. J Hosp Infect. 2007;65(suppl 2):50–54. .
- Detection of methicillin‐resistant Staphylococcus aureus and vancomycin‐resistant enterococci on the gowns and gloves of healthcare workers. Infect Control Hosp Epidemiol. 2008;29:583–589. , , , et al.
- Bacterial contamination of health care workers' white coats. Am J Infect Control. 2009;37:101–105. , , , , , .
- Nursing and physician attire as possible source of nosocomial infections. Am J Infect Control. 2011;39:555–559. , , , , , .
- Newly cleaned physician uniforms and infrequently washed white coats have similar rates of bacterial contamination after an 8‐hour workday: a randomized controlled trial. J Hosp Med. 2011;6:177–182. , , , , , .
- Associations between bacterial contamination of health care workers' hands and contamination of white coats and scrubs. Am J Infect Control. 2012;40:e245–e248. , , , et al.
- Department of Health. Uniforms and workwear: an evidence base for developing local policy. National Health Service, 17 September 2007. Available at: http://www.dh.gov.uk/en/Publicationsandstatistics/Publications/Publicationspolicyandguidance/DH_078433. Accessed January 29, 2010.
- Scottish Government Health Directorates. NHS Scotland dress code. Available at: http://www.sehd.scot.nhs.uk/mels/CEL2008_53.pdf. Accessed February 10, 2010.
- Bio Shield Tech Web site. Bio Gardz–unisex scrub top–antimicrobial treatment. Available at: http://www.bioshieldtech.com/Bio_Gardz_Unisex_Scrub_Top_Antimicrobial_Tre_p/sbt01‐r‐p.htm. Accessed January 9, 2013.
- Doc Froc Web site and informational packet. Available at: http://www.docfroc.com. Accessed July 22, 2011.
- Vestagen Web site and informational packet. Available at: http://www.vestagen.com. Accessed July 22, 2011.
- Under Scrub apparel Web site. Testing. Available at: http://underscrub.com/testing. Accessed March 21, 2013.
- MediThreads Web site. Microban FAQ's. Available at: http://medithreads.com/faq/microban‐faqs. Accessed March 21, 2013.
- Comparison of the Rodac imprint method to selective enrichment broth for recovery of vancomycin‐resistant enterococci and drug‐resistant Enterobacteriaceae from environmental surfaces. J Clin Microbiol. 2000;38:4646–4648. , , , , .
- Research electronic data capture (REDCap)—a metadata‐driven methodology and workflow process for providing translational research informatics support. J Biomed Inform. 2009;42:377–381. , , , , , .
- A crossover trial of antimicrobial scrubs to reduce methicillin‐resistant Staphylococcus aureus burden on healthcare worker apparel. Infect Control Hosp Epidemiol. 2012;33:268–275. , , , et al.
- Pilot study on the microbial contamination of conventional vs. silver‐impregnated uniforms worn by ambulance personnel during one week of emergency medical service. GMS Krankenhhyg Interdiszip. 2010;5.pii: Doc09. , , , , .
- Epidemiology of Staphylococcus aureus blood and skin and soft tissue infections in the US military health system, 2005–2010. JAMA. 2012;308:50–59. , , , et al.
- Health care‐associated invasive MRSA infections, 2005–2008. JAMA. 2010;304:641–648. , , , et al.
Healthcare workers' (HCWs) attire becomes contaminated with bacterial pathogens during the course of the workday,[1, 2, 3, 4, 5, 6, 7, 8, 9, 10, 11, 12] and Munoz‐Price et al.[13] recently demonstrated that finding bacterial pathogens on HCWs' white coats correlated with finding the same pathogens on their hands. Because of concern for an association between attire colonization and nosocomial infection, governmental agencies in England and Scotland banned HCWs from wearing white coats or long‐sleeve garments,[14, 15] despite evidence that such an approach does not reduce contamination.[12]
Newly developed antimicrobial textiles have been incorporated into HCW scrubs,[16, 17, 18, 19, 20] and commercial Web sites and product inserts report that these products can reduce bacterial contamination by 80.9% at 8 hours to greater than 99% under laboratory conditions depending on the product and microbe studied.[16, 17, 19] Because there are limited clinical data pertaining to the effectiveness of antimicrobial scrubs, we performed a prospective study designed to determine whether wearing these products reduced bacterial contamination of HCWs' scrubs or skin at the end of an 8‐hour workday.
METHODS
Design
The study was a prospective, unblinded, randomized, controlled trial that was approved by the Colorado Multiple Institutional Review Board and conducted at Denver Health, a university‐affiliated public safety net hospital. No protocol changes occurred during the study.
Participants
Participants included hospitalist physicians, internal medicine residents, physician assistants, nurse practitioners, and nurses who directly cared for patients hospitalized on internal medicine units between March 12, 2012 and August 28, 2012. Participants known to be pregnant or those who refused to participate in the study were excluded.
Intervention
Standard scrubs issued by the hospital were tested along with 2 different antimicrobial scrubs (scrub A and scrub B). Scrub A was made with a polyester microfiber material embedded with a proprietary antimicrobial chemical. Scrub B was a polyestercotton blend scrub that included 2 proprietary antimicrobial chemicals and silver embedded into the fabric. The standard scrub was made of a polyestercotton blend with no antimicrobial properties. All scrubs consisted of pants and a short‐sleeved shirt, with either a pocket at the left breast or lower front surface, and all were tested new prior to any washing or wear. Preliminary cultures were done on 2 scrubs in each group to assess the extent of preuse contamination. All providers were instructed not to wear white coats at any time during the day that they were wearing the scrubs. Providers were not told the type of scrub they received, but the antimicrobial scrubs had a different appearance and texture than the standard scrubs, so blinding was not possible.
Outcomes
The primary end point was the total bacterial colony count of samples obtained from the breast or lower front pocket, the sleeve cuff of the dominant hand, and the pant leg at the midthigh of the dominant leg on all scrubs after an 8‐hour workday. Secondary outcomes were the bacterial colony counts of cultures obtained from the volar surface of the wrists of the HCWs' dominant arm, and the colony counts of methicillin‐resistant Staphylococcus aureus (MRSA), vancomycin‐resistant enterococci (VRE), and resistant Gram‐negative bacteria on the 3 scrub types, all obtained after the 8‐hour workday.
Cultures were collected using a standardized RODAC imprint method[21] with BBL RODAC plates containing blood agar (Becton Dickinson, Sparks, MD). Cultures were incubated in ambient air at 35 to 37C for 18 to 22 hours. After incubation, visible colonies were counted using a dissecting microscope to a maximum of 200 colonies as recommended by the manufacturer. Colonies morphologically consistent with Staphylococcus species were subsequently tested for coagulase using a BactiStaph rapid latex agglutination test (Remel, Lenexa, KS). If positive, these colonies were subcultured to sheep blood agar (Remel) and BBL MRSA CHROMagar (Becton Dickinson) and incubated for an additional 18 to 24 hours. Characteristic growth on blood agar that also produced mauve‐colored colonies on CHROMagar was taken to indicate MRSA. Colonies morphologically suspicious for being VRE were identified and confirmed as VRE using a positive identification and susceptibility panel (Microscan; Siemens, Deerfield, IL). A negative combination panel (Microscan, Siemens) was also used to identify and confirm resistant Gram‐negative rods.
Each participant completed a survey that included questions that identified their occupation, whether they had had contact with patients who were known to be colonized or infected with MRSA, VRE, or resistant Gram‐negative rods during the testing period, and whether they experienced any adverse events that might relate to wearing the uniform.
Sample Size
We assumed that cultures taken from the sleeve of the control scrubs would have a mean ( standard deviation) colony count of 69 (67) based on data from our previous study.[12] Although the companies making the antimicrobial scrubs indicated that their respective products provided between 80.9% at 8 hours and >99% reduction in bacterial colony counts in laboratory settings, we assumed that a 70% decrease in colony count compared with standard scrubs could be clinically important. After adjusting for multiple comparisons and accounting for using nonparametric analyses with an unknown distribution, we estimated a need to recruit 35 subjects in each of 3 groups.
Randomization
The principal investigator and coinvestigators enrolled and consented participants. After obtaining consent, block randomization, stratified by occupation, occurred 1 day prior to the study using a computer‐generated table of random numbers.
Statistics
Data were collected and managed using REDCap (Research Electronic Data Capture; Vanderbilt UniversityThe Institute for Medicine and Public Health, Nashville, TN) electronic data capture tools hosted at Denver Health. REDCap is a secure Web‐based application designed to support data collection for research studies, providing: (1) an intuitive interface for validated data entry, (2) audit trails for tracking data manipulation and export procedures, (3) automated export procedures for seamless data downloads to common statistical packages, and (4) procedures for importing data from external sources.[22]
Colony counts were compared using a Kruskal‐Wallis 1‐way analysis of variance by ranks. Bonferroni's correction for multiple comparisons resulted in a P<0.01 as indicating statistical significance. Proportions were compared using [2] analysis. All data are presented as medians with interquartile range (IQR) or proportions.
RESULTS
We screened 118 HCWs for participation and randomized 109, 37 in the control and antimicrobial scrub group A, and 35 in antimicrobial scrub group B (during the course of the study we neglected to culture the pockets of 2 participants in the standard scrub group and 2 in antimicrobial scrub group A). Because our primary end point was total colony count from cultures taken from 3 sites, data from these 4 subjects could not be used, and all the data from these 4 subjects were excluded from the primary analysis; 4 additional subjects were subsequently recruited allowing us to meet our block enrollment target (Figure 1). The first and last participants were studied on March 12, 2012 and August 28, 2012, respectively. The trial ended once the defined number of participants was enrolled. The occupations of the 105 participants are summarized in Table 1.
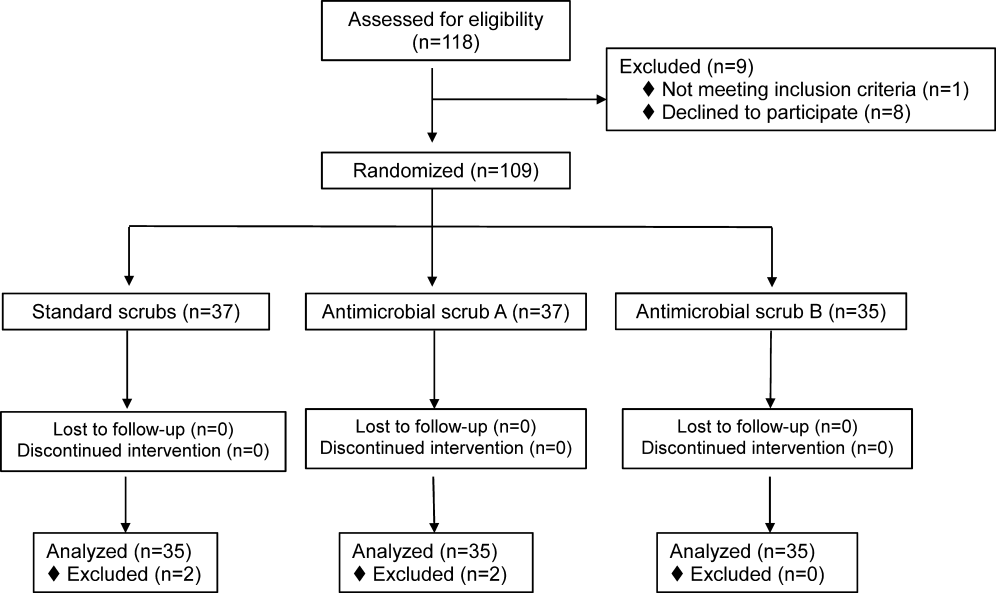
All Subjects, N=105 | Standard Scrub, n=35 | Antimicrobial Scrub A, n=35 | Antimicrobial Scrub B, n=35 | |
---|---|---|---|---|
Healthcare worker type, n (%) | ||||
Attending physician | 11 (10) | 5 (14) | 3 (9) | 3 (9) |
Intern/resident | 51 (49) | 17 (49) | 16 (46) | 18 (51) |
Midlevels | 6 (6) | 2 (6) | 2 (6) | 2 (6) |
Nurse | 37 (35) | 11 (31) | 14 (40) | 12 (34) |
Cared for colonized or infected patient with antibiotic resistant organism, n (%) | 55 (52) | 16 (46) | 20 (57) | 19 (54) |
Number of colonized or infected patients cared for, n (%) | ||||
1 | 37 (67) | 10 (63) | 13 (65) | 14 (74) |
2 | 11 (20) | 4 (25) | 6 (30) | 1 (5) |
3 or more | 6 (11) | 2 (12) | 1 (5) | 3 (16) |
Unknown | 1 (2) | 0 (0) | 0 (0) | 1 (5) |
Colony counts of all scrubs cultured prior to use never exceeded 10 colonies. The median (IQR) total colony counts from all sites on the scrubs was 99 (66182) for standard scrubs, 137 (84289) for antimicrobial scrub type A, and 138 (62274) for antimicrobial scrub type B (P=0.36). We found no significant differences between the colony counts cultured from any of the individual sites among the 3 groups, regardless of occupation (Table 2). No significant difference was observed with respect to colony counts cultured from the wrist among the 3 study groups (Table 2). Comparisons between groups were planned a priori if a difference across all groups was found. Given the nonsignificant P values across all scrub groups, no further comparisons were made.
Total (From All Sites on Scrubs) | Sleeve Cuff | Thigh | Wrist | ||
---|---|---|---|---|---|
| |||||
All subjects, N=105 | |||||
Standard scrub | 99 (66182) | 41 (2070) | 20 (944) | 32 (2161) | 16 (540) |
Antimicrobial scrub A | 137 (84289) | 65 (35117) | 33 (16124) | 41 (1586) | 23 (442) |
Antimicrobial scrub B | 138 (62274) | 41 (2299) | 21 (941) | 40 (18107) | 15 (654) |
P value | 0.36 | 0.17 | 0.07 | 0.57 | 0.92 |
Physicians and midlevels, n=68 | |||||
Standard scrub | 115.5 (72.5173.5) | 44.5 (2270.5) | 27.5 (10.538.5) | 35 (2362.5) | 24.5 (755) |
Antimicrobial scrub A | 210 (114289) | 86 (64120) | 39 (18129) | 49 (2486) | 24 (342) |
Antimicrobial scrub B | 149 (68295) | 52 (26126) | 21 (1069) | 37 (18141) | 19 (872) |
P value | 0.21 | 0.08 | 0.19 | 0.85 | 0.76 |
Nurses, n=37 | |||||
Standard scrub | 89 (31236) | 37 (1348) | 13 (552) | 28 (1342) | 9 (321) |
Antimicrobial scrub A | 105 (43256) | 45.5 (2258) | 21.5 (1654) | 38.5 (1268) | 17 (643) |
Antimicrobial scrub B | 91.5 (60174.5) | 27 (1340) | 16 (7.526) | 51 (2186.5) | 10 (3.543.5) |
P value | 0.86 | 0.39 | 0.19 | 0.49 | 0.41 |
Fifty‐five participants (52%) reported caring for patients who were known to be colonized or infected with an antibiotic‐resistant organism, 16 (46%) randomized to wear standard scrubs, and 20 (57%) and 19 (54%) randomized to wear antimicrobial scrub A or B, respectively (P=0.61). Of these, however, antibiotic‐resistant organisms were only cultured from the scrubs of 2 providers (1 with 1 colony of MRSA from the breast pocket of antimicrobial scrub A, 1 with 1 colony of MRSA cultured from the pocket of antimicrobial scrub B [P=0.55]), and from the wrist of only 1 provider (a multiresistant Gram‐negative rod who wore antimicrobial scrub B).
Adverse Events
Six subjects (5.7%) reported adverse events, all of whom were wearing antimicrobial scrubs (P=0.18). For participants wearing antimicrobial scrub A, 1 (3%) reported itchiness and 2 (6%) reported heaviness or poor breathability. For participants wearing antimicrobial scrub B, 1 (3%) reported redness, 1 (3%) reported itchiness, and 1 (3%) reported heaviness or poor breathability.
DISCUSSION
The important findings of this study are that we found no evidence indicating that either of the 2 antimicrobial scrubs tested reduced bacterial contamination or antibiotic‐resistant contamination on HCWs' scrubs or wrists compared with standard scrubs at the end of an 8‐hour workday, and that despite many HCWs being exposed to patients who were colonized or infected with antibiotic‐resistant bacteria, these organisms were only rarely cultured from their uniforms.
We found that HCWs in all 3 arms of the study had bacterial contamination on their scrubs and skin, consistent with previous studies showing that HCWs' uniforms are frequently contaminated with bacteria, including MRSA, VRE, and other pathogens.[1, 2, 3, 4, 5, 6, 7, 8, 9, 10, 11, 12] We previously found that bacterial contamination of HCWs' uniforms occurs within hours of putting on newly laundered uniforms.[12]
Literature on the effectiveness of antimicrobial HCW uniforms when tested in clinical settings is limited. Bearman and colleagues[23] recently published the results of a study of 31 subjects who wore either standard or antimicrobial scrubs, crossing over every 4 weeks for 4 months, with random culturing done weekly at the beginning and end of a work shift. Scrubs were laundered an average of 1.5 times/week, but the timing of the laundering relative to when cultures were obtained was not reported. Very few isolates of MRSA, Gram‐negative rods, or VRE were found (only 3.9%, 0.4%, and 0.05% of the 2000 samples obtained, respectively), and no differences were observed with respect to the number of HCWs who had antibiotic‐resistant organisms cultured when they were wearing standard versus antimicrobial scrubs. Those who had MRSA cultured, however, had lower mean log colony counts when they were wearing the antimicrobial scrubs. The small number of samples with positive isolates, together with differences in the extent of before‐shift contamination among groups complicates interpreting these data. The authors concluded that a prospective trial was needed. We attempted to include the scrub studied by Bearman and colleagues[23] in our study, but the company had insufficient stock available at the time we tried to purchase the product.
Gross and colleagues[24] found no difference in the mean colony counts of cultures taken from silver‐impregnated versus standard scrubs in a pilot crossover study done with 10 HCWs (although there were trends toward higher colony counts when the subjects wore antimicrobial scrubs).
Antibiotic‐resistant bacteria were only cultured from 3 participants (2.9%) in our current study, compared to 16% of those randomized to wearing white coats in our previous study and 20% of those randomized to wearing standard scrubs.[12] This difference may be explained by several recent studies reporting that rates of MRSA infections in hospitals are decreasing.[25, 26] The rate of hospital‐acquired MRSA infection or colonization at our own institution decreased 80% from 2007 to 2012. At the times of our previous and current studies, providers were expected to wear gowns and gloves when caring for patients as per standard contact precautions. Rates of infection and colonization of VRE and resistant Gram‐negative rods have remained low at our hospital, and our data are consistent with the rates reported on HCWs' uniforms in other studies.[2, 5, 10]
Only 6 of our subjects reported adverse reactions, but all were wearing antimicrobial scrubs (P=0.18). Several of the participants described that the fabrics of the 2 antimicrobial scrubs were heavier and less breathable than the standard scrubs. We believe this difference is more likely to explain the adverse reactions reported than is any type of reaction to the specific chemicals in the fabrics.
Our study has several limitations. Because it was conducted on the general internal medicine units of a single university‐affiliated public hospital, the results may not generalize to other types of institutions or other inpatient services.
As we previously described,[12] the RODAC imprint method only samples a small area of HCWs' uniforms and thus does not represent total bacterial contamination.[21] We specifically cultured areas that are known to be highly contaminated (ie, sleeve cuffs and pockets). Although imprint methods have limitations (as do other methods for culturing clothing), they have been commonly utilized in studies assessing bacterial contamination of HCW clothing.[2, 3, 5]
Although some of the bacterial load we cultured could have come from the providers themselves, previous studies have shown that 80% to 90% of the resistant bacteria cultured from HCWs' attire come from other sources.[1, 2]
Because our sample size was calculated on the basis of being able to detect a difference of 70% in total bacterial colony count, our study was not large enough to exclude a lower level of effectiveness. However, we saw no trends suggesting the antimicrobial products might have a lower level of effectiveness.
We did not observe the hand‐washing practices of the participants, and accordingly, cannot confirm that these practices were the same in each of our 3 study groups. Intermittent, surreptitious monitoring of hand‐washing practices on our internal medicine units over the last several years has found compliance with hand hygiene recommendations varying from 70% to 90%.
Although the participants in our study were not explicitly told to which scrub they were randomized, the colors, appearances, and textures of the antimicrobial fabrics were different from the standard scrubs such that blinding was impossible. Participants wearing antimicrobial scrubs could have changed their hand hygiene practices (ie, less careful hand hygiene). Lack of blinding could also have led to over‐reporting of adverse events by the subjects randomized to wear the antimicrobial scrubs.
In an effort to treat all the scrubs in the same fashion, all were tested new, prior to being washed or previously worn. Studying the scrubs prior to washing or wearing could have increased the reports of adverse effects, as the fabrics could have been stiffer and more uncomfortable than they might have been at a later stage in their use.
Our study also has some strengths. Our participants included physicians, residents, nurses, nurse practitioners, and physician assistants. Accordingly, our results should be generalizable to most HCWs. We also confirmed that the scrubs that were tested were nearly sterile prior to use.
In conclusion, we found no evidence suggesting that either of 2 antimicrobial scrubs tested decreased bacterial contamination of HCWs' scrubs or skin after an 8‐hour workday compared to standard scrubs. We also found that, although HCWs are frequently exposed to patients harboring antibiotic‐resistant bacteria, these bacteria were only rarely cultured from HCWs' scrubs or skin.
Healthcare workers' (HCWs) attire becomes contaminated with bacterial pathogens during the course of the workday,[1, 2, 3, 4, 5, 6, 7, 8, 9, 10, 11, 12] and Munoz‐Price et al.[13] recently demonstrated that finding bacterial pathogens on HCWs' white coats correlated with finding the same pathogens on their hands. Because of concern for an association between attire colonization and nosocomial infection, governmental agencies in England and Scotland banned HCWs from wearing white coats or long‐sleeve garments,[14, 15] despite evidence that such an approach does not reduce contamination.[12]
Newly developed antimicrobial textiles have been incorporated into HCW scrubs,[16, 17, 18, 19, 20] and commercial Web sites and product inserts report that these products can reduce bacterial contamination by 80.9% at 8 hours to greater than 99% under laboratory conditions depending on the product and microbe studied.[16, 17, 19] Because there are limited clinical data pertaining to the effectiveness of antimicrobial scrubs, we performed a prospective study designed to determine whether wearing these products reduced bacterial contamination of HCWs' scrubs or skin at the end of an 8‐hour workday.
METHODS
Design
The study was a prospective, unblinded, randomized, controlled trial that was approved by the Colorado Multiple Institutional Review Board and conducted at Denver Health, a university‐affiliated public safety net hospital. No protocol changes occurred during the study.
Participants
Participants included hospitalist physicians, internal medicine residents, physician assistants, nurse practitioners, and nurses who directly cared for patients hospitalized on internal medicine units between March 12, 2012 and August 28, 2012. Participants known to be pregnant or those who refused to participate in the study were excluded.
Intervention
Standard scrubs issued by the hospital were tested along with 2 different antimicrobial scrubs (scrub A and scrub B). Scrub A was made with a polyester microfiber material embedded with a proprietary antimicrobial chemical. Scrub B was a polyestercotton blend scrub that included 2 proprietary antimicrobial chemicals and silver embedded into the fabric. The standard scrub was made of a polyestercotton blend with no antimicrobial properties. All scrubs consisted of pants and a short‐sleeved shirt, with either a pocket at the left breast or lower front surface, and all were tested new prior to any washing or wear. Preliminary cultures were done on 2 scrubs in each group to assess the extent of preuse contamination. All providers were instructed not to wear white coats at any time during the day that they were wearing the scrubs. Providers were not told the type of scrub they received, but the antimicrobial scrubs had a different appearance and texture than the standard scrubs, so blinding was not possible.
Outcomes
The primary end point was the total bacterial colony count of samples obtained from the breast or lower front pocket, the sleeve cuff of the dominant hand, and the pant leg at the midthigh of the dominant leg on all scrubs after an 8‐hour workday. Secondary outcomes were the bacterial colony counts of cultures obtained from the volar surface of the wrists of the HCWs' dominant arm, and the colony counts of methicillin‐resistant Staphylococcus aureus (MRSA), vancomycin‐resistant enterococci (VRE), and resistant Gram‐negative bacteria on the 3 scrub types, all obtained after the 8‐hour workday.
Cultures were collected using a standardized RODAC imprint method[21] with BBL RODAC plates containing blood agar (Becton Dickinson, Sparks, MD). Cultures were incubated in ambient air at 35 to 37C for 18 to 22 hours. After incubation, visible colonies were counted using a dissecting microscope to a maximum of 200 colonies as recommended by the manufacturer. Colonies morphologically consistent with Staphylococcus species were subsequently tested for coagulase using a BactiStaph rapid latex agglutination test (Remel, Lenexa, KS). If positive, these colonies were subcultured to sheep blood agar (Remel) and BBL MRSA CHROMagar (Becton Dickinson) and incubated for an additional 18 to 24 hours. Characteristic growth on blood agar that also produced mauve‐colored colonies on CHROMagar was taken to indicate MRSA. Colonies morphologically suspicious for being VRE were identified and confirmed as VRE using a positive identification and susceptibility panel (Microscan; Siemens, Deerfield, IL). A negative combination panel (Microscan, Siemens) was also used to identify and confirm resistant Gram‐negative rods.
Each participant completed a survey that included questions that identified their occupation, whether they had had contact with patients who were known to be colonized or infected with MRSA, VRE, or resistant Gram‐negative rods during the testing period, and whether they experienced any adverse events that might relate to wearing the uniform.
Sample Size
We assumed that cultures taken from the sleeve of the control scrubs would have a mean ( standard deviation) colony count of 69 (67) based on data from our previous study.[12] Although the companies making the antimicrobial scrubs indicated that their respective products provided between 80.9% at 8 hours and >99% reduction in bacterial colony counts in laboratory settings, we assumed that a 70% decrease in colony count compared with standard scrubs could be clinically important. After adjusting for multiple comparisons and accounting for using nonparametric analyses with an unknown distribution, we estimated a need to recruit 35 subjects in each of 3 groups.
Randomization
The principal investigator and coinvestigators enrolled and consented participants. After obtaining consent, block randomization, stratified by occupation, occurred 1 day prior to the study using a computer‐generated table of random numbers.
Statistics
Data were collected and managed using REDCap (Research Electronic Data Capture; Vanderbilt UniversityThe Institute for Medicine and Public Health, Nashville, TN) electronic data capture tools hosted at Denver Health. REDCap is a secure Web‐based application designed to support data collection for research studies, providing: (1) an intuitive interface for validated data entry, (2) audit trails for tracking data manipulation and export procedures, (3) automated export procedures for seamless data downloads to common statistical packages, and (4) procedures for importing data from external sources.[22]
Colony counts were compared using a Kruskal‐Wallis 1‐way analysis of variance by ranks. Bonferroni's correction for multiple comparisons resulted in a P<0.01 as indicating statistical significance. Proportions were compared using [2] analysis. All data are presented as medians with interquartile range (IQR) or proportions.
RESULTS
We screened 118 HCWs for participation and randomized 109, 37 in the control and antimicrobial scrub group A, and 35 in antimicrobial scrub group B (during the course of the study we neglected to culture the pockets of 2 participants in the standard scrub group and 2 in antimicrobial scrub group A). Because our primary end point was total colony count from cultures taken from 3 sites, data from these 4 subjects could not be used, and all the data from these 4 subjects were excluded from the primary analysis; 4 additional subjects were subsequently recruited allowing us to meet our block enrollment target (Figure 1). The first and last participants were studied on March 12, 2012 and August 28, 2012, respectively. The trial ended once the defined number of participants was enrolled. The occupations of the 105 participants are summarized in Table 1.
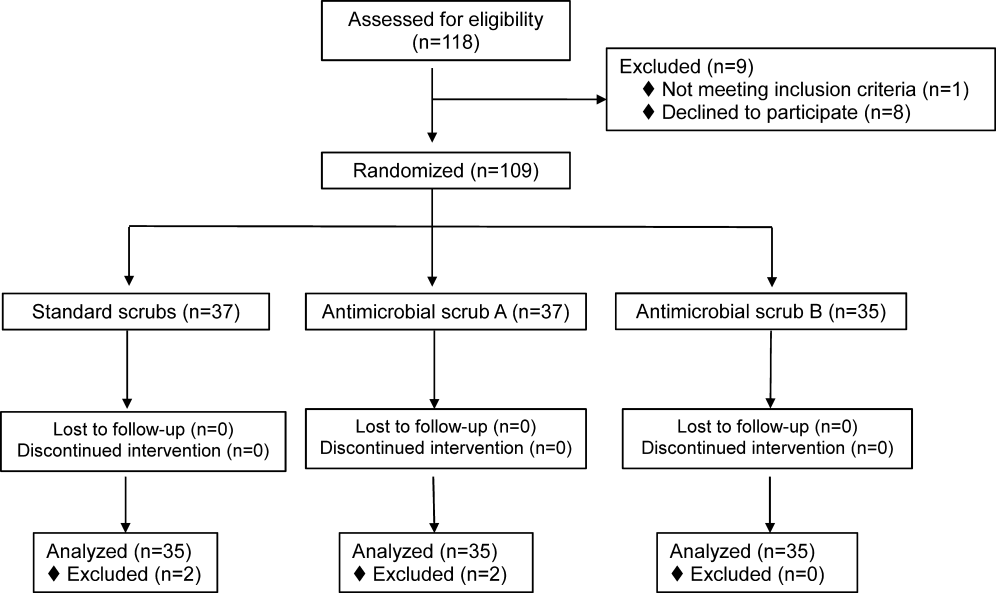
All Subjects, N=105 | Standard Scrub, n=35 | Antimicrobial Scrub A, n=35 | Antimicrobial Scrub B, n=35 | |
---|---|---|---|---|
Healthcare worker type, n (%) | ||||
Attending physician | 11 (10) | 5 (14) | 3 (9) | 3 (9) |
Intern/resident | 51 (49) | 17 (49) | 16 (46) | 18 (51) |
Midlevels | 6 (6) | 2 (6) | 2 (6) | 2 (6) |
Nurse | 37 (35) | 11 (31) | 14 (40) | 12 (34) |
Cared for colonized or infected patient with antibiotic resistant organism, n (%) | 55 (52) | 16 (46) | 20 (57) | 19 (54) |
Number of colonized or infected patients cared for, n (%) | ||||
1 | 37 (67) | 10 (63) | 13 (65) | 14 (74) |
2 | 11 (20) | 4 (25) | 6 (30) | 1 (5) |
3 or more | 6 (11) | 2 (12) | 1 (5) | 3 (16) |
Unknown | 1 (2) | 0 (0) | 0 (0) | 1 (5) |
Colony counts of all scrubs cultured prior to use never exceeded 10 colonies. The median (IQR) total colony counts from all sites on the scrubs was 99 (66182) for standard scrubs, 137 (84289) for antimicrobial scrub type A, and 138 (62274) for antimicrobial scrub type B (P=0.36). We found no significant differences between the colony counts cultured from any of the individual sites among the 3 groups, regardless of occupation (Table 2). No significant difference was observed with respect to colony counts cultured from the wrist among the 3 study groups (Table 2). Comparisons between groups were planned a priori if a difference across all groups was found. Given the nonsignificant P values across all scrub groups, no further comparisons were made.
Total (From All Sites on Scrubs) | Sleeve Cuff | Thigh | Wrist | ||
---|---|---|---|---|---|
| |||||
All subjects, N=105 | |||||
Standard scrub | 99 (66182) | 41 (2070) | 20 (944) | 32 (2161) | 16 (540) |
Antimicrobial scrub A | 137 (84289) | 65 (35117) | 33 (16124) | 41 (1586) | 23 (442) |
Antimicrobial scrub B | 138 (62274) | 41 (2299) | 21 (941) | 40 (18107) | 15 (654) |
P value | 0.36 | 0.17 | 0.07 | 0.57 | 0.92 |
Physicians and midlevels, n=68 | |||||
Standard scrub | 115.5 (72.5173.5) | 44.5 (2270.5) | 27.5 (10.538.5) | 35 (2362.5) | 24.5 (755) |
Antimicrobial scrub A | 210 (114289) | 86 (64120) | 39 (18129) | 49 (2486) | 24 (342) |
Antimicrobial scrub B | 149 (68295) | 52 (26126) | 21 (1069) | 37 (18141) | 19 (872) |
P value | 0.21 | 0.08 | 0.19 | 0.85 | 0.76 |
Nurses, n=37 | |||||
Standard scrub | 89 (31236) | 37 (1348) | 13 (552) | 28 (1342) | 9 (321) |
Antimicrobial scrub A | 105 (43256) | 45.5 (2258) | 21.5 (1654) | 38.5 (1268) | 17 (643) |
Antimicrobial scrub B | 91.5 (60174.5) | 27 (1340) | 16 (7.526) | 51 (2186.5) | 10 (3.543.5) |
P value | 0.86 | 0.39 | 0.19 | 0.49 | 0.41 |
Fifty‐five participants (52%) reported caring for patients who were known to be colonized or infected with an antibiotic‐resistant organism, 16 (46%) randomized to wear standard scrubs, and 20 (57%) and 19 (54%) randomized to wear antimicrobial scrub A or B, respectively (P=0.61). Of these, however, antibiotic‐resistant organisms were only cultured from the scrubs of 2 providers (1 with 1 colony of MRSA from the breast pocket of antimicrobial scrub A, 1 with 1 colony of MRSA cultured from the pocket of antimicrobial scrub B [P=0.55]), and from the wrist of only 1 provider (a multiresistant Gram‐negative rod who wore antimicrobial scrub B).
Adverse Events
Six subjects (5.7%) reported adverse events, all of whom were wearing antimicrobial scrubs (P=0.18). For participants wearing antimicrobial scrub A, 1 (3%) reported itchiness and 2 (6%) reported heaviness or poor breathability. For participants wearing antimicrobial scrub B, 1 (3%) reported redness, 1 (3%) reported itchiness, and 1 (3%) reported heaviness or poor breathability.
DISCUSSION
The important findings of this study are that we found no evidence indicating that either of the 2 antimicrobial scrubs tested reduced bacterial contamination or antibiotic‐resistant contamination on HCWs' scrubs or wrists compared with standard scrubs at the end of an 8‐hour workday, and that despite many HCWs being exposed to patients who were colonized or infected with antibiotic‐resistant bacteria, these organisms were only rarely cultured from their uniforms.
We found that HCWs in all 3 arms of the study had bacterial contamination on their scrubs and skin, consistent with previous studies showing that HCWs' uniforms are frequently contaminated with bacteria, including MRSA, VRE, and other pathogens.[1, 2, 3, 4, 5, 6, 7, 8, 9, 10, 11, 12] We previously found that bacterial contamination of HCWs' uniforms occurs within hours of putting on newly laundered uniforms.[12]
Literature on the effectiveness of antimicrobial HCW uniforms when tested in clinical settings is limited. Bearman and colleagues[23] recently published the results of a study of 31 subjects who wore either standard or antimicrobial scrubs, crossing over every 4 weeks for 4 months, with random culturing done weekly at the beginning and end of a work shift. Scrubs were laundered an average of 1.5 times/week, but the timing of the laundering relative to when cultures were obtained was not reported. Very few isolates of MRSA, Gram‐negative rods, or VRE were found (only 3.9%, 0.4%, and 0.05% of the 2000 samples obtained, respectively), and no differences were observed with respect to the number of HCWs who had antibiotic‐resistant organisms cultured when they were wearing standard versus antimicrobial scrubs. Those who had MRSA cultured, however, had lower mean log colony counts when they were wearing the antimicrobial scrubs. The small number of samples with positive isolates, together with differences in the extent of before‐shift contamination among groups complicates interpreting these data. The authors concluded that a prospective trial was needed. We attempted to include the scrub studied by Bearman and colleagues[23] in our study, but the company had insufficient stock available at the time we tried to purchase the product.
Gross and colleagues[24] found no difference in the mean colony counts of cultures taken from silver‐impregnated versus standard scrubs in a pilot crossover study done with 10 HCWs (although there were trends toward higher colony counts when the subjects wore antimicrobial scrubs).
Antibiotic‐resistant bacteria were only cultured from 3 participants (2.9%) in our current study, compared to 16% of those randomized to wearing white coats in our previous study and 20% of those randomized to wearing standard scrubs.[12] This difference may be explained by several recent studies reporting that rates of MRSA infections in hospitals are decreasing.[25, 26] The rate of hospital‐acquired MRSA infection or colonization at our own institution decreased 80% from 2007 to 2012. At the times of our previous and current studies, providers were expected to wear gowns and gloves when caring for patients as per standard contact precautions. Rates of infection and colonization of VRE and resistant Gram‐negative rods have remained low at our hospital, and our data are consistent with the rates reported on HCWs' uniforms in other studies.[2, 5, 10]
Only 6 of our subjects reported adverse reactions, but all were wearing antimicrobial scrubs (P=0.18). Several of the participants described that the fabrics of the 2 antimicrobial scrubs were heavier and less breathable than the standard scrubs. We believe this difference is more likely to explain the adverse reactions reported than is any type of reaction to the specific chemicals in the fabrics.
Our study has several limitations. Because it was conducted on the general internal medicine units of a single university‐affiliated public hospital, the results may not generalize to other types of institutions or other inpatient services.
As we previously described,[12] the RODAC imprint method only samples a small area of HCWs' uniforms and thus does not represent total bacterial contamination.[21] We specifically cultured areas that are known to be highly contaminated (ie, sleeve cuffs and pockets). Although imprint methods have limitations (as do other methods for culturing clothing), they have been commonly utilized in studies assessing bacterial contamination of HCW clothing.[2, 3, 5]
Although some of the bacterial load we cultured could have come from the providers themselves, previous studies have shown that 80% to 90% of the resistant bacteria cultured from HCWs' attire come from other sources.[1, 2]
Because our sample size was calculated on the basis of being able to detect a difference of 70% in total bacterial colony count, our study was not large enough to exclude a lower level of effectiveness. However, we saw no trends suggesting the antimicrobial products might have a lower level of effectiveness.
We did not observe the hand‐washing practices of the participants, and accordingly, cannot confirm that these practices were the same in each of our 3 study groups. Intermittent, surreptitious monitoring of hand‐washing practices on our internal medicine units over the last several years has found compliance with hand hygiene recommendations varying from 70% to 90%.
Although the participants in our study were not explicitly told to which scrub they were randomized, the colors, appearances, and textures of the antimicrobial fabrics were different from the standard scrubs such that blinding was impossible. Participants wearing antimicrobial scrubs could have changed their hand hygiene practices (ie, less careful hand hygiene). Lack of blinding could also have led to over‐reporting of adverse events by the subjects randomized to wear the antimicrobial scrubs.
In an effort to treat all the scrubs in the same fashion, all were tested new, prior to being washed or previously worn. Studying the scrubs prior to washing or wearing could have increased the reports of adverse effects, as the fabrics could have been stiffer and more uncomfortable than they might have been at a later stage in their use.
Our study also has some strengths. Our participants included physicians, residents, nurses, nurse practitioners, and physician assistants. Accordingly, our results should be generalizable to most HCWs. We also confirmed that the scrubs that were tested were nearly sterile prior to use.
In conclusion, we found no evidence suggesting that either of 2 antimicrobial scrubs tested decreased bacterial contamination of HCWs' scrubs or skin after an 8‐hour workday compared to standard scrubs. We also found that, although HCWs are frequently exposed to patients harboring antibiotic‐resistant bacteria, these bacteria were only rarely cultured from HCWs' scrubs or skin.
- Contamination of nurses' uniforms with Staphylococcus aureus. Lancet. 1969;2:233–235. , , , .
- Contamination of protective clothing and nurses' uniforms in an isolation ward. J Hosp Infect. 1983;4:149–157. , , .
- Microbial flora on doctors' white coats. BMJ. 1991;303:1602–1604. , , .
- Bacterial contamination of nurses' uniforms: a study. Nursing Stand. 1998;13:37–42. .
- Bacterial flora on the white coats of medical students. J Hosp Infect. 2000;45:65–68. , , .
- Bacterial contamination of uniforms. J Hosp Infect. 2001;48:238–241. , , .
- Significance of methicillin‐resistant Staphylococcus aureus (MRSA) survey in a university teaching hospital. J Infect Chemother. 2003;9:172–177. , , , et al.
- Environmental contamination makes an important contribution to hospital infection. J Hosp Infect. 2007;65(suppl 2):50–54. .
- Detection of methicillin‐resistant Staphylococcus aureus and vancomycin‐resistant enterococci on the gowns and gloves of healthcare workers. Infect Control Hosp Epidemiol. 2008;29:583–589. , , , et al.
- Bacterial contamination of health care workers' white coats. Am J Infect Control. 2009;37:101–105. , , , , , .
- Nursing and physician attire as possible source of nosocomial infections. Am J Infect Control. 2011;39:555–559. , , , , , .
- Newly cleaned physician uniforms and infrequently washed white coats have similar rates of bacterial contamination after an 8‐hour workday: a randomized controlled trial. J Hosp Med. 2011;6:177–182. , , , , , .
- Associations between bacterial contamination of health care workers' hands and contamination of white coats and scrubs. Am J Infect Control. 2012;40:e245–e248. , , , et al.
- Department of Health. Uniforms and workwear: an evidence base for developing local policy. National Health Service, 17 September 2007. Available at: http://www.dh.gov.uk/en/Publicationsandstatistics/Publications/Publicationspolicyandguidance/DH_078433. Accessed January 29, 2010.
- Scottish Government Health Directorates. NHS Scotland dress code. Available at: http://www.sehd.scot.nhs.uk/mels/CEL2008_53.pdf. Accessed February 10, 2010.
- Bio Shield Tech Web site. Bio Gardz–unisex scrub top–antimicrobial treatment. Available at: http://www.bioshieldtech.com/Bio_Gardz_Unisex_Scrub_Top_Antimicrobial_Tre_p/sbt01‐r‐p.htm. Accessed January 9, 2013.
- Doc Froc Web site and informational packet. Available at: http://www.docfroc.com. Accessed July 22, 2011.
- Vestagen Web site and informational packet. Available at: http://www.vestagen.com. Accessed July 22, 2011.
- Under Scrub apparel Web site. Testing. Available at: http://underscrub.com/testing. Accessed March 21, 2013.
- MediThreads Web site. Microban FAQ's. Available at: http://medithreads.com/faq/microban‐faqs. Accessed March 21, 2013.
- Comparison of the Rodac imprint method to selective enrichment broth for recovery of vancomycin‐resistant enterococci and drug‐resistant Enterobacteriaceae from environmental surfaces. J Clin Microbiol. 2000;38:4646–4648. , , , , .
- Research electronic data capture (REDCap)—a metadata‐driven methodology and workflow process for providing translational research informatics support. J Biomed Inform. 2009;42:377–381. , , , , , .
- A crossover trial of antimicrobial scrubs to reduce methicillin‐resistant Staphylococcus aureus burden on healthcare worker apparel. Infect Control Hosp Epidemiol. 2012;33:268–275. , , , et al.
- Pilot study on the microbial contamination of conventional vs. silver‐impregnated uniforms worn by ambulance personnel during one week of emergency medical service. GMS Krankenhhyg Interdiszip. 2010;5.pii: Doc09. , , , , .
- Epidemiology of Staphylococcus aureus blood and skin and soft tissue infections in the US military health system, 2005–2010. JAMA. 2012;308:50–59. , , , et al.
- Health care‐associated invasive MRSA infections, 2005–2008. JAMA. 2010;304:641–648. , , , et al.
- Contamination of nurses' uniforms with Staphylococcus aureus. Lancet. 1969;2:233–235. , , , .
- Contamination of protective clothing and nurses' uniforms in an isolation ward. J Hosp Infect. 1983;4:149–157. , , .
- Microbial flora on doctors' white coats. BMJ. 1991;303:1602–1604. , , .
- Bacterial contamination of nurses' uniforms: a study. Nursing Stand. 1998;13:37–42. .
- Bacterial flora on the white coats of medical students. J Hosp Infect. 2000;45:65–68. , , .
- Bacterial contamination of uniforms. J Hosp Infect. 2001;48:238–241. , , .
- Significance of methicillin‐resistant Staphylococcus aureus (MRSA) survey in a university teaching hospital. J Infect Chemother. 2003;9:172–177. , , , et al.
- Environmental contamination makes an important contribution to hospital infection. J Hosp Infect. 2007;65(suppl 2):50–54. .
- Detection of methicillin‐resistant Staphylococcus aureus and vancomycin‐resistant enterococci on the gowns and gloves of healthcare workers. Infect Control Hosp Epidemiol. 2008;29:583–589. , , , et al.
- Bacterial contamination of health care workers' white coats. Am J Infect Control. 2009;37:101–105. , , , , , .
- Nursing and physician attire as possible source of nosocomial infections. Am J Infect Control. 2011;39:555–559. , , , , , .
- Newly cleaned physician uniforms and infrequently washed white coats have similar rates of bacterial contamination after an 8‐hour workday: a randomized controlled trial. J Hosp Med. 2011;6:177–182. , , , , , .
- Associations between bacterial contamination of health care workers' hands and contamination of white coats and scrubs. Am J Infect Control. 2012;40:e245–e248. , , , et al.
- Department of Health. Uniforms and workwear: an evidence base for developing local policy. National Health Service, 17 September 2007. Available at: http://www.dh.gov.uk/en/Publicationsandstatistics/Publications/Publicationspolicyandguidance/DH_078433. Accessed January 29, 2010.
- Scottish Government Health Directorates. NHS Scotland dress code. Available at: http://www.sehd.scot.nhs.uk/mels/CEL2008_53.pdf. Accessed February 10, 2010.
- Bio Shield Tech Web site. Bio Gardz–unisex scrub top–antimicrobial treatment. Available at: http://www.bioshieldtech.com/Bio_Gardz_Unisex_Scrub_Top_Antimicrobial_Tre_p/sbt01‐r‐p.htm. Accessed January 9, 2013.
- Doc Froc Web site and informational packet. Available at: http://www.docfroc.com. Accessed July 22, 2011.
- Vestagen Web site and informational packet. Available at: http://www.vestagen.com. Accessed July 22, 2011.
- Under Scrub apparel Web site. Testing. Available at: http://underscrub.com/testing. Accessed March 21, 2013.
- MediThreads Web site. Microban FAQ's. Available at: http://medithreads.com/faq/microban‐faqs. Accessed March 21, 2013.
- Comparison of the Rodac imprint method to selective enrichment broth for recovery of vancomycin‐resistant enterococci and drug‐resistant Enterobacteriaceae from environmental surfaces. J Clin Microbiol. 2000;38:4646–4648. , , , , .
- Research electronic data capture (REDCap)—a metadata‐driven methodology and workflow process for providing translational research informatics support. J Biomed Inform. 2009;42:377–381. , , , , , .
- A crossover trial of antimicrobial scrubs to reduce methicillin‐resistant Staphylococcus aureus burden on healthcare worker apparel. Infect Control Hosp Epidemiol. 2012;33:268–275. , , , et al.
- Pilot study on the microbial contamination of conventional vs. silver‐impregnated uniforms worn by ambulance personnel during one week of emergency medical service. GMS Krankenhhyg Interdiszip. 2010;5.pii: Doc09. , , , , .
- Epidemiology of Staphylococcus aureus blood and skin and soft tissue infections in the US military health system, 2005–2010. JAMA. 2012;308:50–59. , , , et al.
- Health care‐associated invasive MRSA infections, 2005–2008. JAMA. 2010;304:641–648. , , , et al.
© 2013 Society of Hospital Medicine
Curbside vs Formal Consultation
A curbside consultation is an informal process whereby a consultant is asked to provide information or advice about a patient's care without doing a formal assessment of the patient.14 Curbside consultations are common in the practice of medicine2, 3, 5 and are frequently requested by physicians caring for hospitalized patients. Several surveys have documented the quantity of curbside consultations requested of various subspecialties, the types of questions asked, the time it takes to respond, and physicians' perceptions about the quality of the information exchanged.111 While curbside consultations have a number of advantages, physicians' perceptions are that the information conveyed may be inaccurate or incomplete and that the advice offered may be erroneous.13, 5, 10, 12, 13
Cartmill and White14 performed a random audit of 10% of the telephone referrals they received for neurosurgical consultation over a 1‐year period and noted discrepancies between the Glascow Coma Scores reported during the telephone referrals and those noted in the medical records, but the frequency of these discrepancies was not reported. To our knowledge, no studies have compared the quality of the information provided in curbside consultations with that obtained in formal consultations that included direct face‐to‐face patient evaluations and primary data collection, and whether the advice provided in curbside and formal consultations on the same patient differed.
We performed a prospective cohort study to compare the information received by hospitalists during curbside consultations on hospitalized patients, with that obtained from formal consultations done the same day on the same patients, by different hospitalists who were unaware of any details regarding the curbside consultation. We also compared the advice provided by the 2 hospitalists following their curbside and formal consultations. Our hypotheses were that the information received during curbside consultations was frequently inaccurate or incomplete, that the recommendations made after the formal consultation would frequently differ from those made in the curbside consultation, and that these differences would have important implications on patient care.
METHODS
This was a quality improvement study conducted at Denver Health, a 500‐bed university‐affiliated urban safety net hospital from January 10, 2011 to January 9, 2012. The study design was a prospective cohort that included all curbside consultations on hospitalized patients received between 7 AM and 3 PM, on intermittently selected weekdays, by the Internal Medicine Consultation Service that was staffed by 18 hospitalists. Data were collected intermittently based upon hospitalist availability and was done to limit potential alterations in the consulting practices of the providers requesting consultations.
Consultations were defined as being curbside when the consulting provider asked for advice, suggestions, or opinions about a patient's care but did not ask the hospitalist to see the patient.15, 15 Consultations pertaining to administrative issues (eg, whether a patient should be admitted to an intensive care bed as opposed to an acute care floor bed) or on patients who were already being followed by a hospitalist were excluded.
The hospitalist receiving the curbside consultation was allowed to ask questions as they normally would, but could not verify the accuracy of the information received (eg, could not review any portion of the patient's medical record, such as notes or lab data). A standardized data collection sheet was used to record the service and level of training of the requesting provider, the medical issue(s) of concern, all clinical data offered by the provider, the number of questions asked by the hospitalist of the provider, and whether, on the basis of the information provided, the hospitalist felt that the question(s) being asked was (were) of sufficient complexity that a formal consultation should occur. The hospitalist then offered advice based upon the information given during the curbside consultation.
After completing the curbside consultation, the hospitalist requested verbal permission from the requesting provider to perform a formal consultation. If the request was approved, the hospitalist performing the curbside consultation contacted a different hospitalist who performed the formal consultation within the next few hours. The only information given to the second hospitalist was the patient's identifiers and the clinical question(s) being asked. The formal consultation included a complete face‐to‐face history and physical examination, a review of the patient's medical record, documentation of the provider's findings, and recommendations for care.
Upon completion of the formal consultation, the hospitalists who performed the curbside and the formal consultations met to review the advice each gave to the requesting provider and the information on which this advice was based. The 2 hospitalists jointly determined the following: (a) whether the information received during the curbside consultation was correct and complete, (b) whether the advice provided in the formal consultation differed from that provided in the curbside consultation, (c) whether the advice provided in the formal consultation dealt with issues other than one(s) leading to the curbside consultation, (d) whether differences in the recommendations given in the curbside versus the formal consultation changed patient management in a meaningful way, and (e) whether the curbside consultation alone was felt to be sufficient.
Information obtained by the hospitalist performing the formal consultation that was different from, or not included in, the information recorded during the curbside consultation was considered to be incorrect or incomplete, respectively. A change in management was defined as an alteration in the direction or type of care that the patient would have received as a result of the advice being given. A pulmonary and critical care physician, with >35 years of experience in inpatient medicine, reviewed the information provided in the curbside and formal consultations, and independently assessed whether the curbside consultation alone would have been sufficient and whether the formal consultation changed management.
Curbside consultations were neither solicited nor discouraged during the course of the study. The provider requesting the curbside consultation was not informed or debriefed about the study in an attempt to avoid affecting future consultation practices from that provider or service.
Associations were sought between the frequency of inaccurate or incomplete data and the requesting service and provider, the consultative category and medical issue, the number of questions asked by the hospitalist during the curbside consultation, and whether the hospitalist doing the curbside consultation thought that formal consultation was needed. A chi‐square test was used to analyze all associations. A P value of <0.05 was considered significant. All analyses were performed using SAS Enterprise Guide 4.3 (SAS Institute, Inc, Cary, NC) software. The study was approved by the Colorado Multiple Institutional Review Board.
RESULTS
Fifty curbside consultations were requested on a total of 215 study days. The requesting service declined formal consultation in 3 instances, leaving 47 curbside consultations that had a formal consultation. Curbside consultations came from a variety of services and providers, and addressed a variety of issues and concerns (Table 1).
Curbside Consultations, N (%) | |
---|---|
47 (100) | |
| |
Requesting service | |
Psychiatry | 21 (45) |
Emergency Department | 9 (19) |
Obstetrics/Gynecology | 5 (11) |
Neurology | 4 (8) |
Other (Orthopedics, Anesthesia, General Surgery, Neurosurgery, and Interventional Radiology) | 8 (17) |
Requesting provider | |
Resident | 25 (53) |
Intern | 8 (17) |
Attending | 9 (19) |
Other | 5 (11) |
Consultative issue* | |
Diagnosis | 10 (21) |
Treatment | 29 (62) |
Evaluation | 20 (43) |
Discharge | 13 (28) |
Lab interpretation | 4 (9) |
Medical concern* | |
Cardiac | 27 (57) |
Endocrine | 17 (36) |
Infectious disease | 9 (19) |
Pulmonary | 8 (17) |
Gastroenterology | 6 (13) |
Fluid and electrolyte | 6 (13) |
Others | 23 (49) |
The hospitalists asked 0 to 2 questions during 8/47 (17%) of the curbside consultations, 3 to 5 questions during 26/47 (55%) consultations, and more than 5 questions during 13/47 (28%). Based on the information received during the curbside consultations, the hospitalists thought that the curbside consultations were insufficient for 18/47 (38%) of patients. In all instances, the opinions of the 2 hospitalists concurred with respect to this conclusion, and the independent reviewer agreed with this assessment in 17 of these 18 (94%).
The advice rendered in the formal consultations differed from that provided in 26/47 (55%) of the curbside consultations, and the formal consultation was thought to have changed management for 28/47 (60%) of patients (Table 2). The independent reviewer thought that the advice provided in the formal consultations changed management in 29/47 (62%) of the cases, and in 24/28 cases (86%) where the hospitalist felt that the formal consult changed management.
Curbside Consultations, N (%) | |||
---|---|---|---|
Total | Accurate and Complete | Inaccurate or Incomplete | |
47 (100) | 23 (49) | 24 (51) | |
| |||
Advice in formal consultation differed from advice in curbside consultation | 26 (55) | 7 (30) | 19 (79)* |
Formal consultation changed management | 28 (60) | 6 (26) | 22 (92) |
Minor change | 18 (64) | 6 (100) | 12 (55) |
Major change | 10 (36) | 0 (0) | 10 (45) |
Curbside consultation insufficient | 18 (38) | 2 (9) | 16 (67) |
Information was felt to be inaccurate or incomplete in 24/47 (51%) of the curbside consultations (13/47 inaccurate, 16/47 incomplete, 5/47 both inaccurate and incomplete), and when inaccurate or incomplete information was obtained, the advice given in the formal consultations more commonly differed from that provided in the curbside consultation (19/24, 79% vs 7/23, 30%; P < 0.001), and was more commonly felt to change management (22/24, 92% vs 6/23, 26%; P < 0.0001) (Table 2). No association was found between whether the curbside consultation contained complete or accurate information and the consulting service from which the curbside originated, the consulting provider, the consultative aspect(s) or medical issue(s) addressed, the number of questions asked by the hospitalist during the curbside consultation, nor whether the hospitalists felt that a formal consultation was needed.
DISCUSSION
The important findings of this study are that (a) the recommendations made by hospitalists in curbside versus formal consultations on the same patient frequently differ, (b) these differences frequently result in changes in clinical management, (c) the information presented in curbside consultations by providers is frequently inaccurate or incomplete, regardless of the providers specialty or seniority, (d) when inaccurate or incomplete information is received, the recommendations made in curbside and formal consultations differ more frequently, and (e) we found no way to predict whether the information provided in a curbside consultation was likely to be inaccurate or incomplete.
Our hospitalists thought that 38% of the curbside consultations they received should have had formal consultations. Manian and McKinsey7 reported that as many as 53% of questions asked of infectious disease consultants were thought to be too complex to be addressed in an informal consultation. Others, however, report that only 11%33% of curbside consultations were thought to require formal consultation.1, 9, 10, 16 Our hospitalists asked 3 or more questions of the consulting providers in more than 80% of the curbside consultations, suggesting that the curbside consultations we received might have had a higher complexity than those seen by others.
Our finding that information provided in curbside consultation was frequently inaccurate or incomplete is consistent with a number of previous studies reporting physicians' perceptions of the accuracy of curbside consultations.2, 3 Hospital medicine is not likely to be the only discipline affected by inaccurate curbside consultation practices, as surveys of specialists in infectious disease, gynecology, and neurosurgery report that practitioners in these disciplines have similar concerns.1, 10, 14 In a survey returned by 34 physicians, Myers1 found that 50% thought the information exchanged during curbside consultations was inaccurate, leading him to conclude that inaccuracies presented during curbside consultations required further study.
We found no way of predicting whether curbside consultations were likely to include inaccurate or incomplete information. This observation is consistent with the results of Bergus et al16 who found that the frequency of curbside consultations being converted to formal consultations was independent of the training status of the consulting physician, and with the data of Myers1 who found no way of predicting the likelihood that a curbside consultation should be converted to a formal consultation.
We found that formal consultations resulted in management changes more often than differences in recommendations (ie, 60% vs 55%, respectively). This small difference occurred because, on occasion, the formal consultations found issues to address other than the one(s) for which the curbside consultation was requested. In the majority of these instances, the management changes were minor and the curbside consultation was still felt to be sufficient.
In some instances, the advice given after the curbside and the formal consultations differed to only a minor extent (eg, varying recommendations for oral diabetes management). In other instances, however, the advice differed substantially (eg, change in antibiotic management in a septic patient with a multidrug resistant organism, when the original curbside question was for when to order a follow‐up chest roentgenogram for hypoxia; see Supporting Information, Appendix, in the online version of this article). In 26 patients (55%), formal consultation resulted in different medications being started or stopped, additional tests being performed, or different decisions being made about admission versus discharge.
Our study has a number of strengths. First, while a number of reports document that physicians' perceptions are that curbside consultations frequently contain errors,2, 3, 5, 12 to our knowledge this is the first study that prospectively compared the information collected and advice given in curbside versus formal consultation. Second, while this study was conducted as a quality improvement project, thereby requiring us to conclude that the results are not generalizable, the data presented were collected by 18 different hospitalists, reducing the potential of bias from an individual provider's knowledge base or practice. Third, there was excellent agreement between the independent reviewer and the 2 hospitalists who performed the curbside and formal consultations regarding whether a curbside consultation would have been sufficient, and whether the formal consultation changed patient management. Fourth, the study was conducted over a 1‐year period, which should have reduced potential bias arising from the increasing experience of residents requesting consultations as their training progressed.
Our study has several limitations. First, the number of curbside consultations we received during the study period (50 over 215 days) was lower than anticipated, and lower than the rates of consultation reported by others.1, 7, 9 This likely relates to the fact that, prior to beginning the study, Denver Health hospitalists already provided mandatory consultations for several surgical services (thereby reducing the number of curbside consultations received from these services), because curbside consultations received during evenings, nights, and weekends were not included in the study for reasons of convenience, and because we excluded all administrative curbside consultations. Our hospitalist service also provides consultative services 24 hours a day, thereby reducing the number of consultations received during daytime hours. Second, the frequency with which curbside consultations included inaccurate or incomplete information might be higher than what occurs in other hospitals, as Denver Health is an urban, university‐affiliated public hospital and the patients encountered may be more complex and trainees may be less adept at recognizing the information that would facilitate accurate curbside consultations (although we found no difference in the frequency with which inaccurate or incomplete information was provided as a function of the seniority of the requesting physician). Third, the disparity between curbside and formal consultations that we observed could have been biased by the Hawthorne effect. We attempted to address this by not providing the hospitalists who did the formal consultation with any information collected by the hospitalist involved with the curbside consultation, and by comparing the conclusions reached by the hospitalists performing the curbside and formal consultations with those of a third party reviewer. Fourth, while we found no association between the frequency of curbside consultations in which information was inaccurate or incomplete and the consulting service, there could be a selection bias of the consulting service requesting the curbside consultations as a result of the mandatory consultations already provided by our hospitalists. Finally, our study was not designed or adequately powered to determine why curbside consultations frequently have inaccurate or incomplete information.
In summary, we found that the information provided to hospitalists during a curbside consultation was often inaccurate and incomplete, and that these problems with information exchange adversely affected the accuracy of the resulting recommendations. While there are a number of advantages to curbside consultations,1, 3, 7, 10, 12, 13 our findings indicate that the risk associated with this practice is substantial.
Acknowledgements
Disclosure: Nothing to report.
- Curbside consultation in infectious diseases: a prospective study.J Infect Dis.1984;150:797–802. .
- Physicians' experiences and beliefs regarding informal consultation.JAMA.1998;280:900–904. , , .
- Curbside consultation practices and attitudes among primary care physicians and medical subspecialists.JAMA.1998;280:905–909. , , .
- The complexity, relative value, and financial worth of curbside consultations in an academic infectious diseases unit.Clin Infect Dis.2010;51:651–655. , , , , , .
- Curbside consultations. A closer look at a common practice.JAMA.1996;275:145–147. , .
- Informal advice‐ and information‐seeking between physicians.J Med Educ.1981;56;174–180. , , , .
- A prospective study of 2,092 “curbside” questions asked of two infectious disease consultants in private practice in the midwest.Clin Infect Dis.1996;22:303–307. , .
- Curbside consultation in endocrine practice: a prospective observational study.Endocrinologist.1996;6:328–331. , , , , .
- Informal consultations provided to general internists by the gastroenterology department of an HMO.J Gen Intern Med.1998;13:435–438. , , .
- “Curbside” consultations in gynecologic oncology: a closer look at a common practice.Gynecol Oncol.1999;74:456–459. .
- Informal consultations in infectious diseases and clinical microbiology practice.Clin Microbiol Infect.2003;9:724–726. , , , et al.
- Curbside consultations and the viaduct effect.JAMA.1998;280:929–930. .
- What do we really need to know about consultation and referral?J Gen Intern Med.1998;13:497–498. .
- Telephone advice for neurosurgical referrals. Who assumes duty of care?Br J Neurosurg.2001;15:453–455. , .
- Malpractice liability for informal consultations.Fam Med.2003;35:476–481. , .
- Does the structure of clinical questions affect the outcome of curbside consultations with specialty colleagues?Arch Fam Med.2000;9:541–547. , , , .
A curbside consultation is an informal process whereby a consultant is asked to provide information or advice about a patient's care without doing a formal assessment of the patient.14 Curbside consultations are common in the practice of medicine2, 3, 5 and are frequently requested by physicians caring for hospitalized patients. Several surveys have documented the quantity of curbside consultations requested of various subspecialties, the types of questions asked, the time it takes to respond, and physicians' perceptions about the quality of the information exchanged.111 While curbside consultations have a number of advantages, physicians' perceptions are that the information conveyed may be inaccurate or incomplete and that the advice offered may be erroneous.13, 5, 10, 12, 13
Cartmill and White14 performed a random audit of 10% of the telephone referrals they received for neurosurgical consultation over a 1‐year period and noted discrepancies between the Glascow Coma Scores reported during the telephone referrals and those noted in the medical records, but the frequency of these discrepancies was not reported. To our knowledge, no studies have compared the quality of the information provided in curbside consultations with that obtained in formal consultations that included direct face‐to‐face patient evaluations and primary data collection, and whether the advice provided in curbside and formal consultations on the same patient differed.
We performed a prospective cohort study to compare the information received by hospitalists during curbside consultations on hospitalized patients, with that obtained from formal consultations done the same day on the same patients, by different hospitalists who were unaware of any details regarding the curbside consultation. We also compared the advice provided by the 2 hospitalists following their curbside and formal consultations. Our hypotheses were that the information received during curbside consultations was frequently inaccurate or incomplete, that the recommendations made after the formal consultation would frequently differ from those made in the curbside consultation, and that these differences would have important implications on patient care.
METHODS
This was a quality improvement study conducted at Denver Health, a 500‐bed university‐affiliated urban safety net hospital from January 10, 2011 to January 9, 2012. The study design was a prospective cohort that included all curbside consultations on hospitalized patients received between 7 AM and 3 PM, on intermittently selected weekdays, by the Internal Medicine Consultation Service that was staffed by 18 hospitalists. Data were collected intermittently based upon hospitalist availability and was done to limit potential alterations in the consulting practices of the providers requesting consultations.
Consultations were defined as being curbside when the consulting provider asked for advice, suggestions, or opinions about a patient's care but did not ask the hospitalist to see the patient.15, 15 Consultations pertaining to administrative issues (eg, whether a patient should be admitted to an intensive care bed as opposed to an acute care floor bed) or on patients who were already being followed by a hospitalist were excluded.
The hospitalist receiving the curbside consultation was allowed to ask questions as they normally would, but could not verify the accuracy of the information received (eg, could not review any portion of the patient's medical record, such as notes or lab data). A standardized data collection sheet was used to record the service and level of training of the requesting provider, the medical issue(s) of concern, all clinical data offered by the provider, the number of questions asked by the hospitalist of the provider, and whether, on the basis of the information provided, the hospitalist felt that the question(s) being asked was (were) of sufficient complexity that a formal consultation should occur. The hospitalist then offered advice based upon the information given during the curbside consultation.
After completing the curbside consultation, the hospitalist requested verbal permission from the requesting provider to perform a formal consultation. If the request was approved, the hospitalist performing the curbside consultation contacted a different hospitalist who performed the formal consultation within the next few hours. The only information given to the second hospitalist was the patient's identifiers and the clinical question(s) being asked. The formal consultation included a complete face‐to‐face history and physical examination, a review of the patient's medical record, documentation of the provider's findings, and recommendations for care.
Upon completion of the formal consultation, the hospitalists who performed the curbside and the formal consultations met to review the advice each gave to the requesting provider and the information on which this advice was based. The 2 hospitalists jointly determined the following: (a) whether the information received during the curbside consultation was correct and complete, (b) whether the advice provided in the formal consultation differed from that provided in the curbside consultation, (c) whether the advice provided in the formal consultation dealt with issues other than one(s) leading to the curbside consultation, (d) whether differences in the recommendations given in the curbside versus the formal consultation changed patient management in a meaningful way, and (e) whether the curbside consultation alone was felt to be sufficient.
Information obtained by the hospitalist performing the formal consultation that was different from, or not included in, the information recorded during the curbside consultation was considered to be incorrect or incomplete, respectively. A change in management was defined as an alteration in the direction or type of care that the patient would have received as a result of the advice being given. A pulmonary and critical care physician, with >35 years of experience in inpatient medicine, reviewed the information provided in the curbside and formal consultations, and independently assessed whether the curbside consultation alone would have been sufficient and whether the formal consultation changed management.
Curbside consultations were neither solicited nor discouraged during the course of the study. The provider requesting the curbside consultation was not informed or debriefed about the study in an attempt to avoid affecting future consultation practices from that provider or service.
Associations were sought between the frequency of inaccurate or incomplete data and the requesting service and provider, the consultative category and medical issue, the number of questions asked by the hospitalist during the curbside consultation, and whether the hospitalist doing the curbside consultation thought that formal consultation was needed. A chi‐square test was used to analyze all associations. A P value of <0.05 was considered significant. All analyses were performed using SAS Enterprise Guide 4.3 (SAS Institute, Inc, Cary, NC) software. The study was approved by the Colorado Multiple Institutional Review Board.
RESULTS
Fifty curbside consultations were requested on a total of 215 study days. The requesting service declined formal consultation in 3 instances, leaving 47 curbside consultations that had a formal consultation. Curbside consultations came from a variety of services and providers, and addressed a variety of issues and concerns (Table 1).
Curbside Consultations, N (%) | |
---|---|
47 (100) | |
| |
Requesting service | |
Psychiatry | 21 (45) |
Emergency Department | 9 (19) |
Obstetrics/Gynecology | 5 (11) |
Neurology | 4 (8) |
Other (Orthopedics, Anesthesia, General Surgery, Neurosurgery, and Interventional Radiology) | 8 (17) |
Requesting provider | |
Resident | 25 (53) |
Intern | 8 (17) |
Attending | 9 (19) |
Other | 5 (11) |
Consultative issue* | |
Diagnosis | 10 (21) |
Treatment | 29 (62) |
Evaluation | 20 (43) |
Discharge | 13 (28) |
Lab interpretation | 4 (9) |
Medical concern* | |
Cardiac | 27 (57) |
Endocrine | 17 (36) |
Infectious disease | 9 (19) |
Pulmonary | 8 (17) |
Gastroenterology | 6 (13) |
Fluid and electrolyte | 6 (13) |
Others | 23 (49) |
The hospitalists asked 0 to 2 questions during 8/47 (17%) of the curbside consultations, 3 to 5 questions during 26/47 (55%) consultations, and more than 5 questions during 13/47 (28%). Based on the information received during the curbside consultations, the hospitalists thought that the curbside consultations were insufficient for 18/47 (38%) of patients. In all instances, the opinions of the 2 hospitalists concurred with respect to this conclusion, and the independent reviewer agreed with this assessment in 17 of these 18 (94%).
The advice rendered in the formal consultations differed from that provided in 26/47 (55%) of the curbside consultations, and the formal consultation was thought to have changed management for 28/47 (60%) of patients (Table 2). The independent reviewer thought that the advice provided in the formal consultations changed management in 29/47 (62%) of the cases, and in 24/28 cases (86%) where the hospitalist felt that the formal consult changed management.
Curbside Consultations, N (%) | |||
---|---|---|---|
Total | Accurate and Complete | Inaccurate or Incomplete | |
47 (100) | 23 (49) | 24 (51) | |
| |||
Advice in formal consultation differed from advice in curbside consultation | 26 (55) | 7 (30) | 19 (79)* |
Formal consultation changed management | 28 (60) | 6 (26) | 22 (92) |
Minor change | 18 (64) | 6 (100) | 12 (55) |
Major change | 10 (36) | 0 (0) | 10 (45) |
Curbside consultation insufficient | 18 (38) | 2 (9) | 16 (67) |
Information was felt to be inaccurate or incomplete in 24/47 (51%) of the curbside consultations (13/47 inaccurate, 16/47 incomplete, 5/47 both inaccurate and incomplete), and when inaccurate or incomplete information was obtained, the advice given in the formal consultations more commonly differed from that provided in the curbside consultation (19/24, 79% vs 7/23, 30%; P < 0.001), and was more commonly felt to change management (22/24, 92% vs 6/23, 26%; P < 0.0001) (Table 2). No association was found between whether the curbside consultation contained complete or accurate information and the consulting service from which the curbside originated, the consulting provider, the consultative aspect(s) or medical issue(s) addressed, the number of questions asked by the hospitalist during the curbside consultation, nor whether the hospitalists felt that a formal consultation was needed.
DISCUSSION
The important findings of this study are that (a) the recommendations made by hospitalists in curbside versus formal consultations on the same patient frequently differ, (b) these differences frequently result in changes in clinical management, (c) the information presented in curbside consultations by providers is frequently inaccurate or incomplete, regardless of the providers specialty or seniority, (d) when inaccurate or incomplete information is received, the recommendations made in curbside and formal consultations differ more frequently, and (e) we found no way to predict whether the information provided in a curbside consultation was likely to be inaccurate or incomplete.
Our hospitalists thought that 38% of the curbside consultations they received should have had formal consultations. Manian and McKinsey7 reported that as many as 53% of questions asked of infectious disease consultants were thought to be too complex to be addressed in an informal consultation. Others, however, report that only 11%33% of curbside consultations were thought to require formal consultation.1, 9, 10, 16 Our hospitalists asked 3 or more questions of the consulting providers in more than 80% of the curbside consultations, suggesting that the curbside consultations we received might have had a higher complexity than those seen by others.
Our finding that information provided in curbside consultation was frequently inaccurate or incomplete is consistent with a number of previous studies reporting physicians' perceptions of the accuracy of curbside consultations.2, 3 Hospital medicine is not likely to be the only discipline affected by inaccurate curbside consultation practices, as surveys of specialists in infectious disease, gynecology, and neurosurgery report that practitioners in these disciplines have similar concerns.1, 10, 14 In a survey returned by 34 physicians, Myers1 found that 50% thought the information exchanged during curbside consultations was inaccurate, leading him to conclude that inaccuracies presented during curbside consultations required further study.
We found no way of predicting whether curbside consultations were likely to include inaccurate or incomplete information. This observation is consistent with the results of Bergus et al16 who found that the frequency of curbside consultations being converted to formal consultations was independent of the training status of the consulting physician, and with the data of Myers1 who found no way of predicting the likelihood that a curbside consultation should be converted to a formal consultation.
We found that formal consultations resulted in management changes more often than differences in recommendations (ie, 60% vs 55%, respectively). This small difference occurred because, on occasion, the formal consultations found issues to address other than the one(s) for which the curbside consultation was requested. In the majority of these instances, the management changes were minor and the curbside consultation was still felt to be sufficient.
In some instances, the advice given after the curbside and the formal consultations differed to only a minor extent (eg, varying recommendations for oral diabetes management). In other instances, however, the advice differed substantially (eg, change in antibiotic management in a septic patient with a multidrug resistant organism, when the original curbside question was for when to order a follow‐up chest roentgenogram for hypoxia; see Supporting Information, Appendix, in the online version of this article). In 26 patients (55%), formal consultation resulted in different medications being started or stopped, additional tests being performed, or different decisions being made about admission versus discharge.
Our study has a number of strengths. First, while a number of reports document that physicians' perceptions are that curbside consultations frequently contain errors,2, 3, 5, 12 to our knowledge this is the first study that prospectively compared the information collected and advice given in curbside versus formal consultation. Second, while this study was conducted as a quality improvement project, thereby requiring us to conclude that the results are not generalizable, the data presented were collected by 18 different hospitalists, reducing the potential of bias from an individual provider's knowledge base or practice. Third, there was excellent agreement between the independent reviewer and the 2 hospitalists who performed the curbside and formal consultations regarding whether a curbside consultation would have been sufficient, and whether the formal consultation changed patient management. Fourth, the study was conducted over a 1‐year period, which should have reduced potential bias arising from the increasing experience of residents requesting consultations as their training progressed.
Our study has several limitations. First, the number of curbside consultations we received during the study period (50 over 215 days) was lower than anticipated, and lower than the rates of consultation reported by others.1, 7, 9 This likely relates to the fact that, prior to beginning the study, Denver Health hospitalists already provided mandatory consultations for several surgical services (thereby reducing the number of curbside consultations received from these services), because curbside consultations received during evenings, nights, and weekends were not included in the study for reasons of convenience, and because we excluded all administrative curbside consultations. Our hospitalist service also provides consultative services 24 hours a day, thereby reducing the number of consultations received during daytime hours. Second, the frequency with which curbside consultations included inaccurate or incomplete information might be higher than what occurs in other hospitals, as Denver Health is an urban, university‐affiliated public hospital and the patients encountered may be more complex and trainees may be less adept at recognizing the information that would facilitate accurate curbside consultations (although we found no difference in the frequency with which inaccurate or incomplete information was provided as a function of the seniority of the requesting physician). Third, the disparity between curbside and formal consultations that we observed could have been biased by the Hawthorne effect. We attempted to address this by not providing the hospitalists who did the formal consultation with any information collected by the hospitalist involved with the curbside consultation, and by comparing the conclusions reached by the hospitalists performing the curbside and formal consultations with those of a third party reviewer. Fourth, while we found no association between the frequency of curbside consultations in which information was inaccurate or incomplete and the consulting service, there could be a selection bias of the consulting service requesting the curbside consultations as a result of the mandatory consultations already provided by our hospitalists. Finally, our study was not designed or adequately powered to determine why curbside consultations frequently have inaccurate or incomplete information.
In summary, we found that the information provided to hospitalists during a curbside consultation was often inaccurate and incomplete, and that these problems with information exchange adversely affected the accuracy of the resulting recommendations. While there are a number of advantages to curbside consultations,1, 3, 7, 10, 12, 13 our findings indicate that the risk associated with this practice is substantial.
Acknowledgements
Disclosure: Nothing to report.
A curbside consultation is an informal process whereby a consultant is asked to provide information or advice about a patient's care without doing a formal assessment of the patient.14 Curbside consultations are common in the practice of medicine2, 3, 5 and are frequently requested by physicians caring for hospitalized patients. Several surveys have documented the quantity of curbside consultations requested of various subspecialties, the types of questions asked, the time it takes to respond, and physicians' perceptions about the quality of the information exchanged.111 While curbside consultations have a number of advantages, physicians' perceptions are that the information conveyed may be inaccurate or incomplete and that the advice offered may be erroneous.13, 5, 10, 12, 13
Cartmill and White14 performed a random audit of 10% of the telephone referrals they received for neurosurgical consultation over a 1‐year period and noted discrepancies between the Glascow Coma Scores reported during the telephone referrals and those noted in the medical records, but the frequency of these discrepancies was not reported. To our knowledge, no studies have compared the quality of the information provided in curbside consultations with that obtained in formal consultations that included direct face‐to‐face patient evaluations and primary data collection, and whether the advice provided in curbside and formal consultations on the same patient differed.
We performed a prospective cohort study to compare the information received by hospitalists during curbside consultations on hospitalized patients, with that obtained from formal consultations done the same day on the same patients, by different hospitalists who were unaware of any details regarding the curbside consultation. We also compared the advice provided by the 2 hospitalists following their curbside and formal consultations. Our hypotheses were that the information received during curbside consultations was frequently inaccurate or incomplete, that the recommendations made after the formal consultation would frequently differ from those made in the curbside consultation, and that these differences would have important implications on patient care.
METHODS
This was a quality improvement study conducted at Denver Health, a 500‐bed university‐affiliated urban safety net hospital from January 10, 2011 to January 9, 2012. The study design was a prospective cohort that included all curbside consultations on hospitalized patients received between 7 AM and 3 PM, on intermittently selected weekdays, by the Internal Medicine Consultation Service that was staffed by 18 hospitalists. Data were collected intermittently based upon hospitalist availability and was done to limit potential alterations in the consulting practices of the providers requesting consultations.
Consultations were defined as being curbside when the consulting provider asked for advice, suggestions, or opinions about a patient's care but did not ask the hospitalist to see the patient.15, 15 Consultations pertaining to administrative issues (eg, whether a patient should be admitted to an intensive care bed as opposed to an acute care floor bed) or on patients who were already being followed by a hospitalist were excluded.
The hospitalist receiving the curbside consultation was allowed to ask questions as they normally would, but could not verify the accuracy of the information received (eg, could not review any portion of the patient's medical record, such as notes or lab data). A standardized data collection sheet was used to record the service and level of training of the requesting provider, the medical issue(s) of concern, all clinical data offered by the provider, the number of questions asked by the hospitalist of the provider, and whether, on the basis of the information provided, the hospitalist felt that the question(s) being asked was (were) of sufficient complexity that a formal consultation should occur. The hospitalist then offered advice based upon the information given during the curbside consultation.
After completing the curbside consultation, the hospitalist requested verbal permission from the requesting provider to perform a formal consultation. If the request was approved, the hospitalist performing the curbside consultation contacted a different hospitalist who performed the formal consultation within the next few hours. The only information given to the second hospitalist was the patient's identifiers and the clinical question(s) being asked. The formal consultation included a complete face‐to‐face history and physical examination, a review of the patient's medical record, documentation of the provider's findings, and recommendations for care.
Upon completion of the formal consultation, the hospitalists who performed the curbside and the formal consultations met to review the advice each gave to the requesting provider and the information on which this advice was based. The 2 hospitalists jointly determined the following: (a) whether the information received during the curbside consultation was correct and complete, (b) whether the advice provided in the formal consultation differed from that provided in the curbside consultation, (c) whether the advice provided in the formal consultation dealt with issues other than one(s) leading to the curbside consultation, (d) whether differences in the recommendations given in the curbside versus the formal consultation changed patient management in a meaningful way, and (e) whether the curbside consultation alone was felt to be sufficient.
Information obtained by the hospitalist performing the formal consultation that was different from, or not included in, the information recorded during the curbside consultation was considered to be incorrect or incomplete, respectively. A change in management was defined as an alteration in the direction or type of care that the patient would have received as a result of the advice being given. A pulmonary and critical care physician, with >35 years of experience in inpatient medicine, reviewed the information provided in the curbside and formal consultations, and independently assessed whether the curbside consultation alone would have been sufficient and whether the formal consultation changed management.
Curbside consultations were neither solicited nor discouraged during the course of the study. The provider requesting the curbside consultation was not informed or debriefed about the study in an attempt to avoid affecting future consultation practices from that provider or service.
Associations were sought between the frequency of inaccurate or incomplete data and the requesting service and provider, the consultative category and medical issue, the number of questions asked by the hospitalist during the curbside consultation, and whether the hospitalist doing the curbside consultation thought that formal consultation was needed. A chi‐square test was used to analyze all associations. A P value of <0.05 was considered significant. All analyses were performed using SAS Enterprise Guide 4.3 (SAS Institute, Inc, Cary, NC) software. The study was approved by the Colorado Multiple Institutional Review Board.
RESULTS
Fifty curbside consultations were requested on a total of 215 study days. The requesting service declined formal consultation in 3 instances, leaving 47 curbside consultations that had a formal consultation. Curbside consultations came from a variety of services and providers, and addressed a variety of issues and concerns (Table 1).
Curbside Consultations, N (%) | |
---|---|
47 (100) | |
| |
Requesting service | |
Psychiatry | 21 (45) |
Emergency Department | 9 (19) |
Obstetrics/Gynecology | 5 (11) |
Neurology | 4 (8) |
Other (Orthopedics, Anesthesia, General Surgery, Neurosurgery, and Interventional Radiology) | 8 (17) |
Requesting provider | |
Resident | 25 (53) |
Intern | 8 (17) |
Attending | 9 (19) |
Other | 5 (11) |
Consultative issue* | |
Diagnosis | 10 (21) |
Treatment | 29 (62) |
Evaluation | 20 (43) |
Discharge | 13 (28) |
Lab interpretation | 4 (9) |
Medical concern* | |
Cardiac | 27 (57) |
Endocrine | 17 (36) |
Infectious disease | 9 (19) |
Pulmonary | 8 (17) |
Gastroenterology | 6 (13) |
Fluid and electrolyte | 6 (13) |
Others | 23 (49) |
The hospitalists asked 0 to 2 questions during 8/47 (17%) of the curbside consultations, 3 to 5 questions during 26/47 (55%) consultations, and more than 5 questions during 13/47 (28%). Based on the information received during the curbside consultations, the hospitalists thought that the curbside consultations were insufficient for 18/47 (38%) of patients. In all instances, the opinions of the 2 hospitalists concurred with respect to this conclusion, and the independent reviewer agreed with this assessment in 17 of these 18 (94%).
The advice rendered in the formal consultations differed from that provided in 26/47 (55%) of the curbside consultations, and the formal consultation was thought to have changed management for 28/47 (60%) of patients (Table 2). The independent reviewer thought that the advice provided in the formal consultations changed management in 29/47 (62%) of the cases, and in 24/28 cases (86%) where the hospitalist felt that the formal consult changed management.
Curbside Consultations, N (%) | |||
---|---|---|---|
Total | Accurate and Complete | Inaccurate or Incomplete | |
47 (100) | 23 (49) | 24 (51) | |
| |||
Advice in formal consultation differed from advice in curbside consultation | 26 (55) | 7 (30) | 19 (79)* |
Formal consultation changed management | 28 (60) | 6 (26) | 22 (92) |
Minor change | 18 (64) | 6 (100) | 12 (55) |
Major change | 10 (36) | 0 (0) | 10 (45) |
Curbside consultation insufficient | 18 (38) | 2 (9) | 16 (67) |
Information was felt to be inaccurate or incomplete in 24/47 (51%) of the curbside consultations (13/47 inaccurate, 16/47 incomplete, 5/47 both inaccurate and incomplete), and when inaccurate or incomplete information was obtained, the advice given in the formal consultations more commonly differed from that provided in the curbside consultation (19/24, 79% vs 7/23, 30%; P < 0.001), and was more commonly felt to change management (22/24, 92% vs 6/23, 26%; P < 0.0001) (Table 2). No association was found between whether the curbside consultation contained complete or accurate information and the consulting service from which the curbside originated, the consulting provider, the consultative aspect(s) or medical issue(s) addressed, the number of questions asked by the hospitalist during the curbside consultation, nor whether the hospitalists felt that a formal consultation was needed.
DISCUSSION
The important findings of this study are that (a) the recommendations made by hospitalists in curbside versus formal consultations on the same patient frequently differ, (b) these differences frequently result in changes in clinical management, (c) the information presented in curbside consultations by providers is frequently inaccurate or incomplete, regardless of the providers specialty or seniority, (d) when inaccurate or incomplete information is received, the recommendations made in curbside and formal consultations differ more frequently, and (e) we found no way to predict whether the information provided in a curbside consultation was likely to be inaccurate or incomplete.
Our hospitalists thought that 38% of the curbside consultations they received should have had formal consultations. Manian and McKinsey7 reported that as many as 53% of questions asked of infectious disease consultants were thought to be too complex to be addressed in an informal consultation. Others, however, report that only 11%33% of curbside consultations were thought to require formal consultation.1, 9, 10, 16 Our hospitalists asked 3 or more questions of the consulting providers in more than 80% of the curbside consultations, suggesting that the curbside consultations we received might have had a higher complexity than those seen by others.
Our finding that information provided in curbside consultation was frequently inaccurate or incomplete is consistent with a number of previous studies reporting physicians' perceptions of the accuracy of curbside consultations.2, 3 Hospital medicine is not likely to be the only discipline affected by inaccurate curbside consultation practices, as surveys of specialists in infectious disease, gynecology, and neurosurgery report that practitioners in these disciplines have similar concerns.1, 10, 14 In a survey returned by 34 physicians, Myers1 found that 50% thought the information exchanged during curbside consultations was inaccurate, leading him to conclude that inaccuracies presented during curbside consultations required further study.
We found no way of predicting whether curbside consultations were likely to include inaccurate or incomplete information. This observation is consistent with the results of Bergus et al16 who found that the frequency of curbside consultations being converted to formal consultations was independent of the training status of the consulting physician, and with the data of Myers1 who found no way of predicting the likelihood that a curbside consultation should be converted to a formal consultation.
We found that formal consultations resulted in management changes more often than differences in recommendations (ie, 60% vs 55%, respectively). This small difference occurred because, on occasion, the formal consultations found issues to address other than the one(s) for which the curbside consultation was requested. In the majority of these instances, the management changes were minor and the curbside consultation was still felt to be sufficient.
In some instances, the advice given after the curbside and the formal consultations differed to only a minor extent (eg, varying recommendations for oral diabetes management). In other instances, however, the advice differed substantially (eg, change in antibiotic management in a septic patient with a multidrug resistant organism, when the original curbside question was for when to order a follow‐up chest roentgenogram for hypoxia; see Supporting Information, Appendix, in the online version of this article). In 26 patients (55%), formal consultation resulted in different medications being started or stopped, additional tests being performed, or different decisions being made about admission versus discharge.
Our study has a number of strengths. First, while a number of reports document that physicians' perceptions are that curbside consultations frequently contain errors,2, 3, 5, 12 to our knowledge this is the first study that prospectively compared the information collected and advice given in curbside versus formal consultation. Second, while this study was conducted as a quality improvement project, thereby requiring us to conclude that the results are not generalizable, the data presented were collected by 18 different hospitalists, reducing the potential of bias from an individual provider's knowledge base or practice. Third, there was excellent agreement between the independent reviewer and the 2 hospitalists who performed the curbside and formal consultations regarding whether a curbside consultation would have been sufficient, and whether the formal consultation changed patient management. Fourth, the study was conducted over a 1‐year period, which should have reduced potential bias arising from the increasing experience of residents requesting consultations as their training progressed.
Our study has several limitations. First, the number of curbside consultations we received during the study period (50 over 215 days) was lower than anticipated, and lower than the rates of consultation reported by others.1, 7, 9 This likely relates to the fact that, prior to beginning the study, Denver Health hospitalists already provided mandatory consultations for several surgical services (thereby reducing the number of curbside consultations received from these services), because curbside consultations received during evenings, nights, and weekends were not included in the study for reasons of convenience, and because we excluded all administrative curbside consultations. Our hospitalist service also provides consultative services 24 hours a day, thereby reducing the number of consultations received during daytime hours. Second, the frequency with which curbside consultations included inaccurate or incomplete information might be higher than what occurs in other hospitals, as Denver Health is an urban, university‐affiliated public hospital and the patients encountered may be more complex and trainees may be less adept at recognizing the information that would facilitate accurate curbside consultations (although we found no difference in the frequency with which inaccurate or incomplete information was provided as a function of the seniority of the requesting physician). Third, the disparity between curbside and formal consultations that we observed could have been biased by the Hawthorne effect. We attempted to address this by not providing the hospitalists who did the formal consultation with any information collected by the hospitalist involved with the curbside consultation, and by comparing the conclusions reached by the hospitalists performing the curbside and formal consultations with those of a third party reviewer. Fourth, while we found no association between the frequency of curbside consultations in which information was inaccurate or incomplete and the consulting service, there could be a selection bias of the consulting service requesting the curbside consultations as a result of the mandatory consultations already provided by our hospitalists. Finally, our study was not designed or adequately powered to determine why curbside consultations frequently have inaccurate or incomplete information.
In summary, we found that the information provided to hospitalists during a curbside consultation was often inaccurate and incomplete, and that these problems with information exchange adversely affected the accuracy of the resulting recommendations. While there are a number of advantages to curbside consultations,1, 3, 7, 10, 12, 13 our findings indicate that the risk associated with this practice is substantial.
Acknowledgements
Disclosure: Nothing to report.
- Curbside consultation in infectious diseases: a prospective study.J Infect Dis.1984;150:797–802. .
- Physicians' experiences and beliefs regarding informal consultation.JAMA.1998;280:900–904. , , .
- Curbside consultation practices and attitudes among primary care physicians and medical subspecialists.JAMA.1998;280:905–909. , , .
- The complexity, relative value, and financial worth of curbside consultations in an academic infectious diseases unit.Clin Infect Dis.2010;51:651–655. , , , , , .
- Curbside consultations. A closer look at a common practice.JAMA.1996;275:145–147. , .
- Informal advice‐ and information‐seeking between physicians.J Med Educ.1981;56;174–180. , , , .
- A prospective study of 2,092 “curbside” questions asked of two infectious disease consultants in private practice in the midwest.Clin Infect Dis.1996;22:303–307. , .
- Curbside consultation in endocrine practice: a prospective observational study.Endocrinologist.1996;6:328–331. , , , , .
- Informal consultations provided to general internists by the gastroenterology department of an HMO.J Gen Intern Med.1998;13:435–438. , , .
- “Curbside” consultations in gynecologic oncology: a closer look at a common practice.Gynecol Oncol.1999;74:456–459. .
- Informal consultations in infectious diseases and clinical microbiology practice.Clin Microbiol Infect.2003;9:724–726. , , , et al.
- Curbside consultations and the viaduct effect.JAMA.1998;280:929–930. .
- What do we really need to know about consultation and referral?J Gen Intern Med.1998;13:497–498. .
- Telephone advice for neurosurgical referrals. Who assumes duty of care?Br J Neurosurg.2001;15:453–455. , .
- Malpractice liability for informal consultations.Fam Med.2003;35:476–481. , .
- Does the structure of clinical questions affect the outcome of curbside consultations with specialty colleagues?Arch Fam Med.2000;9:541–547. , , , .
- Curbside consultation in infectious diseases: a prospective study.J Infect Dis.1984;150:797–802. .
- Physicians' experiences and beliefs regarding informal consultation.JAMA.1998;280:900–904. , , .
- Curbside consultation practices and attitudes among primary care physicians and medical subspecialists.JAMA.1998;280:905–909. , , .
- The complexity, relative value, and financial worth of curbside consultations in an academic infectious diseases unit.Clin Infect Dis.2010;51:651–655. , , , , , .
- Curbside consultations. A closer look at a common practice.JAMA.1996;275:145–147. , .
- Informal advice‐ and information‐seeking between physicians.J Med Educ.1981;56;174–180. , , , .
- A prospective study of 2,092 “curbside” questions asked of two infectious disease consultants in private practice in the midwest.Clin Infect Dis.1996;22:303–307. , .
- Curbside consultation in endocrine practice: a prospective observational study.Endocrinologist.1996;6:328–331. , , , , .
- Informal consultations provided to general internists by the gastroenterology department of an HMO.J Gen Intern Med.1998;13:435–438. , , .
- “Curbside” consultations in gynecologic oncology: a closer look at a common practice.Gynecol Oncol.1999;74:456–459. .
- Informal consultations in infectious diseases and clinical microbiology practice.Clin Microbiol Infect.2003;9:724–726. , , , et al.
- Curbside consultations and the viaduct effect.JAMA.1998;280:929–930. .
- What do we really need to know about consultation and referral?J Gen Intern Med.1998;13:497–498. .
- Telephone advice for neurosurgical referrals. Who assumes duty of care?Br J Neurosurg.2001;15:453–455. , .
- Malpractice liability for informal consultations.Fam Med.2003;35:476–481. , .
- Does the structure of clinical questions affect the outcome of curbside consultations with specialty colleagues?Arch Fam Med.2000;9:541–547. , , , .
Copyright © 2012 Society of Hospital Medicine
Hospitalist‐Led Medicine ED Team
Emergency department (ED) crowding leads to ambulance diversion,1 which can delay care and worsen outcomes, including mortality.2 A national survey showed that 90% of EDs were overcrowded, and 70% reported time on diversion.3 One of the causes of ED crowding is boarding of admitted patients.4 Boarding admitted patients decreases quality of care and satisfaction.57
Improved ED triage, bedside registration, physical expansion of hospitals, and regional ambulance programs have been implemented to decrease ED diversion.812 Despite these attempts, ED diversion continues to be prevalent.
Interventions involving hospitalists have been tested to improve throughput and quality of care for admitted medicine patients boarded in the ED. Howell and colleagues decreased ED diversion through active bed management by hospitalists.13 Briones and colleagues dedicated a hospitalist team to patients boarded in the ED and improved their quality of care.14
Denver Health Medical Center (DHMC) is an urban, academic safety net hospital. In 2009, the ED saw an average of 133 patients daily and an average of 25 were admitted to the medical service. DHMC's ED diversion rate was a mean of 12.4% in 2009. Boarded medicine patients occupied 16% of ED medicine bed capacity. Teaching and nonteaching medical floor teams cared for patients in the ED awaiting inpatient beds, who were the last to be seen. Nursing supervisors transferred boarded patients from the ED to hospital units. Patients with the greatest duration of time in the ED had priority for open beds.
ED diversion is costly.15, 16 DHMC implemented codified diversion criteria, calling the administrator on‐call prior to diversion, and increasing frequency of rounding in the ED, with no sustained effect seen in the rate of ED diversion.
In 2009, the DHMC Hospital Medicine Service addressed the issue of ED crowding, ED diversion, and care of boarded ED patients by creating a hospital medicine ED (HMED) team with 2 functions: (1) to provide ongoing care for medicine patients in the ED awaiting inpatient beds; and (2) to work with nursing supervisors to improve patient flow by adding physician clinical expertise to bed management.
METHODS
Setting and Design
This study took place at DHMC, a 477licensed‐bed academic safety net hospital in Denver, Colorado. We used a prepost design to assess measures of patient flow and timeliness of care. We surveyed ED attendings and nursing supervisors after the intervention to determine perceptions of the HMED team. This study was approved by the local institutional review board (IRB protocol number 09‐0892).
Intervention
In 2009, DHMC, which uses Toyota Lean for quality improvement, performed a Rapid Improvement Event (RIE) to address ED diversion and care of admitted patients boarded in the ED. The RIE team consisted of hospital medicine physicians, ED physicians, social workers, and nurses. Over a 4‐day period, the team examined the present state, created an ideal future state, devised a solution, and tested this solution.
Based upon the results of the RIE, DHMC implemented an HMED team to care for admitted patients boarded in the ED and assist in active bed management. The HMED team is a 24/7 service. During the day shift, the HMED team is composed of 1 dedicated attending and 1 allied health provider (AHP). Since the medicine services were already staffing existing patients in the ED, the 2.0 full‐time equivalent (FTE) needed to staff the HMED team attending and the AHP was reallocated from existing FTE within the hospitalist division. During the evening and night shifts, the HMED team's responsibilities were rolled into existing hospitalist duties.
The HMED team provides clinical care for 2 groups of patients in the ED. The first group represents admitted patients who are still awaiting a medicine ward bed as of 7:00 AM. The HMED team provides ongoing care until discharge from the ED or transfer to a medicine floor. The second group of patients includes new admissions that need to stay in the ED due to a lack of available medicine floor beds. For these patients, the HMED team initiates and continues care until discharge from the ED or transfer to a medical floor (Figure 1).
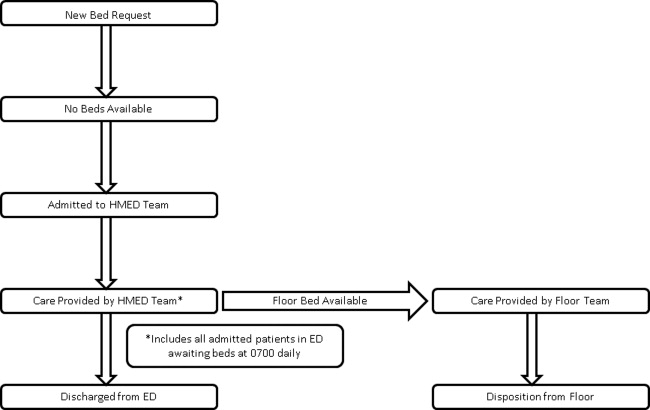
The physician on the HMED team assists nursing supervisors with bed management by providing detailed clinical knowledge, including proximity to discharge as well as updated information on telemetry and intensive care unit (ICU) appropriateness. The HMED team's physician maintains constant knowledge of hospital census via an electronic bed board, and communicates regularly with medical floors about anticipated discharges and transfers to understand the hospital's patient flow status (Figure 2).
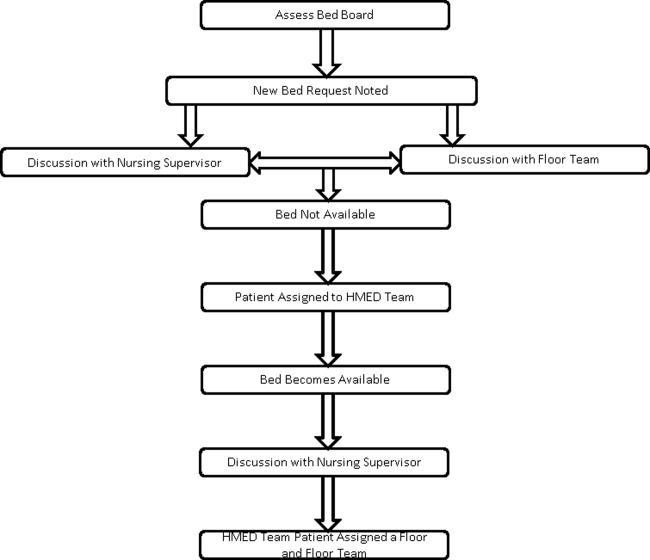
The RIE that resulted in the HMED team was part of the Inpatient Medicine Value Stream, which had the overall goal of saving DHMC $300,000 for 2009. Ten RIEs were planned for this value stream in 2009, with an average of $30,000 of savings expected from each RIE.
Determination of ED Diversion Time
DHMC places responsibility for putting the hospital on an ED Diversion status in the hands of the Emergency Medicine Attending Physician. Diversion is categorized as either due to: (1) excessive ED volume for available ED bedsfull or nearly full department, or full resuscitation rooms without the ability to release a room; or (2) excessive boardingmore than 12 admitted patients awaiting beds in the ED. Other reasons for diversion, such as acute, excessive resource utilization (multiple patients from a single event) and temporary limitation of resources (critical equipment becoming inoperative), are also infrequent causes of diversion that are recorded. The elapsed time during which the ED is on diversion status is recorded and reported as a percentage of the total time on a monthly basis.
Determination of ED Diversion Costs
The cost of diversion at DHMC is calculated by multiplying the average number of ambulance drop‐offs per hour times the number of diversion hours to determine the number of missed patients. The historical mean charges for each ambulance patient are used to determine total missed charge opportunity, which is then applied to the hospital realization rate to calculate missed revenue. In addition, the marginal costs related to Denver Health Medical Plan patients that were unable to be repatriated to DHMC from outlying hospitals, as a result of diversion, is added to the net missed revenue figure. This figure is then divided by the number of diversion hours for the year to determine the cost of each diversion hour. For 2009, the cost of each hour of diversion at DHMC was $5000.
Statistical Analysis
All analyses were performed using SAS Enterprise Guide 4.1 (SAS Institute, Inc, Cary, NC). A Student t test or Wilcoxon rank sum test was used to compare continuous variables, and a chi‐square test was used to compare categorical variables.
Our primary outcome was ED diversion due to hospital bed capacity. These data are recorded, maintained, and analyzed by a DHMC internally developed emergency medical services information system (EMeSIS) that interfaces with computerized laboratory reporting systems, and stores, in part, demographic data as well as real‐time data related to the timing of patient encounters for all patients evaluated in the ED. To assess the effect of the intervention on ED diversion, the proportion of total hours on diversion due to medicine bed capacity was compared preimplementation and postimplementation with a chi‐squared test.
Secondary outcomes for patient flow included: (1) the proportion of patients discharged within 8 hours of transfer to a medical floor; and (2) the proportion of admitted medicine patients discharged from the ED. These data were gathered from the Denver Health Data Warehouse which pools data from both administrative and clinical applications used in patient care. Chi‐squared tests were also used to compare secondary outcomes preintervention and postintervention.
To measure the quality and safety of the HMED team, pre‐ED and post‐ED length of stay (LOS), 48‐hour patient return rate, intensive care unit (ICU) transfer rate, and the total LOS for patients admitted to the HMED team and handed off to a medicine floor team were assessed with the Student t test. To assess timeliness of clinical care provided to boarded medicine patients, self‐reported rounding times were compared preintervention and postintervention with the Student t test.
To assess satisfaction with the HMED team, an anonymous paper survey was administered to ED attendings and nursing supervisors 1 year after the intervention was introduced. The survey consisted of 5 questions, and used a 5‐point Likert scale ranging from strongly disagree (1) to strongly agree (5). Those answering agree or strongly agree were compared to those who were neutral, disagreed, or strongly disagreed.
RESULTS
The ED saw 48,595 patients during the intervention period (August 1, 2009June 30,2010) which did not differ statistically from the 50,469 patients seen in the control period (August 1, 2008June 30, 2009). The number of admissions to the medicine service during the control period (9727) and intervention period (10,013), and the number of total medical/surgical admissions during the control (20,716) and intervention (20,574) periods did not statistically differ. ED staffing during the intervention did not change. The overall number of licensed beds did not increase during the study period. During the control period, staffed medical/surgical beds increased from 395 to 400 beds, while the number of staffed medical/surgical beds decreased from 400 to 397 beds during the intervention period. Patient characteristics were similar during the 2 time periods, with the exception of race (Table 1).
Patients Admitted to Medicine and Transferred to a Medicine Floor | Pre | Post | P Value |
---|---|---|---|
| |||
No. | 1901 | 1828 | |
Age* | 53 15 | 54 14 | 0.59 |
Gender (% male) | 55% | 52% | 0.06 |
Race (% white) | 40% | 34% | <0.0001 |
Insurance (% insured) | 67% | 63% | 0.08 |
Charlson Comorbidity Index | 1.0 [1.0, 1.0] | 1.0 [1.0, 1.0] | 0.52 |
Diversion Hours
After implementation of the HMED team, there was a relative reduction of diversion due to medicine bed capacity of 27% (4.5%3.3%; P < 0.01) (Table 2). During the same time period, the relative proportion of hours on diversion due to ED capacity decreased by 55% (9.9%5.4%).
Pre | Post | P Value | |
---|---|---|---|
| |||
Divert hours due to bed capacity (%, hours)* | 4.5% (3624) | 3.3% (3624) | 0.009 |
Admitted ED patients transferred to floor | |||
Discharged within 8 h (%, N) | 1.3% (1901) | 0.5% (1828) | 0.03 |
Boarded patients rounded on in the ED and transferred to the medical floor | |||
Total length of stay (days, N) | 2.6 [2.4, 3.2] (154) | 2.5 [2.4, 2.6] (364) | 0.21 |
All discharges and transfers to the floor | |||
Discharged from ED [%, (N)] | 4.9% (2009) | 7.5% (1981) | <0.001 |
ED length of stay [hours, (N)] | 12:09 8:44 (2009) | 12:48 10:00 (1981) | 0.46 |
Return to hospital <48 h [%, (N)] | 4.6% (2009) | 4.8% (1981) | 0.75 |
Transfer to the ICU [%, (N)] | 3.3% (2009) | 4.2% (1981) | 0.13 |
Bed Management and Patient Flow
The HMED team rounded on boarded ED patients a mean of 2 hours and 9 minutes earlier (10:59 AM 1:09 vs 8:50 AM 1:20; P < 0.0001). After implementation of the HMED team, patients transferred to a medicine floor and discharged within 8 hours decreased relatively by 67% (1.5%0.5%; P < 0.01), and discharges from the ED of admitted medicine patients increased relatively by 61% (4.9%7.9%; P < 0.001) (Table 2). ED LOS, total LOS, 48‐hour returns to the ED, and ICU transfer rate for patients managed by the HMED team did not change (Table 2).
Perception and Satisfaction
Nine out of 15 (60%) ED attendings and 7 out of 8 (87%) nursing supervisors responded to the survey. The survey demonstrated that ED attendings and nursing supervisors believe the HMED team improves clinical care for boarded patients, communication, collegiality, and patient flow (Table 3).
Postimplementation of the HMED Team | Total (n = 16) | ED Attendings (n = 9) | Nursing Supervisors (n = 7) |
---|---|---|---|
| |||
Quality of care has improved | 94 | 89 | 100 |
Communication has improved | 94 | 89 | 100 |
Collegiality and clinical decision‐making has improved | 94 | 100 | 89 |
Patient flow has improved | 81 | 67 | 100 |
HMED team is an asset to DHMC | 94 | 89 | 100 |
Financial
The 27% relative reduction in ED diversion due to hospital bed capacity extrapolates to 105.1 hours a year of decreased diversion, accounting for $525,600 of increased annual revenues.
DISCUSSION
This study suggests that an HMED team can decrease ED diversion, due to hospital bed capacity, by improving patient flow and timeliness of care for boarded medicine patients in the ED.
After participating in bed management, ED diversion due to a lack of medicine beds decreased. This is consistent with findings by Howell and colleagues who were able to improve throughput and decrease ED diversion with active bed management.13 Howell and colleagues decreased diversion hours due to temporary ED overload, and diversion hours due to a lack of telemetry or critical care beds. At DHMC, diversion is attributed to either a lack of ED capacity or lack of hospital beds. The primary outcome was the diversion rate due to lack of hospital beds, but it is possible that increased discharges directly from the ED contributed to the decrease in diversion due to ED capacity, underestimating the effect our intervention had on total ED diversion. There were no other initiatives to decrease diversion due to ED capacity during the study periods, and ED capacity and volume did not change during the intervention period.
While there were no statistically significant changes in staffed medical/surgical beds or medicine admissions, staffed medical/surgical beds during the intervention period decreased while there were more admissions to medicine. Both of these variables would increase diversion, resulting in an underestimation of the effect of the intervention.
Howell and colleagues improved throughput in the ED by implementing a service which provided active bed management without clinical responsibilities,13 while Briones and colleagues improved clinical care of patients boarded in the ED without affecting throughput.14 The HMED team improved throughput and decreased ED diversion while improving timeliness of care and perception of care quality for patients boarding in the ED.
By decreasing unnecessary transfers to medicine units and increasing discharges from the ED, patient flow was improved. While there was no difference in ED LOS, there was a trend towards decreased total LOS. A larger sample size or a longer period of observation would be necessary to determine if the trend toward decreased total LOS is statistically significant. ED LOS may not have been decreased because patients who would have been sent to the floor only to be discharged within 8 hours were kept in the ED to expedite testing and discharge, while sicker patients were sent to the medical floor. This decreased the turnover time of inpatient beds and allowed more boarded patients to be moved to floor units.
There was concern that an HMED team would fragment care, which would lead to an increased LOS for those patients who were transferred to a medical floor and cared for by an additional medicine team before discharge.17 As noted, there was a trend towards a decreased LOS for patients initially cared for by the HMED team.
In this intervention, hospital medicine physicians provided information regarding ongoing care of patients boarded in the ED to nursing supervisors. Prior to the intervention, nursing supervisors relied upon information from the ED staff and the boarded patient's time in the ED to assign a medical floor. However, ED staff was not providing care to boarded patients and did not know the most up‐to‐date status of the patient. This queuing process and lack of communication resulted in patients ready for discharge being transferred to floor beds and discharged within a few hours of transfer. The HMED team allowed nursing supervisors to have direct knowledge regarding clinical status, including telemetry and ICU criteria (similar to Howell and colleagues13), and readiness for discharge from the physician taking care of the patient.
By managing boarded patients, an HMED team can improve timeliness and coordination of care. Prior to the intervention, boarded ED patients were the last to be seen on rounds. The HMED team rounds only in the ED, expediting care and discharges. The increased proportion of boarded patients discharged from the ED by the HMED team is consistent with Briones and colleagues' clinically oriented team managing boarding patients in the ED.14
Potential adverse effects of our intervention included increased returns to the ED, increased ICU transfer rate, and decreased housestaff satisfaction. There was no increase in the 48‐hour return rate and no increase in the ICU transfer rate for patients cared for by the HMED team. Housestaff at DHMC are satisfied with the HMED team, since the presence of the HMED team allows them to concentrate on patients on the medical floors.
This intervention provides DHMC with an additional $525,600 in revenue annually. Since existing FTE were reallocated to create the HMED team, no additional FTE were required. In our facility, AHPs take on duties of housestaff. However, only 1 physician may be needed to staff an HMED team. This physician's clinical productivity is about 75% of other physicians; therefore, 25% of time is spent in bed management. At DHMC, other medicine teams picked up for the decreased clinical productivity of the HMED team, so the budget was neutral. However, using 2 FTE to staff 1 physician daily for 365 days a year, one would need to allocate 0.5 physician FTE (0.25 decrease in clinical productivity 2 FTE) for an HMED team.
Our study has several limitations. As a single center study, our findings may not extrapolate to other settings. The study used historical controls, therefore, undetected confounders may exist. We could not control for simultaneous changes in the hospital, however, we did not know of any other concurrent interventions aimed at decreasing ED diversion. Also, the decision to admit or not is partially based on individual ED attendings, which causes variability in practice. Finally, while we were able to measure rounding times as a process measure to reflect timeliness of care and staff perceptions of quality of care, due to our data infrastructure and the way our housestaff and attendings rotate, we were not able to assess more downstream measures of quality of care.
CONCLUSION
ED crowding decreases throughput and worsens clinical care; there are few proven solutions. This study demonstrates an intervention that reduced the percentage of patients transferred to a medicine floor and discharged within 8 hours, increased the number of discharges from the ED of admitted medicine patients, and decreased ED diversion while improving the timeliness of clinical care for patients boarded in the ED.
Acknowledgements
Disclosure: Nothing to report.
- Access block causes emergency department overcrowding and ambulance diversion in Perth, Western Australia.Emerg Med J.2005;22:351–354. , , .
- The relationship between distance to hospital and patient mortality in emergencies: an observational study.Emerg Med J.2007;24:665–668. , , , .
- Institute of Medicine.Committee on the Future of Emergency Care in the United States Health System.Hospital‐Based Emergency Care: At the Breaking Point.Washington, DC:National Academies Press;2007.
- Systematic review of emergency department crowding: causes, effects, and solutions.Ann Emerg Med.2008;52:126–136. , .
- Emergency department crowding is associated with poor care for patients with severe pain.Ann Emerg Med.2008;51:1–5. , .
- The impact of emergency department crowding measures on time to antibiotics for patients with community‐acquired pneumonia.Ann Emerg Med.2007;50:510–516. , , , et al.
- for the DELAYED‐ED Study Group.Impact of delayed transfer of critically ill patients from the emergency department to the intensive care unit.Crit Care Med.2007;35:1477–1483. , , , et al;
- Impact of a triage liaison physician on emergency department overcrowding and throughput: a randomized controlled trial.Acad Emerg Med.2007;14:702–708. , , , et al.
- Strategies for dealing with emergency department overcrowding: a one‐year study on how bedside registration affects patient throughput times.Emerg Med J.2007;32:337–342. , , .
- The effect of emergency department expansion on emergency department overcrowding.Acad Emerg Med.2007;14:338–343. , , , et al.
- Effect of increased ICU capacity on emergency department length of stay and ambulance diversion.Ann Emerg Med.2005;5:471–478. , , , , , .
- Ambulance diversion reduction: the Sacramento solution.Am J Emerg Med.2006;357:608–613. , , , , .
- Active bed management by hospitalists and emergency department throughput.Ann Intern Med.2008;149:804–810. , , , , , .
- A model of hospitalist role in the care of admitted patients in the emergency department.J Hosp Med.2010;5:360–364. , , , et al.
- Ambulance diversion and lost hospital revenues.Ann Emerg Med.2006;48(6):702–710. , , , , .
- The financial impact of ambulance diversion and patient elopements.Acad Emerg Med.2007;14(1):58–62. , , , .
- The impact of fragmentation of hospitalist care on length of stay.J. Hosp. Med.2010;5:335–338. , , , , .
Emergency department (ED) crowding leads to ambulance diversion,1 which can delay care and worsen outcomes, including mortality.2 A national survey showed that 90% of EDs were overcrowded, and 70% reported time on diversion.3 One of the causes of ED crowding is boarding of admitted patients.4 Boarding admitted patients decreases quality of care and satisfaction.57
Improved ED triage, bedside registration, physical expansion of hospitals, and regional ambulance programs have been implemented to decrease ED diversion.812 Despite these attempts, ED diversion continues to be prevalent.
Interventions involving hospitalists have been tested to improve throughput and quality of care for admitted medicine patients boarded in the ED. Howell and colleagues decreased ED diversion through active bed management by hospitalists.13 Briones and colleagues dedicated a hospitalist team to patients boarded in the ED and improved their quality of care.14
Denver Health Medical Center (DHMC) is an urban, academic safety net hospital. In 2009, the ED saw an average of 133 patients daily and an average of 25 were admitted to the medical service. DHMC's ED diversion rate was a mean of 12.4% in 2009. Boarded medicine patients occupied 16% of ED medicine bed capacity. Teaching and nonteaching medical floor teams cared for patients in the ED awaiting inpatient beds, who were the last to be seen. Nursing supervisors transferred boarded patients from the ED to hospital units. Patients with the greatest duration of time in the ED had priority for open beds.
ED diversion is costly.15, 16 DHMC implemented codified diversion criteria, calling the administrator on‐call prior to diversion, and increasing frequency of rounding in the ED, with no sustained effect seen in the rate of ED diversion.
In 2009, the DHMC Hospital Medicine Service addressed the issue of ED crowding, ED diversion, and care of boarded ED patients by creating a hospital medicine ED (HMED) team with 2 functions: (1) to provide ongoing care for medicine patients in the ED awaiting inpatient beds; and (2) to work with nursing supervisors to improve patient flow by adding physician clinical expertise to bed management.
METHODS
Setting and Design
This study took place at DHMC, a 477licensed‐bed academic safety net hospital in Denver, Colorado. We used a prepost design to assess measures of patient flow and timeliness of care. We surveyed ED attendings and nursing supervisors after the intervention to determine perceptions of the HMED team. This study was approved by the local institutional review board (IRB protocol number 09‐0892).
Intervention
In 2009, DHMC, which uses Toyota Lean for quality improvement, performed a Rapid Improvement Event (RIE) to address ED diversion and care of admitted patients boarded in the ED. The RIE team consisted of hospital medicine physicians, ED physicians, social workers, and nurses. Over a 4‐day period, the team examined the present state, created an ideal future state, devised a solution, and tested this solution.
Based upon the results of the RIE, DHMC implemented an HMED team to care for admitted patients boarded in the ED and assist in active bed management. The HMED team is a 24/7 service. During the day shift, the HMED team is composed of 1 dedicated attending and 1 allied health provider (AHP). Since the medicine services were already staffing existing patients in the ED, the 2.0 full‐time equivalent (FTE) needed to staff the HMED team attending and the AHP was reallocated from existing FTE within the hospitalist division. During the evening and night shifts, the HMED team's responsibilities were rolled into existing hospitalist duties.
The HMED team provides clinical care for 2 groups of patients in the ED. The first group represents admitted patients who are still awaiting a medicine ward bed as of 7:00 AM. The HMED team provides ongoing care until discharge from the ED or transfer to a medicine floor. The second group of patients includes new admissions that need to stay in the ED due to a lack of available medicine floor beds. For these patients, the HMED team initiates and continues care until discharge from the ED or transfer to a medical floor (Figure 1).
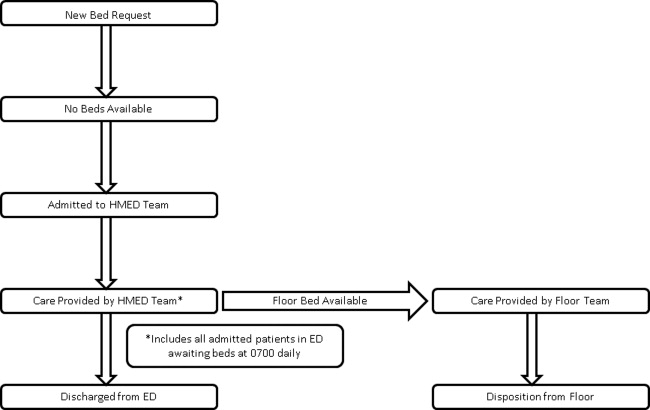
The physician on the HMED team assists nursing supervisors with bed management by providing detailed clinical knowledge, including proximity to discharge as well as updated information on telemetry and intensive care unit (ICU) appropriateness. The HMED team's physician maintains constant knowledge of hospital census via an electronic bed board, and communicates regularly with medical floors about anticipated discharges and transfers to understand the hospital's patient flow status (Figure 2).
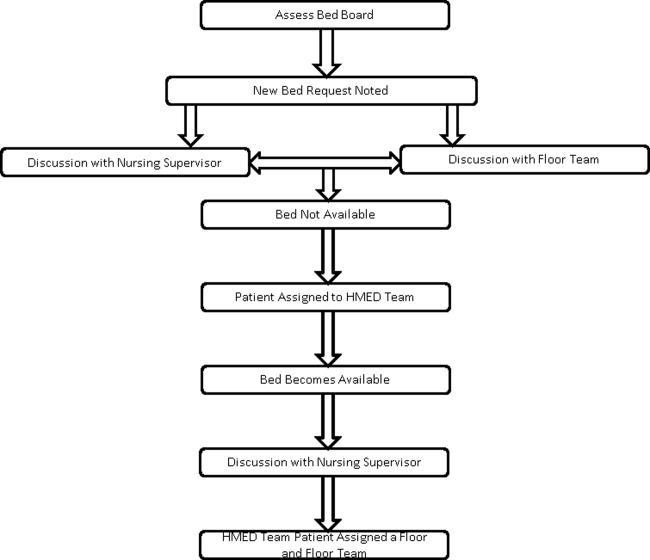
The RIE that resulted in the HMED team was part of the Inpatient Medicine Value Stream, which had the overall goal of saving DHMC $300,000 for 2009. Ten RIEs were planned for this value stream in 2009, with an average of $30,000 of savings expected from each RIE.
Determination of ED Diversion Time
DHMC places responsibility for putting the hospital on an ED Diversion status in the hands of the Emergency Medicine Attending Physician. Diversion is categorized as either due to: (1) excessive ED volume for available ED bedsfull or nearly full department, or full resuscitation rooms without the ability to release a room; or (2) excessive boardingmore than 12 admitted patients awaiting beds in the ED. Other reasons for diversion, such as acute, excessive resource utilization (multiple patients from a single event) and temporary limitation of resources (critical equipment becoming inoperative), are also infrequent causes of diversion that are recorded. The elapsed time during which the ED is on diversion status is recorded and reported as a percentage of the total time on a monthly basis.
Determination of ED Diversion Costs
The cost of diversion at DHMC is calculated by multiplying the average number of ambulance drop‐offs per hour times the number of diversion hours to determine the number of missed patients. The historical mean charges for each ambulance patient are used to determine total missed charge opportunity, which is then applied to the hospital realization rate to calculate missed revenue. In addition, the marginal costs related to Denver Health Medical Plan patients that were unable to be repatriated to DHMC from outlying hospitals, as a result of diversion, is added to the net missed revenue figure. This figure is then divided by the number of diversion hours for the year to determine the cost of each diversion hour. For 2009, the cost of each hour of diversion at DHMC was $5000.
Statistical Analysis
All analyses were performed using SAS Enterprise Guide 4.1 (SAS Institute, Inc, Cary, NC). A Student t test or Wilcoxon rank sum test was used to compare continuous variables, and a chi‐square test was used to compare categorical variables.
Our primary outcome was ED diversion due to hospital bed capacity. These data are recorded, maintained, and analyzed by a DHMC internally developed emergency medical services information system (EMeSIS) that interfaces with computerized laboratory reporting systems, and stores, in part, demographic data as well as real‐time data related to the timing of patient encounters for all patients evaluated in the ED. To assess the effect of the intervention on ED diversion, the proportion of total hours on diversion due to medicine bed capacity was compared preimplementation and postimplementation with a chi‐squared test.
Secondary outcomes for patient flow included: (1) the proportion of patients discharged within 8 hours of transfer to a medical floor; and (2) the proportion of admitted medicine patients discharged from the ED. These data were gathered from the Denver Health Data Warehouse which pools data from both administrative and clinical applications used in patient care. Chi‐squared tests were also used to compare secondary outcomes preintervention and postintervention.
To measure the quality and safety of the HMED team, pre‐ED and post‐ED length of stay (LOS), 48‐hour patient return rate, intensive care unit (ICU) transfer rate, and the total LOS for patients admitted to the HMED team and handed off to a medicine floor team were assessed with the Student t test. To assess timeliness of clinical care provided to boarded medicine patients, self‐reported rounding times were compared preintervention and postintervention with the Student t test.
To assess satisfaction with the HMED team, an anonymous paper survey was administered to ED attendings and nursing supervisors 1 year after the intervention was introduced. The survey consisted of 5 questions, and used a 5‐point Likert scale ranging from strongly disagree (1) to strongly agree (5). Those answering agree or strongly agree were compared to those who were neutral, disagreed, or strongly disagreed.
RESULTS
The ED saw 48,595 patients during the intervention period (August 1, 2009June 30,2010) which did not differ statistically from the 50,469 patients seen in the control period (August 1, 2008June 30, 2009). The number of admissions to the medicine service during the control period (9727) and intervention period (10,013), and the number of total medical/surgical admissions during the control (20,716) and intervention (20,574) periods did not statistically differ. ED staffing during the intervention did not change. The overall number of licensed beds did not increase during the study period. During the control period, staffed medical/surgical beds increased from 395 to 400 beds, while the number of staffed medical/surgical beds decreased from 400 to 397 beds during the intervention period. Patient characteristics were similar during the 2 time periods, with the exception of race (Table 1).
Patients Admitted to Medicine and Transferred to a Medicine Floor | Pre | Post | P Value |
---|---|---|---|
| |||
No. | 1901 | 1828 | |
Age* | 53 15 | 54 14 | 0.59 |
Gender (% male) | 55% | 52% | 0.06 |
Race (% white) | 40% | 34% | <0.0001 |
Insurance (% insured) | 67% | 63% | 0.08 |
Charlson Comorbidity Index | 1.0 [1.0, 1.0] | 1.0 [1.0, 1.0] | 0.52 |
Diversion Hours
After implementation of the HMED team, there was a relative reduction of diversion due to medicine bed capacity of 27% (4.5%3.3%; P < 0.01) (Table 2). During the same time period, the relative proportion of hours on diversion due to ED capacity decreased by 55% (9.9%5.4%).
Pre | Post | P Value | |
---|---|---|---|
| |||
Divert hours due to bed capacity (%, hours)* | 4.5% (3624) | 3.3% (3624) | 0.009 |
Admitted ED patients transferred to floor | |||
Discharged within 8 h (%, N) | 1.3% (1901) | 0.5% (1828) | 0.03 |
Boarded patients rounded on in the ED and transferred to the medical floor | |||
Total length of stay (days, N) | 2.6 [2.4, 3.2] (154) | 2.5 [2.4, 2.6] (364) | 0.21 |
All discharges and transfers to the floor | |||
Discharged from ED [%, (N)] | 4.9% (2009) | 7.5% (1981) | <0.001 |
ED length of stay [hours, (N)] | 12:09 8:44 (2009) | 12:48 10:00 (1981) | 0.46 |
Return to hospital <48 h [%, (N)] | 4.6% (2009) | 4.8% (1981) | 0.75 |
Transfer to the ICU [%, (N)] | 3.3% (2009) | 4.2% (1981) | 0.13 |
Bed Management and Patient Flow
The HMED team rounded on boarded ED patients a mean of 2 hours and 9 minutes earlier (10:59 AM 1:09 vs 8:50 AM 1:20; P < 0.0001). After implementation of the HMED team, patients transferred to a medicine floor and discharged within 8 hours decreased relatively by 67% (1.5%0.5%; P < 0.01), and discharges from the ED of admitted medicine patients increased relatively by 61% (4.9%7.9%; P < 0.001) (Table 2). ED LOS, total LOS, 48‐hour returns to the ED, and ICU transfer rate for patients managed by the HMED team did not change (Table 2).
Perception and Satisfaction
Nine out of 15 (60%) ED attendings and 7 out of 8 (87%) nursing supervisors responded to the survey. The survey demonstrated that ED attendings and nursing supervisors believe the HMED team improves clinical care for boarded patients, communication, collegiality, and patient flow (Table 3).
Postimplementation of the HMED Team | Total (n = 16) | ED Attendings (n = 9) | Nursing Supervisors (n = 7) |
---|---|---|---|
| |||
Quality of care has improved | 94 | 89 | 100 |
Communication has improved | 94 | 89 | 100 |
Collegiality and clinical decision‐making has improved | 94 | 100 | 89 |
Patient flow has improved | 81 | 67 | 100 |
HMED team is an asset to DHMC | 94 | 89 | 100 |
Financial
The 27% relative reduction in ED diversion due to hospital bed capacity extrapolates to 105.1 hours a year of decreased diversion, accounting for $525,600 of increased annual revenues.
DISCUSSION
This study suggests that an HMED team can decrease ED diversion, due to hospital bed capacity, by improving patient flow and timeliness of care for boarded medicine patients in the ED.
After participating in bed management, ED diversion due to a lack of medicine beds decreased. This is consistent with findings by Howell and colleagues who were able to improve throughput and decrease ED diversion with active bed management.13 Howell and colleagues decreased diversion hours due to temporary ED overload, and diversion hours due to a lack of telemetry or critical care beds. At DHMC, diversion is attributed to either a lack of ED capacity or lack of hospital beds. The primary outcome was the diversion rate due to lack of hospital beds, but it is possible that increased discharges directly from the ED contributed to the decrease in diversion due to ED capacity, underestimating the effect our intervention had on total ED diversion. There were no other initiatives to decrease diversion due to ED capacity during the study periods, and ED capacity and volume did not change during the intervention period.
While there were no statistically significant changes in staffed medical/surgical beds or medicine admissions, staffed medical/surgical beds during the intervention period decreased while there were more admissions to medicine. Both of these variables would increase diversion, resulting in an underestimation of the effect of the intervention.
Howell and colleagues improved throughput in the ED by implementing a service which provided active bed management without clinical responsibilities,13 while Briones and colleagues improved clinical care of patients boarded in the ED without affecting throughput.14 The HMED team improved throughput and decreased ED diversion while improving timeliness of care and perception of care quality for patients boarding in the ED.
By decreasing unnecessary transfers to medicine units and increasing discharges from the ED, patient flow was improved. While there was no difference in ED LOS, there was a trend towards decreased total LOS. A larger sample size or a longer period of observation would be necessary to determine if the trend toward decreased total LOS is statistically significant. ED LOS may not have been decreased because patients who would have been sent to the floor only to be discharged within 8 hours were kept in the ED to expedite testing and discharge, while sicker patients were sent to the medical floor. This decreased the turnover time of inpatient beds and allowed more boarded patients to be moved to floor units.
There was concern that an HMED team would fragment care, which would lead to an increased LOS for those patients who were transferred to a medical floor and cared for by an additional medicine team before discharge.17 As noted, there was a trend towards a decreased LOS for patients initially cared for by the HMED team.
In this intervention, hospital medicine physicians provided information regarding ongoing care of patients boarded in the ED to nursing supervisors. Prior to the intervention, nursing supervisors relied upon information from the ED staff and the boarded patient's time in the ED to assign a medical floor. However, ED staff was not providing care to boarded patients and did not know the most up‐to‐date status of the patient. This queuing process and lack of communication resulted in patients ready for discharge being transferred to floor beds and discharged within a few hours of transfer. The HMED team allowed nursing supervisors to have direct knowledge regarding clinical status, including telemetry and ICU criteria (similar to Howell and colleagues13), and readiness for discharge from the physician taking care of the patient.
By managing boarded patients, an HMED team can improve timeliness and coordination of care. Prior to the intervention, boarded ED patients were the last to be seen on rounds. The HMED team rounds only in the ED, expediting care and discharges. The increased proportion of boarded patients discharged from the ED by the HMED team is consistent with Briones and colleagues' clinically oriented team managing boarding patients in the ED.14
Potential adverse effects of our intervention included increased returns to the ED, increased ICU transfer rate, and decreased housestaff satisfaction. There was no increase in the 48‐hour return rate and no increase in the ICU transfer rate for patients cared for by the HMED team. Housestaff at DHMC are satisfied with the HMED team, since the presence of the HMED team allows them to concentrate on patients on the medical floors.
This intervention provides DHMC with an additional $525,600 in revenue annually. Since existing FTE were reallocated to create the HMED team, no additional FTE were required. In our facility, AHPs take on duties of housestaff. However, only 1 physician may be needed to staff an HMED team. This physician's clinical productivity is about 75% of other physicians; therefore, 25% of time is spent in bed management. At DHMC, other medicine teams picked up for the decreased clinical productivity of the HMED team, so the budget was neutral. However, using 2 FTE to staff 1 physician daily for 365 days a year, one would need to allocate 0.5 physician FTE (0.25 decrease in clinical productivity 2 FTE) for an HMED team.
Our study has several limitations. As a single center study, our findings may not extrapolate to other settings. The study used historical controls, therefore, undetected confounders may exist. We could not control for simultaneous changes in the hospital, however, we did not know of any other concurrent interventions aimed at decreasing ED diversion. Also, the decision to admit or not is partially based on individual ED attendings, which causes variability in practice. Finally, while we were able to measure rounding times as a process measure to reflect timeliness of care and staff perceptions of quality of care, due to our data infrastructure and the way our housestaff and attendings rotate, we were not able to assess more downstream measures of quality of care.
CONCLUSION
ED crowding decreases throughput and worsens clinical care; there are few proven solutions. This study demonstrates an intervention that reduced the percentage of patients transferred to a medicine floor and discharged within 8 hours, increased the number of discharges from the ED of admitted medicine patients, and decreased ED diversion while improving the timeliness of clinical care for patients boarded in the ED.
Acknowledgements
Disclosure: Nothing to report.
Emergency department (ED) crowding leads to ambulance diversion,1 which can delay care and worsen outcomes, including mortality.2 A national survey showed that 90% of EDs were overcrowded, and 70% reported time on diversion.3 One of the causes of ED crowding is boarding of admitted patients.4 Boarding admitted patients decreases quality of care and satisfaction.57
Improved ED triage, bedside registration, physical expansion of hospitals, and regional ambulance programs have been implemented to decrease ED diversion.812 Despite these attempts, ED diversion continues to be prevalent.
Interventions involving hospitalists have been tested to improve throughput and quality of care for admitted medicine patients boarded in the ED. Howell and colleagues decreased ED diversion through active bed management by hospitalists.13 Briones and colleagues dedicated a hospitalist team to patients boarded in the ED and improved their quality of care.14
Denver Health Medical Center (DHMC) is an urban, academic safety net hospital. In 2009, the ED saw an average of 133 patients daily and an average of 25 were admitted to the medical service. DHMC's ED diversion rate was a mean of 12.4% in 2009. Boarded medicine patients occupied 16% of ED medicine bed capacity. Teaching and nonteaching medical floor teams cared for patients in the ED awaiting inpatient beds, who were the last to be seen. Nursing supervisors transferred boarded patients from the ED to hospital units. Patients with the greatest duration of time in the ED had priority for open beds.
ED diversion is costly.15, 16 DHMC implemented codified diversion criteria, calling the administrator on‐call prior to diversion, and increasing frequency of rounding in the ED, with no sustained effect seen in the rate of ED diversion.
In 2009, the DHMC Hospital Medicine Service addressed the issue of ED crowding, ED diversion, and care of boarded ED patients by creating a hospital medicine ED (HMED) team with 2 functions: (1) to provide ongoing care for medicine patients in the ED awaiting inpatient beds; and (2) to work with nursing supervisors to improve patient flow by adding physician clinical expertise to bed management.
METHODS
Setting and Design
This study took place at DHMC, a 477licensed‐bed academic safety net hospital in Denver, Colorado. We used a prepost design to assess measures of patient flow and timeliness of care. We surveyed ED attendings and nursing supervisors after the intervention to determine perceptions of the HMED team. This study was approved by the local institutional review board (IRB protocol number 09‐0892).
Intervention
In 2009, DHMC, which uses Toyota Lean for quality improvement, performed a Rapid Improvement Event (RIE) to address ED diversion and care of admitted patients boarded in the ED. The RIE team consisted of hospital medicine physicians, ED physicians, social workers, and nurses. Over a 4‐day period, the team examined the present state, created an ideal future state, devised a solution, and tested this solution.
Based upon the results of the RIE, DHMC implemented an HMED team to care for admitted patients boarded in the ED and assist in active bed management. The HMED team is a 24/7 service. During the day shift, the HMED team is composed of 1 dedicated attending and 1 allied health provider (AHP). Since the medicine services were already staffing existing patients in the ED, the 2.0 full‐time equivalent (FTE) needed to staff the HMED team attending and the AHP was reallocated from existing FTE within the hospitalist division. During the evening and night shifts, the HMED team's responsibilities were rolled into existing hospitalist duties.
The HMED team provides clinical care for 2 groups of patients in the ED. The first group represents admitted patients who are still awaiting a medicine ward bed as of 7:00 AM. The HMED team provides ongoing care until discharge from the ED or transfer to a medicine floor. The second group of patients includes new admissions that need to stay in the ED due to a lack of available medicine floor beds. For these patients, the HMED team initiates and continues care until discharge from the ED or transfer to a medical floor (Figure 1).
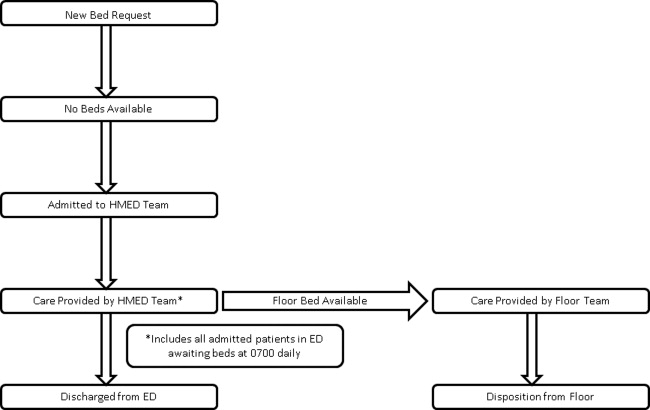
The physician on the HMED team assists nursing supervisors with bed management by providing detailed clinical knowledge, including proximity to discharge as well as updated information on telemetry and intensive care unit (ICU) appropriateness. The HMED team's physician maintains constant knowledge of hospital census via an electronic bed board, and communicates regularly with medical floors about anticipated discharges and transfers to understand the hospital's patient flow status (Figure 2).
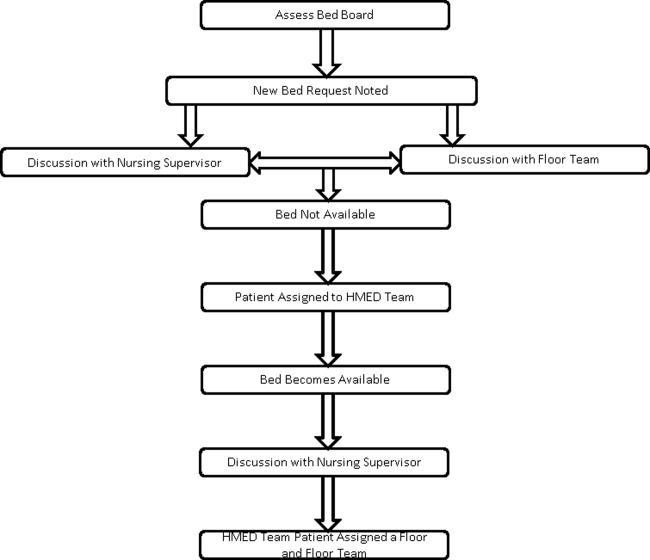
The RIE that resulted in the HMED team was part of the Inpatient Medicine Value Stream, which had the overall goal of saving DHMC $300,000 for 2009. Ten RIEs were planned for this value stream in 2009, with an average of $30,000 of savings expected from each RIE.
Determination of ED Diversion Time
DHMC places responsibility for putting the hospital on an ED Diversion status in the hands of the Emergency Medicine Attending Physician. Diversion is categorized as either due to: (1) excessive ED volume for available ED bedsfull or nearly full department, or full resuscitation rooms without the ability to release a room; or (2) excessive boardingmore than 12 admitted patients awaiting beds in the ED. Other reasons for diversion, such as acute, excessive resource utilization (multiple patients from a single event) and temporary limitation of resources (critical equipment becoming inoperative), are also infrequent causes of diversion that are recorded. The elapsed time during which the ED is on diversion status is recorded and reported as a percentage of the total time on a monthly basis.
Determination of ED Diversion Costs
The cost of diversion at DHMC is calculated by multiplying the average number of ambulance drop‐offs per hour times the number of diversion hours to determine the number of missed patients. The historical mean charges for each ambulance patient are used to determine total missed charge opportunity, which is then applied to the hospital realization rate to calculate missed revenue. In addition, the marginal costs related to Denver Health Medical Plan patients that were unable to be repatriated to DHMC from outlying hospitals, as a result of diversion, is added to the net missed revenue figure. This figure is then divided by the number of diversion hours for the year to determine the cost of each diversion hour. For 2009, the cost of each hour of diversion at DHMC was $5000.
Statistical Analysis
All analyses were performed using SAS Enterprise Guide 4.1 (SAS Institute, Inc, Cary, NC). A Student t test or Wilcoxon rank sum test was used to compare continuous variables, and a chi‐square test was used to compare categorical variables.
Our primary outcome was ED diversion due to hospital bed capacity. These data are recorded, maintained, and analyzed by a DHMC internally developed emergency medical services information system (EMeSIS) that interfaces with computerized laboratory reporting systems, and stores, in part, demographic data as well as real‐time data related to the timing of patient encounters for all patients evaluated in the ED. To assess the effect of the intervention on ED diversion, the proportion of total hours on diversion due to medicine bed capacity was compared preimplementation and postimplementation with a chi‐squared test.
Secondary outcomes for patient flow included: (1) the proportion of patients discharged within 8 hours of transfer to a medical floor; and (2) the proportion of admitted medicine patients discharged from the ED. These data were gathered from the Denver Health Data Warehouse which pools data from both administrative and clinical applications used in patient care. Chi‐squared tests were also used to compare secondary outcomes preintervention and postintervention.
To measure the quality and safety of the HMED team, pre‐ED and post‐ED length of stay (LOS), 48‐hour patient return rate, intensive care unit (ICU) transfer rate, and the total LOS for patients admitted to the HMED team and handed off to a medicine floor team were assessed with the Student t test. To assess timeliness of clinical care provided to boarded medicine patients, self‐reported rounding times were compared preintervention and postintervention with the Student t test.
To assess satisfaction with the HMED team, an anonymous paper survey was administered to ED attendings and nursing supervisors 1 year after the intervention was introduced. The survey consisted of 5 questions, and used a 5‐point Likert scale ranging from strongly disagree (1) to strongly agree (5). Those answering agree or strongly agree were compared to those who were neutral, disagreed, or strongly disagreed.
RESULTS
The ED saw 48,595 patients during the intervention period (August 1, 2009June 30,2010) which did not differ statistically from the 50,469 patients seen in the control period (August 1, 2008June 30, 2009). The number of admissions to the medicine service during the control period (9727) and intervention period (10,013), and the number of total medical/surgical admissions during the control (20,716) and intervention (20,574) periods did not statistically differ. ED staffing during the intervention did not change. The overall number of licensed beds did not increase during the study period. During the control period, staffed medical/surgical beds increased from 395 to 400 beds, while the number of staffed medical/surgical beds decreased from 400 to 397 beds during the intervention period. Patient characteristics were similar during the 2 time periods, with the exception of race (Table 1).
Patients Admitted to Medicine and Transferred to a Medicine Floor | Pre | Post | P Value |
---|---|---|---|
| |||
No. | 1901 | 1828 | |
Age* | 53 15 | 54 14 | 0.59 |
Gender (% male) | 55% | 52% | 0.06 |
Race (% white) | 40% | 34% | <0.0001 |
Insurance (% insured) | 67% | 63% | 0.08 |
Charlson Comorbidity Index | 1.0 [1.0, 1.0] | 1.0 [1.0, 1.0] | 0.52 |
Diversion Hours
After implementation of the HMED team, there was a relative reduction of diversion due to medicine bed capacity of 27% (4.5%3.3%; P < 0.01) (Table 2). During the same time period, the relative proportion of hours on diversion due to ED capacity decreased by 55% (9.9%5.4%).
Pre | Post | P Value | |
---|---|---|---|
| |||
Divert hours due to bed capacity (%, hours)* | 4.5% (3624) | 3.3% (3624) | 0.009 |
Admitted ED patients transferred to floor | |||
Discharged within 8 h (%, N) | 1.3% (1901) | 0.5% (1828) | 0.03 |
Boarded patients rounded on in the ED and transferred to the medical floor | |||
Total length of stay (days, N) | 2.6 [2.4, 3.2] (154) | 2.5 [2.4, 2.6] (364) | 0.21 |
All discharges and transfers to the floor | |||
Discharged from ED [%, (N)] | 4.9% (2009) | 7.5% (1981) | <0.001 |
ED length of stay [hours, (N)] | 12:09 8:44 (2009) | 12:48 10:00 (1981) | 0.46 |
Return to hospital <48 h [%, (N)] | 4.6% (2009) | 4.8% (1981) | 0.75 |
Transfer to the ICU [%, (N)] | 3.3% (2009) | 4.2% (1981) | 0.13 |
Bed Management and Patient Flow
The HMED team rounded on boarded ED patients a mean of 2 hours and 9 minutes earlier (10:59 AM 1:09 vs 8:50 AM 1:20; P < 0.0001). After implementation of the HMED team, patients transferred to a medicine floor and discharged within 8 hours decreased relatively by 67% (1.5%0.5%; P < 0.01), and discharges from the ED of admitted medicine patients increased relatively by 61% (4.9%7.9%; P < 0.001) (Table 2). ED LOS, total LOS, 48‐hour returns to the ED, and ICU transfer rate for patients managed by the HMED team did not change (Table 2).
Perception and Satisfaction
Nine out of 15 (60%) ED attendings and 7 out of 8 (87%) nursing supervisors responded to the survey. The survey demonstrated that ED attendings and nursing supervisors believe the HMED team improves clinical care for boarded patients, communication, collegiality, and patient flow (Table 3).
Postimplementation of the HMED Team | Total (n = 16) | ED Attendings (n = 9) | Nursing Supervisors (n = 7) |
---|---|---|---|
| |||
Quality of care has improved | 94 | 89 | 100 |
Communication has improved | 94 | 89 | 100 |
Collegiality and clinical decision‐making has improved | 94 | 100 | 89 |
Patient flow has improved | 81 | 67 | 100 |
HMED team is an asset to DHMC | 94 | 89 | 100 |
Financial
The 27% relative reduction in ED diversion due to hospital bed capacity extrapolates to 105.1 hours a year of decreased diversion, accounting for $525,600 of increased annual revenues.
DISCUSSION
This study suggests that an HMED team can decrease ED diversion, due to hospital bed capacity, by improving patient flow and timeliness of care for boarded medicine patients in the ED.
After participating in bed management, ED diversion due to a lack of medicine beds decreased. This is consistent with findings by Howell and colleagues who were able to improve throughput and decrease ED diversion with active bed management.13 Howell and colleagues decreased diversion hours due to temporary ED overload, and diversion hours due to a lack of telemetry or critical care beds. At DHMC, diversion is attributed to either a lack of ED capacity or lack of hospital beds. The primary outcome was the diversion rate due to lack of hospital beds, but it is possible that increased discharges directly from the ED contributed to the decrease in diversion due to ED capacity, underestimating the effect our intervention had on total ED diversion. There were no other initiatives to decrease diversion due to ED capacity during the study periods, and ED capacity and volume did not change during the intervention period.
While there were no statistically significant changes in staffed medical/surgical beds or medicine admissions, staffed medical/surgical beds during the intervention period decreased while there were more admissions to medicine. Both of these variables would increase diversion, resulting in an underestimation of the effect of the intervention.
Howell and colleagues improved throughput in the ED by implementing a service which provided active bed management without clinical responsibilities,13 while Briones and colleagues improved clinical care of patients boarded in the ED without affecting throughput.14 The HMED team improved throughput and decreased ED diversion while improving timeliness of care and perception of care quality for patients boarding in the ED.
By decreasing unnecessary transfers to medicine units and increasing discharges from the ED, patient flow was improved. While there was no difference in ED LOS, there was a trend towards decreased total LOS. A larger sample size or a longer period of observation would be necessary to determine if the trend toward decreased total LOS is statistically significant. ED LOS may not have been decreased because patients who would have been sent to the floor only to be discharged within 8 hours were kept in the ED to expedite testing and discharge, while sicker patients were sent to the medical floor. This decreased the turnover time of inpatient beds and allowed more boarded patients to be moved to floor units.
There was concern that an HMED team would fragment care, which would lead to an increased LOS for those patients who were transferred to a medical floor and cared for by an additional medicine team before discharge.17 As noted, there was a trend towards a decreased LOS for patients initially cared for by the HMED team.
In this intervention, hospital medicine physicians provided information regarding ongoing care of patients boarded in the ED to nursing supervisors. Prior to the intervention, nursing supervisors relied upon information from the ED staff and the boarded patient's time in the ED to assign a medical floor. However, ED staff was not providing care to boarded patients and did not know the most up‐to‐date status of the patient. This queuing process and lack of communication resulted in patients ready for discharge being transferred to floor beds and discharged within a few hours of transfer. The HMED team allowed nursing supervisors to have direct knowledge regarding clinical status, including telemetry and ICU criteria (similar to Howell and colleagues13), and readiness for discharge from the physician taking care of the patient.
By managing boarded patients, an HMED team can improve timeliness and coordination of care. Prior to the intervention, boarded ED patients were the last to be seen on rounds. The HMED team rounds only in the ED, expediting care and discharges. The increased proportion of boarded patients discharged from the ED by the HMED team is consistent with Briones and colleagues' clinically oriented team managing boarding patients in the ED.14
Potential adverse effects of our intervention included increased returns to the ED, increased ICU transfer rate, and decreased housestaff satisfaction. There was no increase in the 48‐hour return rate and no increase in the ICU transfer rate for patients cared for by the HMED team. Housestaff at DHMC are satisfied with the HMED team, since the presence of the HMED team allows them to concentrate on patients on the medical floors.
This intervention provides DHMC with an additional $525,600 in revenue annually. Since existing FTE were reallocated to create the HMED team, no additional FTE were required. In our facility, AHPs take on duties of housestaff. However, only 1 physician may be needed to staff an HMED team. This physician's clinical productivity is about 75% of other physicians; therefore, 25% of time is spent in bed management. At DHMC, other medicine teams picked up for the decreased clinical productivity of the HMED team, so the budget was neutral. However, using 2 FTE to staff 1 physician daily for 365 days a year, one would need to allocate 0.5 physician FTE (0.25 decrease in clinical productivity 2 FTE) for an HMED team.
Our study has several limitations. As a single center study, our findings may not extrapolate to other settings. The study used historical controls, therefore, undetected confounders may exist. We could not control for simultaneous changes in the hospital, however, we did not know of any other concurrent interventions aimed at decreasing ED diversion. Also, the decision to admit or not is partially based on individual ED attendings, which causes variability in practice. Finally, while we were able to measure rounding times as a process measure to reflect timeliness of care and staff perceptions of quality of care, due to our data infrastructure and the way our housestaff and attendings rotate, we were not able to assess more downstream measures of quality of care.
CONCLUSION
ED crowding decreases throughput and worsens clinical care; there are few proven solutions. This study demonstrates an intervention that reduced the percentage of patients transferred to a medicine floor and discharged within 8 hours, increased the number of discharges from the ED of admitted medicine patients, and decreased ED diversion while improving the timeliness of clinical care for patients boarded in the ED.
Acknowledgements
Disclosure: Nothing to report.
- Access block causes emergency department overcrowding and ambulance diversion in Perth, Western Australia.Emerg Med J.2005;22:351–354. , , .
- The relationship between distance to hospital and patient mortality in emergencies: an observational study.Emerg Med J.2007;24:665–668. , , , .
- Institute of Medicine.Committee on the Future of Emergency Care in the United States Health System.Hospital‐Based Emergency Care: At the Breaking Point.Washington, DC:National Academies Press;2007.
- Systematic review of emergency department crowding: causes, effects, and solutions.Ann Emerg Med.2008;52:126–136. , .
- Emergency department crowding is associated with poor care for patients with severe pain.Ann Emerg Med.2008;51:1–5. , .
- The impact of emergency department crowding measures on time to antibiotics for patients with community‐acquired pneumonia.Ann Emerg Med.2007;50:510–516. , , , et al.
- for the DELAYED‐ED Study Group.Impact of delayed transfer of critically ill patients from the emergency department to the intensive care unit.Crit Care Med.2007;35:1477–1483. , , , et al;
- Impact of a triage liaison physician on emergency department overcrowding and throughput: a randomized controlled trial.Acad Emerg Med.2007;14:702–708. , , , et al.
- Strategies for dealing with emergency department overcrowding: a one‐year study on how bedside registration affects patient throughput times.Emerg Med J.2007;32:337–342. , , .
- The effect of emergency department expansion on emergency department overcrowding.Acad Emerg Med.2007;14:338–343. , , , et al.
- Effect of increased ICU capacity on emergency department length of stay and ambulance diversion.Ann Emerg Med.2005;5:471–478. , , , , , .
- Ambulance diversion reduction: the Sacramento solution.Am J Emerg Med.2006;357:608–613. , , , , .
- Active bed management by hospitalists and emergency department throughput.Ann Intern Med.2008;149:804–810. , , , , , .
- A model of hospitalist role in the care of admitted patients in the emergency department.J Hosp Med.2010;5:360–364. , , , et al.
- Ambulance diversion and lost hospital revenues.Ann Emerg Med.2006;48(6):702–710. , , , , .
- The financial impact of ambulance diversion and patient elopements.Acad Emerg Med.2007;14(1):58–62. , , , .
- The impact of fragmentation of hospitalist care on length of stay.J. Hosp. Med.2010;5:335–338. , , , , .
- Access block causes emergency department overcrowding and ambulance diversion in Perth, Western Australia.Emerg Med J.2005;22:351–354. , , .
- The relationship between distance to hospital and patient mortality in emergencies: an observational study.Emerg Med J.2007;24:665–668. , , , .
- Institute of Medicine.Committee on the Future of Emergency Care in the United States Health System.Hospital‐Based Emergency Care: At the Breaking Point.Washington, DC:National Academies Press;2007.
- Systematic review of emergency department crowding: causes, effects, and solutions.Ann Emerg Med.2008;52:126–136. , .
- Emergency department crowding is associated with poor care for patients with severe pain.Ann Emerg Med.2008;51:1–5. , .
- The impact of emergency department crowding measures on time to antibiotics for patients with community‐acquired pneumonia.Ann Emerg Med.2007;50:510–516. , , , et al.
- for the DELAYED‐ED Study Group.Impact of delayed transfer of critically ill patients from the emergency department to the intensive care unit.Crit Care Med.2007;35:1477–1483. , , , et al;
- Impact of a triage liaison physician on emergency department overcrowding and throughput: a randomized controlled trial.Acad Emerg Med.2007;14:702–708. , , , et al.
- Strategies for dealing with emergency department overcrowding: a one‐year study on how bedside registration affects patient throughput times.Emerg Med J.2007;32:337–342. , , .
- The effect of emergency department expansion on emergency department overcrowding.Acad Emerg Med.2007;14:338–343. , , , et al.
- Effect of increased ICU capacity on emergency department length of stay and ambulance diversion.Ann Emerg Med.2005;5:471–478. , , , , , .
- Ambulance diversion reduction: the Sacramento solution.Am J Emerg Med.2006;357:608–613. , , , , .
- Active bed management by hospitalists and emergency department throughput.Ann Intern Med.2008;149:804–810. , , , , , .
- A model of hospitalist role in the care of admitted patients in the emergency department.J Hosp Med.2010;5:360–364. , , , et al.
- Ambulance diversion and lost hospital revenues.Ann Emerg Med.2006;48(6):702–710. , , , , .
- The financial impact of ambulance diversion and patient elopements.Acad Emerg Med.2007;14(1):58–62. , , , .
- The impact of fragmentation of hospitalist care on length of stay.J. Hosp. Med.2010;5:335–338. , , , , .
Copyright © 2012 Society of Hospital Medicine