User login
A Method for Attributing Patient-Level Metrics to Rotating Providers in an Inpatient Setting
Hospitalists’ performance is routinely evaluated by third-party payers, employers, and patients. As hospitalist programs mature, there is a need to develop processes to identify, internally measure, and report on individual and group performance. We know from Society of Hospital Medicine (SHM) data that a significant amount of hospitalists’ total compensation is at least partially based on performance. Often this is based at least in part on quality data. In 2006, SHM issued a white paper detailing the key elements of a successful performance monitoring and reporting process.1,2 Recommendations included the identification of meaningful operational and clinical performance metrics, and the ability to monitor and report both group and individual metrics was highlighted as an essential component. There is evidence that comparison of individual provider performance with that of their peers is a necessary element of successful provider dashboards.3 Additionally, regular feedback and a clear, visual presentation of the data are important components of successful provider feedback dashboards.3-6
Much of the literature regarding provider feedback dashboards has been based in the outpatient setting. The majority of these dashboards focus on the management of chronic illnesses (eg, diabetes and hypertension), rates of preventative care services (eg, colonoscopy or mammogram), or avoidance of unnecessary care (eg, antibiotics for sinusitis).4,5 Unlike in the outpatient setting, in which 1 provider often provides a majority of the care for a given episode of care, hospitalized patients are often cared for by multiple providers, challenging the appropriate attribution of patient-level metrics to specific providers. Under the standard approach, an entire hospitalization is attributed to 1 physician, generally the attending of record for the hospitalization, which may be the admitting provider or the discharging provider, depending on the approach used by the hospital. However, assigning responsibility for an entire hospitalization to a provider who may have only seen the patient for a small percentage of a hospitalization may jeopardize the validity of metrics. As provider metrics are increasingly being used for compensation, it is important to ensure that the method for attribution correctly identifies the providers caring for patients. To our knowledge there is no gold standard approach for attributing metrics to providers when patients are cared for by multiple providers, and the standard attending of record–based approach may lack face validity in many cases.
We aimed to develop and operationalize a system to more fairly attribute patient-level data to individual providers across a single hospitalization even when multiple providers cared for the patient. We then compared our methodology to the standard approach, in which the attending of record receives full attribution for each metric, to determine the difference on a provider level between the 2 models.
METHODS
Clinical Setting
The Johns Hopkins Hospital is a 1145-bed, tertiary-care hospital. Over the years of this project, the Johns Hopkins Hospitalist Program was an approximately 20-physician group providing care in a variety of settings, including a dedicated hospitalist floor, where this metrics program was initiated. Hospitalists in this setting work Monday through Friday, with 1 hospitalist and a moonlighter covering on the weekends. Admissions are performed by an admitter, and overnight care is provided by a nocturnist. Initially 17 beds, this unit expanded to 24 beds in June 2012. For the purposes of this article, we included all general medicine patients admitted to this floor between July 1, 2010, and June 30, 2014, who were cared for by hospitalists. During this period, all patients were inpatients; no patients were admitted under observation status. All of these patients were cared for by hospitalists without housestaff or advanced practitioners. Since 2014, the metrics program has been expanded to other hospitalist-run services in the hospital, but for simplicity, we have not presented these more recent data.
Individual Provider Metrics
Metrics were chosen to reflect institutional quality and efficiency priorities. Our choice of metrics was restricted to those that (1) plausibly reflect provider performance, at least in part, and (2) could be accessed in electronic form (without any manual chart review). Whenever possible, we chose metrics with objective data. Additionally, because funding for this effort was provided by the hospital, we sought to ensure that enough of the metrics were related to cost to justify ongoing hospital support of the project. SAS 9.2 (SAS Institute Inc, Cary, NC) was used to calculate metric weights. Specific metrics included American College of Chest Physicians (ACCP)–compliant venous thromboembolism (VTE) prophylaxis,7 observed-to-expected length of stay (LOS) ratio, percentage of discharges per day, discharges before 3
Appropriate prophylaxis for VTE was calculated by using an algorithm embedded within the computerized provider order entry system, which assessed the prescription of ACCP-compliant VTE prophylaxis within 24 hours following admission. This included a risk assessment, and credit was given for no prophylaxis and/or mechanical and/or pharmacologic prophylaxis per the ACCP guidelines.7
Observed-to-expected LOS was defined by using the University HealthSystem Consortium (UHC; now Vizient Inc) expected LOS for the given calendar year. This approach incorporates patient diagnoses, demographics, and other administrative variables to define an expected LOS for each patient.
The percent of patients discharged per day was defined from billing data as the percentage of a provider’s evaluation and management charges that were the final charge of a patient’s stay (regardless of whether a discharge day service was coded).
Discharge prior to 3
Depth of coding was defined as the number of coded diagnoses submitted to the Maryland Health Services Cost Review Commission for determining payment and was viewed as an indicator of the thoroughness of provider documentation.
Patient satisfaction was defined at the patient level (for those patients who turned in patient satisfaction surveys) as the pooled value of the 5 provider questions on the hospital’s patient satisfaction survey administered by Press Ganey: “time the physician spent with you,” “did the physician show concern for your questions/worries,” “did the physician keep you informed,” “friendliness/courtesy of the physician,” and “skill of the physician.”8
Readmission rates were defined as same-hospital readmissions divided by the total number of patients discharged by a given provider, with exclusions based on the Centers for Medicare and Medicaid Services hospital-wide, all-cause readmission measure.1 The expected same-hospital readmission rate was defined for each patient as the observed readmission rate in the entire UHC (Vizient) data set for all patients with the same All Patient Refined Diagnosis Related Group and severity of illness, as we have described previously.9
Communication with the primary care provider was the only self-reported metric used. It was based on a mandatory prompt on the discharge worksheet in the electronic medical record (EMR). Successful communication with the outpatient provider was defined as verbal or electronic communication by the hospitalist with the outpatient provider. Partial (50%) credit was given for providers who attempted but were unsuccessful in communicating with the outpatient provider, for patients for whom the provider had access to the Johns Hopkins EMR system, and for planned admissions without new or important information to convey. No credit was given for providers who indicated that communication was not indicated, who indicated that a patient and/or family would update the provider, or who indicated that the discharge summary would be sufficient.9 Because the discharge worksheet could be initiated at any time during the hospitalization, providers could document communication with the outpatient provider at any point during hospitalization.
Discharge summary turnaround was defined as the average number of days elapsed between the day of discharge and the signing of the discharge summary in the EMR.
Assigning Ownership of Patients to Individual Providers
Using billing data, we assigned ownership of patient care based on the type, timing, and number of charges that occurred during each hospitalization (Figure 1). Eligible charges included all history and physical (codes 99221, 99222, and 99223), subsequent care (codes 99231, 99232, and 99233), and discharge charges (codes 99238 and 99239).
By using a unique identifier assigned for each hospitalization, professional fees submitted by providers were used to identify which provider saw the patient on the admission day, discharge day, as well as subsequent care days. Providers’ productivity, bonus supplements, and policy compliance were determined by using billing data, which encouraged the prompt submittal of charges.
The provider who billed the admission history and physical (codes 99221, 99222, and 99223) within 1 calendar date of the patient’s initial admission was defined as the admitting provider. Patients transferred to the hospitalist service from other services were not assigned an admitting hospitalist. The sole metric assigned to the admitting hospitalist was ACCP-compliant VTE prophylaxis.
The provider who billed the final subsequent care or discharge code (codes 99231, 99232, 99233, 99238, and 99239) within 1 calendar date of discharge was defined as the discharging provider. For hospitalizations characterized by a single provider charge (eg, for patients admitted and discharged on the same day), the provider billing this charge was assigned as both the admitting and discharging physician. Patients upgraded to the intensive care unit (ICU) were not counted as a discharge unless the patient was downgraded and discharged from the hospitalist service. The discharging provider was assigned responsibility for the time of discharge, the percent of patients discharged per day, the discharge summary turnaround time, and hospital readmissions.
Metrics that were assigned to multiple providers for a single hospitalization were termed “provider day–weighted” metrics. The formula for calculating the weight for each provider day–weighted metric was as follows: weight for provider A = [number of daily charges billed by provider A] divided by [LOS +1]. The initial hospital day was counted as day 0. LOS plus 1 was used to recognize that a typical hospitalization will have a charge on the day of admission (day 0) and a charge on the day of discharge such that an LOS of 2 days (eg, a patient admitted on Monday and discharged on Wednesday) will have 3 daily charges. Provider day–weighted metrics included patient satisfaction, communication with the outpatient provider, depth of coding, and observed-to-expected LOS.
Our billing software prevented providers from the same group from billing multiple daily charges, thus ensuring that there were no duplicated charges submitted for a given day.
Presenting Results
Providers were only shown data from the day-weighted approach. For ease of visual interpretation, scores for each metric were scaled ordinally from 1 (worst performance) to 9 (best performance; Table 1). Data were displayed in a dashboard format on a password-protected website for each provider to view his or her own data relative to that of the hospitalist peer group. The dashboard was implemented in this format on July 1, 2011. Data were updated quarterly (Figure 2).
Results were displayed in a polyhedral or spider-web graph (Figure 2). Provider and group metrics were scaled according to predefined benchmarks established for each metric and standardized to a scale ranging from 1 to 9. The scale for each metric was set based on examining historical data and group median performance on the metrics to ensure that there was a range of performance (ie, to avoid having most hospitalists scoring a 1 or 9). Scaling thresholds were periodically adjusted as appropriate to maintain good visual discrimination. Higher scores (creating a larger-volume polygon) are desirable even for metrics such as LOS, for which a low value is desirable. Both a spider-web graph and trends over time were available to the provider (Figure 2). These graphs display a comparison of the individual provider scores for each metric to the hospitalist group average for that metric.
Comparison with the Standard (Attending of Record) Method of Attribution
For the purposes of this report, we sought to determine whether there were meaningful differences between our day-weighted approach versus the standard method of attribution, in which the attending of record is assigned responsibility for each metric that would not have been attributed to the discharging attending under both methods. Our goal was to determine where and whether there was a meaningful difference between the 2 methodologies, recognizing that the degree of difference between these 2 methodologies might vary in other institutions and settings. In our hospital, the attending of record is generally the discharging attending. In order to compare the 2 methodologies, we arbitrarily picked 2015 to retrospectively evaluate the differences between these 2 methods of attribution. We did not display or provide data using the standard methodology to providers at any point; this approach was used only for the purposes of this report. Because these metrics are intended to evaluate relative provider performance, we assigned a percentile to each provider for his or her performance on the given metric using our attribution methodology and then, similarly, assigned a percentile to each provider using the standard methodology. This yielded 2 percentile scores for each provider and each metric. We then compared these percentile ranks for providers in 2 ways: (1) we determined how often providers who scored in the top half of the group for a given metric (above the 50th percentile) also scored in the top half of the group for that metric by using the other calculation method, and (2) we calculated the absolute value of the difference in percentiles between the 2 methods to characterize the impact on a provider’s ranking for that metric that might result from switching to the other method. For instance, if a provider scored at the 20th percentile for the group in patient satisfaction with 1 attribution method and scored at the 40th percentile for the group in patient satisfaction using the other method, the absolute change in percentile would be 20 percentile points. But, this provider would still be below the 50th percentile by both methods (concordant bottom half performance). We did not perform this comparison for metrics assigned to the discharging provider (such as discharge summary turnaround time or readmissions) because the attending of record designation is assigned to the discharging provider at our hospital.
RESULTS
The dashboard was successfully operationalized on July 1, 2011, with displays visible to providers as shown in Figure 2. Consistent with the principles of providing effective performance feedback to providers, the display simultaneously showed providers their individual performance as well as the performance of their peers. Providers were able to view their spider-web plot for prior quarters. Not shown are additional views that allowed providers to see quarterly trends in their data versus their peers across several fiscal years. Also available to providers was their ranking relative to their peers for each metric; specific peers were deidentified in the display.
There was notable discordance between provider rankings between the 2 methodologies, as shown in Table 2. Provider performance above or below the median was concordant 56% to 75% of the time (depending on the particular metric), indicating substantial discordance because top-half or bottom-half concordance would be expected to occur by chance 50% of the time. Although the provider percentile differences between the 2 methods tended to be modest for most providers (the median difference between the methods was 13 to 22 percentile points for the various metrics), there were some providers for whom the method of calculation dramatically impacted their rankings. For 5 of the 6 metrics we examined, at least 1 provider had a 50-percentile or greater change in his or her ranking based on the method used. This indicates that at least some providers would have had markedly different scores relative to their peers had we used the alternative methodology (Table 2). In VTE prophylaxis, for example, at least 1 provider had a 94-percentile change in his or her ranking; similarly, a provider had an 88-perentile change in his or her LOS ranking between the 2 methodologies.
DISCUSSION
We found that it is possible to assign metrics across 1 hospital stay to multiple providers by using billing data. We also found a meaningful discrepancy in how well providers scored (relative to their peers) based on the method used for attribution. These results imply that hospitals should consider attributing performance metrics based on ascribed ownership from billing data and not just from attending of record status.
As hospitalist programs and providers in general are increasingly being asked to develop dashboards to monitor individual and group performance, correctly attributing care to providers is likely to become increasingly important. Experts agree that principles of effective provider performance dashboards include ranking individual provider performance relative to peers, clearly displaying data in an easily accessible format, and ensuring that data can be credibly attributed to the individual provider.3,4,6 However, there appears to be no gold standard method for attribution, especially in the inpatient setting. Our results imply that hospitals should consider attributing performance metrics based on ascribed ownership from billing data and not just from attending of record status.
Several limitations of our findings are important to consider. First, our program is a relatively small, academic group with handoffs that typically occur every 1 to 2 weeks and sometimes with additional handoffs on weekends. Different care patterns and settings might impact the utility of our attribution methodology relative to the standard methodology. Additionally, it is important to note that the relative merits of the different methodologies cannot be ascertained from our comparison. We can demonstrate discordance between the attribution methodologies, but we cannot say that 1 method is correct and the other is flawed. Although we believe that our day-weighted approach feels fairer to providers based on group input and feedback, we did not conduct a formal survey to examine providers’ preferences for the standard versus day-weighted approaches. The appropriateness of a particular attribution method needs to be assessed locally and may vary based on the clinical setting. For instance, on a service in which patients are admitted for procedures, it may make more sense to attribute the outcome of the case to the proceduralist even if that provider did not bill for the patient’s care on a daily basis. Finally, the computational requirements of our methodology are not trivial and require linking billing data with administrative patient-level data, which may be challenging to operationalize in some institutions.
These limitations aside, we believe that our attribution methodology has face validity. For example, a provider might be justifiably frustrated if, using the standard methodology, he or she is charged with the LOS of a patient who had been hospitalized for months, particularly if that patient is discharged shortly after the provider assumes care. Our method addresses this type of misattribution. Particularly when individual provider compensation is based on performance on metrics (as is the case at our institution), optimizing provider attribution to particular patients may be important, and face validity may be required for group buy-in.
In summary, we have demonstrated that it is possible to use billing data to assign ownership of patients to multiple providers over 1 hospital stay. This could be applied to other hospitalist programs as well as other healthcare settings in which multiple providers care for patients during 1 healthcare encounter (eg, ICUs).
Disclosure
The authors declare they have no relevant conflicts of interest.
1. Horwitz L, Partovian C, Lin Z, et al. Hospital-Wide (All-Condition) 30‐Day Risk-Standardized Readmission Measure. https://www.cms.gov/Medicare/Quality-Initiatives-Patient-Assessment-Instruments/MMS/downloads/MMSHospital-WideAll-ConditionReadmissionRate.pdf. Accessed March 6, 2015.
2. Medicine SoH. Measuring Hospitalist Performance: Metrics, Reports, and Dashboards. 2007; https://www.hospitalmedicine.org/Web/Practice_Management/Products_and_Programs/measure_hosp_perf_metrics_reports_dashboards.aspx. Accessed May 12, 2013.
3. Teleki SS, Shaw R, Damberg CL, McGlynn EA. Providing performance feedback to individual physicians: current practice and emerging lessons. Santa Monica, CA: RAND Corporation; 2006. 1-47. https://www.rand.org/content/dam/rand/pubs/working_papers/2006/RAND_WR381.pdf. Accessed August, 2017.
4. Brehaut JC, Colquhoun HL, Eva KW, et al. Practice Feedback Interventions: 15 Suggestions for Optimizing Effectiveness Practice Feedback Interventions. Ann Intern Med. 2016;164(6):435-441. PubMed
5. Dowding D, Randell R, Gardner P, et al. Dashboards for improving patient care: review of the literature. Int J Med Inform. 2015;84(2):87-100. PubMed
6. Landon BE, Normand S-LT, Blumenthal D, Daley J. Physician clinical performance assessment: prospects and barriers. JAMA. 2003;290(9):1183-1189. PubMed
7. Guyatt GH, Akl EA, Crowther M, Gutterman DD, Schuünemann HJ. Executive summary: Antit hrombotic therapy and prevention of thrombosis, 9th ed: American College of Chest Physicians evidence-based clinical practice guidelines. Ann Intern Med. 2012;141(2 suppl):7S-47S. PubMed
8. Siddiqui Z, Qayyum R, Bertram A, et al. Does Provider Self-reporting of Etiquette Behaviors Improve Patient Experience? A Randomized Controlled Trial. J Hosp Med. 2017;12(6):402-406. PubMed
9. Oduyebo I, Lehmann CU, Pollack CE, et al. Association of self-reported hospital discharge handoffs with 30-day readmissions. JAMA Intern Med. 2013;173(8):624-629. PubMed
Hospitalists’ performance is routinely evaluated by third-party payers, employers, and patients. As hospitalist programs mature, there is a need to develop processes to identify, internally measure, and report on individual and group performance. We know from Society of Hospital Medicine (SHM) data that a significant amount of hospitalists’ total compensation is at least partially based on performance. Often this is based at least in part on quality data. In 2006, SHM issued a white paper detailing the key elements of a successful performance monitoring and reporting process.1,2 Recommendations included the identification of meaningful operational and clinical performance metrics, and the ability to monitor and report both group and individual metrics was highlighted as an essential component. There is evidence that comparison of individual provider performance with that of their peers is a necessary element of successful provider dashboards.3 Additionally, regular feedback and a clear, visual presentation of the data are important components of successful provider feedback dashboards.3-6
Much of the literature regarding provider feedback dashboards has been based in the outpatient setting. The majority of these dashboards focus on the management of chronic illnesses (eg, diabetes and hypertension), rates of preventative care services (eg, colonoscopy or mammogram), or avoidance of unnecessary care (eg, antibiotics for sinusitis).4,5 Unlike in the outpatient setting, in which 1 provider often provides a majority of the care for a given episode of care, hospitalized patients are often cared for by multiple providers, challenging the appropriate attribution of patient-level metrics to specific providers. Under the standard approach, an entire hospitalization is attributed to 1 physician, generally the attending of record for the hospitalization, which may be the admitting provider or the discharging provider, depending on the approach used by the hospital. However, assigning responsibility for an entire hospitalization to a provider who may have only seen the patient for a small percentage of a hospitalization may jeopardize the validity of metrics. As provider metrics are increasingly being used for compensation, it is important to ensure that the method for attribution correctly identifies the providers caring for patients. To our knowledge there is no gold standard approach for attributing metrics to providers when patients are cared for by multiple providers, and the standard attending of record–based approach may lack face validity in many cases.
We aimed to develop and operationalize a system to more fairly attribute patient-level data to individual providers across a single hospitalization even when multiple providers cared for the patient. We then compared our methodology to the standard approach, in which the attending of record receives full attribution for each metric, to determine the difference on a provider level between the 2 models.
METHODS
Clinical Setting
The Johns Hopkins Hospital is a 1145-bed, tertiary-care hospital. Over the years of this project, the Johns Hopkins Hospitalist Program was an approximately 20-physician group providing care in a variety of settings, including a dedicated hospitalist floor, where this metrics program was initiated. Hospitalists in this setting work Monday through Friday, with 1 hospitalist and a moonlighter covering on the weekends. Admissions are performed by an admitter, and overnight care is provided by a nocturnist. Initially 17 beds, this unit expanded to 24 beds in June 2012. For the purposes of this article, we included all general medicine patients admitted to this floor between July 1, 2010, and June 30, 2014, who were cared for by hospitalists. During this period, all patients were inpatients; no patients were admitted under observation status. All of these patients were cared for by hospitalists without housestaff or advanced practitioners. Since 2014, the metrics program has been expanded to other hospitalist-run services in the hospital, but for simplicity, we have not presented these more recent data.
Individual Provider Metrics
Metrics were chosen to reflect institutional quality and efficiency priorities. Our choice of metrics was restricted to those that (1) plausibly reflect provider performance, at least in part, and (2) could be accessed in electronic form (without any manual chart review). Whenever possible, we chose metrics with objective data. Additionally, because funding for this effort was provided by the hospital, we sought to ensure that enough of the metrics were related to cost to justify ongoing hospital support of the project. SAS 9.2 (SAS Institute Inc, Cary, NC) was used to calculate metric weights. Specific metrics included American College of Chest Physicians (ACCP)–compliant venous thromboembolism (VTE) prophylaxis,7 observed-to-expected length of stay (LOS) ratio, percentage of discharges per day, discharges before 3
Appropriate prophylaxis for VTE was calculated by using an algorithm embedded within the computerized provider order entry system, which assessed the prescription of ACCP-compliant VTE prophylaxis within 24 hours following admission. This included a risk assessment, and credit was given for no prophylaxis and/or mechanical and/or pharmacologic prophylaxis per the ACCP guidelines.7
Observed-to-expected LOS was defined by using the University HealthSystem Consortium (UHC; now Vizient Inc) expected LOS for the given calendar year. This approach incorporates patient diagnoses, demographics, and other administrative variables to define an expected LOS for each patient.
The percent of patients discharged per day was defined from billing data as the percentage of a provider’s evaluation and management charges that were the final charge of a patient’s stay (regardless of whether a discharge day service was coded).
Discharge prior to 3
Depth of coding was defined as the number of coded diagnoses submitted to the Maryland Health Services Cost Review Commission for determining payment and was viewed as an indicator of the thoroughness of provider documentation.
Patient satisfaction was defined at the patient level (for those patients who turned in patient satisfaction surveys) as the pooled value of the 5 provider questions on the hospital’s patient satisfaction survey administered by Press Ganey: “time the physician spent with you,” “did the physician show concern for your questions/worries,” “did the physician keep you informed,” “friendliness/courtesy of the physician,” and “skill of the physician.”8
Readmission rates were defined as same-hospital readmissions divided by the total number of patients discharged by a given provider, with exclusions based on the Centers for Medicare and Medicaid Services hospital-wide, all-cause readmission measure.1 The expected same-hospital readmission rate was defined for each patient as the observed readmission rate in the entire UHC (Vizient) data set for all patients with the same All Patient Refined Diagnosis Related Group and severity of illness, as we have described previously.9
Communication with the primary care provider was the only self-reported metric used. It was based on a mandatory prompt on the discharge worksheet in the electronic medical record (EMR). Successful communication with the outpatient provider was defined as verbal or electronic communication by the hospitalist with the outpatient provider. Partial (50%) credit was given for providers who attempted but were unsuccessful in communicating with the outpatient provider, for patients for whom the provider had access to the Johns Hopkins EMR system, and for planned admissions without new or important information to convey. No credit was given for providers who indicated that communication was not indicated, who indicated that a patient and/or family would update the provider, or who indicated that the discharge summary would be sufficient.9 Because the discharge worksheet could be initiated at any time during the hospitalization, providers could document communication with the outpatient provider at any point during hospitalization.
Discharge summary turnaround was defined as the average number of days elapsed between the day of discharge and the signing of the discharge summary in the EMR.
Assigning Ownership of Patients to Individual Providers
Using billing data, we assigned ownership of patient care based on the type, timing, and number of charges that occurred during each hospitalization (Figure 1). Eligible charges included all history and physical (codes 99221, 99222, and 99223), subsequent care (codes 99231, 99232, and 99233), and discharge charges (codes 99238 and 99239).
By using a unique identifier assigned for each hospitalization, professional fees submitted by providers were used to identify which provider saw the patient on the admission day, discharge day, as well as subsequent care days. Providers’ productivity, bonus supplements, and policy compliance were determined by using billing data, which encouraged the prompt submittal of charges.
The provider who billed the admission history and physical (codes 99221, 99222, and 99223) within 1 calendar date of the patient’s initial admission was defined as the admitting provider. Patients transferred to the hospitalist service from other services were not assigned an admitting hospitalist. The sole metric assigned to the admitting hospitalist was ACCP-compliant VTE prophylaxis.
The provider who billed the final subsequent care or discharge code (codes 99231, 99232, 99233, 99238, and 99239) within 1 calendar date of discharge was defined as the discharging provider. For hospitalizations characterized by a single provider charge (eg, for patients admitted and discharged on the same day), the provider billing this charge was assigned as both the admitting and discharging physician. Patients upgraded to the intensive care unit (ICU) were not counted as a discharge unless the patient was downgraded and discharged from the hospitalist service. The discharging provider was assigned responsibility for the time of discharge, the percent of patients discharged per day, the discharge summary turnaround time, and hospital readmissions.
Metrics that were assigned to multiple providers for a single hospitalization were termed “provider day–weighted” metrics. The formula for calculating the weight for each provider day–weighted metric was as follows: weight for provider A = [number of daily charges billed by provider A] divided by [LOS +1]. The initial hospital day was counted as day 0. LOS plus 1 was used to recognize that a typical hospitalization will have a charge on the day of admission (day 0) and a charge on the day of discharge such that an LOS of 2 days (eg, a patient admitted on Monday and discharged on Wednesday) will have 3 daily charges. Provider day–weighted metrics included patient satisfaction, communication with the outpatient provider, depth of coding, and observed-to-expected LOS.
Our billing software prevented providers from the same group from billing multiple daily charges, thus ensuring that there were no duplicated charges submitted for a given day.
Presenting Results
Providers were only shown data from the day-weighted approach. For ease of visual interpretation, scores for each metric were scaled ordinally from 1 (worst performance) to 9 (best performance; Table 1). Data were displayed in a dashboard format on a password-protected website for each provider to view his or her own data relative to that of the hospitalist peer group. The dashboard was implemented in this format on July 1, 2011. Data were updated quarterly (Figure 2).
Results were displayed in a polyhedral or spider-web graph (Figure 2). Provider and group metrics were scaled according to predefined benchmarks established for each metric and standardized to a scale ranging from 1 to 9. The scale for each metric was set based on examining historical data and group median performance on the metrics to ensure that there was a range of performance (ie, to avoid having most hospitalists scoring a 1 or 9). Scaling thresholds were periodically adjusted as appropriate to maintain good visual discrimination. Higher scores (creating a larger-volume polygon) are desirable even for metrics such as LOS, for which a low value is desirable. Both a spider-web graph and trends over time were available to the provider (Figure 2). These graphs display a comparison of the individual provider scores for each metric to the hospitalist group average for that metric.
Comparison with the Standard (Attending of Record) Method of Attribution
For the purposes of this report, we sought to determine whether there were meaningful differences between our day-weighted approach versus the standard method of attribution, in which the attending of record is assigned responsibility for each metric that would not have been attributed to the discharging attending under both methods. Our goal was to determine where and whether there was a meaningful difference between the 2 methodologies, recognizing that the degree of difference between these 2 methodologies might vary in other institutions and settings. In our hospital, the attending of record is generally the discharging attending. In order to compare the 2 methodologies, we arbitrarily picked 2015 to retrospectively evaluate the differences between these 2 methods of attribution. We did not display or provide data using the standard methodology to providers at any point; this approach was used only for the purposes of this report. Because these metrics are intended to evaluate relative provider performance, we assigned a percentile to each provider for his or her performance on the given metric using our attribution methodology and then, similarly, assigned a percentile to each provider using the standard methodology. This yielded 2 percentile scores for each provider and each metric. We then compared these percentile ranks for providers in 2 ways: (1) we determined how often providers who scored in the top half of the group for a given metric (above the 50th percentile) also scored in the top half of the group for that metric by using the other calculation method, and (2) we calculated the absolute value of the difference in percentiles between the 2 methods to characterize the impact on a provider’s ranking for that metric that might result from switching to the other method. For instance, if a provider scored at the 20th percentile for the group in patient satisfaction with 1 attribution method and scored at the 40th percentile for the group in patient satisfaction using the other method, the absolute change in percentile would be 20 percentile points. But, this provider would still be below the 50th percentile by both methods (concordant bottom half performance). We did not perform this comparison for metrics assigned to the discharging provider (such as discharge summary turnaround time or readmissions) because the attending of record designation is assigned to the discharging provider at our hospital.
RESULTS
The dashboard was successfully operationalized on July 1, 2011, with displays visible to providers as shown in Figure 2. Consistent with the principles of providing effective performance feedback to providers, the display simultaneously showed providers their individual performance as well as the performance of their peers. Providers were able to view their spider-web plot for prior quarters. Not shown are additional views that allowed providers to see quarterly trends in their data versus their peers across several fiscal years. Also available to providers was their ranking relative to their peers for each metric; specific peers were deidentified in the display.
There was notable discordance between provider rankings between the 2 methodologies, as shown in Table 2. Provider performance above or below the median was concordant 56% to 75% of the time (depending on the particular metric), indicating substantial discordance because top-half or bottom-half concordance would be expected to occur by chance 50% of the time. Although the provider percentile differences between the 2 methods tended to be modest for most providers (the median difference between the methods was 13 to 22 percentile points for the various metrics), there were some providers for whom the method of calculation dramatically impacted their rankings. For 5 of the 6 metrics we examined, at least 1 provider had a 50-percentile or greater change in his or her ranking based on the method used. This indicates that at least some providers would have had markedly different scores relative to their peers had we used the alternative methodology (Table 2). In VTE prophylaxis, for example, at least 1 provider had a 94-percentile change in his or her ranking; similarly, a provider had an 88-perentile change in his or her LOS ranking between the 2 methodologies.
DISCUSSION
We found that it is possible to assign metrics across 1 hospital stay to multiple providers by using billing data. We also found a meaningful discrepancy in how well providers scored (relative to their peers) based on the method used for attribution. These results imply that hospitals should consider attributing performance metrics based on ascribed ownership from billing data and not just from attending of record status.
As hospitalist programs and providers in general are increasingly being asked to develop dashboards to monitor individual and group performance, correctly attributing care to providers is likely to become increasingly important. Experts agree that principles of effective provider performance dashboards include ranking individual provider performance relative to peers, clearly displaying data in an easily accessible format, and ensuring that data can be credibly attributed to the individual provider.3,4,6 However, there appears to be no gold standard method for attribution, especially in the inpatient setting. Our results imply that hospitals should consider attributing performance metrics based on ascribed ownership from billing data and not just from attending of record status.
Several limitations of our findings are important to consider. First, our program is a relatively small, academic group with handoffs that typically occur every 1 to 2 weeks and sometimes with additional handoffs on weekends. Different care patterns and settings might impact the utility of our attribution methodology relative to the standard methodology. Additionally, it is important to note that the relative merits of the different methodologies cannot be ascertained from our comparison. We can demonstrate discordance between the attribution methodologies, but we cannot say that 1 method is correct and the other is flawed. Although we believe that our day-weighted approach feels fairer to providers based on group input and feedback, we did not conduct a formal survey to examine providers’ preferences for the standard versus day-weighted approaches. The appropriateness of a particular attribution method needs to be assessed locally and may vary based on the clinical setting. For instance, on a service in which patients are admitted for procedures, it may make more sense to attribute the outcome of the case to the proceduralist even if that provider did not bill for the patient’s care on a daily basis. Finally, the computational requirements of our methodology are not trivial and require linking billing data with administrative patient-level data, which may be challenging to operationalize in some institutions.
These limitations aside, we believe that our attribution methodology has face validity. For example, a provider might be justifiably frustrated if, using the standard methodology, he or she is charged with the LOS of a patient who had been hospitalized for months, particularly if that patient is discharged shortly after the provider assumes care. Our method addresses this type of misattribution. Particularly when individual provider compensation is based on performance on metrics (as is the case at our institution), optimizing provider attribution to particular patients may be important, and face validity may be required for group buy-in.
In summary, we have demonstrated that it is possible to use billing data to assign ownership of patients to multiple providers over 1 hospital stay. This could be applied to other hospitalist programs as well as other healthcare settings in which multiple providers care for patients during 1 healthcare encounter (eg, ICUs).
Disclosure
The authors declare they have no relevant conflicts of interest.
Hospitalists’ performance is routinely evaluated by third-party payers, employers, and patients. As hospitalist programs mature, there is a need to develop processes to identify, internally measure, and report on individual and group performance. We know from Society of Hospital Medicine (SHM) data that a significant amount of hospitalists’ total compensation is at least partially based on performance. Often this is based at least in part on quality data. In 2006, SHM issued a white paper detailing the key elements of a successful performance monitoring and reporting process.1,2 Recommendations included the identification of meaningful operational and clinical performance metrics, and the ability to monitor and report both group and individual metrics was highlighted as an essential component. There is evidence that comparison of individual provider performance with that of their peers is a necessary element of successful provider dashboards.3 Additionally, regular feedback and a clear, visual presentation of the data are important components of successful provider feedback dashboards.3-6
Much of the literature regarding provider feedback dashboards has been based in the outpatient setting. The majority of these dashboards focus on the management of chronic illnesses (eg, diabetes and hypertension), rates of preventative care services (eg, colonoscopy or mammogram), or avoidance of unnecessary care (eg, antibiotics for sinusitis).4,5 Unlike in the outpatient setting, in which 1 provider often provides a majority of the care for a given episode of care, hospitalized patients are often cared for by multiple providers, challenging the appropriate attribution of patient-level metrics to specific providers. Under the standard approach, an entire hospitalization is attributed to 1 physician, generally the attending of record for the hospitalization, which may be the admitting provider or the discharging provider, depending on the approach used by the hospital. However, assigning responsibility for an entire hospitalization to a provider who may have only seen the patient for a small percentage of a hospitalization may jeopardize the validity of metrics. As provider metrics are increasingly being used for compensation, it is important to ensure that the method for attribution correctly identifies the providers caring for patients. To our knowledge there is no gold standard approach for attributing metrics to providers when patients are cared for by multiple providers, and the standard attending of record–based approach may lack face validity in many cases.
We aimed to develop and operationalize a system to more fairly attribute patient-level data to individual providers across a single hospitalization even when multiple providers cared for the patient. We then compared our methodology to the standard approach, in which the attending of record receives full attribution for each metric, to determine the difference on a provider level between the 2 models.
METHODS
Clinical Setting
The Johns Hopkins Hospital is a 1145-bed, tertiary-care hospital. Over the years of this project, the Johns Hopkins Hospitalist Program was an approximately 20-physician group providing care in a variety of settings, including a dedicated hospitalist floor, where this metrics program was initiated. Hospitalists in this setting work Monday through Friday, with 1 hospitalist and a moonlighter covering on the weekends. Admissions are performed by an admitter, and overnight care is provided by a nocturnist. Initially 17 beds, this unit expanded to 24 beds in June 2012. For the purposes of this article, we included all general medicine patients admitted to this floor between July 1, 2010, and June 30, 2014, who were cared for by hospitalists. During this period, all patients were inpatients; no patients were admitted under observation status. All of these patients were cared for by hospitalists without housestaff or advanced practitioners. Since 2014, the metrics program has been expanded to other hospitalist-run services in the hospital, but for simplicity, we have not presented these more recent data.
Individual Provider Metrics
Metrics were chosen to reflect institutional quality and efficiency priorities. Our choice of metrics was restricted to those that (1) plausibly reflect provider performance, at least in part, and (2) could be accessed in electronic form (without any manual chart review). Whenever possible, we chose metrics with objective data. Additionally, because funding for this effort was provided by the hospital, we sought to ensure that enough of the metrics were related to cost to justify ongoing hospital support of the project. SAS 9.2 (SAS Institute Inc, Cary, NC) was used to calculate metric weights. Specific metrics included American College of Chest Physicians (ACCP)–compliant venous thromboembolism (VTE) prophylaxis,7 observed-to-expected length of stay (LOS) ratio, percentage of discharges per day, discharges before 3
Appropriate prophylaxis for VTE was calculated by using an algorithm embedded within the computerized provider order entry system, which assessed the prescription of ACCP-compliant VTE prophylaxis within 24 hours following admission. This included a risk assessment, and credit was given for no prophylaxis and/or mechanical and/or pharmacologic prophylaxis per the ACCP guidelines.7
Observed-to-expected LOS was defined by using the University HealthSystem Consortium (UHC; now Vizient Inc) expected LOS for the given calendar year. This approach incorporates patient diagnoses, demographics, and other administrative variables to define an expected LOS for each patient.
The percent of patients discharged per day was defined from billing data as the percentage of a provider’s evaluation and management charges that were the final charge of a patient’s stay (regardless of whether a discharge day service was coded).
Discharge prior to 3
Depth of coding was defined as the number of coded diagnoses submitted to the Maryland Health Services Cost Review Commission for determining payment and was viewed as an indicator of the thoroughness of provider documentation.
Patient satisfaction was defined at the patient level (for those patients who turned in patient satisfaction surveys) as the pooled value of the 5 provider questions on the hospital’s patient satisfaction survey administered by Press Ganey: “time the physician spent with you,” “did the physician show concern for your questions/worries,” “did the physician keep you informed,” “friendliness/courtesy of the physician,” and “skill of the physician.”8
Readmission rates were defined as same-hospital readmissions divided by the total number of patients discharged by a given provider, with exclusions based on the Centers for Medicare and Medicaid Services hospital-wide, all-cause readmission measure.1 The expected same-hospital readmission rate was defined for each patient as the observed readmission rate in the entire UHC (Vizient) data set for all patients with the same All Patient Refined Diagnosis Related Group and severity of illness, as we have described previously.9
Communication with the primary care provider was the only self-reported metric used. It was based on a mandatory prompt on the discharge worksheet in the electronic medical record (EMR). Successful communication with the outpatient provider was defined as verbal or electronic communication by the hospitalist with the outpatient provider. Partial (50%) credit was given for providers who attempted but were unsuccessful in communicating with the outpatient provider, for patients for whom the provider had access to the Johns Hopkins EMR system, and for planned admissions without new or important information to convey. No credit was given for providers who indicated that communication was not indicated, who indicated that a patient and/or family would update the provider, or who indicated that the discharge summary would be sufficient.9 Because the discharge worksheet could be initiated at any time during the hospitalization, providers could document communication with the outpatient provider at any point during hospitalization.
Discharge summary turnaround was defined as the average number of days elapsed between the day of discharge and the signing of the discharge summary in the EMR.
Assigning Ownership of Patients to Individual Providers
Using billing data, we assigned ownership of patient care based on the type, timing, and number of charges that occurred during each hospitalization (Figure 1). Eligible charges included all history and physical (codes 99221, 99222, and 99223), subsequent care (codes 99231, 99232, and 99233), and discharge charges (codes 99238 and 99239).
By using a unique identifier assigned for each hospitalization, professional fees submitted by providers were used to identify which provider saw the patient on the admission day, discharge day, as well as subsequent care days. Providers’ productivity, bonus supplements, and policy compliance were determined by using billing data, which encouraged the prompt submittal of charges.
The provider who billed the admission history and physical (codes 99221, 99222, and 99223) within 1 calendar date of the patient’s initial admission was defined as the admitting provider. Patients transferred to the hospitalist service from other services were not assigned an admitting hospitalist. The sole metric assigned to the admitting hospitalist was ACCP-compliant VTE prophylaxis.
The provider who billed the final subsequent care or discharge code (codes 99231, 99232, 99233, 99238, and 99239) within 1 calendar date of discharge was defined as the discharging provider. For hospitalizations characterized by a single provider charge (eg, for patients admitted and discharged on the same day), the provider billing this charge was assigned as both the admitting and discharging physician. Patients upgraded to the intensive care unit (ICU) were not counted as a discharge unless the patient was downgraded and discharged from the hospitalist service. The discharging provider was assigned responsibility for the time of discharge, the percent of patients discharged per day, the discharge summary turnaround time, and hospital readmissions.
Metrics that were assigned to multiple providers for a single hospitalization were termed “provider day–weighted” metrics. The formula for calculating the weight for each provider day–weighted metric was as follows: weight for provider A = [number of daily charges billed by provider A] divided by [LOS +1]. The initial hospital day was counted as day 0. LOS plus 1 was used to recognize that a typical hospitalization will have a charge on the day of admission (day 0) and a charge on the day of discharge such that an LOS of 2 days (eg, a patient admitted on Monday and discharged on Wednesday) will have 3 daily charges. Provider day–weighted metrics included patient satisfaction, communication with the outpatient provider, depth of coding, and observed-to-expected LOS.
Our billing software prevented providers from the same group from billing multiple daily charges, thus ensuring that there were no duplicated charges submitted for a given day.
Presenting Results
Providers were only shown data from the day-weighted approach. For ease of visual interpretation, scores for each metric were scaled ordinally from 1 (worst performance) to 9 (best performance; Table 1). Data were displayed in a dashboard format on a password-protected website for each provider to view his or her own data relative to that of the hospitalist peer group. The dashboard was implemented in this format on July 1, 2011. Data were updated quarterly (Figure 2).
Results were displayed in a polyhedral or spider-web graph (Figure 2). Provider and group metrics were scaled according to predefined benchmarks established for each metric and standardized to a scale ranging from 1 to 9. The scale for each metric was set based on examining historical data and group median performance on the metrics to ensure that there was a range of performance (ie, to avoid having most hospitalists scoring a 1 or 9). Scaling thresholds were periodically adjusted as appropriate to maintain good visual discrimination. Higher scores (creating a larger-volume polygon) are desirable even for metrics such as LOS, for which a low value is desirable. Both a spider-web graph and trends over time were available to the provider (Figure 2). These graphs display a comparison of the individual provider scores for each metric to the hospitalist group average for that metric.
Comparison with the Standard (Attending of Record) Method of Attribution
For the purposes of this report, we sought to determine whether there were meaningful differences between our day-weighted approach versus the standard method of attribution, in which the attending of record is assigned responsibility for each metric that would not have been attributed to the discharging attending under both methods. Our goal was to determine where and whether there was a meaningful difference between the 2 methodologies, recognizing that the degree of difference between these 2 methodologies might vary in other institutions and settings. In our hospital, the attending of record is generally the discharging attending. In order to compare the 2 methodologies, we arbitrarily picked 2015 to retrospectively evaluate the differences between these 2 methods of attribution. We did not display or provide data using the standard methodology to providers at any point; this approach was used only for the purposes of this report. Because these metrics are intended to evaluate relative provider performance, we assigned a percentile to each provider for his or her performance on the given metric using our attribution methodology and then, similarly, assigned a percentile to each provider using the standard methodology. This yielded 2 percentile scores for each provider and each metric. We then compared these percentile ranks for providers in 2 ways: (1) we determined how often providers who scored in the top half of the group for a given metric (above the 50th percentile) also scored in the top half of the group for that metric by using the other calculation method, and (2) we calculated the absolute value of the difference in percentiles between the 2 methods to characterize the impact on a provider’s ranking for that metric that might result from switching to the other method. For instance, if a provider scored at the 20th percentile for the group in patient satisfaction with 1 attribution method and scored at the 40th percentile for the group in patient satisfaction using the other method, the absolute change in percentile would be 20 percentile points. But, this provider would still be below the 50th percentile by both methods (concordant bottom half performance). We did not perform this comparison for metrics assigned to the discharging provider (such as discharge summary turnaround time or readmissions) because the attending of record designation is assigned to the discharging provider at our hospital.
RESULTS
The dashboard was successfully operationalized on July 1, 2011, with displays visible to providers as shown in Figure 2. Consistent with the principles of providing effective performance feedback to providers, the display simultaneously showed providers their individual performance as well as the performance of their peers. Providers were able to view their spider-web plot for prior quarters. Not shown are additional views that allowed providers to see quarterly trends in their data versus their peers across several fiscal years. Also available to providers was their ranking relative to their peers for each metric; specific peers were deidentified in the display.
There was notable discordance between provider rankings between the 2 methodologies, as shown in Table 2. Provider performance above or below the median was concordant 56% to 75% of the time (depending on the particular metric), indicating substantial discordance because top-half or bottom-half concordance would be expected to occur by chance 50% of the time. Although the provider percentile differences between the 2 methods tended to be modest for most providers (the median difference between the methods was 13 to 22 percentile points for the various metrics), there were some providers for whom the method of calculation dramatically impacted their rankings. For 5 of the 6 metrics we examined, at least 1 provider had a 50-percentile or greater change in his or her ranking based on the method used. This indicates that at least some providers would have had markedly different scores relative to their peers had we used the alternative methodology (Table 2). In VTE prophylaxis, for example, at least 1 provider had a 94-percentile change in his or her ranking; similarly, a provider had an 88-perentile change in his or her LOS ranking between the 2 methodologies.
DISCUSSION
We found that it is possible to assign metrics across 1 hospital stay to multiple providers by using billing data. We also found a meaningful discrepancy in how well providers scored (relative to their peers) based on the method used for attribution. These results imply that hospitals should consider attributing performance metrics based on ascribed ownership from billing data and not just from attending of record status.
As hospitalist programs and providers in general are increasingly being asked to develop dashboards to monitor individual and group performance, correctly attributing care to providers is likely to become increasingly important. Experts agree that principles of effective provider performance dashboards include ranking individual provider performance relative to peers, clearly displaying data in an easily accessible format, and ensuring that data can be credibly attributed to the individual provider.3,4,6 However, there appears to be no gold standard method for attribution, especially in the inpatient setting. Our results imply that hospitals should consider attributing performance metrics based on ascribed ownership from billing data and not just from attending of record status.
Several limitations of our findings are important to consider. First, our program is a relatively small, academic group with handoffs that typically occur every 1 to 2 weeks and sometimes with additional handoffs on weekends. Different care patterns and settings might impact the utility of our attribution methodology relative to the standard methodology. Additionally, it is important to note that the relative merits of the different methodologies cannot be ascertained from our comparison. We can demonstrate discordance between the attribution methodologies, but we cannot say that 1 method is correct and the other is flawed. Although we believe that our day-weighted approach feels fairer to providers based on group input and feedback, we did not conduct a formal survey to examine providers’ preferences for the standard versus day-weighted approaches. The appropriateness of a particular attribution method needs to be assessed locally and may vary based on the clinical setting. For instance, on a service in which patients are admitted for procedures, it may make more sense to attribute the outcome of the case to the proceduralist even if that provider did not bill for the patient’s care on a daily basis. Finally, the computational requirements of our methodology are not trivial and require linking billing data with administrative patient-level data, which may be challenging to operationalize in some institutions.
These limitations aside, we believe that our attribution methodology has face validity. For example, a provider might be justifiably frustrated if, using the standard methodology, he or she is charged with the LOS of a patient who had been hospitalized for months, particularly if that patient is discharged shortly after the provider assumes care. Our method addresses this type of misattribution. Particularly when individual provider compensation is based on performance on metrics (as is the case at our institution), optimizing provider attribution to particular patients may be important, and face validity may be required for group buy-in.
In summary, we have demonstrated that it is possible to use billing data to assign ownership of patients to multiple providers over 1 hospital stay. This could be applied to other hospitalist programs as well as other healthcare settings in which multiple providers care for patients during 1 healthcare encounter (eg, ICUs).
Disclosure
The authors declare they have no relevant conflicts of interest.
1. Horwitz L, Partovian C, Lin Z, et al. Hospital-Wide (All-Condition) 30‐Day Risk-Standardized Readmission Measure. https://www.cms.gov/Medicare/Quality-Initiatives-Patient-Assessment-Instruments/MMS/downloads/MMSHospital-WideAll-ConditionReadmissionRate.pdf. Accessed March 6, 2015.
2. Medicine SoH. Measuring Hospitalist Performance: Metrics, Reports, and Dashboards. 2007; https://www.hospitalmedicine.org/Web/Practice_Management/Products_and_Programs/measure_hosp_perf_metrics_reports_dashboards.aspx. Accessed May 12, 2013.
3. Teleki SS, Shaw R, Damberg CL, McGlynn EA. Providing performance feedback to individual physicians: current practice and emerging lessons. Santa Monica, CA: RAND Corporation; 2006. 1-47. https://www.rand.org/content/dam/rand/pubs/working_papers/2006/RAND_WR381.pdf. Accessed August, 2017.
4. Brehaut JC, Colquhoun HL, Eva KW, et al. Practice Feedback Interventions: 15 Suggestions for Optimizing Effectiveness Practice Feedback Interventions. Ann Intern Med. 2016;164(6):435-441. PubMed
5. Dowding D, Randell R, Gardner P, et al. Dashboards for improving patient care: review of the literature. Int J Med Inform. 2015;84(2):87-100. PubMed
6. Landon BE, Normand S-LT, Blumenthal D, Daley J. Physician clinical performance assessment: prospects and barriers. JAMA. 2003;290(9):1183-1189. PubMed
7. Guyatt GH, Akl EA, Crowther M, Gutterman DD, Schuünemann HJ. Executive summary: Antit hrombotic therapy and prevention of thrombosis, 9th ed: American College of Chest Physicians evidence-based clinical practice guidelines. Ann Intern Med. 2012;141(2 suppl):7S-47S. PubMed
8. Siddiqui Z, Qayyum R, Bertram A, et al. Does Provider Self-reporting of Etiquette Behaviors Improve Patient Experience? A Randomized Controlled Trial. J Hosp Med. 2017;12(6):402-406. PubMed
9. Oduyebo I, Lehmann CU, Pollack CE, et al. Association of self-reported hospital discharge handoffs with 30-day readmissions. JAMA Intern Med. 2013;173(8):624-629. PubMed
1. Horwitz L, Partovian C, Lin Z, et al. Hospital-Wide (All-Condition) 30‐Day Risk-Standardized Readmission Measure. https://www.cms.gov/Medicare/Quality-Initiatives-Patient-Assessment-Instruments/MMS/downloads/MMSHospital-WideAll-ConditionReadmissionRate.pdf. Accessed March 6, 2015.
2. Medicine SoH. Measuring Hospitalist Performance: Metrics, Reports, and Dashboards. 2007; https://www.hospitalmedicine.org/Web/Practice_Management/Products_and_Programs/measure_hosp_perf_metrics_reports_dashboards.aspx. Accessed May 12, 2013.
3. Teleki SS, Shaw R, Damberg CL, McGlynn EA. Providing performance feedback to individual physicians: current practice and emerging lessons. Santa Monica, CA: RAND Corporation; 2006. 1-47. https://www.rand.org/content/dam/rand/pubs/working_papers/2006/RAND_WR381.pdf. Accessed August, 2017.
4. Brehaut JC, Colquhoun HL, Eva KW, et al. Practice Feedback Interventions: 15 Suggestions for Optimizing Effectiveness Practice Feedback Interventions. Ann Intern Med. 2016;164(6):435-441. PubMed
5. Dowding D, Randell R, Gardner P, et al. Dashboards for improving patient care: review of the literature. Int J Med Inform. 2015;84(2):87-100. PubMed
6. Landon BE, Normand S-LT, Blumenthal D, Daley J. Physician clinical performance assessment: prospects and barriers. JAMA. 2003;290(9):1183-1189. PubMed
7. Guyatt GH, Akl EA, Crowther M, Gutterman DD, Schuünemann HJ. Executive summary: Antit hrombotic therapy and prevention of thrombosis, 9th ed: American College of Chest Physicians evidence-based clinical practice guidelines. Ann Intern Med. 2012;141(2 suppl):7S-47S. PubMed
8. Siddiqui Z, Qayyum R, Bertram A, et al. Does Provider Self-reporting of Etiquette Behaviors Improve Patient Experience? A Randomized Controlled Trial. J Hosp Med. 2017;12(6):402-406. PubMed
9. Oduyebo I, Lehmann CU, Pollack CE, et al. Association of self-reported hospital discharge handoffs with 30-day readmissions. JAMA Intern Med. 2013;173(8):624-629. PubMed
© 2017 Society of Hospital Medicine
A Comparison of Conventional and Expanded Physician Assistant Hospitalist Staffing Models at a Community Hospital
From Physicians Inpatient Care Specialists (MDICS), Hanover, MD (Dr. Capstack, Ms. Vollono), Versant Statistical Solutions, Raleigh, NC (Ms. Segujja), Anne Arundel Medical Center, Annapolis, MD (Dr. Moser [at the time of the study], Dr. Meisenberg), and Johns Hopkins Hospital, Baltimore, MD (Dr. Michtalik).
Abstract
- Objective: To determine whether a higher than conventional physician assistant (PA)–to-physician hospitalist staffing ratio can achieve similar clinical outcomes for inpatients at a community hospital.
- Methods: Retrospective cohort study comparing 2 hospitalist groups at a 384-bed community hospital, one with a high PA-to-physician ratio model (“expanded PA”), with 3 physicians/3 PAs and the PAs rounding on 14 patients a day (35.73% of all visits), and the other with a low PA-to-physician ratio model (“conventional”), with 9 physicians/2 PAs and the PAs rounding on 9 patients a day (5.89% of all visits). For 16,964 adult patients discharged by the hospitalist groups with a medical principal APR-DRG code between January 2012 and June 2013, in-hospital mortality, cost of care, readmissions, length of stay (LOS) and consultant use were analyzed using logistic regression and adjusted for age, insurance status, severity of illness, and risk of mortality.
- Results: No statistically significant differences were found between the 2 groups for in-hospital mortality (odds ratio [OR], 0.89 [95% confidence interval {CI}, 0.66–1.19]; P = 0.42), readmissions (OR, 0.95 [95% CI, 0.87–1.04]; P = 0.27), length of stay (effect size 0.99 days shorter LOS in expanded PA group, 95% CI, 0.97 to 1.01 days; P = 0.34) or consultant use (OR 1.00, 95% CI 0.94–1.07, P = 0.90). Cost of care was less in the expanded PA group (effect size 3.52% less; estimated cost $2644 vs $2724; 95% CI 2.66%–4.39%, P < 0.001).
- Conclusion: An expanded PA hospitalist staffing model at a community hospital provided similar outcomes at a lower cost of care.
Hospitalist program staffing models must optimize efficiency while maintaining clinical outcomes in order to increase value and decrease costs [1]. The cost of hospitalist programs is burdensome, with nearly 94% of groups nationally requiring financial support beyond professional fees [2]. Nationally, for hospitalist groups serving adults, average institutional support is over $156,000 per physician full time equivalent (FTE) (182 twelve-hour clinical shifts per calendar year) [2]. Significant savings could be achieved if less costly physician assistants could be incorporated into clinical teams to provide similar care without sacrificing quality.
Nurse practitioners (NPs) and physician assistants (PAs) have been successfully employed on academic hospitalist services to complement physician staffing [3–10]. They perform admissions, consults, rounding visits and discharges with physician collaboration as permitted by each group’s policies and in accordance with hospital by-laws and state regulations. A median of 0.25 NP and 0.28 PA FTEs per physician FTE are employed by hospitalist groups that incorporate them, though staffing ratios vary widely [2].
Physicians Inpatient Care Specialists (MDICS) devel-oped a staffing model that deploys PAs to see a large proportion of its patients collaboratively with physicians, and with a higher patient census per PA than has been previously reported [2–5]. The group leaders believed that this would yield similar outcomes for patients at a lower cost to the supporting institution than a conventional staffing model which used fewer PAs to render patient care. Prior inpatient studies have demonstrated comparable clinical outcomes when comparing hospitalist PAs and NPs to residents and fellows [4–10], but to our knowledge no data exist directly comparing hospitalist PAs to hospitalist physicians. This study goes beyond prior work by examining the community, non-teaching setting, and directly comparing outcomes from the expanded use of PAs to those of a hospitalist group staffed with a greater proportion of attending physicians at the same hospital during the same time.
Methods
Setting
The study was performed at Anne Arundel Medical Center (AAMC), a 384-bed community hospital in Annapolis, Maryland, that serves a region of over 1 million people. Approximately 26,000 adult patients are discharged annually. During the study, more than 90% of internal medicine service inpatients were cared for by one of 2 hospitalist groups: a hospital-employed group (“conventional” group, Anne Arundel Medical Group) and a contracted hospitalist group (“expanded PA” group, Physicians Inpatient Care Specialists). The conventional group’s providers received a small incentive for Core Measures compliance for patients with stroke, myocardial infarction, congestive heart failure and pneumonia. The expanded PA group received a flat fee for providing hospitalist services and the group’s providers received a small incentive for productivity from their employer. The study was deemed exempt by the AAMC institutional review board.
Staffing Models, Patient Allocation, and Assignment
Admitted patients were designated to be admitted to one group or the other on the basis of standing arrangements with the patients’ primary care providers. Consultative referrals could also be made from subspecialists, who had discretion as to which group they wished to use.
Each morning, following sign-out report from the night team, each team of day providers determined which patients would be seen by which of their providers. Patients still on service from the previous day would be seen by the same provider again whenever possible in order to maintain continuity. Each individual provider had their own patients for the day who they rounded on independently and were responsible for. Physician involvement with patients seen primarily by PAs occurred as described below. Physicians in both groups were expected to take primary rounding responsibility for patients who were more acute or more complex based on morning sign-out report; there was no more formal mandate for patient allocation to particular provider type.
Physician-PA Collaboration
Patients
Patients discharged between 1 January 2012 and 30 June 2013 by the hospitalist groups were identified by searching AAMC’s Crimson Continuuum of Care (The Advisory Board, Washington, DC), a software analytic tool that is integrated with coded clinical data. Adult patient hospitalizations determined by Crimson to have a medical (non-surgical, non-obstetrical) APR-DRG code as the final principal diagnosis were included. Critically ill patients or those appropriate for “step-down unit” care were cared for by the in-house critical care staff; upon transfer out of critical or step-down care, patients were referred back to the admitting hospitalist team. A diagnosis (and its associated hospitalizations) was excluded for referral bias if the diagnosis was the principal diagnosis for at least 1% of a group’s discharges and the percentage of patients with that diagnosis was at least two times greater in one group than the other. Hospitalizations with a diagnosis of “ungroupable” (APR-DRG 956) were also excluded.
Measurements
Demographic, insurance status, cost of care, length of stay (LOS), APR-DRG (All Patient Refined Diagnosis-Related Group) severity of illness (SOI) and risk of mortality (ROM), consultant utilization, 30-day all-cause readmission (“readmission rate”), and mortality information was obtained from administrative data and exported into a single database for statistical analysis. Readmissions, inpatient mortality, and cost of care were the primary outcomes; consultant use and length of stay were secondary outcomes. A hospitalization was considered a readmission if the patient returned to inpatient status at AAMC for any reason within 30 days of a previous inpatient discharge. Inpatient mortality was defined as patient death during hospitalization. The cost of care was measured using the case charges associated with each encounter. Charge capture data from both groups was analyzed to classify visits as “physician-only,” “physician co-visit,” and “PA-only” visits. A co-visit consists of the physician visiting the patient after the PA has already done so on the same day, taking their own history and performing their own physical exam, and writing a brief progress note. These data were compared against the exported administrative data to find matching encounters and associated visits, with only matching visits included in the analysis. If a duplicate charge was entered on the same day for a patient, any conflict was resolved in favor of the physician visit. A total of 49,883 and 28,663 matching charges were identified for the conventional and expanded PA groups.
Statistical Methods
Odds of inpatient mortality were calculated using logistic regression and adjusted for age, insurance status, APR-DRG ROM, and LOS. Odds of readmission were calculated using logistic regression and adjusted for age, LOS, insurance and APR-DRG SOI. Cost of care (effect size) was examined using multiple linear regression and adjusted for age, APR-DRG SOI, insurance status and LOS. This model was fit using the logarithmic transformations of cost of care and LOS to correct deviation from normality. Robust regression using MM estimation was used to estimate group effects due to the existence of outliers and high leverage points. Length of stay (effect size) was assessed using the log-transformed variable and adjusted for APR-DRG SOI, age, insurance status and consultant use. Finally, category logistic regression models were fit to estimate the odds of consultant use in the study groups and adjusted for age, LOS, insurance status and APR-DRG SOI.
Results
Records review identified 17,294 adult patient hospitalizations determined by Crimson to have a medical (non-surgical, non-obstetrical) APR-DRG code as the final principal diagnosis. We excluded 15 expanded PA and 11 conventional hospitalizations that fell under APR-DRG code 956 “ungroupable.” Exclusion for referral bias resulted in the removal of 304 hospitalizations, 207 (3.03%) from the expanded PA group and 97 (0.92%) from the conventional group. These excluded hospitalizations came from 2 APR-DRG codes, urinary stones (code 465) and “other kidney and urinary tract diagnoses” (code 468). This left 6612 hospitalizations in the expanded PA group and 10,352 in the conventional group.
Charge capture data for both groups was used to determine the proportion of encounters rendered by each provider type or combination. In the expanded PA group, 35.73% of visits (10,241 of 28,663) were conducted by a PA, and 64.27% were conducted by a physician or by a PA with a billable physician “co-visit.” In the conventional group, 5.89% of visits (2938 of 49,883) were conducted by a PA, and 94.11% were conducted by a physician only or by a PA with a billable physician “co-visit”.
Readmissions
Overall, 929 of 6612 (14.05%) and 1417 of 10,352 (13.69%) patients were readmitted after being discharged by the expanded PA and conventional groups, respectively. After multivariate analysis, there was no statistically significant difference in odds of readmission between the groups (OR for conventional group, 0.95 [95% CI, 0.87–1.04]; P = 0.27).
Inpatient Mortality
Unadjusted inpatient mortality for the expanded PA group was 1.30% and 0.99% for the conventional group. After multivariate analysis, there was no statistically significant difference in odds of in-hospital mortality between the groups (OR for conventional group, 0.89 [95% CI, 0.66–1.19]; P = 0.42).
Patient Charges
The unadjusted mean patient charge in the expanded PA group was $7822 ± $7755 and in the conventional group mean patient charge was $8307 ± 10,034. Multivariate analysis found significantly lower adjusted patient charges in the expanded PA group relative to the conventional group (3.52% lower in the expanded PA group [95% CI, 2.66%–4.39%, P < 0.001). When comparing a “standard” patient who was between 80–89 and had Medicare insurance and an SOI of “major,” the cost of care was $2644 in the expanded PA group vs $2724 in the conventional group.
Length of Stay
Unadjusted mean length of stay was 4.1 ± 3.9 days and 4.3 ± 5.6 days for the expanded PA and conventional groups, respectively. After multivariate analysis, when comparing the statistical model “standard” patient, there was no significant difference in the length of stay between the 2 groups (effect size, 0.99 days shorter LOS in the expanded PA group [95% CI, 0.97–1.01 days]; P = 0.34)
Consultant Use
Utilization of consultants was also assessed. The expanded PA group used a mean of 0.55 consultants per case, and the conventional group used 0.56. After multivariate adjustment, there was no significant difference in consulting service use between groups (OR 1.00 [95% CI, 0.94–1.07]; P = 0.90).
Discussion
Maximizing value and minimizing health care costs is a national priority. To our knowledge, this is the first study to compare hospitalist PAs in a community, non-teaching practice directly and contemporaneously to peer PAs and attending physicians and examine the impact on outcomes. In our study, a much larger proportion of patient visits were conducted primarily by PAs without a same-day physician visit in the expanded PA group (35.73%, vs 5.89% in the conventional group). There was no statistically significant difference in inpatient mortality, length of stay or readmissions. In addition, costs of care measured as hospital charges to patients were lower in the expanded PA group. Consultants were not used disproportionately by the expanded PA group in order to achieve these results. Our results are consistent with studies that have compared PAs and NPs at academic centers to traditional housestaff teams and which show that services staffed with PAs or NPs that provide direct care to medical inpatients are non-inferior [4–10].
This study’s expanded PA group’s PAs rounded on 14 patients per day, close to the “magic 15” that is considered by many a good compromise for hospitalist physicians between productivity and quality [11,12]. This is substantially more than the 6 to 10 patients PAs have been responsible for in previously reported studies [3,4,6]. As the median salary for a PA hospitalist is $102,960 compared with the median internal medicine physician hospitalist salary of $253,977 [2], using hospitalist PAs in a collaboration model as described herein could result in significant savings for supporting institutions without sacrificing quality.
We recognize several limitations to this study. First, the data were obtained retrospectively from a single center and patient assignment between groups was nonrandomized. The significant differences in the baseline characteristics of patients between the study groups, however, were adjusted for in multivariate analysis, and potential referral bias was addressed through our exclusion criteria. Second, our comparison relied on coding rather than clinical data for diagnosis grouping. However, administrative data is commonly used to determine the primary diagnosis for study patients and the standard for reimbursement. Third, we recognize that there may have been unmeasured confounders that may have affected the outcomes. However, the same resources, including consultants and procedure services, were readily available to both groups and there was no significant difference in consultation rates. Fourth, “cost of care” was measured as overall charges to patients, not cost to the hospital. However, given that all the encounters occurred at the same hospital in the same time frame, the difference should be proportional and equal between groups. Finally, our readmission rates did not account for patients readmitted to other institutions. However, there should not have been a differential effect between the 2 study groups, given the shared patient catchment area and our exclusion for referral bias.
It should also be noted that the expanded PA group used a structured collaboration framework and incorporated a structured education program for its PAs. These components are integral to the expanded PA model, and our results may not be generalizable outside of a similar framework. The expanded PA group’s PAs were carefully selected at the time of hire, specifically educated, and supported through ongoing collaboration to provide efficient and appropriate care at the “top of their licenses”. Not all medical groups will be able to provide this level of support and education, and not all hospitalist PAs will want to and/or be able to reach this level of proficiency. However, successful implementation is entirely achievable for groups that invest the effort. The MDICS education process included 80 hours of didactic sessions spread over several months and is based on the Society of Hospital Medicine Core Competencies [13] as well as 6 months of supervised bedside education with escalating clinical responsibilities under the tutelage of an experienced physician or PA. Year-long academic PA fellowships have also been developed for purposes of similar training at several institutions [14].
Conclusion
Our results show that expanded use of well-educated PAs functioning within a formal collaboration arrangement with physicians provides similar clinical quality to a conventional PA staffing model with no excess patient care costs. The model also allows substantial salary savings to supporting institutions, which is important to hospital and policy stakeholders given the implications for hospitalist group staffing, increasing value, and allocation of precious time and financial resources.
Acknowledgements: The authors wish to thank Kevin Funk, MBA, of MDICS, Clarence Richardson, MBA, of GeBBs Software International, and Heather Channing, Kayla King, and Laura Knox of Anne Arundel Healthcare Enterprise, who provided invaluable help with the data aggregation used for this study.
Corresponding author: Timothy M. Capstack, MD, 7250 Parkway Dr, Suite 500, Hanover, MD 21076, tcapstack@mdics.com.
Financial disclosures: Dr. Capstack has ownership interest in Physicians Inpatient Care Specialists (MDICS). Ms. Segujja received compensation from MDICS for statistical analysis.
1. Michtalik HJ, Pronovost PJ, Marsteller JA, et al. Developing a model for attending physician workload and outcomes. JAMA Intern Med 2013;173:1026–8.
2. Society of Hospital Medicine. State of hospital medicine report. Philadelphia: Society of Hospital Medicine; 2014.
3. Kartha A, Restuccia J, Burgess J, et al. Nurse practitioner and physician assistant scope of practice in 118 acute care hospitals. J Hosp Med 2014;9:615–20.
4. Dhuper S, Choksi S. Replacing an academic internal medicine residency program with a physician assistant--hospitalist model: a comparative analysis study. Am J Med Qual 2008;24:132–9.
5. Morris D, Reilly P, Rohrbach J, et al. The influence of unit-based nurse practitioners on hospital outcomes and readmission rates for patients with trauma. J Trauma Acute Care Surg 2012;73:474–8.
6. Roy C, Liang C, Lund M, et al. Implementation of a physician assistant/hospitalist service in an academic medical center: impact on efficiency and patient outcomes. J Hosp Med 2008;3:361–8.
7. Singh S, Fletcher K, Schapira M, et al. A comparison of outcomes of general medical inpatient care provided by a hospitalist-physician assistant model vs a traditional resident-based model. J Hosp Med 2011;6:122–30.
8. Hoffman L, Tasota F, Zullo T, et al. Outcomes of care managed by an acute care nurse practitioner/attending physician team in an subacute medical intensive care unit. Am J Crit Care 2005;14:121–30.
9. Kapu A, Kleinpell R, Pilon B. Quality and financial impact of adding nurse practitioners to inpatient care teams. J Nurs Adm 2014;44:87–96.
10. Cowan M, Shapiro M, Hays R, et al. The effect of a multidisciplinary hospitalist/physician and advanced practice nurse collaboration on hospital costs. J Nurs Adm 2006;36:79–85.
11. Michtalik HJ, Yeh HC, Pronovost PJ, Brotman DJ. Impact of attending physician workload on patient care: A survey of hospitalists. JAMA Intern Med 2013;173:375–7.
12. Elliott D, Young R, Brice J, et al. Effect of hospitalist workload on the quality and efficiency of care. JAMA Internal Med 2014;174:786–93.
13. McKean S, Budnitz T, Dressler D, et al. How to use the core competencies in hospital medicine: a framework for curriculum development. J Hosp Med 2006; 1 Suppl 1:57–67.
14. Will K, Budavari A, Wilkens J, et al. A hospitalist postgraduate training program for physician assistants. J Hosp Med 2010;5:94–8.
From Physicians Inpatient Care Specialists (MDICS), Hanover, MD (Dr. Capstack, Ms. Vollono), Versant Statistical Solutions, Raleigh, NC (Ms. Segujja), Anne Arundel Medical Center, Annapolis, MD (Dr. Moser [at the time of the study], Dr. Meisenberg), and Johns Hopkins Hospital, Baltimore, MD (Dr. Michtalik).
Abstract
- Objective: To determine whether a higher than conventional physician assistant (PA)–to-physician hospitalist staffing ratio can achieve similar clinical outcomes for inpatients at a community hospital.
- Methods: Retrospective cohort study comparing 2 hospitalist groups at a 384-bed community hospital, one with a high PA-to-physician ratio model (“expanded PA”), with 3 physicians/3 PAs and the PAs rounding on 14 patients a day (35.73% of all visits), and the other with a low PA-to-physician ratio model (“conventional”), with 9 physicians/2 PAs and the PAs rounding on 9 patients a day (5.89% of all visits). For 16,964 adult patients discharged by the hospitalist groups with a medical principal APR-DRG code between January 2012 and June 2013, in-hospital mortality, cost of care, readmissions, length of stay (LOS) and consultant use were analyzed using logistic regression and adjusted for age, insurance status, severity of illness, and risk of mortality.
- Results: No statistically significant differences were found between the 2 groups for in-hospital mortality (odds ratio [OR], 0.89 [95% confidence interval {CI}, 0.66–1.19]; P = 0.42), readmissions (OR, 0.95 [95% CI, 0.87–1.04]; P = 0.27), length of stay (effect size 0.99 days shorter LOS in expanded PA group, 95% CI, 0.97 to 1.01 days; P = 0.34) or consultant use (OR 1.00, 95% CI 0.94–1.07, P = 0.90). Cost of care was less in the expanded PA group (effect size 3.52% less; estimated cost $2644 vs $2724; 95% CI 2.66%–4.39%, P < 0.001).
- Conclusion: An expanded PA hospitalist staffing model at a community hospital provided similar outcomes at a lower cost of care.
Hospitalist program staffing models must optimize efficiency while maintaining clinical outcomes in order to increase value and decrease costs [1]. The cost of hospitalist programs is burdensome, with nearly 94% of groups nationally requiring financial support beyond professional fees [2]. Nationally, for hospitalist groups serving adults, average institutional support is over $156,000 per physician full time equivalent (FTE) (182 twelve-hour clinical shifts per calendar year) [2]. Significant savings could be achieved if less costly physician assistants could be incorporated into clinical teams to provide similar care without sacrificing quality.
Nurse practitioners (NPs) and physician assistants (PAs) have been successfully employed on academic hospitalist services to complement physician staffing [3–10]. They perform admissions, consults, rounding visits and discharges with physician collaboration as permitted by each group’s policies and in accordance with hospital by-laws and state regulations. A median of 0.25 NP and 0.28 PA FTEs per physician FTE are employed by hospitalist groups that incorporate them, though staffing ratios vary widely [2].
Physicians Inpatient Care Specialists (MDICS) devel-oped a staffing model that deploys PAs to see a large proportion of its patients collaboratively with physicians, and with a higher patient census per PA than has been previously reported [2–5]. The group leaders believed that this would yield similar outcomes for patients at a lower cost to the supporting institution than a conventional staffing model which used fewer PAs to render patient care. Prior inpatient studies have demonstrated comparable clinical outcomes when comparing hospitalist PAs and NPs to residents and fellows [4–10], but to our knowledge no data exist directly comparing hospitalist PAs to hospitalist physicians. This study goes beyond prior work by examining the community, non-teaching setting, and directly comparing outcomes from the expanded use of PAs to those of a hospitalist group staffed with a greater proportion of attending physicians at the same hospital during the same time.
Methods
Setting
The study was performed at Anne Arundel Medical Center (AAMC), a 384-bed community hospital in Annapolis, Maryland, that serves a region of over 1 million people. Approximately 26,000 adult patients are discharged annually. During the study, more than 90% of internal medicine service inpatients were cared for by one of 2 hospitalist groups: a hospital-employed group (“conventional” group, Anne Arundel Medical Group) and a contracted hospitalist group (“expanded PA” group, Physicians Inpatient Care Specialists). The conventional group’s providers received a small incentive for Core Measures compliance for patients with stroke, myocardial infarction, congestive heart failure and pneumonia. The expanded PA group received a flat fee for providing hospitalist services and the group’s providers received a small incentive for productivity from their employer. The study was deemed exempt by the AAMC institutional review board.
Staffing Models, Patient Allocation, and Assignment
Admitted patients were designated to be admitted to one group or the other on the basis of standing arrangements with the patients’ primary care providers. Consultative referrals could also be made from subspecialists, who had discretion as to which group they wished to use.
Each morning, following sign-out report from the night team, each team of day providers determined which patients would be seen by which of their providers. Patients still on service from the previous day would be seen by the same provider again whenever possible in order to maintain continuity. Each individual provider had their own patients for the day who they rounded on independently and were responsible for. Physician involvement with patients seen primarily by PAs occurred as described below. Physicians in both groups were expected to take primary rounding responsibility for patients who were more acute or more complex based on morning sign-out report; there was no more formal mandate for patient allocation to particular provider type.
Physician-PA Collaboration
Patients
Patients discharged between 1 January 2012 and 30 June 2013 by the hospitalist groups were identified by searching AAMC’s Crimson Continuuum of Care (The Advisory Board, Washington, DC), a software analytic tool that is integrated with coded clinical data. Adult patient hospitalizations determined by Crimson to have a medical (non-surgical, non-obstetrical) APR-DRG code as the final principal diagnosis were included. Critically ill patients or those appropriate for “step-down unit” care were cared for by the in-house critical care staff; upon transfer out of critical or step-down care, patients were referred back to the admitting hospitalist team. A diagnosis (and its associated hospitalizations) was excluded for referral bias if the diagnosis was the principal diagnosis for at least 1% of a group’s discharges and the percentage of patients with that diagnosis was at least two times greater in one group than the other. Hospitalizations with a diagnosis of “ungroupable” (APR-DRG 956) were also excluded.
Measurements
Demographic, insurance status, cost of care, length of stay (LOS), APR-DRG (All Patient Refined Diagnosis-Related Group) severity of illness (SOI) and risk of mortality (ROM), consultant utilization, 30-day all-cause readmission (“readmission rate”), and mortality information was obtained from administrative data and exported into a single database for statistical analysis. Readmissions, inpatient mortality, and cost of care were the primary outcomes; consultant use and length of stay were secondary outcomes. A hospitalization was considered a readmission if the patient returned to inpatient status at AAMC for any reason within 30 days of a previous inpatient discharge. Inpatient mortality was defined as patient death during hospitalization. The cost of care was measured using the case charges associated with each encounter. Charge capture data from both groups was analyzed to classify visits as “physician-only,” “physician co-visit,” and “PA-only” visits. A co-visit consists of the physician visiting the patient after the PA has already done so on the same day, taking their own history and performing their own physical exam, and writing a brief progress note. These data were compared against the exported administrative data to find matching encounters and associated visits, with only matching visits included in the analysis. If a duplicate charge was entered on the same day for a patient, any conflict was resolved in favor of the physician visit. A total of 49,883 and 28,663 matching charges were identified for the conventional and expanded PA groups.
Statistical Methods
Odds of inpatient mortality were calculated using logistic regression and adjusted for age, insurance status, APR-DRG ROM, and LOS. Odds of readmission were calculated using logistic regression and adjusted for age, LOS, insurance and APR-DRG SOI. Cost of care (effect size) was examined using multiple linear regression and adjusted for age, APR-DRG SOI, insurance status and LOS. This model was fit using the logarithmic transformations of cost of care and LOS to correct deviation from normality. Robust regression using MM estimation was used to estimate group effects due to the existence of outliers and high leverage points. Length of stay (effect size) was assessed using the log-transformed variable and adjusted for APR-DRG SOI, age, insurance status and consultant use. Finally, category logistic regression models were fit to estimate the odds of consultant use in the study groups and adjusted for age, LOS, insurance status and APR-DRG SOI.
Results
Records review identified 17,294 adult patient hospitalizations determined by Crimson to have a medical (non-surgical, non-obstetrical) APR-DRG code as the final principal diagnosis. We excluded 15 expanded PA and 11 conventional hospitalizations that fell under APR-DRG code 956 “ungroupable.” Exclusion for referral bias resulted in the removal of 304 hospitalizations, 207 (3.03%) from the expanded PA group and 97 (0.92%) from the conventional group. These excluded hospitalizations came from 2 APR-DRG codes, urinary stones (code 465) and “other kidney and urinary tract diagnoses” (code 468). This left 6612 hospitalizations in the expanded PA group and 10,352 in the conventional group.
Charge capture data for both groups was used to determine the proportion of encounters rendered by each provider type or combination. In the expanded PA group, 35.73% of visits (10,241 of 28,663) were conducted by a PA, and 64.27% were conducted by a physician or by a PA with a billable physician “co-visit.” In the conventional group, 5.89% of visits (2938 of 49,883) were conducted by a PA, and 94.11% were conducted by a physician only or by a PA with a billable physician “co-visit”.
Readmissions
Overall, 929 of 6612 (14.05%) and 1417 of 10,352 (13.69%) patients were readmitted after being discharged by the expanded PA and conventional groups, respectively. After multivariate analysis, there was no statistically significant difference in odds of readmission between the groups (OR for conventional group, 0.95 [95% CI, 0.87–1.04]; P = 0.27).
Inpatient Mortality
Unadjusted inpatient mortality for the expanded PA group was 1.30% and 0.99% for the conventional group. After multivariate analysis, there was no statistically significant difference in odds of in-hospital mortality between the groups (OR for conventional group, 0.89 [95% CI, 0.66–1.19]; P = 0.42).
Patient Charges
The unadjusted mean patient charge in the expanded PA group was $7822 ± $7755 and in the conventional group mean patient charge was $8307 ± 10,034. Multivariate analysis found significantly lower adjusted patient charges in the expanded PA group relative to the conventional group (3.52% lower in the expanded PA group [95% CI, 2.66%–4.39%, P < 0.001). When comparing a “standard” patient who was between 80–89 and had Medicare insurance and an SOI of “major,” the cost of care was $2644 in the expanded PA group vs $2724 in the conventional group.
Length of Stay
Unadjusted mean length of stay was 4.1 ± 3.9 days and 4.3 ± 5.6 days for the expanded PA and conventional groups, respectively. After multivariate analysis, when comparing the statistical model “standard” patient, there was no significant difference in the length of stay between the 2 groups (effect size, 0.99 days shorter LOS in the expanded PA group [95% CI, 0.97–1.01 days]; P = 0.34)
Consultant Use
Utilization of consultants was also assessed. The expanded PA group used a mean of 0.55 consultants per case, and the conventional group used 0.56. After multivariate adjustment, there was no significant difference in consulting service use between groups (OR 1.00 [95% CI, 0.94–1.07]; P = 0.90).
Discussion
Maximizing value and minimizing health care costs is a national priority. To our knowledge, this is the first study to compare hospitalist PAs in a community, non-teaching practice directly and contemporaneously to peer PAs and attending physicians and examine the impact on outcomes. In our study, a much larger proportion of patient visits were conducted primarily by PAs without a same-day physician visit in the expanded PA group (35.73%, vs 5.89% in the conventional group). There was no statistically significant difference in inpatient mortality, length of stay or readmissions. In addition, costs of care measured as hospital charges to patients were lower in the expanded PA group. Consultants were not used disproportionately by the expanded PA group in order to achieve these results. Our results are consistent with studies that have compared PAs and NPs at academic centers to traditional housestaff teams and which show that services staffed with PAs or NPs that provide direct care to medical inpatients are non-inferior [4–10].
This study’s expanded PA group’s PAs rounded on 14 patients per day, close to the “magic 15” that is considered by many a good compromise for hospitalist physicians between productivity and quality [11,12]. This is substantially more than the 6 to 10 patients PAs have been responsible for in previously reported studies [3,4,6]. As the median salary for a PA hospitalist is $102,960 compared with the median internal medicine physician hospitalist salary of $253,977 [2], using hospitalist PAs in a collaboration model as described herein could result in significant savings for supporting institutions without sacrificing quality.
We recognize several limitations to this study. First, the data were obtained retrospectively from a single center and patient assignment between groups was nonrandomized. The significant differences in the baseline characteristics of patients between the study groups, however, were adjusted for in multivariate analysis, and potential referral bias was addressed through our exclusion criteria. Second, our comparison relied on coding rather than clinical data for diagnosis grouping. However, administrative data is commonly used to determine the primary diagnosis for study patients and the standard for reimbursement. Third, we recognize that there may have been unmeasured confounders that may have affected the outcomes. However, the same resources, including consultants and procedure services, were readily available to both groups and there was no significant difference in consultation rates. Fourth, “cost of care” was measured as overall charges to patients, not cost to the hospital. However, given that all the encounters occurred at the same hospital in the same time frame, the difference should be proportional and equal between groups. Finally, our readmission rates did not account for patients readmitted to other institutions. However, there should not have been a differential effect between the 2 study groups, given the shared patient catchment area and our exclusion for referral bias.
It should also be noted that the expanded PA group used a structured collaboration framework and incorporated a structured education program for its PAs. These components are integral to the expanded PA model, and our results may not be generalizable outside of a similar framework. The expanded PA group’s PAs were carefully selected at the time of hire, specifically educated, and supported through ongoing collaboration to provide efficient and appropriate care at the “top of their licenses”. Not all medical groups will be able to provide this level of support and education, and not all hospitalist PAs will want to and/or be able to reach this level of proficiency. However, successful implementation is entirely achievable for groups that invest the effort. The MDICS education process included 80 hours of didactic sessions spread over several months and is based on the Society of Hospital Medicine Core Competencies [13] as well as 6 months of supervised bedside education with escalating clinical responsibilities under the tutelage of an experienced physician or PA. Year-long academic PA fellowships have also been developed for purposes of similar training at several institutions [14].
Conclusion
Our results show that expanded use of well-educated PAs functioning within a formal collaboration arrangement with physicians provides similar clinical quality to a conventional PA staffing model with no excess patient care costs. The model also allows substantial salary savings to supporting institutions, which is important to hospital and policy stakeholders given the implications for hospitalist group staffing, increasing value, and allocation of precious time and financial resources.
Acknowledgements: The authors wish to thank Kevin Funk, MBA, of MDICS, Clarence Richardson, MBA, of GeBBs Software International, and Heather Channing, Kayla King, and Laura Knox of Anne Arundel Healthcare Enterprise, who provided invaluable help with the data aggregation used for this study.
Corresponding author: Timothy M. Capstack, MD, 7250 Parkway Dr, Suite 500, Hanover, MD 21076, tcapstack@mdics.com.
Financial disclosures: Dr. Capstack has ownership interest in Physicians Inpatient Care Specialists (MDICS). Ms. Segujja received compensation from MDICS for statistical analysis.
From Physicians Inpatient Care Specialists (MDICS), Hanover, MD (Dr. Capstack, Ms. Vollono), Versant Statistical Solutions, Raleigh, NC (Ms. Segujja), Anne Arundel Medical Center, Annapolis, MD (Dr. Moser [at the time of the study], Dr. Meisenberg), and Johns Hopkins Hospital, Baltimore, MD (Dr. Michtalik).
Abstract
- Objective: To determine whether a higher than conventional physician assistant (PA)–to-physician hospitalist staffing ratio can achieve similar clinical outcomes for inpatients at a community hospital.
- Methods: Retrospective cohort study comparing 2 hospitalist groups at a 384-bed community hospital, one with a high PA-to-physician ratio model (“expanded PA”), with 3 physicians/3 PAs and the PAs rounding on 14 patients a day (35.73% of all visits), and the other with a low PA-to-physician ratio model (“conventional”), with 9 physicians/2 PAs and the PAs rounding on 9 patients a day (5.89% of all visits). For 16,964 adult patients discharged by the hospitalist groups with a medical principal APR-DRG code between January 2012 and June 2013, in-hospital mortality, cost of care, readmissions, length of stay (LOS) and consultant use were analyzed using logistic regression and adjusted for age, insurance status, severity of illness, and risk of mortality.
- Results: No statistically significant differences were found between the 2 groups for in-hospital mortality (odds ratio [OR], 0.89 [95% confidence interval {CI}, 0.66–1.19]; P = 0.42), readmissions (OR, 0.95 [95% CI, 0.87–1.04]; P = 0.27), length of stay (effect size 0.99 days shorter LOS in expanded PA group, 95% CI, 0.97 to 1.01 days; P = 0.34) or consultant use (OR 1.00, 95% CI 0.94–1.07, P = 0.90). Cost of care was less in the expanded PA group (effect size 3.52% less; estimated cost $2644 vs $2724; 95% CI 2.66%–4.39%, P < 0.001).
- Conclusion: An expanded PA hospitalist staffing model at a community hospital provided similar outcomes at a lower cost of care.
Hospitalist program staffing models must optimize efficiency while maintaining clinical outcomes in order to increase value and decrease costs [1]. The cost of hospitalist programs is burdensome, with nearly 94% of groups nationally requiring financial support beyond professional fees [2]. Nationally, for hospitalist groups serving adults, average institutional support is over $156,000 per physician full time equivalent (FTE) (182 twelve-hour clinical shifts per calendar year) [2]. Significant savings could be achieved if less costly physician assistants could be incorporated into clinical teams to provide similar care without sacrificing quality.
Nurse practitioners (NPs) and physician assistants (PAs) have been successfully employed on academic hospitalist services to complement physician staffing [3–10]. They perform admissions, consults, rounding visits and discharges with physician collaboration as permitted by each group’s policies and in accordance with hospital by-laws and state regulations. A median of 0.25 NP and 0.28 PA FTEs per physician FTE are employed by hospitalist groups that incorporate them, though staffing ratios vary widely [2].
Physicians Inpatient Care Specialists (MDICS) devel-oped a staffing model that deploys PAs to see a large proportion of its patients collaboratively with physicians, and with a higher patient census per PA than has been previously reported [2–5]. The group leaders believed that this would yield similar outcomes for patients at a lower cost to the supporting institution than a conventional staffing model which used fewer PAs to render patient care. Prior inpatient studies have demonstrated comparable clinical outcomes when comparing hospitalist PAs and NPs to residents and fellows [4–10], but to our knowledge no data exist directly comparing hospitalist PAs to hospitalist physicians. This study goes beyond prior work by examining the community, non-teaching setting, and directly comparing outcomes from the expanded use of PAs to those of a hospitalist group staffed with a greater proportion of attending physicians at the same hospital during the same time.
Methods
Setting
The study was performed at Anne Arundel Medical Center (AAMC), a 384-bed community hospital in Annapolis, Maryland, that serves a region of over 1 million people. Approximately 26,000 adult patients are discharged annually. During the study, more than 90% of internal medicine service inpatients were cared for by one of 2 hospitalist groups: a hospital-employed group (“conventional” group, Anne Arundel Medical Group) and a contracted hospitalist group (“expanded PA” group, Physicians Inpatient Care Specialists). The conventional group’s providers received a small incentive for Core Measures compliance for patients with stroke, myocardial infarction, congestive heart failure and pneumonia. The expanded PA group received a flat fee for providing hospitalist services and the group’s providers received a small incentive for productivity from their employer. The study was deemed exempt by the AAMC institutional review board.
Staffing Models, Patient Allocation, and Assignment
Admitted patients were designated to be admitted to one group or the other on the basis of standing arrangements with the patients’ primary care providers. Consultative referrals could also be made from subspecialists, who had discretion as to which group they wished to use.
Each morning, following sign-out report from the night team, each team of day providers determined which patients would be seen by which of their providers. Patients still on service from the previous day would be seen by the same provider again whenever possible in order to maintain continuity. Each individual provider had their own patients for the day who they rounded on independently and were responsible for. Physician involvement with patients seen primarily by PAs occurred as described below. Physicians in both groups were expected to take primary rounding responsibility for patients who were more acute or more complex based on morning sign-out report; there was no more formal mandate for patient allocation to particular provider type.
Physician-PA Collaboration
Patients
Patients discharged between 1 January 2012 and 30 June 2013 by the hospitalist groups were identified by searching AAMC’s Crimson Continuuum of Care (The Advisory Board, Washington, DC), a software analytic tool that is integrated with coded clinical data. Adult patient hospitalizations determined by Crimson to have a medical (non-surgical, non-obstetrical) APR-DRG code as the final principal diagnosis were included. Critically ill patients or those appropriate for “step-down unit” care were cared for by the in-house critical care staff; upon transfer out of critical or step-down care, patients were referred back to the admitting hospitalist team. A diagnosis (and its associated hospitalizations) was excluded for referral bias if the diagnosis was the principal diagnosis for at least 1% of a group’s discharges and the percentage of patients with that diagnosis was at least two times greater in one group than the other. Hospitalizations with a diagnosis of “ungroupable” (APR-DRG 956) were also excluded.
Measurements
Demographic, insurance status, cost of care, length of stay (LOS), APR-DRG (All Patient Refined Diagnosis-Related Group) severity of illness (SOI) and risk of mortality (ROM), consultant utilization, 30-day all-cause readmission (“readmission rate”), and mortality information was obtained from administrative data and exported into a single database for statistical analysis. Readmissions, inpatient mortality, and cost of care were the primary outcomes; consultant use and length of stay were secondary outcomes. A hospitalization was considered a readmission if the patient returned to inpatient status at AAMC for any reason within 30 days of a previous inpatient discharge. Inpatient mortality was defined as patient death during hospitalization. The cost of care was measured using the case charges associated with each encounter. Charge capture data from both groups was analyzed to classify visits as “physician-only,” “physician co-visit,” and “PA-only” visits. A co-visit consists of the physician visiting the patient after the PA has already done so on the same day, taking their own history and performing their own physical exam, and writing a brief progress note. These data were compared against the exported administrative data to find matching encounters and associated visits, with only matching visits included in the analysis. If a duplicate charge was entered on the same day for a patient, any conflict was resolved in favor of the physician visit. A total of 49,883 and 28,663 matching charges were identified for the conventional and expanded PA groups.
Statistical Methods
Odds of inpatient mortality were calculated using logistic regression and adjusted for age, insurance status, APR-DRG ROM, and LOS. Odds of readmission were calculated using logistic regression and adjusted for age, LOS, insurance and APR-DRG SOI. Cost of care (effect size) was examined using multiple linear regression and adjusted for age, APR-DRG SOI, insurance status and LOS. This model was fit using the logarithmic transformations of cost of care and LOS to correct deviation from normality. Robust regression using MM estimation was used to estimate group effects due to the existence of outliers and high leverage points. Length of stay (effect size) was assessed using the log-transformed variable and adjusted for APR-DRG SOI, age, insurance status and consultant use. Finally, category logistic regression models were fit to estimate the odds of consultant use in the study groups and adjusted for age, LOS, insurance status and APR-DRG SOI.
Results
Records review identified 17,294 adult patient hospitalizations determined by Crimson to have a medical (non-surgical, non-obstetrical) APR-DRG code as the final principal diagnosis. We excluded 15 expanded PA and 11 conventional hospitalizations that fell under APR-DRG code 956 “ungroupable.” Exclusion for referral bias resulted in the removal of 304 hospitalizations, 207 (3.03%) from the expanded PA group and 97 (0.92%) from the conventional group. These excluded hospitalizations came from 2 APR-DRG codes, urinary stones (code 465) and “other kidney and urinary tract diagnoses” (code 468). This left 6612 hospitalizations in the expanded PA group and 10,352 in the conventional group.
Charge capture data for both groups was used to determine the proportion of encounters rendered by each provider type or combination. In the expanded PA group, 35.73% of visits (10,241 of 28,663) were conducted by a PA, and 64.27% were conducted by a physician or by a PA with a billable physician “co-visit.” In the conventional group, 5.89% of visits (2938 of 49,883) were conducted by a PA, and 94.11% were conducted by a physician only or by a PA with a billable physician “co-visit”.
Readmissions
Overall, 929 of 6612 (14.05%) and 1417 of 10,352 (13.69%) patients were readmitted after being discharged by the expanded PA and conventional groups, respectively. After multivariate analysis, there was no statistically significant difference in odds of readmission between the groups (OR for conventional group, 0.95 [95% CI, 0.87–1.04]; P = 0.27).
Inpatient Mortality
Unadjusted inpatient mortality for the expanded PA group was 1.30% and 0.99% for the conventional group. After multivariate analysis, there was no statistically significant difference in odds of in-hospital mortality between the groups (OR for conventional group, 0.89 [95% CI, 0.66–1.19]; P = 0.42).
Patient Charges
The unadjusted mean patient charge in the expanded PA group was $7822 ± $7755 and in the conventional group mean patient charge was $8307 ± 10,034. Multivariate analysis found significantly lower adjusted patient charges in the expanded PA group relative to the conventional group (3.52% lower in the expanded PA group [95% CI, 2.66%–4.39%, P < 0.001). When comparing a “standard” patient who was between 80–89 and had Medicare insurance and an SOI of “major,” the cost of care was $2644 in the expanded PA group vs $2724 in the conventional group.
Length of Stay
Unadjusted mean length of stay was 4.1 ± 3.9 days and 4.3 ± 5.6 days for the expanded PA and conventional groups, respectively. After multivariate analysis, when comparing the statistical model “standard” patient, there was no significant difference in the length of stay between the 2 groups (effect size, 0.99 days shorter LOS in the expanded PA group [95% CI, 0.97–1.01 days]; P = 0.34)
Consultant Use
Utilization of consultants was also assessed. The expanded PA group used a mean of 0.55 consultants per case, and the conventional group used 0.56. After multivariate adjustment, there was no significant difference in consulting service use between groups (OR 1.00 [95% CI, 0.94–1.07]; P = 0.90).
Discussion
Maximizing value and minimizing health care costs is a national priority. To our knowledge, this is the first study to compare hospitalist PAs in a community, non-teaching practice directly and contemporaneously to peer PAs and attending physicians and examine the impact on outcomes. In our study, a much larger proportion of patient visits were conducted primarily by PAs without a same-day physician visit in the expanded PA group (35.73%, vs 5.89% in the conventional group). There was no statistically significant difference in inpatient mortality, length of stay or readmissions. In addition, costs of care measured as hospital charges to patients were lower in the expanded PA group. Consultants were not used disproportionately by the expanded PA group in order to achieve these results. Our results are consistent with studies that have compared PAs and NPs at academic centers to traditional housestaff teams and which show that services staffed with PAs or NPs that provide direct care to medical inpatients are non-inferior [4–10].
This study’s expanded PA group’s PAs rounded on 14 patients per day, close to the “magic 15” that is considered by many a good compromise for hospitalist physicians between productivity and quality [11,12]. This is substantially more than the 6 to 10 patients PAs have been responsible for in previously reported studies [3,4,6]. As the median salary for a PA hospitalist is $102,960 compared with the median internal medicine physician hospitalist salary of $253,977 [2], using hospitalist PAs in a collaboration model as described herein could result in significant savings for supporting institutions without sacrificing quality.
We recognize several limitations to this study. First, the data were obtained retrospectively from a single center and patient assignment between groups was nonrandomized. The significant differences in the baseline characteristics of patients between the study groups, however, were adjusted for in multivariate analysis, and potential referral bias was addressed through our exclusion criteria. Second, our comparison relied on coding rather than clinical data for diagnosis grouping. However, administrative data is commonly used to determine the primary diagnosis for study patients and the standard for reimbursement. Third, we recognize that there may have been unmeasured confounders that may have affected the outcomes. However, the same resources, including consultants and procedure services, were readily available to both groups and there was no significant difference in consultation rates. Fourth, “cost of care” was measured as overall charges to patients, not cost to the hospital. However, given that all the encounters occurred at the same hospital in the same time frame, the difference should be proportional and equal between groups. Finally, our readmission rates did not account for patients readmitted to other institutions. However, there should not have been a differential effect between the 2 study groups, given the shared patient catchment area and our exclusion for referral bias.
It should also be noted that the expanded PA group used a structured collaboration framework and incorporated a structured education program for its PAs. These components are integral to the expanded PA model, and our results may not be generalizable outside of a similar framework. The expanded PA group’s PAs were carefully selected at the time of hire, specifically educated, and supported through ongoing collaboration to provide efficient and appropriate care at the “top of their licenses”. Not all medical groups will be able to provide this level of support and education, and not all hospitalist PAs will want to and/or be able to reach this level of proficiency. However, successful implementation is entirely achievable for groups that invest the effort. The MDICS education process included 80 hours of didactic sessions spread over several months and is based on the Society of Hospital Medicine Core Competencies [13] as well as 6 months of supervised bedside education with escalating clinical responsibilities under the tutelage of an experienced physician or PA. Year-long academic PA fellowships have also been developed for purposes of similar training at several institutions [14].
Conclusion
Our results show that expanded use of well-educated PAs functioning within a formal collaboration arrangement with physicians provides similar clinical quality to a conventional PA staffing model with no excess patient care costs. The model also allows substantial salary savings to supporting institutions, which is important to hospital and policy stakeholders given the implications for hospitalist group staffing, increasing value, and allocation of precious time and financial resources.
Acknowledgements: The authors wish to thank Kevin Funk, MBA, of MDICS, Clarence Richardson, MBA, of GeBBs Software International, and Heather Channing, Kayla King, and Laura Knox of Anne Arundel Healthcare Enterprise, who provided invaluable help with the data aggregation used for this study.
Corresponding author: Timothy M. Capstack, MD, 7250 Parkway Dr, Suite 500, Hanover, MD 21076, tcapstack@mdics.com.
Financial disclosures: Dr. Capstack has ownership interest in Physicians Inpatient Care Specialists (MDICS). Ms. Segujja received compensation from MDICS for statistical analysis.
1. Michtalik HJ, Pronovost PJ, Marsteller JA, et al. Developing a model for attending physician workload and outcomes. JAMA Intern Med 2013;173:1026–8.
2. Society of Hospital Medicine. State of hospital medicine report. Philadelphia: Society of Hospital Medicine; 2014.
3. Kartha A, Restuccia J, Burgess J, et al. Nurse practitioner and physician assistant scope of practice in 118 acute care hospitals. J Hosp Med 2014;9:615–20.
4. Dhuper S, Choksi S. Replacing an academic internal medicine residency program with a physician assistant--hospitalist model: a comparative analysis study. Am J Med Qual 2008;24:132–9.
5. Morris D, Reilly P, Rohrbach J, et al. The influence of unit-based nurse practitioners on hospital outcomes and readmission rates for patients with trauma. J Trauma Acute Care Surg 2012;73:474–8.
6. Roy C, Liang C, Lund M, et al. Implementation of a physician assistant/hospitalist service in an academic medical center: impact on efficiency and patient outcomes. J Hosp Med 2008;3:361–8.
7. Singh S, Fletcher K, Schapira M, et al. A comparison of outcomes of general medical inpatient care provided by a hospitalist-physician assistant model vs a traditional resident-based model. J Hosp Med 2011;6:122–30.
8. Hoffman L, Tasota F, Zullo T, et al. Outcomes of care managed by an acute care nurse practitioner/attending physician team in an subacute medical intensive care unit. Am J Crit Care 2005;14:121–30.
9. Kapu A, Kleinpell R, Pilon B. Quality and financial impact of adding nurse practitioners to inpatient care teams. J Nurs Adm 2014;44:87–96.
10. Cowan M, Shapiro M, Hays R, et al. The effect of a multidisciplinary hospitalist/physician and advanced practice nurse collaboration on hospital costs. J Nurs Adm 2006;36:79–85.
11. Michtalik HJ, Yeh HC, Pronovost PJ, Brotman DJ. Impact of attending physician workload on patient care: A survey of hospitalists. JAMA Intern Med 2013;173:375–7.
12. Elliott D, Young R, Brice J, et al. Effect of hospitalist workload on the quality and efficiency of care. JAMA Internal Med 2014;174:786–93.
13. McKean S, Budnitz T, Dressler D, et al. How to use the core competencies in hospital medicine: a framework for curriculum development. J Hosp Med 2006; 1 Suppl 1:57–67.
14. Will K, Budavari A, Wilkens J, et al. A hospitalist postgraduate training program for physician assistants. J Hosp Med 2010;5:94–8.
1. Michtalik HJ, Pronovost PJ, Marsteller JA, et al. Developing a model for attending physician workload and outcomes. JAMA Intern Med 2013;173:1026–8.
2. Society of Hospital Medicine. State of hospital medicine report. Philadelphia: Society of Hospital Medicine; 2014.
3. Kartha A, Restuccia J, Burgess J, et al. Nurse practitioner and physician assistant scope of practice in 118 acute care hospitals. J Hosp Med 2014;9:615–20.
4. Dhuper S, Choksi S. Replacing an academic internal medicine residency program with a physician assistant--hospitalist model: a comparative analysis study. Am J Med Qual 2008;24:132–9.
5. Morris D, Reilly P, Rohrbach J, et al. The influence of unit-based nurse practitioners on hospital outcomes and readmission rates for patients with trauma. J Trauma Acute Care Surg 2012;73:474–8.
6. Roy C, Liang C, Lund M, et al. Implementation of a physician assistant/hospitalist service in an academic medical center: impact on efficiency and patient outcomes. J Hosp Med 2008;3:361–8.
7. Singh S, Fletcher K, Schapira M, et al. A comparison of outcomes of general medical inpatient care provided by a hospitalist-physician assistant model vs a traditional resident-based model. J Hosp Med 2011;6:122–30.
8. Hoffman L, Tasota F, Zullo T, et al. Outcomes of care managed by an acute care nurse practitioner/attending physician team in an subacute medical intensive care unit. Am J Crit Care 2005;14:121–30.
9. Kapu A, Kleinpell R, Pilon B. Quality and financial impact of adding nurse practitioners to inpatient care teams. J Nurs Adm 2014;44:87–96.
10. Cowan M, Shapiro M, Hays R, et al. The effect of a multidisciplinary hospitalist/physician and advanced practice nurse collaboration on hospital costs. J Nurs Adm 2006;36:79–85.
11. Michtalik HJ, Yeh HC, Pronovost PJ, Brotman DJ. Impact of attending physician workload on patient care: A survey of hospitalists. JAMA Intern Med 2013;173:375–7.
12. Elliott D, Young R, Brice J, et al. Effect of hospitalist workload on the quality and efficiency of care. JAMA Internal Med 2014;174:786–93.
13. McKean S, Budnitz T, Dressler D, et al. How to use the core competencies in hospital medicine: a framework for curriculum development. J Hosp Med 2006; 1 Suppl 1:57–67.
14. Will K, Budavari A, Wilkens J, et al. A hospitalist postgraduate training program for physician assistants. J Hosp Med 2010;5:94–8.
Dashboards and P4P in VTE Prophylaxis
The Affordable Care Act explicitly outlines improving the value of healthcare by increasing quality and decreasing costs. It emphasizes value‐based purchasing, the transparency of performance metrics, and the use of payment incentives to reward quality.[1, 2] Venous thromboembolism (VTE) prophylaxis is one of these publicly reported performance measures. The National Quality Forum recommends that each patient be evaluated on hospital admission and during their hospitalization for VTE risk level and for appropriate thromboprophylaxis to be used, if required.[3] Similarly, the Joint Commission includes appropriate VTE prophylaxis in its Core Measures.[4] Patient experience and performance metrics, including VTE prophylaxis, constitute the hospital value‐based purchasing (VBP) component of healthcare reform.[5] For a hypothetical 327‐bed hospital, an estimated $1.7 million of a hospital's inpatient payments from Medicare will be at risk from VBP alone.[2]
VTE prophylaxis is a common target of quality improvement projects. Effective, safe, and cost‐effective measures to prevent VTE exist, including pharmacologic and mechanical prophylaxis.[6, 7] Despite these measures, compliance rates are often below 50%.[8] Different interventions have been pursued to ensure appropriate VTE prophylaxis, including computerized provider order entry (CPOE), electronic alerts, mandatory VTE risk assessment and prophylaxis, and provider education campaigns.[9] Recent studies show that CPOE systems with mandatory fields can increase VTE prophylaxis rates to above 80%, yet the goal of a high reliability health system is for 100% of patients to receive recommended therapy.[10, 11, 12, 13, 14, 15] Interventions to improve prophylaxis rates that have included multiple strategies, such as computerized order sets, feedback, and education, have been the most effective, increasing compliance to above 90%.[9, 11, 16] These systems can be enhanced with additional interventions such as providing individualized provider education and feedback, understanding of work flow, and ensuring patients receive the prescribed therapies.[12] For example, a physician dashboard could be employed to provide a snapshot and historical trend of key performance indicators using graphical displays and indicators.[17]
Dashboards and pay‐for‐performance programs have been increasingly used to increase the visibility of these metrics, provide feedback, visually display benchmarks and goals, and proactively monitor for achievements and setbacks.[18] Although these strategies are often addressed at departmental (or greater) levels, applying them at the level of the individual provider may assist hospitals in reducing preventable harm and achieving safety and quality goals, especially at higher benchmarks. With their expanding role, hospitalists provide a key opportunity to lead improvement efforts and to study the impact of dashboards and pay‐for performance at the provider level to achieve VTE prophylaxis performance targets. Hospitalists are often the front‐line provider for inpatients and deliver up to 70% of inpatient general medical services.[19] The objective of our study was to evaluate the impact of providing individual provider feedback and employing a pay‐for‐performance program on baseline performance of VTE prophylaxis among hospitalists. We hypothesized that performance feedback through the use of a dashboard would increase appropriate VTE prophylaxis, and this effect would be further augmented by incorporation of a pay‐for‐performance program.
METHODS
Hospitalist Dashboard
In 2010, hospitalist program leaders met with hospital administrators to create a hospitalist dashboard that would provide regularly updated summaries of performance measures for individual hospitalists. The final set of metrics identified included appropriate VTE prophylaxis, length of stay, patients discharged per day, discharges before 3 pm, depth of coding, patient satisfaction, readmissions, communication with the primary care provider, and time to signature for discharge summaries (Figure 1A). The dashboard was introduced at a general hospitalist meeting during which its purpose, methodology, and accessibility were described; it was subsequently implemented in January 2011.
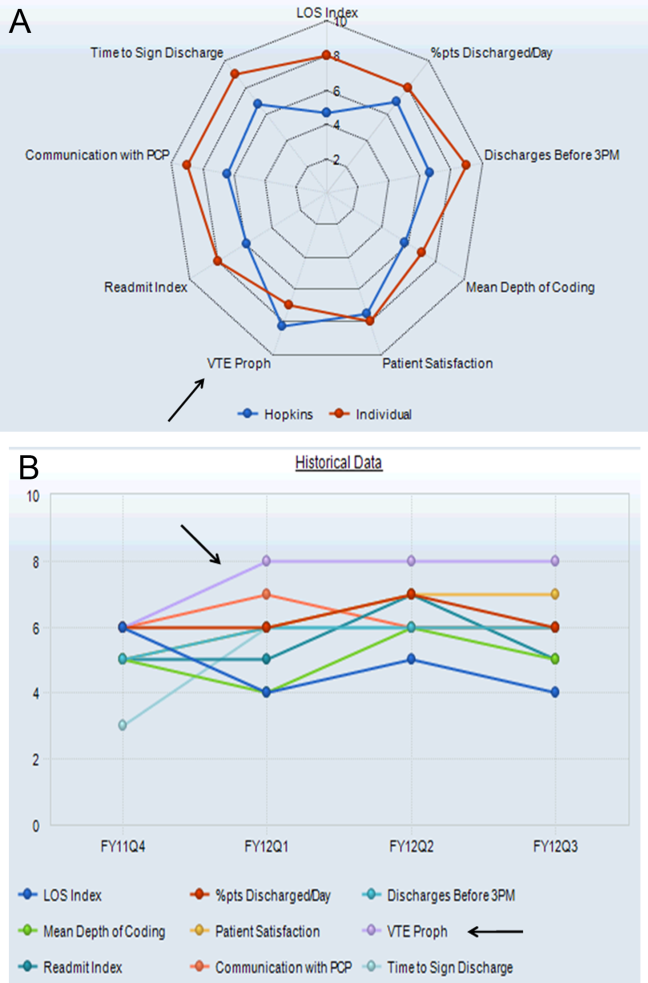
Benchmarks were established for each metric, standardized to establish a scale ranging from 1 through 9, and incorporated into the dashboard (Figure 1A). Higher scores (creating a larger geometric shape) were desirable. For the VTE prophylaxis measure, scores of 1 through 9 corresponded to <60%, 60% to 64.9%, 65% to 69.9%, 70% to 74.9%, 75% to 79.9%, 80% to 84.9%, 85% to 89.9%, 90% to 94.9%, and 95% American College of Chest Physicians (ACCP)‐compliant VTE prophylaxis, respectively.[12, 20] Each provider was able to access the aggregated dashboard (showing the group mean) and his/her individualized dashboard using an individualized login and password for the institutional portal. This portal is used during the provider's workflow, including medical record review and order entry. Both a polygonal summary graphic (Figure 1A) and trend (Figure 1B) view of the dashboard were available to the provider. A comparison of the individual provider to the hospitalist group average was displayed (Figure 1A). At monthly program meetings, the dashboard, group results, and trends were discussed.
Venous Thromboembolism Prophylaxis Compliance
Our study was performed in a tertiary academic medical center with an approximately 20‐member hospitalist group (the precise membership varied over time), whose responsibilities include, among other clinical duties, staffing a 17‐bed general medicine unit with telemetry. The scope of diagnoses and acuity of patients admitted to the hospitalist service is similar to the housestaff services. Some hospitalist faculty serve both as hospitalist and nonhospitalist general medicine service team attendings, but the comparison groups were staffed by hospitalists for <20% of the time. For admissions, all hospitalists use a standardized general medicine admission order set that is integrated into the CPOE system (Sunrise Clinical Manager; Allscripts, Chicago, IL) and completed for all admitted patients. A mandatory VTE risk screen, which includes an assessment of VTE risk factors and pharmacological prophylaxis contraindications, must be completed by the ordering physician as part of this order set (Figure 2A). The system then prompts the provider with a risk‐appropriate VTE prophylaxis recommendation that the provider may subsequently order, including mechanical prophylaxis (Figure 2B). Based on ACCP VTE prevention guidelines, risk‐appropriate prophylaxis was determined using an electronic algorithm that categorized patients into risk categories based on the presence of major VTE risk factors (Figure 2A).[12, 15, 20] If none of these were present, the provider selected No major risk factors known. Both an assessment of current use of anticoagulation and a clinically high risk of bleeding were also included (Figure 2A). If none of these were present, the provider selected No contraindications known. This algorithm is published in detail elsewhere and has been shown to not increase major bleeding episodes.[12, 15] The VTE risk assessment, but not the VTE order itself, was a mandatory field. This allowed the physician discretion to choose among various pharmacological agents and mechanical mechanisms based on patient and physician preferences.
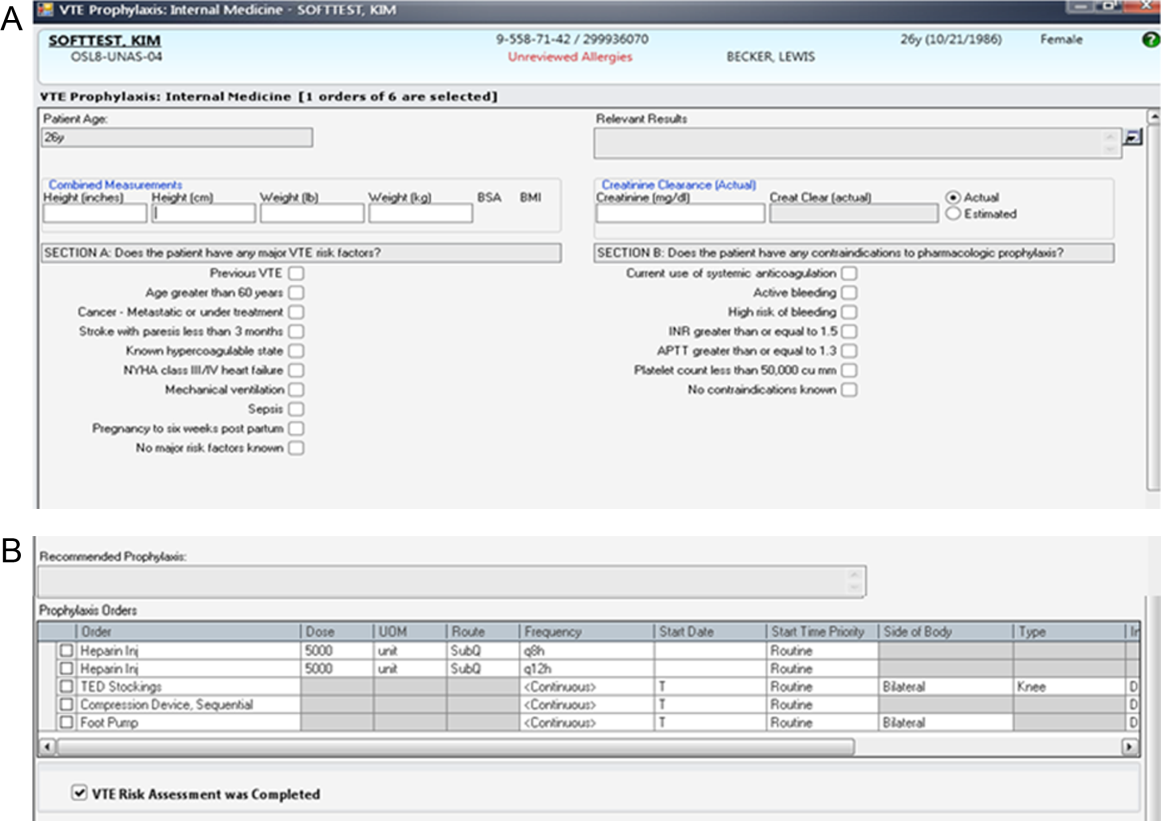
Compliance of risk‐appropriate VTE prophylaxis was determined 24 hours after the admission order set was completed using an automated electronic query of the CPOE system. Low molecular‐weight heparin prescription was included in the compliance algorithm as acceptable prophylaxis. Prescription of pharmacological VTE prophylaxis when a contraindication was present was considered noncompliant. The metric was assigned to the attending physician who billed for the first inpatient encounter.
Pay‐for‐Performance Program
In July 2011, a pay‐for‐performance program was added to the dashboard. All full‐time and part‐time hospitalists were eligible. The financial incentive was determined according to hospital priority and funds available. The VTE prophylaxis metric was prorated by clinical effort, with a maximum of $0.50 per work relative value unit (RVU). To optimize performance, a threshold of 80% compliance had to be surpassed before any payment was made. Progressively increasing percentages of the incentive were earned as compliance increased from 80% to 100%, corresponding to dashboard scores of 6, 7, 8, and 9: <80% (scores 1 to 5)=no payment; 80% to 84.9% (score 6)=$0.125 per RVU; 85% to 89.9% (score 7)=$0.25 per RVU; 90% to 94.9% (score 8)=$0.375 per RVU; and 95% (score 9)=$0.50 per RVU (maximum incentive). Payments were accrued quarterly and paid at the end of the fiscal year as a cumulative, separate performance supplement.
Individualized physician feedback through the dashboard was continued during the pay‐for‐performance period. Average hospitalist group compliance continued to be displayed on the electronic dashboard and was explicitly reviewed at monthly hospitalist meetings.
The VTE prophylaxis order set and data collection and analyses were approved by the Johns Hopkins Medicine Institutional Review Board. The dashboard and pay‐for‐performance program were initiated by the institution as part of a proof of concept quality improvement project.
Analysis
We examined all inpatient admissions to the hospitalist unit from 2008 to 2012. We included patients admitted to and discharged from the hospitalist unit and excluded patients transferred into/out of the unit and encounters with a length of stay <24 hours. VTE prophylaxis orders were queried from the CPOE system 24 hours after the patient was admitted to determine compliance.
After allowing for a run‐in period (2008), we analyzed the change in percent compliance for 3 periods: (1) CPOE‐based VTE order set alone (baseline [BASE], January 2009 to December 2010); (2) group and individual physician feedback using the dashboard (dashboard only [DASH], January to June 2011); and (3) dashboard tied to the pay‐for‐performance program (dashboard with pay‐for‐performance [P4P], July 2011 to December 2012). The CPOE‐based VTE order set was used during all 3 periods. We used the other medical services as a control to ensure that there were no temporal trends toward improved prophylaxis on a service without the intervention. VTE prophylaxis compliance was examined by calculating percent compliance using the same algorithm for the 4 resident‐staffed general medicine service teams at our institution, which utilized the same CPOE system but did not receive the dashboard or pay‐for‐performance interventions. We used locally weighted scatterplot smoothing, a locally weighted regression of percent compliance over time, to graphically display changes in group compliance over time.[21, 22]
We also performed linear regression to assess the rate of change in group compliance and included spline terms that allowed slope to vary for each of the 3 time periods.[23, 24] Clustered analysis accounted for potentially correlated serial measurements of compliance for an individual provider. A separate analysis examined the effect of provider turnover and individual provider improvement during each of the 3 periods. Tests of significance were 2‐sided, with an level of 0.05. Statistical analysis was performed using Stata 12.1 (StataCorp LP, College Station, TX).
RESULTS
Venous Thromboembolism Prophylaxis Compliance
We analyzed 3144 inpatient admissions by 38 hospitalists from 2009 to 2012. The 5 most frequent coded diagnoses were heart failure, acute kidney failure, syncope, pneumonia, and chest pain. Patients had a median length of stay of 3 days [interquartile range: 26]. During the dashboard‐only period, on average, providers improved in compliance by 4% (95% confidence interval [CI]: 35; P<0.001). With the addition of the pay‐for‐performance program, providers improved by an additional 4% (95% CI: 35; P<0.001). Group compliance significantly improved from 86% (95% CI: 8588) during the BASE period of the CPOE‐based VTE order set to 90% (95% CI: 8893) during the DASH period (P=0.01) and 94% (95% CI: 9396) during the subsequent P4P program (P=0.01) (Figure 3). Both inappropriate prophylaxis and lack of prophylaxis, when indicated, resulted in a non‐compliance rating. During the 3 periods, inappropriate prophylaxis decreased from 7.9% to 6.2% to 2.6% during the BASE, DASH, and subsequent P4P periods, respectively. Similarly, lack of prophylaxis when indicated decreased from 6.1% to 3.2% to 3.1% during the BASE, DASH, and subsequent P4P periods, respectively.
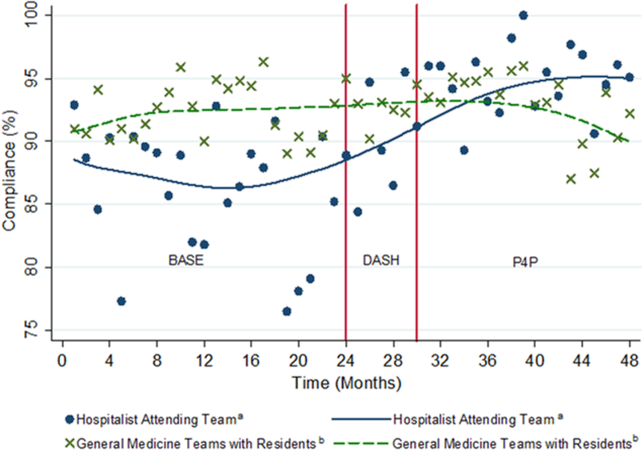
The average compliance of the 4 non‐hospitalist general medicine service teams was initially higher than that of the hospitalist service during the CPOE‐based VTE order set (90%) and DASH (92%) periods, but subsequently plateaued and was exceeded by the hospitalist service during the combined P4P (92%) period (Figure 3). However, there was no statistically significant difference between the general medicine service teams and hospitalist service during the DASH (P=0.15) and subsequent P4P (P=0.76) periods.
We also analyzed the rate of VTE prophylaxis compliance improvement (slope) with cut points at each time period transition (Figure 3). Risk‐appropriate VTE prophylaxis during the BASE period did not exhibit significant improvement as indicated by the slope (P=0.23) (Figure 3). In contrast, during the DASH period, VTE prophylaxis compliance significantly increased by 1.58% per month (95% CI: 0.41‐2.76; P=0.01). The addition of the P4P program, however, did not further significantly increase the rate of compliance (P=0.78).
A subgroup analysis restricted to the 19 providers present during all 3 periods was performed to assess for potential confounding from physician turnover. The percent compliance increased in a similar fashion: BASE period of CPOE‐based VTE order set, 85% (95% CI: 8386); DASH, 90% (95% CI: 8893); and P4P, 94% (95% CI: 9296).
Pay‐for‐Performance Program
Nineteen providers met the threshold for pay‐for‐performance (80% appropriate VTE prophylaxis), with 9 providers in the intermediate categories (80%94.9%) and 10 in the full incentive category (95%). The mean individual payout for the incentive was $633 (standard deviation 350), with a total disbursement of $12,029. The majority of payments (17 of 19) were under $1000.
DISCUSSION
A key component of healthcare reform has been value‐based purchasing, which emphasizes extrinsic motivation through the transparency of performance metrics and use of payment incentives to reward quality. Our study evaluates the impact of both extrinsic (payments) and intrinsic (professionalism and peer norms) motivation. It specifically attributed an individual performance metric, VTE prophylaxis, to an attending physician, provided both individualized and group feedback using an electronic dashboard, and incorporated a pay‐for‐performance program. Prescription of risk‐appropriate VTE prophylaxis significantly increased with the implementation of the dashboard and subsequent pay‐for performance program. The fastest rate of improvement occurred after the addition of the dashboard. Sensitivity analyses for provider turnover and comparisons to the general medicine services showed our results to be independent of a general trend of improvement, both at the provider and institutional levels.
Our prior studies demonstrated that order sets significantly improve performance, from a baseline compliance of risk‐appropriate VTE prophylaxis of 66% to 84%.[13, 15, 25] In the current study, compliance was relatively flat during the BASE period, which included these order sets. The greatest rate of continued improvement in compliance occurred during the DASH period, emphasizing both the importance of provider feedback and receptivity and adaptability in the prescribing behavior of hospitalists. Because the goal of a high‐reliability health system is for 100% of patients to receive recommended therapy, multiple approaches are necessary for success.
Nationally, benchmarks for performance measures continue to be raised, with the highest performers achieving above 95%.[26] Additional interventions, such as dashboards and pay‐for‐performance programs, supplement CPOE systems to achieve high reliability. In our study, the compliance rate during the baseline period, which included a CPOE‐based, clinical support‐enabled VTE order set, was 86%. Initially the compliance of the general medicine teams with residents exceeded that of the hospitalist attending teams, which may reflect a greater willingness of resident teams to comply with order sets and automated recommendations. This emphasizes the importance of continuous individual feedback and provider education at the attending physician level to enhance both guideline compliance and decrease provider care variation. Ultimately, with the addition of the dashboard and subsequent pay‐for‐performance program, compliance was increased to 90% and 94%, respectively. Although the major mechanism used by policymakers to improve quality of care is extrinsic motivation, this study demonstrates that intrinsic motivation through peer norms can enhance extrinsic efforts and may be more influential. Both of these programs, dashboards and pay‐for‐performance, may ultimately assist institutions in changing provider behavior and achieving these harder‐to‐achieve higher benchmarks.
We recognize that there are several limitations to our study. First, this is a single‐site program limited to an attending‐physician‐only service. There was strong data support and a defined CPOE algorithm for this initiative. Multi‐site studies will need to overcome the additional challenges of varying service structures and electronic medical record and provider order entry systems. Second, it is difficult to show actual changes in VTE events over time with appropriate prophylaxis. Although VTE prophylaxis is recommended for patients with VTE risk factors, there are conflicting findings about whether prophylaxis prevents VTE events in lower‐risk patients, and current studies suggest that most patients with VTE events are severely ill and develop VTE despite receiving prophylaxis.[27, 28, 29] Our study was underpowered to detect these potential differences in VTE rates, and although the algorithm has been shown to not increase bleeding rates, we did not measure bleeding rates during this study.[12, 15] Our institutional experience suggests that the majority of VTE events occur despite appropriate prophylaxis.[30] Also, VTE prophylaxis may be ordered, but intervening events, such as procedures and changes in risk status or patient refusal, may prevent patients from receiving appropriate prophylaxis.[31, 32] Similarly, hospitals with higher quality scores have higher VTE prophylaxis rates but worse risk‐adjusted VTE rates, which may result from increased surveillance for VTE, suggesting surveillance bias limits the usefulness of the VTE quality measure.[33, 34] Nevertheless, VTE prophylaxis remains a publicly reported Core Measure tied to financial incentives.[4, 5] Third, there may be an unmeasured factor specific to the hospitalist program, which could potentially account for an overall improvement in quality of care. Although the rate of increase in appropriate prophylaxis was not statistically significant during the baseline period, there did appear to be some improvement in prophylaxis toward the end of the period. However, there were no other VTE‐related provider feedback programs being simultaneously pursued during this study. VTE prophylaxis for the non‐hospitalist services showed a relatively stable, non‐increasing compliance rate for the general medical services. Although it was possible for successful residents to age into the hospitalist service, thereby improving rates of prophylaxis based on changes in group makeup, our subgroup analysis of the providers present throughout all phases of the study showed our results to be robust. Similarly, there may have been a cross‐contamination effect of hospitalist faculty who attended on both hospitalist and non‐hospitalist general medicine service teams. This, however, would attenuate any impact of the programs, and thus the effects may in fact be greater than reported. Fourth, establishment of both the dashboard and pay‐for‐performance program required significant institutional and program leadership and resources. To be successful, the dashboard must be in the provider's workflow, transparent, minimize reporter burden, use existing systems, and be actively fed back to providers, ideally those directly entering orders. Our greatest rate of improvement occurred during the feedback‐only phase of this study, emphasizing the importance of physician feedback, provider‐level accountability, and engagement. We suspect that the relatively modest pay‐for‐performance incentive served mainly as a means of engaging providers in self‐monitoring, rather than as a means to change behavior through true incentivization. Although we did not track individual physician views of the dashboard, we reinforced trends, deviations, and expectations at regularly scheduled meetings and provided feedback and patient‐level data to individual providers. Fifth, the design of the pay‐for‐performance program may have also influenced its effectiveness. These types of programs may be more effective when they provide frequent visible, small payments rather than one large payment, and when the payment is framed as a loss rather than a gain.[35] Finally, physician champions and consistent feedback through departmental meetings or visual displays may be required for program success. The initial resources to create the dashboard, continued maintenance and monitoring of performance, and payment of financial incentives all require institutional commitment. A partnership of physicians, program leaders, and institutional administrators is necessary for both initial and continued success.
To achieve performance goals and benchmarks, multiple strategies that combine extrinsic and intrinsic motivation are necessary. As shown by our study, the use of a dashboard and pay‐for‐performance can be tailored to an institution's goals, in line with national standards. The specific goal (risk‐appropriate VTE prophylaxis) and benchmarks (80%, 85%, 90%, 95%) can be individualized to a particular institution. For example, if readmission rates are above target, readmissions could be added as a dashboard metric. The specific benchmark would be determined by historical trends and administrative targets. Similarly, the overall financial incentives could be adjusted based on the financial resources available. Other process measures, such as influenza vaccination screening and administration, could also be targeted. For all of these objectives, continued provider feedback and engagement are critical for progressive success, especially to decrease variability in care at the attending physician level. Incorporating the value‐based purchasing philosophy from the Affordable Care Act, our study suggests that the combination of standardized order sets, real‐time dashboards, and physician‐level incentives may assist hospitals in achieving quality and safety benchmarks, especially at higher targets.
Acknowledgements
The authors thank Meir Gottlieb, BS, from Salar Inc. for data support; Murali Padmanaban, BS, from Johns Hopkins University for his assistance in linking the administrative billing data with real‐time physician orders; and Hsin‐Chieh Yeh, PhD, from the Bloomberg School of Public Health for her statistical advice and additional review. We also thank Mr. Ronald R. Peterson, President, Johns Hopkins Health System and Johns Hopkins Hospital, for providing funding support for the physician incentive payments.
Disclosures: Drs. Michtalik and Brotman had full access to all of the data in the study and take responsibility for the integrity of the data and accuracy of the data analysis. Study concept and design: Drs. Michtalik, Streiff, Finkelstein, Pronovost, and Brotman. Acquisition of data: Drs. Michtalik, Streiff, Brotman and Mr. Carolan, Mr. Lau, Mrs. Durkin. Analysis and interpretation of data: Drs. Michtalik, Haut, Streiff, Brotman and Mr. Carolan, Mr. Lau. Drafting of the manuscript: Drs. Michtalik and Brotman. Critical revision of the manuscript for important intellectual content: Drs. Michtalik, Haut, Streiff, Finkelstein, Pronovost, Brotman and Mr. Carolan, Mr. Lau, Mrs. Durkin. Statistical analysis and supervision: Drs. Michtalik and Brotman. Obtaining funding: Drs. Streiff and Brotman. Technical support: Dr. Streiff and Mr. Carolan, Mr. Lau, Mrs. Durkin
This study was supported by a National Institutes of Health grant T32 HP10025‐17‐00 (Dr. Michtalik), the National Institutes of Health/Johns Hopkins Institute for Clinical and Translational Research KL2 Award 5KL2RR025006 (Dr. Michtalik), the Agency for Healthcare Research and Quality Mentored Clinical Scientist Development K08 Awards 1K08HS017952‐01 (Dr. Haut) and 1K08HS022331‐01A1 (Dr. Michtalik), and the Johns Hopkins Hospitalist Scholars Fund. The funders had no role in the design and conduct of the study; collection, management, analysis, and interpretation of the data; preparation, review, or approval of the manuscript; and decision to submit the manuscript for publication.
Dr. Haut receives royalties from Lippincott, Williams & Wilkins. Dr. Streiff has received research funding from Portola and Bristol Myers Squibb, honoraria for CME lectures from Sanofi‐Aventis and Ortho‐McNeil, consulted for Eisai, Daiichi‐Sankyo, Boerhinger‐Ingelheim, Janssen Healthcare, and Pfizer. Mr. Lau, Drs. Haut, Streiff, and Pronovost are supported by a contract from the Patient‐Centered Outcomes Research Institute (PCORI) titled Preventing Venous Thromboembolism: Empowering Patients and Enabling Patient‐Centered Care via Health Information Technology (CE‐12‐11‐4489). Dr. Brotman has received research support from Siemens Healthcare Diagnostics, Bristol‐Myers Squibb, the Agency for Healthcare Research and Quality, Centers for Medicare & Medicaid Services, the Amerigroup Corporation, and the Guerrieri Family Foundation. He has received honoraria from the Gerson Lehrman Group, the Dunn Group, and from Quantia Communications, and received royalties from McGraw‐Hill.
- Medicare Program, Centers for Medicare 76(88):26490–26547.
- Quality meets finance: payments at risk with value‐based purchasing, readmission, and hospital‐acquired conditions force hospitalists to focus. Hospitalist. 2013;17(1):31. .
- National Quality Forum. March 2009. Safe practices for better healthcare—2009 update. Available at: http://www.qualityforum.org/Publications/2009/03/Safe_Practices_for_Better_Healthcare%E2%80%932009_Update.aspx. Accessed November 1, 2014.
- Joint Commission on Accreditation of Healthcare Organizations. Approved: more options for hospital core measures. Jt Comm Perspect. 2009;29(4):1–6.
- Centers for Medicare 208(2):227–240.
- Thromboprophylaxis in nonsurgical patients. Hematology Am Soc Hematol Educ Program. 2012;2012:631–637. , .
- Venous thromboembolism risk and prophylaxis in the acute hospital care setting (ENDORSE study): a multinational cross‐sectional study. Lancet. 2008;371(9610):387–394. , , , et al.
- Practices to prevent venous thromboembolism: a brief review. BMJ Qual Saf. 2014;23(3):187–195. , .
- Improving hospital venous thromboembolism prophylaxis with electronic decision support. J Hosp Med. 2013;8(3):115–120. , , , et al.
- Innovative approaches to increase deep vein thrombosis prophylaxis rate resulting in a decrease in hospital‐acquired deep vein thrombosis at a tertiary‐care teaching hospital. J Hosp Med. 2008;3(2):148–155. , , .
- Lessons from the Johns Hopkins Multi‐Disciplinary Venous Thromboembolism (VTE) Prevention Collaborative. BMJ. 2012;344:e3935. , , , et al.
- Improved prophylaxis and decreased rates of preventable harm with the use of a mandatory computerized clinical decision support tool for prophylaxis for venous thromboembolism in trauma. Arch Surg. 2012;147(10):901–907. , , , et al.
- Designing and implementing effective venous thromboembolism prevention protocols: lessons from collaborative efforts. J Thromb Thrombolysis. 2010;29(2):159–166. , .
- Impact of a venous thromboembolism prophylaxis "smart order set": improved compliance, fewer events. Am J Hematol. 2013;88(7):545–549. , , , et al.
- Improving adherence to venous thromoembolism prophylaxis using multiple interventions. BMJ. 2012;344:e3935. , .
- Health Resources and Services Administration of the U.S. Department of Health and Human Services. Managing data for performance improvement. Available at: http://www.hrsa.gov/quality/toolbox/methodology/performanceimprovement/part2.html. Accessed December 18, 2014.
- Improving patient safety by taking systems seriously. JAMA. 2008;299(4):445–447. , .
- Growth in the care of older patients by hospitalists in the United States. N Engl J Med. 2009;360(11):1102–1112. , , , .
- Prevention of venous thromboembolism: American College of Chest Physicians evidence‐based clinical practice guidelines (8th edition). Chest. 2008;133(6 suppl):381S–453S. , , , et al.
- Robust locally weighted regression and smoothing scatterplots. J Am Stat Assoc. 1979;74(368):829–836. .
- Locally weighted regression: An approach to regression analysis by local fitting. J Am Stat Assoc. 1988;83(403):596–610. , .
- Regression Methods in Biostatistics: Linear, Logistic, Survival, and Repeated Measures Models. 2nd ed. New York, NY: Springer; 2012. , , , .
- Regression Modeling Strategies: With Applications to Linear Models, Logistic Regression, and Survival Analysis. New York, NY: Springer‐Verlag; 2001. .
- Med Care. doi: 10.1097/MLR.0000000000000251. , , , et al. Eliminating healthcare disparities via mandatory clinical decision support: the venous thromboembolism (VTE) example [published online ahead of print November 4, 2014].
- Joint Commission. Improving America's hospitals: the Joint Commission's annual report on quality and safety. 2012. Available at: http://www.jointcommission.org/assets/1/18/TJC_Annual_Report_2012.pdf. Accessed September 8, 2013.
- Hospital performance for pharmacologic venous thromboembolism prophylaxis and rate of venous thromboembolism: a cohort study. JAMA Intern Med. 2014;174(10):1577–1584. , , , et al.
- Incidence of hospital‐acquired venous thromboembolic codes in medical patients hospitalized in academic medical centers. J Hosp Med. 2014;9(4):221–225. , , , et al.
- No association between hospital‐reported perioperative venous thromboembolism prophylaxis and outcome rates in publicly reported data. JAMA Surg. 2014;149(4):400–401. , , , , .
- Linking processes and outcomes: a key strategy to prevent and report harm from venous thromboembolism in surgical patients. JAMA Surg. 2013;148(3):299–300. , , , , .
- Patterns of non‐administration of ordered doses of venous thromboembolism prophylaxis: implications for novel intervention strategies. PLoS One. 2013;8(6):e66311. , , , et al.
- Nonadministration of thromboprophylaxis in hospitalized patients with HIV: a missed opportunity for prevention? J Hosp Med. 2014;9(4):215–220. , , , et al.
- Evaluation of surveillance bias and the validity of the venous thromboembolism quality measure. JAMA. 2013;310(14):1482–1489. , , , et al.
- Surveillance bias in outcomes reporting. JAMA. 2011;305(23):2462–2463. , .
- Pay for performance in health care: an international overview of initiatives. Med Care Res Rev. 2012;69(3):251–276. .
The Affordable Care Act explicitly outlines improving the value of healthcare by increasing quality and decreasing costs. It emphasizes value‐based purchasing, the transparency of performance metrics, and the use of payment incentives to reward quality.[1, 2] Venous thromboembolism (VTE) prophylaxis is one of these publicly reported performance measures. The National Quality Forum recommends that each patient be evaluated on hospital admission and during their hospitalization for VTE risk level and for appropriate thromboprophylaxis to be used, if required.[3] Similarly, the Joint Commission includes appropriate VTE prophylaxis in its Core Measures.[4] Patient experience and performance metrics, including VTE prophylaxis, constitute the hospital value‐based purchasing (VBP) component of healthcare reform.[5] For a hypothetical 327‐bed hospital, an estimated $1.7 million of a hospital's inpatient payments from Medicare will be at risk from VBP alone.[2]
VTE prophylaxis is a common target of quality improvement projects. Effective, safe, and cost‐effective measures to prevent VTE exist, including pharmacologic and mechanical prophylaxis.[6, 7] Despite these measures, compliance rates are often below 50%.[8] Different interventions have been pursued to ensure appropriate VTE prophylaxis, including computerized provider order entry (CPOE), electronic alerts, mandatory VTE risk assessment and prophylaxis, and provider education campaigns.[9] Recent studies show that CPOE systems with mandatory fields can increase VTE prophylaxis rates to above 80%, yet the goal of a high reliability health system is for 100% of patients to receive recommended therapy.[10, 11, 12, 13, 14, 15] Interventions to improve prophylaxis rates that have included multiple strategies, such as computerized order sets, feedback, and education, have been the most effective, increasing compliance to above 90%.[9, 11, 16] These systems can be enhanced with additional interventions such as providing individualized provider education and feedback, understanding of work flow, and ensuring patients receive the prescribed therapies.[12] For example, a physician dashboard could be employed to provide a snapshot and historical trend of key performance indicators using graphical displays and indicators.[17]
Dashboards and pay‐for‐performance programs have been increasingly used to increase the visibility of these metrics, provide feedback, visually display benchmarks and goals, and proactively monitor for achievements and setbacks.[18] Although these strategies are often addressed at departmental (or greater) levels, applying them at the level of the individual provider may assist hospitals in reducing preventable harm and achieving safety and quality goals, especially at higher benchmarks. With their expanding role, hospitalists provide a key opportunity to lead improvement efforts and to study the impact of dashboards and pay‐for performance at the provider level to achieve VTE prophylaxis performance targets. Hospitalists are often the front‐line provider for inpatients and deliver up to 70% of inpatient general medical services.[19] The objective of our study was to evaluate the impact of providing individual provider feedback and employing a pay‐for‐performance program on baseline performance of VTE prophylaxis among hospitalists. We hypothesized that performance feedback through the use of a dashboard would increase appropriate VTE prophylaxis, and this effect would be further augmented by incorporation of a pay‐for‐performance program.
METHODS
Hospitalist Dashboard
In 2010, hospitalist program leaders met with hospital administrators to create a hospitalist dashboard that would provide regularly updated summaries of performance measures for individual hospitalists. The final set of metrics identified included appropriate VTE prophylaxis, length of stay, patients discharged per day, discharges before 3 pm, depth of coding, patient satisfaction, readmissions, communication with the primary care provider, and time to signature for discharge summaries (Figure 1A). The dashboard was introduced at a general hospitalist meeting during which its purpose, methodology, and accessibility were described; it was subsequently implemented in January 2011.
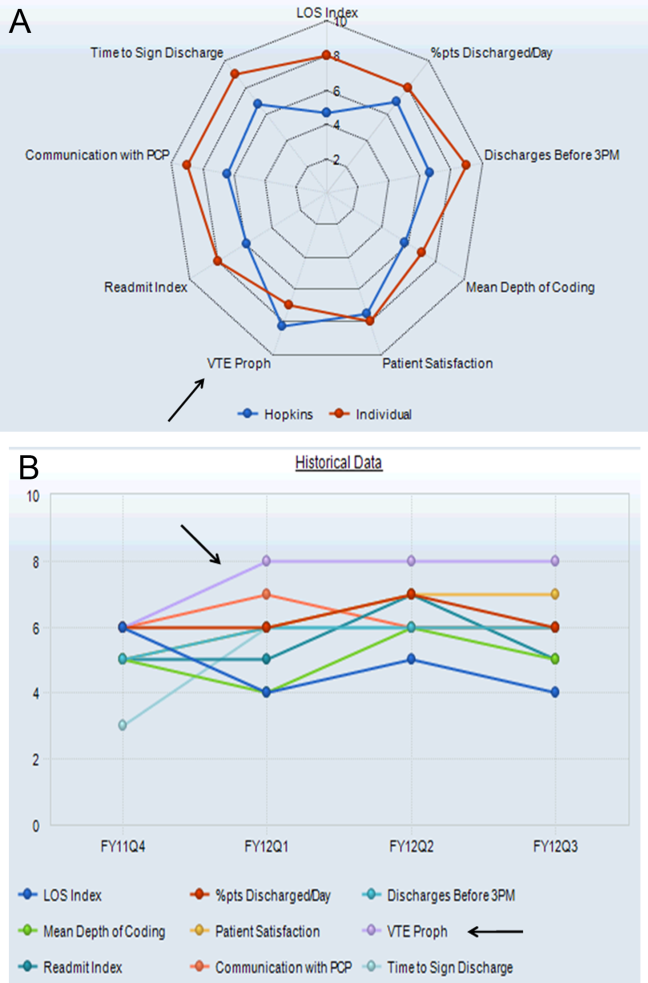
Benchmarks were established for each metric, standardized to establish a scale ranging from 1 through 9, and incorporated into the dashboard (Figure 1A). Higher scores (creating a larger geometric shape) were desirable. For the VTE prophylaxis measure, scores of 1 through 9 corresponded to <60%, 60% to 64.9%, 65% to 69.9%, 70% to 74.9%, 75% to 79.9%, 80% to 84.9%, 85% to 89.9%, 90% to 94.9%, and 95% American College of Chest Physicians (ACCP)‐compliant VTE prophylaxis, respectively.[12, 20] Each provider was able to access the aggregated dashboard (showing the group mean) and his/her individualized dashboard using an individualized login and password for the institutional portal. This portal is used during the provider's workflow, including medical record review and order entry. Both a polygonal summary graphic (Figure 1A) and trend (Figure 1B) view of the dashboard were available to the provider. A comparison of the individual provider to the hospitalist group average was displayed (Figure 1A). At monthly program meetings, the dashboard, group results, and trends were discussed.
Venous Thromboembolism Prophylaxis Compliance
Our study was performed in a tertiary academic medical center with an approximately 20‐member hospitalist group (the precise membership varied over time), whose responsibilities include, among other clinical duties, staffing a 17‐bed general medicine unit with telemetry. The scope of diagnoses and acuity of patients admitted to the hospitalist service is similar to the housestaff services. Some hospitalist faculty serve both as hospitalist and nonhospitalist general medicine service team attendings, but the comparison groups were staffed by hospitalists for <20% of the time. For admissions, all hospitalists use a standardized general medicine admission order set that is integrated into the CPOE system (Sunrise Clinical Manager; Allscripts, Chicago, IL) and completed for all admitted patients. A mandatory VTE risk screen, which includes an assessment of VTE risk factors and pharmacological prophylaxis contraindications, must be completed by the ordering physician as part of this order set (Figure 2A). The system then prompts the provider with a risk‐appropriate VTE prophylaxis recommendation that the provider may subsequently order, including mechanical prophylaxis (Figure 2B). Based on ACCP VTE prevention guidelines, risk‐appropriate prophylaxis was determined using an electronic algorithm that categorized patients into risk categories based on the presence of major VTE risk factors (Figure 2A).[12, 15, 20] If none of these were present, the provider selected No major risk factors known. Both an assessment of current use of anticoagulation and a clinically high risk of bleeding were also included (Figure 2A). If none of these were present, the provider selected No contraindications known. This algorithm is published in detail elsewhere and has been shown to not increase major bleeding episodes.[12, 15] The VTE risk assessment, but not the VTE order itself, was a mandatory field. This allowed the physician discretion to choose among various pharmacological agents and mechanical mechanisms based on patient and physician preferences.
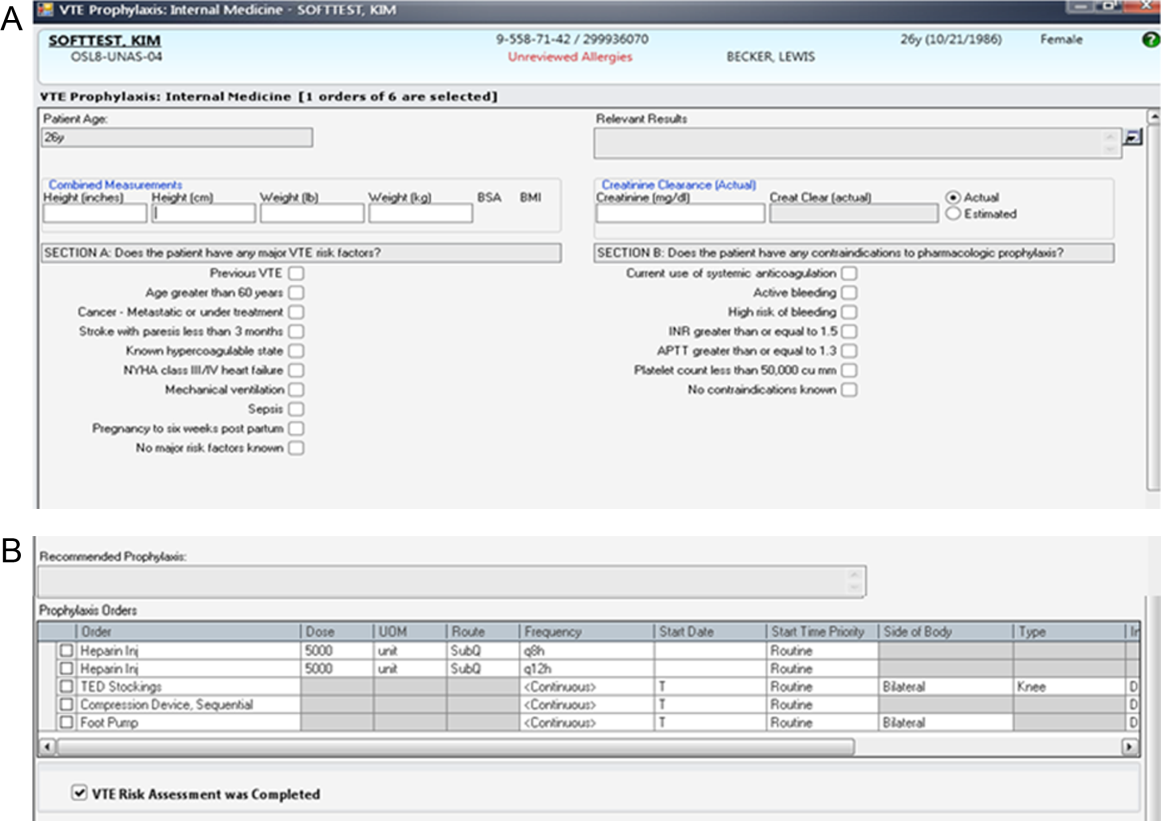
Compliance of risk‐appropriate VTE prophylaxis was determined 24 hours after the admission order set was completed using an automated electronic query of the CPOE system. Low molecular‐weight heparin prescription was included in the compliance algorithm as acceptable prophylaxis. Prescription of pharmacological VTE prophylaxis when a contraindication was present was considered noncompliant. The metric was assigned to the attending physician who billed for the first inpatient encounter.
Pay‐for‐Performance Program
In July 2011, a pay‐for‐performance program was added to the dashboard. All full‐time and part‐time hospitalists were eligible. The financial incentive was determined according to hospital priority and funds available. The VTE prophylaxis metric was prorated by clinical effort, with a maximum of $0.50 per work relative value unit (RVU). To optimize performance, a threshold of 80% compliance had to be surpassed before any payment was made. Progressively increasing percentages of the incentive were earned as compliance increased from 80% to 100%, corresponding to dashboard scores of 6, 7, 8, and 9: <80% (scores 1 to 5)=no payment; 80% to 84.9% (score 6)=$0.125 per RVU; 85% to 89.9% (score 7)=$0.25 per RVU; 90% to 94.9% (score 8)=$0.375 per RVU; and 95% (score 9)=$0.50 per RVU (maximum incentive). Payments were accrued quarterly and paid at the end of the fiscal year as a cumulative, separate performance supplement.
Individualized physician feedback through the dashboard was continued during the pay‐for‐performance period. Average hospitalist group compliance continued to be displayed on the electronic dashboard and was explicitly reviewed at monthly hospitalist meetings.
The VTE prophylaxis order set and data collection and analyses were approved by the Johns Hopkins Medicine Institutional Review Board. The dashboard and pay‐for‐performance program were initiated by the institution as part of a proof of concept quality improvement project.
Analysis
We examined all inpatient admissions to the hospitalist unit from 2008 to 2012. We included patients admitted to and discharged from the hospitalist unit and excluded patients transferred into/out of the unit and encounters with a length of stay <24 hours. VTE prophylaxis orders were queried from the CPOE system 24 hours after the patient was admitted to determine compliance.
After allowing for a run‐in period (2008), we analyzed the change in percent compliance for 3 periods: (1) CPOE‐based VTE order set alone (baseline [BASE], January 2009 to December 2010); (2) group and individual physician feedback using the dashboard (dashboard only [DASH], January to June 2011); and (3) dashboard tied to the pay‐for‐performance program (dashboard with pay‐for‐performance [P4P], July 2011 to December 2012). The CPOE‐based VTE order set was used during all 3 periods. We used the other medical services as a control to ensure that there were no temporal trends toward improved prophylaxis on a service without the intervention. VTE prophylaxis compliance was examined by calculating percent compliance using the same algorithm for the 4 resident‐staffed general medicine service teams at our institution, which utilized the same CPOE system but did not receive the dashboard or pay‐for‐performance interventions. We used locally weighted scatterplot smoothing, a locally weighted regression of percent compliance over time, to graphically display changes in group compliance over time.[21, 22]
We also performed linear regression to assess the rate of change in group compliance and included spline terms that allowed slope to vary for each of the 3 time periods.[23, 24] Clustered analysis accounted for potentially correlated serial measurements of compliance for an individual provider. A separate analysis examined the effect of provider turnover and individual provider improvement during each of the 3 periods. Tests of significance were 2‐sided, with an level of 0.05. Statistical analysis was performed using Stata 12.1 (StataCorp LP, College Station, TX).
RESULTS
Venous Thromboembolism Prophylaxis Compliance
We analyzed 3144 inpatient admissions by 38 hospitalists from 2009 to 2012. The 5 most frequent coded diagnoses were heart failure, acute kidney failure, syncope, pneumonia, and chest pain. Patients had a median length of stay of 3 days [interquartile range: 26]. During the dashboard‐only period, on average, providers improved in compliance by 4% (95% confidence interval [CI]: 35; P<0.001). With the addition of the pay‐for‐performance program, providers improved by an additional 4% (95% CI: 35; P<0.001). Group compliance significantly improved from 86% (95% CI: 8588) during the BASE period of the CPOE‐based VTE order set to 90% (95% CI: 8893) during the DASH period (P=0.01) and 94% (95% CI: 9396) during the subsequent P4P program (P=0.01) (Figure 3). Both inappropriate prophylaxis and lack of prophylaxis, when indicated, resulted in a non‐compliance rating. During the 3 periods, inappropriate prophylaxis decreased from 7.9% to 6.2% to 2.6% during the BASE, DASH, and subsequent P4P periods, respectively. Similarly, lack of prophylaxis when indicated decreased from 6.1% to 3.2% to 3.1% during the BASE, DASH, and subsequent P4P periods, respectively.
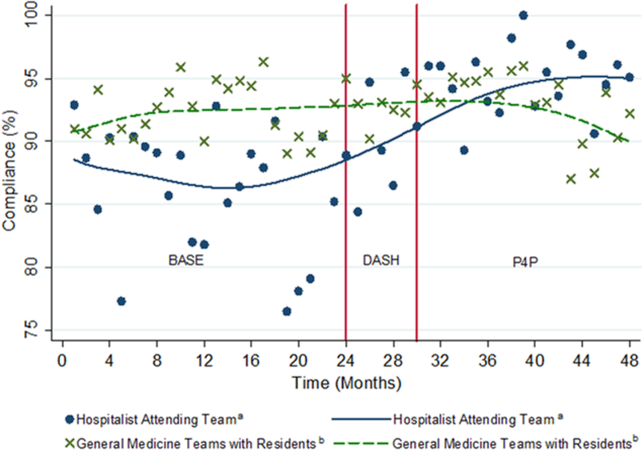
The average compliance of the 4 non‐hospitalist general medicine service teams was initially higher than that of the hospitalist service during the CPOE‐based VTE order set (90%) and DASH (92%) periods, but subsequently plateaued and was exceeded by the hospitalist service during the combined P4P (92%) period (Figure 3). However, there was no statistically significant difference between the general medicine service teams and hospitalist service during the DASH (P=0.15) and subsequent P4P (P=0.76) periods.
We also analyzed the rate of VTE prophylaxis compliance improvement (slope) with cut points at each time period transition (Figure 3). Risk‐appropriate VTE prophylaxis during the BASE period did not exhibit significant improvement as indicated by the slope (P=0.23) (Figure 3). In contrast, during the DASH period, VTE prophylaxis compliance significantly increased by 1.58% per month (95% CI: 0.41‐2.76; P=0.01). The addition of the P4P program, however, did not further significantly increase the rate of compliance (P=0.78).
A subgroup analysis restricted to the 19 providers present during all 3 periods was performed to assess for potential confounding from physician turnover. The percent compliance increased in a similar fashion: BASE period of CPOE‐based VTE order set, 85% (95% CI: 8386); DASH, 90% (95% CI: 8893); and P4P, 94% (95% CI: 9296).
Pay‐for‐Performance Program
Nineteen providers met the threshold for pay‐for‐performance (80% appropriate VTE prophylaxis), with 9 providers in the intermediate categories (80%94.9%) and 10 in the full incentive category (95%). The mean individual payout for the incentive was $633 (standard deviation 350), with a total disbursement of $12,029. The majority of payments (17 of 19) were under $1000.
DISCUSSION
A key component of healthcare reform has been value‐based purchasing, which emphasizes extrinsic motivation through the transparency of performance metrics and use of payment incentives to reward quality. Our study evaluates the impact of both extrinsic (payments) and intrinsic (professionalism and peer norms) motivation. It specifically attributed an individual performance metric, VTE prophylaxis, to an attending physician, provided both individualized and group feedback using an electronic dashboard, and incorporated a pay‐for‐performance program. Prescription of risk‐appropriate VTE prophylaxis significantly increased with the implementation of the dashboard and subsequent pay‐for performance program. The fastest rate of improvement occurred after the addition of the dashboard. Sensitivity analyses for provider turnover and comparisons to the general medicine services showed our results to be independent of a general trend of improvement, both at the provider and institutional levels.
Our prior studies demonstrated that order sets significantly improve performance, from a baseline compliance of risk‐appropriate VTE prophylaxis of 66% to 84%.[13, 15, 25] In the current study, compliance was relatively flat during the BASE period, which included these order sets. The greatest rate of continued improvement in compliance occurred during the DASH period, emphasizing both the importance of provider feedback and receptivity and adaptability in the prescribing behavior of hospitalists. Because the goal of a high‐reliability health system is for 100% of patients to receive recommended therapy, multiple approaches are necessary for success.
Nationally, benchmarks for performance measures continue to be raised, with the highest performers achieving above 95%.[26] Additional interventions, such as dashboards and pay‐for‐performance programs, supplement CPOE systems to achieve high reliability. In our study, the compliance rate during the baseline period, which included a CPOE‐based, clinical support‐enabled VTE order set, was 86%. Initially the compliance of the general medicine teams with residents exceeded that of the hospitalist attending teams, which may reflect a greater willingness of resident teams to comply with order sets and automated recommendations. This emphasizes the importance of continuous individual feedback and provider education at the attending physician level to enhance both guideline compliance and decrease provider care variation. Ultimately, with the addition of the dashboard and subsequent pay‐for‐performance program, compliance was increased to 90% and 94%, respectively. Although the major mechanism used by policymakers to improve quality of care is extrinsic motivation, this study demonstrates that intrinsic motivation through peer norms can enhance extrinsic efforts and may be more influential. Both of these programs, dashboards and pay‐for‐performance, may ultimately assist institutions in changing provider behavior and achieving these harder‐to‐achieve higher benchmarks.
We recognize that there are several limitations to our study. First, this is a single‐site program limited to an attending‐physician‐only service. There was strong data support and a defined CPOE algorithm for this initiative. Multi‐site studies will need to overcome the additional challenges of varying service structures and electronic medical record and provider order entry systems. Second, it is difficult to show actual changes in VTE events over time with appropriate prophylaxis. Although VTE prophylaxis is recommended for patients with VTE risk factors, there are conflicting findings about whether prophylaxis prevents VTE events in lower‐risk patients, and current studies suggest that most patients with VTE events are severely ill and develop VTE despite receiving prophylaxis.[27, 28, 29] Our study was underpowered to detect these potential differences in VTE rates, and although the algorithm has been shown to not increase bleeding rates, we did not measure bleeding rates during this study.[12, 15] Our institutional experience suggests that the majority of VTE events occur despite appropriate prophylaxis.[30] Also, VTE prophylaxis may be ordered, but intervening events, such as procedures and changes in risk status or patient refusal, may prevent patients from receiving appropriate prophylaxis.[31, 32] Similarly, hospitals with higher quality scores have higher VTE prophylaxis rates but worse risk‐adjusted VTE rates, which may result from increased surveillance for VTE, suggesting surveillance bias limits the usefulness of the VTE quality measure.[33, 34] Nevertheless, VTE prophylaxis remains a publicly reported Core Measure tied to financial incentives.[4, 5] Third, there may be an unmeasured factor specific to the hospitalist program, which could potentially account for an overall improvement in quality of care. Although the rate of increase in appropriate prophylaxis was not statistically significant during the baseline period, there did appear to be some improvement in prophylaxis toward the end of the period. However, there were no other VTE‐related provider feedback programs being simultaneously pursued during this study. VTE prophylaxis for the non‐hospitalist services showed a relatively stable, non‐increasing compliance rate for the general medical services. Although it was possible for successful residents to age into the hospitalist service, thereby improving rates of prophylaxis based on changes in group makeup, our subgroup analysis of the providers present throughout all phases of the study showed our results to be robust. Similarly, there may have been a cross‐contamination effect of hospitalist faculty who attended on both hospitalist and non‐hospitalist general medicine service teams. This, however, would attenuate any impact of the programs, and thus the effects may in fact be greater than reported. Fourth, establishment of both the dashboard and pay‐for‐performance program required significant institutional and program leadership and resources. To be successful, the dashboard must be in the provider's workflow, transparent, minimize reporter burden, use existing systems, and be actively fed back to providers, ideally those directly entering orders. Our greatest rate of improvement occurred during the feedback‐only phase of this study, emphasizing the importance of physician feedback, provider‐level accountability, and engagement. We suspect that the relatively modest pay‐for‐performance incentive served mainly as a means of engaging providers in self‐monitoring, rather than as a means to change behavior through true incentivization. Although we did not track individual physician views of the dashboard, we reinforced trends, deviations, and expectations at regularly scheduled meetings and provided feedback and patient‐level data to individual providers. Fifth, the design of the pay‐for‐performance program may have also influenced its effectiveness. These types of programs may be more effective when they provide frequent visible, small payments rather than one large payment, and when the payment is framed as a loss rather than a gain.[35] Finally, physician champions and consistent feedback through departmental meetings or visual displays may be required for program success. The initial resources to create the dashboard, continued maintenance and monitoring of performance, and payment of financial incentives all require institutional commitment. A partnership of physicians, program leaders, and institutional administrators is necessary for both initial and continued success.
To achieve performance goals and benchmarks, multiple strategies that combine extrinsic and intrinsic motivation are necessary. As shown by our study, the use of a dashboard and pay‐for‐performance can be tailored to an institution's goals, in line with national standards. The specific goal (risk‐appropriate VTE prophylaxis) and benchmarks (80%, 85%, 90%, 95%) can be individualized to a particular institution. For example, if readmission rates are above target, readmissions could be added as a dashboard metric. The specific benchmark would be determined by historical trends and administrative targets. Similarly, the overall financial incentives could be adjusted based on the financial resources available. Other process measures, such as influenza vaccination screening and administration, could also be targeted. For all of these objectives, continued provider feedback and engagement are critical for progressive success, especially to decrease variability in care at the attending physician level. Incorporating the value‐based purchasing philosophy from the Affordable Care Act, our study suggests that the combination of standardized order sets, real‐time dashboards, and physician‐level incentives may assist hospitals in achieving quality and safety benchmarks, especially at higher targets.
Acknowledgements
The authors thank Meir Gottlieb, BS, from Salar Inc. for data support; Murali Padmanaban, BS, from Johns Hopkins University for his assistance in linking the administrative billing data with real‐time physician orders; and Hsin‐Chieh Yeh, PhD, from the Bloomberg School of Public Health for her statistical advice and additional review. We also thank Mr. Ronald R. Peterson, President, Johns Hopkins Health System and Johns Hopkins Hospital, for providing funding support for the physician incentive payments.
Disclosures: Drs. Michtalik and Brotman had full access to all of the data in the study and take responsibility for the integrity of the data and accuracy of the data analysis. Study concept and design: Drs. Michtalik, Streiff, Finkelstein, Pronovost, and Brotman. Acquisition of data: Drs. Michtalik, Streiff, Brotman and Mr. Carolan, Mr. Lau, Mrs. Durkin. Analysis and interpretation of data: Drs. Michtalik, Haut, Streiff, Brotman and Mr. Carolan, Mr. Lau. Drafting of the manuscript: Drs. Michtalik and Brotman. Critical revision of the manuscript for important intellectual content: Drs. Michtalik, Haut, Streiff, Finkelstein, Pronovost, Brotman and Mr. Carolan, Mr. Lau, Mrs. Durkin. Statistical analysis and supervision: Drs. Michtalik and Brotman. Obtaining funding: Drs. Streiff and Brotman. Technical support: Dr. Streiff and Mr. Carolan, Mr. Lau, Mrs. Durkin
This study was supported by a National Institutes of Health grant T32 HP10025‐17‐00 (Dr. Michtalik), the National Institutes of Health/Johns Hopkins Institute for Clinical and Translational Research KL2 Award 5KL2RR025006 (Dr. Michtalik), the Agency for Healthcare Research and Quality Mentored Clinical Scientist Development K08 Awards 1K08HS017952‐01 (Dr. Haut) and 1K08HS022331‐01A1 (Dr. Michtalik), and the Johns Hopkins Hospitalist Scholars Fund. The funders had no role in the design and conduct of the study; collection, management, analysis, and interpretation of the data; preparation, review, or approval of the manuscript; and decision to submit the manuscript for publication.
Dr. Haut receives royalties from Lippincott, Williams & Wilkins. Dr. Streiff has received research funding from Portola and Bristol Myers Squibb, honoraria for CME lectures from Sanofi‐Aventis and Ortho‐McNeil, consulted for Eisai, Daiichi‐Sankyo, Boerhinger‐Ingelheim, Janssen Healthcare, and Pfizer. Mr. Lau, Drs. Haut, Streiff, and Pronovost are supported by a contract from the Patient‐Centered Outcomes Research Institute (PCORI) titled Preventing Venous Thromboembolism: Empowering Patients and Enabling Patient‐Centered Care via Health Information Technology (CE‐12‐11‐4489). Dr. Brotman has received research support from Siemens Healthcare Diagnostics, Bristol‐Myers Squibb, the Agency for Healthcare Research and Quality, Centers for Medicare & Medicaid Services, the Amerigroup Corporation, and the Guerrieri Family Foundation. He has received honoraria from the Gerson Lehrman Group, the Dunn Group, and from Quantia Communications, and received royalties from McGraw‐Hill.
The Affordable Care Act explicitly outlines improving the value of healthcare by increasing quality and decreasing costs. It emphasizes value‐based purchasing, the transparency of performance metrics, and the use of payment incentives to reward quality.[1, 2] Venous thromboembolism (VTE) prophylaxis is one of these publicly reported performance measures. The National Quality Forum recommends that each patient be evaluated on hospital admission and during their hospitalization for VTE risk level and for appropriate thromboprophylaxis to be used, if required.[3] Similarly, the Joint Commission includes appropriate VTE prophylaxis in its Core Measures.[4] Patient experience and performance metrics, including VTE prophylaxis, constitute the hospital value‐based purchasing (VBP) component of healthcare reform.[5] For a hypothetical 327‐bed hospital, an estimated $1.7 million of a hospital's inpatient payments from Medicare will be at risk from VBP alone.[2]
VTE prophylaxis is a common target of quality improvement projects. Effective, safe, and cost‐effective measures to prevent VTE exist, including pharmacologic and mechanical prophylaxis.[6, 7] Despite these measures, compliance rates are often below 50%.[8] Different interventions have been pursued to ensure appropriate VTE prophylaxis, including computerized provider order entry (CPOE), electronic alerts, mandatory VTE risk assessment and prophylaxis, and provider education campaigns.[9] Recent studies show that CPOE systems with mandatory fields can increase VTE prophylaxis rates to above 80%, yet the goal of a high reliability health system is for 100% of patients to receive recommended therapy.[10, 11, 12, 13, 14, 15] Interventions to improve prophylaxis rates that have included multiple strategies, such as computerized order sets, feedback, and education, have been the most effective, increasing compliance to above 90%.[9, 11, 16] These systems can be enhanced with additional interventions such as providing individualized provider education and feedback, understanding of work flow, and ensuring patients receive the prescribed therapies.[12] For example, a physician dashboard could be employed to provide a snapshot and historical trend of key performance indicators using graphical displays and indicators.[17]
Dashboards and pay‐for‐performance programs have been increasingly used to increase the visibility of these metrics, provide feedback, visually display benchmarks and goals, and proactively monitor for achievements and setbacks.[18] Although these strategies are often addressed at departmental (or greater) levels, applying them at the level of the individual provider may assist hospitals in reducing preventable harm and achieving safety and quality goals, especially at higher benchmarks. With their expanding role, hospitalists provide a key opportunity to lead improvement efforts and to study the impact of dashboards and pay‐for performance at the provider level to achieve VTE prophylaxis performance targets. Hospitalists are often the front‐line provider for inpatients and deliver up to 70% of inpatient general medical services.[19] The objective of our study was to evaluate the impact of providing individual provider feedback and employing a pay‐for‐performance program on baseline performance of VTE prophylaxis among hospitalists. We hypothesized that performance feedback through the use of a dashboard would increase appropriate VTE prophylaxis, and this effect would be further augmented by incorporation of a pay‐for‐performance program.
METHODS
Hospitalist Dashboard
In 2010, hospitalist program leaders met with hospital administrators to create a hospitalist dashboard that would provide regularly updated summaries of performance measures for individual hospitalists. The final set of metrics identified included appropriate VTE prophylaxis, length of stay, patients discharged per day, discharges before 3 pm, depth of coding, patient satisfaction, readmissions, communication with the primary care provider, and time to signature for discharge summaries (Figure 1A). The dashboard was introduced at a general hospitalist meeting during which its purpose, methodology, and accessibility were described; it was subsequently implemented in January 2011.
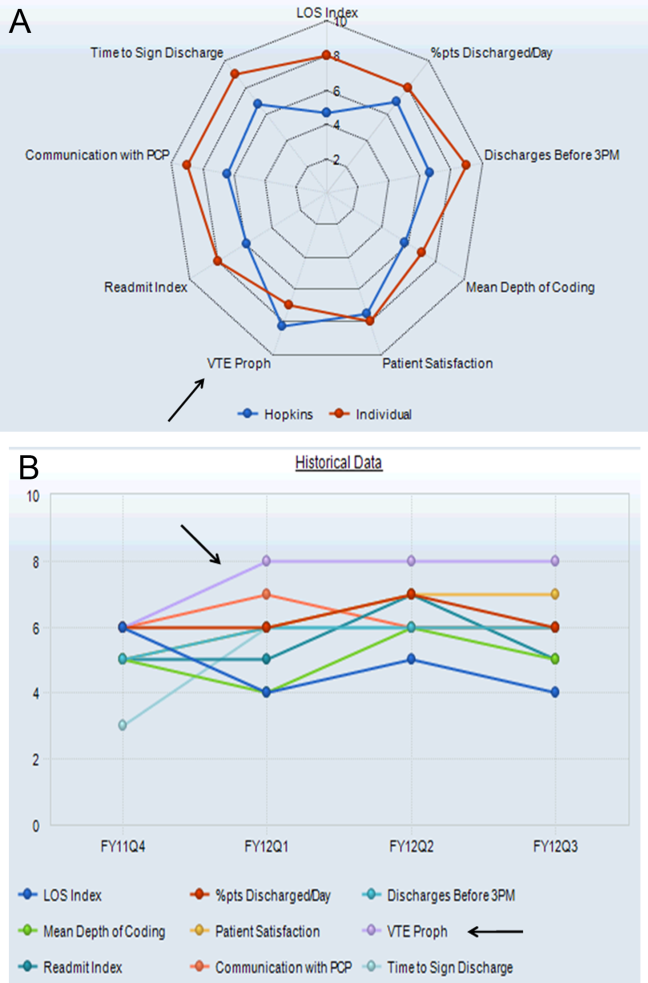
Benchmarks were established for each metric, standardized to establish a scale ranging from 1 through 9, and incorporated into the dashboard (Figure 1A). Higher scores (creating a larger geometric shape) were desirable. For the VTE prophylaxis measure, scores of 1 through 9 corresponded to <60%, 60% to 64.9%, 65% to 69.9%, 70% to 74.9%, 75% to 79.9%, 80% to 84.9%, 85% to 89.9%, 90% to 94.9%, and 95% American College of Chest Physicians (ACCP)‐compliant VTE prophylaxis, respectively.[12, 20] Each provider was able to access the aggregated dashboard (showing the group mean) and his/her individualized dashboard using an individualized login and password for the institutional portal. This portal is used during the provider's workflow, including medical record review and order entry. Both a polygonal summary graphic (Figure 1A) and trend (Figure 1B) view of the dashboard were available to the provider. A comparison of the individual provider to the hospitalist group average was displayed (Figure 1A). At monthly program meetings, the dashboard, group results, and trends were discussed.
Venous Thromboembolism Prophylaxis Compliance
Our study was performed in a tertiary academic medical center with an approximately 20‐member hospitalist group (the precise membership varied over time), whose responsibilities include, among other clinical duties, staffing a 17‐bed general medicine unit with telemetry. The scope of diagnoses and acuity of patients admitted to the hospitalist service is similar to the housestaff services. Some hospitalist faculty serve both as hospitalist and nonhospitalist general medicine service team attendings, but the comparison groups were staffed by hospitalists for <20% of the time. For admissions, all hospitalists use a standardized general medicine admission order set that is integrated into the CPOE system (Sunrise Clinical Manager; Allscripts, Chicago, IL) and completed for all admitted patients. A mandatory VTE risk screen, which includes an assessment of VTE risk factors and pharmacological prophylaxis contraindications, must be completed by the ordering physician as part of this order set (Figure 2A). The system then prompts the provider with a risk‐appropriate VTE prophylaxis recommendation that the provider may subsequently order, including mechanical prophylaxis (Figure 2B). Based on ACCP VTE prevention guidelines, risk‐appropriate prophylaxis was determined using an electronic algorithm that categorized patients into risk categories based on the presence of major VTE risk factors (Figure 2A).[12, 15, 20] If none of these were present, the provider selected No major risk factors known. Both an assessment of current use of anticoagulation and a clinically high risk of bleeding were also included (Figure 2A). If none of these were present, the provider selected No contraindications known. This algorithm is published in detail elsewhere and has been shown to not increase major bleeding episodes.[12, 15] The VTE risk assessment, but not the VTE order itself, was a mandatory field. This allowed the physician discretion to choose among various pharmacological agents and mechanical mechanisms based on patient and physician preferences.
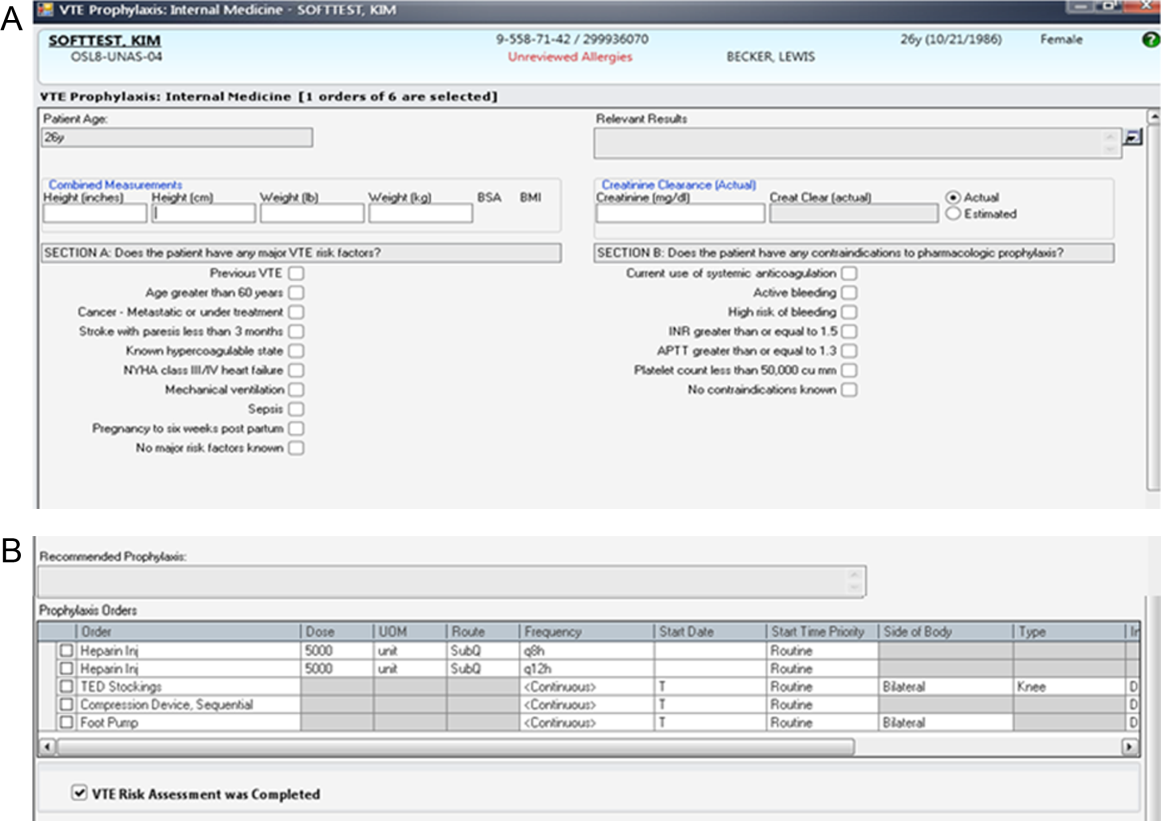
Compliance of risk‐appropriate VTE prophylaxis was determined 24 hours after the admission order set was completed using an automated electronic query of the CPOE system. Low molecular‐weight heparin prescription was included in the compliance algorithm as acceptable prophylaxis. Prescription of pharmacological VTE prophylaxis when a contraindication was present was considered noncompliant. The metric was assigned to the attending physician who billed for the first inpatient encounter.
Pay‐for‐Performance Program
In July 2011, a pay‐for‐performance program was added to the dashboard. All full‐time and part‐time hospitalists were eligible. The financial incentive was determined according to hospital priority and funds available. The VTE prophylaxis metric was prorated by clinical effort, with a maximum of $0.50 per work relative value unit (RVU). To optimize performance, a threshold of 80% compliance had to be surpassed before any payment was made. Progressively increasing percentages of the incentive were earned as compliance increased from 80% to 100%, corresponding to dashboard scores of 6, 7, 8, and 9: <80% (scores 1 to 5)=no payment; 80% to 84.9% (score 6)=$0.125 per RVU; 85% to 89.9% (score 7)=$0.25 per RVU; 90% to 94.9% (score 8)=$0.375 per RVU; and 95% (score 9)=$0.50 per RVU (maximum incentive). Payments were accrued quarterly and paid at the end of the fiscal year as a cumulative, separate performance supplement.
Individualized physician feedback through the dashboard was continued during the pay‐for‐performance period. Average hospitalist group compliance continued to be displayed on the electronic dashboard and was explicitly reviewed at monthly hospitalist meetings.
The VTE prophylaxis order set and data collection and analyses were approved by the Johns Hopkins Medicine Institutional Review Board. The dashboard and pay‐for‐performance program were initiated by the institution as part of a proof of concept quality improvement project.
Analysis
We examined all inpatient admissions to the hospitalist unit from 2008 to 2012. We included patients admitted to and discharged from the hospitalist unit and excluded patients transferred into/out of the unit and encounters with a length of stay <24 hours. VTE prophylaxis orders were queried from the CPOE system 24 hours after the patient was admitted to determine compliance.
After allowing for a run‐in period (2008), we analyzed the change in percent compliance for 3 periods: (1) CPOE‐based VTE order set alone (baseline [BASE], January 2009 to December 2010); (2) group and individual physician feedback using the dashboard (dashboard only [DASH], January to June 2011); and (3) dashboard tied to the pay‐for‐performance program (dashboard with pay‐for‐performance [P4P], July 2011 to December 2012). The CPOE‐based VTE order set was used during all 3 periods. We used the other medical services as a control to ensure that there were no temporal trends toward improved prophylaxis on a service without the intervention. VTE prophylaxis compliance was examined by calculating percent compliance using the same algorithm for the 4 resident‐staffed general medicine service teams at our institution, which utilized the same CPOE system but did not receive the dashboard or pay‐for‐performance interventions. We used locally weighted scatterplot smoothing, a locally weighted regression of percent compliance over time, to graphically display changes in group compliance over time.[21, 22]
We also performed linear regression to assess the rate of change in group compliance and included spline terms that allowed slope to vary for each of the 3 time periods.[23, 24] Clustered analysis accounted for potentially correlated serial measurements of compliance for an individual provider. A separate analysis examined the effect of provider turnover and individual provider improvement during each of the 3 periods. Tests of significance were 2‐sided, with an level of 0.05. Statistical analysis was performed using Stata 12.1 (StataCorp LP, College Station, TX).
RESULTS
Venous Thromboembolism Prophylaxis Compliance
We analyzed 3144 inpatient admissions by 38 hospitalists from 2009 to 2012. The 5 most frequent coded diagnoses were heart failure, acute kidney failure, syncope, pneumonia, and chest pain. Patients had a median length of stay of 3 days [interquartile range: 26]. During the dashboard‐only period, on average, providers improved in compliance by 4% (95% confidence interval [CI]: 35; P<0.001). With the addition of the pay‐for‐performance program, providers improved by an additional 4% (95% CI: 35; P<0.001). Group compliance significantly improved from 86% (95% CI: 8588) during the BASE period of the CPOE‐based VTE order set to 90% (95% CI: 8893) during the DASH period (P=0.01) and 94% (95% CI: 9396) during the subsequent P4P program (P=0.01) (Figure 3). Both inappropriate prophylaxis and lack of prophylaxis, when indicated, resulted in a non‐compliance rating. During the 3 periods, inappropriate prophylaxis decreased from 7.9% to 6.2% to 2.6% during the BASE, DASH, and subsequent P4P periods, respectively. Similarly, lack of prophylaxis when indicated decreased from 6.1% to 3.2% to 3.1% during the BASE, DASH, and subsequent P4P periods, respectively.
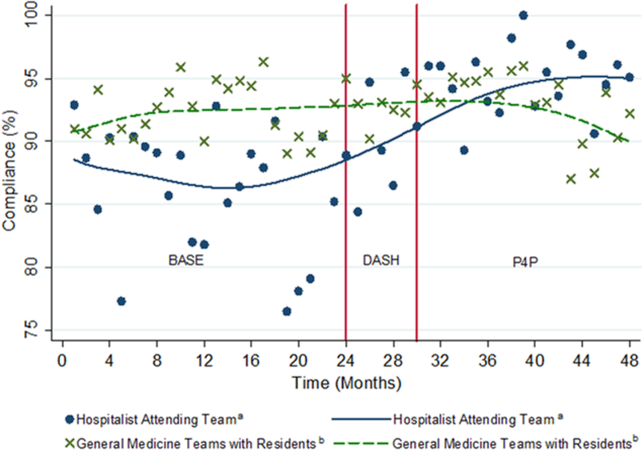
The average compliance of the 4 non‐hospitalist general medicine service teams was initially higher than that of the hospitalist service during the CPOE‐based VTE order set (90%) and DASH (92%) periods, but subsequently plateaued and was exceeded by the hospitalist service during the combined P4P (92%) period (Figure 3). However, there was no statistically significant difference between the general medicine service teams and hospitalist service during the DASH (P=0.15) and subsequent P4P (P=0.76) periods.
We also analyzed the rate of VTE prophylaxis compliance improvement (slope) with cut points at each time period transition (Figure 3). Risk‐appropriate VTE prophylaxis during the BASE period did not exhibit significant improvement as indicated by the slope (P=0.23) (Figure 3). In contrast, during the DASH period, VTE prophylaxis compliance significantly increased by 1.58% per month (95% CI: 0.41‐2.76; P=0.01). The addition of the P4P program, however, did not further significantly increase the rate of compliance (P=0.78).
A subgroup analysis restricted to the 19 providers present during all 3 periods was performed to assess for potential confounding from physician turnover. The percent compliance increased in a similar fashion: BASE period of CPOE‐based VTE order set, 85% (95% CI: 8386); DASH, 90% (95% CI: 8893); and P4P, 94% (95% CI: 9296).
Pay‐for‐Performance Program
Nineteen providers met the threshold for pay‐for‐performance (80% appropriate VTE prophylaxis), with 9 providers in the intermediate categories (80%94.9%) and 10 in the full incentive category (95%). The mean individual payout for the incentive was $633 (standard deviation 350), with a total disbursement of $12,029. The majority of payments (17 of 19) were under $1000.
DISCUSSION
A key component of healthcare reform has been value‐based purchasing, which emphasizes extrinsic motivation through the transparency of performance metrics and use of payment incentives to reward quality. Our study evaluates the impact of both extrinsic (payments) and intrinsic (professionalism and peer norms) motivation. It specifically attributed an individual performance metric, VTE prophylaxis, to an attending physician, provided both individualized and group feedback using an electronic dashboard, and incorporated a pay‐for‐performance program. Prescription of risk‐appropriate VTE prophylaxis significantly increased with the implementation of the dashboard and subsequent pay‐for performance program. The fastest rate of improvement occurred after the addition of the dashboard. Sensitivity analyses for provider turnover and comparisons to the general medicine services showed our results to be independent of a general trend of improvement, both at the provider and institutional levels.
Our prior studies demonstrated that order sets significantly improve performance, from a baseline compliance of risk‐appropriate VTE prophylaxis of 66% to 84%.[13, 15, 25] In the current study, compliance was relatively flat during the BASE period, which included these order sets. The greatest rate of continued improvement in compliance occurred during the DASH period, emphasizing both the importance of provider feedback and receptivity and adaptability in the prescribing behavior of hospitalists. Because the goal of a high‐reliability health system is for 100% of patients to receive recommended therapy, multiple approaches are necessary for success.
Nationally, benchmarks for performance measures continue to be raised, with the highest performers achieving above 95%.[26] Additional interventions, such as dashboards and pay‐for‐performance programs, supplement CPOE systems to achieve high reliability. In our study, the compliance rate during the baseline period, which included a CPOE‐based, clinical support‐enabled VTE order set, was 86%. Initially the compliance of the general medicine teams with residents exceeded that of the hospitalist attending teams, which may reflect a greater willingness of resident teams to comply with order sets and automated recommendations. This emphasizes the importance of continuous individual feedback and provider education at the attending physician level to enhance both guideline compliance and decrease provider care variation. Ultimately, with the addition of the dashboard and subsequent pay‐for‐performance program, compliance was increased to 90% and 94%, respectively. Although the major mechanism used by policymakers to improve quality of care is extrinsic motivation, this study demonstrates that intrinsic motivation through peer norms can enhance extrinsic efforts and may be more influential. Both of these programs, dashboards and pay‐for‐performance, may ultimately assist institutions in changing provider behavior and achieving these harder‐to‐achieve higher benchmarks.
We recognize that there are several limitations to our study. First, this is a single‐site program limited to an attending‐physician‐only service. There was strong data support and a defined CPOE algorithm for this initiative. Multi‐site studies will need to overcome the additional challenges of varying service structures and electronic medical record and provider order entry systems. Second, it is difficult to show actual changes in VTE events over time with appropriate prophylaxis. Although VTE prophylaxis is recommended for patients with VTE risk factors, there are conflicting findings about whether prophylaxis prevents VTE events in lower‐risk patients, and current studies suggest that most patients with VTE events are severely ill and develop VTE despite receiving prophylaxis.[27, 28, 29] Our study was underpowered to detect these potential differences in VTE rates, and although the algorithm has been shown to not increase bleeding rates, we did not measure bleeding rates during this study.[12, 15] Our institutional experience suggests that the majority of VTE events occur despite appropriate prophylaxis.[30] Also, VTE prophylaxis may be ordered, but intervening events, such as procedures and changes in risk status or patient refusal, may prevent patients from receiving appropriate prophylaxis.[31, 32] Similarly, hospitals with higher quality scores have higher VTE prophylaxis rates but worse risk‐adjusted VTE rates, which may result from increased surveillance for VTE, suggesting surveillance bias limits the usefulness of the VTE quality measure.[33, 34] Nevertheless, VTE prophylaxis remains a publicly reported Core Measure tied to financial incentives.[4, 5] Third, there may be an unmeasured factor specific to the hospitalist program, which could potentially account for an overall improvement in quality of care. Although the rate of increase in appropriate prophylaxis was not statistically significant during the baseline period, there did appear to be some improvement in prophylaxis toward the end of the period. However, there were no other VTE‐related provider feedback programs being simultaneously pursued during this study. VTE prophylaxis for the non‐hospitalist services showed a relatively stable, non‐increasing compliance rate for the general medical services. Although it was possible for successful residents to age into the hospitalist service, thereby improving rates of prophylaxis based on changes in group makeup, our subgroup analysis of the providers present throughout all phases of the study showed our results to be robust. Similarly, there may have been a cross‐contamination effect of hospitalist faculty who attended on both hospitalist and non‐hospitalist general medicine service teams. This, however, would attenuate any impact of the programs, and thus the effects may in fact be greater than reported. Fourth, establishment of both the dashboard and pay‐for‐performance program required significant institutional and program leadership and resources. To be successful, the dashboard must be in the provider's workflow, transparent, minimize reporter burden, use existing systems, and be actively fed back to providers, ideally those directly entering orders. Our greatest rate of improvement occurred during the feedback‐only phase of this study, emphasizing the importance of physician feedback, provider‐level accountability, and engagement. We suspect that the relatively modest pay‐for‐performance incentive served mainly as a means of engaging providers in self‐monitoring, rather than as a means to change behavior through true incentivization. Although we did not track individual physician views of the dashboard, we reinforced trends, deviations, and expectations at regularly scheduled meetings and provided feedback and patient‐level data to individual providers. Fifth, the design of the pay‐for‐performance program may have also influenced its effectiveness. These types of programs may be more effective when they provide frequent visible, small payments rather than one large payment, and when the payment is framed as a loss rather than a gain.[35] Finally, physician champions and consistent feedback through departmental meetings or visual displays may be required for program success. The initial resources to create the dashboard, continued maintenance and monitoring of performance, and payment of financial incentives all require institutional commitment. A partnership of physicians, program leaders, and institutional administrators is necessary for both initial and continued success.
To achieve performance goals and benchmarks, multiple strategies that combine extrinsic and intrinsic motivation are necessary. As shown by our study, the use of a dashboard and pay‐for‐performance can be tailored to an institution's goals, in line with national standards. The specific goal (risk‐appropriate VTE prophylaxis) and benchmarks (80%, 85%, 90%, 95%) can be individualized to a particular institution. For example, if readmission rates are above target, readmissions could be added as a dashboard metric. The specific benchmark would be determined by historical trends and administrative targets. Similarly, the overall financial incentives could be adjusted based on the financial resources available. Other process measures, such as influenza vaccination screening and administration, could also be targeted. For all of these objectives, continued provider feedback and engagement are critical for progressive success, especially to decrease variability in care at the attending physician level. Incorporating the value‐based purchasing philosophy from the Affordable Care Act, our study suggests that the combination of standardized order sets, real‐time dashboards, and physician‐level incentives may assist hospitals in achieving quality and safety benchmarks, especially at higher targets.
Acknowledgements
The authors thank Meir Gottlieb, BS, from Salar Inc. for data support; Murali Padmanaban, BS, from Johns Hopkins University for his assistance in linking the administrative billing data with real‐time physician orders; and Hsin‐Chieh Yeh, PhD, from the Bloomberg School of Public Health for her statistical advice and additional review. We also thank Mr. Ronald R. Peterson, President, Johns Hopkins Health System and Johns Hopkins Hospital, for providing funding support for the physician incentive payments.
Disclosures: Drs. Michtalik and Brotman had full access to all of the data in the study and take responsibility for the integrity of the data and accuracy of the data analysis. Study concept and design: Drs. Michtalik, Streiff, Finkelstein, Pronovost, and Brotman. Acquisition of data: Drs. Michtalik, Streiff, Brotman and Mr. Carolan, Mr. Lau, Mrs. Durkin. Analysis and interpretation of data: Drs. Michtalik, Haut, Streiff, Brotman and Mr. Carolan, Mr. Lau. Drafting of the manuscript: Drs. Michtalik and Brotman. Critical revision of the manuscript for important intellectual content: Drs. Michtalik, Haut, Streiff, Finkelstein, Pronovost, Brotman and Mr. Carolan, Mr. Lau, Mrs. Durkin. Statistical analysis and supervision: Drs. Michtalik and Brotman. Obtaining funding: Drs. Streiff and Brotman. Technical support: Dr. Streiff and Mr. Carolan, Mr. Lau, Mrs. Durkin
This study was supported by a National Institutes of Health grant T32 HP10025‐17‐00 (Dr. Michtalik), the National Institutes of Health/Johns Hopkins Institute for Clinical and Translational Research KL2 Award 5KL2RR025006 (Dr. Michtalik), the Agency for Healthcare Research and Quality Mentored Clinical Scientist Development K08 Awards 1K08HS017952‐01 (Dr. Haut) and 1K08HS022331‐01A1 (Dr. Michtalik), and the Johns Hopkins Hospitalist Scholars Fund. The funders had no role in the design and conduct of the study; collection, management, analysis, and interpretation of the data; preparation, review, or approval of the manuscript; and decision to submit the manuscript for publication.
Dr. Haut receives royalties from Lippincott, Williams & Wilkins. Dr. Streiff has received research funding from Portola and Bristol Myers Squibb, honoraria for CME lectures from Sanofi‐Aventis and Ortho‐McNeil, consulted for Eisai, Daiichi‐Sankyo, Boerhinger‐Ingelheim, Janssen Healthcare, and Pfizer. Mr. Lau, Drs. Haut, Streiff, and Pronovost are supported by a contract from the Patient‐Centered Outcomes Research Institute (PCORI) titled Preventing Venous Thromboembolism: Empowering Patients and Enabling Patient‐Centered Care via Health Information Technology (CE‐12‐11‐4489). Dr. Brotman has received research support from Siemens Healthcare Diagnostics, Bristol‐Myers Squibb, the Agency for Healthcare Research and Quality, Centers for Medicare & Medicaid Services, the Amerigroup Corporation, and the Guerrieri Family Foundation. He has received honoraria from the Gerson Lehrman Group, the Dunn Group, and from Quantia Communications, and received royalties from McGraw‐Hill.
- Medicare Program, Centers for Medicare 76(88):26490–26547.
- Quality meets finance: payments at risk with value‐based purchasing, readmission, and hospital‐acquired conditions force hospitalists to focus. Hospitalist. 2013;17(1):31. .
- National Quality Forum. March 2009. Safe practices for better healthcare—2009 update. Available at: http://www.qualityforum.org/Publications/2009/03/Safe_Practices_for_Better_Healthcare%E2%80%932009_Update.aspx. Accessed November 1, 2014.
- Joint Commission on Accreditation of Healthcare Organizations. Approved: more options for hospital core measures. Jt Comm Perspect. 2009;29(4):1–6.
- Centers for Medicare 208(2):227–240.
- Thromboprophylaxis in nonsurgical patients. Hematology Am Soc Hematol Educ Program. 2012;2012:631–637. , .
- Venous thromboembolism risk and prophylaxis in the acute hospital care setting (ENDORSE study): a multinational cross‐sectional study. Lancet. 2008;371(9610):387–394. , , , et al.
- Practices to prevent venous thromboembolism: a brief review. BMJ Qual Saf. 2014;23(3):187–195. , .
- Improving hospital venous thromboembolism prophylaxis with electronic decision support. J Hosp Med. 2013;8(3):115–120. , , , et al.
- Innovative approaches to increase deep vein thrombosis prophylaxis rate resulting in a decrease in hospital‐acquired deep vein thrombosis at a tertiary‐care teaching hospital. J Hosp Med. 2008;3(2):148–155. , , .
- Lessons from the Johns Hopkins Multi‐Disciplinary Venous Thromboembolism (VTE) Prevention Collaborative. BMJ. 2012;344:e3935. , , , et al.
- Improved prophylaxis and decreased rates of preventable harm with the use of a mandatory computerized clinical decision support tool for prophylaxis for venous thromboembolism in trauma. Arch Surg. 2012;147(10):901–907. , , , et al.
- Designing and implementing effective venous thromboembolism prevention protocols: lessons from collaborative efforts. J Thromb Thrombolysis. 2010;29(2):159–166. , .
- Impact of a venous thromboembolism prophylaxis "smart order set": improved compliance, fewer events. Am J Hematol. 2013;88(7):545–549. , , , et al.
- Improving adherence to venous thromoembolism prophylaxis using multiple interventions. BMJ. 2012;344:e3935. , .
- Health Resources and Services Administration of the U.S. Department of Health and Human Services. Managing data for performance improvement. Available at: http://www.hrsa.gov/quality/toolbox/methodology/performanceimprovement/part2.html. Accessed December 18, 2014.
- Improving patient safety by taking systems seriously. JAMA. 2008;299(4):445–447. , .
- Growth in the care of older patients by hospitalists in the United States. N Engl J Med. 2009;360(11):1102–1112. , , , .
- Prevention of venous thromboembolism: American College of Chest Physicians evidence‐based clinical practice guidelines (8th edition). Chest. 2008;133(6 suppl):381S–453S. , , , et al.
- Robust locally weighted regression and smoothing scatterplots. J Am Stat Assoc. 1979;74(368):829–836. .
- Locally weighted regression: An approach to regression analysis by local fitting. J Am Stat Assoc. 1988;83(403):596–610. , .
- Regression Methods in Biostatistics: Linear, Logistic, Survival, and Repeated Measures Models. 2nd ed. New York, NY: Springer; 2012. , , , .
- Regression Modeling Strategies: With Applications to Linear Models, Logistic Regression, and Survival Analysis. New York, NY: Springer‐Verlag; 2001. .
- Med Care. doi: 10.1097/MLR.0000000000000251. , , , et al. Eliminating healthcare disparities via mandatory clinical decision support: the venous thromboembolism (VTE) example [published online ahead of print November 4, 2014].
- Joint Commission. Improving America's hospitals: the Joint Commission's annual report on quality and safety. 2012. Available at: http://www.jointcommission.org/assets/1/18/TJC_Annual_Report_2012.pdf. Accessed September 8, 2013.
- Hospital performance for pharmacologic venous thromboembolism prophylaxis and rate of venous thromboembolism: a cohort study. JAMA Intern Med. 2014;174(10):1577–1584. , , , et al.
- Incidence of hospital‐acquired venous thromboembolic codes in medical patients hospitalized in academic medical centers. J Hosp Med. 2014;9(4):221–225. , , , et al.
- No association between hospital‐reported perioperative venous thromboembolism prophylaxis and outcome rates in publicly reported data. JAMA Surg. 2014;149(4):400–401. , , , , .
- Linking processes and outcomes: a key strategy to prevent and report harm from venous thromboembolism in surgical patients. JAMA Surg. 2013;148(3):299–300. , , , , .
- Patterns of non‐administration of ordered doses of venous thromboembolism prophylaxis: implications for novel intervention strategies. PLoS One. 2013;8(6):e66311. , , , et al.
- Nonadministration of thromboprophylaxis in hospitalized patients with HIV: a missed opportunity for prevention? J Hosp Med. 2014;9(4):215–220. , , , et al.
- Evaluation of surveillance bias and the validity of the venous thromboembolism quality measure. JAMA. 2013;310(14):1482–1489. , , , et al.
- Surveillance bias in outcomes reporting. JAMA. 2011;305(23):2462–2463. , .
- Pay for performance in health care: an international overview of initiatives. Med Care Res Rev. 2012;69(3):251–276. .
- Medicare Program, Centers for Medicare 76(88):26490–26547.
- Quality meets finance: payments at risk with value‐based purchasing, readmission, and hospital‐acquired conditions force hospitalists to focus. Hospitalist. 2013;17(1):31. .
- National Quality Forum. March 2009. Safe practices for better healthcare—2009 update. Available at: http://www.qualityforum.org/Publications/2009/03/Safe_Practices_for_Better_Healthcare%E2%80%932009_Update.aspx. Accessed November 1, 2014.
- Joint Commission on Accreditation of Healthcare Organizations. Approved: more options for hospital core measures. Jt Comm Perspect. 2009;29(4):1–6.
- Centers for Medicare 208(2):227–240.
- Thromboprophylaxis in nonsurgical patients. Hematology Am Soc Hematol Educ Program. 2012;2012:631–637. , .
- Venous thromboembolism risk and prophylaxis in the acute hospital care setting (ENDORSE study): a multinational cross‐sectional study. Lancet. 2008;371(9610):387–394. , , , et al.
- Practices to prevent venous thromboembolism: a brief review. BMJ Qual Saf. 2014;23(3):187–195. , .
- Improving hospital venous thromboembolism prophylaxis with electronic decision support. J Hosp Med. 2013;8(3):115–120. , , , et al.
- Innovative approaches to increase deep vein thrombosis prophylaxis rate resulting in a decrease in hospital‐acquired deep vein thrombosis at a tertiary‐care teaching hospital. J Hosp Med. 2008;3(2):148–155. , , .
- Lessons from the Johns Hopkins Multi‐Disciplinary Venous Thromboembolism (VTE) Prevention Collaborative. BMJ. 2012;344:e3935. , , , et al.
- Improved prophylaxis and decreased rates of preventable harm with the use of a mandatory computerized clinical decision support tool for prophylaxis for venous thromboembolism in trauma. Arch Surg. 2012;147(10):901–907. , , , et al.
- Designing and implementing effective venous thromboembolism prevention protocols: lessons from collaborative efforts. J Thromb Thrombolysis. 2010;29(2):159–166. , .
- Impact of a venous thromboembolism prophylaxis "smart order set": improved compliance, fewer events. Am J Hematol. 2013;88(7):545–549. , , , et al.
- Improving adherence to venous thromoembolism prophylaxis using multiple interventions. BMJ. 2012;344:e3935. , .
- Health Resources and Services Administration of the U.S. Department of Health and Human Services. Managing data for performance improvement. Available at: http://www.hrsa.gov/quality/toolbox/methodology/performanceimprovement/part2.html. Accessed December 18, 2014.
- Improving patient safety by taking systems seriously. JAMA. 2008;299(4):445–447. , .
- Growth in the care of older patients by hospitalists in the United States. N Engl J Med. 2009;360(11):1102–1112. , , , .
- Prevention of venous thromboembolism: American College of Chest Physicians evidence‐based clinical practice guidelines (8th edition). Chest. 2008;133(6 suppl):381S–453S. , , , et al.
- Robust locally weighted regression and smoothing scatterplots. J Am Stat Assoc. 1979;74(368):829–836. .
- Locally weighted regression: An approach to regression analysis by local fitting. J Am Stat Assoc. 1988;83(403):596–610. , .
- Regression Methods in Biostatistics: Linear, Logistic, Survival, and Repeated Measures Models. 2nd ed. New York, NY: Springer; 2012. , , , .
- Regression Modeling Strategies: With Applications to Linear Models, Logistic Regression, and Survival Analysis. New York, NY: Springer‐Verlag; 2001. .
- Med Care. doi: 10.1097/MLR.0000000000000251. , , , et al. Eliminating healthcare disparities via mandatory clinical decision support: the venous thromboembolism (VTE) example [published online ahead of print November 4, 2014].
- Joint Commission. Improving America's hospitals: the Joint Commission's annual report on quality and safety. 2012. Available at: http://www.jointcommission.org/assets/1/18/TJC_Annual_Report_2012.pdf. Accessed September 8, 2013.
- Hospital performance for pharmacologic venous thromboembolism prophylaxis and rate of venous thromboembolism: a cohort study. JAMA Intern Med. 2014;174(10):1577–1584. , , , et al.
- Incidence of hospital‐acquired venous thromboembolic codes in medical patients hospitalized in academic medical centers. J Hosp Med. 2014;9(4):221–225. , , , et al.
- No association between hospital‐reported perioperative venous thromboembolism prophylaxis and outcome rates in publicly reported data. JAMA Surg. 2014;149(4):400–401. , , , , .
- Linking processes and outcomes: a key strategy to prevent and report harm from venous thromboembolism in surgical patients. JAMA Surg. 2013;148(3):299–300. , , , , .
- Patterns of non‐administration of ordered doses of venous thromboembolism prophylaxis: implications for novel intervention strategies. PLoS One. 2013;8(6):e66311. , , , et al.
- Nonadministration of thromboprophylaxis in hospitalized patients with HIV: a missed opportunity for prevention? J Hosp Med. 2014;9(4):215–220. , , , et al.
- Evaluation of surveillance bias and the validity of the venous thromboembolism quality measure. JAMA. 2013;310(14):1482–1489. , , , et al.
- Surveillance bias in outcomes reporting. JAMA. 2011;305(23):2462–2463. , .
- Pay for performance in health care: an international overview of initiatives. Med Care Res Rev. 2012;69(3):251–276. .
© 2014 Society of Hospital Medicine
Predicting Safe Physician Workloads
Attending physician workload may be compromising patient safety and quality of care. Recent studies show hospitalists, intensivists, and surgeons report that excessive attending physician workload has a negative impact on patient care.[1, 2, 3] Because physician teams and hospitals differ in composition, function, and setting, it is difficult to directly compare one service to another within or between institutions. Identifying physician, team, and hospital characteristics associated with clinicians' impressions of unsafe workload provides physician leaders, hospital administrators, and policymakers with potential risk factors and specific targets for interventions.[4] In this study, we use a national survey of hospitalists to identify the physician, team, and hospital factors associated with physician report of an unsafe workload.
METHODS
We electronically surveyed 890 self‐identified hospitalists enrolled in
RESULTS
Of the 890 physicians contacted, 506 (57%) responded. Full characteristics of respondents are reported elsewhere.[1] Forty percent of physicians (n=202) indicated that their typical inpatient census exceeded safe levels at least monthly. A descriptive comparison of the lower and higher reporters of unsafe levels is provided (Table 1). Higher frequency of reporting an unsafe census was associated with higher percentages of clinical (P=0.004) and inpatient responsibilities (P<0.001) and more time seeing patients without midlevel or housestaff assistance (P=0.001) (Table 1). On the other hand, lower reported unsafe census was associated with more years in practice (P=0.02), greater percentage of personal time (P=0.02), and the presence of any system for census control (patient caps, fixed bed capacity, staffing augmentation plans) (P=0.007) (Table 1). Fixed census caps decreased the odds of reporting an unsafe census by 34% and was the only statistically significant workload control mechanism (odds ratio: 0.66; 95% confidence interval: 0.43‐0.99; P=0.04). There was no association between reported unsafe census and physician age (P=0.42), practice area (P=0.63), organization type (P=0.98), or compensation (salary [P=0.23], bonus [P=0.61], or total [P=0.54]).
Characteristic | Report of Unsafe Workloada | Univariate Odds Ratio (95% CI) | Reported Effect on Unsafe Workload Frequency | |
---|---|---|---|---|
Lower | Higher | |||
| ||||
Percentage of total work hours devoted to patient care, median [IQR] | 95 [80100] | 100 [90100] | 1.13b (1.041.23)c | Increased |
Percentage of clinical care that is inpatient, median [IQR] | 75 [5085] | 80 [7090] | 1.21b (1.131.34)d | |
Percentage of clinical work performed with no assistance from housestaff or midlevels, median [IQR] | 80 [25100] | 90 [50100] | 1.08b (1.031.14)c | |
Years in practice, median [IQR] | 6 [311] | 5 [310] | 0.85e (0.750.98)f | Decreased |
Percentage of workday allotted for personal time, median [IQR] | 5 [07] | 3 [05] | 0.50b (0.380.92)f | |
Systems for increased patient volume, No. (%) | ||||
Fixed census cap | 87 (30) | 45 (22) | 0.66 (0.430.99)f | |
Fixed bed capacity | 36 (13) | 24 (12) | 0.94 (0.541.63) | |
Staffing augmentation | 88 (31) | 58 (29) | 0.91 (0.611.35) | |
Any system | 217 (76) | 130 (64) | 0.58 (0.390.86)g | |
Primary practice area of hospital medicine, No. (%) | ||||
Adult | 211 (73) | 173 (86) | 1 | Equivocal |
Pediatric | 7 (2) | 1 (0.5) | 0.24 (0.032.10) | |
Combined, adult and pediatric | 5 (2) | 3 (1) | 0.73 (0.173.10) | |
Primary role, No. (%) | ||||
Clinical | 242 (83) | 186 (92) | 1 | |
Research | 5 (2) | 4 (2) | 1.04 (0.283.93) | |
Administrative | 14 (5) | 6 (3) | 0.56 (0.211.48) | |
Physician age, median [IQR], y | 36 [3242] | 37 [3342] | 0.96e (0.861.07) | |
Compensation, median [IQR], thousands of dollars | ||||
Salary only | 180 [130200] | 180 [150200] | 0.97h (0.981.05) | |
Incentive pay only | 10 [025] | 10 [020] | 0.99h (0.941.04) | |
Total | 190 [140220] | 196 [165220] | 0.99h (0.981.03) | |
Practice area, No. (%) | ||||
Urban | 128 (45) | 98 (49) | 1 | |
Suburban | 126 (44) | 81 (41) | 0.84 (0.571.23) | |
Rural | 33 (11) | 21 (10) | 0.83 (0.451.53) | |
Practice location, No. (%) | ||||
Academic | 82 (29) | 54 (27) | 1 | |
Community | 153 (53) | 110 (55) | 1.09 (0.721.66) | |
Veterans hospital | 7 (2) | 4 (2) | 0.87 (0.243.10) | |
Group | 32 (11) | 25 (13) | 1.19 (0.632.21) | |
Physician group size, median [IQR] | 12 [620] | 12 [822] | 0.99i (0.981.03) | |
Localization of patients, No. (%) | ||||
Multiple units | 179 (61) | 124 (61) | 1 | |
Single or adjacent unit(s) | 87 (30) | 58 (29) | 0.96 (0.641.44) | |
Multiple hospitals | 25 (9) | 20 (10) | 1.15 (0.612.17) |
DISCUSSION
This is the first study to our knowledge to describe factors associated with provider reports of unsafe workload and identifies potential targets for intervention. By identifying modifiable factors affecting workload, such as different team structures with housestaff or midlevels, it may be possible to improve workload, efficiency, and perhaps safety.[5, 6] Less experience, decreased housestaff or midlevel assistance, higher percentages of inpatient and clinical responsibilities, and lack of systems for census control were strongly associated with reports of unsafe workload.
Having any system in place to address increased patient volumes reduced the odds of reporting an unsafe workload. However, only fixed patient census caps were statistically significant. A system that incorporates fixed service or admitting caps may provide greater control on workload but may also result in back‐ups and delays in the emergency room. Similarly, fixed caps may require overflow of patients to less experienced or willing services or increase the number of handoffs, which may adversely affect the quality of patient care. Use of separate admitting teams has the potential to increase efficiency, but is also subject to fluctuations in patient volume and increases the number of handoffs. Each institution should use a multidisciplinary systems approach to address patient throughput and enforce manageable workload such as through the creation of patient flow teams.[7]
Limitations of the study include the relatively small sample of hospitalists and self‐reporting of safety. Because of the diverse characteristics and structures of the individual programs, even if a predictor variable was not missing, if a particular value for that predictor occurred very infrequently, it generated very wide effect estimates. This limited our ability to effectively explore potential confounders and interactions. To our knowledge, this study is the first to explore potential predictors of unsafe attending physician workload. Large national surveys of physicians with greater statistical power can expand upon this initial work and further explore the association between, and interaction of, workload factors and varying perceptions of providers.[4] The most important limitation of this work is that we relied on self‐reporting to define a safe census. We do not have any measured clinical outcomes that can serve to validate the self‐reported impressions. We recognize, however, that adverse events in healthcare require multiple weaknesses to align, and typically, multiple barriers exist to prevent such events. This often makes it difficult to show direct causal links. Additionally, self‐reporting of safety may also be subject to recall bias, because adverse patient outcomes are often particularly memorable. However, high‐reliability organizations recognize the importance of front‐line provider input, such as on the sensitivity of operations (working conditions) and by deferring to expertise (insights and recommendations from providers most knowledgeable of conditions, regardless of seniority).[8]
We acknowledge that several workload factors, such as hospital setting, may not be readily modifiable. However, we also report factors that can be intervened upon, such as assistance[5, 6] or geographic localization of patients.[9, 10] An understanding of both modifiable and fixed factors in healthcare delivery is essential for improving patient care.
This study has significant research implications. It suggests that team structure and physician experience may be used to improve workload safety. Also, particularly if these self‐reported findings are verified using clinical outcomes, providing hospitalists with greater staffing assistance and systems responsive to census fluctuations may improve the safety, quality, and flow of patient care. Future research may identify the association of physician, team, and hospital factors with outcomes and objectively assess targeted interventions to improve both the efficiency and quality of care.
Acknowledgments
The authors thank the Johns Hopkins Clinical Research Network Hospitalists, General Internal Medicine Research in Progress Physicians, and Hospitalist Directors for the Maryland/District of Columbia region for sharing their models of care and comments on the survey content. They also thank Michael Paskavitz, BA (Editor‐in‐Chief) and Brian Driscoll, BA (Managing Editor) from Quantia Communications for all of their technical assistance in administering the survey.
Disclosures: Drs. Michtalik and Brotman had full access to all of the data in the study and take responsibility for the integrity of the data and accuracy of the data analysis. Study concept and design: Michtalik, Pronovost, Brotman. Analysis, interpretation of data: Michtalik, Pronovost, Marsteller, Spetz, Brotman. Drafting of the manuscript: Michtalik, Brotman. Critical revision of the manuscript for important intellectual content: Michtalik, Pronovost, Marsteller, Spetz, Brotman. Dr. Brotman has received compensation from Quantia Communications, not exceeding $10,000 annually, for developing educational content. Dr. Michtalik was supported by NIH grant T32 HP10025‐17‐00 and NIH/Johns Hopkins Institute for Clinical and Translational Research KL2 Award 5KL2RR025006. The Johns Hopkins Hospitalist Scholars Fund provided funding for survey implementation and data acquisition by Quantia Communications. The funders had no role in the design, analysis, and interpretation of the data, or the preparation, review, or approval of the manuscript. The authors report no conflicts of interest.
- Impact of attending physician workload on patient care: a survey of hospitalists. JAMA Intern Med. 2013;173(5):375–377. , , , .
- Does surgeon workload per day affect outcomes after pulmonary lobectomies? Ann Thorac Surg. 2012;94(3):966–972. , , , et al.
- Perceived effects of attending physician workload in academic medical intensive care units: a national survey of training program directors. Crit Care Med. 2012;40(2):400–405. , , , .
- Developing a model for attending physician workload and outcomes. JAMA Intern Med. 2013;173(11):1026–1028. , , , , .
- A comparison of outcomes of general medical inpatient care provided by a hospitalist‐physician assistant model vs a traditional resident‐based model. J Hosp Med. 2011;6(3):122–130. , , , et al.
- Implementation of a physician assistant/hospitalist service in an academic medical center: impact on efficiency and patient outcomes. J Hosp Med. 2008;3(5):361–368. , , , et al.
- Improving patient flow and reducing emergency department crowding: a guide for hospitals. AHRQ publication no. 11(12)−0094. Rockville, MD: Agency for Healthcare Research and Quality; 2011. , , , .
- Becoming a high reliability organization: operational advice for hospital leaders. AHRQ publication no. 08–0022. Rockville, MD: Agency for Healthcare Research and Quality; 2008. , , , et al.
- Impact of localizing general medical teams to a single nursing unit. J Hosp Med. 2012;7(7):551–556. , , , et al.
- Impact of localizing physicians to hospital units on nurse‐physician communication and agreement on the plan of care. J Gen Intern Med. 2009;24(11):1223–1227. , , , et al.
Attending physician workload may be compromising patient safety and quality of care. Recent studies show hospitalists, intensivists, and surgeons report that excessive attending physician workload has a negative impact on patient care.[1, 2, 3] Because physician teams and hospitals differ in composition, function, and setting, it is difficult to directly compare one service to another within or between institutions. Identifying physician, team, and hospital characteristics associated with clinicians' impressions of unsafe workload provides physician leaders, hospital administrators, and policymakers with potential risk factors and specific targets for interventions.[4] In this study, we use a national survey of hospitalists to identify the physician, team, and hospital factors associated with physician report of an unsafe workload.
METHODS
We electronically surveyed 890 self‐identified hospitalists enrolled in
RESULTS
Of the 890 physicians contacted, 506 (57%) responded. Full characteristics of respondents are reported elsewhere.[1] Forty percent of physicians (n=202) indicated that their typical inpatient census exceeded safe levels at least monthly. A descriptive comparison of the lower and higher reporters of unsafe levels is provided (Table 1). Higher frequency of reporting an unsafe census was associated with higher percentages of clinical (P=0.004) and inpatient responsibilities (P<0.001) and more time seeing patients without midlevel or housestaff assistance (P=0.001) (Table 1). On the other hand, lower reported unsafe census was associated with more years in practice (P=0.02), greater percentage of personal time (P=0.02), and the presence of any system for census control (patient caps, fixed bed capacity, staffing augmentation plans) (P=0.007) (Table 1). Fixed census caps decreased the odds of reporting an unsafe census by 34% and was the only statistically significant workload control mechanism (odds ratio: 0.66; 95% confidence interval: 0.43‐0.99; P=0.04). There was no association between reported unsafe census and physician age (P=0.42), practice area (P=0.63), organization type (P=0.98), or compensation (salary [P=0.23], bonus [P=0.61], or total [P=0.54]).
Characteristic | Report of Unsafe Workloada | Univariate Odds Ratio (95% CI) | Reported Effect on Unsafe Workload Frequency | |
---|---|---|---|---|
Lower | Higher | |||
| ||||
Percentage of total work hours devoted to patient care, median [IQR] | 95 [80100] | 100 [90100] | 1.13b (1.041.23)c | Increased |
Percentage of clinical care that is inpatient, median [IQR] | 75 [5085] | 80 [7090] | 1.21b (1.131.34)d | |
Percentage of clinical work performed with no assistance from housestaff or midlevels, median [IQR] | 80 [25100] | 90 [50100] | 1.08b (1.031.14)c | |
Years in practice, median [IQR] | 6 [311] | 5 [310] | 0.85e (0.750.98)f | Decreased |
Percentage of workday allotted for personal time, median [IQR] | 5 [07] | 3 [05] | 0.50b (0.380.92)f | |
Systems for increased patient volume, No. (%) | ||||
Fixed census cap | 87 (30) | 45 (22) | 0.66 (0.430.99)f | |
Fixed bed capacity | 36 (13) | 24 (12) | 0.94 (0.541.63) | |
Staffing augmentation | 88 (31) | 58 (29) | 0.91 (0.611.35) | |
Any system | 217 (76) | 130 (64) | 0.58 (0.390.86)g | |
Primary practice area of hospital medicine, No. (%) | ||||
Adult | 211 (73) | 173 (86) | 1 | Equivocal |
Pediatric | 7 (2) | 1 (0.5) | 0.24 (0.032.10) | |
Combined, adult and pediatric | 5 (2) | 3 (1) | 0.73 (0.173.10) | |
Primary role, No. (%) | ||||
Clinical | 242 (83) | 186 (92) | 1 | |
Research | 5 (2) | 4 (2) | 1.04 (0.283.93) | |
Administrative | 14 (5) | 6 (3) | 0.56 (0.211.48) | |
Physician age, median [IQR], y | 36 [3242] | 37 [3342] | 0.96e (0.861.07) | |
Compensation, median [IQR], thousands of dollars | ||||
Salary only | 180 [130200] | 180 [150200] | 0.97h (0.981.05) | |
Incentive pay only | 10 [025] | 10 [020] | 0.99h (0.941.04) | |
Total | 190 [140220] | 196 [165220] | 0.99h (0.981.03) | |
Practice area, No. (%) | ||||
Urban | 128 (45) | 98 (49) | 1 | |
Suburban | 126 (44) | 81 (41) | 0.84 (0.571.23) | |
Rural | 33 (11) | 21 (10) | 0.83 (0.451.53) | |
Practice location, No. (%) | ||||
Academic | 82 (29) | 54 (27) | 1 | |
Community | 153 (53) | 110 (55) | 1.09 (0.721.66) | |
Veterans hospital | 7 (2) | 4 (2) | 0.87 (0.243.10) | |
Group | 32 (11) | 25 (13) | 1.19 (0.632.21) | |
Physician group size, median [IQR] | 12 [620] | 12 [822] | 0.99i (0.981.03) | |
Localization of patients, No. (%) | ||||
Multiple units | 179 (61) | 124 (61) | 1 | |
Single or adjacent unit(s) | 87 (30) | 58 (29) | 0.96 (0.641.44) | |
Multiple hospitals | 25 (9) | 20 (10) | 1.15 (0.612.17) |
DISCUSSION
This is the first study to our knowledge to describe factors associated with provider reports of unsafe workload and identifies potential targets for intervention. By identifying modifiable factors affecting workload, such as different team structures with housestaff or midlevels, it may be possible to improve workload, efficiency, and perhaps safety.[5, 6] Less experience, decreased housestaff or midlevel assistance, higher percentages of inpatient and clinical responsibilities, and lack of systems for census control were strongly associated with reports of unsafe workload.
Having any system in place to address increased patient volumes reduced the odds of reporting an unsafe workload. However, only fixed patient census caps were statistically significant. A system that incorporates fixed service or admitting caps may provide greater control on workload but may also result in back‐ups and delays in the emergency room. Similarly, fixed caps may require overflow of patients to less experienced or willing services or increase the number of handoffs, which may adversely affect the quality of patient care. Use of separate admitting teams has the potential to increase efficiency, but is also subject to fluctuations in patient volume and increases the number of handoffs. Each institution should use a multidisciplinary systems approach to address patient throughput and enforce manageable workload such as through the creation of patient flow teams.[7]
Limitations of the study include the relatively small sample of hospitalists and self‐reporting of safety. Because of the diverse characteristics and structures of the individual programs, even if a predictor variable was not missing, if a particular value for that predictor occurred very infrequently, it generated very wide effect estimates. This limited our ability to effectively explore potential confounders and interactions. To our knowledge, this study is the first to explore potential predictors of unsafe attending physician workload. Large national surveys of physicians with greater statistical power can expand upon this initial work and further explore the association between, and interaction of, workload factors and varying perceptions of providers.[4] The most important limitation of this work is that we relied on self‐reporting to define a safe census. We do not have any measured clinical outcomes that can serve to validate the self‐reported impressions. We recognize, however, that adverse events in healthcare require multiple weaknesses to align, and typically, multiple barriers exist to prevent such events. This often makes it difficult to show direct causal links. Additionally, self‐reporting of safety may also be subject to recall bias, because adverse patient outcomes are often particularly memorable. However, high‐reliability organizations recognize the importance of front‐line provider input, such as on the sensitivity of operations (working conditions) and by deferring to expertise (insights and recommendations from providers most knowledgeable of conditions, regardless of seniority).[8]
We acknowledge that several workload factors, such as hospital setting, may not be readily modifiable. However, we also report factors that can be intervened upon, such as assistance[5, 6] or geographic localization of patients.[9, 10] An understanding of both modifiable and fixed factors in healthcare delivery is essential for improving patient care.
This study has significant research implications. It suggests that team structure and physician experience may be used to improve workload safety. Also, particularly if these self‐reported findings are verified using clinical outcomes, providing hospitalists with greater staffing assistance and systems responsive to census fluctuations may improve the safety, quality, and flow of patient care. Future research may identify the association of physician, team, and hospital factors with outcomes and objectively assess targeted interventions to improve both the efficiency and quality of care.
Acknowledgments
The authors thank the Johns Hopkins Clinical Research Network Hospitalists, General Internal Medicine Research in Progress Physicians, and Hospitalist Directors for the Maryland/District of Columbia region for sharing their models of care and comments on the survey content. They also thank Michael Paskavitz, BA (Editor‐in‐Chief) and Brian Driscoll, BA (Managing Editor) from Quantia Communications for all of their technical assistance in administering the survey.
Disclosures: Drs. Michtalik and Brotman had full access to all of the data in the study and take responsibility for the integrity of the data and accuracy of the data analysis. Study concept and design: Michtalik, Pronovost, Brotman. Analysis, interpretation of data: Michtalik, Pronovost, Marsteller, Spetz, Brotman. Drafting of the manuscript: Michtalik, Brotman. Critical revision of the manuscript for important intellectual content: Michtalik, Pronovost, Marsteller, Spetz, Brotman. Dr. Brotman has received compensation from Quantia Communications, not exceeding $10,000 annually, for developing educational content. Dr. Michtalik was supported by NIH grant T32 HP10025‐17‐00 and NIH/Johns Hopkins Institute for Clinical and Translational Research KL2 Award 5KL2RR025006. The Johns Hopkins Hospitalist Scholars Fund provided funding for survey implementation and data acquisition by Quantia Communications. The funders had no role in the design, analysis, and interpretation of the data, or the preparation, review, or approval of the manuscript. The authors report no conflicts of interest.
Attending physician workload may be compromising patient safety and quality of care. Recent studies show hospitalists, intensivists, and surgeons report that excessive attending physician workload has a negative impact on patient care.[1, 2, 3] Because physician teams and hospitals differ in composition, function, and setting, it is difficult to directly compare one service to another within or between institutions. Identifying physician, team, and hospital characteristics associated with clinicians' impressions of unsafe workload provides physician leaders, hospital administrators, and policymakers with potential risk factors and specific targets for interventions.[4] In this study, we use a national survey of hospitalists to identify the physician, team, and hospital factors associated with physician report of an unsafe workload.
METHODS
We electronically surveyed 890 self‐identified hospitalists enrolled in
RESULTS
Of the 890 physicians contacted, 506 (57%) responded. Full characteristics of respondents are reported elsewhere.[1] Forty percent of physicians (n=202) indicated that their typical inpatient census exceeded safe levels at least monthly. A descriptive comparison of the lower and higher reporters of unsafe levels is provided (Table 1). Higher frequency of reporting an unsafe census was associated with higher percentages of clinical (P=0.004) and inpatient responsibilities (P<0.001) and more time seeing patients without midlevel or housestaff assistance (P=0.001) (Table 1). On the other hand, lower reported unsafe census was associated with more years in practice (P=0.02), greater percentage of personal time (P=0.02), and the presence of any system for census control (patient caps, fixed bed capacity, staffing augmentation plans) (P=0.007) (Table 1). Fixed census caps decreased the odds of reporting an unsafe census by 34% and was the only statistically significant workload control mechanism (odds ratio: 0.66; 95% confidence interval: 0.43‐0.99; P=0.04). There was no association between reported unsafe census and physician age (P=0.42), practice area (P=0.63), organization type (P=0.98), or compensation (salary [P=0.23], bonus [P=0.61], or total [P=0.54]).
Characteristic | Report of Unsafe Workloada | Univariate Odds Ratio (95% CI) | Reported Effect on Unsafe Workload Frequency | |
---|---|---|---|---|
Lower | Higher | |||
| ||||
Percentage of total work hours devoted to patient care, median [IQR] | 95 [80100] | 100 [90100] | 1.13b (1.041.23)c | Increased |
Percentage of clinical care that is inpatient, median [IQR] | 75 [5085] | 80 [7090] | 1.21b (1.131.34)d | |
Percentage of clinical work performed with no assistance from housestaff or midlevels, median [IQR] | 80 [25100] | 90 [50100] | 1.08b (1.031.14)c | |
Years in practice, median [IQR] | 6 [311] | 5 [310] | 0.85e (0.750.98)f | Decreased |
Percentage of workday allotted for personal time, median [IQR] | 5 [07] | 3 [05] | 0.50b (0.380.92)f | |
Systems for increased patient volume, No. (%) | ||||
Fixed census cap | 87 (30) | 45 (22) | 0.66 (0.430.99)f | |
Fixed bed capacity | 36 (13) | 24 (12) | 0.94 (0.541.63) | |
Staffing augmentation | 88 (31) | 58 (29) | 0.91 (0.611.35) | |
Any system | 217 (76) | 130 (64) | 0.58 (0.390.86)g | |
Primary practice area of hospital medicine, No. (%) | ||||
Adult | 211 (73) | 173 (86) | 1 | Equivocal |
Pediatric | 7 (2) | 1 (0.5) | 0.24 (0.032.10) | |
Combined, adult and pediatric | 5 (2) | 3 (1) | 0.73 (0.173.10) | |
Primary role, No. (%) | ||||
Clinical | 242 (83) | 186 (92) | 1 | |
Research | 5 (2) | 4 (2) | 1.04 (0.283.93) | |
Administrative | 14 (5) | 6 (3) | 0.56 (0.211.48) | |
Physician age, median [IQR], y | 36 [3242] | 37 [3342] | 0.96e (0.861.07) | |
Compensation, median [IQR], thousands of dollars | ||||
Salary only | 180 [130200] | 180 [150200] | 0.97h (0.981.05) | |
Incentive pay only | 10 [025] | 10 [020] | 0.99h (0.941.04) | |
Total | 190 [140220] | 196 [165220] | 0.99h (0.981.03) | |
Practice area, No. (%) | ||||
Urban | 128 (45) | 98 (49) | 1 | |
Suburban | 126 (44) | 81 (41) | 0.84 (0.571.23) | |
Rural | 33 (11) | 21 (10) | 0.83 (0.451.53) | |
Practice location, No. (%) | ||||
Academic | 82 (29) | 54 (27) | 1 | |
Community | 153 (53) | 110 (55) | 1.09 (0.721.66) | |
Veterans hospital | 7 (2) | 4 (2) | 0.87 (0.243.10) | |
Group | 32 (11) | 25 (13) | 1.19 (0.632.21) | |
Physician group size, median [IQR] | 12 [620] | 12 [822] | 0.99i (0.981.03) | |
Localization of patients, No. (%) | ||||
Multiple units | 179 (61) | 124 (61) | 1 | |
Single or adjacent unit(s) | 87 (30) | 58 (29) | 0.96 (0.641.44) | |
Multiple hospitals | 25 (9) | 20 (10) | 1.15 (0.612.17) |
DISCUSSION
This is the first study to our knowledge to describe factors associated with provider reports of unsafe workload and identifies potential targets for intervention. By identifying modifiable factors affecting workload, such as different team structures with housestaff or midlevels, it may be possible to improve workload, efficiency, and perhaps safety.[5, 6] Less experience, decreased housestaff or midlevel assistance, higher percentages of inpatient and clinical responsibilities, and lack of systems for census control were strongly associated with reports of unsafe workload.
Having any system in place to address increased patient volumes reduced the odds of reporting an unsafe workload. However, only fixed patient census caps were statistically significant. A system that incorporates fixed service or admitting caps may provide greater control on workload but may also result in back‐ups and delays in the emergency room. Similarly, fixed caps may require overflow of patients to less experienced or willing services or increase the number of handoffs, which may adversely affect the quality of patient care. Use of separate admitting teams has the potential to increase efficiency, but is also subject to fluctuations in patient volume and increases the number of handoffs. Each institution should use a multidisciplinary systems approach to address patient throughput and enforce manageable workload such as through the creation of patient flow teams.[7]
Limitations of the study include the relatively small sample of hospitalists and self‐reporting of safety. Because of the diverse characteristics and structures of the individual programs, even if a predictor variable was not missing, if a particular value for that predictor occurred very infrequently, it generated very wide effect estimates. This limited our ability to effectively explore potential confounders and interactions. To our knowledge, this study is the first to explore potential predictors of unsafe attending physician workload. Large national surveys of physicians with greater statistical power can expand upon this initial work and further explore the association between, and interaction of, workload factors and varying perceptions of providers.[4] The most important limitation of this work is that we relied on self‐reporting to define a safe census. We do not have any measured clinical outcomes that can serve to validate the self‐reported impressions. We recognize, however, that adverse events in healthcare require multiple weaknesses to align, and typically, multiple barriers exist to prevent such events. This often makes it difficult to show direct causal links. Additionally, self‐reporting of safety may also be subject to recall bias, because adverse patient outcomes are often particularly memorable. However, high‐reliability organizations recognize the importance of front‐line provider input, such as on the sensitivity of operations (working conditions) and by deferring to expertise (insights and recommendations from providers most knowledgeable of conditions, regardless of seniority).[8]
We acknowledge that several workload factors, such as hospital setting, may not be readily modifiable. However, we also report factors that can be intervened upon, such as assistance[5, 6] or geographic localization of patients.[9, 10] An understanding of both modifiable and fixed factors in healthcare delivery is essential for improving patient care.
This study has significant research implications. It suggests that team structure and physician experience may be used to improve workload safety. Also, particularly if these self‐reported findings are verified using clinical outcomes, providing hospitalists with greater staffing assistance and systems responsive to census fluctuations may improve the safety, quality, and flow of patient care. Future research may identify the association of physician, team, and hospital factors with outcomes and objectively assess targeted interventions to improve both the efficiency and quality of care.
Acknowledgments
The authors thank the Johns Hopkins Clinical Research Network Hospitalists, General Internal Medicine Research in Progress Physicians, and Hospitalist Directors for the Maryland/District of Columbia region for sharing their models of care and comments on the survey content. They also thank Michael Paskavitz, BA (Editor‐in‐Chief) and Brian Driscoll, BA (Managing Editor) from Quantia Communications for all of their technical assistance in administering the survey.
Disclosures: Drs. Michtalik and Brotman had full access to all of the data in the study and take responsibility for the integrity of the data and accuracy of the data analysis. Study concept and design: Michtalik, Pronovost, Brotman. Analysis, interpretation of data: Michtalik, Pronovost, Marsteller, Spetz, Brotman. Drafting of the manuscript: Michtalik, Brotman. Critical revision of the manuscript for important intellectual content: Michtalik, Pronovost, Marsteller, Spetz, Brotman. Dr. Brotman has received compensation from Quantia Communications, not exceeding $10,000 annually, for developing educational content. Dr. Michtalik was supported by NIH grant T32 HP10025‐17‐00 and NIH/Johns Hopkins Institute for Clinical and Translational Research KL2 Award 5KL2RR025006. The Johns Hopkins Hospitalist Scholars Fund provided funding for survey implementation and data acquisition by Quantia Communications. The funders had no role in the design, analysis, and interpretation of the data, or the preparation, review, or approval of the manuscript. The authors report no conflicts of interest.
- Impact of attending physician workload on patient care: a survey of hospitalists. JAMA Intern Med. 2013;173(5):375–377. , , , .
- Does surgeon workload per day affect outcomes after pulmonary lobectomies? Ann Thorac Surg. 2012;94(3):966–972. , , , et al.
- Perceived effects of attending physician workload in academic medical intensive care units: a national survey of training program directors. Crit Care Med. 2012;40(2):400–405. , , , .
- Developing a model for attending physician workload and outcomes. JAMA Intern Med. 2013;173(11):1026–1028. , , , , .
- A comparison of outcomes of general medical inpatient care provided by a hospitalist‐physician assistant model vs a traditional resident‐based model. J Hosp Med. 2011;6(3):122–130. , , , et al.
- Implementation of a physician assistant/hospitalist service in an academic medical center: impact on efficiency and patient outcomes. J Hosp Med. 2008;3(5):361–368. , , , et al.
- Improving patient flow and reducing emergency department crowding: a guide for hospitals. AHRQ publication no. 11(12)−0094. Rockville, MD: Agency for Healthcare Research and Quality; 2011. , , , .
- Becoming a high reliability organization: operational advice for hospital leaders. AHRQ publication no. 08–0022. Rockville, MD: Agency for Healthcare Research and Quality; 2008. , , , et al.
- Impact of localizing general medical teams to a single nursing unit. J Hosp Med. 2012;7(7):551–556. , , , et al.
- Impact of localizing physicians to hospital units on nurse‐physician communication and agreement on the plan of care. J Gen Intern Med. 2009;24(11):1223–1227. , , , et al.
- Impact of attending physician workload on patient care: a survey of hospitalists. JAMA Intern Med. 2013;173(5):375–377. , , , .
- Does surgeon workload per day affect outcomes after pulmonary lobectomies? Ann Thorac Surg. 2012;94(3):966–972. , , , et al.
- Perceived effects of attending physician workload in academic medical intensive care units: a national survey of training program directors. Crit Care Med. 2012;40(2):400–405. , , , .
- Developing a model for attending physician workload and outcomes. JAMA Intern Med. 2013;173(11):1026–1028. , , , , .
- A comparison of outcomes of general medical inpatient care provided by a hospitalist‐physician assistant model vs a traditional resident‐based model. J Hosp Med. 2011;6(3):122–130. , , , et al.
- Implementation of a physician assistant/hospitalist service in an academic medical center: impact on efficiency and patient outcomes. J Hosp Med. 2008;3(5):361–368. , , , et al.
- Improving patient flow and reducing emergency department crowding: a guide for hospitals. AHRQ publication no. 11(12)−0094. Rockville, MD: Agency for Healthcare Research and Quality; 2011. , , , .
- Becoming a high reliability organization: operational advice for hospital leaders. AHRQ publication no. 08–0022. Rockville, MD: Agency for Healthcare Research and Quality; 2008. , , , et al.
- Impact of localizing general medical teams to a single nursing unit. J Hosp Med. 2012;7(7):551–556. , , , et al.
- Impact of localizing physicians to hospital units on nurse‐physician communication and agreement on the plan of care. J Gen Intern Med. 2009;24(11):1223–1227. , , , et al.