User login
Does Patient Experience Predict 30-Day Readmission? A Patient-Level Analysis of HCAHPS Data
Patient experience and 30-day readmission are important measures of quality of care for hospitalized patients. Performance on both of these measures impact hospitals financially. Performance on the Hospital Consumer Assessment of Healthcare Systems and Providers (HCAHPS) survey is linked to 25% of the incentive payment under Value Based Purchasing (VBP) Program.1 Starting in 2012, the Centers for Medicare and Medicaid Services (CMS) introduced the Readmission Reduction Program, penalizing hospitals financially for excessive readmissions.2
A relationship between patient experience and readmissions has been explored at the hospital level. Studies have mostly found that higher patient experience scores are associated with lower 30-day readmission rates. In a study of the relationship between 30-day risk-standardized readmission rates for three medical conditions (acute myocardial infarction, heart failure, and pneumonia) and patient experience, the authors noted that higher experience scores for overall care and discharge planning were associated with lower readmission rates for these conditions. They also concluded that patient experience scores were more predictive of 30-day readmission than clinical performance measures. Additionally, the authors predicted that if a hospital increased its total experience scores from the 25th percentile to the 75th percentile, there would be an associated decrease in readmissions by at least 2.3% for each of these conditions.3 Practice management companies and the media have cited this finding to conclude that higher patient experience drives clinical outcomes such as 30-day readmission and that patients are often the best judges of the quality of care delivered.4,5
Other hospital-level studies have found that high 30-day readmission rates are associated with lower overall experience scores in a mixed surgical patient population; worse reports of pain control and overall care in the colorectal surgery population; lower experience scores with discharge preparedness in vascular surgery patients; and lower experience scores with physician communication, nurse communication, and discharge preparedness.6-9 A patient-level study noted higher readmissions are associated with worse experience with physician and nursing communication along with a paradoxically better experience with discharge information.10
Because these studies used an observational design, they demonstrated associations rather than causality. An alternative hypothesis is that readmitted patients complete their patient experience survey after readmission and the low experience is the result, rather than the cause, of their readmission. For patients who are readmitted, it is unclear whether there is an opportunity to complete the survey prior to readmission and whether being readmitted may impact patient perception of quality of care. Using patient-level data, we sought to assess HCAHPS patient-experience responses linked to the index admission of the patients who were readmitted in 30 days and compare it with those patients who were not readmitted during this time period. We paid particular attention to when the surveys were returned.
METHODS
Study Design
We conducted a retrospective analysis of prospectively collected 10-year HCAHPS and Press Ganey patient survey data for a single tertiary care academic hospital.
Participants
All adult patients discharged from the hospital and who responded to the routinely sent patient-experience survey were included. Surveys were sent to a random sample of 50% of the discharged patients.
The exposure group was comprised of patients who responded to the survey and were readmitted within 30 days of discharge. After subtracting 5 days from the survey receipt date for expected delays related to mail delivery time and processing time, survey response date was calculated. The exposure group was further divided into patients who responded to the survey prior to their 30-day readmission (“Pre-readmission responders”) and those that responded to the survey after their readmission (“Postreadmission responders”). A sensitivity analysis was performed by changing the number of days subtracted from the survey receipt date by 2 days in either direction. This approach did not result in any significant changes in the results.
The control group comprised patients who were not readmitted to the hospital within 30 days of discharge and who did not have an admission in the previous 30 days as well (“Not readmitted” group). An additional comparison group for exploratory analysis included patients who had experienced an admission in the prior 30 days but were not readmitted after the admission linked to the survey. These patients responded to the patient-experience surveys that were linked to their second admission in 30 days (“2nd-admission responders” group; Figure).
Time Periods
All survey responders from the third quarter of 2006 to the first quarter of 2016 were included in the study. Additionally, administrative data on non-responders were available from 7/2006 to 8/2012. These data were used to estimate response rates. Patient level experience and administrative data were obtained in a linked fashion for these time periods.
Instruments
Press Ganey and HCAHPS surveys were sent via mail in the same envelope. Fifty percent of the discharged patients were randomized to receive the surveys. The Press Ganey survey contained 33 items encompassing several subdomains, including room, meal, nursing, physician, ancillary staff, visitor, discharge, and overall experience.
The HCAHPS survey contained 29 CMS-mandated items, of which 21 are related to patient experience. The development, testing, and methods for administration and reporting of the HCAHPS survey have been previously described and studies using this instrument have been reported in the literature.11 Press Ganey patient satisfaction survey results have also been reported in the literature.12
Outcome Variables and Covariates
HCAHPS and Press Ganey experience survey individual item responses were the primary outcome variables of this study. Age, self-reported health status, education, primary language spoken, service line, and time taken to respond to the surveys served as the covariates. These variables are used by CMS for patient-mix adjustment and are collected on the HCAHPS survey. Additionally, the number of days to respond to the survey were included in all regression analysis to adjust for early responder effect.13-15
Statistical Analysis
“Percent top-box” scores were calculated for each survey item for patients in each group. The percent top-box scores were calculated as the percent of patients who responded “very good” for a given item on Press Ganey survey items and “always” or “definitely yes” or “yes” or “9” or “10” on HCAHPS survey items. CMS utilizes “percent top-box scores” to calculate payments under the VBP program and to report the results publicly. Numerous studies have also reported percent top-box scores for HCAHPS survey results.12
We hypothesized that whether patients complete the HCAHPS survey before or after the readmission influences their reporting of experience. To test this hypothesis, HCAHPS and Press Ganey item top-box scores of “Pre-readmission responders” and “Postreadmission responders” were compared with those of the control group using multivariate logistic regression. “Pre-readmission responders” were also compared with “Postreadmission responders”.
“2nd-admission responders” were similarly compared with the control group for an exploratory analysis. Finally, “Postreadmission responders” and “2nd-admission responders” were compared in another exploratory analysis since both these groups responded to the survey after being exposed to the readmission, even though the “Postreadmission responders” group is administratively linked to the index admission.
The Johns Hopkins Institutional Review Board approved this study.
RESULTS
There were 43,737 survey responders, among whom 4,707 were subsequently readmitted within 30 days of discharge. Among the readmitted patients who responded to the surveys linked to their index admission, only 15.8% returned the survey before readmission (pre-readmission responders’) and 84.2% returned the survey after readmission (postreadmission responders). Additionally, 1,663 patients responded to experience surveys linked to their readmission. There were 37,365 patients in the control arm (ie, patients who responded to the survey and were not readmitted within 30 days of discharge or in the prior 30 days; Figure 1). The readmission rate among survey responders was 10.6%. Among the readmitted patients, the median number of days to readmission was 10 days while the median number of days to respond to the survey for this group was 33 days. Among the nonreadmitted patients, the median number of days to return the survey was 29 days.
We also conducted an exploratory analysis of the postreadmission responders, comparing them with patients who received patient-experience surveys linked to their second admission in 30 days. Both of these groups were exposed to a readmission before they completed the surveys. There were no significant differences between these two groups on patient experience scores. Additionally, the patients who received the survey linked to their readmission had a broad dissatisfaction pattern on HCAHPS survey items that appeared similar to that of the postreadmission group when compared to the non-readmitted group (Table 3).
DISCUSSION
In this retrospective analysis of prospectively collected Press Ganey and HCAHPS patient-experience survey data, we found that the overwhelming majority of patients readmitted within 30 days of discharge respond to HCAHPS surveys after readmission even though the survey is sent linked to the first admission. This is not unexpected since the median time to survey response is 33 days for this group, while median time to readmission is 10 days. The dissatisfaction pattern of Postreadmission responders was similar to those who responded to the survey linked to the readmission. When a patient is readmitted prior to completing the survey, their responses appear to reflect the cumulative experience of the index admission and the readmission. The lower scores of those who respond to the survey after their readmission appear to be a driver for lower patient-experience scores related to readmissions. Overall, readmission was associated with lower scores on items in five of the nine domains used to calculate patient experience related payments under VBP.16
These findings have important implications in inferring the direction of potential causal relationship between readmissions and patient experience at the hospital level. Additionally, these patients show broad dissatisfaction with areas beyond physician communication and discharge planning. These include staff responsiveness, phlebotomy, meals, hospital cleanliness, and noise level. This pattern of dissatisfaction may represent impatience and frustration with spending additional time in the hospital environment.
Our results are consistent with findings of many of the earlier studies, but our study goes a step further by using patient-level data and incorporating survey response time in our analysis.3,7,9,10 By separating out the readmitted patients who responded to the survey prior to admission, we attempted to address the ability of patients’ perception of care to predict future readmissions. Our results do not support this idea, since pre-readmission responders had similar experience scores to non-readmitted patients. However, because of the low numbers of pre-readmission responders, the comparison lacks precision. Current HCAHPS and Press Ganey questions may lack the ability to predict future readmissions because of the timing of the survey (postdischarge) or the questions themselves.
Overall, postreadmission responders are dissatisfied with multiple domains of hospital care. Many of these survey responses may simply be related to general frustration. Alternatively, they may represent a patient population with a high degree of needs that are not as easily met by a hospital’s routine processes of care. Even though the readmission rates were 10.6% among survey responders, 14.6% of the survey responses were associated with readmissions after accounting for those who respond to surveys linked to readmission. These patients could have significant impact on cumulative experience scores.
Our study has a few limitations. First, it involves a single tertiary care academic center study, and our results may not be generalizable. Second, we did not adjust for some of the patient characteristics associated with readmissions. Patients who were admitted within 30 days are different than those not readmitted based on payor, race, length of stay, and severity of illness, and we did not adjust for these factors in our analysis. This was intentional, however. Our goal was to better understand the relationship between 30-day readmission and patient experience scores as they are used for hospital-level studies, VBP, and public reporting. For these purposes, the scores are not adjusted for factors, such as payor and length of stay. We did adjust for patient-mix adjustment factors used by CMS. Third, the response rates to the HCAHPS were low and may have biased the scores. However, HCAHPS is widely used for comparisons between hospitals has been validated, and our study results have implications with regard to comparing hospital-level performance. HCAHPS results are relevant to policy and have financial consequences.17 Fourth, our study did not directly compare whether the relationship between patient experience for the postreadmission group and nonreadmitted group was different from the relationship between the pre-readmission group and postreadmission group. It is possible that there is no difference in relationship between the groups. However, despite the small number of pre-readmission responders, these patients tended to have more favorable experience responses than those who responded after being readmitted, even after adjusting for response time. Although the P values are nonsignificant for many comparisons, the directionality of the effect is relatively consistent. Also, the vast majority of the patients fall in the postreadmission group, and these patients appear to drive the overall experience related to readmissions. Finally, since relatively few patients turned in surveys prior to readmission, we had limited power to detect a significant difference between these pre-readmission responders and nonreadmitted patients.
Our study has implications for policy makers, researchers, and providers. The HCAHPS scores of patients who are readmitted and completed the survey after being readmitted reflects their experience of both the index admission and the readmission. We did not find evidence to support that HCAHPS survey responses predict future readmissions at the patient level. Our findings do support the concept that lower readmissions rates (whether due to the patient population or processes of care that decrease readmission rates) may improve HCAHPS scores. We suggest caution in assuming that improving patient experience is likely to reduce readmission rates.
Disclosures
The authors declare no conflicts of interest.
1. Hospital value-based purchasing. https://www.cms.gov/Outreach-and-Education/Medicare-Learning-Network-MLN/MLNProducts/downloads/Hospital_VBPurchasing_Fact_Sheet_ICN907664.pdf. Accessed June 25, 2016.
2. Readmissions reduction program (HRRP). Centers for Medicare & Medicaid Services. https://www.cms.gov/medicare/medicare-fee-for-service-payment/acuteinpatientpps/readmissions-reduction-program.html. Accessed June 25, 2016.
3. Boulding W, Glickman SW, Manary MP, Schulman KA, Staelin R. Relationship between patient satisfaction with inpatient care and hospital readmission within 30 days. Am J Manag Care. 2011;17(1):41-48. PubMed
4. Buum HA, Duran-Nelson AM, Menk J, Nixon LJ. Duty-hours monitoring revisited: self-report may not be adequate. Am J Med. 2013;126(4):362-365. doi: 10.1016/j.amjmed.2012.12.003 PubMed
5. Choma NN, Vasilevskis EE, Sponsler KC, Hathaway J, Kripalani S. Effect of the ACGME 16-hour rule on efficiency and quality of care: duty hours 2.0. JAMA Int Med. 2013;173(9):819-821. doi: 10.1001/jamainternmed.2013.3014 PubMed
6. Brooke BS, Samourjian E, Sarfati MR, Nguyen TT, Greer D, Kraiss LW. RR3. Patient-reported readiness at time of discharge predicts readmission following vascular surgery. J Vasc Surg. 2015;61(6):188S. doi: 10.1016/j.jvs.2015.04.356
7. Duraes LC, Merlino J, Stocchi L, et al. 756 readmission decreases patient satisfaction in colorectal surgery. Gastroenterology. 2014;146(5):S-1029. doi: 10.1016/S0016-5085(14)63751-3
8. Mitchell JP. Association of provider communication and discharge instructions on lower readmissions. J Healthc Qual. 2015;37(1):33-40. doi: 10.1097/01.JHQ.0000460126.88382.13 PubMed
9. Tsai TC, Orav EJ, Jha AK. Patient satisfaction and quality of surgical care in US hospitals. Ann Surg. 2015;261(1):2-8. doi: 10.1097/SLA.0000000000000765 PubMed
10. Hachem F, Canar J, Fullam M, Andrew S, Hohmann S, Johnson C. The relationships between HCAHPS communication and discharge satisfaction items and hospital readmissions. Patient Exp J. 2014;1(2):71-77.
11. Irby DM, Cooke M, Lowenstein D, Richards B. The academy movement: a structural approach to reinvigorating the educational mission. Acad Med. 2004;79(8):729-736. doi: 10.1097/00001888-200408000-00003 PubMed
12. Siddiqui ZK, Zuccarelli R, Durkin N, Wu AW, Brotman DJ. Changes in patient satisfaction related to hospital renovation: experience with a new clinical building. J Hosp Med. 2015;10(3):165-171. doi: 10.1002/jhm.2297 PubMed
13. Nair BR, Coughlan JL, Hensley MJ. Student and patient perspectives on bedside teaching. Med Educ. 1997;31(5):341-346. doi: 10.1046/j.1365-2923.1997.00673.x PubMed
14. Elliott MN, Zaslavsky AM, Goldstein E, et al. Effects of survey mode, patient mix, and nonresponse on CAHPS® hospital survey scores. BMC Health Serv Res. 2009;44(2p1):501-518. doi: 10.1111/j.1475-6773.2008.00914.x PubMed
15. Saunders CL, Elliott MN, Lyratzopoulos G, Abel GA. Do differential response rates to patient surveys between organizations lead to unfair performance comparisons?: evidence from the English Cancer Patient Experience Survey. Medical care. 2016;54(1):45. doi: 10.1097/MLR.0000000000000457 PubMed
16. Sabel E, Archer J. “Medical education is the ugly duckling of the medical world” and other challenges to medical educators’ identity construction: a qualitative study. Acad Med. 2014;89(11):1474-1480. doi: 10.1097/ACM.0000000000000420 PubMed
17. O’Malley AJ, Zaslavsky AM, Elliott MN, Zaborski L, Cleary PD. Case‐Mix adjustment of the CAHPS® Hospital Survey. BMC Health Serv Res. 2005;40(6p2):2162-2181. doi: 10.1111/j.1475-6773.2005.00470.x
Patient experience and 30-day readmission are important measures of quality of care for hospitalized patients. Performance on both of these measures impact hospitals financially. Performance on the Hospital Consumer Assessment of Healthcare Systems and Providers (HCAHPS) survey is linked to 25% of the incentive payment under Value Based Purchasing (VBP) Program.1 Starting in 2012, the Centers for Medicare and Medicaid Services (CMS) introduced the Readmission Reduction Program, penalizing hospitals financially for excessive readmissions.2
A relationship between patient experience and readmissions has been explored at the hospital level. Studies have mostly found that higher patient experience scores are associated with lower 30-day readmission rates. In a study of the relationship between 30-day risk-standardized readmission rates for three medical conditions (acute myocardial infarction, heart failure, and pneumonia) and patient experience, the authors noted that higher experience scores for overall care and discharge planning were associated with lower readmission rates for these conditions. They also concluded that patient experience scores were more predictive of 30-day readmission than clinical performance measures. Additionally, the authors predicted that if a hospital increased its total experience scores from the 25th percentile to the 75th percentile, there would be an associated decrease in readmissions by at least 2.3% for each of these conditions.3 Practice management companies and the media have cited this finding to conclude that higher patient experience drives clinical outcomes such as 30-day readmission and that patients are often the best judges of the quality of care delivered.4,5
Other hospital-level studies have found that high 30-day readmission rates are associated with lower overall experience scores in a mixed surgical patient population; worse reports of pain control and overall care in the colorectal surgery population; lower experience scores with discharge preparedness in vascular surgery patients; and lower experience scores with physician communication, nurse communication, and discharge preparedness.6-9 A patient-level study noted higher readmissions are associated with worse experience with physician and nursing communication along with a paradoxically better experience with discharge information.10
Because these studies used an observational design, they demonstrated associations rather than causality. An alternative hypothesis is that readmitted patients complete their patient experience survey after readmission and the low experience is the result, rather than the cause, of their readmission. For patients who are readmitted, it is unclear whether there is an opportunity to complete the survey prior to readmission and whether being readmitted may impact patient perception of quality of care. Using patient-level data, we sought to assess HCAHPS patient-experience responses linked to the index admission of the patients who were readmitted in 30 days and compare it with those patients who were not readmitted during this time period. We paid particular attention to when the surveys were returned.
METHODS
Study Design
We conducted a retrospective analysis of prospectively collected 10-year HCAHPS and Press Ganey patient survey data for a single tertiary care academic hospital.
Participants
All adult patients discharged from the hospital and who responded to the routinely sent patient-experience survey were included. Surveys were sent to a random sample of 50% of the discharged patients.
The exposure group was comprised of patients who responded to the survey and were readmitted within 30 days of discharge. After subtracting 5 days from the survey receipt date for expected delays related to mail delivery time and processing time, survey response date was calculated. The exposure group was further divided into patients who responded to the survey prior to their 30-day readmission (“Pre-readmission responders”) and those that responded to the survey after their readmission (“Postreadmission responders”). A sensitivity analysis was performed by changing the number of days subtracted from the survey receipt date by 2 days in either direction. This approach did not result in any significant changes in the results.
The control group comprised patients who were not readmitted to the hospital within 30 days of discharge and who did not have an admission in the previous 30 days as well (“Not readmitted” group). An additional comparison group for exploratory analysis included patients who had experienced an admission in the prior 30 days but were not readmitted after the admission linked to the survey. These patients responded to the patient-experience surveys that were linked to their second admission in 30 days (“2nd-admission responders” group; Figure).
Time Periods
All survey responders from the third quarter of 2006 to the first quarter of 2016 were included in the study. Additionally, administrative data on non-responders were available from 7/2006 to 8/2012. These data were used to estimate response rates. Patient level experience and administrative data were obtained in a linked fashion for these time periods.
Instruments
Press Ganey and HCAHPS surveys were sent via mail in the same envelope. Fifty percent of the discharged patients were randomized to receive the surveys. The Press Ganey survey contained 33 items encompassing several subdomains, including room, meal, nursing, physician, ancillary staff, visitor, discharge, and overall experience.
The HCAHPS survey contained 29 CMS-mandated items, of which 21 are related to patient experience. The development, testing, and methods for administration and reporting of the HCAHPS survey have been previously described and studies using this instrument have been reported in the literature.11 Press Ganey patient satisfaction survey results have also been reported in the literature.12
Outcome Variables and Covariates
HCAHPS and Press Ganey experience survey individual item responses were the primary outcome variables of this study. Age, self-reported health status, education, primary language spoken, service line, and time taken to respond to the surveys served as the covariates. These variables are used by CMS for patient-mix adjustment and are collected on the HCAHPS survey. Additionally, the number of days to respond to the survey were included in all regression analysis to adjust for early responder effect.13-15
Statistical Analysis
“Percent top-box” scores were calculated for each survey item for patients in each group. The percent top-box scores were calculated as the percent of patients who responded “very good” for a given item on Press Ganey survey items and “always” or “definitely yes” or “yes” or “9” or “10” on HCAHPS survey items. CMS utilizes “percent top-box scores” to calculate payments under the VBP program and to report the results publicly. Numerous studies have also reported percent top-box scores for HCAHPS survey results.12
We hypothesized that whether patients complete the HCAHPS survey before or after the readmission influences their reporting of experience. To test this hypothesis, HCAHPS and Press Ganey item top-box scores of “Pre-readmission responders” and “Postreadmission responders” were compared with those of the control group using multivariate logistic regression. “Pre-readmission responders” were also compared with “Postreadmission responders”.
“2nd-admission responders” were similarly compared with the control group for an exploratory analysis. Finally, “Postreadmission responders” and “2nd-admission responders” were compared in another exploratory analysis since both these groups responded to the survey after being exposed to the readmission, even though the “Postreadmission responders” group is administratively linked to the index admission.
The Johns Hopkins Institutional Review Board approved this study.
RESULTS
There were 43,737 survey responders, among whom 4,707 were subsequently readmitted within 30 days of discharge. Among the readmitted patients who responded to the surveys linked to their index admission, only 15.8% returned the survey before readmission (pre-readmission responders’) and 84.2% returned the survey after readmission (postreadmission responders). Additionally, 1,663 patients responded to experience surveys linked to their readmission. There were 37,365 patients in the control arm (ie, patients who responded to the survey and were not readmitted within 30 days of discharge or in the prior 30 days; Figure 1). The readmission rate among survey responders was 10.6%. Among the readmitted patients, the median number of days to readmission was 10 days while the median number of days to respond to the survey for this group was 33 days. Among the nonreadmitted patients, the median number of days to return the survey was 29 days.
We also conducted an exploratory analysis of the postreadmission responders, comparing them with patients who received patient-experience surveys linked to their second admission in 30 days. Both of these groups were exposed to a readmission before they completed the surveys. There were no significant differences between these two groups on patient experience scores. Additionally, the patients who received the survey linked to their readmission had a broad dissatisfaction pattern on HCAHPS survey items that appeared similar to that of the postreadmission group when compared to the non-readmitted group (Table 3).
DISCUSSION
In this retrospective analysis of prospectively collected Press Ganey and HCAHPS patient-experience survey data, we found that the overwhelming majority of patients readmitted within 30 days of discharge respond to HCAHPS surveys after readmission even though the survey is sent linked to the first admission. This is not unexpected since the median time to survey response is 33 days for this group, while median time to readmission is 10 days. The dissatisfaction pattern of Postreadmission responders was similar to those who responded to the survey linked to the readmission. When a patient is readmitted prior to completing the survey, their responses appear to reflect the cumulative experience of the index admission and the readmission. The lower scores of those who respond to the survey after their readmission appear to be a driver for lower patient-experience scores related to readmissions. Overall, readmission was associated with lower scores on items in five of the nine domains used to calculate patient experience related payments under VBP.16
These findings have important implications in inferring the direction of potential causal relationship between readmissions and patient experience at the hospital level. Additionally, these patients show broad dissatisfaction with areas beyond physician communication and discharge planning. These include staff responsiveness, phlebotomy, meals, hospital cleanliness, and noise level. This pattern of dissatisfaction may represent impatience and frustration with spending additional time in the hospital environment.
Our results are consistent with findings of many of the earlier studies, but our study goes a step further by using patient-level data and incorporating survey response time in our analysis.3,7,9,10 By separating out the readmitted patients who responded to the survey prior to admission, we attempted to address the ability of patients’ perception of care to predict future readmissions. Our results do not support this idea, since pre-readmission responders had similar experience scores to non-readmitted patients. However, because of the low numbers of pre-readmission responders, the comparison lacks precision. Current HCAHPS and Press Ganey questions may lack the ability to predict future readmissions because of the timing of the survey (postdischarge) or the questions themselves.
Overall, postreadmission responders are dissatisfied with multiple domains of hospital care. Many of these survey responses may simply be related to general frustration. Alternatively, they may represent a patient population with a high degree of needs that are not as easily met by a hospital’s routine processes of care. Even though the readmission rates were 10.6% among survey responders, 14.6% of the survey responses were associated with readmissions after accounting for those who respond to surveys linked to readmission. These patients could have significant impact on cumulative experience scores.
Our study has a few limitations. First, it involves a single tertiary care academic center study, and our results may not be generalizable. Second, we did not adjust for some of the patient characteristics associated with readmissions. Patients who were admitted within 30 days are different than those not readmitted based on payor, race, length of stay, and severity of illness, and we did not adjust for these factors in our analysis. This was intentional, however. Our goal was to better understand the relationship between 30-day readmission and patient experience scores as they are used for hospital-level studies, VBP, and public reporting. For these purposes, the scores are not adjusted for factors, such as payor and length of stay. We did adjust for patient-mix adjustment factors used by CMS. Third, the response rates to the HCAHPS were low and may have biased the scores. However, HCAHPS is widely used for comparisons between hospitals has been validated, and our study results have implications with regard to comparing hospital-level performance. HCAHPS results are relevant to policy and have financial consequences.17 Fourth, our study did not directly compare whether the relationship between patient experience for the postreadmission group and nonreadmitted group was different from the relationship between the pre-readmission group and postreadmission group. It is possible that there is no difference in relationship between the groups. However, despite the small number of pre-readmission responders, these patients tended to have more favorable experience responses than those who responded after being readmitted, even after adjusting for response time. Although the P values are nonsignificant for many comparisons, the directionality of the effect is relatively consistent. Also, the vast majority of the patients fall in the postreadmission group, and these patients appear to drive the overall experience related to readmissions. Finally, since relatively few patients turned in surveys prior to readmission, we had limited power to detect a significant difference between these pre-readmission responders and nonreadmitted patients.
Our study has implications for policy makers, researchers, and providers. The HCAHPS scores of patients who are readmitted and completed the survey after being readmitted reflects their experience of both the index admission and the readmission. We did not find evidence to support that HCAHPS survey responses predict future readmissions at the patient level. Our findings do support the concept that lower readmissions rates (whether due to the patient population or processes of care that decrease readmission rates) may improve HCAHPS scores. We suggest caution in assuming that improving patient experience is likely to reduce readmission rates.
Disclosures
The authors declare no conflicts of interest.
Patient experience and 30-day readmission are important measures of quality of care for hospitalized patients. Performance on both of these measures impact hospitals financially. Performance on the Hospital Consumer Assessment of Healthcare Systems and Providers (HCAHPS) survey is linked to 25% of the incentive payment under Value Based Purchasing (VBP) Program.1 Starting in 2012, the Centers for Medicare and Medicaid Services (CMS) introduced the Readmission Reduction Program, penalizing hospitals financially for excessive readmissions.2
A relationship between patient experience and readmissions has been explored at the hospital level. Studies have mostly found that higher patient experience scores are associated with lower 30-day readmission rates. In a study of the relationship between 30-day risk-standardized readmission rates for three medical conditions (acute myocardial infarction, heart failure, and pneumonia) and patient experience, the authors noted that higher experience scores for overall care and discharge planning were associated with lower readmission rates for these conditions. They also concluded that patient experience scores were more predictive of 30-day readmission than clinical performance measures. Additionally, the authors predicted that if a hospital increased its total experience scores from the 25th percentile to the 75th percentile, there would be an associated decrease in readmissions by at least 2.3% for each of these conditions.3 Practice management companies and the media have cited this finding to conclude that higher patient experience drives clinical outcomes such as 30-day readmission and that patients are often the best judges of the quality of care delivered.4,5
Other hospital-level studies have found that high 30-day readmission rates are associated with lower overall experience scores in a mixed surgical patient population; worse reports of pain control and overall care in the colorectal surgery population; lower experience scores with discharge preparedness in vascular surgery patients; and lower experience scores with physician communication, nurse communication, and discharge preparedness.6-9 A patient-level study noted higher readmissions are associated with worse experience with physician and nursing communication along with a paradoxically better experience with discharge information.10
Because these studies used an observational design, they demonstrated associations rather than causality. An alternative hypothesis is that readmitted patients complete their patient experience survey after readmission and the low experience is the result, rather than the cause, of their readmission. For patients who are readmitted, it is unclear whether there is an opportunity to complete the survey prior to readmission and whether being readmitted may impact patient perception of quality of care. Using patient-level data, we sought to assess HCAHPS patient-experience responses linked to the index admission of the patients who were readmitted in 30 days and compare it with those patients who were not readmitted during this time period. We paid particular attention to when the surveys were returned.
METHODS
Study Design
We conducted a retrospective analysis of prospectively collected 10-year HCAHPS and Press Ganey patient survey data for a single tertiary care academic hospital.
Participants
All adult patients discharged from the hospital and who responded to the routinely sent patient-experience survey were included. Surveys were sent to a random sample of 50% of the discharged patients.
The exposure group was comprised of patients who responded to the survey and were readmitted within 30 days of discharge. After subtracting 5 days from the survey receipt date for expected delays related to mail delivery time and processing time, survey response date was calculated. The exposure group was further divided into patients who responded to the survey prior to their 30-day readmission (“Pre-readmission responders”) and those that responded to the survey after their readmission (“Postreadmission responders”). A sensitivity analysis was performed by changing the number of days subtracted from the survey receipt date by 2 days in either direction. This approach did not result in any significant changes in the results.
The control group comprised patients who were not readmitted to the hospital within 30 days of discharge and who did not have an admission in the previous 30 days as well (“Not readmitted” group). An additional comparison group for exploratory analysis included patients who had experienced an admission in the prior 30 days but were not readmitted after the admission linked to the survey. These patients responded to the patient-experience surveys that were linked to their second admission in 30 days (“2nd-admission responders” group; Figure).
Time Periods
All survey responders from the third quarter of 2006 to the first quarter of 2016 were included in the study. Additionally, administrative data on non-responders were available from 7/2006 to 8/2012. These data were used to estimate response rates. Patient level experience and administrative data were obtained in a linked fashion for these time periods.
Instruments
Press Ganey and HCAHPS surveys were sent via mail in the same envelope. Fifty percent of the discharged patients were randomized to receive the surveys. The Press Ganey survey contained 33 items encompassing several subdomains, including room, meal, nursing, physician, ancillary staff, visitor, discharge, and overall experience.
The HCAHPS survey contained 29 CMS-mandated items, of which 21 are related to patient experience. The development, testing, and methods for administration and reporting of the HCAHPS survey have been previously described and studies using this instrument have been reported in the literature.11 Press Ganey patient satisfaction survey results have also been reported in the literature.12
Outcome Variables and Covariates
HCAHPS and Press Ganey experience survey individual item responses were the primary outcome variables of this study. Age, self-reported health status, education, primary language spoken, service line, and time taken to respond to the surveys served as the covariates. These variables are used by CMS for patient-mix adjustment and are collected on the HCAHPS survey. Additionally, the number of days to respond to the survey were included in all regression analysis to adjust for early responder effect.13-15
Statistical Analysis
“Percent top-box” scores were calculated for each survey item for patients in each group. The percent top-box scores were calculated as the percent of patients who responded “very good” for a given item on Press Ganey survey items and “always” or “definitely yes” or “yes” or “9” or “10” on HCAHPS survey items. CMS utilizes “percent top-box scores” to calculate payments under the VBP program and to report the results publicly. Numerous studies have also reported percent top-box scores for HCAHPS survey results.12
We hypothesized that whether patients complete the HCAHPS survey before or after the readmission influences their reporting of experience. To test this hypothesis, HCAHPS and Press Ganey item top-box scores of “Pre-readmission responders” and “Postreadmission responders” were compared with those of the control group using multivariate logistic regression. “Pre-readmission responders” were also compared with “Postreadmission responders”.
“2nd-admission responders” were similarly compared with the control group for an exploratory analysis. Finally, “Postreadmission responders” and “2nd-admission responders” were compared in another exploratory analysis since both these groups responded to the survey after being exposed to the readmission, even though the “Postreadmission responders” group is administratively linked to the index admission.
The Johns Hopkins Institutional Review Board approved this study.
RESULTS
There were 43,737 survey responders, among whom 4,707 were subsequently readmitted within 30 days of discharge. Among the readmitted patients who responded to the surveys linked to their index admission, only 15.8% returned the survey before readmission (pre-readmission responders’) and 84.2% returned the survey after readmission (postreadmission responders). Additionally, 1,663 patients responded to experience surveys linked to their readmission. There were 37,365 patients in the control arm (ie, patients who responded to the survey and were not readmitted within 30 days of discharge or in the prior 30 days; Figure 1). The readmission rate among survey responders was 10.6%. Among the readmitted patients, the median number of days to readmission was 10 days while the median number of days to respond to the survey for this group was 33 days. Among the nonreadmitted patients, the median number of days to return the survey was 29 days.
We also conducted an exploratory analysis of the postreadmission responders, comparing them with patients who received patient-experience surveys linked to their second admission in 30 days. Both of these groups were exposed to a readmission before they completed the surveys. There were no significant differences between these two groups on patient experience scores. Additionally, the patients who received the survey linked to their readmission had a broad dissatisfaction pattern on HCAHPS survey items that appeared similar to that of the postreadmission group when compared to the non-readmitted group (Table 3).
DISCUSSION
In this retrospective analysis of prospectively collected Press Ganey and HCAHPS patient-experience survey data, we found that the overwhelming majority of patients readmitted within 30 days of discharge respond to HCAHPS surveys after readmission even though the survey is sent linked to the first admission. This is not unexpected since the median time to survey response is 33 days for this group, while median time to readmission is 10 days. The dissatisfaction pattern of Postreadmission responders was similar to those who responded to the survey linked to the readmission. When a patient is readmitted prior to completing the survey, their responses appear to reflect the cumulative experience of the index admission and the readmission. The lower scores of those who respond to the survey after their readmission appear to be a driver for lower patient-experience scores related to readmissions. Overall, readmission was associated with lower scores on items in five of the nine domains used to calculate patient experience related payments under VBP.16
These findings have important implications in inferring the direction of potential causal relationship between readmissions and patient experience at the hospital level. Additionally, these patients show broad dissatisfaction with areas beyond physician communication and discharge planning. These include staff responsiveness, phlebotomy, meals, hospital cleanliness, and noise level. This pattern of dissatisfaction may represent impatience and frustration with spending additional time in the hospital environment.
Our results are consistent with findings of many of the earlier studies, but our study goes a step further by using patient-level data and incorporating survey response time in our analysis.3,7,9,10 By separating out the readmitted patients who responded to the survey prior to admission, we attempted to address the ability of patients’ perception of care to predict future readmissions. Our results do not support this idea, since pre-readmission responders had similar experience scores to non-readmitted patients. However, because of the low numbers of pre-readmission responders, the comparison lacks precision. Current HCAHPS and Press Ganey questions may lack the ability to predict future readmissions because of the timing of the survey (postdischarge) or the questions themselves.
Overall, postreadmission responders are dissatisfied with multiple domains of hospital care. Many of these survey responses may simply be related to general frustration. Alternatively, they may represent a patient population with a high degree of needs that are not as easily met by a hospital’s routine processes of care. Even though the readmission rates were 10.6% among survey responders, 14.6% of the survey responses were associated with readmissions after accounting for those who respond to surveys linked to readmission. These patients could have significant impact on cumulative experience scores.
Our study has a few limitations. First, it involves a single tertiary care academic center study, and our results may not be generalizable. Second, we did not adjust for some of the patient characteristics associated with readmissions. Patients who were admitted within 30 days are different than those not readmitted based on payor, race, length of stay, and severity of illness, and we did not adjust for these factors in our analysis. This was intentional, however. Our goal was to better understand the relationship between 30-day readmission and patient experience scores as they are used for hospital-level studies, VBP, and public reporting. For these purposes, the scores are not adjusted for factors, such as payor and length of stay. We did adjust for patient-mix adjustment factors used by CMS. Third, the response rates to the HCAHPS were low and may have biased the scores. However, HCAHPS is widely used for comparisons between hospitals has been validated, and our study results have implications with regard to comparing hospital-level performance. HCAHPS results are relevant to policy and have financial consequences.17 Fourth, our study did not directly compare whether the relationship between patient experience for the postreadmission group and nonreadmitted group was different from the relationship between the pre-readmission group and postreadmission group. It is possible that there is no difference in relationship between the groups. However, despite the small number of pre-readmission responders, these patients tended to have more favorable experience responses than those who responded after being readmitted, even after adjusting for response time. Although the P values are nonsignificant for many comparisons, the directionality of the effect is relatively consistent. Also, the vast majority of the patients fall in the postreadmission group, and these patients appear to drive the overall experience related to readmissions. Finally, since relatively few patients turned in surveys prior to readmission, we had limited power to detect a significant difference between these pre-readmission responders and nonreadmitted patients.
Our study has implications for policy makers, researchers, and providers. The HCAHPS scores of patients who are readmitted and completed the survey after being readmitted reflects their experience of both the index admission and the readmission. We did not find evidence to support that HCAHPS survey responses predict future readmissions at the patient level. Our findings do support the concept that lower readmissions rates (whether due to the patient population or processes of care that decrease readmission rates) may improve HCAHPS scores. We suggest caution in assuming that improving patient experience is likely to reduce readmission rates.
Disclosures
The authors declare no conflicts of interest.
1. Hospital value-based purchasing. https://www.cms.gov/Outreach-and-Education/Medicare-Learning-Network-MLN/MLNProducts/downloads/Hospital_VBPurchasing_Fact_Sheet_ICN907664.pdf. Accessed June 25, 2016.
2. Readmissions reduction program (HRRP). Centers for Medicare & Medicaid Services. https://www.cms.gov/medicare/medicare-fee-for-service-payment/acuteinpatientpps/readmissions-reduction-program.html. Accessed June 25, 2016.
3. Boulding W, Glickman SW, Manary MP, Schulman KA, Staelin R. Relationship between patient satisfaction with inpatient care and hospital readmission within 30 days. Am J Manag Care. 2011;17(1):41-48. PubMed
4. Buum HA, Duran-Nelson AM, Menk J, Nixon LJ. Duty-hours monitoring revisited: self-report may not be adequate. Am J Med. 2013;126(4):362-365. doi: 10.1016/j.amjmed.2012.12.003 PubMed
5. Choma NN, Vasilevskis EE, Sponsler KC, Hathaway J, Kripalani S. Effect of the ACGME 16-hour rule on efficiency and quality of care: duty hours 2.0. JAMA Int Med. 2013;173(9):819-821. doi: 10.1001/jamainternmed.2013.3014 PubMed
6. Brooke BS, Samourjian E, Sarfati MR, Nguyen TT, Greer D, Kraiss LW. RR3. Patient-reported readiness at time of discharge predicts readmission following vascular surgery. J Vasc Surg. 2015;61(6):188S. doi: 10.1016/j.jvs.2015.04.356
7. Duraes LC, Merlino J, Stocchi L, et al. 756 readmission decreases patient satisfaction in colorectal surgery. Gastroenterology. 2014;146(5):S-1029. doi: 10.1016/S0016-5085(14)63751-3
8. Mitchell JP. Association of provider communication and discharge instructions on lower readmissions. J Healthc Qual. 2015;37(1):33-40. doi: 10.1097/01.JHQ.0000460126.88382.13 PubMed
9. Tsai TC, Orav EJ, Jha AK. Patient satisfaction and quality of surgical care in US hospitals. Ann Surg. 2015;261(1):2-8. doi: 10.1097/SLA.0000000000000765 PubMed
10. Hachem F, Canar J, Fullam M, Andrew S, Hohmann S, Johnson C. The relationships between HCAHPS communication and discharge satisfaction items and hospital readmissions. Patient Exp J. 2014;1(2):71-77.
11. Irby DM, Cooke M, Lowenstein D, Richards B. The academy movement: a structural approach to reinvigorating the educational mission. Acad Med. 2004;79(8):729-736. doi: 10.1097/00001888-200408000-00003 PubMed
12. Siddiqui ZK, Zuccarelli R, Durkin N, Wu AW, Brotman DJ. Changes in patient satisfaction related to hospital renovation: experience with a new clinical building. J Hosp Med. 2015;10(3):165-171. doi: 10.1002/jhm.2297 PubMed
13. Nair BR, Coughlan JL, Hensley MJ. Student and patient perspectives on bedside teaching. Med Educ. 1997;31(5):341-346. doi: 10.1046/j.1365-2923.1997.00673.x PubMed
14. Elliott MN, Zaslavsky AM, Goldstein E, et al. Effects of survey mode, patient mix, and nonresponse on CAHPS® hospital survey scores. BMC Health Serv Res. 2009;44(2p1):501-518. doi: 10.1111/j.1475-6773.2008.00914.x PubMed
15. Saunders CL, Elliott MN, Lyratzopoulos G, Abel GA. Do differential response rates to patient surveys between organizations lead to unfair performance comparisons?: evidence from the English Cancer Patient Experience Survey. Medical care. 2016;54(1):45. doi: 10.1097/MLR.0000000000000457 PubMed
16. Sabel E, Archer J. “Medical education is the ugly duckling of the medical world” and other challenges to medical educators’ identity construction: a qualitative study. Acad Med. 2014;89(11):1474-1480. doi: 10.1097/ACM.0000000000000420 PubMed
17. O’Malley AJ, Zaslavsky AM, Elliott MN, Zaborski L, Cleary PD. Case‐Mix adjustment of the CAHPS® Hospital Survey. BMC Health Serv Res. 2005;40(6p2):2162-2181. doi: 10.1111/j.1475-6773.2005.00470.x
1. Hospital value-based purchasing. https://www.cms.gov/Outreach-and-Education/Medicare-Learning-Network-MLN/MLNProducts/downloads/Hospital_VBPurchasing_Fact_Sheet_ICN907664.pdf. Accessed June 25, 2016.
2. Readmissions reduction program (HRRP). Centers for Medicare & Medicaid Services. https://www.cms.gov/medicare/medicare-fee-for-service-payment/acuteinpatientpps/readmissions-reduction-program.html. Accessed June 25, 2016.
3. Boulding W, Glickman SW, Manary MP, Schulman KA, Staelin R. Relationship between patient satisfaction with inpatient care and hospital readmission within 30 days. Am J Manag Care. 2011;17(1):41-48. PubMed
4. Buum HA, Duran-Nelson AM, Menk J, Nixon LJ. Duty-hours monitoring revisited: self-report may not be adequate. Am J Med. 2013;126(4):362-365. doi: 10.1016/j.amjmed.2012.12.003 PubMed
5. Choma NN, Vasilevskis EE, Sponsler KC, Hathaway J, Kripalani S. Effect of the ACGME 16-hour rule on efficiency and quality of care: duty hours 2.0. JAMA Int Med. 2013;173(9):819-821. doi: 10.1001/jamainternmed.2013.3014 PubMed
6. Brooke BS, Samourjian E, Sarfati MR, Nguyen TT, Greer D, Kraiss LW. RR3. Patient-reported readiness at time of discharge predicts readmission following vascular surgery. J Vasc Surg. 2015;61(6):188S. doi: 10.1016/j.jvs.2015.04.356
7. Duraes LC, Merlino J, Stocchi L, et al. 756 readmission decreases patient satisfaction in colorectal surgery. Gastroenterology. 2014;146(5):S-1029. doi: 10.1016/S0016-5085(14)63751-3
8. Mitchell JP. Association of provider communication and discharge instructions on lower readmissions. J Healthc Qual. 2015;37(1):33-40. doi: 10.1097/01.JHQ.0000460126.88382.13 PubMed
9. Tsai TC, Orav EJ, Jha AK. Patient satisfaction and quality of surgical care in US hospitals. Ann Surg. 2015;261(1):2-8. doi: 10.1097/SLA.0000000000000765 PubMed
10. Hachem F, Canar J, Fullam M, Andrew S, Hohmann S, Johnson C. The relationships between HCAHPS communication and discharge satisfaction items and hospital readmissions. Patient Exp J. 2014;1(2):71-77.
11. Irby DM, Cooke M, Lowenstein D, Richards B. The academy movement: a structural approach to reinvigorating the educational mission. Acad Med. 2004;79(8):729-736. doi: 10.1097/00001888-200408000-00003 PubMed
12. Siddiqui ZK, Zuccarelli R, Durkin N, Wu AW, Brotman DJ. Changes in patient satisfaction related to hospital renovation: experience with a new clinical building. J Hosp Med. 2015;10(3):165-171. doi: 10.1002/jhm.2297 PubMed
13. Nair BR, Coughlan JL, Hensley MJ. Student and patient perspectives on bedside teaching. Med Educ. 1997;31(5):341-346. doi: 10.1046/j.1365-2923.1997.00673.x PubMed
14. Elliott MN, Zaslavsky AM, Goldstein E, et al. Effects of survey mode, patient mix, and nonresponse on CAHPS® hospital survey scores. BMC Health Serv Res. 2009;44(2p1):501-518. doi: 10.1111/j.1475-6773.2008.00914.x PubMed
15. Saunders CL, Elliott MN, Lyratzopoulos G, Abel GA. Do differential response rates to patient surveys between organizations lead to unfair performance comparisons?: evidence from the English Cancer Patient Experience Survey. Medical care. 2016;54(1):45. doi: 10.1097/MLR.0000000000000457 PubMed
16. Sabel E, Archer J. “Medical education is the ugly duckling of the medical world” and other challenges to medical educators’ identity construction: a qualitative study. Acad Med. 2014;89(11):1474-1480. doi: 10.1097/ACM.0000000000000420 PubMed
17. O’Malley AJ, Zaslavsky AM, Elliott MN, Zaborski L, Cleary PD. Case‐Mix adjustment of the CAHPS® Hospital Survey. BMC Health Serv Res. 2005;40(6p2):2162-2181. doi: 10.1111/j.1475-6773.2005.00470.x
© 2018 Society of Hospital Medicine
Patient Perceptions of Readmission Risk: An Exploratory Survey
Recent years have seen a proliferation of programs designed to prevent readmissions, including patient education initiatives, financial assistance programs, postdischarge services, and clinical personnel assigned to help patients navigate their posthospitalization clinical care. Although some strategies do not require direct patient participation (such as timely and effective handoffs between inpatient and outpatient care teams), many rely upon a commitment by the patient to participate in the postdischarge care plan. At our hospital, we have found that only about 2/3 of patients who are offered transitional interventions (such as postdischarge phone calls by nurses or home nursing through a “transition guide” program) receive the intended interventions, and those who do not receive them are more likely to be readmitted.1 While limited patient uptake may relate, in part, to factors that are difficult to overcome, such as inadequate housing or phone service, we have also encountered patients whose values, beliefs, or preferences about their care do not align with those of the care team. The purposes of this exploratory study were to (1) assess patient attitudes surrounding readmission, (2) ascertain whether these attitudes are associated with actual readmission, and (3) determine whether patients can estimate their own risk of readmission.
METHODS
From January 2014 to September 2016, we circulated surveys to patients on internal medicine nursing units who were being discharged home within 24 hours. Blank surveys were distributed to nursing units by the researchers. Unit clerks and support staff were educated on the purpose of the project and asked to distribute surveys to patients who were identified by unit case managers or nurses as slated for discharge. Staff members were not asked to help with or supervise survey completion. Surveys were generally filled out by patients, but we allowed family members to assist patients if needed, and to indicate so with a checkbox. There were no exclusion criteria. Because surveys were distributed by clinical staff, the received surveys can be considered a convenience sample. Patients were asked 5 questions with 4- or 5-point Likert scale responses:
(1) “How likely is it that you will be admitted to the hospital (have to stay in the hospital overnight) again within the next 30 days after you leave the hospital this time?” [answers ranging from “Very Unlikely (<5% chance)” to “Very Likely (>50% chance)”];
(2) “How would you feel about being rehospitalized in the next month?” [answers ranging from “Very sad, frustrated, or disappointed” to “Very happy or relieved”];
(3) “How much do you think that you personally can control whether or not you will be rehospitalized (based on what you do to take care of your body, take your medicines, and follow-up with your healthcare team)?” [answers ranging from “I have no control over whether I will be rehospitalized” to “I have complete control over whether I will be rehospitalized”];
(4) “Which of the options below best describes how you plan to follow the medical instructions after you leave the hospital?” [answers ranging from “I do NOT plan to do very much of what I am being asked to do by the doctors, nurses, therapists, and other members of the care team” to “I plan to do EVERYTHING I am being asked to do by the doctors, nurses, therapists and other members of the care team”]; and
(5) “Pick the item below that best describes YOUR OWN VIEW of the care team’s recommendations:” [answers ranging from “I DO NOT AGREE AT ALL that the best way to be healthy is to do exactly what I am being asked to do by the doctors, nurses, therapists, and other members of the care team” to “I FULLY AGREE that the best way to be healthy is to do exactly what I am being asked to do by the doctors, nurses, therapists, and other members of the care team”].
Responses were linked, based on discharge date and medical record number, to administrative data, including age, sex, race, payer, and clinical data. Subsequent hospitalizations to our hospital were ascertained from administrative data. We estimated expected risk of readmission using the all payer refined diagnosis related group coupled with the associated severity-of-illness (SOI) score, as we have reported previously.2-5 We restricted our analysis to patients who answered the question related to the likelihood of readmission. Logistic regression models were constructed using actual 30-day readmission as the dependent variable to determine whether patients could predict their own readmissions and whether patient attitudes and beliefs about their care were predictive of subsequent readmission. Patient survey responses were entered as continuous independent variables (ranging from 1-4 or 1-5, as appropriate). Multivariable logistic regression was used to determine whether patients could predict their readmissions independent of demographic variables and expected readmission rate (modeled continuously); we repeated this model after dichotomizing the patient’s estimate of the likelihood of readmission as either “unlikely” or “likely.” Patients with missing survey responses were excluded from individual models without imputation. The study was approved by the Johns Hopkins institutional review board.
RESULTS
Responses were obtained from 895 patients. Their median age was 56 years [interquartile range, 43-67], 51.4% were female, and 41.7% were white. Mean SOI was 2.53 (on a 1-4 scale), and median length-of-stay was representative for our medical service at 5.2 days (range, 1-66 days). Family members reported filling out the survey in 57 cases. The primary payer was Medicare in 40.7%, Medicaid in 24.9%, and other in 34.4%. A total of 138 patients (15.4%) were readmitted within 30 days. The Table shows survey responses and associated readmission rates. None of the attitudes related to readmission were predictive of actual readmission. However, patients were able to predict their own readmissions (P = .002 for linear trend). After adjustment for expected readmission rate, race, sex, age, and payer, the trend remained significant (P = .005). Other significant predictors of readmissions in this model included expected readmission rate (P = .002), age (P = .02), and payer (P = .002). After dichotomizing the patient estimate of readmission rate as “unlikely” (N = 581) or “likely” (N = 314), the unadjusted odds ratio associating a patient-estimated risk of readmission as “likely” with actual readmission was 1.8 (95% confidence interval, 1.2-2.5). The adjusted odds ratio (including the variables above) was 1.6 (1.1-2.4).
DISCUSSION
Our findings demonstrate that patients are able to quantify their own readmission risk. This was true even after adjustment for expected readmission rate, age, sex, race, and payer. However, we did not identify any patient attitudes, beliefs, or preferences related to readmission or discharge instructions that were associated with subsequent rehospitalization. Reassuringly, more than 80% of patients who responded to the survey indicated that they would be sad, frustrated, or disappointed should readmission occur. This suggests that most patients are invested in preventing rehospitalization. Also reassuring was that patients indicated that they agreed with the discharge care plan and intended to follow their discharge instructions.
The major limitation of this study is that it was a convenience sample. Surveys were distributed inconsistently by nursing unit staff, preventing us from calculating a response rate. Further, it is possible, if not likely, that those patients with higher levels of engagement were more likely to take the time to respond, enriching our sample with activated patients. Although we allowed family members to fill out surveys on behalf of patients, this was done in fewer than 10% of instances; as such, our data may have limited applicability to patients who are physically or cognitively unable to participate in the discharge process. Finally, in this study, we did not capture readmissions to other facilities.
We conclude that patients are able to predict their own readmissions, even after accounting for other potential predictors of readmission. However, we found no evidence to support the possibility that low levels of engagement, limited trust in the healthcare team, or nonchalance about being readmitted are associated with subsequent rehospitalization. Whether asking patients about their perceived risk of readmission might help target readmission prevention programs deserves further study.
Acknowledgments
Dr. Daniel J. Brotman had full access to the data in the study and takes responsibility for the integrity of the study data and the accuracy of the data analysis. The authors also thank the following individuals for their contributions: Drafting the manuscript (Brotman); revising the manuscript for important intellectual content (Brotman, Shihab, Tieu, Cheng, Bertram, Hoyer, Deutschendorf); acquiring the data (Brotman, Shihab, Tieu, Cheng, Bertram, Deutschendorf); interpreting the data (Brotman, Shihab, Tieu, Cheng, Bertram, Hoyer, Deutschendorf); and analyzing the data (Brotman). The authors thank nursing leadership and nursing unit staff for their assistance in distributing surveys.
Funding support: Johns Hopkins Hospitalist Scholars Program
Disclosures: The authors have declared no conflicts of interest.
1. Hoyer EH, Brotman DJ, Apfel A, et al. Improving outcomes after hospitalization: a prospective observational multi-center evaluation of care-coordination strategies on 30-day readmissions to Maryland hospitals. J Gen Int Med. 2017 (in press). PubMed
2. Oduyebo I, Lehmann CU, Pollack CE, et al. Association of self-reported hospital discharge handoffs with 30-day readmissions. JAMA Intern Med. 2013;173(8):624-629. PubMed
3. Hoyer EH, Needham DM, Atanelov L, Knox B, Friedman M, Brotman DJ. Association of impaired functional status at hospital discharge and subsequent rehospitalization. J Hosp Med. 2014;9(5):277-282. PubMed
4. Hoyer EH, Needham DM, Miller J, Deutschendorf A, Friedman M, Brotman DJ. Functional status impairment is associated with unplanned readmissions. Arch Phys Med Rehabil. 2013;94(10):1951-1958. PubMed
5. Hoyer EH, Odonkor CA, Bhatia SN, Leung C, Deutschendorf A, Brotman DJ. Association between days to complete inpatient discharge summaries with all-payer hospital readmissions in Maryland. J Hosp Med. 2016;11(6):393-400. PubMed
Recent years have seen a proliferation of programs designed to prevent readmissions, including patient education initiatives, financial assistance programs, postdischarge services, and clinical personnel assigned to help patients navigate their posthospitalization clinical care. Although some strategies do not require direct patient participation (such as timely and effective handoffs between inpatient and outpatient care teams), many rely upon a commitment by the patient to participate in the postdischarge care plan. At our hospital, we have found that only about 2/3 of patients who are offered transitional interventions (such as postdischarge phone calls by nurses or home nursing through a “transition guide” program) receive the intended interventions, and those who do not receive them are more likely to be readmitted.1 While limited patient uptake may relate, in part, to factors that are difficult to overcome, such as inadequate housing or phone service, we have also encountered patients whose values, beliefs, or preferences about their care do not align with those of the care team. The purposes of this exploratory study were to (1) assess patient attitudes surrounding readmission, (2) ascertain whether these attitudes are associated with actual readmission, and (3) determine whether patients can estimate their own risk of readmission.
METHODS
From January 2014 to September 2016, we circulated surveys to patients on internal medicine nursing units who were being discharged home within 24 hours. Blank surveys were distributed to nursing units by the researchers. Unit clerks and support staff were educated on the purpose of the project and asked to distribute surveys to patients who were identified by unit case managers or nurses as slated for discharge. Staff members were not asked to help with or supervise survey completion. Surveys were generally filled out by patients, but we allowed family members to assist patients if needed, and to indicate so with a checkbox. There were no exclusion criteria. Because surveys were distributed by clinical staff, the received surveys can be considered a convenience sample. Patients were asked 5 questions with 4- or 5-point Likert scale responses:
(1) “How likely is it that you will be admitted to the hospital (have to stay in the hospital overnight) again within the next 30 days after you leave the hospital this time?” [answers ranging from “Very Unlikely (<5% chance)” to “Very Likely (>50% chance)”];
(2) “How would you feel about being rehospitalized in the next month?” [answers ranging from “Very sad, frustrated, or disappointed” to “Very happy or relieved”];
(3) “How much do you think that you personally can control whether or not you will be rehospitalized (based on what you do to take care of your body, take your medicines, and follow-up with your healthcare team)?” [answers ranging from “I have no control over whether I will be rehospitalized” to “I have complete control over whether I will be rehospitalized”];
(4) “Which of the options below best describes how you plan to follow the medical instructions after you leave the hospital?” [answers ranging from “I do NOT plan to do very much of what I am being asked to do by the doctors, nurses, therapists, and other members of the care team” to “I plan to do EVERYTHING I am being asked to do by the doctors, nurses, therapists and other members of the care team”]; and
(5) “Pick the item below that best describes YOUR OWN VIEW of the care team’s recommendations:” [answers ranging from “I DO NOT AGREE AT ALL that the best way to be healthy is to do exactly what I am being asked to do by the doctors, nurses, therapists, and other members of the care team” to “I FULLY AGREE that the best way to be healthy is to do exactly what I am being asked to do by the doctors, nurses, therapists, and other members of the care team”].
Responses were linked, based on discharge date and medical record number, to administrative data, including age, sex, race, payer, and clinical data. Subsequent hospitalizations to our hospital were ascertained from administrative data. We estimated expected risk of readmission using the all payer refined diagnosis related group coupled with the associated severity-of-illness (SOI) score, as we have reported previously.2-5 We restricted our analysis to patients who answered the question related to the likelihood of readmission. Logistic regression models were constructed using actual 30-day readmission as the dependent variable to determine whether patients could predict their own readmissions and whether patient attitudes and beliefs about their care were predictive of subsequent readmission. Patient survey responses were entered as continuous independent variables (ranging from 1-4 or 1-5, as appropriate). Multivariable logistic regression was used to determine whether patients could predict their readmissions independent of demographic variables and expected readmission rate (modeled continuously); we repeated this model after dichotomizing the patient’s estimate of the likelihood of readmission as either “unlikely” or “likely.” Patients with missing survey responses were excluded from individual models without imputation. The study was approved by the Johns Hopkins institutional review board.
RESULTS
Responses were obtained from 895 patients. Their median age was 56 years [interquartile range, 43-67], 51.4% were female, and 41.7% were white. Mean SOI was 2.53 (on a 1-4 scale), and median length-of-stay was representative for our medical service at 5.2 days (range, 1-66 days). Family members reported filling out the survey in 57 cases. The primary payer was Medicare in 40.7%, Medicaid in 24.9%, and other in 34.4%. A total of 138 patients (15.4%) were readmitted within 30 days. The Table shows survey responses and associated readmission rates. None of the attitudes related to readmission were predictive of actual readmission. However, patients were able to predict their own readmissions (P = .002 for linear trend). After adjustment for expected readmission rate, race, sex, age, and payer, the trend remained significant (P = .005). Other significant predictors of readmissions in this model included expected readmission rate (P = .002), age (P = .02), and payer (P = .002). After dichotomizing the patient estimate of readmission rate as “unlikely” (N = 581) or “likely” (N = 314), the unadjusted odds ratio associating a patient-estimated risk of readmission as “likely” with actual readmission was 1.8 (95% confidence interval, 1.2-2.5). The adjusted odds ratio (including the variables above) was 1.6 (1.1-2.4).
DISCUSSION
Our findings demonstrate that patients are able to quantify their own readmission risk. This was true even after adjustment for expected readmission rate, age, sex, race, and payer. However, we did not identify any patient attitudes, beliefs, or preferences related to readmission or discharge instructions that were associated with subsequent rehospitalization. Reassuringly, more than 80% of patients who responded to the survey indicated that they would be sad, frustrated, or disappointed should readmission occur. This suggests that most patients are invested in preventing rehospitalization. Also reassuring was that patients indicated that they agreed with the discharge care plan and intended to follow their discharge instructions.
The major limitation of this study is that it was a convenience sample. Surveys were distributed inconsistently by nursing unit staff, preventing us from calculating a response rate. Further, it is possible, if not likely, that those patients with higher levels of engagement were more likely to take the time to respond, enriching our sample with activated patients. Although we allowed family members to fill out surveys on behalf of patients, this was done in fewer than 10% of instances; as such, our data may have limited applicability to patients who are physically or cognitively unable to participate in the discharge process. Finally, in this study, we did not capture readmissions to other facilities.
We conclude that patients are able to predict their own readmissions, even after accounting for other potential predictors of readmission. However, we found no evidence to support the possibility that low levels of engagement, limited trust in the healthcare team, or nonchalance about being readmitted are associated with subsequent rehospitalization. Whether asking patients about their perceived risk of readmission might help target readmission prevention programs deserves further study.
Acknowledgments
Dr. Daniel J. Brotman had full access to the data in the study and takes responsibility for the integrity of the study data and the accuracy of the data analysis. The authors also thank the following individuals for their contributions: Drafting the manuscript (Brotman); revising the manuscript for important intellectual content (Brotman, Shihab, Tieu, Cheng, Bertram, Hoyer, Deutschendorf); acquiring the data (Brotman, Shihab, Tieu, Cheng, Bertram, Deutschendorf); interpreting the data (Brotman, Shihab, Tieu, Cheng, Bertram, Hoyer, Deutschendorf); and analyzing the data (Brotman). The authors thank nursing leadership and nursing unit staff for their assistance in distributing surveys.
Funding support: Johns Hopkins Hospitalist Scholars Program
Disclosures: The authors have declared no conflicts of interest.
Recent years have seen a proliferation of programs designed to prevent readmissions, including patient education initiatives, financial assistance programs, postdischarge services, and clinical personnel assigned to help patients navigate their posthospitalization clinical care. Although some strategies do not require direct patient participation (such as timely and effective handoffs between inpatient and outpatient care teams), many rely upon a commitment by the patient to participate in the postdischarge care plan. At our hospital, we have found that only about 2/3 of patients who are offered transitional interventions (such as postdischarge phone calls by nurses or home nursing through a “transition guide” program) receive the intended interventions, and those who do not receive them are more likely to be readmitted.1 While limited patient uptake may relate, in part, to factors that are difficult to overcome, such as inadequate housing or phone service, we have also encountered patients whose values, beliefs, or preferences about their care do not align with those of the care team. The purposes of this exploratory study were to (1) assess patient attitudes surrounding readmission, (2) ascertain whether these attitudes are associated with actual readmission, and (3) determine whether patients can estimate their own risk of readmission.
METHODS
From January 2014 to September 2016, we circulated surveys to patients on internal medicine nursing units who were being discharged home within 24 hours. Blank surveys were distributed to nursing units by the researchers. Unit clerks and support staff were educated on the purpose of the project and asked to distribute surveys to patients who were identified by unit case managers or nurses as slated for discharge. Staff members were not asked to help with or supervise survey completion. Surveys were generally filled out by patients, but we allowed family members to assist patients if needed, and to indicate so with a checkbox. There were no exclusion criteria. Because surveys were distributed by clinical staff, the received surveys can be considered a convenience sample. Patients were asked 5 questions with 4- or 5-point Likert scale responses:
(1) “How likely is it that you will be admitted to the hospital (have to stay in the hospital overnight) again within the next 30 days after you leave the hospital this time?” [answers ranging from “Very Unlikely (<5% chance)” to “Very Likely (>50% chance)”];
(2) “How would you feel about being rehospitalized in the next month?” [answers ranging from “Very sad, frustrated, or disappointed” to “Very happy or relieved”];
(3) “How much do you think that you personally can control whether or not you will be rehospitalized (based on what you do to take care of your body, take your medicines, and follow-up with your healthcare team)?” [answers ranging from “I have no control over whether I will be rehospitalized” to “I have complete control over whether I will be rehospitalized”];
(4) “Which of the options below best describes how you plan to follow the medical instructions after you leave the hospital?” [answers ranging from “I do NOT plan to do very much of what I am being asked to do by the doctors, nurses, therapists, and other members of the care team” to “I plan to do EVERYTHING I am being asked to do by the doctors, nurses, therapists and other members of the care team”]; and
(5) “Pick the item below that best describes YOUR OWN VIEW of the care team’s recommendations:” [answers ranging from “I DO NOT AGREE AT ALL that the best way to be healthy is to do exactly what I am being asked to do by the doctors, nurses, therapists, and other members of the care team” to “I FULLY AGREE that the best way to be healthy is to do exactly what I am being asked to do by the doctors, nurses, therapists, and other members of the care team”].
Responses were linked, based on discharge date and medical record number, to administrative data, including age, sex, race, payer, and clinical data. Subsequent hospitalizations to our hospital were ascertained from administrative data. We estimated expected risk of readmission using the all payer refined diagnosis related group coupled with the associated severity-of-illness (SOI) score, as we have reported previously.2-5 We restricted our analysis to patients who answered the question related to the likelihood of readmission. Logistic regression models were constructed using actual 30-day readmission as the dependent variable to determine whether patients could predict their own readmissions and whether patient attitudes and beliefs about their care were predictive of subsequent readmission. Patient survey responses were entered as continuous independent variables (ranging from 1-4 or 1-5, as appropriate). Multivariable logistic regression was used to determine whether patients could predict their readmissions independent of demographic variables and expected readmission rate (modeled continuously); we repeated this model after dichotomizing the patient’s estimate of the likelihood of readmission as either “unlikely” or “likely.” Patients with missing survey responses were excluded from individual models without imputation. The study was approved by the Johns Hopkins institutional review board.
RESULTS
Responses were obtained from 895 patients. Their median age was 56 years [interquartile range, 43-67], 51.4% were female, and 41.7% were white. Mean SOI was 2.53 (on a 1-4 scale), and median length-of-stay was representative for our medical service at 5.2 days (range, 1-66 days). Family members reported filling out the survey in 57 cases. The primary payer was Medicare in 40.7%, Medicaid in 24.9%, and other in 34.4%. A total of 138 patients (15.4%) were readmitted within 30 days. The Table shows survey responses and associated readmission rates. None of the attitudes related to readmission were predictive of actual readmission. However, patients were able to predict their own readmissions (P = .002 for linear trend). After adjustment for expected readmission rate, race, sex, age, and payer, the trend remained significant (P = .005). Other significant predictors of readmissions in this model included expected readmission rate (P = .002), age (P = .02), and payer (P = .002). After dichotomizing the patient estimate of readmission rate as “unlikely” (N = 581) or “likely” (N = 314), the unadjusted odds ratio associating a patient-estimated risk of readmission as “likely” with actual readmission was 1.8 (95% confidence interval, 1.2-2.5). The adjusted odds ratio (including the variables above) was 1.6 (1.1-2.4).
DISCUSSION
Our findings demonstrate that patients are able to quantify their own readmission risk. This was true even after adjustment for expected readmission rate, age, sex, race, and payer. However, we did not identify any patient attitudes, beliefs, or preferences related to readmission or discharge instructions that were associated with subsequent rehospitalization. Reassuringly, more than 80% of patients who responded to the survey indicated that they would be sad, frustrated, or disappointed should readmission occur. This suggests that most patients are invested in preventing rehospitalization. Also reassuring was that patients indicated that they agreed with the discharge care plan and intended to follow their discharge instructions.
The major limitation of this study is that it was a convenience sample. Surveys were distributed inconsistently by nursing unit staff, preventing us from calculating a response rate. Further, it is possible, if not likely, that those patients with higher levels of engagement were more likely to take the time to respond, enriching our sample with activated patients. Although we allowed family members to fill out surveys on behalf of patients, this was done in fewer than 10% of instances; as such, our data may have limited applicability to patients who are physically or cognitively unable to participate in the discharge process. Finally, in this study, we did not capture readmissions to other facilities.
We conclude that patients are able to predict their own readmissions, even after accounting for other potential predictors of readmission. However, we found no evidence to support the possibility that low levels of engagement, limited trust in the healthcare team, or nonchalance about being readmitted are associated with subsequent rehospitalization. Whether asking patients about their perceived risk of readmission might help target readmission prevention programs deserves further study.
Acknowledgments
Dr. Daniel J. Brotman had full access to the data in the study and takes responsibility for the integrity of the study data and the accuracy of the data analysis. The authors also thank the following individuals for their contributions: Drafting the manuscript (Brotman); revising the manuscript for important intellectual content (Brotman, Shihab, Tieu, Cheng, Bertram, Hoyer, Deutschendorf); acquiring the data (Brotman, Shihab, Tieu, Cheng, Bertram, Deutschendorf); interpreting the data (Brotman, Shihab, Tieu, Cheng, Bertram, Hoyer, Deutschendorf); and analyzing the data (Brotman). The authors thank nursing leadership and nursing unit staff for their assistance in distributing surveys.
Funding support: Johns Hopkins Hospitalist Scholars Program
Disclosures: The authors have declared no conflicts of interest.
1. Hoyer EH, Brotman DJ, Apfel A, et al. Improving outcomes after hospitalization: a prospective observational multi-center evaluation of care-coordination strategies on 30-day readmissions to Maryland hospitals. J Gen Int Med. 2017 (in press). PubMed
2. Oduyebo I, Lehmann CU, Pollack CE, et al. Association of self-reported hospital discharge handoffs with 30-day readmissions. JAMA Intern Med. 2013;173(8):624-629. PubMed
3. Hoyer EH, Needham DM, Atanelov L, Knox B, Friedman M, Brotman DJ. Association of impaired functional status at hospital discharge and subsequent rehospitalization. J Hosp Med. 2014;9(5):277-282. PubMed
4. Hoyer EH, Needham DM, Miller J, Deutschendorf A, Friedman M, Brotman DJ. Functional status impairment is associated with unplanned readmissions. Arch Phys Med Rehabil. 2013;94(10):1951-1958. PubMed
5. Hoyer EH, Odonkor CA, Bhatia SN, Leung C, Deutschendorf A, Brotman DJ. Association between days to complete inpatient discharge summaries with all-payer hospital readmissions in Maryland. J Hosp Med. 2016;11(6):393-400. PubMed
1. Hoyer EH, Brotman DJ, Apfel A, et al. Improving outcomes after hospitalization: a prospective observational multi-center evaluation of care-coordination strategies on 30-day readmissions to Maryland hospitals. J Gen Int Med. 2017 (in press). PubMed
2. Oduyebo I, Lehmann CU, Pollack CE, et al. Association of self-reported hospital discharge handoffs with 30-day readmissions. JAMA Intern Med. 2013;173(8):624-629. PubMed
3. Hoyer EH, Needham DM, Atanelov L, Knox B, Friedman M, Brotman DJ. Association of impaired functional status at hospital discharge and subsequent rehospitalization. J Hosp Med. 2014;9(5):277-282. PubMed
4. Hoyer EH, Needham DM, Miller J, Deutschendorf A, Friedman M, Brotman DJ. Functional status impairment is associated with unplanned readmissions. Arch Phys Med Rehabil. 2013;94(10):1951-1958. PubMed
5. Hoyer EH, Odonkor CA, Bhatia SN, Leung C, Deutschendorf A, Brotman DJ. Association between days to complete inpatient discharge summaries with all-payer hospital readmissions in Maryland. J Hosp Med. 2016;11(6):393-400. PubMed
© 2018 Society of Hospital Medicine
Hospital Renovation Patient Satisfaction
Hospitals are expensive and complex facilities to build and renovate. It is estimated $200 billion is being spent in the United States during this decade on hospital construction and renovation, and further expenditures in this area are expected.[1] Aging hospital infrastructure, competition, and health system expansion have motivated institutions to invest in renovation and new hospital building construction.[2, 3, 4, 5, 6, 7] There is a trend toward patient‐centered design in new hospital construction. Features of this trend include same‐handed design (ie, rooms on a unit have all beds oriented in the same direction and do not share headwalls); use of sound absorbent materials to reduced ambient noise[7, 8, 9]; rooms with improved view and increased natural lighting to reduce anxiety, decrease delirium, and increase sense of wellbeing[10, 11, 12]; incorporation of natural elements like gardens, water features, and art[12, 13, 14, 15, 16, 17, 18]; single‐patient rooms to reduce transmission of infection and enhance privacy and visitor comfort[7, 19, 20]; presence of comfortable waiting rooms and visitor accommodations to enhance comfort and family participation[21, 22, 23]; and hotel‐like amenities such as on‐demand entertainment and room service menus.[24, 25]
There is a belief among some hospital leaders that patients are generally unable to distinguish their positive experience with a pleasing healthcare environment from their positive experience with care, and thus improving facilities will lead to improved satisfaction across the board.[26, 27] In a controlled study of hospitalized patients, appealing rooms were associated with increased satisfaction with services including housekeeping and food service staff, meals, as well as physicians and overall satisfaction.[26] A 2012 survey of hospital leadership found that expanding and renovating facilities was considered a top priority in improving patient satisfaction, with 82% of the respondents stating that this was important.[27]
Despite these attitudes, the impact of patient‐centered design on patient satisfaction is not well understood. Studies have shown that renovations and hospital construction that incorporates noise reduction strategies, positive distraction, patient and caregiver control, attractive waiting rooms, improved patient room appearance, private rooms, and large windows result in improved satisfaction with nursing, noise level, unit environment and cleanliness, perceived wait time, discharge preparedness, and overall care. [7, 19, 20, 23, 28] However, these studies were limited by small sample size, inclusion of a narrow group of patients (eg, ambulatory, obstetric, geriatric rehabilitation, intensive care unit), and concurrent use of interventions other than design improvement (eg, nurse and patient education). Many of these studies did not use the ubiquitous Hospital Consumer Assessment of Healthcare Providers and Systems (HCAHPS) and Press Ganey patient satisfaction surveys.
We sought to determine the changes in patient satisfaction that occurred during a natural experiment, in which clinical units (comprising stable nursing, physician, and unit teams) were relocated from an historic clinical building to a new clinical building that featured patient‐centered design, using HCAHPS and Press Ganey surveys and a large study population. We hypothesized that new building features would positively impact both facility related (eg, noise level), nonfacility related (eg, physician and housekeeping service related), and overall satisfaction.
METHODS
This was a retrospective analysis of prospectively collected Press Ganey and HCAPHS patient satisfaction survey data for a single academic tertiary care hospital.[29] The research project was reviewed and approved by the institutional review board.
Participants
All patients discharged from 12 clinical units that relocated to the new clinical building and returned patient satisfaction surveys served as study patients. The moved units included the coronary care unit, cardiac step down unit, medical intensive care unit, neuro critical care unit, surgical intensive care unit, orthopedic unit, neurology unit, neurosurgery unit, obstetrics units, gynecology unit, urology unit, cardiothoracic surgery unit, and the transplant surgery and renal transplant unit. Patients on clinical units that did not move served as concurrent controls.
Exposure
Patients admitted to the new clinical building experienced several patient‐centered design features. These features included easy access to healing gardens with a water feature, soaring lobbies, a collection of more than 500 works of art, well‐decorated and light‐filled patient rooms with sleeping accommodations for family members, sound‐absorbing features in patient care corridors ranging from acoustical ceiling tiles to a quiet nurse‐call system, and an interactive television network with Internet, movies, and games. All patients during the baseline period and control patients during the study period were located in typical patient rooms with standard hospital amenities. No other major patient satisfaction interventions were initiated during the pre‐ or postperiod in either arm of the study; ongoing patient satisfaction efforts (such as unit‐based customer care representatives) were deployed broadly and not restricted to the new clinical building. Clinical teams comprised of physicians, nurses, and ancillary staff did not change significantly after the move.
Time Periods
The move to new clinical building occurred on May 1, 2012. After allowing for a 15‐day washout period, the postmove period included Press Ganey and HCAHPS surveys returned for discharges that occurred during a 7.5‐month period between May 15, 2102 and December 31, 2012. Baseline data included Press Ganey and HCAHPS surveys returned for discharges in the preceding 12 months (May 1, 2011 to April 30, 2012). Sensitivity analysis using only 7.5 months of baseline data did not reveal any significant difference when compared with 12‐month baseline data, and we report only data from the 12‐month baseline period.
Instruments
Press Ganey and HCAHPS patient satisfaction surveys were sent via mail in the same envelope. Fifty percent of the discharged patients were randomized to receive the surveys. The Press Ganey survey contained 33 items covering across several subdomains including room, meal, nursing, physician, ancillary staff, visitor, discharge, and overall satisfaction. The HCAHPS survey contained 29 Centers for Medicare and Medicaid Services (CMS)‐mandated items, of which 21 are related to patient satisfaction. The development and testing and methods for administration and reporting of the HCAHPS survey have been previously described.[30, 31] Press Ganey patient satisfaction survey results have been reported in the literature.[32, 33]
Outcome Variables
Press Ganey and HCAHPS patient satisfaction survey responses were the primary outcome variables of the study. The survey items were categorized as facility related (eg, noise level), nonfacility related (eg, physician and nursing staff satisfaction), and overall satisfaction related.
Covariates
Age, sex, length of stay (LOS), insurance type, and all‐payer refined diagnosis‐related groupassociated illness complexity were included as covariates.
Statistical Analysis
Percent top‐box scores were calculated for each survey item as the percent of patients who responded very good for a given item on Press Ganey survey items and always or definitely yes or 9 or 10 on HCAHPS survey items. CMS utilizes percent top‐box scores to calculate payments under the Value Based Purchasing (VBP) program and to report the results publicly. Numerous studies have also reported percent top‐box scores for HCAHPS survey results.[31, 32, 33, 34]
Odds ratios of premove versus postmove percentage of top‐box scores, adjusted for age, sex, LOS, complexity of illness, and insurance type were determined using logistic regression for the units that moved. Similar scores were calculated for unmoved units to detect secular trends. To determine whether the differences between the moved and unmoved units were significant, we introduced the interaction term (moved vs unmoved unit status) (pre‐ vs postmove time period) into the logistic regression models and examined the adjusted P value for this term. All statistical analysis was performed using SAS Institute Inc.'s (Cary, NC) JMP Pro 10.0.0.
RESULTS
The study included 1648 respondents in the moved units in the baseline period (ie, units designated to move to a new clinical building) and 1373 respondents in the postmove period. There were 1593 respondents in the control group during the baseline period and 1049 respondents in the postmove period. For the units that moved, survey response rates were 28.5% prior to the move and 28.3% after the move. For the units that did not move, survey response rates were 20.9% prior to the move and 22.7% after the move. A majority of survey respondents on the nursing units that moved were white, male, and had private insurance (Table 1). There were no significant differences between respondents across these characteristics between the pre‐ and postmove periods. Mean age and LOS were also similar. For these units, there were 70.5% private rooms prior to the move and 100% after the move. For the unmoved units, 58.9% of the rooms were private in the baseline period and 72.7% were private in the study period. Similar to the units that moved, characteristics of the respondents on the unmoved units also did not differ significantly in the postmove period.
Patient demographics | Moved Units (N=3,021) | Unmoved Units (N=2,642) | ||||
---|---|---|---|---|---|---|
Pre | Post | P Value | Pre | Post | P Value | |
| ||||||
White | 75.3% | 78.2% | 0.07 | 66.7% | 68.5% | 0.31 |
Mean age, y | 57.3 | 57.4 | 0.84 | 57.3 | 57.1 | 0.81 |
Male | 54.3% | 53.0% | 0.48 | 40.5% | 42.3% | 0.23 |
Self‐reported health | ||||||
Excellent or very good | 54.7% | 51.2% | 0.04 | 38.7% | 39.5% | 0.11 |
Good | 27.8% | 32.0% | 29.3% | 32.2% | ||
Fair or poor | 17.5% | 16.9% | 32.0% | 28.3% | ||
Self‐reported language | ||||||
English | 96.0% | 97.2% | 0.06 | 96.8% | 97.1% | 0.63 |
Other | 4.0% | 2.8% | 3.2% | 2.9% | ||
Self‐reported education | ||||||
Less than high school | 5.8% | 5.0% | 0.24 | 10.8% | 10.4% | 0.24 |
High school grad | 46.4% | 44.2% | 48.6% | 45.5% | ||
College grad or more | 47.7% | 50.7% | 40.7% | 44.7% | ||
Insurance type | ||||||
Medicaid | 6.7% | 5.5% | 0.11 | 10.8% | 9.0% | 0.32 |
Medicare | 32.0% | 35.5% | 36.0% | 36.1% | ||
Private insurance | 55.6% | 52.8% | 48.0% | 50.3% | ||
Mean APRDRG complexity* | 2.1 | 2.1 | 0.09 | 2.3 | 2.3 | 0.14 |
Mean LOS | 4.7 | 5.0 | 0.12 | 4.9 | 5.0 | 0.77 |
Service | ||||||
Medicine | 15.4% | 16.2% | 0.51 | 40.0% | 34.5% | 0.10 |
Surgery | 50.7% | 45.7% | 40.1% | 44.1% | ||
Neurosciences | 20.3% | 24.1% | 6.0% | 6.0% | ||
Obstetrics/gynecology | 7.5% | 8.2% | 5.7% | 5.6% |
The move was associated with significant improvements in facility‐related satisfaction (Tables 2 and 3). The most prominent increases in satisfaction were with pleasantness of dcor (33.6% vs 66.2%), noise level (39.9% vs 59.3%), and visitor accommodation and comfort (50.0% vs 70.3 %). There was improvement in satisfaction related to cleanliness of the room (49.0% vs 68.6 %), but no significant increase in satisfaction with courtesy of the person cleaning the room (59.8% vs 67.7%) when compared with units that did move.
Satisfaction Domain | Moved Units | Unmoved Units | P Value of the Difference in Odds Ratio Between Moved and Unmoved Units | |||||
---|---|---|---|---|---|---|---|---|
% Top Box | Adjusted Odds Ratio* (95% CI) | % Top Box | Adjusted Odds Ratio* (95% CI) | |||||
Pre | Post | Pre | Post | |||||
| ||||||||
FACILITY RELATED | ||||||||
Hospital environment | ||||||||
Cleanliness of the room and bathroom | 61.0 | 70.8 | 1.62 (1.40‐1.90) | 64.0 | 69.2 | 1.24 (1.03‐1.48) | 0.03 | |
Quietness of the room | 51.3 | 65.4 | 1.89 (1.63‐2.19) | 58.6 | 60.3 | 1.08 (0.90‐1.28) | <0.0001 | |
NONFACILITY RELATED | ||||||||
Nursing communication | ||||||||
Nurses treated with courtesy/respect | 84.0 | 86.7 | 1.28 (1.05‐1.57) | 83.6 | 87.1 | 1.29 (1.02‐1.64) | 0.92 | |
Nurses listened | 73.1 | 76.4 | 1.21 (1.03‐1.43) | 74.2 | 75.5 | 1.05 (0.86‐1.27) | 0.26 | |
Nurses explained | 75.0 | 76.6 | 1.10 (0.94‐1.30) | 76.0 | 76.2 | 1.00 (0.82‐1.21) | 0.43 | |
Physician communication | ||||||||
Doctors treated with courtesy/respect | 89.5 | 90.5 | 1.13 (0.89‐1.42) | 84.9 | 87.3 | 1.20 (0.94‐1.53) | 0.77 | |
Doctors listened | 81.4 | 81.0 | 0.93 (0.83‐1.19) | 77.7 | 77.1 | 0.94 (0.77‐1.15) | 0.68 | |
Doctors explained | 79.2 | 79.0 | 1.00(0.84‐1.19) | 75.7 | 74.4 | 0.92 (0.76‐1.12) | 0.49 | |
Other | ||||||||
Help toileting as soon as you wanted | 61.8 | 63.7 | 1.08 (0.89‐1.32) | 62.3 | 60.6 | 0.92 (0.71‐1.18) | 0.31 | |
Pain well controlled | 63.2 | 63.8 | 1.06 (0.90‐1.25) | 62.0 | 62.6 | 0.99 (0.81‐1.20) | 060 | |
Staff do everything to help with pain | 77.7 | 80.1 | 1.19 (0.99‐1.44) | 76.8 | 75.7 | 0.90 (0.75‐1.13) | 0.07 | |
Staff describe medicine side effects | 47.0 | 47.6 | 1.05 (0.89‐1.24) | 49.2 | 47.1 | 0.91 (0.74‐1.11) | 0.32 | |
Tell you what new medicine was for | 76.4 | 76.4 | 1.02 (0.84‐1.25) | 77.1 | 78.8 | 1.09(0.85‐1.39) | 0.65 | |
Overall | ||||||||
Rate hospital (010) | 75.0 | 83.3 | 1.71 (1.44‐2.05) | 75.7 | 77.6 | 1.06 (0.87‐1.29) | 0.006 | |
Recommend hospital | 82.5 | 87.1 | 1.43 (1.18‐1.76) | 81.4 | 82.0 | 0.98 (0.79‐1.22) | 0.03 |
Satisfaction Domain | Moved Unit | Unmoved Unit | P Value of the Difference in Odds Ratio Between Moved and Unmoved Units | ||||
---|---|---|---|---|---|---|---|
% Top Box | Adjusted Odds Ratio* (95% CI) | % Top Box | Adjusted Odds Ratio* (95% CI) | ||||
Pre | Post | Pre | Post | ||||
| |||||||
FACILITY RELATED | |||||||
Room | |||||||
Pleasantness of room dcor | 33.6 | 64.8 | 3.77 (3.24‐4.38) | 41.6 | 47.0 | 1.21 (1.02‐1.44) | <0.0001 |
Room cleanliness | 49.0 | 68.6 | 2.35 (2.02‐2.73) | 51.6 | 59.1 | 1.32 (1.12‐1.58) | <0.0001 |
Room temperature | 43.1 | 54.9 | 1.64 (1.43‐1.90) | 45.0 | 48.8 | 1.14 (0.96‐1.36) | 0.002 |
Noise level in and around the room | 40.2 | 59.2 | 2.23 (1.92‐2.58) | 45.5 | 47.6 | 1.07 (0.90‐1.22) | <0.0001 |
Visitor related | |||||||
Accommodations and comfort of visitors | 50.0 | 70.3 | 2.44 (2.10‐2.83) | 55.3 | 59.1 | 1.14 (0.96‐1.35) | <0.0001 |
NONFACILITY RELATED | |||||||
Food | |||||||
Temperature of the food | 31.1 | 33.6 | 1.15 (0.99‐1.34) | 34.0 | 38.9 | 1.23 (1.02‐1.47) | 0.51 |
Quality of the food | 25.8 | 27.1 | 1.10 (0.93‐1.30) | 30.2 | 36.2 | 1.32 (1.10‐1.59) | 0.12 |
Courtesy of the person who served food | 63.9 | 62.3 | 0.93 (0.80‐1.10) | 66.0 | 61.4 | 0.82 (0.69‐0.98) | 0.26 |
Nursing | |||||||
Friendliness/courtesy of the nurses | 76.3 | 82.8 | 1.49 (1.26‐1.79) | 77.7 | 80.1 | 1.10 (0.90‐1.37) | 0.04 |
Promptness of response to call | 60.1 | 62.6 | 1.14 (0.98‐1.33) | 59.2 | 62.0 | 1.10 (0.91‐1.31) | 0.80 |
Nurses' attitude toward requests | 71.0 | 75.8 | 1.30 (1.11‐1.54) | 70.5 | 72.4 | 1.06 (0.88‐1.28) | 0.13 |
Attention to special/personal needs | 66.7 | 72.2 | 1.32 (1.13‐1.54) | 67.8 | 70.3 | 1.09 (0.91‐1.31) | 0.16 |
Nurses kept you informed | 64.3 | 72.2 | 1.46 (1.25‐1.70) | 65.8 | 69.8 | 1.17 (0.98‐1.41) | 0.88 |
Skill of the nurses | 75.3 | 79.5 | 1.28 (1.08‐1.52) | 74.3 | 78.6 | 1.23 (1.01‐1.51) | 0.89 |
Ancillary staff | |||||||
Courtesy of the person cleaning the room | 59.8 | 67.7 | 1.41 (1.21‐1.65) | 61.2 | 66.5 | 1.24 (1.03‐1.49) | 0.28 |
Courtesy of the person who took blood | 66.5 | 68.1 | 1.10 (0.94‐1.28) | 63.2 | 63.1 | 0.96 (0.76‐1.08) | 0.34 |
Courtesy of the person who started the IV | 70.0 | 71.7 | 1.09 (0.93‐1.28) | 66.6 | 69.3 | 1.11 (0.92‐1.33) | 0.88 |
Visitor related | |||||||
Staff attitude toward visitors | 68.1 | 79.4 | 1.84 (1.56‐2.18) | 70.3 | 72.2 | 1.06 (0.87‐1.28) | <0.0001 |
Physician | |||||||
Time physician spent with you | 55.0 | 58.9 | 1.20 (1.04‐1.39) | 53.2 | 55.9 | 1.10 (0.92‐1.30) | 0.46 |
Physician concern questions/worries | 67.2 | 70.7 | 1.20 (1.03‐1.40) | 64.3 | 66.1 | 1.05 (0.88‐1.26) | 0.31 |
Physician kept you informed | 65.3 | 67.5 | 1.12 (0.96‐1.30) | 61.6 | 63.2 | 1.05 (0.88‐1.25) | 0.58 |
Friendliness/courtesy of physician | 76.3 | 78.1 | 1.11 (0.93‐1.31) | 71.0 | 73.3 | 1.08 (0.90‐1.31) | 0.89 |
Skill of physician | 85.4 | 88.5 | 1.35 (1.09‐1.68) | 78.0 | 81.0 | 1.15 (0.93‐1.43) | 0.34 |
Discharge | |||||||
Extent felt ready for discharge | 62.0 | 66.7 | 1.23 (1.07‐1.44) | 59.2 | 62.3 | 1.10 (0.92‐1.30) | 0.35 |
Speed of discharge process | 50.7 | 54.2 | 1.16 (1.01‐1.33) | 47.8 | 50.0 | 1.07 (0.90‐1.27) | 0.49 |
Instructions for care at home | 66.4 | 71.1 | 1.25 (1.06‐1.46) | 64.0 | 67.7 | 1.16 (0.97‐1.39) | 0.54 |
Staff concern for your privacy | 65.3 | 71.8 | 1.37 (1.17‐0.85) | 63.6 | 66.2 | 1.10 (0.91‐1.31) | 0.07 |
Miscellaneous | |||||||
How well your pain was controlled | 64.2 | 66.5 | 1.14 (0.97‐1.32) | 60.2 | 62.6 | 1.07 (0.89‐1.28) | 0.66 |
Staff addressed emotional needs | 60.0 | 63.4 | 1.19 (1.02‐1.38) | 55.1 | 60.2 | 1.20 (1.01‐1.42) | 0.90 |
Response to concerns/complaints | 61.1 | 64.5 | 1.19 (1.02‐1.38) | 57.2 | 60.1 | 1.10 (0.92‐1.31) | 0.57 |
Overall | |||||||
Staff worked together to care for you | 72.6 | 77.2 | 1.29 (1.10‐1.52) | 70.3 | 73.2 | 1.13 (0.93‐1.37) | 0.30 |
Likelihood of recommending hospital | 79.1 | 84.3 | 1.44 (1.20‐1.74) | 76.3 | 79.2 | 1.14 (0.93‐1.39) | 0.10 |
Overall rating of care given | 76.8 | 83.0 | 1.50 (1.25‐1.80) | 74.7 | 77.2 | 1.10 (0.90‐1.34) | 0.03 |
With regard to nonfacility‐related satisfaction, there were statistically higher scores in several nursing, physician, and discharge‐related satisfaction domains after the move. However, these changes were not associated with the move to the new clinical building as they were not significantly different from improvements on the unmoved units. Among nonfacility‐related items, only staff attitude toward visitors showed significant improvement (68.1% vs 79.4%). There was a significant improvement in hospital rating (75.0% vs 83.3% in the moved units and 75.7% vs 77.6% in the unmoved units). However, the other 3 measures of overall satisfaction did not show significant improvement associated with the move to the new clinical building when compared to the concurrent controls.
DISCUSSION
Contrary to our hypothesis and a belief held by many, we found that patients appeared able to distinguish their experience with hospital environment from their experience with providers and other services. Improvement in hospital facilities with incorporation of patient‐centered features was associated with improvements that were largely limited to increases in satisfaction with quietness, cleanliness, temperature, and dcor of the room along with visitor‐related satisfaction. Notably, there was no significant improvement in satisfaction related to physicians, nurses, housekeeping, and other service staff. There was improvement in satisfaction with staff attitude toward visitors, but this can be attributed to availability of visitor‐friendly facilities. There was a significant improvement in 1 of the 4 measures of overall satisfaction. Our findings also support the construct validity of HCAHPS and Press Ganey patient satisfaction surveys.
Ours is one of the largest studies on patient satisfaction related to patient‐centered design features in the inpatient acute care setting. Swan et al. also studied patients in an acute inpatient setting and compared satisfaction related to appealing versus typical hospital rooms. Patients were matched for case mix, insurance, gender, types of medical services received and LOS, and were served by the same set of physicians and similar food service and housekeeping staff.[26] Unlike our study, they found improved satisfaction related to physicians, housekeeping staff, food service staff, meals, and overall satisfaction. However, the study had some limitations. In particular, the study sample was self‐selected because the patients in this group were required to pay an extra daily fee to utilize the appealing room. Additionally, there were only 177 patients across the 2 groups, and the actual differences in satisfaction scores were small. Our sample was larger and patients in the study group were admitted to units in the new clinical buildings by the same criteria as they were admitted to the historic building prior to the move, and there were no significant differences in baseline characteristics between the comparison groups.
Jansen et al. also found broad improvements in patient satisfaction in a study of over 309 maternity unit patients in a new construction, all private‐room maternity unit with more appealing design elements and comfort features for visitors.[7] Improved satisfaction was noted with the physical environment, nursing care, assistance with feeding, respect for privacy, and discharge planning. However, it is difficult to extrapolate the results of this study to other settings, as maternity unit patients constitute a unique patient demographic with unique care needs. Additionally, when compared with patients in the control group, the patients in the study group were cared for by nurses who had a lower workload and who were not assigned other patients with more complex needs. Because nursing availability may be expected to impact satisfaction with clinical domains, the impact of private and appealing room may very well have been limited to improved satisfaction with the physical environment.
Despite the widespread belief among healthcare leadership that facility renovation or expansion is a vital strategy for improving patient satisfaction, our study shows that this may not be a dominant factor.[27] In fact, the Planetree model showed that improvement in satisfaction related to physical environment and nursing care was associated with implementation of both patient‐centered design features as well as with utilization of nurses that were trained to provide personalized care, educate patients, and involve patients and family.[28] It is more likely that provider‐level interventions will have a greater impact on provider level and overall satisfaction. This idea is supported by a recent JD Powers study suggesting that facilities represent only 19% of overall satisfaction in the inpatient setting.[35]
Although our study focused on patient‐centered design features, several renovation and construction projects have also focused on design features that improve patient safety and provider satisfaction, workflow, efficiency, productivity, stress, and time spent in direct care.[9] Interventions in these areas may lead to improvement in patient outcomes and perhaps lead to improvement in patient satisfaction; however, this relationship has not been well established at present.
In an era of cost containment, healthcare administrators are faced with high‐priced interventions, competing needs, limited resources, low profit margins, and often unclear evidence on cost‐effectiveness and return on investment of healthcare design features. Benefits are related to competitive advantage, higher reputation, patient retention, decreased malpractice costs, and increased Medicare payments through VBP programs that incentivize improved performance on quality metrics and patient satisfaction surveys. Our study supports the idea that a significant improvement in patient satisfaction related to creature comforts can be achieved with investment in patient‐centered design features. However, our findings also suggest that institutions should perform an individualized cost‐benefit analysis related to improvements in this narrow area of patient satisfaction. In our study, incorporation of patient‐centered design features resulted in improvement on 2 VBP HCAHPS measures, and its contribution toward total performance score under the VBP program would be limited.
Strengths of our study include the use of concurrent controls and our ability to capitalize on a natural experiment in which care teams remained constant before and after a move to a new clinical building. However, our study has some limitations. It was conducted at a single tertiary care academic center that predominantly serves an inner city population and referral patients seeking specialized care. Drivers of patient satisfaction may be different in community hospitals, and a different relationship may be observed between patient‐centered design and domains of patient satisfaction in this setting. Further studies in different hospital settings are needed to confirm our findings. Additionally, we were limited by the low response rate of the surveys. However, this is a widespread problem with all patient satisfaction research utilizing voluntary surveys, and our response rates are consistent with those previously reported.[34, 36, 37, 38] Furthermore, low response rates have not impeded the implementation of pay‐for‐performance programs on a national scale using HCHAPS.
In conclusion, our study suggests that hospitals should not use outdated facilities as an excuse for achievement of suboptimal satisfaction scores. Patients respond positively to creature comforts, pleasing surroundings, and visitor‐friendly facilities but can distinguish these positive experiences from experiences in other patient satisfaction domains. In our study, the move to a higher‐amenity building had only a modest impact on overall patient satisfaction, perhaps because clinical care is the primary driver of this outcome. Contrary to belief held by some hospital leaders, major strides in overall satisfaction across the board and other subdomains of satisfaction likely require intervention in areas other than facility renovation and expansion.
Disclosures
Zishan Siddiqui, MD, was supported by the Osler Center of Clinical Excellence Faculty Scholarship Grant. Funds from Johns Hopkins Hospitalist Scholars Program supported the research project. The authors have no conflict of interests to disclose.
- Create a blueprint for successful hospital construction. Nurs Manage. 2006;37(6):39–44. , .
- Walter Reed National Military Medical Center website. Facts at a glance. Available at: http://www.wrnmmc.capmed.mil/About%20Us/SitePages/Facts.aspx. Accessed June 19, 2013.
- http://www.healthcaredesignmagazine.com/building‐ideas/keys‐collaboration. Accessed June 19, 2013. . Keys to collaboration. Healthcare Design website. Available at:
- http://www.healthcaredesignmagazine.com/building‐ideas/tale‐4‐hospitals. Accessed June 19, 2013. . A tale of 4 hospitals. Healthcare Design website. Available at:
- http://www.healthcaredesignmagazine.com/building‐ideas/gateway‐east. Accessed June 19, 2013. . Gateway to the east. Healthcare Design website. Available at:
- http://www.healthcaredesignmagazine.com/building‐ideas/lessons‐learned. Accessed June 19, 2013. . Lessons learned. Healthcare Design website. Available at:
- Single room maternity care and client satisfaction. Birth. 2000;27(4):235–243. , , , , .
- Same‐handed and mirrored unit configurations: is there a difference in patient and nurse outcomes? J Nurs Adm. 2011;41(6):273–279. , , , .
- The Pebble Projects: coordinated evidence‐based case studies. Build Res Inform. 2008;36(2):129–145. , .
- Effects of exposure to nature and abstract pictures on patients recovering from open heart surgery. J Soc Psychophysiol Res. 1993;30:7. , , .
- Postoperative delirium. Curr Drug Targets. 2005;6(7):807–814. , , , .
- Stimulus deprivation in windowless rooms. Anaesthesia. 1977;32(7):598–602. .
- Post‐occupancy evaluation of healing gardens in a pediatric cancer center. Landsc Urban Plan. 2005;73(2):167–183. , , , .
- Healing gardens in hospitals. Interdiscip Des Res J. 2007;1(1):1–27. .
- Restorative gardens. BMJ. 1993;306(6885):1080–1081. , .
- Effects of interior design on wellness: theory and recent scientific research. J Health Care Inter Des. 1991;3:97–109. .
- Sunny hospital rooms expedite recovery from severe and refractory depressions. J Affect Disord. 1996;40(1‐2):49–51. , .
- Art in hospital spaces: the role of hospitals in an aestheticised society. Int J Cult Policy. 2007;13(1):85–101. .
- Renovation of a semiprivate patient room. Bowman Center Geriatric Rehabilitation Unit. Nurs Clin North Am 1995;30(1):97–115. , , .
- (2013). Effect of intensive care environment on family and patient satisfaction: a before‐after study. Intensive Care Med. 2013;39(9):1626–1634. , , , et al.
- Outcomes of environmental appraisal of different hospital waiting areas. Environ Behav. 2003;35(6):842–869. , , , , .
- Redesigning the neurocritical care unit to enhance family participation and improve outcomes. Cleve Clin J Med. 2009;76(suppl 2):S70–S74. .
- The ecology of the patient visit: physical attractiveness, waiting times, and perceived quality of care. J Ambul Care Manage. 2008;31(2):128–141. , .
- Patient satisfaction and the new consumer. Hosp Health Netw. 2006;80(57):59–62. .
- Patient satisfaction. Hospitals embrace hotel‐like amenities. Hosp Health Netw. 2007;81(11):24–26. .
- Do appealing hospital rooms increase patient evaluations of physicians, nurses, and hospital services? Health Care Manage Rev. 2003;28(3):254–264. , , .
- http://www.healthleadersmedia.com/intelligence/detail.cfm?content_id=28289334(2):125–133. . Patient experience and HCAHPS: little consensus on a top priority. Health Leaders Media website. Available at
- Centers for Medicare 67:27–37.
- Hospital Consumer Assessment of Healthcare Providers and Systems. Summary analysis. http://www.hcahpsonline.org/SummaryAnalyses.aspx. Accessed October 1, 2014.
- Centers for Medicare 44(2 pt 1):501–518.
- J.D. Power and Associates. Patient satisfaction influenced more by hospital staff than by the hospital facilities. Available at: http://www.jdpower.com/press‐releases/2012‐national‐patient‐experience‐study#sthash.gSv6wAdc.dpuf. Accessed December 10, 2013.
- Racial and ethnic differences in a patient survey: patients' values, ratings, and reports regarding physician primary care performance in a large health maintenance organization. Med Care. 2000;38(3): 300–310. , , , , .
- Patient experience in safety‐net hospitals implications for improving care and Value‐Based Purchasing patient experience in safety‐net hospitals. Arch Intern Med. 2012;172(16):1204–1210. , , , .
- Comparison of Hospital Consumer Assessment of Healthcare Providers and Systems patient satisfaction scores for specialty hospitals and general medical hospitals: confounding effect of survey response rate. J Hosp Med. 2014;9(9):590–593. , , , .
Hospitals are expensive and complex facilities to build and renovate. It is estimated $200 billion is being spent in the United States during this decade on hospital construction and renovation, and further expenditures in this area are expected.[1] Aging hospital infrastructure, competition, and health system expansion have motivated institutions to invest in renovation and new hospital building construction.[2, 3, 4, 5, 6, 7] There is a trend toward patient‐centered design in new hospital construction. Features of this trend include same‐handed design (ie, rooms on a unit have all beds oriented in the same direction and do not share headwalls); use of sound absorbent materials to reduced ambient noise[7, 8, 9]; rooms with improved view and increased natural lighting to reduce anxiety, decrease delirium, and increase sense of wellbeing[10, 11, 12]; incorporation of natural elements like gardens, water features, and art[12, 13, 14, 15, 16, 17, 18]; single‐patient rooms to reduce transmission of infection and enhance privacy and visitor comfort[7, 19, 20]; presence of comfortable waiting rooms and visitor accommodations to enhance comfort and family participation[21, 22, 23]; and hotel‐like amenities such as on‐demand entertainment and room service menus.[24, 25]
There is a belief among some hospital leaders that patients are generally unable to distinguish their positive experience with a pleasing healthcare environment from their positive experience with care, and thus improving facilities will lead to improved satisfaction across the board.[26, 27] In a controlled study of hospitalized patients, appealing rooms were associated with increased satisfaction with services including housekeeping and food service staff, meals, as well as physicians and overall satisfaction.[26] A 2012 survey of hospital leadership found that expanding and renovating facilities was considered a top priority in improving patient satisfaction, with 82% of the respondents stating that this was important.[27]
Despite these attitudes, the impact of patient‐centered design on patient satisfaction is not well understood. Studies have shown that renovations and hospital construction that incorporates noise reduction strategies, positive distraction, patient and caregiver control, attractive waiting rooms, improved patient room appearance, private rooms, and large windows result in improved satisfaction with nursing, noise level, unit environment and cleanliness, perceived wait time, discharge preparedness, and overall care. [7, 19, 20, 23, 28] However, these studies were limited by small sample size, inclusion of a narrow group of patients (eg, ambulatory, obstetric, geriatric rehabilitation, intensive care unit), and concurrent use of interventions other than design improvement (eg, nurse and patient education). Many of these studies did not use the ubiquitous Hospital Consumer Assessment of Healthcare Providers and Systems (HCAHPS) and Press Ganey patient satisfaction surveys.
We sought to determine the changes in patient satisfaction that occurred during a natural experiment, in which clinical units (comprising stable nursing, physician, and unit teams) were relocated from an historic clinical building to a new clinical building that featured patient‐centered design, using HCAHPS and Press Ganey surveys and a large study population. We hypothesized that new building features would positively impact both facility related (eg, noise level), nonfacility related (eg, physician and housekeeping service related), and overall satisfaction.
METHODS
This was a retrospective analysis of prospectively collected Press Ganey and HCAPHS patient satisfaction survey data for a single academic tertiary care hospital.[29] The research project was reviewed and approved by the institutional review board.
Participants
All patients discharged from 12 clinical units that relocated to the new clinical building and returned patient satisfaction surveys served as study patients. The moved units included the coronary care unit, cardiac step down unit, medical intensive care unit, neuro critical care unit, surgical intensive care unit, orthopedic unit, neurology unit, neurosurgery unit, obstetrics units, gynecology unit, urology unit, cardiothoracic surgery unit, and the transplant surgery and renal transplant unit. Patients on clinical units that did not move served as concurrent controls.
Exposure
Patients admitted to the new clinical building experienced several patient‐centered design features. These features included easy access to healing gardens with a water feature, soaring lobbies, a collection of more than 500 works of art, well‐decorated and light‐filled patient rooms with sleeping accommodations for family members, sound‐absorbing features in patient care corridors ranging from acoustical ceiling tiles to a quiet nurse‐call system, and an interactive television network with Internet, movies, and games. All patients during the baseline period and control patients during the study period were located in typical patient rooms with standard hospital amenities. No other major patient satisfaction interventions were initiated during the pre‐ or postperiod in either arm of the study; ongoing patient satisfaction efforts (such as unit‐based customer care representatives) were deployed broadly and not restricted to the new clinical building. Clinical teams comprised of physicians, nurses, and ancillary staff did not change significantly after the move.
Time Periods
The move to new clinical building occurred on May 1, 2012. After allowing for a 15‐day washout period, the postmove period included Press Ganey and HCAHPS surveys returned for discharges that occurred during a 7.5‐month period between May 15, 2102 and December 31, 2012. Baseline data included Press Ganey and HCAHPS surveys returned for discharges in the preceding 12 months (May 1, 2011 to April 30, 2012). Sensitivity analysis using only 7.5 months of baseline data did not reveal any significant difference when compared with 12‐month baseline data, and we report only data from the 12‐month baseline period.
Instruments
Press Ganey and HCAHPS patient satisfaction surveys were sent via mail in the same envelope. Fifty percent of the discharged patients were randomized to receive the surveys. The Press Ganey survey contained 33 items covering across several subdomains including room, meal, nursing, physician, ancillary staff, visitor, discharge, and overall satisfaction. The HCAHPS survey contained 29 Centers for Medicare and Medicaid Services (CMS)‐mandated items, of which 21 are related to patient satisfaction. The development and testing and methods for administration and reporting of the HCAHPS survey have been previously described.[30, 31] Press Ganey patient satisfaction survey results have been reported in the literature.[32, 33]
Outcome Variables
Press Ganey and HCAHPS patient satisfaction survey responses were the primary outcome variables of the study. The survey items were categorized as facility related (eg, noise level), nonfacility related (eg, physician and nursing staff satisfaction), and overall satisfaction related.
Covariates
Age, sex, length of stay (LOS), insurance type, and all‐payer refined diagnosis‐related groupassociated illness complexity were included as covariates.
Statistical Analysis
Percent top‐box scores were calculated for each survey item as the percent of patients who responded very good for a given item on Press Ganey survey items and always or definitely yes or 9 or 10 on HCAHPS survey items. CMS utilizes percent top‐box scores to calculate payments under the Value Based Purchasing (VBP) program and to report the results publicly. Numerous studies have also reported percent top‐box scores for HCAHPS survey results.[31, 32, 33, 34]
Odds ratios of premove versus postmove percentage of top‐box scores, adjusted for age, sex, LOS, complexity of illness, and insurance type were determined using logistic regression for the units that moved. Similar scores were calculated for unmoved units to detect secular trends. To determine whether the differences between the moved and unmoved units were significant, we introduced the interaction term (moved vs unmoved unit status) (pre‐ vs postmove time period) into the logistic regression models and examined the adjusted P value for this term. All statistical analysis was performed using SAS Institute Inc.'s (Cary, NC) JMP Pro 10.0.0.
RESULTS
The study included 1648 respondents in the moved units in the baseline period (ie, units designated to move to a new clinical building) and 1373 respondents in the postmove period. There were 1593 respondents in the control group during the baseline period and 1049 respondents in the postmove period. For the units that moved, survey response rates were 28.5% prior to the move and 28.3% after the move. For the units that did not move, survey response rates were 20.9% prior to the move and 22.7% after the move. A majority of survey respondents on the nursing units that moved were white, male, and had private insurance (Table 1). There were no significant differences between respondents across these characteristics between the pre‐ and postmove periods. Mean age and LOS were also similar. For these units, there were 70.5% private rooms prior to the move and 100% after the move. For the unmoved units, 58.9% of the rooms were private in the baseline period and 72.7% were private in the study period. Similar to the units that moved, characteristics of the respondents on the unmoved units also did not differ significantly in the postmove period.
Patient demographics | Moved Units (N=3,021) | Unmoved Units (N=2,642) | ||||
---|---|---|---|---|---|---|
Pre | Post | P Value | Pre | Post | P Value | |
| ||||||
White | 75.3% | 78.2% | 0.07 | 66.7% | 68.5% | 0.31 |
Mean age, y | 57.3 | 57.4 | 0.84 | 57.3 | 57.1 | 0.81 |
Male | 54.3% | 53.0% | 0.48 | 40.5% | 42.3% | 0.23 |
Self‐reported health | ||||||
Excellent or very good | 54.7% | 51.2% | 0.04 | 38.7% | 39.5% | 0.11 |
Good | 27.8% | 32.0% | 29.3% | 32.2% | ||
Fair or poor | 17.5% | 16.9% | 32.0% | 28.3% | ||
Self‐reported language | ||||||
English | 96.0% | 97.2% | 0.06 | 96.8% | 97.1% | 0.63 |
Other | 4.0% | 2.8% | 3.2% | 2.9% | ||
Self‐reported education | ||||||
Less than high school | 5.8% | 5.0% | 0.24 | 10.8% | 10.4% | 0.24 |
High school grad | 46.4% | 44.2% | 48.6% | 45.5% | ||
College grad or more | 47.7% | 50.7% | 40.7% | 44.7% | ||
Insurance type | ||||||
Medicaid | 6.7% | 5.5% | 0.11 | 10.8% | 9.0% | 0.32 |
Medicare | 32.0% | 35.5% | 36.0% | 36.1% | ||
Private insurance | 55.6% | 52.8% | 48.0% | 50.3% | ||
Mean APRDRG complexity* | 2.1 | 2.1 | 0.09 | 2.3 | 2.3 | 0.14 |
Mean LOS | 4.7 | 5.0 | 0.12 | 4.9 | 5.0 | 0.77 |
Service | ||||||
Medicine | 15.4% | 16.2% | 0.51 | 40.0% | 34.5% | 0.10 |
Surgery | 50.7% | 45.7% | 40.1% | 44.1% | ||
Neurosciences | 20.3% | 24.1% | 6.0% | 6.0% | ||
Obstetrics/gynecology | 7.5% | 8.2% | 5.7% | 5.6% |
The move was associated with significant improvements in facility‐related satisfaction (Tables 2 and 3). The most prominent increases in satisfaction were with pleasantness of dcor (33.6% vs 66.2%), noise level (39.9% vs 59.3%), and visitor accommodation and comfort (50.0% vs 70.3 %). There was improvement in satisfaction related to cleanliness of the room (49.0% vs 68.6 %), but no significant increase in satisfaction with courtesy of the person cleaning the room (59.8% vs 67.7%) when compared with units that did move.
Satisfaction Domain | Moved Units | Unmoved Units | P Value of the Difference in Odds Ratio Between Moved and Unmoved Units | |||||
---|---|---|---|---|---|---|---|---|
% Top Box | Adjusted Odds Ratio* (95% CI) | % Top Box | Adjusted Odds Ratio* (95% CI) | |||||
Pre | Post | Pre | Post | |||||
| ||||||||
FACILITY RELATED | ||||||||
Hospital environment | ||||||||
Cleanliness of the room and bathroom | 61.0 | 70.8 | 1.62 (1.40‐1.90) | 64.0 | 69.2 | 1.24 (1.03‐1.48) | 0.03 | |
Quietness of the room | 51.3 | 65.4 | 1.89 (1.63‐2.19) | 58.6 | 60.3 | 1.08 (0.90‐1.28) | <0.0001 | |
NONFACILITY RELATED | ||||||||
Nursing communication | ||||||||
Nurses treated with courtesy/respect | 84.0 | 86.7 | 1.28 (1.05‐1.57) | 83.6 | 87.1 | 1.29 (1.02‐1.64) | 0.92 | |
Nurses listened | 73.1 | 76.4 | 1.21 (1.03‐1.43) | 74.2 | 75.5 | 1.05 (0.86‐1.27) | 0.26 | |
Nurses explained | 75.0 | 76.6 | 1.10 (0.94‐1.30) | 76.0 | 76.2 | 1.00 (0.82‐1.21) | 0.43 | |
Physician communication | ||||||||
Doctors treated with courtesy/respect | 89.5 | 90.5 | 1.13 (0.89‐1.42) | 84.9 | 87.3 | 1.20 (0.94‐1.53) | 0.77 | |
Doctors listened | 81.4 | 81.0 | 0.93 (0.83‐1.19) | 77.7 | 77.1 | 0.94 (0.77‐1.15) | 0.68 | |
Doctors explained | 79.2 | 79.0 | 1.00(0.84‐1.19) | 75.7 | 74.4 | 0.92 (0.76‐1.12) | 0.49 | |
Other | ||||||||
Help toileting as soon as you wanted | 61.8 | 63.7 | 1.08 (0.89‐1.32) | 62.3 | 60.6 | 0.92 (0.71‐1.18) | 0.31 | |
Pain well controlled | 63.2 | 63.8 | 1.06 (0.90‐1.25) | 62.0 | 62.6 | 0.99 (0.81‐1.20) | 060 | |
Staff do everything to help with pain | 77.7 | 80.1 | 1.19 (0.99‐1.44) | 76.8 | 75.7 | 0.90 (0.75‐1.13) | 0.07 | |
Staff describe medicine side effects | 47.0 | 47.6 | 1.05 (0.89‐1.24) | 49.2 | 47.1 | 0.91 (0.74‐1.11) | 0.32 | |
Tell you what new medicine was for | 76.4 | 76.4 | 1.02 (0.84‐1.25) | 77.1 | 78.8 | 1.09(0.85‐1.39) | 0.65 | |
Overall | ||||||||
Rate hospital (010) | 75.0 | 83.3 | 1.71 (1.44‐2.05) | 75.7 | 77.6 | 1.06 (0.87‐1.29) | 0.006 | |
Recommend hospital | 82.5 | 87.1 | 1.43 (1.18‐1.76) | 81.4 | 82.0 | 0.98 (0.79‐1.22) | 0.03 |
Satisfaction Domain | Moved Unit | Unmoved Unit | P Value of the Difference in Odds Ratio Between Moved and Unmoved Units | ||||
---|---|---|---|---|---|---|---|
% Top Box | Adjusted Odds Ratio* (95% CI) | % Top Box | Adjusted Odds Ratio* (95% CI) | ||||
Pre | Post | Pre | Post | ||||
| |||||||
FACILITY RELATED | |||||||
Room | |||||||
Pleasantness of room dcor | 33.6 | 64.8 | 3.77 (3.24‐4.38) | 41.6 | 47.0 | 1.21 (1.02‐1.44) | <0.0001 |
Room cleanliness | 49.0 | 68.6 | 2.35 (2.02‐2.73) | 51.6 | 59.1 | 1.32 (1.12‐1.58) | <0.0001 |
Room temperature | 43.1 | 54.9 | 1.64 (1.43‐1.90) | 45.0 | 48.8 | 1.14 (0.96‐1.36) | 0.002 |
Noise level in and around the room | 40.2 | 59.2 | 2.23 (1.92‐2.58) | 45.5 | 47.6 | 1.07 (0.90‐1.22) | <0.0001 |
Visitor related | |||||||
Accommodations and comfort of visitors | 50.0 | 70.3 | 2.44 (2.10‐2.83) | 55.3 | 59.1 | 1.14 (0.96‐1.35) | <0.0001 |
NONFACILITY RELATED | |||||||
Food | |||||||
Temperature of the food | 31.1 | 33.6 | 1.15 (0.99‐1.34) | 34.0 | 38.9 | 1.23 (1.02‐1.47) | 0.51 |
Quality of the food | 25.8 | 27.1 | 1.10 (0.93‐1.30) | 30.2 | 36.2 | 1.32 (1.10‐1.59) | 0.12 |
Courtesy of the person who served food | 63.9 | 62.3 | 0.93 (0.80‐1.10) | 66.0 | 61.4 | 0.82 (0.69‐0.98) | 0.26 |
Nursing | |||||||
Friendliness/courtesy of the nurses | 76.3 | 82.8 | 1.49 (1.26‐1.79) | 77.7 | 80.1 | 1.10 (0.90‐1.37) | 0.04 |
Promptness of response to call | 60.1 | 62.6 | 1.14 (0.98‐1.33) | 59.2 | 62.0 | 1.10 (0.91‐1.31) | 0.80 |
Nurses' attitude toward requests | 71.0 | 75.8 | 1.30 (1.11‐1.54) | 70.5 | 72.4 | 1.06 (0.88‐1.28) | 0.13 |
Attention to special/personal needs | 66.7 | 72.2 | 1.32 (1.13‐1.54) | 67.8 | 70.3 | 1.09 (0.91‐1.31) | 0.16 |
Nurses kept you informed | 64.3 | 72.2 | 1.46 (1.25‐1.70) | 65.8 | 69.8 | 1.17 (0.98‐1.41) | 0.88 |
Skill of the nurses | 75.3 | 79.5 | 1.28 (1.08‐1.52) | 74.3 | 78.6 | 1.23 (1.01‐1.51) | 0.89 |
Ancillary staff | |||||||
Courtesy of the person cleaning the room | 59.8 | 67.7 | 1.41 (1.21‐1.65) | 61.2 | 66.5 | 1.24 (1.03‐1.49) | 0.28 |
Courtesy of the person who took blood | 66.5 | 68.1 | 1.10 (0.94‐1.28) | 63.2 | 63.1 | 0.96 (0.76‐1.08) | 0.34 |
Courtesy of the person who started the IV | 70.0 | 71.7 | 1.09 (0.93‐1.28) | 66.6 | 69.3 | 1.11 (0.92‐1.33) | 0.88 |
Visitor related | |||||||
Staff attitude toward visitors | 68.1 | 79.4 | 1.84 (1.56‐2.18) | 70.3 | 72.2 | 1.06 (0.87‐1.28) | <0.0001 |
Physician | |||||||
Time physician spent with you | 55.0 | 58.9 | 1.20 (1.04‐1.39) | 53.2 | 55.9 | 1.10 (0.92‐1.30) | 0.46 |
Physician concern questions/worries | 67.2 | 70.7 | 1.20 (1.03‐1.40) | 64.3 | 66.1 | 1.05 (0.88‐1.26) | 0.31 |
Physician kept you informed | 65.3 | 67.5 | 1.12 (0.96‐1.30) | 61.6 | 63.2 | 1.05 (0.88‐1.25) | 0.58 |
Friendliness/courtesy of physician | 76.3 | 78.1 | 1.11 (0.93‐1.31) | 71.0 | 73.3 | 1.08 (0.90‐1.31) | 0.89 |
Skill of physician | 85.4 | 88.5 | 1.35 (1.09‐1.68) | 78.0 | 81.0 | 1.15 (0.93‐1.43) | 0.34 |
Discharge | |||||||
Extent felt ready for discharge | 62.0 | 66.7 | 1.23 (1.07‐1.44) | 59.2 | 62.3 | 1.10 (0.92‐1.30) | 0.35 |
Speed of discharge process | 50.7 | 54.2 | 1.16 (1.01‐1.33) | 47.8 | 50.0 | 1.07 (0.90‐1.27) | 0.49 |
Instructions for care at home | 66.4 | 71.1 | 1.25 (1.06‐1.46) | 64.0 | 67.7 | 1.16 (0.97‐1.39) | 0.54 |
Staff concern for your privacy | 65.3 | 71.8 | 1.37 (1.17‐0.85) | 63.6 | 66.2 | 1.10 (0.91‐1.31) | 0.07 |
Miscellaneous | |||||||
How well your pain was controlled | 64.2 | 66.5 | 1.14 (0.97‐1.32) | 60.2 | 62.6 | 1.07 (0.89‐1.28) | 0.66 |
Staff addressed emotional needs | 60.0 | 63.4 | 1.19 (1.02‐1.38) | 55.1 | 60.2 | 1.20 (1.01‐1.42) | 0.90 |
Response to concerns/complaints | 61.1 | 64.5 | 1.19 (1.02‐1.38) | 57.2 | 60.1 | 1.10 (0.92‐1.31) | 0.57 |
Overall | |||||||
Staff worked together to care for you | 72.6 | 77.2 | 1.29 (1.10‐1.52) | 70.3 | 73.2 | 1.13 (0.93‐1.37) | 0.30 |
Likelihood of recommending hospital | 79.1 | 84.3 | 1.44 (1.20‐1.74) | 76.3 | 79.2 | 1.14 (0.93‐1.39) | 0.10 |
Overall rating of care given | 76.8 | 83.0 | 1.50 (1.25‐1.80) | 74.7 | 77.2 | 1.10 (0.90‐1.34) | 0.03 |
With regard to nonfacility‐related satisfaction, there were statistically higher scores in several nursing, physician, and discharge‐related satisfaction domains after the move. However, these changes were not associated with the move to the new clinical building as they were not significantly different from improvements on the unmoved units. Among nonfacility‐related items, only staff attitude toward visitors showed significant improvement (68.1% vs 79.4%). There was a significant improvement in hospital rating (75.0% vs 83.3% in the moved units and 75.7% vs 77.6% in the unmoved units). However, the other 3 measures of overall satisfaction did not show significant improvement associated with the move to the new clinical building when compared to the concurrent controls.
DISCUSSION
Contrary to our hypothesis and a belief held by many, we found that patients appeared able to distinguish their experience with hospital environment from their experience with providers and other services. Improvement in hospital facilities with incorporation of patient‐centered features was associated with improvements that were largely limited to increases in satisfaction with quietness, cleanliness, temperature, and dcor of the room along with visitor‐related satisfaction. Notably, there was no significant improvement in satisfaction related to physicians, nurses, housekeeping, and other service staff. There was improvement in satisfaction with staff attitude toward visitors, but this can be attributed to availability of visitor‐friendly facilities. There was a significant improvement in 1 of the 4 measures of overall satisfaction. Our findings also support the construct validity of HCAHPS and Press Ganey patient satisfaction surveys.
Ours is one of the largest studies on patient satisfaction related to patient‐centered design features in the inpatient acute care setting. Swan et al. also studied patients in an acute inpatient setting and compared satisfaction related to appealing versus typical hospital rooms. Patients were matched for case mix, insurance, gender, types of medical services received and LOS, and were served by the same set of physicians and similar food service and housekeeping staff.[26] Unlike our study, they found improved satisfaction related to physicians, housekeeping staff, food service staff, meals, and overall satisfaction. However, the study had some limitations. In particular, the study sample was self‐selected because the patients in this group were required to pay an extra daily fee to utilize the appealing room. Additionally, there were only 177 patients across the 2 groups, and the actual differences in satisfaction scores were small. Our sample was larger and patients in the study group were admitted to units in the new clinical buildings by the same criteria as they were admitted to the historic building prior to the move, and there were no significant differences in baseline characteristics between the comparison groups.
Jansen et al. also found broad improvements in patient satisfaction in a study of over 309 maternity unit patients in a new construction, all private‐room maternity unit with more appealing design elements and comfort features for visitors.[7] Improved satisfaction was noted with the physical environment, nursing care, assistance with feeding, respect for privacy, and discharge planning. However, it is difficult to extrapolate the results of this study to other settings, as maternity unit patients constitute a unique patient demographic with unique care needs. Additionally, when compared with patients in the control group, the patients in the study group were cared for by nurses who had a lower workload and who were not assigned other patients with more complex needs. Because nursing availability may be expected to impact satisfaction with clinical domains, the impact of private and appealing room may very well have been limited to improved satisfaction with the physical environment.
Despite the widespread belief among healthcare leadership that facility renovation or expansion is a vital strategy for improving patient satisfaction, our study shows that this may not be a dominant factor.[27] In fact, the Planetree model showed that improvement in satisfaction related to physical environment and nursing care was associated with implementation of both patient‐centered design features as well as with utilization of nurses that were trained to provide personalized care, educate patients, and involve patients and family.[28] It is more likely that provider‐level interventions will have a greater impact on provider level and overall satisfaction. This idea is supported by a recent JD Powers study suggesting that facilities represent only 19% of overall satisfaction in the inpatient setting.[35]
Although our study focused on patient‐centered design features, several renovation and construction projects have also focused on design features that improve patient safety and provider satisfaction, workflow, efficiency, productivity, stress, and time spent in direct care.[9] Interventions in these areas may lead to improvement in patient outcomes and perhaps lead to improvement in patient satisfaction; however, this relationship has not been well established at present.
In an era of cost containment, healthcare administrators are faced with high‐priced interventions, competing needs, limited resources, low profit margins, and often unclear evidence on cost‐effectiveness and return on investment of healthcare design features. Benefits are related to competitive advantage, higher reputation, patient retention, decreased malpractice costs, and increased Medicare payments through VBP programs that incentivize improved performance on quality metrics and patient satisfaction surveys. Our study supports the idea that a significant improvement in patient satisfaction related to creature comforts can be achieved with investment in patient‐centered design features. However, our findings also suggest that institutions should perform an individualized cost‐benefit analysis related to improvements in this narrow area of patient satisfaction. In our study, incorporation of patient‐centered design features resulted in improvement on 2 VBP HCAHPS measures, and its contribution toward total performance score under the VBP program would be limited.
Strengths of our study include the use of concurrent controls and our ability to capitalize on a natural experiment in which care teams remained constant before and after a move to a new clinical building. However, our study has some limitations. It was conducted at a single tertiary care academic center that predominantly serves an inner city population and referral patients seeking specialized care. Drivers of patient satisfaction may be different in community hospitals, and a different relationship may be observed between patient‐centered design and domains of patient satisfaction in this setting. Further studies in different hospital settings are needed to confirm our findings. Additionally, we were limited by the low response rate of the surveys. However, this is a widespread problem with all patient satisfaction research utilizing voluntary surveys, and our response rates are consistent with those previously reported.[34, 36, 37, 38] Furthermore, low response rates have not impeded the implementation of pay‐for‐performance programs on a national scale using HCHAPS.
In conclusion, our study suggests that hospitals should not use outdated facilities as an excuse for achievement of suboptimal satisfaction scores. Patients respond positively to creature comforts, pleasing surroundings, and visitor‐friendly facilities but can distinguish these positive experiences from experiences in other patient satisfaction domains. In our study, the move to a higher‐amenity building had only a modest impact on overall patient satisfaction, perhaps because clinical care is the primary driver of this outcome. Contrary to belief held by some hospital leaders, major strides in overall satisfaction across the board and other subdomains of satisfaction likely require intervention in areas other than facility renovation and expansion.
Disclosures
Zishan Siddiqui, MD, was supported by the Osler Center of Clinical Excellence Faculty Scholarship Grant. Funds from Johns Hopkins Hospitalist Scholars Program supported the research project. The authors have no conflict of interests to disclose.
Hospitals are expensive and complex facilities to build and renovate. It is estimated $200 billion is being spent in the United States during this decade on hospital construction and renovation, and further expenditures in this area are expected.[1] Aging hospital infrastructure, competition, and health system expansion have motivated institutions to invest in renovation and new hospital building construction.[2, 3, 4, 5, 6, 7] There is a trend toward patient‐centered design in new hospital construction. Features of this trend include same‐handed design (ie, rooms on a unit have all beds oriented in the same direction and do not share headwalls); use of sound absorbent materials to reduced ambient noise[7, 8, 9]; rooms with improved view and increased natural lighting to reduce anxiety, decrease delirium, and increase sense of wellbeing[10, 11, 12]; incorporation of natural elements like gardens, water features, and art[12, 13, 14, 15, 16, 17, 18]; single‐patient rooms to reduce transmission of infection and enhance privacy and visitor comfort[7, 19, 20]; presence of comfortable waiting rooms and visitor accommodations to enhance comfort and family participation[21, 22, 23]; and hotel‐like amenities such as on‐demand entertainment and room service menus.[24, 25]
There is a belief among some hospital leaders that patients are generally unable to distinguish their positive experience with a pleasing healthcare environment from their positive experience with care, and thus improving facilities will lead to improved satisfaction across the board.[26, 27] In a controlled study of hospitalized patients, appealing rooms were associated with increased satisfaction with services including housekeeping and food service staff, meals, as well as physicians and overall satisfaction.[26] A 2012 survey of hospital leadership found that expanding and renovating facilities was considered a top priority in improving patient satisfaction, with 82% of the respondents stating that this was important.[27]
Despite these attitudes, the impact of patient‐centered design on patient satisfaction is not well understood. Studies have shown that renovations and hospital construction that incorporates noise reduction strategies, positive distraction, patient and caregiver control, attractive waiting rooms, improved patient room appearance, private rooms, and large windows result in improved satisfaction with nursing, noise level, unit environment and cleanliness, perceived wait time, discharge preparedness, and overall care. [7, 19, 20, 23, 28] However, these studies were limited by small sample size, inclusion of a narrow group of patients (eg, ambulatory, obstetric, geriatric rehabilitation, intensive care unit), and concurrent use of interventions other than design improvement (eg, nurse and patient education). Many of these studies did not use the ubiquitous Hospital Consumer Assessment of Healthcare Providers and Systems (HCAHPS) and Press Ganey patient satisfaction surveys.
We sought to determine the changes in patient satisfaction that occurred during a natural experiment, in which clinical units (comprising stable nursing, physician, and unit teams) were relocated from an historic clinical building to a new clinical building that featured patient‐centered design, using HCAHPS and Press Ganey surveys and a large study population. We hypothesized that new building features would positively impact both facility related (eg, noise level), nonfacility related (eg, physician and housekeeping service related), and overall satisfaction.
METHODS
This was a retrospective analysis of prospectively collected Press Ganey and HCAPHS patient satisfaction survey data for a single academic tertiary care hospital.[29] The research project was reviewed and approved by the institutional review board.
Participants
All patients discharged from 12 clinical units that relocated to the new clinical building and returned patient satisfaction surveys served as study patients. The moved units included the coronary care unit, cardiac step down unit, medical intensive care unit, neuro critical care unit, surgical intensive care unit, orthopedic unit, neurology unit, neurosurgery unit, obstetrics units, gynecology unit, urology unit, cardiothoracic surgery unit, and the transplant surgery and renal transplant unit. Patients on clinical units that did not move served as concurrent controls.
Exposure
Patients admitted to the new clinical building experienced several patient‐centered design features. These features included easy access to healing gardens with a water feature, soaring lobbies, a collection of more than 500 works of art, well‐decorated and light‐filled patient rooms with sleeping accommodations for family members, sound‐absorbing features in patient care corridors ranging from acoustical ceiling tiles to a quiet nurse‐call system, and an interactive television network with Internet, movies, and games. All patients during the baseline period and control patients during the study period were located in typical patient rooms with standard hospital amenities. No other major patient satisfaction interventions were initiated during the pre‐ or postperiod in either arm of the study; ongoing patient satisfaction efforts (such as unit‐based customer care representatives) were deployed broadly and not restricted to the new clinical building. Clinical teams comprised of physicians, nurses, and ancillary staff did not change significantly after the move.
Time Periods
The move to new clinical building occurred on May 1, 2012. After allowing for a 15‐day washout period, the postmove period included Press Ganey and HCAHPS surveys returned for discharges that occurred during a 7.5‐month period between May 15, 2102 and December 31, 2012. Baseline data included Press Ganey and HCAHPS surveys returned for discharges in the preceding 12 months (May 1, 2011 to April 30, 2012). Sensitivity analysis using only 7.5 months of baseline data did not reveal any significant difference when compared with 12‐month baseline data, and we report only data from the 12‐month baseline period.
Instruments
Press Ganey and HCAHPS patient satisfaction surveys were sent via mail in the same envelope. Fifty percent of the discharged patients were randomized to receive the surveys. The Press Ganey survey contained 33 items covering across several subdomains including room, meal, nursing, physician, ancillary staff, visitor, discharge, and overall satisfaction. The HCAHPS survey contained 29 Centers for Medicare and Medicaid Services (CMS)‐mandated items, of which 21 are related to patient satisfaction. The development and testing and methods for administration and reporting of the HCAHPS survey have been previously described.[30, 31] Press Ganey patient satisfaction survey results have been reported in the literature.[32, 33]
Outcome Variables
Press Ganey and HCAHPS patient satisfaction survey responses were the primary outcome variables of the study. The survey items were categorized as facility related (eg, noise level), nonfacility related (eg, physician and nursing staff satisfaction), and overall satisfaction related.
Covariates
Age, sex, length of stay (LOS), insurance type, and all‐payer refined diagnosis‐related groupassociated illness complexity were included as covariates.
Statistical Analysis
Percent top‐box scores were calculated for each survey item as the percent of patients who responded very good for a given item on Press Ganey survey items and always or definitely yes or 9 or 10 on HCAHPS survey items. CMS utilizes percent top‐box scores to calculate payments under the Value Based Purchasing (VBP) program and to report the results publicly. Numerous studies have also reported percent top‐box scores for HCAHPS survey results.[31, 32, 33, 34]
Odds ratios of premove versus postmove percentage of top‐box scores, adjusted for age, sex, LOS, complexity of illness, and insurance type were determined using logistic regression for the units that moved. Similar scores were calculated for unmoved units to detect secular trends. To determine whether the differences between the moved and unmoved units were significant, we introduced the interaction term (moved vs unmoved unit status) (pre‐ vs postmove time period) into the logistic regression models and examined the adjusted P value for this term. All statistical analysis was performed using SAS Institute Inc.'s (Cary, NC) JMP Pro 10.0.0.
RESULTS
The study included 1648 respondents in the moved units in the baseline period (ie, units designated to move to a new clinical building) and 1373 respondents in the postmove period. There were 1593 respondents in the control group during the baseline period and 1049 respondents in the postmove period. For the units that moved, survey response rates were 28.5% prior to the move and 28.3% after the move. For the units that did not move, survey response rates were 20.9% prior to the move and 22.7% after the move. A majority of survey respondents on the nursing units that moved were white, male, and had private insurance (Table 1). There were no significant differences between respondents across these characteristics between the pre‐ and postmove periods. Mean age and LOS were also similar. For these units, there were 70.5% private rooms prior to the move and 100% after the move. For the unmoved units, 58.9% of the rooms were private in the baseline period and 72.7% were private in the study period. Similar to the units that moved, characteristics of the respondents on the unmoved units also did not differ significantly in the postmove period.
Patient demographics | Moved Units (N=3,021) | Unmoved Units (N=2,642) | ||||
---|---|---|---|---|---|---|
Pre | Post | P Value | Pre | Post | P Value | |
| ||||||
White | 75.3% | 78.2% | 0.07 | 66.7% | 68.5% | 0.31 |
Mean age, y | 57.3 | 57.4 | 0.84 | 57.3 | 57.1 | 0.81 |
Male | 54.3% | 53.0% | 0.48 | 40.5% | 42.3% | 0.23 |
Self‐reported health | ||||||
Excellent or very good | 54.7% | 51.2% | 0.04 | 38.7% | 39.5% | 0.11 |
Good | 27.8% | 32.0% | 29.3% | 32.2% | ||
Fair or poor | 17.5% | 16.9% | 32.0% | 28.3% | ||
Self‐reported language | ||||||
English | 96.0% | 97.2% | 0.06 | 96.8% | 97.1% | 0.63 |
Other | 4.0% | 2.8% | 3.2% | 2.9% | ||
Self‐reported education | ||||||
Less than high school | 5.8% | 5.0% | 0.24 | 10.8% | 10.4% | 0.24 |
High school grad | 46.4% | 44.2% | 48.6% | 45.5% | ||
College grad or more | 47.7% | 50.7% | 40.7% | 44.7% | ||
Insurance type | ||||||
Medicaid | 6.7% | 5.5% | 0.11 | 10.8% | 9.0% | 0.32 |
Medicare | 32.0% | 35.5% | 36.0% | 36.1% | ||
Private insurance | 55.6% | 52.8% | 48.0% | 50.3% | ||
Mean APRDRG complexity* | 2.1 | 2.1 | 0.09 | 2.3 | 2.3 | 0.14 |
Mean LOS | 4.7 | 5.0 | 0.12 | 4.9 | 5.0 | 0.77 |
Service | ||||||
Medicine | 15.4% | 16.2% | 0.51 | 40.0% | 34.5% | 0.10 |
Surgery | 50.7% | 45.7% | 40.1% | 44.1% | ||
Neurosciences | 20.3% | 24.1% | 6.0% | 6.0% | ||
Obstetrics/gynecology | 7.5% | 8.2% | 5.7% | 5.6% |
The move was associated with significant improvements in facility‐related satisfaction (Tables 2 and 3). The most prominent increases in satisfaction were with pleasantness of dcor (33.6% vs 66.2%), noise level (39.9% vs 59.3%), and visitor accommodation and comfort (50.0% vs 70.3 %). There was improvement in satisfaction related to cleanliness of the room (49.0% vs 68.6 %), but no significant increase in satisfaction with courtesy of the person cleaning the room (59.8% vs 67.7%) when compared with units that did move.
Satisfaction Domain | Moved Units | Unmoved Units | P Value of the Difference in Odds Ratio Between Moved and Unmoved Units | |||||
---|---|---|---|---|---|---|---|---|
% Top Box | Adjusted Odds Ratio* (95% CI) | % Top Box | Adjusted Odds Ratio* (95% CI) | |||||
Pre | Post | Pre | Post | |||||
| ||||||||
FACILITY RELATED | ||||||||
Hospital environment | ||||||||
Cleanliness of the room and bathroom | 61.0 | 70.8 | 1.62 (1.40‐1.90) | 64.0 | 69.2 | 1.24 (1.03‐1.48) | 0.03 | |
Quietness of the room | 51.3 | 65.4 | 1.89 (1.63‐2.19) | 58.6 | 60.3 | 1.08 (0.90‐1.28) | <0.0001 | |
NONFACILITY RELATED | ||||||||
Nursing communication | ||||||||
Nurses treated with courtesy/respect | 84.0 | 86.7 | 1.28 (1.05‐1.57) | 83.6 | 87.1 | 1.29 (1.02‐1.64) | 0.92 | |
Nurses listened | 73.1 | 76.4 | 1.21 (1.03‐1.43) | 74.2 | 75.5 | 1.05 (0.86‐1.27) | 0.26 | |
Nurses explained | 75.0 | 76.6 | 1.10 (0.94‐1.30) | 76.0 | 76.2 | 1.00 (0.82‐1.21) | 0.43 | |
Physician communication | ||||||||
Doctors treated with courtesy/respect | 89.5 | 90.5 | 1.13 (0.89‐1.42) | 84.9 | 87.3 | 1.20 (0.94‐1.53) | 0.77 | |
Doctors listened | 81.4 | 81.0 | 0.93 (0.83‐1.19) | 77.7 | 77.1 | 0.94 (0.77‐1.15) | 0.68 | |
Doctors explained | 79.2 | 79.0 | 1.00(0.84‐1.19) | 75.7 | 74.4 | 0.92 (0.76‐1.12) | 0.49 | |
Other | ||||||||
Help toileting as soon as you wanted | 61.8 | 63.7 | 1.08 (0.89‐1.32) | 62.3 | 60.6 | 0.92 (0.71‐1.18) | 0.31 | |
Pain well controlled | 63.2 | 63.8 | 1.06 (0.90‐1.25) | 62.0 | 62.6 | 0.99 (0.81‐1.20) | 060 | |
Staff do everything to help with pain | 77.7 | 80.1 | 1.19 (0.99‐1.44) | 76.8 | 75.7 | 0.90 (0.75‐1.13) | 0.07 | |
Staff describe medicine side effects | 47.0 | 47.6 | 1.05 (0.89‐1.24) | 49.2 | 47.1 | 0.91 (0.74‐1.11) | 0.32 | |
Tell you what new medicine was for | 76.4 | 76.4 | 1.02 (0.84‐1.25) | 77.1 | 78.8 | 1.09(0.85‐1.39) | 0.65 | |
Overall | ||||||||
Rate hospital (010) | 75.0 | 83.3 | 1.71 (1.44‐2.05) | 75.7 | 77.6 | 1.06 (0.87‐1.29) | 0.006 | |
Recommend hospital | 82.5 | 87.1 | 1.43 (1.18‐1.76) | 81.4 | 82.0 | 0.98 (0.79‐1.22) | 0.03 |
Satisfaction Domain | Moved Unit | Unmoved Unit | P Value of the Difference in Odds Ratio Between Moved and Unmoved Units | ||||
---|---|---|---|---|---|---|---|
% Top Box | Adjusted Odds Ratio* (95% CI) | % Top Box | Adjusted Odds Ratio* (95% CI) | ||||
Pre | Post | Pre | Post | ||||
| |||||||
FACILITY RELATED | |||||||
Room | |||||||
Pleasantness of room dcor | 33.6 | 64.8 | 3.77 (3.24‐4.38) | 41.6 | 47.0 | 1.21 (1.02‐1.44) | <0.0001 |
Room cleanliness | 49.0 | 68.6 | 2.35 (2.02‐2.73) | 51.6 | 59.1 | 1.32 (1.12‐1.58) | <0.0001 |
Room temperature | 43.1 | 54.9 | 1.64 (1.43‐1.90) | 45.0 | 48.8 | 1.14 (0.96‐1.36) | 0.002 |
Noise level in and around the room | 40.2 | 59.2 | 2.23 (1.92‐2.58) | 45.5 | 47.6 | 1.07 (0.90‐1.22) | <0.0001 |
Visitor related | |||||||
Accommodations and comfort of visitors | 50.0 | 70.3 | 2.44 (2.10‐2.83) | 55.3 | 59.1 | 1.14 (0.96‐1.35) | <0.0001 |
NONFACILITY RELATED | |||||||
Food | |||||||
Temperature of the food | 31.1 | 33.6 | 1.15 (0.99‐1.34) | 34.0 | 38.9 | 1.23 (1.02‐1.47) | 0.51 |
Quality of the food | 25.8 | 27.1 | 1.10 (0.93‐1.30) | 30.2 | 36.2 | 1.32 (1.10‐1.59) | 0.12 |
Courtesy of the person who served food | 63.9 | 62.3 | 0.93 (0.80‐1.10) | 66.0 | 61.4 | 0.82 (0.69‐0.98) | 0.26 |
Nursing | |||||||
Friendliness/courtesy of the nurses | 76.3 | 82.8 | 1.49 (1.26‐1.79) | 77.7 | 80.1 | 1.10 (0.90‐1.37) | 0.04 |
Promptness of response to call | 60.1 | 62.6 | 1.14 (0.98‐1.33) | 59.2 | 62.0 | 1.10 (0.91‐1.31) | 0.80 |
Nurses' attitude toward requests | 71.0 | 75.8 | 1.30 (1.11‐1.54) | 70.5 | 72.4 | 1.06 (0.88‐1.28) | 0.13 |
Attention to special/personal needs | 66.7 | 72.2 | 1.32 (1.13‐1.54) | 67.8 | 70.3 | 1.09 (0.91‐1.31) | 0.16 |
Nurses kept you informed | 64.3 | 72.2 | 1.46 (1.25‐1.70) | 65.8 | 69.8 | 1.17 (0.98‐1.41) | 0.88 |
Skill of the nurses | 75.3 | 79.5 | 1.28 (1.08‐1.52) | 74.3 | 78.6 | 1.23 (1.01‐1.51) | 0.89 |
Ancillary staff | |||||||
Courtesy of the person cleaning the room | 59.8 | 67.7 | 1.41 (1.21‐1.65) | 61.2 | 66.5 | 1.24 (1.03‐1.49) | 0.28 |
Courtesy of the person who took blood | 66.5 | 68.1 | 1.10 (0.94‐1.28) | 63.2 | 63.1 | 0.96 (0.76‐1.08) | 0.34 |
Courtesy of the person who started the IV | 70.0 | 71.7 | 1.09 (0.93‐1.28) | 66.6 | 69.3 | 1.11 (0.92‐1.33) | 0.88 |
Visitor related | |||||||
Staff attitude toward visitors | 68.1 | 79.4 | 1.84 (1.56‐2.18) | 70.3 | 72.2 | 1.06 (0.87‐1.28) | <0.0001 |
Physician | |||||||
Time physician spent with you | 55.0 | 58.9 | 1.20 (1.04‐1.39) | 53.2 | 55.9 | 1.10 (0.92‐1.30) | 0.46 |
Physician concern questions/worries | 67.2 | 70.7 | 1.20 (1.03‐1.40) | 64.3 | 66.1 | 1.05 (0.88‐1.26) | 0.31 |
Physician kept you informed | 65.3 | 67.5 | 1.12 (0.96‐1.30) | 61.6 | 63.2 | 1.05 (0.88‐1.25) | 0.58 |
Friendliness/courtesy of physician | 76.3 | 78.1 | 1.11 (0.93‐1.31) | 71.0 | 73.3 | 1.08 (0.90‐1.31) | 0.89 |
Skill of physician | 85.4 | 88.5 | 1.35 (1.09‐1.68) | 78.0 | 81.0 | 1.15 (0.93‐1.43) | 0.34 |
Discharge | |||||||
Extent felt ready for discharge | 62.0 | 66.7 | 1.23 (1.07‐1.44) | 59.2 | 62.3 | 1.10 (0.92‐1.30) | 0.35 |
Speed of discharge process | 50.7 | 54.2 | 1.16 (1.01‐1.33) | 47.8 | 50.0 | 1.07 (0.90‐1.27) | 0.49 |
Instructions for care at home | 66.4 | 71.1 | 1.25 (1.06‐1.46) | 64.0 | 67.7 | 1.16 (0.97‐1.39) | 0.54 |
Staff concern for your privacy | 65.3 | 71.8 | 1.37 (1.17‐0.85) | 63.6 | 66.2 | 1.10 (0.91‐1.31) | 0.07 |
Miscellaneous | |||||||
How well your pain was controlled | 64.2 | 66.5 | 1.14 (0.97‐1.32) | 60.2 | 62.6 | 1.07 (0.89‐1.28) | 0.66 |
Staff addressed emotional needs | 60.0 | 63.4 | 1.19 (1.02‐1.38) | 55.1 | 60.2 | 1.20 (1.01‐1.42) | 0.90 |
Response to concerns/complaints | 61.1 | 64.5 | 1.19 (1.02‐1.38) | 57.2 | 60.1 | 1.10 (0.92‐1.31) | 0.57 |
Overall | |||||||
Staff worked together to care for you | 72.6 | 77.2 | 1.29 (1.10‐1.52) | 70.3 | 73.2 | 1.13 (0.93‐1.37) | 0.30 |
Likelihood of recommending hospital | 79.1 | 84.3 | 1.44 (1.20‐1.74) | 76.3 | 79.2 | 1.14 (0.93‐1.39) | 0.10 |
Overall rating of care given | 76.8 | 83.0 | 1.50 (1.25‐1.80) | 74.7 | 77.2 | 1.10 (0.90‐1.34) | 0.03 |
With regard to nonfacility‐related satisfaction, there were statistically higher scores in several nursing, physician, and discharge‐related satisfaction domains after the move. However, these changes were not associated with the move to the new clinical building as they were not significantly different from improvements on the unmoved units. Among nonfacility‐related items, only staff attitude toward visitors showed significant improvement (68.1% vs 79.4%). There was a significant improvement in hospital rating (75.0% vs 83.3% in the moved units and 75.7% vs 77.6% in the unmoved units). However, the other 3 measures of overall satisfaction did not show significant improvement associated with the move to the new clinical building when compared to the concurrent controls.
DISCUSSION
Contrary to our hypothesis and a belief held by many, we found that patients appeared able to distinguish their experience with hospital environment from their experience with providers and other services. Improvement in hospital facilities with incorporation of patient‐centered features was associated with improvements that were largely limited to increases in satisfaction with quietness, cleanliness, temperature, and dcor of the room along with visitor‐related satisfaction. Notably, there was no significant improvement in satisfaction related to physicians, nurses, housekeeping, and other service staff. There was improvement in satisfaction with staff attitude toward visitors, but this can be attributed to availability of visitor‐friendly facilities. There was a significant improvement in 1 of the 4 measures of overall satisfaction. Our findings also support the construct validity of HCAHPS and Press Ganey patient satisfaction surveys.
Ours is one of the largest studies on patient satisfaction related to patient‐centered design features in the inpatient acute care setting. Swan et al. also studied patients in an acute inpatient setting and compared satisfaction related to appealing versus typical hospital rooms. Patients were matched for case mix, insurance, gender, types of medical services received and LOS, and were served by the same set of physicians and similar food service and housekeeping staff.[26] Unlike our study, they found improved satisfaction related to physicians, housekeeping staff, food service staff, meals, and overall satisfaction. However, the study had some limitations. In particular, the study sample was self‐selected because the patients in this group were required to pay an extra daily fee to utilize the appealing room. Additionally, there were only 177 patients across the 2 groups, and the actual differences in satisfaction scores were small. Our sample was larger and patients in the study group were admitted to units in the new clinical buildings by the same criteria as they were admitted to the historic building prior to the move, and there were no significant differences in baseline characteristics between the comparison groups.
Jansen et al. also found broad improvements in patient satisfaction in a study of over 309 maternity unit patients in a new construction, all private‐room maternity unit with more appealing design elements and comfort features for visitors.[7] Improved satisfaction was noted with the physical environment, nursing care, assistance with feeding, respect for privacy, and discharge planning. However, it is difficult to extrapolate the results of this study to other settings, as maternity unit patients constitute a unique patient demographic with unique care needs. Additionally, when compared with patients in the control group, the patients in the study group were cared for by nurses who had a lower workload and who were not assigned other patients with more complex needs. Because nursing availability may be expected to impact satisfaction with clinical domains, the impact of private and appealing room may very well have been limited to improved satisfaction with the physical environment.
Despite the widespread belief among healthcare leadership that facility renovation or expansion is a vital strategy for improving patient satisfaction, our study shows that this may not be a dominant factor.[27] In fact, the Planetree model showed that improvement in satisfaction related to physical environment and nursing care was associated with implementation of both patient‐centered design features as well as with utilization of nurses that were trained to provide personalized care, educate patients, and involve patients and family.[28] It is more likely that provider‐level interventions will have a greater impact on provider level and overall satisfaction. This idea is supported by a recent JD Powers study suggesting that facilities represent only 19% of overall satisfaction in the inpatient setting.[35]
Although our study focused on patient‐centered design features, several renovation and construction projects have also focused on design features that improve patient safety and provider satisfaction, workflow, efficiency, productivity, stress, and time spent in direct care.[9] Interventions in these areas may lead to improvement in patient outcomes and perhaps lead to improvement in patient satisfaction; however, this relationship has not been well established at present.
In an era of cost containment, healthcare administrators are faced with high‐priced interventions, competing needs, limited resources, low profit margins, and often unclear evidence on cost‐effectiveness and return on investment of healthcare design features. Benefits are related to competitive advantage, higher reputation, patient retention, decreased malpractice costs, and increased Medicare payments through VBP programs that incentivize improved performance on quality metrics and patient satisfaction surveys. Our study supports the idea that a significant improvement in patient satisfaction related to creature comforts can be achieved with investment in patient‐centered design features. However, our findings also suggest that institutions should perform an individualized cost‐benefit analysis related to improvements in this narrow area of patient satisfaction. In our study, incorporation of patient‐centered design features resulted in improvement on 2 VBP HCAHPS measures, and its contribution toward total performance score under the VBP program would be limited.
Strengths of our study include the use of concurrent controls and our ability to capitalize on a natural experiment in which care teams remained constant before and after a move to a new clinical building. However, our study has some limitations. It was conducted at a single tertiary care academic center that predominantly serves an inner city population and referral patients seeking specialized care. Drivers of patient satisfaction may be different in community hospitals, and a different relationship may be observed between patient‐centered design and domains of patient satisfaction in this setting. Further studies in different hospital settings are needed to confirm our findings. Additionally, we were limited by the low response rate of the surveys. However, this is a widespread problem with all patient satisfaction research utilizing voluntary surveys, and our response rates are consistent with those previously reported.[34, 36, 37, 38] Furthermore, low response rates have not impeded the implementation of pay‐for‐performance programs on a national scale using HCHAPS.
In conclusion, our study suggests that hospitals should not use outdated facilities as an excuse for achievement of suboptimal satisfaction scores. Patients respond positively to creature comforts, pleasing surroundings, and visitor‐friendly facilities but can distinguish these positive experiences from experiences in other patient satisfaction domains. In our study, the move to a higher‐amenity building had only a modest impact on overall patient satisfaction, perhaps because clinical care is the primary driver of this outcome. Contrary to belief held by some hospital leaders, major strides in overall satisfaction across the board and other subdomains of satisfaction likely require intervention in areas other than facility renovation and expansion.
Disclosures
Zishan Siddiqui, MD, was supported by the Osler Center of Clinical Excellence Faculty Scholarship Grant. Funds from Johns Hopkins Hospitalist Scholars Program supported the research project. The authors have no conflict of interests to disclose.
- Create a blueprint for successful hospital construction. Nurs Manage. 2006;37(6):39–44. , .
- Walter Reed National Military Medical Center website. Facts at a glance. Available at: http://www.wrnmmc.capmed.mil/About%20Us/SitePages/Facts.aspx. Accessed June 19, 2013.
- http://www.healthcaredesignmagazine.com/building‐ideas/keys‐collaboration. Accessed June 19, 2013. . Keys to collaboration. Healthcare Design website. Available at:
- http://www.healthcaredesignmagazine.com/building‐ideas/tale‐4‐hospitals. Accessed June 19, 2013. . A tale of 4 hospitals. Healthcare Design website. Available at:
- http://www.healthcaredesignmagazine.com/building‐ideas/gateway‐east. Accessed June 19, 2013. . Gateway to the east. Healthcare Design website. Available at:
- http://www.healthcaredesignmagazine.com/building‐ideas/lessons‐learned. Accessed June 19, 2013. . Lessons learned. Healthcare Design website. Available at:
- Single room maternity care and client satisfaction. Birth. 2000;27(4):235–243. , , , , .
- Same‐handed and mirrored unit configurations: is there a difference in patient and nurse outcomes? J Nurs Adm. 2011;41(6):273–279. , , , .
- The Pebble Projects: coordinated evidence‐based case studies. Build Res Inform. 2008;36(2):129–145. , .
- Effects of exposure to nature and abstract pictures on patients recovering from open heart surgery. J Soc Psychophysiol Res. 1993;30:7. , , .
- Postoperative delirium. Curr Drug Targets. 2005;6(7):807–814. , , , .
- Stimulus deprivation in windowless rooms. Anaesthesia. 1977;32(7):598–602. .
- Post‐occupancy evaluation of healing gardens in a pediatric cancer center. Landsc Urban Plan. 2005;73(2):167–183. , , , .
- Healing gardens in hospitals. Interdiscip Des Res J. 2007;1(1):1–27. .
- Restorative gardens. BMJ. 1993;306(6885):1080–1081. , .
- Effects of interior design on wellness: theory and recent scientific research. J Health Care Inter Des. 1991;3:97–109. .
- Sunny hospital rooms expedite recovery from severe and refractory depressions. J Affect Disord. 1996;40(1‐2):49–51. , .
- Art in hospital spaces: the role of hospitals in an aestheticised society. Int J Cult Policy. 2007;13(1):85–101. .
- Renovation of a semiprivate patient room. Bowman Center Geriatric Rehabilitation Unit. Nurs Clin North Am 1995;30(1):97–115. , , .
- (2013). Effect of intensive care environment on family and patient satisfaction: a before‐after study. Intensive Care Med. 2013;39(9):1626–1634. , , , et al.
- Outcomes of environmental appraisal of different hospital waiting areas. Environ Behav. 2003;35(6):842–869. , , , , .
- Redesigning the neurocritical care unit to enhance family participation and improve outcomes. Cleve Clin J Med. 2009;76(suppl 2):S70–S74. .
- The ecology of the patient visit: physical attractiveness, waiting times, and perceived quality of care. J Ambul Care Manage. 2008;31(2):128–141. , .
- Patient satisfaction and the new consumer. Hosp Health Netw. 2006;80(57):59–62. .
- Patient satisfaction. Hospitals embrace hotel‐like amenities. Hosp Health Netw. 2007;81(11):24–26. .
- Do appealing hospital rooms increase patient evaluations of physicians, nurses, and hospital services? Health Care Manage Rev. 2003;28(3):254–264. , , .
- http://www.healthleadersmedia.com/intelligence/detail.cfm?content_id=28289334(2):125–133. . Patient experience and HCAHPS: little consensus on a top priority. Health Leaders Media website. Available at
- Centers for Medicare 67:27–37.
- Hospital Consumer Assessment of Healthcare Providers and Systems. Summary analysis. http://www.hcahpsonline.org/SummaryAnalyses.aspx. Accessed October 1, 2014.
- Centers for Medicare 44(2 pt 1):501–518.
- J.D. Power and Associates. Patient satisfaction influenced more by hospital staff than by the hospital facilities. Available at: http://www.jdpower.com/press‐releases/2012‐national‐patient‐experience‐study#sthash.gSv6wAdc.dpuf. Accessed December 10, 2013.
- Racial and ethnic differences in a patient survey: patients' values, ratings, and reports regarding physician primary care performance in a large health maintenance organization. Med Care. 2000;38(3): 300–310. , , , , .
- Patient experience in safety‐net hospitals implications for improving care and Value‐Based Purchasing patient experience in safety‐net hospitals. Arch Intern Med. 2012;172(16):1204–1210. , , , .
- Comparison of Hospital Consumer Assessment of Healthcare Providers and Systems patient satisfaction scores for specialty hospitals and general medical hospitals: confounding effect of survey response rate. J Hosp Med. 2014;9(9):590–593. , , , .
- Create a blueprint for successful hospital construction. Nurs Manage. 2006;37(6):39–44. , .
- Walter Reed National Military Medical Center website. Facts at a glance. Available at: http://www.wrnmmc.capmed.mil/About%20Us/SitePages/Facts.aspx. Accessed June 19, 2013.
- http://www.healthcaredesignmagazine.com/building‐ideas/keys‐collaboration. Accessed June 19, 2013. . Keys to collaboration. Healthcare Design website. Available at:
- http://www.healthcaredesignmagazine.com/building‐ideas/tale‐4‐hospitals. Accessed June 19, 2013. . A tale of 4 hospitals. Healthcare Design website. Available at:
- http://www.healthcaredesignmagazine.com/building‐ideas/gateway‐east. Accessed June 19, 2013. . Gateway to the east. Healthcare Design website. Available at:
- http://www.healthcaredesignmagazine.com/building‐ideas/lessons‐learned. Accessed June 19, 2013. . Lessons learned. Healthcare Design website. Available at:
- Single room maternity care and client satisfaction. Birth. 2000;27(4):235–243. , , , , .
- Same‐handed and mirrored unit configurations: is there a difference in patient and nurse outcomes? J Nurs Adm. 2011;41(6):273–279. , , , .
- The Pebble Projects: coordinated evidence‐based case studies. Build Res Inform. 2008;36(2):129–145. , .
- Effects of exposure to nature and abstract pictures on patients recovering from open heart surgery. J Soc Psychophysiol Res. 1993;30:7. , , .
- Postoperative delirium. Curr Drug Targets. 2005;6(7):807–814. , , , .
- Stimulus deprivation in windowless rooms. Anaesthesia. 1977;32(7):598–602. .
- Post‐occupancy evaluation of healing gardens in a pediatric cancer center. Landsc Urban Plan. 2005;73(2):167–183. , , , .
- Healing gardens in hospitals. Interdiscip Des Res J. 2007;1(1):1–27. .
- Restorative gardens. BMJ. 1993;306(6885):1080–1081. , .
- Effects of interior design on wellness: theory and recent scientific research. J Health Care Inter Des. 1991;3:97–109. .
- Sunny hospital rooms expedite recovery from severe and refractory depressions. J Affect Disord. 1996;40(1‐2):49–51. , .
- Art in hospital spaces: the role of hospitals in an aestheticised society. Int J Cult Policy. 2007;13(1):85–101. .
- Renovation of a semiprivate patient room. Bowman Center Geriatric Rehabilitation Unit. Nurs Clin North Am 1995;30(1):97–115. , , .
- (2013). Effect of intensive care environment on family and patient satisfaction: a before‐after study. Intensive Care Med. 2013;39(9):1626–1634. , , , et al.
- Outcomes of environmental appraisal of different hospital waiting areas. Environ Behav. 2003;35(6):842–869. , , , , .
- Redesigning the neurocritical care unit to enhance family participation and improve outcomes. Cleve Clin J Med. 2009;76(suppl 2):S70–S74. .
- The ecology of the patient visit: physical attractiveness, waiting times, and perceived quality of care. J Ambul Care Manage. 2008;31(2):128–141. , .
- Patient satisfaction and the new consumer. Hosp Health Netw. 2006;80(57):59–62. .
- Patient satisfaction. Hospitals embrace hotel‐like amenities. Hosp Health Netw. 2007;81(11):24–26. .
- Do appealing hospital rooms increase patient evaluations of physicians, nurses, and hospital services? Health Care Manage Rev. 2003;28(3):254–264. , , .
- http://www.healthleadersmedia.com/intelligence/detail.cfm?content_id=28289334(2):125–133. . Patient experience and HCAHPS: little consensus on a top priority. Health Leaders Media website. Available at
- Centers for Medicare 67:27–37.
- Hospital Consumer Assessment of Healthcare Providers and Systems. Summary analysis. http://www.hcahpsonline.org/SummaryAnalyses.aspx. Accessed October 1, 2014.
- Centers for Medicare 44(2 pt 1):501–518.
- J.D. Power and Associates. Patient satisfaction influenced more by hospital staff than by the hospital facilities. Available at: http://www.jdpower.com/press‐releases/2012‐national‐patient‐experience‐study#sthash.gSv6wAdc.dpuf. Accessed December 10, 2013.
- Racial and ethnic differences in a patient survey: patients' values, ratings, and reports regarding physician primary care performance in a large health maintenance organization. Med Care. 2000;38(3): 300–310. , , , , .
- Patient experience in safety‐net hospitals implications for improving care and Value‐Based Purchasing patient experience in safety‐net hospitals. Arch Intern Med. 2012;172(16):1204–1210. , , , .
- Comparison of Hospital Consumer Assessment of Healthcare Providers and Systems patient satisfaction scores for specialty hospitals and general medical hospitals: confounding effect of survey response rate. J Hosp Med. 2014;9(9):590–593. , , , .
© 2014 Society of Hospital Medicine
Dashboards and P4P in VTE Prophylaxis
The Affordable Care Act explicitly outlines improving the value of healthcare by increasing quality and decreasing costs. It emphasizes value‐based purchasing, the transparency of performance metrics, and the use of payment incentives to reward quality.[1, 2] Venous thromboembolism (VTE) prophylaxis is one of these publicly reported performance measures. The National Quality Forum recommends that each patient be evaluated on hospital admission and during their hospitalization for VTE risk level and for appropriate thromboprophylaxis to be used, if required.[3] Similarly, the Joint Commission includes appropriate VTE prophylaxis in its Core Measures.[4] Patient experience and performance metrics, including VTE prophylaxis, constitute the hospital value‐based purchasing (VBP) component of healthcare reform.[5] For a hypothetical 327‐bed hospital, an estimated $1.7 million of a hospital's inpatient payments from Medicare will be at risk from VBP alone.[2]
VTE prophylaxis is a common target of quality improvement projects. Effective, safe, and cost‐effective measures to prevent VTE exist, including pharmacologic and mechanical prophylaxis.[6, 7] Despite these measures, compliance rates are often below 50%.[8] Different interventions have been pursued to ensure appropriate VTE prophylaxis, including computerized provider order entry (CPOE), electronic alerts, mandatory VTE risk assessment and prophylaxis, and provider education campaigns.[9] Recent studies show that CPOE systems with mandatory fields can increase VTE prophylaxis rates to above 80%, yet the goal of a high reliability health system is for 100% of patients to receive recommended therapy.[10, 11, 12, 13, 14, 15] Interventions to improve prophylaxis rates that have included multiple strategies, such as computerized order sets, feedback, and education, have been the most effective, increasing compliance to above 90%.[9, 11, 16] These systems can be enhanced with additional interventions such as providing individualized provider education and feedback, understanding of work flow, and ensuring patients receive the prescribed therapies.[12] For example, a physician dashboard could be employed to provide a snapshot and historical trend of key performance indicators using graphical displays and indicators.[17]
Dashboards and pay‐for‐performance programs have been increasingly used to increase the visibility of these metrics, provide feedback, visually display benchmarks and goals, and proactively monitor for achievements and setbacks.[18] Although these strategies are often addressed at departmental (or greater) levels, applying them at the level of the individual provider may assist hospitals in reducing preventable harm and achieving safety and quality goals, especially at higher benchmarks. With their expanding role, hospitalists provide a key opportunity to lead improvement efforts and to study the impact of dashboards and pay‐for performance at the provider level to achieve VTE prophylaxis performance targets. Hospitalists are often the front‐line provider for inpatients and deliver up to 70% of inpatient general medical services.[19] The objective of our study was to evaluate the impact of providing individual provider feedback and employing a pay‐for‐performance program on baseline performance of VTE prophylaxis among hospitalists. We hypothesized that performance feedback through the use of a dashboard would increase appropriate VTE prophylaxis, and this effect would be further augmented by incorporation of a pay‐for‐performance program.
METHODS
Hospitalist Dashboard
In 2010, hospitalist program leaders met with hospital administrators to create a hospitalist dashboard that would provide regularly updated summaries of performance measures for individual hospitalists. The final set of metrics identified included appropriate VTE prophylaxis, length of stay, patients discharged per day, discharges before 3 pm, depth of coding, patient satisfaction, readmissions, communication with the primary care provider, and time to signature for discharge summaries (Figure 1A). The dashboard was introduced at a general hospitalist meeting during which its purpose, methodology, and accessibility were described; it was subsequently implemented in January 2011.
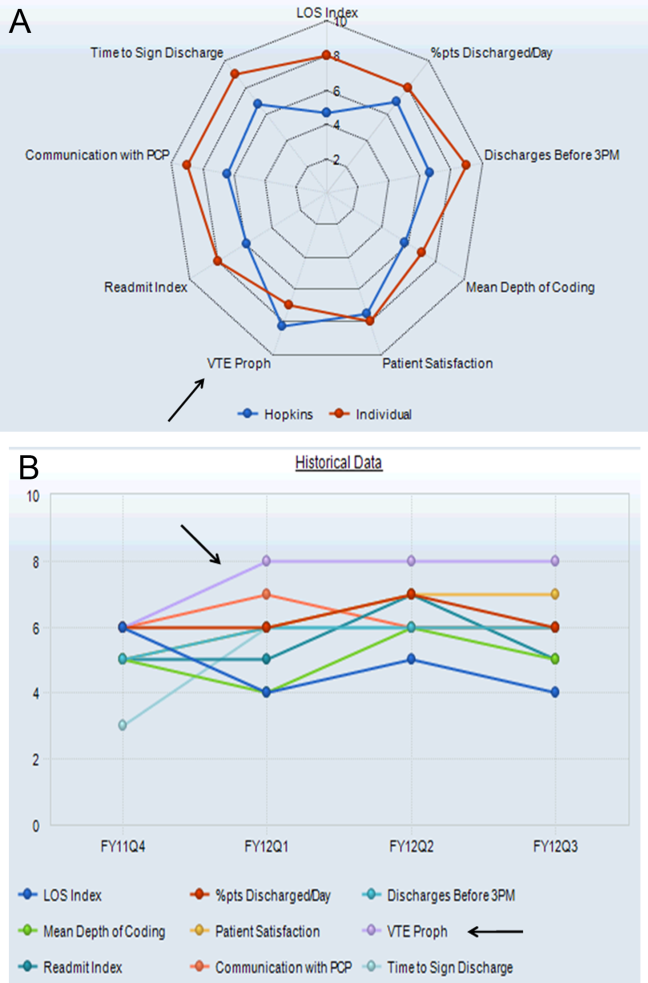
Benchmarks were established for each metric, standardized to establish a scale ranging from 1 through 9, and incorporated into the dashboard (Figure 1A). Higher scores (creating a larger geometric shape) were desirable. For the VTE prophylaxis measure, scores of 1 through 9 corresponded to <60%, 60% to 64.9%, 65% to 69.9%, 70% to 74.9%, 75% to 79.9%, 80% to 84.9%, 85% to 89.9%, 90% to 94.9%, and 95% American College of Chest Physicians (ACCP)‐compliant VTE prophylaxis, respectively.[12, 20] Each provider was able to access the aggregated dashboard (showing the group mean) and his/her individualized dashboard using an individualized login and password for the institutional portal. This portal is used during the provider's workflow, including medical record review and order entry. Both a polygonal summary graphic (Figure 1A) and trend (Figure 1B) view of the dashboard were available to the provider. A comparison of the individual provider to the hospitalist group average was displayed (Figure 1A). At monthly program meetings, the dashboard, group results, and trends were discussed.
Venous Thromboembolism Prophylaxis Compliance
Our study was performed in a tertiary academic medical center with an approximately 20‐member hospitalist group (the precise membership varied over time), whose responsibilities include, among other clinical duties, staffing a 17‐bed general medicine unit with telemetry. The scope of diagnoses and acuity of patients admitted to the hospitalist service is similar to the housestaff services. Some hospitalist faculty serve both as hospitalist and nonhospitalist general medicine service team attendings, but the comparison groups were staffed by hospitalists for <20% of the time. For admissions, all hospitalists use a standardized general medicine admission order set that is integrated into the CPOE system (Sunrise Clinical Manager; Allscripts, Chicago, IL) and completed for all admitted patients. A mandatory VTE risk screen, which includes an assessment of VTE risk factors and pharmacological prophylaxis contraindications, must be completed by the ordering physician as part of this order set (Figure 2A). The system then prompts the provider with a risk‐appropriate VTE prophylaxis recommendation that the provider may subsequently order, including mechanical prophylaxis (Figure 2B). Based on ACCP VTE prevention guidelines, risk‐appropriate prophylaxis was determined using an electronic algorithm that categorized patients into risk categories based on the presence of major VTE risk factors (Figure 2A).[12, 15, 20] If none of these were present, the provider selected No major risk factors known. Both an assessment of current use of anticoagulation and a clinically high risk of bleeding were also included (Figure 2A). If none of these were present, the provider selected No contraindications known. This algorithm is published in detail elsewhere and has been shown to not increase major bleeding episodes.[12, 15] The VTE risk assessment, but not the VTE order itself, was a mandatory field. This allowed the physician discretion to choose among various pharmacological agents and mechanical mechanisms based on patient and physician preferences.
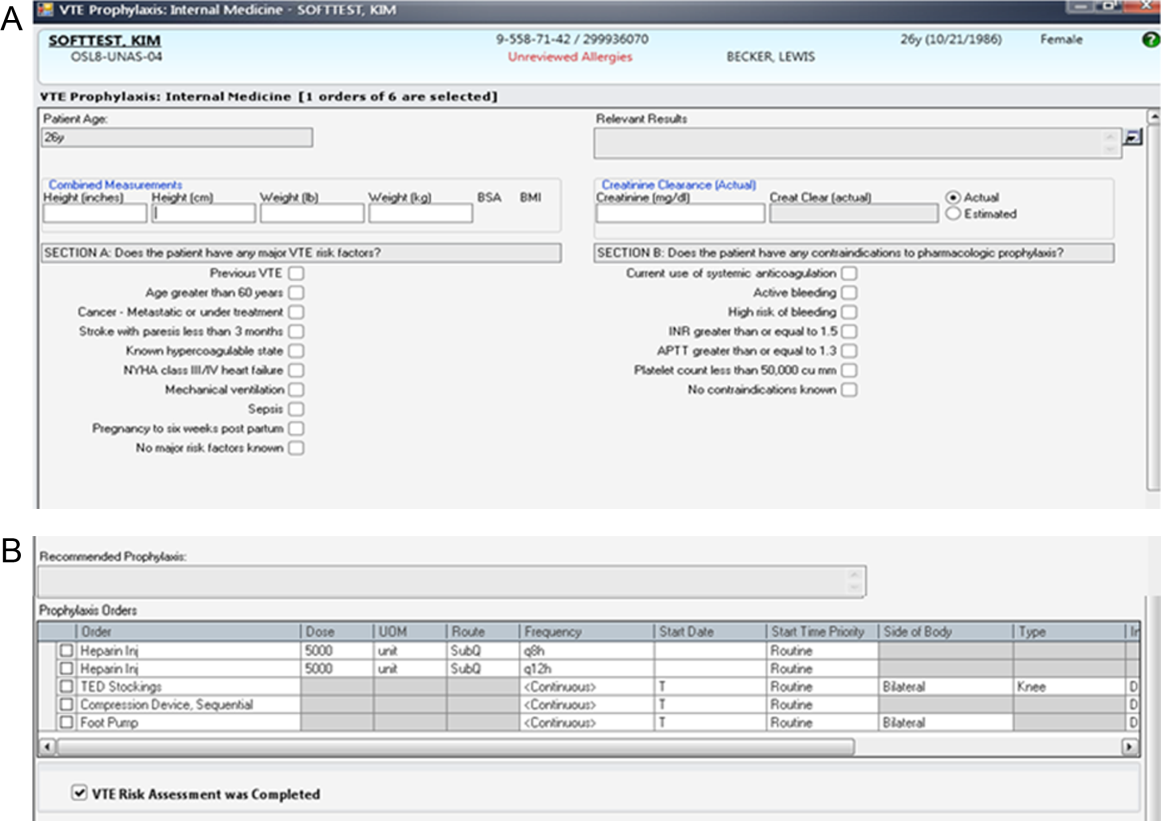
Compliance of risk‐appropriate VTE prophylaxis was determined 24 hours after the admission order set was completed using an automated electronic query of the CPOE system. Low molecular‐weight heparin prescription was included in the compliance algorithm as acceptable prophylaxis. Prescription of pharmacological VTE prophylaxis when a contraindication was present was considered noncompliant. The metric was assigned to the attending physician who billed for the first inpatient encounter.
Pay‐for‐Performance Program
In July 2011, a pay‐for‐performance program was added to the dashboard. All full‐time and part‐time hospitalists were eligible. The financial incentive was determined according to hospital priority and funds available. The VTE prophylaxis metric was prorated by clinical effort, with a maximum of $0.50 per work relative value unit (RVU). To optimize performance, a threshold of 80% compliance had to be surpassed before any payment was made. Progressively increasing percentages of the incentive were earned as compliance increased from 80% to 100%, corresponding to dashboard scores of 6, 7, 8, and 9: <80% (scores 1 to 5)=no payment; 80% to 84.9% (score 6)=$0.125 per RVU; 85% to 89.9% (score 7)=$0.25 per RVU; 90% to 94.9% (score 8)=$0.375 per RVU; and 95% (score 9)=$0.50 per RVU (maximum incentive). Payments were accrued quarterly and paid at the end of the fiscal year as a cumulative, separate performance supplement.
Individualized physician feedback through the dashboard was continued during the pay‐for‐performance period. Average hospitalist group compliance continued to be displayed on the electronic dashboard and was explicitly reviewed at monthly hospitalist meetings.
The VTE prophylaxis order set and data collection and analyses were approved by the Johns Hopkins Medicine Institutional Review Board. The dashboard and pay‐for‐performance program were initiated by the institution as part of a proof of concept quality improvement project.
Analysis
We examined all inpatient admissions to the hospitalist unit from 2008 to 2012. We included patients admitted to and discharged from the hospitalist unit and excluded patients transferred into/out of the unit and encounters with a length of stay <24 hours. VTE prophylaxis orders were queried from the CPOE system 24 hours after the patient was admitted to determine compliance.
After allowing for a run‐in period (2008), we analyzed the change in percent compliance for 3 periods: (1) CPOE‐based VTE order set alone (baseline [BASE], January 2009 to December 2010); (2) group and individual physician feedback using the dashboard (dashboard only [DASH], January to June 2011); and (3) dashboard tied to the pay‐for‐performance program (dashboard with pay‐for‐performance [P4P], July 2011 to December 2012). The CPOE‐based VTE order set was used during all 3 periods. We used the other medical services as a control to ensure that there were no temporal trends toward improved prophylaxis on a service without the intervention. VTE prophylaxis compliance was examined by calculating percent compliance using the same algorithm for the 4 resident‐staffed general medicine service teams at our institution, which utilized the same CPOE system but did not receive the dashboard or pay‐for‐performance interventions. We used locally weighted scatterplot smoothing, a locally weighted regression of percent compliance over time, to graphically display changes in group compliance over time.[21, 22]
We also performed linear regression to assess the rate of change in group compliance and included spline terms that allowed slope to vary for each of the 3 time periods.[23, 24] Clustered analysis accounted for potentially correlated serial measurements of compliance for an individual provider. A separate analysis examined the effect of provider turnover and individual provider improvement during each of the 3 periods. Tests of significance were 2‐sided, with an level of 0.05. Statistical analysis was performed using Stata 12.1 (StataCorp LP, College Station, TX).
RESULTS
Venous Thromboembolism Prophylaxis Compliance
We analyzed 3144 inpatient admissions by 38 hospitalists from 2009 to 2012. The 5 most frequent coded diagnoses were heart failure, acute kidney failure, syncope, pneumonia, and chest pain. Patients had a median length of stay of 3 days [interquartile range: 26]. During the dashboard‐only period, on average, providers improved in compliance by 4% (95% confidence interval [CI]: 35; P<0.001). With the addition of the pay‐for‐performance program, providers improved by an additional 4% (95% CI: 35; P<0.001). Group compliance significantly improved from 86% (95% CI: 8588) during the BASE period of the CPOE‐based VTE order set to 90% (95% CI: 8893) during the DASH period (P=0.01) and 94% (95% CI: 9396) during the subsequent P4P program (P=0.01) (Figure 3). Both inappropriate prophylaxis and lack of prophylaxis, when indicated, resulted in a non‐compliance rating. During the 3 periods, inappropriate prophylaxis decreased from 7.9% to 6.2% to 2.6% during the BASE, DASH, and subsequent P4P periods, respectively. Similarly, lack of prophylaxis when indicated decreased from 6.1% to 3.2% to 3.1% during the BASE, DASH, and subsequent P4P periods, respectively.
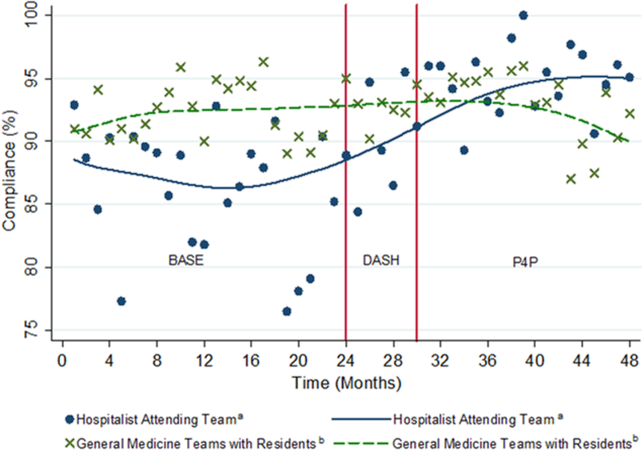
The average compliance of the 4 non‐hospitalist general medicine service teams was initially higher than that of the hospitalist service during the CPOE‐based VTE order set (90%) and DASH (92%) periods, but subsequently plateaued and was exceeded by the hospitalist service during the combined P4P (92%) period (Figure 3). However, there was no statistically significant difference between the general medicine service teams and hospitalist service during the DASH (P=0.15) and subsequent P4P (P=0.76) periods.
We also analyzed the rate of VTE prophylaxis compliance improvement (slope) with cut points at each time period transition (Figure 3). Risk‐appropriate VTE prophylaxis during the BASE period did not exhibit significant improvement as indicated by the slope (P=0.23) (Figure 3). In contrast, during the DASH period, VTE prophylaxis compliance significantly increased by 1.58% per month (95% CI: 0.41‐2.76; P=0.01). The addition of the P4P program, however, did not further significantly increase the rate of compliance (P=0.78).
A subgroup analysis restricted to the 19 providers present during all 3 periods was performed to assess for potential confounding from physician turnover. The percent compliance increased in a similar fashion: BASE period of CPOE‐based VTE order set, 85% (95% CI: 8386); DASH, 90% (95% CI: 8893); and P4P, 94% (95% CI: 9296).
Pay‐for‐Performance Program
Nineteen providers met the threshold for pay‐for‐performance (80% appropriate VTE prophylaxis), with 9 providers in the intermediate categories (80%94.9%) and 10 in the full incentive category (95%). The mean individual payout for the incentive was $633 (standard deviation 350), with a total disbursement of $12,029. The majority of payments (17 of 19) were under $1000.
DISCUSSION
A key component of healthcare reform has been value‐based purchasing, which emphasizes extrinsic motivation through the transparency of performance metrics and use of payment incentives to reward quality. Our study evaluates the impact of both extrinsic (payments) and intrinsic (professionalism and peer norms) motivation. It specifically attributed an individual performance metric, VTE prophylaxis, to an attending physician, provided both individualized and group feedback using an electronic dashboard, and incorporated a pay‐for‐performance program. Prescription of risk‐appropriate VTE prophylaxis significantly increased with the implementation of the dashboard and subsequent pay‐for performance program. The fastest rate of improvement occurred after the addition of the dashboard. Sensitivity analyses for provider turnover and comparisons to the general medicine services showed our results to be independent of a general trend of improvement, both at the provider and institutional levels.
Our prior studies demonstrated that order sets significantly improve performance, from a baseline compliance of risk‐appropriate VTE prophylaxis of 66% to 84%.[13, 15, 25] In the current study, compliance was relatively flat during the BASE period, which included these order sets. The greatest rate of continued improvement in compliance occurred during the DASH period, emphasizing both the importance of provider feedback and receptivity and adaptability in the prescribing behavior of hospitalists. Because the goal of a high‐reliability health system is for 100% of patients to receive recommended therapy, multiple approaches are necessary for success.
Nationally, benchmarks for performance measures continue to be raised, with the highest performers achieving above 95%.[26] Additional interventions, such as dashboards and pay‐for‐performance programs, supplement CPOE systems to achieve high reliability. In our study, the compliance rate during the baseline period, which included a CPOE‐based, clinical support‐enabled VTE order set, was 86%. Initially the compliance of the general medicine teams with residents exceeded that of the hospitalist attending teams, which may reflect a greater willingness of resident teams to comply with order sets and automated recommendations. This emphasizes the importance of continuous individual feedback and provider education at the attending physician level to enhance both guideline compliance and decrease provider care variation. Ultimately, with the addition of the dashboard and subsequent pay‐for‐performance program, compliance was increased to 90% and 94%, respectively. Although the major mechanism used by policymakers to improve quality of care is extrinsic motivation, this study demonstrates that intrinsic motivation through peer norms can enhance extrinsic efforts and may be more influential. Both of these programs, dashboards and pay‐for‐performance, may ultimately assist institutions in changing provider behavior and achieving these harder‐to‐achieve higher benchmarks.
We recognize that there are several limitations to our study. First, this is a single‐site program limited to an attending‐physician‐only service. There was strong data support and a defined CPOE algorithm for this initiative. Multi‐site studies will need to overcome the additional challenges of varying service structures and electronic medical record and provider order entry systems. Second, it is difficult to show actual changes in VTE events over time with appropriate prophylaxis. Although VTE prophylaxis is recommended for patients with VTE risk factors, there are conflicting findings about whether prophylaxis prevents VTE events in lower‐risk patients, and current studies suggest that most patients with VTE events are severely ill and develop VTE despite receiving prophylaxis.[27, 28, 29] Our study was underpowered to detect these potential differences in VTE rates, and although the algorithm has been shown to not increase bleeding rates, we did not measure bleeding rates during this study.[12, 15] Our institutional experience suggests that the majority of VTE events occur despite appropriate prophylaxis.[30] Also, VTE prophylaxis may be ordered, but intervening events, such as procedures and changes in risk status or patient refusal, may prevent patients from receiving appropriate prophylaxis.[31, 32] Similarly, hospitals with higher quality scores have higher VTE prophylaxis rates but worse risk‐adjusted VTE rates, which may result from increased surveillance for VTE, suggesting surveillance bias limits the usefulness of the VTE quality measure.[33, 34] Nevertheless, VTE prophylaxis remains a publicly reported Core Measure tied to financial incentives.[4, 5] Third, there may be an unmeasured factor specific to the hospitalist program, which could potentially account for an overall improvement in quality of care. Although the rate of increase in appropriate prophylaxis was not statistically significant during the baseline period, there did appear to be some improvement in prophylaxis toward the end of the period. However, there were no other VTE‐related provider feedback programs being simultaneously pursued during this study. VTE prophylaxis for the non‐hospitalist services showed a relatively stable, non‐increasing compliance rate for the general medical services. Although it was possible for successful residents to age into the hospitalist service, thereby improving rates of prophylaxis based on changes in group makeup, our subgroup analysis of the providers present throughout all phases of the study showed our results to be robust. Similarly, there may have been a cross‐contamination effect of hospitalist faculty who attended on both hospitalist and non‐hospitalist general medicine service teams. This, however, would attenuate any impact of the programs, and thus the effects may in fact be greater than reported. Fourth, establishment of both the dashboard and pay‐for‐performance program required significant institutional and program leadership and resources. To be successful, the dashboard must be in the provider's workflow, transparent, minimize reporter burden, use existing systems, and be actively fed back to providers, ideally those directly entering orders. Our greatest rate of improvement occurred during the feedback‐only phase of this study, emphasizing the importance of physician feedback, provider‐level accountability, and engagement. We suspect that the relatively modest pay‐for‐performance incentive served mainly as a means of engaging providers in self‐monitoring, rather than as a means to change behavior through true incentivization. Although we did not track individual physician views of the dashboard, we reinforced trends, deviations, and expectations at regularly scheduled meetings and provided feedback and patient‐level data to individual providers. Fifth, the design of the pay‐for‐performance program may have also influenced its effectiveness. These types of programs may be more effective when they provide frequent visible, small payments rather than one large payment, and when the payment is framed as a loss rather than a gain.[35] Finally, physician champions and consistent feedback through departmental meetings or visual displays may be required for program success. The initial resources to create the dashboard, continued maintenance and monitoring of performance, and payment of financial incentives all require institutional commitment. A partnership of physicians, program leaders, and institutional administrators is necessary for both initial and continued success.
To achieve performance goals and benchmarks, multiple strategies that combine extrinsic and intrinsic motivation are necessary. As shown by our study, the use of a dashboard and pay‐for‐performance can be tailored to an institution's goals, in line with national standards. The specific goal (risk‐appropriate VTE prophylaxis) and benchmarks (80%, 85%, 90%, 95%) can be individualized to a particular institution. For example, if readmission rates are above target, readmissions could be added as a dashboard metric. The specific benchmark would be determined by historical trends and administrative targets. Similarly, the overall financial incentives could be adjusted based on the financial resources available. Other process measures, such as influenza vaccination screening and administration, could also be targeted. For all of these objectives, continued provider feedback and engagement are critical for progressive success, especially to decrease variability in care at the attending physician level. Incorporating the value‐based purchasing philosophy from the Affordable Care Act, our study suggests that the combination of standardized order sets, real‐time dashboards, and physician‐level incentives may assist hospitals in achieving quality and safety benchmarks, especially at higher targets.
Acknowledgements
The authors thank Meir Gottlieb, BS, from Salar Inc. for data support; Murali Padmanaban, BS, from Johns Hopkins University for his assistance in linking the administrative billing data with real‐time physician orders; and Hsin‐Chieh Yeh, PhD, from the Bloomberg School of Public Health for her statistical advice and additional review. We also thank Mr. Ronald R. Peterson, President, Johns Hopkins Health System and Johns Hopkins Hospital, for providing funding support for the physician incentive payments.
Disclosures: Drs. Michtalik and Brotman had full access to all of the data in the study and take responsibility for the integrity of the data and accuracy of the data analysis. Study concept and design: Drs. Michtalik, Streiff, Finkelstein, Pronovost, and Brotman. Acquisition of data: Drs. Michtalik, Streiff, Brotman and Mr. Carolan, Mr. Lau, Mrs. Durkin. Analysis and interpretation of data: Drs. Michtalik, Haut, Streiff, Brotman and Mr. Carolan, Mr. Lau. Drafting of the manuscript: Drs. Michtalik and Brotman. Critical revision of the manuscript for important intellectual content: Drs. Michtalik, Haut, Streiff, Finkelstein, Pronovost, Brotman and Mr. Carolan, Mr. Lau, Mrs. Durkin. Statistical analysis and supervision: Drs. Michtalik and Brotman. Obtaining funding: Drs. Streiff and Brotman. Technical support: Dr. Streiff and Mr. Carolan, Mr. Lau, Mrs. Durkin
This study was supported by a National Institutes of Health grant T32 HP10025‐17‐00 (Dr. Michtalik), the National Institutes of Health/Johns Hopkins Institute for Clinical and Translational Research KL2 Award 5KL2RR025006 (Dr. Michtalik), the Agency for Healthcare Research and Quality Mentored Clinical Scientist Development K08 Awards 1K08HS017952‐01 (Dr. Haut) and 1K08HS022331‐01A1 (Dr. Michtalik), and the Johns Hopkins Hospitalist Scholars Fund. The funders had no role in the design and conduct of the study; collection, management, analysis, and interpretation of the data; preparation, review, or approval of the manuscript; and decision to submit the manuscript for publication.
Dr. Haut receives royalties from Lippincott, Williams & Wilkins. Dr. Streiff has received research funding from Portola and Bristol Myers Squibb, honoraria for CME lectures from Sanofi‐Aventis and Ortho‐McNeil, consulted for Eisai, Daiichi‐Sankyo, Boerhinger‐Ingelheim, Janssen Healthcare, and Pfizer. Mr. Lau, Drs. Haut, Streiff, and Pronovost are supported by a contract from the Patient‐Centered Outcomes Research Institute (PCORI) titled Preventing Venous Thromboembolism: Empowering Patients and Enabling Patient‐Centered Care via Health Information Technology (CE‐12‐11‐4489). Dr. Brotman has received research support from Siemens Healthcare Diagnostics, Bristol‐Myers Squibb, the Agency for Healthcare Research and Quality, Centers for Medicare & Medicaid Services, the Amerigroup Corporation, and the Guerrieri Family Foundation. He has received honoraria from the Gerson Lehrman Group, the Dunn Group, and from Quantia Communications, and received royalties from McGraw‐Hill.
- Medicare Program, Centers for Medicare 76(88):26490–26547.
- Quality meets finance: payments at risk with value‐based purchasing, readmission, and hospital‐acquired conditions force hospitalists to focus. Hospitalist. 2013;17(1):31. .
- National Quality Forum. March 2009. Safe practices for better healthcare—2009 update. Available at: http://www.qualityforum.org/Publications/2009/03/Safe_Practices_for_Better_Healthcare%E2%80%932009_Update.aspx. Accessed November 1, 2014.
- Joint Commission on Accreditation of Healthcare Organizations. Approved: more options for hospital core measures. Jt Comm Perspect. 2009;29(4):1–6.
- Centers for Medicare 208(2):227–240.
- Thromboprophylaxis in nonsurgical patients. Hematology Am Soc Hematol Educ Program. 2012;2012:631–637. , .
- Venous thromboembolism risk and prophylaxis in the acute hospital care setting (ENDORSE study): a multinational cross‐sectional study. Lancet. 2008;371(9610):387–394. , , , et al.
- Practices to prevent venous thromboembolism: a brief review. BMJ Qual Saf. 2014;23(3):187–195. , .
- Improving hospital venous thromboembolism prophylaxis with electronic decision support. J Hosp Med. 2013;8(3):115–120. , , , et al.
- Innovative approaches to increase deep vein thrombosis prophylaxis rate resulting in a decrease in hospital‐acquired deep vein thrombosis at a tertiary‐care teaching hospital. J Hosp Med. 2008;3(2):148–155. , , .
- Lessons from the Johns Hopkins Multi‐Disciplinary Venous Thromboembolism (VTE) Prevention Collaborative. BMJ. 2012;344:e3935. , , , et al.
- Improved prophylaxis and decreased rates of preventable harm with the use of a mandatory computerized clinical decision support tool for prophylaxis for venous thromboembolism in trauma. Arch Surg. 2012;147(10):901–907. , , , et al.
- Designing and implementing effective venous thromboembolism prevention protocols: lessons from collaborative efforts. J Thromb Thrombolysis. 2010;29(2):159–166. , .
- Impact of a venous thromboembolism prophylaxis "smart order set": improved compliance, fewer events. Am J Hematol. 2013;88(7):545–549. , , , et al.
- Improving adherence to venous thromoembolism prophylaxis using multiple interventions. BMJ. 2012;344:e3935. , .
- Health Resources and Services Administration of the U.S. Department of Health and Human Services. Managing data for performance improvement. Available at: http://www.hrsa.gov/quality/toolbox/methodology/performanceimprovement/part2.html. Accessed December 18, 2014.
- Improving patient safety by taking systems seriously. JAMA. 2008;299(4):445–447. , .
- Growth in the care of older patients by hospitalists in the United States. N Engl J Med. 2009;360(11):1102–1112. , , , .
- Prevention of venous thromboembolism: American College of Chest Physicians evidence‐based clinical practice guidelines (8th edition). Chest. 2008;133(6 suppl):381S–453S. , , , et al.
- Robust locally weighted regression and smoothing scatterplots. J Am Stat Assoc. 1979;74(368):829–836. .
- Locally weighted regression: An approach to regression analysis by local fitting. J Am Stat Assoc. 1988;83(403):596–610. , .
- Regression Methods in Biostatistics: Linear, Logistic, Survival, and Repeated Measures Models. 2nd ed. New York, NY: Springer; 2012. , , , .
- Regression Modeling Strategies: With Applications to Linear Models, Logistic Regression, and Survival Analysis. New York, NY: Springer‐Verlag; 2001. .
- Med Care. doi: 10.1097/MLR.0000000000000251. , , , et al. Eliminating healthcare disparities via mandatory clinical decision support: the venous thromboembolism (VTE) example [published online ahead of print November 4, 2014].
- Joint Commission. Improving America's hospitals: the Joint Commission's annual report on quality and safety. 2012. Available at: http://www.jointcommission.org/assets/1/18/TJC_Annual_Report_2012.pdf. Accessed September 8, 2013.
- Hospital performance for pharmacologic venous thromboembolism prophylaxis and rate of venous thromboembolism: a cohort study. JAMA Intern Med. 2014;174(10):1577–1584. , , , et al.
- Incidence of hospital‐acquired venous thromboembolic codes in medical patients hospitalized in academic medical centers. J Hosp Med. 2014;9(4):221–225. , , , et al.
- No association between hospital‐reported perioperative venous thromboembolism prophylaxis and outcome rates in publicly reported data. JAMA Surg. 2014;149(4):400–401. , , , , .
- Linking processes and outcomes: a key strategy to prevent and report harm from venous thromboembolism in surgical patients. JAMA Surg. 2013;148(3):299–300. , , , , .
- Patterns of non‐administration of ordered doses of venous thromboembolism prophylaxis: implications for novel intervention strategies. PLoS One. 2013;8(6):e66311. , , , et al.
- Nonadministration of thromboprophylaxis in hospitalized patients with HIV: a missed opportunity for prevention? J Hosp Med. 2014;9(4):215–220. , , , et al.
- Evaluation of surveillance bias and the validity of the venous thromboembolism quality measure. JAMA. 2013;310(14):1482–1489. , , , et al.
- Surveillance bias in outcomes reporting. JAMA. 2011;305(23):2462–2463. , .
- Pay for performance in health care: an international overview of initiatives. Med Care Res Rev. 2012;69(3):251–276. .
The Affordable Care Act explicitly outlines improving the value of healthcare by increasing quality and decreasing costs. It emphasizes value‐based purchasing, the transparency of performance metrics, and the use of payment incentives to reward quality.[1, 2] Venous thromboembolism (VTE) prophylaxis is one of these publicly reported performance measures. The National Quality Forum recommends that each patient be evaluated on hospital admission and during their hospitalization for VTE risk level and for appropriate thromboprophylaxis to be used, if required.[3] Similarly, the Joint Commission includes appropriate VTE prophylaxis in its Core Measures.[4] Patient experience and performance metrics, including VTE prophylaxis, constitute the hospital value‐based purchasing (VBP) component of healthcare reform.[5] For a hypothetical 327‐bed hospital, an estimated $1.7 million of a hospital's inpatient payments from Medicare will be at risk from VBP alone.[2]
VTE prophylaxis is a common target of quality improvement projects. Effective, safe, and cost‐effective measures to prevent VTE exist, including pharmacologic and mechanical prophylaxis.[6, 7] Despite these measures, compliance rates are often below 50%.[8] Different interventions have been pursued to ensure appropriate VTE prophylaxis, including computerized provider order entry (CPOE), electronic alerts, mandatory VTE risk assessment and prophylaxis, and provider education campaigns.[9] Recent studies show that CPOE systems with mandatory fields can increase VTE prophylaxis rates to above 80%, yet the goal of a high reliability health system is for 100% of patients to receive recommended therapy.[10, 11, 12, 13, 14, 15] Interventions to improve prophylaxis rates that have included multiple strategies, such as computerized order sets, feedback, and education, have been the most effective, increasing compliance to above 90%.[9, 11, 16] These systems can be enhanced with additional interventions such as providing individualized provider education and feedback, understanding of work flow, and ensuring patients receive the prescribed therapies.[12] For example, a physician dashboard could be employed to provide a snapshot and historical trend of key performance indicators using graphical displays and indicators.[17]
Dashboards and pay‐for‐performance programs have been increasingly used to increase the visibility of these metrics, provide feedback, visually display benchmarks and goals, and proactively monitor for achievements and setbacks.[18] Although these strategies are often addressed at departmental (or greater) levels, applying them at the level of the individual provider may assist hospitals in reducing preventable harm and achieving safety and quality goals, especially at higher benchmarks. With their expanding role, hospitalists provide a key opportunity to lead improvement efforts and to study the impact of dashboards and pay‐for performance at the provider level to achieve VTE prophylaxis performance targets. Hospitalists are often the front‐line provider for inpatients and deliver up to 70% of inpatient general medical services.[19] The objective of our study was to evaluate the impact of providing individual provider feedback and employing a pay‐for‐performance program on baseline performance of VTE prophylaxis among hospitalists. We hypothesized that performance feedback through the use of a dashboard would increase appropriate VTE prophylaxis, and this effect would be further augmented by incorporation of a pay‐for‐performance program.
METHODS
Hospitalist Dashboard
In 2010, hospitalist program leaders met with hospital administrators to create a hospitalist dashboard that would provide regularly updated summaries of performance measures for individual hospitalists. The final set of metrics identified included appropriate VTE prophylaxis, length of stay, patients discharged per day, discharges before 3 pm, depth of coding, patient satisfaction, readmissions, communication with the primary care provider, and time to signature for discharge summaries (Figure 1A). The dashboard was introduced at a general hospitalist meeting during which its purpose, methodology, and accessibility were described; it was subsequently implemented in January 2011.
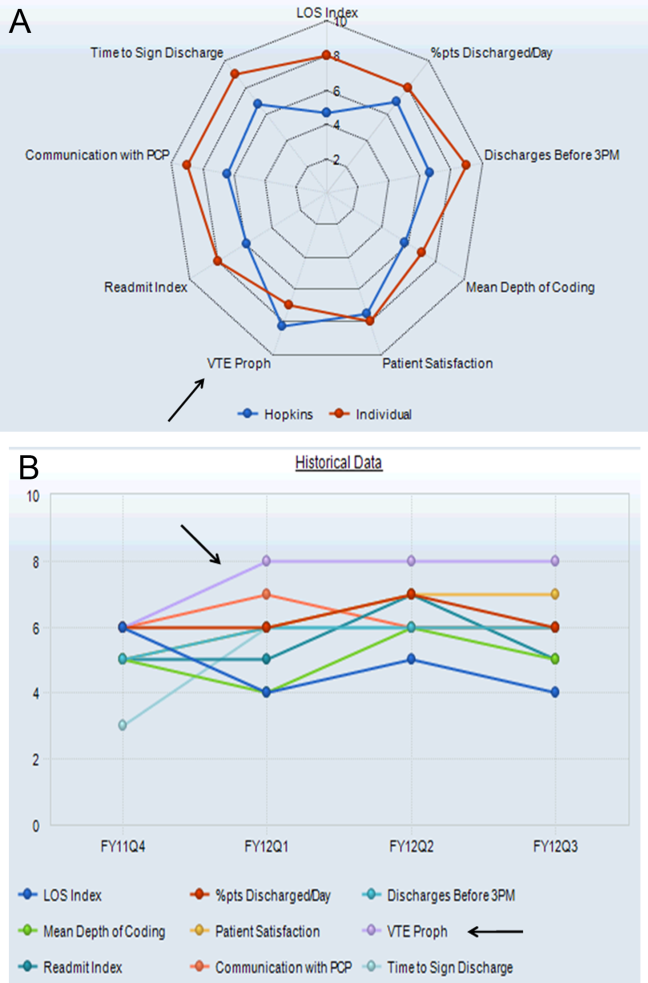
Benchmarks were established for each metric, standardized to establish a scale ranging from 1 through 9, and incorporated into the dashboard (Figure 1A). Higher scores (creating a larger geometric shape) were desirable. For the VTE prophylaxis measure, scores of 1 through 9 corresponded to <60%, 60% to 64.9%, 65% to 69.9%, 70% to 74.9%, 75% to 79.9%, 80% to 84.9%, 85% to 89.9%, 90% to 94.9%, and 95% American College of Chest Physicians (ACCP)‐compliant VTE prophylaxis, respectively.[12, 20] Each provider was able to access the aggregated dashboard (showing the group mean) and his/her individualized dashboard using an individualized login and password for the institutional portal. This portal is used during the provider's workflow, including medical record review and order entry. Both a polygonal summary graphic (Figure 1A) and trend (Figure 1B) view of the dashboard were available to the provider. A comparison of the individual provider to the hospitalist group average was displayed (Figure 1A). At monthly program meetings, the dashboard, group results, and trends were discussed.
Venous Thromboembolism Prophylaxis Compliance
Our study was performed in a tertiary academic medical center with an approximately 20‐member hospitalist group (the precise membership varied over time), whose responsibilities include, among other clinical duties, staffing a 17‐bed general medicine unit with telemetry. The scope of diagnoses and acuity of patients admitted to the hospitalist service is similar to the housestaff services. Some hospitalist faculty serve both as hospitalist and nonhospitalist general medicine service team attendings, but the comparison groups were staffed by hospitalists for <20% of the time. For admissions, all hospitalists use a standardized general medicine admission order set that is integrated into the CPOE system (Sunrise Clinical Manager; Allscripts, Chicago, IL) and completed for all admitted patients. A mandatory VTE risk screen, which includes an assessment of VTE risk factors and pharmacological prophylaxis contraindications, must be completed by the ordering physician as part of this order set (Figure 2A). The system then prompts the provider with a risk‐appropriate VTE prophylaxis recommendation that the provider may subsequently order, including mechanical prophylaxis (Figure 2B). Based on ACCP VTE prevention guidelines, risk‐appropriate prophylaxis was determined using an electronic algorithm that categorized patients into risk categories based on the presence of major VTE risk factors (Figure 2A).[12, 15, 20] If none of these were present, the provider selected No major risk factors known. Both an assessment of current use of anticoagulation and a clinically high risk of bleeding were also included (Figure 2A). If none of these were present, the provider selected No contraindications known. This algorithm is published in detail elsewhere and has been shown to not increase major bleeding episodes.[12, 15] The VTE risk assessment, but not the VTE order itself, was a mandatory field. This allowed the physician discretion to choose among various pharmacological agents and mechanical mechanisms based on patient and physician preferences.
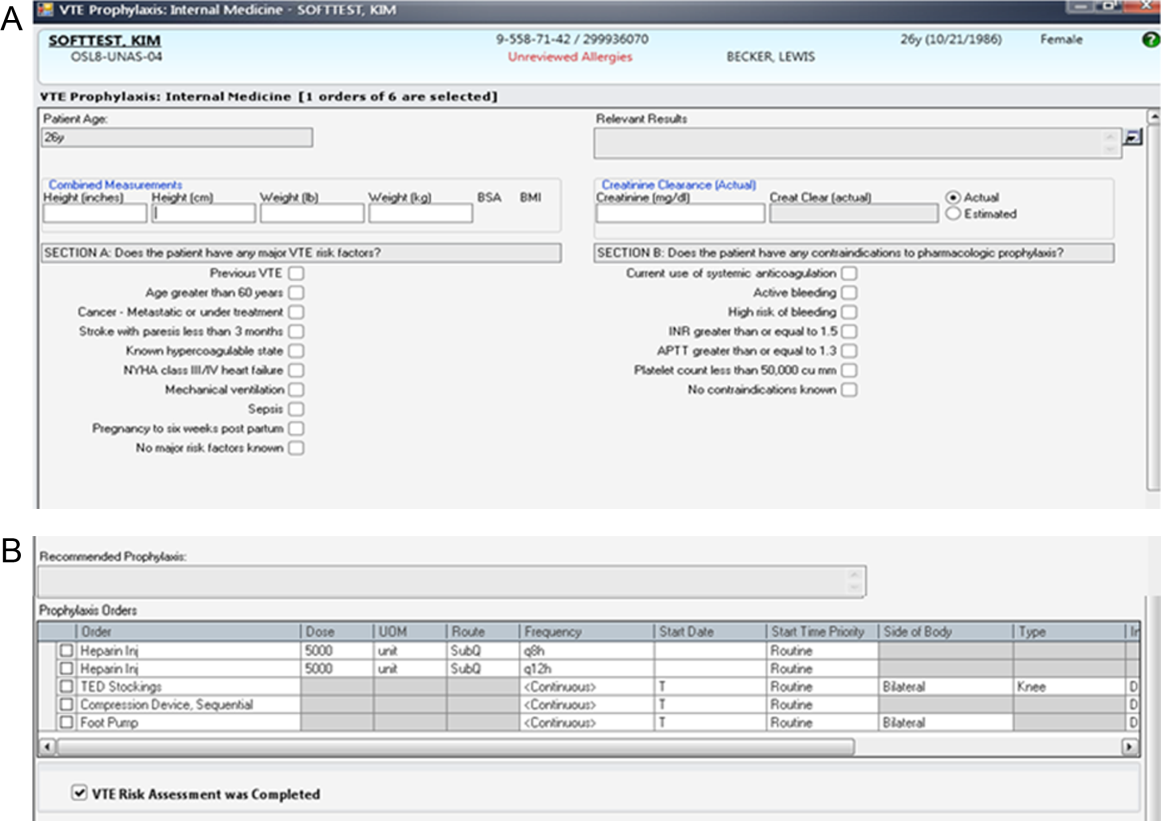
Compliance of risk‐appropriate VTE prophylaxis was determined 24 hours after the admission order set was completed using an automated electronic query of the CPOE system. Low molecular‐weight heparin prescription was included in the compliance algorithm as acceptable prophylaxis. Prescription of pharmacological VTE prophylaxis when a contraindication was present was considered noncompliant. The metric was assigned to the attending physician who billed for the first inpatient encounter.
Pay‐for‐Performance Program
In July 2011, a pay‐for‐performance program was added to the dashboard. All full‐time and part‐time hospitalists were eligible. The financial incentive was determined according to hospital priority and funds available. The VTE prophylaxis metric was prorated by clinical effort, with a maximum of $0.50 per work relative value unit (RVU). To optimize performance, a threshold of 80% compliance had to be surpassed before any payment was made. Progressively increasing percentages of the incentive were earned as compliance increased from 80% to 100%, corresponding to dashboard scores of 6, 7, 8, and 9: <80% (scores 1 to 5)=no payment; 80% to 84.9% (score 6)=$0.125 per RVU; 85% to 89.9% (score 7)=$0.25 per RVU; 90% to 94.9% (score 8)=$0.375 per RVU; and 95% (score 9)=$0.50 per RVU (maximum incentive). Payments were accrued quarterly and paid at the end of the fiscal year as a cumulative, separate performance supplement.
Individualized physician feedback through the dashboard was continued during the pay‐for‐performance period. Average hospitalist group compliance continued to be displayed on the electronic dashboard and was explicitly reviewed at monthly hospitalist meetings.
The VTE prophylaxis order set and data collection and analyses were approved by the Johns Hopkins Medicine Institutional Review Board. The dashboard and pay‐for‐performance program were initiated by the institution as part of a proof of concept quality improvement project.
Analysis
We examined all inpatient admissions to the hospitalist unit from 2008 to 2012. We included patients admitted to and discharged from the hospitalist unit and excluded patients transferred into/out of the unit and encounters with a length of stay <24 hours. VTE prophylaxis orders were queried from the CPOE system 24 hours after the patient was admitted to determine compliance.
After allowing for a run‐in period (2008), we analyzed the change in percent compliance for 3 periods: (1) CPOE‐based VTE order set alone (baseline [BASE], January 2009 to December 2010); (2) group and individual physician feedback using the dashboard (dashboard only [DASH], January to June 2011); and (3) dashboard tied to the pay‐for‐performance program (dashboard with pay‐for‐performance [P4P], July 2011 to December 2012). The CPOE‐based VTE order set was used during all 3 periods. We used the other medical services as a control to ensure that there were no temporal trends toward improved prophylaxis on a service without the intervention. VTE prophylaxis compliance was examined by calculating percent compliance using the same algorithm for the 4 resident‐staffed general medicine service teams at our institution, which utilized the same CPOE system but did not receive the dashboard or pay‐for‐performance interventions. We used locally weighted scatterplot smoothing, a locally weighted regression of percent compliance over time, to graphically display changes in group compliance over time.[21, 22]
We also performed linear regression to assess the rate of change in group compliance and included spline terms that allowed slope to vary for each of the 3 time periods.[23, 24] Clustered analysis accounted for potentially correlated serial measurements of compliance for an individual provider. A separate analysis examined the effect of provider turnover and individual provider improvement during each of the 3 periods. Tests of significance were 2‐sided, with an level of 0.05. Statistical analysis was performed using Stata 12.1 (StataCorp LP, College Station, TX).
RESULTS
Venous Thromboembolism Prophylaxis Compliance
We analyzed 3144 inpatient admissions by 38 hospitalists from 2009 to 2012. The 5 most frequent coded diagnoses were heart failure, acute kidney failure, syncope, pneumonia, and chest pain. Patients had a median length of stay of 3 days [interquartile range: 26]. During the dashboard‐only period, on average, providers improved in compliance by 4% (95% confidence interval [CI]: 35; P<0.001). With the addition of the pay‐for‐performance program, providers improved by an additional 4% (95% CI: 35; P<0.001). Group compliance significantly improved from 86% (95% CI: 8588) during the BASE period of the CPOE‐based VTE order set to 90% (95% CI: 8893) during the DASH period (P=0.01) and 94% (95% CI: 9396) during the subsequent P4P program (P=0.01) (Figure 3). Both inappropriate prophylaxis and lack of prophylaxis, when indicated, resulted in a non‐compliance rating. During the 3 periods, inappropriate prophylaxis decreased from 7.9% to 6.2% to 2.6% during the BASE, DASH, and subsequent P4P periods, respectively. Similarly, lack of prophylaxis when indicated decreased from 6.1% to 3.2% to 3.1% during the BASE, DASH, and subsequent P4P periods, respectively.
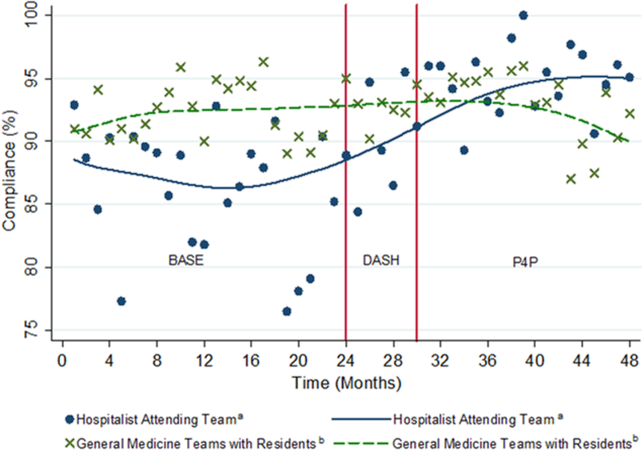
The average compliance of the 4 non‐hospitalist general medicine service teams was initially higher than that of the hospitalist service during the CPOE‐based VTE order set (90%) and DASH (92%) periods, but subsequently plateaued and was exceeded by the hospitalist service during the combined P4P (92%) period (Figure 3). However, there was no statistically significant difference between the general medicine service teams and hospitalist service during the DASH (P=0.15) and subsequent P4P (P=0.76) periods.
We also analyzed the rate of VTE prophylaxis compliance improvement (slope) with cut points at each time period transition (Figure 3). Risk‐appropriate VTE prophylaxis during the BASE period did not exhibit significant improvement as indicated by the slope (P=0.23) (Figure 3). In contrast, during the DASH period, VTE prophylaxis compliance significantly increased by 1.58% per month (95% CI: 0.41‐2.76; P=0.01). The addition of the P4P program, however, did not further significantly increase the rate of compliance (P=0.78).
A subgroup analysis restricted to the 19 providers present during all 3 periods was performed to assess for potential confounding from physician turnover. The percent compliance increased in a similar fashion: BASE period of CPOE‐based VTE order set, 85% (95% CI: 8386); DASH, 90% (95% CI: 8893); and P4P, 94% (95% CI: 9296).
Pay‐for‐Performance Program
Nineteen providers met the threshold for pay‐for‐performance (80% appropriate VTE prophylaxis), with 9 providers in the intermediate categories (80%94.9%) and 10 in the full incentive category (95%). The mean individual payout for the incentive was $633 (standard deviation 350), with a total disbursement of $12,029. The majority of payments (17 of 19) were under $1000.
DISCUSSION
A key component of healthcare reform has been value‐based purchasing, which emphasizes extrinsic motivation through the transparency of performance metrics and use of payment incentives to reward quality. Our study evaluates the impact of both extrinsic (payments) and intrinsic (professionalism and peer norms) motivation. It specifically attributed an individual performance metric, VTE prophylaxis, to an attending physician, provided both individualized and group feedback using an electronic dashboard, and incorporated a pay‐for‐performance program. Prescription of risk‐appropriate VTE prophylaxis significantly increased with the implementation of the dashboard and subsequent pay‐for performance program. The fastest rate of improvement occurred after the addition of the dashboard. Sensitivity analyses for provider turnover and comparisons to the general medicine services showed our results to be independent of a general trend of improvement, both at the provider and institutional levels.
Our prior studies demonstrated that order sets significantly improve performance, from a baseline compliance of risk‐appropriate VTE prophylaxis of 66% to 84%.[13, 15, 25] In the current study, compliance was relatively flat during the BASE period, which included these order sets. The greatest rate of continued improvement in compliance occurred during the DASH period, emphasizing both the importance of provider feedback and receptivity and adaptability in the prescribing behavior of hospitalists. Because the goal of a high‐reliability health system is for 100% of patients to receive recommended therapy, multiple approaches are necessary for success.
Nationally, benchmarks for performance measures continue to be raised, with the highest performers achieving above 95%.[26] Additional interventions, such as dashboards and pay‐for‐performance programs, supplement CPOE systems to achieve high reliability. In our study, the compliance rate during the baseline period, which included a CPOE‐based, clinical support‐enabled VTE order set, was 86%. Initially the compliance of the general medicine teams with residents exceeded that of the hospitalist attending teams, which may reflect a greater willingness of resident teams to comply with order sets and automated recommendations. This emphasizes the importance of continuous individual feedback and provider education at the attending physician level to enhance both guideline compliance and decrease provider care variation. Ultimately, with the addition of the dashboard and subsequent pay‐for‐performance program, compliance was increased to 90% and 94%, respectively. Although the major mechanism used by policymakers to improve quality of care is extrinsic motivation, this study demonstrates that intrinsic motivation through peer norms can enhance extrinsic efforts and may be more influential. Both of these programs, dashboards and pay‐for‐performance, may ultimately assist institutions in changing provider behavior and achieving these harder‐to‐achieve higher benchmarks.
We recognize that there are several limitations to our study. First, this is a single‐site program limited to an attending‐physician‐only service. There was strong data support and a defined CPOE algorithm for this initiative. Multi‐site studies will need to overcome the additional challenges of varying service structures and electronic medical record and provider order entry systems. Second, it is difficult to show actual changes in VTE events over time with appropriate prophylaxis. Although VTE prophylaxis is recommended for patients with VTE risk factors, there are conflicting findings about whether prophylaxis prevents VTE events in lower‐risk patients, and current studies suggest that most patients with VTE events are severely ill and develop VTE despite receiving prophylaxis.[27, 28, 29] Our study was underpowered to detect these potential differences in VTE rates, and although the algorithm has been shown to not increase bleeding rates, we did not measure bleeding rates during this study.[12, 15] Our institutional experience suggests that the majority of VTE events occur despite appropriate prophylaxis.[30] Also, VTE prophylaxis may be ordered, but intervening events, such as procedures and changes in risk status or patient refusal, may prevent patients from receiving appropriate prophylaxis.[31, 32] Similarly, hospitals with higher quality scores have higher VTE prophylaxis rates but worse risk‐adjusted VTE rates, which may result from increased surveillance for VTE, suggesting surveillance bias limits the usefulness of the VTE quality measure.[33, 34] Nevertheless, VTE prophylaxis remains a publicly reported Core Measure tied to financial incentives.[4, 5] Third, there may be an unmeasured factor specific to the hospitalist program, which could potentially account for an overall improvement in quality of care. Although the rate of increase in appropriate prophylaxis was not statistically significant during the baseline period, there did appear to be some improvement in prophylaxis toward the end of the period. However, there were no other VTE‐related provider feedback programs being simultaneously pursued during this study. VTE prophylaxis for the non‐hospitalist services showed a relatively stable, non‐increasing compliance rate for the general medical services. Although it was possible for successful residents to age into the hospitalist service, thereby improving rates of prophylaxis based on changes in group makeup, our subgroup analysis of the providers present throughout all phases of the study showed our results to be robust. Similarly, there may have been a cross‐contamination effect of hospitalist faculty who attended on both hospitalist and non‐hospitalist general medicine service teams. This, however, would attenuate any impact of the programs, and thus the effects may in fact be greater than reported. Fourth, establishment of both the dashboard and pay‐for‐performance program required significant institutional and program leadership and resources. To be successful, the dashboard must be in the provider's workflow, transparent, minimize reporter burden, use existing systems, and be actively fed back to providers, ideally those directly entering orders. Our greatest rate of improvement occurred during the feedback‐only phase of this study, emphasizing the importance of physician feedback, provider‐level accountability, and engagement. We suspect that the relatively modest pay‐for‐performance incentive served mainly as a means of engaging providers in self‐monitoring, rather than as a means to change behavior through true incentivization. Although we did not track individual physician views of the dashboard, we reinforced trends, deviations, and expectations at regularly scheduled meetings and provided feedback and patient‐level data to individual providers. Fifth, the design of the pay‐for‐performance program may have also influenced its effectiveness. These types of programs may be more effective when they provide frequent visible, small payments rather than one large payment, and when the payment is framed as a loss rather than a gain.[35] Finally, physician champions and consistent feedback through departmental meetings or visual displays may be required for program success. The initial resources to create the dashboard, continued maintenance and monitoring of performance, and payment of financial incentives all require institutional commitment. A partnership of physicians, program leaders, and institutional administrators is necessary for both initial and continued success.
To achieve performance goals and benchmarks, multiple strategies that combine extrinsic and intrinsic motivation are necessary. As shown by our study, the use of a dashboard and pay‐for‐performance can be tailored to an institution's goals, in line with national standards. The specific goal (risk‐appropriate VTE prophylaxis) and benchmarks (80%, 85%, 90%, 95%) can be individualized to a particular institution. For example, if readmission rates are above target, readmissions could be added as a dashboard metric. The specific benchmark would be determined by historical trends and administrative targets. Similarly, the overall financial incentives could be adjusted based on the financial resources available. Other process measures, such as influenza vaccination screening and administration, could also be targeted. For all of these objectives, continued provider feedback and engagement are critical for progressive success, especially to decrease variability in care at the attending physician level. Incorporating the value‐based purchasing philosophy from the Affordable Care Act, our study suggests that the combination of standardized order sets, real‐time dashboards, and physician‐level incentives may assist hospitals in achieving quality and safety benchmarks, especially at higher targets.
Acknowledgements
The authors thank Meir Gottlieb, BS, from Salar Inc. for data support; Murali Padmanaban, BS, from Johns Hopkins University for his assistance in linking the administrative billing data with real‐time physician orders; and Hsin‐Chieh Yeh, PhD, from the Bloomberg School of Public Health for her statistical advice and additional review. We also thank Mr. Ronald R. Peterson, President, Johns Hopkins Health System and Johns Hopkins Hospital, for providing funding support for the physician incentive payments.
Disclosures: Drs. Michtalik and Brotman had full access to all of the data in the study and take responsibility for the integrity of the data and accuracy of the data analysis. Study concept and design: Drs. Michtalik, Streiff, Finkelstein, Pronovost, and Brotman. Acquisition of data: Drs. Michtalik, Streiff, Brotman and Mr. Carolan, Mr. Lau, Mrs. Durkin. Analysis and interpretation of data: Drs. Michtalik, Haut, Streiff, Brotman and Mr. Carolan, Mr. Lau. Drafting of the manuscript: Drs. Michtalik and Brotman. Critical revision of the manuscript for important intellectual content: Drs. Michtalik, Haut, Streiff, Finkelstein, Pronovost, Brotman and Mr. Carolan, Mr. Lau, Mrs. Durkin. Statistical analysis and supervision: Drs. Michtalik and Brotman. Obtaining funding: Drs. Streiff and Brotman. Technical support: Dr. Streiff and Mr. Carolan, Mr. Lau, Mrs. Durkin
This study was supported by a National Institutes of Health grant T32 HP10025‐17‐00 (Dr. Michtalik), the National Institutes of Health/Johns Hopkins Institute for Clinical and Translational Research KL2 Award 5KL2RR025006 (Dr. Michtalik), the Agency for Healthcare Research and Quality Mentored Clinical Scientist Development K08 Awards 1K08HS017952‐01 (Dr. Haut) and 1K08HS022331‐01A1 (Dr. Michtalik), and the Johns Hopkins Hospitalist Scholars Fund. The funders had no role in the design and conduct of the study; collection, management, analysis, and interpretation of the data; preparation, review, or approval of the manuscript; and decision to submit the manuscript for publication.
Dr. Haut receives royalties from Lippincott, Williams & Wilkins. Dr. Streiff has received research funding from Portola and Bristol Myers Squibb, honoraria for CME lectures from Sanofi‐Aventis and Ortho‐McNeil, consulted for Eisai, Daiichi‐Sankyo, Boerhinger‐Ingelheim, Janssen Healthcare, and Pfizer. Mr. Lau, Drs. Haut, Streiff, and Pronovost are supported by a contract from the Patient‐Centered Outcomes Research Institute (PCORI) titled Preventing Venous Thromboembolism: Empowering Patients and Enabling Patient‐Centered Care via Health Information Technology (CE‐12‐11‐4489). Dr. Brotman has received research support from Siemens Healthcare Diagnostics, Bristol‐Myers Squibb, the Agency for Healthcare Research and Quality, Centers for Medicare & Medicaid Services, the Amerigroup Corporation, and the Guerrieri Family Foundation. He has received honoraria from the Gerson Lehrman Group, the Dunn Group, and from Quantia Communications, and received royalties from McGraw‐Hill.
The Affordable Care Act explicitly outlines improving the value of healthcare by increasing quality and decreasing costs. It emphasizes value‐based purchasing, the transparency of performance metrics, and the use of payment incentives to reward quality.[1, 2] Venous thromboembolism (VTE) prophylaxis is one of these publicly reported performance measures. The National Quality Forum recommends that each patient be evaluated on hospital admission and during their hospitalization for VTE risk level and for appropriate thromboprophylaxis to be used, if required.[3] Similarly, the Joint Commission includes appropriate VTE prophylaxis in its Core Measures.[4] Patient experience and performance metrics, including VTE prophylaxis, constitute the hospital value‐based purchasing (VBP) component of healthcare reform.[5] For a hypothetical 327‐bed hospital, an estimated $1.7 million of a hospital's inpatient payments from Medicare will be at risk from VBP alone.[2]
VTE prophylaxis is a common target of quality improvement projects. Effective, safe, and cost‐effective measures to prevent VTE exist, including pharmacologic and mechanical prophylaxis.[6, 7] Despite these measures, compliance rates are often below 50%.[8] Different interventions have been pursued to ensure appropriate VTE prophylaxis, including computerized provider order entry (CPOE), electronic alerts, mandatory VTE risk assessment and prophylaxis, and provider education campaigns.[9] Recent studies show that CPOE systems with mandatory fields can increase VTE prophylaxis rates to above 80%, yet the goal of a high reliability health system is for 100% of patients to receive recommended therapy.[10, 11, 12, 13, 14, 15] Interventions to improve prophylaxis rates that have included multiple strategies, such as computerized order sets, feedback, and education, have been the most effective, increasing compliance to above 90%.[9, 11, 16] These systems can be enhanced with additional interventions such as providing individualized provider education and feedback, understanding of work flow, and ensuring patients receive the prescribed therapies.[12] For example, a physician dashboard could be employed to provide a snapshot and historical trend of key performance indicators using graphical displays and indicators.[17]
Dashboards and pay‐for‐performance programs have been increasingly used to increase the visibility of these metrics, provide feedback, visually display benchmarks and goals, and proactively monitor for achievements and setbacks.[18] Although these strategies are often addressed at departmental (or greater) levels, applying them at the level of the individual provider may assist hospitals in reducing preventable harm and achieving safety and quality goals, especially at higher benchmarks. With their expanding role, hospitalists provide a key opportunity to lead improvement efforts and to study the impact of dashboards and pay‐for performance at the provider level to achieve VTE prophylaxis performance targets. Hospitalists are often the front‐line provider for inpatients and deliver up to 70% of inpatient general medical services.[19] The objective of our study was to evaluate the impact of providing individual provider feedback and employing a pay‐for‐performance program on baseline performance of VTE prophylaxis among hospitalists. We hypothesized that performance feedback through the use of a dashboard would increase appropriate VTE prophylaxis, and this effect would be further augmented by incorporation of a pay‐for‐performance program.
METHODS
Hospitalist Dashboard
In 2010, hospitalist program leaders met with hospital administrators to create a hospitalist dashboard that would provide regularly updated summaries of performance measures for individual hospitalists. The final set of metrics identified included appropriate VTE prophylaxis, length of stay, patients discharged per day, discharges before 3 pm, depth of coding, patient satisfaction, readmissions, communication with the primary care provider, and time to signature for discharge summaries (Figure 1A). The dashboard was introduced at a general hospitalist meeting during which its purpose, methodology, and accessibility were described; it was subsequently implemented in January 2011.
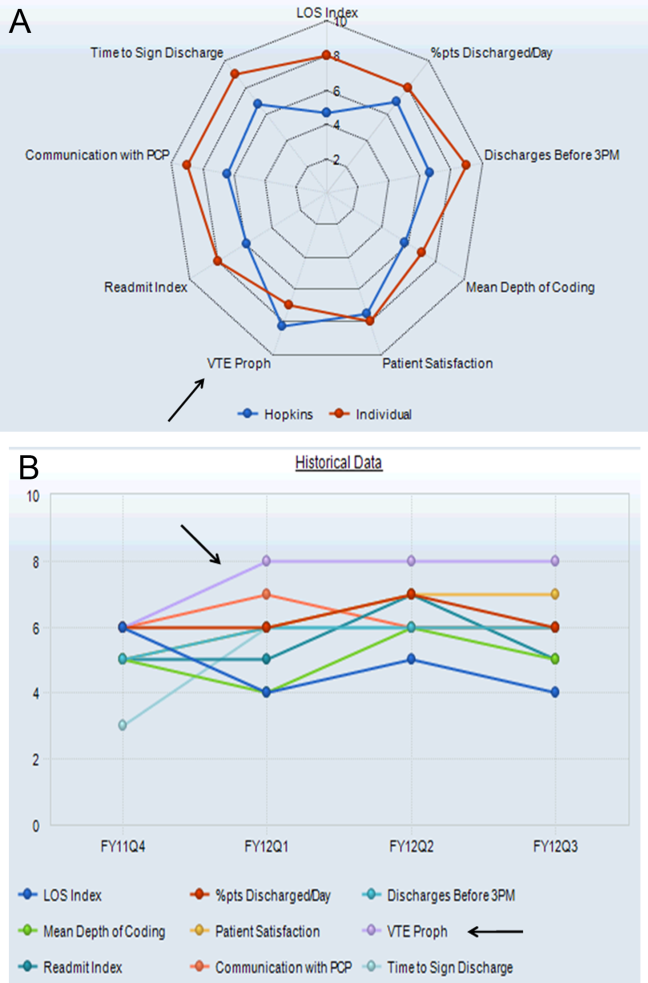
Benchmarks were established for each metric, standardized to establish a scale ranging from 1 through 9, and incorporated into the dashboard (Figure 1A). Higher scores (creating a larger geometric shape) were desirable. For the VTE prophylaxis measure, scores of 1 through 9 corresponded to <60%, 60% to 64.9%, 65% to 69.9%, 70% to 74.9%, 75% to 79.9%, 80% to 84.9%, 85% to 89.9%, 90% to 94.9%, and 95% American College of Chest Physicians (ACCP)‐compliant VTE prophylaxis, respectively.[12, 20] Each provider was able to access the aggregated dashboard (showing the group mean) and his/her individualized dashboard using an individualized login and password for the institutional portal. This portal is used during the provider's workflow, including medical record review and order entry. Both a polygonal summary graphic (Figure 1A) and trend (Figure 1B) view of the dashboard were available to the provider. A comparison of the individual provider to the hospitalist group average was displayed (Figure 1A). At monthly program meetings, the dashboard, group results, and trends were discussed.
Venous Thromboembolism Prophylaxis Compliance
Our study was performed in a tertiary academic medical center with an approximately 20‐member hospitalist group (the precise membership varied over time), whose responsibilities include, among other clinical duties, staffing a 17‐bed general medicine unit with telemetry. The scope of diagnoses and acuity of patients admitted to the hospitalist service is similar to the housestaff services. Some hospitalist faculty serve both as hospitalist and nonhospitalist general medicine service team attendings, but the comparison groups were staffed by hospitalists for <20% of the time. For admissions, all hospitalists use a standardized general medicine admission order set that is integrated into the CPOE system (Sunrise Clinical Manager; Allscripts, Chicago, IL) and completed for all admitted patients. A mandatory VTE risk screen, which includes an assessment of VTE risk factors and pharmacological prophylaxis contraindications, must be completed by the ordering physician as part of this order set (Figure 2A). The system then prompts the provider with a risk‐appropriate VTE prophylaxis recommendation that the provider may subsequently order, including mechanical prophylaxis (Figure 2B). Based on ACCP VTE prevention guidelines, risk‐appropriate prophylaxis was determined using an electronic algorithm that categorized patients into risk categories based on the presence of major VTE risk factors (Figure 2A).[12, 15, 20] If none of these were present, the provider selected No major risk factors known. Both an assessment of current use of anticoagulation and a clinically high risk of bleeding were also included (Figure 2A). If none of these were present, the provider selected No contraindications known. This algorithm is published in detail elsewhere and has been shown to not increase major bleeding episodes.[12, 15] The VTE risk assessment, but not the VTE order itself, was a mandatory field. This allowed the physician discretion to choose among various pharmacological agents and mechanical mechanisms based on patient and physician preferences.
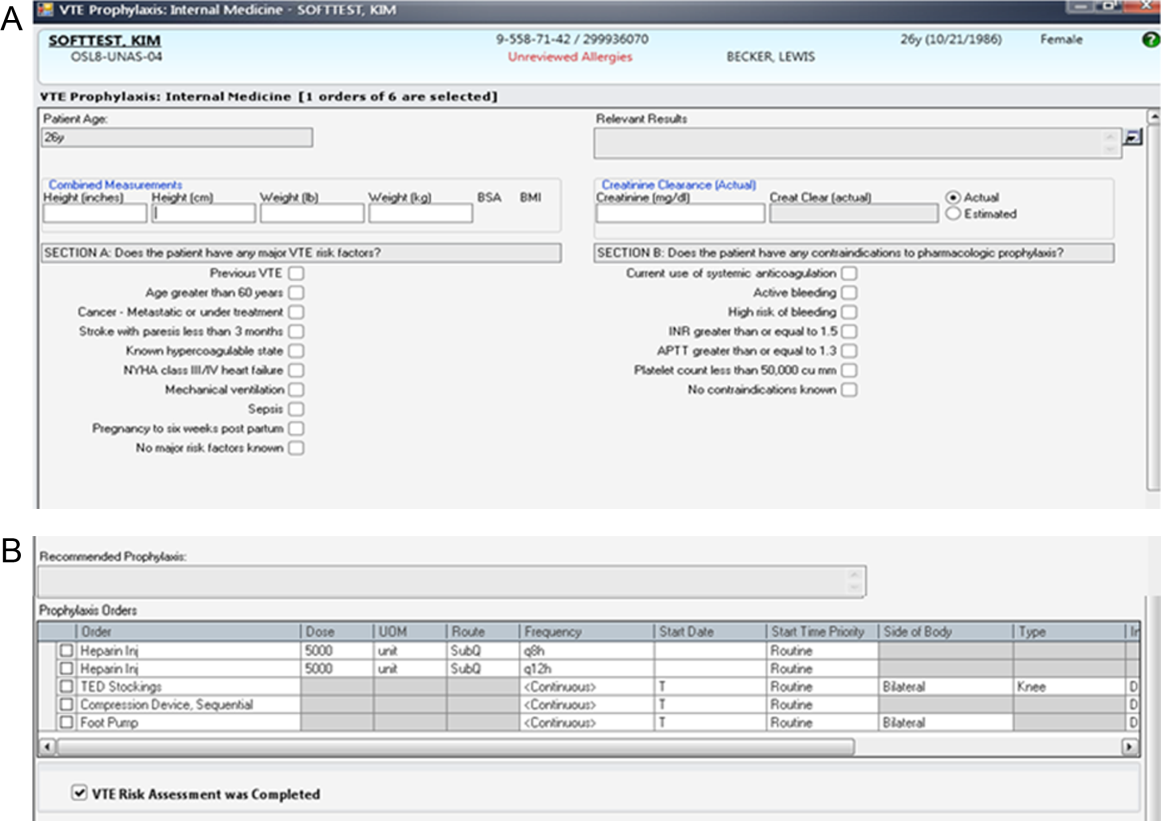
Compliance of risk‐appropriate VTE prophylaxis was determined 24 hours after the admission order set was completed using an automated electronic query of the CPOE system. Low molecular‐weight heparin prescription was included in the compliance algorithm as acceptable prophylaxis. Prescription of pharmacological VTE prophylaxis when a contraindication was present was considered noncompliant. The metric was assigned to the attending physician who billed for the first inpatient encounter.
Pay‐for‐Performance Program
In July 2011, a pay‐for‐performance program was added to the dashboard. All full‐time and part‐time hospitalists were eligible. The financial incentive was determined according to hospital priority and funds available. The VTE prophylaxis metric was prorated by clinical effort, with a maximum of $0.50 per work relative value unit (RVU). To optimize performance, a threshold of 80% compliance had to be surpassed before any payment was made. Progressively increasing percentages of the incentive were earned as compliance increased from 80% to 100%, corresponding to dashboard scores of 6, 7, 8, and 9: <80% (scores 1 to 5)=no payment; 80% to 84.9% (score 6)=$0.125 per RVU; 85% to 89.9% (score 7)=$0.25 per RVU; 90% to 94.9% (score 8)=$0.375 per RVU; and 95% (score 9)=$0.50 per RVU (maximum incentive). Payments were accrued quarterly and paid at the end of the fiscal year as a cumulative, separate performance supplement.
Individualized physician feedback through the dashboard was continued during the pay‐for‐performance period. Average hospitalist group compliance continued to be displayed on the electronic dashboard and was explicitly reviewed at monthly hospitalist meetings.
The VTE prophylaxis order set and data collection and analyses were approved by the Johns Hopkins Medicine Institutional Review Board. The dashboard and pay‐for‐performance program were initiated by the institution as part of a proof of concept quality improvement project.
Analysis
We examined all inpatient admissions to the hospitalist unit from 2008 to 2012. We included patients admitted to and discharged from the hospitalist unit and excluded patients transferred into/out of the unit and encounters with a length of stay <24 hours. VTE prophylaxis orders were queried from the CPOE system 24 hours after the patient was admitted to determine compliance.
After allowing for a run‐in period (2008), we analyzed the change in percent compliance for 3 periods: (1) CPOE‐based VTE order set alone (baseline [BASE], January 2009 to December 2010); (2) group and individual physician feedback using the dashboard (dashboard only [DASH], January to June 2011); and (3) dashboard tied to the pay‐for‐performance program (dashboard with pay‐for‐performance [P4P], July 2011 to December 2012). The CPOE‐based VTE order set was used during all 3 periods. We used the other medical services as a control to ensure that there were no temporal trends toward improved prophylaxis on a service without the intervention. VTE prophylaxis compliance was examined by calculating percent compliance using the same algorithm for the 4 resident‐staffed general medicine service teams at our institution, which utilized the same CPOE system but did not receive the dashboard or pay‐for‐performance interventions. We used locally weighted scatterplot smoothing, a locally weighted regression of percent compliance over time, to graphically display changes in group compliance over time.[21, 22]
We also performed linear regression to assess the rate of change in group compliance and included spline terms that allowed slope to vary for each of the 3 time periods.[23, 24] Clustered analysis accounted for potentially correlated serial measurements of compliance for an individual provider. A separate analysis examined the effect of provider turnover and individual provider improvement during each of the 3 periods. Tests of significance were 2‐sided, with an level of 0.05. Statistical analysis was performed using Stata 12.1 (StataCorp LP, College Station, TX).
RESULTS
Venous Thromboembolism Prophylaxis Compliance
We analyzed 3144 inpatient admissions by 38 hospitalists from 2009 to 2012. The 5 most frequent coded diagnoses were heart failure, acute kidney failure, syncope, pneumonia, and chest pain. Patients had a median length of stay of 3 days [interquartile range: 26]. During the dashboard‐only period, on average, providers improved in compliance by 4% (95% confidence interval [CI]: 35; P<0.001). With the addition of the pay‐for‐performance program, providers improved by an additional 4% (95% CI: 35; P<0.001). Group compliance significantly improved from 86% (95% CI: 8588) during the BASE period of the CPOE‐based VTE order set to 90% (95% CI: 8893) during the DASH period (P=0.01) and 94% (95% CI: 9396) during the subsequent P4P program (P=0.01) (Figure 3). Both inappropriate prophylaxis and lack of prophylaxis, when indicated, resulted in a non‐compliance rating. During the 3 periods, inappropriate prophylaxis decreased from 7.9% to 6.2% to 2.6% during the BASE, DASH, and subsequent P4P periods, respectively. Similarly, lack of prophylaxis when indicated decreased from 6.1% to 3.2% to 3.1% during the BASE, DASH, and subsequent P4P periods, respectively.
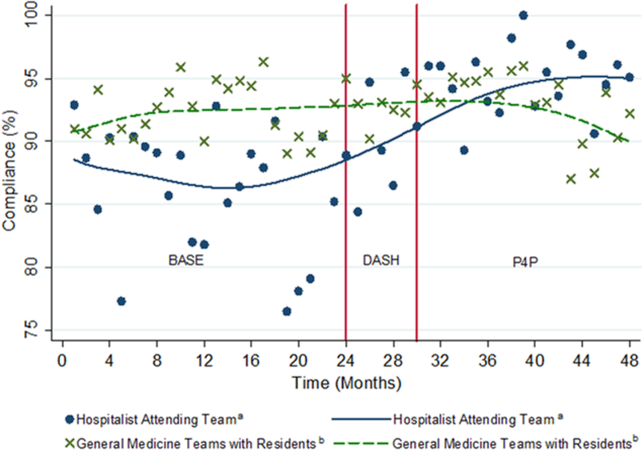
The average compliance of the 4 non‐hospitalist general medicine service teams was initially higher than that of the hospitalist service during the CPOE‐based VTE order set (90%) and DASH (92%) periods, but subsequently plateaued and was exceeded by the hospitalist service during the combined P4P (92%) period (Figure 3). However, there was no statistically significant difference between the general medicine service teams and hospitalist service during the DASH (P=0.15) and subsequent P4P (P=0.76) periods.
We also analyzed the rate of VTE prophylaxis compliance improvement (slope) with cut points at each time period transition (Figure 3). Risk‐appropriate VTE prophylaxis during the BASE period did not exhibit significant improvement as indicated by the slope (P=0.23) (Figure 3). In contrast, during the DASH period, VTE prophylaxis compliance significantly increased by 1.58% per month (95% CI: 0.41‐2.76; P=0.01). The addition of the P4P program, however, did not further significantly increase the rate of compliance (P=0.78).
A subgroup analysis restricted to the 19 providers present during all 3 periods was performed to assess for potential confounding from physician turnover. The percent compliance increased in a similar fashion: BASE period of CPOE‐based VTE order set, 85% (95% CI: 8386); DASH, 90% (95% CI: 8893); and P4P, 94% (95% CI: 9296).
Pay‐for‐Performance Program
Nineteen providers met the threshold for pay‐for‐performance (80% appropriate VTE prophylaxis), with 9 providers in the intermediate categories (80%94.9%) and 10 in the full incentive category (95%). The mean individual payout for the incentive was $633 (standard deviation 350), with a total disbursement of $12,029. The majority of payments (17 of 19) were under $1000.
DISCUSSION
A key component of healthcare reform has been value‐based purchasing, which emphasizes extrinsic motivation through the transparency of performance metrics and use of payment incentives to reward quality. Our study evaluates the impact of both extrinsic (payments) and intrinsic (professionalism and peer norms) motivation. It specifically attributed an individual performance metric, VTE prophylaxis, to an attending physician, provided both individualized and group feedback using an electronic dashboard, and incorporated a pay‐for‐performance program. Prescription of risk‐appropriate VTE prophylaxis significantly increased with the implementation of the dashboard and subsequent pay‐for performance program. The fastest rate of improvement occurred after the addition of the dashboard. Sensitivity analyses for provider turnover and comparisons to the general medicine services showed our results to be independent of a general trend of improvement, both at the provider and institutional levels.
Our prior studies demonstrated that order sets significantly improve performance, from a baseline compliance of risk‐appropriate VTE prophylaxis of 66% to 84%.[13, 15, 25] In the current study, compliance was relatively flat during the BASE period, which included these order sets. The greatest rate of continued improvement in compliance occurred during the DASH period, emphasizing both the importance of provider feedback and receptivity and adaptability in the prescribing behavior of hospitalists. Because the goal of a high‐reliability health system is for 100% of patients to receive recommended therapy, multiple approaches are necessary for success.
Nationally, benchmarks for performance measures continue to be raised, with the highest performers achieving above 95%.[26] Additional interventions, such as dashboards and pay‐for‐performance programs, supplement CPOE systems to achieve high reliability. In our study, the compliance rate during the baseline period, which included a CPOE‐based, clinical support‐enabled VTE order set, was 86%. Initially the compliance of the general medicine teams with residents exceeded that of the hospitalist attending teams, which may reflect a greater willingness of resident teams to comply with order sets and automated recommendations. This emphasizes the importance of continuous individual feedback and provider education at the attending physician level to enhance both guideline compliance and decrease provider care variation. Ultimately, with the addition of the dashboard and subsequent pay‐for‐performance program, compliance was increased to 90% and 94%, respectively. Although the major mechanism used by policymakers to improve quality of care is extrinsic motivation, this study demonstrates that intrinsic motivation through peer norms can enhance extrinsic efforts and may be more influential. Both of these programs, dashboards and pay‐for‐performance, may ultimately assist institutions in changing provider behavior and achieving these harder‐to‐achieve higher benchmarks.
We recognize that there are several limitations to our study. First, this is a single‐site program limited to an attending‐physician‐only service. There was strong data support and a defined CPOE algorithm for this initiative. Multi‐site studies will need to overcome the additional challenges of varying service structures and electronic medical record and provider order entry systems. Second, it is difficult to show actual changes in VTE events over time with appropriate prophylaxis. Although VTE prophylaxis is recommended for patients with VTE risk factors, there are conflicting findings about whether prophylaxis prevents VTE events in lower‐risk patients, and current studies suggest that most patients with VTE events are severely ill and develop VTE despite receiving prophylaxis.[27, 28, 29] Our study was underpowered to detect these potential differences in VTE rates, and although the algorithm has been shown to not increase bleeding rates, we did not measure bleeding rates during this study.[12, 15] Our institutional experience suggests that the majority of VTE events occur despite appropriate prophylaxis.[30] Also, VTE prophylaxis may be ordered, but intervening events, such as procedures and changes in risk status or patient refusal, may prevent patients from receiving appropriate prophylaxis.[31, 32] Similarly, hospitals with higher quality scores have higher VTE prophylaxis rates but worse risk‐adjusted VTE rates, which may result from increased surveillance for VTE, suggesting surveillance bias limits the usefulness of the VTE quality measure.[33, 34] Nevertheless, VTE prophylaxis remains a publicly reported Core Measure tied to financial incentives.[4, 5] Third, there may be an unmeasured factor specific to the hospitalist program, which could potentially account for an overall improvement in quality of care. Although the rate of increase in appropriate prophylaxis was not statistically significant during the baseline period, there did appear to be some improvement in prophylaxis toward the end of the period. However, there were no other VTE‐related provider feedback programs being simultaneously pursued during this study. VTE prophylaxis for the non‐hospitalist services showed a relatively stable, non‐increasing compliance rate for the general medical services. Although it was possible for successful residents to age into the hospitalist service, thereby improving rates of prophylaxis based on changes in group makeup, our subgroup analysis of the providers present throughout all phases of the study showed our results to be robust. Similarly, there may have been a cross‐contamination effect of hospitalist faculty who attended on both hospitalist and non‐hospitalist general medicine service teams. This, however, would attenuate any impact of the programs, and thus the effects may in fact be greater than reported. Fourth, establishment of both the dashboard and pay‐for‐performance program required significant institutional and program leadership and resources. To be successful, the dashboard must be in the provider's workflow, transparent, minimize reporter burden, use existing systems, and be actively fed back to providers, ideally those directly entering orders. Our greatest rate of improvement occurred during the feedback‐only phase of this study, emphasizing the importance of physician feedback, provider‐level accountability, and engagement. We suspect that the relatively modest pay‐for‐performance incentive served mainly as a means of engaging providers in self‐monitoring, rather than as a means to change behavior through true incentivization. Although we did not track individual physician views of the dashboard, we reinforced trends, deviations, and expectations at regularly scheduled meetings and provided feedback and patient‐level data to individual providers. Fifth, the design of the pay‐for‐performance program may have also influenced its effectiveness. These types of programs may be more effective when they provide frequent visible, small payments rather than one large payment, and when the payment is framed as a loss rather than a gain.[35] Finally, physician champions and consistent feedback through departmental meetings or visual displays may be required for program success. The initial resources to create the dashboard, continued maintenance and monitoring of performance, and payment of financial incentives all require institutional commitment. A partnership of physicians, program leaders, and institutional administrators is necessary for both initial and continued success.
To achieve performance goals and benchmarks, multiple strategies that combine extrinsic and intrinsic motivation are necessary. As shown by our study, the use of a dashboard and pay‐for‐performance can be tailored to an institution's goals, in line with national standards. The specific goal (risk‐appropriate VTE prophylaxis) and benchmarks (80%, 85%, 90%, 95%) can be individualized to a particular institution. For example, if readmission rates are above target, readmissions could be added as a dashboard metric. The specific benchmark would be determined by historical trends and administrative targets. Similarly, the overall financial incentives could be adjusted based on the financial resources available. Other process measures, such as influenza vaccination screening and administration, could also be targeted. For all of these objectives, continued provider feedback and engagement are critical for progressive success, especially to decrease variability in care at the attending physician level. Incorporating the value‐based purchasing philosophy from the Affordable Care Act, our study suggests that the combination of standardized order sets, real‐time dashboards, and physician‐level incentives may assist hospitals in achieving quality and safety benchmarks, especially at higher targets.
Acknowledgements
The authors thank Meir Gottlieb, BS, from Salar Inc. for data support; Murali Padmanaban, BS, from Johns Hopkins University for his assistance in linking the administrative billing data with real‐time physician orders; and Hsin‐Chieh Yeh, PhD, from the Bloomberg School of Public Health for her statistical advice and additional review. We also thank Mr. Ronald R. Peterson, President, Johns Hopkins Health System and Johns Hopkins Hospital, for providing funding support for the physician incentive payments.
Disclosures: Drs. Michtalik and Brotman had full access to all of the data in the study and take responsibility for the integrity of the data and accuracy of the data analysis. Study concept and design: Drs. Michtalik, Streiff, Finkelstein, Pronovost, and Brotman. Acquisition of data: Drs. Michtalik, Streiff, Brotman and Mr. Carolan, Mr. Lau, Mrs. Durkin. Analysis and interpretation of data: Drs. Michtalik, Haut, Streiff, Brotman and Mr. Carolan, Mr. Lau. Drafting of the manuscript: Drs. Michtalik and Brotman. Critical revision of the manuscript for important intellectual content: Drs. Michtalik, Haut, Streiff, Finkelstein, Pronovost, Brotman and Mr. Carolan, Mr. Lau, Mrs. Durkin. Statistical analysis and supervision: Drs. Michtalik and Brotman. Obtaining funding: Drs. Streiff and Brotman. Technical support: Dr. Streiff and Mr. Carolan, Mr. Lau, Mrs. Durkin
This study was supported by a National Institutes of Health grant T32 HP10025‐17‐00 (Dr. Michtalik), the National Institutes of Health/Johns Hopkins Institute for Clinical and Translational Research KL2 Award 5KL2RR025006 (Dr. Michtalik), the Agency for Healthcare Research and Quality Mentored Clinical Scientist Development K08 Awards 1K08HS017952‐01 (Dr. Haut) and 1K08HS022331‐01A1 (Dr. Michtalik), and the Johns Hopkins Hospitalist Scholars Fund. The funders had no role in the design and conduct of the study; collection, management, analysis, and interpretation of the data; preparation, review, or approval of the manuscript; and decision to submit the manuscript for publication.
Dr. Haut receives royalties from Lippincott, Williams & Wilkins. Dr. Streiff has received research funding from Portola and Bristol Myers Squibb, honoraria for CME lectures from Sanofi‐Aventis and Ortho‐McNeil, consulted for Eisai, Daiichi‐Sankyo, Boerhinger‐Ingelheim, Janssen Healthcare, and Pfizer. Mr. Lau, Drs. Haut, Streiff, and Pronovost are supported by a contract from the Patient‐Centered Outcomes Research Institute (PCORI) titled Preventing Venous Thromboembolism: Empowering Patients and Enabling Patient‐Centered Care via Health Information Technology (CE‐12‐11‐4489). Dr. Brotman has received research support from Siemens Healthcare Diagnostics, Bristol‐Myers Squibb, the Agency for Healthcare Research and Quality, Centers for Medicare & Medicaid Services, the Amerigroup Corporation, and the Guerrieri Family Foundation. He has received honoraria from the Gerson Lehrman Group, the Dunn Group, and from Quantia Communications, and received royalties from McGraw‐Hill.
- Medicare Program, Centers for Medicare 76(88):26490–26547.
- Quality meets finance: payments at risk with value‐based purchasing, readmission, and hospital‐acquired conditions force hospitalists to focus. Hospitalist. 2013;17(1):31. .
- National Quality Forum. March 2009. Safe practices for better healthcare—2009 update. Available at: http://www.qualityforum.org/Publications/2009/03/Safe_Practices_for_Better_Healthcare%E2%80%932009_Update.aspx. Accessed November 1, 2014.
- Joint Commission on Accreditation of Healthcare Organizations. Approved: more options for hospital core measures. Jt Comm Perspect. 2009;29(4):1–6.
- Centers for Medicare 208(2):227–240.
- Thromboprophylaxis in nonsurgical patients. Hematology Am Soc Hematol Educ Program. 2012;2012:631–637. , .
- Venous thromboembolism risk and prophylaxis in the acute hospital care setting (ENDORSE study): a multinational cross‐sectional study. Lancet. 2008;371(9610):387–394. , , , et al.
- Practices to prevent venous thromboembolism: a brief review. BMJ Qual Saf. 2014;23(3):187–195. , .
- Improving hospital venous thromboembolism prophylaxis with electronic decision support. J Hosp Med. 2013;8(3):115–120. , , , et al.
- Innovative approaches to increase deep vein thrombosis prophylaxis rate resulting in a decrease in hospital‐acquired deep vein thrombosis at a tertiary‐care teaching hospital. J Hosp Med. 2008;3(2):148–155. , , .
- Lessons from the Johns Hopkins Multi‐Disciplinary Venous Thromboembolism (VTE) Prevention Collaborative. BMJ. 2012;344:e3935. , , , et al.
- Improved prophylaxis and decreased rates of preventable harm with the use of a mandatory computerized clinical decision support tool for prophylaxis for venous thromboembolism in trauma. Arch Surg. 2012;147(10):901–907. , , , et al.
- Designing and implementing effective venous thromboembolism prevention protocols: lessons from collaborative efforts. J Thromb Thrombolysis. 2010;29(2):159–166. , .
- Impact of a venous thromboembolism prophylaxis "smart order set": improved compliance, fewer events. Am J Hematol. 2013;88(7):545–549. , , , et al.
- Improving adherence to venous thromoembolism prophylaxis using multiple interventions. BMJ. 2012;344:e3935. , .
- Health Resources and Services Administration of the U.S. Department of Health and Human Services. Managing data for performance improvement. Available at: http://www.hrsa.gov/quality/toolbox/methodology/performanceimprovement/part2.html. Accessed December 18, 2014.
- Improving patient safety by taking systems seriously. JAMA. 2008;299(4):445–447. , .
- Growth in the care of older patients by hospitalists in the United States. N Engl J Med. 2009;360(11):1102–1112. , , , .
- Prevention of venous thromboembolism: American College of Chest Physicians evidence‐based clinical practice guidelines (8th edition). Chest. 2008;133(6 suppl):381S–453S. , , , et al.
- Robust locally weighted regression and smoothing scatterplots. J Am Stat Assoc. 1979;74(368):829–836. .
- Locally weighted regression: An approach to regression analysis by local fitting. J Am Stat Assoc. 1988;83(403):596–610. , .
- Regression Methods in Biostatistics: Linear, Logistic, Survival, and Repeated Measures Models. 2nd ed. New York, NY: Springer; 2012. , , , .
- Regression Modeling Strategies: With Applications to Linear Models, Logistic Regression, and Survival Analysis. New York, NY: Springer‐Verlag; 2001. .
- Med Care. doi: 10.1097/MLR.0000000000000251. , , , et al. Eliminating healthcare disparities via mandatory clinical decision support: the venous thromboembolism (VTE) example [published online ahead of print November 4, 2014].
- Joint Commission. Improving America's hospitals: the Joint Commission's annual report on quality and safety. 2012. Available at: http://www.jointcommission.org/assets/1/18/TJC_Annual_Report_2012.pdf. Accessed September 8, 2013.
- Hospital performance for pharmacologic venous thromboembolism prophylaxis and rate of venous thromboembolism: a cohort study. JAMA Intern Med. 2014;174(10):1577–1584. , , , et al.
- Incidence of hospital‐acquired venous thromboembolic codes in medical patients hospitalized in academic medical centers. J Hosp Med. 2014;9(4):221–225. , , , et al.
- No association between hospital‐reported perioperative venous thromboembolism prophylaxis and outcome rates in publicly reported data. JAMA Surg. 2014;149(4):400–401. , , , , .
- Linking processes and outcomes: a key strategy to prevent and report harm from venous thromboembolism in surgical patients. JAMA Surg. 2013;148(3):299–300. , , , , .
- Patterns of non‐administration of ordered doses of venous thromboembolism prophylaxis: implications for novel intervention strategies. PLoS One. 2013;8(6):e66311. , , , et al.
- Nonadministration of thromboprophylaxis in hospitalized patients with HIV: a missed opportunity for prevention? J Hosp Med. 2014;9(4):215–220. , , , et al.
- Evaluation of surveillance bias and the validity of the venous thromboembolism quality measure. JAMA. 2013;310(14):1482–1489. , , , et al.
- Surveillance bias in outcomes reporting. JAMA. 2011;305(23):2462–2463. , .
- Pay for performance in health care: an international overview of initiatives. Med Care Res Rev. 2012;69(3):251–276. .
- Medicare Program, Centers for Medicare 76(88):26490–26547.
- Quality meets finance: payments at risk with value‐based purchasing, readmission, and hospital‐acquired conditions force hospitalists to focus. Hospitalist. 2013;17(1):31. .
- National Quality Forum. March 2009. Safe practices for better healthcare—2009 update. Available at: http://www.qualityforum.org/Publications/2009/03/Safe_Practices_for_Better_Healthcare%E2%80%932009_Update.aspx. Accessed November 1, 2014.
- Joint Commission on Accreditation of Healthcare Organizations. Approved: more options for hospital core measures. Jt Comm Perspect. 2009;29(4):1–6.
- Centers for Medicare 208(2):227–240.
- Thromboprophylaxis in nonsurgical patients. Hematology Am Soc Hematol Educ Program. 2012;2012:631–637. , .
- Venous thromboembolism risk and prophylaxis in the acute hospital care setting (ENDORSE study): a multinational cross‐sectional study. Lancet. 2008;371(9610):387–394. , , , et al.
- Practices to prevent venous thromboembolism: a brief review. BMJ Qual Saf. 2014;23(3):187–195. , .
- Improving hospital venous thromboembolism prophylaxis with electronic decision support. J Hosp Med. 2013;8(3):115–120. , , , et al.
- Innovative approaches to increase deep vein thrombosis prophylaxis rate resulting in a decrease in hospital‐acquired deep vein thrombosis at a tertiary‐care teaching hospital. J Hosp Med. 2008;3(2):148–155. , , .
- Lessons from the Johns Hopkins Multi‐Disciplinary Venous Thromboembolism (VTE) Prevention Collaborative. BMJ. 2012;344:e3935. , , , et al.
- Improved prophylaxis and decreased rates of preventable harm with the use of a mandatory computerized clinical decision support tool for prophylaxis for venous thromboembolism in trauma. Arch Surg. 2012;147(10):901–907. , , , et al.
- Designing and implementing effective venous thromboembolism prevention protocols: lessons from collaborative efforts. J Thromb Thrombolysis. 2010;29(2):159–166. , .
- Impact of a venous thromboembolism prophylaxis "smart order set": improved compliance, fewer events. Am J Hematol. 2013;88(7):545–549. , , , et al.
- Improving adherence to venous thromoembolism prophylaxis using multiple interventions. BMJ. 2012;344:e3935. , .
- Health Resources and Services Administration of the U.S. Department of Health and Human Services. Managing data for performance improvement. Available at: http://www.hrsa.gov/quality/toolbox/methodology/performanceimprovement/part2.html. Accessed December 18, 2014.
- Improving patient safety by taking systems seriously. JAMA. 2008;299(4):445–447. , .
- Growth in the care of older patients by hospitalists in the United States. N Engl J Med. 2009;360(11):1102–1112. , , , .
- Prevention of venous thromboembolism: American College of Chest Physicians evidence‐based clinical practice guidelines (8th edition). Chest. 2008;133(6 suppl):381S–453S. , , , et al.
- Robust locally weighted regression and smoothing scatterplots. J Am Stat Assoc. 1979;74(368):829–836. .
- Locally weighted regression: An approach to regression analysis by local fitting. J Am Stat Assoc. 1988;83(403):596–610. , .
- Regression Methods in Biostatistics: Linear, Logistic, Survival, and Repeated Measures Models. 2nd ed. New York, NY: Springer; 2012. , , , .
- Regression Modeling Strategies: With Applications to Linear Models, Logistic Regression, and Survival Analysis. New York, NY: Springer‐Verlag; 2001. .
- Med Care. doi: 10.1097/MLR.0000000000000251. , , , et al. Eliminating healthcare disparities via mandatory clinical decision support: the venous thromboembolism (VTE) example [published online ahead of print November 4, 2014].
- Joint Commission. Improving America's hospitals: the Joint Commission's annual report on quality and safety. 2012. Available at: http://www.jointcommission.org/assets/1/18/TJC_Annual_Report_2012.pdf. Accessed September 8, 2013.
- Hospital performance for pharmacologic venous thromboembolism prophylaxis and rate of venous thromboembolism: a cohort study. JAMA Intern Med. 2014;174(10):1577–1584. , , , et al.
- Incidence of hospital‐acquired venous thromboembolic codes in medical patients hospitalized in academic medical centers. J Hosp Med. 2014;9(4):221–225. , , , et al.
- No association between hospital‐reported perioperative venous thromboembolism prophylaxis and outcome rates in publicly reported data. JAMA Surg. 2014;149(4):400–401. , , , , .
- Linking processes and outcomes: a key strategy to prevent and report harm from venous thromboembolism in surgical patients. JAMA Surg. 2013;148(3):299–300. , , , , .
- Patterns of non‐administration of ordered doses of venous thromboembolism prophylaxis: implications for novel intervention strategies. PLoS One. 2013;8(6):e66311. , , , et al.
- Nonadministration of thromboprophylaxis in hospitalized patients with HIV: a missed opportunity for prevention? J Hosp Med. 2014;9(4):215–220. , , , et al.
- Evaluation of surveillance bias and the validity of the venous thromboembolism quality measure. JAMA. 2013;310(14):1482–1489. , , , et al.
- Surveillance bias in outcomes reporting. JAMA. 2011;305(23):2462–2463. , .
- Pay for performance in health care: an international overview of initiatives. Med Care Res Rev. 2012;69(3):251–276. .
© 2014 Society of Hospital Medicine