User login
Hospitalist‐Run Postdischarge Clinic
Currently, healthcare systems rarely provide ideal transitions of care for discharged patients,[1] resulting in fragmented care,[2, 3, 4, 5] significant patient uncertainty about how to manage at home,[6, 7] and frequent adverse events.[8, 9] These factors are so commonly experienced by discharged patients that they are recognizable as a postdischarge syndrome.[10]
One element important for reducing the postdischarge risk of adverse events is provision of adequate follow‐up.[11, 12] However, supplying this care is challenging in the modern era, and it will become progressively more difficult to achieve. In 2004, 50% of readmitted Medicare fee‐for‐service patients had no postdischarge visit within 30 days of their discharge,[9] likely due in part to difficulty arranging such care. Changes in insurance coverage and demographics are expected to result in more than 100 million newly insured patients by 2019, yet the primary‐care workforce is projected to begin shrinking by 2016.[13, 14] In the increasingly uncommon situation that a primary‐care clinician is available promptly after discharge, information transfer is often inadequate[4, 15, 16, 17] and can be exacerbated by the growing discontinuity between inpatient and outpatient care.[2, 3, 4] Efforts to increase the supply of primary‐care clinicians and thereby improve early access to postdischarge care are important for the future, but hospitals, particularly those penalized for high risk‐adjusted readmission rates, are seeking novel solutions now.
One increasingly common innovation is to extend the role of inpatient providers (usually hospitalists) into the postdischarge period.[18] Preliminary evidence suggests improved continuity[19] and access[20] achieved by providing this care may decrease postdischarge adverse events,[19, 20, 21] though evidence is conflicting.[22]
As a closed, multilevel healthcare system, the Denver VA Medical Center is uniquely positioned to evaluate the influence of alternative postdischarge‐care strategies on subsequent adverse events. Discharged patients are seen in a well‐established hospitalist‐run postdischarge clinic (PDC), a robust urgent‐care system (UC), or by a large primary‐care provider (PCP) practice. The purpose of this study was to evaluate whether patients seen in a hospitalist‐run PDC have reduced adverse outcomes in the 30 days following hospital discharge compared with follow‐up with the patient's PCP or in an UC clinic.
METHODS
Patients
This was a retrospective cohort study of consecutive adult patients discharged from the general medical services of the Denver VA Medical Center after a nonelective admission between January 2005 and August 2012. This time range was chosen because all 3 clinics were fully operational during this period. The Denver VA Medical Center is an academically‐affiliated 128‐bed hospital that provides a full range of tertiary services. All medical patients, including intensive care unit (ICU) patients, are cared for on general medical teams by University of Colorado housestaff with hospitalists or subspecialty attendings. Patients who lived in the Denver metropolitan area, were discharged home, and who followed up with a PCP, UC clinic, or PDC within 30 days of discharge were included. Patients discharged to subacute facilities, hospice, or this tends to be capitalized as a special program at our VA were excluded. For patients with multiple admissions, only the first was included.
Clinics
Primary Care
Primary‐care clinics in the VA system are organized into Patient‐Aligned Care Teams (PACTs) and are available for appointments 5 days per week. Patients discharged from the medical service who have PCPs are called within 48 hours of discharge by PACT nurses to evaluate their postdischarge state. Primary‐care physicians could be resident housestaff or ambulatory attending physicians. Seventy‐two percent of patients seen at the Denver VA have an assigned PCP.
Urgent Care
The Office‐based Medical Team provides UC and short‐term regular appointments for recently discharged medical patients or patients who require frequent follow‐up (such as those that require serial paracenteses). It is a separate clinic from an emergency department (ED)‐based walk‐in clinic. It is also available 5 days per week; patients are seen by resident housestaff unfamiliar with the patient, and the clinic is staffed with an ambulatory attending physician. Patients are commonly seen multiple times in the same clinic, though usually with different providers.
Postdischarge Clinic
The hospitalist‐run PDC is scheduled 2 afternoons per week. Patients are always seen by housestaff and medical students from the team that cared for them as an inpatient, then staffed with a rotating hospitalist attending who may have been the supervising inpatient attending during the patient's inpatient stay. Thus, continuity is preserved with the housestaff team in all cases, although attending continuity is variable. This is added to the daily responsibility of the resident and hospitalist physicians who are providing care on the inpatient service at the time of the clinic. Capabilities of the clinic are similar to UC and PCP clinics. Patients are usually seen once postdischarge with referral to the PCP for further follow‐up; however, patients can be seen multiple times by the same provider team.
If a patient followed up with multiple clinics, the first clinic visited determined the group to which that patient was allocated for the purpose of analysis. If a patient was scheduled for clinic follow‐up but did not attend within 30 days of discharge, he or she was excluded. We did not collect data on visits outside of these 3 clinics, as pilot data demonstrated they accounted for nearly all (>90%) of posthospitalization follow‐up visits. During the study period, there were no guidelines for discharging physicians about which clinic to have the patient follow up in. The UC and PDC were known to have better early access to follow‐up appointments and thus tended to see patients requiring early follow‐up in the judgment of the discharging clinician.
Statistical Analysis
The VA's Computing and Informatics Infrastructure (VINCI) was used to collect predischarge patient data for descriptive and analytic purposes. Pertinent potential confounders included patient age, sex, marital status, comorbidities, number of prescribed medications on discharge, previous hospital admissions in the last year, ICU admission (as a dichotomous variable), ICU length of stay (LOS), and hospital LOS. Postdischarge variables included time to first follow‐up appointment and hospital LOS if readmitted.
The primary outcome was a composite of ED visits, hospital readmissions, and mortality in the 30 days following hospital discharge. These outcomes were captured in the VA system; we did not measure outside utilization. A power analysis indicated that the sample has >90% power to detect small differences (4%) in the composite outcome between types of outpatient care. We also evaluated the effect of different types of follow‐up on the 3 individual components of the primary outcome. To compare baseline categorical variables across 3 groups, 2 trend tests were used; analysis of variance (ANOVA) or Kruskal‐Wallis test was used for continuous variables in univariate analysis.
We then used propensity scoring to adjust for baseline differences between groups in an attempt to adjust for referral bias, using multivariate logistic regression to calculate a propensity score for each patient in 2‐way comparisons, and a single score for every patient in a multinomial comparison.[23] Our final propensity score incorporated age, number of hospital admissions in the past year, and Elixhauser comorbidity score,[24] with excellent overlap in propensity scores between groups. Although hospital LOS was different between groups, inclusion in the propensity score did not reduce this significant difference, and its inclusion in the propensity model decreased model fit. Limitations of the accessible data prevented high‐dimensional propensity scoring and limited the outcome of the propensity score to attendance at the clinic assigned, rather than referral to the clinic assigned. The propensity score, hospital LOS, time to the first outpatient visit, and group assignment (PDC, PCP, UC) were entered into a multivariate logistic regression model.
To find a subgroup who may benefit most from follow‐up in the PDC, we a priori identified patients with one of the 5 discharge diagnosis‐related groups (DRGs) most commonly associated with subsequent readmission[9] and examined outcomes between the 3 different kinds of follow‐up, restricted to patients discharged with one of these diagnoses. All analyses were conducted using SAS 9.3 (SAS Institute, Inc., Cary, NC).
RESULTS
9952 patients who met criteria were discharged during this time period; however, 48.9% did not follow up with one of these clinics within 30 days, leaving 5085 patients in our analysis. Of these, 538 followed up in PDC (10.6%), 1848 followed up with their PCP (36.3%), and 2699 followed up in UC (53.1%). Table 1 presents predischarge characteristics of these patients. Patients seen in PDC were older and had a more significant comorbidity burden.
PDC, N=538 | UC, N=2699 | PCP, N=1848 | P Value | P Value After Propensity Adjustment | |
---|---|---|---|---|---|
| |||||
Age, years (SD) | 67.8 (12.6) | 67.1 (13.0) | 64.8 (13.0) | <0.01 | 0.86 |
Male sex, % | 95.0 | 95.4 | 94.4 | 0.33 | |
Marital status, % | |||||
Divorced | 40.2 | 36.2 | 35.0 | 0.09 | |
Married | 35.9 | 37.3 | 39.8 | 0.13 | |
Never married | 12.3 | 13.7 | 14.3 | 0.48 | |
LOS, days (SD) | 3.8 (3.6) | 5.0 (11.7) | 6.2 (10.8) | 0.04 | |
Elixhauser score (SD) | 0.80 (1.1) | 0.69 (1.0) | 0.75 (1.0) | 0.02 | 0.06 |
Admitted to ICU, % | 19.0 | 19.9 | 23.0 | 0.12 | |
ICU LOS, days (SD) | 2.8 (4.4) | 2.8 (3.4) | 2.3 (1.5) | 0.15 | |
Discharge medications, mean (SD) | 10.0 (6.7) | 10.4 (7.4) | 10.4 (8.2) | 0.37 | |
Admissions per patient in prior year, mean (SD) | 0.18 (0.5) | 0.21 (0.6) | 0.23 (0.6) | 0.08 | 0.78 |
Patients seen in PDC had a mean 2.4‐day shorter LOS than those seen by their PCPs (PDC: 3.8 days, UC: 5.0 days, PCP: 6.2 days; P=0.04 for comparison). Neither the percentage of patients admitted to the ICU during their index hospitalization nor the ICU LOS was different between groups. Patients were seen earlier postdischarge in PDC than in other types of follow‐up (PDC: 5.0 days, UC: 9.4 days, PCP: 13.7 days; P<0.01 for comparison). In univariate analysis, there was no difference between groups in the composite 30‐day outcome (Table 2). Analysis of the individual components of the primary outcome revealed significant differences in readmission rates, with PDC having the highest rate.
PDC | UC | PCP | P Value | P Value After Propensity Adjustment | |
---|---|---|---|---|---|
| |||||
Composite outcome, % | 19.9 | 18.3 | 17.5 | 0.42 | 0.30 |
Hospital readmission | 13.0 | 11.1 | 9.4 | 0.03 | 0.03 |
ED visit | 10.2 | 9.9 | 10.5 | 0.78 | 0.93 |
Mortality | 1.1 | 0.7 | 0.7 | 0.58 | 0.65 |
LOS if readmitted, days (SD) | 6.9 (18.1) | 4.9 (7.8) | 4.8 (6.5) | 0.28 | 0.23 |
Time to first visit after discharge, days (SD) | 5.0 (3.0) | 9.4 (6.1) | 13.8 (8.5) | <0.01 | <0.01 |
Univariate analyses conducted on predischarge characteristics after multinomial propensity scoring revealed significant differences between groups no longer existed for the variables that were included in the propensity score (age, Elixhauser score, and inpatient stays prior to visit; Table 1).
In multivariate analysis comparing PDC to PCP follow‐up, there was no difference in the composite outcome after controlling for propensity score and time to outpatient visit (odds ratio [OR]: 1.07, 95% confidence interval [CI]: 0.81‐1.40). Similar results were obtained in comparing PDC with UC (OR: 1.05, 95% CI: 0.82‐1.34) and in multinomial logistic regression comparing PDC with other types of follow‐up (PDC vs PCP: OR: 1.01, 95% CI: 0.78‐1.31; PDC vs UC: OR: 0.99, 95% CI: 0.78‐1.26).
Restricting the multivariate analysis to those patients discharged with one of the 5 discharge DRGs most associated with readmission did not alter our findings regarding the primary outcome. We also found no change in the composite outcome or any subcomponent of the composite outcome when restricting the analysis to 7‐day outcomes or when excluding scheduled readmissions (which represented <5% of all readmission).
DISCUSSION
A hospitalist‐run postdischarge clinic did not reduce a composite of 30‐day postdischarge adverse outcomes in our study when compared with primary‐care or urgent‐care follow‐up. In fact, patients who followed up in PDC had a small increase in 30‐day readmissions. However, they also were sicker at baseline, considered higher risk by the discharging physician, were able to be seen significantly earlier, and had an associated 2.4‐day shorter hospital LOS than patients seen by their PCPs.
Our findings do not confirm those of prior research in this area, which indicated outpatient follow‐up by the same physician who was the treating inpatient physician was linked to lower mortality rates, hospital readmissions, and ED utilization.[19, 20, 21] In fact, in our study, there was a significant (albeit small) increase in 30‐day readmissions in patients seen in PDC. There are significant challenges to the generalizability and validity of these prior studies. In one study, inpatient care was provided by outpatient primary‐care doctors in Canada,[19] a payer and care model rare in the United States.[25] In a second, usual care was not specified, and it is likely the reduction in ED visits resulted from provision of follow‐up care of any kind compared with those who did not follow up after discharge.[20] In a third, the PDC was part of a larger bundle of postdischarge interventions, and it only reduced ED visits when compared with patients who did not have follow‐up; rates of ED visits were similar in a comparison with PCP follow‐up.[21]
There are several possible explanations for the lack of improvement in 30‐day adverse outcomes with a hospitalist‐run PDC. First, although early access to early postdischarge care was improved and evidence suggests this is important in reducing readmissions,[11, 12] in populations similar to that studied, more postdischarge care has also been linked to increased readmissions.[22] This may be due to more frequent re‐evaluation of fragile, chronically ill patients, presenting more options for readmission. Second, the intervention currently only addresses some components of the Ideal Transition of Care[1] (Figure 1) and may benefit from an enhanced visit structure using a multidisciplinary approach. Third, the intervention took place in the context of a robust primary‐care system with a universal electronic medical record; the effects of improved access and continuity may be magnified in a system without these advantages. Fourth, there was a low readmission rate overall, and it is unclear how many of these readmissions were preventable. Finally, it may be that although the initial postdischarge care was adequate, readmissions occurred after the first visit, suggesting subsequent care during the 30 days postdischarge could have been improved.
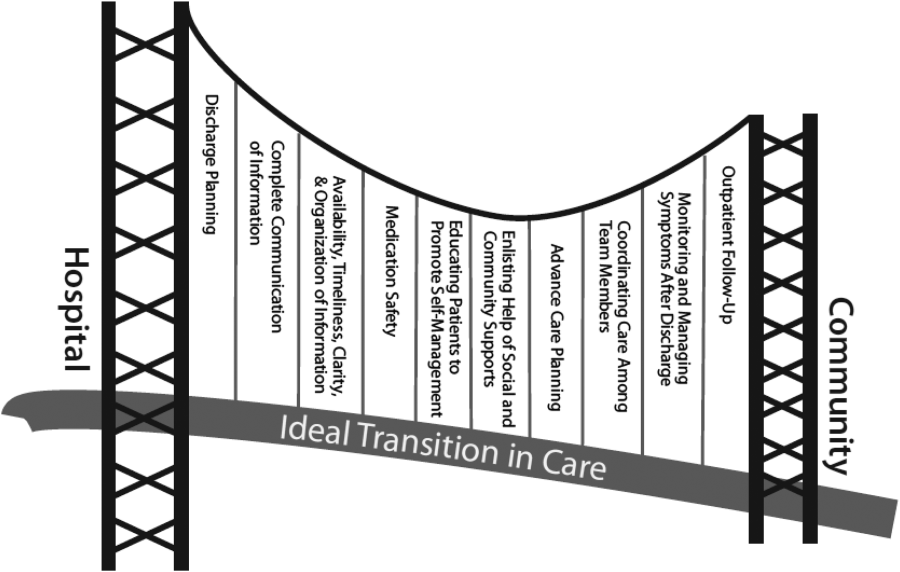
The most likely explanation for the substantially decreased LOS associated with follow‐up in PDC is that inpatient physicians who knew they could see their own patients early in the postdischarge process were more tolerant of uncertainty surrounding the patients' clinical course.
For example, a frequent clinical conundrum for hospitalists is when to discharge patients improving on diuretic therapy for a heart failure exacerbation or antibiotics for cellulitis. Provided a PDC, these hospitalists may choose to discharge a patient still actively being treated, because they may feel they have access to early follow‐up to change course if needed as well as the ability to see the patient themselves, allowing precise evaluation of the change in their condition. Without this clinic, the hospitalist may wonder when postdischarge follow‐up will occur. They may be more hesitant to discharge a patient who has not fully completed treatment for fear he or she will still appear decompensated to the postdischarge provider (though greatly improved from admission), or will not have timely‐enough follow‐up to change treatment if the condition worsens.
Our finding that the LOS was still shorter when comparing PDC with UC suggests continuity may be a significant component of this effect. It seems unlikely that patients following up in PDC had less complex hospitalizations given similar ICU exposure and LOS, as well as older age and larger baseline comorbidity burden.
The LOS seen in patients who followed up in PDC was lower than Medicare rates[26] but similar to reported rates at other VA acute‐care hospitals.[27] It is consistent with prior findings that hospitalist care reduces LOS,[26] though the magnitude in our study was much larger than that in prior reports. Prior studies have suggested this decreased LOS is linked to increased adverse postdischarge outcomes, such as ED visits and readmissions, as well as increased costs and decreased discharges to home.[28] The PDC was not associated with increased postdischarge adverse events measured, though a formal cost analysis and analysis of other postdischarge outcomes, such as placement in a skilled nursing or rehabilitation facility after return home, could be assessed in future work.
The findings of our study should be interpreted in the context of the study design. Our study was retrospective, observational, and single‐center. There may have been additional baseline differences between groups predisposing to bias we did not capture in the propensity score. For example, we could not measure rates of attendance at the different clinics and cannot rule out that outcomes associated with PDC were also associated with increased attendance rates. However, none of the clinics had mechanisms in place to improve follow‐up rates; patients referred to PDC were those considered highest risk for readmission and were sicker at baseline, making it very unlikely that they were predisposed to attend clinic more frequently and/or to have better outcomes; and even if PDC improved follow‐up rates, this would be a significant contribution given the limitations of primary‐care access. Our propensity score could not perfectly mimic randomization to a treatment assignment, but rather to treatment received, because of this limitation.
We did not ascertain ED visits or readmissions outside the VA system; it is possible these differentially affected one group more than another, though this seems unlikely. Our patient population was representative of veteran populations elsewhere who are at high risk of adverse postdischarge outcomes, but our findings may not be generalizable to younger, more ethnically diverse populations or to women.
CONCLUSIONS
Provision of postdischarge care by hospitalists may reduce LOS without increasing postdischarge adverse events. Further work is required to evaluate the role of hospitalist‐run PDCs in healthcare systems with more limited postdischarge access to care, to formally evaluate the costs associated with extending hospitalists to the outpatient setting, and to prospectively evaluate the role of a PDC compared with other kinds of hospital follow‐up.
Acknowledgments
The authors thank Melver Anderson, MD, for editorial assistance with the manuscript.
Disclosures: Dr. Burke had full access to all of the data in the study and takes responsibility for the integrity of the data and the accuracy of the data analysis. The views expressed in this article are those of the authors and do not necessarily represent the views of the Department of Veterans Affairs. Dr. Burke was supported by grant funding from the Colorado Research Enhancement Award Program to Improve Care Coordination for Veterans. Initial results of this study were presented at the Society of General Internal Medicine National Meeting in Denver, Colorado, April 24, 2013.
- Moving beyond readmission penalties: creating an ideal process to improve transitional care. J Hosp Med. 2013;8(2):102–109. , , , .
- Continuity of outpatient and inpatient care by primary care physicians for hospitalized older adults. JAMA. 2009;301(16):1671–1680. , , , , , .
- Trends in inpatient continuity of care for a cohort of Medicare patients 1996–2006. J Hosp Med. 2011;6(8):438–444. , , , , .
- Deficits in communication and information transfer between hospital‐based and primary care physicians: implications for patient safety and continuity of care. JAMA. 2007;297(8):831–841. , , , , , .
- Posthospital care transitions: patterns, complications, and risk identification. Health Serv Res. 2004;39(5):1449–1465. , , , .
- Patient experiences of transitioning from hospital to home: an ethnographic quality improvement project. J Hosp Med. 2012;7(5):382–387. , , , , .
- Understanding and execution of discharge instructions. Am J Med Qual. 2013;28(5):383–391. , , , et al.
- The incidence and severity of adverse events affecting patients after discharge from the hospital. Ann Intern Med. 2003;138(3):161–167. , , , , .
- Rehospitalizations among patients in the Medicare fee‐for‐service program. N Engl J Med. 2009;360(14):1418–1428. , , .
- Post‐hospital syndrome—an acquired, transient condition of generalized risk. N Engl J Med. 2013;368(2):100–102. .
- Relationship between early physician follow‐up and 30‐day readmission among Medicare beneficiaries hospitalized for heart failure. JAMA. 2010;303(17):1716–1722. , , , et al.
- Post‐hospitalization transitions: examining the effects of timing of primary care provider follow‐up. J Hosp Med. 2010;5(7):392–397. , , .
- Will generalist physician supply meet demands of an increasing and aging population? Health Aff (Millwood). 2008;27(3):w232–w241. , , .
- Association of American Medical Colleges. The Impact of Health Care Reform on the Future Supply and Demand for Physicians: Updated Projections Through 2025. Available at: http://www.aamc.org/download/158076/data/updated_projections_through_2025.pdf. Published June 2010. Accessed May 1, 2012.
- Effect of discharge summary availability during post‐discharge visits on hospital readmission. J Gen Intern Med. 2002;17(3):186–192. , , , .
- Comprehensive quality of discharge summaries at an academic medical center. J Hosp Med. 2013;8(8):436–443. , , , et al.
- Association of communication between hospital‐based physicians and primary care providers with patient outcomes. J Gen Intern Med. 2009;24(3):381–386. , , , et al.
- Is a post‐discharge clinic in your hospital's future? Available at: http://www.the‐hospitalist.org/details/article/1409011/Is_a_Post‐Discharge_Clinic_in_Your_Hospitals_Future.html. Published December 2011. Accessed May 1, 2013. .
- Continuity of care and patient outcomes after hospital discharge. J Gen Intern Med. 2004;19(6):624–631. , , , .
- Effects of a postdischarge clinic on housestaff satisfaction and utilization of hospital services. J Gen Intern Med. 1996;11(3):179–181. , , , .
- Integrated postdischarge transitional care in a hospitalist system to improve discharge outcome: an experimental study. BMC Med. 2011;9:96. , , , , , .
- Does increased access to primary care reduce hospital readmissions? Veterans Affairs Cooperative Study Group on Primary Care and Hospital Readmission. N Engl J Med. 1996;334(22):1441–1447. , , .
- An overview of the objectives of and the approaches to propensity score analyses. Eur Heart J. 2011;32(14):1704–1708. , .
- Comparison of the Elixhauser and Charlson/Deyo methods of comorbidity measurement in administrative data. Med Care. 2004;42(4):355–360. , , .
- Growth in the care of older patients by hospitalists in the United States. N Engl J Med. 2009;360(11):1102–1112. , , , .
- Association of hospitalist care with medical utilization after discharge: evidence of cost shift from a cohort study. Ann Intern Med. 2011;155(3):152–159. , .
- Associations between reduced hospital length of stay and 30‐day readmission rate and mortality: 14‐year experience in 129 Veterans Affairs hospitals. Ann Intern Med. 2012;157(12):837–845. , , , et al.
- Variation in length of stay and outcomes among hospitalized patients attributable to hospitals and hospitalists. J Gen Intern Med. 2013;28(3):370–376. , , , .
Currently, healthcare systems rarely provide ideal transitions of care for discharged patients,[1] resulting in fragmented care,[2, 3, 4, 5] significant patient uncertainty about how to manage at home,[6, 7] and frequent adverse events.[8, 9] These factors are so commonly experienced by discharged patients that they are recognizable as a postdischarge syndrome.[10]
One element important for reducing the postdischarge risk of adverse events is provision of adequate follow‐up.[11, 12] However, supplying this care is challenging in the modern era, and it will become progressively more difficult to achieve. In 2004, 50% of readmitted Medicare fee‐for‐service patients had no postdischarge visit within 30 days of their discharge,[9] likely due in part to difficulty arranging such care. Changes in insurance coverage and demographics are expected to result in more than 100 million newly insured patients by 2019, yet the primary‐care workforce is projected to begin shrinking by 2016.[13, 14] In the increasingly uncommon situation that a primary‐care clinician is available promptly after discharge, information transfer is often inadequate[4, 15, 16, 17] and can be exacerbated by the growing discontinuity between inpatient and outpatient care.[2, 3, 4] Efforts to increase the supply of primary‐care clinicians and thereby improve early access to postdischarge care are important for the future, but hospitals, particularly those penalized for high risk‐adjusted readmission rates, are seeking novel solutions now.
One increasingly common innovation is to extend the role of inpatient providers (usually hospitalists) into the postdischarge period.[18] Preliminary evidence suggests improved continuity[19] and access[20] achieved by providing this care may decrease postdischarge adverse events,[19, 20, 21] though evidence is conflicting.[22]
As a closed, multilevel healthcare system, the Denver VA Medical Center is uniquely positioned to evaluate the influence of alternative postdischarge‐care strategies on subsequent adverse events. Discharged patients are seen in a well‐established hospitalist‐run postdischarge clinic (PDC), a robust urgent‐care system (UC), or by a large primary‐care provider (PCP) practice. The purpose of this study was to evaluate whether patients seen in a hospitalist‐run PDC have reduced adverse outcomes in the 30 days following hospital discharge compared with follow‐up with the patient's PCP or in an UC clinic.
METHODS
Patients
This was a retrospective cohort study of consecutive adult patients discharged from the general medical services of the Denver VA Medical Center after a nonelective admission between January 2005 and August 2012. This time range was chosen because all 3 clinics were fully operational during this period. The Denver VA Medical Center is an academically‐affiliated 128‐bed hospital that provides a full range of tertiary services. All medical patients, including intensive care unit (ICU) patients, are cared for on general medical teams by University of Colorado housestaff with hospitalists or subspecialty attendings. Patients who lived in the Denver metropolitan area, were discharged home, and who followed up with a PCP, UC clinic, or PDC within 30 days of discharge were included. Patients discharged to subacute facilities, hospice, or this tends to be capitalized as a special program at our VA were excluded. For patients with multiple admissions, only the first was included.
Clinics
Primary Care
Primary‐care clinics in the VA system are organized into Patient‐Aligned Care Teams (PACTs) and are available for appointments 5 days per week. Patients discharged from the medical service who have PCPs are called within 48 hours of discharge by PACT nurses to evaluate their postdischarge state. Primary‐care physicians could be resident housestaff or ambulatory attending physicians. Seventy‐two percent of patients seen at the Denver VA have an assigned PCP.
Urgent Care
The Office‐based Medical Team provides UC and short‐term regular appointments for recently discharged medical patients or patients who require frequent follow‐up (such as those that require serial paracenteses). It is a separate clinic from an emergency department (ED)‐based walk‐in clinic. It is also available 5 days per week; patients are seen by resident housestaff unfamiliar with the patient, and the clinic is staffed with an ambulatory attending physician. Patients are commonly seen multiple times in the same clinic, though usually with different providers.
Postdischarge Clinic
The hospitalist‐run PDC is scheduled 2 afternoons per week. Patients are always seen by housestaff and medical students from the team that cared for them as an inpatient, then staffed with a rotating hospitalist attending who may have been the supervising inpatient attending during the patient's inpatient stay. Thus, continuity is preserved with the housestaff team in all cases, although attending continuity is variable. This is added to the daily responsibility of the resident and hospitalist physicians who are providing care on the inpatient service at the time of the clinic. Capabilities of the clinic are similar to UC and PCP clinics. Patients are usually seen once postdischarge with referral to the PCP for further follow‐up; however, patients can be seen multiple times by the same provider team.
If a patient followed up with multiple clinics, the first clinic visited determined the group to which that patient was allocated for the purpose of analysis. If a patient was scheduled for clinic follow‐up but did not attend within 30 days of discharge, he or she was excluded. We did not collect data on visits outside of these 3 clinics, as pilot data demonstrated they accounted for nearly all (>90%) of posthospitalization follow‐up visits. During the study period, there were no guidelines for discharging physicians about which clinic to have the patient follow up in. The UC and PDC were known to have better early access to follow‐up appointments and thus tended to see patients requiring early follow‐up in the judgment of the discharging clinician.
Statistical Analysis
The VA's Computing and Informatics Infrastructure (VINCI) was used to collect predischarge patient data for descriptive and analytic purposes. Pertinent potential confounders included patient age, sex, marital status, comorbidities, number of prescribed medications on discharge, previous hospital admissions in the last year, ICU admission (as a dichotomous variable), ICU length of stay (LOS), and hospital LOS. Postdischarge variables included time to first follow‐up appointment and hospital LOS if readmitted.
The primary outcome was a composite of ED visits, hospital readmissions, and mortality in the 30 days following hospital discharge. These outcomes were captured in the VA system; we did not measure outside utilization. A power analysis indicated that the sample has >90% power to detect small differences (4%) in the composite outcome between types of outpatient care. We also evaluated the effect of different types of follow‐up on the 3 individual components of the primary outcome. To compare baseline categorical variables across 3 groups, 2 trend tests were used; analysis of variance (ANOVA) or Kruskal‐Wallis test was used for continuous variables in univariate analysis.
We then used propensity scoring to adjust for baseline differences between groups in an attempt to adjust for referral bias, using multivariate logistic regression to calculate a propensity score for each patient in 2‐way comparisons, and a single score for every patient in a multinomial comparison.[23] Our final propensity score incorporated age, number of hospital admissions in the past year, and Elixhauser comorbidity score,[24] with excellent overlap in propensity scores between groups. Although hospital LOS was different between groups, inclusion in the propensity score did not reduce this significant difference, and its inclusion in the propensity model decreased model fit. Limitations of the accessible data prevented high‐dimensional propensity scoring and limited the outcome of the propensity score to attendance at the clinic assigned, rather than referral to the clinic assigned. The propensity score, hospital LOS, time to the first outpatient visit, and group assignment (PDC, PCP, UC) were entered into a multivariate logistic regression model.
To find a subgroup who may benefit most from follow‐up in the PDC, we a priori identified patients with one of the 5 discharge diagnosis‐related groups (DRGs) most commonly associated with subsequent readmission[9] and examined outcomes between the 3 different kinds of follow‐up, restricted to patients discharged with one of these diagnoses. All analyses were conducted using SAS 9.3 (SAS Institute, Inc., Cary, NC).
RESULTS
9952 patients who met criteria were discharged during this time period; however, 48.9% did not follow up with one of these clinics within 30 days, leaving 5085 patients in our analysis. Of these, 538 followed up in PDC (10.6%), 1848 followed up with their PCP (36.3%), and 2699 followed up in UC (53.1%). Table 1 presents predischarge characteristics of these patients. Patients seen in PDC were older and had a more significant comorbidity burden.
PDC, N=538 | UC, N=2699 | PCP, N=1848 | P Value | P Value After Propensity Adjustment | |
---|---|---|---|---|---|
| |||||
Age, years (SD) | 67.8 (12.6) | 67.1 (13.0) | 64.8 (13.0) | <0.01 | 0.86 |
Male sex, % | 95.0 | 95.4 | 94.4 | 0.33 | |
Marital status, % | |||||
Divorced | 40.2 | 36.2 | 35.0 | 0.09 | |
Married | 35.9 | 37.3 | 39.8 | 0.13 | |
Never married | 12.3 | 13.7 | 14.3 | 0.48 | |
LOS, days (SD) | 3.8 (3.6) | 5.0 (11.7) | 6.2 (10.8) | 0.04 | |
Elixhauser score (SD) | 0.80 (1.1) | 0.69 (1.0) | 0.75 (1.0) | 0.02 | 0.06 |
Admitted to ICU, % | 19.0 | 19.9 | 23.0 | 0.12 | |
ICU LOS, days (SD) | 2.8 (4.4) | 2.8 (3.4) | 2.3 (1.5) | 0.15 | |
Discharge medications, mean (SD) | 10.0 (6.7) | 10.4 (7.4) | 10.4 (8.2) | 0.37 | |
Admissions per patient in prior year, mean (SD) | 0.18 (0.5) | 0.21 (0.6) | 0.23 (0.6) | 0.08 | 0.78 |
Patients seen in PDC had a mean 2.4‐day shorter LOS than those seen by their PCPs (PDC: 3.8 days, UC: 5.0 days, PCP: 6.2 days; P=0.04 for comparison). Neither the percentage of patients admitted to the ICU during their index hospitalization nor the ICU LOS was different between groups. Patients were seen earlier postdischarge in PDC than in other types of follow‐up (PDC: 5.0 days, UC: 9.4 days, PCP: 13.7 days; P<0.01 for comparison). In univariate analysis, there was no difference between groups in the composite 30‐day outcome (Table 2). Analysis of the individual components of the primary outcome revealed significant differences in readmission rates, with PDC having the highest rate.
PDC | UC | PCP | P Value | P Value After Propensity Adjustment | |
---|---|---|---|---|---|
| |||||
Composite outcome, % | 19.9 | 18.3 | 17.5 | 0.42 | 0.30 |
Hospital readmission | 13.0 | 11.1 | 9.4 | 0.03 | 0.03 |
ED visit | 10.2 | 9.9 | 10.5 | 0.78 | 0.93 |
Mortality | 1.1 | 0.7 | 0.7 | 0.58 | 0.65 |
LOS if readmitted, days (SD) | 6.9 (18.1) | 4.9 (7.8) | 4.8 (6.5) | 0.28 | 0.23 |
Time to first visit after discharge, days (SD) | 5.0 (3.0) | 9.4 (6.1) | 13.8 (8.5) | <0.01 | <0.01 |
Univariate analyses conducted on predischarge characteristics after multinomial propensity scoring revealed significant differences between groups no longer existed for the variables that were included in the propensity score (age, Elixhauser score, and inpatient stays prior to visit; Table 1).
In multivariate analysis comparing PDC to PCP follow‐up, there was no difference in the composite outcome after controlling for propensity score and time to outpatient visit (odds ratio [OR]: 1.07, 95% confidence interval [CI]: 0.81‐1.40). Similar results were obtained in comparing PDC with UC (OR: 1.05, 95% CI: 0.82‐1.34) and in multinomial logistic regression comparing PDC with other types of follow‐up (PDC vs PCP: OR: 1.01, 95% CI: 0.78‐1.31; PDC vs UC: OR: 0.99, 95% CI: 0.78‐1.26).
Restricting the multivariate analysis to those patients discharged with one of the 5 discharge DRGs most associated with readmission did not alter our findings regarding the primary outcome. We also found no change in the composite outcome or any subcomponent of the composite outcome when restricting the analysis to 7‐day outcomes or when excluding scheduled readmissions (which represented <5% of all readmission).
DISCUSSION
A hospitalist‐run postdischarge clinic did not reduce a composite of 30‐day postdischarge adverse outcomes in our study when compared with primary‐care or urgent‐care follow‐up. In fact, patients who followed up in PDC had a small increase in 30‐day readmissions. However, they also were sicker at baseline, considered higher risk by the discharging physician, were able to be seen significantly earlier, and had an associated 2.4‐day shorter hospital LOS than patients seen by their PCPs.
Our findings do not confirm those of prior research in this area, which indicated outpatient follow‐up by the same physician who was the treating inpatient physician was linked to lower mortality rates, hospital readmissions, and ED utilization.[19, 20, 21] In fact, in our study, there was a significant (albeit small) increase in 30‐day readmissions in patients seen in PDC. There are significant challenges to the generalizability and validity of these prior studies. In one study, inpatient care was provided by outpatient primary‐care doctors in Canada,[19] a payer and care model rare in the United States.[25] In a second, usual care was not specified, and it is likely the reduction in ED visits resulted from provision of follow‐up care of any kind compared with those who did not follow up after discharge.[20] In a third, the PDC was part of a larger bundle of postdischarge interventions, and it only reduced ED visits when compared with patients who did not have follow‐up; rates of ED visits were similar in a comparison with PCP follow‐up.[21]
There are several possible explanations for the lack of improvement in 30‐day adverse outcomes with a hospitalist‐run PDC. First, although early access to early postdischarge care was improved and evidence suggests this is important in reducing readmissions,[11, 12] in populations similar to that studied, more postdischarge care has also been linked to increased readmissions.[22] This may be due to more frequent re‐evaluation of fragile, chronically ill patients, presenting more options for readmission. Second, the intervention currently only addresses some components of the Ideal Transition of Care[1] (Figure 1) and may benefit from an enhanced visit structure using a multidisciplinary approach. Third, the intervention took place in the context of a robust primary‐care system with a universal electronic medical record; the effects of improved access and continuity may be magnified in a system without these advantages. Fourth, there was a low readmission rate overall, and it is unclear how many of these readmissions were preventable. Finally, it may be that although the initial postdischarge care was adequate, readmissions occurred after the first visit, suggesting subsequent care during the 30 days postdischarge could have been improved.
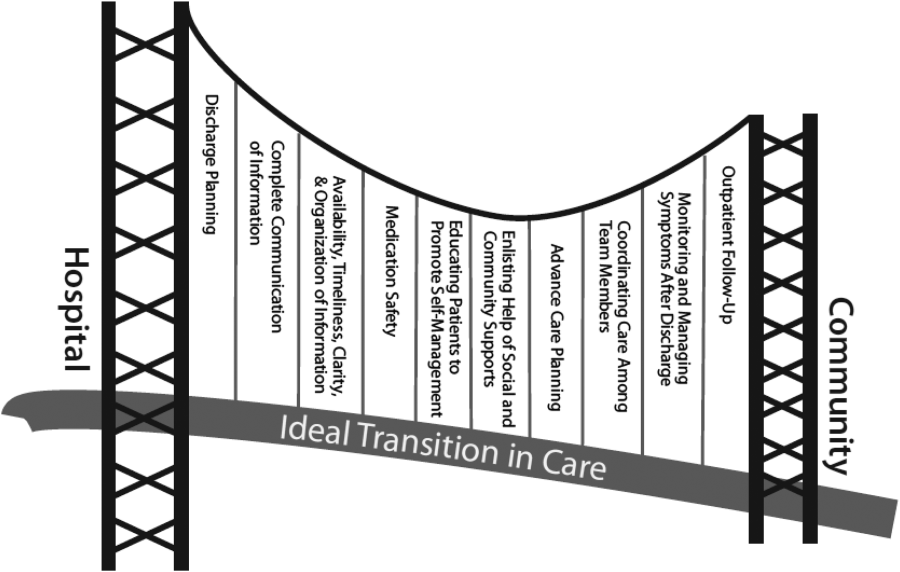
The most likely explanation for the substantially decreased LOS associated with follow‐up in PDC is that inpatient physicians who knew they could see their own patients early in the postdischarge process were more tolerant of uncertainty surrounding the patients' clinical course.
For example, a frequent clinical conundrum for hospitalists is when to discharge patients improving on diuretic therapy for a heart failure exacerbation or antibiotics for cellulitis. Provided a PDC, these hospitalists may choose to discharge a patient still actively being treated, because they may feel they have access to early follow‐up to change course if needed as well as the ability to see the patient themselves, allowing precise evaluation of the change in their condition. Without this clinic, the hospitalist may wonder when postdischarge follow‐up will occur. They may be more hesitant to discharge a patient who has not fully completed treatment for fear he or she will still appear decompensated to the postdischarge provider (though greatly improved from admission), or will not have timely‐enough follow‐up to change treatment if the condition worsens.
Our finding that the LOS was still shorter when comparing PDC with UC suggests continuity may be a significant component of this effect. It seems unlikely that patients following up in PDC had less complex hospitalizations given similar ICU exposure and LOS, as well as older age and larger baseline comorbidity burden.
The LOS seen in patients who followed up in PDC was lower than Medicare rates[26] but similar to reported rates at other VA acute‐care hospitals.[27] It is consistent with prior findings that hospitalist care reduces LOS,[26] though the magnitude in our study was much larger than that in prior reports. Prior studies have suggested this decreased LOS is linked to increased adverse postdischarge outcomes, such as ED visits and readmissions, as well as increased costs and decreased discharges to home.[28] The PDC was not associated with increased postdischarge adverse events measured, though a formal cost analysis and analysis of other postdischarge outcomes, such as placement in a skilled nursing or rehabilitation facility after return home, could be assessed in future work.
The findings of our study should be interpreted in the context of the study design. Our study was retrospective, observational, and single‐center. There may have been additional baseline differences between groups predisposing to bias we did not capture in the propensity score. For example, we could not measure rates of attendance at the different clinics and cannot rule out that outcomes associated with PDC were also associated with increased attendance rates. However, none of the clinics had mechanisms in place to improve follow‐up rates; patients referred to PDC were those considered highest risk for readmission and were sicker at baseline, making it very unlikely that they were predisposed to attend clinic more frequently and/or to have better outcomes; and even if PDC improved follow‐up rates, this would be a significant contribution given the limitations of primary‐care access. Our propensity score could not perfectly mimic randomization to a treatment assignment, but rather to treatment received, because of this limitation.
We did not ascertain ED visits or readmissions outside the VA system; it is possible these differentially affected one group more than another, though this seems unlikely. Our patient population was representative of veteran populations elsewhere who are at high risk of adverse postdischarge outcomes, but our findings may not be generalizable to younger, more ethnically diverse populations or to women.
CONCLUSIONS
Provision of postdischarge care by hospitalists may reduce LOS without increasing postdischarge adverse events. Further work is required to evaluate the role of hospitalist‐run PDCs in healthcare systems with more limited postdischarge access to care, to formally evaluate the costs associated with extending hospitalists to the outpatient setting, and to prospectively evaluate the role of a PDC compared with other kinds of hospital follow‐up.
Acknowledgments
The authors thank Melver Anderson, MD, for editorial assistance with the manuscript.
Disclosures: Dr. Burke had full access to all of the data in the study and takes responsibility for the integrity of the data and the accuracy of the data analysis. The views expressed in this article are those of the authors and do not necessarily represent the views of the Department of Veterans Affairs. Dr. Burke was supported by grant funding from the Colorado Research Enhancement Award Program to Improve Care Coordination for Veterans. Initial results of this study were presented at the Society of General Internal Medicine National Meeting in Denver, Colorado, April 24, 2013.
Currently, healthcare systems rarely provide ideal transitions of care for discharged patients,[1] resulting in fragmented care,[2, 3, 4, 5] significant patient uncertainty about how to manage at home,[6, 7] and frequent adverse events.[8, 9] These factors are so commonly experienced by discharged patients that they are recognizable as a postdischarge syndrome.[10]
One element important for reducing the postdischarge risk of adverse events is provision of adequate follow‐up.[11, 12] However, supplying this care is challenging in the modern era, and it will become progressively more difficult to achieve. In 2004, 50% of readmitted Medicare fee‐for‐service patients had no postdischarge visit within 30 days of their discharge,[9] likely due in part to difficulty arranging such care. Changes in insurance coverage and demographics are expected to result in more than 100 million newly insured patients by 2019, yet the primary‐care workforce is projected to begin shrinking by 2016.[13, 14] In the increasingly uncommon situation that a primary‐care clinician is available promptly after discharge, information transfer is often inadequate[4, 15, 16, 17] and can be exacerbated by the growing discontinuity between inpatient and outpatient care.[2, 3, 4] Efforts to increase the supply of primary‐care clinicians and thereby improve early access to postdischarge care are important for the future, but hospitals, particularly those penalized for high risk‐adjusted readmission rates, are seeking novel solutions now.
One increasingly common innovation is to extend the role of inpatient providers (usually hospitalists) into the postdischarge period.[18] Preliminary evidence suggests improved continuity[19] and access[20] achieved by providing this care may decrease postdischarge adverse events,[19, 20, 21] though evidence is conflicting.[22]
As a closed, multilevel healthcare system, the Denver VA Medical Center is uniquely positioned to evaluate the influence of alternative postdischarge‐care strategies on subsequent adverse events. Discharged patients are seen in a well‐established hospitalist‐run postdischarge clinic (PDC), a robust urgent‐care system (UC), or by a large primary‐care provider (PCP) practice. The purpose of this study was to evaluate whether patients seen in a hospitalist‐run PDC have reduced adverse outcomes in the 30 days following hospital discharge compared with follow‐up with the patient's PCP or in an UC clinic.
METHODS
Patients
This was a retrospective cohort study of consecutive adult patients discharged from the general medical services of the Denver VA Medical Center after a nonelective admission between January 2005 and August 2012. This time range was chosen because all 3 clinics were fully operational during this period. The Denver VA Medical Center is an academically‐affiliated 128‐bed hospital that provides a full range of tertiary services. All medical patients, including intensive care unit (ICU) patients, are cared for on general medical teams by University of Colorado housestaff with hospitalists or subspecialty attendings. Patients who lived in the Denver metropolitan area, were discharged home, and who followed up with a PCP, UC clinic, or PDC within 30 days of discharge were included. Patients discharged to subacute facilities, hospice, or this tends to be capitalized as a special program at our VA were excluded. For patients with multiple admissions, only the first was included.
Clinics
Primary Care
Primary‐care clinics in the VA system are organized into Patient‐Aligned Care Teams (PACTs) and are available for appointments 5 days per week. Patients discharged from the medical service who have PCPs are called within 48 hours of discharge by PACT nurses to evaluate their postdischarge state. Primary‐care physicians could be resident housestaff or ambulatory attending physicians. Seventy‐two percent of patients seen at the Denver VA have an assigned PCP.
Urgent Care
The Office‐based Medical Team provides UC and short‐term regular appointments for recently discharged medical patients or patients who require frequent follow‐up (such as those that require serial paracenteses). It is a separate clinic from an emergency department (ED)‐based walk‐in clinic. It is also available 5 days per week; patients are seen by resident housestaff unfamiliar with the patient, and the clinic is staffed with an ambulatory attending physician. Patients are commonly seen multiple times in the same clinic, though usually with different providers.
Postdischarge Clinic
The hospitalist‐run PDC is scheduled 2 afternoons per week. Patients are always seen by housestaff and medical students from the team that cared for them as an inpatient, then staffed with a rotating hospitalist attending who may have been the supervising inpatient attending during the patient's inpatient stay. Thus, continuity is preserved with the housestaff team in all cases, although attending continuity is variable. This is added to the daily responsibility of the resident and hospitalist physicians who are providing care on the inpatient service at the time of the clinic. Capabilities of the clinic are similar to UC and PCP clinics. Patients are usually seen once postdischarge with referral to the PCP for further follow‐up; however, patients can be seen multiple times by the same provider team.
If a patient followed up with multiple clinics, the first clinic visited determined the group to which that patient was allocated for the purpose of analysis. If a patient was scheduled for clinic follow‐up but did not attend within 30 days of discharge, he or she was excluded. We did not collect data on visits outside of these 3 clinics, as pilot data demonstrated they accounted for nearly all (>90%) of posthospitalization follow‐up visits. During the study period, there were no guidelines for discharging physicians about which clinic to have the patient follow up in. The UC and PDC were known to have better early access to follow‐up appointments and thus tended to see patients requiring early follow‐up in the judgment of the discharging clinician.
Statistical Analysis
The VA's Computing and Informatics Infrastructure (VINCI) was used to collect predischarge patient data for descriptive and analytic purposes. Pertinent potential confounders included patient age, sex, marital status, comorbidities, number of prescribed medications on discharge, previous hospital admissions in the last year, ICU admission (as a dichotomous variable), ICU length of stay (LOS), and hospital LOS. Postdischarge variables included time to first follow‐up appointment and hospital LOS if readmitted.
The primary outcome was a composite of ED visits, hospital readmissions, and mortality in the 30 days following hospital discharge. These outcomes were captured in the VA system; we did not measure outside utilization. A power analysis indicated that the sample has >90% power to detect small differences (4%) in the composite outcome between types of outpatient care. We also evaluated the effect of different types of follow‐up on the 3 individual components of the primary outcome. To compare baseline categorical variables across 3 groups, 2 trend tests were used; analysis of variance (ANOVA) or Kruskal‐Wallis test was used for continuous variables in univariate analysis.
We then used propensity scoring to adjust for baseline differences between groups in an attempt to adjust for referral bias, using multivariate logistic regression to calculate a propensity score for each patient in 2‐way comparisons, and a single score for every patient in a multinomial comparison.[23] Our final propensity score incorporated age, number of hospital admissions in the past year, and Elixhauser comorbidity score,[24] with excellent overlap in propensity scores between groups. Although hospital LOS was different between groups, inclusion in the propensity score did not reduce this significant difference, and its inclusion in the propensity model decreased model fit. Limitations of the accessible data prevented high‐dimensional propensity scoring and limited the outcome of the propensity score to attendance at the clinic assigned, rather than referral to the clinic assigned. The propensity score, hospital LOS, time to the first outpatient visit, and group assignment (PDC, PCP, UC) were entered into a multivariate logistic regression model.
To find a subgroup who may benefit most from follow‐up in the PDC, we a priori identified patients with one of the 5 discharge diagnosis‐related groups (DRGs) most commonly associated with subsequent readmission[9] and examined outcomes between the 3 different kinds of follow‐up, restricted to patients discharged with one of these diagnoses. All analyses were conducted using SAS 9.3 (SAS Institute, Inc., Cary, NC).
RESULTS
9952 patients who met criteria were discharged during this time period; however, 48.9% did not follow up with one of these clinics within 30 days, leaving 5085 patients in our analysis. Of these, 538 followed up in PDC (10.6%), 1848 followed up with their PCP (36.3%), and 2699 followed up in UC (53.1%). Table 1 presents predischarge characteristics of these patients. Patients seen in PDC were older and had a more significant comorbidity burden.
PDC, N=538 | UC, N=2699 | PCP, N=1848 | P Value | P Value After Propensity Adjustment | |
---|---|---|---|---|---|
| |||||
Age, years (SD) | 67.8 (12.6) | 67.1 (13.0) | 64.8 (13.0) | <0.01 | 0.86 |
Male sex, % | 95.0 | 95.4 | 94.4 | 0.33 | |
Marital status, % | |||||
Divorced | 40.2 | 36.2 | 35.0 | 0.09 | |
Married | 35.9 | 37.3 | 39.8 | 0.13 | |
Never married | 12.3 | 13.7 | 14.3 | 0.48 | |
LOS, days (SD) | 3.8 (3.6) | 5.0 (11.7) | 6.2 (10.8) | 0.04 | |
Elixhauser score (SD) | 0.80 (1.1) | 0.69 (1.0) | 0.75 (1.0) | 0.02 | 0.06 |
Admitted to ICU, % | 19.0 | 19.9 | 23.0 | 0.12 | |
ICU LOS, days (SD) | 2.8 (4.4) | 2.8 (3.4) | 2.3 (1.5) | 0.15 | |
Discharge medications, mean (SD) | 10.0 (6.7) | 10.4 (7.4) | 10.4 (8.2) | 0.37 | |
Admissions per patient in prior year, mean (SD) | 0.18 (0.5) | 0.21 (0.6) | 0.23 (0.6) | 0.08 | 0.78 |
Patients seen in PDC had a mean 2.4‐day shorter LOS than those seen by their PCPs (PDC: 3.8 days, UC: 5.0 days, PCP: 6.2 days; P=0.04 for comparison). Neither the percentage of patients admitted to the ICU during their index hospitalization nor the ICU LOS was different between groups. Patients were seen earlier postdischarge in PDC than in other types of follow‐up (PDC: 5.0 days, UC: 9.4 days, PCP: 13.7 days; P<0.01 for comparison). In univariate analysis, there was no difference between groups in the composite 30‐day outcome (Table 2). Analysis of the individual components of the primary outcome revealed significant differences in readmission rates, with PDC having the highest rate.
PDC | UC | PCP | P Value | P Value After Propensity Adjustment | |
---|---|---|---|---|---|
| |||||
Composite outcome, % | 19.9 | 18.3 | 17.5 | 0.42 | 0.30 |
Hospital readmission | 13.0 | 11.1 | 9.4 | 0.03 | 0.03 |
ED visit | 10.2 | 9.9 | 10.5 | 0.78 | 0.93 |
Mortality | 1.1 | 0.7 | 0.7 | 0.58 | 0.65 |
LOS if readmitted, days (SD) | 6.9 (18.1) | 4.9 (7.8) | 4.8 (6.5) | 0.28 | 0.23 |
Time to first visit after discharge, days (SD) | 5.0 (3.0) | 9.4 (6.1) | 13.8 (8.5) | <0.01 | <0.01 |
Univariate analyses conducted on predischarge characteristics after multinomial propensity scoring revealed significant differences between groups no longer existed for the variables that were included in the propensity score (age, Elixhauser score, and inpatient stays prior to visit; Table 1).
In multivariate analysis comparing PDC to PCP follow‐up, there was no difference in the composite outcome after controlling for propensity score and time to outpatient visit (odds ratio [OR]: 1.07, 95% confidence interval [CI]: 0.81‐1.40). Similar results were obtained in comparing PDC with UC (OR: 1.05, 95% CI: 0.82‐1.34) and in multinomial logistic regression comparing PDC with other types of follow‐up (PDC vs PCP: OR: 1.01, 95% CI: 0.78‐1.31; PDC vs UC: OR: 0.99, 95% CI: 0.78‐1.26).
Restricting the multivariate analysis to those patients discharged with one of the 5 discharge DRGs most associated with readmission did not alter our findings regarding the primary outcome. We also found no change in the composite outcome or any subcomponent of the composite outcome when restricting the analysis to 7‐day outcomes or when excluding scheduled readmissions (which represented <5% of all readmission).
DISCUSSION
A hospitalist‐run postdischarge clinic did not reduce a composite of 30‐day postdischarge adverse outcomes in our study when compared with primary‐care or urgent‐care follow‐up. In fact, patients who followed up in PDC had a small increase in 30‐day readmissions. However, they also were sicker at baseline, considered higher risk by the discharging physician, were able to be seen significantly earlier, and had an associated 2.4‐day shorter hospital LOS than patients seen by their PCPs.
Our findings do not confirm those of prior research in this area, which indicated outpatient follow‐up by the same physician who was the treating inpatient physician was linked to lower mortality rates, hospital readmissions, and ED utilization.[19, 20, 21] In fact, in our study, there was a significant (albeit small) increase in 30‐day readmissions in patients seen in PDC. There are significant challenges to the generalizability and validity of these prior studies. In one study, inpatient care was provided by outpatient primary‐care doctors in Canada,[19] a payer and care model rare in the United States.[25] In a second, usual care was not specified, and it is likely the reduction in ED visits resulted from provision of follow‐up care of any kind compared with those who did not follow up after discharge.[20] In a third, the PDC was part of a larger bundle of postdischarge interventions, and it only reduced ED visits when compared with patients who did not have follow‐up; rates of ED visits were similar in a comparison with PCP follow‐up.[21]
There are several possible explanations for the lack of improvement in 30‐day adverse outcomes with a hospitalist‐run PDC. First, although early access to early postdischarge care was improved and evidence suggests this is important in reducing readmissions,[11, 12] in populations similar to that studied, more postdischarge care has also been linked to increased readmissions.[22] This may be due to more frequent re‐evaluation of fragile, chronically ill patients, presenting more options for readmission. Second, the intervention currently only addresses some components of the Ideal Transition of Care[1] (Figure 1) and may benefit from an enhanced visit structure using a multidisciplinary approach. Third, the intervention took place in the context of a robust primary‐care system with a universal electronic medical record; the effects of improved access and continuity may be magnified in a system without these advantages. Fourth, there was a low readmission rate overall, and it is unclear how many of these readmissions were preventable. Finally, it may be that although the initial postdischarge care was adequate, readmissions occurred after the first visit, suggesting subsequent care during the 30 days postdischarge could have been improved.
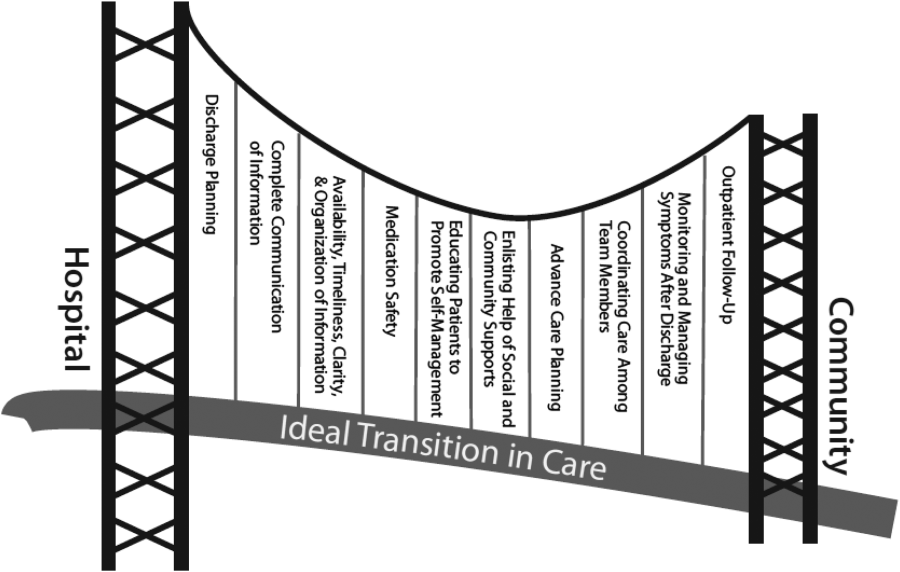
The most likely explanation for the substantially decreased LOS associated with follow‐up in PDC is that inpatient physicians who knew they could see their own patients early in the postdischarge process were more tolerant of uncertainty surrounding the patients' clinical course.
For example, a frequent clinical conundrum for hospitalists is when to discharge patients improving on diuretic therapy for a heart failure exacerbation or antibiotics for cellulitis. Provided a PDC, these hospitalists may choose to discharge a patient still actively being treated, because they may feel they have access to early follow‐up to change course if needed as well as the ability to see the patient themselves, allowing precise evaluation of the change in their condition. Without this clinic, the hospitalist may wonder when postdischarge follow‐up will occur. They may be more hesitant to discharge a patient who has not fully completed treatment for fear he or she will still appear decompensated to the postdischarge provider (though greatly improved from admission), or will not have timely‐enough follow‐up to change treatment if the condition worsens.
Our finding that the LOS was still shorter when comparing PDC with UC suggests continuity may be a significant component of this effect. It seems unlikely that patients following up in PDC had less complex hospitalizations given similar ICU exposure and LOS, as well as older age and larger baseline comorbidity burden.
The LOS seen in patients who followed up in PDC was lower than Medicare rates[26] but similar to reported rates at other VA acute‐care hospitals.[27] It is consistent with prior findings that hospitalist care reduces LOS,[26] though the magnitude in our study was much larger than that in prior reports. Prior studies have suggested this decreased LOS is linked to increased adverse postdischarge outcomes, such as ED visits and readmissions, as well as increased costs and decreased discharges to home.[28] The PDC was not associated with increased postdischarge adverse events measured, though a formal cost analysis and analysis of other postdischarge outcomes, such as placement in a skilled nursing or rehabilitation facility after return home, could be assessed in future work.
The findings of our study should be interpreted in the context of the study design. Our study was retrospective, observational, and single‐center. There may have been additional baseline differences between groups predisposing to bias we did not capture in the propensity score. For example, we could not measure rates of attendance at the different clinics and cannot rule out that outcomes associated with PDC were also associated with increased attendance rates. However, none of the clinics had mechanisms in place to improve follow‐up rates; patients referred to PDC were those considered highest risk for readmission and were sicker at baseline, making it very unlikely that they were predisposed to attend clinic more frequently and/or to have better outcomes; and even if PDC improved follow‐up rates, this would be a significant contribution given the limitations of primary‐care access. Our propensity score could not perfectly mimic randomization to a treatment assignment, but rather to treatment received, because of this limitation.
We did not ascertain ED visits or readmissions outside the VA system; it is possible these differentially affected one group more than another, though this seems unlikely. Our patient population was representative of veteran populations elsewhere who are at high risk of adverse postdischarge outcomes, but our findings may not be generalizable to younger, more ethnically diverse populations or to women.
CONCLUSIONS
Provision of postdischarge care by hospitalists may reduce LOS without increasing postdischarge adverse events. Further work is required to evaluate the role of hospitalist‐run PDCs in healthcare systems with more limited postdischarge access to care, to formally evaluate the costs associated with extending hospitalists to the outpatient setting, and to prospectively evaluate the role of a PDC compared with other kinds of hospital follow‐up.
Acknowledgments
The authors thank Melver Anderson, MD, for editorial assistance with the manuscript.
Disclosures: Dr. Burke had full access to all of the data in the study and takes responsibility for the integrity of the data and the accuracy of the data analysis. The views expressed in this article are those of the authors and do not necessarily represent the views of the Department of Veterans Affairs. Dr. Burke was supported by grant funding from the Colorado Research Enhancement Award Program to Improve Care Coordination for Veterans. Initial results of this study were presented at the Society of General Internal Medicine National Meeting in Denver, Colorado, April 24, 2013.
- Moving beyond readmission penalties: creating an ideal process to improve transitional care. J Hosp Med. 2013;8(2):102–109. , , , .
- Continuity of outpatient and inpatient care by primary care physicians for hospitalized older adults. JAMA. 2009;301(16):1671–1680. , , , , , .
- Trends in inpatient continuity of care for a cohort of Medicare patients 1996–2006. J Hosp Med. 2011;6(8):438–444. , , , , .
- Deficits in communication and information transfer between hospital‐based and primary care physicians: implications for patient safety and continuity of care. JAMA. 2007;297(8):831–841. , , , , , .
- Posthospital care transitions: patterns, complications, and risk identification. Health Serv Res. 2004;39(5):1449–1465. , , , .
- Patient experiences of transitioning from hospital to home: an ethnographic quality improvement project. J Hosp Med. 2012;7(5):382–387. , , , , .
- Understanding and execution of discharge instructions. Am J Med Qual. 2013;28(5):383–391. , , , et al.
- The incidence and severity of adverse events affecting patients after discharge from the hospital. Ann Intern Med. 2003;138(3):161–167. , , , , .
- Rehospitalizations among patients in the Medicare fee‐for‐service program. N Engl J Med. 2009;360(14):1418–1428. , , .
- Post‐hospital syndrome—an acquired, transient condition of generalized risk. N Engl J Med. 2013;368(2):100–102. .
- Relationship between early physician follow‐up and 30‐day readmission among Medicare beneficiaries hospitalized for heart failure. JAMA. 2010;303(17):1716–1722. , , , et al.
- Post‐hospitalization transitions: examining the effects of timing of primary care provider follow‐up. J Hosp Med. 2010;5(7):392–397. , , .
- Will generalist physician supply meet demands of an increasing and aging population? Health Aff (Millwood). 2008;27(3):w232–w241. , , .
- Association of American Medical Colleges. The Impact of Health Care Reform on the Future Supply and Demand for Physicians: Updated Projections Through 2025. Available at: http://www.aamc.org/download/158076/data/updated_projections_through_2025.pdf. Published June 2010. Accessed May 1, 2012.
- Effect of discharge summary availability during post‐discharge visits on hospital readmission. J Gen Intern Med. 2002;17(3):186–192. , , , .
- Comprehensive quality of discharge summaries at an academic medical center. J Hosp Med. 2013;8(8):436–443. , , , et al.
- Association of communication between hospital‐based physicians and primary care providers with patient outcomes. J Gen Intern Med. 2009;24(3):381–386. , , , et al.
- Is a post‐discharge clinic in your hospital's future? Available at: http://www.the‐hospitalist.org/details/article/1409011/Is_a_Post‐Discharge_Clinic_in_Your_Hospitals_Future.html. Published December 2011. Accessed May 1, 2013. .
- Continuity of care and patient outcomes after hospital discharge. J Gen Intern Med. 2004;19(6):624–631. , , , .
- Effects of a postdischarge clinic on housestaff satisfaction and utilization of hospital services. J Gen Intern Med. 1996;11(3):179–181. , , , .
- Integrated postdischarge transitional care in a hospitalist system to improve discharge outcome: an experimental study. BMC Med. 2011;9:96. , , , , , .
- Does increased access to primary care reduce hospital readmissions? Veterans Affairs Cooperative Study Group on Primary Care and Hospital Readmission. N Engl J Med. 1996;334(22):1441–1447. , , .
- An overview of the objectives of and the approaches to propensity score analyses. Eur Heart J. 2011;32(14):1704–1708. , .
- Comparison of the Elixhauser and Charlson/Deyo methods of comorbidity measurement in administrative data. Med Care. 2004;42(4):355–360. , , .
- Growth in the care of older patients by hospitalists in the United States. N Engl J Med. 2009;360(11):1102–1112. , , , .
- Association of hospitalist care with medical utilization after discharge: evidence of cost shift from a cohort study. Ann Intern Med. 2011;155(3):152–159. , .
- Associations between reduced hospital length of stay and 30‐day readmission rate and mortality: 14‐year experience in 129 Veterans Affairs hospitals. Ann Intern Med. 2012;157(12):837–845. , , , et al.
- Variation in length of stay and outcomes among hospitalized patients attributable to hospitals and hospitalists. J Gen Intern Med. 2013;28(3):370–376. , , , .
- Moving beyond readmission penalties: creating an ideal process to improve transitional care. J Hosp Med. 2013;8(2):102–109. , , , .
- Continuity of outpatient and inpatient care by primary care physicians for hospitalized older adults. JAMA. 2009;301(16):1671–1680. , , , , , .
- Trends in inpatient continuity of care for a cohort of Medicare patients 1996–2006. J Hosp Med. 2011;6(8):438–444. , , , , .
- Deficits in communication and information transfer between hospital‐based and primary care physicians: implications for patient safety and continuity of care. JAMA. 2007;297(8):831–841. , , , , , .
- Posthospital care transitions: patterns, complications, and risk identification. Health Serv Res. 2004;39(5):1449–1465. , , , .
- Patient experiences of transitioning from hospital to home: an ethnographic quality improvement project. J Hosp Med. 2012;7(5):382–387. , , , , .
- Understanding and execution of discharge instructions. Am J Med Qual. 2013;28(5):383–391. , , , et al.
- The incidence and severity of adverse events affecting patients after discharge from the hospital. Ann Intern Med. 2003;138(3):161–167. , , , , .
- Rehospitalizations among patients in the Medicare fee‐for‐service program. N Engl J Med. 2009;360(14):1418–1428. , , .
- Post‐hospital syndrome—an acquired, transient condition of generalized risk. N Engl J Med. 2013;368(2):100–102. .
- Relationship between early physician follow‐up and 30‐day readmission among Medicare beneficiaries hospitalized for heart failure. JAMA. 2010;303(17):1716–1722. , , , et al.
- Post‐hospitalization transitions: examining the effects of timing of primary care provider follow‐up. J Hosp Med. 2010;5(7):392–397. , , .
- Will generalist physician supply meet demands of an increasing and aging population? Health Aff (Millwood). 2008;27(3):w232–w241. , , .
- Association of American Medical Colleges. The Impact of Health Care Reform on the Future Supply and Demand for Physicians: Updated Projections Through 2025. Available at: http://www.aamc.org/download/158076/data/updated_projections_through_2025.pdf. Published June 2010. Accessed May 1, 2012.
- Effect of discharge summary availability during post‐discharge visits on hospital readmission. J Gen Intern Med. 2002;17(3):186–192. , , , .
- Comprehensive quality of discharge summaries at an academic medical center. J Hosp Med. 2013;8(8):436–443. , , , et al.
- Association of communication between hospital‐based physicians and primary care providers with patient outcomes. J Gen Intern Med. 2009;24(3):381–386. , , , et al.
- Is a post‐discharge clinic in your hospital's future? Available at: http://www.the‐hospitalist.org/details/article/1409011/Is_a_Post‐Discharge_Clinic_in_Your_Hospitals_Future.html. Published December 2011. Accessed May 1, 2013. .
- Continuity of care and patient outcomes after hospital discharge. J Gen Intern Med. 2004;19(6):624–631. , , , .
- Effects of a postdischarge clinic on housestaff satisfaction and utilization of hospital services. J Gen Intern Med. 1996;11(3):179–181. , , , .
- Integrated postdischarge transitional care in a hospitalist system to improve discharge outcome: an experimental study. BMC Med. 2011;9:96. , , , , , .
- Does increased access to primary care reduce hospital readmissions? Veterans Affairs Cooperative Study Group on Primary Care and Hospital Readmission. N Engl J Med. 1996;334(22):1441–1447. , , .
- An overview of the objectives of and the approaches to propensity score analyses. Eur Heart J. 2011;32(14):1704–1708. , .
- Comparison of the Elixhauser and Charlson/Deyo methods of comorbidity measurement in administrative data. Med Care. 2004;42(4):355–360. , , .
- Growth in the care of older patients by hospitalists in the United States. N Engl J Med. 2009;360(11):1102–1112. , , , .
- Association of hospitalist care with medical utilization after discharge: evidence of cost shift from a cohort study. Ann Intern Med. 2011;155(3):152–159. , .
- Associations between reduced hospital length of stay and 30‐day readmission rate and mortality: 14‐year experience in 129 Veterans Affairs hospitals. Ann Intern Med. 2012;157(12):837–845. , , , et al.
- Variation in length of stay and outcomes among hospitalized patients attributable to hospitals and hospitalists. J Gen Intern Med. 2013;28(3):370–376. , , , .
© 2013 Society of Hospital Medicine
Postdischarge Clinics
Transitions of care, which encompass the patient experience of hospital discharge to the community, are frequently associated with clinically and financially costly adverse events.[1, 2] One important element for reducing the risk of postdischarge adverse events is provision of timely follow‐up by a clinician familiar with the patient and hospital course.[3, 4]
However, achieving this ideal is becoming more difficult because of an increased demand for primary care services (due to expanding coverage of Medicare and Medicaid) and the decreased supply of primary care physicians.[5, 6] When a timely visit with a clinician is available postdischarge, the widening discontinuity between inpatient and outpatient care providers often means this clinician is lacking essential details of the hospitalization.[7, 8]
One increasingly common innovation to improve postdischarge care access and continuity is to extend the role of inpatient providers (usually hospitalists) to provide care after discharge in a postdischarge clinic (PDC).[9, 10, 11] These clinics require an expansion of a hospitalist's duties to the outpatient setting, a requirement that has met with hospitalist resistance in initial reports.[12] However, little is known about hospitalists' experience with PDCs or attitudes toward postdischarge care. We aimed to explore these attitudes and experiences surrounding postdischarge care and PDCs.
METHODS
We conducted a cross‐sectional 17‐question Web‐based survey of hospitalists at 20 academic and 17 VA medical centers across the United States. Hospital medicine faculty at each site were identified by their group leader; members of each group then received an email survey up to 3 times. To collect responses from nonacademic hospitalists, the survey was also distributed to a large national private hospitalist employer. Due to internal limitations at the employer site, sampling was not feasible, and thus a convenience sample was obtained. Hospitalists who were not clinically active or did not have computer access to complete the survey were excluded. Responses were initially gathered on a 4‐point Likert scale; for comparisons between groups the scale was collapsed to a binary comparison using Fisher exact or 2 tests. We included questions answered in partially completed surveys in both the numerator and denominator; questions not answered were excluded from both numerator and denominator. The denominator of all responses was noted. All analyses were conducted using SAS 9.3 (SAS Institute, Inc., Cary, NC). The study was approved by the Colorado Multiple Institutional Review Board.
RESULTS
Of 814 hospitalists, 228 responded to the survey (28.3%). Table 1 illustrates characteristics of responding hospitalists, who were divided between university hospitals, community teaching hospitals, and community nonteaching hospitals in diverse practices in terms of location and group size.
Characteristic | Respondents, No. (%) |
---|---|
| |
Employing institution | |
University hospital | 79 (37.4) |
Community, nonteaching | 62 (29.4) |
Community, teaching | 70 (33.2) |
Care environment | |
Hospitalist providers | 98 (46.4) |
Housestaff providers | 94 (44.6) |
Primary care providers | 10 (4.7) |
Combination | 9 (4.3) |
Hospitalist group size (number of hospitalists) | |
15 | 39 (18.5) |
610 | 50 (23.7) |
1120 | 52 (24.6) |
2150 | 59 (28.0) |
>50 | 9 (4.3) |
Hospital locationpopulation | |
Rural | 20 (9.5) |
Suburban | 47 (22.3) |
Urban | 144 (68.2) |
Hospital locationgeographic | |
West Coast | 11 (5.2) |
Midwest | 47 (22.3) |
Southern | 57 (27.1) |
East Coast | 21 (10) |
Southwest | 36 (17.1) |
Mountain | 32 (15.2) |
Sixty‐one percent of responding hospitalists believed most patient problems after discharge were due to poor follow‐up with primary care providers, and 55% found it difficult to arrange timely primary care follow up (Table 2). Despite this, 87% thought patient problems after discharge should be cared for by primary care physicians, and 62% opposed the idea of hospitalists seeing patients in the clinic after discharge.
Agree, No. (%) | Disagree, No. (%) | |
---|---|---|
| ||
Hospitalists should see patients in clinic after discharge | 87 (38.2) | 141 (61.8) |
Primary care responsible for problems after discharge | 198 (86.8) | 30 (13.2) |
Hospitalists responsible for patients after discharge | 113 (49.6) | 115 (50.4) |
Would welcome a PDC if employer required | 113 (49.6) | 115 (50.4) |
Would require extra compensation to work in a PDC | 175 (76.8) | 53 (23.2) |
Believe a PDC would reduce ED visits after discharge | 168 (73.7) | 60 (26.3) |
Would discharge patients earlier if could see after discharge | 116 (50.9) | 112 (49.1) |
Most postdischarge problems due to poor PCP access | 138 (60.5) | 90 (39.5) |
Easy to arrange timely follow‐up with patient's PCP | 100 (44.2) | 126 (55.3) |
When asked if hospitalists were responsible for patients after discharge from the hospital, only 50% responded positively. However, when asked how long hospitalists were responsible for patients after discharge, 71% gave a response longer than hospital discharge, including 60% who believed this responsibility ended at 1 week or less following discharge. A minority (12%) felt it extended to 1 month following discharge (Table 3).
Respondents, No. (%) | |
---|---|
| |
Length of time inpatient providers responsible after discharge | |
Responsibility ends at time of discharge | 65 (28.5) |
13 days | 40 (17.5) |
47 days | 57 (25.0) |
2 weeks | 41 (18.0) |
4 weeks | 20 (8.8) |
3 months | 3 (1.3) |
3 months | 2 (0.9) |
Postdischarge clinic present | 20 (8.8) |
Considered starting a postdischarge clinica | 62 (30.5) |
Starting in next yearb | 6 (3.3) |
Are satisfied with experience in postdischarge clinicc | 17 (85) |
Think patients are satisfied/highly satisfiedc | 14 (70) |
Responding hospitalists expressed confidence in a PDC to reduce postdischarge emergency department visits (74%). However, most felt they would require extra compensation to staff a PDC (77%). They were divided on whether they would discharge patients from the hospital earlier if they could see those patients in postdischarge follow‐up (51% would discharge patients earlier).
Compared to those who had not experienced a PDC, responding hospitalists who had provided care in a PDC trended toward responding more positively that hospitalists should provide postdischarge care (P = 0.054). Few responding hospitalists had such exposure (8.8%) at the time of the survey. Although 31% had considering starting a PDC, only 3% were starting in the next year. Of responding hospitalists with exposure to a PDC, 70% were satisfied with the experience and 85% felt their patients were satisfied. Responses did not vary by type of practice (academic vs nonacademic), group size, geographic location, or by exposure to a PDC except as above.
DISCUSSION
Responding hospitalists reported encountering significant difficulty arranging appropriate postdischarge appointments with primary care providers and feel this contributes to postdischarge complications. Nearly 75% of those surveyed felt a hospitalist‐run PDC would be effective in reducing postdischarge emergency department visits, presumably in part due to improved access to postdischarge care. However, 62% of responding hospitalists opposed providing this type of care, though those who had experienced a PDC were somewhat more likely to view providing care in a PDC favorably. Survey responses largely reflect attitudes rather than experience with PDCs, because very few respondents had ever worked in a PDC.
The juxtaposition of the confidence expressed in PDCs to reduce postdischarge emergency department visits with the less enthusiastic views of respondents about providing care in a PDC was surprising. Several explanations are possible. First, providing such care is outside the usual scope of practice of most hospitalists, and preliminary reports indicate hospitalists, as self‐selected inpatient providers, may not initially welcome this opportunity.[12] Second, responding hospitalists identified the need for extra compensation for providing this care, suggesting they would see staffing a PDC as a burden requiring extra payment. Third, only 12% of respondents felt their responsibility to their discharged patients extended to 1 month following discharge. Given this, hospitalists may not feel enough personal ownership over 30‐day readmission rates to justify the additional clinical demand of staffing a PDC.[13]
In fact, 29% of responding hospitalists felt their responsibility to the patient ended at the time of discharge. Respondents may have interpreted responsibility differently, and we cannot rule out response bias given our lower‐than‐expected response rate. However, we had anticipated many fewer hospitalists would respond this way given professional hospitalist societies have endorsed guidelines for improved transitions of care, which clearly delineate the key role hospitalists play in care transitions.[14]
Although fewer than 10% of respondents had worked in a PDC, nearly one‐third reported considering starting such a practice in the future, underscoring the importance of understanding hospitalist attitudes and experiences when creating a PDC and the significant barriers to arranging appropriate postdischarge care identified by survey respondents. The barriers to establishing a PDC may explain why few planned to start a PDC in the next year.
This study should be interpreted in the context of its design. Due to limitations in survey delivery, more rigorous sampling designs could not be used, and efforts were instead made to deliver the survey to a diverse group of hospitalists. The survey response rate was lower than anticipated and this increases the risk of response bias. Though this response rate is characteristic of other surveys of hospitalists, responses may have been from a selected population and therefore not representative of all hospitalists.[15] We sampled from a variety of practice venues, locations, academic and community practices, and practice group sizes to try to minimize this bias. Due to the low exposure rate to PDCs, hospitalist responses to experiences with PDCs should be considered exploratory.
We asked about similar content areas in the survey in multiple questions to maximize content validity; this resulted in variations in the degree of agreement or disagreement to similar prompts. For example, 62% of hospitalists opposed seeing patients in the clinic after discharge when directly asked, but nearly 50% said they would welcome the opportunity to work in a PDC if their employer required it. In another example, 50% of respondents said their responsibility for the patient ended at time of discharge, but when asked about duration of responsibility, 30% identified time of discharge as the limit. When reporting and interpreting results, we have tried to highlight responses to questions that ask most clearly and directly about the content of interest (rather than general themes), but this interpretation may also be subject to bias.
The time after hospital discharge is one of heightened risk for adverse events for the recently discharged patient. Hospitalist‐run postdischarge clinics may offer improved postdischarge care access and continuity; more research is needed on the effects of such clinics on patient outcomes, including postdischarge utilization. Until then, physicians and hospitals considering establishing PDCs should consider the barriers responding hospitalists identified to working in such a clinic, as well as the confidence they expressed in PDCs to reduce subsequent utilization.
Disclosures: Dr. Burke had full access to all of the data in the study and takes responsibility for the integrity of the data and the accuracy of the data analysis. The views expressed in this article are those of the authors and do not necessarily represent the views of the Department of Veterans Affairs. Dr. Burke was supported by grant funding from the Colorado Research Enhancement Award Program to Improve Care Coordination for Veterans. Dr. Ryan has no conflicts of interest to disclose.
- The incidence and severity of adverse events affecting patients after discharge from the hospital. Ann Intern Med. 2003;138(3):161–167. , , , , .
- Rehospitalizations among patients in the Medicare fee‐for‐service program. N Engl J Med. 2009;360(14):1418–1428. , , .
- Relationship between early physician follow‐up and 30‐day readmission among Medicare beneficiaries hospitalized for heart failure. JAMA. 2010;303(17):1716–1722. , , , et al.
- Post‐hospitalization transitions: Examining the effects of timing of primary care provider follow‐up. J Hosp Med. 2010;5(7):392–397. , , .
- Will generalist physician supply meet demands of an increasing and aging population? Health Aff (Millwood). 2008;27(3):w232–w241. , , .
- Association of American Medical Colleges. June 2010.The impact of health care reform on the future supply and demand for physicians updated projections through 2025. Available at: http://www.aamc.org/download/158076/data/updated_projections_through_2025.pdf. Accessed May 1, 2012.
- Continuity of outpatient and inpatient care by primary care physicians for hospitalized older adults. JAMA. 2009;301(16):1671–1680. , , , , , .
- Trends in inpatient continuity of care for a cohort of Medicare patients 1996–2006. J Hosp Med. 2011;6(8):438–444. , , , , .
- Is a post‐discharge clinic in your hospital's future? The Hospitalist. December 2011 Available at: http://www.the‐hospitalist.org/details/article/1409011/Is_a_Post_Discharge_Clinic_in_Your_Hospitals_future.html. Accessed May 1, 2013. .
- Continuity of care and patient outcomes after hospital discharge. J Gen Intern Med. 2004;19(6):624–631. , , , .
- Effects of a postdischarge clinic on housestaff satisfaction and utilization of hospital services. J Gen Intern Med. 1996;11(3):179–181. , , , .
- Interval examination: establishment of a hospitalist‐staffed discharge clinic. J Gen Intern Med. 2012;27(10):1377–1382. .
- Patient Protection and Affordable Care Act (PPACA).Public Law 111–148 2010. Available at: http://www.gpo.gov/fdsys/pkg/PLAW‐111publ148/pdf/PLAW‐111publ148.pdf. Accessed January 10, 2013.
- Transitions of Care Consensus policy statement: American College of Physicians, Society of General Internal Medicine, Society of Hospital Medicine, American Geriatrics Society, American College of Emergency Physicians, and Society for Academic Emergency Medicine. J Hosp Med. 2009;4(6):364–370. , , , et al.
- Person‐job fit: an exploratory cross‐sectional analysis of hospitalists. J Hosp Med. 2013;8(2):96–101. , , , , .
Transitions of care, which encompass the patient experience of hospital discharge to the community, are frequently associated with clinically and financially costly adverse events.[1, 2] One important element for reducing the risk of postdischarge adverse events is provision of timely follow‐up by a clinician familiar with the patient and hospital course.[3, 4]
However, achieving this ideal is becoming more difficult because of an increased demand for primary care services (due to expanding coverage of Medicare and Medicaid) and the decreased supply of primary care physicians.[5, 6] When a timely visit with a clinician is available postdischarge, the widening discontinuity between inpatient and outpatient care providers often means this clinician is lacking essential details of the hospitalization.[7, 8]
One increasingly common innovation to improve postdischarge care access and continuity is to extend the role of inpatient providers (usually hospitalists) to provide care after discharge in a postdischarge clinic (PDC).[9, 10, 11] These clinics require an expansion of a hospitalist's duties to the outpatient setting, a requirement that has met with hospitalist resistance in initial reports.[12] However, little is known about hospitalists' experience with PDCs or attitudes toward postdischarge care. We aimed to explore these attitudes and experiences surrounding postdischarge care and PDCs.
METHODS
We conducted a cross‐sectional 17‐question Web‐based survey of hospitalists at 20 academic and 17 VA medical centers across the United States. Hospital medicine faculty at each site were identified by their group leader; members of each group then received an email survey up to 3 times. To collect responses from nonacademic hospitalists, the survey was also distributed to a large national private hospitalist employer. Due to internal limitations at the employer site, sampling was not feasible, and thus a convenience sample was obtained. Hospitalists who were not clinically active or did not have computer access to complete the survey were excluded. Responses were initially gathered on a 4‐point Likert scale; for comparisons between groups the scale was collapsed to a binary comparison using Fisher exact or 2 tests. We included questions answered in partially completed surveys in both the numerator and denominator; questions not answered were excluded from both numerator and denominator. The denominator of all responses was noted. All analyses were conducted using SAS 9.3 (SAS Institute, Inc., Cary, NC). The study was approved by the Colorado Multiple Institutional Review Board.
RESULTS
Of 814 hospitalists, 228 responded to the survey (28.3%). Table 1 illustrates characteristics of responding hospitalists, who were divided between university hospitals, community teaching hospitals, and community nonteaching hospitals in diverse practices in terms of location and group size.
Characteristic | Respondents, No. (%) |
---|---|
| |
Employing institution | |
University hospital | 79 (37.4) |
Community, nonteaching | 62 (29.4) |
Community, teaching | 70 (33.2) |
Care environment | |
Hospitalist providers | 98 (46.4) |
Housestaff providers | 94 (44.6) |
Primary care providers | 10 (4.7) |
Combination | 9 (4.3) |
Hospitalist group size (number of hospitalists) | |
15 | 39 (18.5) |
610 | 50 (23.7) |
1120 | 52 (24.6) |
2150 | 59 (28.0) |
>50 | 9 (4.3) |
Hospital locationpopulation | |
Rural | 20 (9.5) |
Suburban | 47 (22.3) |
Urban | 144 (68.2) |
Hospital locationgeographic | |
West Coast | 11 (5.2) |
Midwest | 47 (22.3) |
Southern | 57 (27.1) |
East Coast | 21 (10) |
Southwest | 36 (17.1) |
Mountain | 32 (15.2) |
Sixty‐one percent of responding hospitalists believed most patient problems after discharge were due to poor follow‐up with primary care providers, and 55% found it difficult to arrange timely primary care follow up (Table 2). Despite this, 87% thought patient problems after discharge should be cared for by primary care physicians, and 62% opposed the idea of hospitalists seeing patients in the clinic after discharge.
Agree, No. (%) | Disagree, No. (%) | |
---|---|---|
| ||
Hospitalists should see patients in clinic after discharge | 87 (38.2) | 141 (61.8) |
Primary care responsible for problems after discharge | 198 (86.8) | 30 (13.2) |
Hospitalists responsible for patients after discharge | 113 (49.6) | 115 (50.4) |
Would welcome a PDC if employer required | 113 (49.6) | 115 (50.4) |
Would require extra compensation to work in a PDC | 175 (76.8) | 53 (23.2) |
Believe a PDC would reduce ED visits after discharge | 168 (73.7) | 60 (26.3) |
Would discharge patients earlier if could see after discharge | 116 (50.9) | 112 (49.1) |
Most postdischarge problems due to poor PCP access | 138 (60.5) | 90 (39.5) |
Easy to arrange timely follow‐up with patient's PCP | 100 (44.2) | 126 (55.3) |
When asked if hospitalists were responsible for patients after discharge from the hospital, only 50% responded positively. However, when asked how long hospitalists were responsible for patients after discharge, 71% gave a response longer than hospital discharge, including 60% who believed this responsibility ended at 1 week or less following discharge. A minority (12%) felt it extended to 1 month following discharge (Table 3).
Respondents, No. (%) | |
---|---|
| |
Length of time inpatient providers responsible after discharge | |
Responsibility ends at time of discharge | 65 (28.5) |
13 days | 40 (17.5) |
47 days | 57 (25.0) |
2 weeks | 41 (18.0) |
4 weeks | 20 (8.8) |
3 months | 3 (1.3) |
3 months | 2 (0.9) |
Postdischarge clinic present | 20 (8.8) |
Considered starting a postdischarge clinica | 62 (30.5) |
Starting in next yearb | 6 (3.3) |
Are satisfied with experience in postdischarge clinicc | 17 (85) |
Think patients are satisfied/highly satisfiedc | 14 (70) |
Responding hospitalists expressed confidence in a PDC to reduce postdischarge emergency department visits (74%). However, most felt they would require extra compensation to staff a PDC (77%). They were divided on whether they would discharge patients from the hospital earlier if they could see those patients in postdischarge follow‐up (51% would discharge patients earlier).
Compared to those who had not experienced a PDC, responding hospitalists who had provided care in a PDC trended toward responding more positively that hospitalists should provide postdischarge care (P = 0.054). Few responding hospitalists had such exposure (8.8%) at the time of the survey. Although 31% had considering starting a PDC, only 3% were starting in the next year. Of responding hospitalists with exposure to a PDC, 70% were satisfied with the experience and 85% felt their patients were satisfied. Responses did not vary by type of practice (academic vs nonacademic), group size, geographic location, or by exposure to a PDC except as above.
DISCUSSION
Responding hospitalists reported encountering significant difficulty arranging appropriate postdischarge appointments with primary care providers and feel this contributes to postdischarge complications. Nearly 75% of those surveyed felt a hospitalist‐run PDC would be effective in reducing postdischarge emergency department visits, presumably in part due to improved access to postdischarge care. However, 62% of responding hospitalists opposed providing this type of care, though those who had experienced a PDC were somewhat more likely to view providing care in a PDC favorably. Survey responses largely reflect attitudes rather than experience with PDCs, because very few respondents had ever worked in a PDC.
The juxtaposition of the confidence expressed in PDCs to reduce postdischarge emergency department visits with the less enthusiastic views of respondents about providing care in a PDC was surprising. Several explanations are possible. First, providing such care is outside the usual scope of practice of most hospitalists, and preliminary reports indicate hospitalists, as self‐selected inpatient providers, may not initially welcome this opportunity.[12] Second, responding hospitalists identified the need for extra compensation for providing this care, suggesting they would see staffing a PDC as a burden requiring extra payment. Third, only 12% of respondents felt their responsibility to their discharged patients extended to 1 month following discharge. Given this, hospitalists may not feel enough personal ownership over 30‐day readmission rates to justify the additional clinical demand of staffing a PDC.[13]
In fact, 29% of responding hospitalists felt their responsibility to the patient ended at the time of discharge. Respondents may have interpreted responsibility differently, and we cannot rule out response bias given our lower‐than‐expected response rate. However, we had anticipated many fewer hospitalists would respond this way given professional hospitalist societies have endorsed guidelines for improved transitions of care, which clearly delineate the key role hospitalists play in care transitions.[14]
Although fewer than 10% of respondents had worked in a PDC, nearly one‐third reported considering starting such a practice in the future, underscoring the importance of understanding hospitalist attitudes and experiences when creating a PDC and the significant barriers to arranging appropriate postdischarge care identified by survey respondents. The barriers to establishing a PDC may explain why few planned to start a PDC in the next year.
This study should be interpreted in the context of its design. Due to limitations in survey delivery, more rigorous sampling designs could not be used, and efforts were instead made to deliver the survey to a diverse group of hospitalists. The survey response rate was lower than anticipated and this increases the risk of response bias. Though this response rate is characteristic of other surveys of hospitalists, responses may have been from a selected population and therefore not representative of all hospitalists.[15] We sampled from a variety of practice venues, locations, academic and community practices, and practice group sizes to try to minimize this bias. Due to the low exposure rate to PDCs, hospitalist responses to experiences with PDCs should be considered exploratory.
We asked about similar content areas in the survey in multiple questions to maximize content validity; this resulted in variations in the degree of agreement or disagreement to similar prompts. For example, 62% of hospitalists opposed seeing patients in the clinic after discharge when directly asked, but nearly 50% said they would welcome the opportunity to work in a PDC if their employer required it. In another example, 50% of respondents said their responsibility for the patient ended at time of discharge, but when asked about duration of responsibility, 30% identified time of discharge as the limit. When reporting and interpreting results, we have tried to highlight responses to questions that ask most clearly and directly about the content of interest (rather than general themes), but this interpretation may also be subject to bias.
The time after hospital discharge is one of heightened risk for adverse events for the recently discharged patient. Hospitalist‐run postdischarge clinics may offer improved postdischarge care access and continuity; more research is needed on the effects of such clinics on patient outcomes, including postdischarge utilization. Until then, physicians and hospitals considering establishing PDCs should consider the barriers responding hospitalists identified to working in such a clinic, as well as the confidence they expressed in PDCs to reduce subsequent utilization.
Disclosures: Dr. Burke had full access to all of the data in the study and takes responsibility for the integrity of the data and the accuracy of the data analysis. The views expressed in this article are those of the authors and do not necessarily represent the views of the Department of Veterans Affairs. Dr. Burke was supported by grant funding from the Colorado Research Enhancement Award Program to Improve Care Coordination for Veterans. Dr. Ryan has no conflicts of interest to disclose.
Transitions of care, which encompass the patient experience of hospital discharge to the community, are frequently associated with clinically and financially costly adverse events.[1, 2] One important element for reducing the risk of postdischarge adverse events is provision of timely follow‐up by a clinician familiar with the patient and hospital course.[3, 4]
However, achieving this ideal is becoming more difficult because of an increased demand for primary care services (due to expanding coverage of Medicare and Medicaid) and the decreased supply of primary care physicians.[5, 6] When a timely visit with a clinician is available postdischarge, the widening discontinuity between inpatient and outpatient care providers often means this clinician is lacking essential details of the hospitalization.[7, 8]
One increasingly common innovation to improve postdischarge care access and continuity is to extend the role of inpatient providers (usually hospitalists) to provide care after discharge in a postdischarge clinic (PDC).[9, 10, 11] These clinics require an expansion of a hospitalist's duties to the outpatient setting, a requirement that has met with hospitalist resistance in initial reports.[12] However, little is known about hospitalists' experience with PDCs or attitudes toward postdischarge care. We aimed to explore these attitudes and experiences surrounding postdischarge care and PDCs.
METHODS
We conducted a cross‐sectional 17‐question Web‐based survey of hospitalists at 20 academic and 17 VA medical centers across the United States. Hospital medicine faculty at each site were identified by their group leader; members of each group then received an email survey up to 3 times. To collect responses from nonacademic hospitalists, the survey was also distributed to a large national private hospitalist employer. Due to internal limitations at the employer site, sampling was not feasible, and thus a convenience sample was obtained. Hospitalists who were not clinically active or did not have computer access to complete the survey were excluded. Responses were initially gathered on a 4‐point Likert scale; for comparisons between groups the scale was collapsed to a binary comparison using Fisher exact or 2 tests. We included questions answered in partially completed surveys in both the numerator and denominator; questions not answered were excluded from both numerator and denominator. The denominator of all responses was noted. All analyses were conducted using SAS 9.3 (SAS Institute, Inc., Cary, NC). The study was approved by the Colorado Multiple Institutional Review Board.
RESULTS
Of 814 hospitalists, 228 responded to the survey (28.3%). Table 1 illustrates characteristics of responding hospitalists, who were divided between university hospitals, community teaching hospitals, and community nonteaching hospitals in diverse practices in terms of location and group size.
Characteristic | Respondents, No. (%) |
---|---|
| |
Employing institution | |
University hospital | 79 (37.4) |
Community, nonteaching | 62 (29.4) |
Community, teaching | 70 (33.2) |
Care environment | |
Hospitalist providers | 98 (46.4) |
Housestaff providers | 94 (44.6) |
Primary care providers | 10 (4.7) |
Combination | 9 (4.3) |
Hospitalist group size (number of hospitalists) | |
15 | 39 (18.5) |
610 | 50 (23.7) |
1120 | 52 (24.6) |
2150 | 59 (28.0) |
>50 | 9 (4.3) |
Hospital locationpopulation | |
Rural | 20 (9.5) |
Suburban | 47 (22.3) |
Urban | 144 (68.2) |
Hospital locationgeographic | |
West Coast | 11 (5.2) |
Midwest | 47 (22.3) |
Southern | 57 (27.1) |
East Coast | 21 (10) |
Southwest | 36 (17.1) |
Mountain | 32 (15.2) |
Sixty‐one percent of responding hospitalists believed most patient problems after discharge were due to poor follow‐up with primary care providers, and 55% found it difficult to arrange timely primary care follow up (Table 2). Despite this, 87% thought patient problems after discharge should be cared for by primary care physicians, and 62% opposed the idea of hospitalists seeing patients in the clinic after discharge.
Agree, No. (%) | Disagree, No. (%) | |
---|---|---|
| ||
Hospitalists should see patients in clinic after discharge | 87 (38.2) | 141 (61.8) |
Primary care responsible for problems after discharge | 198 (86.8) | 30 (13.2) |
Hospitalists responsible for patients after discharge | 113 (49.6) | 115 (50.4) |
Would welcome a PDC if employer required | 113 (49.6) | 115 (50.4) |
Would require extra compensation to work in a PDC | 175 (76.8) | 53 (23.2) |
Believe a PDC would reduce ED visits after discharge | 168 (73.7) | 60 (26.3) |
Would discharge patients earlier if could see after discharge | 116 (50.9) | 112 (49.1) |
Most postdischarge problems due to poor PCP access | 138 (60.5) | 90 (39.5) |
Easy to arrange timely follow‐up with patient's PCP | 100 (44.2) | 126 (55.3) |
When asked if hospitalists were responsible for patients after discharge from the hospital, only 50% responded positively. However, when asked how long hospitalists were responsible for patients after discharge, 71% gave a response longer than hospital discharge, including 60% who believed this responsibility ended at 1 week or less following discharge. A minority (12%) felt it extended to 1 month following discharge (Table 3).
Respondents, No. (%) | |
---|---|
| |
Length of time inpatient providers responsible after discharge | |
Responsibility ends at time of discharge | 65 (28.5) |
13 days | 40 (17.5) |
47 days | 57 (25.0) |
2 weeks | 41 (18.0) |
4 weeks | 20 (8.8) |
3 months | 3 (1.3) |
3 months | 2 (0.9) |
Postdischarge clinic present | 20 (8.8) |
Considered starting a postdischarge clinica | 62 (30.5) |
Starting in next yearb | 6 (3.3) |
Are satisfied with experience in postdischarge clinicc | 17 (85) |
Think patients are satisfied/highly satisfiedc | 14 (70) |
Responding hospitalists expressed confidence in a PDC to reduce postdischarge emergency department visits (74%). However, most felt they would require extra compensation to staff a PDC (77%). They were divided on whether they would discharge patients from the hospital earlier if they could see those patients in postdischarge follow‐up (51% would discharge patients earlier).
Compared to those who had not experienced a PDC, responding hospitalists who had provided care in a PDC trended toward responding more positively that hospitalists should provide postdischarge care (P = 0.054). Few responding hospitalists had such exposure (8.8%) at the time of the survey. Although 31% had considering starting a PDC, only 3% were starting in the next year. Of responding hospitalists with exposure to a PDC, 70% were satisfied with the experience and 85% felt their patients were satisfied. Responses did not vary by type of practice (academic vs nonacademic), group size, geographic location, or by exposure to a PDC except as above.
DISCUSSION
Responding hospitalists reported encountering significant difficulty arranging appropriate postdischarge appointments with primary care providers and feel this contributes to postdischarge complications. Nearly 75% of those surveyed felt a hospitalist‐run PDC would be effective in reducing postdischarge emergency department visits, presumably in part due to improved access to postdischarge care. However, 62% of responding hospitalists opposed providing this type of care, though those who had experienced a PDC were somewhat more likely to view providing care in a PDC favorably. Survey responses largely reflect attitudes rather than experience with PDCs, because very few respondents had ever worked in a PDC.
The juxtaposition of the confidence expressed in PDCs to reduce postdischarge emergency department visits with the less enthusiastic views of respondents about providing care in a PDC was surprising. Several explanations are possible. First, providing such care is outside the usual scope of practice of most hospitalists, and preliminary reports indicate hospitalists, as self‐selected inpatient providers, may not initially welcome this opportunity.[12] Second, responding hospitalists identified the need for extra compensation for providing this care, suggesting they would see staffing a PDC as a burden requiring extra payment. Third, only 12% of respondents felt their responsibility to their discharged patients extended to 1 month following discharge. Given this, hospitalists may not feel enough personal ownership over 30‐day readmission rates to justify the additional clinical demand of staffing a PDC.[13]
In fact, 29% of responding hospitalists felt their responsibility to the patient ended at the time of discharge. Respondents may have interpreted responsibility differently, and we cannot rule out response bias given our lower‐than‐expected response rate. However, we had anticipated many fewer hospitalists would respond this way given professional hospitalist societies have endorsed guidelines for improved transitions of care, which clearly delineate the key role hospitalists play in care transitions.[14]
Although fewer than 10% of respondents had worked in a PDC, nearly one‐third reported considering starting such a practice in the future, underscoring the importance of understanding hospitalist attitudes and experiences when creating a PDC and the significant barriers to arranging appropriate postdischarge care identified by survey respondents. The barriers to establishing a PDC may explain why few planned to start a PDC in the next year.
This study should be interpreted in the context of its design. Due to limitations in survey delivery, more rigorous sampling designs could not be used, and efforts were instead made to deliver the survey to a diverse group of hospitalists. The survey response rate was lower than anticipated and this increases the risk of response bias. Though this response rate is characteristic of other surveys of hospitalists, responses may have been from a selected population and therefore not representative of all hospitalists.[15] We sampled from a variety of practice venues, locations, academic and community practices, and practice group sizes to try to minimize this bias. Due to the low exposure rate to PDCs, hospitalist responses to experiences with PDCs should be considered exploratory.
We asked about similar content areas in the survey in multiple questions to maximize content validity; this resulted in variations in the degree of agreement or disagreement to similar prompts. For example, 62% of hospitalists opposed seeing patients in the clinic after discharge when directly asked, but nearly 50% said they would welcome the opportunity to work in a PDC if their employer required it. In another example, 50% of respondents said their responsibility for the patient ended at time of discharge, but when asked about duration of responsibility, 30% identified time of discharge as the limit. When reporting and interpreting results, we have tried to highlight responses to questions that ask most clearly and directly about the content of interest (rather than general themes), but this interpretation may also be subject to bias.
The time after hospital discharge is one of heightened risk for adverse events for the recently discharged patient. Hospitalist‐run postdischarge clinics may offer improved postdischarge care access and continuity; more research is needed on the effects of such clinics on patient outcomes, including postdischarge utilization. Until then, physicians and hospitals considering establishing PDCs should consider the barriers responding hospitalists identified to working in such a clinic, as well as the confidence they expressed in PDCs to reduce subsequent utilization.
Disclosures: Dr. Burke had full access to all of the data in the study and takes responsibility for the integrity of the data and the accuracy of the data analysis. The views expressed in this article are those of the authors and do not necessarily represent the views of the Department of Veterans Affairs. Dr. Burke was supported by grant funding from the Colorado Research Enhancement Award Program to Improve Care Coordination for Veterans. Dr. Ryan has no conflicts of interest to disclose.
- The incidence and severity of adverse events affecting patients after discharge from the hospital. Ann Intern Med. 2003;138(3):161–167. , , , , .
- Rehospitalizations among patients in the Medicare fee‐for‐service program. N Engl J Med. 2009;360(14):1418–1428. , , .
- Relationship between early physician follow‐up and 30‐day readmission among Medicare beneficiaries hospitalized for heart failure. JAMA. 2010;303(17):1716–1722. , , , et al.
- Post‐hospitalization transitions: Examining the effects of timing of primary care provider follow‐up. J Hosp Med. 2010;5(7):392–397. , , .
- Will generalist physician supply meet demands of an increasing and aging population? Health Aff (Millwood). 2008;27(3):w232–w241. , , .
- Association of American Medical Colleges. June 2010.The impact of health care reform on the future supply and demand for physicians updated projections through 2025. Available at: http://www.aamc.org/download/158076/data/updated_projections_through_2025.pdf. Accessed May 1, 2012.
- Continuity of outpatient and inpatient care by primary care physicians for hospitalized older adults. JAMA. 2009;301(16):1671–1680. , , , , , .
- Trends in inpatient continuity of care for a cohort of Medicare patients 1996–2006. J Hosp Med. 2011;6(8):438–444. , , , , .
- Is a post‐discharge clinic in your hospital's future? The Hospitalist. December 2011 Available at: http://www.the‐hospitalist.org/details/article/1409011/Is_a_Post_Discharge_Clinic_in_Your_Hospitals_future.html. Accessed May 1, 2013. .
- Continuity of care and patient outcomes after hospital discharge. J Gen Intern Med. 2004;19(6):624–631. , , , .
- Effects of a postdischarge clinic on housestaff satisfaction and utilization of hospital services. J Gen Intern Med. 1996;11(3):179–181. , , , .
- Interval examination: establishment of a hospitalist‐staffed discharge clinic. J Gen Intern Med. 2012;27(10):1377–1382. .
- Patient Protection and Affordable Care Act (PPACA).Public Law 111–148 2010. Available at: http://www.gpo.gov/fdsys/pkg/PLAW‐111publ148/pdf/PLAW‐111publ148.pdf. Accessed January 10, 2013.
- Transitions of Care Consensus policy statement: American College of Physicians, Society of General Internal Medicine, Society of Hospital Medicine, American Geriatrics Society, American College of Emergency Physicians, and Society for Academic Emergency Medicine. J Hosp Med. 2009;4(6):364–370. , , , et al.
- Person‐job fit: an exploratory cross‐sectional analysis of hospitalists. J Hosp Med. 2013;8(2):96–101. , , , , .
- The incidence and severity of adverse events affecting patients after discharge from the hospital. Ann Intern Med. 2003;138(3):161–167. , , , , .
- Rehospitalizations among patients in the Medicare fee‐for‐service program. N Engl J Med. 2009;360(14):1418–1428. , , .
- Relationship between early physician follow‐up and 30‐day readmission among Medicare beneficiaries hospitalized for heart failure. JAMA. 2010;303(17):1716–1722. , , , et al.
- Post‐hospitalization transitions: Examining the effects of timing of primary care provider follow‐up. J Hosp Med. 2010;5(7):392–397. , , .
- Will generalist physician supply meet demands of an increasing and aging population? Health Aff (Millwood). 2008;27(3):w232–w241. , , .
- Association of American Medical Colleges. June 2010.The impact of health care reform on the future supply and demand for physicians updated projections through 2025. Available at: http://www.aamc.org/download/158076/data/updated_projections_through_2025.pdf. Accessed May 1, 2012.
- Continuity of outpatient and inpatient care by primary care physicians for hospitalized older adults. JAMA. 2009;301(16):1671–1680. , , , , , .
- Trends in inpatient continuity of care for a cohort of Medicare patients 1996–2006. J Hosp Med. 2011;6(8):438–444. , , , , .
- Is a post‐discharge clinic in your hospital's future? The Hospitalist. December 2011 Available at: http://www.the‐hospitalist.org/details/article/1409011/Is_a_Post_Discharge_Clinic_in_Your_Hospitals_future.html. Accessed May 1, 2013. .
- Continuity of care and patient outcomes after hospital discharge. J Gen Intern Med. 2004;19(6):624–631. , , , .
- Effects of a postdischarge clinic on housestaff satisfaction and utilization of hospital services. J Gen Intern Med. 1996;11(3):179–181. , , , .
- Interval examination: establishment of a hospitalist‐staffed discharge clinic. J Gen Intern Med. 2012;27(10):1377–1382. .
- Patient Protection and Affordable Care Act (PPACA).Public Law 111–148 2010. Available at: http://www.gpo.gov/fdsys/pkg/PLAW‐111publ148/pdf/PLAW‐111publ148.pdf. Accessed January 10, 2013.
- Transitions of Care Consensus policy statement: American College of Physicians, Society of General Internal Medicine, Society of Hospital Medicine, American Geriatrics Society, American College of Emergency Physicians, and Society for Academic Emergency Medicine. J Hosp Med. 2009;4(6):364–370. , , , et al.
- Person‐job fit: an exploratory cross‐sectional analysis of hospitalists. J Hosp Med. 2013;8(2):96–101. , , , , .
Patients at Risk for 30‐Day Readmission
Readmissions to the hospital are common and costly.[1] However, identifying patients prospectively who are likely to be readmitted and who may benefit from interventions to reduce readmission risk has proven challenging, with published risk scores having only moderate ability to discriminate between patients likely and unlikely to be readmitted.[2] One reason for this may be that published studies have not typically focused on patients who are cognitively impaired, psychiatrically ill, have low health or English literacy, or have poor social supports, all of whom may represent a substantial fraction of readmitted patients.[2, 3, 4, 5]
Psychiatric disease, in particular, may contribute to increased readmission risk for nonpsychiatric (medical) illness, and is associated with increased utilization of healthcare resources.[6, 7, 8, 9, 10, 11] For example, patients with mental illness who were discharged from New York hospitals were more likely to be rehospitalized and had more costly readmissions than patients without these comorbidities, including a length of stay nearly 1 day longer on average.[7] An unmet need for treatment of substance abuse was projected to cost Tennessee $772 million of excess healthcare costs in 2000, mostly incurred through repeat hospitalizations and emergency department (ED) visits.[10]
Despite this, few investigators have considered the role of psychiatric disease and/or substance abuse in medical readmission risk. The purpose of the current study was to evaluate the role of psychiatric illness and substance abuse in unselected medical patients to determine their relative contributions to 30‐day all‐cause readmissions (ACR) and potentially avoidable readmissions (PAR).
METHODS
Patients and Setting
We conducted a retrospective cohort study of consecutive adult patients discharged from medicine services at Brigham and Women's Hospital (BWH), a 747‐bed tertiary referral center and teaching hospital, between July 1, 2009 and June 30, 2010. Most patients are cared for by resident housestaff teams at BWH (approximately 25% are cared for by physician assistants working directly with attending physicians), and approximately half receive primary care in the Partners system, which has a shared electronic medical record (EMR). Outpatient mental health services are provided by Partners‐associated mental health professionals including those at McLean Hospital and MassHealth (Medicaid)‐associated sites through the Massachusetts Behavioral Health Partnership. Exclusion criteria were death in the hospital or discharge to another acute care facility. We also excluded patients who left against medical advice (AMA). The study protocol was approved by the Partners Institutional Review Board.
Outcome
The primary outcomes were ACR and PAR within 30 days of discharge. First, we identified all 30‐day readmissions to BWH or to 2 other hospitals in the Partners Healthcare Network (previous studies have shown that 80% of all readmitted patients are readmitted to 1 of these 3 hospitals).[12] For patients with multiple readmissions, only the first readmission was included in the dataset.
To find potentially avoidable readmissions, administrative and billing data for these patients were processed using the SQLape (SQLape s.a.r.l., Corseaux, Switzerland) algorithm, which identifies PAR by excluding patients who undergo planned follow‐up treatment (such as a cycle of planned chemotherapy) or are readmitted for conditions unrelated in any way to the index hospitalization.[13, 14] Common complications of treatment are categorized as potentially avoidable, such as development of a deep venous thrombosis, a decubitus ulcer after prolonged bed rest, or bleeding complications after starting anticoagulation. Although the algorithm identifies theoretically preventable readmissions, the algorithm does not quantify how preventable they are, and these are thus referred to as potentially avoidable. This is similar to other admission metrics, such as the Agency for Healthcare Research and Quality's prevention quality indicators, which are created from a list of ambulatory care‐sensitive conditions.[15] SQLape has the advantage of being a specific tool for readmissions. Patients with 30‐day readmissions identified by SQLape as planned or unlikely to be avoidable were excluded in the PAR analysis, although still included in ACR analysis. In each case, the comparison group is patients without any readmission.
Predictors
Our predictors of interest included the overall prevalence of a psychiatric diagnosis or diagnosis of substance abuse, the presence of specific psychiatric diagnoses, and prescription of psychiatric medications to help assess the independent contribution of these comorbidities to readmission risk.
We used a combination of easily obtainable inpatient and outpatient clinical and administrative data to identify relevant patients. Patients were considered likely to be psychiatrically ill if they: (1) had a psychiatric diagnosis on their Partners outpatient EMR problem list and were prescribed a medication to treat that condition as an outpatient, or (2) had an International Classification of Diseases, 9th Revision diagnosis of a psychiatric illness at hospital discharge. Patients were considered to have moderate probability of disease if they: (1) had a psychiatric diagnosis on their outpatient problem list, or (2) were prescribed a medication intended to treat a psychiatric condition as an outpatient. Patients were considered unlikely to have psychiatric disease if none of these criteria were met. Patients were considered likely to have a substance abuse disorder if they had this diagnosis on their outpatient EMR, or were prescribed a medication to treat this condition (eg, buprenorphine/naloxone), or received inpatient consultation from a substance abuse treatment team during their inpatient hospitalization, and were considered unlikely if none of these were true. We also evaluated individual categories of psychiatric illness (schizophrenia, depression, anxiety, bipolar disorder) and of psychotropic medications (antidepressants, antipsychotics, anxiolytics).
Potential Confounders
Data on potential confounders, based on prior literature,[16, 17] collected at the index admission were derived from electronic administrative, clinical, and billing sources, including the Brigham Integrated Computer System and the Partners Clinical Data Repository. They included patient age, gender, ethnicity, primary language, marital status, insurance status, living situation prior to admission, discharge location, length of stay, Elixhauser comorbidity index,[18] total number of medications prescribed, and number of prior admissions and ED visits in the prior year.
Statistical Analysis
Bivariate comparisons of each of the predictors of ACR and PAR risk (ie, patients with a 30‐day ACR or PAR vs those not readmitted within 30 days) were conducted using 2 trend tests for ordinal predictors (eg, likelihood of psychiatric disease), and 2 or Fisher exact test for dichotomous predictors (eg, receipt of inpatient substance abuse counseling).
We then used multivariate logistic regression analysis to adjust for all of the potential confounders noted above, entering each variable related to psychiatric illness into the model separately (eg, likely psychiatric illness, number of psychiatric medications). In a secondary analysis, we removed potentially collinear variables from the final model; as this did not alter the results, the full model is presented. We also conducted a secondary analysis where we included patients who left against medical advice (AMA), which also did not alter the results. Two‐sided P values <0.05 were considered significant, and all analyses were performed using the SAS version 9.2 (SAS Institute, Inc., Cary, NC).
RESULTS
There were 7984 unique patients discharged during the study period. Patients were generally white and English speaking; just over half of admissions came from the ED (Table 1). Of note, nearly all patients were insured, as are almost all patients in Massachusetts. They had high degrees of comorbid illness and large numbers of prescribed medications. Nearly 30% had at least 1 hospital admission within the prior year.
Characteristic | All Patients, N (%) | Not Readmitted, N (%) | ACR, N (%) | PAR N (%)a |
---|---|---|---|---|
| ||||
Study cohort | 6987 (100) | 5727 (72) | 1260 (18) | 388 (5.6) |
Age, y | ||||
<50 | 1663 (23.8) | 1343 (23.5) | 320 (25.4) | 85 (21.9) |
5165 | 2273 (32.5) | 1859 (32.5) | 414 (32.9) | 136 (35.1) |
6679 | 1444 (20.7) | 1176 (20.5) | 268 (18.6) | 80 (20.6) |
>80 | 1607 (23.0) | 1349 (23.6) | 258 (16.1) | 87 (22.4) |
Female | 3604 (51.6) | 2967 (51.8) | 637 (50.6) | 206 (53.1) |
Race | ||||
White | 5126 (73.4) | 4153 (72.5) | 973 (77.2) | 300 (77.3) |
Black | 1075 (15.4) | 899 (15.7) | 176 (14.0) | 53 (13.7) |
Hispanic | 562 (8.0) | 477 (8.3) | 85 (6.8) | 28 (7.2) |
Other | 224 (3.2) | 198 (3.5) | 26 (2.1) | 7 (1.8) |
Primary language | ||||
English | 6345 (90.8) | 5180 (90.5) | 1165 (92.5) | 356 (91.8) |
Marital status | ||||
Married | 3642 (52.1) | 2942 (51.4) | 702 (55.7) | 214 (55.2) |
Single, never married | 1662 (23.8) | 1393 (24.3) | 269 (21.4) | 73 (18.8) |
Previously married | 1683 (24.1) | 1386 (24.2) | 289 (22.9) | 101 (26.0) |
Insurance | ||||
Medicare | 3550 (50.8) | 2949 (51.5) | 601 (47.7) | 188 (48.5) |
Medicaid | 539 (7.7) | 430 (7.5) | 109 (8.7) | 33 (8.5) |
Private | 2892 (41.4) | 2344 (40.9) | 548 (43.5) | 167 (43.0) |
Uninsured | 6 (0.1) | 4 (0.1) | 2 (0.1) | 0 (0) |
Source of index admission | ||||
Clinic or home | 2136 (30.6) | 1711 (29.9) | 425 (33.7) | 117 (30.2) |
Emergency department | 3592 (51.4) | 2999 (52.4) | 593 (47.1) | 181 (46.7) |
Nursing facility | 1204 (17.2) | 977 (17.1) | 227 (18.0) | 84 (21.7) |
Other | 55 (0.1) | 40 (0.7) | 15 (1.1) | 6 (1.6) |
Length of stay, d | ||||
02 | 1757 (25.2) | 1556 (27.2) | 201 (16.0) | 55 (14.2) |
34 | 2200 (31.5) | 1842 (32.2) | 358 (28.4) | 105 (27.1) |
57 | 1521 (21.8) | 1214 (21.2) | 307 (24.4) | 101 (26.0) |
>7 | 1509 (21.6) | 1115 (19.5) | 394 (31.3) | 127 (32.7) |
Elixhauser comorbidity index score | ||||
01 | 1987 (28.4) | 1729 (30.2) | 258 (20.5) | 66 (17.0) |
27 | 1773 (25.4) | 1541 (26.9) | 232 (18.4) | 67 (17.3) |
813 | 1535 (22.0) | 1212 (21.2) | 323 (25.6) | 86 (22.2) |
>13 | 1692 (24.2) | 1245 (21.7) | 447 (35.5) | 169 (43.6) |
Medications prescribed as outpatient | ||||
06 | 1684 (24.1) | 1410 (24.6) | 274 (21.8) | 72 (18.6) |
79 | 1601 (22.9) | 1349 (23.6) | 252 (20.0) | 77 (19.9) |
1013 | 1836 (26.3) | 1508 (26.3) | 328 (26.0) | 107 (27.6) |
>13 | 1866 (26.7) | 1460 (25.5) | 406 (32.2) | 132 (34.0) |
Number of admissions in past year | ||||
0 | 4816 (68.9) | 4032 (70.4) | 784 (62.2) | 279 (71.9) |
15 | 2075 (29.7) | 1640 (28.6) | 435 (34.5) | 107 (27.6) |
>5 | 96 (1.4) | 55 (1.0) | 41 (3.3) | 2 (0.5) |
Number of ED visits in past year | ||||
0 | 4661 (66.7) | 3862 (67.4) | 799 (63.4) | 261 (67.3) |
15 | 2326 (33.3) | 1865 (32.6) | 461 (36.6) | 127 (32.7) |
All‐Cause Readmissions
After exclusion of 997 patients who died, were discharged to skilled nursing or rehabilitation facilities, or left AMA, 6987 patients were included (Figure 1). Of these, 1260 had a readmission (18%). Approximately half were considered unlikely to be psychiatrically ill, 22% were considered moderately likely, and 29% likely (Table 2).
All‐Cause Readmission Analysis | Potentially Avoidable Readmission Analysis | |||||
---|---|---|---|---|---|---|
No. in Cohort (%) | % of Patients With ACR | P Valuea | No. in Cohort (%) | % of Patients With PAR | P Valuea | |
| ||||||
Entire cohort | 6987 | 18.0 | 6115 | 6.3 | ||
Likelihood of psychiatric illness | ||||||
Unlikely | 3424 (49) | 16.5 | 3026 (49) | 5.6 | ||
Moderate | 1564 (22) | 23.5 | 1302 (21) | 7.1 | ||
Likely | 1999 (29) | 16.4 | 1787 (29) | 6.4 | ||
Likely versus unlikely | 0.87 | 0.20 | ||||
Moderate+likely versus unlikely | 0.001 | 0.02 | ||||
Likelihood of substance abuse | 0.01 | 0.20 | ||||
Unlikely | 5804 (83) | 18.7 | 5104 (83) | 6.5 | ||
Likely | 1183 (17) | 14.8 | 1011 (17) | 5.4 | 0.14 | |
Number of prescribed outpatient psychotropic medications | <0.001 | 0.04 | ||||
0 | 4420 (63) | 16.3 | 3931 (64) | 5.9 | ||
1 | 1725 (25) | 20.4 | 1481 (24) | 7.2 | ||
2 | 781 (11) | 22.3 | 653 (11) | 7.0 | ||
>2 | 61 (1) | 23.0 | 50 (1) | 6.0 | ||
Prescribed antidepressant | 1474 (21) | 20.6 | 0.005 | 1248 (20) | 6.2 | 0.77 |
Prescribed antipsychotic | 375 (5) | 22.4 | 0.02 | 315 (5) | 7.6 | 0.34 |
Prescribed mood stabilizer | 81 (1) | 18.5 | 0.91 | 69 (1) | 4.4 | 0.49 |
Prescribed anxiolytic | 1814 (26) | 21.8 | <0.001 | 1537 (25) | 7.7 | 0.01 |
Prescribed stimulant | 101 (2) | 26.7 | 0.02 | 83 (1) | 10.8 | 0.09 |
Prescribed pharmacologic treatment for substance abuse | 79 (1) | 25.3 | 0.09 | 60 (1) | 1.7 | 0.14 |
Number of psychiatric diagnoses on outpatient problem list | 0.31 | 0.74 | ||||
0 | 6405 (92) | 18.2 | 5509 (90) | 6.3 | ||
1 or more | 582 (8) | 16.5 | 474 (8) | 7.0 | ||
Outpatient diagnosis of substance abuse | 159 (2) | 13.2 | 0.11 | 144 (2) | 4.2 | 0.28 |
Outpatient diagnosis of any psychiatric illness | 582 (8) | 16.5 | 0.31 | 517 (8) | 8.0 | 0.73 |
Discharge diagnosis of depression | 774 (11) | 17.7 | 0.80 | 690 (11) | 7.7 | 0.13 |
Discharge diagnosis of schizophrenia | 56 (1) | 23.2 | 0.31 | 50 (1) | 14 | 0.03 |
Discharge diagnosis of bipolar disorder | 101 (1) | 10.9 | 0.06 | 92 (2) | 2.2 | 0.10 |
Discharge diagnosis of anxiety | 1192 (17) | 15.0 | 0.003 | 1080 (18) | 6.2 | 0.83 |
Discharge diagnosis of substance abuse | 885 (13) | 14.8 | 0.008 | 803 (13) | 6.1 | 0.76 |
Discharge diagnosis of any psychiatric illness | 1839 (26) | 16.0 | 0.008 | 1654 (27) | 6.6 | 0.63 |
Substance abuse consultation as inpatient | 284 (4) | 14.4 | 0.11 | 252 (4) | 3.6 | 0.07 |
In bivariate analysis (Table 2), likelihood of psychiatric illness (P<0.01) and increasing numbers of prescribed outpatient psychiatric medications (P<0.01) were significantly associated with ACR. In multivariate analysis, each additional prescribed outpatient psychiatric medication increased ACR risk (odds ratio [OR]: 1.10, 95% confidence interval [CI]: 1.01‐1.20) or any prescription of an anxiolytic in particular (OR: 1.16, 95% CI: 1.001.35) was associated with increased risk of ACR, whereas discharge diagnoses of anxiety (OR: 0.82, 95% CI: 0.68‐0.99) and substance abuse (OR: 0.80, 95% CI: 0.65‐0.99) were associated with lower risk of ACR (Table 3).
ACR, OR (95% CI) | PAR, OR (95% CI)a | |
---|---|---|
| ||
Likely psychiatric disease | 0.97 (0.82‐1.14) | 1.20 (0.92‐1.56) |
Likely and possible psychiatric disease | 1.07 (0.94‐1.22) | 1.18 (0.94‐1.47) |
Likely substance abuse | 0.83 (0.69‐0.99) | 0.85 (0.63‐1.16) |
Psychiatric diagnosis on outpatient problem list | 0.97 (0.76‐1.23) | 1.04 (0.70‐1.55) |
Substance abuse diagnosis on outpatient problem list | 0.63 (0.39‐1.02) | 0.65 (0.28‐1.52) |
Increasing number of prescribed psychiatric medications | 1.10 (1.01‐1.20) | 1.00 (0.86‐1.16) |
Outpatient prescription for antidepressant | 1.10 (0.94‐1.29) | 0.86 (0.66‐1.13) |
Outpatient prescription for antipsychotic | 1.03 (0.79‐1.34) | 0.93 (0.59‐1.45) |
Outpatient prescription for anxiolytic | 1.16 (1.001.35) | 1.13 (0.88‐1.44) |
Outpatient prescription for methadone or buprenorphine | 1.15 (0.67‐1.98) | 0.18 (0.03‐1.36) |
Discharge diagnosis of depression | 1.06 (0.86‐1.30) | 1.49 (1.09‐2.04) |
Discharge diagnosis of schizophrenia | 1.43 (0.75‐2.74) | 2.63 (1.13‐6.13) |
Discharge diagnosis of bipolar disorder | 0.53 (0.28‐1.02) | 0.35 (0.09‐1.45) |
Discharge diagnosis of anxiety | 0.82 (0.68‐0.99) | 1.11 (0.83‐1.49) |
Discharge diagnosis of substance abuse | 0.80 (0.65‐0.99) | 1.05 (0.75‐1.46) |
Discharge diagnosis of any psychiatric illness | 0.88 (0.75‐1.02) | 1.22 (0.96‐1.56) |
Addiction team consult while inpatient | 0.82 (0.58‐1.17) | 0.58 (0.29‐1.17) |
Potentially Avoidable Readmissions
After further exclusion of 872 patients who had unavoidable readmissions according to the SQLape algorithm, 6115 patients remained. Of these, 388 had a PAR within 30 days (6.3%, Table 1).
In bivariate analysis (Table 2), the likelihood of psychiatric illness (P=0.02), number of outpatient psychiatric medications (P=0.04), and prescription of anxiolytics (P=0.01) were significantly associated with PAR, as they were with ACR. A discharge diagnosis of schizophrenia was also associated with PAR (P=0.03).
In multivariate analysis, only discharge diagnoses of depression (OR: 1.49, 95% CI: 1.09‐2.04) and schizophrenia (OR: 2.63, 95% CI: 1.13‐6.13) were associated with PAR.
DISCUSSION
Comorbid psychiatric illness was common among patients admitted to the medicine wards. Patients with documented discharge diagnoses of depression or schizophrenia were at highest risk for a potentially avoidable 30‐day readmission, whereas those prescribed more psychiatric medications were at increased risk for ACR. These findings were independent of a comprehensive set of risk factors among medicine inpatients in this retrospective cohort study.
This study extends prior work indicating patients with psychiatric disease have increased healthcare utilization,[6, 7, 8, 9, 10, 11] by identifying at least 2 subpopulations of the psychiatrically ill (those with depression and schizophrenia) at particularly high risk for 30‐day PAR. To our knowledge, this is the first study to identify schizophrenia as a predictor of hospital readmission for medical illnesses. One prior study prospectively identified depression as increasing the 90‐day risk of readmission 3‐fold, although medication usage was not assessed,[6] and our report strengthens this association.
There are several possible explanations why these two subpopulations in particular would be more predisposed to readmissions that are potentially avoidable. It is known that patients with schizophrenia, for example, live on average 20 years less than the general population, and most of this excess mortality is due to medical illnesses.[20, 21] Reasons for this may include poor healthcare access, adverse effects of medication, and socioeconomic factors among others.[21, 22] All of these reasons may contribute to the increased PAR risk in this population, mediated, for example, by decreased ability to adhere to postdischarge care plans. Successful community‐based interventions to decrease these inequities have been described and could serve as a model for addressing the increased readmission risk in this population.[23]
Our finding that patients with a greater number of prescribed psychiatric medications are at increased risk for ACR may be expected, given other studies that have highlighted the crucial importance of medications in postdischarge adverse events, including readmissions.[24] Indeed, medication‐related errors and toxicities are the most common postdischarge adverse events experienced by patients.[25] Whether psychiatric medications are particularly prone to causing postdischarge adverse events or whether these medications represent greater psychiatric comorbidity cannot be answered by this study.
It was surprising but reassuring that substance abuse was not a predictor of short‐term readmissions as identified using our measures; in fact, a discharge diagnosis of substance abuse was associated with lower risk of ACR than comparator patients. It seems unlikely that we would have inadequate power to find such a result, as we found a statistically significant negative association in the ACR population, and 17% of our population overall was considered likely to have a substance abuse comorbidity. However, it is likely the burden of disease was underestimated given that we did not try to determine the contribution of long‐term substance abuse to medical diseases that may increase readmission risk (eg, liver cirrhosis from alcohol use). Unlike other conditions in our study, patients with substance abuse diagnoses at BWH can be seen by a dedicated multidisciplinary team while an inpatient to start treatment and plan for postdischarge follow‐up; this may have played a role in our findings.
A discharge diagnosis of anxiety was also somewhat protective against readmission, whereas a prescription of an anxiolytic (predominantly benzodiazepines) increased risk; many patients prescribed a benzodiazepine do not have a Diagnostic and Statistical Manual of Mental Disorders4th Edition (DSM‐IV) diagnosis of anxiety disorder, and thus these findings may reflect different patient populations. Discharging physicians may have used anxiety as a discharge diagnosis in patients in whom they suspected somatic complaints without organic basis; these patients may be at lower risk of readmission.
Discharge diagnoses of psychiatric illnesses were associated with ACR and PAR in our study, but outpatient diagnoses were not. This likely reflects greater severity of illness (documentation as a treated diagnosis on discharge indicates the illness was relevant during the hospitalization), but may also reflect inaccuracies of diagnosis and lack of assessment of severity in outpatient coding, which would bias toward null findings. Although many of the patients in our study were seen by primary care doctors within the Partners system, some patients had outside primary care physicians and we did not have access to these records. This may also have decreased our ability to find associations.
The findings of our study should be interpreted in the context of the study design. Our study was retrospective, which limited our ability to conclusively diagnose psychiatric disease presence or severity (as is true of most institutions, validated psychiatric screening was not routinely used at our institutions on hospital admission or discharge). However, we used a conservative scale to classify the likelihood of patients having psychiatric or substance abuse disorders, and we used other metrics to establish the presence of illness, such as the number of prescribed medications, inpatient consultation with a substance abuse service, and hospital discharge diagnoses. This approach also allowed us to quickly identify a large cohort unaffected by selection bias. Our study was single center, potentially limiting generalizability. Although we capture at least 80% of readmissions, we were not able to capture all readmissions, and we cannot rule out that patients readmitted elsewhere are different than those readmitted within the Partners system. Last, the SQLape algorithm is not perfectly sensitive or specific in identifying avoidable readmissions,[13] but it does eliminate many readmissions that are clearly unavoidable, creating an enriched cohort of patients whose readmissions are more likely to be avoidable and therefore potentially actionable.
We suggest that our study findings first be considered when risk stratifying patients before hospital discharge in terms of readmission risk. Patients with depression and schizophrenia would seem to merit postdischarge interventions to decrease their potentially avoidable readmissions. Compulsory community treatment (a feature of treatment in Canada and Australia that is ordered by clinicians) has been shown to decrease mortality due to medical illness in patients who have been hospitalized and are psychiatrically ill, and addition of these services to postdischarge care may be useful.[23] Inpatient physicians could work to ensure follow‐up not just with medical providers but with robust outpatient mental health programs to decrease potentially avoidable readmission risk, and administrators could work to ensure close linkages with these community resources. Studies evaluating the impact of these types of interventions would need to be conducted. Patients with polypharmacy, including psychiatric medications, may benefit from interventions to improve medication safety, such as enhanced medication reconciliation and pharmacist counseling.[26]
Our study suggests that patients with depression, those with schizophrenia, and those who have increased numbers of prescribed psychiatric medications should be considered at high risk for readmission for medical illnesses. Targeting interventions to these patients may be fruitful in preventing avoidable readmissions.
Acknowledgements
The authors thank Dr. Yves Eggli for screening the database for potentially avoidable readmissions using the SQLape algorithm.
Disclosures
Dr. Donz was supported by the Swiss National Science Foundation and the Swiss Foundation for MedicalBiological Scholarships. The authors otherwise have no conflicts of interest to disclose. The content is solely the responsibility of the authors and does not necessarily represent the official views of the US Department of Veterans Affairs.
- Rehospitalizations among patients in the Medicare fee‐for‐service program. N Engl J Med. 2009;360(14):1418–1428. , , .
- Risk prediction models for hospital readmission: a systematic review. JAMA. 2011;306(15):1688–1698. , , , et al.
- Hospital readmission as an accountability measure. JAMA. 2011;305(5):504–505. , .
- Postdischarge environmental and socioeconomic factors and the likelihood of early hospital readmission among community‐dwelling Medicare beneficiaries. Gerontologist. 2008;48(4):495–504. , , , , , .
- Low health literacy and health outcomes: an updated systematic review. Ann Intern Med. 2011;155(2):97–107. , , , , .
- Depression is a risk factor for rehospitalization in medical inpatients. Prim Care Companion J Clin Psychiatry. 2007;9(4):256–262. , , , et al.
- Mental illness and hospitalization for ambulatory care sensitive medical conditions. Med Care. 2008;46(12):1249–1256. , , , .
- Substance use treatment barriers for patients with frequent hospital admissions. J Subst Abuse Treat. 2010;38(1):22–30. , , , , , .
- Managed care and the quality of substance abuse treatment. J Ment Health Policy Econ. 2002;5(4):163–174. , , , , .
- Unmet substance abuse treatment need, health services utilization, and cost: a population‐based emergency department study. Ann Emerg Med. 2005;45(2):118–127. , , , , .
- Elderly Medicare inpatients with substance use disorders: characteristics and predictors of hospital readmissions over a four‐year interval. J Stud Alcohol. 2000;61(6):891–895. , , , .
- Rationale and design of the Pharmacist Intervention for Low Literacy in Cardiovascular Disease (PILL‐CVD) study. Circ Cardiovasc Qual Outcomes. 2010;3(2):212–219. , , , et al.
- Validation of the potentially avoidable hospital readmission rate as a routine indicator of the quality of hospital care. Med Care. 2006;44(11);972–981. , , , , , .
- Measuring potentially avoidable hospital readmissions. J Clin Epidemiol. 2002;55:573–587. , , , , , .
- Agency for Healthcare Research and Quality Quality Indicators. (April 7, 2006). Prevention Quality Indicators (PQI) Composite Measure Workgroup Final Report. Available at: http://www.qualityindicators.ahrq.gov/modules/pqi_resources.aspx. Accessed June 1, 2012.
- Hospital readmission in general medicine patients: a prediction model. J Gen Intern Med. 2010;25(3):211–219. , , , et al.
- Derivation and validation of an index to predict early death or unplanned readmission after discharge from hospital to the community. CMAJ. 2010;182(6):551–557. , , , et al.
- Comorbidity measures for use with administrative data. Med Care. Jan 1998;36(1):8–27. , , , .
- Morbidity and mortality in people with serious mental illness. October 2006. National Association of State Mental Health Directors, Medical Directors Council. Available at: http://www.nasmhpd.org/docs/publications/MDCdocs/Mortality%20and%20 Mo rbidity%20Final%20Report%208.18.08.pdf. Accessed January 13, 2013. , , , , eds.
- Mortality in individuals who have had psychiatric treatment: population‐based study in Nova Scotia. Br J Psychiat. 2005;187:552–558. , , , .
- Inequitable access for mentally ill patients to some medically necessary procedures. CMAJ. 2007;176(6):779–784. , , , , , .
- Quality of medical care for people with and without comorbid mental illness and substance misuse: systematic review of comparative studies. Br J Psychiat. 2009;194:491–499. , , .
- Reducing all‐cause mortality among patients with psychiatric disorders: a population‐based study. CMAJ. 2013;185(1):E50–E56. , , , , , .
- Incidence of potentially avoidable urgent readmissions and their relation to all‐cause urgent readmissions. CMAJ. 2011;183(14):E1067–E1072. , , , et al.
- The incidence and severity of adverse events affecting patients after discharge from the hospital. Ann Intern Med. 2003;138(3):161–167. , , , , .
- Hospital‐based medication reconciliation practices: a systematic review. Arch Intern Med. 2012;172(14):1057–1069. , , , .
Readmissions to the hospital are common and costly.[1] However, identifying patients prospectively who are likely to be readmitted and who may benefit from interventions to reduce readmission risk has proven challenging, with published risk scores having only moderate ability to discriminate between patients likely and unlikely to be readmitted.[2] One reason for this may be that published studies have not typically focused on patients who are cognitively impaired, psychiatrically ill, have low health or English literacy, or have poor social supports, all of whom may represent a substantial fraction of readmitted patients.[2, 3, 4, 5]
Psychiatric disease, in particular, may contribute to increased readmission risk for nonpsychiatric (medical) illness, and is associated with increased utilization of healthcare resources.[6, 7, 8, 9, 10, 11] For example, patients with mental illness who were discharged from New York hospitals were more likely to be rehospitalized and had more costly readmissions than patients without these comorbidities, including a length of stay nearly 1 day longer on average.[7] An unmet need for treatment of substance abuse was projected to cost Tennessee $772 million of excess healthcare costs in 2000, mostly incurred through repeat hospitalizations and emergency department (ED) visits.[10]
Despite this, few investigators have considered the role of psychiatric disease and/or substance abuse in medical readmission risk. The purpose of the current study was to evaluate the role of psychiatric illness and substance abuse in unselected medical patients to determine their relative contributions to 30‐day all‐cause readmissions (ACR) and potentially avoidable readmissions (PAR).
METHODS
Patients and Setting
We conducted a retrospective cohort study of consecutive adult patients discharged from medicine services at Brigham and Women's Hospital (BWH), a 747‐bed tertiary referral center and teaching hospital, between July 1, 2009 and June 30, 2010. Most patients are cared for by resident housestaff teams at BWH (approximately 25% are cared for by physician assistants working directly with attending physicians), and approximately half receive primary care in the Partners system, which has a shared electronic medical record (EMR). Outpatient mental health services are provided by Partners‐associated mental health professionals including those at McLean Hospital and MassHealth (Medicaid)‐associated sites through the Massachusetts Behavioral Health Partnership. Exclusion criteria were death in the hospital or discharge to another acute care facility. We also excluded patients who left against medical advice (AMA). The study protocol was approved by the Partners Institutional Review Board.
Outcome
The primary outcomes were ACR and PAR within 30 days of discharge. First, we identified all 30‐day readmissions to BWH or to 2 other hospitals in the Partners Healthcare Network (previous studies have shown that 80% of all readmitted patients are readmitted to 1 of these 3 hospitals).[12] For patients with multiple readmissions, only the first readmission was included in the dataset.
To find potentially avoidable readmissions, administrative and billing data for these patients were processed using the SQLape (SQLape s.a.r.l., Corseaux, Switzerland) algorithm, which identifies PAR by excluding patients who undergo planned follow‐up treatment (such as a cycle of planned chemotherapy) or are readmitted for conditions unrelated in any way to the index hospitalization.[13, 14] Common complications of treatment are categorized as potentially avoidable, such as development of a deep venous thrombosis, a decubitus ulcer after prolonged bed rest, or bleeding complications after starting anticoagulation. Although the algorithm identifies theoretically preventable readmissions, the algorithm does not quantify how preventable they are, and these are thus referred to as potentially avoidable. This is similar to other admission metrics, such as the Agency for Healthcare Research and Quality's prevention quality indicators, which are created from a list of ambulatory care‐sensitive conditions.[15] SQLape has the advantage of being a specific tool for readmissions. Patients with 30‐day readmissions identified by SQLape as planned or unlikely to be avoidable were excluded in the PAR analysis, although still included in ACR analysis. In each case, the comparison group is patients without any readmission.
Predictors
Our predictors of interest included the overall prevalence of a psychiatric diagnosis or diagnosis of substance abuse, the presence of specific psychiatric diagnoses, and prescription of psychiatric medications to help assess the independent contribution of these comorbidities to readmission risk.
We used a combination of easily obtainable inpatient and outpatient clinical and administrative data to identify relevant patients. Patients were considered likely to be psychiatrically ill if they: (1) had a psychiatric diagnosis on their Partners outpatient EMR problem list and were prescribed a medication to treat that condition as an outpatient, or (2) had an International Classification of Diseases, 9th Revision diagnosis of a psychiatric illness at hospital discharge. Patients were considered to have moderate probability of disease if they: (1) had a psychiatric diagnosis on their outpatient problem list, or (2) were prescribed a medication intended to treat a psychiatric condition as an outpatient. Patients were considered unlikely to have psychiatric disease if none of these criteria were met. Patients were considered likely to have a substance abuse disorder if they had this diagnosis on their outpatient EMR, or were prescribed a medication to treat this condition (eg, buprenorphine/naloxone), or received inpatient consultation from a substance abuse treatment team during their inpatient hospitalization, and were considered unlikely if none of these were true. We also evaluated individual categories of psychiatric illness (schizophrenia, depression, anxiety, bipolar disorder) and of psychotropic medications (antidepressants, antipsychotics, anxiolytics).
Potential Confounders
Data on potential confounders, based on prior literature,[16, 17] collected at the index admission were derived from electronic administrative, clinical, and billing sources, including the Brigham Integrated Computer System and the Partners Clinical Data Repository. They included patient age, gender, ethnicity, primary language, marital status, insurance status, living situation prior to admission, discharge location, length of stay, Elixhauser comorbidity index,[18] total number of medications prescribed, and number of prior admissions and ED visits in the prior year.
Statistical Analysis
Bivariate comparisons of each of the predictors of ACR and PAR risk (ie, patients with a 30‐day ACR or PAR vs those not readmitted within 30 days) were conducted using 2 trend tests for ordinal predictors (eg, likelihood of psychiatric disease), and 2 or Fisher exact test for dichotomous predictors (eg, receipt of inpatient substance abuse counseling).
We then used multivariate logistic regression analysis to adjust for all of the potential confounders noted above, entering each variable related to psychiatric illness into the model separately (eg, likely psychiatric illness, number of psychiatric medications). In a secondary analysis, we removed potentially collinear variables from the final model; as this did not alter the results, the full model is presented. We also conducted a secondary analysis where we included patients who left against medical advice (AMA), which also did not alter the results. Two‐sided P values <0.05 were considered significant, and all analyses were performed using the SAS version 9.2 (SAS Institute, Inc., Cary, NC).
RESULTS
There were 7984 unique patients discharged during the study period. Patients were generally white and English speaking; just over half of admissions came from the ED (Table 1). Of note, nearly all patients were insured, as are almost all patients in Massachusetts. They had high degrees of comorbid illness and large numbers of prescribed medications. Nearly 30% had at least 1 hospital admission within the prior year.
Characteristic | All Patients, N (%) | Not Readmitted, N (%) | ACR, N (%) | PAR N (%)a |
---|---|---|---|---|
| ||||
Study cohort | 6987 (100) | 5727 (72) | 1260 (18) | 388 (5.6) |
Age, y | ||||
<50 | 1663 (23.8) | 1343 (23.5) | 320 (25.4) | 85 (21.9) |
5165 | 2273 (32.5) | 1859 (32.5) | 414 (32.9) | 136 (35.1) |
6679 | 1444 (20.7) | 1176 (20.5) | 268 (18.6) | 80 (20.6) |
>80 | 1607 (23.0) | 1349 (23.6) | 258 (16.1) | 87 (22.4) |
Female | 3604 (51.6) | 2967 (51.8) | 637 (50.6) | 206 (53.1) |
Race | ||||
White | 5126 (73.4) | 4153 (72.5) | 973 (77.2) | 300 (77.3) |
Black | 1075 (15.4) | 899 (15.7) | 176 (14.0) | 53 (13.7) |
Hispanic | 562 (8.0) | 477 (8.3) | 85 (6.8) | 28 (7.2) |
Other | 224 (3.2) | 198 (3.5) | 26 (2.1) | 7 (1.8) |
Primary language | ||||
English | 6345 (90.8) | 5180 (90.5) | 1165 (92.5) | 356 (91.8) |
Marital status | ||||
Married | 3642 (52.1) | 2942 (51.4) | 702 (55.7) | 214 (55.2) |
Single, never married | 1662 (23.8) | 1393 (24.3) | 269 (21.4) | 73 (18.8) |
Previously married | 1683 (24.1) | 1386 (24.2) | 289 (22.9) | 101 (26.0) |
Insurance | ||||
Medicare | 3550 (50.8) | 2949 (51.5) | 601 (47.7) | 188 (48.5) |
Medicaid | 539 (7.7) | 430 (7.5) | 109 (8.7) | 33 (8.5) |
Private | 2892 (41.4) | 2344 (40.9) | 548 (43.5) | 167 (43.0) |
Uninsured | 6 (0.1) | 4 (0.1) | 2 (0.1) | 0 (0) |
Source of index admission | ||||
Clinic or home | 2136 (30.6) | 1711 (29.9) | 425 (33.7) | 117 (30.2) |
Emergency department | 3592 (51.4) | 2999 (52.4) | 593 (47.1) | 181 (46.7) |
Nursing facility | 1204 (17.2) | 977 (17.1) | 227 (18.0) | 84 (21.7) |
Other | 55 (0.1) | 40 (0.7) | 15 (1.1) | 6 (1.6) |
Length of stay, d | ||||
02 | 1757 (25.2) | 1556 (27.2) | 201 (16.0) | 55 (14.2) |
34 | 2200 (31.5) | 1842 (32.2) | 358 (28.4) | 105 (27.1) |
57 | 1521 (21.8) | 1214 (21.2) | 307 (24.4) | 101 (26.0) |
>7 | 1509 (21.6) | 1115 (19.5) | 394 (31.3) | 127 (32.7) |
Elixhauser comorbidity index score | ||||
01 | 1987 (28.4) | 1729 (30.2) | 258 (20.5) | 66 (17.0) |
27 | 1773 (25.4) | 1541 (26.9) | 232 (18.4) | 67 (17.3) |
813 | 1535 (22.0) | 1212 (21.2) | 323 (25.6) | 86 (22.2) |
>13 | 1692 (24.2) | 1245 (21.7) | 447 (35.5) | 169 (43.6) |
Medications prescribed as outpatient | ||||
06 | 1684 (24.1) | 1410 (24.6) | 274 (21.8) | 72 (18.6) |
79 | 1601 (22.9) | 1349 (23.6) | 252 (20.0) | 77 (19.9) |
1013 | 1836 (26.3) | 1508 (26.3) | 328 (26.0) | 107 (27.6) |
>13 | 1866 (26.7) | 1460 (25.5) | 406 (32.2) | 132 (34.0) |
Number of admissions in past year | ||||
0 | 4816 (68.9) | 4032 (70.4) | 784 (62.2) | 279 (71.9) |
15 | 2075 (29.7) | 1640 (28.6) | 435 (34.5) | 107 (27.6) |
>5 | 96 (1.4) | 55 (1.0) | 41 (3.3) | 2 (0.5) |
Number of ED visits in past year | ||||
0 | 4661 (66.7) | 3862 (67.4) | 799 (63.4) | 261 (67.3) |
15 | 2326 (33.3) | 1865 (32.6) | 461 (36.6) | 127 (32.7) |
All‐Cause Readmissions
After exclusion of 997 patients who died, were discharged to skilled nursing or rehabilitation facilities, or left AMA, 6987 patients were included (Figure 1). Of these, 1260 had a readmission (18%). Approximately half were considered unlikely to be psychiatrically ill, 22% were considered moderately likely, and 29% likely (Table 2).
All‐Cause Readmission Analysis | Potentially Avoidable Readmission Analysis | |||||
---|---|---|---|---|---|---|
No. in Cohort (%) | % of Patients With ACR | P Valuea | No. in Cohort (%) | % of Patients With PAR | P Valuea | |
| ||||||
Entire cohort | 6987 | 18.0 | 6115 | 6.3 | ||
Likelihood of psychiatric illness | ||||||
Unlikely | 3424 (49) | 16.5 | 3026 (49) | 5.6 | ||
Moderate | 1564 (22) | 23.5 | 1302 (21) | 7.1 | ||
Likely | 1999 (29) | 16.4 | 1787 (29) | 6.4 | ||
Likely versus unlikely | 0.87 | 0.20 | ||||
Moderate+likely versus unlikely | 0.001 | 0.02 | ||||
Likelihood of substance abuse | 0.01 | 0.20 | ||||
Unlikely | 5804 (83) | 18.7 | 5104 (83) | 6.5 | ||
Likely | 1183 (17) | 14.8 | 1011 (17) | 5.4 | 0.14 | |
Number of prescribed outpatient psychotropic medications | <0.001 | 0.04 | ||||
0 | 4420 (63) | 16.3 | 3931 (64) | 5.9 | ||
1 | 1725 (25) | 20.4 | 1481 (24) | 7.2 | ||
2 | 781 (11) | 22.3 | 653 (11) | 7.0 | ||
>2 | 61 (1) | 23.0 | 50 (1) | 6.0 | ||
Prescribed antidepressant | 1474 (21) | 20.6 | 0.005 | 1248 (20) | 6.2 | 0.77 |
Prescribed antipsychotic | 375 (5) | 22.4 | 0.02 | 315 (5) | 7.6 | 0.34 |
Prescribed mood stabilizer | 81 (1) | 18.5 | 0.91 | 69 (1) | 4.4 | 0.49 |
Prescribed anxiolytic | 1814 (26) | 21.8 | <0.001 | 1537 (25) | 7.7 | 0.01 |
Prescribed stimulant | 101 (2) | 26.7 | 0.02 | 83 (1) | 10.8 | 0.09 |
Prescribed pharmacologic treatment for substance abuse | 79 (1) | 25.3 | 0.09 | 60 (1) | 1.7 | 0.14 |
Number of psychiatric diagnoses on outpatient problem list | 0.31 | 0.74 | ||||
0 | 6405 (92) | 18.2 | 5509 (90) | 6.3 | ||
1 or more | 582 (8) | 16.5 | 474 (8) | 7.0 | ||
Outpatient diagnosis of substance abuse | 159 (2) | 13.2 | 0.11 | 144 (2) | 4.2 | 0.28 |
Outpatient diagnosis of any psychiatric illness | 582 (8) | 16.5 | 0.31 | 517 (8) | 8.0 | 0.73 |
Discharge diagnosis of depression | 774 (11) | 17.7 | 0.80 | 690 (11) | 7.7 | 0.13 |
Discharge diagnosis of schizophrenia | 56 (1) | 23.2 | 0.31 | 50 (1) | 14 | 0.03 |
Discharge diagnosis of bipolar disorder | 101 (1) | 10.9 | 0.06 | 92 (2) | 2.2 | 0.10 |
Discharge diagnosis of anxiety | 1192 (17) | 15.0 | 0.003 | 1080 (18) | 6.2 | 0.83 |
Discharge diagnosis of substance abuse | 885 (13) | 14.8 | 0.008 | 803 (13) | 6.1 | 0.76 |
Discharge diagnosis of any psychiatric illness | 1839 (26) | 16.0 | 0.008 | 1654 (27) | 6.6 | 0.63 |
Substance abuse consultation as inpatient | 284 (4) | 14.4 | 0.11 | 252 (4) | 3.6 | 0.07 |
In bivariate analysis (Table 2), likelihood of psychiatric illness (P<0.01) and increasing numbers of prescribed outpatient psychiatric medications (P<0.01) were significantly associated with ACR. In multivariate analysis, each additional prescribed outpatient psychiatric medication increased ACR risk (odds ratio [OR]: 1.10, 95% confidence interval [CI]: 1.01‐1.20) or any prescription of an anxiolytic in particular (OR: 1.16, 95% CI: 1.001.35) was associated with increased risk of ACR, whereas discharge diagnoses of anxiety (OR: 0.82, 95% CI: 0.68‐0.99) and substance abuse (OR: 0.80, 95% CI: 0.65‐0.99) were associated with lower risk of ACR (Table 3).
ACR, OR (95% CI) | PAR, OR (95% CI)a | |
---|---|---|
| ||
Likely psychiatric disease | 0.97 (0.82‐1.14) | 1.20 (0.92‐1.56) |
Likely and possible psychiatric disease | 1.07 (0.94‐1.22) | 1.18 (0.94‐1.47) |
Likely substance abuse | 0.83 (0.69‐0.99) | 0.85 (0.63‐1.16) |
Psychiatric diagnosis on outpatient problem list | 0.97 (0.76‐1.23) | 1.04 (0.70‐1.55) |
Substance abuse diagnosis on outpatient problem list | 0.63 (0.39‐1.02) | 0.65 (0.28‐1.52) |
Increasing number of prescribed psychiatric medications | 1.10 (1.01‐1.20) | 1.00 (0.86‐1.16) |
Outpatient prescription for antidepressant | 1.10 (0.94‐1.29) | 0.86 (0.66‐1.13) |
Outpatient prescription for antipsychotic | 1.03 (0.79‐1.34) | 0.93 (0.59‐1.45) |
Outpatient prescription for anxiolytic | 1.16 (1.001.35) | 1.13 (0.88‐1.44) |
Outpatient prescription for methadone or buprenorphine | 1.15 (0.67‐1.98) | 0.18 (0.03‐1.36) |
Discharge diagnosis of depression | 1.06 (0.86‐1.30) | 1.49 (1.09‐2.04) |
Discharge diagnosis of schizophrenia | 1.43 (0.75‐2.74) | 2.63 (1.13‐6.13) |
Discharge diagnosis of bipolar disorder | 0.53 (0.28‐1.02) | 0.35 (0.09‐1.45) |
Discharge diagnosis of anxiety | 0.82 (0.68‐0.99) | 1.11 (0.83‐1.49) |
Discharge diagnosis of substance abuse | 0.80 (0.65‐0.99) | 1.05 (0.75‐1.46) |
Discharge diagnosis of any psychiatric illness | 0.88 (0.75‐1.02) | 1.22 (0.96‐1.56) |
Addiction team consult while inpatient | 0.82 (0.58‐1.17) | 0.58 (0.29‐1.17) |
Potentially Avoidable Readmissions
After further exclusion of 872 patients who had unavoidable readmissions according to the SQLape algorithm, 6115 patients remained. Of these, 388 had a PAR within 30 days (6.3%, Table 1).
In bivariate analysis (Table 2), the likelihood of psychiatric illness (P=0.02), number of outpatient psychiatric medications (P=0.04), and prescription of anxiolytics (P=0.01) were significantly associated with PAR, as they were with ACR. A discharge diagnosis of schizophrenia was also associated with PAR (P=0.03).
In multivariate analysis, only discharge diagnoses of depression (OR: 1.49, 95% CI: 1.09‐2.04) and schizophrenia (OR: 2.63, 95% CI: 1.13‐6.13) were associated with PAR.
DISCUSSION
Comorbid psychiatric illness was common among patients admitted to the medicine wards. Patients with documented discharge diagnoses of depression or schizophrenia were at highest risk for a potentially avoidable 30‐day readmission, whereas those prescribed more psychiatric medications were at increased risk for ACR. These findings were independent of a comprehensive set of risk factors among medicine inpatients in this retrospective cohort study.
This study extends prior work indicating patients with psychiatric disease have increased healthcare utilization,[6, 7, 8, 9, 10, 11] by identifying at least 2 subpopulations of the psychiatrically ill (those with depression and schizophrenia) at particularly high risk for 30‐day PAR. To our knowledge, this is the first study to identify schizophrenia as a predictor of hospital readmission for medical illnesses. One prior study prospectively identified depression as increasing the 90‐day risk of readmission 3‐fold, although medication usage was not assessed,[6] and our report strengthens this association.
There are several possible explanations why these two subpopulations in particular would be more predisposed to readmissions that are potentially avoidable. It is known that patients with schizophrenia, for example, live on average 20 years less than the general population, and most of this excess mortality is due to medical illnesses.[20, 21] Reasons for this may include poor healthcare access, adverse effects of medication, and socioeconomic factors among others.[21, 22] All of these reasons may contribute to the increased PAR risk in this population, mediated, for example, by decreased ability to adhere to postdischarge care plans. Successful community‐based interventions to decrease these inequities have been described and could serve as a model for addressing the increased readmission risk in this population.[23]
Our finding that patients with a greater number of prescribed psychiatric medications are at increased risk for ACR may be expected, given other studies that have highlighted the crucial importance of medications in postdischarge adverse events, including readmissions.[24] Indeed, medication‐related errors and toxicities are the most common postdischarge adverse events experienced by patients.[25] Whether psychiatric medications are particularly prone to causing postdischarge adverse events or whether these medications represent greater psychiatric comorbidity cannot be answered by this study.
It was surprising but reassuring that substance abuse was not a predictor of short‐term readmissions as identified using our measures; in fact, a discharge diagnosis of substance abuse was associated with lower risk of ACR than comparator patients. It seems unlikely that we would have inadequate power to find such a result, as we found a statistically significant negative association in the ACR population, and 17% of our population overall was considered likely to have a substance abuse comorbidity. However, it is likely the burden of disease was underestimated given that we did not try to determine the contribution of long‐term substance abuse to medical diseases that may increase readmission risk (eg, liver cirrhosis from alcohol use). Unlike other conditions in our study, patients with substance abuse diagnoses at BWH can be seen by a dedicated multidisciplinary team while an inpatient to start treatment and plan for postdischarge follow‐up; this may have played a role in our findings.
A discharge diagnosis of anxiety was also somewhat protective against readmission, whereas a prescription of an anxiolytic (predominantly benzodiazepines) increased risk; many patients prescribed a benzodiazepine do not have a Diagnostic and Statistical Manual of Mental Disorders4th Edition (DSM‐IV) diagnosis of anxiety disorder, and thus these findings may reflect different patient populations. Discharging physicians may have used anxiety as a discharge diagnosis in patients in whom they suspected somatic complaints without organic basis; these patients may be at lower risk of readmission.
Discharge diagnoses of psychiatric illnesses were associated with ACR and PAR in our study, but outpatient diagnoses were not. This likely reflects greater severity of illness (documentation as a treated diagnosis on discharge indicates the illness was relevant during the hospitalization), but may also reflect inaccuracies of diagnosis and lack of assessment of severity in outpatient coding, which would bias toward null findings. Although many of the patients in our study were seen by primary care doctors within the Partners system, some patients had outside primary care physicians and we did not have access to these records. This may also have decreased our ability to find associations.
The findings of our study should be interpreted in the context of the study design. Our study was retrospective, which limited our ability to conclusively diagnose psychiatric disease presence or severity (as is true of most institutions, validated psychiatric screening was not routinely used at our institutions on hospital admission or discharge). However, we used a conservative scale to classify the likelihood of patients having psychiatric or substance abuse disorders, and we used other metrics to establish the presence of illness, such as the number of prescribed medications, inpatient consultation with a substance abuse service, and hospital discharge diagnoses. This approach also allowed us to quickly identify a large cohort unaffected by selection bias. Our study was single center, potentially limiting generalizability. Although we capture at least 80% of readmissions, we were not able to capture all readmissions, and we cannot rule out that patients readmitted elsewhere are different than those readmitted within the Partners system. Last, the SQLape algorithm is not perfectly sensitive or specific in identifying avoidable readmissions,[13] but it does eliminate many readmissions that are clearly unavoidable, creating an enriched cohort of patients whose readmissions are more likely to be avoidable and therefore potentially actionable.
We suggest that our study findings first be considered when risk stratifying patients before hospital discharge in terms of readmission risk. Patients with depression and schizophrenia would seem to merit postdischarge interventions to decrease their potentially avoidable readmissions. Compulsory community treatment (a feature of treatment in Canada and Australia that is ordered by clinicians) has been shown to decrease mortality due to medical illness in patients who have been hospitalized and are psychiatrically ill, and addition of these services to postdischarge care may be useful.[23] Inpatient physicians could work to ensure follow‐up not just with medical providers but with robust outpatient mental health programs to decrease potentially avoidable readmission risk, and administrators could work to ensure close linkages with these community resources. Studies evaluating the impact of these types of interventions would need to be conducted. Patients with polypharmacy, including psychiatric medications, may benefit from interventions to improve medication safety, such as enhanced medication reconciliation and pharmacist counseling.[26]
Our study suggests that patients with depression, those with schizophrenia, and those who have increased numbers of prescribed psychiatric medications should be considered at high risk for readmission for medical illnesses. Targeting interventions to these patients may be fruitful in preventing avoidable readmissions.
Acknowledgements
The authors thank Dr. Yves Eggli for screening the database for potentially avoidable readmissions using the SQLape algorithm.
Disclosures
Dr. Donz was supported by the Swiss National Science Foundation and the Swiss Foundation for MedicalBiological Scholarships. The authors otherwise have no conflicts of interest to disclose. The content is solely the responsibility of the authors and does not necessarily represent the official views of the US Department of Veterans Affairs.
Readmissions to the hospital are common and costly.[1] However, identifying patients prospectively who are likely to be readmitted and who may benefit from interventions to reduce readmission risk has proven challenging, with published risk scores having only moderate ability to discriminate between patients likely and unlikely to be readmitted.[2] One reason for this may be that published studies have not typically focused on patients who are cognitively impaired, psychiatrically ill, have low health or English literacy, or have poor social supports, all of whom may represent a substantial fraction of readmitted patients.[2, 3, 4, 5]
Psychiatric disease, in particular, may contribute to increased readmission risk for nonpsychiatric (medical) illness, and is associated with increased utilization of healthcare resources.[6, 7, 8, 9, 10, 11] For example, patients with mental illness who were discharged from New York hospitals were more likely to be rehospitalized and had more costly readmissions than patients without these comorbidities, including a length of stay nearly 1 day longer on average.[7] An unmet need for treatment of substance abuse was projected to cost Tennessee $772 million of excess healthcare costs in 2000, mostly incurred through repeat hospitalizations and emergency department (ED) visits.[10]
Despite this, few investigators have considered the role of psychiatric disease and/or substance abuse in medical readmission risk. The purpose of the current study was to evaluate the role of psychiatric illness and substance abuse in unselected medical patients to determine their relative contributions to 30‐day all‐cause readmissions (ACR) and potentially avoidable readmissions (PAR).
METHODS
Patients and Setting
We conducted a retrospective cohort study of consecutive adult patients discharged from medicine services at Brigham and Women's Hospital (BWH), a 747‐bed tertiary referral center and teaching hospital, between July 1, 2009 and June 30, 2010. Most patients are cared for by resident housestaff teams at BWH (approximately 25% are cared for by physician assistants working directly with attending physicians), and approximately half receive primary care in the Partners system, which has a shared electronic medical record (EMR). Outpatient mental health services are provided by Partners‐associated mental health professionals including those at McLean Hospital and MassHealth (Medicaid)‐associated sites through the Massachusetts Behavioral Health Partnership. Exclusion criteria were death in the hospital or discharge to another acute care facility. We also excluded patients who left against medical advice (AMA). The study protocol was approved by the Partners Institutional Review Board.
Outcome
The primary outcomes were ACR and PAR within 30 days of discharge. First, we identified all 30‐day readmissions to BWH or to 2 other hospitals in the Partners Healthcare Network (previous studies have shown that 80% of all readmitted patients are readmitted to 1 of these 3 hospitals).[12] For patients with multiple readmissions, only the first readmission was included in the dataset.
To find potentially avoidable readmissions, administrative and billing data for these patients were processed using the SQLape (SQLape s.a.r.l., Corseaux, Switzerland) algorithm, which identifies PAR by excluding patients who undergo planned follow‐up treatment (such as a cycle of planned chemotherapy) or are readmitted for conditions unrelated in any way to the index hospitalization.[13, 14] Common complications of treatment are categorized as potentially avoidable, such as development of a deep venous thrombosis, a decubitus ulcer after prolonged bed rest, or bleeding complications after starting anticoagulation. Although the algorithm identifies theoretically preventable readmissions, the algorithm does not quantify how preventable they are, and these are thus referred to as potentially avoidable. This is similar to other admission metrics, such as the Agency for Healthcare Research and Quality's prevention quality indicators, which are created from a list of ambulatory care‐sensitive conditions.[15] SQLape has the advantage of being a specific tool for readmissions. Patients with 30‐day readmissions identified by SQLape as planned or unlikely to be avoidable were excluded in the PAR analysis, although still included in ACR analysis. In each case, the comparison group is patients without any readmission.
Predictors
Our predictors of interest included the overall prevalence of a psychiatric diagnosis or diagnosis of substance abuse, the presence of specific psychiatric diagnoses, and prescription of psychiatric medications to help assess the independent contribution of these comorbidities to readmission risk.
We used a combination of easily obtainable inpatient and outpatient clinical and administrative data to identify relevant patients. Patients were considered likely to be psychiatrically ill if they: (1) had a psychiatric diagnosis on their Partners outpatient EMR problem list and were prescribed a medication to treat that condition as an outpatient, or (2) had an International Classification of Diseases, 9th Revision diagnosis of a psychiatric illness at hospital discharge. Patients were considered to have moderate probability of disease if they: (1) had a psychiatric diagnosis on their outpatient problem list, or (2) were prescribed a medication intended to treat a psychiatric condition as an outpatient. Patients were considered unlikely to have psychiatric disease if none of these criteria were met. Patients were considered likely to have a substance abuse disorder if they had this diagnosis on their outpatient EMR, or were prescribed a medication to treat this condition (eg, buprenorphine/naloxone), or received inpatient consultation from a substance abuse treatment team during their inpatient hospitalization, and were considered unlikely if none of these were true. We also evaluated individual categories of psychiatric illness (schizophrenia, depression, anxiety, bipolar disorder) and of psychotropic medications (antidepressants, antipsychotics, anxiolytics).
Potential Confounders
Data on potential confounders, based on prior literature,[16, 17] collected at the index admission were derived from electronic administrative, clinical, and billing sources, including the Brigham Integrated Computer System and the Partners Clinical Data Repository. They included patient age, gender, ethnicity, primary language, marital status, insurance status, living situation prior to admission, discharge location, length of stay, Elixhauser comorbidity index,[18] total number of medications prescribed, and number of prior admissions and ED visits in the prior year.
Statistical Analysis
Bivariate comparisons of each of the predictors of ACR and PAR risk (ie, patients with a 30‐day ACR or PAR vs those not readmitted within 30 days) were conducted using 2 trend tests for ordinal predictors (eg, likelihood of psychiatric disease), and 2 or Fisher exact test for dichotomous predictors (eg, receipt of inpatient substance abuse counseling).
We then used multivariate logistic regression analysis to adjust for all of the potential confounders noted above, entering each variable related to psychiatric illness into the model separately (eg, likely psychiatric illness, number of psychiatric medications). In a secondary analysis, we removed potentially collinear variables from the final model; as this did not alter the results, the full model is presented. We also conducted a secondary analysis where we included patients who left against medical advice (AMA), which also did not alter the results. Two‐sided P values <0.05 were considered significant, and all analyses were performed using the SAS version 9.2 (SAS Institute, Inc., Cary, NC).
RESULTS
There were 7984 unique patients discharged during the study period. Patients were generally white and English speaking; just over half of admissions came from the ED (Table 1). Of note, nearly all patients were insured, as are almost all patients in Massachusetts. They had high degrees of comorbid illness and large numbers of prescribed medications. Nearly 30% had at least 1 hospital admission within the prior year.
Characteristic | All Patients, N (%) | Not Readmitted, N (%) | ACR, N (%) | PAR N (%)a |
---|---|---|---|---|
| ||||
Study cohort | 6987 (100) | 5727 (72) | 1260 (18) | 388 (5.6) |
Age, y | ||||
<50 | 1663 (23.8) | 1343 (23.5) | 320 (25.4) | 85 (21.9) |
5165 | 2273 (32.5) | 1859 (32.5) | 414 (32.9) | 136 (35.1) |
6679 | 1444 (20.7) | 1176 (20.5) | 268 (18.6) | 80 (20.6) |
>80 | 1607 (23.0) | 1349 (23.6) | 258 (16.1) | 87 (22.4) |
Female | 3604 (51.6) | 2967 (51.8) | 637 (50.6) | 206 (53.1) |
Race | ||||
White | 5126 (73.4) | 4153 (72.5) | 973 (77.2) | 300 (77.3) |
Black | 1075 (15.4) | 899 (15.7) | 176 (14.0) | 53 (13.7) |
Hispanic | 562 (8.0) | 477 (8.3) | 85 (6.8) | 28 (7.2) |
Other | 224 (3.2) | 198 (3.5) | 26 (2.1) | 7 (1.8) |
Primary language | ||||
English | 6345 (90.8) | 5180 (90.5) | 1165 (92.5) | 356 (91.8) |
Marital status | ||||
Married | 3642 (52.1) | 2942 (51.4) | 702 (55.7) | 214 (55.2) |
Single, never married | 1662 (23.8) | 1393 (24.3) | 269 (21.4) | 73 (18.8) |
Previously married | 1683 (24.1) | 1386 (24.2) | 289 (22.9) | 101 (26.0) |
Insurance | ||||
Medicare | 3550 (50.8) | 2949 (51.5) | 601 (47.7) | 188 (48.5) |
Medicaid | 539 (7.7) | 430 (7.5) | 109 (8.7) | 33 (8.5) |
Private | 2892 (41.4) | 2344 (40.9) | 548 (43.5) | 167 (43.0) |
Uninsured | 6 (0.1) | 4 (0.1) | 2 (0.1) | 0 (0) |
Source of index admission | ||||
Clinic or home | 2136 (30.6) | 1711 (29.9) | 425 (33.7) | 117 (30.2) |
Emergency department | 3592 (51.4) | 2999 (52.4) | 593 (47.1) | 181 (46.7) |
Nursing facility | 1204 (17.2) | 977 (17.1) | 227 (18.0) | 84 (21.7) |
Other | 55 (0.1) | 40 (0.7) | 15 (1.1) | 6 (1.6) |
Length of stay, d | ||||
02 | 1757 (25.2) | 1556 (27.2) | 201 (16.0) | 55 (14.2) |
34 | 2200 (31.5) | 1842 (32.2) | 358 (28.4) | 105 (27.1) |
57 | 1521 (21.8) | 1214 (21.2) | 307 (24.4) | 101 (26.0) |
>7 | 1509 (21.6) | 1115 (19.5) | 394 (31.3) | 127 (32.7) |
Elixhauser comorbidity index score | ||||
01 | 1987 (28.4) | 1729 (30.2) | 258 (20.5) | 66 (17.0) |
27 | 1773 (25.4) | 1541 (26.9) | 232 (18.4) | 67 (17.3) |
813 | 1535 (22.0) | 1212 (21.2) | 323 (25.6) | 86 (22.2) |
>13 | 1692 (24.2) | 1245 (21.7) | 447 (35.5) | 169 (43.6) |
Medications prescribed as outpatient | ||||
06 | 1684 (24.1) | 1410 (24.6) | 274 (21.8) | 72 (18.6) |
79 | 1601 (22.9) | 1349 (23.6) | 252 (20.0) | 77 (19.9) |
1013 | 1836 (26.3) | 1508 (26.3) | 328 (26.0) | 107 (27.6) |
>13 | 1866 (26.7) | 1460 (25.5) | 406 (32.2) | 132 (34.0) |
Number of admissions in past year | ||||
0 | 4816 (68.9) | 4032 (70.4) | 784 (62.2) | 279 (71.9) |
15 | 2075 (29.7) | 1640 (28.6) | 435 (34.5) | 107 (27.6) |
>5 | 96 (1.4) | 55 (1.0) | 41 (3.3) | 2 (0.5) |
Number of ED visits in past year | ||||
0 | 4661 (66.7) | 3862 (67.4) | 799 (63.4) | 261 (67.3) |
15 | 2326 (33.3) | 1865 (32.6) | 461 (36.6) | 127 (32.7) |
All‐Cause Readmissions
After exclusion of 997 patients who died, were discharged to skilled nursing or rehabilitation facilities, or left AMA, 6987 patients were included (Figure 1). Of these, 1260 had a readmission (18%). Approximately half were considered unlikely to be psychiatrically ill, 22% were considered moderately likely, and 29% likely (Table 2).
All‐Cause Readmission Analysis | Potentially Avoidable Readmission Analysis | |||||
---|---|---|---|---|---|---|
No. in Cohort (%) | % of Patients With ACR | P Valuea | No. in Cohort (%) | % of Patients With PAR | P Valuea | |
| ||||||
Entire cohort | 6987 | 18.0 | 6115 | 6.3 | ||
Likelihood of psychiatric illness | ||||||
Unlikely | 3424 (49) | 16.5 | 3026 (49) | 5.6 | ||
Moderate | 1564 (22) | 23.5 | 1302 (21) | 7.1 | ||
Likely | 1999 (29) | 16.4 | 1787 (29) | 6.4 | ||
Likely versus unlikely | 0.87 | 0.20 | ||||
Moderate+likely versus unlikely | 0.001 | 0.02 | ||||
Likelihood of substance abuse | 0.01 | 0.20 | ||||
Unlikely | 5804 (83) | 18.7 | 5104 (83) | 6.5 | ||
Likely | 1183 (17) | 14.8 | 1011 (17) | 5.4 | 0.14 | |
Number of prescribed outpatient psychotropic medications | <0.001 | 0.04 | ||||
0 | 4420 (63) | 16.3 | 3931 (64) | 5.9 | ||
1 | 1725 (25) | 20.4 | 1481 (24) | 7.2 | ||
2 | 781 (11) | 22.3 | 653 (11) | 7.0 | ||
>2 | 61 (1) | 23.0 | 50 (1) | 6.0 | ||
Prescribed antidepressant | 1474 (21) | 20.6 | 0.005 | 1248 (20) | 6.2 | 0.77 |
Prescribed antipsychotic | 375 (5) | 22.4 | 0.02 | 315 (5) | 7.6 | 0.34 |
Prescribed mood stabilizer | 81 (1) | 18.5 | 0.91 | 69 (1) | 4.4 | 0.49 |
Prescribed anxiolytic | 1814 (26) | 21.8 | <0.001 | 1537 (25) | 7.7 | 0.01 |
Prescribed stimulant | 101 (2) | 26.7 | 0.02 | 83 (1) | 10.8 | 0.09 |
Prescribed pharmacologic treatment for substance abuse | 79 (1) | 25.3 | 0.09 | 60 (1) | 1.7 | 0.14 |
Number of psychiatric diagnoses on outpatient problem list | 0.31 | 0.74 | ||||
0 | 6405 (92) | 18.2 | 5509 (90) | 6.3 | ||
1 or more | 582 (8) | 16.5 | 474 (8) | 7.0 | ||
Outpatient diagnosis of substance abuse | 159 (2) | 13.2 | 0.11 | 144 (2) | 4.2 | 0.28 |
Outpatient diagnosis of any psychiatric illness | 582 (8) | 16.5 | 0.31 | 517 (8) | 8.0 | 0.73 |
Discharge diagnosis of depression | 774 (11) | 17.7 | 0.80 | 690 (11) | 7.7 | 0.13 |
Discharge diagnosis of schizophrenia | 56 (1) | 23.2 | 0.31 | 50 (1) | 14 | 0.03 |
Discharge diagnosis of bipolar disorder | 101 (1) | 10.9 | 0.06 | 92 (2) | 2.2 | 0.10 |
Discharge diagnosis of anxiety | 1192 (17) | 15.0 | 0.003 | 1080 (18) | 6.2 | 0.83 |
Discharge diagnosis of substance abuse | 885 (13) | 14.8 | 0.008 | 803 (13) | 6.1 | 0.76 |
Discharge diagnosis of any psychiatric illness | 1839 (26) | 16.0 | 0.008 | 1654 (27) | 6.6 | 0.63 |
Substance abuse consultation as inpatient | 284 (4) | 14.4 | 0.11 | 252 (4) | 3.6 | 0.07 |
In bivariate analysis (Table 2), likelihood of psychiatric illness (P<0.01) and increasing numbers of prescribed outpatient psychiatric medications (P<0.01) were significantly associated with ACR. In multivariate analysis, each additional prescribed outpatient psychiatric medication increased ACR risk (odds ratio [OR]: 1.10, 95% confidence interval [CI]: 1.01‐1.20) or any prescription of an anxiolytic in particular (OR: 1.16, 95% CI: 1.001.35) was associated with increased risk of ACR, whereas discharge diagnoses of anxiety (OR: 0.82, 95% CI: 0.68‐0.99) and substance abuse (OR: 0.80, 95% CI: 0.65‐0.99) were associated with lower risk of ACR (Table 3).
ACR, OR (95% CI) | PAR, OR (95% CI)a | |
---|---|---|
| ||
Likely psychiatric disease | 0.97 (0.82‐1.14) | 1.20 (0.92‐1.56) |
Likely and possible psychiatric disease | 1.07 (0.94‐1.22) | 1.18 (0.94‐1.47) |
Likely substance abuse | 0.83 (0.69‐0.99) | 0.85 (0.63‐1.16) |
Psychiatric diagnosis on outpatient problem list | 0.97 (0.76‐1.23) | 1.04 (0.70‐1.55) |
Substance abuse diagnosis on outpatient problem list | 0.63 (0.39‐1.02) | 0.65 (0.28‐1.52) |
Increasing number of prescribed psychiatric medications | 1.10 (1.01‐1.20) | 1.00 (0.86‐1.16) |
Outpatient prescription for antidepressant | 1.10 (0.94‐1.29) | 0.86 (0.66‐1.13) |
Outpatient prescription for antipsychotic | 1.03 (0.79‐1.34) | 0.93 (0.59‐1.45) |
Outpatient prescription for anxiolytic | 1.16 (1.001.35) | 1.13 (0.88‐1.44) |
Outpatient prescription for methadone or buprenorphine | 1.15 (0.67‐1.98) | 0.18 (0.03‐1.36) |
Discharge diagnosis of depression | 1.06 (0.86‐1.30) | 1.49 (1.09‐2.04) |
Discharge diagnosis of schizophrenia | 1.43 (0.75‐2.74) | 2.63 (1.13‐6.13) |
Discharge diagnosis of bipolar disorder | 0.53 (0.28‐1.02) | 0.35 (0.09‐1.45) |
Discharge diagnosis of anxiety | 0.82 (0.68‐0.99) | 1.11 (0.83‐1.49) |
Discharge diagnosis of substance abuse | 0.80 (0.65‐0.99) | 1.05 (0.75‐1.46) |
Discharge diagnosis of any psychiatric illness | 0.88 (0.75‐1.02) | 1.22 (0.96‐1.56) |
Addiction team consult while inpatient | 0.82 (0.58‐1.17) | 0.58 (0.29‐1.17) |
Potentially Avoidable Readmissions
After further exclusion of 872 patients who had unavoidable readmissions according to the SQLape algorithm, 6115 patients remained. Of these, 388 had a PAR within 30 days (6.3%, Table 1).
In bivariate analysis (Table 2), the likelihood of psychiatric illness (P=0.02), number of outpatient psychiatric medications (P=0.04), and prescription of anxiolytics (P=0.01) were significantly associated with PAR, as they were with ACR. A discharge diagnosis of schizophrenia was also associated with PAR (P=0.03).
In multivariate analysis, only discharge diagnoses of depression (OR: 1.49, 95% CI: 1.09‐2.04) and schizophrenia (OR: 2.63, 95% CI: 1.13‐6.13) were associated with PAR.
DISCUSSION
Comorbid psychiatric illness was common among patients admitted to the medicine wards. Patients with documented discharge diagnoses of depression or schizophrenia were at highest risk for a potentially avoidable 30‐day readmission, whereas those prescribed more psychiatric medications were at increased risk for ACR. These findings were independent of a comprehensive set of risk factors among medicine inpatients in this retrospective cohort study.
This study extends prior work indicating patients with psychiatric disease have increased healthcare utilization,[6, 7, 8, 9, 10, 11] by identifying at least 2 subpopulations of the psychiatrically ill (those with depression and schizophrenia) at particularly high risk for 30‐day PAR. To our knowledge, this is the first study to identify schizophrenia as a predictor of hospital readmission for medical illnesses. One prior study prospectively identified depression as increasing the 90‐day risk of readmission 3‐fold, although medication usage was not assessed,[6] and our report strengthens this association.
There are several possible explanations why these two subpopulations in particular would be more predisposed to readmissions that are potentially avoidable. It is known that patients with schizophrenia, for example, live on average 20 years less than the general population, and most of this excess mortality is due to medical illnesses.[20, 21] Reasons for this may include poor healthcare access, adverse effects of medication, and socioeconomic factors among others.[21, 22] All of these reasons may contribute to the increased PAR risk in this population, mediated, for example, by decreased ability to adhere to postdischarge care plans. Successful community‐based interventions to decrease these inequities have been described and could serve as a model for addressing the increased readmission risk in this population.[23]
Our finding that patients with a greater number of prescribed psychiatric medications are at increased risk for ACR may be expected, given other studies that have highlighted the crucial importance of medications in postdischarge adverse events, including readmissions.[24] Indeed, medication‐related errors and toxicities are the most common postdischarge adverse events experienced by patients.[25] Whether psychiatric medications are particularly prone to causing postdischarge adverse events or whether these medications represent greater psychiatric comorbidity cannot be answered by this study.
It was surprising but reassuring that substance abuse was not a predictor of short‐term readmissions as identified using our measures; in fact, a discharge diagnosis of substance abuse was associated with lower risk of ACR than comparator patients. It seems unlikely that we would have inadequate power to find such a result, as we found a statistically significant negative association in the ACR population, and 17% of our population overall was considered likely to have a substance abuse comorbidity. However, it is likely the burden of disease was underestimated given that we did not try to determine the contribution of long‐term substance abuse to medical diseases that may increase readmission risk (eg, liver cirrhosis from alcohol use). Unlike other conditions in our study, patients with substance abuse diagnoses at BWH can be seen by a dedicated multidisciplinary team while an inpatient to start treatment and plan for postdischarge follow‐up; this may have played a role in our findings.
A discharge diagnosis of anxiety was also somewhat protective against readmission, whereas a prescription of an anxiolytic (predominantly benzodiazepines) increased risk; many patients prescribed a benzodiazepine do not have a Diagnostic and Statistical Manual of Mental Disorders4th Edition (DSM‐IV) diagnosis of anxiety disorder, and thus these findings may reflect different patient populations. Discharging physicians may have used anxiety as a discharge diagnosis in patients in whom they suspected somatic complaints without organic basis; these patients may be at lower risk of readmission.
Discharge diagnoses of psychiatric illnesses were associated with ACR and PAR in our study, but outpatient diagnoses were not. This likely reflects greater severity of illness (documentation as a treated diagnosis on discharge indicates the illness was relevant during the hospitalization), but may also reflect inaccuracies of diagnosis and lack of assessment of severity in outpatient coding, which would bias toward null findings. Although many of the patients in our study were seen by primary care doctors within the Partners system, some patients had outside primary care physicians and we did not have access to these records. This may also have decreased our ability to find associations.
The findings of our study should be interpreted in the context of the study design. Our study was retrospective, which limited our ability to conclusively diagnose psychiatric disease presence or severity (as is true of most institutions, validated psychiatric screening was not routinely used at our institutions on hospital admission or discharge). However, we used a conservative scale to classify the likelihood of patients having psychiatric or substance abuse disorders, and we used other metrics to establish the presence of illness, such as the number of prescribed medications, inpatient consultation with a substance abuse service, and hospital discharge diagnoses. This approach also allowed us to quickly identify a large cohort unaffected by selection bias. Our study was single center, potentially limiting generalizability. Although we capture at least 80% of readmissions, we were not able to capture all readmissions, and we cannot rule out that patients readmitted elsewhere are different than those readmitted within the Partners system. Last, the SQLape algorithm is not perfectly sensitive or specific in identifying avoidable readmissions,[13] but it does eliminate many readmissions that are clearly unavoidable, creating an enriched cohort of patients whose readmissions are more likely to be avoidable and therefore potentially actionable.
We suggest that our study findings first be considered when risk stratifying patients before hospital discharge in terms of readmission risk. Patients with depression and schizophrenia would seem to merit postdischarge interventions to decrease their potentially avoidable readmissions. Compulsory community treatment (a feature of treatment in Canada and Australia that is ordered by clinicians) has been shown to decrease mortality due to medical illness in patients who have been hospitalized and are psychiatrically ill, and addition of these services to postdischarge care may be useful.[23] Inpatient physicians could work to ensure follow‐up not just with medical providers but with robust outpatient mental health programs to decrease potentially avoidable readmission risk, and administrators could work to ensure close linkages with these community resources. Studies evaluating the impact of these types of interventions would need to be conducted. Patients with polypharmacy, including psychiatric medications, may benefit from interventions to improve medication safety, such as enhanced medication reconciliation and pharmacist counseling.[26]
Our study suggests that patients with depression, those with schizophrenia, and those who have increased numbers of prescribed psychiatric medications should be considered at high risk for readmission for medical illnesses. Targeting interventions to these patients may be fruitful in preventing avoidable readmissions.
Acknowledgements
The authors thank Dr. Yves Eggli for screening the database for potentially avoidable readmissions using the SQLape algorithm.
Disclosures
Dr. Donz was supported by the Swiss National Science Foundation and the Swiss Foundation for MedicalBiological Scholarships. The authors otherwise have no conflicts of interest to disclose. The content is solely the responsibility of the authors and does not necessarily represent the official views of the US Department of Veterans Affairs.
- Rehospitalizations among patients in the Medicare fee‐for‐service program. N Engl J Med. 2009;360(14):1418–1428. , , .
- Risk prediction models for hospital readmission: a systematic review. JAMA. 2011;306(15):1688–1698. , , , et al.
- Hospital readmission as an accountability measure. JAMA. 2011;305(5):504–505. , .
- Postdischarge environmental and socioeconomic factors and the likelihood of early hospital readmission among community‐dwelling Medicare beneficiaries. Gerontologist. 2008;48(4):495–504. , , , , , .
- Low health literacy and health outcomes: an updated systematic review. Ann Intern Med. 2011;155(2):97–107. , , , , .
- Depression is a risk factor for rehospitalization in medical inpatients. Prim Care Companion J Clin Psychiatry. 2007;9(4):256–262. , , , et al.
- Mental illness and hospitalization for ambulatory care sensitive medical conditions. Med Care. 2008;46(12):1249–1256. , , , .
- Substance use treatment barriers for patients with frequent hospital admissions. J Subst Abuse Treat. 2010;38(1):22–30. , , , , , .
- Managed care and the quality of substance abuse treatment. J Ment Health Policy Econ. 2002;5(4):163–174. , , , , .
- Unmet substance abuse treatment need, health services utilization, and cost: a population‐based emergency department study. Ann Emerg Med. 2005;45(2):118–127. , , , , .
- Elderly Medicare inpatients with substance use disorders: characteristics and predictors of hospital readmissions over a four‐year interval. J Stud Alcohol. 2000;61(6):891–895. , , , .
- Rationale and design of the Pharmacist Intervention for Low Literacy in Cardiovascular Disease (PILL‐CVD) study. Circ Cardiovasc Qual Outcomes. 2010;3(2):212–219. , , , et al.
- Validation of the potentially avoidable hospital readmission rate as a routine indicator of the quality of hospital care. Med Care. 2006;44(11);972–981. , , , , , .
- Measuring potentially avoidable hospital readmissions. J Clin Epidemiol. 2002;55:573–587. , , , , , .
- Agency for Healthcare Research and Quality Quality Indicators. (April 7, 2006). Prevention Quality Indicators (PQI) Composite Measure Workgroup Final Report. Available at: http://www.qualityindicators.ahrq.gov/modules/pqi_resources.aspx. Accessed June 1, 2012.
- Hospital readmission in general medicine patients: a prediction model. J Gen Intern Med. 2010;25(3):211–219. , , , et al.
- Derivation and validation of an index to predict early death or unplanned readmission after discharge from hospital to the community. CMAJ. 2010;182(6):551–557. , , , et al.
- Comorbidity measures for use with administrative data. Med Care. Jan 1998;36(1):8–27. , , , .
- Morbidity and mortality in people with serious mental illness. October 2006. National Association of State Mental Health Directors, Medical Directors Council. Available at: http://www.nasmhpd.org/docs/publications/MDCdocs/Mortality%20and%20 Mo rbidity%20Final%20Report%208.18.08.pdf. Accessed January 13, 2013. , , , , eds.
- Mortality in individuals who have had psychiatric treatment: population‐based study in Nova Scotia. Br J Psychiat. 2005;187:552–558. , , , .
- Inequitable access for mentally ill patients to some medically necessary procedures. CMAJ. 2007;176(6):779–784. , , , , , .
- Quality of medical care for people with and without comorbid mental illness and substance misuse: systematic review of comparative studies. Br J Psychiat. 2009;194:491–499. , , .
- Reducing all‐cause mortality among patients with psychiatric disorders: a population‐based study. CMAJ. 2013;185(1):E50–E56. , , , , , .
- Incidence of potentially avoidable urgent readmissions and their relation to all‐cause urgent readmissions. CMAJ. 2011;183(14):E1067–E1072. , , , et al.
- The incidence and severity of adverse events affecting patients after discharge from the hospital. Ann Intern Med. 2003;138(3):161–167. , , , , .
- Hospital‐based medication reconciliation practices: a systematic review. Arch Intern Med. 2012;172(14):1057–1069. , , , .
- Rehospitalizations among patients in the Medicare fee‐for‐service program. N Engl J Med. 2009;360(14):1418–1428. , , .
- Risk prediction models for hospital readmission: a systematic review. JAMA. 2011;306(15):1688–1698. , , , et al.
- Hospital readmission as an accountability measure. JAMA. 2011;305(5):504–505. , .
- Postdischarge environmental and socioeconomic factors and the likelihood of early hospital readmission among community‐dwelling Medicare beneficiaries. Gerontologist. 2008;48(4):495–504. , , , , , .
- Low health literacy and health outcomes: an updated systematic review. Ann Intern Med. 2011;155(2):97–107. , , , , .
- Depression is a risk factor for rehospitalization in medical inpatients. Prim Care Companion J Clin Psychiatry. 2007;9(4):256–262. , , , et al.
- Mental illness and hospitalization for ambulatory care sensitive medical conditions. Med Care. 2008;46(12):1249–1256. , , , .
- Substance use treatment barriers for patients with frequent hospital admissions. J Subst Abuse Treat. 2010;38(1):22–30. , , , , , .
- Managed care and the quality of substance abuse treatment. J Ment Health Policy Econ. 2002;5(4):163–174. , , , , .
- Unmet substance abuse treatment need, health services utilization, and cost: a population‐based emergency department study. Ann Emerg Med. 2005;45(2):118–127. , , , , .
- Elderly Medicare inpatients with substance use disorders: characteristics and predictors of hospital readmissions over a four‐year interval. J Stud Alcohol. 2000;61(6):891–895. , , , .
- Rationale and design of the Pharmacist Intervention for Low Literacy in Cardiovascular Disease (PILL‐CVD) study. Circ Cardiovasc Qual Outcomes. 2010;3(2):212–219. , , , et al.
- Validation of the potentially avoidable hospital readmission rate as a routine indicator of the quality of hospital care. Med Care. 2006;44(11);972–981. , , , , , .
- Measuring potentially avoidable hospital readmissions. J Clin Epidemiol. 2002;55:573–587. , , , , , .
- Agency for Healthcare Research and Quality Quality Indicators. (April 7, 2006). Prevention Quality Indicators (PQI) Composite Measure Workgroup Final Report. Available at: http://www.qualityindicators.ahrq.gov/modules/pqi_resources.aspx. Accessed June 1, 2012.
- Hospital readmission in general medicine patients: a prediction model. J Gen Intern Med. 2010;25(3):211–219. , , , et al.
- Derivation and validation of an index to predict early death or unplanned readmission after discharge from hospital to the community. CMAJ. 2010;182(6):551–557. , , , et al.
- Comorbidity measures for use with administrative data. Med Care. Jan 1998;36(1):8–27. , , , .
- Morbidity and mortality in people with serious mental illness. October 2006. National Association of State Mental Health Directors, Medical Directors Council. Available at: http://www.nasmhpd.org/docs/publications/MDCdocs/Mortality%20and%20 Mo rbidity%20Final%20Report%208.18.08.pdf. Accessed January 13, 2013. , , , , eds.
- Mortality in individuals who have had psychiatric treatment: population‐based study in Nova Scotia. Br J Psychiat. 2005;187:552–558. , , , .
- Inequitable access for mentally ill patients to some medically necessary procedures. CMAJ. 2007;176(6):779–784. , , , , , .
- Quality of medical care for people with and without comorbid mental illness and substance misuse: systematic review of comparative studies. Br J Psychiat. 2009;194:491–499. , , .
- Reducing all‐cause mortality among patients with psychiatric disorders: a population‐based study. CMAJ. 2013;185(1):E50–E56. , , , , , .
- Incidence of potentially avoidable urgent readmissions and their relation to all‐cause urgent readmissions. CMAJ. 2011;183(14):E1067–E1072. , , , et al.
- The incidence and severity of adverse events affecting patients after discharge from the hospital. Ann Intern Med. 2003;138(3):161–167. , , , , .
- Hospital‐based medication reconciliation practices: a systematic review. Arch Intern Med. 2012;172(14):1057–1069. , , , .
Copyright © 2013 Society of Hospital Medicine
Moving Beyond Readmission Penalties
Containing the rise of healthcare costs has taken on a new sense of urgency in the wake of the recent economic recession and continued growth in the cost of healthcare. Accordingly, many stakeholders seek solutions to improve value (reducing costs while improving care)[1]; hospital readmissions, which are common and costly,[2] have emerged as a key target. The Centers for Medicare and Medicaid Services (CMS) have instituted several programs intended to reduce readmissions, including funding for community‐based, care‐transition programs; penalties for hospitals with elevated risk‐adjusted readmission rates for selected diagnoses; pioneer Accountable Care Organizations (ACOs) with incentives to reduce global costs of care; and Hospital Engagement Networks (HENs) through the Partnership for Patients.[3] A primary aim of these initiatives is to enhance the quality of care transitions as patients are discharged from the hospital.
Though the recent focus on hospital readmissions has appropriately drawn attention to transitions in care, some have expressed concerns. Among these are questions about: 1) the extent to which readmissions truly reflect the quality of hospital care[4]; 2) the preventability of readmissions[5]; 3) limitations in risk‐adjustment techniques[6]; and 4) best practices for preventing readmissions.[7] We believe these concerns stem in part from deficiencies in the state of the science of transitional care, and that future efforts in this area will be hindered without a clear vision of an ideal transition in care. We propose the key components of an ideal transition in care and discuss the implications of this concept as it pertains to hospital readmissions.
THE IDEAL TRANSITION IN CARE
We propose the key components of an ideal transition in care in Figure 1 and Table 1. Figure 1 represents 10 domains described more fully below as structural supports of the bridge patients must cross from one care environment to another during a care transition. This figure highlights key domains and suggests that lack of a domain makes the bridge weaker and more prone to gaps in care and poor outcomes. It also implies that the more components are missing, the less safe is the bridge or transition. Those domains that mainly take place prior to discharge are placed closer to the hospital side of the bridge, those that mainly take place after discharge are placed closer to the community side of the bridge, while those that take place both prior to and after discharge are in the middle. Table 1 provides descriptions of the key content for each of these domains, as well as guidance about which personnel might be involved and where in the transition process that domain should be implemented. We support these domains with supporting evidence where available.
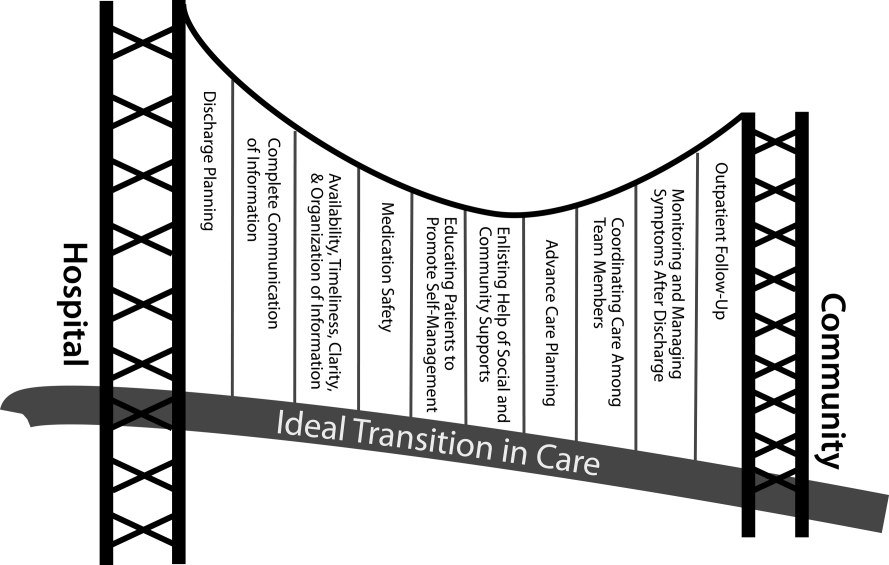
Domain | Who | When | References |
---|---|---|---|
| |||
Discharge planning | |||
Use a multidisciplinary team to create a discharge plan | Discharging clinician | Predischarge | 911 |
Collaborate with PCP regarding discharge and follow‐up plan | Care managers/discharge planners | ||
Arrange follow‐up appointments prior to discharge | Nurses | ||
Make timely appointments for follow‐up care | |||
Make appointments that take patient and caregiver's schedules and transportation needs into account | |||
Complete communication of information | |||
Includes: | Discharging clinician | Time of discharge | 1214 |
Patient's full name | |||
Age | |||
Dates of admission and discharge | |||
Names of responsible hospital physicians | |||
Name of physician preparing discharge summary | |||
Name of PCP | |||
Main diagnosis | |||
Other relevant diagnoses, procedures, and complications | |||
Relevant findings at admission | |||
Treatment and response for each active problem | |||
Results of procedures and abnormal laboratory test results | |||
Recommendations of any subspecialty consultants | |||
Patient's functional status at discharge | |||
Discharge medications | |||
Follow‐up appointments made and those to be made | |||
Tests to be ordered and pending tests to be followed‐up | |||
Counseling provided to patient and caregiver, when applicable | |||
Contingency planning | |||
Code status | |||
Availability, timeliness, clarity, and organization of information | |||
Timely communication with postdischarge providers verbally (preferred) or by fax/e‐mail | Discharging clinician | Time of discharge | 1214 |
Timely completion of discharge summary and reliable transmission to postdischarge providers | |||
Availability of information in medical record | |||
Use of a structured template with subheadings in discharge communication | |||
Medication safety | |||
Take an accurate preadmission medication history | Clinicians | Admission | 1521 |
Reconcile preadmission medications with all ordered medications at all transfers in care, including discharge | Pharmacists | Throughout hospitalization | |
Communicate discharge medications to all outpatient providers, including all changes and rationale for those changes | Nurses | Time of discharge | |
Educating patients, promoting self‐management | |||
Focus discharge counseling on major diagnoses, medication changes, dates of follow‐up appointments, self‐care instructions, warning signs and symptoms, and who to contact for problems | Clinicians | Daily | 911, 2228, 30 |
Include caregivers as appropriate | Nurses | Time of discharge | |
Ensure staff members provide consistent messages | Care managers/discharge planners | Postdischarge | |
Provide simply written patient‐centered materials with instructions | Transition coaches | ||
Use teach‐back methods to confirm understanding | |||
Encourage questions | |||
Continue teaching during postdischarge follow‐up | |||
Use transition coaches in high‐risk patients: focus on medication management, keeping a personal medical record, follow‐up appointments, and knowledge of red flags | |||
Enlisting help of social and community supports | |||
Assess needs and appropriately arrange for home services | Clinicians | Predischarge and postdischarge | 29, 30 |
Enlist help of caregivers | Nurses | ||
Enlist help of community supports | Care managers | ||
Home health staff | |||
Advanced care planning | |||
Establish healthcare proxy | Clinicians | Predischarge and postdischarge | 31, 32 |
Discuss goals of care | Palliative care staff | ||
Palliative care consultation (if appropriate) | Social workers | ||
Enlist hospice services (if appropriate) | Nurses | ||
Hospice workers | |||
Coordinating care among team members | |||
Share medical records | Clinicians | Predischarge and postdischarge | 33 |
Communicate involving all team members | Nurses | ||
Optimize continuity of providers and formal handoffs of care | Office personnel | ||
IT staff | |||
Monitoring and managing symptoms after discharge | |||
Monitor for: | Clinicians | Postdischarge | 1113, 28, 3436 |
Worsening disease control | Nurses | ||
Medication side effects, discrepancies, nonadherence | Pharmacists | ||
Therapeutic drug monitoring | Care managers | ||
Inability to manage conditions at home | Visiting nurses and other home health staff | ||
Via: | |||
Postdischarge phone calls | |||
Home visits | |||
Postdischarge clinic visits | |||
Patient hotline | |||
Availability of inpatient providers after discharge | |||
Follow‐up with outpatient providers | |||
Within an appropriate time frame (eg, 7 d or sooner for high‐risk patients) | Clinicians | Postdischarge | 3740 |
With appropriate providers (eg, most related to reasons for hospitalization, who manage least stable conditions, and/or PCP) | Nurses Pharmacists | ||
Utilize multidisciplinary teams as appropriate | Care managers | ||
Ensure appropriate progress along plan of care and safe transition | Office personnel | ||
Other clinical staff as appropriate |
Our concept of an ideal transition in care began with work by Naylor, who described several important components of a safe transition in care, including complete communication of information, patient education, enlisting the help of social and community supports, ensuring continuity of care, and coordinating care among team members.[8] It is supplemented by the Transitions of Care Consensus Policy Statement proposed by representatives from hospital medicine, primary care, and emergency medicine, which emphasized aspects of timeliness and content of communication between providers.[9] Our present articulation of these key components includes 10 organizing domains.
The Discharge Planning domain highlights the important principle of planning ahead for hospital discharge while the patient is still being treated in the hospital, a paradigm espoused by Project RED[10] and other successful care transitions interventions.[11, 12] Collaborating with the outpatient provider and taking the patient and caregiver's preferences for appointment scheduling into account can help ensure optimal outpatient follow‐up.
Complete Communication of Information refers to the content that should be included in discharge summaries and other means of information transfer from hospital to postdischarge care. The specific content areas are based on the Society of Hospital Medicine and Society of General Internal Medicine Continuity of Care Task Force systematic review and recommendations,[13] which takes into account information requested by primary care physicians after discharge.
Availability, Timeliness, Clarity, and Organization of that information is as important as the content because postdischarge providers must be able to access and quickly understand the information they have been provided before assuming care of the patient.[14, 15]
The Medication Safety domain is of central importance because medications are responsible for most postdischarge adverse events.[16] Taking an accurate medication history,[17] reconciling changes throughout the hospitalization,[18] and communicating the reconciled medication regimen to patients and providers across transitions of care can reduce medication errors and improve patient safety.[19, 20, 21, 22]
The Patient Education and Promotion of Self‐Management domain involves teaching patients and their caregivers about the main hospital diagnoses and instructions for self‐care, including medication changes, appointments, and whom to contact if issues arise. Confirming comprehension of instructions through assessments of acute (delirium) and chronic (dementia) cognitive impairments[23, 24, 25, 26] and teach‐back from the patient (or caregiver) is an important aspect of such counseling, as is providing patients and caregivers with educational materials that are appropriate for their level of health literacy and preferred language.[14] High‐risk patients may benefit from patient coaching to improve their self‐management skills.[12] These recommendations are based on years of health literacy research,[27, 28, 29] and such elements are generally included in effective interventions (including Project RED,[10] Naylor and colleagues' Transitional Care Model,[11] and Coleman and colleagues' Care Transitions Intervention[12]).
Enlisting the help of Social and Community Supports is an important adjunct to medical care and is the rationale for the recent increase in CMS funding for community‐based, care‐transition programs. These programs are crucial for assisting patients with household activities, meals, and other necessities during the period of recovery, though they should be distinguished from care management or care coordination interventions, which have not been found to be helpful in preventing readmissions unless high touch in nature.[30, 31]
The Advanced Care Planning domain may begin in the hospital or outpatient setting, and involves establishing goals of care and healthcare proxies, as well as engaging with palliative care or hospice services if appropriate. Emerging evidence supports the intuitive conclusion that this approach prevents readmissions, particularly in patients who do not benefit from hospital readmission.[32, 33]
Attention to the Coordinating Care Among Team Members domain is needed to synchronize efforts across settings and providers. Clearly, many healthcare professionals as well as other involved parties can be involved in helping a single patient during transitions in care. It is vital that they coordinate information, assessments, and plans as a team.[34]
We recognize the domain of Monitoring and Managing Symptoms After Discharge as increasingly crucial as reflected in our growing understanding of the reasons for readmission, especially among patients with fragile conditions such as heart failure, chronic lung disease, gastrointestinal disorders, dementia,[23, 24, 25, 26] and vascular disease.[35] Monitoring for new or worsening symptoms; medication side effects, discrepancies, or nonadherence; and other self‐management challenges will allow problems to be detected and addressed early, before they result in unplanned healthcare utilization. It is noteworthy that successful interventions in this regard rely on in‐home evaluation[13, 14, 29] by nurses rather than telemonitoring, which in isolation has not been effective to date.[36, 37]
Finally, optimal Outpatient Follow‐Up with appropriate postdischarge providers is crucial for providing ideal transitions. These appointments need to be prompt[38, 39] (eg, within 7 days if not sooner for high‐risk patients) and with providers who have a longitudinal relationship to the patient, as prior work has shown increased readmissions when the provider is unfamiliar with the patient.[40] The advantages and disadvantages of hospitalist‐run postdischarge clinics as one way to increase access and expedite follow‐up are currently being explored. Although the optimal content of a postdischarge visit has not been defined, logical tasks to be completed are myriad and imply the need for checklists, adequate time, and a multidisciplinary team of providers.[41]
IMPLICATIONS OF THE IDEAL TRANSITION IN CARE
Our conceptualization of an ideal transition in care provides insight for hospital and healthcare system leadership, policymakers, researchers, clinicians, and educators seeking to improve transitions of care and reduce hospital readmissions. In the sections below, we briefly review commonly cited concerns about the recent focus on readmissions as a quality measure, illustrate how the Ideal Transition in Care addresses these concerns, and propose fruitful areas for future work.
How Does the Framework Address the Extent to Which Readmissions Reflect Hospital Quality?
One of the chief problems with readmissionrates as a hospital quality measure is that many of the factors that influence readmission may not currently be under the hospital's control. The healthcare environment to which a patient is being discharged (and was admitted from in the first place) is an important determinant of readmission.[42] In this context, it is noteworthy that successful interventions to reduce readmission are generally those that focus on outpatient follow‐up, while inpatient‐only interventions have had less success.[7] This is reflected in our framework above, informed by the literature, highlighting the importance of coordination between inpatient and outpatient providers and the importance of postdischarge care, including monitoring and managing symptoms after discharge, prompt follow‐up appointments, the continuation of patient self‐management activities, monitoring for drug‐related problems after discharge, and the effective utilization of community supports. Accountable care organizations, once established, would be responsible for several components of this environment, including the provision of prompt and effective follow‐up care.
The implication of the framework is that if a hospital does not have control over most of the factors that influence its readmission rate, it should see financial incentives to reduce readmission rates as an opportunity to invest in relationships with the outpatient environment from which their patients are admitted and to which they are discharged. One can envision hospitals growing ever‐closer relationships with their network of primary care physician groups, community agencies, and home health services, rehabilitation facilities, and nursing homes through coordinated discharge planning, medication management, patient education, shared electronic medical records, structured handoffs in care, and systems of intensive outpatient monitoring. Our proposed framework, in other words, emphasizes that hospitals cannot reduce their readmission rates by focusing on aspects of care within their walls. They must forge new and stronger relationships with their communities if they are to be successful.
How Does the Framework Help Us Understand Which Readmissions Are Preventable?
Public reporting and financial penalties are currently tied to all‐cause readmission, but preventable readmissions are a more appealing outcome to target. In one study, the ranking of hospitals by all‐cause readmission rate had very little correlation with the ranking by preventable readmission rate.[5] However, researchers have struggled to establish standardized, valid, and reliable measures for determining what proportion of readmissions are in fact preventable, with estimates ranging from 5% to 79% in the published literature.[43]
The difficulty of accurately determining preventability stems from an inadequate understanding of the roles that patient comorbidities, transitional processes of care, individual patient behaviors, and social and environmental determinants of health play in the complex process of hospital recidivism. Our proposed elements of an ideal transition in care provide a structure to frame this discussion and suggest future research opportunities to allow a more accurate and reliable understanding of the spectrum of preventability. Care system leadership can use the framework to rigorously evaluate their readmissions and determine the extent to which the transitions process approached the ideal. For example, if a readmission occurs despite care processes that addressed most of the domains with high fidelity, it becomes much less likely that the readmission was preventable. It should be noted that the converse is not always true: When a transition falls well short of the ideal, it does not always imply that provision of a more ideal transition would necessarily have prevented the readmission, but it does make it more likely.
For educators, the framework may provide insights for trainees into the complexity of the transitions process and vulnerability of patients during this time, highlighting preventable aspects of readmissions that are within the grasp of the discharging clinician or team. It highlights the importance of medication reconciliation, synchronous communication, and predischarge teaching, which are measurable and teachable skills for non‐physician providers, housestaff, and medical students. It also may allow for more structured feedback, for example, on the quality of discharge summaries produced by trainees.
How Could the Framework Improve Risk Adjustment for Between‐Hospital Comparisons?
Under the Patient Protection and Affordable Care Act (PPACA), hospitals will be compared to one another using risk‐standardized readmission rates as a way to penalize poorly performing hospitals. However, risk‐adjustment models have only modest ability to predict hospital readmission.[6] Moreover, current approaches predominantly adjust for patients' medical comorbidities (which are easily measurable), but they do not adequately take into account the growing literature on other factors that influence readmission rates, including a patient's health literacy, visual or cognitive impairment, functional status, language barriers, and community‐level factors such as social supports.[44, 45]
The Ideal Transition of Care provides a comprehensive framework of hospital discharge quality that provides additional process measures on which hospitals could be compared rather than focusing solely on (inadequately) risk‐adjusted readmission rates. Indeed, most other quality and safety measures (such as the National Quality Forum's Safe Practices[46] and The Joint Commission's National Patient Safety Goals),[47] emphasize process over outcome, in part because of issues of fairness. Process measures are less subject to differences in patient populations and also change the focus from simply reducing readmissions to improving transitional care more broadly. These process measures should be based on our framework and should attempt to capture as many dimensions of an optimal care transition as possible.
Possible examples of process measures include: the accuracy of medication reconciliation at admission and discharge; provision of prompt outpatient follow‐up; provision of adequate systems to monitor and manage symptoms after discharge; advanced care planning in appropriate patients; and the quality of discharge education, incorporating measurements of the patient's understanding and ability to self‐manage their illness. At least some of these could be used now as part of a performance measurement set that highlights opportunities for immediate system change and can serve as performance milestones.
The framework could also be used to validate risk‐adjustment techniques. After accounting for patient factors, the remaining variability in outcomes should be accounted for by processes of care that are in the transitions framework. Once these processes are accurately measured, one can determine if indeed the remaining variability is due to transitions processes, or rather unaccounted factors that are not being measured and that hospitals may have little control over. Such work can lead to iterative refinement of patient risk‐adjustment models.
What Does the Framework Imply About Best Practices for Reducing Readmission Rates?
Despite the limitations of readmission rates as a quality measure noted above, hospitals presently face potentially large financial penalties for readmissions and are allocating resources to readmission reduction efforts. However, hospitals currently may not have enough guidance to know what actions to take to reduce readmissions, and thus could be spending money inefficiently and reducing the value proposition of focusing on readmissions.
A recent systematic review of interventions hospitals could employ to reduce readmissions identified several positive studies, but also many negative studies, and there were significant barriers to understanding what works to reduce readmissions.[7] For example, most of the interventions described in both positive and negative studies were multifaceted, and the authors were unable to identify which components of the intervention were most effective. Also, while several studies have identified risk factors for readmission,[6, 48, 49] very few studies have identified which subgroups of patients benefit most from specific interventions. Few of the studies described key contextual factors that may have led to successful or failed implementation, or the fidelity with which the intervention was implemented.[50, 51, 52]
Few if any of the studies were guided by a concept of the ideal transition in care.[10] Such a framework will better guide development of multifaceted interventions and provide an improved means for interpreting the results. Clearly, rigorously conducted, multicenter studies of readmission prevention interventions are needed to move the field forward. These studies should: 1) correlate implementation of specific intervention components with reductions in readmission rates to better understand the most effective components; 2) be adequately powered to show effect modification, ie, which patients benefit most from these interventions; and 3) rigorously measure environmental context and intervention fidelity, and employ mixed methods to better understand predictors of implementation success and failure.
Our framework can be used in the design and evaluation of such interventions. For example, interventions could be designed that incorporate as many of the domains of an ideal transition as possible, in particular those that span the inpatient and outpatient settings. Processes of care metrics can be developed that measure the extent to which each domain is delivered, analogous to the way the Joint Commission might aggregate individual scores on the 10 items in Acute Myocardial Infarction Core Measure Set[53] to provide a composite of the quality of care provided to patients with this diagnosis. These can be used to correlate certain intervention components with success in reducing readmissions and also in measuring intervention fidelity.
NEXT STEPS
For hospital and healthcare system leaders, who need to take action now to avoid financial penalties, we recommend starting with proven, high‐touch interventions such as Project RED and the Care Transitions Intervention, which are durable, cost‐effective, robustly address multiple domains of the Ideal Transition in Care, and have been implemented at numerous sites.[54, 55] Each hospital or group will need to decide on a bundle of interventions and customize them based on local workflow, resources, and culture.
Risk‐stratification, to match the intensity of the intervention to the risk of readmission of the patient, will undoubtedly be a key component for the efficient use of resources. We anticipate future research will allow risk stratification to be a robust part of any implementation plan. However, as noted above, current risk prediction models are imperfect,[6] and more work is needed to determine which patients benefit most from which interventions. Few if any studies have described interventions tailored to risk for this reason.
Based on our ideal transition in care, our collective experience, and published evidence,[7, 10, 11, 12] potential elements to start with include: early discharge planning; medication reconciliation[56]; patient/caregiver education using health literacy principles, cognitive assessments, and teach‐back to confirm understanding; synchronous communication (eg, by phone) between inpatient and postdischarge providers; follow‐up phone calls to patients within 72 hours of discharge; 24/7 availability of a responsible inpatient provider to address questions and problems (both from the patient/caregiver and from postdischarge providers); and prompt appointments for patients discharged home. High‐risk patients will likely require additional interventions, including in‐home assessments, disease‐monitoring programs, and/or patient coaching. Lastly, patients with certain conditions prone to readmission (such as heart failure and chronic obstructive pulmonary disease) may benefit from disease‐specific programs, including patient education, outpatient disease management, and monitoring protocols.
It is likely that the most effective interventions are those that come from combined, coordinated interventions shared between inpatient and outpatient settings, and are intensive in nature. We expect that the more domains in the framework that are addressed, the safer and more seamless transitions in care will be, with improvement in patient outcomes. To the extent that fragmentation of care has been a barrier to the implementation of these types of interventions in the past, ACOs, perhaps with imbedded Patient‐Centered Medical Homes, may be in the best position to take advantage of newly aligned financial incentives to design comprehensive transitional care. Indeed, we anticipate that Figure 1 may provide substrate for a discussion of postdischarge care and division of responsibilities between inpatient and outpatient care teams at the time of transition, so effort is not duplicated and multiple domains are addressed.
Other barriers to implementation of ideal transitions in care will continue to be an issue for most healthcare systems. Financial constraints that have been a barrier up until now will be partially overcome by penalties for high readmission rates and by ACOs, bundled payments, and alternative care contracts (ie, global payments), but the extent to which each institution feels rewarded for investing in transitional interventions will vary greatly. Healthcare leadership that sees the value of improving transitions in care will be critical to overcoming this barrier. Competing demands (such as lowering hospital length of stay and carrying out other patient care responsibilities),[57] lack of coordination and diffusion of responsibility among various clinical personnel, and lack of standards are other barriers[58] that will require clear prioritization from leadership, policy changes, team‐based care, provider education and feedback, and adequate allocation of personnel resources. In short, process redesign using continuous quality improvement efforts and effective tools will be required to maximize the possibility of success.
CONCLUSIONS
Readmissions are costly and undesirable. Intuition suggests they are a marker of poor care and that hospitals should be capable of reducing them, thereby improving care and decreasing costs. In a potential future world of ACOs based on global payments, financial incentives would be aligned for each system to reduce readmissions below their current baseline, therefore obviating the need for external financial rewards and penalties. In the meantime, financial penalties do exist, and controversy exists over their fairness and likelihood of driving appropriate behavior. To address these controversies and promote better transitional care, we call for the development and use of multifaceted, collaborative transitions interventions that span settings, risk‐adjustment models that allow for fairer comparisons among hospitals, better and more widespread measurement of processes of transitional care, a better understanding of what interventions are most effective and in whom, and better guidance in how to implement these interventions. Our conceptualization of an ideal transition of care serves as a guide and provides a common vocabulary for these efforts. Such research is likely to produce the knowledge needed for healthcare systems to improve transitions in care, reduce readmissions, and reduce costs.
Disclosure
Funding for Dr Vasilevskis has been provided by the National Institutes of Health (K23AG040157) and the VA Tennessee Valley Geriatric Research, Education and Clinical Center (GRECC). The content is solely the responsibility of the authors and does not necessarily represent the official views of the National Institute on Aging, the National Institutes of Health, or the US Department of Veterans Affairs.
- Redefining Health Care: Creating Value‐Based Competition on Results. Boston, MA:Harvard Business School Press;2006. , .
- Rehospitalizations among patients in the Medicare fee‐for‐service program. N Engl J Med. 2009;360(14):1418–1428. , , .
- Patient Protection and Affordable Care Act (PPACA). Public Law 111–148; 2010. Available at: http://www.gpo.gov/fdsys/pkg/PLAW‐111publ148/pdf/PLAW‐111publ148.pdf. Accessed on June 4, 2012.
- Hospital readmission as an accountability measure. JAMA. 2011;305(5):504–505. , .
- Incidence of potentially avoidable urgent readmissions and their relation to all‐cause urgent readmissions. Can Med Assoc J. 2011;183(14):E1067–E1072. , , , et al.
- Risk prediction models for hospital readmission: a systematic review. JAMA. 2011;306(15):1688–1698. , , , et al.
- Interventions to reduce 30‐day rehospitalization: a systematic review. Ann Intern Med. 2011;155(8):520–528. , , , , .
- A decade of transitional care research with vulnerable elders. J Cardiovasc Nurs. 2000;14(3):1–14. .
- for the American College of Physicians; Society of General Internal Medicine; Society of Hospital Medicine; American Geriatrics Society; American College of Emergency Physicians; Society of Academic Emergency Medicine. Transitions of Care Consensus Policy Statement American College of Physicians–Society of General Internal Medicine–Society of Hospital Medicine–American Geriatrics Society–American College of Emergency Physicians–Society of Academic Emergency Medicine. J Gen Intern Med. 2009;24(8):971–976. , , , et al;
- A reengineered hospital discharge program to decrease rehospitalization: a randomized trial. Ann Intern Med. 2009;150(3):178–187. , , , et al.
- Comprehensive discharge planning and home follow‐up of hospitalized elders: a randomized clinical trial. JAMA. 1999;281(7):613–620. , , , et al.
- The care transitions intervention: results of a randomized controlled trial. Arch Intern Med. 2006;166(17):1822–1828. , , , .
- Deficits in communication and information transfer between hospital‐based and primary care physicians: implications for patient safety and continuity of care. JAMA. 2007;297(8):831–841. , , , , , .
- Promoting effective transitions of care at hospital discharge: a review of key issues for hospitalists. J Hosp Med. 2007;2(5):314–323. , , , .
- Discharge documentation of patients discharged to subacute facilities: a three‐year quality improvement process across an integrated health care system. Jt Comm J Qual Patient Saf. 2010;36(6):243–251. , , , , , .
- The incidence and severity of adverse events affecting patients after discharge from the hospital. Ann Intern Med. 2003;138(3):161–167. , , , , .
- Frequency, type and clinical importance of medication history errors at admission to hospital: a systematic review. Can Med Assoc J. 2005;173(5):510–515. , , , , , .
- Classifying and predicting errors of inpatient medication reconciliation. J Gen Intern Med. 2008;23(9):1414–1422. , , , et al.
- for the PILL‐CVD (Pharmacist Intervention for Low Literacy in Cardiovascular Disease) Study Group. Effect of a pharmacist intervention on clinically important medication errors after hospital discharge: a randomized trial. Ann Intern Med. 2012;157(1):1–10. , , et al;
- Hospital‐based medication reconciliation practices: a systematic review. Arch Intern Med. 2012;172(14):1057–1069. , , , .
- Effect of an electronic medication reconciliation application and process redesign on potential adverse drug events: a cluster‐randomized trial. Arch Intern Med. 2009;169(8):771–780. , , , et al.
- Role of pharmacist counseling in preventing adverse drug events after hospitalization. Arch Intern Med. 2006;166(5):565–571. , , , et al.
- Insufficient help for activity of daily living disabilities and risk of all–cause hospitalization. J Am Geriatr Soc. 2012;60(5):927–933. , , , , .
- Transitions in care for older adults with and without dementia. J Am Geriatr Soc. 2012;60(5):813–820. , , , et al.
- Association of incident dementia with hospitalizations. JAMA. 2012;307(2):165–172. , , , , .
- Potentially avoidable hospitalizations of dually eligible Medicare and Medicaid beneficiaries from nursing facility and home– and community–based services waiver programs. J Am Geriatr Soc. 2012;60(5):821–829. , , , et al.
- Teaching about health literacy and clear communication. J Gen Intern Med. 2006;21(8):888–890. , .
- Health literacy and outcomes among patients with heart failure. JAMA. 2011;305(16):1695–1701. , , , et al.
- Patient experiences of transitioning from hospital to home: an ethnographic quality improvement project. J Hosp Med. 2012;7(5):382–387. , , , , .
- Effects of care coordination on hospitalization, quality of care, and health care expenditures among Medicare beneficiaries: 15 randomized trials. JAMA. 2009;301(6):603–618. , , , .
- How changes in Washington University's Medicare coordinated care demonstration pilot ultimately achieved savings. Health Aff (Millwood). 2012;31(6):1216–1226. , , , , .
- Quality of care and rehospitalization rate in the last stage of disease in brain tumor patients assisted at home: a cost effectiveness study. J Palliat Med. 2012;15(2):225–227. , , , et al.
- Inpatient palliative care consults and the probability of hospital readmission. Perm J. 2011;15(2):48–51. , , , , .
- TeamSTEPPS™: team strategies and tools to enhance performance and patient safety. In: Henriksen K, Battles JB, Keyes MA, Grady ML, ed. Advances in Patient Safety: New Directions and Alternative Approaches. Vol 3: Performance and Tools. Rockville, MD:Agency for Healthcare Research and Quality; August2008. , , , et al.
- Factors contributing to all‐cause 30‐day readmissions: a structured case series across 18 hospitals. Med Care. 2012;50(7):599–605. , , , et al.
- Telemonitoring in patients with heart failure [erratum, N Engl J Med. 2011;364(5):490]. N Engl J Med. 2010;363(24):2301–2309. , , , et al.
- A randomized controlled trial of telemonitoring in older adults with multiple health issues to prevent hospitalizations and emergency department visits. Arch Intern Med. 2012;172(10):773–779. , , , et al.
- Relationship between early physician follow‐up and 30‐day readmission among Medicare beneficiaries hospitalized for heart failure. JAMA. 2010;303(17):1716–1722. , , , et al.
- Post‐hospitalization transitions: examining the effects of timing of primary care provider follow‐up. J Hosp Med. 2010;5(7):392–397. , , .
- Does increased access to primary care reduce hospital readmissions? Veterans Affairs Cooperative Study Group on Primary Care and Hospital Readmission. N Engl J Med. 1996;334(22):1441–1447. , , .
- The Post‐Hospital Follow‐Up Visit: A Physician Checklist to Reduce Readmissions. California Healthcare Foundation; October 2010. Available at: http://www.chcf.org/publications/2010/10/the‐post‐hospital‐follow‐up‐visit‐a‐physician‐checklist. Accessed on January 10, 2012. .
- Thirty‐day readmission rates for Medicare beneficiaries by race and site of care. JAMA. 2011;305(7):675–681. , , .
- Proportion of hospital readmissions deemed avoidable: a systematic review. Can Med Assoc J. 2011;183(7):E391–E402. , , , , .
- Postdischarge environmental and socioeconomic factors and the likelihood of early hospital readmission among community‐dwelling Medicare beneficiaries. Gerontologist. 2008;48(4):495–504. , , , et al.
- Low health literacy and health outcomes: an updated systematic review. Ann Intern Med. 2011;155(2):97–107. , , , , .
- National Quality Forum. Safe Practices for Better Healthcare—2010 Update: A Consensus Report. Washington, DC;2010.
- Joint Commission on Accreditation of Healthcare Organizations. Accreditation Program: Hospital 2010 National Patient Safety Goals (NPSGs). 2010. Available at: http://www.jointcommission.org/PatientSafety/NationalPatientSafetyGoals/. Accessed on March 20, 2012.
- Hospital readmission in general medicine patients: a prediction model. J Gen Intern Med. 2010;25(3):211–219. , , , et al.
- Derivation and validation of an index to predict early death or unplanned readmission after discharge from hospital to the community. Can Med Assoc J. 2010;182(6):551–557. , , , et al.
- Evaluating service delivery interventions to enhance patient safety. BMJ. 2008;337:a2764. , .
- Assessing the Evidence for Context‐Sensitive Effectiveness and Safety of Patient Safety Practices: Developing Criteria. Rockville, MD:Agency for Healthcare Research and Quality; December2010. , , .
- Advancing the science of patient safety. Ann Intern Med. 2011;154(10):693–696. , , , et al.
- The Joint Commission. Acute Myocardial Infarction Core Measure Set. Available at: http://www.jointcommission.org/assets/1/6/Acute%20Myocardial%20Infarction.pdf. Accessed August 20,2012.
- The care transitions intervention: translating from efficacy to effectiveness. Arch Intern Med. 2011;171(14):1232–1237. , , , , , .
- Project RED toolkit, AHRQ Innovations Exchange. Available at:http://www.innovations.ahrq.gov/content.aspx?id=2180. Accessed on July 2, 2012.
- A comprehensive pharmacist intervention to reduce morbidity in patients 80 years or older: a randomized controlled trial. Arch Intern Med. 2009;169(9):894–900. , , , et al.
- Thirty‐day readmissions—truth and consequences. N Engl J Med. 2012;366(15):1366–1369. , .
- “Out of sight, out of mind”: housestaff perceptions of quality‐limiting factors in discharge care at teaching hospitals. J Hosp Med. 2012;7(5):376–381. , , , , .
Containing the rise of healthcare costs has taken on a new sense of urgency in the wake of the recent economic recession and continued growth in the cost of healthcare. Accordingly, many stakeholders seek solutions to improve value (reducing costs while improving care)[1]; hospital readmissions, which are common and costly,[2] have emerged as a key target. The Centers for Medicare and Medicaid Services (CMS) have instituted several programs intended to reduce readmissions, including funding for community‐based, care‐transition programs; penalties for hospitals with elevated risk‐adjusted readmission rates for selected diagnoses; pioneer Accountable Care Organizations (ACOs) with incentives to reduce global costs of care; and Hospital Engagement Networks (HENs) through the Partnership for Patients.[3] A primary aim of these initiatives is to enhance the quality of care transitions as patients are discharged from the hospital.
Though the recent focus on hospital readmissions has appropriately drawn attention to transitions in care, some have expressed concerns. Among these are questions about: 1) the extent to which readmissions truly reflect the quality of hospital care[4]; 2) the preventability of readmissions[5]; 3) limitations in risk‐adjustment techniques[6]; and 4) best practices for preventing readmissions.[7] We believe these concerns stem in part from deficiencies in the state of the science of transitional care, and that future efforts in this area will be hindered without a clear vision of an ideal transition in care. We propose the key components of an ideal transition in care and discuss the implications of this concept as it pertains to hospital readmissions.
THE IDEAL TRANSITION IN CARE
We propose the key components of an ideal transition in care in Figure 1 and Table 1. Figure 1 represents 10 domains described more fully below as structural supports of the bridge patients must cross from one care environment to another during a care transition. This figure highlights key domains and suggests that lack of a domain makes the bridge weaker and more prone to gaps in care and poor outcomes. It also implies that the more components are missing, the less safe is the bridge or transition. Those domains that mainly take place prior to discharge are placed closer to the hospital side of the bridge, those that mainly take place after discharge are placed closer to the community side of the bridge, while those that take place both prior to and after discharge are in the middle. Table 1 provides descriptions of the key content for each of these domains, as well as guidance about which personnel might be involved and where in the transition process that domain should be implemented. We support these domains with supporting evidence where available.
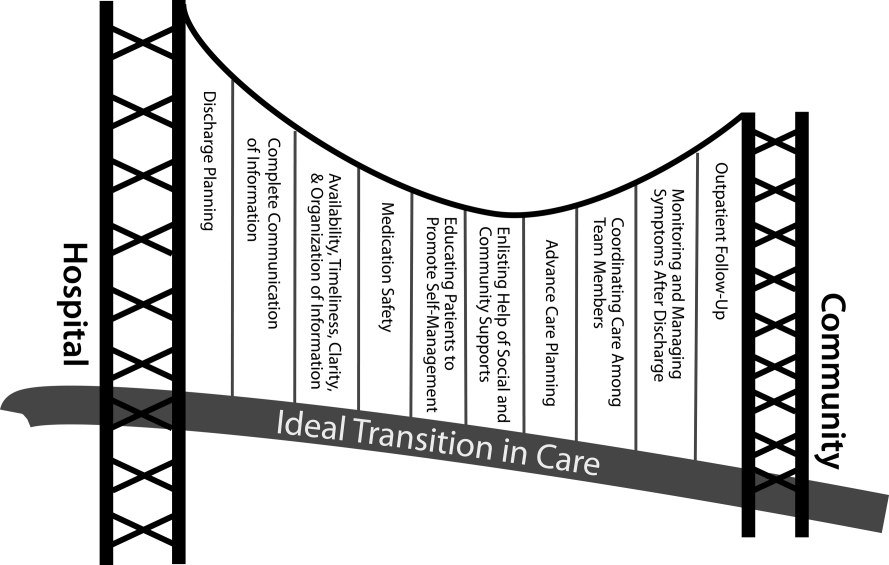
Domain | Who | When | References |
---|---|---|---|
| |||
Discharge planning | |||
Use a multidisciplinary team to create a discharge plan | Discharging clinician | Predischarge | 911 |
Collaborate with PCP regarding discharge and follow‐up plan | Care managers/discharge planners | ||
Arrange follow‐up appointments prior to discharge | Nurses | ||
Make timely appointments for follow‐up care | |||
Make appointments that take patient and caregiver's schedules and transportation needs into account | |||
Complete communication of information | |||
Includes: | Discharging clinician | Time of discharge | 1214 |
Patient's full name | |||
Age | |||
Dates of admission and discharge | |||
Names of responsible hospital physicians | |||
Name of physician preparing discharge summary | |||
Name of PCP | |||
Main diagnosis | |||
Other relevant diagnoses, procedures, and complications | |||
Relevant findings at admission | |||
Treatment and response for each active problem | |||
Results of procedures and abnormal laboratory test results | |||
Recommendations of any subspecialty consultants | |||
Patient's functional status at discharge | |||
Discharge medications | |||
Follow‐up appointments made and those to be made | |||
Tests to be ordered and pending tests to be followed‐up | |||
Counseling provided to patient and caregiver, when applicable | |||
Contingency planning | |||
Code status | |||
Availability, timeliness, clarity, and organization of information | |||
Timely communication with postdischarge providers verbally (preferred) or by fax/e‐mail | Discharging clinician | Time of discharge | 1214 |
Timely completion of discharge summary and reliable transmission to postdischarge providers | |||
Availability of information in medical record | |||
Use of a structured template with subheadings in discharge communication | |||
Medication safety | |||
Take an accurate preadmission medication history | Clinicians | Admission | 1521 |
Reconcile preadmission medications with all ordered medications at all transfers in care, including discharge | Pharmacists | Throughout hospitalization | |
Communicate discharge medications to all outpatient providers, including all changes and rationale for those changes | Nurses | Time of discharge | |
Educating patients, promoting self‐management | |||
Focus discharge counseling on major diagnoses, medication changes, dates of follow‐up appointments, self‐care instructions, warning signs and symptoms, and who to contact for problems | Clinicians | Daily | 911, 2228, 30 |
Include caregivers as appropriate | Nurses | Time of discharge | |
Ensure staff members provide consistent messages | Care managers/discharge planners | Postdischarge | |
Provide simply written patient‐centered materials with instructions | Transition coaches | ||
Use teach‐back methods to confirm understanding | |||
Encourage questions | |||
Continue teaching during postdischarge follow‐up | |||
Use transition coaches in high‐risk patients: focus on medication management, keeping a personal medical record, follow‐up appointments, and knowledge of red flags | |||
Enlisting help of social and community supports | |||
Assess needs and appropriately arrange for home services | Clinicians | Predischarge and postdischarge | 29, 30 |
Enlist help of caregivers | Nurses | ||
Enlist help of community supports | Care managers | ||
Home health staff | |||
Advanced care planning | |||
Establish healthcare proxy | Clinicians | Predischarge and postdischarge | 31, 32 |
Discuss goals of care | Palliative care staff | ||
Palliative care consultation (if appropriate) | Social workers | ||
Enlist hospice services (if appropriate) | Nurses | ||
Hospice workers | |||
Coordinating care among team members | |||
Share medical records | Clinicians | Predischarge and postdischarge | 33 |
Communicate involving all team members | Nurses | ||
Optimize continuity of providers and formal handoffs of care | Office personnel | ||
IT staff | |||
Monitoring and managing symptoms after discharge | |||
Monitor for: | Clinicians | Postdischarge | 1113, 28, 3436 |
Worsening disease control | Nurses | ||
Medication side effects, discrepancies, nonadherence | Pharmacists | ||
Therapeutic drug monitoring | Care managers | ||
Inability to manage conditions at home | Visiting nurses and other home health staff | ||
Via: | |||
Postdischarge phone calls | |||
Home visits | |||
Postdischarge clinic visits | |||
Patient hotline | |||
Availability of inpatient providers after discharge | |||
Follow‐up with outpatient providers | |||
Within an appropriate time frame (eg, 7 d or sooner for high‐risk patients) | Clinicians | Postdischarge | 3740 |
With appropriate providers (eg, most related to reasons for hospitalization, who manage least stable conditions, and/or PCP) | Nurses Pharmacists | ||
Utilize multidisciplinary teams as appropriate | Care managers | ||
Ensure appropriate progress along plan of care and safe transition | Office personnel | ||
Other clinical staff as appropriate |
Our concept of an ideal transition in care began with work by Naylor, who described several important components of a safe transition in care, including complete communication of information, patient education, enlisting the help of social and community supports, ensuring continuity of care, and coordinating care among team members.[8] It is supplemented by the Transitions of Care Consensus Policy Statement proposed by representatives from hospital medicine, primary care, and emergency medicine, which emphasized aspects of timeliness and content of communication between providers.[9] Our present articulation of these key components includes 10 organizing domains.
The Discharge Planning domain highlights the important principle of planning ahead for hospital discharge while the patient is still being treated in the hospital, a paradigm espoused by Project RED[10] and other successful care transitions interventions.[11, 12] Collaborating with the outpatient provider and taking the patient and caregiver's preferences for appointment scheduling into account can help ensure optimal outpatient follow‐up.
Complete Communication of Information refers to the content that should be included in discharge summaries and other means of information transfer from hospital to postdischarge care. The specific content areas are based on the Society of Hospital Medicine and Society of General Internal Medicine Continuity of Care Task Force systematic review and recommendations,[13] which takes into account information requested by primary care physicians after discharge.
Availability, Timeliness, Clarity, and Organization of that information is as important as the content because postdischarge providers must be able to access and quickly understand the information they have been provided before assuming care of the patient.[14, 15]
The Medication Safety domain is of central importance because medications are responsible for most postdischarge adverse events.[16] Taking an accurate medication history,[17] reconciling changes throughout the hospitalization,[18] and communicating the reconciled medication regimen to patients and providers across transitions of care can reduce medication errors and improve patient safety.[19, 20, 21, 22]
The Patient Education and Promotion of Self‐Management domain involves teaching patients and their caregivers about the main hospital diagnoses and instructions for self‐care, including medication changes, appointments, and whom to contact if issues arise. Confirming comprehension of instructions through assessments of acute (delirium) and chronic (dementia) cognitive impairments[23, 24, 25, 26] and teach‐back from the patient (or caregiver) is an important aspect of such counseling, as is providing patients and caregivers with educational materials that are appropriate for their level of health literacy and preferred language.[14] High‐risk patients may benefit from patient coaching to improve their self‐management skills.[12] These recommendations are based on years of health literacy research,[27, 28, 29] and such elements are generally included in effective interventions (including Project RED,[10] Naylor and colleagues' Transitional Care Model,[11] and Coleman and colleagues' Care Transitions Intervention[12]).
Enlisting the help of Social and Community Supports is an important adjunct to medical care and is the rationale for the recent increase in CMS funding for community‐based, care‐transition programs. These programs are crucial for assisting patients with household activities, meals, and other necessities during the period of recovery, though they should be distinguished from care management or care coordination interventions, which have not been found to be helpful in preventing readmissions unless high touch in nature.[30, 31]
The Advanced Care Planning domain may begin in the hospital or outpatient setting, and involves establishing goals of care and healthcare proxies, as well as engaging with palliative care or hospice services if appropriate. Emerging evidence supports the intuitive conclusion that this approach prevents readmissions, particularly in patients who do not benefit from hospital readmission.[32, 33]
Attention to the Coordinating Care Among Team Members domain is needed to synchronize efforts across settings and providers. Clearly, many healthcare professionals as well as other involved parties can be involved in helping a single patient during transitions in care. It is vital that they coordinate information, assessments, and plans as a team.[34]
We recognize the domain of Monitoring and Managing Symptoms After Discharge as increasingly crucial as reflected in our growing understanding of the reasons for readmission, especially among patients with fragile conditions such as heart failure, chronic lung disease, gastrointestinal disorders, dementia,[23, 24, 25, 26] and vascular disease.[35] Monitoring for new or worsening symptoms; medication side effects, discrepancies, or nonadherence; and other self‐management challenges will allow problems to be detected and addressed early, before they result in unplanned healthcare utilization. It is noteworthy that successful interventions in this regard rely on in‐home evaluation[13, 14, 29] by nurses rather than telemonitoring, which in isolation has not been effective to date.[36, 37]
Finally, optimal Outpatient Follow‐Up with appropriate postdischarge providers is crucial for providing ideal transitions. These appointments need to be prompt[38, 39] (eg, within 7 days if not sooner for high‐risk patients) and with providers who have a longitudinal relationship to the patient, as prior work has shown increased readmissions when the provider is unfamiliar with the patient.[40] The advantages and disadvantages of hospitalist‐run postdischarge clinics as one way to increase access and expedite follow‐up are currently being explored. Although the optimal content of a postdischarge visit has not been defined, logical tasks to be completed are myriad and imply the need for checklists, adequate time, and a multidisciplinary team of providers.[41]
IMPLICATIONS OF THE IDEAL TRANSITION IN CARE
Our conceptualization of an ideal transition in care provides insight for hospital and healthcare system leadership, policymakers, researchers, clinicians, and educators seeking to improve transitions of care and reduce hospital readmissions. In the sections below, we briefly review commonly cited concerns about the recent focus on readmissions as a quality measure, illustrate how the Ideal Transition in Care addresses these concerns, and propose fruitful areas for future work.
How Does the Framework Address the Extent to Which Readmissions Reflect Hospital Quality?
One of the chief problems with readmissionrates as a hospital quality measure is that many of the factors that influence readmission may not currently be under the hospital's control. The healthcare environment to which a patient is being discharged (and was admitted from in the first place) is an important determinant of readmission.[42] In this context, it is noteworthy that successful interventions to reduce readmission are generally those that focus on outpatient follow‐up, while inpatient‐only interventions have had less success.[7] This is reflected in our framework above, informed by the literature, highlighting the importance of coordination between inpatient and outpatient providers and the importance of postdischarge care, including monitoring and managing symptoms after discharge, prompt follow‐up appointments, the continuation of patient self‐management activities, monitoring for drug‐related problems after discharge, and the effective utilization of community supports. Accountable care organizations, once established, would be responsible for several components of this environment, including the provision of prompt and effective follow‐up care.
The implication of the framework is that if a hospital does not have control over most of the factors that influence its readmission rate, it should see financial incentives to reduce readmission rates as an opportunity to invest in relationships with the outpatient environment from which their patients are admitted and to which they are discharged. One can envision hospitals growing ever‐closer relationships with their network of primary care physician groups, community agencies, and home health services, rehabilitation facilities, and nursing homes through coordinated discharge planning, medication management, patient education, shared electronic medical records, structured handoffs in care, and systems of intensive outpatient monitoring. Our proposed framework, in other words, emphasizes that hospitals cannot reduce their readmission rates by focusing on aspects of care within their walls. They must forge new and stronger relationships with their communities if they are to be successful.
How Does the Framework Help Us Understand Which Readmissions Are Preventable?
Public reporting and financial penalties are currently tied to all‐cause readmission, but preventable readmissions are a more appealing outcome to target. In one study, the ranking of hospitals by all‐cause readmission rate had very little correlation with the ranking by preventable readmission rate.[5] However, researchers have struggled to establish standardized, valid, and reliable measures for determining what proportion of readmissions are in fact preventable, with estimates ranging from 5% to 79% in the published literature.[43]
The difficulty of accurately determining preventability stems from an inadequate understanding of the roles that patient comorbidities, transitional processes of care, individual patient behaviors, and social and environmental determinants of health play in the complex process of hospital recidivism. Our proposed elements of an ideal transition in care provide a structure to frame this discussion and suggest future research opportunities to allow a more accurate and reliable understanding of the spectrum of preventability. Care system leadership can use the framework to rigorously evaluate their readmissions and determine the extent to which the transitions process approached the ideal. For example, if a readmission occurs despite care processes that addressed most of the domains with high fidelity, it becomes much less likely that the readmission was preventable. It should be noted that the converse is not always true: When a transition falls well short of the ideal, it does not always imply that provision of a more ideal transition would necessarily have prevented the readmission, but it does make it more likely.
For educators, the framework may provide insights for trainees into the complexity of the transitions process and vulnerability of patients during this time, highlighting preventable aspects of readmissions that are within the grasp of the discharging clinician or team. It highlights the importance of medication reconciliation, synchronous communication, and predischarge teaching, which are measurable and teachable skills for non‐physician providers, housestaff, and medical students. It also may allow for more structured feedback, for example, on the quality of discharge summaries produced by trainees.
How Could the Framework Improve Risk Adjustment for Between‐Hospital Comparisons?
Under the Patient Protection and Affordable Care Act (PPACA), hospitals will be compared to one another using risk‐standardized readmission rates as a way to penalize poorly performing hospitals. However, risk‐adjustment models have only modest ability to predict hospital readmission.[6] Moreover, current approaches predominantly adjust for patients' medical comorbidities (which are easily measurable), but they do not adequately take into account the growing literature on other factors that influence readmission rates, including a patient's health literacy, visual or cognitive impairment, functional status, language barriers, and community‐level factors such as social supports.[44, 45]
The Ideal Transition of Care provides a comprehensive framework of hospital discharge quality that provides additional process measures on which hospitals could be compared rather than focusing solely on (inadequately) risk‐adjusted readmission rates. Indeed, most other quality and safety measures (such as the National Quality Forum's Safe Practices[46] and The Joint Commission's National Patient Safety Goals),[47] emphasize process over outcome, in part because of issues of fairness. Process measures are less subject to differences in patient populations and also change the focus from simply reducing readmissions to improving transitional care more broadly. These process measures should be based on our framework and should attempt to capture as many dimensions of an optimal care transition as possible.
Possible examples of process measures include: the accuracy of medication reconciliation at admission and discharge; provision of prompt outpatient follow‐up; provision of adequate systems to monitor and manage symptoms after discharge; advanced care planning in appropriate patients; and the quality of discharge education, incorporating measurements of the patient's understanding and ability to self‐manage their illness. At least some of these could be used now as part of a performance measurement set that highlights opportunities for immediate system change and can serve as performance milestones.
The framework could also be used to validate risk‐adjustment techniques. After accounting for patient factors, the remaining variability in outcomes should be accounted for by processes of care that are in the transitions framework. Once these processes are accurately measured, one can determine if indeed the remaining variability is due to transitions processes, or rather unaccounted factors that are not being measured and that hospitals may have little control over. Such work can lead to iterative refinement of patient risk‐adjustment models.
What Does the Framework Imply About Best Practices for Reducing Readmission Rates?
Despite the limitations of readmission rates as a quality measure noted above, hospitals presently face potentially large financial penalties for readmissions and are allocating resources to readmission reduction efforts. However, hospitals currently may not have enough guidance to know what actions to take to reduce readmissions, and thus could be spending money inefficiently and reducing the value proposition of focusing on readmissions.
A recent systematic review of interventions hospitals could employ to reduce readmissions identified several positive studies, but also many negative studies, and there were significant barriers to understanding what works to reduce readmissions.[7] For example, most of the interventions described in both positive and negative studies were multifaceted, and the authors were unable to identify which components of the intervention were most effective. Also, while several studies have identified risk factors for readmission,[6, 48, 49] very few studies have identified which subgroups of patients benefit most from specific interventions. Few of the studies described key contextual factors that may have led to successful or failed implementation, or the fidelity with which the intervention was implemented.[50, 51, 52]
Few if any of the studies were guided by a concept of the ideal transition in care.[10] Such a framework will better guide development of multifaceted interventions and provide an improved means for interpreting the results. Clearly, rigorously conducted, multicenter studies of readmission prevention interventions are needed to move the field forward. These studies should: 1) correlate implementation of specific intervention components with reductions in readmission rates to better understand the most effective components; 2) be adequately powered to show effect modification, ie, which patients benefit most from these interventions; and 3) rigorously measure environmental context and intervention fidelity, and employ mixed methods to better understand predictors of implementation success and failure.
Our framework can be used in the design and evaluation of such interventions. For example, interventions could be designed that incorporate as many of the domains of an ideal transition as possible, in particular those that span the inpatient and outpatient settings. Processes of care metrics can be developed that measure the extent to which each domain is delivered, analogous to the way the Joint Commission might aggregate individual scores on the 10 items in Acute Myocardial Infarction Core Measure Set[53] to provide a composite of the quality of care provided to patients with this diagnosis. These can be used to correlate certain intervention components with success in reducing readmissions and also in measuring intervention fidelity.
NEXT STEPS
For hospital and healthcare system leaders, who need to take action now to avoid financial penalties, we recommend starting with proven, high‐touch interventions such as Project RED and the Care Transitions Intervention, which are durable, cost‐effective, robustly address multiple domains of the Ideal Transition in Care, and have been implemented at numerous sites.[54, 55] Each hospital or group will need to decide on a bundle of interventions and customize them based on local workflow, resources, and culture.
Risk‐stratification, to match the intensity of the intervention to the risk of readmission of the patient, will undoubtedly be a key component for the efficient use of resources. We anticipate future research will allow risk stratification to be a robust part of any implementation plan. However, as noted above, current risk prediction models are imperfect,[6] and more work is needed to determine which patients benefit most from which interventions. Few if any studies have described interventions tailored to risk for this reason.
Based on our ideal transition in care, our collective experience, and published evidence,[7, 10, 11, 12] potential elements to start with include: early discharge planning; medication reconciliation[56]; patient/caregiver education using health literacy principles, cognitive assessments, and teach‐back to confirm understanding; synchronous communication (eg, by phone) between inpatient and postdischarge providers; follow‐up phone calls to patients within 72 hours of discharge; 24/7 availability of a responsible inpatient provider to address questions and problems (both from the patient/caregiver and from postdischarge providers); and prompt appointments for patients discharged home. High‐risk patients will likely require additional interventions, including in‐home assessments, disease‐monitoring programs, and/or patient coaching. Lastly, patients with certain conditions prone to readmission (such as heart failure and chronic obstructive pulmonary disease) may benefit from disease‐specific programs, including patient education, outpatient disease management, and monitoring protocols.
It is likely that the most effective interventions are those that come from combined, coordinated interventions shared between inpatient and outpatient settings, and are intensive in nature. We expect that the more domains in the framework that are addressed, the safer and more seamless transitions in care will be, with improvement in patient outcomes. To the extent that fragmentation of care has been a barrier to the implementation of these types of interventions in the past, ACOs, perhaps with imbedded Patient‐Centered Medical Homes, may be in the best position to take advantage of newly aligned financial incentives to design comprehensive transitional care. Indeed, we anticipate that Figure 1 may provide substrate for a discussion of postdischarge care and division of responsibilities between inpatient and outpatient care teams at the time of transition, so effort is not duplicated and multiple domains are addressed.
Other barriers to implementation of ideal transitions in care will continue to be an issue for most healthcare systems. Financial constraints that have been a barrier up until now will be partially overcome by penalties for high readmission rates and by ACOs, bundled payments, and alternative care contracts (ie, global payments), but the extent to which each institution feels rewarded for investing in transitional interventions will vary greatly. Healthcare leadership that sees the value of improving transitions in care will be critical to overcoming this barrier. Competing demands (such as lowering hospital length of stay and carrying out other patient care responsibilities),[57] lack of coordination and diffusion of responsibility among various clinical personnel, and lack of standards are other barriers[58] that will require clear prioritization from leadership, policy changes, team‐based care, provider education and feedback, and adequate allocation of personnel resources. In short, process redesign using continuous quality improvement efforts and effective tools will be required to maximize the possibility of success.
CONCLUSIONS
Readmissions are costly and undesirable. Intuition suggests they are a marker of poor care and that hospitals should be capable of reducing them, thereby improving care and decreasing costs. In a potential future world of ACOs based on global payments, financial incentives would be aligned for each system to reduce readmissions below their current baseline, therefore obviating the need for external financial rewards and penalties. In the meantime, financial penalties do exist, and controversy exists over their fairness and likelihood of driving appropriate behavior. To address these controversies and promote better transitional care, we call for the development and use of multifaceted, collaborative transitions interventions that span settings, risk‐adjustment models that allow for fairer comparisons among hospitals, better and more widespread measurement of processes of transitional care, a better understanding of what interventions are most effective and in whom, and better guidance in how to implement these interventions. Our conceptualization of an ideal transition of care serves as a guide and provides a common vocabulary for these efforts. Such research is likely to produce the knowledge needed for healthcare systems to improve transitions in care, reduce readmissions, and reduce costs.
Disclosure
Funding for Dr Vasilevskis has been provided by the National Institutes of Health (K23AG040157) and the VA Tennessee Valley Geriatric Research, Education and Clinical Center (GRECC). The content is solely the responsibility of the authors and does not necessarily represent the official views of the National Institute on Aging, the National Institutes of Health, or the US Department of Veterans Affairs.
Containing the rise of healthcare costs has taken on a new sense of urgency in the wake of the recent economic recession and continued growth in the cost of healthcare. Accordingly, many stakeholders seek solutions to improve value (reducing costs while improving care)[1]; hospital readmissions, which are common and costly,[2] have emerged as a key target. The Centers for Medicare and Medicaid Services (CMS) have instituted several programs intended to reduce readmissions, including funding for community‐based, care‐transition programs; penalties for hospitals with elevated risk‐adjusted readmission rates for selected diagnoses; pioneer Accountable Care Organizations (ACOs) with incentives to reduce global costs of care; and Hospital Engagement Networks (HENs) through the Partnership for Patients.[3] A primary aim of these initiatives is to enhance the quality of care transitions as patients are discharged from the hospital.
Though the recent focus on hospital readmissions has appropriately drawn attention to transitions in care, some have expressed concerns. Among these are questions about: 1) the extent to which readmissions truly reflect the quality of hospital care[4]; 2) the preventability of readmissions[5]; 3) limitations in risk‐adjustment techniques[6]; and 4) best practices for preventing readmissions.[7] We believe these concerns stem in part from deficiencies in the state of the science of transitional care, and that future efforts in this area will be hindered without a clear vision of an ideal transition in care. We propose the key components of an ideal transition in care and discuss the implications of this concept as it pertains to hospital readmissions.
THE IDEAL TRANSITION IN CARE
We propose the key components of an ideal transition in care in Figure 1 and Table 1. Figure 1 represents 10 domains described more fully below as structural supports of the bridge patients must cross from one care environment to another during a care transition. This figure highlights key domains and suggests that lack of a domain makes the bridge weaker and more prone to gaps in care and poor outcomes. It also implies that the more components are missing, the less safe is the bridge or transition. Those domains that mainly take place prior to discharge are placed closer to the hospital side of the bridge, those that mainly take place after discharge are placed closer to the community side of the bridge, while those that take place both prior to and after discharge are in the middle. Table 1 provides descriptions of the key content for each of these domains, as well as guidance about which personnel might be involved and where in the transition process that domain should be implemented. We support these domains with supporting evidence where available.
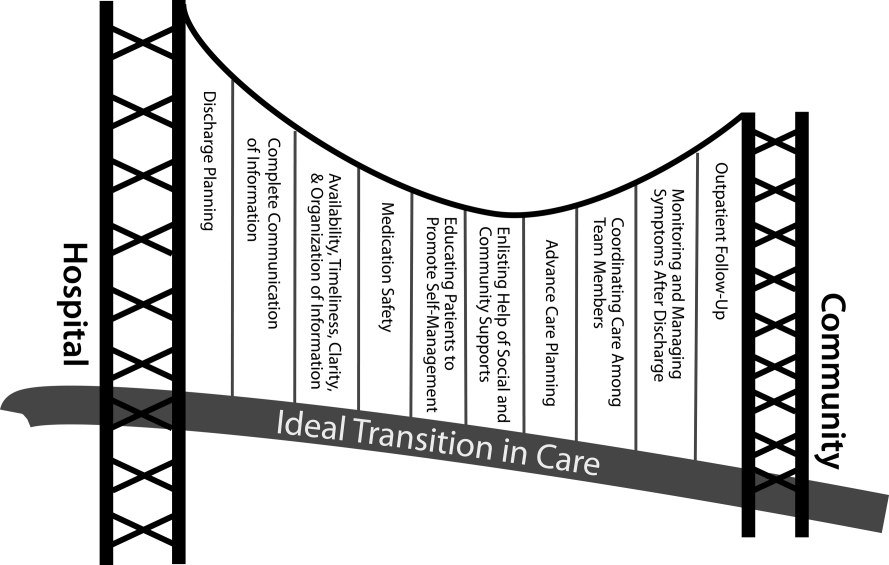
Domain | Who | When | References |
---|---|---|---|
| |||
Discharge planning | |||
Use a multidisciplinary team to create a discharge plan | Discharging clinician | Predischarge | 911 |
Collaborate with PCP regarding discharge and follow‐up plan | Care managers/discharge planners | ||
Arrange follow‐up appointments prior to discharge | Nurses | ||
Make timely appointments for follow‐up care | |||
Make appointments that take patient and caregiver's schedules and transportation needs into account | |||
Complete communication of information | |||
Includes: | Discharging clinician | Time of discharge | 1214 |
Patient's full name | |||
Age | |||
Dates of admission and discharge | |||
Names of responsible hospital physicians | |||
Name of physician preparing discharge summary | |||
Name of PCP | |||
Main diagnosis | |||
Other relevant diagnoses, procedures, and complications | |||
Relevant findings at admission | |||
Treatment and response for each active problem | |||
Results of procedures and abnormal laboratory test results | |||
Recommendations of any subspecialty consultants | |||
Patient's functional status at discharge | |||
Discharge medications | |||
Follow‐up appointments made and those to be made | |||
Tests to be ordered and pending tests to be followed‐up | |||
Counseling provided to patient and caregiver, when applicable | |||
Contingency planning | |||
Code status | |||
Availability, timeliness, clarity, and organization of information | |||
Timely communication with postdischarge providers verbally (preferred) or by fax/e‐mail | Discharging clinician | Time of discharge | 1214 |
Timely completion of discharge summary and reliable transmission to postdischarge providers | |||
Availability of information in medical record | |||
Use of a structured template with subheadings in discharge communication | |||
Medication safety | |||
Take an accurate preadmission medication history | Clinicians | Admission | 1521 |
Reconcile preadmission medications with all ordered medications at all transfers in care, including discharge | Pharmacists | Throughout hospitalization | |
Communicate discharge medications to all outpatient providers, including all changes and rationale for those changes | Nurses | Time of discharge | |
Educating patients, promoting self‐management | |||
Focus discharge counseling on major diagnoses, medication changes, dates of follow‐up appointments, self‐care instructions, warning signs and symptoms, and who to contact for problems | Clinicians | Daily | 911, 2228, 30 |
Include caregivers as appropriate | Nurses | Time of discharge | |
Ensure staff members provide consistent messages | Care managers/discharge planners | Postdischarge | |
Provide simply written patient‐centered materials with instructions | Transition coaches | ||
Use teach‐back methods to confirm understanding | |||
Encourage questions | |||
Continue teaching during postdischarge follow‐up | |||
Use transition coaches in high‐risk patients: focus on medication management, keeping a personal medical record, follow‐up appointments, and knowledge of red flags | |||
Enlisting help of social and community supports | |||
Assess needs and appropriately arrange for home services | Clinicians | Predischarge and postdischarge | 29, 30 |
Enlist help of caregivers | Nurses | ||
Enlist help of community supports | Care managers | ||
Home health staff | |||
Advanced care planning | |||
Establish healthcare proxy | Clinicians | Predischarge and postdischarge | 31, 32 |
Discuss goals of care | Palliative care staff | ||
Palliative care consultation (if appropriate) | Social workers | ||
Enlist hospice services (if appropriate) | Nurses | ||
Hospice workers | |||
Coordinating care among team members | |||
Share medical records | Clinicians | Predischarge and postdischarge | 33 |
Communicate involving all team members | Nurses | ||
Optimize continuity of providers and formal handoffs of care | Office personnel | ||
IT staff | |||
Monitoring and managing symptoms after discharge | |||
Monitor for: | Clinicians | Postdischarge | 1113, 28, 3436 |
Worsening disease control | Nurses | ||
Medication side effects, discrepancies, nonadherence | Pharmacists | ||
Therapeutic drug monitoring | Care managers | ||
Inability to manage conditions at home | Visiting nurses and other home health staff | ||
Via: | |||
Postdischarge phone calls | |||
Home visits | |||
Postdischarge clinic visits | |||
Patient hotline | |||
Availability of inpatient providers after discharge | |||
Follow‐up with outpatient providers | |||
Within an appropriate time frame (eg, 7 d or sooner for high‐risk patients) | Clinicians | Postdischarge | 3740 |
With appropriate providers (eg, most related to reasons for hospitalization, who manage least stable conditions, and/or PCP) | Nurses Pharmacists | ||
Utilize multidisciplinary teams as appropriate | Care managers | ||
Ensure appropriate progress along plan of care and safe transition | Office personnel | ||
Other clinical staff as appropriate |
Our concept of an ideal transition in care began with work by Naylor, who described several important components of a safe transition in care, including complete communication of information, patient education, enlisting the help of social and community supports, ensuring continuity of care, and coordinating care among team members.[8] It is supplemented by the Transitions of Care Consensus Policy Statement proposed by representatives from hospital medicine, primary care, and emergency medicine, which emphasized aspects of timeliness and content of communication between providers.[9] Our present articulation of these key components includes 10 organizing domains.
The Discharge Planning domain highlights the important principle of planning ahead for hospital discharge while the patient is still being treated in the hospital, a paradigm espoused by Project RED[10] and other successful care transitions interventions.[11, 12] Collaborating with the outpatient provider and taking the patient and caregiver's preferences for appointment scheduling into account can help ensure optimal outpatient follow‐up.
Complete Communication of Information refers to the content that should be included in discharge summaries and other means of information transfer from hospital to postdischarge care. The specific content areas are based on the Society of Hospital Medicine and Society of General Internal Medicine Continuity of Care Task Force systematic review and recommendations,[13] which takes into account information requested by primary care physicians after discharge.
Availability, Timeliness, Clarity, and Organization of that information is as important as the content because postdischarge providers must be able to access and quickly understand the information they have been provided before assuming care of the patient.[14, 15]
The Medication Safety domain is of central importance because medications are responsible for most postdischarge adverse events.[16] Taking an accurate medication history,[17] reconciling changes throughout the hospitalization,[18] and communicating the reconciled medication regimen to patients and providers across transitions of care can reduce medication errors and improve patient safety.[19, 20, 21, 22]
The Patient Education and Promotion of Self‐Management domain involves teaching patients and their caregivers about the main hospital diagnoses and instructions for self‐care, including medication changes, appointments, and whom to contact if issues arise. Confirming comprehension of instructions through assessments of acute (delirium) and chronic (dementia) cognitive impairments[23, 24, 25, 26] and teach‐back from the patient (or caregiver) is an important aspect of such counseling, as is providing patients and caregivers with educational materials that are appropriate for their level of health literacy and preferred language.[14] High‐risk patients may benefit from patient coaching to improve their self‐management skills.[12] These recommendations are based on years of health literacy research,[27, 28, 29] and such elements are generally included in effective interventions (including Project RED,[10] Naylor and colleagues' Transitional Care Model,[11] and Coleman and colleagues' Care Transitions Intervention[12]).
Enlisting the help of Social and Community Supports is an important adjunct to medical care and is the rationale for the recent increase in CMS funding for community‐based, care‐transition programs. These programs are crucial for assisting patients with household activities, meals, and other necessities during the period of recovery, though they should be distinguished from care management or care coordination interventions, which have not been found to be helpful in preventing readmissions unless high touch in nature.[30, 31]
The Advanced Care Planning domain may begin in the hospital or outpatient setting, and involves establishing goals of care and healthcare proxies, as well as engaging with palliative care or hospice services if appropriate. Emerging evidence supports the intuitive conclusion that this approach prevents readmissions, particularly in patients who do not benefit from hospital readmission.[32, 33]
Attention to the Coordinating Care Among Team Members domain is needed to synchronize efforts across settings and providers. Clearly, many healthcare professionals as well as other involved parties can be involved in helping a single patient during transitions in care. It is vital that they coordinate information, assessments, and plans as a team.[34]
We recognize the domain of Monitoring and Managing Symptoms After Discharge as increasingly crucial as reflected in our growing understanding of the reasons for readmission, especially among patients with fragile conditions such as heart failure, chronic lung disease, gastrointestinal disorders, dementia,[23, 24, 25, 26] and vascular disease.[35] Monitoring for new or worsening symptoms; medication side effects, discrepancies, or nonadherence; and other self‐management challenges will allow problems to be detected and addressed early, before they result in unplanned healthcare utilization. It is noteworthy that successful interventions in this regard rely on in‐home evaluation[13, 14, 29] by nurses rather than telemonitoring, which in isolation has not been effective to date.[36, 37]
Finally, optimal Outpatient Follow‐Up with appropriate postdischarge providers is crucial for providing ideal transitions. These appointments need to be prompt[38, 39] (eg, within 7 days if not sooner for high‐risk patients) and with providers who have a longitudinal relationship to the patient, as prior work has shown increased readmissions when the provider is unfamiliar with the patient.[40] The advantages and disadvantages of hospitalist‐run postdischarge clinics as one way to increase access and expedite follow‐up are currently being explored. Although the optimal content of a postdischarge visit has not been defined, logical tasks to be completed are myriad and imply the need for checklists, adequate time, and a multidisciplinary team of providers.[41]
IMPLICATIONS OF THE IDEAL TRANSITION IN CARE
Our conceptualization of an ideal transition in care provides insight for hospital and healthcare system leadership, policymakers, researchers, clinicians, and educators seeking to improve transitions of care and reduce hospital readmissions. In the sections below, we briefly review commonly cited concerns about the recent focus on readmissions as a quality measure, illustrate how the Ideal Transition in Care addresses these concerns, and propose fruitful areas for future work.
How Does the Framework Address the Extent to Which Readmissions Reflect Hospital Quality?
One of the chief problems with readmissionrates as a hospital quality measure is that many of the factors that influence readmission may not currently be under the hospital's control. The healthcare environment to which a patient is being discharged (and was admitted from in the first place) is an important determinant of readmission.[42] In this context, it is noteworthy that successful interventions to reduce readmission are generally those that focus on outpatient follow‐up, while inpatient‐only interventions have had less success.[7] This is reflected in our framework above, informed by the literature, highlighting the importance of coordination between inpatient and outpatient providers and the importance of postdischarge care, including monitoring and managing symptoms after discharge, prompt follow‐up appointments, the continuation of patient self‐management activities, monitoring for drug‐related problems after discharge, and the effective utilization of community supports. Accountable care organizations, once established, would be responsible for several components of this environment, including the provision of prompt and effective follow‐up care.
The implication of the framework is that if a hospital does not have control over most of the factors that influence its readmission rate, it should see financial incentives to reduce readmission rates as an opportunity to invest in relationships with the outpatient environment from which their patients are admitted and to which they are discharged. One can envision hospitals growing ever‐closer relationships with their network of primary care physician groups, community agencies, and home health services, rehabilitation facilities, and nursing homes through coordinated discharge planning, medication management, patient education, shared electronic medical records, structured handoffs in care, and systems of intensive outpatient monitoring. Our proposed framework, in other words, emphasizes that hospitals cannot reduce their readmission rates by focusing on aspects of care within their walls. They must forge new and stronger relationships with their communities if they are to be successful.
How Does the Framework Help Us Understand Which Readmissions Are Preventable?
Public reporting and financial penalties are currently tied to all‐cause readmission, but preventable readmissions are a more appealing outcome to target. In one study, the ranking of hospitals by all‐cause readmission rate had very little correlation with the ranking by preventable readmission rate.[5] However, researchers have struggled to establish standardized, valid, and reliable measures for determining what proportion of readmissions are in fact preventable, with estimates ranging from 5% to 79% in the published literature.[43]
The difficulty of accurately determining preventability stems from an inadequate understanding of the roles that patient comorbidities, transitional processes of care, individual patient behaviors, and social and environmental determinants of health play in the complex process of hospital recidivism. Our proposed elements of an ideal transition in care provide a structure to frame this discussion and suggest future research opportunities to allow a more accurate and reliable understanding of the spectrum of preventability. Care system leadership can use the framework to rigorously evaluate their readmissions and determine the extent to which the transitions process approached the ideal. For example, if a readmission occurs despite care processes that addressed most of the domains with high fidelity, it becomes much less likely that the readmission was preventable. It should be noted that the converse is not always true: When a transition falls well short of the ideal, it does not always imply that provision of a more ideal transition would necessarily have prevented the readmission, but it does make it more likely.
For educators, the framework may provide insights for trainees into the complexity of the transitions process and vulnerability of patients during this time, highlighting preventable aspects of readmissions that are within the grasp of the discharging clinician or team. It highlights the importance of medication reconciliation, synchronous communication, and predischarge teaching, which are measurable and teachable skills for non‐physician providers, housestaff, and medical students. It also may allow for more structured feedback, for example, on the quality of discharge summaries produced by trainees.
How Could the Framework Improve Risk Adjustment for Between‐Hospital Comparisons?
Under the Patient Protection and Affordable Care Act (PPACA), hospitals will be compared to one another using risk‐standardized readmission rates as a way to penalize poorly performing hospitals. However, risk‐adjustment models have only modest ability to predict hospital readmission.[6] Moreover, current approaches predominantly adjust for patients' medical comorbidities (which are easily measurable), but they do not adequately take into account the growing literature on other factors that influence readmission rates, including a patient's health literacy, visual or cognitive impairment, functional status, language barriers, and community‐level factors such as social supports.[44, 45]
The Ideal Transition of Care provides a comprehensive framework of hospital discharge quality that provides additional process measures on which hospitals could be compared rather than focusing solely on (inadequately) risk‐adjusted readmission rates. Indeed, most other quality and safety measures (such as the National Quality Forum's Safe Practices[46] and The Joint Commission's National Patient Safety Goals),[47] emphasize process over outcome, in part because of issues of fairness. Process measures are less subject to differences in patient populations and also change the focus from simply reducing readmissions to improving transitional care more broadly. These process measures should be based on our framework and should attempt to capture as many dimensions of an optimal care transition as possible.
Possible examples of process measures include: the accuracy of medication reconciliation at admission and discharge; provision of prompt outpatient follow‐up; provision of adequate systems to monitor and manage symptoms after discharge; advanced care planning in appropriate patients; and the quality of discharge education, incorporating measurements of the patient's understanding and ability to self‐manage their illness. At least some of these could be used now as part of a performance measurement set that highlights opportunities for immediate system change and can serve as performance milestones.
The framework could also be used to validate risk‐adjustment techniques. After accounting for patient factors, the remaining variability in outcomes should be accounted for by processes of care that are in the transitions framework. Once these processes are accurately measured, one can determine if indeed the remaining variability is due to transitions processes, or rather unaccounted factors that are not being measured and that hospitals may have little control over. Such work can lead to iterative refinement of patient risk‐adjustment models.
What Does the Framework Imply About Best Practices for Reducing Readmission Rates?
Despite the limitations of readmission rates as a quality measure noted above, hospitals presently face potentially large financial penalties for readmissions and are allocating resources to readmission reduction efforts. However, hospitals currently may not have enough guidance to know what actions to take to reduce readmissions, and thus could be spending money inefficiently and reducing the value proposition of focusing on readmissions.
A recent systematic review of interventions hospitals could employ to reduce readmissions identified several positive studies, but also many negative studies, and there were significant barriers to understanding what works to reduce readmissions.[7] For example, most of the interventions described in both positive and negative studies were multifaceted, and the authors were unable to identify which components of the intervention were most effective. Also, while several studies have identified risk factors for readmission,[6, 48, 49] very few studies have identified which subgroups of patients benefit most from specific interventions. Few of the studies described key contextual factors that may have led to successful or failed implementation, or the fidelity with which the intervention was implemented.[50, 51, 52]
Few if any of the studies were guided by a concept of the ideal transition in care.[10] Such a framework will better guide development of multifaceted interventions and provide an improved means for interpreting the results. Clearly, rigorously conducted, multicenter studies of readmission prevention interventions are needed to move the field forward. These studies should: 1) correlate implementation of specific intervention components with reductions in readmission rates to better understand the most effective components; 2) be adequately powered to show effect modification, ie, which patients benefit most from these interventions; and 3) rigorously measure environmental context and intervention fidelity, and employ mixed methods to better understand predictors of implementation success and failure.
Our framework can be used in the design and evaluation of such interventions. For example, interventions could be designed that incorporate as many of the domains of an ideal transition as possible, in particular those that span the inpatient and outpatient settings. Processes of care metrics can be developed that measure the extent to which each domain is delivered, analogous to the way the Joint Commission might aggregate individual scores on the 10 items in Acute Myocardial Infarction Core Measure Set[53] to provide a composite of the quality of care provided to patients with this diagnosis. These can be used to correlate certain intervention components with success in reducing readmissions and also in measuring intervention fidelity.
NEXT STEPS
For hospital and healthcare system leaders, who need to take action now to avoid financial penalties, we recommend starting with proven, high‐touch interventions such as Project RED and the Care Transitions Intervention, which are durable, cost‐effective, robustly address multiple domains of the Ideal Transition in Care, and have been implemented at numerous sites.[54, 55] Each hospital or group will need to decide on a bundle of interventions and customize them based on local workflow, resources, and culture.
Risk‐stratification, to match the intensity of the intervention to the risk of readmission of the patient, will undoubtedly be a key component for the efficient use of resources. We anticipate future research will allow risk stratification to be a robust part of any implementation plan. However, as noted above, current risk prediction models are imperfect,[6] and more work is needed to determine which patients benefit most from which interventions. Few if any studies have described interventions tailored to risk for this reason.
Based on our ideal transition in care, our collective experience, and published evidence,[7, 10, 11, 12] potential elements to start with include: early discharge planning; medication reconciliation[56]; patient/caregiver education using health literacy principles, cognitive assessments, and teach‐back to confirm understanding; synchronous communication (eg, by phone) between inpatient and postdischarge providers; follow‐up phone calls to patients within 72 hours of discharge; 24/7 availability of a responsible inpatient provider to address questions and problems (both from the patient/caregiver and from postdischarge providers); and prompt appointments for patients discharged home. High‐risk patients will likely require additional interventions, including in‐home assessments, disease‐monitoring programs, and/or patient coaching. Lastly, patients with certain conditions prone to readmission (such as heart failure and chronic obstructive pulmonary disease) may benefit from disease‐specific programs, including patient education, outpatient disease management, and monitoring protocols.
It is likely that the most effective interventions are those that come from combined, coordinated interventions shared between inpatient and outpatient settings, and are intensive in nature. We expect that the more domains in the framework that are addressed, the safer and more seamless transitions in care will be, with improvement in patient outcomes. To the extent that fragmentation of care has been a barrier to the implementation of these types of interventions in the past, ACOs, perhaps with imbedded Patient‐Centered Medical Homes, may be in the best position to take advantage of newly aligned financial incentives to design comprehensive transitional care. Indeed, we anticipate that Figure 1 may provide substrate for a discussion of postdischarge care and division of responsibilities between inpatient and outpatient care teams at the time of transition, so effort is not duplicated and multiple domains are addressed.
Other barriers to implementation of ideal transitions in care will continue to be an issue for most healthcare systems. Financial constraints that have been a barrier up until now will be partially overcome by penalties for high readmission rates and by ACOs, bundled payments, and alternative care contracts (ie, global payments), but the extent to which each institution feels rewarded for investing in transitional interventions will vary greatly. Healthcare leadership that sees the value of improving transitions in care will be critical to overcoming this barrier. Competing demands (such as lowering hospital length of stay and carrying out other patient care responsibilities),[57] lack of coordination and diffusion of responsibility among various clinical personnel, and lack of standards are other barriers[58] that will require clear prioritization from leadership, policy changes, team‐based care, provider education and feedback, and adequate allocation of personnel resources. In short, process redesign using continuous quality improvement efforts and effective tools will be required to maximize the possibility of success.
CONCLUSIONS
Readmissions are costly and undesirable. Intuition suggests they are a marker of poor care and that hospitals should be capable of reducing them, thereby improving care and decreasing costs. In a potential future world of ACOs based on global payments, financial incentives would be aligned for each system to reduce readmissions below their current baseline, therefore obviating the need for external financial rewards and penalties. In the meantime, financial penalties do exist, and controversy exists over their fairness and likelihood of driving appropriate behavior. To address these controversies and promote better transitional care, we call for the development and use of multifaceted, collaborative transitions interventions that span settings, risk‐adjustment models that allow for fairer comparisons among hospitals, better and more widespread measurement of processes of transitional care, a better understanding of what interventions are most effective and in whom, and better guidance in how to implement these interventions. Our conceptualization of an ideal transition of care serves as a guide and provides a common vocabulary for these efforts. Such research is likely to produce the knowledge needed for healthcare systems to improve transitions in care, reduce readmissions, and reduce costs.
Disclosure
Funding for Dr Vasilevskis has been provided by the National Institutes of Health (K23AG040157) and the VA Tennessee Valley Geriatric Research, Education and Clinical Center (GRECC). The content is solely the responsibility of the authors and does not necessarily represent the official views of the National Institute on Aging, the National Institutes of Health, or the US Department of Veterans Affairs.
- Redefining Health Care: Creating Value‐Based Competition on Results. Boston, MA:Harvard Business School Press;2006. , .
- Rehospitalizations among patients in the Medicare fee‐for‐service program. N Engl J Med. 2009;360(14):1418–1428. , , .
- Patient Protection and Affordable Care Act (PPACA). Public Law 111–148; 2010. Available at: http://www.gpo.gov/fdsys/pkg/PLAW‐111publ148/pdf/PLAW‐111publ148.pdf. Accessed on June 4, 2012.
- Hospital readmission as an accountability measure. JAMA. 2011;305(5):504–505. , .
- Incidence of potentially avoidable urgent readmissions and their relation to all‐cause urgent readmissions. Can Med Assoc J. 2011;183(14):E1067–E1072. , , , et al.
- Risk prediction models for hospital readmission: a systematic review. JAMA. 2011;306(15):1688–1698. , , , et al.
- Interventions to reduce 30‐day rehospitalization: a systematic review. Ann Intern Med. 2011;155(8):520–528. , , , , .
- A decade of transitional care research with vulnerable elders. J Cardiovasc Nurs. 2000;14(3):1–14. .
- for the American College of Physicians; Society of General Internal Medicine; Society of Hospital Medicine; American Geriatrics Society; American College of Emergency Physicians; Society of Academic Emergency Medicine. Transitions of Care Consensus Policy Statement American College of Physicians–Society of General Internal Medicine–Society of Hospital Medicine–American Geriatrics Society–American College of Emergency Physicians–Society of Academic Emergency Medicine. J Gen Intern Med. 2009;24(8):971–976. , , , et al;
- A reengineered hospital discharge program to decrease rehospitalization: a randomized trial. Ann Intern Med. 2009;150(3):178–187. , , , et al.
- Comprehensive discharge planning and home follow‐up of hospitalized elders: a randomized clinical trial. JAMA. 1999;281(7):613–620. , , , et al.
- The care transitions intervention: results of a randomized controlled trial. Arch Intern Med. 2006;166(17):1822–1828. , , , .
- Deficits in communication and information transfer between hospital‐based and primary care physicians: implications for patient safety and continuity of care. JAMA. 2007;297(8):831–841. , , , , , .
- Promoting effective transitions of care at hospital discharge: a review of key issues for hospitalists. J Hosp Med. 2007;2(5):314–323. , , , .
- Discharge documentation of patients discharged to subacute facilities: a three‐year quality improvement process across an integrated health care system. Jt Comm J Qual Patient Saf. 2010;36(6):243–251. , , , , , .
- The incidence and severity of adverse events affecting patients after discharge from the hospital. Ann Intern Med. 2003;138(3):161–167. , , , , .
- Frequency, type and clinical importance of medication history errors at admission to hospital: a systematic review. Can Med Assoc J. 2005;173(5):510–515. , , , , , .
- Classifying and predicting errors of inpatient medication reconciliation. J Gen Intern Med. 2008;23(9):1414–1422. , , , et al.
- for the PILL‐CVD (Pharmacist Intervention for Low Literacy in Cardiovascular Disease) Study Group. Effect of a pharmacist intervention on clinically important medication errors after hospital discharge: a randomized trial. Ann Intern Med. 2012;157(1):1–10. , , et al;
- Hospital‐based medication reconciliation practices: a systematic review. Arch Intern Med. 2012;172(14):1057–1069. , , , .
- Effect of an electronic medication reconciliation application and process redesign on potential adverse drug events: a cluster‐randomized trial. Arch Intern Med. 2009;169(8):771–780. , , , et al.
- Role of pharmacist counseling in preventing adverse drug events after hospitalization. Arch Intern Med. 2006;166(5):565–571. , , , et al.
- Insufficient help for activity of daily living disabilities and risk of all–cause hospitalization. J Am Geriatr Soc. 2012;60(5):927–933. , , , , .
- Transitions in care for older adults with and without dementia. J Am Geriatr Soc. 2012;60(5):813–820. , , , et al.
- Association of incident dementia with hospitalizations. JAMA. 2012;307(2):165–172. , , , , .
- Potentially avoidable hospitalizations of dually eligible Medicare and Medicaid beneficiaries from nursing facility and home– and community–based services waiver programs. J Am Geriatr Soc. 2012;60(5):821–829. , , , et al.
- Teaching about health literacy and clear communication. J Gen Intern Med. 2006;21(8):888–890. , .
- Health literacy and outcomes among patients with heart failure. JAMA. 2011;305(16):1695–1701. , , , et al.
- Patient experiences of transitioning from hospital to home: an ethnographic quality improvement project. J Hosp Med. 2012;7(5):382–387. , , , , .
- Effects of care coordination on hospitalization, quality of care, and health care expenditures among Medicare beneficiaries: 15 randomized trials. JAMA. 2009;301(6):603–618. , , , .
- How changes in Washington University's Medicare coordinated care demonstration pilot ultimately achieved savings. Health Aff (Millwood). 2012;31(6):1216–1226. , , , , .
- Quality of care and rehospitalization rate in the last stage of disease in brain tumor patients assisted at home: a cost effectiveness study. J Palliat Med. 2012;15(2):225–227. , , , et al.
- Inpatient palliative care consults and the probability of hospital readmission. Perm J. 2011;15(2):48–51. , , , , .
- TeamSTEPPS™: team strategies and tools to enhance performance and patient safety. In: Henriksen K, Battles JB, Keyes MA, Grady ML, ed. Advances in Patient Safety: New Directions and Alternative Approaches. Vol 3: Performance and Tools. Rockville, MD:Agency for Healthcare Research and Quality; August2008. , , , et al.
- Factors contributing to all‐cause 30‐day readmissions: a structured case series across 18 hospitals. Med Care. 2012;50(7):599–605. , , , et al.
- Telemonitoring in patients with heart failure [erratum, N Engl J Med. 2011;364(5):490]. N Engl J Med. 2010;363(24):2301–2309. , , , et al.
- A randomized controlled trial of telemonitoring in older adults with multiple health issues to prevent hospitalizations and emergency department visits. Arch Intern Med. 2012;172(10):773–779. , , , et al.
- Relationship between early physician follow‐up and 30‐day readmission among Medicare beneficiaries hospitalized for heart failure. JAMA. 2010;303(17):1716–1722. , , , et al.
- Post‐hospitalization transitions: examining the effects of timing of primary care provider follow‐up. J Hosp Med. 2010;5(7):392–397. , , .
- Does increased access to primary care reduce hospital readmissions? Veterans Affairs Cooperative Study Group on Primary Care and Hospital Readmission. N Engl J Med. 1996;334(22):1441–1447. , , .
- The Post‐Hospital Follow‐Up Visit: A Physician Checklist to Reduce Readmissions. California Healthcare Foundation; October 2010. Available at: http://www.chcf.org/publications/2010/10/the‐post‐hospital‐follow‐up‐visit‐a‐physician‐checklist. Accessed on January 10, 2012. .
- Thirty‐day readmission rates for Medicare beneficiaries by race and site of care. JAMA. 2011;305(7):675–681. , , .
- Proportion of hospital readmissions deemed avoidable: a systematic review. Can Med Assoc J. 2011;183(7):E391–E402. , , , , .
- Postdischarge environmental and socioeconomic factors and the likelihood of early hospital readmission among community‐dwelling Medicare beneficiaries. Gerontologist. 2008;48(4):495–504. , , , et al.
- Low health literacy and health outcomes: an updated systematic review. Ann Intern Med. 2011;155(2):97–107. , , , , .
- National Quality Forum. Safe Practices for Better Healthcare—2010 Update: A Consensus Report. Washington, DC;2010.
- Joint Commission on Accreditation of Healthcare Organizations. Accreditation Program: Hospital 2010 National Patient Safety Goals (NPSGs). 2010. Available at: http://www.jointcommission.org/PatientSafety/NationalPatientSafetyGoals/. Accessed on March 20, 2012.
- Hospital readmission in general medicine patients: a prediction model. J Gen Intern Med. 2010;25(3):211–219. , , , et al.
- Derivation and validation of an index to predict early death or unplanned readmission after discharge from hospital to the community. Can Med Assoc J. 2010;182(6):551–557. , , , et al.
- Evaluating service delivery interventions to enhance patient safety. BMJ. 2008;337:a2764. , .
- Assessing the Evidence for Context‐Sensitive Effectiveness and Safety of Patient Safety Practices: Developing Criteria. Rockville, MD:Agency for Healthcare Research and Quality; December2010. , , .
- Advancing the science of patient safety. Ann Intern Med. 2011;154(10):693–696. , , , et al.
- The Joint Commission. Acute Myocardial Infarction Core Measure Set. Available at: http://www.jointcommission.org/assets/1/6/Acute%20Myocardial%20Infarction.pdf. Accessed August 20,2012.
- The care transitions intervention: translating from efficacy to effectiveness. Arch Intern Med. 2011;171(14):1232–1237. , , , , , .
- Project RED toolkit, AHRQ Innovations Exchange. Available at:http://www.innovations.ahrq.gov/content.aspx?id=2180. Accessed on July 2, 2012.
- A comprehensive pharmacist intervention to reduce morbidity in patients 80 years or older: a randomized controlled trial. Arch Intern Med. 2009;169(9):894–900. , , , et al.
- Thirty‐day readmissions—truth and consequences. N Engl J Med. 2012;366(15):1366–1369. , .
- “Out of sight, out of mind”: housestaff perceptions of quality‐limiting factors in discharge care at teaching hospitals. J Hosp Med. 2012;7(5):376–381. , , , , .
- Redefining Health Care: Creating Value‐Based Competition on Results. Boston, MA:Harvard Business School Press;2006. , .
- Rehospitalizations among patients in the Medicare fee‐for‐service program. N Engl J Med. 2009;360(14):1418–1428. , , .
- Patient Protection and Affordable Care Act (PPACA). Public Law 111–148; 2010. Available at: http://www.gpo.gov/fdsys/pkg/PLAW‐111publ148/pdf/PLAW‐111publ148.pdf. Accessed on June 4, 2012.
- Hospital readmission as an accountability measure. JAMA. 2011;305(5):504–505. , .
- Incidence of potentially avoidable urgent readmissions and their relation to all‐cause urgent readmissions. Can Med Assoc J. 2011;183(14):E1067–E1072. , , , et al.
- Risk prediction models for hospital readmission: a systematic review. JAMA. 2011;306(15):1688–1698. , , , et al.
- Interventions to reduce 30‐day rehospitalization: a systematic review. Ann Intern Med. 2011;155(8):520–528. , , , , .
- A decade of transitional care research with vulnerable elders. J Cardiovasc Nurs. 2000;14(3):1–14. .
- for the American College of Physicians; Society of General Internal Medicine; Society of Hospital Medicine; American Geriatrics Society; American College of Emergency Physicians; Society of Academic Emergency Medicine. Transitions of Care Consensus Policy Statement American College of Physicians–Society of General Internal Medicine–Society of Hospital Medicine–American Geriatrics Society–American College of Emergency Physicians–Society of Academic Emergency Medicine. J Gen Intern Med. 2009;24(8):971–976. , , , et al;
- A reengineered hospital discharge program to decrease rehospitalization: a randomized trial. Ann Intern Med. 2009;150(3):178–187. , , , et al.
- Comprehensive discharge planning and home follow‐up of hospitalized elders: a randomized clinical trial. JAMA. 1999;281(7):613–620. , , , et al.
- The care transitions intervention: results of a randomized controlled trial. Arch Intern Med. 2006;166(17):1822–1828. , , , .
- Deficits in communication and information transfer between hospital‐based and primary care physicians: implications for patient safety and continuity of care. JAMA. 2007;297(8):831–841. , , , , , .
- Promoting effective transitions of care at hospital discharge: a review of key issues for hospitalists. J Hosp Med. 2007;2(5):314–323. , , , .
- Discharge documentation of patients discharged to subacute facilities: a three‐year quality improvement process across an integrated health care system. Jt Comm J Qual Patient Saf. 2010;36(6):243–251. , , , , , .
- The incidence and severity of adverse events affecting patients after discharge from the hospital. Ann Intern Med. 2003;138(3):161–167. , , , , .
- Frequency, type and clinical importance of medication history errors at admission to hospital: a systematic review. Can Med Assoc J. 2005;173(5):510–515. , , , , , .
- Classifying and predicting errors of inpatient medication reconciliation. J Gen Intern Med. 2008;23(9):1414–1422. , , , et al.
- for the PILL‐CVD (Pharmacist Intervention for Low Literacy in Cardiovascular Disease) Study Group. Effect of a pharmacist intervention on clinically important medication errors after hospital discharge: a randomized trial. Ann Intern Med. 2012;157(1):1–10. , , et al;
- Hospital‐based medication reconciliation practices: a systematic review. Arch Intern Med. 2012;172(14):1057–1069. , , , .
- Effect of an electronic medication reconciliation application and process redesign on potential adverse drug events: a cluster‐randomized trial. Arch Intern Med. 2009;169(8):771–780. , , , et al.
- Role of pharmacist counseling in preventing adverse drug events after hospitalization. Arch Intern Med. 2006;166(5):565–571. , , , et al.
- Insufficient help for activity of daily living disabilities and risk of all–cause hospitalization. J Am Geriatr Soc. 2012;60(5):927–933. , , , , .
- Transitions in care for older adults with and without dementia. J Am Geriatr Soc. 2012;60(5):813–820. , , , et al.
- Association of incident dementia with hospitalizations. JAMA. 2012;307(2):165–172. , , , , .
- Potentially avoidable hospitalizations of dually eligible Medicare and Medicaid beneficiaries from nursing facility and home– and community–based services waiver programs. J Am Geriatr Soc. 2012;60(5):821–829. , , , et al.
- Teaching about health literacy and clear communication. J Gen Intern Med. 2006;21(8):888–890. , .
- Health literacy and outcomes among patients with heart failure. JAMA. 2011;305(16):1695–1701. , , , et al.
- Patient experiences of transitioning from hospital to home: an ethnographic quality improvement project. J Hosp Med. 2012;7(5):382–387. , , , , .
- Effects of care coordination on hospitalization, quality of care, and health care expenditures among Medicare beneficiaries: 15 randomized trials. JAMA. 2009;301(6):603–618. , , , .
- How changes in Washington University's Medicare coordinated care demonstration pilot ultimately achieved savings. Health Aff (Millwood). 2012;31(6):1216–1226. , , , , .
- Quality of care and rehospitalization rate in the last stage of disease in brain tumor patients assisted at home: a cost effectiveness study. J Palliat Med. 2012;15(2):225–227. , , , et al.
- Inpatient palliative care consults and the probability of hospital readmission. Perm J. 2011;15(2):48–51. , , , , .
- TeamSTEPPS™: team strategies and tools to enhance performance and patient safety. In: Henriksen K, Battles JB, Keyes MA, Grady ML, ed. Advances in Patient Safety: New Directions and Alternative Approaches. Vol 3: Performance and Tools. Rockville, MD:Agency for Healthcare Research and Quality; August2008. , , , et al.
- Factors contributing to all‐cause 30‐day readmissions: a structured case series across 18 hospitals. Med Care. 2012;50(7):599–605. , , , et al.
- Telemonitoring in patients with heart failure [erratum, N Engl J Med. 2011;364(5):490]. N Engl J Med. 2010;363(24):2301–2309. , , , et al.
- A randomized controlled trial of telemonitoring in older adults with multiple health issues to prevent hospitalizations and emergency department visits. Arch Intern Med. 2012;172(10):773–779. , , , et al.
- Relationship between early physician follow‐up and 30‐day readmission among Medicare beneficiaries hospitalized for heart failure. JAMA. 2010;303(17):1716–1722. , , , et al.
- Post‐hospitalization transitions: examining the effects of timing of primary care provider follow‐up. J Hosp Med. 2010;5(7):392–397. , , .
- Does increased access to primary care reduce hospital readmissions? Veterans Affairs Cooperative Study Group on Primary Care and Hospital Readmission. N Engl J Med. 1996;334(22):1441–1447. , , .
- The Post‐Hospital Follow‐Up Visit: A Physician Checklist to Reduce Readmissions. California Healthcare Foundation; October 2010. Available at: http://www.chcf.org/publications/2010/10/the‐post‐hospital‐follow‐up‐visit‐a‐physician‐checklist. Accessed on January 10, 2012. .
- Thirty‐day readmission rates for Medicare beneficiaries by race and site of care. JAMA. 2011;305(7):675–681. , , .
- Proportion of hospital readmissions deemed avoidable: a systematic review. Can Med Assoc J. 2011;183(7):E391–E402. , , , , .
- Postdischarge environmental and socioeconomic factors and the likelihood of early hospital readmission among community‐dwelling Medicare beneficiaries. Gerontologist. 2008;48(4):495–504. , , , et al.
- Low health literacy and health outcomes: an updated systematic review. Ann Intern Med. 2011;155(2):97–107. , , , , .
- National Quality Forum. Safe Practices for Better Healthcare—2010 Update: A Consensus Report. Washington, DC;2010.
- Joint Commission on Accreditation of Healthcare Organizations. Accreditation Program: Hospital 2010 National Patient Safety Goals (NPSGs). 2010. Available at: http://www.jointcommission.org/PatientSafety/NationalPatientSafetyGoals/. Accessed on March 20, 2012.
- Hospital readmission in general medicine patients: a prediction model. J Gen Intern Med. 2010;25(3):211–219. , , , et al.
- Derivation and validation of an index to predict early death or unplanned readmission after discharge from hospital to the community. Can Med Assoc J. 2010;182(6):551–557. , , , et al.
- Evaluating service delivery interventions to enhance patient safety. BMJ. 2008;337:a2764. , .
- Assessing the Evidence for Context‐Sensitive Effectiveness and Safety of Patient Safety Practices: Developing Criteria. Rockville, MD:Agency for Healthcare Research and Quality; December2010. , , .
- Advancing the science of patient safety. Ann Intern Med. 2011;154(10):693–696. , , , et al.
- The Joint Commission. Acute Myocardial Infarction Core Measure Set. Available at: http://www.jointcommission.org/assets/1/6/Acute%20Myocardial%20Infarction.pdf. Accessed August 20,2012.
- The care transitions intervention: translating from efficacy to effectiveness. Arch Intern Med. 2011;171(14):1232–1237. , , , , , .
- Project RED toolkit, AHRQ Innovations Exchange. Available at:http://www.innovations.ahrq.gov/content.aspx?id=2180. Accessed on July 2, 2012.
- A comprehensive pharmacist intervention to reduce morbidity in patients 80 years or older: a randomized controlled trial. Arch Intern Med. 2009;169(9):894–900. , , , et al.
- Thirty‐day readmissions—truth and consequences. N Engl J Med. 2012;366(15):1366–1369. , .
- “Out of sight, out of mind”: housestaff perceptions of quality‐limiting factors in discharge care at teaching hospitals. J Hosp Med. 2012;7(5):376–381. , , , , .
In the Literature: Research You Need to Know
Clinical question: What is the current level of continuity of care, and what factors affect continuity of care in the hospital setting? Has this changed with increasing use of hospitalists and limits on residency duty hours?
Background: Outpatient continuity of care leads to lower costs, better quality of life, and less emergency room use. Recent changes in residency hours have increased hand-offs and decreased inpatient continuity, but to what extent is unknown.
Study design: Retrospective cohort of 5% of Medicare claims data (530,000 patients in all) from 1996 to 2006, including patients admitted for COPD, congestive heart failure, or pneumonia who were cared for by a general internist or family practitioner.
Setting: Nationwide in the U.S.
Synopsis: The authors defined patients as having a primary-care physician (PCP) if they had three billed visits with the PCP in the last year, hospitalists as those who derived at least 90% of their Medicare claims from inpatient billing, and other generalists as those who met criteria as a generalist but did not fit these categories. Inpatient continuity of care decreased to 59% of patients seeing a single physician in the hospital in 2006 from 71% in 1996, with an accompanying decrease in the length of stay of one full day. There were large variations by geographic region, population size, and hospital characteristics. Patients cared for by hospitalists had slightly better continuity of care than those cared for by nonhospitalist generalists, and those who were cared for by both hospitalists and nonhospitalist generalists had the worst continuity of care. Having a PCP was associated with increased discontinuity of care as an inpatient, perhaps because of individual members of a practice rounding on all of the practice’s inpatients.
Bottom line: Patients were 5% less likely per year between 1996 and 2006 to have a single physician be their primary caregiver in the hospital, but the rise of the hospitalist movement does not seem to be the cause.
Citation: Fletcher KE, Sharma G, Zhang D, Kuo YF, Goodwin JS. Trends in inpatient continuity of care for a cohort of Medicare patients 1996-2006. J Hosp Med. 2011;6:441-447.
For more physician reviews of HM-relevant literature, visit our website.
Clinical question: What is the current level of continuity of care, and what factors affect continuity of care in the hospital setting? Has this changed with increasing use of hospitalists and limits on residency duty hours?
Background: Outpatient continuity of care leads to lower costs, better quality of life, and less emergency room use. Recent changes in residency hours have increased hand-offs and decreased inpatient continuity, but to what extent is unknown.
Study design: Retrospective cohort of 5% of Medicare claims data (530,000 patients in all) from 1996 to 2006, including patients admitted for COPD, congestive heart failure, or pneumonia who were cared for by a general internist or family practitioner.
Setting: Nationwide in the U.S.
Synopsis: The authors defined patients as having a primary-care physician (PCP) if they had three billed visits with the PCP in the last year, hospitalists as those who derived at least 90% of their Medicare claims from inpatient billing, and other generalists as those who met criteria as a generalist but did not fit these categories. Inpatient continuity of care decreased to 59% of patients seeing a single physician in the hospital in 2006 from 71% in 1996, with an accompanying decrease in the length of stay of one full day. There were large variations by geographic region, population size, and hospital characteristics. Patients cared for by hospitalists had slightly better continuity of care than those cared for by nonhospitalist generalists, and those who were cared for by both hospitalists and nonhospitalist generalists had the worst continuity of care. Having a PCP was associated with increased discontinuity of care as an inpatient, perhaps because of individual members of a practice rounding on all of the practice’s inpatients.
Bottom line: Patients were 5% less likely per year between 1996 and 2006 to have a single physician be their primary caregiver in the hospital, but the rise of the hospitalist movement does not seem to be the cause.
Citation: Fletcher KE, Sharma G, Zhang D, Kuo YF, Goodwin JS. Trends in inpatient continuity of care for a cohort of Medicare patients 1996-2006. J Hosp Med. 2011;6:441-447.
For more physician reviews of HM-relevant literature, visit our website.
Clinical question: What is the current level of continuity of care, and what factors affect continuity of care in the hospital setting? Has this changed with increasing use of hospitalists and limits on residency duty hours?
Background: Outpatient continuity of care leads to lower costs, better quality of life, and less emergency room use. Recent changes in residency hours have increased hand-offs and decreased inpatient continuity, but to what extent is unknown.
Study design: Retrospective cohort of 5% of Medicare claims data (530,000 patients in all) from 1996 to 2006, including patients admitted for COPD, congestive heart failure, or pneumonia who were cared for by a general internist or family practitioner.
Setting: Nationwide in the U.S.
Synopsis: The authors defined patients as having a primary-care physician (PCP) if they had three billed visits with the PCP in the last year, hospitalists as those who derived at least 90% of their Medicare claims from inpatient billing, and other generalists as those who met criteria as a generalist but did not fit these categories. Inpatient continuity of care decreased to 59% of patients seeing a single physician in the hospital in 2006 from 71% in 1996, with an accompanying decrease in the length of stay of one full day. There were large variations by geographic region, population size, and hospital characteristics. Patients cared for by hospitalists had slightly better continuity of care than those cared for by nonhospitalist generalists, and those who were cared for by both hospitalists and nonhospitalist generalists had the worst continuity of care. Having a PCP was associated with increased discontinuity of care as an inpatient, perhaps because of individual members of a practice rounding on all of the practice’s inpatients.
Bottom line: Patients were 5% less likely per year between 1996 and 2006 to have a single physician be their primary caregiver in the hospital, but the rise of the hospitalist movement does not seem to be the cause.
Citation: Fletcher KE, Sharma G, Zhang D, Kuo YF, Goodwin JS. Trends in inpatient continuity of care for a cohort of Medicare patients 1996-2006. J Hosp Med. 2011;6:441-447.
For more physician reviews of HM-relevant literature, visit our website.
In the Literature: Physician Reviews of HM-Relevant Research
In This Edition
Literature At A Glance
A guide to this month’s studies
- Higher loading dose of clopidogrel in STEMI
- Early vs. late surgery following hip fracture
- Beta-blockers in chronic kidney disease
- Long-term azithromycin in COPD
- CT screening for lung cancer
- Timing of parenteral nutrition in the ICU
- Intrapleural management of empyema with DNase and t-PA
- Effect of weekend elective admissions on hospital flow
- Expectations and outcomes of medical comanagement
Higher-Dose Clopidogrel Improves Outcomes at 30 Days in STEMI Patients
Clinical question: Does a 600-mg loading dose of clopidogrel given before percutaneous coronary intervention (PCI) in patients with an ST-segment elevation myocardial infarction (STEMI) provide more protection from thrombotic complications at 30 days than a 300-mg dose?
Background: Clopidogrel at 600 mg is active more quickly (two hours versus 12 hours) and inhibits platelets more completely than does a 300-mg dose, but it has never been tested prospectively in patients undergoing percutaneous intervention with a STEMI.
Study design: Prospective, multicenter, randomized controlled trial.
Setting: Five hospitals in Italy, Belgium, Serbia, and Hungary.
Synopsis: Two-hundred-one patients with STEMI were randomized to either 600 mg or 300 mg of clopidogrel, given an average of 30 minutes before initial PCI, as well as other standard treatment for STEMI. The primary outcome was “infarct size,” judged as the area under the curve (AUC) of serial creatine kinase-MB (CK-MB) and troponin measurements. At 30 days, patients who received the 600-mg dose of clopidogrel had lower AUCs of cardiac biomarkers, statistically significant (though clinically small) improvement in left ventricle ejection fraction at discharge, lower incidence of severely impaired post-PCI thrombolitis in myocardial infarction (TIMI) flow, and fewer “major cardiovascular events,” driven mainly by a reduction in revascularizations. Measurement of biomarkers to calculate infarct size can be confounded by hypertrophy, and the trial was underpowered for cardiovascular events. However, there was no increase in bleeding events.
Bottom line: In patients with STEMI, treatment with a higher loading dose of clopidogrel before PCI reduces revascularizations and might decrease infarct size without an increase in adverse events.
Citation: Patti G, Bárczi G, Orlic D, et al. Outcome comparison of 600- and 300-mg loading doses of clopidogrel in patients undergoing primary percutaneous coronary intervention for ST-segment elevation myocardial infarction: results from the ARMYDA-6 MI (Antiplatelet therapy for Reduction of MYocardial Damage during Angioplasty-Myocardial Infarction) randomized study. J Am Coll Cardiol. 2011;58:1592-1599.
Medical Comorbidities Explain Most of Excess Risk Seen in Patients with Delayed Hip Fracture Repair
Clinical question: Could the increased mortality found with delays in hip fracture surgery be confounded by the premorbid functional status and medical comorbidities of patients whose surgery is more likely to be delayed?
Background: Guidelines recommend operating on patients with hip fracture within 24 hours, but the supporting evidence has not adjusted for underlying medical comorbidities, which could delay surgery and contribute to poor outcomes, making delays look harmful.
Study design: Prospective cohort, single-center design.
Setting: University hospital in Spain.
Synopsis: The study included 2,250 consecutive elderly patients admitted to the hospital for hip fracture who had their functional status and medical comorbidities assessed at enrollment. If their surgery was delayed beyond 24 hours, the reason was sought. Medical outcomes assessed daily while in hospital were delirium, pneumonia, heart failure, urinary tract infection, and new pressure sores, while the dose of pain medication, surgical complications, and in-hospital mortality were also compiled. No post-discharge data were available.
The median time to surgery was 72 hours. Patients with more medical comorbidities and poorer preoperative functional status had longer delays to operation, most commonly due to interrupting antiplatelet treatment or need for preoperative “echocardiography or other examinations.” When these medical factors were included in logistic regression analysis, the increased mortality seen with delays of surgery in the cohort was no longer statistically significant, suggesting the underlying comorbidities of these patients, rather than the delay to surgery itself, explained the increased mortality.
Bottom line: Delaying hip fracture surgery is less important than preoperative functional status and medical comorbidity in contributing to poor outcomes.
Citation: Vidán MT, Sánchez E, Gracia Y, Marañón E, Vaquero J, Serra JA. Causes and effects of surgical delay in patients with hip fracture: a cohort study. Ann Intern Med. 2011;155:226-233.
Beta-Blockers Decrease All-Cause and Cardiovascular Mortality in Patients with Chronic Kidney Disease
Clinical question: Are beta-blockers as effective in patients on dialysis and with end-stage chronic kidney disease (CKD) as they are in patients without severe renal disease?
Background: Patients with CKD have been largely excluded from trials of beta-blocker therapy even though they have high rates of cardiovascular disease and might be most likely to benefit. However, patients on dialysis might be predisposed to adverse complications of beta-blocker therapy, including hypotension.
Study design: Meta-analysis of eight trials of beta-blockade versus placebo (six heart failure trials) or ACE-I (two non-heart-failure trials) that included post-hoc analyses of CKD patients.
Setting: Varied, usually multinational RCTs.
Synopsis: The six congestive heart failure (CHF) studies were not designed to evaluate patients with CKD, and the two non-CHF studies were intended to evaluate progression of CKD, not cardiac outcomes. Thus, this is a meta-analysis of post-hoc CKD subgroups included in these trials. Compared with placebo, beta-blockers reduced all-cause and cardiovascular mortality without significant heterogeneity between trials. The magnitude of the effect was similar in CKD and non-CKD patients. Patients with CKD treated with beta-blockers were at increased risk of bradycardia and hypotension, but this did not lead to increased discontinuation of the drug. Only 114 dialysis patients were included in one of the eight trials (7,000 patients overall) and no outcomes were assessed.
Bottom line: Beta-blockers lower all-cause and cardiovascular mortality in patients with CKD similarly to patients without kidney disease but are associated with an increased risk for hypotension and bradycardia. Their effect in dialysis patients is unknown.
Citation: Badve SV, Roberts MA, Hawley CM, et al. Effects of beta-adrenergic antagonists in patients with chronic kidney disease: a systematic review and meta-analysis. J Am Coll Cardiol. 2011;58:1152-1161.
Daily Long-Term Azithromycin in COPD Patients Decreases Frequency of Exacerbations
Clinical question: Does long-term treatment with azithromycin decrease COPD exacerbations and improve quality of life with an acceptable risk profile?
Background: Patients with acute exacerbations of COPD have increased risks of death and more rapid decline in lung function. Macrolide antibiotics might decrease exacerbations via antibacterial and anti-inflammatory effects. Small studies of long-term treatment with macrolides in COPD have had conflicting results.
Study design: Prospective, multicenter, parallel-group, placebo-controlled trial.
Setting: Twelve U.S. academic health centers.
Synopsis: Eligible patients were those older than 40 with pulmonary function test-proven obstructive disease, a significant smoking history, a use of supplemental oxygen, or an oral glucocorticoid treatment in the previous year, and those who had previously come to healthcare attention for a COPD exacerbation. They were randomized to daily azithromycin (250 mg) or placebo. The primary outcome was time to first COPD exacerbation at one year. The investigators used deep nasopharyngeal swabs to evaluate the development of microbial resistance, and all patients were regularly screened for hearing loss. Time to first exacerbation and frequency of exacerbations decreased significantly with azithromycin treatment; the number needed to treat to prevent a COPD exacerbation was 2.86. More than half of nasopharyngeal samples had evidence of macrolide resistance at enrollment, and this increased to more than 80% at one year. Audiometric testing did show a decrease in hearing in those treated with azithromycin, but even those who did not stop their medication had recovery on subsequent testing.
Bottom line: Daily azithromycin decreases COPD exacerbations in those with a history of previous exacerbation at the cost of increased macrolide resistance and possible reversible decrements in hearing. The high degree of macrolide resistance at enrollment suggests non-antibacterial mechanisms might be responsible.
Citation: Albert RK, Connett J, Bailey WC, et al. Azithromycin for prevention of exacerbations of COPD. N Engl J Med. 2011;365:689-698.
Serial Low-Dose CT Scans Decrease Lung Cancer Mortality
Clinical question: Does annual screening with low-dose CT scans reduce mortality from lung cancer in current or former heavy smokers?
Background: Lung cancer is still the top cause of cancer death in the United States, and its prevalence is increasing in the developing world. Prior trials of screening chest radiography and sputum samples have not shown a decrease in lung cancer mortality, but CT scans could help identify lung cancer at an earlier, more treatable stage.
Study design: Prospective, multicenter, randomized trial of low-dose (roughly one-fifth the radiation of traditional CT) annual CT versus annual chest radiography.
Setting: Thirty-three U.S. medical institutions.
Synopsis: The study enrolled 53,454 current or prior smokers with a history of smoking more than 30 packs a year who were randomly assigned to undergo three annual CT scans or chest X-rays. Any CT scans that showed a noncalcified nodule greater than 4 mm and chest X-rays that showed a noncalcified nodule or mass of any size were classified as “positive,” but follow-up was left to the discretion of the treating physicians. Participants were followed for 6.5 years on average.
Of those screened with CT, 40% had a positive screening test at some point, more than three times the rate of chest radiography, and 96.4% of these were false-positives, which was similar to chest radiography. Adverse events as a result of workup of eventual false positives were uncommon, occurring in around 1% of those who did not have lung cancer and in 11% of those who did. Screening reduced lung cancer mortality by 20% compared with chest radiography, with a number needed to screen to prevent one lung cancer death of 320. Cost-effectiveness and effects of radiation were not assessed.
Bottom line: Serial screening with low-dose CT reduces lung cancer mortality at the cost of a high rate of false-positives. Questions remain about cost-effectiveness and radiation exposure.
Citation: National Lung Screening Trial Research Team, Aberle DR, Adams AM, Berg CD, et al. Reduced lung-cancer mortality with low-dose computed tomographic screening. N Engl J Med. 2011;365:395-409.
One-Week Delay in Starting Parenteral Nutrition in ICU Patients Is Associated with Better Outcomes
Clinical question: In patients admitted to the ICU who are at risk for malnutrition, does supplementing enteral feeding with parenteral feeding on the day of admission improve outcomes when compared with supplementation starting on Day 8?
Background: Patients with critical illness are at risk for malnutrition, which may lead to worsened outcomes. Many are unable to tolerate enteral feeding. However, adding parenteral nutrition has risks, including overfeeding and hyperglycemia.
Study design: Prospective, multicenter, randomized controlled trial. Neither patients/families nor ICU physicians were blinded.
Setting: Two university hospitals in Belgium.
Synopsis: The study included 4,640 patients admitted to the ICU who were “at risk” for malnutrition (assessed using a Nutritional Risk Score of >3). They were randomized to early initiation of parenteral nutrition on Day 1 or late initiation of parenteral nutrition on Day 8, in both cases only if needed to supplement enteral feeding. Both groups received intensive IV insulin protocols to keep blood glucose at 80 mg/dL to 110 mg/dL. The study populations were well-matched, with similar APACHE II scores. Of the total patients, 42% were emergency admissions to the ICU (more than half of which had sepsis), while scheduled cardiac surgery patients made up the majority of the rest of the study participants.
Patients with late initiation of total parenteral nutrition (TPN) were more likely to be discharged alive from the ICU within eight days despite having increased risk of hypoglycemia and more elevation of inflammatory markers. They had shorter ICU and hospital stays, fewer days on the ventilator, fewer infectious complications, were less likely to develop renal failure, and had lower overall costs. The unblinded management of these patients raises concern in interpreting infectious outcomes because study investigators could have been biased to look for infection more often in the early initiation group.
Bottom line: In ICU patients at risk for malnutrition, delaying initiation of TPN to supplement enteral feeding shortens ICU stay and reduces infectious complications when compared with early initiation of TPN.
Citation: Casaer MP, Mesotten D, Hermans G, et al. Early versus late parenteral nutrition in critically ill adults. N Engl J Med. 2011;365:506-17.
Intrapleural Instillation of Combined DNase and t-PA Improve Outcomes in Patients with Empyema
Clinical question: Does the intrapleural administration of a fibrinolytic, a recombinant DNase, or a combination of the two improve outcomes in patients with pleural infections compared with placebo?
Background: Pleural-based infections confer high morbidity and mortality, especially in the one-third of cases in which chest tube drainage fails. Observational data suggest intrapleural administration of fibrinolytics can improve drainage; however, a large randomized trial (MIST1) failed to show improvement with streptokinase.
Study design: Randomized, double-blind, double-dummy, factorial, multicenter trial.
Setting: University hospitals in the U.K.
Synopsis: A total of 193 patients with clinical evidence of an infection as well as laboratory evidence of pleural infection were randomized in factorial design to placebo, DNase alone, t-PA alone, and DNase plus t-PA as a twice-daily, hourlong instillation into the pleural space on hospital days 1 through 3. The combination group had improvements in the size of the pleural effusion compared with placebo, the primary endpoint. This group also was less likely to be referred for surgery, had shorter hospital stays, had less fever, and had lower inflammatory markers by hospital Day 7. Neither of the single-agent groups were better than placebo. The confidence intervals for all outcomes besides radiographic size of the effusion were large, as the trial had limited power for secondary endpoints.
Bottom line: In patients with empyema, the addition of twice-daily instillations of DNase and tPA can improve drainage and decrease risk of treatment failure when combined with chest tube drainage and antibiotics.
Citation: Rahman NM, Maskell NA, West A, et al. Intrapleural use of tissue plasminogen activator and DNase in pleural infection. N Engl J Med. 2011;365:518-526.
Smoothing Admissions over the Week Improves Patient Flow
Clinical question: What is the difference between weekday and weekend hospital occupancy, and what might the effect be of redistributing, or “smoothing,” elective admissions across the week?
Background: Efforts to improve patient flow have largely focused on reducing the average length of stay (ALOS). As the ALOS decreases, though, further reductions have limited yield in improving patient flow and could occur at the expense of patient safety. Smoothing admissions is a recognized but underutilized tool to address patient flow and hospital overcrowding.
Study design: Retrospective cohort.
Setting: Thirty-nine freestanding, tertiary-care children’s hospitals in the U.S.
Synopsis: Hospital occupancy ranged from 70.9% to 108.1% for weekdays, and from 65.7% to 94.4% on weekends. The mean difference between weekday and weekend occupancy was 8.2%. Using a mathematical model to redistribute admissions from peak days to nonpeak days (within a one-week time frame), the investigators found that percent occupancy on average dropped by 6.6%—the same number of patients over the same time interval, but with lower average occupancy. And, while not projected by these authors, the correlate of lower average occupancy would be reduced waits and delays for admission and within-hospital services. Only 2.6%, or about 7.5 admissions per week per hospital, would need to be rescheduled in order to realize this gain.
Bottom line: Where feasible, reshuffling elective admissions to “smooth” demand across the week is associated with improved hospital flow.
Citation: Fieldston ES, Hall M, Shah SS, et al. Addressing inpatient crowding by smoothing occupancy at children’s hospitals. J Hosp Med. 2011;6:466-473.
Variability in Preferences, Experiences, and Expectations for Hospitalist Roles in Comanagement
Clinical question: Do provider expectations and experiences in comanagement differ from the traditional medical consultation model?
Background: Hospitalists participate in traditional medical consultation and, increasingly, a variety of comanagement with surgical and various medical specialists. It is uncertain what preferences and expectations on either side of the comanagement relationship might be. Learning more might lead to a better conceptual understanding and working definition of inpatient comanagement.
Study design: Baseline and follow-up surveys.
Setting: Large single-site academic medical center, hospitalist-hepatologist comanagement service.
Synopsis: All 41 respondents completed the baseline survey, with 32 respondents completing a total of 177 follow-up surveys. Respondents included hospitalists, hepatologists, nonphysician providers (NPPs), and GI fellows. Hospitalists were more likely than hepatologists to want to be informed about every patient care decision (83% versus 17%). The majority of respondents reported understanding their roles. At baseline, nearly all agreed that “comanagement tends to improve patient care.” However, at follow-up, fewer NPP and fewer GI fellows reported that patients on their service had received “better care than they would without comanagement.” At follow-up, fewer hospitalists than at baseline reported that there was one “physician leader to direct the overall management of the patient’s hospital course.”
Bottom line: Hospitalists were more likely than hepatologists, within a comanagement relationship, to want to be informed about every decision. Most expected—but fewer actually later reported—that the comanagement model improved patient care.
Citation: Hinami K, Whelan CT, Konetzka RT, and Meltzer DO. Provider expectations and experiences of comanagement. J Hosp Med. 2011;6:401-404.
In This Edition
Literature At A Glance
A guide to this month’s studies
- Higher loading dose of clopidogrel in STEMI
- Early vs. late surgery following hip fracture
- Beta-blockers in chronic kidney disease
- Long-term azithromycin in COPD
- CT screening for lung cancer
- Timing of parenteral nutrition in the ICU
- Intrapleural management of empyema with DNase and t-PA
- Effect of weekend elective admissions on hospital flow
- Expectations and outcomes of medical comanagement
Higher-Dose Clopidogrel Improves Outcomes at 30 Days in STEMI Patients
Clinical question: Does a 600-mg loading dose of clopidogrel given before percutaneous coronary intervention (PCI) in patients with an ST-segment elevation myocardial infarction (STEMI) provide more protection from thrombotic complications at 30 days than a 300-mg dose?
Background: Clopidogrel at 600 mg is active more quickly (two hours versus 12 hours) and inhibits platelets more completely than does a 300-mg dose, but it has never been tested prospectively in patients undergoing percutaneous intervention with a STEMI.
Study design: Prospective, multicenter, randomized controlled trial.
Setting: Five hospitals in Italy, Belgium, Serbia, and Hungary.
Synopsis: Two-hundred-one patients with STEMI were randomized to either 600 mg or 300 mg of clopidogrel, given an average of 30 minutes before initial PCI, as well as other standard treatment for STEMI. The primary outcome was “infarct size,” judged as the area under the curve (AUC) of serial creatine kinase-MB (CK-MB) and troponin measurements. At 30 days, patients who received the 600-mg dose of clopidogrel had lower AUCs of cardiac biomarkers, statistically significant (though clinically small) improvement in left ventricle ejection fraction at discharge, lower incidence of severely impaired post-PCI thrombolitis in myocardial infarction (TIMI) flow, and fewer “major cardiovascular events,” driven mainly by a reduction in revascularizations. Measurement of biomarkers to calculate infarct size can be confounded by hypertrophy, and the trial was underpowered for cardiovascular events. However, there was no increase in bleeding events.
Bottom line: In patients with STEMI, treatment with a higher loading dose of clopidogrel before PCI reduces revascularizations and might decrease infarct size without an increase in adverse events.
Citation: Patti G, Bárczi G, Orlic D, et al. Outcome comparison of 600- and 300-mg loading doses of clopidogrel in patients undergoing primary percutaneous coronary intervention for ST-segment elevation myocardial infarction: results from the ARMYDA-6 MI (Antiplatelet therapy for Reduction of MYocardial Damage during Angioplasty-Myocardial Infarction) randomized study. J Am Coll Cardiol. 2011;58:1592-1599.
Medical Comorbidities Explain Most of Excess Risk Seen in Patients with Delayed Hip Fracture Repair
Clinical question: Could the increased mortality found with delays in hip fracture surgery be confounded by the premorbid functional status and medical comorbidities of patients whose surgery is more likely to be delayed?
Background: Guidelines recommend operating on patients with hip fracture within 24 hours, but the supporting evidence has not adjusted for underlying medical comorbidities, which could delay surgery and contribute to poor outcomes, making delays look harmful.
Study design: Prospective cohort, single-center design.
Setting: University hospital in Spain.
Synopsis: The study included 2,250 consecutive elderly patients admitted to the hospital for hip fracture who had their functional status and medical comorbidities assessed at enrollment. If their surgery was delayed beyond 24 hours, the reason was sought. Medical outcomes assessed daily while in hospital were delirium, pneumonia, heart failure, urinary tract infection, and new pressure sores, while the dose of pain medication, surgical complications, and in-hospital mortality were also compiled. No post-discharge data were available.
The median time to surgery was 72 hours. Patients with more medical comorbidities and poorer preoperative functional status had longer delays to operation, most commonly due to interrupting antiplatelet treatment or need for preoperative “echocardiography or other examinations.” When these medical factors were included in logistic regression analysis, the increased mortality seen with delays of surgery in the cohort was no longer statistically significant, suggesting the underlying comorbidities of these patients, rather than the delay to surgery itself, explained the increased mortality.
Bottom line: Delaying hip fracture surgery is less important than preoperative functional status and medical comorbidity in contributing to poor outcomes.
Citation: Vidán MT, Sánchez E, Gracia Y, Marañón E, Vaquero J, Serra JA. Causes and effects of surgical delay in patients with hip fracture: a cohort study. Ann Intern Med. 2011;155:226-233.
Beta-Blockers Decrease All-Cause and Cardiovascular Mortality in Patients with Chronic Kidney Disease
Clinical question: Are beta-blockers as effective in patients on dialysis and with end-stage chronic kidney disease (CKD) as they are in patients without severe renal disease?
Background: Patients with CKD have been largely excluded from trials of beta-blocker therapy even though they have high rates of cardiovascular disease and might be most likely to benefit. However, patients on dialysis might be predisposed to adverse complications of beta-blocker therapy, including hypotension.
Study design: Meta-analysis of eight trials of beta-blockade versus placebo (six heart failure trials) or ACE-I (two non-heart-failure trials) that included post-hoc analyses of CKD patients.
Setting: Varied, usually multinational RCTs.
Synopsis: The six congestive heart failure (CHF) studies were not designed to evaluate patients with CKD, and the two non-CHF studies were intended to evaluate progression of CKD, not cardiac outcomes. Thus, this is a meta-analysis of post-hoc CKD subgroups included in these trials. Compared with placebo, beta-blockers reduced all-cause and cardiovascular mortality without significant heterogeneity between trials. The magnitude of the effect was similar in CKD and non-CKD patients. Patients with CKD treated with beta-blockers were at increased risk of bradycardia and hypotension, but this did not lead to increased discontinuation of the drug. Only 114 dialysis patients were included in one of the eight trials (7,000 patients overall) and no outcomes were assessed.
Bottom line: Beta-blockers lower all-cause and cardiovascular mortality in patients with CKD similarly to patients without kidney disease but are associated with an increased risk for hypotension and bradycardia. Their effect in dialysis patients is unknown.
Citation: Badve SV, Roberts MA, Hawley CM, et al. Effects of beta-adrenergic antagonists in patients with chronic kidney disease: a systematic review and meta-analysis. J Am Coll Cardiol. 2011;58:1152-1161.
Daily Long-Term Azithromycin in COPD Patients Decreases Frequency of Exacerbations
Clinical question: Does long-term treatment with azithromycin decrease COPD exacerbations and improve quality of life with an acceptable risk profile?
Background: Patients with acute exacerbations of COPD have increased risks of death and more rapid decline in lung function. Macrolide antibiotics might decrease exacerbations via antibacterial and anti-inflammatory effects. Small studies of long-term treatment with macrolides in COPD have had conflicting results.
Study design: Prospective, multicenter, parallel-group, placebo-controlled trial.
Setting: Twelve U.S. academic health centers.
Synopsis: Eligible patients were those older than 40 with pulmonary function test-proven obstructive disease, a significant smoking history, a use of supplemental oxygen, or an oral glucocorticoid treatment in the previous year, and those who had previously come to healthcare attention for a COPD exacerbation. They were randomized to daily azithromycin (250 mg) or placebo. The primary outcome was time to first COPD exacerbation at one year. The investigators used deep nasopharyngeal swabs to evaluate the development of microbial resistance, and all patients were regularly screened for hearing loss. Time to first exacerbation and frequency of exacerbations decreased significantly with azithromycin treatment; the number needed to treat to prevent a COPD exacerbation was 2.86. More than half of nasopharyngeal samples had evidence of macrolide resistance at enrollment, and this increased to more than 80% at one year. Audiometric testing did show a decrease in hearing in those treated with azithromycin, but even those who did not stop their medication had recovery on subsequent testing.
Bottom line: Daily azithromycin decreases COPD exacerbations in those with a history of previous exacerbation at the cost of increased macrolide resistance and possible reversible decrements in hearing. The high degree of macrolide resistance at enrollment suggests non-antibacterial mechanisms might be responsible.
Citation: Albert RK, Connett J, Bailey WC, et al. Azithromycin for prevention of exacerbations of COPD. N Engl J Med. 2011;365:689-698.
Serial Low-Dose CT Scans Decrease Lung Cancer Mortality
Clinical question: Does annual screening with low-dose CT scans reduce mortality from lung cancer in current or former heavy smokers?
Background: Lung cancer is still the top cause of cancer death in the United States, and its prevalence is increasing in the developing world. Prior trials of screening chest radiography and sputum samples have not shown a decrease in lung cancer mortality, but CT scans could help identify lung cancer at an earlier, more treatable stage.
Study design: Prospective, multicenter, randomized trial of low-dose (roughly one-fifth the radiation of traditional CT) annual CT versus annual chest radiography.
Setting: Thirty-three U.S. medical institutions.
Synopsis: The study enrolled 53,454 current or prior smokers with a history of smoking more than 30 packs a year who were randomly assigned to undergo three annual CT scans or chest X-rays. Any CT scans that showed a noncalcified nodule greater than 4 mm and chest X-rays that showed a noncalcified nodule or mass of any size were classified as “positive,” but follow-up was left to the discretion of the treating physicians. Participants were followed for 6.5 years on average.
Of those screened with CT, 40% had a positive screening test at some point, more than three times the rate of chest radiography, and 96.4% of these were false-positives, which was similar to chest radiography. Adverse events as a result of workup of eventual false positives were uncommon, occurring in around 1% of those who did not have lung cancer and in 11% of those who did. Screening reduced lung cancer mortality by 20% compared with chest radiography, with a number needed to screen to prevent one lung cancer death of 320. Cost-effectiveness and effects of radiation were not assessed.
Bottom line: Serial screening with low-dose CT reduces lung cancer mortality at the cost of a high rate of false-positives. Questions remain about cost-effectiveness and radiation exposure.
Citation: National Lung Screening Trial Research Team, Aberle DR, Adams AM, Berg CD, et al. Reduced lung-cancer mortality with low-dose computed tomographic screening. N Engl J Med. 2011;365:395-409.
One-Week Delay in Starting Parenteral Nutrition in ICU Patients Is Associated with Better Outcomes
Clinical question: In patients admitted to the ICU who are at risk for malnutrition, does supplementing enteral feeding with parenteral feeding on the day of admission improve outcomes when compared with supplementation starting on Day 8?
Background: Patients with critical illness are at risk for malnutrition, which may lead to worsened outcomes. Many are unable to tolerate enteral feeding. However, adding parenteral nutrition has risks, including overfeeding and hyperglycemia.
Study design: Prospective, multicenter, randomized controlled trial. Neither patients/families nor ICU physicians were blinded.
Setting: Two university hospitals in Belgium.
Synopsis: The study included 4,640 patients admitted to the ICU who were “at risk” for malnutrition (assessed using a Nutritional Risk Score of >3). They were randomized to early initiation of parenteral nutrition on Day 1 or late initiation of parenteral nutrition on Day 8, in both cases only if needed to supplement enteral feeding. Both groups received intensive IV insulin protocols to keep blood glucose at 80 mg/dL to 110 mg/dL. The study populations were well-matched, with similar APACHE II scores. Of the total patients, 42% were emergency admissions to the ICU (more than half of which had sepsis), while scheduled cardiac surgery patients made up the majority of the rest of the study participants.
Patients with late initiation of total parenteral nutrition (TPN) were more likely to be discharged alive from the ICU within eight days despite having increased risk of hypoglycemia and more elevation of inflammatory markers. They had shorter ICU and hospital stays, fewer days on the ventilator, fewer infectious complications, were less likely to develop renal failure, and had lower overall costs. The unblinded management of these patients raises concern in interpreting infectious outcomes because study investigators could have been biased to look for infection more often in the early initiation group.
Bottom line: In ICU patients at risk for malnutrition, delaying initiation of TPN to supplement enteral feeding shortens ICU stay and reduces infectious complications when compared with early initiation of TPN.
Citation: Casaer MP, Mesotten D, Hermans G, et al. Early versus late parenteral nutrition in critically ill adults. N Engl J Med. 2011;365:506-17.
Intrapleural Instillation of Combined DNase and t-PA Improve Outcomes in Patients with Empyema
Clinical question: Does the intrapleural administration of a fibrinolytic, a recombinant DNase, or a combination of the two improve outcomes in patients with pleural infections compared with placebo?
Background: Pleural-based infections confer high morbidity and mortality, especially in the one-third of cases in which chest tube drainage fails. Observational data suggest intrapleural administration of fibrinolytics can improve drainage; however, a large randomized trial (MIST1) failed to show improvement with streptokinase.
Study design: Randomized, double-blind, double-dummy, factorial, multicenter trial.
Setting: University hospitals in the U.K.
Synopsis: A total of 193 patients with clinical evidence of an infection as well as laboratory evidence of pleural infection were randomized in factorial design to placebo, DNase alone, t-PA alone, and DNase plus t-PA as a twice-daily, hourlong instillation into the pleural space on hospital days 1 through 3. The combination group had improvements in the size of the pleural effusion compared with placebo, the primary endpoint. This group also was less likely to be referred for surgery, had shorter hospital stays, had less fever, and had lower inflammatory markers by hospital Day 7. Neither of the single-agent groups were better than placebo. The confidence intervals for all outcomes besides radiographic size of the effusion were large, as the trial had limited power for secondary endpoints.
Bottom line: In patients with empyema, the addition of twice-daily instillations of DNase and tPA can improve drainage and decrease risk of treatment failure when combined with chest tube drainage and antibiotics.
Citation: Rahman NM, Maskell NA, West A, et al. Intrapleural use of tissue plasminogen activator and DNase in pleural infection. N Engl J Med. 2011;365:518-526.
Smoothing Admissions over the Week Improves Patient Flow
Clinical question: What is the difference between weekday and weekend hospital occupancy, and what might the effect be of redistributing, or “smoothing,” elective admissions across the week?
Background: Efforts to improve patient flow have largely focused on reducing the average length of stay (ALOS). As the ALOS decreases, though, further reductions have limited yield in improving patient flow and could occur at the expense of patient safety. Smoothing admissions is a recognized but underutilized tool to address patient flow and hospital overcrowding.
Study design: Retrospective cohort.
Setting: Thirty-nine freestanding, tertiary-care children’s hospitals in the U.S.
Synopsis: Hospital occupancy ranged from 70.9% to 108.1% for weekdays, and from 65.7% to 94.4% on weekends. The mean difference between weekday and weekend occupancy was 8.2%. Using a mathematical model to redistribute admissions from peak days to nonpeak days (within a one-week time frame), the investigators found that percent occupancy on average dropped by 6.6%—the same number of patients over the same time interval, but with lower average occupancy. And, while not projected by these authors, the correlate of lower average occupancy would be reduced waits and delays for admission and within-hospital services. Only 2.6%, or about 7.5 admissions per week per hospital, would need to be rescheduled in order to realize this gain.
Bottom line: Where feasible, reshuffling elective admissions to “smooth” demand across the week is associated with improved hospital flow.
Citation: Fieldston ES, Hall M, Shah SS, et al. Addressing inpatient crowding by smoothing occupancy at children’s hospitals. J Hosp Med. 2011;6:466-473.
Variability in Preferences, Experiences, and Expectations for Hospitalist Roles in Comanagement
Clinical question: Do provider expectations and experiences in comanagement differ from the traditional medical consultation model?
Background: Hospitalists participate in traditional medical consultation and, increasingly, a variety of comanagement with surgical and various medical specialists. It is uncertain what preferences and expectations on either side of the comanagement relationship might be. Learning more might lead to a better conceptual understanding and working definition of inpatient comanagement.
Study design: Baseline and follow-up surveys.
Setting: Large single-site academic medical center, hospitalist-hepatologist comanagement service.
Synopsis: All 41 respondents completed the baseline survey, with 32 respondents completing a total of 177 follow-up surveys. Respondents included hospitalists, hepatologists, nonphysician providers (NPPs), and GI fellows. Hospitalists were more likely than hepatologists to want to be informed about every patient care decision (83% versus 17%). The majority of respondents reported understanding their roles. At baseline, nearly all agreed that “comanagement tends to improve patient care.” However, at follow-up, fewer NPP and fewer GI fellows reported that patients on their service had received “better care than they would without comanagement.” At follow-up, fewer hospitalists than at baseline reported that there was one “physician leader to direct the overall management of the patient’s hospital course.”
Bottom line: Hospitalists were more likely than hepatologists, within a comanagement relationship, to want to be informed about every decision. Most expected—but fewer actually later reported—that the comanagement model improved patient care.
Citation: Hinami K, Whelan CT, Konetzka RT, and Meltzer DO. Provider expectations and experiences of comanagement. J Hosp Med. 2011;6:401-404.
In This Edition
Literature At A Glance
A guide to this month’s studies
- Higher loading dose of clopidogrel in STEMI
- Early vs. late surgery following hip fracture
- Beta-blockers in chronic kidney disease
- Long-term azithromycin in COPD
- CT screening for lung cancer
- Timing of parenteral nutrition in the ICU
- Intrapleural management of empyema with DNase and t-PA
- Effect of weekend elective admissions on hospital flow
- Expectations and outcomes of medical comanagement
Higher-Dose Clopidogrel Improves Outcomes at 30 Days in STEMI Patients
Clinical question: Does a 600-mg loading dose of clopidogrel given before percutaneous coronary intervention (PCI) in patients with an ST-segment elevation myocardial infarction (STEMI) provide more protection from thrombotic complications at 30 days than a 300-mg dose?
Background: Clopidogrel at 600 mg is active more quickly (two hours versus 12 hours) and inhibits platelets more completely than does a 300-mg dose, but it has never been tested prospectively in patients undergoing percutaneous intervention with a STEMI.
Study design: Prospective, multicenter, randomized controlled trial.
Setting: Five hospitals in Italy, Belgium, Serbia, and Hungary.
Synopsis: Two-hundred-one patients with STEMI were randomized to either 600 mg or 300 mg of clopidogrel, given an average of 30 minutes before initial PCI, as well as other standard treatment for STEMI. The primary outcome was “infarct size,” judged as the area under the curve (AUC) of serial creatine kinase-MB (CK-MB) and troponin measurements. At 30 days, patients who received the 600-mg dose of clopidogrel had lower AUCs of cardiac biomarkers, statistically significant (though clinically small) improvement in left ventricle ejection fraction at discharge, lower incidence of severely impaired post-PCI thrombolitis in myocardial infarction (TIMI) flow, and fewer “major cardiovascular events,” driven mainly by a reduction in revascularizations. Measurement of biomarkers to calculate infarct size can be confounded by hypertrophy, and the trial was underpowered for cardiovascular events. However, there was no increase in bleeding events.
Bottom line: In patients with STEMI, treatment with a higher loading dose of clopidogrel before PCI reduces revascularizations and might decrease infarct size without an increase in adverse events.
Citation: Patti G, Bárczi G, Orlic D, et al. Outcome comparison of 600- and 300-mg loading doses of clopidogrel in patients undergoing primary percutaneous coronary intervention for ST-segment elevation myocardial infarction: results from the ARMYDA-6 MI (Antiplatelet therapy for Reduction of MYocardial Damage during Angioplasty-Myocardial Infarction) randomized study. J Am Coll Cardiol. 2011;58:1592-1599.
Medical Comorbidities Explain Most of Excess Risk Seen in Patients with Delayed Hip Fracture Repair
Clinical question: Could the increased mortality found with delays in hip fracture surgery be confounded by the premorbid functional status and medical comorbidities of patients whose surgery is more likely to be delayed?
Background: Guidelines recommend operating on patients with hip fracture within 24 hours, but the supporting evidence has not adjusted for underlying medical comorbidities, which could delay surgery and contribute to poor outcomes, making delays look harmful.
Study design: Prospective cohort, single-center design.
Setting: University hospital in Spain.
Synopsis: The study included 2,250 consecutive elderly patients admitted to the hospital for hip fracture who had their functional status and medical comorbidities assessed at enrollment. If their surgery was delayed beyond 24 hours, the reason was sought. Medical outcomes assessed daily while in hospital were delirium, pneumonia, heart failure, urinary tract infection, and new pressure sores, while the dose of pain medication, surgical complications, and in-hospital mortality were also compiled. No post-discharge data were available.
The median time to surgery was 72 hours. Patients with more medical comorbidities and poorer preoperative functional status had longer delays to operation, most commonly due to interrupting antiplatelet treatment or need for preoperative “echocardiography or other examinations.” When these medical factors were included in logistic regression analysis, the increased mortality seen with delays of surgery in the cohort was no longer statistically significant, suggesting the underlying comorbidities of these patients, rather than the delay to surgery itself, explained the increased mortality.
Bottom line: Delaying hip fracture surgery is less important than preoperative functional status and medical comorbidity in contributing to poor outcomes.
Citation: Vidán MT, Sánchez E, Gracia Y, Marañón E, Vaquero J, Serra JA. Causes and effects of surgical delay in patients with hip fracture: a cohort study. Ann Intern Med. 2011;155:226-233.
Beta-Blockers Decrease All-Cause and Cardiovascular Mortality in Patients with Chronic Kidney Disease
Clinical question: Are beta-blockers as effective in patients on dialysis and with end-stage chronic kidney disease (CKD) as they are in patients without severe renal disease?
Background: Patients with CKD have been largely excluded from trials of beta-blocker therapy even though they have high rates of cardiovascular disease and might be most likely to benefit. However, patients on dialysis might be predisposed to adverse complications of beta-blocker therapy, including hypotension.
Study design: Meta-analysis of eight trials of beta-blockade versus placebo (six heart failure trials) or ACE-I (two non-heart-failure trials) that included post-hoc analyses of CKD patients.
Setting: Varied, usually multinational RCTs.
Synopsis: The six congestive heart failure (CHF) studies were not designed to evaluate patients with CKD, and the two non-CHF studies were intended to evaluate progression of CKD, not cardiac outcomes. Thus, this is a meta-analysis of post-hoc CKD subgroups included in these trials. Compared with placebo, beta-blockers reduced all-cause and cardiovascular mortality without significant heterogeneity between trials. The magnitude of the effect was similar in CKD and non-CKD patients. Patients with CKD treated with beta-blockers were at increased risk of bradycardia and hypotension, but this did not lead to increased discontinuation of the drug. Only 114 dialysis patients were included in one of the eight trials (7,000 patients overall) and no outcomes were assessed.
Bottom line: Beta-blockers lower all-cause and cardiovascular mortality in patients with CKD similarly to patients without kidney disease but are associated with an increased risk for hypotension and bradycardia. Their effect in dialysis patients is unknown.
Citation: Badve SV, Roberts MA, Hawley CM, et al. Effects of beta-adrenergic antagonists in patients with chronic kidney disease: a systematic review and meta-analysis. J Am Coll Cardiol. 2011;58:1152-1161.
Daily Long-Term Azithromycin in COPD Patients Decreases Frequency of Exacerbations
Clinical question: Does long-term treatment with azithromycin decrease COPD exacerbations and improve quality of life with an acceptable risk profile?
Background: Patients with acute exacerbations of COPD have increased risks of death and more rapid decline in lung function. Macrolide antibiotics might decrease exacerbations via antibacterial and anti-inflammatory effects. Small studies of long-term treatment with macrolides in COPD have had conflicting results.
Study design: Prospective, multicenter, parallel-group, placebo-controlled trial.
Setting: Twelve U.S. academic health centers.
Synopsis: Eligible patients were those older than 40 with pulmonary function test-proven obstructive disease, a significant smoking history, a use of supplemental oxygen, or an oral glucocorticoid treatment in the previous year, and those who had previously come to healthcare attention for a COPD exacerbation. They were randomized to daily azithromycin (250 mg) or placebo. The primary outcome was time to first COPD exacerbation at one year. The investigators used deep nasopharyngeal swabs to evaluate the development of microbial resistance, and all patients were regularly screened for hearing loss. Time to first exacerbation and frequency of exacerbations decreased significantly with azithromycin treatment; the number needed to treat to prevent a COPD exacerbation was 2.86. More than half of nasopharyngeal samples had evidence of macrolide resistance at enrollment, and this increased to more than 80% at one year. Audiometric testing did show a decrease in hearing in those treated with azithromycin, but even those who did not stop their medication had recovery on subsequent testing.
Bottom line: Daily azithromycin decreases COPD exacerbations in those with a history of previous exacerbation at the cost of increased macrolide resistance and possible reversible decrements in hearing. The high degree of macrolide resistance at enrollment suggests non-antibacterial mechanisms might be responsible.
Citation: Albert RK, Connett J, Bailey WC, et al. Azithromycin for prevention of exacerbations of COPD. N Engl J Med. 2011;365:689-698.
Serial Low-Dose CT Scans Decrease Lung Cancer Mortality
Clinical question: Does annual screening with low-dose CT scans reduce mortality from lung cancer in current or former heavy smokers?
Background: Lung cancer is still the top cause of cancer death in the United States, and its prevalence is increasing in the developing world. Prior trials of screening chest radiography and sputum samples have not shown a decrease in lung cancer mortality, but CT scans could help identify lung cancer at an earlier, more treatable stage.
Study design: Prospective, multicenter, randomized trial of low-dose (roughly one-fifth the radiation of traditional CT) annual CT versus annual chest radiography.
Setting: Thirty-three U.S. medical institutions.
Synopsis: The study enrolled 53,454 current or prior smokers with a history of smoking more than 30 packs a year who were randomly assigned to undergo three annual CT scans or chest X-rays. Any CT scans that showed a noncalcified nodule greater than 4 mm and chest X-rays that showed a noncalcified nodule or mass of any size were classified as “positive,” but follow-up was left to the discretion of the treating physicians. Participants were followed for 6.5 years on average.
Of those screened with CT, 40% had a positive screening test at some point, more than three times the rate of chest radiography, and 96.4% of these were false-positives, which was similar to chest radiography. Adverse events as a result of workup of eventual false positives were uncommon, occurring in around 1% of those who did not have lung cancer and in 11% of those who did. Screening reduced lung cancer mortality by 20% compared with chest radiography, with a number needed to screen to prevent one lung cancer death of 320. Cost-effectiveness and effects of radiation were not assessed.
Bottom line: Serial screening with low-dose CT reduces lung cancer mortality at the cost of a high rate of false-positives. Questions remain about cost-effectiveness and radiation exposure.
Citation: National Lung Screening Trial Research Team, Aberle DR, Adams AM, Berg CD, et al. Reduced lung-cancer mortality with low-dose computed tomographic screening. N Engl J Med. 2011;365:395-409.
One-Week Delay in Starting Parenteral Nutrition in ICU Patients Is Associated with Better Outcomes
Clinical question: In patients admitted to the ICU who are at risk for malnutrition, does supplementing enteral feeding with parenteral feeding on the day of admission improve outcomes when compared with supplementation starting on Day 8?
Background: Patients with critical illness are at risk for malnutrition, which may lead to worsened outcomes. Many are unable to tolerate enteral feeding. However, adding parenteral nutrition has risks, including overfeeding and hyperglycemia.
Study design: Prospective, multicenter, randomized controlled trial. Neither patients/families nor ICU physicians were blinded.
Setting: Two university hospitals in Belgium.
Synopsis: The study included 4,640 patients admitted to the ICU who were “at risk” for malnutrition (assessed using a Nutritional Risk Score of >3). They were randomized to early initiation of parenteral nutrition on Day 1 or late initiation of parenteral nutrition on Day 8, in both cases only if needed to supplement enteral feeding. Both groups received intensive IV insulin protocols to keep blood glucose at 80 mg/dL to 110 mg/dL. The study populations were well-matched, with similar APACHE II scores. Of the total patients, 42% were emergency admissions to the ICU (more than half of which had sepsis), while scheduled cardiac surgery patients made up the majority of the rest of the study participants.
Patients with late initiation of total parenteral nutrition (TPN) were more likely to be discharged alive from the ICU within eight days despite having increased risk of hypoglycemia and more elevation of inflammatory markers. They had shorter ICU and hospital stays, fewer days on the ventilator, fewer infectious complications, were less likely to develop renal failure, and had lower overall costs. The unblinded management of these patients raises concern in interpreting infectious outcomes because study investigators could have been biased to look for infection more often in the early initiation group.
Bottom line: In ICU patients at risk for malnutrition, delaying initiation of TPN to supplement enteral feeding shortens ICU stay and reduces infectious complications when compared with early initiation of TPN.
Citation: Casaer MP, Mesotten D, Hermans G, et al. Early versus late parenteral nutrition in critically ill adults. N Engl J Med. 2011;365:506-17.
Intrapleural Instillation of Combined DNase and t-PA Improve Outcomes in Patients with Empyema
Clinical question: Does the intrapleural administration of a fibrinolytic, a recombinant DNase, or a combination of the two improve outcomes in patients with pleural infections compared with placebo?
Background: Pleural-based infections confer high morbidity and mortality, especially in the one-third of cases in which chest tube drainage fails. Observational data suggest intrapleural administration of fibrinolytics can improve drainage; however, a large randomized trial (MIST1) failed to show improvement with streptokinase.
Study design: Randomized, double-blind, double-dummy, factorial, multicenter trial.
Setting: University hospitals in the U.K.
Synopsis: A total of 193 patients with clinical evidence of an infection as well as laboratory evidence of pleural infection were randomized in factorial design to placebo, DNase alone, t-PA alone, and DNase plus t-PA as a twice-daily, hourlong instillation into the pleural space on hospital days 1 through 3. The combination group had improvements in the size of the pleural effusion compared with placebo, the primary endpoint. This group also was less likely to be referred for surgery, had shorter hospital stays, had less fever, and had lower inflammatory markers by hospital Day 7. Neither of the single-agent groups were better than placebo. The confidence intervals for all outcomes besides radiographic size of the effusion were large, as the trial had limited power for secondary endpoints.
Bottom line: In patients with empyema, the addition of twice-daily instillations of DNase and tPA can improve drainage and decrease risk of treatment failure when combined with chest tube drainage and antibiotics.
Citation: Rahman NM, Maskell NA, West A, et al. Intrapleural use of tissue plasminogen activator and DNase in pleural infection. N Engl J Med. 2011;365:518-526.
Smoothing Admissions over the Week Improves Patient Flow
Clinical question: What is the difference between weekday and weekend hospital occupancy, and what might the effect be of redistributing, or “smoothing,” elective admissions across the week?
Background: Efforts to improve patient flow have largely focused on reducing the average length of stay (ALOS). As the ALOS decreases, though, further reductions have limited yield in improving patient flow and could occur at the expense of patient safety. Smoothing admissions is a recognized but underutilized tool to address patient flow and hospital overcrowding.
Study design: Retrospective cohort.
Setting: Thirty-nine freestanding, tertiary-care children’s hospitals in the U.S.
Synopsis: Hospital occupancy ranged from 70.9% to 108.1% for weekdays, and from 65.7% to 94.4% on weekends. The mean difference between weekday and weekend occupancy was 8.2%. Using a mathematical model to redistribute admissions from peak days to nonpeak days (within a one-week time frame), the investigators found that percent occupancy on average dropped by 6.6%—the same number of patients over the same time interval, but with lower average occupancy. And, while not projected by these authors, the correlate of lower average occupancy would be reduced waits and delays for admission and within-hospital services. Only 2.6%, or about 7.5 admissions per week per hospital, would need to be rescheduled in order to realize this gain.
Bottom line: Where feasible, reshuffling elective admissions to “smooth” demand across the week is associated with improved hospital flow.
Citation: Fieldston ES, Hall M, Shah SS, et al. Addressing inpatient crowding by smoothing occupancy at children’s hospitals. J Hosp Med. 2011;6:466-473.
Variability in Preferences, Experiences, and Expectations for Hospitalist Roles in Comanagement
Clinical question: Do provider expectations and experiences in comanagement differ from the traditional medical consultation model?
Background: Hospitalists participate in traditional medical consultation and, increasingly, a variety of comanagement with surgical and various medical specialists. It is uncertain what preferences and expectations on either side of the comanagement relationship might be. Learning more might lead to a better conceptual understanding and working definition of inpatient comanagement.
Study design: Baseline and follow-up surveys.
Setting: Large single-site academic medical center, hospitalist-hepatologist comanagement service.
Synopsis: All 41 respondents completed the baseline survey, with 32 respondents completing a total of 177 follow-up surveys. Respondents included hospitalists, hepatologists, nonphysician providers (NPPs), and GI fellows. Hospitalists were more likely than hepatologists to want to be informed about every patient care decision (83% versus 17%). The majority of respondents reported understanding their roles. At baseline, nearly all agreed that “comanagement tends to improve patient care.” However, at follow-up, fewer NPP and fewer GI fellows reported that patients on their service had received “better care than they would without comanagement.” At follow-up, fewer hospitalists than at baseline reported that there was one “physician leader to direct the overall management of the patient’s hospital course.”
Bottom line: Hospitalists were more likely than hepatologists, within a comanagement relationship, to want to be informed about every decision. Most expected—but fewer actually later reported—that the comanagement model improved patient care.
Citation: Hinami K, Whelan CT, Konetzka RT, and Meltzer DO. Provider expectations and experiences of comanagement. J Hosp Med. 2011;6:401-404.