User login
Hospital medicine resident training tracks: Developing the hospital medicine pipeline
The field of hospital medicine (HM) is rapidly expanding in the areas of clinical medicine, administration, and quality improvement (QI).1 Emerging with this growth is a gap in the traditional internal medicine (IM) training and skills needed to be effective in HM.1,2 These skills include clinical and nonclinical aptitudes, such as process improvement, health care economics, and leadership.1-3 However, resident education on these topics must compete with other required curricular content in IM residency training.2,4 Few IM residencies offer focused HM training that emphasizes key components of successful HM careers.3,5
Within the past decade, designated HM tracks within IM residency programs have been proposed as a potential solution. Initially, calls for such tracks focused on gaps in the clinical competencies required of hospitalists.1 Tracks have since evolved to also include skills required to drive high-value care, process improvement, and scholarship. Designated HM tracks address these areas through greater breadth of curricula, additional time for reflection, participation in group projects, and active application to clinical care.4 We conducted a study to identify themes that could inform the ongoing evolution of dedicated HM tracks.
METHODS
Programs were initially identified through communication among professional networks. The phrases hospital medicine residency track and internal medicine residency hospitalist track were used in broader Google searches, as there is no database of such tracks. Searches were performed quarterly during the 2015–2016 academic year. The top 20 hits were manually filtered to identify tracks affiliated with major academic centers. IM residency program websites provided basic information for programs with tracks. We excluded tracks focused entirely on QI6 because, though a crucial part of HM, QI training alone is probably insufficient for preparing residents for success as hospitalists on residency completion. Similarly, IM residencies with stand-alone HM clinical rotations without longitudinal HM curricula were excluded.
Semistructured interviews with track directors were conducted by e-mail or telephone for all tracks except one, the details of which are published.7 We tabulated data and reviewed qualitative information to identify themes among the different tracks. As this study did not involve human participants, Institutional Review Board approval was not needed.
RESULTS
We identified 11 HM residency training programs at major academic centers across the United States: Cleveland Clinic, Stanford University, Tulane University, University of California Davis, University of California Irvine, University of Colorado, University of Kentucky, University of Minnesota, University of New Mexico, Virginia Commonwealth University, and Wake Forest University (Table 1). We reviewed the websites of about 10 other programs, but none suggested existence of a track. Additional programs contacted reported no current track.
Track Participants and Structure
HM tracks mainly target third-year residents (Table 1). Some extend into the second year of residency, and 4 have opportunities for intern involvement, including a separate match number at Colorado. Tracks accept up to 12 residents per class. Two programs, at Colorado and Virginia, are part of IM programs in which all residents belong to a track (eg, HM, primary care, research).
HM track structures vary widely and are heavily influenced by the content delivery platforms of their IM residency programs. Several HM track directors emphasized the importance of fitting into existing educational frameworks to ensure access to residents and to minimize the burden of participation. Four programs deliver the bulk of their nonclinical content in dedicated blocks; 6 others use brief recurring sessions to deliver smaller aliquots longitudinally (Table 1). The number of protected hours for content delivery ranges from 10 to more than 40 annually. All tracks use multiple content delivery modes, including didactic sessions and journal clubs. Four tracks employ panel discussions to explore career options within HM. Several also use online platforms, including discussions, readings, and modules.
Quality Improvement
The vast majority of curricula prominently feature experiential QI project involvement (Table 2). These mentored longitudinal projects allow applied delivery of content, such as QI methods and management skills. Four tracks use material from the Institute for Healthcare Improvement.8 Several also offer dedicated QI rotations that immerse residents in ongoing QI efforts.
Institutional partnerships support these initiatives at several sites. The Minnesota track is a joint venture of the university and Regions Hospital, a nonprofit community hospital. The Virginia track positions HM residents to lead university-wide interdisciplinary QI teams. For project support, the Colorado and Kentucky tracks partner with local QI resources—the Institute for Healthcare Quality, Safety, and Efficiency at Colorado and the Office of Value and Innovation in Healthcare Delivery at Kentucky.
Health Care Economics and Value
Many programs leverage the rapidly growing emphasis on health care “value” as an opportunity for synergy between IM programs and HM tracks. Examples include involving residents in efforts to improve documentation or didactic instruction on topics such as health care finance. The New Mexico and Wake Forest tracks offer elective rotations on health care economics. Several track directors mentioned successfully expanding curricula on health care value from the HM track into IM residency programs at large, providing a measurable service to the residency programs while ensuring content delivery and freeing up additional time for track activities.
Scholarship and Career Development
Most programs provide targeted career development for residents. Six tracks provide sessions on job procurement skills, such as curriculum vitae preparation and interviewing (Table 2). Many also provide content on venues for disseminating scholarly activity. The Colorado, Kentucky, New Mexico, and Tulane programs feature content on abstract and poster creation. Leadership development is addressed in several tracks through dedicated track activities or participation in discrete, outside-track events. Specifically, Colorado offers a leadership track for residents interested in hospital administration, Cleveland has a leadership journal club, Wake Forest enrolls HM residents in leadership training available through the university, and Minnesota sends residents to the Society of Hospital Medicine’s Leadership Academy (Table 2).
Clinical Rotations
Almost all tracks include a clinical rotation, typically pairing residents directly with hospitalist attendings to encourage autonomy and mentorship. Several also offer elective rotations in various disciplines within HM (Table 2). The Kentucky and Virginia tracks incorporate working with advanced practice providers into their practicums. The Cleveland, Minnesota, Tulane, and Virginia tracks offer HM rotations in community hospitals or postacute settings.
HM rotations also pair clinical experiences with didactic education on relevant topics (eg, billing and coding). The Cleveland, Minnesota, and Virginia tracks developed clinical rotations reflecting the common 7-on and 7-off schedule with nonclinical obligations, such as seminars linking specific content to clinical experiences, during nonclinical time.
DISCUSSION
Our investigation into the current state of HM training found that HM track curricula focus largely on QI, health care economics, and professional development. This focus likely developed in response to hospitalists’ increasing engagement in related endeavors. HM tracks have dynamic and variable structures, reflecting an evolving field and the need to fit into existing IM residency program structures. Similarly, the content covered in HM tracks is tightly linked to perceived opportunities within IM residency curricula. The heterogeneity of content suggests the breadth and ambiguity of necessary competencies for aspiring hospitalists. One of the 11 tracks has not had any residents enroll within the past few years—a testament to the continued effort necessary to sustain such tracks, including curricular updates and recruiting. Conversely, many programs now share track content with the larger IM residency program, suggesting HM tracks may be near the forefront of medical education in some areas.
Our study had several limitations. As we are unaware of any databases of HM tracks, we discussed tracks with professional contacts, performed Internet searches, and reviewed IM residency program websites. Our search, however, was not exhaustive; despite our best efforts, we may have missed or mischaracterized some track offerings. Nevertheless, we think that our analysis represents the first thorough compilation of HM tracks and that it will be useful to institutions seeking to create or enhance HM-specific training.
As the field continues to evolve, we are optimistic about the future of HM training. We suspect that HM residency training tracks will continue to expand. More work is needed so these tracks can adjust to the changing HM and IM residency program landscapes and supply well-trained physicians for the HM workforce.
Acknowledgment
The authors thank track directors Alpesh Amin, David Gugliotti, Rick Hilger, Karnjit Johl, Nasir Majeed, Georgia McIntosh, Charles Pizanis, and Jeff Wiese for making this study possible.
Disclosure
Nothing to report.
1. Glasheen JJ, Siegal EM, Epstein K, Kutner J, Prochazka AV. Fulfilling the promise of hospital medicine: tailoring internal medicine training to address hospitalists’ needs [published correction appears in J Gen Intern Med. 2008;23(11):1931]. J Gen Intern Med. 2008;23(7):1110-1115. PubMed
2. Arora V, Guardiano S, Donaldson D, Storch I, Hemstreet P. Closing the gap between internal medicine training and practice: recommendations from recent graduates. Am J Med. 2005;118(6):680-685. PubMed
3. Glasheen JJ, Goldenberg J, Nelson JR. Achieving hospital medicine’s promise through internal medicine residency redesign. Mt Sinai J Med. 2008;75(5):436-441. PubMed
4. Wiese J. Residency training: beginning with the end in mind. J Gen Intern Med. 2008;23(7):1122-1123. PubMed
5. Glasheen JJ, Epstein KR, Siegal E, Kutner JS, Prochazka AV. The spectrum of community-based hospitalist practice: a call to tailor internal medicine residency training. Arch Intern Med. 2007;167(7):727-728. PubMed
6. Patel N, Brennan PJ, Metlay J, Bellini L, Shannon RP, Myers JS. Building the pipeline: the creation of a residency training pathway for future physician leaders in health care quality. Acad Med. 2015;90(2):185-190. PubMed
7. Kumar A, Smeraglio A, Witteles R, et al. A resident-created hospitalist curriculum for internal medicine housestaff. J Hosp Med. 2016;11(9):646-649. PubMed
8. Institute for Healthcare Improvement website. http://www.ihi.org. Accessed December 15, 2015.
The field of hospital medicine (HM) is rapidly expanding in the areas of clinical medicine, administration, and quality improvement (QI).1 Emerging with this growth is a gap in the traditional internal medicine (IM) training and skills needed to be effective in HM.1,2 These skills include clinical and nonclinical aptitudes, such as process improvement, health care economics, and leadership.1-3 However, resident education on these topics must compete with other required curricular content in IM residency training.2,4 Few IM residencies offer focused HM training that emphasizes key components of successful HM careers.3,5
Within the past decade, designated HM tracks within IM residency programs have been proposed as a potential solution. Initially, calls for such tracks focused on gaps in the clinical competencies required of hospitalists.1 Tracks have since evolved to also include skills required to drive high-value care, process improvement, and scholarship. Designated HM tracks address these areas through greater breadth of curricula, additional time for reflection, participation in group projects, and active application to clinical care.4 We conducted a study to identify themes that could inform the ongoing evolution of dedicated HM tracks.
METHODS
Programs were initially identified through communication among professional networks. The phrases hospital medicine residency track and internal medicine residency hospitalist track were used in broader Google searches, as there is no database of such tracks. Searches were performed quarterly during the 2015–2016 academic year. The top 20 hits were manually filtered to identify tracks affiliated with major academic centers. IM residency program websites provided basic information for programs with tracks. We excluded tracks focused entirely on QI6 because, though a crucial part of HM, QI training alone is probably insufficient for preparing residents for success as hospitalists on residency completion. Similarly, IM residencies with stand-alone HM clinical rotations without longitudinal HM curricula were excluded.
Semistructured interviews with track directors were conducted by e-mail or telephone for all tracks except one, the details of which are published.7 We tabulated data and reviewed qualitative information to identify themes among the different tracks. As this study did not involve human participants, Institutional Review Board approval was not needed.
RESULTS
We identified 11 HM residency training programs at major academic centers across the United States: Cleveland Clinic, Stanford University, Tulane University, University of California Davis, University of California Irvine, University of Colorado, University of Kentucky, University of Minnesota, University of New Mexico, Virginia Commonwealth University, and Wake Forest University (Table 1). We reviewed the websites of about 10 other programs, but none suggested existence of a track. Additional programs contacted reported no current track.
Track Participants and Structure
HM tracks mainly target third-year residents (Table 1). Some extend into the second year of residency, and 4 have opportunities for intern involvement, including a separate match number at Colorado. Tracks accept up to 12 residents per class. Two programs, at Colorado and Virginia, are part of IM programs in which all residents belong to a track (eg, HM, primary care, research).
HM track structures vary widely and are heavily influenced by the content delivery platforms of their IM residency programs. Several HM track directors emphasized the importance of fitting into existing educational frameworks to ensure access to residents and to minimize the burden of participation. Four programs deliver the bulk of their nonclinical content in dedicated blocks; 6 others use brief recurring sessions to deliver smaller aliquots longitudinally (Table 1). The number of protected hours for content delivery ranges from 10 to more than 40 annually. All tracks use multiple content delivery modes, including didactic sessions and journal clubs. Four tracks employ panel discussions to explore career options within HM. Several also use online platforms, including discussions, readings, and modules.
Quality Improvement
The vast majority of curricula prominently feature experiential QI project involvement (Table 2). These mentored longitudinal projects allow applied delivery of content, such as QI methods and management skills. Four tracks use material from the Institute for Healthcare Improvement.8 Several also offer dedicated QI rotations that immerse residents in ongoing QI efforts.
Institutional partnerships support these initiatives at several sites. The Minnesota track is a joint venture of the university and Regions Hospital, a nonprofit community hospital. The Virginia track positions HM residents to lead university-wide interdisciplinary QI teams. For project support, the Colorado and Kentucky tracks partner with local QI resources—the Institute for Healthcare Quality, Safety, and Efficiency at Colorado and the Office of Value and Innovation in Healthcare Delivery at Kentucky.
Health Care Economics and Value
Many programs leverage the rapidly growing emphasis on health care “value” as an opportunity for synergy between IM programs and HM tracks. Examples include involving residents in efforts to improve documentation or didactic instruction on topics such as health care finance. The New Mexico and Wake Forest tracks offer elective rotations on health care economics. Several track directors mentioned successfully expanding curricula on health care value from the HM track into IM residency programs at large, providing a measurable service to the residency programs while ensuring content delivery and freeing up additional time for track activities.
Scholarship and Career Development
Most programs provide targeted career development for residents. Six tracks provide sessions on job procurement skills, such as curriculum vitae preparation and interviewing (Table 2). Many also provide content on venues for disseminating scholarly activity. The Colorado, Kentucky, New Mexico, and Tulane programs feature content on abstract and poster creation. Leadership development is addressed in several tracks through dedicated track activities or participation in discrete, outside-track events. Specifically, Colorado offers a leadership track for residents interested in hospital administration, Cleveland has a leadership journal club, Wake Forest enrolls HM residents in leadership training available through the university, and Minnesota sends residents to the Society of Hospital Medicine’s Leadership Academy (Table 2).
Clinical Rotations
Almost all tracks include a clinical rotation, typically pairing residents directly with hospitalist attendings to encourage autonomy and mentorship. Several also offer elective rotations in various disciplines within HM (Table 2). The Kentucky and Virginia tracks incorporate working with advanced practice providers into their practicums. The Cleveland, Minnesota, Tulane, and Virginia tracks offer HM rotations in community hospitals or postacute settings.
HM rotations also pair clinical experiences with didactic education on relevant topics (eg, billing and coding). The Cleveland, Minnesota, and Virginia tracks developed clinical rotations reflecting the common 7-on and 7-off schedule with nonclinical obligations, such as seminars linking specific content to clinical experiences, during nonclinical time.
DISCUSSION
Our investigation into the current state of HM training found that HM track curricula focus largely on QI, health care economics, and professional development. This focus likely developed in response to hospitalists’ increasing engagement in related endeavors. HM tracks have dynamic and variable structures, reflecting an evolving field and the need to fit into existing IM residency program structures. Similarly, the content covered in HM tracks is tightly linked to perceived opportunities within IM residency curricula. The heterogeneity of content suggests the breadth and ambiguity of necessary competencies for aspiring hospitalists. One of the 11 tracks has not had any residents enroll within the past few years—a testament to the continued effort necessary to sustain such tracks, including curricular updates and recruiting. Conversely, many programs now share track content with the larger IM residency program, suggesting HM tracks may be near the forefront of medical education in some areas.
Our study had several limitations. As we are unaware of any databases of HM tracks, we discussed tracks with professional contacts, performed Internet searches, and reviewed IM residency program websites. Our search, however, was not exhaustive; despite our best efforts, we may have missed or mischaracterized some track offerings. Nevertheless, we think that our analysis represents the first thorough compilation of HM tracks and that it will be useful to institutions seeking to create or enhance HM-specific training.
As the field continues to evolve, we are optimistic about the future of HM training. We suspect that HM residency training tracks will continue to expand. More work is needed so these tracks can adjust to the changing HM and IM residency program landscapes and supply well-trained physicians for the HM workforce.
Acknowledgment
The authors thank track directors Alpesh Amin, David Gugliotti, Rick Hilger, Karnjit Johl, Nasir Majeed, Georgia McIntosh, Charles Pizanis, and Jeff Wiese for making this study possible.
Disclosure
Nothing to report.
The field of hospital medicine (HM) is rapidly expanding in the areas of clinical medicine, administration, and quality improvement (QI).1 Emerging with this growth is a gap in the traditional internal medicine (IM) training and skills needed to be effective in HM.1,2 These skills include clinical and nonclinical aptitudes, such as process improvement, health care economics, and leadership.1-3 However, resident education on these topics must compete with other required curricular content in IM residency training.2,4 Few IM residencies offer focused HM training that emphasizes key components of successful HM careers.3,5
Within the past decade, designated HM tracks within IM residency programs have been proposed as a potential solution. Initially, calls for such tracks focused on gaps in the clinical competencies required of hospitalists.1 Tracks have since evolved to also include skills required to drive high-value care, process improvement, and scholarship. Designated HM tracks address these areas through greater breadth of curricula, additional time for reflection, participation in group projects, and active application to clinical care.4 We conducted a study to identify themes that could inform the ongoing evolution of dedicated HM tracks.
METHODS
Programs were initially identified through communication among professional networks. The phrases hospital medicine residency track and internal medicine residency hospitalist track were used in broader Google searches, as there is no database of such tracks. Searches were performed quarterly during the 2015–2016 academic year. The top 20 hits were manually filtered to identify tracks affiliated with major academic centers. IM residency program websites provided basic information for programs with tracks. We excluded tracks focused entirely on QI6 because, though a crucial part of HM, QI training alone is probably insufficient for preparing residents for success as hospitalists on residency completion. Similarly, IM residencies with stand-alone HM clinical rotations without longitudinal HM curricula were excluded.
Semistructured interviews with track directors were conducted by e-mail or telephone for all tracks except one, the details of which are published.7 We tabulated data and reviewed qualitative information to identify themes among the different tracks. As this study did not involve human participants, Institutional Review Board approval was not needed.
RESULTS
We identified 11 HM residency training programs at major academic centers across the United States: Cleveland Clinic, Stanford University, Tulane University, University of California Davis, University of California Irvine, University of Colorado, University of Kentucky, University of Minnesota, University of New Mexico, Virginia Commonwealth University, and Wake Forest University (Table 1). We reviewed the websites of about 10 other programs, but none suggested existence of a track. Additional programs contacted reported no current track.
Track Participants and Structure
HM tracks mainly target third-year residents (Table 1). Some extend into the second year of residency, and 4 have opportunities for intern involvement, including a separate match number at Colorado. Tracks accept up to 12 residents per class. Two programs, at Colorado and Virginia, are part of IM programs in which all residents belong to a track (eg, HM, primary care, research).
HM track structures vary widely and are heavily influenced by the content delivery platforms of their IM residency programs. Several HM track directors emphasized the importance of fitting into existing educational frameworks to ensure access to residents and to minimize the burden of participation. Four programs deliver the bulk of their nonclinical content in dedicated blocks; 6 others use brief recurring sessions to deliver smaller aliquots longitudinally (Table 1). The number of protected hours for content delivery ranges from 10 to more than 40 annually. All tracks use multiple content delivery modes, including didactic sessions and journal clubs. Four tracks employ panel discussions to explore career options within HM. Several also use online platforms, including discussions, readings, and modules.
Quality Improvement
The vast majority of curricula prominently feature experiential QI project involvement (Table 2). These mentored longitudinal projects allow applied delivery of content, such as QI methods and management skills. Four tracks use material from the Institute for Healthcare Improvement.8 Several also offer dedicated QI rotations that immerse residents in ongoing QI efforts.
Institutional partnerships support these initiatives at several sites. The Minnesota track is a joint venture of the university and Regions Hospital, a nonprofit community hospital. The Virginia track positions HM residents to lead university-wide interdisciplinary QI teams. For project support, the Colorado and Kentucky tracks partner with local QI resources—the Institute for Healthcare Quality, Safety, and Efficiency at Colorado and the Office of Value and Innovation in Healthcare Delivery at Kentucky.
Health Care Economics and Value
Many programs leverage the rapidly growing emphasis on health care “value” as an opportunity for synergy between IM programs and HM tracks. Examples include involving residents in efforts to improve documentation or didactic instruction on topics such as health care finance. The New Mexico and Wake Forest tracks offer elective rotations on health care economics. Several track directors mentioned successfully expanding curricula on health care value from the HM track into IM residency programs at large, providing a measurable service to the residency programs while ensuring content delivery and freeing up additional time for track activities.
Scholarship and Career Development
Most programs provide targeted career development for residents. Six tracks provide sessions on job procurement skills, such as curriculum vitae preparation and interviewing (Table 2). Many also provide content on venues for disseminating scholarly activity. The Colorado, Kentucky, New Mexico, and Tulane programs feature content on abstract and poster creation. Leadership development is addressed in several tracks through dedicated track activities or participation in discrete, outside-track events. Specifically, Colorado offers a leadership track for residents interested in hospital administration, Cleveland has a leadership journal club, Wake Forest enrolls HM residents in leadership training available through the university, and Minnesota sends residents to the Society of Hospital Medicine’s Leadership Academy (Table 2).
Clinical Rotations
Almost all tracks include a clinical rotation, typically pairing residents directly with hospitalist attendings to encourage autonomy and mentorship. Several also offer elective rotations in various disciplines within HM (Table 2). The Kentucky and Virginia tracks incorporate working with advanced practice providers into their practicums. The Cleveland, Minnesota, Tulane, and Virginia tracks offer HM rotations in community hospitals or postacute settings.
HM rotations also pair clinical experiences with didactic education on relevant topics (eg, billing and coding). The Cleveland, Minnesota, and Virginia tracks developed clinical rotations reflecting the common 7-on and 7-off schedule with nonclinical obligations, such as seminars linking specific content to clinical experiences, during nonclinical time.
DISCUSSION
Our investigation into the current state of HM training found that HM track curricula focus largely on QI, health care economics, and professional development. This focus likely developed in response to hospitalists’ increasing engagement in related endeavors. HM tracks have dynamic and variable structures, reflecting an evolving field and the need to fit into existing IM residency program structures. Similarly, the content covered in HM tracks is tightly linked to perceived opportunities within IM residency curricula. The heterogeneity of content suggests the breadth and ambiguity of necessary competencies for aspiring hospitalists. One of the 11 tracks has not had any residents enroll within the past few years—a testament to the continued effort necessary to sustain such tracks, including curricular updates and recruiting. Conversely, many programs now share track content with the larger IM residency program, suggesting HM tracks may be near the forefront of medical education in some areas.
Our study had several limitations. As we are unaware of any databases of HM tracks, we discussed tracks with professional contacts, performed Internet searches, and reviewed IM residency program websites. Our search, however, was not exhaustive; despite our best efforts, we may have missed or mischaracterized some track offerings. Nevertheless, we think that our analysis represents the first thorough compilation of HM tracks and that it will be useful to institutions seeking to create or enhance HM-specific training.
As the field continues to evolve, we are optimistic about the future of HM training. We suspect that HM residency training tracks will continue to expand. More work is needed so these tracks can adjust to the changing HM and IM residency program landscapes and supply well-trained physicians for the HM workforce.
Acknowledgment
The authors thank track directors Alpesh Amin, David Gugliotti, Rick Hilger, Karnjit Johl, Nasir Majeed, Georgia McIntosh, Charles Pizanis, and Jeff Wiese for making this study possible.
Disclosure
Nothing to report.
1. Glasheen JJ, Siegal EM, Epstein K, Kutner J, Prochazka AV. Fulfilling the promise of hospital medicine: tailoring internal medicine training to address hospitalists’ needs [published correction appears in J Gen Intern Med. 2008;23(11):1931]. J Gen Intern Med. 2008;23(7):1110-1115. PubMed
2. Arora V, Guardiano S, Donaldson D, Storch I, Hemstreet P. Closing the gap between internal medicine training and practice: recommendations from recent graduates. Am J Med. 2005;118(6):680-685. PubMed
3. Glasheen JJ, Goldenberg J, Nelson JR. Achieving hospital medicine’s promise through internal medicine residency redesign. Mt Sinai J Med. 2008;75(5):436-441. PubMed
4. Wiese J. Residency training: beginning with the end in mind. J Gen Intern Med. 2008;23(7):1122-1123. PubMed
5. Glasheen JJ, Epstein KR, Siegal E, Kutner JS, Prochazka AV. The spectrum of community-based hospitalist practice: a call to tailor internal medicine residency training. Arch Intern Med. 2007;167(7):727-728. PubMed
6. Patel N, Brennan PJ, Metlay J, Bellini L, Shannon RP, Myers JS. Building the pipeline: the creation of a residency training pathway for future physician leaders in health care quality. Acad Med. 2015;90(2):185-190. PubMed
7. Kumar A, Smeraglio A, Witteles R, et al. A resident-created hospitalist curriculum for internal medicine housestaff. J Hosp Med. 2016;11(9):646-649. PubMed
8. Institute for Healthcare Improvement website. http://www.ihi.org. Accessed December 15, 2015.
1. Glasheen JJ, Siegal EM, Epstein K, Kutner J, Prochazka AV. Fulfilling the promise of hospital medicine: tailoring internal medicine training to address hospitalists’ needs [published correction appears in J Gen Intern Med. 2008;23(11):1931]. J Gen Intern Med. 2008;23(7):1110-1115. PubMed
2. Arora V, Guardiano S, Donaldson D, Storch I, Hemstreet P. Closing the gap between internal medicine training and practice: recommendations from recent graduates. Am J Med. 2005;118(6):680-685. PubMed
3. Glasheen JJ, Goldenberg J, Nelson JR. Achieving hospital medicine’s promise through internal medicine residency redesign. Mt Sinai J Med. 2008;75(5):436-441. PubMed
4. Wiese J. Residency training: beginning with the end in mind. J Gen Intern Med. 2008;23(7):1122-1123. PubMed
5. Glasheen JJ, Epstein KR, Siegal E, Kutner JS, Prochazka AV. The spectrum of community-based hospitalist practice: a call to tailor internal medicine residency training. Arch Intern Med. 2007;167(7):727-728. PubMed
6. Patel N, Brennan PJ, Metlay J, Bellini L, Shannon RP, Myers JS. Building the pipeline: the creation of a residency training pathway for future physician leaders in health care quality. Acad Med. 2015;90(2):185-190. PubMed
7. Kumar A, Smeraglio A, Witteles R, et al. A resident-created hospitalist curriculum for internal medicine housestaff. J Hosp Med. 2016;11(9):646-649. PubMed
8. Institute for Healthcare Improvement website. http://www.ihi.org. Accessed December 15, 2015.
© 2017 Society of Hospital Medicine
State Medicaid Expansion Status
On January 1, 2014, several major provisions of the Affordable Care Act (ACA) took effect, including introduction of the individual mandate for health insurance coverage, opening of the Health Insurance Marketplace, and expansion of Medicaid eligibility to Americans earning up to 133% of the federal poverty level.[1] Nearly 9 million US adults have enrolled in Medicaid since that time, primarily in the 31 states and Washington, DC that have opted into Medicaid expansion.[2, 3] ACA implementation has also had a significant impact on hospital payer mix, primarily by reducing the volume of uncompensated care in Medicaid‐expansion states.[4, 5]
The differential shift in payer mix in Medicaid‐expansion versus nonexpansion states may be relevant to hospitals beyond reimbursement. Medicaid insurance has historically been associated with longer hospitalizations and higher in‐hospital mortality in diverse patient populations, more so than commercial insurance and often even uninsured payer status.[6, 7, 8, 9, 10, 11, 12, 13, 14, 15] The disparity in outcomes between patients with Medicaid versus other insurance persists even after adjustment for disease severity and baseline comorbidities. Insurance type may influence the delivery of inpatient care through variation in access to invasive procedures and adherence to guideline‐concordant medical therapies.[9, 10, 11, 12] Medicaid patients may be more likely than uninsured patients to remain hospitalized pending postacute care placement rather than be discharged home with family support.[16] Medicaid patients are also less likely to leave against medical advice than uninsured patients.[17]
Currently, little is known about the impact of state Medicaid expansion status on length of stay (LOS) or mortality nationally. It is possible that hospitals in Medicaid‐expansion states have experienced relative worsening in LOS and mortality as their share of Medicaid patients has grown. Determining the impact of ACA implementation on payer mix and patient outcomes is particularly important for academic medical centers (AMCs), as they traditionally care for the greatest percentage of both Medicaid and uninsured patients.[18] We sought to characterize the impact of state Medicaid expansion status on payer mix, LOS, and in‐hospital mortality for general medicine patients at AMCs in the United States.
METHODS
The University HealthSystem Consortium (UHC) is an alliance of 117 AMCs and 310 affiliated hospitals, representing >90% of such institutions in the US. We queried the online UHC Clinical Data Base/Resource Manager (CDB/RM) to obtain hospital‐level insurance, LOS, and mortality data for inpatients discharged from a general medicine service between October 1, 2012 and September 30, 2015. We excluded hospitals that were missing data for any month within the study period. No patient‐level data were accessed.
Our outcomes of interest were the proportion of discharges by primary payer (Medicare, commercial, Medicaid, uninsured, or other [eg, Tri‐Care or Workers' Compensation]), as well as the LOS index and mortality index. Both indices were defined as the ratio of the observed to expected values. To determine the expected LOS and mortality, the UHC 2015 risk adjustment models were applied to all cases, adjusting for variables such as patient demographics, low socioeconomic status, admit source and status, severity of illness, and comorbid conditions, as described by International Classification of Diseases, Ninth Revision codes. These models have been validated and are used for research and quality benchmarking for member institutions.[19]
We next stratified hospitals according to state Medicaid expansion status. We defined Medicaid‐expansion states as those that had expanded Medicaid by the end of the study period: Arizona, Arkansas, California, Colorado, Connecticut, Illinois, Indiana, Iowa, Kentucky, Maryland, Massachusetts, Michigan, Minnesota, Nevada, New Hampshire, New Jersey, New Mexico, New York, Ohio, Oregon, Pennsylvania, Rhode Island, Washington, Washington DC, and West Virginia. Nonexpansion states included Alabama, Florida, Georgia, Kansas, Louisiana, Missouri, Nebraska, North Carolina, South Carolina, Tennessee, Texas, Utah, Virginia, and Wisconsin. We excluded 12 states due to incomplete data: Alaska, Delaware, Hawaii, Idaho, North Dakota, Maine, Mississippi, Montana, Oklahoma, South Dakota, Vermont, and Wyoming.
We then identified our pre‐ and post‐ACA implementation periods. Medicaid coverage expansion took effect in all expansion states on January 1, 2014, with the exception of Michigan (April 1, 2014), New Hampshire (August 15, 2014), Pennsylvania (January 1, 2015), and Indiana (February 1, 2015).[3] We therefore defined October 1, 2012 to December 31, 2013 as the pre‐ACA implementation period and January 1, 2014 to September 30, 2015 as the post‐ACA implementation period for all states except for Michigan, New Hampshire, Pennsylvania, and Indiana. For these 4 states, we customized the pre‐ and post‐ACA implementation periods to their respective dates of Medicaid expansion; for New Hampshire, we designated October 1, 2012 to July 31, 2014 as the pre‐ACA implementation period and September 1, 2014 to September 30, 2015 as the post‐ACA implementation period, as we were unable to distinguish before versus after data in August 2014 based on the midmonth expansion of Medicaid.
After stratifying hospitals into groups based on whether they were located in Medicaid‐expansion or nonexpansion states, the proportion of discharges by payer was compared between pre‐ and post‐ACA implementation periods both graphically by quarter and using linear regression models weighted for the number of cases from each hospital. Next, for both Medicaid‐expansion and nonexpansion hospitals, LOS index and mortality index were compared before and after ACA implementation using linear regression models weighted for the number of cases from each hospital, both overall and by payer. Difference‐in‐differences estimations were then completed to compare the proportion of discharges by payer, LOS index, and mortality index between Medicaid‐expansion and nonexpansion hospitals before and after ACA implementation. Post hoc linear regression analyses were completed to evaluate the effect of clustering by state level strata on payer mix and LOS and mortality indices. A 2‐sided P value of <0.05 was considered statistically significant. Data analyses were performed using Stata 12.0 (StataCorp, College Station, TX).
RESULTS
We identified 4,258,952 discharges among general medicine patients from 211 hospitals in 38 states and Washington, DC between October 1, 2012, and September 30, 2015. This included 3,144,488 discharges from 156 hospitals in 24 Medicaid‐expansion states and Washington, DC and 1,114,464 discharges from 55 hospitals in 14 nonexpansion states.
Figure 1 shows the trends in payer mix over time for hospitals in both Medicaid‐expansion and nonexpansion states. As summarized in Table 1, hospitals in Medicaid‐expansion states experienced a significant 3.7‐percentage point increase in Medicaid discharges (P = 0.013) and 2.9‐percentage point decrease in uninsured discharges (P < 0.001) after ACA implementation. This represented an approximately 19% jump and 60% drop in Medicaid and uninsured discharges, respectively. Hospitals in nonexpansion states saw no significant change in the proportion of discharges by payer after ACA implementation. In the difference‐in‐differences analysis, there was a trend toward a greater change in the proportion of Medicaid discharges pre‐ to post‐ACA implementation among hospitals in Medicaid‐expansion states compared to hospitals in nonexpansion states (mean difference‐in‐differences 4.1%, 95% confidence interval [CI]: 0.3%, 8.6%, P = 0.070).
Medicaid‐expansion n=156 hospitals; 3,144,488 cases | Non‐expansion n=55 hospitals; 1,114,464 cases | |||||||||
---|---|---|---|---|---|---|---|---|---|---|
Pre‐ACA Implementation (1,453,090 Cases) | Post‐ACA Implementation (1,691,398 Cases) | Mean Difference | P Value | Pre‐ACA Implementation (455,440 Cases) | Post‐ACA Implementation (659,024 Cases) | Mean Difference | P Value | Mean Difference‐in‐Differences | P Value | |
| ||||||||||
Payer mix, % (95% CI) | ||||||||||
Medicare | 48.6 (46.2, 51.0)* | 48.3 (45.9, 50.7) | 0.3 (3.6, 3.1) | 0.865 | 44.3 (40.7, 47.7)* | 45.3 (41.9, 48.6) | 1.0 (3.8, 5.8) | 0.671 | 1.3 (7.1, 4.5) | 0.655 |
Commercial | 23.1 (21.4, 24.7) | 23.2 (21.8, 24.6) | 0.2 (2.0, 2.3) | 0.882 | 21.5 (18.5, 24.6) | 22.7 (19.7, 25.8) | 1.2 (3.0, 5.4) | 0.574 | 1.0 (5.7, 3.6) | 0.662 |
Medicaid | 19.6 (17.6, 21.6) | 23.3 (21.2, 25.5) | 3.7 (0.8, 6.6) | 0.013 | 19.4 (16.9, 21.9) | 19.0 (16.5, 21.4) | 0.4 (3.8, 3.0) | 0.812 | 4.1 (0.3, 8.6) | 0.070 |
Uninsured | 5.0 (4.0, 5.9) | 2.0 (1.7, 2.3) | 2.9 (3.9, 2.0) | <0.001 | 10.9 (8.1, 13.7) | 9.4 (7.0, 11.7) | 1.5 (5.1, 2.1) | 0.407 | 1.4 (5.1, 2.2) | 0.442 |
Other | 3.8 (2.6, 4.9) | 3.1 (2.0, 4.3) | 0.7 (2.3, 1.0) | 0.435 | 4.0 (2.9, 5.0) | 3.7 (2.6, 4.7) | 0.3 (1.7, 1.1) | 0.662 | 0.3 (2.5, 1.8) | 0.762 |
LOS index, mean (95% CI) | ||||||||||
Overall | 1.017 (0.996, 1.038) | 1.006 (0.981, 1.031) | 0.011 (0.044, 0.021) | 0.488 | 1.008 (0.974, 1.042) | 0.995 (0.961, 1.029) | 0.013 (0.061, 0.034) | 0.574 | 0.002 (0.055, 0.059) | 0.943 |
Medicare | 1.012 (0.989, 1.035) | 0.999 (0.971, 1.027) | 0.013 (0.049, 0.023) | 0.488 | 0.982 (0.946, 1.017) | 0.979 (0.944, 1.013) | 0.003 (0.052, 0.046) | 0.899 | 0.010 (0.070, 0.051) | 0.754 |
Commercial | 0.993 (0.974, 1.012) | 0.977 (0.955, 0.998) | 0.016 (0.045, 0.013) | 0.271 | 1.009 (0.978, 1.039) | 0.986 (0.956, 1.016) | 0.022 (0.065, 0.020) | 0.298 | 0.006 (0.044, 0.057) | 0.809 |
Medicaid | 1.059 (1.036, 1.082) | 1.043 (1.018, 1.067) | 0.016 (0.049, 0.017) | 0.349 | 1.064 (1.020, 1.108) | 1.060 (1.015, 1.106) | 0.004 (0.066, 0.059) | 0.911 | 0.012 (0.082, 0.057) | 0.727 |
Uninsured | 0.960 (0.933, 0.988) | 0.925 (0.890, 0.961) | 0.035 (0.080, 0.010) | 0.126 | 0.972 (0.935, 1.009) | 0.944 (0.909, 0.979) | 0.028 (0.078, 0.022) | 0.273 | 0.007 (0.074, 0.060) | 0.835 |
Other | 0.988 (0.960, 1.017) | 0.984 (0.952, 1.015) | 0.005 (0.047, 0.037) | 0.822 | 1.022 (0.973, 1.071) | 0.984 (0.944, 1.024) | 0.038 (0.100, 0.024) | 0.232 | 0.033 (0.042, 0.107) | 0.386 |
Mortality index, mean (95% CI) | ||||||||||
Overall | 1.000 (0.955, 1.045) | 0.878 (0.836, 0.921) | 0.122 (0.183, 0.061) | <0.001 | 0.997 (0.931, 1.062) | 0.850 (0.800, 0.900) | 0.147 (0.227, 0.066) | 0.001 | 0.025 (0.076, 0.125) | 0.628 |
Medicare | 0.990 (0.942, 1.038) | 0.871 (0.826, 0.917) | 0.119 (0.185, 0.053) | <0.001 | 1.000 (0.925, 1.076) | 0.844 (0.788, 0.900) | 0.156 (0.249, 0.064) | 0.001 | 0.038 (0.075, 0.150) | 0.513 |
Commercial | 1.045 (0.934, 1.155) | 0.908 (0.842, 0.975) | 0.136 (0.264, 0.008) | 0.037 | 1.023 (0.935, 1.111) | 0.820 (0.758, 0.883) | 0.203 (0.309, 0.096) | <0.001 | 0.067 (0.099, 0.232) | 0.430 |
Medicaid | 0.894 (0.845, 0.942) | 0.786 (0.748, 0.824) | 0.107 (0.168, 0.046) | 0.001 | 0.937 (0.861, 1.013) | 0.789 (0.733, 0.844) | 0.148 (0.242, 0.055) | 0.002 | 0.041 (0.069, 0.151) | 0.464 |
Uninsured | 1.172 (1.007, 1.337)∥ | 1.136 (0.968, 1.303) | 0.037 (0.271, 0.197) | 0.758 | 0.868 (0.768, 0.968)∥ | 0.850 (0.761, 0.939) | 0.017 (0.149, 0.115) | 0.795 | 0.019 (0.287, 0.248) | 0.887 |
Other | 1.376 (1.052, 1.700)# | 1.156 (0.910, 1.402) | 0.220 (0.624, 0.184) | 0.285 | 1.009 (0.868, 1.150) # | 0.874 (0.682, 1.066) | 0.135 (0.369, 0.099) | 0.254 | 0.085 (0.555, 0.380) | 0.720 |
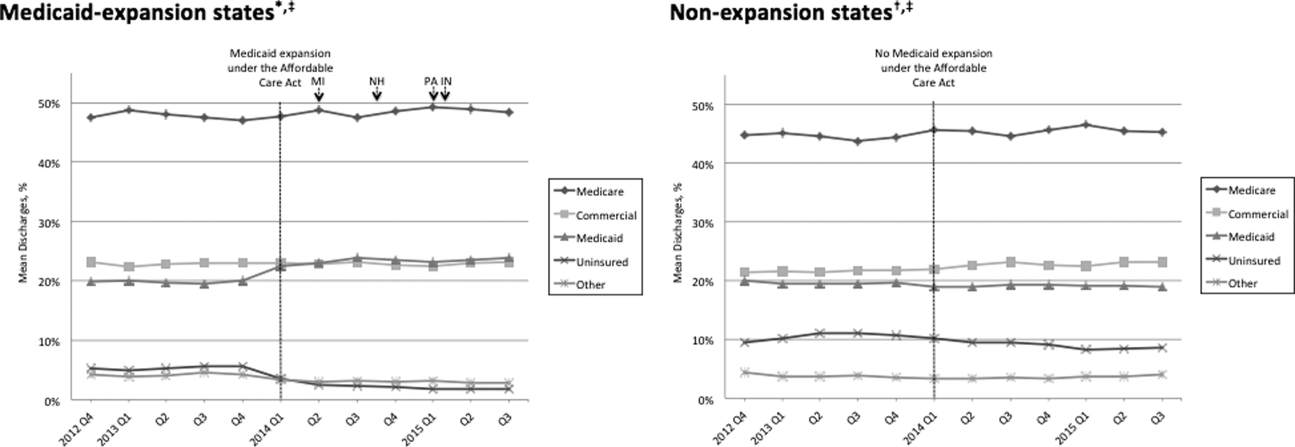
Table 1 shows that the overall LOS index remained unchanged pre‐ to post‐ACA implementation for both Medicaid‐expansion (1.017 to 1.006, P = 0.488) and nonexpansion hospitals (1.008 to 0.995, P = 0.574). LOS indices for each payer type also remained unchanged. The overall mortality index significantly improved pre‐ to post‐ACA implementation for both Medicaid‐expansion (1.000 to 0.878, P < 0.001) and nonexpansion hospitals (0.997 to 0.850, P = 0.001). Among both Medicaid‐expansion and nonexpansion hospitals, the mortality index significantly improved for Medicare, commercial, and Medicaid discharges but not for uninsured or other discharges. In the difference‐in‐differences analysis, the changes in LOS indices and mortality indices pre‐ to post‐ACA implementation did not differ significantly between hospitals in Medicaid‐expansion versus nonexpansion states.
In post hoc linear regression analyses of payer mix and LOS and mortality indices clustered by state‐level strata, point estimates were minimally changed. Although 95% CIs were slightly wider, statistical significance was unchanged from our primary analyses (data not shown).
DISCUSSION
We found that ACA implementation had a significant impact on payer mix for general medicine patients at AMCs in the United States, primarily by increasing the number of Medicaid beneficiaries and by decreasing the number of uninsured patients in Medicaid‐expansion states. State Medicaid expansion status did not appear to influence either LOS or in‐hospital mortality.
Our study offers some of the longest‐term data currently available on the impact of ACA implementation on payer mix trends and encompasses more states than others have previously. Although we uniquely focused on general medicine patients at AMCs, our results are similar to those seen for US hospitals overall. Nikpay and colleagues evaluated payer mix trends for non‐Medicare adult inpatient stays in 16 states through the second quarter of 2014 using the Healthcare Cost and Utilization Project database through the Agency for Healthcare Research and Quality.[4] They found a relative 20% increase and 50% decrease in Medicaid and uninsured discharges in Medicaid‐expansion states, along with nonsignificant changes in nonexpansion states. Hempstead and Cantor assessed payer mix for non‐Medicare discharges using state hospital association data from 21 states through the fourth quarter of 2014 and found a significant increase in Medicaid patients as well as a nearly significant decrease in uninsured patients in expansion states relative to nonexpansion states.[5] The Department of Health and Human Services also reported that uninsured/self‐pay discharges fell substantially (65%73%) in Medicaid‐expansion states by the end of 2014, with slight decreases in nonexpansion states.[20]
In contrast to our hypothesis, the overall LOS and in‐hospital mortality indices were not influenced by state Medicaid expansion status. From a purely mathematical standpoint, the contribution of Medicaid patients to the overall LOS and mortality indices may have been eclipsed by Medicare and commercially insured patients, who represented a higher proportion of total discharges. The lack of impact of state Medicaid expansion status on overall LOS and mortality indices did not appear to occur as a result of indices for Medicaid patients trending toward the mean. As predicted based on observational studies, Medicaid patients in our study tended to have a higher LOS index than those with other insurance types. Medicaid patients actually tended to have a lower mortality index in our analysis; the reason for this latter finding is unclear and in contrast to other published studies.[6, 7, 8, 9, 10, 11, 12, 13, 14, 15, 21]
To our knowledge, no other studies have evaluated the effect of payer mix changes under the ACA on inpatient outcomes. However, new evidence is emerging on outpatient outcomes. Low‐income adults in Medicaid‐expansion states have reported greater gains in access to primary care services and in the diagnosis of certain chronic health conditions than those in nonexpansion states as a result of ACA implementation.[22, 23] Such improvements in the outpatient setting might be expected to reduce patient acuity on admission. However, they would not necessarily translate to relative improvements in LOS or mortality indices for Medicaid‐expansion hospitals, as the UHC risk adjustment models controlled for disease severity on admission.
Similarly, few studies have assessed the impact of payer mix changes under previous state Medicaid expansions on inpatient outcomes. After Massachusetts expanded Medicaid and enacted near‐universal healthcare coverage in 2006, a minimal LOS reduction of just 0.05 days was observed.[24] New York expanded Medicaid eligibility to nondisabled childless adults with incomes below 100% of the federal poverty level in September 2001, whereas Arizona did so in November 2001 and Maine in October 2002. A study comparing outcomes in these states to 4 neighboring nonexpansion states found a relative reduction in annual all‐cause mortality of 6.1% population wide; however, it did not assess in‐hospital mortality.[25] The Oregon Health Insurance Experiment that randomized low‐income adults to expanded Medicaid coverage or not in 2008 has also reported on outpatient rather than inpatient outcomes.[26]
Our findings have potential implications for health policymakers. That Medicaid expansion status had a neutral effect on both LOS and mortality indices in our analysis should be reassuring for states contemplating Medicaid expansion in the future. Our results also highlight the need for further efforts to reduce disparities in inpatient care based on payer status. For example, although Medicare, commercially insured, and Medicaid patients witnessed significant improvements in mortality indices pre‐ to post‐ACA implementation in hospitals in both Medicaid‐expansion and nonexpansion states, uninsured patients did not.
This study has several limitations. First, our analysis of the impact of ACA implementation on payer mix did not account for concurrent socioeconomic trends that may have influenced insurance coverage across the United States. However, the main goal of this analysis was to demonstrate that changes in payer mix did in fact occur over time, to provide rationale for our subsequent LOS and mortality analyses. Second, we could not control for variation in the design and implementation of Medicaid expansions across states as permitted under the federal Section 1115 waiver process. Third, we only had access to hospital‐level data through the UHC CDB/RM, rather than individual patient data. We attempted to mitigate this limitation by weighting data according to the number of cases per hospital. Lastly, additional patient‐level factors that may influence LOS or mortality may not be included in the UHC risk adjustment models.
In summary, the differential shift in payer mix between Medicaid‐expansion and nonexpansion states did not influence overall LOS or in‐hospital mortality for general medicine patients at AMCs in the United States. Additional research could help to determine the impact of ACA implementation on other patient outcomes that may be dependent on insurance status, such as readmissions or hospital‐acquired complications.
Disclosures: M.E.A. conceived of the study concept and design, assisted with data acquisition, and drafted the manuscript. J.J.G. assisted with study design and made critical revisions to the manuscript. D.A. assisted with study design and made critical revisions to the manuscript. R.P. assisted with study design and made critical revisions to the manuscript. M.L. assisted with study design and data acquisition and made critical revisions to the manuscript. C.D.J. assisted with study design, performed data analyses, and made critical revisions to the manuscript. A modified abstract was presented in poster format at the University HealthSystem Consortium Annual Conference held September 30 to October 2, 2015 in Orlando, Florida, as well as at the Society of Hospital Medicine Research, Innovations, and Vignettes 2016 Annual Meeting held March 69, 2016, in San Diego, California. The authors report no conflicts of interest.
- Department of Health and Human Services. Key features of the Affordable Care Act by year. Available at: http://www.hhs.gov/healthcare/facts‐and‐features/key‐features‐of‐aca‐by‐year/index.html#2014. Accessed April 4, 2016.
- Centers for Medicare and Medicaid Services. Medicaid enrollment data collected through MBES. Available at: https://www.medicaid.gov/medicaid‐chip‐program‐information/program‐information/medicaid‐and‐chip‐enrollment‐data/medicaid‐enrollment‐data‐collected‐through‐mbes.html. Accessed April 4, 2016.
- The Henry J. Kaiser Family Foundation. Status of state action on the Medicaid expansion decision. Available at: http://kff.org/health‐reform/state‐indicator/state‐activity‐around‐expanding‐medicaid‐under‐the‐affordable‐care‐act. Accessed April 4, 2016.
- Affordable Care Act Medicaid expansion reduced uninsured hospital stays in 2014. Health Aff (Millwood). 2016;35(1):106–110. , , .
- State Medicaid expansion and changes in hospital volume according to payer. N Engl J Med. 2016;374(2):196–198. , .
- Understanding predictors of prolonged hospitalizations among general medicine patients: a guide and preliminary analysis. J Hosp Med. 2015;10(9):623–626. , , , , , .
- Impact of insurance and hospital ownership on hospital length of stay among patients with ambulatory care‐sensitive conditions. Ann Fam Med. 2011;9:489–495. , , , .
- Insurance status and hospital care for myocardial infarction, stroke, and pneumonia. J Hosp Med. 2010;5:452–459. , , .
- Payment source, quality of care, and outcomes in patients hospitalized with heart failure. J Am Coll Cardiol. 2011;58(14):1465–1471. , , , , , .
- The inpatient experience and predictors of length of stay for patients hospitalized with systolic heart failure: comparison by commercial, Medicaid, and Medicare payer type. J Med Econ. 2013;16(1):43–54. , , , , .
- Insurance coverage and care of patients with non‐ST‐segment elevation acute coronary syndromes. Ann Intern Med. 2006;145:739–748. , , et al.
- Association of insurance status with inpatient treatment for coronary artery disease: findings from the Get with the Guidelines Program. Am Heart J. 2010;159:1026–1036. , , , et al.
- Primary payer status affects mortality for major surgical operations. Ann Surg. 2010;252:544–551. , , , et al.
- Medicaid payer status is associated with in‐hospital morbidity and resource utilization following primary total joint arthroplasty. J Bone Joint Surg Am. 2014;96(21):e180. , , .
- The quality of care delivered to patients within the same hospital varies by insurance type. Health Aff (Millwood). 2013;32(10):1731–1739. , , .
- Effect of insurance status on postacute care among working age stroke survivors. Neurology. 2012;78(20):1590–1595. , , , , .
- Hospitalizations in which patients leave the hospital against medical advice (AMA), 2007. HCUP statistical brief #78. August 2009. Rockville, MD: Agency for Healthcare Research and Quality; 2009. Available at: http://www.hcup‐us.ahrq.gov/reports/statbriefs/sb78.pdf. Accessed May 12, 2016. , , , .
- Characteristics of Medicaid and uninsured hospitalizations, 2012. HCUP statistical brief #182. Rockville, MD: Agency for Healthcare Research and Quality; 2014. Available at: http://www.hcup‐us.ahrq.gov/reports/statbriefs/sb182‐Medicaid‐Uninsured‐Hospitalizations‐2012.pdf. Accessed March 9, 2016. , , , .
- Agency for Healthcare Research and Quality. Mortality measurement: mortality risk adjustment methodology for University HealthSystem Consortium. Available at: http://archive.ahrq.gov/professionals/quality‐patient‐safety/quality‐resources/tools/mortality/Meurer.pdf. Accessed May 10, 2016.
- Department of Health and Human Services. Insurance expansion, hospital uncompensated care, and the Affordable Care Act. Available at: https://aspe.hhs.gov/pdf‐report/insurance‐expansion‐hospital‐uncompensated‐care‐and‐affordable‐care‐act. Accessed May 27, 2016.
- Our flawed but beneficial Medicaid program. N Engl J Med. 2011;364(16):e31. , , , .
- Changes in self‐reported insurance coverage, access to care, and health under the Affordable Care Act. JAMA. 2015;314(4):366–374. , , , .
- Early coverage, access, utilization, and health effects associated with the Affordable Care Act Medicaid Expansions: a quasi‐experimental study. Ann Intern Med. 2016;164(12):795–803. , .
- The impact of health care reform on hospital and preventive care: evidence from Massachusetts. J Public Econ. 2012;96(11–12):909–929. , .
- Mortality and access to care among adults after state Medicaid expansions. N Engl J Med. 2012;367:1025–1034. , , .
- The Oregon Experiment—effects of Medicaid on clinical outcomes. N Engl J Med. 2013;368(18):1713–1722. , , , et al.
On January 1, 2014, several major provisions of the Affordable Care Act (ACA) took effect, including introduction of the individual mandate for health insurance coverage, opening of the Health Insurance Marketplace, and expansion of Medicaid eligibility to Americans earning up to 133% of the federal poverty level.[1] Nearly 9 million US adults have enrolled in Medicaid since that time, primarily in the 31 states and Washington, DC that have opted into Medicaid expansion.[2, 3] ACA implementation has also had a significant impact on hospital payer mix, primarily by reducing the volume of uncompensated care in Medicaid‐expansion states.[4, 5]
The differential shift in payer mix in Medicaid‐expansion versus nonexpansion states may be relevant to hospitals beyond reimbursement. Medicaid insurance has historically been associated with longer hospitalizations and higher in‐hospital mortality in diverse patient populations, more so than commercial insurance and often even uninsured payer status.[6, 7, 8, 9, 10, 11, 12, 13, 14, 15] The disparity in outcomes between patients with Medicaid versus other insurance persists even after adjustment for disease severity and baseline comorbidities. Insurance type may influence the delivery of inpatient care through variation in access to invasive procedures and adherence to guideline‐concordant medical therapies.[9, 10, 11, 12] Medicaid patients may be more likely than uninsured patients to remain hospitalized pending postacute care placement rather than be discharged home with family support.[16] Medicaid patients are also less likely to leave against medical advice than uninsured patients.[17]
Currently, little is known about the impact of state Medicaid expansion status on length of stay (LOS) or mortality nationally. It is possible that hospitals in Medicaid‐expansion states have experienced relative worsening in LOS and mortality as their share of Medicaid patients has grown. Determining the impact of ACA implementation on payer mix and patient outcomes is particularly important for academic medical centers (AMCs), as they traditionally care for the greatest percentage of both Medicaid and uninsured patients.[18] We sought to characterize the impact of state Medicaid expansion status on payer mix, LOS, and in‐hospital mortality for general medicine patients at AMCs in the United States.
METHODS
The University HealthSystem Consortium (UHC) is an alliance of 117 AMCs and 310 affiliated hospitals, representing >90% of such institutions in the US. We queried the online UHC Clinical Data Base/Resource Manager (CDB/RM) to obtain hospital‐level insurance, LOS, and mortality data for inpatients discharged from a general medicine service between October 1, 2012 and September 30, 2015. We excluded hospitals that were missing data for any month within the study period. No patient‐level data were accessed.
Our outcomes of interest were the proportion of discharges by primary payer (Medicare, commercial, Medicaid, uninsured, or other [eg, Tri‐Care or Workers' Compensation]), as well as the LOS index and mortality index. Both indices were defined as the ratio of the observed to expected values. To determine the expected LOS and mortality, the UHC 2015 risk adjustment models were applied to all cases, adjusting for variables such as patient demographics, low socioeconomic status, admit source and status, severity of illness, and comorbid conditions, as described by International Classification of Diseases, Ninth Revision codes. These models have been validated and are used for research and quality benchmarking for member institutions.[19]
We next stratified hospitals according to state Medicaid expansion status. We defined Medicaid‐expansion states as those that had expanded Medicaid by the end of the study period: Arizona, Arkansas, California, Colorado, Connecticut, Illinois, Indiana, Iowa, Kentucky, Maryland, Massachusetts, Michigan, Minnesota, Nevada, New Hampshire, New Jersey, New Mexico, New York, Ohio, Oregon, Pennsylvania, Rhode Island, Washington, Washington DC, and West Virginia. Nonexpansion states included Alabama, Florida, Georgia, Kansas, Louisiana, Missouri, Nebraska, North Carolina, South Carolina, Tennessee, Texas, Utah, Virginia, and Wisconsin. We excluded 12 states due to incomplete data: Alaska, Delaware, Hawaii, Idaho, North Dakota, Maine, Mississippi, Montana, Oklahoma, South Dakota, Vermont, and Wyoming.
We then identified our pre‐ and post‐ACA implementation periods. Medicaid coverage expansion took effect in all expansion states on January 1, 2014, with the exception of Michigan (April 1, 2014), New Hampshire (August 15, 2014), Pennsylvania (January 1, 2015), and Indiana (February 1, 2015).[3] We therefore defined October 1, 2012 to December 31, 2013 as the pre‐ACA implementation period and January 1, 2014 to September 30, 2015 as the post‐ACA implementation period for all states except for Michigan, New Hampshire, Pennsylvania, and Indiana. For these 4 states, we customized the pre‐ and post‐ACA implementation periods to their respective dates of Medicaid expansion; for New Hampshire, we designated October 1, 2012 to July 31, 2014 as the pre‐ACA implementation period and September 1, 2014 to September 30, 2015 as the post‐ACA implementation period, as we were unable to distinguish before versus after data in August 2014 based on the midmonth expansion of Medicaid.
After stratifying hospitals into groups based on whether they were located in Medicaid‐expansion or nonexpansion states, the proportion of discharges by payer was compared between pre‐ and post‐ACA implementation periods both graphically by quarter and using linear regression models weighted for the number of cases from each hospital. Next, for both Medicaid‐expansion and nonexpansion hospitals, LOS index and mortality index were compared before and after ACA implementation using linear regression models weighted for the number of cases from each hospital, both overall and by payer. Difference‐in‐differences estimations were then completed to compare the proportion of discharges by payer, LOS index, and mortality index between Medicaid‐expansion and nonexpansion hospitals before and after ACA implementation. Post hoc linear regression analyses were completed to evaluate the effect of clustering by state level strata on payer mix and LOS and mortality indices. A 2‐sided P value of <0.05 was considered statistically significant. Data analyses were performed using Stata 12.0 (StataCorp, College Station, TX).
RESULTS
We identified 4,258,952 discharges among general medicine patients from 211 hospitals in 38 states and Washington, DC between October 1, 2012, and September 30, 2015. This included 3,144,488 discharges from 156 hospitals in 24 Medicaid‐expansion states and Washington, DC and 1,114,464 discharges from 55 hospitals in 14 nonexpansion states.
Figure 1 shows the trends in payer mix over time for hospitals in both Medicaid‐expansion and nonexpansion states. As summarized in Table 1, hospitals in Medicaid‐expansion states experienced a significant 3.7‐percentage point increase in Medicaid discharges (P = 0.013) and 2.9‐percentage point decrease in uninsured discharges (P < 0.001) after ACA implementation. This represented an approximately 19% jump and 60% drop in Medicaid and uninsured discharges, respectively. Hospitals in nonexpansion states saw no significant change in the proportion of discharges by payer after ACA implementation. In the difference‐in‐differences analysis, there was a trend toward a greater change in the proportion of Medicaid discharges pre‐ to post‐ACA implementation among hospitals in Medicaid‐expansion states compared to hospitals in nonexpansion states (mean difference‐in‐differences 4.1%, 95% confidence interval [CI]: 0.3%, 8.6%, P = 0.070).
Medicaid‐expansion n=156 hospitals; 3,144,488 cases | Non‐expansion n=55 hospitals; 1,114,464 cases | |||||||||
---|---|---|---|---|---|---|---|---|---|---|
Pre‐ACA Implementation (1,453,090 Cases) | Post‐ACA Implementation (1,691,398 Cases) | Mean Difference | P Value | Pre‐ACA Implementation (455,440 Cases) | Post‐ACA Implementation (659,024 Cases) | Mean Difference | P Value | Mean Difference‐in‐Differences | P Value | |
| ||||||||||
Payer mix, % (95% CI) | ||||||||||
Medicare | 48.6 (46.2, 51.0)* | 48.3 (45.9, 50.7) | 0.3 (3.6, 3.1) | 0.865 | 44.3 (40.7, 47.7)* | 45.3 (41.9, 48.6) | 1.0 (3.8, 5.8) | 0.671 | 1.3 (7.1, 4.5) | 0.655 |
Commercial | 23.1 (21.4, 24.7) | 23.2 (21.8, 24.6) | 0.2 (2.0, 2.3) | 0.882 | 21.5 (18.5, 24.6) | 22.7 (19.7, 25.8) | 1.2 (3.0, 5.4) | 0.574 | 1.0 (5.7, 3.6) | 0.662 |
Medicaid | 19.6 (17.6, 21.6) | 23.3 (21.2, 25.5) | 3.7 (0.8, 6.6) | 0.013 | 19.4 (16.9, 21.9) | 19.0 (16.5, 21.4) | 0.4 (3.8, 3.0) | 0.812 | 4.1 (0.3, 8.6) | 0.070 |
Uninsured | 5.0 (4.0, 5.9) | 2.0 (1.7, 2.3) | 2.9 (3.9, 2.0) | <0.001 | 10.9 (8.1, 13.7) | 9.4 (7.0, 11.7) | 1.5 (5.1, 2.1) | 0.407 | 1.4 (5.1, 2.2) | 0.442 |
Other | 3.8 (2.6, 4.9) | 3.1 (2.0, 4.3) | 0.7 (2.3, 1.0) | 0.435 | 4.0 (2.9, 5.0) | 3.7 (2.6, 4.7) | 0.3 (1.7, 1.1) | 0.662 | 0.3 (2.5, 1.8) | 0.762 |
LOS index, mean (95% CI) | ||||||||||
Overall | 1.017 (0.996, 1.038) | 1.006 (0.981, 1.031) | 0.011 (0.044, 0.021) | 0.488 | 1.008 (0.974, 1.042) | 0.995 (0.961, 1.029) | 0.013 (0.061, 0.034) | 0.574 | 0.002 (0.055, 0.059) | 0.943 |
Medicare | 1.012 (0.989, 1.035) | 0.999 (0.971, 1.027) | 0.013 (0.049, 0.023) | 0.488 | 0.982 (0.946, 1.017) | 0.979 (0.944, 1.013) | 0.003 (0.052, 0.046) | 0.899 | 0.010 (0.070, 0.051) | 0.754 |
Commercial | 0.993 (0.974, 1.012) | 0.977 (0.955, 0.998) | 0.016 (0.045, 0.013) | 0.271 | 1.009 (0.978, 1.039) | 0.986 (0.956, 1.016) | 0.022 (0.065, 0.020) | 0.298 | 0.006 (0.044, 0.057) | 0.809 |
Medicaid | 1.059 (1.036, 1.082) | 1.043 (1.018, 1.067) | 0.016 (0.049, 0.017) | 0.349 | 1.064 (1.020, 1.108) | 1.060 (1.015, 1.106) | 0.004 (0.066, 0.059) | 0.911 | 0.012 (0.082, 0.057) | 0.727 |
Uninsured | 0.960 (0.933, 0.988) | 0.925 (0.890, 0.961) | 0.035 (0.080, 0.010) | 0.126 | 0.972 (0.935, 1.009) | 0.944 (0.909, 0.979) | 0.028 (0.078, 0.022) | 0.273 | 0.007 (0.074, 0.060) | 0.835 |
Other | 0.988 (0.960, 1.017) | 0.984 (0.952, 1.015) | 0.005 (0.047, 0.037) | 0.822 | 1.022 (0.973, 1.071) | 0.984 (0.944, 1.024) | 0.038 (0.100, 0.024) | 0.232 | 0.033 (0.042, 0.107) | 0.386 |
Mortality index, mean (95% CI) | ||||||||||
Overall | 1.000 (0.955, 1.045) | 0.878 (0.836, 0.921) | 0.122 (0.183, 0.061) | <0.001 | 0.997 (0.931, 1.062) | 0.850 (0.800, 0.900) | 0.147 (0.227, 0.066) | 0.001 | 0.025 (0.076, 0.125) | 0.628 |
Medicare | 0.990 (0.942, 1.038) | 0.871 (0.826, 0.917) | 0.119 (0.185, 0.053) | <0.001 | 1.000 (0.925, 1.076) | 0.844 (0.788, 0.900) | 0.156 (0.249, 0.064) | 0.001 | 0.038 (0.075, 0.150) | 0.513 |
Commercial | 1.045 (0.934, 1.155) | 0.908 (0.842, 0.975) | 0.136 (0.264, 0.008) | 0.037 | 1.023 (0.935, 1.111) | 0.820 (0.758, 0.883) | 0.203 (0.309, 0.096) | <0.001 | 0.067 (0.099, 0.232) | 0.430 |
Medicaid | 0.894 (0.845, 0.942) | 0.786 (0.748, 0.824) | 0.107 (0.168, 0.046) | 0.001 | 0.937 (0.861, 1.013) | 0.789 (0.733, 0.844) | 0.148 (0.242, 0.055) | 0.002 | 0.041 (0.069, 0.151) | 0.464 |
Uninsured | 1.172 (1.007, 1.337)∥ | 1.136 (0.968, 1.303) | 0.037 (0.271, 0.197) | 0.758 | 0.868 (0.768, 0.968)∥ | 0.850 (0.761, 0.939) | 0.017 (0.149, 0.115) | 0.795 | 0.019 (0.287, 0.248) | 0.887 |
Other | 1.376 (1.052, 1.700)# | 1.156 (0.910, 1.402) | 0.220 (0.624, 0.184) | 0.285 | 1.009 (0.868, 1.150) # | 0.874 (0.682, 1.066) | 0.135 (0.369, 0.099) | 0.254 | 0.085 (0.555, 0.380) | 0.720 |
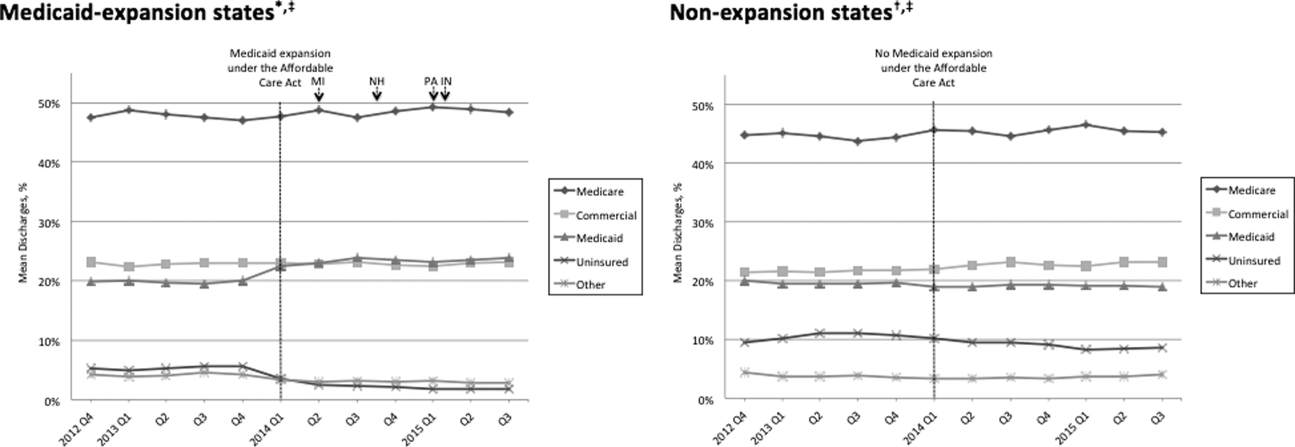
Table 1 shows that the overall LOS index remained unchanged pre‐ to post‐ACA implementation for both Medicaid‐expansion (1.017 to 1.006, P = 0.488) and nonexpansion hospitals (1.008 to 0.995, P = 0.574). LOS indices for each payer type also remained unchanged. The overall mortality index significantly improved pre‐ to post‐ACA implementation for both Medicaid‐expansion (1.000 to 0.878, P < 0.001) and nonexpansion hospitals (0.997 to 0.850, P = 0.001). Among both Medicaid‐expansion and nonexpansion hospitals, the mortality index significantly improved for Medicare, commercial, and Medicaid discharges but not for uninsured or other discharges. In the difference‐in‐differences analysis, the changes in LOS indices and mortality indices pre‐ to post‐ACA implementation did not differ significantly between hospitals in Medicaid‐expansion versus nonexpansion states.
In post hoc linear regression analyses of payer mix and LOS and mortality indices clustered by state‐level strata, point estimates were minimally changed. Although 95% CIs were slightly wider, statistical significance was unchanged from our primary analyses (data not shown).
DISCUSSION
We found that ACA implementation had a significant impact on payer mix for general medicine patients at AMCs in the United States, primarily by increasing the number of Medicaid beneficiaries and by decreasing the number of uninsured patients in Medicaid‐expansion states. State Medicaid expansion status did not appear to influence either LOS or in‐hospital mortality.
Our study offers some of the longest‐term data currently available on the impact of ACA implementation on payer mix trends and encompasses more states than others have previously. Although we uniquely focused on general medicine patients at AMCs, our results are similar to those seen for US hospitals overall. Nikpay and colleagues evaluated payer mix trends for non‐Medicare adult inpatient stays in 16 states through the second quarter of 2014 using the Healthcare Cost and Utilization Project database through the Agency for Healthcare Research and Quality.[4] They found a relative 20% increase and 50% decrease in Medicaid and uninsured discharges in Medicaid‐expansion states, along with nonsignificant changes in nonexpansion states. Hempstead and Cantor assessed payer mix for non‐Medicare discharges using state hospital association data from 21 states through the fourth quarter of 2014 and found a significant increase in Medicaid patients as well as a nearly significant decrease in uninsured patients in expansion states relative to nonexpansion states.[5] The Department of Health and Human Services also reported that uninsured/self‐pay discharges fell substantially (65%73%) in Medicaid‐expansion states by the end of 2014, with slight decreases in nonexpansion states.[20]
In contrast to our hypothesis, the overall LOS and in‐hospital mortality indices were not influenced by state Medicaid expansion status. From a purely mathematical standpoint, the contribution of Medicaid patients to the overall LOS and mortality indices may have been eclipsed by Medicare and commercially insured patients, who represented a higher proportion of total discharges. The lack of impact of state Medicaid expansion status on overall LOS and mortality indices did not appear to occur as a result of indices for Medicaid patients trending toward the mean. As predicted based on observational studies, Medicaid patients in our study tended to have a higher LOS index than those with other insurance types. Medicaid patients actually tended to have a lower mortality index in our analysis; the reason for this latter finding is unclear and in contrast to other published studies.[6, 7, 8, 9, 10, 11, 12, 13, 14, 15, 21]
To our knowledge, no other studies have evaluated the effect of payer mix changes under the ACA on inpatient outcomes. However, new evidence is emerging on outpatient outcomes. Low‐income adults in Medicaid‐expansion states have reported greater gains in access to primary care services and in the diagnosis of certain chronic health conditions than those in nonexpansion states as a result of ACA implementation.[22, 23] Such improvements in the outpatient setting might be expected to reduce patient acuity on admission. However, they would not necessarily translate to relative improvements in LOS or mortality indices for Medicaid‐expansion hospitals, as the UHC risk adjustment models controlled for disease severity on admission.
Similarly, few studies have assessed the impact of payer mix changes under previous state Medicaid expansions on inpatient outcomes. After Massachusetts expanded Medicaid and enacted near‐universal healthcare coverage in 2006, a minimal LOS reduction of just 0.05 days was observed.[24] New York expanded Medicaid eligibility to nondisabled childless adults with incomes below 100% of the federal poverty level in September 2001, whereas Arizona did so in November 2001 and Maine in October 2002. A study comparing outcomes in these states to 4 neighboring nonexpansion states found a relative reduction in annual all‐cause mortality of 6.1% population wide; however, it did not assess in‐hospital mortality.[25] The Oregon Health Insurance Experiment that randomized low‐income adults to expanded Medicaid coverage or not in 2008 has also reported on outpatient rather than inpatient outcomes.[26]
Our findings have potential implications for health policymakers. That Medicaid expansion status had a neutral effect on both LOS and mortality indices in our analysis should be reassuring for states contemplating Medicaid expansion in the future. Our results also highlight the need for further efforts to reduce disparities in inpatient care based on payer status. For example, although Medicare, commercially insured, and Medicaid patients witnessed significant improvements in mortality indices pre‐ to post‐ACA implementation in hospitals in both Medicaid‐expansion and nonexpansion states, uninsured patients did not.
This study has several limitations. First, our analysis of the impact of ACA implementation on payer mix did not account for concurrent socioeconomic trends that may have influenced insurance coverage across the United States. However, the main goal of this analysis was to demonstrate that changes in payer mix did in fact occur over time, to provide rationale for our subsequent LOS and mortality analyses. Second, we could not control for variation in the design and implementation of Medicaid expansions across states as permitted under the federal Section 1115 waiver process. Third, we only had access to hospital‐level data through the UHC CDB/RM, rather than individual patient data. We attempted to mitigate this limitation by weighting data according to the number of cases per hospital. Lastly, additional patient‐level factors that may influence LOS or mortality may not be included in the UHC risk adjustment models.
In summary, the differential shift in payer mix between Medicaid‐expansion and nonexpansion states did not influence overall LOS or in‐hospital mortality for general medicine patients at AMCs in the United States. Additional research could help to determine the impact of ACA implementation on other patient outcomes that may be dependent on insurance status, such as readmissions or hospital‐acquired complications.
Disclosures: M.E.A. conceived of the study concept and design, assisted with data acquisition, and drafted the manuscript. J.J.G. assisted with study design and made critical revisions to the manuscript. D.A. assisted with study design and made critical revisions to the manuscript. R.P. assisted with study design and made critical revisions to the manuscript. M.L. assisted with study design and data acquisition and made critical revisions to the manuscript. C.D.J. assisted with study design, performed data analyses, and made critical revisions to the manuscript. A modified abstract was presented in poster format at the University HealthSystem Consortium Annual Conference held September 30 to October 2, 2015 in Orlando, Florida, as well as at the Society of Hospital Medicine Research, Innovations, and Vignettes 2016 Annual Meeting held March 69, 2016, in San Diego, California. The authors report no conflicts of interest.
On January 1, 2014, several major provisions of the Affordable Care Act (ACA) took effect, including introduction of the individual mandate for health insurance coverage, opening of the Health Insurance Marketplace, and expansion of Medicaid eligibility to Americans earning up to 133% of the federal poverty level.[1] Nearly 9 million US adults have enrolled in Medicaid since that time, primarily in the 31 states and Washington, DC that have opted into Medicaid expansion.[2, 3] ACA implementation has also had a significant impact on hospital payer mix, primarily by reducing the volume of uncompensated care in Medicaid‐expansion states.[4, 5]
The differential shift in payer mix in Medicaid‐expansion versus nonexpansion states may be relevant to hospitals beyond reimbursement. Medicaid insurance has historically been associated with longer hospitalizations and higher in‐hospital mortality in diverse patient populations, more so than commercial insurance and often even uninsured payer status.[6, 7, 8, 9, 10, 11, 12, 13, 14, 15] The disparity in outcomes between patients with Medicaid versus other insurance persists even after adjustment for disease severity and baseline comorbidities. Insurance type may influence the delivery of inpatient care through variation in access to invasive procedures and adherence to guideline‐concordant medical therapies.[9, 10, 11, 12] Medicaid patients may be more likely than uninsured patients to remain hospitalized pending postacute care placement rather than be discharged home with family support.[16] Medicaid patients are also less likely to leave against medical advice than uninsured patients.[17]
Currently, little is known about the impact of state Medicaid expansion status on length of stay (LOS) or mortality nationally. It is possible that hospitals in Medicaid‐expansion states have experienced relative worsening in LOS and mortality as their share of Medicaid patients has grown. Determining the impact of ACA implementation on payer mix and patient outcomes is particularly important for academic medical centers (AMCs), as they traditionally care for the greatest percentage of both Medicaid and uninsured patients.[18] We sought to characterize the impact of state Medicaid expansion status on payer mix, LOS, and in‐hospital mortality for general medicine patients at AMCs in the United States.
METHODS
The University HealthSystem Consortium (UHC) is an alliance of 117 AMCs and 310 affiliated hospitals, representing >90% of such institutions in the US. We queried the online UHC Clinical Data Base/Resource Manager (CDB/RM) to obtain hospital‐level insurance, LOS, and mortality data for inpatients discharged from a general medicine service between October 1, 2012 and September 30, 2015. We excluded hospitals that were missing data for any month within the study period. No patient‐level data were accessed.
Our outcomes of interest were the proportion of discharges by primary payer (Medicare, commercial, Medicaid, uninsured, or other [eg, Tri‐Care or Workers' Compensation]), as well as the LOS index and mortality index. Both indices were defined as the ratio of the observed to expected values. To determine the expected LOS and mortality, the UHC 2015 risk adjustment models were applied to all cases, adjusting for variables such as patient demographics, low socioeconomic status, admit source and status, severity of illness, and comorbid conditions, as described by International Classification of Diseases, Ninth Revision codes. These models have been validated and are used for research and quality benchmarking for member institutions.[19]
We next stratified hospitals according to state Medicaid expansion status. We defined Medicaid‐expansion states as those that had expanded Medicaid by the end of the study period: Arizona, Arkansas, California, Colorado, Connecticut, Illinois, Indiana, Iowa, Kentucky, Maryland, Massachusetts, Michigan, Minnesota, Nevada, New Hampshire, New Jersey, New Mexico, New York, Ohio, Oregon, Pennsylvania, Rhode Island, Washington, Washington DC, and West Virginia. Nonexpansion states included Alabama, Florida, Georgia, Kansas, Louisiana, Missouri, Nebraska, North Carolina, South Carolina, Tennessee, Texas, Utah, Virginia, and Wisconsin. We excluded 12 states due to incomplete data: Alaska, Delaware, Hawaii, Idaho, North Dakota, Maine, Mississippi, Montana, Oklahoma, South Dakota, Vermont, and Wyoming.
We then identified our pre‐ and post‐ACA implementation periods. Medicaid coverage expansion took effect in all expansion states on January 1, 2014, with the exception of Michigan (April 1, 2014), New Hampshire (August 15, 2014), Pennsylvania (January 1, 2015), and Indiana (February 1, 2015).[3] We therefore defined October 1, 2012 to December 31, 2013 as the pre‐ACA implementation period and January 1, 2014 to September 30, 2015 as the post‐ACA implementation period for all states except for Michigan, New Hampshire, Pennsylvania, and Indiana. For these 4 states, we customized the pre‐ and post‐ACA implementation periods to their respective dates of Medicaid expansion; for New Hampshire, we designated October 1, 2012 to July 31, 2014 as the pre‐ACA implementation period and September 1, 2014 to September 30, 2015 as the post‐ACA implementation period, as we were unable to distinguish before versus after data in August 2014 based on the midmonth expansion of Medicaid.
After stratifying hospitals into groups based on whether they were located in Medicaid‐expansion or nonexpansion states, the proportion of discharges by payer was compared between pre‐ and post‐ACA implementation periods both graphically by quarter and using linear regression models weighted for the number of cases from each hospital. Next, for both Medicaid‐expansion and nonexpansion hospitals, LOS index and mortality index were compared before and after ACA implementation using linear regression models weighted for the number of cases from each hospital, both overall and by payer. Difference‐in‐differences estimations were then completed to compare the proportion of discharges by payer, LOS index, and mortality index between Medicaid‐expansion and nonexpansion hospitals before and after ACA implementation. Post hoc linear regression analyses were completed to evaluate the effect of clustering by state level strata on payer mix and LOS and mortality indices. A 2‐sided P value of <0.05 was considered statistically significant. Data analyses were performed using Stata 12.0 (StataCorp, College Station, TX).
RESULTS
We identified 4,258,952 discharges among general medicine patients from 211 hospitals in 38 states and Washington, DC between October 1, 2012, and September 30, 2015. This included 3,144,488 discharges from 156 hospitals in 24 Medicaid‐expansion states and Washington, DC and 1,114,464 discharges from 55 hospitals in 14 nonexpansion states.
Figure 1 shows the trends in payer mix over time for hospitals in both Medicaid‐expansion and nonexpansion states. As summarized in Table 1, hospitals in Medicaid‐expansion states experienced a significant 3.7‐percentage point increase in Medicaid discharges (P = 0.013) and 2.9‐percentage point decrease in uninsured discharges (P < 0.001) after ACA implementation. This represented an approximately 19% jump and 60% drop in Medicaid and uninsured discharges, respectively. Hospitals in nonexpansion states saw no significant change in the proportion of discharges by payer after ACA implementation. In the difference‐in‐differences analysis, there was a trend toward a greater change in the proportion of Medicaid discharges pre‐ to post‐ACA implementation among hospitals in Medicaid‐expansion states compared to hospitals in nonexpansion states (mean difference‐in‐differences 4.1%, 95% confidence interval [CI]: 0.3%, 8.6%, P = 0.070).
Medicaid‐expansion n=156 hospitals; 3,144,488 cases | Non‐expansion n=55 hospitals; 1,114,464 cases | |||||||||
---|---|---|---|---|---|---|---|---|---|---|
Pre‐ACA Implementation (1,453,090 Cases) | Post‐ACA Implementation (1,691,398 Cases) | Mean Difference | P Value | Pre‐ACA Implementation (455,440 Cases) | Post‐ACA Implementation (659,024 Cases) | Mean Difference | P Value | Mean Difference‐in‐Differences | P Value | |
| ||||||||||
Payer mix, % (95% CI) | ||||||||||
Medicare | 48.6 (46.2, 51.0)* | 48.3 (45.9, 50.7) | 0.3 (3.6, 3.1) | 0.865 | 44.3 (40.7, 47.7)* | 45.3 (41.9, 48.6) | 1.0 (3.8, 5.8) | 0.671 | 1.3 (7.1, 4.5) | 0.655 |
Commercial | 23.1 (21.4, 24.7) | 23.2 (21.8, 24.6) | 0.2 (2.0, 2.3) | 0.882 | 21.5 (18.5, 24.6) | 22.7 (19.7, 25.8) | 1.2 (3.0, 5.4) | 0.574 | 1.0 (5.7, 3.6) | 0.662 |
Medicaid | 19.6 (17.6, 21.6) | 23.3 (21.2, 25.5) | 3.7 (0.8, 6.6) | 0.013 | 19.4 (16.9, 21.9) | 19.0 (16.5, 21.4) | 0.4 (3.8, 3.0) | 0.812 | 4.1 (0.3, 8.6) | 0.070 |
Uninsured | 5.0 (4.0, 5.9) | 2.0 (1.7, 2.3) | 2.9 (3.9, 2.0) | <0.001 | 10.9 (8.1, 13.7) | 9.4 (7.0, 11.7) | 1.5 (5.1, 2.1) | 0.407 | 1.4 (5.1, 2.2) | 0.442 |
Other | 3.8 (2.6, 4.9) | 3.1 (2.0, 4.3) | 0.7 (2.3, 1.0) | 0.435 | 4.0 (2.9, 5.0) | 3.7 (2.6, 4.7) | 0.3 (1.7, 1.1) | 0.662 | 0.3 (2.5, 1.8) | 0.762 |
LOS index, mean (95% CI) | ||||||||||
Overall | 1.017 (0.996, 1.038) | 1.006 (0.981, 1.031) | 0.011 (0.044, 0.021) | 0.488 | 1.008 (0.974, 1.042) | 0.995 (0.961, 1.029) | 0.013 (0.061, 0.034) | 0.574 | 0.002 (0.055, 0.059) | 0.943 |
Medicare | 1.012 (0.989, 1.035) | 0.999 (0.971, 1.027) | 0.013 (0.049, 0.023) | 0.488 | 0.982 (0.946, 1.017) | 0.979 (0.944, 1.013) | 0.003 (0.052, 0.046) | 0.899 | 0.010 (0.070, 0.051) | 0.754 |
Commercial | 0.993 (0.974, 1.012) | 0.977 (0.955, 0.998) | 0.016 (0.045, 0.013) | 0.271 | 1.009 (0.978, 1.039) | 0.986 (0.956, 1.016) | 0.022 (0.065, 0.020) | 0.298 | 0.006 (0.044, 0.057) | 0.809 |
Medicaid | 1.059 (1.036, 1.082) | 1.043 (1.018, 1.067) | 0.016 (0.049, 0.017) | 0.349 | 1.064 (1.020, 1.108) | 1.060 (1.015, 1.106) | 0.004 (0.066, 0.059) | 0.911 | 0.012 (0.082, 0.057) | 0.727 |
Uninsured | 0.960 (0.933, 0.988) | 0.925 (0.890, 0.961) | 0.035 (0.080, 0.010) | 0.126 | 0.972 (0.935, 1.009) | 0.944 (0.909, 0.979) | 0.028 (0.078, 0.022) | 0.273 | 0.007 (0.074, 0.060) | 0.835 |
Other | 0.988 (0.960, 1.017) | 0.984 (0.952, 1.015) | 0.005 (0.047, 0.037) | 0.822 | 1.022 (0.973, 1.071) | 0.984 (0.944, 1.024) | 0.038 (0.100, 0.024) | 0.232 | 0.033 (0.042, 0.107) | 0.386 |
Mortality index, mean (95% CI) | ||||||||||
Overall | 1.000 (0.955, 1.045) | 0.878 (0.836, 0.921) | 0.122 (0.183, 0.061) | <0.001 | 0.997 (0.931, 1.062) | 0.850 (0.800, 0.900) | 0.147 (0.227, 0.066) | 0.001 | 0.025 (0.076, 0.125) | 0.628 |
Medicare | 0.990 (0.942, 1.038) | 0.871 (0.826, 0.917) | 0.119 (0.185, 0.053) | <0.001 | 1.000 (0.925, 1.076) | 0.844 (0.788, 0.900) | 0.156 (0.249, 0.064) | 0.001 | 0.038 (0.075, 0.150) | 0.513 |
Commercial | 1.045 (0.934, 1.155) | 0.908 (0.842, 0.975) | 0.136 (0.264, 0.008) | 0.037 | 1.023 (0.935, 1.111) | 0.820 (0.758, 0.883) | 0.203 (0.309, 0.096) | <0.001 | 0.067 (0.099, 0.232) | 0.430 |
Medicaid | 0.894 (0.845, 0.942) | 0.786 (0.748, 0.824) | 0.107 (0.168, 0.046) | 0.001 | 0.937 (0.861, 1.013) | 0.789 (0.733, 0.844) | 0.148 (0.242, 0.055) | 0.002 | 0.041 (0.069, 0.151) | 0.464 |
Uninsured | 1.172 (1.007, 1.337)∥ | 1.136 (0.968, 1.303) | 0.037 (0.271, 0.197) | 0.758 | 0.868 (0.768, 0.968)∥ | 0.850 (0.761, 0.939) | 0.017 (0.149, 0.115) | 0.795 | 0.019 (0.287, 0.248) | 0.887 |
Other | 1.376 (1.052, 1.700)# | 1.156 (0.910, 1.402) | 0.220 (0.624, 0.184) | 0.285 | 1.009 (0.868, 1.150) # | 0.874 (0.682, 1.066) | 0.135 (0.369, 0.099) | 0.254 | 0.085 (0.555, 0.380) | 0.720 |
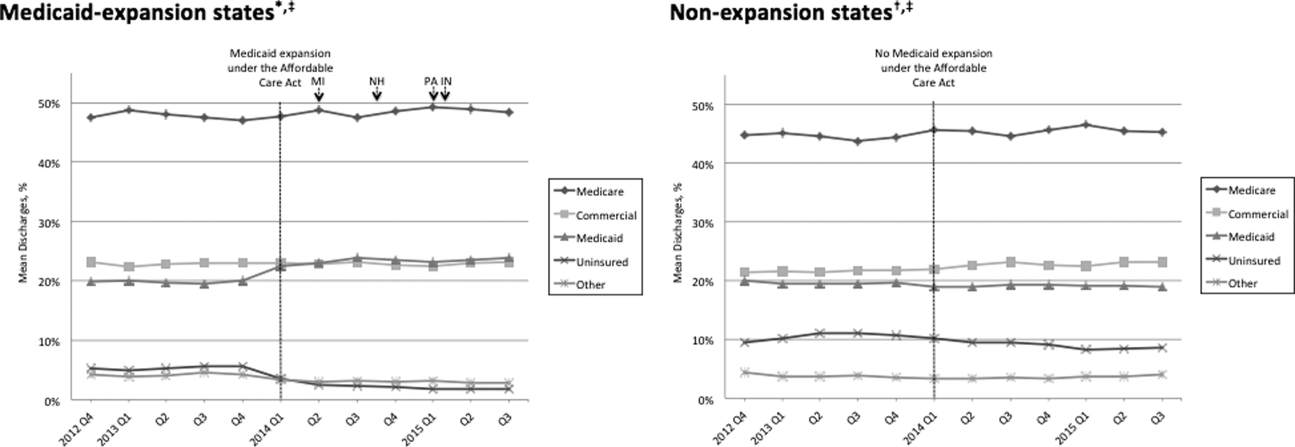
Table 1 shows that the overall LOS index remained unchanged pre‐ to post‐ACA implementation for both Medicaid‐expansion (1.017 to 1.006, P = 0.488) and nonexpansion hospitals (1.008 to 0.995, P = 0.574). LOS indices for each payer type also remained unchanged. The overall mortality index significantly improved pre‐ to post‐ACA implementation for both Medicaid‐expansion (1.000 to 0.878, P < 0.001) and nonexpansion hospitals (0.997 to 0.850, P = 0.001). Among both Medicaid‐expansion and nonexpansion hospitals, the mortality index significantly improved for Medicare, commercial, and Medicaid discharges but not for uninsured or other discharges. In the difference‐in‐differences analysis, the changes in LOS indices and mortality indices pre‐ to post‐ACA implementation did not differ significantly between hospitals in Medicaid‐expansion versus nonexpansion states.
In post hoc linear regression analyses of payer mix and LOS and mortality indices clustered by state‐level strata, point estimates were minimally changed. Although 95% CIs were slightly wider, statistical significance was unchanged from our primary analyses (data not shown).
DISCUSSION
We found that ACA implementation had a significant impact on payer mix for general medicine patients at AMCs in the United States, primarily by increasing the number of Medicaid beneficiaries and by decreasing the number of uninsured patients in Medicaid‐expansion states. State Medicaid expansion status did not appear to influence either LOS or in‐hospital mortality.
Our study offers some of the longest‐term data currently available on the impact of ACA implementation on payer mix trends and encompasses more states than others have previously. Although we uniquely focused on general medicine patients at AMCs, our results are similar to those seen for US hospitals overall. Nikpay and colleagues evaluated payer mix trends for non‐Medicare adult inpatient stays in 16 states through the second quarter of 2014 using the Healthcare Cost and Utilization Project database through the Agency for Healthcare Research and Quality.[4] They found a relative 20% increase and 50% decrease in Medicaid and uninsured discharges in Medicaid‐expansion states, along with nonsignificant changes in nonexpansion states. Hempstead and Cantor assessed payer mix for non‐Medicare discharges using state hospital association data from 21 states through the fourth quarter of 2014 and found a significant increase in Medicaid patients as well as a nearly significant decrease in uninsured patients in expansion states relative to nonexpansion states.[5] The Department of Health and Human Services also reported that uninsured/self‐pay discharges fell substantially (65%73%) in Medicaid‐expansion states by the end of 2014, with slight decreases in nonexpansion states.[20]
In contrast to our hypothesis, the overall LOS and in‐hospital mortality indices were not influenced by state Medicaid expansion status. From a purely mathematical standpoint, the contribution of Medicaid patients to the overall LOS and mortality indices may have been eclipsed by Medicare and commercially insured patients, who represented a higher proportion of total discharges. The lack of impact of state Medicaid expansion status on overall LOS and mortality indices did not appear to occur as a result of indices for Medicaid patients trending toward the mean. As predicted based on observational studies, Medicaid patients in our study tended to have a higher LOS index than those with other insurance types. Medicaid patients actually tended to have a lower mortality index in our analysis; the reason for this latter finding is unclear and in contrast to other published studies.[6, 7, 8, 9, 10, 11, 12, 13, 14, 15, 21]
To our knowledge, no other studies have evaluated the effect of payer mix changes under the ACA on inpatient outcomes. However, new evidence is emerging on outpatient outcomes. Low‐income adults in Medicaid‐expansion states have reported greater gains in access to primary care services and in the diagnosis of certain chronic health conditions than those in nonexpansion states as a result of ACA implementation.[22, 23] Such improvements in the outpatient setting might be expected to reduce patient acuity on admission. However, they would not necessarily translate to relative improvements in LOS or mortality indices for Medicaid‐expansion hospitals, as the UHC risk adjustment models controlled for disease severity on admission.
Similarly, few studies have assessed the impact of payer mix changes under previous state Medicaid expansions on inpatient outcomes. After Massachusetts expanded Medicaid and enacted near‐universal healthcare coverage in 2006, a minimal LOS reduction of just 0.05 days was observed.[24] New York expanded Medicaid eligibility to nondisabled childless adults with incomes below 100% of the federal poverty level in September 2001, whereas Arizona did so in November 2001 and Maine in October 2002. A study comparing outcomes in these states to 4 neighboring nonexpansion states found a relative reduction in annual all‐cause mortality of 6.1% population wide; however, it did not assess in‐hospital mortality.[25] The Oregon Health Insurance Experiment that randomized low‐income adults to expanded Medicaid coverage or not in 2008 has also reported on outpatient rather than inpatient outcomes.[26]
Our findings have potential implications for health policymakers. That Medicaid expansion status had a neutral effect on both LOS and mortality indices in our analysis should be reassuring for states contemplating Medicaid expansion in the future. Our results also highlight the need for further efforts to reduce disparities in inpatient care based on payer status. For example, although Medicare, commercially insured, and Medicaid patients witnessed significant improvements in mortality indices pre‐ to post‐ACA implementation in hospitals in both Medicaid‐expansion and nonexpansion states, uninsured patients did not.
This study has several limitations. First, our analysis of the impact of ACA implementation on payer mix did not account for concurrent socioeconomic trends that may have influenced insurance coverage across the United States. However, the main goal of this analysis was to demonstrate that changes in payer mix did in fact occur over time, to provide rationale for our subsequent LOS and mortality analyses. Second, we could not control for variation in the design and implementation of Medicaid expansions across states as permitted under the federal Section 1115 waiver process. Third, we only had access to hospital‐level data through the UHC CDB/RM, rather than individual patient data. We attempted to mitigate this limitation by weighting data according to the number of cases per hospital. Lastly, additional patient‐level factors that may influence LOS or mortality may not be included in the UHC risk adjustment models.
In summary, the differential shift in payer mix between Medicaid‐expansion and nonexpansion states did not influence overall LOS or in‐hospital mortality for general medicine patients at AMCs in the United States. Additional research could help to determine the impact of ACA implementation on other patient outcomes that may be dependent on insurance status, such as readmissions or hospital‐acquired complications.
Disclosures: M.E.A. conceived of the study concept and design, assisted with data acquisition, and drafted the manuscript. J.J.G. assisted with study design and made critical revisions to the manuscript. D.A. assisted with study design and made critical revisions to the manuscript. R.P. assisted with study design and made critical revisions to the manuscript. M.L. assisted with study design and data acquisition and made critical revisions to the manuscript. C.D.J. assisted with study design, performed data analyses, and made critical revisions to the manuscript. A modified abstract was presented in poster format at the University HealthSystem Consortium Annual Conference held September 30 to October 2, 2015 in Orlando, Florida, as well as at the Society of Hospital Medicine Research, Innovations, and Vignettes 2016 Annual Meeting held March 69, 2016, in San Diego, California. The authors report no conflicts of interest.
- Department of Health and Human Services. Key features of the Affordable Care Act by year. Available at: http://www.hhs.gov/healthcare/facts‐and‐features/key‐features‐of‐aca‐by‐year/index.html#2014. Accessed April 4, 2016.
- Centers for Medicare and Medicaid Services. Medicaid enrollment data collected through MBES. Available at: https://www.medicaid.gov/medicaid‐chip‐program‐information/program‐information/medicaid‐and‐chip‐enrollment‐data/medicaid‐enrollment‐data‐collected‐through‐mbes.html. Accessed April 4, 2016.
- The Henry J. Kaiser Family Foundation. Status of state action on the Medicaid expansion decision. Available at: http://kff.org/health‐reform/state‐indicator/state‐activity‐around‐expanding‐medicaid‐under‐the‐affordable‐care‐act. Accessed April 4, 2016.
- Affordable Care Act Medicaid expansion reduced uninsured hospital stays in 2014. Health Aff (Millwood). 2016;35(1):106–110. , , .
- State Medicaid expansion and changes in hospital volume according to payer. N Engl J Med. 2016;374(2):196–198. , .
- Understanding predictors of prolonged hospitalizations among general medicine patients: a guide and preliminary analysis. J Hosp Med. 2015;10(9):623–626. , , , , , .
- Impact of insurance and hospital ownership on hospital length of stay among patients with ambulatory care‐sensitive conditions. Ann Fam Med. 2011;9:489–495. , , , .
- Insurance status and hospital care for myocardial infarction, stroke, and pneumonia. J Hosp Med. 2010;5:452–459. , , .
- Payment source, quality of care, and outcomes in patients hospitalized with heart failure. J Am Coll Cardiol. 2011;58(14):1465–1471. , , , , , .
- The inpatient experience and predictors of length of stay for patients hospitalized with systolic heart failure: comparison by commercial, Medicaid, and Medicare payer type. J Med Econ. 2013;16(1):43–54. , , , , .
- Insurance coverage and care of patients with non‐ST‐segment elevation acute coronary syndromes. Ann Intern Med. 2006;145:739–748. , , et al.
- Association of insurance status with inpatient treatment for coronary artery disease: findings from the Get with the Guidelines Program. Am Heart J. 2010;159:1026–1036. , , , et al.
- Primary payer status affects mortality for major surgical operations. Ann Surg. 2010;252:544–551. , , , et al.
- Medicaid payer status is associated with in‐hospital morbidity and resource utilization following primary total joint arthroplasty. J Bone Joint Surg Am. 2014;96(21):e180. , , .
- The quality of care delivered to patients within the same hospital varies by insurance type. Health Aff (Millwood). 2013;32(10):1731–1739. , , .
- Effect of insurance status on postacute care among working age stroke survivors. Neurology. 2012;78(20):1590–1595. , , , , .
- Hospitalizations in which patients leave the hospital against medical advice (AMA), 2007. HCUP statistical brief #78. August 2009. Rockville, MD: Agency for Healthcare Research and Quality; 2009. Available at: http://www.hcup‐us.ahrq.gov/reports/statbriefs/sb78.pdf. Accessed May 12, 2016. , , , .
- Characteristics of Medicaid and uninsured hospitalizations, 2012. HCUP statistical brief #182. Rockville, MD: Agency for Healthcare Research and Quality; 2014. Available at: http://www.hcup‐us.ahrq.gov/reports/statbriefs/sb182‐Medicaid‐Uninsured‐Hospitalizations‐2012.pdf. Accessed March 9, 2016. , , , .
- Agency for Healthcare Research and Quality. Mortality measurement: mortality risk adjustment methodology for University HealthSystem Consortium. Available at: http://archive.ahrq.gov/professionals/quality‐patient‐safety/quality‐resources/tools/mortality/Meurer.pdf. Accessed May 10, 2016.
- Department of Health and Human Services. Insurance expansion, hospital uncompensated care, and the Affordable Care Act. Available at: https://aspe.hhs.gov/pdf‐report/insurance‐expansion‐hospital‐uncompensated‐care‐and‐affordable‐care‐act. Accessed May 27, 2016.
- Our flawed but beneficial Medicaid program. N Engl J Med. 2011;364(16):e31. , , , .
- Changes in self‐reported insurance coverage, access to care, and health under the Affordable Care Act. JAMA. 2015;314(4):366–374. , , , .
- Early coverage, access, utilization, and health effects associated with the Affordable Care Act Medicaid Expansions: a quasi‐experimental study. Ann Intern Med. 2016;164(12):795–803. , .
- The impact of health care reform on hospital and preventive care: evidence from Massachusetts. J Public Econ. 2012;96(11–12):909–929. , .
- Mortality and access to care among adults after state Medicaid expansions. N Engl J Med. 2012;367:1025–1034. , , .
- The Oregon Experiment—effects of Medicaid on clinical outcomes. N Engl J Med. 2013;368(18):1713–1722. , , , et al.
- Department of Health and Human Services. Key features of the Affordable Care Act by year. Available at: http://www.hhs.gov/healthcare/facts‐and‐features/key‐features‐of‐aca‐by‐year/index.html#2014. Accessed April 4, 2016.
- Centers for Medicare and Medicaid Services. Medicaid enrollment data collected through MBES. Available at: https://www.medicaid.gov/medicaid‐chip‐program‐information/program‐information/medicaid‐and‐chip‐enrollment‐data/medicaid‐enrollment‐data‐collected‐through‐mbes.html. Accessed April 4, 2016.
- The Henry J. Kaiser Family Foundation. Status of state action on the Medicaid expansion decision. Available at: http://kff.org/health‐reform/state‐indicator/state‐activity‐around‐expanding‐medicaid‐under‐the‐affordable‐care‐act. Accessed April 4, 2016.
- Affordable Care Act Medicaid expansion reduced uninsured hospital stays in 2014. Health Aff (Millwood). 2016;35(1):106–110. , , .
- State Medicaid expansion and changes in hospital volume according to payer. N Engl J Med. 2016;374(2):196–198. , .
- Understanding predictors of prolonged hospitalizations among general medicine patients: a guide and preliminary analysis. J Hosp Med. 2015;10(9):623–626. , , , , , .
- Impact of insurance and hospital ownership on hospital length of stay among patients with ambulatory care‐sensitive conditions. Ann Fam Med. 2011;9:489–495. , , , .
- Insurance status and hospital care for myocardial infarction, stroke, and pneumonia. J Hosp Med. 2010;5:452–459. , , .
- Payment source, quality of care, and outcomes in patients hospitalized with heart failure. J Am Coll Cardiol. 2011;58(14):1465–1471. , , , , , .
- The inpatient experience and predictors of length of stay for patients hospitalized with systolic heart failure: comparison by commercial, Medicaid, and Medicare payer type. J Med Econ. 2013;16(1):43–54. , , , , .
- Insurance coverage and care of patients with non‐ST‐segment elevation acute coronary syndromes. Ann Intern Med. 2006;145:739–748. , , et al.
- Association of insurance status with inpatient treatment for coronary artery disease: findings from the Get with the Guidelines Program. Am Heart J. 2010;159:1026–1036. , , , et al.
- Primary payer status affects mortality for major surgical operations. Ann Surg. 2010;252:544–551. , , , et al.
- Medicaid payer status is associated with in‐hospital morbidity and resource utilization following primary total joint arthroplasty. J Bone Joint Surg Am. 2014;96(21):e180. , , .
- The quality of care delivered to patients within the same hospital varies by insurance type. Health Aff (Millwood). 2013;32(10):1731–1739. , , .
- Effect of insurance status on postacute care among working age stroke survivors. Neurology. 2012;78(20):1590–1595. , , , , .
- Hospitalizations in which patients leave the hospital against medical advice (AMA), 2007. HCUP statistical brief #78. August 2009. Rockville, MD: Agency for Healthcare Research and Quality; 2009. Available at: http://www.hcup‐us.ahrq.gov/reports/statbriefs/sb78.pdf. Accessed May 12, 2016. , , , .
- Characteristics of Medicaid and uninsured hospitalizations, 2012. HCUP statistical brief #182. Rockville, MD: Agency for Healthcare Research and Quality; 2014. Available at: http://www.hcup‐us.ahrq.gov/reports/statbriefs/sb182‐Medicaid‐Uninsured‐Hospitalizations‐2012.pdf. Accessed March 9, 2016. , , , .
- Agency for Healthcare Research and Quality. Mortality measurement: mortality risk adjustment methodology for University HealthSystem Consortium. Available at: http://archive.ahrq.gov/professionals/quality‐patient‐safety/quality‐resources/tools/mortality/Meurer.pdf. Accessed May 10, 2016.
- Department of Health and Human Services. Insurance expansion, hospital uncompensated care, and the Affordable Care Act. Available at: https://aspe.hhs.gov/pdf‐report/insurance‐expansion‐hospital‐uncompensated‐care‐and‐affordable‐care‐act. Accessed May 27, 2016.
- Our flawed but beneficial Medicaid program. N Engl J Med. 2011;364(16):e31. , , , .
- Changes in self‐reported insurance coverage, access to care, and health under the Affordable Care Act. JAMA. 2015;314(4):366–374. , , , .
- Early coverage, access, utilization, and health effects associated with the Affordable Care Act Medicaid Expansions: a quasi‐experimental study. Ann Intern Med. 2016;164(12):795–803. , .
- The impact of health care reform on hospital and preventive care: evidence from Massachusetts. J Public Econ. 2012;96(11–12):909–929. , .
- Mortality and access to care among adults after state Medicaid expansions. N Engl J Med. 2012;367:1025–1034. , , .
- The Oregon Experiment—effects of Medicaid on clinical outcomes. N Engl J Med. 2013;368(18):1713–1722. , , , et al.
Visiting Professor in Hospital Medicine
Hospital medicine is an emerging specialty comprised predominantly of early‐career faculty, often less than 5 years postresidency and predominately at instructor or assistant professor level.[1] Effective mentoring has been identified as a critical component of academic success.[2, 3] Published data suggest that most academic hospitalists do not have a mentor, and when they do, the majority of them spend less than 4 hours per year with their mentor.[2] The reasons for this are multifactorial but largely result from the lack of structure, opportunities, and local senior academic hospitalists.[1, 4] Early‐career faculty have difficulty establishing external mentoring relationships, and new models beyond the traditional intrainstitutional dyad are needed.[3, 4] The need for mentors and structured mentorship networks may be particularly high in hospital medicine.[5]
The Visiting Professorship in Hospital Medicine Program was designed to promote cross‐institutional mentorship, share hospitalist innovations, and facilitate academic collaboration between hospitalist groups. We describe the design and early experience with this program across 5 academic hospital medicine programs.
PROGRAM DESIGN
Objectives
The program was designed to promote mentoring relationships between early‐career hospitalist faculty and a visiting professor from another academic hospital medicine group. The program sought to provide immediate career advice during the visits, but also create opportunities for long‐term mentorship and collaboration between institutions. Goals for each visiting professorship included: (1) follow‐up contact between early‐career faculty and visiting professor in the 6 months following the visit, (2) long‐term mentoring relationship with at least 1 early‐career faculty at the visited institution, and (3) identification of opportunities for interinstitutional collaboration to disseminate innovations.
Selection of Sites and Faculty
The first 2 academic medical centers (AMCs) for the visiting professorship exchange designed the program (University of Colorado and University of New Mexico). In subsequent years, each participating AMC was able to solicit additional sites for faculty exchange. This model can expand without requiring ongoing central direction. No criteria were set for selection of AMCs. Visiting professors in hospital medicine were explicitly targeted to be at midcareer stage of late assistant professor or early associate professor and within 1 to 2 years of promotion. It was felt that this group would gain the maximal career benefit from delivering an invited visit to an external AMC, yet have a sufficient track record to deliver effective mentoring advice to early‐career hospitalists. The hospitalist group sending the visiting professor would propose a few candidates, with the innovations they would be able to present, and the hosting site would select 1 for the visit. Early‐career faculty at the hosting institution were generally instructor to early assistant professors.
Visit Itinerary
The visit itinerary was set up as follows:
- Visiting professor delivers a formal 1‐hour presentation to hospitalist faculty, describing an innovation in clinical care, quality improvement, patient safety, or education.
- Individual meetings with 3 to 5 early‐career hospitalists to review faculty portfolios and provide career advice.
- Group lunch between the visiting professor and faculty with similar interests to promote cross‐institutional networking and spark potential collaborations.
- Meeting with hospital medicine program leadership.
- Visiting professor receives exposure to an innovation developed at the hosting institution.
- Dinner with the hosting faculty including the senior hospitalist coordinating the visit.
In advance of the visit, both early‐career faculty and visiting professors receive written materials describing the program, its objectives, and tips to prepare for the visit (see Supporting Information in the online version of this article). The curricula vitae of early‐career faculty at the hosting institution were provided to the visiting professor. Visit costs were covered by the visiting professor's institution. Honoraria were not offered.
Program Evaluation
Within a month of each visit, a paper survey was administered to the visiting professor and the faculty with whom she/he met. In addition to demographic data including gender, self‐reported minority status, academic rank, years at rank, and total years in academic medicine, the survey asked faculty to rate on a 5‐point Likert scale their assessment of the usefulness of the visit to accomplish the 4 core goals of the program: (1) cross‐institutional dissemination of innovations in clinical medicine, education, or research; (2) advancing the respondent's academic career; (3) fostering cross‐institutional mentor‐mentee relationships; and (4) promoting cross‐institutional collaborations. Free‐text responses for overall impression of program and suggestions for improvement were solicited.
At the time of this writing, 1 year has passed from the initial visits for the first 3 visiting professorships. A 1‐year follow‐up survey was administered assessing (1) total number of contacts with the visiting professor in the year following the visit, (2) whether a letter of recommendation resulted from the visit, (3) whether the respondent had seen evidence of spread of innovative ideas as a result of the program, (4) participation in a cross‐institutional collaboration as a result of the program, and (5) assessment of benefit in continuing the program in the next year. The respondents were also asked to rate the global utility of the program to their professional development on a 5‐point scale ranging from not at all useful to very useful (Thinking about what has happened to you since the visit a year ago, please rate the usefulness of the entire program to your professional life: overall usefulness for my professional development.). Domain‐specific utility in improving clinical, research, quality improvement, and administrative skills were also elicited (results not shown). Finally, suggestions to improve the program for the future were solicited. The Colorado Multiple Institutional Review Board determined that the study of this faculty development program did not qualify as human subjects research, and subjects were therefore not asked to provide informed consent for participation.
RESULTS
To date, 5 academic medical centers have participated in the visiting professorship program, with 7 visiting professors interacting with 29 early‐career faculty. Of the 29 early‐career faculty, 72% (21/29) were at the rank of assistant professor, 17% (5/29) instructor, 7% (2/29) residents with plans to hire, and 3% (1/29) associate professor. The median was 2 years in academic medicine and 1 year at current academic rank. Forty‐one percent (12/29) were women and 7% (2/29) identified as ethnic minority. Of the 7 visiting professors, 57% (4/7) were assistant professor and 43% (3/7) were associate professors. The median was 5 years in academic medicine, 29% (2/7) were women, and none identified as ethnic minority.
Immediate postvisit survey response was obtained for all participating faculty. In the immediate postvisit survey, on a 5‐point Likert scale, the 29 early‐career faculty rated the visit: 4.4 for promoting cross‐institutional dissemination of innovations, 4.2 for advancing my academic career, 4.2 for fostering cross‐institutional mentor‐mentee relationships, and 4.4 for promoting cross‐institutional collaborations. Ninety‐three percent (26/28 accounting for 1 nonresponse to this question) reported the visiting professorship had high potential to disseminate innovation (rated greater than 3 on the 5‐point Likert score). Eighty‐three percent (24/29) of the early‐career faculty rated the visit highly useful in advancing their career, 76% (22/29) responded that the visit was highly likely to foster external mentorship relationships, and 90% (26/29) reported the visit highly effective in promoting cross‐institutional collaborations. In the immediate postvisit survey, the 7 visiting professors rated the visit 4.9 for promoting cross‐institutional dissemination of innovations, 4.3 for advancing my academic career, 4.0 for fostering cross‐institutional mentor‐mentee relationships, and 4.3 for promoting cross‐institutional collaborations.
Free‐text comments from both visiting professors and early‐career faculty were generally favorable (Table 1). Some comments offered constructive input on appropriate matching of faculty, previsit preparation, or desire for more time in sessions (Table 1).
Visiting Professors (n = 7) | Early‐Career Faculty (n = 29) |
---|---|
I was very impressed with the degree of organization, preparation, and structure from [host institution]. The project is a great concept and may well lead to similar and even more developed ones in the future. It is very helpful to get the pulse on another program and to hear of some of the same struggles and successes of another hospitalist program. The potential for cross‐site mentor‐mentee relationships and collaborations is a win‐win for both programs. | I really enjoyed my individual meeting with [visiting professor]. She was helpful in reviewing current projects from another perspective and very helpful in making suggestions for future projects. Also enjoyed her Grand Rounds and plan to follow‐up on this issue for possible cross‐institutional collaboration. |
Overall, this exchange is a great program. It is fun, promotes idea exchange, and is immensely helpful to the visiting professor for promotion. Every meeting I had with faculty at [host institution] was interesting and worthwhile. The primary challenge is maintaining mentorship ties and momentum after the visit. I personally e‐mailed every person I met and received many responses, including several explicit requests for ongoing advising and collaboration. | I think this is a great program. It definitely gives us the opportunity to meet people outside of the [host institution] community and foster relationships, mentorship, and possible collaborations with projects and programs. |
I liked multidisciplinary rounding. Research club. Meeting with faculty and trying to find common areas of interest. | I think this is a fantastic program so far. [Visiting professor] was very energetic and interested in making the most of the day. She contacted me after the visit and offered to keep in touch in the future. Right now I can see the program as being most useful in establishing new mentor/mentee relationships. |
Most of the faculty I met with see value in being involved in systems/quality improvement, but most do not express interest in specific projects. Areas needing improvement were identified by everyone I met with so developing projects around these areas should be doable. They might benefit from access to mentoring in quality improvement. | It was fantastic to meet with [visiting professor] and get a sense for his work and also brainstorm about how we might do similar work here in the future (eg, in high‐value care). It was also great to then see him 2 days later at [national conference]. I feel this is a great program to improve our connections cross‐institutionally and hopefully to spark some future collaborations. |
Very worthwhile. Was really helpful to meet with various faculty and leadership to see similarities and differences between our institutions. Generated several ideas for collaborative activities already. Also really helpful to have a somewhat structured way to share my work at an outside institution, as well as to create opportunities for mentor‐mentee relationships outside my home institution. | Incredibly valuable to promote this kind of cross‐pollination for both collaboration and innovation. |
Wonderful, inspiring, professionally advantageous. | |
Good idea. Good way to help midcareer faculty with advancement. Offers promise for collaboration of research/workshops. | |
Suggestions for Improvement | |
Please have e‐mails of the folks we meet available immediately after the visit. It is hard to know if anyone felt enough of a connection to want mentorship from me. | I feel like I may be a bit early on to benefit as much as I could have. |
Develop a mentorship program for quality improvement. As part of this exchange, consider treating visits as similar to a consultation. Have visitor with specific focus that they can offer help with. | Nice to have personal access to accomplished faculty from other institutions. Their perspective and career trajectory don't always align due to differences in institution culture, specifics of promotion process, and so on, but still a useful experience. |
Share any possible more‐formal topics for discussion with leadership prior to the visit so can prepare ahead of time (eg, gather information they may have questions on). Otherwise it was great! | For early career faculty, more discussions prior in regard to what to expect. |
A question is who should continue to push? Is it the prospective mentee, the mentee's institution, an so on? | Great idea. Would have loved to be involved in more aspects. More time for discussion would have been good. Did not get to discuss collaboration in person. |
Great to get to talk to someone from totally different system. Wish we had more time to talk. |
One‐year follow‐up was obtained for all but 1 early‐career faculty member receiving the follow‐up survey, and all 3 visiting professors. Of the 3 visiting professorships that occurred more than 1 year ago, 16 mentorship contacts occurred in total (phone, e‐mail, or in person) between 13 early‐career faculty and 3 visiting professors in the year after the initial visits (range, 04 contacts). Follow‐up contact occurred for 3 of 4 early‐career faculty from the first visiting professorship, 3 of 5 from the second visiting professorship, and 2 of 4 from the third visiting professorship. One early‐career faculty member from each host academic medical center had 3 or more additional contacts with the visiting professor in the year following the initial visit. Overall, 8/13 (62%) of early‐career faculty had at least 1 follow‐up mentoring discussion. On 1‐year follow‐up, overall utility for professional development was rated an average of 3.5 by early‐career faculty (with a trend of higher ratings of efficacy with increasing number of follow‐up contacts) and 4.7 by visiting professors. Half (8/16) of the involved faculty report having seen evidence of cross‐institutional dissemination of innovation. Ninety‐four percent (15/16) of participants at 1‐year follow‐up felt there was benefit to their institution in continuing the program for the next year.
Objective evidence of cross‐institutional scholarship, assessed by email query of both visiting professors and senior hospitalists coordinating the visits, includes 2 collaborative peer reviewed publications including mentors and mentees participating in the visiting professorship.[6, 7] Joint educational curriculum development on high‐value care between sites is planned. The Visiting Professorship in Hospital Medicine Program has resulted in 1 external letter to support a visiting professor's promotion to date.
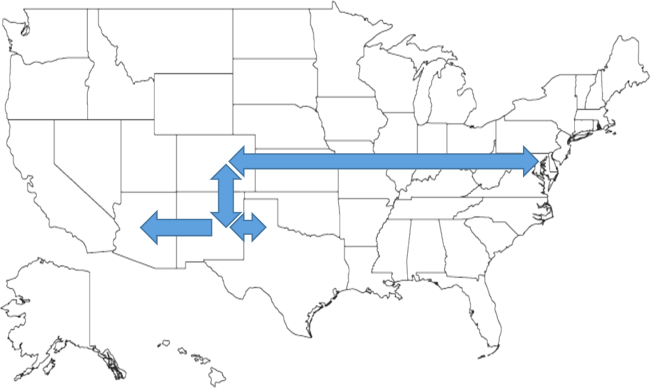
DISCUSSION
Hospital Medicine is a young, rapidly growing field, hence the number of experienced academic hospitalist mentors with expertise in successfully navigating an academic career is limited. A national study of hospitalist leaders found that 75% of clinician‐educators and 58% of research faculty feel that lack of mentorship is a major issue.[1] Mentorship for hospitalist clinician‐investigators is often delivered by nonhospitalists.[2, 8] There is little evidence of external mentorship for academic clinician‐educators in hospital medicine.[1] Without explicit programmatic support, many faculty may find this to be a barrier to career advancement. A study of successfully promoted hospitalists identified difficulty identifying external senior hospitalists to write letters in support of promotion as an obstacle.[9] Our study of the Visiting Professorship in Hospital Medicine Program found that early‐career faculty rated the visit as useful in advancing their career and fostered external mentorship relationships. Subsequent experience suggests more than half of the early‐career faculty will maintain contact with the visiting professor over the year following the visit. Visiting professors rate the experience particularly highly in their own career advancement.
The hospitalist movement is built on a foundation of innovation. The focus of each presentation was on an innovation developed by the visiting professor, and each visit showcased an innovation of the visited institution. This is distinct from traditional Hospital Grand Rounds, which more often focus on basic science research or clinical pathophysiology/disease management based on subspecialty topics.[10] The Visiting Professorship in Hospital Medicine Program was judged by participants to be an effective means of spreading innovation.
Insights from experience with the Visiting Professorship in Hospital Medicine Program include the importance of preliminary work prior to each visit. Program directors need to attend closely to the fit between the interests and career path of the visiting professor and those of the early‐career faculty. The innovations being shared should be aligned with organizational interests to maximize the chance of subsequent spread of the innovation and future collaboration. Providing faculty information about the objectives of the program in advance of the visit and arranging an exchange of curricula vitae between the early‐career faculty and the visiting professor allows participants to prepare for the in‐person coaching. Based on comments from participants, prompting contact from the visiting professor after the visit may be helpful to initiate the longitudinal relationship. We also found that early‐career faculty may not be aware of how to effectively use a mentoring relationship with an external faculty member. Training sessions for both mentors and mentees on effective mentorship relationships before visiting professorships might improve early‐career faculty confidence in initiating relationships and maximize value from mentor coaching.
A key issue is finding the right level of career maturity for the visiting professor. Our approach in selecting visiting professors was congruent with utilization of midcareer peer coaches employed by intrainstitutional hospital medicine mentoring programs.[11] The visiting professor should have sufficient experience and accomplishments to be able to effectively counsel junior faculty. However, it is important that the visiting professor also has sufficient time and interest to take on additional mentees and to be a full participant in shared scholarship projects emerging from the experience.
This study represents the experience of 5 mature academic hospitalist groups, and results may not be generalizable to dissimilar institutions or if only the most senior faculty are selected to perform visits. There is an inherent selection bias in the choice of both visiting professor and early‐career faculty. The small sample size of the faculty exposed to this program is a limitation to generalizability of the results of this evaluation. Whether this program will result in greater success in promotion of academic hospitalists cannot be assessed based on the follow‐up available. The Visiting Professorship in Hospital Medicine Program has continued to be sustained with an additional academic medical center enrolled and 2 additional site visits planned. The costs of the program are low, largely air travel and a night of lodging, as well as nominal administrative logistical support. Perceived benefits by participants and academic medical centers make this modest investment worth considering for academic hospitalist groups.
CONCLUSIONS
The Visiting Professorship in Hospital Medicine Program offers structure, opportunities, and access to senior mentors to advance the development of early‐career hospitalists while spreading innovation to distant sites. It is assessed by participants to facilitate external mentoring relationships and has the potential to advance the careers of both early‐career faculty as well as the visiting professors.
Disclosure
Nothing to report.
- Survey of US academic hospitalist leaders about mentorship and academic activities in hospitalist groups. J Hosp Med. 2011;6:5–9. , , , .
- Mentorship, productivity, and promotion among academic hospitalists. J Gen Intern Med. 2012;27(1):23–27. , , , , , .
- Mentoring faculty in academic medicine: a new paradigm? J Gen Intern Med. 2005;20(9):866–870. , .
- Challenges and opportunities in academic hospital medicine: report from the Academic Hospital Medicine Summit. J Hosp Med. 2009;4:240–246. , , , , , .
- The need for mentors in the odyssey of the academic hospitalist. J Hosp Med. 2011;6:1–2. , .
- Procedural skills for hospitalists. Hosp Med Clin. 2016;5:114–136. , , , .
- Cedecea davisae' s role in a polymicrobial lung infection in a cystic fibrosis patient. Case reports in infectious diseases. Case Rep Infect Dis. 2012;2012:176864. , , .
- Innovative approach to supporting hospitalist physicians towards academic success. J Hosp Med. 2008;3:314–318. , , , .
- Tried and true: a survey of successfully promoted academic hospitalists. J Hosp Med. 2011;6:411–415. , , , , .
- A case study of medical grand rounds: are we using effective methods? Acad Med. 2009;84(8):1144–1151. , , , , .
- Investing in the future: building an academic hospitalist faculty development program. J Hosp Med. 2011;6:161–166. , , , .
Hospital medicine is an emerging specialty comprised predominantly of early‐career faculty, often less than 5 years postresidency and predominately at instructor or assistant professor level.[1] Effective mentoring has been identified as a critical component of academic success.[2, 3] Published data suggest that most academic hospitalists do not have a mentor, and when they do, the majority of them spend less than 4 hours per year with their mentor.[2] The reasons for this are multifactorial but largely result from the lack of structure, opportunities, and local senior academic hospitalists.[1, 4] Early‐career faculty have difficulty establishing external mentoring relationships, and new models beyond the traditional intrainstitutional dyad are needed.[3, 4] The need for mentors and structured mentorship networks may be particularly high in hospital medicine.[5]
The Visiting Professorship in Hospital Medicine Program was designed to promote cross‐institutional mentorship, share hospitalist innovations, and facilitate academic collaboration between hospitalist groups. We describe the design and early experience with this program across 5 academic hospital medicine programs.
PROGRAM DESIGN
Objectives
The program was designed to promote mentoring relationships between early‐career hospitalist faculty and a visiting professor from another academic hospital medicine group. The program sought to provide immediate career advice during the visits, but also create opportunities for long‐term mentorship and collaboration between institutions. Goals for each visiting professorship included: (1) follow‐up contact between early‐career faculty and visiting professor in the 6 months following the visit, (2) long‐term mentoring relationship with at least 1 early‐career faculty at the visited institution, and (3) identification of opportunities for interinstitutional collaboration to disseminate innovations.
Selection of Sites and Faculty
The first 2 academic medical centers (AMCs) for the visiting professorship exchange designed the program (University of Colorado and University of New Mexico). In subsequent years, each participating AMC was able to solicit additional sites for faculty exchange. This model can expand without requiring ongoing central direction. No criteria were set for selection of AMCs. Visiting professors in hospital medicine were explicitly targeted to be at midcareer stage of late assistant professor or early associate professor and within 1 to 2 years of promotion. It was felt that this group would gain the maximal career benefit from delivering an invited visit to an external AMC, yet have a sufficient track record to deliver effective mentoring advice to early‐career hospitalists. The hospitalist group sending the visiting professor would propose a few candidates, with the innovations they would be able to present, and the hosting site would select 1 for the visit. Early‐career faculty at the hosting institution were generally instructor to early assistant professors.
Visit Itinerary
The visit itinerary was set up as follows:
- Visiting professor delivers a formal 1‐hour presentation to hospitalist faculty, describing an innovation in clinical care, quality improvement, patient safety, or education.
- Individual meetings with 3 to 5 early‐career hospitalists to review faculty portfolios and provide career advice.
- Group lunch between the visiting professor and faculty with similar interests to promote cross‐institutional networking and spark potential collaborations.
- Meeting with hospital medicine program leadership.
- Visiting professor receives exposure to an innovation developed at the hosting institution.
- Dinner with the hosting faculty including the senior hospitalist coordinating the visit.
In advance of the visit, both early‐career faculty and visiting professors receive written materials describing the program, its objectives, and tips to prepare for the visit (see Supporting Information in the online version of this article). The curricula vitae of early‐career faculty at the hosting institution were provided to the visiting professor. Visit costs were covered by the visiting professor's institution. Honoraria were not offered.
Program Evaluation
Within a month of each visit, a paper survey was administered to the visiting professor and the faculty with whom she/he met. In addition to demographic data including gender, self‐reported minority status, academic rank, years at rank, and total years in academic medicine, the survey asked faculty to rate on a 5‐point Likert scale their assessment of the usefulness of the visit to accomplish the 4 core goals of the program: (1) cross‐institutional dissemination of innovations in clinical medicine, education, or research; (2) advancing the respondent's academic career; (3) fostering cross‐institutional mentor‐mentee relationships; and (4) promoting cross‐institutional collaborations. Free‐text responses for overall impression of program and suggestions for improvement were solicited.
At the time of this writing, 1 year has passed from the initial visits for the first 3 visiting professorships. A 1‐year follow‐up survey was administered assessing (1) total number of contacts with the visiting professor in the year following the visit, (2) whether a letter of recommendation resulted from the visit, (3) whether the respondent had seen evidence of spread of innovative ideas as a result of the program, (4) participation in a cross‐institutional collaboration as a result of the program, and (5) assessment of benefit in continuing the program in the next year. The respondents were also asked to rate the global utility of the program to their professional development on a 5‐point scale ranging from not at all useful to very useful (Thinking about what has happened to you since the visit a year ago, please rate the usefulness of the entire program to your professional life: overall usefulness for my professional development.). Domain‐specific utility in improving clinical, research, quality improvement, and administrative skills were also elicited (results not shown). Finally, suggestions to improve the program for the future were solicited. The Colorado Multiple Institutional Review Board determined that the study of this faculty development program did not qualify as human subjects research, and subjects were therefore not asked to provide informed consent for participation.
RESULTS
To date, 5 academic medical centers have participated in the visiting professorship program, with 7 visiting professors interacting with 29 early‐career faculty. Of the 29 early‐career faculty, 72% (21/29) were at the rank of assistant professor, 17% (5/29) instructor, 7% (2/29) residents with plans to hire, and 3% (1/29) associate professor. The median was 2 years in academic medicine and 1 year at current academic rank. Forty‐one percent (12/29) were women and 7% (2/29) identified as ethnic minority. Of the 7 visiting professors, 57% (4/7) were assistant professor and 43% (3/7) were associate professors. The median was 5 years in academic medicine, 29% (2/7) were women, and none identified as ethnic minority.
Immediate postvisit survey response was obtained for all participating faculty. In the immediate postvisit survey, on a 5‐point Likert scale, the 29 early‐career faculty rated the visit: 4.4 for promoting cross‐institutional dissemination of innovations, 4.2 for advancing my academic career, 4.2 for fostering cross‐institutional mentor‐mentee relationships, and 4.4 for promoting cross‐institutional collaborations. Ninety‐three percent (26/28 accounting for 1 nonresponse to this question) reported the visiting professorship had high potential to disseminate innovation (rated greater than 3 on the 5‐point Likert score). Eighty‐three percent (24/29) of the early‐career faculty rated the visit highly useful in advancing their career, 76% (22/29) responded that the visit was highly likely to foster external mentorship relationships, and 90% (26/29) reported the visit highly effective in promoting cross‐institutional collaborations. In the immediate postvisit survey, the 7 visiting professors rated the visit 4.9 for promoting cross‐institutional dissemination of innovations, 4.3 for advancing my academic career, 4.0 for fostering cross‐institutional mentor‐mentee relationships, and 4.3 for promoting cross‐institutional collaborations.
Free‐text comments from both visiting professors and early‐career faculty were generally favorable (Table 1). Some comments offered constructive input on appropriate matching of faculty, previsit preparation, or desire for more time in sessions (Table 1).
Visiting Professors (n = 7) | Early‐Career Faculty (n = 29) |
---|---|
I was very impressed with the degree of organization, preparation, and structure from [host institution]. The project is a great concept and may well lead to similar and even more developed ones in the future. It is very helpful to get the pulse on another program and to hear of some of the same struggles and successes of another hospitalist program. The potential for cross‐site mentor‐mentee relationships and collaborations is a win‐win for both programs. | I really enjoyed my individual meeting with [visiting professor]. She was helpful in reviewing current projects from another perspective and very helpful in making suggestions for future projects. Also enjoyed her Grand Rounds and plan to follow‐up on this issue for possible cross‐institutional collaboration. |
Overall, this exchange is a great program. It is fun, promotes idea exchange, and is immensely helpful to the visiting professor for promotion. Every meeting I had with faculty at [host institution] was interesting and worthwhile. The primary challenge is maintaining mentorship ties and momentum after the visit. I personally e‐mailed every person I met and received many responses, including several explicit requests for ongoing advising and collaboration. | I think this is a great program. It definitely gives us the opportunity to meet people outside of the [host institution] community and foster relationships, mentorship, and possible collaborations with projects and programs. |
I liked multidisciplinary rounding. Research club. Meeting with faculty and trying to find common areas of interest. | I think this is a fantastic program so far. [Visiting professor] was very energetic and interested in making the most of the day. She contacted me after the visit and offered to keep in touch in the future. Right now I can see the program as being most useful in establishing new mentor/mentee relationships. |
Most of the faculty I met with see value in being involved in systems/quality improvement, but most do not express interest in specific projects. Areas needing improvement were identified by everyone I met with so developing projects around these areas should be doable. They might benefit from access to mentoring in quality improvement. | It was fantastic to meet with [visiting professor] and get a sense for his work and also brainstorm about how we might do similar work here in the future (eg, in high‐value care). It was also great to then see him 2 days later at [national conference]. I feel this is a great program to improve our connections cross‐institutionally and hopefully to spark some future collaborations. |
Very worthwhile. Was really helpful to meet with various faculty and leadership to see similarities and differences between our institutions. Generated several ideas for collaborative activities already. Also really helpful to have a somewhat structured way to share my work at an outside institution, as well as to create opportunities for mentor‐mentee relationships outside my home institution. | Incredibly valuable to promote this kind of cross‐pollination for both collaboration and innovation. |
Wonderful, inspiring, professionally advantageous. | |
Good idea. Good way to help midcareer faculty with advancement. Offers promise for collaboration of research/workshops. | |
Suggestions for Improvement | |
Please have e‐mails of the folks we meet available immediately after the visit. It is hard to know if anyone felt enough of a connection to want mentorship from me. | I feel like I may be a bit early on to benefit as much as I could have. |
Develop a mentorship program for quality improvement. As part of this exchange, consider treating visits as similar to a consultation. Have visitor with specific focus that they can offer help with. | Nice to have personal access to accomplished faculty from other institutions. Their perspective and career trajectory don't always align due to differences in institution culture, specifics of promotion process, and so on, but still a useful experience. |
Share any possible more‐formal topics for discussion with leadership prior to the visit so can prepare ahead of time (eg, gather information they may have questions on). Otherwise it was great! | For early career faculty, more discussions prior in regard to what to expect. |
A question is who should continue to push? Is it the prospective mentee, the mentee's institution, an so on? | Great idea. Would have loved to be involved in more aspects. More time for discussion would have been good. Did not get to discuss collaboration in person. |
Great to get to talk to someone from totally different system. Wish we had more time to talk. |
One‐year follow‐up was obtained for all but 1 early‐career faculty member receiving the follow‐up survey, and all 3 visiting professors. Of the 3 visiting professorships that occurred more than 1 year ago, 16 mentorship contacts occurred in total (phone, e‐mail, or in person) between 13 early‐career faculty and 3 visiting professors in the year after the initial visits (range, 04 contacts). Follow‐up contact occurred for 3 of 4 early‐career faculty from the first visiting professorship, 3 of 5 from the second visiting professorship, and 2 of 4 from the third visiting professorship. One early‐career faculty member from each host academic medical center had 3 or more additional contacts with the visiting professor in the year following the initial visit. Overall, 8/13 (62%) of early‐career faculty had at least 1 follow‐up mentoring discussion. On 1‐year follow‐up, overall utility for professional development was rated an average of 3.5 by early‐career faculty (with a trend of higher ratings of efficacy with increasing number of follow‐up contacts) and 4.7 by visiting professors. Half (8/16) of the involved faculty report having seen evidence of cross‐institutional dissemination of innovation. Ninety‐four percent (15/16) of participants at 1‐year follow‐up felt there was benefit to their institution in continuing the program for the next year.
Objective evidence of cross‐institutional scholarship, assessed by email query of both visiting professors and senior hospitalists coordinating the visits, includes 2 collaborative peer reviewed publications including mentors and mentees participating in the visiting professorship.[6, 7] Joint educational curriculum development on high‐value care between sites is planned. The Visiting Professorship in Hospital Medicine Program has resulted in 1 external letter to support a visiting professor's promotion to date.
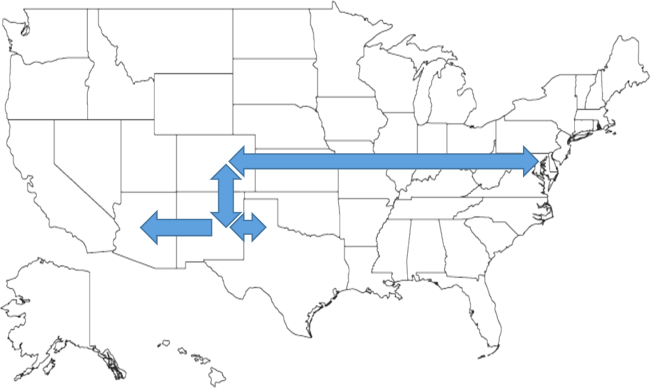
DISCUSSION
Hospital Medicine is a young, rapidly growing field, hence the number of experienced academic hospitalist mentors with expertise in successfully navigating an academic career is limited. A national study of hospitalist leaders found that 75% of clinician‐educators and 58% of research faculty feel that lack of mentorship is a major issue.[1] Mentorship for hospitalist clinician‐investigators is often delivered by nonhospitalists.[2, 8] There is little evidence of external mentorship for academic clinician‐educators in hospital medicine.[1] Without explicit programmatic support, many faculty may find this to be a barrier to career advancement. A study of successfully promoted hospitalists identified difficulty identifying external senior hospitalists to write letters in support of promotion as an obstacle.[9] Our study of the Visiting Professorship in Hospital Medicine Program found that early‐career faculty rated the visit as useful in advancing their career and fostered external mentorship relationships. Subsequent experience suggests more than half of the early‐career faculty will maintain contact with the visiting professor over the year following the visit. Visiting professors rate the experience particularly highly in their own career advancement.
The hospitalist movement is built on a foundation of innovation. The focus of each presentation was on an innovation developed by the visiting professor, and each visit showcased an innovation of the visited institution. This is distinct from traditional Hospital Grand Rounds, which more often focus on basic science research or clinical pathophysiology/disease management based on subspecialty topics.[10] The Visiting Professorship in Hospital Medicine Program was judged by participants to be an effective means of spreading innovation.
Insights from experience with the Visiting Professorship in Hospital Medicine Program include the importance of preliminary work prior to each visit. Program directors need to attend closely to the fit between the interests and career path of the visiting professor and those of the early‐career faculty. The innovations being shared should be aligned with organizational interests to maximize the chance of subsequent spread of the innovation and future collaboration. Providing faculty information about the objectives of the program in advance of the visit and arranging an exchange of curricula vitae between the early‐career faculty and the visiting professor allows participants to prepare for the in‐person coaching. Based on comments from participants, prompting contact from the visiting professor after the visit may be helpful to initiate the longitudinal relationship. We also found that early‐career faculty may not be aware of how to effectively use a mentoring relationship with an external faculty member. Training sessions for both mentors and mentees on effective mentorship relationships before visiting professorships might improve early‐career faculty confidence in initiating relationships and maximize value from mentor coaching.
A key issue is finding the right level of career maturity for the visiting professor. Our approach in selecting visiting professors was congruent with utilization of midcareer peer coaches employed by intrainstitutional hospital medicine mentoring programs.[11] The visiting professor should have sufficient experience and accomplishments to be able to effectively counsel junior faculty. However, it is important that the visiting professor also has sufficient time and interest to take on additional mentees and to be a full participant in shared scholarship projects emerging from the experience.
This study represents the experience of 5 mature academic hospitalist groups, and results may not be generalizable to dissimilar institutions or if only the most senior faculty are selected to perform visits. There is an inherent selection bias in the choice of both visiting professor and early‐career faculty. The small sample size of the faculty exposed to this program is a limitation to generalizability of the results of this evaluation. Whether this program will result in greater success in promotion of academic hospitalists cannot be assessed based on the follow‐up available. The Visiting Professorship in Hospital Medicine Program has continued to be sustained with an additional academic medical center enrolled and 2 additional site visits planned. The costs of the program are low, largely air travel and a night of lodging, as well as nominal administrative logistical support. Perceived benefits by participants and academic medical centers make this modest investment worth considering for academic hospitalist groups.
CONCLUSIONS
The Visiting Professorship in Hospital Medicine Program offers structure, opportunities, and access to senior mentors to advance the development of early‐career hospitalists while spreading innovation to distant sites. It is assessed by participants to facilitate external mentoring relationships and has the potential to advance the careers of both early‐career faculty as well as the visiting professors.
Disclosure
Nothing to report.
Hospital medicine is an emerging specialty comprised predominantly of early‐career faculty, often less than 5 years postresidency and predominately at instructor or assistant professor level.[1] Effective mentoring has been identified as a critical component of academic success.[2, 3] Published data suggest that most academic hospitalists do not have a mentor, and when they do, the majority of them spend less than 4 hours per year with their mentor.[2] The reasons for this are multifactorial but largely result from the lack of structure, opportunities, and local senior academic hospitalists.[1, 4] Early‐career faculty have difficulty establishing external mentoring relationships, and new models beyond the traditional intrainstitutional dyad are needed.[3, 4] The need for mentors and structured mentorship networks may be particularly high in hospital medicine.[5]
The Visiting Professorship in Hospital Medicine Program was designed to promote cross‐institutional mentorship, share hospitalist innovations, and facilitate academic collaboration between hospitalist groups. We describe the design and early experience with this program across 5 academic hospital medicine programs.
PROGRAM DESIGN
Objectives
The program was designed to promote mentoring relationships between early‐career hospitalist faculty and a visiting professor from another academic hospital medicine group. The program sought to provide immediate career advice during the visits, but also create opportunities for long‐term mentorship and collaboration between institutions. Goals for each visiting professorship included: (1) follow‐up contact between early‐career faculty and visiting professor in the 6 months following the visit, (2) long‐term mentoring relationship with at least 1 early‐career faculty at the visited institution, and (3) identification of opportunities for interinstitutional collaboration to disseminate innovations.
Selection of Sites and Faculty
The first 2 academic medical centers (AMCs) for the visiting professorship exchange designed the program (University of Colorado and University of New Mexico). In subsequent years, each participating AMC was able to solicit additional sites for faculty exchange. This model can expand without requiring ongoing central direction. No criteria were set for selection of AMCs. Visiting professors in hospital medicine were explicitly targeted to be at midcareer stage of late assistant professor or early associate professor and within 1 to 2 years of promotion. It was felt that this group would gain the maximal career benefit from delivering an invited visit to an external AMC, yet have a sufficient track record to deliver effective mentoring advice to early‐career hospitalists. The hospitalist group sending the visiting professor would propose a few candidates, with the innovations they would be able to present, and the hosting site would select 1 for the visit. Early‐career faculty at the hosting institution were generally instructor to early assistant professors.
Visit Itinerary
The visit itinerary was set up as follows:
- Visiting professor delivers a formal 1‐hour presentation to hospitalist faculty, describing an innovation in clinical care, quality improvement, patient safety, or education.
- Individual meetings with 3 to 5 early‐career hospitalists to review faculty portfolios and provide career advice.
- Group lunch between the visiting professor and faculty with similar interests to promote cross‐institutional networking and spark potential collaborations.
- Meeting with hospital medicine program leadership.
- Visiting professor receives exposure to an innovation developed at the hosting institution.
- Dinner with the hosting faculty including the senior hospitalist coordinating the visit.
In advance of the visit, both early‐career faculty and visiting professors receive written materials describing the program, its objectives, and tips to prepare for the visit (see Supporting Information in the online version of this article). The curricula vitae of early‐career faculty at the hosting institution were provided to the visiting professor. Visit costs were covered by the visiting professor's institution. Honoraria were not offered.
Program Evaluation
Within a month of each visit, a paper survey was administered to the visiting professor and the faculty with whom she/he met. In addition to demographic data including gender, self‐reported minority status, academic rank, years at rank, and total years in academic medicine, the survey asked faculty to rate on a 5‐point Likert scale their assessment of the usefulness of the visit to accomplish the 4 core goals of the program: (1) cross‐institutional dissemination of innovations in clinical medicine, education, or research; (2) advancing the respondent's academic career; (3) fostering cross‐institutional mentor‐mentee relationships; and (4) promoting cross‐institutional collaborations. Free‐text responses for overall impression of program and suggestions for improvement were solicited.
At the time of this writing, 1 year has passed from the initial visits for the first 3 visiting professorships. A 1‐year follow‐up survey was administered assessing (1) total number of contacts with the visiting professor in the year following the visit, (2) whether a letter of recommendation resulted from the visit, (3) whether the respondent had seen evidence of spread of innovative ideas as a result of the program, (4) participation in a cross‐institutional collaboration as a result of the program, and (5) assessment of benefit in continuing the program in the next year. The respondents were also asked to rate the global utility of the program to their professional development on a 5‐point scale ranging from not at all useful to very useful (Thinking about what has happened to you since the visit a year ago, please rate the usefulness of the entire program to your professional life: overall usefulness for my professional development.). Domain‐specific utility in improving clinical, research, quality improvement, and administrative skills were also elicited (results not shown). Finally, suggestions to improve the program for the future were solicited. The Colorado Multiple Institutional Review Board determined that the study of this faculty development program did not qualify as human subjects research, and subjects were therefore not asked to provide informed consent for participation.
RESULTS
To date, 5 academic medical centers have participated in the visiting professorship program, with 7 visiting professors interacting with 29 early‐career faculty. Of the 29 early‐career faculty, 72% (21/29) were at the rank of assistant professor, 17% (5/29) instructor, 7% (2/29) residents with plans to hire, and 3% (1/29) associate professor. The median was 2 years in academic medicine and 1 year at current academic rank. Forty‐one percent (12/29) were women and 7% (2/29) identified as ethnic minority. Of the 7 visiting professors, 57% (4/7) were assistant professor and 43% (3/7) were associate professors. The median was 5 years in academic medicine, 29% (2/7) were women, and none identified as ethnic minority.
Immediate postvisit survey response was obtained for all participating faculty. In the immediate postvisit survey, on a 5‐point Likert scale, the 29 early‐career faculty rated the visit: 4.4 for promoting cross‐institutional dissemination of innovations, 4.2 for advancing my academic career, 4.2 for fostering cross‐institutional mentor‐mentee relationships, and 4.4 for promoting cross‐institutional collaborations. Ninety‐three percent (26/28 accounting for 1 nonresponse to this question) reported the visiting professorship had high potential to disseminate innovation (rated greater than 3 on the 5‐point Likert score). Eighty‐three percent (24/29) of the early‐career faculty rated the visit highly useful in advancing their career, 76% (22/29) responded that the visit was highly likely to foster external mentorship relationships, and 90% (26/29) reported the visit highly effective in promoting cross‐institutional collaborations. In the immediate postvisit survey, the 7 visiting professors rated the visit 4.9 for promoting cross‐institutional dissemination of innovations, 4.3 for advancing my academic career, 4.0 for fostering cross‐institutional mentor‐mentee relationships, and 4.3 for promoting cross‐institutional collaborations.
Free‐text comments from both visiting professors and early‐career faculty were generally favorable (Table 1). Some comments offered constructive input on appropriate matching of faculty, previsit preparation, or desire for more time in sessions (Table 1).
Visiting Professors (n = 7) | Early‐Career Faculty (n = 29) |
---|---|
I was very impressed with the degree of organization, preparation, and structure from [host institution]. The project is a great concept and may well lead to similar and even more developed ones in the future. It is very helpful to get the pulse on another program and to hear of some of the same struggles and successes of another hospitalist program. The potential for cross‐site mentor‐mentee relationships and collaborations is a win‐win for both programs. | I really enjoyed my individual meeting with [visiting professor]. She was helpful in reviewing current projects from another perspective and very helpful in making suggestions for future projects. Also enjoyed her Grand Rounds and plan to follow‐up on this issue for possible cross‐institutional collaboration. |
Overall, this exchange is a great program. It is fun, promotes idea exchange, and is immensely helpful to the visiting professor for promotion. Every meeting I had with faculty at [host institution] was interesting and worthwhile. The primary challenge is maintaining mentorship ties and momentum after the visit. I personally e‐mailed every person I met and received many responses, including several explicit requests for ongoing advising and collaboration. | I think this is a great program. It definitely gives us the opportunity to meet people outside of the [host institution] community and foster relationships, mentorship, and possible collaborations with projects and programs. |
I liked multidisciplinary rounding. Research club. Meeting with faculty and trying to find common areas of interest. | I think this is a fantastic program so far. [Visiting professor] was very energetic and interested in making the most of the day. She contacted me after the visit and offered to keep in touch in the future. Right now I can see the program as being most useful in establishing new mentor/mentee relationships. |
Most of the faculty I met with see value in being involved in systems/quality improvement, but most do not express interest in specific projects. Areas needing improvement were identified by everyone I met with so developing projects around these areas should be doable. They might benefit from access to mentoring in quality improvement. | It was fantastic to meet with [visiting professor] and get a sense for his work and also brainstorm about how we might do similar work here in the future (eg, in high‐value care). It was also great to then see him 2 days later at [national conference]. I feel this is a great program to improve our connections cross‐institutionally and hopefully to spark some future collaborations. |
Very worthwhile. Was really helpful to meet with various faculty and leadership to see similarities and differences between our institutions. Generated several ideas for collaborative activities already. Also really helpful to have a somewhat structured way to share my work at an outside institution, as well as to create opportunities for mentor‐mentee relationships outside my home institution. | Incredibly valuable to promote this kind of cross‐pollination for both collaboration and innovation. |
Wonderful, inspiring, professionally advantageous. | |
Good idea. Good way to help midcareer faculty with advancement. Offers promise for collaboration of research/workshops. | |
Suggestions for Improvement | |
Please have e‐mails of the folks we meet available immediately after the visit. It is hard to know if anyone felt enough of a connection to want mentorship from me. | I feel like I may be a bit early on to benefit as much as I could have. |
Develop a mentorship program for quality improvement. As part of this exchange, consider treating visits as similar to a consultation. Have visitor with specific focus that they can offer help with. | Nice to have personal access to accomplished faculty from other institutions. Their perspective and career trajectory don't always align due to differences in institution culture, specifics of promotion process, and so on, but still a useful experience. |
Share any possible more‐formal topics for discussion with leadership prior to the visit so can prepare ahead of time (eg, gather information they may have questions on). Otherwise it was great! | For early career faculty, more discussions prior in regard to what to expect. |
A question is who should continue to push? Is it the prospective mentee, the mentee's institution, an so on? | Great idea. Would have loved to be involved in more aspects. More time for discussion would have been good. Did not get to discuss collaboration in person. |
Great to get to talk to someone from totally different system. Wish we had more time to talk. |
One‐year follow‐up was obtained for all but 1 early‐career faculty member receiving the follow‐up survey, and all 3 visiting professors. Of the 3 visiting professorships that occurred more than 1 year ago, 16 mentorship contacts occurred in total (phone, e‐mail, or in person) between 13 early‐career faculty and 3 visiting professors in the year after the initial visits (range, 04 contacts). Follow‐up contact occurred for 3 of 4 early‐career faculty from the first visiting professorship, 3 of 5 from the second visiting professorship, and 2 of 4 from the third visiting professorship. One early‐career faculty member from each host academic medical center had 3 or more additional contacts with the visiting professor in the year following the initial visit. Overall, 8/13 (62%) of early‐career faculty had at least 1 follow‐up mentoring discussion. On 1‐year follow‐up, overall utility for professional development was rated an average of 3.5 by early‐career faculty (with a trend of higher ratings of efficacy with increasing number of follow‐up contacts) and 4.7 by visiting professors. Half (8/16) of the involved faculty report having seen evidence of cross‐institutional dissemination of innovation. Ninety‐four percent (15/16) of participants at 1‐year follow‐up felt there was benefit to their institution in continuing the program for the next year.
Objective evidence of cross‐institutional scholarship, assessed by email query of both visiting professors and senior hospitalists coordinating the visits, includes 2 collaborative peer reviewed publications including mentors and mentees participating in the visiting professorship.[6, 7] Joint educational curriculum development on high‐value care between sites is planned. The Visiting Professorship in Hospital Medicine Program has resulted in 1 external letter to support a visiting professor's promotion to date.
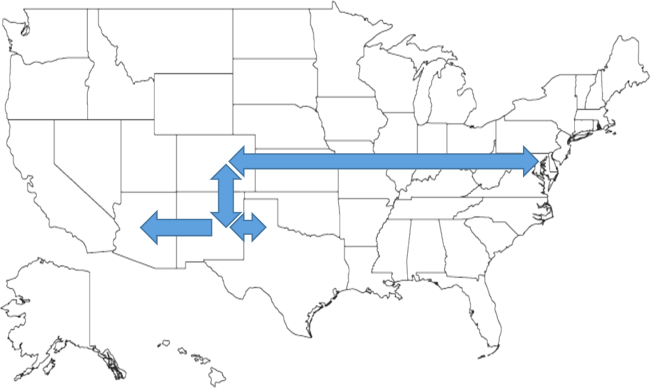
DISCUSSION
Hospital Medicine is a young, rapidly growing field, hence the number of experienced academic hospitalist mentors with expertise in successfully navigating an academic career is limited. A national study of hospitalist leaders found that 75% of clinician‐educators and 58% of research faculty feel that lack of mentorship is a major issue.[1] Mentorship for hospitalist clinician‐investigators is often delivered by nonhospitalists.[2, 8] There is little evidence of external mentorship for academic clinician‐educators in hospital medicine.[1] Without explicit programmatic support, many faculty may find this to be a barrier to career advancement. A study of successfully promoted hospitalists identified difficulty identifying external senior hospitalists to write letters in support of promotion as an obstacle.[9] Our study of the Visiting Professorship in Hospital Medicine Program found that early‐career faculty rated the visit as useful in advancing their career and fostered external mentorship relationships. Subsequent experience suggests more than half of the early‐career faculty will maintain contact with the visiting professor over the year following the visit. Visiting professors rate the experience particularly highly in their own career advancement.
The hospitalist movement is built on a foundation of innovation. The focus of each presentation was on an innovation developed by the visiting professor, and each visit showcased an innovation of the visited institution. This is distinct from traditional Hospital Grand Rounds, which more often focus on basic science research or clinical pathophysiology/disease management based on subspecialty topics.[10] The Visiting Professorship in Hospital Medicine Program was judged by participants to be an effective means of spreading innovation.
Insights from experience with the Visiting Professorship in Hospital Medicine Program include the importance of preliminary work prior to each visit. Program directors need to attend closely to the fit between the interests and career path of the visiting professor and those of the early‐career faculty. The innovations being shared should be aligned with organizational interests to maximize the chance of subsequent spread of the innovation and future collaboration. Providing faculty information about the objectives of the program in advance of the visit and arranging an exchange of curricula vitae between the early‐career faculty and the visiting professor allows participants to prepare for the in‐person coaching. Based on comments from participants, prompting contact from the visiting professor after the visit may be helpful to initiate the longitudinal relationship. We also found that early‐career faculty may not be aware of how to effectively use a mentoring relationship with an external faculty member. Training sessions for both mentors and mentees on effective mentorship relationships before visiting professorships might improve early‐career faculty confidence in initiating relationships and maximize value from mentor coaching.
A key issue is finding the right level of career maturity for the visiting professor. Our approach in selecting visiting professors was congruent with utilization of midcareer peer coaches employed by intrainstitutional hospital medicine mentoring programs.[11] The visiting professor should have sufficient experience and accomplishments to be able to effectively counsel junior faculty. However, it is important that the visiting professor also has sufficient time and interest to take on additional mentees and to be a full participant in shared scholarship projects emerging from the experience.
This study represents the experience of 5 mature academic hospitalist groups, and results may not be generalizable to dissimilar institutions or if only the most senior faculty are selected to perform visits. There is an inherent selection bias in the choice of both visiting professor and early‐career faculty. The small sample size of the faculty exposed to this program is a limitation to generalizability of the results of this evaluation. Whether this program will result in greater success in promotion of academic hospitalists cannot be assessed based on the follow‐up available. The Visiting Professorship in Hospital Medicine Program has continued to be sustained with an additional academic medical center enrolled and 2 additional site visits planned. The costs of the program are low, largely air travel and a night of lodging, as well as nominal administrative logistical support. Perceived benefits by participants and academic medical centers make this modest investment worth considering for academic hospitalist groups.
CONCLUSIONS
The Visiting Professorship in Hospital Medicine Program offers structure, opportunities, and access to senior mentors to advance the development of early‐career hospitalists while spreading innovation to distant sites. It is assessed by participants to facilitate external mentoring relationships and has the potential to advance the careers of both early‐career faculty as well as the visiting professors.
Disclosure
Nothing to report.
- Survey of US academic hospitalist leaders about mentorship and academic activities in hospitalist groups. J Hosp Med. 2011;6:5–9. , , , .
- Mentorship, productivity, and promotion among academic hospitalists. J Gen Intern Med. 2012;27(1):23–27. , , , , , .
- Mentoring faculty in academic medicine: a new paradigm? J Gen Intern Med. 2005;20(9):866–870. , .
- Challenges and opportunities in academic hospital medicine: report from the Academic Hospital Medicine Summit. J Hosp Med. 2009;4:240–246. , , , , , .
- The need for mentors in the odyssey of the academic hospitalist. J Hosp Med. 2011;6:1–2. , .
- Procedural skills for hospitalists. Hosp Med Clin. 2016;5:114–136. , , , .
- Cedecea davisae' s role in a polymicrobial lung infection in a cystic fibrosis patient. Case reports in infectious diseases. Case Rep Infect Dis. 2012;2012:176864. , , .
- Innovative approach to supporting hospitalist physicians towards academic success. J Hosp Med. 2008;3:314–318. , , , .
- Tried and true: a survey of successfully promoted academic hospitalists. J Hosp Med. 2011;6:411–415. , , , , .
- A case study of medical grand rounds: are we using effective methods? Acad Med. 2009;84(8):1144–1151. , , , , .
- Investing in the future: building an academic hospitalist faculty development program. J Hosp Med. 2011;6:161–166. , , , .
- Survey of US academic hospitalist leaders about mentorship and academic activities in hospitalist groups. J Hosp Med. 2011;6:5–9. , , , .
- Mentorship, productivity, and promotion among academic hospitalists. J Gen Intern Med. 2012;27(1):23–27. , , , , , .
- Mentoring faculty in academic medicine: a new paradigm? J Gen Intern Med. 2005;20(9):866–870. , .
- Challenges and opportunities in academic hospital medicine: report from the Academic Hospital Medicine Summit. J Hosp Med. 2009;4:240–246. , , , , , .
- The need for mentors in the odyssey of the academic hospitalist. J Hosp Med. 2011;6:1–2. , .
- Procedural skills for hospitalists. Hosp Med Clin. 2016;5:114–136. , , , .
- Cedecea davisae' s role in a polymicrobial lung infection in a cystic fibrosis patient. Case reports in infectious diseases. Case Rep Infect Dis. 2012;2012:176864. , , .
- Innovative approach to supporting hospitalist physicians towards academic success. J Hosp Med. 2008;3:314–318. , , , .
- Tried and true: a survey of successfully promoted academic hospitalists. J Hosp Med. 2011;6:411–415. , , , , .
- A case study of medical grand rounds: are we using effective methods? Acad Med. 2009;84(8):1144–1151. , , , , .
- Investing in the future: building an academic hospitalist faculty development program. J Hosp Med. 2011;6:161–166. , , , .
Predictors of Prolonged Hospitalizations
Hospitalizations frequently last longer than warranted by medical necessity alone, due to inefficiencies within the US healthcare system.[1, 2] Discharge delays place patients at risk for hospital‐acquired complications and increase costs. With the growing emphasis on high‐value care, hospital length of stay (LOS) has emerged as a key metric for inpatient care and will remain a central focus of hospital‐based improvement initiatives for the foreseeable future.
Hospitals may find it difficult to identify the primary drivers of inpatient LOS in a dynamic and increasingly complex healthcare system. Multiple recent policy changes have affected inpatient care. The Health Information Technology for Economic and Clinical Health Act of 2009 has led to widespread adoption of electronic health records (EHRs) that have markedly impacted provider workflows.[3] In October 2013, the Centers for Medicare & Medicaid Services implemented the 2‐midnight rule, which reclassified lower acuity inpatients with an expected stay <48 hours to observation status.[4] In January 2014, expansion of insurance coverage under the Affordable Care Act (ACA) altered payer mix for hospitals nationwide.[5] At a local level, hospitals that are rapidly adjusting resource allocation, capital investments, and marketing efforts and making complex operational decisions (eg, to open new units or change admission or referral algorithms) may simultaneously experience shifts in patient volumes, case‐mix index, and staffing ratios with downstream effects on LOS.
Given the myriad factors influencing inpatient LOS, hospital leaders may encounter real challenges in designing effective LOS reduction strategies. For example, they may expend significant resources on real‐time demand‐capacity management systems to improve hospital‐wide patient flow, but the resultant emphasis on bed placement and early discharges may shave only hours off average LOS.[6, 7] An alternative approach may be to target the small percentage of patients with prolonged hospitalizations who contribute disproportionately to the average LOS, as other initiatives focused on high utilizers have done.[8, 9, 10]
Our institution noted an increase in the average inpatient LOS for general medicine patients from 2012 to 2014, prompting a call to action by hospital leaders. We sought to characterize the predictors of prolonged hospitalizations among medicine patients to guide future efforts aimed at mitigating the contribution of prolonged LOS to overall LOS.
METHODS
Study Design
We performed a retrospective analysis of medicine patients discharged between January 1, 2012 and December 31, 2014, from the University of Colorado Hospital, a 551‐bed urban, quaternary‐care academic medical center in Aurora, Colorado. Patients were included if they were admitted under inpatient status, 18 years of age, and discharged from 1 of our 10 medicine services: 7 services with residents, staffed by hospitalists, general internists, or subspecialists; and 3 services with advanced practice providers, staffed by hospitalists.
Data Collection
We obtained LOS, calendar year of discharge, demographic data, insurance type, discharge disposition, number of medications, consults, intensive care unit (ICU) stays, surgeries (ie, procedures requiring anesthesia), and primary diagnosis by International Classification of Diseases, Ninth Revision codes from an administrative database that had been developed, validated, and maintained by our hospital medicine group. This database was populated with variables from our EHR, which was implemented in September 2011; to minimize variability in data input during the EHR rollout, we excluded data from September 2011 through December 2011. The Colorado Multiple Institutional Review Board reviewed and exempted this database (protocol 13‐2953) as a program evaluation.
Outcomes
We defined a prolonged hospitalization or LOS as >21 days in duration. This represented approximately 2 standard deviations above the mean LOS in our cohort. This cutoff also helped to remove provider‐level variability, as each medicine service was staffed by 2 attendings per month, each working approximately 7 days on and 7 days off. We examined LOS >14 and >30 days in sensitivity analyses to ensure that the selection of >21 days did not impose an arbitrary and invalid limitation on our statistical analysis.
Statistical Analysis
Demographic and clinical data were compared in the group with LOS 21 days versus the group with LOS >21 days with a 2 test for dichotomous variables and Student t test for continuous variables. We then built a multivariable logistic regression model to predict LOS >21 days using the variables that were significantly different between groups in bivariate analyses. A two‐sided P value of <0.05 was considered statistically significant. All data analyses were performed using Stata 12.0 (StataCorp, College Station, TX).
RESULTS
We identified 18,363 inpatient discharges among 12,511 medicine patients between January 1, 2012 and December 31, 2014. Of these discharges, 416 (2.3%) demonstrated prolonged LOS. Prolonged hospitalizations accounted for 18.6% of total inpatient days. The average LOS during the study period was 4.8 days including patients with prolonged LOS and 4.0 days excluding patients with prolonged LOS, a contribution of 0.8 days.
Table 1 compares the characteristics of patients with and without prolonged LOS. Age, insurance, discharge disposition, palliative care consults, ICU stays, and surgeries were among the variables that differed significantly between the 2 groups. Among patients undergoing surgery, those with prolonged LOS were more likely to have surgery >24 hours after admission than those without prolonged LOS (85.7% vs 51.4%, P<0.001).
Variable | LOS 21 Days, N=17,947 | LOS >21 Days, N=416 | P Value |
---|---|---|---|
| |||
Age, y, mean (SD) | 56.4 (18.7) | 54.4 (17.1) | 0.030 |
Female | 9,256 (52%) | 199 (48%) | 0.132 |
Year of discharge | <0.001 | ||
2012 | 5,486 (31%) | 69 (17%) | |
2013 | 6,193 (35%) | 162 (39%) | |
2014 | 6,268 (35%) | 185 (44%) | |
Race/ethnicity | 0.003 | ||
White non‐Hispanic | 9,702 (54%) | 242 (58%) | |
Black non‐Hispanic | 4,000 (22%) | 68 (16%) | |
Hispanic | 2,872(16%) | 67 (16%) | |
Asian | 578 (3%) | 9 (2%) | |
Other or unknown | 795 (4%) | 30 (7%) | |
Language preference | 0.795 | ||
English | 16,049 (89%) | 376 (90%) | |
Spanish | 1,052 (6%) | 23 (6%) | |
Other | 846 (5%) | 17 (4%) | |
Insurance | <0.001 | ||
Medicare | 5,462 (30%) | 109 (26%) | |
Medicaid | 3,406 (19%) | 126 (30%) | |
Dual | 2,815 (16%) | 64 (15%) | |
Private | 2,714 (15%) | 60 (14%) | |
Indigent/self‐pay | 2,829 (16%) | 42 (10%) | |
Other | 721 (4%) | 15 (4%) | |
Length of stay, d (SD) | 4.0 (3.5) | 39.5 (37.3) | <0.001 |
Discharge disposition | <0.001 | ||
Home with self‐care | 13,276 (74%) | 115 (28%) | |
Home with home health | 1,584 (9%) | 79 (19%) | |
Hospicehome or inpatient | 369 (2%) | 19 (5%) | |
Postacute‐care facility or LTAC | 1,761 (10%) | 141 (34%) | |
Expired | 113 (1%) | 18 (4%) | |
Other | 844 (5%) | 44 (11%) | |
No. of admission medications (SD) | 9.7 (7.4) | 10.9 (7.8) | 0.002 |
Primary diagnosis by ICD‐9 code* | |||
Sepsis, unspecified | 1,548 (9%) | 55 (13%) | 0.001 |
Acute respiratory failure | 293 (2%) | 9 (2%) | 0.400 |
MSSA septicemia | 36 (0.2%) | 8 (2%) | <0.001 |
MRSA septicemia | 13 (0.1%) | 7 (2%) | <0.001 |
Alcoholic cirrhosis of the liver | 111 (1%) | 7 (2%) | 0.007 |
Palliative care consult | 398 (2%) | 64 (15%) | <0.001 |
ICU stay | 2,030 (11%) | 246 (59%) | <0.001 |
Surgical procedure | 1,800 (10%) | 182 (44%) | <0.001 |
Unspecified sepsis was the most frequent primary diagnosis, regardless of LOS category (Table 2). However, the second through fifth most frequent diagnoses differed for patients with and without prolonged LOS.
N | % | |
---|---|---|
| ||
LOS 21 days | ||
1. Sepsis, unspecified | 1,548 | 8.6% |
2. Acute pancreatitis | 435 | 2.4% |
3. Pneumonia | 431 | 2.4% |
4. Acute kidney failure | 363 | 2.0% |
5. COPD exacerbation | 320 | 1.8% |
LOS >21 days | ||
1. Sepsis, unspecified | 55 | 13.0% |
2. Acute respiratory failure | 9 | 2.2% |
3. Methicillin‐sensitive Staphylococcus aureus septicemia | 8 | 1.9% |
4. Methicillin‐resistant Staphylococcus aureus septicemia | 7 | 1.7% |
5. Alcoholic cirrhosis of the liver | 7 | 1.7% |
In an adjusted logistic regression model (Table 3), we found lower odds of prolonged LOS for each 10‐year increase in age and higher odds of prolonged LOS for Medicaid insurance, discharge to home with home health, discharge to a postacute‐care or long‐term acute‐care facility, and in‐hospital death. Methicillin‐resistant Staphylococcus aureus (MRSA) septicemia, palliative care consults, ICU stays, and surgeries were all also associated with increased odds of prolonged LOS. We identified a statistically significant interaction between ICU stay and surgical procedure (odds ratio: 2.53, 95% confidence interval: 1.51‐4.26, P<0.001).
Outcome: LOS >21 Days | Odds Ratio | 95% CI | P Value |
---|---|---|---|
| |||
Age, per 10 years increase in age | 0.80 | 0.73‐0.87 | <0.001 |
Year of discharge | |||
2012 | 0.47 | 0.34‐0.67 | <0.001 |
2013 | 1.10 | 0.84‐1.43 | 0.493 |
2014 | Ref | ||
Race/ethnicity | |||
White non‐Hispanic | Ref | ||
Black non‐Hispanic | 0.89 | 0.64‐1.22 | 0.454 |
Hispanic | 1.01 | 0.70‐1.46 | 0.952 |
Asian | 0.85 | 0.40‐1.83 | 0.679 |
Other or unknown | 1.29 | 0.73‐2.26 | 0.378 |
Insurance | |||
Medicare | Ref | ||
Medicaid | 1.99 | 1.29‐3.05 | 0.002 |
Dual | 1.06 | 0.72‐1.57 | 0.765 |
Private | 1.13 | 0.70‐1.82 | 0.620 |
Indigent/self‐pay | 1.66 | 0.95‐2.88 | 0.073 |
Other | 0.96 | 0.47‐1.96 | 0.908 |
Discharge disposition | |||
Home with self‐care | Ref | ||
Home with home health | 4.48 | 3.10‐6.48 | <0.001 |
Hospicehome or inpatient | 2.11 | 0.98‐4.55 | 0.057 |
Postacute‐care facility or LTAC | 10.37 | 6.92‐15.56 | <0.001 |
Expired | 5.38 | 2.27‐12.75 | <0.001 |
Other | 4.04 | 2.64‐6.18 | <0.001 |
No. of admission medications | 1.00 | 0.99‐1.02 | 0.775 |
Primary diagnosis by ICD‐9 code | |||
Sepsis, unspecified | 1.11 | 0.78‐1.58 | 0.575 |
MSSA septicemia | 2.44 | 0.68‐8.67 | 0.074 |
MRSA septicemia | 8.83 | 1.72‐45.36 | 0.009 |
Alcoholic cirrhosis of the liver | 1.25 | 0.43‐3.65 | 0.687 |
Palliative care consult | 4.63 | 2.86‐7.49 | <0.001 |
ICU stay | 6.66 | 5.22‐8.50 | <0.001 |
Surgical procedure | 5.04 | 3.90‐6.52 | <0.001 |
Sensitivity analyses using LOS >14 and >30 days yielded similar results to LOS >21 days (see Supporting Appendix Table 1 in the online version of this article).
DISCUSSION
We found that a small proportion of medicine patients with prolonged hospitalizations contributed substantially to both total inpatient days and average inpatient LOS. Such disproportionate healthcare utilization is concerning in light of the Institute of Medicine's charge for health systems to deliver timely, efficient, and equitable care.[11]
Few studies in the United States have analyzed patient characteristics that predict prolonged LOS, and to our knowledge, none have evaluated prolonged LOS specifically in general medicine patients.[12, 13, 14] Among selected surgical populations, prolonged hospitalizations are most often related to placement difficulties, operational delays, and payer‐related issues, rather than severity of illness, baseline comorbidities, or in‐hospital complications.[12, 13] In our study, we found that patients with prolonged LOS were more likely to require a palliative care consult, ICU stay, or surgery, all proxies for disease severity. Patients with prolonged LOS were also more likely to undergo surgery >24 hours after admission than those without prolonged LOS, suggesting that the former were either too unstable to proceed directly to surgery or developed complications later during their hospitalization. Even after controlling for palliative care consults, ICU stays, and surgeries, placement at a postacute‐care facility was strongly associated with prolonged LOS. Patients with prolonged LOS were also more likely to have Medicaid compared to other insurance types.
Our findings have several potential implications for efforts aimed at decreasing the number of and length of prolonged hospitalizations. Although demographic and clinical factors such as Medicaid insurance, ICU stays, and surgeries are generally not modifiable, they could, particularly in combination, be used to trigger earlier and more intensive case management involvement. A streamlined insurance approval process for Medicaid pending inpatients could be beneficial, given the recent expansion of Medicaid eligibility under the ACA. Hospital partnerships with postacute‐care facilities could also relieve bottlenecks in placement.[8] Chart review of the patients with MRSA septicemia and prolonged LOS indicated that development of an intensive outpatient parenteral antimicrobial therapy pathway with substance abuse counseling could provide an alternative to extended inpatient treatment for intravenous drug users with complicated infections.[15]
This study has several limitations. First, given the lack of consensus in the literature regarding the definition of a prolonged hospitalization, it is difficult to directly compare our results with existing studies.[8, 12, 13, 14] However, we believe LOS >21 days to be a meaningful cutoff for our cohort. Most demographic and clinical variables that were predictive at >21 days were also predictive at >14 and >30 days, which reassured us that the relationship between variables and prolonged LOS was stable at different thresholds. Second, our database did not allow us to fully adjust for baseline comorbidities or categorize the reasons for discharge delays. Finally, this was a single‐center program evaluation. Although this limits generalization to other institutions, we believe our approach may serve as a guide for others interested in reducing prolonged hospitalizations.
In summary, prolonged hospitalizations represent a potentially high‐yield target for LOS reduction efforts. Prolonged hospitalizations among medicine patients at our institution particularly affected Medicaid enrollees with complex hospital stays who were not discharged home. Further studies are needed to determine the specific reasons for unnecessary hospital days in this population.
Acknowledgements
The authors thank Essey Yirdaw for her contributions to the building and management of the database that informed this work.
Disclosure: M.E.A. conceived of the study concept and design and drafted the manuscript. J.J.G. assisted with the study design and made critical revisions to the final manuscript. D.A., R.P., and R.C. assisted with the study design and made critical revisions to the manuscript. C.D.J. assisted with the study design, performed data analyses, and made critical revisions to the manuscript. RC has disclosed that her time is funded by a National Institutes of Health grant unrelated to this study. A modified abstract was presented in poster format at the Society of Hospital Medicine Research, Innovations, and Vignettes Competition 2015 Annual Meeting, held March 29 April 1, 2015, in National Harbor, Maryland. The authors have no conflicts of interest to disclose.
- Excess hospitalization days in an academic medical center: perceptions of hospitalists and discharge planners. Am J Manag Care. 2011;17(2):e34–e42. , , , et al.
- A prospective study of reasons for prolonged hospitalizations on a general medicine teaching service. J Gen Intern Med. 2005;20(2):108–115. , ,
- Adoption of electronic health records grows rapidly, but fewer than half of U.S. hospitals had at least a basic system in 2012. Health Aff (Millwood). 2013;32(8):1478–1485. , , , et al.
- Observation and inpatient status: clinical impact of the 2‐midnight rule. J Hosp Med. 2014;9(4):203–209. , , , et al.
- Impact of insurance expansion on hospital uncompensated care costs in 2014. Department of Health and Human Services Office of the Assistant Secretary for Planning and Evaluation. Available at: http://aspe.hhs.gov/health/reports/2014/uncompensatedcare/ib_uncompensatedcare.pdf. Accessed March 28, 2015. , ,
- Using real‐time demand capacity management to improve hospital‐wide patient flow. Jt Comm J Qual Patient Saf. 2011;37(5):217–227. , , ,
- Natural history of late discharges from a general medical ward. J Hosp Med. 2009;4(4):226–233. , , , , ,
- Addressing hospital length of stay outlier patients: a community‐wide approach. Adv Biosci Biotechol. 2014;5:188–196. , , ,
- American medical home runs. Health Aff (Millwood). 2009;28(5):1317–1326. ,
- The hot spotters: can we lower medical costs by giving the neediest patients better care? New Yorker. January 2011:40–51.
- Institute of Medicine. Crossing the Quality Chasm. Washington, DC: National Academies Press; 2001.
- Excessively long hospital stays after trauma are not related to the severity of illness: let's aim to the right target! JAMA Surg. 2013;148(1):956–961. , , , et al.
- Extended length of stay after surgery: complications, inefficient practice, or sick patients? JAMA Surg. 2014;149(8):815–820. , ,
- Nonmedical factors associated with prolonged hospital length of stay in an urban homebound population. J Hosp Med. 2012;7(2):73–78. , , , ,
- Safe and successful treatment of intravenous drug users with a peripherally inserted central catheter in an outpatient parenteral antibiotic treatment service. J Antimicrob Chemother. 2010;65(12):2641–2644. , , ,
Hospitalizations frequently last longer than warranted by medical necessity alone, due to inefficiencies within the US healthcare system.[1, 2] Discharge delays place patients at risk for hospital‐acquired complications and increase costs. With the growing emphasis on high‐value care, hospital length of stay (LOS) has emerged as a key metric for inpatient care and will remain a central focus of hospital‐based improvement initiatives for the foreseeable future.
Hospitals may find it difficult to identify the primary drivers of inpatient LOS in a dynamic and increasingly complex healthcare system. Multiple recent policy changes have affected inpatient care. The Health Information Technology for Economic and Clinical Health Act of 2009 has led to widespread adoption of electronic health records (EHRs) that have markedly impacted provider workflows.[3] In October 2013, the Centers for Medicare & Medicaid Services implemented the 2‐midnight rule, which reclassified lower acuity inpatients with an expected stay <48 hours to observation status.[4] In January 2014, expansion of insurance coverage under the Affordable Care Act (ACA) altered payer mix for hospitals nationwide.[5] At a local level, hospitals that are rapidly adjusting resource allocation, capital investments, and marketing efforts and making complex operational decisions (eg, to open new units or change admission or referral algorithms) may simultaneously experience shifts in patient volumes, case‐mix index, and staffing ratios with downstream effects on LOS.
Given the myriad factors influencing inpatient LOS, hospital leaders may encounter real challenges in designing effective LOS reduction strategies. For example, they may expend significant resources on real‐time demand‐capacity management systems to improve hospital‐wide patient flow, but the resultant emphasis on bed placement and early discharges may shave only hours off average LOS.[6, 7] An alternative approach may be to target the small percentage of patients with prolonged hospitalizations who contribute disproportionately to the average LOS, as other initiatives focused on high utilizers have done.[8, 9, 10]
Our institution noted an increase in the average inpatient LOS for general medicine patients from 2012 to 2014, prompting a call to action by hospital leaders. We sought to characterize the predictors of prolonged hospitalizations among medicine patients to guide future efforts aimed at mitigating the contribution of prolonged LOS to overall LOS.
METHODS
Study Design
We performed a retrospective analysis of medicine patients discharged between January 1, 2012 and December 31, 2014, from the University of Colorado Hospital, a 551‐bed urban, quaternary‐care academic medical center in Aurora, Colorado. Patients were included if they were admitted under inpatient status, 18 years of age, and discharged from 1 of our 10 medicine services: 7 services with residents, staffed by hospitalists, general internists, or subspecialists; and 3 services with advanced practice providers, staffed by hospitalists.
Data Collection
We obtained LOS, calendar year of discharge, demographic data, insurance type, discharge disposition, number of medications, consults, intensive care unit (ICU) stays, surgeries (ie, procedures requiring anesthesia), and primary diagnosis by International Classification of Diseases, Ninth Revision codes from an administrative database that had been developed, validated, and maintained by our hospital medicine group. This database was populated with variables from our EHR, which was implemented in September 2011; to minimize variability in data input during the EHR rollout, we excluded data from September 2011 through December 2011. The Colorado Multiple Institutional Review Board reviewed and exempted this database (protocol 13‐2953) as a program evaluation.
Outcomes
We defined a prolonged hospitalization or LOS as >21 days in duration. This represented approximately 2 standard deviations above the mean LOS in our cohort. This cutoff also helped to remove provider‐level variability, as each medicine service was staffed by 2 attendings per month, each working approximately 7 days on and 7 days off. We examined LOS >14 and >30 days in sensitivity analyses to ensure that the selection of >21 days did not impose an arbitrary and invalid limitation on our statistical analysis.
Statistical Analysis
Demographic and clinical data were compared in the group with LOS 21 days versus the group with LOS >21 days with a 2 test for dichotomous variables and Student t test for continuous variables. We then built a multivariable logistic regression model to predict LOS >21 days using the variables that were significantly different between groups in bivariate analyses. A two‐sided P value of <0.05 was considered statistically significant. All data analyses were performed using Stata 12.0 (StataCorp, College Station, TX).
RESULTS
We identified 18,363 inpatient discharges among 12,511 medicine patients between January 1, 2012 and December 31, 2014. Of these discharges, 416 (2.3%) demonstrated prolonged LOS. Prolonged hospitalizations accounted for 18.6% of total inpatient days. The average LOS during the study period was 4.8 days including patients with prolonged LOS and 4.0 days excluding patients with prolonged LOS, a contribution of 0.8 days.
Table 1 compares the characteristics of patients with and without prolonged LOS. Age, insurance, discharge disposition, palliative care consults, ICU stays, and surgeries were among the variables that differed significantly between the 2 groups. Among patients undergoing surgery, those with prolonged LOS were more likely to have surgery >24 hours after admission than those without prolonged LOS (85.7% vs 51.4%, P<0.001).
Variable | LOS 21 Days, N=17,947 | LOS >21 Days, N=416 | P Value |
---|---|---|---|
| |||
Age, y, mean (SD) | 56.4 (18.7) | 54.4 (17.1) | 0.030 |
Female | 9,256 (52%) | 199 (48%) | 0.132 |
Year of discharge | <0.001 | ||
2012 | 5,486 (31%) | 69 (17%) | |
2013 | 6,193 (35%) | 162 (39%) | |
2014 | 6,268 (35%) | 185 (44%) | |
Race/ethnicity | 0.003 | ||
White non‐Hispanic | 9,702 (54%) | 242 (58%) | |
Black non‐Hispanic | 4,000 (22%) | 68 (16%) | |
Hispanic | 2,872(16%) | 67 (16%) | |
Asian | 578 (3%) | 9 (2%) | |
Other or unknown | 795 (4%) | 30 (7%) | |
Language preference | 0.795 | ||
English | 16,049 (89%) | 376 (90%) | |
Spanish | 1,052 (6%) | 23 (6%) | |
Other | 846 (5%) | 17 (4%) | |
Insurance | <0.001 | ||
Medicare | 5,462 (30%) | 109 (26%) | |
Medicaid | 3,406 (19%) | 126 (30%) | |
Dual | 2,815 (16%) | 64 (15%) | |
Private | 2,714 (15%) | 60 (14%) | |
Indigent/self‐pay | 2,829 (16%) | 42 (10%) | |
Other | 721 (4%) | 15 (4%) | |
Length of stay, d (SD) | 4.0 (3.5) | 39.5 (37.3) | <0.001 |
Discharge disposition | <0.001 | ||
Home with self‐care | 13,276 (74%) | 115 (28%) | |
Home with home health | 1,584 (9%) | 79 (19%) | |
Hospicehome or inpatient | 369 (2%) | 19 (5%) | |
Postacute‐care facility or LTAC | 1,761 (10%) | 141 (34%) | |
Expired | 113 (1%) | 18 (4%) | |
Other | 844 (5%) | 44 (11%) | |
No. of admission medications (SD) | 9.7 (7.4) | 10.9 (7.8) | 0.002 |
Primary diagnosis by ICD‐9 code* | |||
Sepsis, unspecified | 1,548 (9%) | 55 (13%) | 0.001 |
Acute respiratory failure | 293 (2%) | 9 (2%) | 0.400 |
MSSA septicemia | 36 (0.2%) | 8 (2%) | <0.001 |
MRSA septicemia | 13 (0.1%) | 7 (2%) | <0.001 |
Alcoholic cirrhosis of the liver | 111 (1%) | 7 (2%) | 0.007 |
Palliative care consult | 398 (2%) | 64 (15%) | <0.001 |
ICU stay | 2,030 (11%) | 246 (59%) | <0.001 |
Surgical procedure | 1,800 (10%) | 182 (44%) | <0.001 |
Unspecified sepsis was the most frequent primary diagnosis, regardless of LOS category (Table 2). However, the second through fifth most frequent diagnoses differed for patients with and without prolonged LOS.
N | % | |
---|---|---|
| ||
LOS 21 days | ||
1. Sepsis, unspecified | 1,548 | 8.6% |
2. Acute pancreatitis | 435 | 2.4% |
3. Pneumonia | 431 | 2.4% |
4. Acute kidney failure | 363 | 2.0% |
5. COPD exacerbation | 320 | 1.8% |
LOS >21 days | ||
1. Sepsis, unspecified | 55 | 13.0% |
2. Acute respiratory failure | 9 | 2.2% |
3. Methicillin‐sensitive Staphylococcus aureus septicemia | 8 | 1.9% |
4. Methicillin‐resistant Staphylococcus aureus septicemia | 7 | 1.7% |
5. Alcoholic cirrhosis of the liver | 7 | 1.7% |
In an adjusted logistic regression model (Table 3), we found lower odds of prolonged LOS for each 10‐year increase in age and higher odds of prolonged LOS for Medicaid insurance, discharge to home with home health, discharge to a postacute‐care or long‐term acute‐care facility, and in‐hospital death. Methicillin‐resistant Staphylococcus aureus (MRSA) septicemia, palliative care consults, ICU stays, and surgeries were all also associated with increased odds of prolonged LOS. We identified a statistically significant interaction between ICU stay and surgical procedure (odds ratio: 2.53, 95% confidence interval: 1.51‐4.26, P<0.001).
Outcome: LOS >21 Days | Odds Ratio | 95% CI | P Value |
---|---|---|---|
| |||
Age, per 10 years increase in age | 0.80 | 0.73‐0.87 | <0.001 |
Year of discharge | |||
2012 | 0.47 | 0.34‐0.67 | <0.001 |
2013 | 1.10 | 0.84‐1.43 | 0.493 |
2014 | Ref | ||
Race/ethnicity | |||
White non‐Hispanic | Ref | ||
Black non‐Hispanic | 0.89 | 0.64‐1.22 | 0.454 |
Hispanic | 1.01 | 0.70‐1.46 | 0.952 |
Asian | 0.85 | 0.40‐1.83 | 0.679 |
Other or unknown | 1.29 | 0.73‐2.26 | 0.378 |
Insurance | |||
Medicare | Ref | ||
Medicaid | 1.99 | 1.29‐3.05 | 0.002 |
Dual | 1.06 | 0.72‐1.57 | 0.765 |
Private | 1.13 | 0.70‐1.82 | 0.620 |
Indigent/self‐pay | 1.66 | 0.95‐2.88 | 0.073 |
Other | 0.96 | 0.47‐1.96 | 0.908 |
Discharge disposition | |||
Home with self‐care | Ref | ||
Home with home health | 4.48 | 3.10‐6.48 | <0.001 |
Hospicehome or inpatient | 2.11 | 0.98‐4.55 | 0.057 |
Postacute‐care facility or LTAC | 10.37 | 6.92‐15.56 | <0.001 |
Expired | 5.38 | 2.27‐12.75 | <0.001 |
Other | 4.04 | 2.64‐6.18 | <0.001 |
No. of admission medications | 1.00 | 0.99‐1.02 | 0.775 |
Primary diagnosis by ICD‐9 code | |||
Sepsis, unspecified | 1.11 | 0.78‐1.58 | 0.575 |
MSSA septicemia | 2.44 | 0.68‐8.67 | 0.074 |
MRSA septicemia | 8.83 | 1.72‐45.36 | 0.009 |
Alcoholic cirrhosis of the liver | 1.25 | 0.43‐3.65 | 0.687 |
Palliative care consult | 4.63 | 2.86‐7.49 | <0.001 |
ICU stay | 6.66 | 5.22‐8.50 | <0.001 |
Surgical procedure | 5.04 | 3.90‐6.52 | <0.001 |
Sensitivity analyses using LOS >14 and >30 days yielded similar results to LOS >21 days (see Supporting Appendix Table 1 in the online version of this article).
DISCUSSION
We found that a small proportion of medicine patients with prolonged hospitalizations contributed substantially to both total inpatient days and average inpatient LOS. Such disproportionate healthcare utilization is concerning in light of the Institute of Medicine's charge for health systems to deliver timely, efficient, and equitable care.[11]
Few studies in the United States have analyzed patient characteristics that predict prolonged LOS, and to our knowledge, none have evaluated prolonged LOS specifically in general medicine patients.[12, 13, 14] Among selected surgical populations, prolonged hospitalizations are most often related to placement difficulties, operational delays, and payer‐related issues, rather than severity of illness, baseline comorbidities, or in‐hospital complications.[12, 13] In our study, we found that patients with prolonged LOS were more likely to require a palliative care consult, ICU stay, or surgery, all proxies for disease severity. Patients with prolonged LOS were also more likely to undergo surgery >24 hours after admission than those without prolonged LOS, suggesting that the former were either too unstable to proceed directly to surgery or developed complications later during their hospitalization. Even after controlling for palliative care consults, ICU stays, and surgeries, placement at a postacute‐care facility was strongly associated with prolonged LOS. Patients with prolonged LOS were also more likely to have Medicaid compared to other insurance types.
Our findings have several potential implications for efforts aimed at decreasing the number of and length of prolonged hospitalizations. Although demographic and clinical factors such as Medicaid insurance, ICU stays, and surgeries are generally not modifiable, they could, particularly in combination, be used to trigger earlier and more intensive case management involvement. A streamlined insurance approval process for Medicaid pending inpatients could be beneficial, given the recent expansion of Medicaid eligibility under the ACA. Hospital partnerships with postacute‐care facilities could also relieve bottlenecks in placement.[8] Chart review of the patients with MRSA septicemia and prolonged LOS indicated that development of an intensive outpatient parenteral antimicrobial therapy pathway with substance abuse counseling could provide an alternative to extended inpatient treatment for intravenous drug users with complicated infections.[15]
This study has several limitations. First, given the lack of consensus in the literature regarding the definition of a prolonged hospitalization, it is difficult to directly compare our results with existing studies.[8, 12, 13, 14] However, we believe LOS >21 days to be a meaningful cutoff for our cohort. Most demographic and clinical variables that were predictive at >21 days were also predictive at >14 and >30 days, which reassured us that the relationship between variables and prolonged LOS was stable at different thresholds. Second, our database did not allow us to fully adjust for baseline comorbidities or categorize the reasons for discharge delays. Finally, this was a single‐center program evaluation. Although this limits generalization to other institutions, we believe our approach may serve as a guide for others interested in reducing prolonged hospitalizations.
In summary, prolonged hospitalizations represent a potentially high‐yield target for LOS reduction efforts. Prolonged hospitalizations among medicine patients at our institution particularly affected Medicaid enrollees with complex hospital stays who were not discharged home. Further studies are needed to determine the specific reasons for unnecessary hospital days in this population.
Acknowledgements
The authors thank Essey Yirdaw for her contributions to the building and management of the database that informed this work.
Disclosure: M.E.A. conceived of the study concept and design and drafted the manuscript. J.J.G. assisted with the study design and made critical revisions to the final manuscript. D.A., R.P., and R.C. assisted with the study design and made critical revisions to the manuscript. C.D.J. assisted with the study design, performed data analyses, and made critical revisions to the manuscript. RC has disclosed that her time is funded by a National Institutes of Health grant unrelated to this study. A modified abstract was presented in poster format at the Society of Hospital Medicine Research, Innovations, and Vignettes Competition 2015 Annual Meeting, held March 29 April 1, 2015, in National Harbor, Maryland. The authors have no conflicts of interest to disclose.
Hospitalizations frequently last longer than warranted by medical necessity alone, due to inefficiencies within the US healthcare system.[1, 2] Discharge delays place patients at risk for hospital‐acquired complications and increase costs. With the growing emphasis on high‐value care, hospital length of stay (LOS) has emerged as a key metric for inpatient care and will remain a central focus of hospital‐based improvement initiatives for the foreseeable future.
Hospitals may find it difficult to identify the primary drivers of inpatient LOS in a dynamic and increasingly complex healthcare system. Multiple recent policy changes have affected inpatient care. The Health Information Technology for Economic and Clinical Health Act of 2009 has led to widespread adoption of electronic health records (EHRs) that have markedly impacted provider workflows.[3] In October 2013, the Centers for Medicare & Medicaid Services implemented the 2‐midnight rule, which reclassified lower acuity inpatients with an expected stay <48 hours to observation status.[4] In January 2014, expansion of insurance coverage under the Affordable Care Act (ACA) altered payer mix for hospitals nationwide.[5] At a local level, hospitals that are rapidly adjusting resource allocation, capital investments, and marketing efforts and making complex operational decisions (eg, to open new units or change admission or referral algorithms) may simultaneously experience shifts in patient volumes, case‐mix index, and staffing ratios with downstream effects on LOS.
Given the myriad factors influencing inpatient LOS, hospital leaders may encounter real challenges in designing effective LOS reduction strategies. For example, they may expend significant resources on real‐time demand‐capacity management systems to improve hospital‐wide patient flow, but the resultant emphasis on bed placement and early discharges may shave only hours off average LOS.[6, 7] An alternative approach may be to target the small percentage of patients with prolonged hospitalizations who contribute disproportionately to the average LOS, as other initiatives focused on high utilizers have done.[8, 9, 10]
Our institution noted an increase in the average inpatient LOS for general medicine patients from 2012 to 2014, prompting a call to action by hospital leaders. We sought to characterize the predictors of prolonged hospitalizations among medicine patients to guide future efforts aimed at mitigating the contribution of prolonged LOS to overall LOS.
METHODS
Study Design
We performed a retrospective analysis of medicine patients discharged between January 1, 2012 and December 31, 2014, from the University of Colorado Hospital, a 551‐bed urban, quaternary‐care academic medical center in Aurora, Colorado. Patients were included if they were admitted under inpatient status, 18 years of age, and discharged from 1 of our 10 medicine services: 7 services with residents, staffed by hospitalists, general internists, or subspecialists; and 3 services with advanced practice providers, staffed by hospitalists.
Data Collection
We obtained LOS, calendar year of discharge, demographic data, insurance type, discharge disposition, number of medications, consults, intensive care unit (ICU) stays, surgeries (ie, procedures requiring anesthesia), and primary diagnosis by International Classification of Diseases, Ninth Revision codes from an administrative database that had been developed, validated, and maintained by our hospital medicine group. This database was populated with variables from our EHR, which was implemented in September 2011; to minimize variability in data input during the EHR rollout, we excluded data from September 2011 through December 2011. The Colorado Multiple Institutional Review Board reviewed and exempted this database (protocol 13‐2953) as a program evaluation.
Outcomes
We defined a prolonged hospitalization or LOS as >21 days in duration. This represented approximately 2 standard deviations above the mean LOS in our cohort. This cutoff also helped to remove provider‐level variability, as each medicine service was staffed by 2 attendings per month, each working approximately 7 days on and 7 days off. We examined LOS >14 and >30 days in sensitivity analyses to ensure that the selection of >21 days did not impose an arbitrary and invalid limitation on our statistical analysis.
Statistical Analysis
Demographic and clinical data were compared in the group with LOS 21 days versus the group with LOS >21 days with a 2 test for dichotomous variables and Student t test for continuous variables. We then built a multivariable logistic regression model to predict LOS >21 days using the variables that were significantly different between groups in bivariate analyses. A two‐sided P value of <0.05 was considered statistically significant. All data analyses were performed using Stata 12.0 (StataCorp, College Station, TX).
RESULTS
We identified 18,363 inpatient discharges among 12,511 medicine patients between January 1, 2012 and December 31, 2014. Of these discharges, 416 (2.3%) demonstrated prolonged LOS. Prolonged hospitalizations accounted for 18.6% of total inpatient days. The average LOS during the study period was 4.8 days including patients with prolonged LOS and 4.0 days excluding patients with prolonged LOS, a contribution of 0.8 days.
Table 1 compares the characteristics of patients with and without prolonged LOS. Age, insurance, discharge disposition, palliative care consults, ICU stays, and surgeries were among the variables that differed significantly between the 2 groups. Among patients undergoing surgery, those with prolonged LOS were more likely to have surgery >24 hours after admission than those without prolonged LOS (85.7% vs 51.4%, P<0.001).
Variable | LOS 21 Days, N=17,947 | LOS >21 Days, N=416 | P Value |
---|---|---|---|
| |||
Age, y, mean (SD) | 56.4 (18.7) | 54.4 (17.1) | 0.030 |
Female | 9,256 (52%) | 199 (48%) | 0.132 |
Year of discharge | <0.001 | ||
2012 | 5,486 (31%) | 69 (17%) | |
2013 | 6,193 (35%) | 162 (39%) | |
2014 | 6,268 (35%) | 185 (44%) | |
Race/ethnicity | 0.003 | ||
White non‐Hispanic | 9,702 (54%) | 242 (58%) | |
Black non‐Hispanic | 4,000 (22%) | 68 (16%) | |
Hispanic | 2,872(16%) | 67 (16%) | |
Asian | 578 (3%) | 9 (2%) | |
Other or unknown | 795 (4%) | 30 (7%) | |
Language preference | 0.795 | ||
English | 16,049 (89%) | 376 (90%) | |
Spanish | 1,052 (6%) | 23 (6%) | |
Other | 846 (5%) | 17 (4%) | |
Insurance | <0.001 | ||
Medicare | 5,462 (30%) | 109 (26%) | |
Medicaid | 3,406 (19%) | 126 (30%) | |
Dual | 2,815 (16%) | 64 (15%) | |
Private | 2,714 (15%) | 60 (14%) | |
Indigent/self‐pay | 2,829 (16%) | 42 (10%) | |
Other | 721 (4%) | 15 (4%) | |
Length of stay, d (SD) | 4.0 (3.5) | 39.5 (37.3) | <0.001 |
Discharge disposition | <0.001 | ||
Home with self‐care | 13,276 (74%) | 115 (28%) | |
Home with home health | 1,584 (9%) | 79 (19%) | |
Hospicehome or inpatient | 369 (2%) | 19 (5%) | |
Postacute‐care facility or LTAC | 1,761 (10%) | 141 (34%) | |
Expired | 113 (1%) | 18 (4%) | |
Other | 844 (5%) | 44 (11%) | |
No. of admission medications (SD) | 9.7 (7.4) | 10.9 (7.8) | 0.002 |
Primary diagnosis by ICD‐9 code* | |||
Sepsis, unspecified | 1,548 (9%) | 55 (13%) | 0.001 |
Acute respiratory failure | 293 (2%) | 9 (2%) | 0.400 |
MSSA septicemia | 36 (0.2%) | 8 (2%) | <0.001 |
MRSA septicemia | 13 (0.1%) | 7 (2%) | <0.001 |
Alcoholic cirrhosis of the liver | 111 (1%) | 7 (2%) | 0.007 |
Palliative care consult | 398 (2%) | 64 (15%) | <0.001 |
ICU stay | 2,030 (11%) | 246 (59%) | <0.001 |
Surgical procedure | 1,800 (10%) | 182 (44%) | <0.001 |
Unspecified sepsis was the most frequent primary diagnosis, regardless of LOS category (Table 2). However, the second through fifth most frequent diagnoses differed for patients with and without prolonged LOS.
N | % | |
---|---|---|
| ||
LOS 21 days | ||
1. Sepsis, unspecified | 1,548 | 8.6% |
2. Acute pancreatitis | 435 | 2.4% |
3. Pneumonia | 431 | 2.4% |
4. Acute kidney failure | 363 | 2.0% |
5. COPD exacerbation | 320 | 1.8% |
LOS >21 days | ||
1. Sepsis, unspecified | 55 | 13.0% |
2. Acute respiratory failure | 9 | 2.2% |
3. Methicillin‐sensitive Staphylococcus aureus septicemia | 8 | 1.9% |
4. Methicillin‐resistant Staphylococcus aureus septicemia | 7 | 1.7% |
5. Alcoholic cirrhosis of the liver | 7 | 1.7% |
In an adjusted logistic regression model (Table 3), we found lower odds of prolonged LOS for each 10‐year increase in age and higher odds of prolonged LOS for Medicaid insurance, discharge to home with home health, discharge to a postacute‐care or long‐term acute‐care facility, and in‐hospital death. Methicillin‐resistant Staphylococcus aureus (MRSA) septicemia, palliative care consults, ICU stays, and surgeries were all also associated with increased odds of prolonged LOS. We identified a statistically significant interaction between ICU stay and surgical procedure (odds ratio: 2.53, 95% confidence interval: 1.51‐4.26, P<0.001).
Outcome: LOS >21 Days | Odds Ratio | 95% CI | P Value |
---|---|---|---|
| |||
Age, per 10 years increase in age | 0.80 | 0.73‐0.87 | <0.001 |
Year of discharge | |||
2012 | 0.47 | 0.34‐0.67 | <0.001 |
2013 | 1.10 | 0.84‐1.43 | 0.493 |
2014 | Ref | ||
Race/ethnicity | |||
White non‐Hispanic | Ref | ||
Black non‐Hispanic | 0.89 | 0.64‐1.22 | 0.454 |
Hispanic | 1.01 | 0.70‐1.46 | 0.952 |
Asian | 0.85 | 0.40‐1.83 | 0.679 |
Other or unknown | 1.29 | 0.73‐2.26 | 0.378 |
Insurance | |||
Medicare | Ref | ||
Medicaid | 1.99 | 1.29‐3.05 | 0.002 |
Dual | 1.06 | 0.72‐1.57 | 0.765 |
Private | 1.13 | 0.70‐1.82 | 0.620 |
Indigent/self‐pay | 1.66 | 0.95‐2.88 | 0.073 |
Other | 0.96 | 0.47‐1.96 | 0.908 |
Discharge disposition | |||
Home with self‐care | Ref | ||
Home with home health | 4.48 | 3.10‐6.48 | <0.001 |
Hospicehome or inpatient | 2.11 | 0.98‐4.55 | 0.057 |
Postacute‐care facility or LTAC | 10.37 | 6.92‐15.56 | <0.001 |
Expired | 5.38 | 2.27‐12.75 | <0.001 |
Other | 4.04 | 2.64‐6.18 | <0.001 |
No. of admission medications | 1.00 | 0.99‐1.02 | 0.775 |
Primary diagnosis by ICD‐9 code | |||
Sepsis, unspecified | 1.11 | 0.78‐1.58 | 0.575 |
MSSA septicemia | 2.44 | 0.68‐8.67 | 0.074 |
MRSA septicemia | 8.83 | 1.72‐45.36 | 0.009 |
Alcoholic cirrhosis of the liver | 1.25 | 0.43‐3.65 | 0.687 |
Palliative care consult | 4.63 | 2.86‐7.49 | <0.001 |
ICU stay | 6.66 | 5.22‐8.50 | <0.001 |
Surgical procedure | 5.04 | 3.90‐6.52 | <0.001 |
Sensitivity analyses using LOS >14 and >30 days yielded similar results to LOS >21 days (see Supporting Appendix Table 1 in the online version of this article).
DISCUSSION
We found that a small proportion of medicine patients with prolonged hospitalizations contributed substantially to both total inpatient days and average inpatient LOS. Such disproportionate healthcare utilization is concerning in light of the Institute of Medicine's charge for health systems to deliver timely, efficient, and equitable care.[11]
Few studies in the United States have analyzed patient characteristics that predict prolonged LOS, and to our knowledge, none have evaluated prolonged LOS specifically in general medicine patients.[12, 13, 14] Among selected surgical populations, prolonged hospitalizations are most often related to placement difficulties, operational delays, and payer‐related issues, rather than severity of illness, baseline comorbidities, or in‐hospital complications.[12, 13] In our study, we found that patients with prolonged LOS were more likely to require a palliative care consult, ICU stay, or surgery, all proxies for disease severity. Patients with prolonged LOS were also more likely to undergo surgery >24 hours after admission than those without prolonged LOS, suggesting that the former were either too unstable to proceed directly to surgery or developed complications later during their hospitalization. Even after controlling for palliative care consults, ICU stays, and surgeries, placement at a postacute‐care facility was strongly associated with prolonged LOS. Patients with prolonged LOS were also more likely to have Medicaid compared to other insurance types.
Our findings have several potential implications for efforts aimed at decreasing the number of and length of prolonged hospitalizations. Although demographic and clinical factors such as Medicaid insurance, ICU stays, and surgeries are generally not modifiable, they could, particularly in combination, be used to trigger earlier and more intensive case management involvement. A streamlined insurance approval process for Medicaid pending inpatients could be beneficial, given the recent expansion of Medicaid eligibility under the ACA. Hospital partnerships with postacute‐care facilities could also relieve bottlenecks in placement.[8] Chart review of the patients with MRSA septicemia and prolonged LOS indicated that development of an intensive outpatient parenteral antimicrobial therapy pathway with substance abuse counseling could provide an alternative to extended inpatient treatment for intravenous drug users with complicated infections.[15]
This study has several limitations. First, given the lack of consensus in the literature regarding the definition of a prolonged hospitalization, it is difficult to directly compare our results with existing studies.[8, 12, 13, 14] However, we believe LOS >21 days to be a meaningful cutoff for our cohort. Most demographic and clinical variables that were predictive at >21 days were also predictive at >14 and >30 days, which reassured us that the relationship between variables and prolonged LOS was stable at different thresholds. Second, our database did not allow us to fully adjust for baseline comorbidities or categorize the reasons for discharge delays. Finally, this was a single‐center program evaluation. Although this limits generalization to other institutions, we believe our approach may serve as a guide for others interested in reducing prolonged hospitalizations.
In summary, prolonged hospitalizations represent a potentially high‐yield target for LOS reduction efforts. Prolonged hospitalizations among medicine patients at our institution particularly affected Medicaid enrollees with complex hospital stays who were not discharged home. Further studies are needed to determine the specific reasons for unnecessary hospital days in this population.
Acknowledgements
The authors thank Essey Yirdaw for her contributions to the building and management of the database that informed this work.
Disclosure: M.E.A. conceived of the study concept and design and drafted the manuscript. J.J.G. assisted with the study design and made critical revisions to the final manuscript. D.A., R.P., and R.C. assisted with the study design and made critical revisions to the manuscript. C.D.J. assisted with the study design, performed data analyses, and made critical revisions to the manuscript. RC has disclosed that her time is funded by a National Institutes of Health grant unrelated to this study. A modified abstract was presented in poster format at the Society of Hospital Medicine Research, Innovations, and Vignettes Competition 2015 Annual Meeting, held March 29 April 1, 2015, in National Harbor, Maryland. The authors have no conflicts of interest to disclose.
- Excess hospitalization days in an academic medical center: perceptions of hospitalists and discharge planners. Am J Manag Care. 2011;17(2):e34–e42. , , , et al.
- A prospective study of reasons for prolonged hospitalizations on a general medicine teaching service. J Gen Intern Med. 2005;20(2):108–115. , ,
- Adoption of electronic health records grows rapidly, but fewer than half of U.S. hospitals had at least a basic system in 2012. Health Aff (Millwood). 2013;32(8):1478–1485. , , , et al.
- Observation and inpatient status: clinical impact of the 2‐midnight rule. J Hosp Med. 2014;9(4):203–209. , , , et al.
- Impact of insurance expansion on hospital uncompensated care costs in 2014. Department of Health and Human Services Office of the Assistant Secretary for Planning and Evaluation. Available at: http://aspe.hhs.gov/health/reports/2014/uncompensatedcare/ib_uncompensatedcare.pdf. Accessed March 28, 2015. , ,
- Using real‐time demand capacity management to improve hospital‐wide patient flow. Jt Comm J Qual Patient Saf. 2011;37(5):217–227. , , ,
- Natural history of late discharges from a general medical ward. J Hosp Med. 2009;4(4):226–233. , , , , ,
- Addressing hospital length of stay outlier patients: a community‐wide approach. Adv Biosci Biotechol. 2014;5:188–196. , , ,
- American medical home runs. Health Aff (Millwood). 2009;28(5):1317–1326. ,
- The hot spotters: can we lower medical costs by giving the neediest patients better care? New Yorker. January 2011:40–51.
- Institute of Medicine. Crossing the Quality Chasm. Washington, DC: National Academies Press; 2001.
- Excessively long hospital stays after trauma are not related to the severity of illness: let's aim to the right target! JAMA Surg. 2013;148(1):956–961. , , , et al.
- Extended length of stay after surgery: complications, inefficient practice, or sick patients? JAMA Surg. 2014;149(8):815–820. , ,
- Nonmedical factors associated with prolonged hospital length of stay in an urban homebound population. J Hosp Med. 2012;7(2):73–78. , , , ,
- Safe and successful treatment of intravenous drug users with a peripherally inserted central catheter in an outpatient parenteral antibiotic treatment service. J Antimicrob Chemother. 2010;65(12):2641–2644. , , ,
- Excess hospitalization days in an academic medical center: perceptions of hospitalists and discharge planners. Am J Manag Care. 2011;17(2):e34–e42. , , , et al.
- A prospective study of reasons for prolonged hospitalizations on a general medicine teaching service. J Gen Intern Med. 2005;20(2):108–115. , ,
- Adoption of electronic health records grows rapidly, but fewer than half of U.S. hospitals had at least a basic system in 2012. Health Aff (Millwood). 2013;32(8):1478–1485. , , , et al.
- Observation and inpatient status: clinical impact of the 2‐midnight rule. J Hosp Med. 2014;9(4):203–209. , , , et al.
- Impact of insurance expansion on hospital uncompensated care costs in 2014. Department of Health and Human Services Office of the Assistant Secretary for Planning and Evaluation. Available at: http://aspe.hhs.gov/health/reports/2014/uncompensatedcare/ib_uncompensatedcare.pdf. Accessed March 28, 2015. , ,
- Using real‐time demand capacity management to improve hospital‐wide patient flow. Jt Comm J Qual Patient Saf. 2011;37(5):217–227. , , ,
- Natural history of late discharges from a general medical ward. J Hosp Med. 2009;4(4):226–233. , , , , ,
- Addressing hospital length of stay outlier patients: a community‐wide approach. Adv Biosci Biotechol. 2014;5:188–196. , , ,
- American medical home runs. Health Aff (Millwood). 2009;28(5):1317–1326. ,
- The hot spotters: can we lower medical costs by giving the neediest patients better care? New Yorker. January 2011:40–51.
- Institute of Medicine. Crossing the Quality Chasm. Washington, DC: National Academies Press; 2001.
- Excessively long hospital stays after trauma are not related to the severity of illness: let's aim to the right target! JAMA Surg. 2013;148(1):956–961. , , , et al.
- Extended length of stay after surgery: complications, inefficient practice, or sick patients? JAMA Surg. 2014;149(8):815–820. , ,
- Nonmedical factors associated with prolonged hospital length of stay in an urban homebound population. J Hosp Med. 2012;7(2):73–78. , , , ,
- Safe and successful treatment of intravenous drug users with a peripherally inserted central catheter in an outpatient parenteral antibiotic treatment service. J Antimicrob Chemother. 2010;65(12):2641–2644. , , ,
Handoffs
In this issue of the Journal of Hospital Medicine, the results of 2 inpatient handoff studies further shape our evolving understanding of in‐hospital care transitions. Schouten and colleagues,[1] report no difference in adverse outcomes when admissions were handed off to the primary team using face‐to‐face compared to nonface‐to‐face interactions. Meanwhile, Hanson and colleagues[2] report that a written handoff tool is used infrequently by covering interns.
Schouten et al.'s study attempted to isolate the impact of the verbal portion of the handoff between admitting and accepting team by evaluating whether early adverse outcomes differed between patients whose teams performed a face‐to‐face handoffs compared to those who did not. Their study was a retrospective chart review, and no additional process changes, training, or instruction regarding handoffs were implemented or measured. Handoffs occurred primarily between advanced practice providers, hospitalists, and a small number of resident physicians, so generalizability of this study to other institutions may be limited. No difference in adverse events was noted between admissions with face‐to‐face compared to those without face‐to‐face handoffs (2.6% vs 3.2%). Unfortunately, this study was likely underpowered to detect significant changes in adverse events, with a sample size of 805 total patients with a 3% baseline rate of adverse events (by our estimate, over 5000 patients would be needed in each group10,000 overallto detect a 30% relative difference in event rates). Further, this study did not examine other outcomes that could be impacted by the handoff process such as provider efficiency or patient experience.
Face‐to‐face handoffs, the gold standard for handoffs between providers, was 1 of the sign‐out approaches examined in a study by Graham and colleagues.[3] This study, in contrast to the Schouten et al. study, prospectively evaluated adverse events before and after implementation of face‐to‐face handoffs, with structured written sign‐out from the primary team to nighttime covering physicians. Prior to implementation, handoffs consisted of a double handoff involving an intermediary physician and unstructured written sign‐out. Although no statistically significant reduction in adverse events was found in the Graham et al. study, significant improvements were noted in physician satisfaction, documentation of key elements in handoffs, and reduced data omissions; importantly, a trend of fewer near misses was noted comparing the pre‐ and postintervention periods. Although the Schouten et al. and Graham et al. studies suggest questionable benefit of face‐to‐face handoffs, we would caution that limitations in sample size and methodological sensitivity to detect adverse events in both studies could explain the lack of association between face‐to‐face handoffs and reduced adverse events. Furthermore, the promising findings of fewer data omissions and near misses in the intervention group in the Graham et al. study suggest benefit from a multipronged approach to improving handoffs including both face‐to‐face interactions and a structured written component.
In this issue, Hanson and colleagues also evaluated the use of a handoff tool by cross‐covering interns in a convenience sample of overnight clinical interactions. Despite finding that standard written documentation was considered beneficial by nearly all respondents (94.3%), the interns reported that the handoff tool was used in only 27.7% of encounters. This pales in comparison to the use of the nurse or chart in 94.4% of cross‐coverage encounters. The authors speculate that a handoff tool, for many years the only timely source of information, may not be as useful when information can be easily accessed in an electronic health record. Yet, in a prior systematic review that included 6 studies of computerized handoff tools, Li and colleagues found that computerized handoff tools may improve physician efficiency, enhance the completeness of handoff information, and even potentially reduce adverse events.[4]
The Schouten et al. and Hanson et al. studies raise important questions for the fields of hospital medicine and patient safety. Is it time to do away with the written and verbal portions of the handoff process? Should the handoff of patients simply consist of transferring a list of patients to covering providers? We do not believe this is the correct course of action. Rather, we recommend a more evolutionary, not revolutionary, interpretation of these results, especially when considered as part of a broader story of in‐hospital transitions of care.
For example, a recently published evaluation of a resident handoff‐improvement program in 9 hospitals and 10,740 patient admissions by Starmer and collegues[5] focused on a handoff bundle, I‐PASS, which is a pneumonic for Illness severity, Patient summary, Action items, Situation awareness and contingency planning, Synthesis by receiver. The authors report a reduction in medical errors and preventable adverse events without significant increases in the duration of oral handoff per patient. The handoff in this study included both oral and written elements in the I‐PASS format. Implementation was multipronged, and the I‐PASS bundle included (1) use of the I‐PASS pneumonic to standardize handoffs; (2) resident physician training in handoffs and communication through a 2‐hour workshop, followed by a 1‐hour role‐playing and simulation session, and a computer module for practice; (3) faculty development and observation with use of direct‐observation tools to provide structured feedback to residents; (4) active surveillance for errors (rather than relying on self‐report); and (5) a sustainability campaign to promote continuation of culture change. The complexity and robust nature of the I‐PASS handoff bundle suggests that having multiple structured components included in a handoff program with active, rather than retrospective, evaluation might increase the likelihood of improved, sustained outcomes. In addition, one might also conclude from the Starmer et al. study that it takes commitment from all levels, including residents, faculty, and administration, to improve handoffs between teams for inpatient care.
We commend Schouten et al. and Hanson et al. on their contributions to the literature, but believe that the story of the in‐hospital handoff has yet to be fully written. Although results from these 2 articles may cause speculation about the value of oral and written handoffs, we believe that the balance of evidence favors the use of a multipronged approach that involves both structured oral and written handoffs to improve the value and efficiency of handoffs. In addition, findings from the I‐PASS study support dedicated handoff training for providers, evaluation of handoffs using structured tools, and active surveillance for medical errors. Future areas of work should include a systematic review of the inpatient handoff literature and further evaluation of precisely which specific intervention components (eg, structured content of handoffs, sensemaking content) or modes of delivery (eg, face‐to‐face vs other) are most likely to reduce medical errors and improve patient outcomes. As the hospital medicine movement continues to grow, handoffs will continue to be paramount. Establishing the safest method to complete handoffs to promote patient safety should be a common goal for hospitalists.
The handoff story is still in evolution; as hospitalists, we are poised to be its author.
- Association of Face‐to‐Face Handoffs and Outcomes of Hospitalized Internal Medicine Patients. J Hosp Med. 2015;10(3):137–141. , , , et al.
- Nighttime Clinical Encounters: How Residents Perceive and Respond to Calls at Night. J Hosp Med. 2015;10(3):142–146. , , , et al.
- Effect of a systems intervention on the quality and safety of patient handoffs in an internal medicine residency program. J Gen Intern Med. 2013;28(8):986–993. , , , , , .
- Review of computerized physician handoff tools for improving the quality of patient care. J Hosp Med. 2013;8(8):456–463. , , , , .
- Changes in medical errors after implementation of a handoff program. N Engl J Med. 2014;371(19):1803–1812. , , , et al.
In this issue of the Journal of Hospital Medicine, the results of 2 inpatient handoff studies further shape our evolving understanding of in‐hospital care transitions. Schouten and colleagues,[1] report no difference in adverse outcomes when admissions were handed off to the primary team using face‐to‐face compared to nonface‐to‐face interactions. Meanwhile, Hanson and colleagues[2] report that a written handoff tool is used infrequently by covering interns.
Schouten et al.'s study attempted to isolate the impact of the verbal portion of the handoff between admitting and accepting team by evaluating whether early adverse outcomes differed between patients whose teams performed a face‐to‐face handoffs compared to those who did not. Their study was a retrospective chart review, and no additional process changes, training, or instruction regarding handoffs were implemented or measured. Handoffs occurred primarily between advanced practice providers, hospitalists, and a small number of resident physicians, so generalizability of this study to other institutions may be limited. No difference in adverse events was noted between admissions with face‐to‐face compared to those without face‐to‐face handoffs (2.6% vs 3.2%). Unfortunately, this study was likely underpowered to detect significant changes in adverse events, with a sample size of 805 total patients with a 3% baseline rate of adverse events (by our estimate, over 5000 patients would be needed in each group10,000 overallto detect a 30% relative difference in event rates). Further, this study did not examine other outcomes that could be impacted by the handoff process such as provider efficiency or patient experience.
Face‐to‐face handoffs, the gold standard for handoffs between providers, was 1 of the sign‐out approaches examined in a study by Graham and colleagues.[3] This study, in contrast to the Schouten et al. study, prospectively evaluated adverse events before and after implementation of face‐to‐face handoffs, with structured written sign‐out from the primary team to nighttime covering physicians. Prior to implementation, handoffs consisted of a double handoff involving an intermediary physician and unstructured written sign‐out. Although no statistically significant reduction in adverse events was found in the Graham et al. study, significant improvements were noted in physician satisfaction, documentation of key elements in handoffs, and reduced data omissions; importantly, a trend of fewer near misses was noted comparing the pre‐ and postintervention periods. Although the Schouten et al. and Graham et al. studies suggest questionable benefit of face‐to‐face handoffs, we would caution that limitations in sample size and methodological sensitivity to detect adverse events in both studies could explain the lack of association between face‐to‐face handoffs and reduced adverse events. Furthermore, the promising findings of fewer data omissions and near misses in the intervention group in the Graham et al. study suggest benefit from a multipronged approach to improving handoffs including both face‐to‐face interactions and a structured written component.
In this issue, Hanson and colleagues also evaluated the use of a handoff tool by cross‐covering interns in a convenience sample of overnight clinical interactions. Despite finding that standard written documentation was considered beneficial by nearly all respondents (94.3%), the interns reported that the handoff tool was used in only 27.7% of encounters. This pales in comparison to the use of the nurse or chart in 94.4% of cross‐coverage encounters. The authors speculate that a handoff tool, for many years the only timely source of information, may not be as useful when information can be easily accessed in an electronic health record. Yet, in a prior systematic review that included 6 studies of computerized handoff tools, Li and colleagues found that computerized handoff tools may improve physician efficiency, enhance the completeness of handoff information, and even potentially reduce adverse events.[4]
The Schouten et al. and Hanson et al. studies raise important questions for the fields of hospital medicine and patient safety. Is it time to do away with the written and verbal portions of the handoff process? Should the handoff of patients simply consist of transferring a list of patients to covering providers? We do not believe this is the correct course of action. Rather, we recommend a more evolutionary, not revolutionary, interpretation of these results, especially when considered as part of a broader story of in‐hospital transitions of care.
For example, a recently published evaluation of a resident handoff‐improvement program in 9 hospitals and 10,740 patient admissions by Starmer and collegues[5] focused on a handoff bundle, I‐PASS, which is a pneumonic for Illness severity, Patient summary, Action items, Situation awareness and contingency planning, Synthesis by receiver. The authors report a reduction in medical errors and preventable adverse events without significant increases in the duration of oral handoff per patient. The handoff in this study included both oral and written elements in the I‐PASS format. Implementation was multipronged, and the I‐PASS bundle included (1) use of the I‐PASS pneumonic to standardize handoffs; (2) resident physician training in handoffs and communication through a 2‐hour workshop, followed by a 1‐hour role‐playing and simulation session, and a computer module for practice; (3) faculty development and observation with use of direct‐observation tools to provide structured feedback to residents; (4) active surveillance for errors (rather than relying on self‐report); and (5) a sustainability campaign to promote continuation of culture change. The complexity and robust nature of the I‐PASS handoff bundle suggests that having multiple structured components included in a handoff program with active, rather than retrospective, evaluation might increase the likelihood of improved, sustained outcomes. In addition, one might also conclude from the Starmer et al. study that it takes commitment from all levels, including residents, faculty, and administration, to improve handoffs between teams for inpatient care.
We commend Schouten et al. and Hanson et al. on their contributions to the literature, but believe that the story of the in‐hospital handoff has yet to be fully written. Although results from these 2 articles may cause speculation about the value of oral and written handoffs, we believe that the balance of evidence favors the use of a multipronged approach that involves both structured oral and written handoffs to improve the value and efficiency of handoffs. In addition, findings from the I‐PASS study support dedicated handoff training for providers, evaluation of handoffs using structured tools, and active surveillance for medical errors. Future areas of work should include a systematic review of the inpatient handoff literature and further evaluation of precisely which specific intervention components (eg, structured content of handoffs, sensemaking content) or modes of delivery (eg, face‐to‐face vs other) are most likely to reduce medical errors and improve patient outcomes. As the hospital medicine movement continues to grow, handoffs will continue to be paramount. Establishing the safest method to complete handoffs to promote patient safety should be a common goal for hospitalists.
The handoff story is still in evolution; as hospitalists, we are poised to be its author.
In this issue of the Journal of Hospital Medicine, the results of 2 inpatient handoff studies further shape our evolving understanding of in‐hospital care transitions. Schouten and colleagues,[1] report no difference in adverse outcomes when admissions were handed off to the primary team using face‐to‐face compared to nonface‐to‐face interactions. Meanwhile, Hanson and colleagues[2] report that a written handoff tool is used infrequently by covering interns.
Schouten et al.'s study attempted to isolate the impact of the verbal portion of the handoff between admitting and accepting team by evaluating whether early adverse outcomes differed between patients whose teams performed a face‐to‐face handoffs compared to those who did not. Their study was a retrospective chart review, and no additional process changes, training, or instruction regarding handoffs were implemented or measured. Handoffs occurred primarily between advanced practice providers, hospitalists, and a small number of resident physicians, so generalizability of this study to other institutions may be limited. No difference in adverse events was noted between admissions with face‐to‐face compared to those without face‐to‐face handoffs (2.6% vs 3.2%). Unfortunately, this study was likely underpowered to detect significant changes in adverse events, with a sample size of 805 total patients with a 3% baseline rate of adverse events (by our estimate, over 5000 patients would be needed in each group10,000 overallto detect a 30% relative difference in event rates). Further, this study did not examine other outcomes that could be impacted by the handoff process such as provider efficiency or patient experience.
Face‐to‐face handoffs, the gold standard for handoffs between providers, was 1 of the sign‐out approaches examined in a study by Graham and colleagues.[3] This study, in contrast to the Schouten et al. study, prospectively evaluated adverse events before and after implementation of face‐to‐face handoffs, with structured written sign‐out from the primary team to nighttime covering physicians. Prior to implementation, handoffs consisted of a double handoff involving an intermediary physician and unstructured written sign‐out. Although no statistically significant reduction in adverse events was found in the Graham et al. study, significant improvements were noted in physician satisfaction, documentation of key elements in handoffs, and reduced data omissions; importantly, a trend of fewer near misses was noted comparing the pre‐ and postintervention periods. Although the Schouten et al. and Graham et al. studies suggest questionable benefit of face‐to‐face handoffs, we would caution that limitations in sample size and methodological sensitivity to detect adverse events in both studies could explain the lack of association between face‐to‐face handoffs and reduced adverse events. Furthermore, the promising findings of fewer data omissions and near misses in the intervention group in the Graham et al. study suggest benefit from a multipronged approach to improving handoffs including both face‐to‐face interactions and a structured written component.
In this issue, Hanson and colleagues also evaluated the use of a handoff tool by cross‐covering interns in a convenience sample of overnight clinical interactions. Despite finding that standard written documentation was considered beneficial by nearly all respondents (94.3%), the interns reported that the handoff tool was used in only 27.7% of encounters. This pales in comparison to the use of the nurse or chart in 94.4% of cross‐coverage encounters. The authors speculate that a handoff tool, for many years the only timely source of information, may not be as useful when information can be easily accessed in an electronic health record. Yet, in a prior systematic review that included 6 studies of computerized handoff tools, Li and colleagues found that computerized handoff tools may improve physician efficiency, enhance the completeness of handoff information, and even potentially reduce adverse events.[4]
The Schouten et al. and Hanson et al. studies raise important questions for the fields of hospital medicine and patient safety. Is it time to do away with the written and verbal portions of the handoff process? Should the handoff of patients simply consist of transferring a list of patients to covering providers? We do not believe this is the correct course of action. Rather, we recommend a more evolutionary, not revolutionary, interpretation of these results, especially when considered as part of a broader story of in‐hospital transitions of care.
For example, a recently published evaluation of a resident handoff‐improvement program in 9 hospitals and 10,740 patient admissions by Starmer and collegues[5] focused on a handoff bundle, I‐PASS, which is a pneumonic for Illness severity, Patient summary, Action items, Situation awareness and contingency planning, Synthesis by receiver. The authors report a reduction in medical errors and preventable adverse events without significant increases in the duration of oral handoff per patient. The handoff in this study included both oral and written elements in the I‐PASS format. Implementation was multipronged, and the I‐PASS bundle included (1) use of the I‐PASS pneumonic to standardize handoffs; (2) resident physician training in handoffs and communication through a 2‐hour workshop, followed by a 1‐hour role‐playing and simulation session, and a computer module for practice; (3) faculty development and observation with use of direct‐observation tools to provide structured feedback to residents; (4) active surveillance for errors (rather than relying on self‐report); and (5) a sustainability campaign to promote continuation of culture change. The complexity and robust nature of the I‐PASS handoff bundle suggests that having multiple structured components included in a handoff program with active, rather than retrospective, evaluation might increase the likelihood of improved, sustained outcomes. In addition, one might also conclude from the Starmer et al. study that it takes commitment from all levels, including residents, faculty, and administration, to improve handoffs between teams for inpatient care.
We commend Schouten et al. and Hanson et al. on their contributions to the literature, but believe that the story of the in‐hospital handoff has yet to be fully written. Although results from these 2 articles may cause speculation about the value of oral and written handoffs, we believe that the balance of evidence favors the use of a multipronged approach that involves both structured oral and written handoffs to improve the value and efficiency of handoffs. In addition, findings from the I‐PASS study support dedicated handoff training for providers, evaluation of handoffs using structured tools, and active surveillance for medical errors. Future areas of work should include a systematic review of the inpatient handoff literature and further evaluation of precisely which specific intervention components (eg, structured content of handoffs, sensemaking content) or modes of delivery (eg, face‐to‐face vs other) are most likely to reduce medical errors and improve patient outcomes. As the hospital medicine movement continues to grow, handoffs will continue to be paramount. Establishing the safest method to complete handoffs to promote patient safety should be a common goal for hospitalists.
The handoff story is still in evolution; as hospitalists, we are poised to be its author.
- Association of Face‐to‐Face Handoffs and Outcomes of Hospitalized Internal Medicine Patients. J Hosp Med. 2015;10(3):137–141. , , , et al.
- Nighttime Clinical Encounters: How Residents Perceive and Respond to Calls at Night. J Hosp Med. 2015;10(3):142–146. , , , et al.
- Effect of a systems intervention on the quality and safety of patient handoffs in an internal medicine residency program. J Gen Intern Med. 2013;28(8):986–993. , , , , , .
- Review of computerized physician handoff tools for improving the quality of patient care. J Hosp Med. 2013;8(8):456–463. , , , , .
- Changes in medical errors after implementation of a handoff program. N Engl J Med. 2014;371(19):1803–1812. , , , et al.
- Association of Face‐to‐Face Handoffs and Outcomes of Hospitalized Internal Medicine Patients. J Hosp Med. 2015;10(3):137–141. , , , et al.
- Nighttime Clinical Encounters: How Residents Perceive and Respond to Calls at Night. J Hosp Med. 2015;10(3):142–146. , , , et al.
- Effect of a systems intervention on the quality and safety of patient handoffs in an internal medicine residency program. J Gen Intern Med. 2013;28(8):986–993. , , , , , .
- Review of computerized physician handoff tools for improving the quality of patient care. J Hosp Med. 2013;8(8):456–463. , , , , .
- Changes in medical errors after implementation of a handoff program. N Engl J Med. 2014;371(19):1803–1812. , , , et al.
Hospitalist‐Run Acute Care for Elderly
For the frail older patient, hospitalization marks a period of high risk of poor outcomes and adverse events including functional decline, delirium, pressure ulcers, adverse drug events, nosocomial infections, and falls.1, 2 Physician recognition of elderly patients at risk for adverse outcomes is poor, making it difficult to intervene to prevent them.3, 4 Among frail, elderly inpatients at an urban academic medical center, doctors documented cognitive assessments in only 5% of patients. Functional assessments are appropriately documented in 40%80% of inpatients.3, 5
The Acute Care for Elders (ACE) unit is one of several models of comprehensive inpatient geriatric care that have been developed by geriatrician researchers to address the adverse events and functional decline that often accompany hospitalization.6 The ACE unit model generally incorporates: 1) a modified hospital environment, 2) early assessment and intensive management to minimize the adverse effects of hospital care, 3) early discharge planning, 4) patient centered care protocols, and 5) a consistent nursing staff.7 Two randomized, controlled trials have shown the ACE unit model to be successful in reducing functional decline among frail older inpatients during and after hospitalization.7, 8 While meta‐analyses data also suggests the ACE unit model reduces functional decline and future institutionalization, significant impact on other outcomes is not proven.9, 10
Several barriers have prevented the successful dissemination of the ACE unit model. The chief limitations are the upfront resources required to create and maintain a modified, dedicated unit, as well as the lack of a geriatrics trained workforce.7, 1113 The rapid growth of hospital medicine presents opportunities for innovation in the care of older patients. Still, a 2006 census demonstrated that few hospitalist groups had identified geriatric care as a priority.14
In response to these challenges, the University of Colorado Hospital Medicine Group created a hospitalist‐run inpatient medical service designed for the care of the frail older patient. This Hospitalist‐Acute Care for the Elderly (Hospitalist‐ACE) unit is a hybrid of a general medical service and an inpatient geriatrics unit.7 The goals of the Hospitalist‐ACE service are to provide high quality care tailored to older inpatients, thus minimizing the risks of functional decline and adverse events associate with hospitalization, and to provide a clinical geriatrics teaching experience for Hospitalist Training Track Residents within the Internal Medicine Residency Training Program and medical students at the University of Colorado Denver School of Medicine. The Hospitalist‐ACE unit is staffed with a core group of hospitalist attendings who have, at a minimum, attended an intensive mini‐course in inpatient geriatrics. The service employs interdisciplinary rounds; a brief, standardized geriatric assessment including screens of function, cognition, and mood; a clinical focus on mitigating the hazards of hospitalization, early discharge planning; and a novel geriatric educational curriculum for medicine residents and medical students.
This article will: 1) describe the creation of the Hospitalist‐ACE service at the University of Colorado Hospital; and 2) summarize the evaluation of the Hospitalist‐ACE service in a quasi‐randomized, controlled manner during its first year. We hypothesized that, when compared to patients receiving usual care, patients cared for on the Hospitalist‐ACE service would have increased recognition of abnormal functional status; recognition of abnormal cognitive status and delirium; equivalent lengths of stay and hospital charges; and decreased falls, 30‐day readmissions, and restraint use.
METHODS
Design
We performed a quasi‐randomized, controlled study of the Hospitalist‐ACE service.
Setting
The study setting was the inpatient general medical services of the Anschutz Inpatient Pavilion (AIP) of the University of Colorado Hospital (UCH). The AIP is a 425‐bed tertiary care hospital that is the major teaching affiliate of the University of Colorado School of Medicine and a regional referral center. The control services, hereafter referred to as usual care, were comprised of the four inpatient general medicine teaching services that take admissions on a four‐day rotation (in general, two were staffed by outpatient general internists and medical subspecialists, and two were staffed by academic hospitalists). The Hospitalist‐ACE service was a novel hospitalist teaching service that began in July 2007. Hospitalist‐ACE patients were admitted to a single 12‐bed medical unit (12 West) when beds were available; 12 West is similar to the other medical/surgical units at UCH and did not have any modifications to the rooms, equipment, or common areas for the intervention. The nursing staff on this unit had no formal geriatric nursing training. The Hospitalist‐ACE team admitted patients daily (between 7 AM and 3 PM MondayFriday; between 7 AM and 12 noon Saturday and Sunday). Patients assigned to the Hospitalist‐ACE service after hours were admitted by the internal medicine resident on call for the usual care services and handed off to the Hospitalist‐ACE team at 7 AM the next morning.
Study Subjects
Eligible subjects were inpatients age 70 years admitted to the usual care or Hospitalist‐ACE services at the AIP from November 2, 2007 to April 15, 2008. All patients age 70 years were randomized to the Hospitalist‐ACE service or usual care on a general internal medicine service by the last digit of the medical record number (odd numbers admitted to the Hospitalist‐ACE service and even numbers admitted to usual care). Patients followed by the Hospitalist‐ACE service but not admitted to 12 West were included in the study. To isolate the impact of the intervention, patients admitted to a medicine subspecialty service (such as cardiology, pulmonary, or oncology), or transferred to or from the Hospitalist‐ACE or control services to another service (eg, intensive care unit [ICU] or orthopedic surgery service) were excluded from the study.
Intervention
The Hospitalist‐ACE unit implemented an interdisciplinary team approach to identify and address geriatric syndromes in patients aged 70 and over. The Hospitalist‐ACE model of care consisted of clinical care provided by a hospitalist attending with additional training in geriatric medicine, administration of standardized geriatric screens assessing function, cognition, and mood, 15 minute daily (MondayFriday) interdisciplinary rounds focusing on recognition and management of geriatric syndromes and early discharge planning, and a standardized educational curriculum for medical residents and medical students addressing hazards of hospitalization.
The Hospitalist‐ACE service was a unique rotation within the Hospitalist Training Track of the Internal Medicine Residency that was developed with the support of the University of Colorado Hospital and the Internal Medicine Residency Training Program, and input from the Geriatrics Division at the University of Colorado Denver. The director received additional training from the Donald W. Reynolds FoundationUCLA Faculty Development to Advance Geriatric Education Mini‐Fellowship for hospitalist faculty. The mission of the service was to excel at educating the next generation of hospitalists while providing a model for excellence of care for hospitalized elderly patients. Important stakeholders were identified, and a leadership teamincluding representatives from nursing, physical and occupational therapy, pharmacy, social work, case management, and later, volunteer servicescreated the model daily interdisciplinary rounds. As geographic concentration was essential for the viability of interdisciplinary rounds, one unit (12 West) within the hospital was designated as the preferred location for patients admitted to the Hospitalist‐ACE service.
The Hospitalist‐ACE unit team consisted of one attending hospitalist, one resident, one intern, and medical students. The attending was one of five hospitalists, with additional training in geriatric medicine, who rotated attending responsibilities on the service. One of the hospitalists was board certified in geriatric medicine. Each of the other four hospitalists attended the Reynolds FoundationUCLA mini‐fellowship in geriatric medicine. Hospitalist‐ACE attendings rotated on a variety of other hospitalist services throughout the academic year, including the usual care services.
The brief standardized geriatric assessment consisted of six validated instruments, and was completed by house staff or medical students on admission, following instruction by the attending physician. The complete assessment tool is shown in Figure 1. The cognitive items included the Mini‐Cog,15 a two‐item depression screen,16 and the Confusion Assessment Method.17 The functional items included the Vulnerable Elders Survey (VES‐13),18 the Timed Get Up and Go test,19 and a two‐question falls screen.20 The elements of the assessment tool were selected by the Hospitalist‐ACE attendings for brevity and the potential to inform clinical management. To standardize the clinical and educational approach, the Hospitalist‐ACE attendings regularly discussed appropriate orders recommended in response to each positive screen, but no templated order sets were used during the study period.
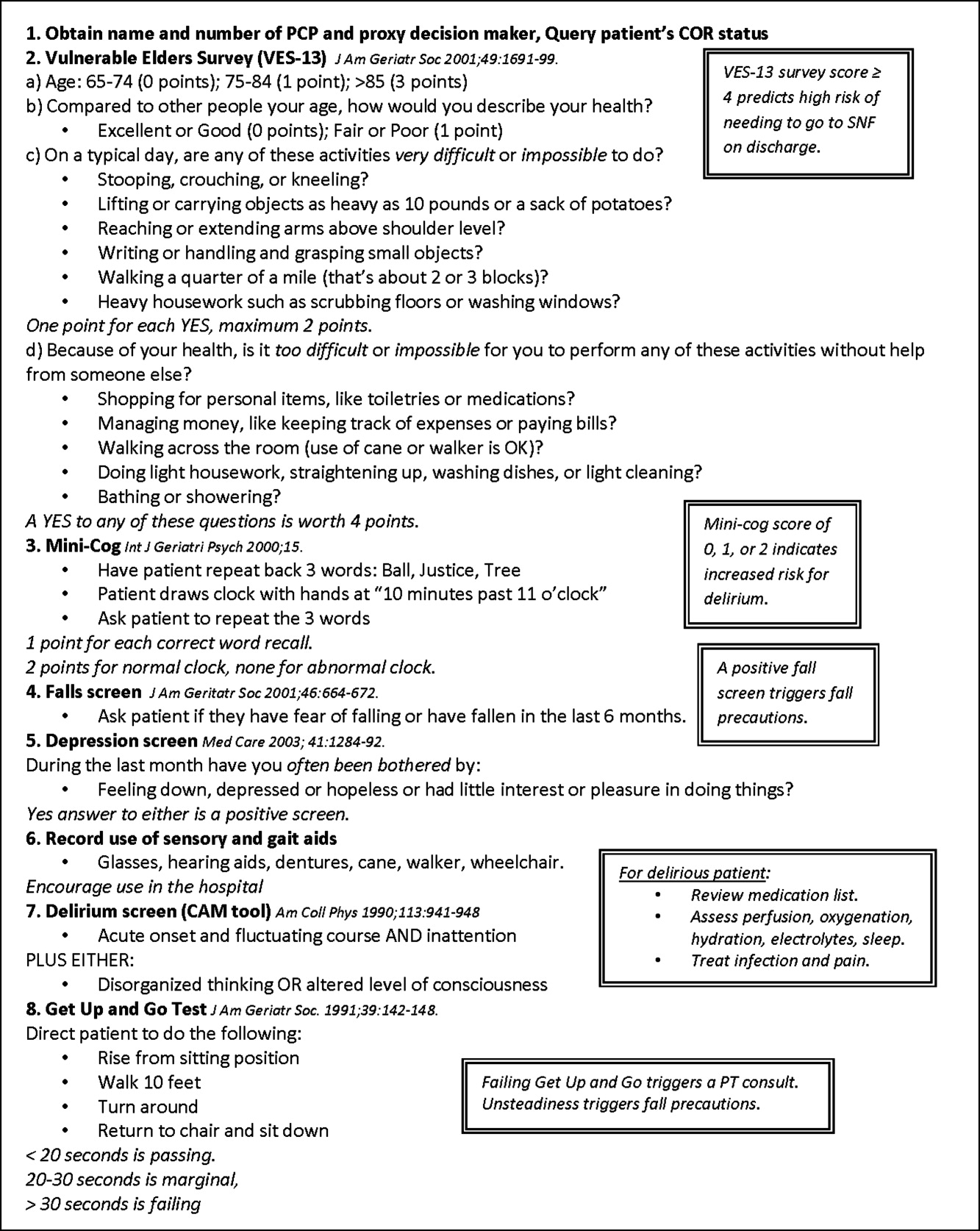
Interdisciplinary rounds were attended by Hospitalist‐ACE physicians, nurses, case managers, social workers, physical or occupational therapists, pharmacists, and volunteers. Rounds were led by the attending or medical resident.
The educational curriculum encompassed 13 modules created by the attending faculty that cover delirium, falls, dementia, pressure ulcers, physiology of aging, movement disorders, medication safety, end of life care, advance directives, care transitions, financing of health care for the elderly, and ethical conundrums in the care of the elderly. A full table of contents appears in online Appendix 1. Additionally, portions of the curriculum have been published online.21, 22 Topic selection was guided by the Accreditation Council for Graduate Medical Education (ACGME) core geriatrics topics determined most relevant for the inpatient setting. Formal instruction of 3045 minutes duration occurred three to four days a week and was presented in addition to routine internal medicine educational conferences. Attendings coordinated teaching to ensure that each trainee was exposed to all of the content during the course of their four‐week rotation.
In contrast to the Hospitalist‐ACE service, usual care on the control general medical services consisted of either a hospitalist, a general internist, or an internal medicine subspecialist attending physician, with one medical resident, one intern, and medical students admitting every fourth day. The general medical teams attended daily discharge planning rounds with a discharge planner and social worker focused exclusively on discharge planning. The content of teaching rounds on the general medical services was largely left to the discretion of the attending physician.
This program evaluation of the Hospitalist‐ACE service was granted a waiver of consent and Health Insurance Portability and Accountability Act (HIPAA) by the Colorado Multiple Institutional Review Board.
Measures
Primary Outcome
The primary outcome for the study was the recognition of abnormal functional status by the primary team. Recognition of abnormal functional status was determined from chart review and consisted of both the physician's detection of abnormal functional status and evidence of a corresponding treatment plan identified in the notes or orders of a physician member of the primary team (Table 1).
Measure | Criterion | Source | Content Examples |
---|---|---|---|
| |||
Recognition of abnormal functional status* | 1) Detection | MD's documentation of history | Presentation with change in function (new gait instability); use of gait aides (wheelchair) |
OR | |||
MD's documentation of physical exam | Observation of abnormal gait (eg, unsteady, wide‐based, shuffling) and/or balance Abnormal Get Up and Go test | ||
AND | |||
2) Treatment | MD's order | PT/OT consult; home safety evaluation | |
OR | |||
MD's documentation assessment/plan | Inclusion of functional status (rehabilitation, PT/OT needs) on the MD's problem list | ||
Recognition of abnormal cognitive status | Any of the following: | ||
Delirium | 1) Detection | MD's history | Presentation of confusion or altered mental status |
OR | |||
MD's physical exam | Abnormal confusion assessment method | ||
AND | |||
2) Treatment | MD's order | Sitter, reorienting communication, new halperidol order | |
OR | |||
MD's documentation of assessment/plan | Inclusion of delirium on the problem list | ||
OR | |||
Dementia | 1) Detection | MD's history | Dementia in medical history |
OR | OR | ||
MD's physical exam | Abnormal Folstein Mini‐Mental Status Exam or Mini‐Cog | ||
AND | |||
2) Treatment | MD's order | Cholinesterase inhibitor ordered | |
OR | OR | ||
MD's documentation of assessment/plan | Inclusion of dementia on the problem list | ||
OR | |||
Depression | 1) Detection | MD's history | Depression in medical history |
OR | OR | ||
MD's physical exam | Positive depression screen | ||
AND | |||
2) Treatment | MD's order | New antidepressant order | |
OR | |||
MD's documentation of assessment/plan | Inclusion of depression on the problem list |
Secondary Outcomes
Recognition of abnormal cognitive status was determined from chart review and consisted of both the physician's detection of dementia, depression, or delirium, and evidence of a corresponding treatment plan for any of the documented conditions identified in the notes or orders of a physician member of the primary team (Table 1). Additionally, we measured recognition and treatment of delirium alone.
Falls were determined from mandatory event reporting collected by the hospital on the University Hospitals Consortium Patient Safety Net web‐based reporting system and based on clinical assessment as reported by the nursing staff. The reports are validated by the appropriate clinical managers within 45 days of the event according to standard procedure.
Physical restraint use (type of restraint and duration) was determined from query of mandatory clinical documentation in the electronic medical record. Use of sleep aids was determined from review of the physician's order sheets in the medical record. The chart review captured any of 39 commonly prescribed hypnotic medications ordered at hour of sleep or for insomnia. The sleep medication list was compiled with the assistance of a pharmacist for an earlier chart review and included non‐benzodiazepine hypnotics, benzodiazepines, antidepressants, antihistamines, and antipsychotics.23
Length of stay, hospital charges, 30‐day readmissions to UCH (calculated from date of discharge), and discharge location were determined from administrative data.
Additional Descriptive Variables
Name, medical record number, gender, date of birth, date of admission and discharge, and primary diagnosis were obtained from the medical record. The Case Mix Index for each group of patients was determined from the average Medicare Severity‐adjusted Diagnosis Related Group (MS‐DRG) weight obtained from administrative data.
Data Collection
A two‐step, retrospective chart abstraction was employed. A professional research assistant (P.R.A.) hand‐abstracted process measures from the paper medical chart onto a data collection form designed for this study. A physician investigator performed a secondary review (H.L.W.). Discrepancies were resolved by the physician reviewer.
Data Analysis
Descriptive statistics were performed on intervention and control subjects. Means and standard deviations (age) or frequencies (gender, primary diagnoses) were calculated as appropriate. T tests were used for continuous variables, chi‐square tests for gender, and the Wilcoxon rank sum test for categorical variables.
Outcomes were reported as means and standard deviations for continuous variables (length of stay and charges) and frequencies for categorical variables (all other outcomes). T tests were used for continuous variables, Fisher's exact test for restraint use, and chi‐square tests were used for categorical variable to compare the impact of the intervention between intervention and control patients. For falls, confidence intervals were calculated for the incidence rate differences based on Poisson approximations.
Sample Size Considerations
An a priori sample size calculation was performed. A 2001 study showed that functional status is poorly documented in at least 60% of hospital charts of elderly patients.5 Given an estimated sample size of 120 per group and a power of 80%, this study was powered to be able to detect an absolute difference in the documentation of functional status of as little as 18%.
RESULTS
Two hundred seventeen patients met the study entry criteria (Table 2): 122 were admitted to the Hospitalist‐ACE service, and 95 were admitted to usual care on the general medical services. The average age of the study patients was 80.5 years, 55.3% were female. Twenty‐eight percent of subjects were admitted for pulmonary diagnoses. The two groups of patients were similar with respect to age, gender, and distribution of primary diagnoses. The Hospitalist‐ACE patients had a mean MS‐DRG weight of 1.15, which was slightly higher than that of usual care patients at 1.05 (P = 0.06). Typically, 70% of Hospitalist‐ACE patients are admitted to the designated ACE medical unit (12 West).
Characteristic | Hospitalist‐ACE | Usual Care | P Value |
---|---|---|---|
N = 122 | N = 95 | ||
| |||
Age (years), mean (SD) | 80.5 (6.5) | 80.7 (7.0) | 0.86 |
Gender (% female) | 52.5 | 59 | 0.34 |
Case Mix Index (mean MS‐DRG weight [SD]) | 1.15 (0.43) | 1.05 (0.31) | 0.06 |
Primary ICD‐9 diagnosis (%) | 0.59 | ||
Pulmonary | 27.9 | 28.4 | |
General medicine | 15.6 | 11.6 | |
Surgery | 13.9 | 11.6 | |
Cardiology | 9.8 | 6.3 | |
Nephrology | 8.2 | 7.4 |
Processes of Care
Processes of care for older patients are displayed in Table 3. Patients on the Hospitalist‐ACE service had recognition and treatment of abnormal functional status at a rate that was nearly double that of patients on the usual care services (68.9% vs 35.8%, P < 0.0001). In addition, patients on the Hospitalist‐ACE service were significantly more likely to have had recognition and treatment of any abnormal cognitive status (55.7% vs 40.0%, P = 0.02). When delirium was evaluated alone, the Hospitalist‐ACE patients were also more likely to have had recognition and treatment of delirium (27.1% vs 17.0%, P = 0.08), although this finding did not reach statistical significance.
Measure | Percent of Hospitalist‐ACE Patients | Percent of Usual Care Patients | P Value |
---|---|---|---|
N = 122 | N = 95 | ||
| |||
Recognition and treatment of abnormal functional status | 68.9 | 35.8 | <0.0001 |
Recognition and treatment of abnormal cognitive status* | 55.7 | 40.0 | 0.02 |
Recognition and treatment of delirium | 27.1 | 17.0 | 0.08 |
Documentation of resuscitation preferences | 95.1 | 91.6 | 0.3 |
Do Not Attempt Resuscitation orders | 39.3 | 26.3 | 0.04 |
Use of sleep medications | 28.1 | 27.4 | 0.91 |
Use of physical restraints | 2.5 | 0 | 0.26 |
While patients on the Hospitalist‐ACE and usual care services had similar percentages of documentation of resuscitation preferences (95.1% vs 91.6%, P = 0.3), the percentage of Hospitalist‐ACE patients who had Do Not Attempt Resuscitation (DNAR) orders was significantly greater than that of the usual care patients (39.3% vs 26.3%, P = 0.04).
There were no differences in the use of physical restraints or sleep medications for Hospitalist‐ACE patients as compared to usual care patients, although the types of sleep mediations used on each service were markedly different: trazadone was employed as the first‐line sleep agent on the Hospitalist‐ACE service (77.7%), and non‐benzodiazepine hypnotics (primarily zolpidem) were employed most commonly on the usual care services (35%). There were no differences noted in the percentage of patients with benzodiazepines prescribed as sleep aids.
Outcomes
Resource utilization outcomes are reported in Table 4. Of note, there were no significant differences between Hospitalist‐ACE discharges and usual care discharges in mean length of stay (3.4 2.7 days vs 3.1 2.7 days, P = 0.52), mean charges ($24,617 15,828 vs $21,488 13,407, P = 0.12), or 30‐day readmissions to UCH (12.3% vs 9.5%, P = 0.51). Hospitalist‐ACE discharges and usual care patients were equally likely to be discharged to home (68.6% vs 67.4%, P = 0.84), with a similar proportion of Hospitalist‐ACE discharges receiving home health care or home hospice services (14.1% vs 7.4%, P = 12).
Measure | Hospitalist‐ACE | Usual Care | P Value |
---|---|---|---|
N = 122 | N = 95 | ||
| |||
Length of stay in days (mean [SD]) | 3.4 (2.7) | 3.1 (2.7) | 0.52 |
Charges in dollars (mean [SD]) | 24,617 (15,828) | 21,488 (13,407) | 0.12 |
30‐Day readmissions to UCH (%) | 12.3 | 9.5 | 0.51 |
Discharges to home (%) | 68.8* | 67.4 | 0.84 |
Discharges to home with services (%) | 14%* | 7.4% | 0.12 |
In addition, the fall rate for Hospitalist‐ACE patients was not significantly different from the fall rate for usual care patients (4.8 falls/1000 patient days vs 6.7 falls/1000 patient days, 95% confidence interval 9.613.3).
DISCUSSION
We report the implementation and evaluation of a medical service tailored to the care of the acutely ill older patient that draws from elements of the hospitalist model and the ACE unit model.7, 14, 24 For this Hospitalist‐ACE service, we developed a specialized hospitalist workforce, assembled a brief geriatric assessment tailored to the inpatient setting, instituted an interdisciplinary rounding model, and created a novel inpatient geriatrics curriculum.
During the study period, we improved performance of important processes of care for hospitalized elders, including recognition of abnormal cognitive and functional status; maintained comparable resource use; and implemented a novel, inpatient‐focused geriatric medicine educational experience. We were unable to demonstrate an impact on key clinical outcomes such as falls, physical restraint use, and readmissions. Nonetheless, there is evidence that the performance of selected processes of care is associated with improved three‐year survival status in the community‐dwelling vulnerable older patient, and may also be associated with a mortality benefit in the hospitalized vulnerable older patient.25, 26 Therefore, methods to improve the performance of these processes of care may be of clinical importance.
The finding of increased use of DNAR orders in the face of equivalent documentation of code status is of interest and generates hypotheses for further study. It is possible that the educational experience and use of geriatric assessment provides a more complete context for the code status discussion (one that incorporates the patient's social, physical, and cognitive function). However, we do not know if the patients on the ACE service had improved concordance between their code status and their goals of care.
We believe that there was no difference in key clinical outcomes between Hospitalist‐ACE and control patients because the population in this study was relatively low acuity and, therefore, the occurrence of falls and the use of physical restraints were quite low in the study population. In particular, the readmission rate was much lower than is typical for the Medicare population at our hospital, making it challenging to draw conclusions about the impact of the intervention on readmissions, however, we cannot rule out the possibility that our early discharge planning did not address the determinants of readmission for this population.
The ACE unit paradigmcharacterized by 1) closed, modified hospital units; 2) staffing by geriatricians and nurses with geriatrics training; 3) employing geriatric nursing care protocolsrequires significant resources and is not feasible for all settings.6 There is a need for alternative models of comprehensive care for hospitalized elders that require fewer resources in the form of dedicated units and specialist personnel, and can be more responsive to institutional needs. For example, in a 2005 report, one institution reported the creation of a geriatric medicine service that utilized a geriatrician and hospitalist co‐attending model.14 More recently, a large geriatrics program replaced its inpatient geriatrics unit with a mobile inpatient geriatrics service staffed by an attending geriatricianhospitalist, a geriatrics fellow, and a nurse practitioner.27 While these innovative models have eliminated the dedicated unit, they rely on board certified geriatricians, a group in short supply nationally.28 Hospitalists are a rapidly growing provider group that, with appropriate training and building on the work of geriatricians, is poised to provide leadership in acute geriatric care.29, 30
In contrast to the comprehensive inpatient geriatric care models described above, the Hospitalist‐ACE service uses a specialized hospitalist workforce and is not dependent on continuous staffing by geriatricians. Although geographic concentration is important for the success of interdisciplinary rounds, the Hospitalist‐ACE service does not require a closed or modified unit. The nursing staff caring for Hospitalist‐ACE patients have generalist nursing training and, at the time of the study, did not utilize geriatric‐care protocols. Our results need to be interpreted in the light of these differences from the ACE unit model which is a significantly more intensive intervention than the Hospitalist‐ACE service. In addition, the current practice environment is quite different from the mid‐1990s when ACE units were developed and studied. Development and maintenance of models of comprehensive inpatient geriatric care require demonstration of both value as well as return on investment. The alignment of financial and regulatory incentives for programs that provide comprehensive care to complex patients, such as those anticipated by the Affordable Care Act, may encourage the growth of such models.
These data represent findings from a six‐month evaluation of a novel inpatient service in the middle of its first year. There are several limitations related to our study design. First, the results of this small study at a single academic medical center may be of limited generalizability to other settings. Second, the program was evaluated only three months after its inception; we did not capture further improvements in methods, training, and outcomes expected as the program matured. Third, most of the Hospitalist‐ACE service attendings and residents rotate on the UCH general medical services throughout the year. Consequently, we were unable to eliminate the possibility of contamination of the control group, and we were unable to blind the physicians to the study. Fourth, the study population had a relatively low severity of illnessthe average MS‐DRG weight was near 1and low rates of important adverse events such falls and restraint use. This may have occurred because we excluded patients transferred from the ICUs and other services. It is possible that the Hospitalist‐ACE intervention might have demonstrated a larger benefit in a sicker population that would have presented greater opportunities for reductions in length of stay, costs, and adverse events. Fifth, given the retrospective nature of the data collection, we were not able to prospectively assess the incidence of important geriatric outcomes such as delirium and functional decline, nor can we make conclusions about changes in function during the hospitalization.
While the outcome measures we used are conceptually similar to several measures developed by RAND's Assessing Care of Vulnerable Elders (ACOVE) project, this study did not explicitly rely on those constructs.31 To do so would have required prospective screening by clinical staff independent from the care team for vulnerability that was beyond the scope of this project. In addition, the ACOVE measures of interest for functional and cognitive decline are limited to documentation of cognitive or functional assessments in the medical record. The ACE service's adoption of a brief standardized geriatric assessment was almost certain to meet that documentation requirement. While documentation is important, it is not clear that documentation, in and of itself, improves outcomes. Therefore, we expanded upon the ACOVE constructs to include the need for the additional evidence of a treatment plan when abnormal physical or cognitive function was documented. These constructs are important process of care for vulnerable elders. While we demonstrated improvements in several of these important processes of care for elderly patients, we are unable to draw conclusions about the impact of these differences in care on important clinical outcomes such as development of delirium, long‐term institutionalization, or mortality.
CONCLUSIONS
The risks of hospitalization for older persons are numerous, and present challenges and opportunities for inpatient physicians. As the hospitalized population agesmirroring national demographic trends and trends in use of acute care hospitalsthe challenge of avoiding harm in the older hospitalized patient will intensify. Innovations in care to improve the experience and outcomes of hospitalization for older patients are needed in the face of limited geriatrics‐trained workforce and few discretionary funds for unit redesign. The Hospitalist‐ACE service is a promising strategy for hospitalist programs with sufficient numbers of older patients and hospitalists with interest in improving clinical care for older adults. It provides a model for hospitalists to employ geriatrics principles targeted at reducing harm to their most vulnerable patients. Hospitalist‐run geriatric care models offer great promise for improving the care of acutely ill elderly patients. Future investigation should focus on demonstrating the impact of such care on important clinical outcomes between admission and discharge; on model refinement and adaptation, such as determining what components of comprehensive geriatric care are essential to success; and on how complementary interventions, such as the use of templated orders for the hospitalized elderly, impact outcomes. Additional research is needed, with a focus on demonstrating value with regard to an array of outcomes including cost, readmissions, and preventable harms of care.
Acknowledgements
Jean Kutner, MD, MSPH; Daniel Sandy, MPH; Shelly Limon, RN; nurses of 12 West; the UCH staff on the interdisciplinary team; and ACE patients and their families.
- Functional outcomes of acute medical illness and hospitalization in older persons.Arch Intern Med.1996;156:645–652. , , , et al.
- Delirium: a symptom of how hospital care is failing older persons and a window to improve quality of hospital care.Am J Med.1999;106:565–573. , , .
- Using assessing care of vulnerable elders quality indicators to measure quality of hospital care for vulnerable elders.J Am Geriatr Soc.2007;55(11):1705–1711. , , , et al.
- Impact and recognition of cognitive impairment among hospitalized elders.J Hosp Med.2010;5:69–75. , , , et al.
- What does the medical record reveal about functional status? A comparison of medical record and interview data.J Gen Intern Med.2001;16(11):728–736. , , , , .
- Successful models of comprehensive care for older adults with chronic conditions: evidence for the Institute of Medicine's “Retooling for an Aging America” report.J Am Geriatr Soc.2009;57(12):2328–2337. , , , , , .
- A randomized trial of care in a hospital medical unit especially designed to improve the functional outcomes of acutely ill older patients.N Engl J Med.1995;332:1338–1344. , , , , .
- Effects of a multicomponent intervention on functional outcomes and process of care in hospitalized older adults: a randomized controlled trial of Acute Care for Elders (ACE) in a community hospital.J Am Geriatr Soc.2000;48:1572–1581. , , , et al.
- The effectiveness of inpatient geriatric evaluation and management units: a systematic review and meta‐analysis.J Am Geriatr Soc.2010;58:83–92. , , , et al.
- Effectiveness of acute geriatric units on functional decline, living at home, and case fatality among older patients admitted to hospital for acute medical disorders: meta‐analysis.BMJ.2009;338:b50. , , , , .
- A randomized, controlled clinical trial of a geriatrics consultation team: compliance with recommendations.JAMA.1986;255:2617–2621. , , , et al.
- A multicomponent intervention to prevent delirium in hospitalized older patients.N Engl J Med.1999;340:669–676. , , , et al.
- Dissemination and characteristics of Acute Care of Elders (ACE) units in the United States.Int J Technol Assess Health Care.2003;19:220–227. , , , .
- Is there a geriatrician in the house? Geriatric care approaches in hospitalist programs.J Hosp Med.2006;1:29–35. , , .
- The Mini‐Cog: a cognitive “vital signs” measure for dementia screening in multi‐lingual elderly.Int J Geriatr Psychiatry.2000;15(11):1021–1027. , , , , .
- The Patient Health Questionnaire‐2: validity of a two‐item depression screener.Med Care.2003;41:1284–1292. , , .
- Clarifying confusion: the Confusion Assessment Method.Ann Intern Med.1990;113(12):941–948. , , , , , .
- The Vulnerable Elders Survey: a tool for identifying vulnerable older people in the community.J Am Geriatr Soc.2001;49:1691–1699. , , , et al.
- The timed “Up and Go”: a test of basic functional mobility for frail elderly persons.J Am Geriatr Soc.1991;39:142–148. , .
- American Geriatrics Society, British Geriatrics Society, and American Academy of Orthopedic Surgeons Panel on Falls Prevention.Guideline for the prevention of falls in older persons.J Am Geriatr Soc.2001;49:664–672.
- http://www.pogoe.org/productid/20212. . Falls for the inpatient physician. Translating knowledge into action. The Portal of Online Geriatric Education (POGOe). 6–19‐2008. Available at:
- 11–27‐0008. Available at: http://www.pogoe.org/productid/20296. , , , . Incontinence and urinary catheters for the inpatient physician. The Portal of Online Geriatric Education (POGOe).
- Use of medications for insomnia in the hospitalized geriatric population.J Am Geriatr Soc.2008;56(3):579–581. , , , .
- Hospitalists and the practice of inpatient medicine: results of a survey of the National Association of Inpatient Physicians.Ann Intern Med.1999;130(4 pt 2):343–349. , , , .
- Quality of care associated with survival in vulnerable older patients.Ann Intern Med.2005;143:274–281. , , , et al.
- Higher quality of care for hosptialized frail older adults is associated with improved survival one year after discharge.J Hosp Med.2009;4(S1):24. , , , et al.
- Operational and quality outcomes of a novel mobile acute care for the elderly service.J Am Geriatr Soc.2009;57:S1. , , , .
- Institute of Medicine (IOM).Retooling for an Aging America: Building the Health Care Workforce.Washington, DC:The National Academies Press;2008.
- Alternative solutions to the geriatric workforce deficit.Am J Med.2008;121:e23. , , .
- Fulfilling the promise of hospital medicine: tailoring internal medicine training to address hospitalists' needs.J Gen Intern Med.2008;23(7):1110–1115. , , , , .
- Assessing care of vulnerable elders: ACOVE project overview.Ann Intern Med.2001;135(8 pt 2):642–646. , .
For the frail older patient, hospitalization marks a period of high risk of poor outcomes and adverse events including functional decline, delirium, pressure ulcers, adverse drug events, nosocomial infections, and falls.1, 2 Physician recognition of elderly patients at risk for adverse outcomes is poor, making it difficult to intervene to prevent them.3, 4 Among frail, elderly inpatients at an urban academic medical center, doctors documented cognitive assessments in only 5% of patients. Functional assessments are appropriately documented in 40%80% of inpatients.3, 5
The Acute Care for Elders (ACE) unit is one of several models of comprehensive inpatient geriatric care that have been developed by geriatrician researchers to address the adverse events and functional decline that often accompany hospitalization.6 The ACE unit model generally incorporates: 1) a modified hospital environment, 2) early assessment and intensive management to minimize the adverse effects of hospital care, 3) early discharge planning, 4) patient centered care protocols, and 5) a consistent nursing staff.7 Two randomized, controlled trials have shown the ACE unit model to be successful in reducing functional decline among frail older inpatients during and after hospitalization.7, 8 While meta‐analyses data also suggests the ACE unit model reduces functional decline and future institutionalization, significant impact on other outcomes is not proven.9, 10
Several barriers have prevented the successful dissemination of the ACE unit model. The chief limitations are the upfront resources required to create and maintain a modified, dedicated unit, as well as the lack of a geriatrics trained workforce.7, 1113 The rapid growth of hospital medicine presents opportunities for innovation in the care of older patients. Still, a 2006 census demonstrated that few hospitalist groups had identified geriatric care as a priority.14
In response to these challenges, the University of Colorado Hospital Medicine Group created a hospitalist‐run inpatient medical service designed for the care of the frail older patient. This Hospitalist‐Acute Care for the Elderly (Hospitalist‐ACE) unit is a hybrid of a general medical service and an inpatient geriatrics unit.7 The goals of the Hospitalist‐ACE service are to provide high quality care tailored to older inpatients, thus minimizing the risks of functional decline and adverse events associate with hospitalization, and to provide a clinical geriatrics teaching experience for Hospitalist Training Track Residents within the Internal Medicine Residency Training Program and medical students at the University of Colorado Denver School of Medicine. The Hospitalist‐ACE unit is staffed with a core group of hospitalist attendings who have, at a minimum, attended an intensive mini‐course in inpatient geriatrics. The service employs interdisciplinary rounds; a brief, standardized geriatric assessment including screens of function, cognition, and mood; a clinical focus on mitigating the hazards of hospitalization, early discharge planning; and a novel geriatric educational curriculum for medicine residents and medical students.
This article will: 1) describe the creation of the Hospitalist‐ACE service at the University of Colorado Hospital; and 2) summarize the evaluation of the Hospitalist‐ACE service in a quasi‐randomized, controlled manner during its first year. We hypothesized that, when compared to patients receiving usual care, patients cared for on the Hospitalist‐ACE service would have increased recognition of abnormal functional status; recognition of abnormal cognitive status and delirium; equivalent lengths of stay and hospital charges; and decreased falls, 30‐day readmissions, and restraint use.
METHODS
Design
We performed a quasi‐randomized, controlled study of the Hospitalist‐ACE service.
Setting
The study setting was the inpatient general medical services of the Anschutz Inpatient Pavilion (AIP) of the University of Colorado Hospital (UCH). The AIP is a 425‐bed tertiary care hospital that is the major teaching affiliate of the University of Colorado School of Medicine and a regional referral center. The control services, hereafter referred to as usual care, were comprised of the four inpatient general medicine teaching services that take admissions on a four‐day rotation (in general, two were staffed by outpatient general internists and medical subspecialists, and two were staffed by academic hospitalists). The Hospitalist‐ACE service was a novel hospitalist teaching service that began in July 2007. Hospitalist‐ACE patients were admitted to a single 12‐bed medical unit (12 West) when beds were available; 12 West is similar to the other medical/surgical units at UCH and did not have any modifications to the rooms, equipment, or common areas for the intervention. The nursing staff on this unit had no formal geriatric nursing training. The Hospitalist‐ACE team admitted patients daily (between 7 AM and 3 PM MondayFriday; between 7 AM and 12 noon Saturday and Sunday). Patients assigned to the Hospitalist‐ACE service after hours were admitted by the internal medicine resident on call for the usual care services and handed off to the Hospitalist‐ACE team at 7 AM the next morning.
Study Subjects
Eligible subjects were inpatients age 70 years admitted to the usual care or Hospitalist‐ACE services at the AIP from November 2, 2007 to April 15, 2008. All patients age 70 years were randomized to the Hospitalist‐ACE service or usual care on a general internal medicine service by the last digit of the medical record number (odd numbers admitted to the Hospitalist‐ACE service and even numbers admitted to usual care). Patients followed by the Hospitalist‐ACE service but not admitted to 12 West were included in the study. To isolate the impact of the intervention, patients admitted to a medicine subspecialty service (such as cardiology, pulmonary, or oncology), or transferred to or from the Hospitalist‐ACE or control services to another service (eg, intensive care unit [ICU] or orthopedic surgery service) were excluded from the study.
Intervention
The Hospitalist‐ACE unit implemented an interdisciplinary team approach to identify and address geriatric syndromes in patients aged 70 and over. The Hospitalist‐ACE model of care consisted of clinical care provided by a hospitalist attending with additional training in geriatric medicine, administration of standardized geriatric screens assessing function, cognition, and mood, 15 minute daily (MondayFriday) interdisciplinary rounds focusing on recognition and management of geriatric syndromes and early discharge planning, and a standardized educational curriculum for medical residents and medical students addressing hazards of hospitalization.
The Hospitalist‐ACE service was a unique rotation within the Hospitalist Training Track of the Internal Medicine Residency that was developed with the support of the University of Colorado Hospital and the Internal Medicine Residency Training Program, and input from the Geriatrics Division at the University of Colorado Denver. The director received additional training from the Donald W. Reynolds FoundationUCLA Faculty Development to Advance Geriatric Education Mini‐Fellowship for hospitalist faculty. The mission of the service was to excel at educating the next generation of hospitalists while providing a model for excellence of care for hospitalized elderly patients. Important stakeholders were identified, and a leadership teamincluding representatives from nursing, physical and occupational therapy, pharmacy, social work, case management, and later, volunteer servicescreated the model daily interdisciplinary rounds. As geographic concentration was essential for the viability of interdisciplinary rounds, one unit (12 West) within the hospital was designated as the preferred location for patients admitted to the Hospitalist‐ACE service.
The Hospitalist‐ACE unit team consisted of one attending hospitalist, one resident, one intern, and medical students. The attending was one of five hospitalists, with additional training in geriatric medicine, who rotated attending responsibilities on the service. One of the hospitalists was board certified in geriatric medicine. Each of the other four hospitalists attended the Reynolds FoundationUCLA mini‐fellowship in geriatric medicine. Hospitalist‐ACE attendings rotated on a variety of other hospitalist services throughout the academic year, including the usual care services.
The brief standardized geriatric assessment consisted of six validated instruments, and was completed by house staff or medical students on admission, following instruction by the attending physician. The complete assessment tool is shown in Figure 1. The cognitive items included the Mini‐Cog,15 a two‐item depression screen,16 and the Confusion Assessment Method.17 The functional items included the Vulnerable Elders Survey (VES‐13),18 the Timed Get Up and Go test,19 and a two‐question falls screen.20 The elements of the assessment tool were selected by the Hospitalist‐ACE attendings for brevity and the potential to inform clinical management. To standardize the clinical and educational approach, the Hospitalist‐ACE attendings regularly discussed appropriate orders recommended in response to each positive screen, but no templated order sets were used during the study period.
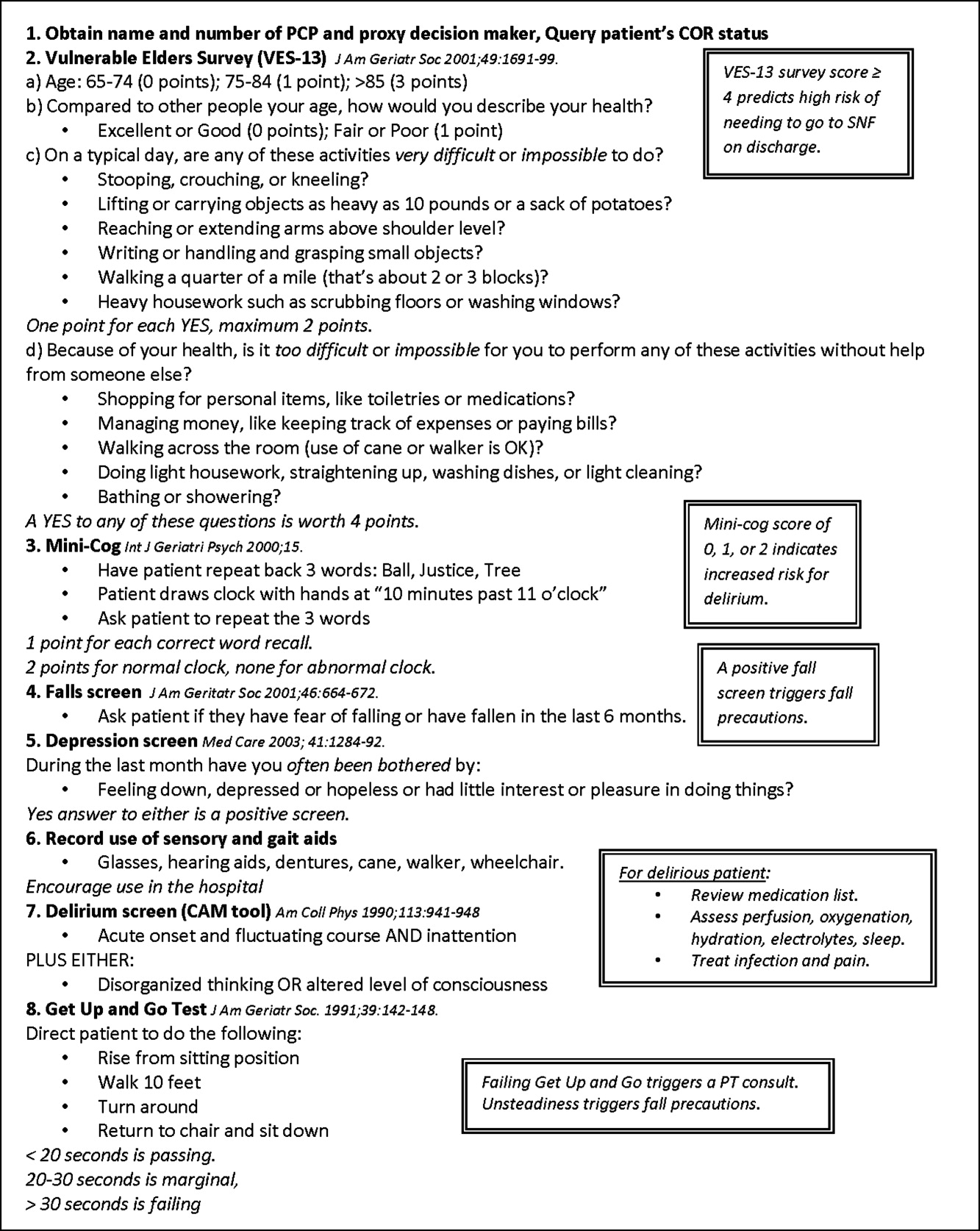
Interdisciplinary rounds were attended by Hospitalist‐ACE physicians, nurses, case managers, social workers, physical or occupational therapists, pharmacists, and volunteers. Rounds were led by the attending or medical resident.
The educational curriculum encompassed 13 modules created by the attending faculty that cover delirium, falls, dementia, pressure ulcers, physiology of aging, movement disorders, medication safety, end of life care, advance directives, care transitions, financing of health care for the elderly, and ethical conundrums in the care of the elderly. A full table of contents appears in online Appendix 1. Additionally, portions of the curriculum have been published online.21, 22 Topic selection was guided by the Accreditation Council for Graduate Medical Education (ACGME) core geriatrics topics determined most relevant for the inpatient setting. Formal instruction of 3045 minutes duration occurred three to four days a week and was presented in addition to routine internal medicine educational conferences. Attendings coordinated teaching to ensure that each trainee was exposed to all of the content during the course of their four‐week rotation.
In contrast to the Hospitalist‐ACE service, usual care on the control general medical services consisted of either a hospitalist, a general internist, or an internal medicine subspecialist attending physician, with one medical resident, one intern, and medical students admitting every fourth day. The general medical teams attended daily discharge planning rounds with a discharge planner and social worker focused exclusively on discharge planning. The content of teaching rounds on the general medical services was largely left to the discretion of the attending physician.
This program evaluation of the Hospitalist‐ACE service was granted a waiver of consent and Health Insurance Portability and Accountability Act (HIPAA) by the Colorado Multiple Institutional Review Board.
Measures
Primary Outcome
The primary outcome for the study was the recognition of abnormal functional status by the primary team. Recognition of abnormal functional status was determined from chart review and consisted of both the physician's detection of abnormal functional status and evidence of a corresponding treatment plan identified in the notes or orders of a physician member of the primary team (Table 1).
Measure | Criterion | Source | Content Examples |
---|---|---|---|
| |||
Recognition of abnormal functional status* | 1) Detection | MD's documentation of history | Presentation with change in function (new gait instability); use of gait aides (wheelchair) |
OR | |||
MD's documentation of physical exam | Observation of abnormal gait (eg, unsteady, wide‐based, shuffling) and/or balance Abnormal Get Up and Go test | ||
AND | |||
2) Treatment | MD's order | PT/OT consult; home safety evaluation | |
OR | |||
MD's documentation assessment/plan | Inclusion of functional status (rehabilitation, PT/OT needs) on the MD's problem list | ||
Recognition of abnormal cognitive status | Any of the following: | ||
Delirium | 1) Detection | MD's history | Presentation of confusion or altered mental status |
OR | |||
MD's physical exam | Abnormal confusion assessment method | ||
AND | |||
2) Treatment | MD's order | Sitter, reorienting communication, new halperidol order | |
OR | |||
MD's documentation of assessment/plan | Inclusion of delirium on the problem list | ||
OR | |||
Dementia | 1) Detection | MD's history | Dementia in medical history |
OR | OR | ||
MD's physical exam | Abnormal Folstein Mini‐Mental Status Exam or Mini‐Cog | ||
AND | |||
2) Treatment | MD's order | Cholinesterase inhibitor ordered | |
OR | OR | ||
MD's documentation of assessment/plan | Inclusion of dementia on the problem list | ||
OR | |||
Depression | 1) Detection | MD's history | Depression in medical history |
OR | OR | ||
MD's physical exam | Positive depression screen | ||
AND | |||
2) Treatment | MD's order | New antidepressant order | |
OR | |||
MD's documentation of assessment/plan | Inclusion of depression on the problem list |
Secondary Outcomes
Recognition of abnormal cognitive status was determined from chart review and consisted of both the physician's detection of dementia, depression, or delirium, and evidence of a corresponding treatment plan for any of the documented conditions identified in the notes or orders of a physician member of the primary team (Table 1). Additionally, we measured recognition and treatment of delirium alone.
Falls were determined from mandatory event reporting collected by the hospital on the University Hospitals Consortium Patient Safety Net web‐based reporting system and based on clinical assessment as reported by the nursing staff. The reports are validated by the appropriate clinical managers within 45 days of the event according to standard procedure.
Physical restraint use (type of restraint and duration) was determined from query of mandatory clinical documentation in the electronic medical record. Use of sleep aids was determined from review of the physician's order sheets in the medical record. The chart review captured any of 39 commonly prescribed hypnotic medications ordered at hour of sleep or for insomnia. The sleep medication list was compiled with the assistance of a pharmacist for an earlier chart review and included non‐benzodiazepine hypnotics, benzodiazepines, antidepressants, antihistamines, and antipsychotics.23
Length of stay, hospital charges, 30‐day readmissions to UCH (calculated from date of discharge), and discharge location were determined from administrative data.
Additional Descriptive Variables
Name, medical record number, gender, date of birth, date of admission and discharge, and primary diagnosis were obtained from the medical record. The Case Mix Index for each group of patients was determined from the average Medicare Severity‐adjusted Diagnosis Related Group (MS‐DRG) weight obtained from administrative data.
Data Collection
A two‐step, retrospective chart abstraction was employed. A professional research assistant (P.R.A.) hand‐abstracted process measures from the paper medical chart onto a data collection form designed for this study. A physician investigator performed a secondary review (H.L.W.). Discrepancies were resolved by the physician reviewer.
Data Analysis
Descriptive statistics were performed on intervention and control subjects. Means and standard deviations (age) or frequencies (gender, primary diagnoses) were calculated as appropriate. T tests were used for continuous variables, chi‐square tests for gender, and the Wilcoxon rank sum test for categorical variables.
Outcomes were reported as means and standard deviations for continuous variables (length of stay and charges) and frequencies for categorical variables (all other outcomes). T tests were used for continuous variables, Fisher's exact test for restraint use, and chi‐square tests were used for categorical variable to compare the impact of the intervention between intervention and control patients. For falls, confidence intervals were calculated for the incidence rate differences based on Poisson approximations.
Sample Size Considerations
An a priori sample size calculation was performed. A 2001 study showed that functional status is poorly documented in at least 60% of hospital charts of elderly patients.5 Given an estimated sample size of 120 per group and a power of 80%, this study was powered to be able to detect an absolute difference in the documentation of functional status of as little as 18%.
RESULTS
Two hundred seventeen patients met the study entry criteria (Table 2): 122 were admitted to the Hospitalist‐ACE service, and 95 were admitted to usual care on the general medical services. The average age of the study patients was 80.5 years, 55.3% were female. Twenty‐eight percent of subjects were admitted for pulmonary diagnoses. The two groups of patients were similar with respect to age, gender, and distribution of primary diagnoses. The Hospitalist‐ACE patients had a mean MS‐DRG weight of 1.15, which was slightly higher than that of usual care patients at 1.05 (P = 0.06). Typically, 70% of Hospitalist‐ACE patients are admitted to the designated ACE medical unit (12 West).
Characteristic | Hospitalist‐ACE | Usual Care | P Value |
---|---|---|---|
N = 122 | N = 95 | ||
| |||
Age (years), mean (SD) | 80.5 (6.5) | 80.7 (7.0) | 0.86 |
Gender (% female) | 52.5 | 59 | 0.34 |
Case Mix Index (mean MS‐DRG weight [SD]) | 1.15 (0.43) | 1.05 (0.31) | 0.06 |
Primary ICD‐9 diagnosis (%) | 0.59 | ||
Pulmonary | 27.9 | 28.4 | |
General medicine | 15.6 | 11.6 | |
Surgery | 13.9 | 11.6 | |
Cardiology | 9.8 | 6.3 | |
Nephrology | 8.2 | 7.4 |
Processes of Care
Processes of care for older patients are displayed in Table 3. Patients on the Hospitalist‐ACE service had recognition and treatment of abnormal functional status at a rate that was nearly double that of patients on the usual care services (68.9% vs 35.8%, P < 0.0001). In addition, patients on the Hospitalist‐ACE service were significantly more likely to have had recognition and treatment of any abnormal cognitive status (55.7% vs 40.0%, P = 0.02). When delirium was evaluated alone, the Hospitalist‐ACE patients were also more likely to have had recognition and treatment of delirium (27.1% vs 17.0%, P = 0.08), although this finding did not reach statistical significance.
Measure | Percent of Hospitalist‐ACE Patients | Percent of Usual Care Patients | P Value |
---|---|---|---|
N = 122 | N = 95 | ||
| |||
Recognition and treatment of abnormal functional status | 68.9 | 35.8 | <0.0001 |
Recognition and treatment of abnormal cognitive status* | 55.7 | 40.0 | 0.02 |
Recognition and treatment of delirium | 27.1 | 17.0 | 0.08 |
Documentation of resuscitation preferences | 95.1 | 91.6 | 0.3 |
Do Not Attempt Resuscitation orders | 39.3 | 26.3 | 0.04 |
Use of sleep medications | 28.1 | 27.4 | 0.91 |
Use of physical restraints | 2.5 | 0 | 0.26 |
While patients on the Hospitalist‐ACE and usual care services had similar percentages of documentation of resuscitation preferences (95.1% vs 91.6%, P = 0.3), the percentage of Hospitalist‐ACE patients who had Do Not Attempt Resuscitation (DNAR) orders was significantly greater than that of the usual care patients (39.3% vs 26.3%, P = 0.04).
There were no differences in the use of physical restraints or sleep medications for Hospitalist‐ACE patients as compared to usual care patients, although the types of sleep mediations used on each service were markedly different: trazadone was employed as the first‐line sleep agent on the Hospitalist‐ACE service (77.7%), and non‐benzodiazepine hypnotics (primarily zolpidem) were employed most commonly on the usual care services (35%). There were no differences noted in the percentage of patients with benzodiazepines prescribed as sleep aids.
Outcomes
Resource utilization outcomes are reported in Table 4. Of note, there were no significant differences between Hospitalist‐ACE discharges and usual care discharges in mean length of stay (3.4 2.7 days vs 3.1 2.7 days, P = 0.52), mean charges ($24,617 15,828 vs $21,488 13,407, P = 0.12), or 30‐day readmissions to UCH (12.3% vs 9.5%, P = 0.51). Hospitalist‐ACE discharges and usual care patients were equally likely to be discharged to home (68.6% vs 67.4%, P = 0.84), with a similar proportion of Hospitalist‐ACE discharges receiving home health care or home hospice services (14.1% vs 7.4%, P = 12).
Measure | Hospitalist‐ACE | Usual Care | P Value |
---|---|---|---|
N = 122 | N = 95 | ||
| |||
Length of stay in days (mean [SD]) | 3.4 (2.7) | 3.1 (2.7) | 0.52 |
Charges in dollars (mean [SD]) | 24,617 (15,828) | 21,488 (13,407) | 0.12 |
30‐Day readmissions to UCH (%) | 12.3 | 9.5 | 0.51 |
Discharges to home (%) | 68.8* | 67.4 | 0.84 |
Discharges to home with services (%) | 14%* | 7.4% | 0.12 |
In addition, the fall rate for Hospitalist‐ACE patients was not significantly different from the fall rate for usual care patients (4.8 falls/1000 patient days vs 6.7 falls/1000 patient days, 95% confidence interval 9.613.3).
DISCUSSION
We report the implementation and evaluation of a medical service tailored to the care of the acutely ill older patient that draws from elements of the hospitalist model and the ACE unit model.7, 14, 24 For this Hospitalist‐ACE service, we developed a specialized hospitalist workforce, assembled a brief geriatric assessment tailored to the inpatient setting, instituted an interdisciplinary rounding model, and created a novel inpatient geriatrics curriculum.
During the study period, we improved performance of important processes of care for hospitalized elders, including recognition of abnormal cognitive and functional status; maintained comparable resource use; and implemented a novel, inpatient‐focused geriatric medicine educational experience. We were unable to demonstrate an impact on key clinical outcomes such as falls, physical restraint use, and readmissions. Nonetheless, there is evidence that the performance of selected processes of care is associated with improved three‐year survival status in the community‐dwelling vulnerable older patient, and may also be associated with a mortality benefit in the hospitalized vulnerable older patient.25, 26 Therefore, methods to improve the performance of these processes of care may be of clinical importance.
The finding of increased use of DNAR orders in the face of equivalent documentation of code status is of interest and generates hypotheses for further study. It is possible that the educational experience and use of geriatric assessment provides a more complete context for the code status discussion (one that incorporates the patient's social, physical, and cognitive function). However, we do not know if the patients on the ACE service had improved concordance between their code status and their goals of care.
We believe that there was no difference in key clinical outcomes between Hospitalist‐ACE and control patients because the population in this study was relatively low acuity and, therefore, the occurrence of falls and the use of physical restraints were quite low in the study population. In particular, the readmission rate was much lower than is typical for the Medicare population at our hospital, making it challenging to draw conclusions about the impact of the intervention on readmissions, however, we cannot rule out the possibility that our early discharge planning did not address the determinants of readmission for this population.
The ACE unit paradigmcharacterized by 1) closed, modified hospital units; 2) staffing by geriatricians and nurses with geriatrics training; 3) employing geriatric nursing care protocolsrequires significant resources and is not feasible for all settings.6 There is a need for alternative models of comprehensive care for hospitalized elders that require fewer resources in the form of dedicated units and specialist personnel, and can be more responsive to institutional needs. For example, in a 2005 report, one institution reported the creation of a geriatric medicine service that utilized a geriatrician and hospitalist co‐attending model.14 More recently, a large geriatrics program replaced its inpatient geriatrics unit with a mobile inpatient geriatrics service staffed by an attending geriatricianhospitalist, a geriatrics fellow, and a nurse practitioner.27 While these innovative models have eliminated the dedicated unit, they rely on board certified geriatricians, a group in short supply nationally.28 Hospitalists are a rapidly growing provider group that, with appropriate training and building on the work of geriatricians, is poised to provide leadership in acute geriatric care.29, 30
In contrast to the comprehensive inpatient geriatric care models described above, the Hospitalist‐ACE service uses a specialized hospitalist workforce and is not dependent on continuous staffing by geriatricians. Although geographic concentration is important for the success of interdisciplinary rounds, the Hospitalist‐ACE service does not require a closed or modified unit. The nursing staff caring for Hospitalist‐ACE patients have generalist nursing training and, at the time of the study, did not utilize geriatric‐care protocols. Our results need to be interpreted in the light of these differences from the ACE unit model which is a significantly more intensive intervention than the Hospitalist‐ACE service. In addition, the current practice environment is quite different from the mid‐1990s when ACE units were developed and studied. Development and maintenance of models of comprehensive inpatient geriatric care require demonstration of both value as well as return on investment. The alignment of financial and regulatory incentives for programs that provide comprehensive care to complex patients, such as those anticipated by the Affordable Care Act, may encourage the growth of such models.
These data represent findings from a six‐month evaluation of a novel inpatient service in the middle of its first year. There are several limitations related to our study design. First, the results of this small study at a single academic medical center may be of limited generalizability to other settings. Second, the program was evaluated only three months after its inception; we did not capture further improvements in methods, training, and outcomes expected as the program matured. Third, most of the Hospitalist‐ACE service attendings and residents rotate on the UCH general medical services throughout the year. Consequently, we were unable to eliminate the possibility of contamination of the control group, and we were unable to blind the physicians to the study. Fourth, the study population had a relatively low severity of illnessthe average MS‐DRG weight was near 1and low rates of important adverse events such falls and restraint use. This may have occurred because we excluded patients transferred from the ICUs and other services. It is possible that the Hospitalist‐ACE intervention might have demonstrated a larger benefit in a sicker population that would have presented greater opportunities for reductions in length of stay, costs, and adverse events. Fifth, given the retrospective nature of the data collection, we were not able to prospectively assess the incidence of important geriatric outcomes such as delirium and functional decline, nor can we make conclusions about changes in function during the hospitalization.
While the outcome measures we used are conceptually similar to several measures developed by RAND's Assessing Care of Vulnerable Elders (ACOVE) project, this study did not explicitly rely on those constructs.31 To do so would have required prospective screening by clinical staff independent from the care team for vulnerability that was beyond the scope of this project. In addition, the ACOVE measures of interest for functional and cognitive decline are limited to documentation of cognitive or functional assessments in the medical record. The ACE service's adoption of a brief standardized geriatric assessment was almost certain to meet that documentation requirement. While documentation is important, it is not clear that documentation, in and of itself, improves outcomes. Therefore, we expanded upon the ACOVE constructs to include the need for the additional evidence of a treatment plan when abnormal physical or cognitive function was documented. These constructs are important process of care for vulnerable elders. While we demonstrated improvements in several of these important processes of care for elderly patients, we are unable to draw conclusions about the impact of these differences in care on important clinical outcomes such as development of delirium, long‐term institutionalization, or mortality.
CONCLUSIONS
The risks of hospitalization for older persons are numerous, and present challenges and opportunities for inpatient physicians. As the hospitalized population agesmirroring national demographic trends and trends in use of acute care hospitalsthe challenge of avoiding harm in the older hospitalized patient will intensify. Innovations in care to improve the experience and outcomes of hospitalization for older patients are needed in the face of limited geriatrics‐trained workforce and few discretionary funds for unit redesign. The Hospitalist‐ACE service is a promising strategy for hospitalist programs with sufficient numbers of older patients and hospitalists with interest in improving clinical care for older adults. It provides a model for hospitalists to employ geriatrics principles targeted at reducing harm to their most vulnerable patients. Hospitalist‐run geriatric care models offer great promise for improving the care of acutely ill elderly patients. Future investigation should focus on demonstrating the impact of such care on important clinical outcomes between admission and discharge; on model refinement and adaptation, such as determining what components of comprehensive geriatric care are essential to success; and on how complementary interventions, such as the use of templated orders for the hospitalized elderly, impact outcomes. Additional research is needed, with a focus on demonstrating value with regard to an array of outcomes including cost, readmissions, and preventable harms of care.
Acknowledgements
Jean Kutner, MD, MSPH; Daniel Sandy, MPH; Shelly Limon, RN; nurses of 12 West; the UCH staff on the interdisciplinary team; and ACE patients and their families.
For the frail older patient, hospitalization marks a period of high risk of poor outcomes and adverse events including functional decline, delirium, pressure ulcers, adverse drug events, nosocomial infections, and falls.1, 2 Physician recognition of elderly patients at risk for adverse outcomes is poor, making it difficult to intervene to prevent them.3, 4 Among frail, elderly inpatients at an urban academic medical center, doctors documented cognitive assessments in only 5% of patients. Functional assessments are appropriately documented in 40%80% of inpatients.3, 5
The Acute Care for Elders (ACE) unit is one of several models of comprehensive inpatient geriatric care that have been developed by geriatrician researchers to address the adverse events and functional decline that often accompany hospitalization.6 The ACE unit model generally incorporates: 1) a modified hospital environment, 2) early assessment and intensive management to minimize the adverse effects of hospital care, 3) early discharge planning, 4) patient centered care protocols, and 5) a consistent nursing staff.7 Two randomized, controlled trials have shown the ACE unit model to be successful in reducing functional decline among frail older inpatients during and after hospitalization.7, 8 While meta‐analyses data also suggests the ACE unit model reduces functional decline and future institutionalization, significant impact on other outcomes is not proven.9, 10
Several barriers have prevented the successful dissemination of the ACE unit model. The chief limitations are the upfront resources required to create and maintain a modified, dedicated unit, as well as the lack of a geriatrics trained workforce.7, 1113 The rapid growth of hospital medicine presents opportunities for innovation in the care of older patients. Still, a 2006 census demonstrated that few hospitalist groups had identified geriatric care as a priority.14
In response to these challenges, the University of Colorado Hospital Medicine Group created a hospitalist‐run inpatient medical service designed for the care of the frail older patient. This Hospitalist‐Acute Care for the Elderly (Hospitalist‐ACE) unit is a hybrid of a general medical service and an inpatient geriatrics unit.7 The goals of the Hospitalist‐ACE service are to provide high quality care tailored to older inpatients, thus minimizing the risks of functional decline and adverse events associate with hospitalization, and to provide a clinical geriatrics teaching experience for Hospitalist Training Track Residents within the Internal Medicine Residency Training Program and medical students at the University of Colorado Denver School of Medicine. The Hospitalist‐ACE unit is staffed with a core group of hospitalist attendings who have, at a minimum, attended an intensive mini‐course in inpatient geriatrics. The service employs interdisciplinary rounds; a brief, standardized geriatric assessment including screens of function, cognition, and mood; a clinical focus on mitigating the hazards of hospitalization, early discharge planning; and a novel geriatric educational curriculum for medicine residents and medical students.
This article will: 1) describe the creation of the Hospitalist‐ACE service at the University of Colorado Hospital; and 2) summarize the evaluation of the Hospitalist‐ACE service in a quasi‐randomized, controlled manner during its first year. We hypothesized that, when compared to patients receiving usual care, patients cared for on the Hospitalist‐ACE service would have increased recognition of abnormal functional status; recognition of abnormal cognitive status and delirium; equivalent lengths of stay and hospital charges; and decreased falls, 30‐day readmissions, and restraint use.
METHODS
Design
We performed a quasi‐randomized, controlled study of the Hospitalist‐ACE service.
Setting
The study setting was the inpatient general medical services of the Anschutz Inpatient Pavilion (AIP) of the University of Colorado Hospital (UCH). The AIP is a 425‐bed tertiary care hospital that is the major teaching affiliate of the University of Colorado School of Medicine and a regional referral center. The control services, hereafter referred to as usual care, were comprised of the four inpatient general medicine teaching services that take admissions on a four‐day rotation (in general, two were staffed by outpatient general internists and medical subspecialists, and two were staffed by academic hospitalists). The Hospitalist‐ACE service was a novel hospitalist teaching service that began in July 2007. Hospitalist‐ACE patients were admitted to a single 12‐bed medical unit (12 West) when beds were available; 12 West is similar to the other medical/surgical units at UCH and did not have any modifications to the rooms, equipment, or common areas for the intervention. The nursing staff on this unit had no formal geriatric nursing training. The Hospitalist‐ACE team admitted patients daily (between 7 AM and 3 PM MondayFriday; between 7 AM and 12 noon Saturday and Sunday). Patients assigned to the Hospitalist‐ACE service after hours were admitted by the internal medicine resident on call for the usual care services and handed off to the Hospitalist‐ACE team at 7 AM the next morning.
Study Subjects
Eligible subjects were inpatients age 70 years admitted to the usual care or Hospitalist‐ACE services at the AIP from November 2, 2007 to April 15, 2008. All patients age 70 years were randomized to the Hospitalist‐ACE service or usual care on a general internal medicine service by the last digit of the medical record number (odd numbers admitted to the Hospitalist‐ACE service and even numbers admitted to usual care). Patients followed by the Hospitalist‐ACE service but not admitted to 12 West were included in the study. To isolate the impact of the intervention, patients admitted to a medicine subspecialty service (such as cardiology, pulmonary, or oncology), or transferred to or from the Hospitalist‐ACE or control services to another service (eg, intensive care unit [ICU] or orthopedic surgery service) were excluded from the study.
Intervention
The Hospitalist‐ACE unit implemented an interdisciplinary team approach to identify and address geriatric syndromes in patients aged 70 and over. The Hospitalist‐ACE model of care consisted of clinical care provided by a hospitalist attending with additional training in geriatric medicine, administration of standardized geriatric screens assessing function, cognition, and mood, 15 minute daily (MondayFriday) interdisciplinary rounds focusing on recognition and management of geriatric syndromes and early discharge planning, and a standardized educational curriculum for medical residents and medical students addressing hazards of hospitalization.
The Hospitalist‐ACE service was a unique rotation within the Hospitalist Training Track of the Internal Medicine Residency that was developed with the support of the University of Colorado Hospital and the Internal Medicine Residency Training Program, and input from the Geriatrics Division at the University of Colorado Denver. The director received additional training from the Donald W. Reynolds FoundationUCLA Faculty Development to Advance Geriatric Education Mini‐Fellowship for hospitalist faculty. The mission of the service was to excel at educating the next generation of hospitalists while providing a model for excellence of care for hospitalized elderly patients. Important stakeholders were identified, and a leadership teamincluding representatives from nursing, physical and occupational therapy, pharmacy, social work, case management, and later, volunteer servicescreated the model daily interdisciplinary rounds. As geographic concentration was essential for the viability of interdisciplinary rounds, one unit (12 West) within the hospital was designated as the preferred location for patients admitted to the Hospitalist‐ACE service.
The Hospitalist‐ACE unit team consisted of one attending hospitalist, one resident, one intern, and medical students. The attending was one of five hospitalists, with additional training in geriatric medicine, who rotated attending responsibilities on the service. One of the hospitalists was board certified in geriatric medicine. Each of the other four hospitalists attended the Reynolds FoundationUCLA mini‐fellowship in geriatric medicine. Hospitalist‐ACE attendings rotated on a variety of other hospitalist services throughout the academic year, including the usual care services.
The brief standardized geriatric assessment consisted of six validated instruments, and was completed by house staff or medical students on admission, following instruction by the attending physician. The complete assessment tool is shown in Figure 1. The cognitive items included the Mini‐Cog,15 a two‐item depression screen,16 and the Confusion Assessment Method.17 The functional items included the Vulnerable Elders Survey (VES‐13),18 the Timed Get Up and Go test,19 and a two‐question falls screen.20 The elements of the assessment tool were selected by the Hospitalist‐ACE attendings for brevity and the potential to inform clinical management. To standardize the clinical and educational approach, the Hospitalist‐ACE attendings regularly discussed appropriate orders recommended in response to each positive screen, but no templated order sets were used during the study period.
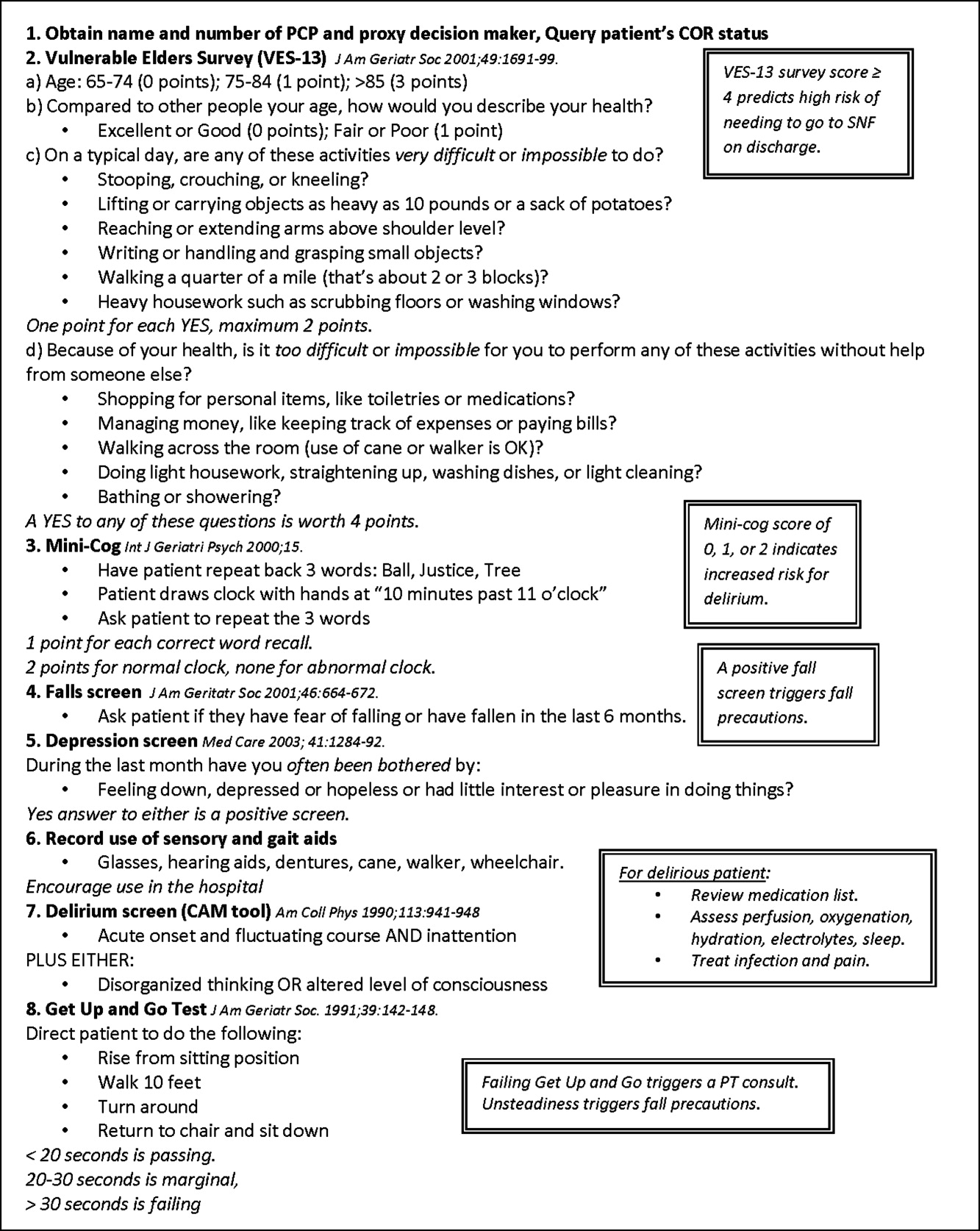
Interdisciplinary rounds were attended by Hospitalist‐ACE physicians, nurses, case managers, social workers, physical or occupational therapists, pharmacists, and volunteers. Rounds were led by the attending or medical resident.
The educational curriculum encompassed 13 modules created by the attending faculty that cover delirium, falls, dementia, pressure ulcers, physiology of aging, movement disorders, medication safety, end of life care, advance directives, care transitions, financing of health care for the elderly, and ethical conundrums in the care of the elderly. A full table of contents appears in online Appendix 1. Additionally, portions of the curriculum have been published online.21, 22 Topic selection was guided by the Accreditation Council for Graduate Medical Education (ACGME) core geriatrics topics determined most relevant for the inpatient setting. Formal instruction of 3045 minutes duration occurred three to four days a week and was presented in addition to routine internal medicine educational conferences. Attendings coordinated teaching to ensure that each trainee was exposed to all of the content during the course of their four‐week rotation.
In contrast to the Hospitalist‐ACE service, usual care on the control general medical services consisted of either a hospitalist, a general internist, or an internal medicine subspecialist attending physician, with one medical resident, one intern, and medical students admitting every fourth day. The general medical teams attended daily discharge planning rounds with a discharge planner and social worker focused exclusively on discharge planning. The content of teaching rounds on the general medical services was largely left to the discretion of the attending physician.
This program evaluation of the Hospitalist‐ACE service was granted a waiver of consent and Health Insurance Portability and Accountability Act (HIPAA) by the Colorado Multiple Institutional Review Board.
Measures
Primary Outcome
The primary outcome for the study was the recognition of abnormal functional status by the primary team. Recognition of abnormal functional status was determined from chart review and consisted of both the physician's detection of abnormal functional status and evidence of a corresponding treatment plan identified in the notes or orders of a physician member of the primary team (Table 1).
Measure | Criterion | Source | Content Examples |
---|---|---|---|
| |||
Recognition of abnormal functional status* | 1) Detection | MD's documentation of history | Presentation with change in function (new gait instability); use of gait aides (wheelchair) |
OR | |||
MD's documentation of physical exam | Observation of abnormal gait (eg, unsteady, wide‐based, shuffling) and/or balance Abnormal Get Up and Go test | ||
AND | |||
2) Treatment | MD's order | PT/OT consult; home safety evaluation | |
OR | |||
MD's documentation assessment/plan | Inclusion of functional status (rehabilitation, PT/OT needs) on the MD's problem list | ||
Recognition of abnormal cognitive status | Any of the following: | ||
Delirium | 1) Detection | MD's history | Presentation of confusion or altered mental status |
OR | |||
MD's physical exam | Abnormal confusion assessment method | ||
AND | |||
2) Treatment | MD's order | Sitter, reorienting communication, new halperidol order | |
OR | |||
MD's documentation of assessment/plan | Inclusion of delirium on the problem list | ||
OR | |||
Dementia | 1) Detection | MD's history | Dementia in medical history |
OR | OR | ||
MD's physical exam | Abnormal Folstein Mini‐Mental Status Exam or Mini‐Cog | ||
AND | |||
2) Treatment | MD's order | Cholinesterase inhibitor ordered | |
OR | OR | ||
MD's documentation of assessment/plan | Inclusion of dementia on the problem list | ||
OR | |||
Depression | 1) Detection | MD's history | Depression in medical history |
OR | OR | ||
MD's physical exam | Positive depression screen | ||
AND | |||
2) Treatment | MD's order | New antidepressant order | |
OR | |||
MD's documentation of assessment/plan | Inclusion of depression on the problem list |
Secondary Outcomes
Recognition of abnormal cognitive status was determined from chart review and consisted of both the physician's detection of dementia, depression, or delirium, and evidence of a corresponding treatment plan for any of the documented conditions identified in the notes or orders of a physician member of the primary team (Table 1). Additionally, we measured recognition and treatment of delirium alone.
Falls were determined from mandatory event reporting collected by the hospital on the University Hospitals Consortium Patient Safety Net web‐based reporting system and based on clinical assessment as reported by the nursing staff. The reports are validated by the appropriate clinical managers within 45 days of the event according to standard procedure.
Physical restraint use (type of restraint and duration) was determined from query of mandatory clinical documentation in the electronic medical record. Use of sleep aids was determined from review of the physician's order sheets in the medical record. The chart review captured any of 39 commonly prescribed hypnotic medications ordered at hour of sleep or for insomnia. The sleep medication list was compiled with the assistance of a pharmacist for an earlier chart review and included non‐benzodiazepine hypnotics, benzodiazepines, antidepressants, antihistamines, and antipsychotics.23
Length of stay, hospital charges, 30‐day readmissions to UCH (calculated from date of discharge), and discharge location were determined from administrative data.
Additional Descriptive Variables
Name, medical record number, gender, date of birth, date of admission and discharge, and primary diagnosis were obtained from the medical record. The Case Mix Index for each group of patients was determined from the average Medicare Severity‐adjusted Diagnosis Related Group (MS‐DRG) weight obtained from administrative data.
Data Collection
A two‐step, retrospective chart abstraction was employed. A professional research assistant (P.R.A.) hand‐abstracted process measures from the paper medical chart onto a data collection form designed for this study. A physician investigator performed a secondary review (H.L.W.). Discrepancies were resolved by the physician reviewer.
Data Analysis
Descriptive statistics were performed on intervention and control subjects. Means and standard deviations (age) or frequencies (gender, primary diagnoses) were calculated as appropriate. T tests were used for continuous variables, chi‐square tests for gender, and the Wilcoxon rank sum test for categorical variables.
Outcomes were reported as means and standard deviations for continuous variables (length of stay and charges) and frequencies for categorical variables (all other outcomes). T tests were used for continuous variables, Fisher's exact test for restraint use, and chi‐square tests were used for categorical variable to compare the impact of the intervention between intervention and control patients. For falls, confidence intervals were calculated for the incidence rate differences based on Poisson approximations.
Sample Size Considerations
An a priori sample size calculation was performed. A 2001 study showed that functional status is poorly documented in at least 60% of hospital charts of elderly patients.5 Given an estimated sample size of 120 per group and a power of 80%, this study was powered to be able to detect an absolute difference in the documentation of functional status of as little as 18%.
RESULTS
Two hundred seventeen patients met the study entry criteria (Table 2): 122 were admitted to the Hospitalist‐ACE service, and 95 were admitted to usual care on the general medical services. The average age of the study patients was 80.5 years, 55.3% were female. Twenty‐eight percent of subjects were admitted for pulmonary diagnoses. The two groups of patients were similar with respect to age, gender, and distribution of primary diagnoses. The Hospitalist‐ACE patients had a mean MS‐DRG weight of 1.15, which was slightly higher than that of usual care patients at 1.05 (P = 0.06). Typically, 70% of Hospitalist‐ACE patients are admitted to the designated ACE medical unit (12 West).
Characteristic | Hospitalist‐ACE | Usual Care | P Value |
---|---|---|---|
N = 122 | N = 95 | ||
| |||
Age (years), mean (SD) | 80.5 (6.5) | 80.7 (7.0) | 0.86 |
Gender (% female) | 52.5 | 59 | 0.34 |
Case Mix Index (mean MS‐DRG weight [SD]) | 1.15 (0.43) | 1.05 (0.31) | 0.06 |
Primary ICD‐9 diagnosis (%) | 0.59 | ||
Pulmonary | 27.9 | 28.4 | |
General medicine | 15.6 | 11.6 | |
Surgery | 13.9 | 11.6 | |
Cardiology | 9.8 | 6.3 | |
Nephrology | 8.2 | 7.4 |
Processes of Care
Processes of care for older patients are displayed in Table 3. Patients on the Hospitalist‐ACE service had recognition and treatment of abnormal functional status at a rate that was nearly double that of patients on the usual care services (68.9% vs 35.8%, P < 0.0001). In addition, patients on the Hospitalist‐ACE service were significantly more likely to have had recognition and treatment of any abnormal cognitive status (55.7% vs 40.0%, P = 0.02). When delirium was evaluated alone, the Hospitalist‐ACE patients were also more likely to have had recognition and treatment of delirium (27.1% vs 17.0%, P = 0.08), although this finding did not reach statistical significance.
Measure | Percent of Hospitalist‐ACE Patients | Percent of Usual Care Patients | P Value |
---|---|---|---|
N = 122 | N = 95 | ||
| |||
Recognition and treatment of abnormal functional status | 68.9 | 35.8 | <0.0001 |
Recognition and treatment of abnormal cognitive status* | 55.7 | 40.0 | 0.02 |
Recognition and treatment of delirium | 27.1 | 17.0 | 0.08 |
Documentation of resuscitation preferences | 95.1 | 91.6 | 0.3 |
Do Not Attempt Resuscitation orders | 39.3 | 26.3 | 0.04 |
Use of sleep medications | 28.1 | 27.4 | 0.91 |
Use of physical restraints | 2.5 | 0 | 0.26 |
While patients on the Hospitalist‐ACE and usual care services had similar percentages of documentation of resuscitation preferences (95.1% vs 91.6%, P = 0.3), the percentage of Hospitalist‐ACE patients who had Do Not Attempt Resuscitation (DNAR) orders was significantly greater than that of the usual care patients (39.3% vs 26.3%, P = 0.04).
There were no differences in the use of physical restraints or sleep medications for Hospitalist‐ACE patients as compared to usual care patients, although the types of sleep mediations used on each service were markedly different: trazadone was employed as the first‐line sleep agent on the Hospitalist‐ACE service (77.7%), and non‐benzodiazepine hypnotics (primarily zolpidem) were employed most commonly on the usual care services (35%). There were no differences noted in the percentage of patients with benzodiazepines prescribed as sleep aids.
Outcomes
Resource utilization outcomes are reported in Table 4. Of note, there were no significant differences between Hospitalist‐ACE discharges and usual care discharges in mean length of stay (3.4 2.7 days vs 3.1 2.7 days, P = 0.52), mean charges ($24,617 15,828 vs $21,488 13,407, P = 0.12), or 30‐day readmissions to UCH (12.3% vs 9.5%, P = 0.51). Hospitalist‐ACE discharges and usual care patients were equally likely to be discharged to home (68.6% vs 67.4%, P = 0.84), with a similar proportion of Hospitalist‐ACE discharges receiving home health care or home hospice services (14.1% vs 7.4%, P = 12).
Measure | Hospitalist‐ACE | Usual Care | P Value |
---|---|---|---|
N = 122 | N = 95 | ||
| |||
Length of stay in days (mean [SD]) | 3.4 (2.7) | 3.1 (2.7) | 0.52 |
Charges in dollars (mean [SD]) | 24,617 (15,828) | 21,488 (13,407) | 0.12 |
30‐Day readmissions to UCH (%) | 12.3 | 9.5 | 0.51 |
Discharges to home (%) | 68.8* | 67.4 | 0.84 |
Discharges to home with services (%) | 14%* | 7.4% | 0.12 |
In addition, the fall rate for Hospitalist‐ACE patients was not significantly different from the fall rate for usual care patients (4.8 falls/1000 patient days vs 6.7 falls/1000 patient days, 95% confidence interval 9.613.3).
DISCUSSION
We report the implementation and evaluation of a medical service tailored to the care of the acutely ill older patient that draws from elements of the hospitalist model and the ACE unit model.7, 14, 24 For this Hospitalist‐ACE service, we developed a specialized hospitalist workforce, assembled a brief geriatric assessment tailored to the inpatient setting, instituted an interdisciplinary rounding model, and created a novel inpatient geriatrics curriculum.
During the study period, we improved performance of important processes of care for hospitalized elders, including recognition of abnormal cognitive and functional status; maintained comparable resource use; and implemented a novel, inpatient‐focused geriatric medicine educational experience. We were unable to demonstrate an impact on key clinical outcomes such as falls, physical restraint use, and readmissions. Nonetheless, there is evidence that the performance of selected processes of care is associated with improved three‐year survival status in the community‐dwelling vulnerable older patient, and may also be associated with a mortality benefit in the hospitalized vulnerable older patient.25, 26 Therefore, methods to improve the performance of these processes of care may be of clinical importance.
The finding of increased use of DNAR orders in the face of equivalent documentation of code status is of interest and generates hypotheses for further study. It is possible that the educational experience and use of geriatric assessment provides a more complete context for the code status discussion (one that incorporates the patient's social, physical, and cognitive function). However, we do not know if the patients on the ACE service had improved concordance between their code status and their goals of care.
We believe that there was no difference in key clinical outcomes between Hospitalist‐ACE and control patients because the population in this study was relatively low acuity and, therefore, the occurrence of falls and the use of physical restraints were quite low in the study population. In particular, the readmission rate was much lower than is typical for the Medicare population at our hospital, making it challenging to draw conclusions about the impact of the intervention on readmissions, however, we cannot rule out the possibility that our early discharge planning did not address the determinants of readmission for this population.
The ACE unit paradigmcharacterized by 1) closed, modified hospital units; 2) staffing by geriatricians and nurses with geriatrics training; 3) employing geriatric nursing care protocolsrequires significant resources and is not feasible for all settings.6 There is a need for alternative models of comprehensive care for hospitalized elders that require fewer resources in the form of dedicated units and specialist personnel, and can be more responsive to institutional needs. For example, in a 2005 report, one institution reported the creation of a geriatric medicine service that utilized a geriatrician and hospitalist co‐attending model.14 More recently, a large geriatrics program replaced its inpatient geriatrics unit with a mobile inpatient geriatrics service staffed by an attending geriatricianhospitalist, a geriatrics fellow, and a nurse practitioner.27 While these innovative models have eliminated the dedicated unit, they rely on board certified geriatricians, a group in short supply nationally.28 Hospitalists are a rapidly growing provider group that, with appropriate training and building on the work of geriatricians, is poised to provide leadership in acute geriatric care.29, 30
In contrast to the comprehensive inpatient geriatric care models described above, the Hospitalist‐ACE service uses a specialized hospitalist workforce and is not dependent on continuous staffing by geriatricians. Although geographic concentration is important for the success of interdisciplinary rounds, the Hospitalist‐ACE service does not require a closed or modified unit. The nursing staff caring for Hospitalist‐ACE patients have generalist nursing training and, at the time of the study, did not utilize geriatric‐care protocols. Our results need to be interpreted in the light of these differences from the ACE unit model which is a significantly more intensive intervention than the Hospitalist‐ACE service. In addition, the current practice environment is quite different from the mid‐1990s when ACE units were developed and studied. Development and maintenance of models of comprehensive inpatient geriatric care require demonstration of both value as well as return on investment. The alignment of financial and regulatory incentives for programs that provide comprehensive care to complex patients, such as those anticipated by the Affordable Care Act, may encourage the growth of such models.
These data represent findings from a six‐month evaluation of a novel inpatient service in the middle of its first year. There are several limitations related to our study design. First, the results of this small study at a single academic medical center may be of limited generalizability to other settings. Second, the program was evaluated only three months after its inception; we did not capture further improvements in methods, training, and outcomes expected as the program matured. Third, most of the Hospitalist‐ACE service attendings and residents rotate on the UCH general medical services throughout the year. Consequently, we were unable to eliminate the possibility of contamination of the control group, and we were unable to blind the physicians to the study. Fourth, the study population had a relatively low severity of illnessthe average MS‐DRG weight was near 1and low rates of important adverse events such falls and restraint use. This may have occurred because we excluded patients transferred from the ICUs and other services. It is possible that the Hospitalist‐ACE intervention might have demonstrated a larger benefit in a sicker population that would have presented greater opportunities for reductions in length of stay, costs, and adverse events. Fifth, given the retrospective nature of the data collection, we were not able to prospectively assess the incidence of important geriatric outcomes such as delirium and functional decline, nor can we make conclusions about changes in function during the hospitalization.
While the outcome measures we used are conceptually similar to several measures developed by RAND's Assessing Care of Vulnerable Elders (ACOVE) project, this study did not explicitly rely on those constructs.31 To do so would have required prospective screening by clinical staff independent from the care team for vulnerability that was beyond the scope of this project. In addition, the ACOVE measures of interest for functional and cognitive decline are limited to documentation of cognitive or functional assessments in the medical record. The ACE service's adoption of a brief standardized geriatric assessment was almost certain to meet that documentation requirement. While documentation is important, it is not clear that documentation, in and of itself, improves outcomes. Therefore, we expanded upon the ACOVE constructs to include the need for the additional evidence of a treatment plan when abnormal physical or cognitive function was documented. These constructs are important process of care for vulnerable elders. While we demonstrated improvements in several of these important processes of care for elderly patients, we are unable to draw conclusions about the impact of these differences in care on important clinical outcomes such as development of delirium, long‐term institutionalization, or mortality.
CONCLUSIONS
The risks of hospitalization for older persons are numerous, and present challenges and opportunities for inpatient physicians. As the hospitalized population agesmirroring national demographic trends and trends in use of acute care hospitalsthe challenge of avoiding harm in the older hospitalized patient will intensify. Innovations in care to improve the experience and outcomes of hospitalization for older patients are needed in the face of limited geriatrics‐trained workforce and few discretionary funds for unit redesign. The Hospitalist‐ACE service is a promising strategy for hospitalist programs with sufficient numbers of older patients and hospitalists with interest in improving clinical care for older adults. It provides a model for hospitalists to employ geriatrics principles targeted at reducing harm to their most vulnerable patients. Hospitalist‐run geriatric care models offer great promise for improving the care of acutely ill elderly patients. Future investigation should focus on demonstrating the impact of such care on important clinical outcomes between admission and discharge; on model refinement and adaptation, such as determining what components of comprehensive geriatric care are essential to success; and on how complementary interventions, such as the use of templated orders for the hospitalized elderly, impact outcomes. Additional research is needed, with a focus on demonstrating value with regard to an array of outcomes including cost, readmissions, and preventable harms of care.
Acknowledgements
Jean Kutner, MD, MSPH; Daniel Sandy, MPH; Shelly Limon, RN; nurses of 12 West; the UCH staff on the interdisciplinary team; and ACE patients and their families.
- Functional outcomes of acute medical illness and hospitalization in older persons.Arch Intern Med.1996;156:645–652. , , , et al.
- Delirium: a symptom of how hospital care is failing older persons and a window to improve quality of hospital care.Am J Med.1999;106:565–573. , , .
- Using assessing care of vulnerable elders quality indicators to measure quality of hospital care for vulnerable elders.J Am Geriatr Soc.2007;55(11):1705–1711. , , , et al.
- Impact and recognition of cognitive impairment among hospitalized elders.J Hosp Med.2010;5:69–75. , , , et al.
- What does the medical record reveal about functional status? A comparison of medical record and interview data.J Gen Intern Med.2001;16(11):728–736. , , , , .
- Successful models of comprehensive care for older adults with chronic conditions: evidence for the Institute of Medicine's “Retooling for an Aging America” report.J Am Geriatr Soc.2009;57(12):2328–2337. , , , , , .
- A randomized trial of care in a hospital medical unit especially designed to improve the functional outcomes of acutely ill older patients.N Engl J Med.1995;332:1338–1344. , , , , .
- Effects of a multicomponent intervention on functional outcomes and process of care in hospitalized older adults: a randomized controlled trial of Acute Care for Elders (ACE) in a community hospital.J Am Geriatr Soc.2000;48:1572–1581. , , , et al.
- The effectiveness of inpatient geriatric evaluation and management units: a systematic review and meta‐analysis.J Am Geriatr Soc.2010;58:83–92. , , , et al.
- Effectiveness of acute geriatric units on functional decline, living at home, and case fatality among older patients admitted to hospital for acute medical disorders: meta‐analysis.BMJ.2009;338:b50. , , , , .
- A randomized, controlled clinical trial of a geriatrics consultation team: compliance with recommendations.JAMA.1986;255:2617–2621. , , , et al.
- A multicomponent intervention to prevent delirium in hospitalized older patients.N Engl J Med.1999;340:669–676. , , , et al.
- Dissemination and characteristics of Acute Care of Elders (ACE) units in the United States.Int J Technol Assess Health Care.2003;19:220–227. , , , .
- Is there a geriatrician in the house? Geriatric care approaches in hospitalist programs.J Hosp Med.2006;1:29–35. , , .
- The Mini‐Cog: a cognitive “vital signs” measure for dementia screening in multi‐lingual elderly.Int J Geriatr Psychiatry.2000;15(11):1021–1027. , , , , .
- The Patient Health Questionnaire‐2: validity of a two‐item depression screener.Med Care.2003;41:1284–1292. , , .
- Clarifying confusion: the Confusion Assessment Method.Ann Intern Med.1990;113(12):941–948. , , , , , .
- The Vulnerable Elders Survey: a tool for identifying vulnerable older people in the community.J Am Geriatr Soc.2001;49:1691–1699. , , , et al.
- The timed “Up and Go”: a test of basic functional mobility for frail elderly persons.J Am Geriatr Soc.1991;39:142–148. , .
- American Geriatrics Society, British Geriatrics Society, and American Academy of Orthopedic Surgeons Panel on Falls Prevention.Guideline for the prevention of falls in older persons.J Am Geriatr Soc.2001;49:664–672.
- http://www.pogoe.org/productid/20212. . Falls for the inpatient physician. Translating knowledge into action. The Portal of Online Geriatric Education (POGOe). 6–19‐2008. Available at:
- 11–27‐0008. Available at: http://www.pogoe.org/productid/20296. , , , . Incontinence and urinary catheters for the inpatient physician. The Portal of Online Geriatric Education (POGOe).
- Use of medications for insomnia in the hospitalized geriatric population.J Am Geriatr Soc.2008;56(3):579–581. , , , .
- Hospitalists and the practice of inpatient medicine: results of a survey of the National Association of Inpatient Physicians.Ann Intern Med.1999;130(4 pt 2):343–349. , , , .
- Quality of care associated with survival in vulnerable older patients.Ann Intern Med.2005;143:274–281. , , , et al.
- Higher quality of care for hosptialized frail older adults is associated with improved survival one year after discharge.J Hosp Med.2009;4(S1):24. , , , et al.
- Operational and quality outcomes of a novel mobile acute care for the elderly service.J Am Geriatr Soc.2009;57:S1. , , , .
- Institute of Medicine (IOM).Retooling for an Aging America: Building the Health Care Workforce.Washington, DC:The National Academies Press;2008.
- Alternative solutions to the geriatric workforce deficit.Am J Med.2008;121:e23. , , .
- Fulfilling the promise of hospital medicine: tailoring internal medicine training to address hospitalists' needs.J Gen Intern Med.2008;23(7):1110–1115. , , , , .
- Assessing care of vulnerable elders: ACOVE project overview.Ann Intern Med.2001;135(8 pt 2):642–646. , .
- Functional outcomes of acute medical illness and hospitalization in older persons.Arch Intern Med.1996;156:645–652. , , , et al.
- Delirium: a symptom of how hospital care is failing older persons and a window to improve quality of hospital care.Am J Med.1999;106:565–573. , , .
- Using assessing care of vulnerable elders quality indicators to measure quality of hospital care for vulnerable elders.J Am Geriatr Soc.2007;55(11):1705–1711. , , , et al.
- Impact and recognition of cognitive impairment among hospitalized elders.J Hosp Med.2010;5:69–75. , , , et al.
- What does the medical record reveal about functional status? A comparison of medical record and interview data.J Gen Intern Med.2001;16(11):728–736. , , , , .
- Successful models of comprehensive care for older adults with chronic conditions: evidence for the Institute of Medicine's “Retooling for an Aging America” report.J Am Geriatr Soc.2009;57(12):2328–2337. , , , , , .
- A randomized trial of care in a hospital medical unit especially designed to improve the functional outcomes of acutely ill older patients.N Engl J Med.1995;332:1338–1344. , , , , .
- Effects of a multicomponent intervention on functional outcomes and process of care in hospitalized older adults: a randomized controlled trial of Acute Care for Elders (ACE) in a community hospital.J Am Geriatr Soc.2000;48:1572–1581. , , , et al.
- The effectiveness of inpatient geriatric evaluation and management units: a systematic review and meta‐analysis.J Am Geriatr Soc.2010;58:83–92. , , , et al.
- Effectiveness of acute geriatric units on functional decline, living at home, and case fatality among older patients admitted to hospital for acute medical disorders: meta‐analysis.BMJ.2009;338:b50. , , , , .
- A randomized, controlled clinical trial of a geriatrics consultation team: compliance with recommendations.JAMA.1986;255:2617–2621. , , , et al.
- A multicomponent intervention to prevent delirium in hospitalized older patients.N Engl J Med.1999;340:669–676. , , , et al.
- Dissemination and characteristics of Acute Care of Elders (ACE) units in the United States.Int J Technol Assess Health Care.2003;19:220–227. , , , .
- Is there a geriatrician in the house? Geriatric care approaches in hospitalist programs.J Hosp Med.2006;1:29–35. , , .
- The Mini‐Cog: a cognitive “vital signs” measure for dementia screening in multi‐lingual elderly.Int J Geriatr Psychiatry.2000;15(11):1021–1027. , , , , .
- The Patient Health Questionnaire‐2: validity of a two‐item depression screener.Med Care.2003;41:1284–1292. , , .
- Clarifying confusion: the Confusion Assessment Method.Ann Intern Med.1990;113(12):941–948. , , , , , .
- The Vulnerable Elders Survey: a tool for identifying vulnerable older people in the community.J Am Geriatr Soc.2001;49:1691–1699. , , , et al.
- The timed “Up and Go”: a test of basic functional mobility for frail elderly persons.J Am Geriatr Soc.1991;39:142–148. , .
- American Geriatrics Society, British Geriatrics Society, and American Academy of Orthopedic Surgeons Panel on Falls Prevention.Guideline for the prevention of falls in older persons.J Am Geriatr Soc.2001;49:664–672.
- http://www.pogoe.org/productid/20212. . Falls for the inpatient physician. Translating knowledge into action. The Portal of Online Geriatric Education (POGOe). 6–19‐2008. Available at:
- 11–27‐0008. Available at: http://www.pogoe.org/productid/20296. , , , . Incontinence and urinary catheters for the inpatient physician. The Portal of Online Geriatric Education (POGOe).
- Use of medications for insomnia in the hospitalized geriatric population.J Am Geriatr Soc.2008;56(3):579–581. , , , .
- Hospitalists and the practice of inpatient medicine: results of a survey of the National Association of Inpatient Physicians.Ann Intern Med.1999;130(4 pt 2):343–349. , , , .
- Quality of care associated with survival in vulnerable older patients.Ann Intern Med.2005;143:274–281. , , , et al.
- Higher quality of care for hosptialized frail older adults is associated with improved survival one year after discharge.J Hosp Med.2009;4(S1):24. , , , et al.
- Operational and quality outcomes of a novel mobile acute care for the elderly service.J Am Geriatr Soc.2009;57:S1. , , , .
- Institute of Medicine (IOM).Retooling for an Aging America: Building the Health Care Workforce.Washington, DC:The National Academies Press;2008.
- Alternative solutions to the geriatric workforce deficit.Am J Med.2008;121:e23. , , .
- Fulfilling the promise of hospital medicine: tailoring internal medicine training to address hospitalists' needs.J Gen Intern Med.2008;23(7):1110–1115. , , , , .
- Assessing care of vulnerable elders: ACOVE project overview.Ann Intern Med.2001;135(8 pt 2):642–646. , .
Copyright © 2011 Society of Hospital Medicine
Risk Stratification Tools for TIA
Transient ischemic attacks (TIAs) are common and represent a clarion call to action to prevent disabling stroke. Incidence estimates for TIA range from 37 to 107 per 100,000 persons each year.1 Extrapolating from these data, there are likely greater than 100,000 to 300,000 TIAs in the US annually. Within 3 months, approximately 10% of these patients will suffer a stroke, with approximately one‐half of these events occurring within the first 48 hours after the sentinel TIA.26 Nearly two‐thirds of secondary strokes result in disability and 21% are fatal.3 Hospitalists are frequently called to provide care for patients with TIA and, as such, in order to establish an appropriate care plan, they require tools to better predict the likelihood and timing of a disabling stroke.7 In this review we examine the rationale for early aggressive TIA evaluation and treatment in the hospital, overview risk stratification models to identify the patients at highest risk for early recurrent ischemia, and explore application of these tools to admission policy and individualized patient care planning.
Definition
TIA is defined as a brief episode of neurological dysfunction caused by focal brain or retinal ischemia with clinical symptoms typically lasting less than 1 hour and without evidence of brain infarction.8, 9 Prior arbitrary time limits are being abandoned as advanced imaging techniques demonstrate that clinical examination lacks the sensitivity to detect small cerebral infarctions leading to misclassification of as many as 30% to 40% of strokes as TIAs.811 For cases in which imaging is not available, the diagnosis of clinically probable TIA is suggested. Patients with imaging consistent with stroke appear to be at 4‐fold to 10‐fold higher risk for subsequent ischemic events, thus the presence of subclinical infarcts may have clinical importance.2, 12 The majority of TIAs resolve within 1 hour of onset and neurologic deficit continuance beyond this time frame is more consistent with a stroke.13 Continuing symptoms after 1 hour mandates aggressive therapy in lieu of withholding intervention in the hopes of a spontaneous recovery.
Rationale for Hospitalization
Urgent evaluation and treatment within 24 to 48 hours of a TIA is recommended by the National Stroke Association (Table 1).14 These guidelines also recommend hospital admission for high‐risk patients. There are a number of compelling arguments for the hospitalization of a patient at high risk for subsequent stroke.
Test | Rationale | Therapy |
---|---|---|
| ||
Electrocardiogram and rhythm strip | To detect atrial fibrillation. | Long‐term warfarin indicated for suspected cardioembolic etiology. |
Echocardiogram | To detect intracardiac thrombus or vegetations. Bubble study to detect patent foramen ovale in young patients. | Warfarin indicated for suspected cardioembolic etiology. Patent foramen ovale closure is an option for selected patients. |
Carotid ultrasound | To detect large vessel atherosclerotic disease. | Antiplatelet therapy* indicated for atherosclerotic etiologies. Early carotid endarterectomy following TIA considered for 50% symptomatic ipsilateral stenosis. |
Fasting lipid profile, complete blood count, serum electrolytes and creatinine | Secondary prevention of stroke by treating hyperlipidemia. Signs and symptoms associated with severe laboratory abnormalities may mimic TIA. | LDL >100 mg/dL (optional goal >70 mg/dL) is indication for cholesterol lowering therapy. |
Neuroimaging‐MRI with diffusion images MRA or CT CTA | To detect clinically inapparent lesions of stroke. Useful in ruling out some mimics of TIA. | Patients with abnormal MRI diffusion images represent a population at increased risk for recurrent stroke. |
First, hospitalization offers potential for reduced time to thrombolysis for those patients who have a second ischemic event in the early period following TIA. Outpatients with new ischemic stroke may see hours pass between symptom onset and presentation to the emergency department (ED). This delay frequently places them outside of the thrombolytic window.1517 Hospitalization, assuming a well‐designed inpatient stroke care system, has great potential to reduce this delay. Approximately 50% of the stroke risk following a TIA is evident within 48 hours and rapid thrombolysis, available in an inpatient setting, is associated with improved outcome after stroke.3, 18 A cost‐utility analysis found that a 24‐hour admission for TIA patients to allow tissue plasminogen activator (t‐PA) for recurrent ischemia has a cost‐effectiveness ratio of $55,044 per quality‐adjusted life year with increasing cost effectiveness for the highest risk patients, such as those with a 24‐hour stroke risk of >5%.19
Second, hospital admission often facilitates the reliable and efficient evaluation for etiology and early initiation of secondary prevention. Neuroimaging, carotid ultrasound, echocardiography, and telemetry can be expedited with rapid initiation of proven secondary preventive therapies such as statin treatment, blood pressure control, and antithrombotic therapy. When indicated, carotid revascularization is recommended as soon as possible following TIA, with retrospective reviews suggesting improved outcomes when performed within 2 weeks of the event.1420 In one analysis, a negative association between hospitalization for TIA and subsequent stroke was discovered by review of Canadian population‐based administrative databases.5 While the mechanism for the negative association could not be established, the literature provides some support for hospitalization being associated with decreased risk for second strokes (hazard ratio [HR], 0.73; 95% confidence interval [CI], 0.570.95).5
Theoretically, much of this evaluation and treatment could occur in the outpatient setting but delays commonly seen in outpatient evaluation and the high potential for early second strokes for some patients may make this a risky care plan. Despite the high likelihood for serious outcomes following TIA and clear guidelines for early evaluation and management, current care often lacks a sense of urgency. A 2004 Canadian study revealed that three‐quarters of patients with a TIA were discharged directly from the ED with a resultant delay in diagnostic investigation.4 Over one‐third of patients were discharged without a prescription for antithrombotic therapy. American primary care practice patterns reveal even more significant delays in therapy, with only 2% of patients admitted to a hospital on the day of presentation for TIA, despite 80% of patients presenting for evaluation on the day of symptom onset.21 In this study less than one‐half of patients with atrial fibrillation were started on immediate anticoagulation.21 Further, as many as one‐third of patients did not have any evaluation in the month after the index event.21 Hospitalization for high‐risk patients has the potential to avoid these delays in outpatient evaluation and initiation of therapy.
Still, not all patients will require admission to a hospital setting. American EDs admit approximately one‐half of all TIAs, with regional variability not explained exclusively by clinical characteristics.22 Focusing on identifying the cohort of patients who would most benefit from hospitalization is paramount. In general, hospitalization should be reserved for patients with higher risk of an early secondary stroke. Specifically, admission is generally recommended for patients with crescendo symptoms, TIA on antithrombotic therapy, or symptoms lasting >1 hour.14 Additionally, patients with symptomatic carotid stenosis of 50% and presumed cardioembolic or hypercoagulable etiology merit hospital admission.14 In many cases these etiologies may not be known at time of presentation. Evaluation, such as carotid ultrasound, may not be readily available in the ED to inform the admission decision. Several new scoring systems that utilize routine clinical features available within an hour of presentation have been developed to more objectively assess the risk of secondary stroke following a TIA. The use of these prognostic scoring systems is recommended by the National Stroke Association to aid in triaging this cohort of patients.14
Prognostic Scoring Systems
California Score
The 2000 California Score (Tables 2 and 3) is a 5‐point tool retrospectively developed from a database of 1,707 TIA patients seen in the ED of a California hospital system to predict the likelihood of stroke within 90 days of an initial presentation with transient neurologic deficits.3 Multivariate logistic regression models were used to test the clinical factors associated with stroke risk, resulting in a tool using clinical features of age, diabetes, symptom duration, and the type of deficit to provide quantitative estimates of intermediate term risk. Within 90 days, none of the patients with a score of zero had a stroke whereas the highest score had a 34% risk of stroke (Table 3). While it is possible that some patients with lower scores had a nonischemic etiology for their transient neurological symptoms, clinical practice contains similar ambiguity, and such patients would be correctly predicted to have a low risk for subsequent strokes. Additionally, the derivation and validation of this tool did not include a neurologist confirmation of TIA diagnosis; however, this likely mirrors the practice in most EDs. The California Score has subsequently been validated and expanded upon during the development of the ABCD2 score.23 The California Score's utility is limited in the acute decision‐making process, especially regarding the decision to admit, as it focuses on 90‐day outcomes. For that, shorter‐term risk assessments are more useful.
Clinical Feature | California Score (points) | ABCD (points) | ABCD2 (points) | |
---|---|---|---|---|
| ||||
Age | 60 years | 1 | 1 | 1 |
Blood pressure | Systolic blood pressure 140 or diastolic blood pressure 90 mmHg | N/A | 1 | 1 |
Clinical deficits* | Unilateral weakness (focal motor weakness of 1 or more of face, arm, hand, or leg) | 1 | 2 | 2 |
Speech impairment (dysarthria, dysphasia, or both) | 1 | 1 | 1 | |
Duration | 60 minutes | 1 | 2 | 2 |
10‐59 minutes | 1 | 1 | 1 | |
Diabetes | Present | 1 | N/A | 1 |
Maximum score | 5 | 6 | 7 |
California Score | 0 | 1 | 2 | 3 | 4 | 5 |
---|---|---|---|---|---|---|
| ||||||
90‐day stroke risk (%) | 0 | 3 | 7 | 11 | 15 | 34 |
ABCD Score
The 2005 ABCD (Tables 2 and 4) is a 6‐point tool designed to predict 7‐day risk of stroke following TIA from the Oxfordshire Community Stroke Project, a UK prospective population‐based cohort of 209 patients with diagnosis of TIA made by a neurologist.24 It evaluated factors previously found to be independent predictors of stroke after TIA, and determined that risk factors of age, blood pressure, type of clinical deficit, and symptom duration predicted 7‐day risk of stroke following TIA. Unlike the California score, the ABCD authors found presenting blood pressure, but not diabetes, to be independent predictors of future events. The authors validated the score with a second population of TIA patients in the Oxford Vascular Study and in a hospital‐based TIA clinic cohort.24 In the validation cohorts the score was highly predictive of stroke at 7 days (P < 0.001). Patients with the lowest scores of 0 to 3 had no strokes in the week following the index TIA, whereas patients with the highest score of 6 carried a 35.5% risk of early second stroke. The primary limitation of the ABCD score lies in the small sample size, with only 18 recurrent strokes in the week after TIA in the derivation cohort.
ABCD Score | 0 | 1 | 2 | 3 | 4 | 5 | 6 |
---|---|---|---|---|---|---|---|
| |||||||
7‐Day stroke risk (%) | 0 | 0 | 0 | 0 | 2.2 | 16.3 | 35.5 |
The ABCD score has subsequently been tested in other settings with mixed results. Two studies found limited utility.25, 26 Other trials found high scores to be overly inclusive but highly predictive and thus the majority of evidence appears to support the validity of the ABCD score in predicting risk of early recurrent ischemic events.2729 The ABCD score has been used to triage patients with high scores to inpatient management although the benefit of this strategy on outcomes has not been proven.30
ABCD2 Score
The 2007 ABCD2 (Tables 2 and 5) is a 7‐point tool that uses the original ABCD criteria along with an additional point for diabetes.23 The ABCD2 derived a unified prognostic score for optimal prediction of the 2‐day stroke risk from multivariate analysis of the original data sets used to create the California and ABCD scores. This score was then validated by the authors in 4 independent cohorts from the United States and the United Kingdom.23 In sum, 4809 patients with TIA were included in the ABCD2 analysis. Similar to prior studies, stroke occurred in 9.2% of patients by 90 days of which 20% were fatal. The authors created low (03 points), intermediate (45 points), and high (67 points) risk categories. In the validation cohorts the prediction rule for the ABCD2 functioned better than the California or ABCD scores with c statistics of 0.62 to 0.83 (ideal prediction produces a c statistic of 1 and prediction no better than chance would have a c statistic of 0.5). They found a 2‐day risk of stroke of 1% for low, 4.1% for intermediate, and 8.1% for the high risk group. Data from the study suggest 34% of TIA patients will be in low‐risk, 45% in intermediate‐risk, and 21% in high‐risk categories. While the ABCD2 score overcomes some of the problems with the 2 prior systems it shares many of the limitations as it was derived from the combined data sets. All scoring systems lack the ability to provide guidance on the management of TIAs associated with rare conditions, such as vasculitis, that are underrepresented in the derivation data sets. The ABCD2 also does not incorporate imaging data and this will likely require further exploration.
ABCD2 Score | 0‐3 | 4‐5 | 6‐7 |
---|---|---|---|
| |||
Risk stratification | Low | Intermediate | High |
2‐Day stroke risk (%) | 1 | 4.1 | 8.1 |
7‐Day stroke risk (%) | 1.2 | 5.9 | 11.7 |
90‐Day stroke risk (%) | 3.1 | 9.8 | 17.8 |
The ABCD2 score can be used to predict risk for a variety of time intervals, has now been validated in independent Greek and British populations, and appears to be the best performing tool at predicting early risk of stroke regardless of underlying etiology.23, 31, 32 The authors suggest that admission for patients in the high‐risk group is prudent whereas outpatient evaluation is reasonable for patients in the low‐risk group.23 Admission for patients in the intermediate‐risk group will depend on individualized decision making, local practice standards, and available community resources.
New Models of Care: An Opportunity for Hospitalists
The key to improving TIA outcomes appears to be more contingent on the speed of evaluation and initiation of appropriate therapy than on the location of the care. The EXPRESS trial studied the effect of an immediate access neurovascular clinic providing urgent evaluation and immediate treatment of nonhospitalized TIA patients versus usual care. Statistically significant reductions were seen in time to evaluation, first treatment prescription, and in 90‐day risk of recurrent stroke (10.3% versus 2.1%, P < 0.0001) after the clinic was changed to the rapid evaluation and treatment model.33
The SOS‐TIA study used a 24‐hour access hospital‐based TIA clinic to evaluate the effects of rapid assessment and interventions on hospital length of stay and clinical outcomes.34 The 90‐day stroke rate was 1.24% (95% CI, 0.712.12), which represents a 79% reduction compared to the predicted stroke rate from the ABCD2 scores. With expedited evaluation and treatment, 74% of patients were able to be sent home on the same day.
The results of these 2 new studies provide compelling evidence that rapid evaluation and treatment in the first 48 hours after TIA has the potential to alter outcomes. Unfortunately not all communities have access to same day TIA clinics. Still, these findings should embolden hospitalists to advocate for urgent evaluation, such as neurology and cardiac imaging and carotid evaluation, with immediate initiation of secondary preventive therapy and early surgical intervention when appropriate. In most cases these changes will require process transformations that present prime opportunities for hospitalists to reengineer systems of care.
Incorporating Prognostic Scores into Clinical Practice
Applying the evidence to practice requires calculation of the early risk but also awareness of the community resources available. High‐risk patients with an ABCD2 score of 6 or 7 have a very high 8.1% risk of stroke within the next 48 hours. Given the catastrophic outcomes frequently seen after second strokes, these patients warrant inpatient admission to facilitate the immediate initiation of appropriate secondary prevention and potentially shorten time to thrombolysis if an early stroke occurs. Intermediate‐risk patients with ABCD2 scores of 4 and 5 have a 4.1% 2‐day risk of stroke and may be considered for admission, hospital observation, or expedited clinic evaluation contingent on local availability. As many as one‐third of TIA patients will be categorized as low risk with a score of 0 to 3. These patients have a 2‐day risk of stroke of only 1% and are likely safe for prompt outpatient evaluation and management. The new, validated, ABCD2 score is not a substitute for individualized judgment, but is helpful in developing admission guidelines in cooperation between neurologists, emergency room physicians, and hospitalists, and in using evidence‐based medicine to provide optimal care for the patient presenting with a TIA.
Stroke and TIA arise from identical etiologies, respond to the same secondary preventive measures, and should be considered part of the spectrum of an ischemic cerebral syndrome. Recognizing TIA as a medical emergency with high rates of secondary stroke and subsequent disability allows institution of therapies with appropriate urgency. Hospitalization offers the ability to rapidly coordinate the testing and secondary prevention measures but also, for high‐risk patients, offers the opportunity to reduce the time to thrombolysis for early recurrent strokes. New, validated scoring systems such as the ABCD2 score help the hospitalist to decide which patients are appropriate for admission and which can be managed in progressive and traditional outpatient settings.
- Epidemiological impact in the United States of a tissue‐based definition of transient ischemic attack.Stroke.2003;34:919–924. , , .
- Head computed tomography findings predict short‐term stroke risk after transient ischemic attack.Stroke.2003;34:2894–2899. , , , et al.
- Short‐term prognosis after emergency department diagnosis of TIA.JAMA.2000;284:2901–2906. , , , et al.
- Management and outcomes of transient ischemic attacks in Ontario.CMAJ.2004;170:1099–1104. , , , et al.
- The high risk of stroke immediately after transient ischemic attack: a population based study.Neurology.2004;62:2015–2020. , , , et al.
- Early risk of stroke after transient ischemic attack: a systematic review and meta‐analysis.Arch Intern Med.2007;167:2417–2422. , , , et al.
- The spectrum of community‐based hospitalist practice: a call to tailor internal medicine residency training.Arch Intern Med.2007;167:727–729. , , , et al.
- Transient ischemic attack with abnormal diffusion‐weighted imaging results: what's in a name?Arch Neurol.2007;64:1080–1082. .
- A reappraisal of the definition and pathophysiology of the transient ischemic attack.Med Sci Monit.2007;13:RA50–53. , .
- Diffusion‐weighted imaging‐negative patients with transient ischemic attack are at risk of recurrent transient events.Stroke.2007;38:2367–2369. , , , et al.
- Transient ischemic attack—proposal for a new definition.N Engl J Med.2002;347:1713–1716. , , , et al.
- Management and outcome of patients with transient ischemic attack admitted to a stroke unit.Cerebrovasc Dis.2007;24:80–85. , , , et al.
- How transient are transient ischemic attacks.Neurology.1988;38:674–677. .
- National Stroke Association guidelines for the management of transient ischemic attacks.Ann Neurol.2006;60:301–313. , , , et al.
- Out‐of‐hospital delays in patients with acute stroke.Ann Emerg Med.2004;44:476–483. , , , et al.
- Factors associated with delayed admission to hospital and in‐hospital delays in acute stroke and TIA: a prospective multicenter study.Stroke.1999;30:40–48. , , , et al.
- Prehospital and emergency department delays after acute stroke: the Genentech Stroke Presentation Survey.Stroke.2000;31:2585–2590. , , , et al.
- Tissue plasminogen activator for acute ischemic stroke.The National Institute of Neurological Disorders and Stroke rt‐PA Stroke Study Group.N Engl J Med.1995;333:1581–1587.
- Is hospitalization after TIA cost effective on the basis of treatment with tPA?Neurology.2005;65:1799–1801. , .
- Endarterectomy for symptomatic carotid stenosis in relation to clinical subgroups and timing of surgery.Lancet.2004;363:915–924. , , , et al.
- New transient ischemic attack and stroke: outpatient management by primary care physicians.Arch Intern Med.2000;160:2941–2946. , , , et al.
- Hospital and demographic influences on the disposition of transient ischemic attack.Acad Emerg Med.2008;15:171–176. , , , et al.
- Validation and refinement of scores to predict very early stroke risk after transient ischaemic attack.Lancet.2007;369:283–292. , , , et al.
- A simple score (ABCD) to identify individuals at high early risk of stroke after transient ischaemic attack.Lancet.2005;366:29–36. , , , et al.
- Is the ABCD score useful for risk stratification of patients with acute transient ischemic attack?Stroke.2006;37:1710–1714. , , , et al.
- Absence of usefulness of ABCD score in the early risk of recurrent stroke in transient ischemic attack patients.Stroke.2007;38:855–856. , , , et al.
- Validation of the ABCD score in identifying individuals at high early risk of stroke after a transient ischemic attack: a hospital‐based case series study.Stroke.2006;37:2892–2897. , , , et al.
- Rapid identification of high‐risk transient ischemic attacks: prospective validation of the ABCD score.Stroke.2008;39:297–302. , .
- Can the ABCD score be dichotomized to identify high‐risk patients with transient ischaemic attack in the emergency department?Emerg Med J.2007;24:92–95. , , .
- Can risk stratification of transient ischaemic attacks improve patient care in the emergency department?Emerg Med J.2007;24:637–640. , , , et al.
- Prognosis in patients with transient ischaemic attack (TIA) and minor stroke attending TIA services in the north west of England: The NORTHSTAR Study.J Neurol Neurosurg Psychiatry.2007:1–6. , , , et al.
- Potential applicability of the ABCD2 in triaging TIA patients.Lancet.2007;369:1082. , , .
- Effect of urgent treatment of transient ischemic attack and minor stroke on early recurrent stroke (EXPRESS Study): a prospective population‐based sequential comparison.Lancet.2007;370;1432–1442. , , , et al.
- A transient ischemic attack clinic with round‐the‐clock access (SOS‐TIA): feasibility and effects.Lancet Neurol.2007;6:953–960. , , , et al.
Transient ischemic attacks (TIAs) are common and represent a clarion call to action to prevent disabling stroke. Incidence estimates for TIA range from 37 to 107 per 100,000 persons each year.1 Extrapolating from these data, there are likely greater than 100,000 to 300,000 TIAs in the US annually. Within 3 months, approximately 10% of these patients will suffer a stroke, with approximately one‐half of these events occurring within the first 48 hours after the sentinel TIA.26 Nearly two‐thirds of secondary strokes result in disability and 21% are fatal.3 Hospitalists are frequently called to provide care for patients with TIA and, as such, in order to establish an appropriate care plan, they require tools to better predict the likelihood and timing of a disabling stroke.7 In this review we examine the rationale for early aggressive TIA evaluation and treatment in the hospital, overview risk stratification models to identify the patients at highest risk for early recurrent ischemia, and explore application of these tools to admission policy and individualized patient care planning.
Definition
TIA is defined as a brief episode of neurological dysfunction caused by focal brain or retinal ischemia with clinical symptoms typically lasting less than 1 hour and without evidence of brain infarction.8, 9 Prior arbitrary time limits are being abandoned as advanced imaging techniques demonstrate that clinical examination lacks the sensitivity to detect small cerebral infarctions leading to misclassification of as many as 30% to 40% of strokes as TIAs.811 For cases in which imaging is not available, the diagnosis of clinically probable TIA is suggested. Patients with imaging consistent with stroke appear to be at 4‐fold to 10‐fold higher risk for subsequent ischemic events, thus the presence of subclinical infarcts may have clinical importance.2, 12 The majority of TIAs resolve within 1 hour of onset and neurologic deficit continuance beyond this time frame is more consistent with a stroke.13 Continuing symptoms after 1 hour mandates aggressive therapy in lieu of withholding intervention in the hopes of a spontaneous recovery.
Rationale for Hospitalization
Urgent evaluation and treatment within 24 to 48 hours of a TIA is recommended by the National Stroke Association (Table 1).14 These guidelines also recommend hospital admission for high‐risk patients. There are a number of compelling arguments for the hospitalization of a patient at high risk for subsequent stroke.
Test | Rationale | Therapy |
---|---|---|
| ||
Electrocardiogram and rhythm strip | To detect atrial fibrillation. | Long‐term warfarin indicated for suspected cardioembolic etiology. |
Echocardiogram | To detect intracardiac thrombus or vegetations. Bubble study to detect patent foramen ovale in young patients. | Warfarin indicated for suspected cardioembolic etiology. Patent foramen ovale closure is an option for selected patients. |
Carotid ultrasound | To detect large vessel atherosclerotic disease. | Antiplatelet therapy* indicated for atherosclerotic etiologies. Early carotid endarterectomy following TIA considered for 50% symptomatic ipsilateral stenosis. |
Fasting lipid profile, complete blood count, serum electrolytes and creatinine | Secondary prevention of stroke by treating hyperlipidemia. Signs and symptoms associated with severe laboratory abnormalities may mimic TIA. | LDL >100 mg/dL (optional goal >70 mg/dL) is indication for cholesterol lowering therapy. |
Neuroimaging‐MRI with diffusion images MRA or CT CTA | To detect clinically inapparent lesions of stroke. Useful in ruling out some mimics of TIA. | Patients with abnormal MRI diffusion images represent a population at increased risk for recurrent stroke. |
First, hospitalization offers potential for reduced time to thrombolysis for those patients who have a second ischemic event in the early period following TIA. Outpatients with new ischemic stroke may see hours pass between symptom onset and presentation to the emergency department (ED). This delay frequently places them outside of the thrombolytic window.1517 Hospitalization, assuming a well‐designed inpatient stroke care system, has great potential to reduce this delay. Approximately 50% of the stroke risk following a TIA is evident within 48 hours and rapid thrombolysis, available in an inpatient setting, is associated with improved outcome after stroke.3, 18 A cost‐utility analysis found that a 24‐hour admission for TIA patients to allow tissue plasminogen activator (t‐PA) for recurrent ischemia has a cost‐effectiveness ratio of $55,044 per quality‐adjusted life year with increasing cost effectiveness for the highest risk patients, such as those with a 24‐hour stroke risk of >5%.19
Second, hospital admission often facilitates the reliable and efficient evaluation for etiology and early initiation of secondary prevention. Neuroimaging, carotid ultrasound, echocardiography, and telemetry can be expedited with rapid initiation of proven secondary preventive therapies such as statin treatment, blood pressure control, and antithrombotic therapy. When indicated, carotid revascularization is recommended as soon as possible following TIA, with retrospective reviews suggesting improved outcomes when performed within 2 weeks of the event.1420 In one analysis, a negative association between hospitalization for TIA and subsequent stroke was discovered by review of Canadian population‐based administrative databases.5 While the mechanism for the negative association could not be established, the literature provides some support for hospitalization being associated with decreased risk for second strokes (hazard ratio [HR], 0.73; 95% confidence interval [CI], 0.570.95).5
Theoretically, much of this evaluation and treatment could occur in the outpatient setting but delays commonly seen in outpatient evaluation and the high potential for early second strokes for some patients may make this a risky care plan. Despite the high likelihood for serious outcomes following TIA and clear guidelines for early evaluation and management, current care often lacks a sense of urgency. A 2004 Canadian study revealed that three‐quarters of patients with a TIA were discharged directly from the ED with a resultant delay in diagnostic investigation.4 Over one‐third of patients were discharged without a prescription for antithrombotic therapy. American primary care practice patterns reveal even more significant delays in therapy, with only 2% of patients admitted to a hospital on the day of presentation for TIA, despite 80% of patients presenting for evaluation on the day of symptom onset.21 In this study less than one‐half of patients with atrial fibrillation were started on immediate anticoagulation.21 Further, as many as one‐third of patients did not have any evaluation in the month after the index event.21 Hospitalization for high‐risk patients has the potential to avoid these delays in outpatient evaluation and initiation of therapy.
Still, not all patients will require admission to a hospital setting. American EDs admit approximately one‐half of all TIAs, with regional variability not explained exclusively by clinical characteristics.22 Focusing on identifying the cohort of patients who would most benefit from hospitalization is paramount. In general, hospitalization should be reserved for patients with higher risk of an early secondary stroke. Specifically, admission is generally recommended for patients with crescendo symptoms, TIA on antithrombotic therapy, or symptoms lasting >1 hour.14 Additionally, patients with symptomatic carotid stenosis of 50% and presumed cardioembolic or hypercoagulable etiology merit hospital admission.14 In many cases these etiologies may not be known at time of presentation. Evaluation, such as carotid ultrasound, may not be readily available in the ED to inform the admission decision. Several new scoring systems that utilize routine clinical features available within an hour of presentation have been developed to more objectively assess the risk of secondary stroke following a TIA. The use of these prognostic scoring systems is recommended by the National Stroke Association to aid in triaging this cohort of patients.14
Prognostic Scoring Systems
California Score
The 2000 California Score (Tables 2 and 3) is a 5‐point tool retrospectively developed from a database of 1,707 TIA patients seen in the ED of a California hospital system to predict the likelihood of stroke within 90 days of an initial presentation with transient neurologic deficits.3 Multivariate logistic regression models were used to test the clinical factors associated with stroke risk, resulting in a tool using clinical features of age, diabetes, symptom duration, and the type of deficit to provide quantitative estimates of intermediate term risk. Within 90 days, none of the patients with a score of zero had a stroke whereas the highest score had a 34% risk of stroke (Table 3). While it is possible that some patients with lower scores had a nonischemic etiology for their transient neurological symptoms, clinical practice contains similar ambiguity, and such patients would be correctly predicted to have a low risk for subsequent strokes. Additionally, the derivation and validation of this tool did not include a neurologist confirmation of TIA diagnosis; however, this likely mirrors the practice in most EDs. The California Score has subsequently been validated and expanded upon during the development of the ABCD2 score.23 The California Score's utility is limited in the acute decision‐making process, especially regarding the decision to admit, as it focuses on 90‐day outcomes. For that, shorter‐term risk assessments are more useful.
Clinical Feature | California Score (points) | ABCD (points) | ABCD2 (points) | |
---|---|---|---|---|
| ||||
Age | 60 years | 1 | 1 | 1 |
Blood pressure | Systolic blood pressure 140 or diastolic blood pressure 90 mmHg | N/A | 1 | 1 |
Clinical deficits* | Unilateral weakness (focal motor weakness of 1 or more of face, arm, hand, or leg) | 1 | 2 | 2 |
Speech impairment (dysarthria, dysphasia, or both) | 1 | 1 | 1 | |
Duration | 60 minutes | 1 | 2 | 2 |
10‐59 minutes | 1 | 1 | 1 | |
Diabetes | Present | 1 | N/A | 1 |
Maximum score | 5 | 6 | 7 |
California Score | 0 | 1 | 2 | 3 | 4 | 5 |
---|---|---|---|---|---|---|
| ||||||
90‐day stroke risk (%) | 0 | 3 | 7 | 11 | 15 | 34 |
ABCD Score
The 2005 ABCD (Tables 2 and 4) is a 6‐point tool designed to predict 7‐day risk of stroke following TIA from the Oxfordshire Community Stroke Project, a UK prospective population‐based cohort of 209 patients with diagnosis of TIA made by a neurologist.24 It evaluated factors previously found to be independent predictors of stroke after TIA, and determined that risk factors of age, blood pressure, type of clinical deficit, and symptom duration predicted 7‐day risk of stroke following TIA. Unlike the California score, the ABCD authors found presenting blood pressure, but not diabetes, to be independent predictors of future events. The authors validated the score with a second population of TIA patients in the Oxford Vascular Study and in a hospital‐based TIA clinic cohort.24 In the validation cohorts the score was highly predictive of stroke at 7 days (P < 0.001). Patients with the lowest scores of 0 to 3 had no strokes in the week following the index TIA, whereas patients with the highest score of 6 carried a 35.5% risk of early second stroke. The primary limitation of the ABCD score lies in the small sample size, with only 18 recurrent strokes in the week after TIA in the derivation cohort.
ABCD Score | 0 | 1 | 2 | 3 | 4 | 5 | 6 |
---|---|---|---|---|---|---|---|
| |||||||
7‐Day stroke risk (%) | 0 | 0 | 0 | 0 | 2.2 | 16.3 | 35.5 |
The ABCD score has subsequently been tested in other settings with mixed results. Two studies found limited utility.25, 26 Other trials found high scores to be overly inclusive but highly predictive and thus the majority of evidence appears to support the validity of the ABCD score in predicting risk of early recurrent ischemic events.2729 The ABCD score has been used to triage patients with high scores to inpatient management although the benefit of this strategy on outcomes has not been proven.30
ABCD2 Score
The 2007 ABCD2 (Tables 2 and 5) is a 7‐point tool that uses the original ABCD criteria along with an additional point for diabetes.23 The ABCD2 derived a unified prognostic score for optimal prediction of the 2‐day stroke risk from multivariate analysis of the original data sets used to create the California and ABCD scores. This score was then validated by the authors in 4 independent cohorts from the United States and the United Kingdom.23 In sum, 4809 patients with TIA were included in the ABCD2 analysis. Similar to prior studies, stroke occurred in 9.2% of patients by 90 days of which 20% were fatal. The authors created low (03 points), intermediate (45 points), and high (67 points) risk categories. In the validation cohorts the prediction rule for the ABCD2 functioned better than the California or ABCD scores with c statistics of 0.62 to 0.83 (ideal prediction produces a c statistic of 1 and prediction no better than chance would have a c statistic of 0.5). They found a 2‐day risk of stroke of 1% for low, 4.1% for intermediate, and 8.1% for the high risk group. Data from the study suggest 34% of TIA patients will be in low‐risk, 45% in intermediate‐risk, and 21% in high‐risk categories. While the ABCD2 score overcomes some of the problems with the 2 prior systems it shares many of the limitations as it was derived from the combined data sets. All scoring systems lack the ability to provide guidance on the management of TIAs associated with rare conditions, such as vasculitis, that are underrepresented in the derivation data sets. The ABCD2 also does not incorporate imaging data and this will likely require further exploration.
ABCD2 Score | 0‐3 | 4‐5 | 6‐7 |
---|---|---|---|
| |||
Risk stratification | Low | Intermediate | High |
2‐Day stroke risk (%) | 1 | 4.1 | 8.1 |
7‐Day stroke risk (%) | 1.2 | 5.9 | 11.7 |
90‐Day stroke risk (%) | 3.1 | 9.8 | 17.8 |
The ABCD2 score can be used to predict risk for a variety of time intervals, has now been validated in independent Greek and British populations, and appears to be the best performing tool at predicting early risk of stroke regardless of underlying etiology.23, 31, 32 The authors suggest that admission for patients in the high‐risk group is prudent whereas outpatient evaluation is reasonable for patients in the low‐risk group.23 Admission for patients in the intermediate‐risk group will depend on individualized decision making, local practice standards, and available community resources.
New Models of Care: An Opportunity for Hospitalists
The key to improving TIA outcomes appears to be more contingent on the speed of evaluation and initiation of appropriate therapy than on the location of the care. The EXPRESS trial studied the effect of an immediate access neurovascular clinic providing urgent evaluation and immediate treatment of nonhospitalized TIA patients versus usual care. Statistically significant reductions were seen in time to evaluation, first treatment prescription, and in 90‐day risk of recurrent stroke (10.3% versus 2.1%, P < 0.0001) after the clinic was changed to the rapid evaluation and treatment model.33
The SOS‐TIA study used a 24‐hour access hospital‐based TIA clinic to evaluate the effects of rapid assessment and interventions on hospital length of stay and clinical outcomes.34 The 90‐day stroke rate was 1.24% (95% CI, 0.712.12), which represents a 79% reduction compared to the predicted stroke rate from the ABCD2 scores. With expedited evaluation and treatment, 74% of patients were able to be sent home on the same day.
The results of these 2 new studies provide compelling evidence that rapid evaluation and treatment in the first 48 hours after TIA has the potential to alter outcomes. Unfortunately not all communities have access to same day TIA clinics. Still, these findings should embolden hospitalists to advocate for urgent evaluation, such as neurology and cardiac imaging and carotid evaluation, with immediate initiation of secondary preventive therapy and early surgical intervention when appropriate. In most cases these changes will require process transformations that present prime opportunities for hospitalists to reengineer systems of care.
Incorporating Prognostic Scores into Clinical Practice
Applying the evidence to practice requires calculation of the early risk but also awareness of the community resources available. High‐risk patients with an ABCD2 score of 6 or 7 have a very high 8.1% risk of stroke within the next 48 hours. Given the catastrophic outcomes frequently seen after second strokes, these patients warrant inpatient admission to facilitate the immediate initiation of appropriate secondary prevention and potentially shorten time to thrombolysis if an early stroke occurs. Intermediate‐risk patients with ABCD2 scores of 4 and 5 have a 4.1% 2‐day risk of stroke and may be considered for admission, hospital observation, or expedited clinic evaluation contingent on local availability. As many as one‐third of TIA patients will be categorized as low risk with a score of 0 to 3. These patients have a 2‐day risk of stroke of only 1% and are likely safe for prompt outpatient evaluation and management. The new, validated, ABCD2 score is not a substitute for individualized judgment, but is helpful in developing admission guidelines in cooperation between neurologists, emergency room physicians, and hospitalists, and in using evidence‐based medicine to provide optimal care for the patient presenting with a TIA.
Stroke and TIA arise from identical etiologies, respond to the same secondary preventive measures, and should be considered part of the spectrum of an ischemic cerebral syndrome. Recognizing TIA as a medical emergency with high rates of secondary stroke and subsequent disability allows institution of therapies with appropriate urgency. Hospitalization offers the ability to rapidly coordinate the testing and secondary prevention measures but also, for high‐risk patients, offers the opportunity to reduce the time to thrombolysis for early recurrent strokes. New, validated scoring systems such as the ABCD2 score help the hospitalist to decide which patients are appropriate for admission and which can be managed in progressive and traditional outpatient settings.
Transient ischemic attacks (TIAs) are common and represent a clarion call to action to prevent disabling stroke. Incidence estimates for TIA range from 37 to 107 per 100,000 persons each year.1 Extrapolating from these data, there are likely greater than 100,000 to 300,000 TIAs in the US annually. Within 3 months, approximately 10% of these patients will suffer a stroke, with approximately one‐half of these events occurring within the first 48 hours after the sentinel TIA.26 Nearly two‐thirds of secondary strokes result in disability and 21% are fatal.3 Hospitalists are frequently called to provide care for patients with TIA and, as such, in order to establish an appropriate care plan, they require tools to better predict the likelihood and timing of a disabling stroke.7 In this review we examine the rationale for early aggressive TIA evaluation and treatment in the hospital, overview risk stratification models to identify the patients at highest risk for early recurrent ischemia, and explore application of these tools to admission policy and individualized patient care planning.
Definition
TIA is defined as a brief episode of neurological dysfunction caused by focal brain or retinal ischemia with clinical symptoms typically lasting less than 1 hour and without evidence of brain infarction.8, 9 Prior arbitrary time limits are being abandoned as advanced imaging techniques demonstrate that clinical examination lacks the sensitivity to detect small cerebral infarctions leading to misclassification of as many as 30% to 40% of strokes as TIAs.811 For cases in which imaging is not available, the diagnosis of clinically probable TIA is suggested. Patients with imaging consistent with stroke appear to be at 4‐fold to 10‐fold higher risk for subsequent ischemic events, thus the presence of subclinical infarcts may have clinical importance.2, 12 The majority of TIAs resolve within 1 hour of onset and neurologic deficit continuance beyond this time frame is more consistent with a stroke.13 Continuing symptoms after 1 hour mandates aggressive therapy in lieu of withholding intervention in the hopes of a spontaneous recovery.
Rationale for Hospitalization
Urgent evaluation and treatment within 24 to 48 hours of a TIA is recommended by the National Stroke Association (Table 1).14 These guidelines also recommend hospital admission for high‐risk patients. There are a number of compelling arguments for the hospitalization of a patient at high risk for subsequent stroke.
Test | Rationale | Therapy |
---|---|---|
| ||
Electrocardiogram and rhythm strip | To detect atrial fibrillation. | Long‐term warfarin indicated for suspected cardioembolic etiology. |
Echocardiogram | To detect intracardiac thrombus or vegetations. Bubble study to detect patent foramen ovale in young patients. | Warfarin indicated for suspected cardioembolic etiology. Patent foramen ovale closure is an option for selected patients. |
Carotid ultrasound | To detect large vessel atherosclerotic disease. | Antiplatelet therapy* indicated for atherosclerotic etiologies. Early carotid endarterectomy following TIA considered for 50% symptomatic ipsilateral stenosis. |
Fasting lipid profile, complete blood count, serum electrolytes and creatinine | Secondary prevention of stroke by treating hyperlipidemia. Signs and symptoms associated with severe laboratory abnormalities may mimic TIA. | LDL >100 mg/dL (optional goal >70 mg/dL) is indication for cholesterol lowering therapy. |
Neuroimaging‐MRI with diffusion images MRA or CT CTA | To detect clinically inapparent lesions of stroke. Useful in ruling out some mimics of TIA. | Patients with abnormal MRI diffusion images represent a population at increased risk for recurrent stroke. |
First, hospitalization offers potential for reduced time to thrombolysis for those patients who have a second ischemic event in the early period following TIA. Outpatients with new ischemic stroke may see hours pass between symptom onset and presentation to the emergency department (ED). This delay frequently places them outside of the thrombolytic window.1517 Hospitalization, assuming a well‐designed inpatient stroke care system, has great potential to reduce this delay. Approximately 50% of the stroke risk following a TIA is evident within 48 hours and rapid thrombolysis, available in an inpatient setting, is associated with improved outcome after stroke.3, 18 A cost‐utility analysis found that a 24‐hour admission for TIA patients to allow tissue plasminogen activator (t‐PA) for recurrent ischemia has a cost‐effectiveness ratio of $55,044 per quality‐adjusted life year with increasing cost effectiveness for the highest risk patients, such as those with a 24‐hour stroke risk of >5%.19
Second, hospital admission often facilitates the reliable and efficient evaluation for etiology and early initiation of secondary prevention. Neuroimaging, carotid ultrasound, echocardiography, and telemetry can be expedited with rapid initiation of proven secondary preventive therapies such as statin treatment, blood pressure control, and antithrombotic therapy. When indicated, carotid revascularization is recommended as soon as possible following TIA, with retrospective reviews suggesting improved outcomes when performed within 2 weeks of the event.1420 In one analysis, a negative association between hospitalization for TIA and subsequent stroke was discovered by review of Canadian population‐based administrative databases.5 While the mechanism for the negative association could not be established, the literature provides some support for hospitalization being associated with decreased risk for second strokes (hazard ratio [HR], 0.73; 95% confidence interval [CI], 0.570.95).5
Theoretically, much of this evaluation and treatment could occur in the outpatient setting but delays commonly seen in outpatient evaluation and the high potential for early second strokes for some patients may make this a risky care plan. Despite the high likelihood for serious outcomes following TIA and clear guidelines for early evaluation and management, current care often lacks a sense of urgency. A 2004 Canadian study revealed that three‐quarters of patients with a TIA were discharged directly from the ED with a resultant delay in diagnostic investigation.4 Over one‐third of patients were discharged without a prescription for antithrombotic therapy. American primary care practice patterns reveal even more significant delays in therapy, with only 2% of patients admitted to a hospital on the day of presentation for TIA, despite 80% of patients presenting for evaluation on the day of symptom onset.21 In this study less than one‐half of patients with atrial fibrillation were started on immediate anticoagulation.21 Further, as many as one‐third of patients did not have any evaluation in the month after the index event.21 Hospitalization for high‐risk patients has the potential to avoid these delays in outpatient evaluation and initiation of therapy.
Still, not all patients will require admission to a hospital setting. American EDs admit approximately one‐half of all TIAs, with regional variability not explained exclusively by clinical characteristics.22 Focusing on identifying the cohort of patients who would most benefit from hospitalization is paramount. In general, hospitalization should be reserved for patients with higher risk of an early secondary stroke. Specifically, admission is generally recommended for patients with crescendo symptoms, TIA on antithrombotic therapy, or symptoms lasting >1 hour.14 Additionally, patients with symptomatic carotid stenosis of 50% and presumed cardioembolic or hypercoagulable etiology merit hospital admission.14 In many cases these etiologies may not be known at time of presentation. Evaluation, such as carotid ultrasound, may not be readily available in the ED to inform the admission decision. Several new scoring systems that utilize routine clinical features available within an hour of presentation have been developed to more objectively assess the risk of secondary stroke following a TIA. The use of these prognostic scoring systems is recommended by the National Stroke Association to aid in triaging this cohort of patients.14
Prognostic Scoring Systems
California Score
The 2000 California Score (Tables 2 and 3) is a 5‐point tool retrospectively developed from a database of 1,707 TIA patients seen in the ED of a California hospital system to predict the likelihood of stroke within 90 days of an initial presentation with transient neurologic deficits.3 Multivariate logistic regression models were used to test the clinical factors associated with stroke risk, resulting in a tool using clinical features of age, diabetes, symptom duration, and the type of deficit to provide quantitative estimates of intermediate term risk. Within 90 days, none of the patients with a score of zero had a stroke whereas the highest score had a 34% risk of stroke (Table 3). While it is possible that some patients with lower scores had a nonischemic etiology for their transient neurological symptoms, clinical practice contains similar ambiguity, and such patients would be correctly predicted to have a low risk for subsequent strokes. Additionally, the derivation and validation of this tool did not include a neurologist confirmation of TIA diagnosis; however, this likely mirrors the practice in most EDs. The California Score has subsequently been validated and expanded upon during the development of the ABCD2 score.23 The California Score's utility is limited in the acute decision‐making process, especially regarding the decision to admit, as it focuses on 90‐day outcomes. For that, shorter‐term risk assessments are more useful.
Clinical Feature | California Score (points) | ABCD (points) | ABCD2 (points) | |
---|---|---|---|---|
| ||||
Age | 60 years | 1 | 1 | 1 |
Blood pressure | Systolic blood pressure 140 or diastolic blood pressure 90 mmHg | N/A | 1 | 1 |
Clinical deficits* | Unilateral weakness (focal motor weakness of 1 or more of face, arm, hand, or leg) | 1 | 2 | 2 |
Speech impairment (dysarthria, dysphasia, or both) | 1 | 1 | 1 | |
Duration | 60 minutes | 1 | 2 | 2 |
10‐59 minutes | 1 | 1 | 1 | |
Diabetes | Present | 1 | N/A | 1 |
Maximum score | 5 | 6 | 7 |
California Score | 0 | 1 | 2 | 3 | 4 | 5 |
---|---|---|---|---|---|---|
| ||||||
90‐day stroke risk (%) | 0 | 3 | 7 | 11 | 15 | 34 |
ABCD Score
The 2005 ABCD (Tables 2 and 4) is a 6‐point tool designed to predict 7‐day risk of stroke following TIA from the Oxfordshire Community Stroke Project, a UK prospective population‐based cohort of 209 patients with diagnosis of TIA made by a neurologist.24 It evaluated factors previously found to be independent predictors of stroke after TIA, and determined that risk factors of age, blood pressure, type of clinical deficit, and symptom duration predicted 7‐day risk of stroke following TIA. Unlike the California score, the ABCD authors found presenting blood pressure, but not diabetes, to be independent predictors of future events. The authors validated the score with a second population of TIA patients in the Oxford Vascular Study and in a hospital‐based TIA clinic cohort.24 In the validation cohorts the score was highly predictive of stroke at 7 days (P < 0.001). Patients with the lowest scores of 0 to 3 had no strokes in the week following the index TIA, whereas patients with the highest score of 6 carried a 35.5% risk of early second stroke. The primary limitation of the ABCD score lies in the small sample size, with only 18 recurrent strokes in the week after TIA in the derivation cohort.
ABCD Score | 0 | 1 | 2 | 3 | 4 | 5 | 6 |
---|---|---|---|---|---|---|---|
| |||||||
7‐Day stroke risk (%) | 0 | 0 | 0 | 0 | 2.2 | 16.3 | 35.5 |
The ABCD score has subsequently been tested in other settings with mixed results. Two studies found limited utility.25, 26 Other trials found high scores to be overly inclusive but highly predictive and thus the majority of evidence appears to support the validity of the ABCD score in predicting risk of early recurrent ischemic events.2729 The ABCD score has been used to triage patients with high scores to inpatient management although the benefit of this strategy on outcomes has not been proven.30
ABCD2 Score
The 2007 ABCD2 (Tables 2 and 5) is a 7‐point tool that uses the original ABCD criteria along with an additional point for diabetes.23 The ABCD2 derived a unified prognostic score for optimal prediction of the 2‐day stroke risk from multivariate analysis of the original data sets used to create the California and ABCD scores. This score was then validated by the authors in 4 independent cohorts from the United States and the United Kingdom.23 In sum, 4809 patients with TIA were included in the ABCD2 analysis. Similar to prior studies, stroke occurred in 9.2% of patients by 90 days of which 20% were fatal. The authors created low (03 points), intermediate (45 points), and high (67 points) risk categories. In the validation cohorts the prediction rule for the ABCD2 functioned better than the California or ABCD scores with c statistics of 0.62 to 0.83 (ideal prediction produces a c statistic of 1 and prediction no better than chance would have a c statistic of 0.5). They found a 2‐day risk of stroke of 1% for low, 4.1% for intermediate, and 8.1% for the high risk group. Data from the study suggest 34% of TIA patients will be in low‐risk, 45% in intermediate‐risk, and 21% in high‐risk categories. While the ABCD2 score overcomes some of the problems with the 2 prior systems it shares many of the limitations as it was derived from the combined data sets. All scoring systems lack the ability to provide guidance on the management of TIAs associated with rare conditions, such as vasculitis, that are underrepresented in the derivation data sets. The ABCD2 also does not incorporate imaging data and this will likely require further exploration.
ABCD2 Score | 0‐3 | 4‐5 | 6‐7 |
---|---|---|---|
| |||
Risk stratification | Low | Intermediate | High |
2‐Day stroke risk (%) | 1 | 4.1 | 8.1 |
7‐Day stroke risk (%) | 1.2 | 5.9 | 11.7 |
90‐Day stroke risk (%) | 3.1 | 9.8 | 17.8 |
The ABCD2 score can be used to predict risk for a variety of time intervals, has now been validated in independent Greek and British populations, and appears to be the best performing tool at predicting early risk of stroke regardless of underlying etiology.23, 31, 32 The authors suggest that admission for patients in the high‐risk group is prudent whereas outpatient evaluation is reasonable for patients in the low‐risk group.23 Admission for patients in the intermediate‐risk group will depend on individualized decision making, local practice standards, and available community resources.
New Models of Care: An Opportunity for Hospitalists
The key to improving TIA outcomes appears to be more contingent on the speed of evaluation and initiation of appropriate therapy than on the location of the care. The EXPRESS trial studied the effect of an immediate access neurovascular clinic providing urgent evaluation and immediate treatment of nonhospitalized TIA patients versus usual care. Statistically significant reductions were seen in time to evaluation, first treatment prescription, and in 90‐day risk of recurrent stroke (10.3% versus 2.1%, P < 0.0001) after the clinic was changed to the rapid evaluation and treatment model.33
The SOS‐TIA study used a 24‐hour access hospital‐based TIA clinic to evaluate the effects of rapid assessment and interventions on hospital length of stay and clinical outcomes.34 The 90‐day stroke rate was 1.24% (95% CI, 0.712.12), which represents a 79% reduction compared to the predicted stroke rate from the ABCD2 scores. With expedited evaluation and treatment, 74% of patients were able to be sent home on the same day.
The results of these 2 new studies provide compelling evidence that rapid evaluation and treatment in the first 48 hours after TIA has the potential to alter outcomes. Unfortunately not all communities have access to same day TIA clinics. Still, these findings should embolden hospitalists to advocate for urgent evaluation, such as neurology and cardiac imaging and carotid evaluation, with immediate initiation of secondary preventive therapy and early surgical intervention when appropriate. In most cases these changes will require process transformations that present prime opportunities for hospitalists to reengineer systems of care.
Incorporating Prognostic Scores into Clinical Practice
Applying the evidence to practice requires calculation of the early risk but also awareness of the community resources available. High‐risk patients with an ABCD2 score of 6 or 7 have a very high 8.1% risk of stroke within the next 48 hours. Given the catastrophic outcomes frequently seen after second strokes, these patients warrant inpatient admission to facilitate the immediate initiation of appropriate secondary prevention and potentially shorten time to thrombolysis if an early stroke occurs. Intermediate‐risk patients with ABCD2 scores of 4 and 5 have a 4.1% 2‐day risk of stroke and may be considered for admission, hospital observation, or expedited clinic evaluation contingent on local availability. As many as one‐third of TIA patients will be categorized as low risk with a score of 0 to 3. These patients have a 2‐day risk of stroke of only 1% and are likely safe for prompt outpatient evaluation and management. The new, validated, ABCD2 score is not a substitute for individualized judgment, but is helpful in developing admission guidelines in cooperation between neurologists, emergency room physicians, and hospitalists, and in using evidence‐based medicine to provide optimal care for the patient presenting with a TIA.
Stroke and TIA arise from identical etiologies, respond to the same secondary preventive measures, and should be considered part of the spectrum of an ischemic cerebral syndrome. Recognizing TIA as a medical emergency with high rates of secondary stroke and subsequent disability allows institution of therapies with appropriate urgency. Hospitalization offers the ability to rapidly coordinate the testing and secondary prevention measures but also, for high‐risk patients, offers the opportunity to reduce the time to thrombolysis for early recurrent strokes. New, validated scoring systems such as the ABCD2 score help the hospitalist to decide which patients are appropriate for admission and which can be managed in progressive and traditional outpatient settings.
- Epidemiological impact in the United States of a tissue‐based definition of transient ischemic attack.Stroke.2003;34:919–924. , , .
- Head computed tomography findings predict short‐term stroke risk after transient ischemic attack.Stroke.2003;34:2894–2899. , , , et al.
- Short‐term prognosis after emergency department diagnosis of TIA.JAMA.2000;284:2901–2906. , , , et al.
- Management and outcomes of transient ischemic attacks in Ontario.CMAJ.2004;170:1099–1104. , , , et al.
- The high risk of stroke immediately after transient ischemic attack: a population based study.Neurology.2004;62:2015–2020. , , , et al.
- Early risk of stroke after transient ischemic attack: a systematic review and meta‐analysis.Arch Intern Med.2007;167:2417–2422. , , , et al.
- The spectrum of community‐based hospitalist practice: a call to tailor internal medicine residency training.Arch Intern Med.2007;167:727–729. , , , et al.
- Transient ischemic attack with abnormal diffusion‐weighted imaging results: what's in a name?Arch Neurol.2007;64:1080–1082. .
- A reappraisal of the definition and pathophysiology of the transient ischemic attack.Med Sci Monit.2007;13:RA50–53. , .
- Diffusion‐weighted imaging‐negative patients with transient ischemic attack are at risk of recurrent transient events.Stroke.2007;38:2367–2369. , , , et al.
- Transient ischemic attack—proposal for a new definition.N Engl J Med.2002;347:1713–1716. , , , et al.
- Management and outcome of patients with transient ischemic attack admitted to a stroke unit.Cerebrovasc Dis.2007;24:80–85. , , , et al.
- How transient are transient ischemic attacks.Neurology.1988;38:674–677. .
- National Stroke Association guidelines for the management of transient ischemic attacks.Ann Neurol.2006;60:301–313. , , , et al.
- Out‐of‐hospital delays in patients with acute stroke.Ann Emerg Med.2004;44:476–483. , , , et al.
- Factors associated with delayed admission to hospital and in‐hospital delays in acute stroke and TIA: a prospective multicenter study.Stroke.1999;30:40–48. , , , et al.
- Prehospital and emergency department delays after acute stroke: the Genentech Stroke Presentation Survey.Stroke.2000;31:2585–2590. , , , et al.
- Tissue plasminogen activator for acute ischemic stroke.The National Institute of Neurological Disorders and Stroke rt‐PA Stroke Study Group.N Engl J Med.1995;333:1581–1587.
- Is hospitalization after TIA cost effective on the basis of treatment with tPA?Neurology.2005;65:1799–1801. , .
- Endarterectomy for symptomatic carotid stenosis in relation to clinical subgroups and timing of surgery.Lancet.2004;363:915–924. , , , et al.
- New transient ischemic attack and stroke: outpatient management by primary care physicians.Arch Intern Med.2000;160:2941–2946. , , , et al.
- Hospital and demographic influences on the disposition of transient ischemic attack.Acad Emerg Med.2008;15:171–176. , , , et al.
- Validation and refinement of scores to predict very early stroke risk after transient ischaemic attack.Lancet.2007;369:283–292. , , , et al.
- A simple score (ABCD) to identify individuals at high early risk of stroke after transient ischaemic attack.Lancet.2005;366:29–36. , , , et al.
- Is the ABCD score useful for risk stratification of patients with acute transient ischemic attack?Stroke.2006;37:1710–1714. , , , et al.
- Absence of usefulness of ABCD score in the early risk of recurrent stroke in transient ischemic attack patients.Stroke.2007;38:855–856. , , , et al.
- Validation of the ABCD score in identifying individuals at high early risk of stroke after a transient ischemic attack: a hospital‐based case series study.Stroke.2006;37:2892–2897. , , , et al.
- Rapid identification of high‐risk transient ischemic attacks: prospective validation of the ABCD score.Stroke.2008;39:297–302. , .
- Can the ABCD score be dichotomized to identify high‐risk patients with transient ischaemic attack in the emergency department?Emerg Med J.2007;24:92–95. , , .
- Can risk stratification of transient ischaemic attacks improve patient care in the emergency department?Emerg Med J.2007;24:637–640. , , , et al.
- Prognosis in patients with transient ischaemic attack (TIA) and minor stroke attending TIA services in the north west of England: The NORTHSTAR Study.J Neurol Neurosurg Psychiatry.2007:1–6. , , , et al.
- Potential applicability of the ABCD2 in triaging TIA patients.Lancet.2007;369:1082. , , .
- Effect of urgent treatment of transient ischemic attack and minor stroke on early recurrent stroke (EXPRESS Study): a prospective population‐based sequential comparison.Lancet.2007;370;1432–1442. , , , et al.
- A transient ischemic attack clinic with round‐the‐clock access (SOS‐TIA): feasibility and effects.Lancet Neurol.2007;6:953–960. , , , et al.
- Epidemiological impact in the United States of a tissue‐based definition of transient ischemic attack.Stroke.2003;34:919–924. , , .
- Head computed tomography findings predict short‐term stroke risk after transient ischemic attack.Stroke.2003;34:2894–2899. , , , et al.
- Short‐term prognosis after emergency department diagnosis of TIA.JAMA.2000;284:2901–2906. , , , et al.
- Management and outcomes of transient ischemic attacks in Ontario.CMAJ.2004;170:1099–1104. , , , et al.
- The high risk of stroke immediately after transient ischemic attack: a population based study.Neurology.2004;62:2015–2020. , , , et al.
- Early risk of stroke after transient ischemic attack: a systematic review and meta‐analysis.Arch Intern Med.2007;167:2417–2422. , , , et al.
- The spectrum of community‐based hospitalist practice: a call to tailor internal medicine residency training.Arch Intern Med.2007;167:727–729. , , , et al.
- Transient ischemic attack with abnormal diffusion‐weighted imaging results: what's in a name?Arch Neurol.2007;64:1080–1082. .
- A reappraisal of the definition and pathophysiology of the transient ischemic attack.Med Sci Monit.2007;13:RA50–53. , .
- Diffusion‐weighted imaging‐negative patients with transient ischemic attack are at risk of recurrent transient events.Stroke.2007;38:2367–2369. , , , et al.
- Transient ischemic attack—proposal for a new definition.N Engl J Med.2002;347:1713–1716. , , , et al.
- Management and outcome of patients with transient ischemic attack admitted to a stroke unit.Cerebrovasc Dis.2007;24:80–85. , , , et al.
- How transient are transient ischemic attacks.Neurology.1988;38:674–677. .
- National Stroke Association guidelines for the management of transient ischemic attacks.Ann Neurol.2006;60:301–313. , , , et al.
- Out‐of‐hospital delays in patients with acute stroke.Ann Emerg Med.2004;44:476–483. , , , et al.
- Factors associated with delayed admission to hospital and in‐hospital delays in acute stroke and TIA: a prospective multicenter study.Stroke.1999;30:40–48. , , , et al.
- Prehospital and emergency department delays after acute stroke: the Genentech Stroke Presentation Survey.Stroke.2000;31:2585–2590. , , , et al.
- Tissue plasminogen activator for acute ischemic stroke.The National Institute of Neurological Disorders and Stroke rt‐PA Stroke Study Group.N Engl J Med.1995;333:1581–1587.
- Is hospitalization after TIA cost effective on the basis of treatment with tPA?Neurology.2005;65:1799–1801. , .
- Endarterectomy for symptomatic carotid stenosis in relation to clinical subgroups and timing of surgery.Lancet.2004;363:915–924. , , , et al.
- New transient ischemic attack and stroke: outpatient management by primary care physicians.Arch Intern Med.2000;160:2941–2946. , , , et al.
- Hospital and demographic influences on the disposition of transient ischemic attack.Acad Emerg Med.2008;15:171–176. , , , et al.
- Validation and refinement of scores to predict very early stroke risk after transient ischaemic attack.Lancet.2007;369:283–292. , , , et al.
- A simple score (ABCD) to identify individuals at high early risk of stroke after transient ischaemic attack.Lancet.2005;366:29–36. , , , et al.
- Is the ABCD score useful for risk stratification of patients with acute transient ischemic attack?Stroke.2006;37:1710–1714. , , , et al.
- Absence of usefulness of ABCD score in the early risk of recurrent stroke in transient ischemic attack patients.Stroke.2007;38:855–856. , , , et al.
- Validation of the ABCD score in identifying individuals at high early risk of stroke after a transient ischemic attack: a hospital‐based case series study.Stroke.2006;37:2892–2897. , , , et al.
- Rapid identification of high‐risk transient ischemic attacks: prospective validation of the ABCD score.Stroke.2008;39:297–302. , .
- Can the ABCD score be dichotomized to identify high‐risk patients with transient ischaemic attack in the emergency department?Emerg Med J.2007;24:92–95. , , .
- Can risk stratification of transient ischaemic attacks improve patient care in the emergency department?Emerg Med J.2007;24:637–640. , , , et al.
- Prognosis in patients with transient ischaemic attack (TIA) and minor stroke attending TIA services in the north west of England: The NORTHSTAR Study.J Neurol Neurosurg Psychiatry.2007:1–6. , , , et al.
- Potential applicability of the ABCD2 in triaging TIA patients.Lancet.2007;369:1082. , , .
- Effect of urgent treatment of transient ischemic attack and minor stroke on early recurrent stroke (EXPRESS Study): a prospective population‐based sequential comparison.Lancet.2007;370;1432–1442. , , , et al.
- A transient ischemic attack clinic with round‐the‐clock access (SOS‐TIA): feasibility and effects.Lancet Neurol.2007;6:953–960. , , , et al.