User login
Health Literacy and Hospital Length of Stay: An Inpatient Cohort Study
Health literacy (HL), defined as patients’ ability to understand health information and make health decisions,1 is a prevalent problem in the outpatient and inpatient settings.2,3 In both settings, low HL has adverse implications for self-care including interpreting health labels4 and taking medications correctly.5 Among outpatient cohorts, HL has been associated with worse outcomes and acute care utilization.6 Associations with low HL include increased hospitalizations,7 rehospitalizations,8,9 emergency department visits,10 and decreased preventative care use.11 Among the elderly, low HL is associated with increased mortality12 and decreased self-perception of health.13
A systematic review revealed that most high-quality HL outcome studies were conducted in the outpatient setting.6 There have been very few studies assessing effects of low HL in an acute-care setting.7,14 These studies have evaluated postdischarge outcomes, including admissions or readmissions,7-9 and medication knowledge.14 To the best of our knowledge, there are no studies evaluating associations between HL and hospital length of stay (LOS).
LOS has received much attention as providers and payers focus more on resource utilization and eliminating adverse effects of prolonged hospitalization.15 LOS is multifactorial, depending on clinical characteristics like disease severity, as well as on sociocultural, demographic, and geographic factors.16 Despite evidence that LOS reductions translate into improved resource allocation and potentially fewer complications, there remains a tension between the appropriate LOS and one that is too short for a given condition.17
Because low HL is associated with inefficient resource utilization, we hypothesized that low HL would be associated with increased LOS after controlling for illness severity. Our objectives were to evaluate the association between low HL and LOS and whether such an association was modified by illness severity and sociodemographics.
METHODS
Study Design, Setting, Participants
An in-hospital, cohort study design of patients who were admitted or transferred to the general medicine service at the University of Chicago between October 2012 and November 2015 and screened for inclusion as part of a large, ongoing study of inpatient care quality was conducted.18 Exclusion criteria included observation status, age under 18 years, non-English speaking, and repeat participants. Those who died during hospitalization or whose discharge status was missing were excluded because the primary goal was to examine the association of HL and time to discharge, which could not be evaluated among those who died. We excluded participants with LOS >30 days to limit overly influential effects of extreme outliers (1% of the population).
Variables
HL was screened using the Brief Health Literacy Screen (BHLS), a validated, 3-question verbal survey not requiring adequate visual acuity to assess HL.19,20 The 3 questions are as follows: (1) “How confident are you filling out medical forms by yourself?”, (2) “How often do you have someone help you read hospital materials?”, and (3) “How often do you have problems learning about your medical condition because of difficulty understanding written information?” Responses to the questions were scored on a 5-point Likert scale in which higher scores corresponded to higher HL.21,22 The scores for each of the 3 questions were summed to yield a range between 3 and 15. On the individual questions, prior work has demonstrated improved test performance with a cutoff of ≤3, which corresponds to a response of “some of the time” or “somewhat”; therefore, when the 3 questions were summed together, scores of ≤9 were considered indicative of low HL.21,23
For severity of illness adjustment, we used relative weights derived from the 3M (3M, Maplewood, MN) All Patient Refined Diagnosis Related Groups (APR-DRG) classification system, which uses administrative data to classify the severity. The APR-DRG system assigns each admission to a DRG based on principal diagnosis; for each DRG, patients are then subdivided into 4 severity classes based on age, comorbidity, and interactions between these variables and the admitting diagnosis.24 Using the base DRG and severity score, the system assigns relative weights that reflect differences in expected hospital resource utilization.
LOS was derived from hospital administrative data and counted from the date of admission to the hospital. Participants who were discharged on the day of admission were counted as having an LOS of 1. Insurance status (Medicare, Medicaid, no payer, private) also was obtained from administrative data. Age, sex (male or female), education (junior high or less, some high school, high school graduate, some college, college graduate, postgraduate), and race (black/African American, white, Asian or Pacific Islander [including Asian Indian, Chinese, Filipino, Japanese, Korean, Vietnamese, other Asian, Native Hawaiian, Guam/Chamorro, Samoan, other Pacific], American Indian or Alaskan Native, multiple race) were obtained from administrative data based on information provided by the patient. Participants with missing data on any of the sociodemographic variables or on the APR-DRG score were excluded from the analysis.
Statistical Analysis
χ2 and 2-tailed t tests were used to compare categorical and continuous variables, respectively. Multivariate linear regressions were employed to measure associations between the independent variables (HL, illness severity, race, gender, education, and insurance status) and the dependent variable, LOS. Independent variables were chosen for clinical significance and retained in the model regardless of statistical significance. The adjusted R2 values of models with and without the HL variable included were reported to provide information on the contribution of HL to the overall model.
Because LOS was observed to be right skewed and residuals of the untransformed regression were observed to be non-normally distributed, the decision was made to natural log transform LOS, which is consistent with previous hospital LOS studies.16 Regression coefficients and confidence intervals were then transformed into percentage estimates using the following equation: 100(eβ–1). Adjusted R2 was reported for the transformed regression.
The APR-DRG relative weight was treated as a continuous variable. Sociodemographic variables were dichotomized as follows: female vs male; high school graduates vs not; African American vs not; Medicaid/no payer vs Medicare/private payer. Age was not included in the multivariate model because it has been incorporated into the weighted APR-DRG illness severity scores.
Each of the sociodemographic variables and the APR-DRG score were examined for effect modification via the same multivariate linear equation described above, with the addition of an interaction term. A separate regression was performed with an interaction term between age (dichotomized at ≥65) and HL to investigate whether age modified the association between HL and LOS. Finally, we explored whether effects were isolated to long vs short LOS by dividing the sample based on the mean LOS (≥6 days) and performing separate multivariate comparisons.
Sensitivity analyses were performed to exclude those with LOS greater than the 90th percentile and those with APR-DRG score greater than the 90th percentile; age was added to the model as a continuous variable to evaluate whether the illness severity score fully adjusted for the effects of age on LOS. Furthermore, we compared the participants with missing data to those with complete data across both dependent and independent variables. Alpha was set at 0.05; analyses were performed using Stata Version 14 (Stata, College Station, TX).
RESULTS
A total of 5983 participants met inclusion criteria and completed the HL assessment; of these participants, 75 (1%) died during hospitalization, 9 (0.2%) had missing discharge status, and 79 (1%) had LOS >30 days. Two hundred eighty (5%) were missing data on sociodemographic variables or APR-DRG score. Of the remaining (n = 5540), the mean age was 57 years (standard deviation [SD] = 19 years), over half of participants were female (57%), and the majority were African American (73%) and had graduated from high school (81%). The sample was divided into those with private insurance (25%), those with Medicare (46%), and those with Medicaid (26%); 2% had no payer. The mean APR-DRG score was 1.3 (SD = 1.2), and the scores ranged from 0.3 to 15.8.
On the BHLS screen for HL, 20% (1104/5540) had inadequate HL. Participants with low HL had higher weighted illness severity scores (average 1.4 vs 1.3; P = 0.003). Participants with low HL were also more likely to be 65 or older (55% vs 33%; P < 0.001), non-high school graduates (35% vs 15%; P < 0.001), and African American (78% vs 72%; P < 0.001), and to have Medicare or private insurance (75% vs 71%; P = 0.02). There was no significant difference with respect to gender (54% male vs 57% female; P = 0.1)
Finally, we compared the group with missing data (n = 280) to the group with complete data (n = 5540). The participants with missing data were more likely to have low HL (31% [86/280] vs 20%; P < 0.001) and to have Medicare or private insurance (82% [177/217] vs 72%; P = 0.002); however, they were not more likely to be 65 or older (40% [112/280] vs 37%; P = 0.3), high school graduates (88% [113/129] vs 81%; P = 0.06), African American (69% [177/256] vs 73%; P = 0.1), or female (57% [158/279] vs 57%; P = 1), nor were they more likely to have longer LOS (5.7 [n = 280] vs 5.5 days; P = 0.6) or higher illness severity scores (1.3 [n = 231] vs 1.3; P = 0.7).
DISCUSSION
To our knowledge, this study is the first to evaluate the association between low HL and an important in-hospital outcome measure, hospital LOS. We found that low HL was associated with a longer hospital LOS, a result which remained significant when controlling for severity of illness and sociodemographic variables and when testing the model for sensitivity to the highest values of LOS and illness severity. Additionally, the association of HL with LOS appeared concentrated among participants with shorter LOS. Relative to other predictors, the contribution of HL to the overall LOS model was small, as evidenced by the change in adjusted R2 values with HL excluded.
Among the covariates, only gender modified the association between HL and LOS; the findings suggested that men were more susceptible to the effect of low HL on increased LOS. Illness severity and other sociodemographics, including age ≥65, did not appear to modify the association. We also found that being African American and having Medicaid or no insurance were associated with a significantly shorter LOS in multivariate analysis.
Previous work suggested that the adverse health effects of low HL may be mediated through several pathways, including health knowledge, self-efficacy, health skills, and illness stigma.25-27 The finding of a small but significant relationship between HL and LOS was not surprising given these known associations; nevertheless, there may be an additional patient-dependent effect of low HL on LOS not discovered here. For instance, patients with poor health knowledge and self-efficacy might stay in the hospital longer if they or their providers do not feel comfortable with their self-care ability.
This finding may be useful in developing hospital-based interventions. HL-specific interventions, several of which have been tested in the inpatient setting,14,28,29 have shown promise toward improving health knowledge,30 disease severity,31 and health resource utilization.32
Those with low HL may lack the self-efficacy to participate in discharge planning; in fact, previous work has related low HL to posthospital readmissions.8,9 Conversely, patients with low HL might struggle to engage in the inpatient milieu, advocating for shorter LOS if they feel alienated by the inpatient experience.
These possibilities show that LOS is a complex measure shown to depend on patient-level characteristics and on provider-based, geographical, and sociocultural factors.16,33 With these forces at play, additional effects of lower levels of HL may be lost without phenotyping patients by both level of HL and related characteristics, such as self-efficacy, health skills, and stigma. By gathering these additional data, future work should explore whether subpopulations of patients with low HL may be at risk for too-short vs too-long hospital admissions.
For instance, in this study, both race and Medicaid insurance were associated with shorter LOS. Being African American was associated with shorter LOS in our study but has been found to be associated with longer LOS in another study specifically focused on diabetes.34 Prior findings found uninsured patients have shorter LOS.35 Therefore, these findings in our study are difficult to explain without further work to understand whether there are health disparities in the way patients are cared for during hospitalization that may shorten or lengthen their LOS because of factors outside of their clinical need.
The finding that gender modified the effect of low HL on LOS was unexpected. There were similar proportions of men and women with low HL. There is evidence to support that women make the majority of health decisions for themselves and their familes36; therefore, there may be unmeasured aspects of HL that provide an advantage for female vs male inpatients. Furthermore, omitted confounders, such as social support, may not fully capture potential gender-related differences. Future work is needed to understand the role of gender in relationship to HL and LOS.
Limitations of this study include its observational, single-centered design with information derived from administrative data; positive and negative confounding cannot be ruled out. For instance, we did not control for complex aspects affecting LOS, such as discharge disposition and goals of care (eg, aggressive care after discharge vs hospice). To address this limitation, multivariate analyses were performed, which were adjusted for illness severity scores and took into account both comorbidity and severity of the current illness. Additionally, although it is important to study such populations, our largely urban, minority sample is not representative of the U.S. population, and within our large sample, there were participants with missing data who had lower HL on average, although this group represented only 5% of the sample. Finally, different HL tools have noncomplete concordance, which has been seen when comparing the BHLS with more objective tools.20,37 Furthermore, certain in-hospital clinical scenarios (eg, recent stroke or prolonged intensive care unit stay) may present unique challenges in establishing a baseline HL level. However, the BHLS was used in this study because of its greater feasibility.
In conclusion, this study is the first to evaluate the relationship between low HL and LOS. The findings suggest that HL may play a role in shaping outcomes in the inpatient setting and that targeting interventions toward screened patients may be a pathway toward mitigating adverse effects. Our findings need to be replicated in larger, more representative samples, and further work understanding subpopulations within the low HL population is needed. Future work should measure this association in diverse inpatient settings (eg, psychiatric, surgical, and specialty), in addition to assessing associations between HL and other important in-hospital outcome measures, including mortality and discharge disposition.
Acknowledgments
The authors thank the Hospitalist Project team for their assistance with data collection. The authors especially thank Chuanhong Liao and Ashley Snyder for assistance with statistical analyses; Andrea Flores, Ainoa Coltri, and Tom Best for their assistance with data management. The authors would also like to thank Nicole Twu for her help with preparing and editing the manuscript.
Disclosures
Dr. Jaffee was supported by a Calvin Fentress Research Fellowship and NIH R25MH094612. Dr. Press was supported by a career development award (NHLBI K23HL118151). This work was also supported by a seed grant from the Center for Health Administration Studies. All other authors declare no conflicts of interest.
1. U.S. Department of Health and Human Services. Healthy People 2010: Understanding and Improving Health. Washington, DC: U.S. Government Printing Office; 2000.
2. “What Did the Doctor Say”? Improving Health Literacy to Protect Patient Safety. The Joint Commission; 2007.
3. Kutner M, Greenberg E, Jin Y, Paulsen C. The Health Literacy of America’s Adults: Results from the 2003 National Assessment of Adult Literacy. National Center for Education Statistics; 2006.
4. Davis TC, Wolf MS, Bass PF, et al. Literacy and misunderstanding prescription drug labels. Ann Intern Med. 2006;145(12):887-894. PubMed
5. Kripalani S, Henderson LE, Chiu EY, Robertson R, Kolm P, Jacobson TA. Predictors of medication self-management skill in a low-literacy population. J Gen Intern Med. 2006;21(8):852-856. PubMed
6. Berkman ND, Sheridan SL, Donahue KE, Halpern DJ, Crotty K. Low health literacy and health outcomes: an updated systematic review. Ann Intern Med. 2011;155(2):97-107. PubMed
7. Baker DW, Parker RM, Williams MV, Clark WS. Health literacy and the risk of hospital admission. J Gen Intern Med. 1998;13(12):791-798. PubMed
8. Mitchell SE, Sadikova E, Jack BW, Paasche-Orlow MK. Health literacy and 30-day postdischarge hospital utilization. J Health Commun. 2012;17(Suppl 3):325-338. PubMed
9. Jaffee EG, Arora VM, Matthiesen MI, Hariprasad SM, Meltzer DO, Press VG. Postdischarge Falls and Readmissions: Associations with Insufficient Vision and Low Health Literacy among Hospitalized Seniors. J Health Commun. 2016;21(sup2):135-140. PubMed
10. Hope CJ, Wu J, Tu W, Young J, Murray MD. Association of medication adherence, knowledge, and skills with emergency department visits by adults 50 years or older with congestive heart failure. Am J Health Syst Pharm. 2004;61(19):2043-2049. PubMed
11. Bennett IM, Chen J, Soroui JS, White S. The contribution of health literacy to disparities in self-rated health status and preventive health behaviors in older adults. Ann Fam Med. 2009;7(3):204-211. PubMed
12. Baker DW, Wolf MS, Feinglass J, Thompson JA. Health literacy, cognitive abilities, and mortality among elderly persons. J Gen Intern Med. 2008;23(6):723-726. PubMed
13. Cho YI, Lee SY, Arozullah AM, Crittenden KS. Effects of health literacy on health status and health service utilization amongst the elderly. Soc Sci Med. 2008;66(8):1809-1816. PubMed
14. Paasche-Orlow MK, Riekert KA, Bilderback A, et al. Tailored education may reduce health literacy disparities in asthma self-management. Am J Respir Crit Care Med. 2005;172(8):980-986. PubMed
15. Soria-Aledo V, Carrillo-Alcaraz A, Campillo-Soto Á, et al. Associated factors and cost of inappropriate hospital admissions and stays in a second-level hospital. Am J Med Qual. 2009;24(4):321-332. PubMed
16. Lu M, Sajobi T, Lucyk K, Lorenzetti D, Quan H. Systematic review of risk adjustment models of hospital length of stay (LOS). Med Care. 2015;53(4):355-365. PubMed
17. Clarke A, Rosen R. Length of stay. How short should hospital care be? Eur J Public Health. 2001;11(2):166-170. PubMed
18. Meltzer D, Manning WG, Morrison J, et al. Effects of physician experience on costs and outcomes on an academic general medicine service: results of a trial of hospitalists. Ann Intern Med. 2002;137(11):866-874. PubMed
19. Chew LD, Bradley KA, Boyko EJ. Brief questions to identify patients with inadequate health literacy. Fam Med. 2004;36(8):588-594. PubMed
20. Press VG, Shapiro MI, Mayo AM, Meltzer DO, Arora VM. More than meets the eye: relationship between low health literacy and poor vision in hospitalized patients. J Health Commun. 2013;18 Suppl 1:197-204. PubMed
21. Willens DE, Kripalani S, Schildcrout JS, et al. Association of brief health literacy screening and blood pressure in primary care. J Health Commun. 2013;18 Suppl 1:129-142. PubMed
22. Peterson PN, Shetterly SM, Clarke CL, et al. Health literacy and outcomes among patients with heart failure. JAMA. 2011;305(16):1695-1701. PubMed
23. Chew LD, Griffin JM, Partin MR, et al. Validation of screening questions for limited health literacy in a large VA outpatient population. J Gen Intern Med. 2008;23(5):561-566. PubMed
24. Averill RF, Goldfield N, Hughes JS, et al. All Patient Refined Diagnosis Related Groups (APR-DRGs): Methodology Overview. 3M Health Information Systems; 2003.
25. Waite KR, Paasche-Orlow M, Rintamaki LS, Davis TC, Wolf MS. Literacy, social stigma, and HIV medication adherence. J Gen Intern Med. 2008;23(9):1367-1372. PubMed
26. Paasche-Orlow MK, Wolf MS. The causal pathways linking health literacy to health outcomes. Am J Health Behav. 2007;31 Suppl 1:S19-26. PubMed
27. Berkman ND, Sheridan SL, Donahue KE, et al. Health literacy interventions and outcomes: an updated systematic review. Evid Rep Technol Assess (Full Rep). 2011;(199):1-941. PubMed
28. Kripalani S, Roumie CL, Dalal AK, et al. Effect of a pharmacist intervention on clinically important medication errors after hospital discharge: a randomized trial. Ann Intern Med. 2012;157(1):1-10. PubMed
29. Press VG, Arora VM, Shah LM, et al. Teaching the use of respiratory inhalers to hospitalized patients with asthma or COPD: a randomized trial. J Gen Intern Med. 2012;27(10):1317-1325. PubMed
30. Sobel RM, Paasche-Orlow MK, Waite KR, Rittner SS, Wilson EAH, Wolf MS. Asthma 1-2-3: a low literacy multimedia tool to educate African American adults about asthma. J Community Health. 2009;34(4):321-327. PubMed
31. Rothman RL, DeWalt DA, Malone R, et al. Influence of patient literacy on the effectiveness of a primary care-based diabetes disease management program. JAMA. 2004;292(14):1711-1716. PubMed
32. DeWalt DA, Malone RM, Bryant ME, et al. A heart failure self-management
program for patients of all literacy levels: a randomized, controlled trial [ISRCTN11535170].
BMC Health Serv Res. 2006;6:30. PubMed
33. Hasan O, Orav EJ, Hicks LS. Insurance status and hospital care for myocardial
infarction, stroke, and pneumonia. J Hosp Med. 2010;5(8):452-459. PubMed
34. Cook CB, Naylor DB, Hentz JG, et al. Disparities in diabetes-related hospitalizations:
relationship of age, sex, and race/ethnicity with hospital discharges, lengths
of stay, and direct inpatient charges. Ethn Dis. 2006;16(1):126-131. PubMed
35. Hadley J, Steinberg EP, Feder J. Comparison of uninsured and privately insured
hospital patients. Condition on admission, resource use, and outcome. JAMA.
1991;265(3):374-379. PubMed
36. Women’s Health Care Chartbook: Key Findings From the Kaiser Women’s
Health Survey. May 2011. https://kaiserfamilyfoundation.files.wordpress.
com/2013/01/8164.pdf. Accessed August 1, 2017.
37. Louis AJ, Arora VM, Matthiesen MI, Meltzer DO, Press VG. Screening Hospitalized Patients for Low Health Literacy: Beyond the REALM of Possibility? PubMed
Health literacy (HL), defined as patients’ ability to understand health information and make health decisions,1 is a prevalent problem in the outpatient and inpatient settings.2,3 In both settings, low HL has adverse implications for self-care including interpreting health labels4 and taking medications correctly.5 Among outpatient cohorts, HL has been associated with worse outcomes and acute care utilization.6 Associations with low HL include increased hospitalizations,7 rehospitalizations,8,9 emergency department visits,10 and decreased preventative care use.11 Among the elderly, low HL is associated with increased mortality12 and decreased self-perception of health.13
A systematic review revealed that most high-quality HL outcome studies were conducted in the outpatient setting.6 There have been very few studies assessing effects of low HL in an acute-care setting.7,14 These studies have evaluated postdischarge outcomes, including admissions or readmissions,7-9 and medication knowledge.14 To the best of our knowledge, there are no studies evaluating associations between HL and hospital length of stay (LOS).
LOS has received much attention as providers and payers focus more on resource utilization and eliminating adverse effects of prolonged hospitalization.15 LOS is multifactorial, depending on clinical characteristics like disease severity, as well as on sociocultural, demographic, and geographic factors.16 Despite evidence that LOS reductions translate into improved resource allocation and potentially fewer complications, there remains a tension between the appropriate LOS and one that is too short for a given condition.17
Because low HL is associated with inefficient resource utilization, we hypothesized that low HL would be associated with increased LOS after controlling for illness severity. Our objectives were to evaluate the association between low HL and LOS and whether such an association was modified by illness severity and sociodemographics.
METHODS
Study Design, Setting, Participants
An in-hospital, cohort study design of patients who were admitted or transferred to the general medicine service at the University of Chicago between October 2012 and November 2015 and screened for inclusion as part of a large, ongoing study of inpatient care quality was conducted.18 Exclusion criteria included observation status, age under 18 years, non-English speaking, and repeat participants. Those who died during hospitalization or whose discharge status was missing were excluded because the primary goal was to examine the association of HL and time to discharge, which could not be evaluated among those who died. We excluded participants with LOS >30 days to limit overly influential effects of extreme outliers (1% of the population).
Variables
HL was screened using the Brief Health Literacy Screen (BHLS), a validated, 3-question verbal survey not requiring adequate visual acuity to assess HL.19,20 The 3 questions are as follows: (1) “How confident are you filling out medical forms by yourself?”, (2) “How often do you have someone help you read hospital materials?”, and (3) “How often do you have problems learning about your medical condition because of difficulty understanding written information?” Responses to the questions were scored on a 5-point Likert scale in which higher scores corresponded to higher HL.21,22 The scores for each of the 3 questions were summed to yield a range between 3 and 15. On the individual questions, prior work has demonstrated improved test performance with a cutoff of ≤3, which corresponds to a response of “some of the time” or “somewhat”; therefore, when the 3 questions were summed together, scores of ≤9 were considered indicative of low HL.21,23
For severity of illness adjustment, we used relative weights derived from the 3M (3M, Maplewood, MN) All Patient Refined Diagnosis Related Groups (APR-DRG) classification system, which uses administrative data to classify the severity. The APR-DRG system assigns each admission to a DRG based on principal diagnosis; for each DRG, patients are then subdivided into 4 severity classes based on age, comorbidity, and interactions between these variables and the admitting diagnosis.24 Using the base DRG and severity score, the system assigns relative weights that reflect differences in expected hospital resource utilization.
LOS was derived from hospital administrative data and counted from the date of admission to the hospital. Participants who were discharged on the day of admission were counted as having an LOS of 1. Insurance status (Medicare, Medicaid, no payer, private) also was obtained from administrative data. Age, sex (male or female), education (junior high or less, some high school, high school graduate, some college, college graduate, postgraduate), and race (black/African American, white, Asian or Pacific Islander [including Asian Indian, Chinese, Filipino, Japanese, Korean, Vietnamese, other Asian, Native Hawaiian, Guam/Chamorro, Samoan, other Pacific], American Indian or Alaskan Native, multiple race) were obtained from administrative data based on information provided by the patient. Participants with missing data on any of the sociodemographic variables or on the APR-DRG score were excluded from the analysis.
Statistical Analysis
χ2 and 2-tailed t tests were used to compare categorical and continuous variables, respectively. Multivariate linear regressions were employed to measure associations between the independent variables (HL, illness severity, race, gender, education, and insurance status) and the dependent variable, LOS. Independent variables were chosen for clinical significance and retained in the model regardless of statistical significance. The adjusted R2 values of models with and without the HL variable included were reported to provide information on the contribution of HL to the overall model.
Because LOS was observed to be right skewed and residuals of the untransformed regression were observed to be non-normally distributed, the decision was made to natural log transform LOS, which is consistent with previous hospital LOS studies.16 Regression coefficients and confidence intervals were then transformed into percentage estimates using the following equation: 100(eβ–1). Adjusted R2 was reported for the transformed regression.
The APR-DRG relative weight was treated as a continuous variable. Sociodemographic variables were dichotomized as follows: female vs male; high school graduates vs not; African American vs not; Medicaid/no payer vs Medicare/private payer. Age was not included in the multivariate model because it has been incorporated into the weighted APR-DRG illness severity scores.
Each of the sociodemographic variables and the APR-DRG score were examined for effect modification via the same multivariate linear equation described above, with the addition of an interaction term. A separate regression was performed with an interaction term between age (dichotomized at ≥65) and HL to investigate whether age modified the association between HL and LOS. Finally, we explored whether effects were isolated to long vs short LOS by dividing the sample based on the mean LOS (≥6 days) and performing separate multivariate comparisons.
Sensitivity analyses were performed to exclude those with LOS greater than the 90th percentile and those with APR-DRG score greater than the 90th percentile; age was added to the model as a continuous variable to evaluate whether the illness severity score fully adjusted for the effects of age on LOS. Furthermore, we compared the participants with missing data to those with complete data across both dependent and independent variables. Alpha was set at 0.05; analyses were performed using Stata Version 14 (Stata, College Station, TX).
RESULTS
A total of 5983 participants met inclusion criteria and completed the HL assessment; of these participants, 75 (1%) died during hospitalization, 9 (0.2%) had missing discharge status, and 79 (1%) had LOS >30 days. Two hundred eighty (5%) were missing data on sociodemographic variables or APR-DRG score. Of the remaining (n = 5540), the mean age was 57 years (standard deviation [SD] = 19 years), over half of participants were female (57%), and the majority were African American (73%) and had graduated from high school (81%). The sample was divided into those with private insurance (25%), those with Medicare (46%), and those with Medicaid (26%); 2% had no payer. The mean APR-DRG score was 1.3 (SD = 1.2), and the scores ranged from 0.3 to 15.8.
On the BHLS screen for HL, 20% (1104/5540) had inadequate HL. Participants with low HL had higher weighted illness severity scores (average 1.4 vs 1.3; P = 0.003). Participants with low HL were also more likely to be 65 or older (55% vs 33%; P < 0.001), non-high school graduates (35% vs 15%; P < 0.001), and African American (78% vs 72%; P < 0.001), and to have Medicare or private insurance (75% vs 71%; P = 0.02). There was no significant difference with respect to gender (54% male vs 57% female; P = 0.1)
Finally, we compared the group with missing data (n = 280) to the group with complete data (n = 5540). The participants with missing data were more likely to have low HL (31% [86/280] vs 20%; P < 0.001) and to have Medicare or private insurance (82% [177/217] vs 72%; P = 0.002); however, they were not more likely to be 65 or older (40% [112/280] vs 37%; P = 0.3), high school graduates (88% [113/129] vs 81%; P = 0.06), African American (69% [177/256] vs 73%; P = 0.1), or female (57% [158/279] vs 57%; P = 1), nor were they more likely to have longer LOS (5.7 [n = 280] vs 5.5 days; P = 0.6) or higher illness severity scores (1.3 [n = 231] vs 1.3; P = 0.7).
DISCUSSION
To our knowledge, this study is the first to evaluate the association between low HL and an important in-hospital outcome measure, hospital LOS. We found that low HL was associated with a longer hospital LOS, a result which remained significant when controlling for severity of illness and sociodemographic variables and when testing the model for sensitivity to the highest values of LOS and illness severity. Additionally, the association of HL with LOS appeared concentrated among participants with shorter LOS. Relative to other predictors, the contribution of HL to the overall LOS model was small, as evidenced by the change in adjusted R2 values with HL excluded.
Among the covariates, only gender modified the association between HL and LOS; the findings suggested that men were more susceptible to the effect of low HL on increased LOS. Illness severity and other sociodemographics, including age ≥65, did not appear to modify the association. We also found that being African American and having Medicaid or no insurance were associated with a significantly shorter LOS in multivariate analysis.
Previous work suggested that the adverse health effects of low HL may be mediated through several pathways, including health knowledge, self-efficacy, health skills, and illness stigma.25-27 The finding of a small but significant relationship between HL and LOS was not surprising given these known associations; nevertheless, there may be an additional patient-dependent effect of low HL on LOS not discovered here. For instance, patients with poor health knowledge and self-efficacy might stay in the hospital longer if they or their providers do not feel comfortable with their self-care ability.
This finding may be useful in developing hospital-based interventions. HL-specific interventions, several of which have been tested in the inpatient setting,14,28,29 have shown promise toward improving health knowledge,30 disease severity,31 and health resource utilization.32
Those with low HL may lack the self-efficacy to participate in discharge planning; in fact, previous work has related low HL to posthospital readmissions.8,9 Conversely, patients with low HL might struggle to engage in the inpatient milieu, advocating for shorter LOS if they feel alienated by the inpatient experience.
These possibilities show that LOS is a complex measure shown to depend on patient-level characteristics and on provider-based, geographical, and sociocultural factors.16,33 With these forces at play, additional effects of lower levels of HL may be lost without phenotyping patients by both level of HL and related characteristics, such as self-efficacy, health skills, and stigma. By gathering these additional data, future work should explore whether subpopulations of patients with low HL may be at risk for too-short vs too-long hospital admissions.
For instance, in this study, both race and Medicaid insurance were associated with shorter LOS. Being African American was associated with shorter LOS in our study but has been found to be associated with longer LOS in another study specifically focused on diabetes.34 Prior findings found uninsured patients have shorter LOS.35 Therefore, these findings in our study are difficult to explain without further work to understand whether there are health disparities in the way patients are cared for during hospitalization that may shorten or lengthen their LOS because of factors outside of their clinical need.
The finding that gender modified the effect of low HL on LOS was unexpected. There were similar proportions of men and women with low HL. There is evidence to support that women make the majority of health decisions for themselves and their familes36; therefore, there may be unmeasured aspects of HL that provide an advantage for female vs male inpatients. Furthermore, omitted confounders, such as social support, may not fully capture potential gender-related differences. Future work is needed to understand the role of gender in relationship to HL and LOS.
Limitations of this study include its observational, single-centered design with information derived from administrative data; positive and negative confounding cannot be ruled out. For instance, we did not control for complex aspects affecting LOS, such as discharge disposition and goals of care (eg, aggressive care after discharge vs hospice). To address this limitation, multivariate analyses were performed, which were adjusted for illness severity scores and took into account both comorbidity and severity of the current illness. Additionally, although it is important to study such populations, our largely urban, minority sample is not representative of the U.S. population, and within our large sample, there were participants with missing data who had lower HL on average, although this group represented only 5% of the sample. Finally, different HL tools have noncomplete concordance, which has been seen when comparing the BHLS with more objective tools.20,37 Furthermore, certain in-hospital clinical scenarios (eg, recent stroke or prolonged intensive care unit stay) may present unique challenges in establishing a baseline HL level. However, the BHLS was used in this study because of its greater feasibility.
In conclusion, this study is the first to evaluate the relationship between low HL and LOS. The findings suggest that HL may play a role in shaping outcomes in the inpatient setting and that targeting interventions toward screened patients may be a pathway toward mitigating adverse effects. Our findings need to be replicated in larger, more representative samples, and further work understanding subpopulations within the low HL population is needed. Future work should measure this association in diverse inpatient settings (eg, psychiatric, surgical, and specialty), in addition to assessing associations between HL and other important in-hospital outcome measures, including mortality and discharge disposition.
Acknowledgments
The authors thank the Hospitalist Project team for their assistance with data collection. The authors especially thank Chuanhong Liao and Ashley Snyder for assistance with statistical analyses; Andrea Flores, Ainoa Coltri, and Tom Best for their assistance with data management. The authors would also like to thank Nicole Twu for her help with preparing and editing the manuscript.
Disclosures
Dr. Jaffee was supported by a Calvin Fentress Research Fellowship and NIH R25MH094612. Dr. Press was supported by a career development award (NHLBI K23HL118151). This work was also supported by a seed grant from the Center for Health Administration Studies. All other authors declare no conflicts of interest.
Health literacy (HL), defined as patients’ ability to understand health information and make health decisions,1 is a prevalent problem in the outpatient and inpatient settings.2,3 In both settings, low HL has adverse implications for self-care including interpreting health labels4 and taking medications correctly.5 Among outpatient cohorts, HL has been associated with worse outcomes and acute care utilization.6 Associations with low HL include increased hospitalizations,7 rehospitalizations,8,9 emergency department visits,10 and decreased preventative care use.11 Among the elderly, low HL is associated with increased mortality12 and decreased self-perception of health.13
A systematic review revealed that most high-quality HL outcome studies were conducted in the outpatient setting.6 There have been very few studies assessing effects of low HL in an acute-care setting.7,14 These studies have evaluated postdischarge outcomes, including admissions or readmissions,7-9 and medication knowledge.14 To the best of our knowledge, there are no studies evaluating associations between HL and hospital length of stay (LOS).
LOS has received much attention as providers and payers focus more on resource utilization and eliminating adverse effects of prolonged hospitalization.15 LOS is multifactorial, depending on clinical characteristics like disease severity, as well as on sociocultural, demographic, and geographic factors.16 Despite evidence that LOS reductions translate into improved resource allocation and potentially fewer complications, there remains a tension between the appropriate LOS and one that is too short for a given condition.17
Because low HL is associated with inefficient resource utilization, we hypothesized that low HL would be associated with increased LOS after controlling for illness severity. Our objectives were to evaluate the association between low HL and LOS and whether such an association was modified by illness severity and sociodemographics.
METHODS
Study Design, Setting, Participants
An in-hospital, cohort study design of patients who were admitted or transferred to the general medicine service at the University of Chicago between October 2012 and November 2015 and screened for inclusion as part of a large, ongoing study of inpatient care quality was conducted.18 Exclusion criteria included observation status, age under 18 years, non-English speaking, and repeat participants. Those who died during hospitalization or whose discharge status was missing were excluded because the primary goal was to examine the association of HL and time to discharge, which could not be evaluated among those who died. We excluded participants with LOS >30 days to limit overly influential effects of extreme outliers (1% of the population).
Variables
HL was screened using the Brief Health Literacy Screen (BHLS), a validated, 3-question verbal survey not requiring adequate visual acuity to assess HL.19,20 The 3 questions are as follows: (1) “How confident are you filling out medical forms by yourself?”, (2) “How often do you have someone help you read hospital materials?”, and (3) “How often do you have problems learning about your medical condition because of difficulty understanding written information?” Responses to the questions were scored on a 5-point Likert scale in which higher scores corresponded to higher HL.21,22 The scores for each of the 3 questions were summed to yield a range between 3 and 15. On the individual questions, prior work has demonstrated improved test performance with a cutoff of ≤3, which corresponds to a response of “some of the time” or “somewhat”; therefore, when the 3 questions were summed together, scores of ≤9 were considered indicative of low HL.21,23
For severity of illness adjustment, we used relative weights derived from the 3M (3M, Maplewood, MN) All Patient Refined Diagnosis Related Groups (APR-DRG) classification system, which uses administrative data to classify the severity. The APR-DRG system assigns each admission to a DRG based on principal diagnosis; for each DRG, patients are then subdivided into 4 severity classes based on age, comorbidity, and interactions between these variables and the admitting diagnosis.24 Using the base DRG and severity score, the system assigns relative weights that reflect differences in expected hospital resource utilization.
LOS was derived from hospital administrative data and counted from the date of admission to the hospital. Participants who were discharged on the day of admission were counted as having an LOS of 1. Insurance status (Medicare, Medicaid, no payer, private) also was obtained from administrative data. Age, sex (male or female), education (junior high or less, some high school, high school graduate, some college, college graduate, postgraduate), and race (black/African American, white, Asian or Pacific Islander [including Asian Indian, Chinese, Filipino, Japanese, Korean, Vietnamese, other Asian, Native Hawaiian, Guam/Chamorro, Samoan, other Pacific], American Indian or Alaskan Native, multiple race) were obtained from administrative data based on information provided by the patient. Participants with missing data on any of the sociodemographic variables or on the APR-DRG score were excluded from the analysis.
Statistical Analysis
χ2 and 2-tailed t tests were used to compare categorical and continuous variables, respectively. Multivariate linear regressions were employed to measure associations between the independent variables (HL, illness severity, race, gender, education, and insurance status) and the dependent variable, LOS. Independent variables were chosen for clinical significance and retained in the model regardless of statistical significance. The adjusted R2 values of models with and without the HL variable included were reported to provide information on the contribution of HL to the overall model.
Because LOS was observed to be right skewed and residuals of the untransformed regression were observed to be non-normally distributed, the decision was made to natural log transform LOS, which is consistent with previous hospital LOS studies.16 Regression coefficients and confidence intervals were then transformed into percentage estimates using the following equation: 100(eβ–1). Adjusted R2 was reported for the transformed regression.
The APR-DRG relative weight was treated as a continuous variable. Sociodemographic variables were dichotomized as follows: female vs male; high school graduates vs not; African American vs not; Medicaid/no payer vs Medicare/private payer. Age was not included in the multivariate model because it has been incorporated into the weighted APR-DRG illness severity scores.
Each of the sociodemographic variables and the APR-DRG score were examined for effect modification via the same multivariate linear equation described above, with the addition of an interaction term. A separate regression was performed with an interaction term between age (dichotomized at ≥65) and HL to investigate whether age modified the association between HL and LOS. Finally, we explored whether effects were isolated to long vs short LOS by dividing the sample based on the mean LOS (≥6 days) and performing separate multivariate comparisons.
Sensitivity analyses were performed to exclude those with LOS greater than the 90th percentile and those with APR-DRG score greater than the 90th percentile; age was added to the model as a continuous variable to evaluate whether the illness severity score fully adjusted for the effects of age on LOS. Furthermore, we compared the participants with missing data to those with complete data across both dependent and independent variables. Alpha was set at 0.05; analyses were performed using Stata Version 14 (Stata, College Station, TX).
RESULTS
A total of 5983 participants met inclusion criteria and completed the HL assessment; of these participants, 75 (1%) died during hospitalization, 9 (0.2%) had missing discharge status, and 79 (1%) had LOS >30 days. Two hundred eighty (5%) were missing data on sociodemographic variables or APR-DRG score. Of the remaining (n = 5540), the mean age was 57 years (standard deviation [SD] = 19 years), over half of participants were female (57%), and the majority were African American (73%) and had graduated from high school (81%). The sample was divided into those with private insurance (25%), those with Medicare (46%), and those with Medicaid (26%); 2% had no payer. The mean APR-DRG score was 1.3 (SD = 1.2), and the scores ranged from 0.3 to 15.8.
On the BHLS screen for HL, 20% (1104/5540) had inadequate HL. Participants with low HL had higher weighted illness severity scores (average 1.4 vs 1.3; P = 0.003). Participants with low HL were also more likely to be 65 or older (55% vs 33%; P < 0.001), non-high school graduates (35% vs 15%; P < 0.001), and African American (78% vs 72%; P < 0.001), and to have Medicare or private insurance (75% vs 71%; P = 0.02). There was no significant difference with respect to gender (54% male vs 57% female; P = 0.1)
Finally, we compared the group with missing data (n = 280) to the group with complete data (n = 5540). The participants with missing data were more likely to have low HL (31% [86/280] vs 20%; P < 0.001) and to have Medicare or private insurance (82% [177/217] vs 72%; P = 0.002); however, they were not more likely to be 65 or older (40% [112/280] vs 37%; P = 0.3), high school graduates (88% [113/129] vs 81%; P = 0.06), African American (69% [177/256] vs 73%; P = 0.1), or female (57% [158/279] vs 57%; P = 1), nor were they more likely to have longer LOS (5.7 [n = 280] vs 5.5 days; P = 0.6) or higher illness severity scores (1.3 [n = 231] vs 1.3; P = 0.7).
DISCUSSION
To our knowledge, this study is the first to evaluate the association between low HL and an important in-hospital outcome measure, hospital LOS. We found that low HL was associated with a longer hospital LOS, a result which remained significant when controlling for severity of illness and sociodemographic variables and when testing the model for sensitivity to the highest values of LOS and illness severity. Additionally, the association of HL with LOS appeared concentrated among participants with shorter LOS. Relative to other predictors, the contribution of HL to the overall LOS model was small, as evidenced by the change in adjusted R2 values with HL excluded.
Among the covariates, only gender modified the association between HL and LOS; the findings suggested that men were more susceptible to the effect of low HL on increased LOS. Illness severity and other sociodemographics, including age ≥65, did not appear to modify the association. We also found that being African American and having Medicaid or no insurance were associated with a significantly shorter LOS in multivariate analysis.
Previous work suggested that the adverse health effects of low HL may be mediated through several pathways, including health knowledge, self-efficacy, health skills, and illness stigma.25-27 The finding of a small but significant relationship between HL and LOS was not surprising given these known associations; nevertheless, there may be an additional patient-dependent effect of low HL on LOS not discovered here. For instance, patients with poor health knowledge and self-efficacy might stay in the hospital longer if they or their providers do not feel comfortable with their self-care ability.
This finding may be useful in developing hospital-based interventions. HL-specific interventions, several of which have been tested in the inpatient setting,14,28,29 have shown promise toward improving health knowledge,30 disease severity,31 and health resource utilization.32
Those with low HL may lack the self-efficacy to participate in discharge planning; in fact, previous work has related low HL to posthospital readmissions.8,9 Conversely, patients with low HL might struggle to engage in the inpatient milieu, advocating for shorter LOS if they feel alienated by the inpatient experience.
These possibilities show that LOS is a complex measure shown to depend on patient-level characteristics and on provider-based, geographical, and sociocultural factors.16,33 With these forces at play, additional effects of lower levels of HL may be lost without phenotyping patients by both level of HL and related characteristics, such as self-efficacy, health skills, and stigma. By gathering these additional data, future work should explore whether subpopulations of patients with low HL may be at risk for too-short vs too-long hospital admissions.
For instance, in this study, both race and Medicaid insurance were associated with shorter LOS. Being African American was associated with shorter LOS in our study but has been found to be associated with longer LOS in another study specifically focused on diabetes.34 Prior findings found uninsured patients have shorter LOS.35 Therefore, these findings in our study are difficult to explain without further work to understand whether there are health disparities in the way patients are cared for during hospitalization that may shorten or lengthen their LOS because of factors outside of their clinical need.
The finding that gender modified the effect of low HL on LOS was unexpected. There were similar proportions of men and women with low HL. There is evidence to support that women make the majority of health decisions for themselves and their familes36; therefore, there may be unmeasured aspects of HL that provide an advantage for female vs male inpatients. Furthermore, omitted confounders, such as social support, may not fully capture potential gender-related differences. Future work is needed to understand the role of gender in relationship to HL and LOS.
Limitations of this study include its observational, single-centered design with information derived from administrative data; positive and negative confounding cannot be ruled out. For instance, we did not control for complex aspects affecting LOS, such as discharge disposition and goals of care (eg, aggressive care after discharge vs hospice). To address this limitation, multivariate analyses were performed, which were adjusted for illness severity scores and took into account both comorbidity and severity of the current illness. Additionally, although it is important to study such populations, our largely urban, minority sample is not representative of the U.S. population, and within our large sample, there were participants with missing data who had lower HL on average, although this group represented only 5% of the sample. Finally, different HL tools have noncomplete concordance, which has been seen when comparing the BHLS with more objective tools.20,37 Furthermore, certain in-hospital clinical scenarios (eg, recent stroke or prolonged intensive care unit stay) may present unique challenges in establishing a baseline HL level. However, the BHLS was used in this study because of its greater feasibility.
In conclusion, this study is the first to evaluate the relationship between low HL and LOS. The findings suggest that HL may play a role in shaping outcomes in the inpatient setting and that targeting interventions toward screened patients may be a pathway toward mitigating adverse effects. Our findings need to be replicated in larger, more representative samples, and further work understanding subpopulations within the low HL population is needed. Future work should measure this association in diverse inpatient settings (eg, psychiatric, surgical, and specialty), in addition to assessing associations between HL and other important in-hospital outcome measures, including mortality and discharge disposition.
Acknowledgments
The authors thank the Hospitalist Project team for their assistance with data collection. The authors especially thank Chuanhong Liao and Ashley Snyder for assistance with statistical analyses; Andrea Flores, Ainoa Coltri, and Tom Best for their assistance with data management. The authors would also like to thank Nicole Twu for her help with preparing and editing the manuscript.
Disclosures
Dr. Jaffee was supported by a Calvin Fentress Research Fellowship and NIH R25MH094612. Dr. Press was supported by a career development award (NHLBI K23HL118151). This work was also supported by a seed grant from the Center for Health Administration Studies. All other authors declare no conflicts of interest.
1. U.S. Department of Health and Human Services. Healthy People 2010: Understanding and Improving Health. Washington, DC: U.S. Government Printing Office; 2000.
2. “What Did the Doctor Say”? Improving Health Literacy to Protect Patient Safety. The Joint Commission; 2007.
3. Kutner M, Greenberg E, Jin Y, Paulsen C. The Health Literacy of America’s Adults: Results from the 2003 National Assessment of Adult Literacy. National Center for Education Statistics; 2006.
4. Davis TC, Wolf MS, Bass PF, et al. Literacy and misunderstanding prescription drug labels. Ann Intern Med. 2006;145(12):887-894. PubMed
5. Kripalani S, Henderson LE, Chiu EY, Robertson R, Kolm P, Jacobson TA. Predictors of medication self-management skill in a low-literacy population. J Gen Intern Med. 2006;21(8):852-856. PubMed
6. Berkman ND, Sheridan SL, Donahue KE, Halpern DJ, Crotty K. Low health literacy and health outcomes: an updated systematic review. Ann Intern Med. 2011;155(2):97-107. PubMed
7. Baker DW, Parker RM, Williams MV, Clark WS. Health literacy and the risk of hospital admission. J Gen Intern Med. 1998;13(12):791-798. PubMed
8. Mitchell SE, Sadikova E, Jack BW, Paasche-Orlow MK. Health literacy and 30-day postdischarge hospital utilization. J Health Commun. 2012;17(Suppl 3):325-338. PubMed
9. Jaffee EG, Arora VM, Matthiesen MI, Hariprasad SM, Meltzer DO, Press VG. Postdischarge Falls and Readmissions: Associations with Insufficient Vision and Low Health Literacy among Hospitalized Seniors. J Health Commun. 2016;21(sup2):135-140. PubMed
10. Hope CJ, Wu J, Tu W, Young J, Murray MD. Association of medication adherence, knowledge, and skills with emergency department visits by adults 50 years or older with congestive heart failure. Am J Health Syst Pharm. 2004;61(19):2043-2049. PubMed
11. Bennett IM, Chen J, Soroui JS, White S. The contribution of health literacy to disparities in self-rated health status and preventive health behaviors in older adults. Ann Fam Med. 2009;7(3):204-211. PubMed
12. Baker DW, Wolf MS, Feinglass J, Thompson JA. Health literacy, cognitive abilities, and mortality among elderly persons. J Gen Intern Med. 2008;23(6):723-726. PubMed
13. Cho YI, Lee SY, Arozullah AM, Crittenden KS. Effects of health literacy on health status and health service utilization amongst the elderly. Soc Sci Med. 2008;66(8):1809-1816. PubMed
14. Paasche-Orlow MK, Riekert KA, Bilderback A, et al. Tailored education may reduce health literacy disparities in asthma self-management. Am J Respir Crit Care Med. 2005;172(8):980-986. PubMed
15. Soria-Aledo V, Carrillo-Alcaraz A, Campillo-Soto Á, et al. Associated factors and cost of inappropriate hospital admissions and stays in a second-level hospital. Am J Med Qual. 2009;24(4):321-332. PubMed
16. Lu M, Sajobi T, Lucyk K, Lorenzetti D, Quan H. Systematic review of risk adjustment models of hospital length of stay (LOS). Med Care. 2015;53(4):355-365. PubMed
17. Clarke A, Rosen R. Length of stay. How short should hospital care be? Eur J Public Health. 2001;11(2):166-170. PubMed
18. Meltzer D, Manning WG, Morrison J, et al. Effects of physician experience on costs and outcomes on an academic general medicine service: results of a trial of hospitalists. Ann Intern Med. 2002;137(11):866-874. PubMed
19. Chew LD, Bradley KA, Boyko EJ. Brief questions to identify patients with inadequate health literacy. Fam Med. 2004;36(8):588-594. PubMed
20. Press VG, Shapiro MI, Mayo AM, Meltzer DO, Arora VM. More than meets the eye: relationship between low health literacy and poor vision in hospitalized patients. J Health Commun. 2013;18 Suppl 1:197-204. PubMed
21. Willens DE, Kripalani S, Schildcrout JS, et al. Association of brief health literacy screening and blood pressure in primary care. J Health Commun. 2013;18 Suppl 1:129-142. PubMed
22. Peterson PN, Shetterly SM, Clarke CL, et al. Health literacy and outcomes among patients with heart failure. JAMA. 2011;305(16):1695-1701. PubMed
23. Chew LD, Griffin JM, Partin MR, et al. Validation of screening questions for limited health literacy in a large VA outpatient population. J Gen Intern Med. 2008;23(5):561-566. PubMed
24. Averill RF, Goldfield N, Hughes JS, et al. All Patient Refined Diagnosis Related Groups (APR-DRGs): Methodology Overview. 3M Health Information Systems; 2003.
25. Waite KR, Paasche-Orlow M, Rintamaki LS, Davis TC, Wolf MS. Literacy, social stigma, and HIV medication adherence. J Gen Intern Med. 2008;23(9):1367-1372. PubMed
26. Paasche-Orlow MK, Wolf MS. The causal pathways linking health literacy to health outcomes. Am J Health Behav. 2007;31 Suppl 1:S19-26. PubMed
27. Berkman ND, Sheridan SL, Donahue KE, et al. Health literacy interventions and outcomes: an updated systematic review. Evid Rep Technol Assess (Full Rep). 2011;(199):1-941. PubMed
28. Kripalani S, Roumie CL, Dalal AK, et al. Effect of a pharmacist intervention on clinically important medication errors after hospital discharge: a randomized trial. Ann Intern Med. 2012;157(1):1-10. PubMed
29. Press VG, Arora VM, Shah LM, et al. Teaching the use of respiratory inhalers to hospitalized patients with asthma or COPD: a randomized trial. J Gen Intern Med. 2012;27(10):1317-1325. PubMed
30. Sobel RM, Paasche-Orlow MK, Waite KR, Rittner SS, Wilson EAH, Wolf MS. Asthma 1-2-3: a low literacy multimedia tool to educate African American adults about asthma. J Community Health. 2009;34(4):321-327. PubMed
31. Rothman RL, DeWalt DA, Malone R, et al. Influence of patient literacy on the effectiveness of a primary care-based diabetes disease management program. JAMA. 2004;292(14):1711-1716. PubMed
32. DeWalt DA, Malone RM, Bryant ME, et al. A heart failure self-management
program for patients of all literacy levels: a randomized, controlled trial [ISRCTN11535170].
BMC Health Serv Res. 2006;6:30. PubMed
33. Hasan O, Orav EJ, Hicks LS. Insurance status and hospital care for myocardial
infarction, stroke, and pneumonia. J Hosp Med. 2010;5(8):452-459. PubMed
34. Cook CB, Naylor DB, Hentz JG, et al. Disparities in diabetes-related hospitalizations:
relationship of age, sex, and race/ethnicity with hospital discharges, lengths
of stay, and direct inpatient charges. Ethn Dis. 2006;16(1):126-131. PubMed
35. Hadley J, Steinberg EP, Feder J. Comparison of uninsured and privately insured
hospital patients. Condition on admission, resource use, and outcome. JAMA.
1991;265(3):374-379. PubMed
36. Women’s Health Care Chartbook: Key Findings From the Kaiser Women’s
Health Survey. May 2011. https://kaiserfamilyfoundation.files.wordpress.
com/2013/01/8164.pdf. Accessed August 1, 2017.
37. Louis AJ, Arora VM, Matthiesen MI, Meltzer DO, Press VG. Screening Hospitalized Patients for Low Health Literacy: Beyond the REALM of Possibility? PubMed
1. U.S. Department of Health and Human Services. Healthy People 2010: Understanding and Improving Health. Washington, DC: U.S. Government Printing Office; 2000.
2. “What Did the Doctor Say”? Improving Health Literacy to Protect Patient Safety. The Joint Commission; 2007.
3. Kutner M, Greenberg E, Jin Y, Paulsen C. The Health Literacy of America’s Adults: Results from the 2003 National Assessment of Adult Literacy. National Center for Education Statistics; 2006.
4. Davis TC, Wolf MS, Bass PF, et al. Literacy and misunderstanding prescription drug labels. Ann Intern Med. 2006;145(12):887-894. PubMed
5. Kripalani S, Henderson LE, Chiu EY, Robertson R, Kolm P, Jacobson TA. Predictors of medication self-management skill in a low-literacy population. J Gen Intern Med. 2006;21(8):852-856. PubMed
6. Berkman ND, Sheridan SL, Donahue KE, Halpern DJ, Crotty K. Low health literacy and health outcomes: an updated systematic review. Ann Intern Med. 2011;155(2):97-107. PubMed
7. Baker DW, Parker RM, Williams MV, Clark WS. Health literacy and the risk of hospital admission. J Gen Intern Med. 1998;13(12):791-798. PubMed
8. Mitchell SE, Sadikova E, Jack BW, Paasche-Orlow MK. Health literacy and 30-day postdischarge hospital utilization. J Health Commun. 2012;17(Suppl 3):325-338. PubMed
9. Jaffee EG, Arora VM, Matthiesen MI, Hariprasad SM, Meltzer DO, Press VG. Postdischarge Falls and Readmissions: Associations with Insufficient Vision and Low Health Literacy among Hospitalized Seniors. J Health Commun. 2016;21(sup2):135-140. PubMed
10. Hope CJ, Wu J, Tu W, Young J, Murray MD. Association of medication adherence, knowledge, and skills with emergency department visits by adults 50 years or older with congestive heart failure. Am J Health Syst Pharm. 2004;61(19):2043-2049. PubMed
11. Bennett IM, Chen J, Soroui JS, White S. The contribution of health literacy to disparities in self-rated health status and preventive health behaviors in older adults. Ann Fam Med. 2009;7(3):204-211. PubMed
12. Baker DW, Wolf MS, Feinglass J, Thompson JA. Health literacy, cognitive abilities, and mortality among elderly persons. J Gen Intern Med. 2008;23(6):723-726. PubMed
13. Cho YI, Lee SY, Arozullah AM, Crittenden KS. Effects of health literacy on health status and health service utilization amongst the elderly. Soc Sci Med. 2008;66(8):1809-1816. PubMed
14. Paasche-Orlow MK, Riekert KA, Bilderback A, et al. Tailored education may reduce health literacy disparities in asthma self-management. Am J Respir Crit Care Med. 2005;172(8):980-986. PubMed
15. Soria-Aledo V, Carrillo-Alcaraz A, Campillo-Soto Á, et al. Associated factors and cost of inappropriate hospital admissions and stays in a second-level hospital. Am J Med Qual. 2009;24(4):321-332. PubMed
16. Lu M, Sajobi T, Lucyk K, Lorenzetti D, Quan H. Systematic review of risk adjustment models of hospital length of stay (LOS). Med Care. 2015;53(4):355-365. PubMed
17. Clarke A, Rosen R. Length of stay. How short should hospital care be? Eur J Public Health. 2001;11(2):166-170. PubMed
18. Meltzer D, Manning WG, Morrison J, et al. Effects of physician experience on costs and outcomes on an academic general medicine service: results of a trial of hospitalists. Ann Intern Med. 2002;137(11):866-874. PubMed
19. Chew LD, Bradley KA, Boyko EJ. Brief questions to identify patients with inadequate health literacy. Fam Med. 2004;36(8):588-594. PubMed
20. Press VG, Shapiro MI, Mayo AM, Meltzer DO, Arora VM. More than meets the eye: relationship between low health literacy and poor vision in hospitalized patients. J Health Commun. 2013;18 Suppl 1:197-204. PubMed
21. Willens DE, Kripalani S, Schildcrout JS, et al. Association of brief health literacy screening and blood pressure in primary care. J Health Commun. 2013;18 Suppl 1:129-142. PubMed
22. Peterson PN, Shetterly SM, Clarke CL, et al. Health literacy and outcomes among patients with heart failure. JAMA. 2011;305(16):1695-1701. PubMed
23. Chew LD, Griffin JM, Partin MR, et al. Validation of screening questions for limited health literacy in a large VA outpatient population. J Gen Intern Med. 2008;23(5):561-566. PubMed
24. Averill RF, Goldfield N, Hughes JS, et al. All Patient Refined Diagnosis Related Groups (APR-DRGs): Methodology Overview. 3M Health Information Systems; 2003.
25. Waite KR, Paasche-Orlow M, Rintamaki LS, Davis TC, Wolf MS. Literacy, social stigma, and HIV medication adherence. J Gen Intern Med. 2008;23(9):1367-1372. PubMed
26. Paasche-Orlow MK, Wolf MS. The causal pathways linking health literacy to health outcomes. Am J Health Behav. 2007;31 Suppl 1:S19-26. PubMed
27. Berkman ND, Sheridan SL, Donahue KE, et al. Health literacy interventions and outcomes: an updated systematic review. Evid Rep Technol Assess (Full Rep). 2011;(199):1-941. PubMed
28. Kripalani S, Roumie CL, Dalal AK, et al. Effect of a pharmacist intervention on clinically important medication errors after hospital discharge: a randomized trial. Ann Intern Med. 2012;157(1):1-10. PubMed
29. Press VG, Arora VM, Shah LM, et al. Teaching the use of respiratory inhalers to hospitalized patients with asthma or COPD: a randomized trial. J Gen Intern Med. 2012;27(10):1317-1325. PubMed
30. Sobel RM, Paasche-Orlow MK, Waite KR, Rittner SS, Wilson EAH, Wolf MS. Asthma 1-2-3: a low literacy multimedia tool to educate African American adults about asthma. J Community Health. 2009;34(4):321-327. PubMed
31. Rothman RL, DeWalt DA, Malone R, et al. Influence of patient literacy on the effectiveness of a primary care-based diabetes disease management program. JAMA. 2004;292(14):1711-1716. PubMed
32. DeWalt DA, Malone RM, Bryant ME, et al. A heart failure self-management
program for patients of all literacy levels: a randomized, controlled trial [ISRCTN11535170].
BMC Health Serv Res. 2006;6:30. PubMed
33. Hasan O, Orav EJ, Hicks LS. Insurance status and hospital care for myocardial
infarction, stroke, and pneumonia. J Hosp Med. 2010;5(8):452-459. PubMed
34. Cook CB, Naylor DB, Hentz JG, et al. Disparities in diabetes-related hospitalizations:
relationship of age, sex, and race/ethnicity with hospital discharges, lengths
of stay, and direct inpatient charges. Ethn Dis. 2006;16(1):126-131. PubMed
35. Hadley J, Steinberg EP, Feder J. Comparison of uninsured and privately insured
hospital patients. Condition on admission, resource use, and outcome. JAMA.
1991;265(3):374-379. PubMed
36. Women’s Health Care Chartbook: Key Findings From the Kaiser Women’s
Health Survey. May 2011. https://kaiserfamilyfoundation.files.wordpress.
com/2013/01/8164.pdf. Accessed August 1, 2017.
37. Louis AJ, Arora VM, Matthiesen MI, Meltzer DO, Press VG. Screening Hospitalized Patients for Low Health Literacy: Beyond the REALM of Possibility? PubMed
© 2017 Society of Hospital Medicine
Analysis of Hospitalist Discontinuity
Studies examining the importance of continuity of care have shown that patients who maintain a continuous relationship with a single physician have improved outcomes.[1, 2] However, most of these studies were performed in the outpatient, rather than the inpatient setting. With over 35 million patients admitted to hospitals in 2013, along with the significant increase in hospital discontinuity over recent years, the impact of inpatient continuity of care on quality outcomes and patient satisfaction is becoming increasingly relevant.[3, 4]
Service handoffs, when a physician hands over treatment responsibility for a panel of patients and is not expected to return, are a type of handoff that contributes to inpatient discontinuity. In particular, service handoffs between hospitalists are an especially common and inherently risky type of transition, as there is a severing of an established relationship during a patient's hospitalization. Unfortunately, due to the lack of evidence on the effects of service handoffs, current guidelines are limited in their recommendations.[5] Whereas several recent studies have begun to explore the effects of these handoffs, no prior study has examined this issue from a patient's perspective.[6, 7, 8]
Patients are uniquely positioned to inform us about their experiences in care transitions. Furthermore, with patient satisfaction now affecting Medicare reimbursement rates, patient experiences while in the hospital are becoming even more significant.[9] Despite this emphasis toward more patient‐centered care, no study has explored the hospitalized patient's experience with hospitalist service handoffs. Our goal was to qualitatively assess the hospitalized patients' experiences with transitions between hospitalists to develop a conceptual model to inform future work on improving inpatient transitions of care.
METHODS
Sampling and Recruitment
We conducted bedside interviews of hospitalized patients at an urban academic medical center from October 2014 through December 2014. The hospitalist service consists of a physician and an advanced nurse practitioner (ANP) who divide a panel of patients that consist of general medicine and subspecialty patients who are often comanaged with hepatology, oncology, and nephrology subspecialists. We performed a purposive selection of patients who could potentially comment on their experience with a hospitalist service transition using the following method: 48 hours after a service handoff (ie, an outgoing physician completing 1 week on service, then transfers the care of the patient to a new oncoming hospitalist), oncoming hospitalists were approached and asked if any patient on their service had experienced a service handoff and still remained in the hospital. A 48‐hour time period was chosen to give the patients time to familiarize themselves with their new hospitalist, allowing them to properly comment on the handoff. Patients who were managed by the ANP, who were non‐English speaking, or who were deemed to have an altered mental status based on clinical suspicion by the interviewing physician (C.M.W.) were excluded from participation. Following each weekly service transition, a list of patients who met the above criteria was collected from 4 nonteaching hospitalist services, and were approached by the primary investigator (C.M.W.) and asked if they would be willing to participate. All patients were general medicine patients and no exclusions were made based on physical location within the hospital. Those who agreed provided signed written consent prior to participation to allow access to the electronic health records (EHRs) by study personnel.
Data Collection
Patients were administered a 9‐question, semistructured interview that was informed by expert opinion and existing literature, which was developed to elicit their perspective regarding their transition between hospitalists.[10, 11] No formal changes were made to the interview guide during the study period, and all patients were asked the same questions. Outcomes from interim analysis guided further questioning in subsequent interviews so as to increase the depth of patient responses (ie, Can you explain your response in greater depth?). Prior to the interview, patients were read a description of a hospitalist, and were reminded which hospitalists had cared for them during their stay (see Supporting Information, Appendix 1, in the online version of this article). If family members or a caregiver were present at the time of interview, they were asked not to comment. No repeat interviews were carried out.
All interviews were performed privately in single‐occupancy rooms, digitally recorded using an iPad (Apple, Cupertino, CA) and professionally transcribed verbatim (Rev, San Francisco, CA). All analysis was performed using MAXQDA Software (VERBI Software GmbH, Berlin, Germany). We obtained demographic information about each patient through chart review
Data Analysis
Grounded theory was utilized, with an inductive approach with no a priori hypothesis.[12] The constant comparative method was used to generate emerging and reoccurring themes.[13] Units of analysis were sentences and phrases. Our research team consisted of 4 academic hospitalists, 2 with backgrounds in clinical medicine, medical education, and qualitative analysis (J.M.F., V.M.A.), 1 as a clinician (C.M.W.), and 1 in health economics (D.O.M.). Interim analysis was performed on a weekly basis (C.M.W.), during which time a coding template was created and refined through an iterative process (C.M.W., J.M.F.). All disagreements in coded themes were resolved through group discussion until full consensus was reached. Each week, responses were assessed for thematic saturation.[14] Interviews were continued if new themes arose during this analysis. Data collection was ended once we ceased to extract new topics from participants. A summary of all themes was then presented to a group of 10 patients who met the same inclusion criteria for respondent validation and member checking. All reporting was performed within the Standards for Reporting Qualitative Research, with additional guidance derived from the Consolidated Criteria for Reporting Qualitative Research.[15, 16] The University of Chicago Institutional Review Board approved this protocol.
RESULTS
In total, 43 eligible patients were recruited, and 40 (93%) agreed to participate. Interviewed patients were between 51 and 65 (39%) years old, had a mean age of 54.5 (15) years, were predominantly female (65%), African American (58%), had a median length of stay at the time of interview of 6.5 days (interquartile range [IQR]: 48), and had an average of 2.0 (IQR: 13) hospitalists oversee their care at the time of interview (Table 1). Interview times ranged from 10:25 to 25:48 minutes, with an average of 15:32 minutes.
Value | |
---|---|
| |
Response rate, n (%) | 40/43 (93) |
Age, mean SD | 54.5 15 |
Sex, n (%) | |
Female | 26 (65) |
Male | 14 (35) |
Race, n (%) | |
African American | 23 (58) |
White | 16 (40) |
Hispanic | 1 (2) |
Median LOS at time of interview, d (IQR) | 6.5 (48) |
Median no. of hospitalists at time of interview, n (IQR) | 2.0 (13) |
We identified 6 major themes on patient perceptions of hospitalist service handoffs including (1) physician‐patient communication, (2) transparency in the hospitalist transition process, (3) indifference toward the hospitalist transition, (4) hospitalist‐subspecialist communication, (5) recognition of new opportunities due to a transition, and (6) hospitalists' bedside manner (Table 2).
Themes | Subthemes | Representative Quotes |
---|---|---|
Physician‐patient communication | Patients dislike redundant communication with oncoming hospitalist. | I mean it's just you always have to explain your situation over and over and over again. (patient 14) |
When I said it once already, then you're repeating it to another doctor. I feel as if that hospitalist didn't talk to the other hospitalist. (patient 7) | ||
Poor communication can negatively affect the doctor‐patient relationship. | They don't really want to explain things. They don't think I'll understand. I think & yeah, I'm okay. You don't even have to put it in layman's terms. I know medical. I'm in nursing school. I have a year left. But even if you didn't know that, I would still hope you would try to tell me what was going on instead of just doing it in your head, and treating it. (patient 2) | |
I mean it's just you always have to explain your situation over and over and over again. After a while you just stop trusting them. (patient 20) | ||
Good communication can positively affect the doctor‐patient relationship. | Just continue with the communication, the open communication, and always stress to me that I have a voice and just going out of their way to do whatever they can to help me through whatever I'm going through. (patient 1) | |
Transparency in transition | Patients want to be informed prior to a service changeover. | I think they should be told immediately, even maybe given prior notice, like this may happen, just so you're not surprised when it happens. (patient 15) |
When the doctor approached me, he let me know that he wasn't going to be here the next day and there was going to be another doctor coming in. That made me feel comfortable. (patient 9) | ||
Patients desire a more formalized process in the service changeover. | People want things to be consistent. People don't like change. They like routine. So, if he's leaving, you're coming on, I'd like for him to bring you in, introduce you to me, and for you just assure me that I'll take care of you. (patient 4) | |
Just like when you get a new medication, you're given all this information on it. So when you get a new hospitalist, shouldn't I get all the information on them? Like where they went to school, what they look like. (patient 23) | ||
Patients want clearer definition of the roles the physicians will play in their care. | The first time I was hospitalized for the first time I had all these different doctors coming in, and I had the residency, and the specialists, and the department, and I don't know who's who. What I asked them to do is when they come in the room, which they did, but introduce it a little more for me. Write it down like these are the special team and these are the doctors because even though they come in and give me their name, I have no idea what they're doing. (patient 5) | |
Someone should explain the setup and who people are. Someone would say, Okay when you're in a hospital this is your [doctor's] role. Like they should have booklets and everything. (patient 19) | ||
Indifference toward transition | Many patients have trust in service changeovers. | [S]o as long as everybody's on board and communicates well and efficiently, I don't have a problem with it. (patient 6) |
To me, it really wasn't no preference, as long as I was getting the care that I needed. (patient 21) | ||
It's not a concern as long as they're on the same page. (patient 17) | ||
Hospitalist‐specialist communication | Patients are concerned about communication between their hospitalist and their subspecialists. | The more cooks you get in the kitchen, the more things get to get lost, so I'm always concerned that they're not sharing the same information, especially when you're getting asked the same questions that you might have just answered the last hour ago. (patient 9) |
I don't know if the hospitalist are talking to them [subspecialist]. They haven't got time. (patient 35) | ||
Patients place trust in the communication between hospitalist and subspecialist. | I think among the teams themselveswhich is my pain doctor, Dr. K's group, the oncology group itself, they switch off and trade with each other and they all speak the same language so that works out good. (patient 3) | |
Lack of interprofessional communication can lead to patient concern. | I was afraid that one was going to drop the ball on something and not pass something on, or you know. (patient 11) | |
I had numerous doctors who all seemed to not communicate with each other at all or did so by email or whatever. They didn't just sit down together and say we feel this way and we feel that way. I didn't like that at all. (patient 10) | ||
New opportunities due to transition | Patients see new doctor as opportunity for medical reevaluation. | I see it as two heads are better than one, three heads are better than one, four heads are better than one. When people put their heads together to work towards a common goal, especially when they're, you know, people working their craft, it can't be bad. (patient 9) |
I finally got my ears looked atbecause I've asked to have my ears looked at since Mondayand the new doc is trying to make an effort to look at them. (patient 39) | ||
Patients see service changeover as an opportunity to form a better personal relationship. | Having a new hospitalist it gives you opportunity for a new beginning. (patient 11) | |
Bedside manner | Good bedside manner can assist in a service changeover. | Some of them are all business‐like but some of them are, Well how do you feel today? Hi, how are you? So this made a little difference. You feel more comfortable. You're going to be more comfortable with them. Their bedside manner helps. (patient 16) |
It's just like when a doctor sits down and talks to you, they just seem more relaxed and more .... I know they're very busy and they have lots of things to do and other patients to see, but while they're in there with you, you know, you don't get too much time with them. So bedside manner is just so important. (patient 24) | ||
Poor bedside manner can be detrimental in transition. | [B]ecause they be so busy they claim they don't have time just to sit and talk to a patient, and make sure they all right. (patient 17) |
Physician‐Patient Communication
Communication between the physician and the patient was an important element in patients' assessment of their experience. Patient's tended to divide physician‐patient communication into 2 categories: good communication, which consisted of open communication (patient 1) and patient engagement, and bad communication, which was described as physicians not sharing information or taking the time to explain the course of care in words that I'll understand (patient 2). Patients also described dissatisfaction with redundant communication between multiple hospitalists and the frustration of often having to describe their clinical course to multiple providers.
Transparency in Communication
The desire to have greater transparency in the handoff process was another common theme. This was likely due to the fact that 34/40 (85%) of surveyed patients were unaware that a service changeover had ever taken place. This lack of transparency was viewed to have further downstream consequences as patients stated that there should be a level of transparency, and when it's not, then there is always trust issues (patient 1). Upon further questioning as to how to make the process more transparent, many patients recommended a formalized, face‐to‐face introduction involving the patient and both hospitalists, in which the outgoing hospitalist would, bring you [oncoming hospitalist] in, and introduce you to me (patient 4).
Patients often stated that given the large spectrum of physicians they might encounter during their stay (ie, medical student, resident, hospitalist attending, subspecialty fellow, subspecialist attending), clearer definitions of physicians' roles are needed.
Hospitalist‐Specialist Communication
Concern about the communication between their hospitalist and subspecialist was another predominant theme. Conflicting and unclear directions from multiple services were especially frustrating, as a patient stated, One guy took me off this pill, the other guy wants me on that pill, I'm like okay, I can't do both (patient 8). Furthermore, a subset of patients referenced their subspecialist as their primary care provider and preferred their subspecialist for guidance in their hospital course, rather than their hospitalist. This often appeared in cases where the patient had an established relationship with the subspecialist prior to their hospitalization.
New Opportunities Due to Transition
Patients expressed positive feelings toward service handoffs by viewing the transition as an opportunity for medical reevaluation by a new physician. Patients told of instances in which a specific complaint was not being addressed by the first physician, but would be addressed by the second (oncoming) physician. A commonly expressed idea was that the oncoming physician might know something that he [Dr. B] didn't know, and since Dr. B was only here for a week, why not give him [oncoming hospitalist] a chance (patient 10). Patients would also describe the transition as an opportunity to form, and possibly improve, therapeutic alliances with a new hospitalist.
Bedside Manner
Bedside manner was another commonly mentioned thematic element. Patients were often quick to forget prior problems or issues that they may have suffered because of the transition if the oncoming physician was perceived to have a good bedside manner, often described as someone who formally introduced themselves, was considered relaxed, and would take the time to sit and talk with the patient. As a patient put it, [S]he sat down and got to know meand asked me what I wanted to do (patient 12). Conversely, patients described instances in which a perceived bad bedside manner led to a poor relationship between the physician and the patient, in which trust and comfort (patient 11) were sacrificed.
Indifference Toward Transition
In contrast to some of the previous findings, which called for improved interactions between physicians and patients, we also discovered a theme of indifference toward the transition. Several patients stated feelings of trust with the medical system, and were content with the service changeover as long as they felt that their medical needs were being met. Patients also tended to express a level of acceptance with the transition, and tended to believe that this was the price we pay for being here [in the hospital] (patient 7).
Conceptual Model
Following the collection and analysis of all patient responses, all themes were utilized to construct the ideal patient‐centered service handoff. The ideal transition describes open lines of communication between all involved parties, is facilitated by multiple modalities, such as the EHRs and nursing staff, and recognizes the patient as the primary stakeholder (Figure 1).
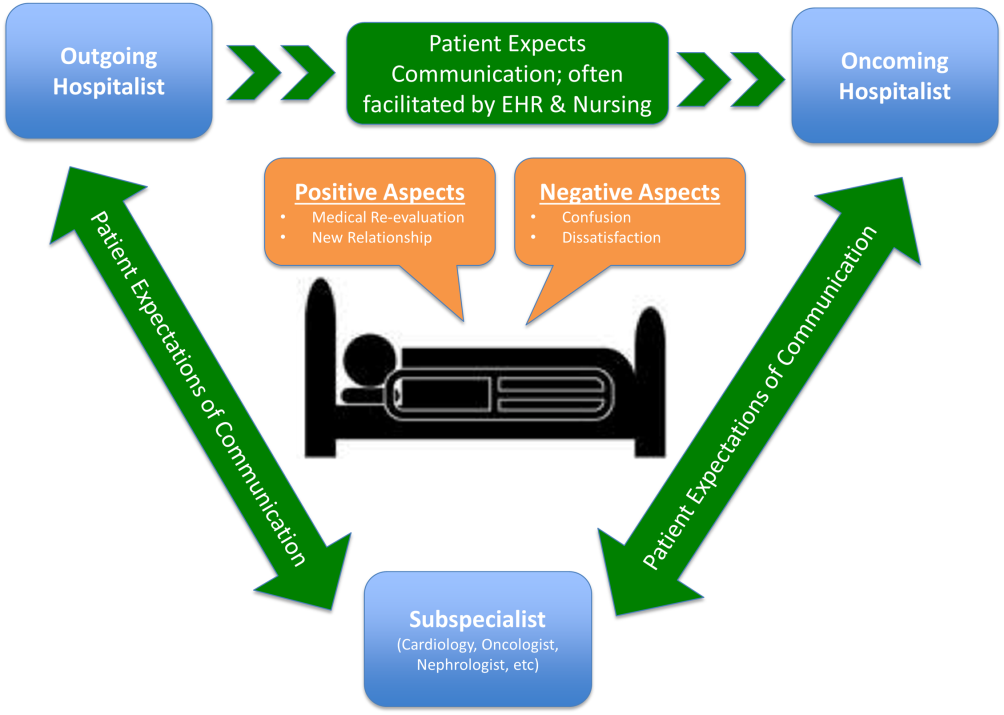
DISCUSSION
To our knowledge, this is the first qualitative investigation of the hospitalized patient's experience with service handoffs between hospitalists. The patient perspective adds a personal and first‐hand description of how fragmented care may impact the hospitalized patient experience.
Of the 6 themes, communication was found to be the most pertinent to our respondents. Because much of patient care is an inherently communicative activity, it is not surprising that patients, as well as patient safety experts, have focused on communication as an area in need of improvement in transition processes.[17, 18] Moreover, multiple medical societies have directly called for improvements within this area, and have specifically recommended clear and direct communication of treatment plans between the patient and physician, timely exchange of information, and knowledge of who is primarily in charge of the patients care.[11] Not surprisingly, each of these recommendations appears to be echoed by our participants. This theme is especially important given that good physician‐patient communication has been noted to be a major goal in achieving patient‐centered care, and has been positively correlated to medication adherence, patient satisfaction, and physical health outcomes.[19, 20, 21, 22, 23]
Although not a substitute for face‐to‐face interactions, other communication interventions between physicians and patients should be considered. For example, get to know me posters placed in patient rooms have been shown to encourage communication between patients and physicians.[24] Additionally, physician face cards have been used to improve patients' abilities to identify and clarify physicians' roles in patient care.[25] As a patient put it, If they got a new one [hospitalist], just as if I got a new medicationprint out information on themlike where they went to med school, and stuff(patient 13). These modalities may represent highly implementable, cost‐effective adjuncts to current handoff methods that may improve lines of communication between physicians and patients.
In addition to the importance placed on physician‐patient communication, interprofessional communication between hospitalists and subspecialists was also highly regarded. Studies have shown that practice‐based interprofessional communication, such as daily interdisciplinary rounds and the use of external facilitators, can improve healthcare processes and outcomes.[26] However, these interventions must be weighed with the many conflicting factors that both hospitalists and subspecialists face on daily basis, including high patient volumes, time limitations, patient availability, and scheduling conflicts.[27] None the less, the strong emphasis patients placed on this line of communication highlights this domain as an area in which hospitalist and subspecialist can work together for systematic improvement.
Patients also recognized the complexity of the transfer process between hospitalists and called for improved transparency. For example, patients repeatedly requested to be informed prior to any changes in their hospitalists, a request that remains consistent with current guidelines.[11] There also existed a strong desire for a more formalized process of transitioning between hospitalists, which often described a handoff procedure that would occur at the patient's bedside. This desire seems to be mirrored in the data that show that patients prefer to interact with their care team at the bedside and report higher satisfaction when they are involved with their care.[28, 29] Unfortunately, this desire for more direct interaction with physicians runs counter to the current paradigm of patient care, where most activities on rounds do not take place at the bedside.[30]
In contrast to patient's calls for improved transparency, an equally large portion of patients expressed relative indifference to the transition. Whereas on the surface this may seem salutary, some studies suggest that a lack of patient activation and engagement may have adverse effects toward patients' overall care.[31] Furthermore, others have shown evidence of better healthcare experiences, improved health outcomes, and lower costs in patients who are more active in their care.[30, 31] Altogether, this suggests that despite some patients' indifference, physicians should continue to engage patients in their hospital care.[32]
Although prevailing sentiments among patient safety advocates are that patient handoffs are inherently dangerous and place patients at increased risk of adverse events, patients did not always share this concern. A frequently occurring theme was that the transition is an opportunity for medical reevaluation or the establishment of a new, possibly improved therapeutic alliance. Recognizing this viewpoint offers oncoming hospitalists the opportunity to focus on issues that the patient may have felt were not being properly addressed with their prior physician.
Finally, although our conceptual model is not a strict guideline, we believe that any future studies should consider this framework when constructing interventions to improve service‐level handoffs. Several interventions already exist. For instance, educational interventions, such as patient‐centered interviewing, have been shown to improve patient satisfaction, compliance with medications, lead to fewer lawsuits, and improve health outcomes.[33, 34, 35] Additional methods of keeping the patient more informed include physician face sheets and performance of the handoff at the patient's bedside. Although well known in nursing literature, the idea of physicians performing handoffs at the patient's bedside is a particularly patient‐centric process.[36] This type of intervention may have the ability to transform the handoff from the current state of a 2‐way street, in which information is passed between 2 hospitalists, to a 3‐way stop, in which both hospitalists and the patient are able to communicate at this critical junction of care.
Although our study does offer new insight into the effects of discontinuous care, its exploratory nature does have limitations. First, being performed at a single academic center limits our ability to generalize our findings. Second, perspectives of those who did not wish to participate, patients' family members or caregivers, and those who were not queried, could highly differ from those we interviewed. Additionally, we did not collect data on patients' diagnoses or reason for admission, thus limiting our ability to assess if certain diagnosis or subpopulations predispose patients to experiencing a service handoff. Third, although our study was restricted to English‐speaking patients only, we must consider that non‐English speakers would likely suffer from even greater communication barriers than those who took part in our study. Finally, our interviews and data analysis were conducted by hospitalists, which could have subconsciously influenced the interview process, and the interpretation of patient responses. However, we tried to mitigate these issues by having the same individual interview all participants, by using an interview guide to ensure cross‐cohort consistency, by using open‐ended questions, and by attempting to give patients every opportunity to express themselves.
CONCLUSIONS
From a patients' perspective, inpatient service handoffs are often opaque experiences that are highlighted by poor communication between physicians and patients. Although deficits in communication and transparency acted as barriers to a patient‐centered handoff, physicians should recognize that service handoffs may also represent opportunities for improvement, and should focus on these domains when they start on a new service.
Disclosures
All funding for this project was provided by the Section of Hospital Medicine at The University of Chicago Medical Center. The data from this article were presented at the Society of Hospital Medicine Annual Conference, National Harbor, March 31, 2015, and at the Society of General Internal Medicine National Meeting in Toronto, Canada, April 23, 2015. The authors report that no conflicts of interest, financial or otherwise, exist.
- Continuity of outpatient and inpatient care by primary care physicians for hospitalized older adults. JAMA. 2009;301(16):1671–1680. , , , , , .
- Continuity of care and the risk of preventable hospitalization in older adults. JAMA Intern Med. 2013;173(20):1879–1885. , , , et al.
- Agency for Healthcare Research and Quality. HCUPnet: a tool for identifying, tracking, and analyzing national hospital statistics. Available at: http://hcupnet.ahrq.gov/HCUPnet.jsp?Id=82B37DA366A36BAD6(8):438–444.
- Hospitalist handoffs: a systematic review and task force recommendations. J Hosp Med. 2009;4(7):433–440. , , , , , .
- The impact of fragmentation of hospitalist care on length of stay. J Hosp Med. 2010;5(6):335–338. , , , , .
- The impact of hospitalist discontinuity on hospital cost, readmissions, and patient satisfaction. J Gen Intern Med. 2014;29(7):1004–1008. , , , et al.
- The effect of hospitalist discontinuity on adverse events. J Hosp Med. 2015;10(3):147–151. , , , et al.
- Agency for Healthcare Research and Quality. HCAHPS Fact Sheet. CAHPS Hospital Survey August 2013. Available at: http://www.hcahpsonline.org/files/August_2013_HCAHPS_Fact_Sheet3.pdf. Accessed February 2, 2015.
- A conceptual framework for studying the safety of transitions in emergency care. In: Henriksen K, Battles JB, Marks ES, eds. Advances in Patient Safety: From Research to Implementation. Rockville, MD: Agency for Healthcare Research and Quality; 2005:309–321. Concepts and Methodology; vol 2. Available at: http://www.ncbi.nlm.nih.gov/books/NBK20522. Accessed January 15, 2015. , , , et al.
- Transitions of care consensus policy statement American College of Physicians‐Society of General Internal Medicine‐Society of Hospital Medicine‐American Geriatrics Society‐American College of Emergency Physicians‐Society of Academic Emergency Medicine. J Gen Intern Med. 2009;24(8):971–976. , , , et al.
- Grounded theory in medical education research: AMEE guide no. 70. Med Teach. 2012;34(10):850–861. , .
- A purposeful approach to the constant comparative method in the analysis of qualitative interviews. Qual Quant. 2002;36(4):391–409. .
- The significance of saturation. Qual Health Res. 1995;5(2):147–149. .
- Standards for reporting qualitative research: a synthesis of recommendations. Acad Med. 2014;89(9):1245–1251. , , , , .
- Consolidated criteria for reporting qualitative research (COREQ): a 32‐item checklist for interviews and focus groups. Int J Qual Health Care. 2007;19(6):349–357. , , .
- Promoting effective transitions of care at hospital discharge: a review of key issues for hospitalists. J Hosp Med. 2007;2(5):314–323. , , , .
- The Joint Commission. Hot Topics in Healthcare, Issue 2. Transitions of care: the need for collaboration across entire care continuum. Available at: http://www.jointcommission.org/assets/1/6/TOC_Hot_Topics.pdf. Accessed April 9, 2015.
- Physician communication and patient adherence to treatment: a meta‐analysis. Med Care. 2009;47(8):826–834. , .
- Impediments to adherence to post myocardial infarction medications. Curr Cardiol Rep. 2013;15(1):322. , .
- Medical specialists' patient‐centered communication and patient‐reported outcomes. Med Care. 2007;45(4):330–339. , , , , .
- Does doctor‐patient communication affect patient satisfaction with hospital care? Results of an analysis with a novel instrumental variable. Health Serv Res. 2008;43(5 pt 1):1505–1519. , , , .
- Patient‐centredness in chronic illness: what is it and does it matter? Patient Educ Couns. 2003;51(3):197–206. , , .
- Merging cultures: palliative care specialists in the medical intensive care unit. Crit Care Med. 2006;34(11 suppl):S388–S393. , , , et al.
- Improving inpatients' identification of their doctors: use of FACE cards. Jt Comm J Qual Patient Saf. 2009;35(12):613–619. , , , et al.
- Interprofessional collaboration: effects of practice‐based interventions on professional practice and healthcare outcomes. Cochrane Database Syst Rev. 2009;(3):CD000072. , , .
- Identifying and overcoming the barriers to bedside rounds: a multicenter qualitative study. Acad Med. 2014;89(2):326–334. , , , et al.
- The effect of bedside case presentations on patients' perceptions of their medical care. N Engl J Med. 1997;336(16):1150–1155. , , , , .
- Patient‐centered interprofessional collaborative care: factors associated with bedside interprofessional rounds. J Gen Intern Med. 2014;29(7):1040–1047. , , , .
- Attending rounds in the current era: what is and is not happening. JAMA Intern Med. 2013;173(12):1084–1089. , , , et al.
- What the evidence shows about patient activation: better health outcomes and care experiences; fewer data on costs. Health Aff (Millwood). 2013;32(2):207–214. , .
- When patient activation levels change, health outcomes and costs change, too. Health Aff Proj Hope. 2015;34(3):431–437. , , , , .
- Evidence‐based guidelines for teaching patient‐centered interviewing. Patient Educ Couns. 2000;39(1):27–36. , , , et al.
- Meta‐analysis of correlates of provider behavior in medical encounters. Med Care. 1988;26(7):657–675. , , .
- Characteristics of potential plaintiffs in malpractice litigation. Ann Intern Med. 1994;120(9):792–798. , .
- Bedside shift reports: what does the evidence say? J Nurs Adm. 2014;44(10):541–545. , , , , .
Studies examining the importance of continuity of care have shown that patients who maintain a continuous relationship with a single physician have improved outcomes.[1, 2] However, most of these studies were performed in the outpatient, rather than the inpatient setting. With over 35 million patients admitted to hospitals in 2013, along with the significant increase in hospital discontinuity over recent years, the impact of inpatient continuity of care on quality outcomes and patient satisfaction is becoming increasingly relevant.[3, 4]
Service handoffs, when a physician hands over treatment responsibility for a panel of patients and is not expected to return, are a type of handoff that contributes to inpatient discontinuity. In particular, service handoffs between hospitalists are an especially common and inherently risky type of transition, as there is a severing of an established relationship during a patient's hospitalization. Unfortunately, due to the lack of evidence on the effects of service handoffs, current guidelines are limited in their recommendations.[5] Whereas several recent studies have begun to explore the effects of these handoffs, no prior study has examined this issue from a patient's perspective.[6, 7, 8]
Patients are uniquely positioned to inform us about their experiences in care transitions. Furthermore, with patient satisfaction now affecting Medicare reimbursement rates, patient experiences while in the hospital are becoming even more significant.[9] Despite this emphasis toward more patient‐centered care, no study has explored the hospitalized patient's experience with hospitalist service handoffs. Our goal was to qualitatively assess the hospitalized patients' experiences with transitions between hospitalists to develop a conceptual model to inform future work on improving inpatient transitions of care.
METHODS
Sampling and Recruitment
We conducted bedside interviews of hospitalized patients at an urban academic medical center from October 2014 through December 2014. The hospitalist service consists of a physician and an advanced nurse practitioner (ANP) who divide a panel of patients that consist of general medicine and subspecialty patients who are often comanaged with hepatology, oncology, and nephrology subspecialists. We performed a purposive selection of patients who could potentially comment on their experience with a hospitalist service transition using the following method: 48 hours after a service handoff (ie, an outgoing physician completing 1 week on service, then transfers the care of the patient to a new oncoming hospitalist), oncoming hospitalists were approached and asked if any patient on their service had experienced a service handoff and still remained in the hospital. A 48‐hour time period was chosen to give the patients time to familiarize themselves with their new hospitalist, allowing them to properly comment on the handoff. Patients who were managed by the ANP, who were non‐English speaking, or who were deemed to have an altered mental status based on clinical suspicion by the interviewing physician (C.M.W.) were excluded from participation. Following each weekly service transition, a list of patients who met the above criteria was collected from 4 nonteaching hospitalist services, and were approached by the primary investigator (C.M.W.) and asked if they would be willing to participate. All patients were general medicine patients and no exclusions were made based on physical location within the hospital. Those who agreed provided signed written consent prior to participation to allow access to the electronic health records (EHRs) by study personnel.
Data Collection
Patients were administered a 9‐question, semistructured interview that was informed by expert opinion and existing literature, which was developed to elicit their perspective regarding their transition between hospitalists.[10, 11] No formal changes were made to the interview guide during the study period, and all patients were asked the same questions. Outcomes from interim analysis guided further questioning in subsequent interviews so as to increase the depth of patient responses (ie, Can you explain your response in greater depth?). Prior to the interview, patients were read a description of a hospitalist, and were reminded which hospitalists had cared for them during their stay (see Supporting Information, Appendix 1, in the online version of this article). If family members or a caregiver were present at the time of interview, they were asked not to comment. No repeat interviews were carried out.
All interviews were performed privately in single‐occupancy rooms, digitally recorded using an iPad (Apple, Cupertino, CA) and professionally transcribed verbatim (Rev, San Francisco, CA). All analysis was performed using MAXQDA Software (VERBI Software GmbH, Berlin, Germany). We obtained demographic information about each patient through chart review
Data Analysis
Grounded theory was utilized, with an inductive approach with no a priori hypothesis.[12] The constant comparative method was used to generate emerging and reoccurring themes.[13] Units of analysis were sentences and phrases. Our research team consisted of 4 academic hospitalists, 2 with backgrounds in clinical medicine, medical education, and qualitative analysis (J.M.F., V.M.A.), 1 as a clinician (C.M.W.), and 1 in health economics (D.O.M.). Interim analysis was performed on a weekly basis (C.M.W.), during which time a coding template was created and refined through an iterative process (C.M.W., J.M.F.). All disagreements in coded themes were resolved through group discussion until full consensus was reached. Each week, responses were assessed for thematic saturation.[14] Interviews were continued if new themes arose during this analysis. Data collection was ended once we ceased to extract new topics from participants. A summary of all themes was then presented to a group of 10 patients who met the same inclusion criteria for respondent validation and member checking. All reporting was performed within the Standards for Reporting Qualitative Research, with additional guidance derived from the Consolidated Criteria for Reporting Qualitative Research.[15, 16] The University of Chicago Institutional Review Board approved this protocol.
RESULTS
In total, 43 eligible patients were recruited, and 40 (93%) agreed to participate. Interviewed patients were between 51 and 65 (39%) years old, had a mean age of 54.5 (15) years, were predominantly female (65%), African American (58%), had a median length of stay at the time of interview of 6.5 days (interquartile range [IQR]: 48), and had an average of 2.0 (IQR: 13) hospitalists oversee their care at the time of interview (Table 1). Interview times ranged from 10:25 to 25:48 minutes, with an average of 15:32 minutes.
Value | |
---|---|
| |
Response rate, n (%) | 40/43 (93) |
Age, mean SD | 54.5 15 |
Sex, n (%) | |
Female | 26 (65) |
Male | 14 (35) |
Race, n (%) | |
African American | 23 (58) |
White | 16 (40) |
Hispanic | 1 (2) |
Median LOS at time of interview, d (IQR) | 6.5 (48) |
Median no. of hospitalists at time of interview, n (IQR) | 2.0 (13) |
We identified 6 major themes on patient perceptions of hospitalist service handoffs including (1) physician‐patient communication, (2) transparency in the hospitalist transition process, (3) indifference toward the hospitalist transition, (4) hospitalist‐subspecialist communication, (5) recognition of new opportunities due to a transition, and (6) hospitalists' bedside manner (Table 2).
Themes | Subthemes | Representative Quotes |
---|---|---|
Physician‐patient communication | Patients dislike redundant communication with oncoming hospitalist. | I mean it's just you always have to explain your situation over and over and over again. (patient 14) |
When I said it once already, then you're repeating it to another doctor. I feel as if that hospitalist didn't talk to the other hospitalist. (patient 7) | ||
Poor communication can negatively affect the doctor‐patient relationship. | They don't really want to explain things. They don't think I'll understand. I think & yeah, I'm okay. You don't even have to put it in layman's terms. I know medical. I'm in nursing school. I have a year left. But even if you didn't know that, I would still hope you would try to tell me what was going on instead of just doing it in your head, and treating it. (patient 2) | |
I mean it's just you always have to explain your situation over and over and over again. After a while you just stop trusting them. (patient 20) | ||
Good communication can positively affect the doctor‐patient relationship. | Just continue with the communication, the open communication, and always stress to me that I have a voice and just going out of their way to do whatever they can to help me through whatever I'm going through. (patient 1) | |
Transparency in transition | Patients want to be informed prior to a service changeover. | I think they should be told immediately, even maybe given prior notice, like this may happen, just so you're not surprised when it happens. (patient 15) |
When the doctor approached me, he let me know that he wasn't going to be here the next day and there was going to be another doctor coming in. That made me feel comfortable. (patient 9) | ||
Patients desire a more formalized process in the service changeover. | People want things to be consistent. People don't like change. They like routine. So, if he's leaving, you're coming on, I'd like for him to bring you in, introduce you to me, and for you just assure me that I'll take care of you. (patient 4) | |
Just like when you get a new medication, you're given all this information on it. So when you get a new hospitalist, shouldn't I get all the information on them? Like where they went to school, what they look like. (patient 23) | ||
Patients want clearer definition of the roles the physicians will play in their care. | The first time I was hospitalized for the first time I had all these different doctors coming in, and I had the residency, and the specialists, and the department, and I don't know who's who. What I asked them to do is when they come in the room, which they did, but introduce it a little more for me. Write it down like these are the special team and these are the doctors because even though they come in and give me their name, I have no idea what they're doing. (patient 5) | |
Someone should explain the setup and who people are. Someone would say, Okay when you're in a hospital this is your [doctor's] role. Like they should have booklets and everything. (patient 19) | ||
Indifference toward transition | Many patients have trust in service changeovers. | [S]o as long as everybody's on board and communicates well and efficiently, I don't have a problem with it. (patient 6) |
To me, it really wasn't no preference, as long as I was getting the care that I needed. (patient 21) | ||
It's not a concern as long as they're on the same page. (patient 17) | ||
Hospitalist‐specialist communication | Patients are concerned about communication between their hospitalist and their subspecialists. | The more cooks you get in the kitchen, the more things get to get lost, so I'm always concerned that they're not sharing the same information, especially when you're getting asked the same questions that you might have just answered the last hour ago. (patient 9) |
I don't know if the hospitalist are talking to them [subspecialist]. They haven't got time. (patient 35) | ||
Patients place trust in the communication between hospitalist and subspecialist. | I think among the teams themselveswhich is my pain doctor, Dr. K's group, the oncology group itself, they switch off and trade with each other and they all speak the same language so that works out good. (patient 3) | |
Lack of interprofessional communication can lead to patient concern. | I was afraid that one was going to drop the ball on something and not pass something on, or you know. (patient 11) | |
I had numerous doctors who all seemed to not communicate with each other at all or did so by email or whatever. They didn't just sit down together and say we feel this way and we feel that way. I didn't like that at all. (patient 10) | ||
New opportunities due to transition | Patients see new doctor as opportunity for medical reevaluation. | I see it as two heads are better than one, three heads are better than one, four heads are better than one. When people put their heads together to work towards a common goal, especially when they're, you know, people working their craft, it can't be bad. (patient 9) |
I finally got my ears looked atbecause I've asked to have my ears looked at since Mondayand the new doc is trying to make an effort to look at them. (patient 39) | ||
Patients see service changeover as an opportunity to form a better personal relationship. | Having a new hospitalist it gives you opportunity for a new beginning. (patient 11) | |
Bedside manner | Good bedside manner can assist in a service changeover. | Some of them are all business‐like but some of them are, Well how do you feel today? Hi, how are you? So this made a little difference. You feel more comfortable. You're going to be more comfortable with them. Their bedside manner helps. (patient 16) |
It's just like when a doctor sits down and talks to you, they just seem more relaxed and more .... I know they're very busy and they have lots of things to do and other patients to see, but while they're in there with you, you know, you don't get too much time with them. So bedside manner is just so important. (patient 24) | ||
Poor bedside manner can be detrimental in transition. | [B]ecause they be so busy they claim they don't have time just to sit and talk to a patient, and make sure they all right. (patient 17) |
Physician‐Patient Communication
Communication between the physician and the patient was an important element in patients' assessment of their experience. Patient's tended to divide physician‐patient communication into 2 categories: good communication, which consisted of open communication (patient 1) and patient engagement, and bad communication, which was described as physicians not sharing information or taking the time to explain the course of care in words that I'll understand (patient 2). Patients also described dissatisfaction with redundant communication between multiple hospitalists and the frustration of often having to describe their clinical course to multiple providers.
Transparency in Communication
The desire to have greater transparency in the handoff process was another common theme. This was likely due to the fact that 34/40 (85%) of surveyed patients were unaware that a service changeover had ever taken place. This lack of transparency was viewed to have further downstream consequences as patients stated that there should be a level of transparency, and when it's not, then there is always trust issues (patient 1). Upon further questioning as to how to make the process more transparent, many patients recommended a formalized, face‐to‐face introduction involving the patient and both hospitalists, in which the outgoing hospitalist would, bring you [oncoming hospitalist] in, and introduce you to me (patient 4).
Patients often stated that given the large spectrum of physicians they might encounter during their stay (ie, medical student, resident, hospitalist attending, subspecialty fellow, subspecialist attending), clearer definitions of physicians' roles are needed.
Hospitalist‐Specialist Communication
Concern about the communication between their hospitalist and subspecialist was another predominant theme. Conflicting and unclear directions from multiple services were especially frustrating, as a patient stated, One guy took me off this pill, the other guy wants me on that pill, I'm like okay, I can't do both (patient 8). Furthermore, a subset of patients referenced their subspecialist as their primary care provider and preferred their subspecialist for guidance in their hospital course, rather than their hospitalist. This often appeared in cases where the patient had an established relationship with the subspecialist prior to their hospitalization.
New Opportunities Due to Transition
Patients expressed positive feelings toward service handoffs by viewing the transition as an opportunity for medical reevaluation by a new physician. Patients told of instances in which a specific complaint was not being addressed by the first physician, but would be addressed by the second (oncoming) physician. A commonly expressed idea was that the oncoming physician might know something that he [Dr. B] didn't know, and since Dr. B was only here for a week, why not give him [oncoming hospitalist] a chance (patient 10). Patients would also describe the transition as an opportunity to form, and possibly improve, therapeutic alliances with a new hospitalist.
Bedside Manner
Bedside manner was another commonly mentioned thematic element. Patients were often quick to forget prior problems or issues that they may have suffered because of the transition if the oncoming physician was perceived to have a good bedside manner, often described as someone who formally introduced themselves, was considered relaxed, and would take the time to sit and talk with the patient. As a patient put it, [S]he sat down and got to know meand asked me what I wanted to do (patient 12). Conversely, patients described instances in which a perceived bad bedside manner led to a poor relationship between the physician and the patient, in which trust and comfort (patient 11) were sacrificed.
Indifference Toward Transition
In contrast to some of the previous findings, which called for improved interactions between physicians and patients, we also discovered a theme of indifference toward the transition. Several patients stated feelings of trust with the medical system, and were content with the service changeover as long as they felt that their medical needs were being met. Patients also tended to express a level of acceptance with the transition, and tended to believe that this was the price we pay for being here [in the hospital] (patient 7).
Conceptual Model
Following the collection and analysis of all patient responses, all themes were utilized to construct the ideal patient‐centered service handoff. The ideal transition describes open lines of communication between all involved parties, is facilitated by multiple modalities, such as the EHRs and nursing staff, and recognizes the patient as the primary stakeholder (Figure 1).
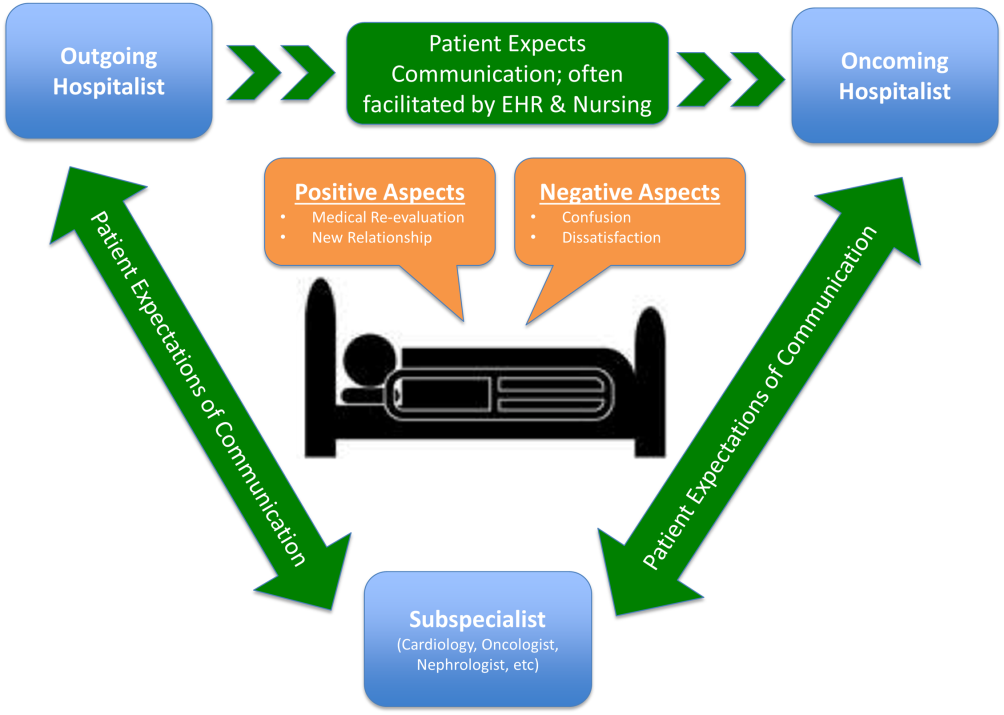
DISCUSSION
To our knowledge, this is the first qualitative investigation of the hospitalized patient's experience with service handoffs between hospitalists. The patient perspective adds a personal and first‐hand description of how fragmented care may impact the hospitalized patient experience.
Of the 6 themes, communication was found to be the most pertinent to our respondents. Because much of patient care is an inherently communicative activity, it is not surprising that patients, as well as patient safety experts, have focused on communication as an area in need of improvement in transition processes.[17, 18] Moreover, multiple medical societies have directly called for improvements within this area, and have specifically recommended clear and direct communication of treatment plans between the patient and physician, timely exchange of information, and knowledge of who is primarily in charge of the patients care.[11] Not surprisingly, each of these recommendations appears to be echoed by our participants. This theme is especially important given that good physician‐patient communication has been noted to be a major goal in achieving patient‐centered care, and has been positively correlated to medication adherence, patient satisfaction, and physical health outcomes.[19, 20, 21, 22, 23]
Although not a substitute for face‐to‐face interactions, other communication interventions between physicians and patients should be considered. For example, get to know me posters placed in patient rooms have been shown to encourage communication between patients and physicians.[24] Additionally, physician face cards have been used to improve patients' abilities to identify and clarify physicians' roles in patient care.[25] As a patient put it, If they got a new one [hospitalist], just as if I got a new medicationprint out information on themlike where they went to med school, and stuff(patient 13). These modalities may represent highly implementable, cost‐effective adjuncts to current handoff methods that may improve lines of communication between physicians and patients.
In addition to the importance placed on physician‐patient communication, interprofessional communication between hospitalists and subspecialists was also highly regarded. Studies have shown that practice‐based interprofessional communication, such as daily interdisciplinary rounds and the use of external facilitators, can improve healthcare processes and outcomes.[26] However, these interventions must be weighed with the many conflicting factors that both hospitalists and subspecialists face on daily basis, including high patient volumes, time limitations, patient availability, and scheduling conflicts.[27] None the less, the strong emphasis patients placed on this line of communication highlights this domain as an area in which hospitalist and subspecialist can work together for systematic improvement.
Patients also recognized the complexity of the transfer process between hospitalists and called for improved transparency. For example, patients repeatedly requested to be informed prior to any changes in their hospitalists, a request that remains consistent with current guidelines.[11] There also existed a strong desire for a more formalized process of transitioning between hospitalists, which often described a handoff procedure that would occur at the patient's bedside. This desire seems to be mirrored in the data that show that patients prefer to interact with their care team at the bedside and report higher satisfaction when they are involved with their care.[28, 29] Unfortunately, this desire for more direct interaction with physicians runs counter to the current paradigm of patient care, where most activities on rounds do not take place at the bedside.[30]
In contrast to patient's calls for improved transparency, an equally large portion of patients expressed relative indifference to the transition. Whereas on the surface this may seem salutary, some studies suggest that a lack of patient activation and engagement may have adverse effects toward patients' overall care.[31] Furthermore, others have shown evidence of better healthcare experiences, improved health outcomes, and lower costs in patients who are more active in their care.[30, 31] Altogether, this suggests that despite some patients' indifference, physicians should continue to engage patients in their hospital care.[32]
Although prevailing sentiments among patient safety advocates are that patient handoffs are inherently dangerous and place patients at increased risk of adverse events, patients did not always share this concern. A frequently occurring theme was that the transition is an opportunity for medical reevaluation or the establishment of a new, possibly improved therapeutic alliance. Recognizing this viewpoint offers oncoming hospitalists the opportunity to focus on issues that the patient may have felt were not being properly addressed with their prior physician.
Finally, although our conceptual model is not a strict guideline, we believe that any future studies should consider this framework when constructing interventions to improve service‐level handoffs. Several interventions already exist. For instance, educational interventions, such as patient‐centered interviewing, have been shown to improve patient satisfaction, compliance with medications, lead to fewer lawsuits, and improve health outcomes.[33, 34, 35] Additional methods of keeping the patient more informed include physician face sheets and performance of the handoff at the patient's bedside. Although well known in nursing literature, the idea of physicians performing handoffs at the patient's bedside is a particularly patient‐centric process.[36] This type of intervention may have the ability to transform the handoff from the current state of a 2‐way street, in which information is passed between 2 hospitalists, to a 3‐way stop, in which both hospitalists and the patient are able to communicate at this critical junction of care.
Although our study does offer new insight into the effects of discontinuous care, its exploratory nature does have limitations. First, being performed at a single academic center limits our ability to generalize our findings. Second, perspectives of those who did not wish to participate, patients' family members or caregivers, and those who were not queried, could highly differ from those we interviewed. Additionally, we did not collect data on patients' diagnoses or reason for admission, thus limiting our ability to assess if certain diagnosis or subpopulations predispose patients to experiencing a service handoff. Third, although our study was restricted to English‐speaking patients only, we must consider that non‐English speakers would likely suffer from even greater communication barriers than those who took part in our study. Finally, our interviews and data analysis were conducted by hospitalists, which could have subconsciously influenced the interview process, and the interpretation of patient responses. However, we tried to mitigate these issues by having the same individual interview all participants, by using an interview guide to ensure cross‐cohort consistency, by using open‐ended questions, and by attempting to give patients every opportunity to express themselves.
CONCLUSIONS
From a patients' perspective, inpatient service handoffs are often opaque experiences that are highlighted by poor communication between physicians and patients. Although deficits in communication and transparency acted as barriers to a patient‐centered handoff, physicians should recognize that service handoffs may also represent opportunities for improvement, and should focus on these domains when they start on a new service.
Disclosures
All funding for this project was provided by the Section of Hospital Medicine at The University of Chicago Medical Center. The data from this article were presented at the Society of Hospital Medicine Annual Conference, National Harbor, March 31, 2015, and at the Society of General Internal Medicine National Meeting in Toronto, Canada, April 23, 2015. The authors report that no conflicts of interest, financial or otherwise, exist.
Studies examining the importance of continuity of care have shown that patients who maintain a continuous relationship with a single physician have improved outcomes.[1, 2] However, most of these studies were performed in the outpatient, rather than the inpatient setting. With over 35 million patients admitted to hospitals in 2013, along with the significant increase in hospital discontinuity over recent years, the impact of inpatient continuity of care on quality outcomes and patient satisfaction is becoming increasingly relevant.[3, 4]
Service handoffs, when a physician hands over treatment responsibility for a panel of patients and is not expected to return, are a type of handoff that contributes to inpatient discontinuity. In particular, service handoffs between hospitalists are an especially common and inherently risky type of transition, as there is a severing of an established relationship during a patient's hospitalization. Unfortunately, due to the lack of evidence on the effects of service handoffs, current guidelines are limited in their recommendations.[5] Whereas several recent studies have begun to explore the effects of these handoffs, no prior study has examined this issue from a patient's perspective.[6, 7, 8]
Patients are uniquely positioned to inform us about their experiences in care transitions. Furthermore, with patient satisfaction now affecting Medicare reimbursement rates, patient experiences while in the hospital are becoming even more significant.[9] Despite this emphasis toward more patient‐centered care, no study has explored the hospitalized patient's experience with hospitalist service handoffs. Our goal was to qualitatively assess the hospitalized patients' experiences with transitions between hospitalists to develop a conceptual model to inform future work on improving inpatient transitions of care.
METHODS
Sampling and Recruitment
We conducted bedside interviews of hospitalized patients at an urban academic medical center from October 2014 through December 2014. The hospitalist service consists of a physician and an advanced nurse practitioner (ANP) who divide a panel of patients that consist of general medicine and subspecialty patients who are often comanaged with hepatology, oncology, and nephrology subspecialists. We performed a purposive selection of patients who could potentially comment on their experience with a hospitalist service transition using the following method: 48 hours after a service handoff (ie, an outgoing physician completing 1 week on service, then transfers the care of the patient to a new oncoming hospitalist), oncoming hospitalists were approached and asked if any patient on their service had experienced a service handoff and still remained in the hospital. A 48‐hour time period was chosen to give the patients time to familiarize themselves with their new hospitalist, allowing them to properly comment on the handoff. Patients who were managed by the ANP, who were non‐English speaking, or who were deemed to have an altered mental status based on clinical suspicion by the interviewing physician (C.M.W.) were excluded from participation. Following each weekly service transition, a list of patients who met the above criteria was collected from 4 nonteaching hospitalist services, and were approached by the primary investigator (C.M.W.) and asked if they would be willing to participate. All patients were general medicine patients and no exclusions were made based on physical location within the hospital. Those who agreed provided signed written consent prior to participation to allow access to the electronic health records (EHRs) by study personnel.
Data Collection
Patients were administered a 9‐question, semistructured interview that was informed by expert opinion and existing literature, which was developed to elicit their perspective regarding their transition between hospitalists.[10, 11] No formal changes were made to the interview guide during the study period, and all patients were asked the same questions. Outcomes from interim analysis guided further questioning in subsequent interviews so as to increase the depth of patient responses (ie, Can you explain your response in greater depth?). Prior to the interview, patients were read a description of a hospitalist, and were reminded which hospitalists had cared for them during their stay (see Supporting Information, Appendix 1, in the online version of this article). If family members or a caregiver were present at the time of interview, they were asked not to comment. No repeat interviews were carried out.
All interviews were performed privately in single‐occupancy rooms, digitally recorded using an iPad (Apple, Cupertino, CA) and professionally transcribed verbatim (Rev, San Francisco, CA). All analysis was performed using MAXQDA Software (VERBI Software GmbH, Berlin, Germany). We obtained demographic information about each patient through chart review
Data Analysis
Grounded theory was utilized, with an inductive approach with no a priori hypothesis.[12] The constant comparative method was used to generate emerging and reoccurring themes.[13] Units of analysis were sentences and phrases. Our research team consisted of 4 academic hospitalists, 2 with backgrounds in clinical medicine, medical education, and qualitative analysis (J.M.F., V.M.A.), 1 as a clinician (C.M.W.), and 1 in health economics (D.O.M.). Interim analysis was performed on a weekly basis (C.M.W.), during which time a coding template was created and refined through an iterative process (C.M.W., J.M.F.). All disagreements in coded themes were resolved through group discussion until full consensus was reached. Each week, responses were assessed for thematic saturation.[14] Interviews were continued if new themes arose during this analysis. Data collection was ended once we ceased to extract new topics from participants. A summary of all themes was then presented to a group of 10 patients who met the same inclusion criteria for respondent validation and member checking. All reporting was performed within the Standards for Reporting Qualitative Research, with additional guidance derived from the Consolidated Criteria for Reporting Qualitative Research.[15, 16] The University of Chicago Institutional Review Board approved this protocol.
RESULTS
In total, 43 eligible patients were recruited, and 40 (93%) agreed to participate. Interviewed patients were between 51 and 65 (39%) years old, had a mean age of 54.5 (15) years, were predominantly female (65%), African American (58%), had a median length of stay at the time of interview of 6.5 days (interquartile range [IQR]: 48), and had an average of 2.0 (IQR: 13) hospitalists oversee their care at the time of interview (Table 1). Interview times ranged from 10:25 to 25:48 minutes, with an average of 15:32 minutes.
Value | |
---|---|
| |
Response rate, n (%) | 40/43 (93) |
Age, mean SD | 54.5 15 |
Sex, n (%) | |
Female | 26 (65) |
Male | 14 (35) |
Race, n (%) | |
African American | 23 (58) |
White | 16 (40) |
Hispanic | 1 (2) |
Median LOS at time of interview, d (IQR) | 6.5 (48) |
Median no. of hospitalists at time of interview, n (IQR) | 2.0 (13) |
We identified 6 major themes on patient perceptions of hospitalist service handoffs including (1) physician‐patient communication, (2) transparency in the hospitalist transition process, (3) indifference toward the hospitalist transition, (4) hospitalist‐subspecialist communication, (5) recognition of new opportunities due to a transition, and (6) hospitalists' bedside manner (Table 2).
Themes | Subthemes | Representative Quotes |
---|---|---|
Physician‐patient communication | Patients dislike redundant communication with oncoming hospitalist. | I mean it's just you always have to explain your situation over and over and over again. (patient 14) |
When I said it once already, then you're repeating it to another doctor. I feel as if that hospitalist didn't talk to the other hospitalist. (patient 7) | ||
Poor communication can negatively affect the doctor‐patient relationship. | They don't really want to explain things. They don't think I'll understand. I think & yeah, I'm okay. You don't even have to put it in layman's terms. I know medical. I'm in nursing school. I have a year left. But even if you didn't know that, I would still hope you would try to tell me what was going on instead of just doing it in your head, and treating it. (patient 2) | |
I mean it's just you always have to explain your situation over and over and over again. After a while you just stop trusting them. (patient 20) | ||
Good communication can positively affect the doctor‐patient relationship. | Just continue with the communication, the open communication, and always stress to me that I have a voice and just going out of their way to do whatever they can to help me through whatever I'm going through. (patient 1) | |
Transparency in transition | Patients want to be informed prior to a service changeover. | I think they should be told immediately, even maybe given prior notice, like this may happen, just so you're not surprised when it happens. (patient 15) |
When the doctor approached me, he let me know that he wasn't going to be here the next day and there was going to be another doctor coming in. That made me feel comfortable. (patient 9) | ||
Patients desire a more formalized process in the service changeover. | People want things to be consistent. People don't like change. They like routine. So, if he's leaving, you're coming on, I'd like for him to bring you in, introduce you to me, and for you just assure me that I'll take care of you. (patient 4) | |
Just like when you get a new medication, you're given all this information on it. So when you get a new hospitalist, shouldn't I get all the information on them? Like where they went to school, what they look like. (patient 23) | ||
Patients want clearer definition of the roles the physicians will play in their care. | The first time I was hospitalized for the first time I had all these different doctors coming in, and I had the residency, and the specialists, and the department, and I don't know who's who. What I asked them to do is when they come in the room, which they did, but introduce it a little more for me. Write it down like these are the special team and these are the doctors because even though they come in and give me their name, I have no idea what they're doing. (patient 5) | |
Someone should explain the setup and who people are. Someone would say, Okay when you're in a hospital this is your [doctor's] role. Like they should have booklets and everything. (patient 19) | ||
Indifference toward transition | Many patients have trust in service changeovers. | [S]o as long as everybody's on board and communicates well and efficiently, I don't have a problem with it. (patient 6) |
To me, it really wasn't no preference, as long as I was getting the care that I needed. (patient 21) | ||
It's not a concern as long as they're on the same page. (patient 17) | ||
Hospitalist‐specialist communication | Patients are concerned about communication between their hospitalist and their subspecialists. | The more cooks you get in the kitchen, the more things get to get lost, so I'm always concerned that they're not sharing the same information, especially when you're getting asked the same questions that you might have just answered the last hour ago. (patient 9) |
I don't know if the hospitalist are talking to them [subspecialist]. They haven't got time. (patient 35) | ||
Patients place trust in the communication between hospitalist and subspecialist. | I think among the teams themselveswhich is my pain doctor, Dr. K's group, the oncology group itself, they switch off and trade with each other and they all speak the same language so that works out good. (patient 3) | |
Lack of interprofessional communication can lead to patient concern. | I was afraid that one was going to drop the ball on something and not pass something on, or you know. (patient 11) | |
I had numerous doctors who all seemed to not communicate with each other at all or did so by email or whatever. They didn't just sit down together and say we feel this way and we feel that way. I didn't like that at all. (patient 10) | ||
New opportunities due to transition | Patients see new doctor as opportunity for medical reevaluation. | I see it as two heads are better than one, three heads are better than one, four heads are better than one. When people put their heads together to work towards a common goal, especially when they're, you know, people working their craft, it can't be bad. (patient 9) |
I finally got my ears looked atbecause I've asked to have my ears looked at since Mondayand the new doc is trying to make an effort to look at them. (patient 39) | ||
Patients see service changeover as an opportunity to form a better personal relationship. | Having a new hospitalist it gives you opportunity for a new beginning. (patient 11) | |
Bedside manner | Good bedside manner can assist in a service changeover. | Some of them are all business‐like but some of them are, Well how do you feel today? Hi, how are you? So this made a little difference. You feel more comfortable. You're going to be more comfortable with them. Their bedside manner helps. (patient 16) |
It's just like when a doctor sits down and talks to you, they just seem more relaxed and more .... I know they're very busy and they have lots of things to do and other patients to see, but while they're in there with you, you know, you don't get too much time with them. So bedside manner is just so important. (patient 24) | ||
Poor bedside manner can be detrimental in transition. | [B]ecause they be so busy they claim they don't have time just to sit and talk to a patient, and make sure they all right. (patient 17) |
Physician‐Patient Communication
Communication between the physician and the patient was an important element in patients' assessment of their experience. Patient's tended to divide physician‐patient communication into 2 categories: good communication, which consisted of open communication (patient 1) and patient engagement, and bad communication, which was described as physicians not sharing information or taking the time to explain the course of care in words that I'll understand (patient 2). Patients also described dissatisfaction with redundant communication between multiple hospitalists and the frustration of often having to describe their clinical course to multiple providers.
Transparency in Communication
The desire to have greater transparency in the handoff process was another common theme. This was likely due to the fact that 34/40 (85%) of surveyed patients were unaware that a service changeover had ever taken place. This lack of transparency was viewed to have further downstream consequences as patients stated that there should be a level of transparency, and when it's not, then there is always trust issues (patient 1). Upon further questioning as to how to make the process more transparent, many patients recommended a formalized, face‐to‐face introduction involving the patient and both hospitalists, in which the outgoing hospitalist would, bring you [oncoming hospitalist] in, and introduce you to me (patient 4).
Patients often stated that given the large spectrum of physicians they might encounter during their stay (ie, medical student, resident, hospitalist attending, subspecialty fellow, subspecialist attending), clearer definitions of physicians' roles are needed.
Hospitalist‐Specialist Communication
Concern about the communication between their hospitalist and subspecialist was another predominant theme. Conflicting and unclear directions from multiple services were especially frustrating, as a patient stated, One guy took me off this pill, the other guy wants me on that pill, I'm like okay, I can't do both (patient 8). Furthermore, a subset of patients referenced their subspecialist as their primary care provider and preferred their subspecialist for guidance in their hospital course, rather than their hospitalist. This often appeared in cases where the patient had an established relationship with the subspecialist prior to their hospitalization.
New Opportunities Due to Transition
Patients expressed positive feelings toward service handoffs by viewing the transition as an opportunity for medical reevaluation by a new physician. Patients told of instances in which a specific complaint was not being addressed by the first physician, but would be addressed by the second (oncoming) physician. A commonly expressed idea was that the oncoming physician might know something that he [Dr. B] didn't know, and since Dr. B was only here for a week, why not give him [oncoming hospitalist] a chance (patient 10). Patients would also describe the transition as an opportunity to form, and possibly improve, therapeutic alliances with a new hospitalist.
Bedside Manner
Bedside manner was another commonly mentioned thematic element. Patients were often quick to forget prior problems or issues that they may have suffered because of the transition if the oncoming physician was perceived to have a good bedside manner, often described as someone who formally introduced themselves, was considered relaxed, and would take the time to sit and talk with the patient. As a patient put it, [S]he sat down and got to know meand asked me what I wanted to do (patient 12). Conversely, patients described instances in which a perceived bad bedside manner led to a poor relationship between the physician and the patient, in which trust and comfort (patient 11) were sacrificed.
Indifference Toward Transition
In contrast to some of the previous findings, which called for improved interactions between physicians and patients, we also discovered a theme of indifference toward the transition. Several patients stated feelings of trust with the medical system, and were content with the service changeover as long as they felt that their medical needs were being met. Patients also tended to express a level of acceptance with the transition, and tended to believe that this was the price we pay for being here [in the hospital] (patient 7).
Conceptual Model
Following the collection and analysis of all patient responses, all themes were utilized to construct the ideal patient‐centered service handoff. The ideal transition describes open lines of communication between all involved parties, is facilitated by multiple modalities, such as the EHRs and nursing staff, and recognizes the patient as the primary stakeholder (Figure 1).
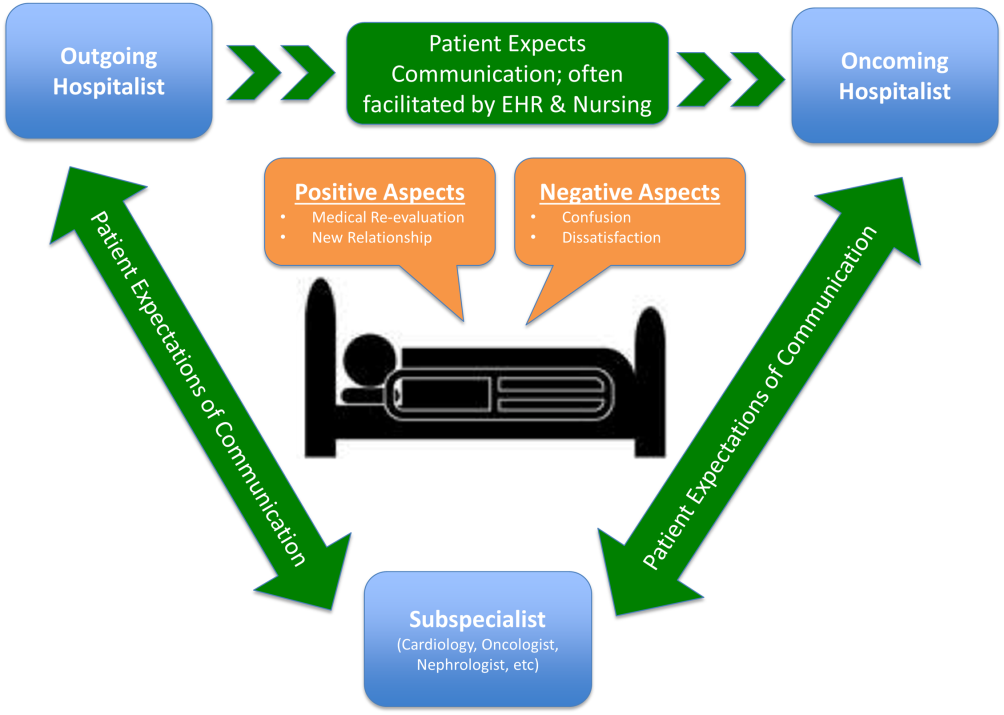
DISCUSSION
To our knowledge, this is the first qualitative investigation of the hospitalized patient's experience with service handoffs between hospitalists. The patient perspective adds a personal and first‐hand description of how fragmented care may impact the hospitalized patient experience.
Of the 6 themes, communication was found to be the most pertinent to our respondents. Because much of patient care is an inherently communicative activity, it is not surprising that patients, as well as patient safety experts, have focused on communication as an area in need of improvement in transition processes.[17, 18] Moreover, multiple medical societies have directly called for improvements within this area, and have specifically recommended clear and direct communication of treatment plans between the patient and physician, timely exchange of information, and knowledge of who is primarily in charge of the patients care.[11] Not surprisingly, each of these recommendations appears to be echoed by our participants. This theme is especially important given that good physician‐patient communication has been noted to be a major goal in achieving patient‐centered care, and has been positively correlated to medication adherence, patient satisfaction, and physical health outcomes.[19, 20, 21, 22, 23]
Although not a substitute for face‐to‐face interactions, other communication interventions between physicians and patients should be considered. For example, get to know me posters placed in patient rooms have been shown to encourage communication between patients and physicians.[24] Additionally, physician face cards have been used to improve patients' abilities to identify and clarify physicians' roles in patient care.[25] As a patient put it, If they got a new one [hospitalist], just as if I got a new medicationprint out information on themlike where they went to med school, and stuff(patient 13). These modalities may represent highly implementable, cost‐effective adjuncts to current handoff methods that may improve lines of communication between physicians and patients.
In addition to the importance placed on physician‐patient communication, interprofessional communication between hospitalists and subspecialists was also highly regarded. Studies have shown that practice‐based interprofessional communication, such as daily interdisciplinary rounds and the use of external facilitators, can improve healthcare processes and outcomes.[26] However, these interventions must be weighed with the many conflicting factors that both hospitalists and subspecialists face on daily basis, including high patient volumes, time limitations, patient availability, and scheduling conflicts.[27] None the less, the strong emphasis patients placed on this line of communication highlights this domain as an area in which hospitalist and subspecialist can work together for systematic improvement.
Patients also recognized the complexity of the transfer process between hospitalists and called for improved transparency. For example, patients repeatedly requested to be informed prior to any changes in their hospitalists, a request that remains consistent with current guidelines.[11] There also existed a strong desire for a more formalized process of transitioning between hospitalists, which often described a handoff procedure that would occur at the patient's bedside. This desire seems to be mirrored in the data that show that patients prefer to interact with their care team at the bedside and report higher satisfaction when they are involved with their care.[28, 29] Unfortunately, this desire for more direct interaction with physicians runs counter to the current paradigm of patient care, where most activities on rounds do not take place at the bedside.[30]
In contrast to patient's calls for improved transparency, an equally large portion of patients expressed relative indifference to the transition. Whereas on the surface this may seem salutary, some studies suggest that a lack of patient activation and engagement may have adverse effects toward patients' overall care.[31] Furthermore, others have shown evidence of better healthcare experiences, improved health outcomes, and lower costs in patients who are more active in their care.[30, 31] Altogether, this suggests that despite some patients' indifference, physicians should continue to engage patients in their hospital care.[32]
Although prevailing sentiments among patient safety advocates are that patient handoffs are inherently dangerous and place patients at increased risk of adverse events, patients did not always share this concern. A frequently occurring theme was that the transition is an opportunity for medical reevaluation or the establishment of a new, possibly improved therapeutic alliance. Recognizing this viewpoint offers oncoming hospitalists the opportunity to focus on issues that the patient may have felt were not being properly addressed with their prior physician.
Finally, although our conceptual model is not a strict guideline, we believe that any future studies should consider this framework when constructing interventions to improve service‐level handoffs. Several interventions already exist. For instance, educational interventions, such as patient‐centered interviewing, have been shown to improve patient satisfaction, compliance with medications, lead to fewer lawsuits, and improve health outcomes.[33, 34, 35] Additional methods of keeping the patient more informed include physician face sheets and performance of the handoff at the patient's bedside. Although well known in nursing literature, the idea of physicians performing handoffs at the patient's bedside is a particularly patient‐centric process.[36] This type of intervention may have the ability to transform the handoff from the current state of a 2‐way street, in which information is passed between 2 hospitalists, to a 3‐way stop, in which both hospitalists and the patient are able to communicate at this critical junction of care.
Although our study does offer new insight into the effects of discontinuous care, its exploratory nature does have limitations. First, being performed at a single academic center limits our ability to generalize our findings. Second, perspectives of those who did not wish to participate, patients' family members or caregivers, and those who were not queried, could highly differ from those we interviewed. Additionally, we did not collect data on patients' diagnoses or reason for admission, thus limiting our ability to assess if certain diagnosis or subpopulations predispose patients to experiencing a service handoff. Third, although our study was restricted to English‐speaking patients only, we must consider that non‐English speakers would likely suffer from even greater communication barriers than those who took part in our study. Finally, our interviews and data analysis were conducted by hospitalists, which could have subconsciously influenced the interview process, and the interpretation of patient responses. However, we tried to mitigate these issues by having the same individual interview all participants, by using an interview guide to ensure cross‐cohort consistency, by using open‐ended questions, and by attempting to give patients every opportunity to express themselves.
CONCLUSIONS
From a patients' perspective, inpatient service handoffs are often opaque experiences that are highlighted by poor communication between physicians and patients. Although deficits in communication and transparency acted as barriers to a patient‐centered handoff, physicians should recognize that service handoffs may also represent opportunities for improvement, and should focus on these domains when they start on a new service.
Disclosures
All funding for this project was provided by the Section of Hospital Medicine at The University of Chicago Medical Center. The data from this article were presented at the Society of Hospital Medicine Annual Conference, National Harbor, March 31, 2015, and at the Society of General Internal Medicine National Meeting in Toronto, Canada, April 23, 2015. The authors report that no conflicts of interest, financial or otherwise, exist.
- Continuity of outpatient and inpatient care by primary care physicians for hospitalized older adults. JAMA. 2009;301(16):1671–1680. , , , , , .
- Continuity of care and the risk of preventable hospitalization in older adults. JAMA Intern Med. 2013;173(20):1879–1885. , , , et al.
- Agency for Healthcare Research and Quality. HCUPnet: a tool for identifying, tracking, and analyzing national hospital statistics. Available at: http://hcupnet.ahrq.gov/HCUPnet.jsp?Id=82B37DA366A36BAD6(8):438–444.
- Hospitalist handoffs: a systematic review and task force recommendations. J Hosp Med. 2009;4(7):433–440. , , , , , .
- The impact of fragmentation of hospitalist care on length of stay. J Hosp Med. 2010;5(6):335–338. , , , , .
- The impact of hospitalist discontinuity on hospital cost, readmissions, and patient satisfaction. J Gen Intern Med. 2014;29(7):1004–1008. , , , et al.
- The effect of hospitalist discontinuity on adverse events. J Hosp Med. 2015;10(3):147–151. , , , et al.
- Agency for Healthcare Research and Quality. HCAHPS Fact Sheet. CAHPS Hospital Survey August 2013. Available at: http://www.hcahpsonline.org/files/August_2013_HCAHPS_Fact_Sheet3.pdf. Accessed February 2, 2015.
- A conceptual framework for studying the safety of transitions in emergency care. In: Henriksen K, Battles JB, Marks ES, eds. Advances in Patient Safety: From Research to Implementation. Rockville, MD: Agency for Healthcare Research and Quality; 2005:309–321. Concepts and Methodology; vol 2. Available at: http://www.ncbi.nlm.nih.gov/books/NBK20522. Accessed January 15, 2015. , , , et al.
- Transitions of care consensus policy statement American College of Physicians‐Society of General Internal Medicine‐Society of Hospital Medicine‐American Geriatrics Society‐American College of Emergency Physicians‐Society of Academic Emergency Medicine. J Gen Intern Med. 2009;24(8):971–976. , , , et al.
- Grounded theory in medical education research: AMEE guide no. 70. Med Teach. 2012;34(10):850–861. , .
- A purposeful approach to the constant comparative method in the analysis of qualitative interviews. Qual Quant. 2002;36(4):391–409. .
- The significance of saturation. Qual Health Res. 1995;5(2):147–149. .
- Standards for reporting qualitative research: a synthesis of recommendations. Acad Med. 2014;89(9):1245–1251. , , , , .
- Consolidated criteria for reporting qualitative research (COREQ): a 32‐item checklist for interviews and focus groups. Int J Qual Health Care. 2007;19(6):349–357. , , .
- Promoting effective transitions of care at hospital discharge: a review of key issues for hospitalists. J Hosp Med. 2007;2(5):314–323. , , , .
- The Joint Commission. Hot Topics in Healthcare, Issue 2. Transitions of care: the need for collaboration across entire care continuum. Available at: http://www.jointcommission.org/assets/1/6/TOC_Hot_Topics.pdf. Accessed April 9, 2015.
- Physician communication and patient adherence to treatment: a meta‐analysis. Med Care. 2009;47(8):826–834. , .
- Impediments to adherence to post myocardial infarction medications. Curr Cardiol Rep. 2013;15(1):322. , .
- Medical specialists' patient‐centered communication and patient‐reported outcomes. Med Care. 2007;45(4):330–339. , , , , .
- Does doctor‐patient communication affect patient satisfaction with hospital care? Results of an analysis with a novel instrumental variable. Health Serv Res. 2008;43(5 pt 1):1505–1519. , , , .
- Patient‐centredness in chronic illness: what is it and does it matter? Patient Educ Couns. 2003;51(3):197–206. , , .
- Merging cultures: palliative care specialists in the medical intensive care unit. Crit Care Med. 2006;34(11 suppl):S388–S393. , , , et al.
- Improving inpatients' identification of their doctors: use of FACE cards. Jt Comm J Qual Patient Saf. 2009;35(12):613–619. , , , et al.
- Interprofessional collaboration: effects of practice‐based interventions on professional practice and healthcare outcomes. Cochrane Database Syst Rev. 2009;(3):CD000072. , , .
- Identifying and overcoming the barriers to bedside rounds: a multicenter qualitative study. Acad Med. 2014;89(2):326–334. , , , et al.
- The effect of bedside case presentations on patients' perceptions of their medical care. N Engl J Med. 1997;336(16):1150–1155. , , , , .
- Patient‐centered interprofessional collaborative care: factors associated with bedside interprofessional rounds. J Gen Intern Med. 2014;29(7):1040–1047. , , , .
- Attending rounds in the current era: what is and is not happening. JAMA Intern Med. 2013;173(12):1084–1089. , , , et al.
- What the evidence shows about patient activation: better health outcomes and care experiences; fewer data on costs. Health Aff (Millwood). 2013;32(2):207–214. , .
- When patient activation levels change, health outcomes and costs change, too. Health Aff Proj Hope. 2015;34(3):431–437. , , , , .
- Evidence‐based guidelines for teaching patient‐centered interviewing. Patient Educ Couns. 2000;39(1):27–36. , , , et al.
- Meta‐analysis of correlates of provider behavior in medical encounters. Med Care. 1988;26(7):657–675. , , .
- Characteristics of potential plaintiffs in malpractice litigation. Ann Intern Med. 1994;120(9):792–798. , .
- Bedside shift reports: what does the evidence say? J Nurs Adm. 2014;44(10):541–545. , , , , .
- Continuity of outpatient and inpatient care by primary care physicians for hospitalized older adults. JAMA. 2009;301(16):1671–1680. , , , , , .
- Continuity of care and the risk of preventable hospitalization in older adults. JAMA Intern Med. 2013;173(20):1879–1885. , , , et al.
- Agency for Healthcare Research and Quality. HCUPnet: a tool for identifying, tracking, and analyzing national hospital statistics. Available at: http://hcupnet.ahrq.gov/HCUPnet.jsp?Id=82B37DA366A36BAD6(8):438–444.
- Hospitalist handoffs: a systematic review and task force recommendations. J Hosp Med. 2009;4(7):433–440. , , , , , .
- The impact of fragmentation of hospitalist care on length of stay. J Hosp Med. 2010;5(6):335–338. , , , , .
- The impact of hospitalist discontinuity on hospital cost, readmissions, and patient satisfaction. J Gen Intern Med. 2014;29(7):1004–1008. , , , et al.
- The effect of hospitalist discontinuity on adverse events. J Hosp Med. 2015;10(3):147–151. , , , et al.
- Agency for Healthcare Research and Quality. HCAHPS Fact Sheet. CAHPS Hospital Survey August 2013. Available at: http://www.hcahpsonline.org/files/August_2013_HCAHPS_Fact_Sheet3.pdf. Accessed February 2, 2015.
- A conceptual framework for studying the safety of transitions in emergency care. In: Henriksen K, Battles JB, Marks ES, eds. Advances in Patient Safety: From Research to Implementation. Rockville, MD: Agency for Healthcare Research and Quality; 2005:309–321. Concepts and Methodology; vol 2. Available at: http://www.ncbi.nlm.nih.gov/books/NBK20522. Accessed January 15, 2015. , , , et al.
- Transitions of care consensus policy statement American College of Physicians‐Society of General Internal Medicine‐Society of Hospital Medicine‐American Geriatrics Society‐American College of Emergency Physicians‐Society of Academic Emergency Medicine. J Gen Intern Med. 2009;24(8):971–976. , , , et al.
- Grounded theory in medical education research: AMEE guide no. 70. Med Teach. 2012;34(10):850–861. , .
- A purposeful approach to the constant comparative method in the analysis of qualitative interviews. Qual Quant. 2002;36(4):391–409. .
- The significance of saturation. Qual Health Res. 1995;5(2):147–149. .
- Standards for reporting qualitative research: a synthesis of recommendations. Acad Med. 2014;89(9):1245–1251. , , , , .
- Consolidated criteria for reporting qualitative research (COREQ): a 32‐item checklist for interviews and focus groups. Int J Qual Health Care. 2007;19(6):349–357. , , .
- Promoting effective transitions of care at hospital discharge: a review of key issues for hospitalists. J Hosp Med. 2007;2(5):314–323. , , , .
- The Joint Commission. Hot Topics in Healthcare, Issue 2. Transitions of care: the need for collaboration across entire care continuum. Available at: http://www.jointcommission.org/assets/1/6/TOC_Hot_Topics.pdf. Accessed April 9, 2015.
- Physician communication and patient adherence to treatment: a meta‐analysis. Med Care. 2009;47(8):826–834. , .
- Impediments to adherence to post myocardial infarction medications. Curr Cardiol Rep. 2013;15(1):322. , .
- Medical specialists' patient‐centered communication and patient‐reported outcomes. Med Care. 2007;45(4):330–339. , , , , .
- Does doctor‐patient communication affect patient satisfaction with hospital care? Results of an analysis with a novel instrumental variable. Health Serv Res. 2008;43(5 pt 1):1505–1519. , , , .
- Patient‐centredness in chronic illness: what is it and does it matter? Patient Educ Couns. 2003;51(3):197–206. , , .
- Merging cultures: palliative care specialists in the medical intensive care unit. Crit Care Med. 2006;34(11 suppl):S388–S393. , , , et al.
- Improving inpatients' identification of their doctors: use of FACE cards. Jt Comm J Qual Patient Saf. 2009;35(12):613–619. , , , et al.
- Interprofessional collaboration: effects of practice‐based interventions on professional practice and healthcare outcomes. Cochrane Database Syst Rev. 2009;(3):CD000072. , , .
- Identifying and overcoming the barriers to bedside rounds: a multicenter qualitative study. Acad Med. 2014;89(2):326–334. , , , et al.
- The effect of bedside case presentations on patients' perceptions of their medical care. N Engl J Med. 1997;336(16):1150–1155. , , , , .
- Patient‐centered interprofessional collaborative care: factors associated with bedside interprofessional rounds. J Gen Intern Med. 2014;29(7):1040–1047. , , , .
- Attending rounds in the current era: what is and is not happening. JAMA Intern Med. 2013;173(12):1084–1089. , , , et al.
- What the evidence shows about patient activation: better health outcomes and care experiences; fewer data on costs. Health Aff (Millwood). 2013;32(2):207–214. , .
- When patient activation levels change, health outcomes and costs change, too. Health Aff Proj Hope. 2015;34(3):431–437. , , , , .
- Evidence‐based guidelines for teaching patient‐centered interviewing. Patient Educ Couns. 2000;39(1):27–36. , , , et al.
- Meta‐analysis of correlates of provider behavior in medical encounters. Med Care. 1988;26(7):657–675. , , .
- Characteristics of potential plaintiffs in malpractice litigation. Ann Intern Med. 1994;120(9):792–798. , .
- Bedside shift reports: what does the evidence say? J Nurs Adm. 2014;44(10):541–545. , , , , .
Measuring Patient Experiences
The hospitalized patient experience has become an area of increased focus for hospitals given the recent coupling of patient satisfaction to reimbursement rates for Medicare patients.[1] Although patient experiences are multifactorial, 1 component is the relationship that hospitalized patients develop with their inpatient physicians. In recognition of the importance of this relationship, several organizations including the Society of Hospital Medicine, Society of General Internal Medicine, American College of Physicians, the American College of Emergency Physicians, and the Accreditation Council for Graduate Medical Education have recommended that patients know and understand who is guiding their care at all times during their hospitalization.[2, 3] Unfortunately, previous studies have shown that hospitalized patients often lack the ability to identify[4, 5] and understand their course of care.[6, 7] This may be due to numerous clinical factors including lack of a prior relationship, rapid pace of clinical care, and the frequent transitions of care found in both hospitalists and general medicine teaching services.[5, 8, 9] Regardless of the cause, one could hypothesize that patients who are unable to identify or understand the role of their physician may be less informed about their hospitalization, which may lead to further confusion, dissatisfaction, and ultimately a poor experience.
Given the proliferation of nonteaching hospitalist services in teaching hospitals, it is important to understand if patient experiences differ between general medicine teaching and hospitalist services. Several reasons could explain why patient experiences may vary on these services. For example, patients on a hospitalist service will likely interact with a single physician caretaker, which may give a feeling of more personalized care. In contrast, patients on general medicine teaching services are cared for by larger teams of residents under the supervision of an attending physician. Residents are also subjected to duty‐hour restrictions, clinic responsibilities, and other educational requirements that may impede the continuity of care for hospitalized patients.[10, 11, 12] Although 1 study has shown that hospitalist‐intensive hospitals perform better on patient satisfaction measures,[13] no study to date has compared patient‐reported experiences on general medicine teaching and nonteaching hospitalist services. This study aimed to evaluate the hospitalized patient experience on both teaching and nonteaching hospitalist services by assessing several patient‐reported measures of their experience, namely their confidence in their ability to identify their physician(s), understand their roles, and their rating of both the coordination and overall care.
METHODS
Study Design
We performed a retrospective cohort analysis at the University of Chicago Medical Center between July 2007 and June 2013. Data were acquired as part of the Hospitalist Project, an ongoing study that is used to evaluate the impact of hospitalists, and now serves as infrastructure to continue research related to hospital care at University of Chicago.[14] Patients were cared for by either the general medicine teaching service or the nonteaching hospitalist service. General medicine teaching services were composed of an attending physician who rotates for 2 weeks at a time, a second‐ or third‐year medicine resident, 1 to 2 medicine interns, and 1 to 2 medical students.[15] The attending physician assigned to the patient's hospitalization was the attending listed on the first day of hospitalization, regardless of the length of hospitalization. Nonteaching hospitalist services consisted of a single hospitalist who worked 7‐day shifts, and were assisted by a nurse practitioner/physician's assistant (NPA). The majority of attendings on the hospitalist service were less than 5 years out of residency. Both services admitted 7 days a week, with patients initially admitted to the general medicine teaching service until resident caps were met, after which all subsequent admissions were admitted to the hospitalist service. In addition, the hospitalist service is also responsible for specific patient subpopulations, such as lung and renal transplants, and oncologic patients who have previously established care with our institution.
Data Collection
During a 30‐day posthospitalization follow‐up questionnaire, patients were surveyed regarding their confidence in their ability to identify and understand the roles of their physician(s) and their perceptions of the overall coordination of care and their overall care, using a 5‐point Likert scale (1 = poor understanding to 5 = excellent understanding). Questions related to satisfaction with care and coordination were derived from the Picker‐Commonwealth Survey, a previously validated survey meant to evaluate patient‐centered care.[16] Patients were also asked to report their race, level of education, comorbid diseases, and whether they had any prior hospitalizations within 1 year. Chart review was performed to obtain patient age, gender, and hospital length of stay (LOS), and calculated Charlson Comorbidity Index (CCI).[17] Patients with missing data or responses to survey questions were excluded from final analysis. The University of Chicago Institutional Review Board approved the study protocol, and all patients provided written consented prior to participation.
Data Analysis
After initial analysis noted that outcomes were skewed, the decision was made to dichotomize the data and use logistic rather than linear regression models. Patient responses to the follow‐up phone questionnaire were dichotomized to reflect the top 2 categories (excellent and very good). Pearson 2 analysis was used to assess for any differences in demographic characteristics, disease severity, and measures of patient experience between the 2 services. To assess if service type was associated with differences in our 4 measures of patient experience, we created a 3‐level mixed‐effects logistic regression using a logit function while controlling for age, gender, race, CCI, LOS, previous hospitalizations within 1 year, level of education, and academic year. These models studied the longitudinal association between teaching service and the 4 outcome measures, while also controlling for the cluster effect of time nested within individual patients who were clustered within physicians. The model included random intercepts at both the patient and physician level and also included a random effect of service (teaching vs nonteaching) at the patient level. A Hausman test was used to determine if these random‐effects models improved fit over a fixed‐effects model, and the intraclass correlations were compared using likelihood ratio tests to determine the appropriateness of a 3‐level versus 2‐level model. Data management and 2 analyses were performed using Stata version 13.0 (StataCorp, College Station, TX), and mixed‐effects regression models were done in SuperMix (Scientific Software International, Skokie, IL).
RESULTS
In total, 14,855 patients were enrolled during their hospitalization with 57% and 61% completing the 30‐day follow‐up survey on the hospitalist and general medicine teaching service, respectively. In total, 4131 (69%) and 4322 (48%) of the hospitalist and general medicine services, respectively, either did not answer all survey questions, or were missing basic demographic data, and thus were excluded. Data from 4591 patients on the general medicine teaching (52% of those enrolled at hospitalization) and 1811 on the hospitalist service (31% of those enrolled at hospitalization) were used for final analysis (Figure 1). Respondents were predominantly female (61% and 56%), African American (75% and 63%), with a mean age of 56.2 (19.4) and 57.1 (16.1) years, for the general medicine teaching and hospitalist services, respectively. A majority of patients (71% and 66%) had a CCI of 0 to 3 on both services. There were differences in self‐reported comorbidities between the 2 groups, with hospitalist services having a higher prevalence of cancer (20% vs 7%), renal disease (25% vs 18%), and liver disease (23% vs 7%). Patients on the hospitalist service had a longer mean LOS (5.5 vs 4.8 days), a greater percentage of a hospitalization within 1 year (58% vs 52%), and a larger proportion who were admitted in 2011 to 2013 compared to 2007 to 2010 (75% vs 39%), when compared to the general medicine teaching services. Median LOS and interquartile ranges were similar between both groups. Although most baseline demographics were statistically different between the 2 groups (Table 1), these differences were likely clinically insignificant. Compared to those who responded to the follow‐up survey, nonresponders were more likely to be African American (73% and 64%, P < 0.001) and female (60% and 56%, P < 0.01). The nonresponders were more likely to be hospitalized in the past 1 year (62% and 53%, P < 0.001) and have a lower CCI (CCI 03 [75% and 80%, P < 0.001]) compared to responders. Demographics between responders and nonresponders were also statistically different from one another.
Variable | General Medicine Teaching | Nonteaching Hospitalist | P Value |
---|---|---|---|
| |||
Total (n) | 4,591 | 1,811 | <0.001 |
Attending classification, hospitalist, n (%) | 1,147 (25) | 1,811 (100) | |
Response rate, % | 61 | 57 | <0.01 |
Age, y, mean SD | 56.2 19.4 | 57.1 16.1 | <0.01 |
Gender, n (%) | <0.01 | ||
Male | 1,796 (39) | 805 (44) | |
Female | 2,795 (61) | 1,004 (56) | |
Race, n (%) | <0.01 | ||
African American | 3,440 (75) | 1,092 (63) | |
White | 900 (20) | 571 (32) | |
Asian/Pacific | 38 (1) | 17 (1) | |
Other | 20 (1) | 10 (1) | |
Unknown | 134 (3) | 52 (3) | |
Charlson Comorbidity Index, n (%) | <0.001 | ||
0 | 1,635 (36) | 532 (29) | |
12 | 1,590 (35) | 675 (37) | |
39 | 1,366 (30) | 602 (33) | |
Self‐reported comorbidities | |||
Anemia/sickle cell disease | 1,201 (26) | 408 (23) | 0.003 |
Asthma/COPD | 1,251 (28) | 432 (24) | 0.006 |
Cancer* | 300 (7) | 371 (20) | <0.001 |
Depression | 1,035 (23) | 411 (23) | 0.887 |
Diabetes | 1,381 (30) | 584 (32) | 0.087 |
Gastrointestinal | 1,140 (25) | 485 (27) | 0.104 |
Cardiac | 1,336 (29) | 520 (29) | 0.770 |
Hypertension | 2,566 (56) | 1,042 (58) | 0.222 |
HIV/AIDS | 151 (3) | 40 (2) | 0.022 |
Kidney disease | 828 (18) | 459 (25) | <0.001 |
Liver disease | 313 (7) | 417 (23) | <0.001 |
Stroke | 543 (12) | 201 (11) | 0.417 |
Education level | 0.066 | ||
High school | 2,248 (49) | 832 (46) | |
Junior college/college | 1,878 (41) | 781 (43) | |
Postgraduate | 388 (8) | 173 (10) | |
Don't know | 77 (2) | 23 (1) | |
Academic year, n (%) | <0.001 | ||
July 2007 June 2008 | 938 (20) | 90 (5) | |
July 2008 June 2009 | 702 (15) | 148 (8) | |
July 2009 June 2010 | 576(13) | 85 (5) | |
July 2010 June 2011 | 602 (13) | 138 (8) | |
July 2011 June 2012 | 769 (17) | 574 (32) | |
July 2012 June 2013 | 1,004 (22) | 774 (43) | |
Length of stay, d, mean SD | 4.8 7.3 | 5.5 6.4 | <0.01 |
Prior hospitalization (within 1 year), yes, n (%) | 2,379 (52) | 1,039 (58) | <0.01 |
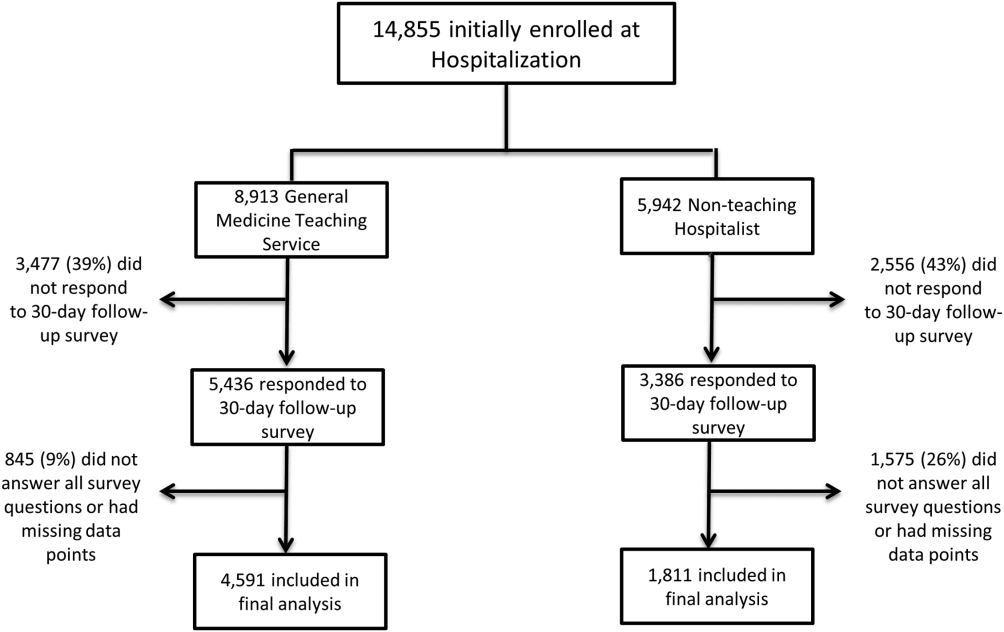
Unadjusted results revealed that patients on the hospitalist service were more confident in their abilities to identify their physician(s) (50% vs 45%, P < 0.001), perceived greater ability in understanding the role of their physician(s) (54% vs 50%, P < 0.001), and reported greater satisfaction with coordination and teamwork (68% vs 64%, P = 0.006) and with overall care (73% vs 67%, P < 0.001) (Figure 2).
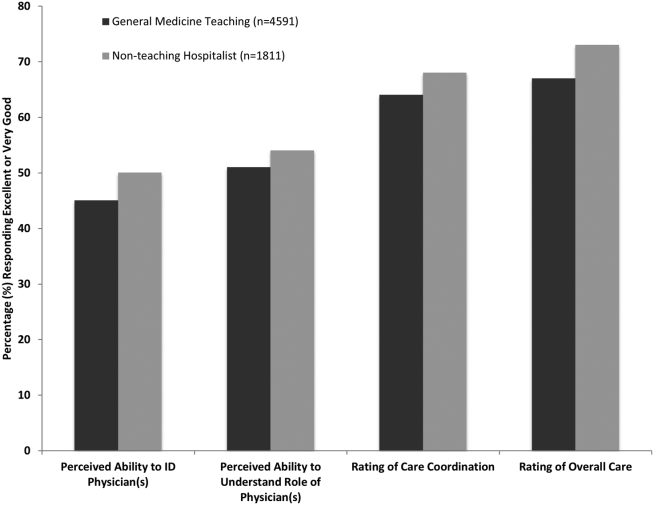
From the mixed‐effects regression models it was discovered that admission to the hospitalist service was associated with a higher odds ratio (OR) of reporting overall care as excellent or very good (OR: 1.33; 95% confidence interval [CI]: 1.15‐1.47). There was no difference between services in patients' ability to identify their physician(s) (OR: 0.89; 95% CI: 0.61‐1.11), in patients reporting a better understanding of the role of their physician(s) (OR: 1.09; 95% CI: 0.94‐1.23), or in their rating of overall coordination and teamwork (OR: 0.71; 95% CI: 0.42‐1.89).
A subgroup analysis was performed on the 25% of hospitalist attendings in the general medicine teaching service comparing this cohort to the hospitalist services, and it was found that patients perceived better overall care on the hospitalist service (OR: 1.17; 95% CI: 1.01‐ 1.31) than on the general medicine service (Table 2). All other domains in the subgroup analysis were not statistically significant. Finally, an ordinal logistic regression was performed for each of these outcomes, but it did not show any major differences compared to the logistic regression of dichotomous outcomes.
Domains in Patient Experience* | Odds Ratio (95% CI) | P Value |
---|---|---|
| ||
How would you rate your ability to identify the physicians and trainees on your general medicine team during the hospitalization? | ||
Model 1 | 0.89 (0.611.11) | 0.32 |
Model 2 | 0.98 (0.671.22) | 0.86 |
How would you rate your understanding of the roles of the physicians and trainees on your general medicine team? | ||
Model 1 | 1.09 (0.941.23) | 0.25 |
Model 2 | 1.19 (0.981.36) | 0.08 |
How would you rate the overall coordination and teamwork among the doctors and nurses who care for you during your hospital stay? | ||
Model 1 | 0.71 (0.421.89) | 0.18 |
Model 2 | 0.82 (0.651.20) | 0.23 |
Overall, how would you rate the care you received at the hospital? | ||
Model 1 | 1.33 (1.151.47) | 0.001 |
Model 2 | 1.17 (1.011.31) | 0.04 |
DISCUSSION
This study is the first to directly compare measures of patient experience on hospitalist and general medicine teaching services in a large, multiyear comparison across multiple domains. In adjusted analysis, we found that patients on nonteaching hospitalist services rated their overall care better than those on general medicine teaching services, whereas no differences in patients' ability to identify their physician(s), understand their role in their care, or rating of coordination of care were found. Although the magnitude of the differences in rating of overall care may appear small, it remains noteworthy because of the recent focus on patient experience at the reimbursement level, where small differences in performance can lead to large changes in payment. Because of the observational design of this study, it is important to consider mechanisms that could account for our findings.
The first are the structural differences between the 2 services. Our subgroup analysis comparing patients rating of overall care on a general medicine service with a hospitalist attending to a pure hospitalist cohort found a significant difference between the groups, indicating that the structural differences between the 2 groups may be a significant contributor to patient satisfaction ratings. Under the care of a hospitalist service, a patient would only interact with a single physician on a daily basis, possibly leading to a more meaningful relationship and improved communication between patient and provider. Alternatively, while on a general medicine teaching service, patients would likely interact with multiple physicians, as a result making their confidence in their ability to identify and perception at understanding physicians' roles more challenging.[18] This dilemma is further compounded by duty hour restrictions, which have subsequently led to increased fragmentation in housestaff scheduling. The patient experience on the general medicine teaching service may be further complicated by recent data that show residents spend a minority of time in direct patient care,[19, 20] which could additionally contribute to patients' inability to understand who their physicians are and to the decreased satisfaction with their care. This combination of structural complexity, duty hour reform, and reduced direct patient interaction would likely decrease the chance a patient will interact with the same resident on a consistent basis,[5, 21] thus making the ability to truly understand who their caretakers are, and the role they play, more difficult.
Another contributing factor could be the use of NPAs on our hospitalist service. Given that these providers often see the patient on a more continual basis, hospitalized patients' exposure to a single, continuous caretaker may be a factor in our findings.[22] Furthermore, with studies showing that hospitalists also spend a small fraction of their day in direct patient care,[23, 24, 25] the use of NPAs may allow our hospitalists to spend greater amounts of time with their patients, thus improving patients' rating of their overall care and influencing their perceived ability to understand their physician's role.
Although there was no difference between general medicine teaching and hospitalist services with respect to patient understanding of their roles, our data suggest that both groups would benefit from interventions to target this area. Focused attempts at improving patient's ability to identify and explain the roles of their inpatient physician(s) have been performed. For example, previous studies have attempted to improve a patient's ability to identify their physician through physician facecards[8, 9] or the use of other simple interventions (ie, bedside whiteboards).[4, 26] Results from such interventions are mixed, as they have demonstrated the capacity to improve patients' ability to identify who their physician is, whereas few have shown any appreciable improvement in patient satisfaction.[26]
Although our findings suggest that structural differences in team composition may be a possible explanation, it is also important to consider how the quality of care a patient receives affects their experience. For instance, hospitalists have been shown to produce moderate improvements in patient‐centered outcomes such as 30‐day readmission[27] and hospital length of stay[14, 28, 29, 30, 31] when compared to other care providers, which in turn could be reflected in the patient's perception of their overall care. In a large national study of acute care hospitals using the Hospital Consumer Assessment of Healthcare Providers and Systems survey, Chen and colleagues found that for most measures of patient satisfaction, hospitals with greater use of hospitalist care were associated with better patient‐centered care.[13] These outcomes were in part driven by patient‐centered domains such as discharge planning, pain control, and medication management. It is possible that patients are sensitive to the improved outcomes that are associated with hospitalist services, and reflect this in their measures of patient satisfaction.
Last, because this is an observational study and not a randomized trial, it is possible that the clinical differences in the patients cared for by these services could have led to our findings. Although the clinical significance of the differences in patient demographics were small, patients seen on the hospitalist service were more likely to be older white males, with a slightly longer LOS, greater comorbidities, and more hospitalizations in the previous year than those seen on the general medicine teaching service. Additionally, our hospitalist service frequently cares for highly specific subpopulations (ie, liver and renal transplant patients, and oncology patients), which could have influenced our results. For example, transplant patients who may be very grateful for their second chance, are preferentially admitted to the hospitalist service, which could have biased our results in favor of hospitalists.[32] Unfortunately, we were unable to control for all such factors.
Although we hope that multivariable analysis can adjust for many of these differences, we are not able to account for possible unmeasured confounders such as time of day of admission, health literacy, personality differences, physician turnover, or nursing and other ancillary care that could contribute to these findings. In addition to its observational study design, our study has several other limitations. First, our study was performed at a single institution, thus limiting its generalizability. Second, as a retrospective study based on observational data, no definitive conclusions regarding causality can be made. Third, although our response rate was low, it is comparable to other studies that have examined underserved populations.[33, 34] Fourth, because our survey was performed 30 days after hospitalization, this may impart imprecision on our outcomes measures. Finally, we were not able to mitigate selection bias through imputation for missing data .
All together, given the small absolute differences between the groups in patients' ratings of their overall care compared to large differences in possible confounders, these findings call for further exploration into the significance and possible mechanisms of these outcomes. Our study raises the potential possibility that the structural component of a care team may play a role in overall patient satisfaction. If this is the case, future studies of team structure could help inform how best to optimize this component for the patient experience. On the other hand, if process differences are to explain our findings, it is important to distill the types of processes hospitalists are using to improve the patient experience and potentially export this to resident services.
Finally, if similar results were found in other institutions, these findings could have implications on how hospitals respond to new payment models that are linked to patient‐experience measures. For example, the Hospital Value‐Based Purchasing Program currently links the Centers for Medicare and Medicaid Services payments to a set of quality measures that consist of (1) clinical processes of care (70%) and (2) the patient experience (30%).[1] Given this linkage, any small changes in the domain of patient satisfaction could have large payment implications on a national level.
CONCLUSION
In summary, in this large‐scale multiyear study, patients cared for by a nonteaching hospitalist service reported greater satisfaction with their overall care than patients cared for by a general medicine teaching service. This difference could be mediated by the structural differences between these 2 services. As hospitals seek to optimize patient experiences in an era where reimbursement models are now being linked to patient‐experience measures, future work should focus on further understanding the mechanisms for these findings.
Disclosures
Financial support for this work was provided by the Robert Wood Johnson Investigator Program (RWJF Grant ID 63910 PI Meltzer), a Midcareer Career Development Award from the National Institute of Aging (1 K24 AG031326‐01, PI Meltzer), and a Clinical and Translational Science Award (NIH/NCATS 2UL1TR000430‐08, PI Solway, Meltzer Core Leader). The authors report no conflicts of interest.
- Hospital Consumer Assessment of Healthcare Providers and Systems. HCAHPS fact sheet. CAHPS hospital survey August 2013. Available at: http://www.hcahpsonline.org/files/August_2013_HCAHPS_Fact_Sheet3.pdf. Accessed February 2, 2015.
- Transitions of Care Consensus policy statement: American College of Physicians, Society of General Internal Medicine, Society of Hospital Medicine, American Geriatrics Society, American College Of Emergency Physicians, and Society for Academic Emergency Medicine. J Hosp Med. 2009;4(6):364–370. , , , et al.
- Accreditation Council for Graduate Medical Education. Common program requirements. Available at: http://www.acgme.org/acgmeweb/Portals/0/PFAssets/ProgramRequirements/CPRs2013.pdf. Accessed January 15, 2015.
- Increasing a patient's ability to identify his or her attending physician using a patient room display. Arch Intern Med. 2010;170(12):1084–1085. , , .
- Ability of hospitalized patients to identify their in‐hospital physicians. Arch Intern Med. 2009;169(2):199–201. , , , , , .
- Hospitalized patients' understanding of their plan of care. Mayo Clin Proc. 2010;85(1):47–52. , , , et al.
- Patient‐physician communication at hospital discharge and patients' understanding of the postdischarge treatment plan. Arch Intern Med. 1997;157(9):1026–1030. , , , et al.
- Improving inpatients' identification of their doctors: use of FACE cards. Jt Comm J Qual Patient Saf. 2009;35(12):613–619. , , , et al.
- The impact of facecards on patients' knowledge, satisfaction, trust, and agreement with hospital physicians: a pilot study. J Hosp Med. 2014;9(3):137–141. , , , , , .
- Restructuring an inpatient resident service to improve outcomes for residents, students, and patients. Acad Med. 2011;86(12):1500–1507. , , .
- Residency training in the modern era: the pipe dream of less time to learn more, care better, and be more professional. Arch Intern Med. 2005;165(22):2561–2562. , , .
- Managing discontinuity in academic medical centers: strategies for a safe and effective resident sign‐out. J Hosp Med. 2006;1(4):257–266. , , , , .
- Hospitalist staffing and patient satisfaction in the national Medicare population. J Hosp Med. 2013;8(3):126–131. , , , .
- Effects of physician experience on costs and outcomes on an academic general medicine service: results of a trial of hospitalists. Ann Intern Med. 2002;137(11):866–874. , , , et al.
- The Effects of on‐duty napping on intern sleep time and fatigue. Ann Intern Med. 2006;144(11):792–798. , , , , , .
- Patients evaluate their hospital care: a national survey. Health Aff (Millwood). 1991;10(4):254–267. , , , et al.
- A new method of classifying prognostic comorbidity in longitudinal studies: development and validation. J Chronic Dis. 1987;40(5):373–383. , , , .
- Agency for Healthcare Research and Quality. Welcome to HCUPnet. Available at: http://hcupnet.ahrq.gov/HCUPnet.jsp?Id=F70FC59C286BADCB371(4):293–295.
- In the wake of the 2003 and 2011 duty hours regulations, how do internal medicine interns spend their time? J Gen Intern Med. 2013;28(8):1042–1047. , , , et al.
- The composition of intern work while on call. J Gen Intern Med. 2012;27(11):1432–1437. , , , , , .
- Effect of the 2011 vs 2003 duty hour regulation‐compliant models on sleep duration, trainee education, and continuity of patient care among internal medicine house staff: a randomized trial. JAMA Intern Med. 2013;173(8):649–655. , , , et al.
- The impact of hospitalist discontinuity on hospital cost, readmissions, and patient satisfaction. J Gen Intern Med. 2014;29(7):1004–1008. , , , et al.
- Hospitalist time usage and cyclicality: opportunities to improve efficiency. J Hosp Med. 2010;5(6):329–334. , , , , .
- Where did the day go?—a time‐motion study of hospitalists. J Hosp Med. 2010;5(6):323–328. , , , et al.
- How hospitalists spend their time: insights on efficiency and safety. J Hosp Med. 2006;1(2):88–93. , , .
- Patient satisfaction associated with correct identification of physician's photographs. Mayo Clin Proc. 2001;76(6):604–608. , , .
- Comparing patient outcomes of academician‐preceptors, hospitalist‐preceptors, and hospitalists on internal medicine services in an academic medical center. J Gen Intern Med. 2014;29(12):1672–1678. , , , .
- Comparison of processes and outcomes of pneumonia care between hospitalists and community‐based primary care physicians. Mayo Clin Proc. 2002;77(10):1053–1058. , , , .
- Outcomes of care by hospitalists, general internists, and family physicians. N Engl J Med. 2007;357(25):2589–2600. , , , , , .
- A systematic review of outcomes and quality measures in adult patients cared for by hospitalists vs nonhospitalists. Mayo Clin Proc. 2009;84(3):248–254. .
- Do hospitalist physicians improve the quality of inpatient care delivery? A systematic review of process, efficiency and outcome measures. BMC Med. 2011;9(1):58. , .
- Patients' experiences of everyday life after lung transplantation. J Clin Nurs. 2009;18(24):3472–3479. , .
- Optimal design features for surveying low‐income populations. J Health Care Poor Underserved. 2005;16(4):677–690. , , , et al.
The hospitalized patient experience has become an area of increased focus for hospitals given the recent coupling of patient satisfaction to reimbursement rates for Medicare patients.[1] Although patient experiences are multifactorial, 1 component is the relationship that hospitalized patients develop with their inpatient physicians. In recognition of the importance of this relationship, several organizations including the Society of Hospital Medicine, Society of General Internal Medicine, American College of Physicians, the American College of Emergency Physicians, and the Accreditation Council for Graduate Medical Education have recommended that patients know and understand who is guiding their care at all times during their hospitalization.[2, 3] Unfortunately, previous studies have shown that hospitalized patients often lack the ability to identify[4, 5] and understand their course of care.[6, 7] This may be due to numerous clinical factors including lack of a prior relationship, rapid pace of clinical care, and the frequent transitions of care found in both hospitalists and general medicine teaching services.[5, 8, 9] Regardless of the cause, one could hypothesize that patients who are unable to identify or understand the role of their physician may be less informed about their hospitalization, which may lead to further confusion, dissatisfaction, and ultimately a poor experience.
Given the proliferation of nonteaching hospitalist services in teaching hospitals, it is important to understand if patient experiences differ between general medicine teaching and hospitalist services. Several reasons could explain why patient experiences may vary on these services. For example, patients on a hospitalist service will likely interact with a single physician caretaker, which may give a feeling of more personalized care. In contrast, patients on general medicine teaching services are cared for by larger teams of residents under the supervision of an attending physician. Residents are also subjected to duty‐hour restrictions, clinic responsibilities, and other educational requirements that may impede the continuity of care for hospitalized patients.[10, 11, 12] Although 1 study has shown that hospitalist‐intensive hospitals perform better on patient satisfaction measures,[13] no study to date has compared patient‐reported experiences on general medicine teaching and nonteaching hospitalist services. This study aimed to evaluate the hospitalized patient experience on both teaching and nonteaching hospitalist services by assessing several patient‐reported measures of their experience, namely their confidence in their ability to identify their physician(s), understand their roles, and their rating of both the coordination and overall care.
METHODS
Study Design
We performed a retrospective cohort analysis at the University of Chicago Medical Center between July 2007 and June 2013. Data were acquired as part of the Hospitalist Project, an ongoing study that is used to evaluate the impact of hospitalists, and now serves as infrastructure to continue research related to hospital care at University of Chicago.[14] Patients were cared for by either the general medicine teaching service or the nonteaching hospitalist service. General medicine teaching services were composed of an attending physician who rotates for 2 weeks at a time, a second‐ or third‐year medicine resident, 1 to 2 medicine interns, and 1 to 2 medical students.[15] The attending physician assigned to the patient's hospitalization was the attending listed on the first day of hospitalization, regardless of the length of hospitalization. Nonteaching hospitalist services consisted of a single hospitalist who worked 7‐day shifts, and were assisted by a nurse practitioner/physician's assistant (NPA). The majority of attendings on the hospitalist service were less than 5 years out of residency. Both services admitted 7 days a week, with patients initially admitted to the general medicine teaching service until resident caps were met, after which all subsequent admissions were admitted to the hospitalist service. In addition, the hospitalist service is also responsible for specific patient subpopulations, such as lung and renal transplants, and oncologic patients who have previously established care with our institution.
Data Collection
During a 30‐day posthospitalization follow‐up questionnaire, patients were surveyed regarding their confidence in their ability to identify and understand the roles of their physician(s) and their perceptions of the overall coordination of care and their overall care, using a 5‐point Likert scale (1 = poor understanding to 5 = excellent understanding). Questions related to satisfaction with care and coordination were derived from the Picker‐Commonwealth Survey, a previously validated survey meant to evaluate patient‐centered care.[16] Patients were also asked to report their race, level of education, comorbid diseases, and whether they had any prior hospitalizations within 1 year. Chart review was performed to obtain patient age, gender, and hospital length of stay (LOS), and calculated Charlson Comorbidity Index (CCI).[17] Patients with missing data or responses to survey questions were excluded from final analysis. The University of Chicago Institutional Review Board approved the study protocol, and all patients provided written consented prior to participation.
Data Analysis
After initial analysis noted that outcomes were skewed, the decision was made to dichotomize the data and use logistic rather than linear regression models. Patient responses to the follow‐up phone questionnaire were dichotomized to reflect the top 2 categories (excellent and very good). Pearson 2 analysis was used to assess for any differences in demographic characteristics, disease severity, and measures of patient experience between the 2 services. To assess if service type was associated with differences in our 4 measures of patient experience, we created a 3‐level mixed‐effects logistic regression using a logit function while controlling for age, gender, race, CCI, LOS, previous hospitalizations within 1 year, level of education, and academic year. These models studied the longitudinal association between teaching service and the 4 outcome measures, while also controlling for the cluster effect of time nested within individual patients who were clustered within physicians. The model included random intercepts at both the patient and physician level and also included a random effect of service (teaching vs nonteaching) at the patient level. A Hausman test was used to determine if these random‐effects models improved fit over a fixed‐effects model, and the intraclass correlations were compared using likelihood ratio tests to determine the appropriateness of a 3‐level versus 2‐level model. Data management and 2 analyses were performed using Stata version 13.0 (StataCorp, College Station, TX), and mixed‐effects regression models were done in SuperMix (Scientific Software International, Skokie, IL).
RESULTS
In total, 14,855 patients were enrolled during their hospitalization with 57% and 61% completing the 30‐day follow‐up survey on the hospitalist and general medicine teaching service, respectively. In total, 4131 (69%) and 4322 (48%) of the hospitalist and general medicine services, respectively, either did not answer all survey questions, or were missing basic demographic data, and thus were excluded. Data from 4591 patients on the general medicine teaching (52% of those enrolled at hospitalization) and 1811 on the hospitalist service (31% of those enrolled at hospitalization) were used for final analysis (Figure 1). Respondents were predominantly female (61% and 56%), African American (75% and 63%), with a mean age of 56.2 (19.4) and 57.1 (16.1) years, for the general medicine teaching and hospitalist services, respectively. A majority of patients (71% and 66%) had a CCI of 0 to 3 on both services. There were differences in self‐reported comorbidities between the 2 groups, with hospitalist services having a higher prevalence of cancer (20% vs 7%), renal disease (25% vs 18%), and liver disease (23% vs 7%). Patients on the hospitalist service had a longer mean LOS (5.5 vs 4.8 days), a greater percentage of a hospitalization within 1 year (58% vs 52%), and a larger proportion who were admitted in 2011 to 2013 compared to 2007 to 2010 (75% vs 39%), when compared to the general medicine teaching services. Median LOS and interquartile ranges were similar between both groups. Although most baseline demographics were statistically different between the 2 groups (Table 1), these differences were likely clinically insignificant. Compared to those who responded to the follow‐up survey, nonresponders were more likely to be African American (73% and 64%, P < 0.001) and female (60% and 56%, P < 0.01). The nonresponders were more likely to be hospitalized in the past 1 year (62% and 53%, P < 0.001) and have a lower CCI (CCI 03 [75% and 80%, P < 0.001]) compared to responders. Demographics between responders and nonresponders were also statistically different from one another.
Variable | General Medicine Teaching | Nonteaching Hospitalist | P Value |
---|---|---|---|
| |||
Total (n) | 4,591 | 1,811 | <0.001 |
Attending classification, hospitalist, n (%) | 1,147 (25) | 1,811 (100) | |
Response rate, % | 61 | 57 | <0.01 |
Age, y, mean SD | 56.2 19.4 | 57.1 16.1 | <0.01 |
Gender, n (%) | <0.01 | ||
Male | 1,796 (39) | 805 (44) | |
Female | 2,795 (61) | 1,004 (56) | |
Race, n (%) | <0.01 | ||
African American | 3,440 (75) | 1,092 (63) | |
White | 900 (20) | 571 (32) | |
Asian/Pacific | 38 (1) | 17 (1) | |
Other | 20 (1) | 10 (1) | |
Unknown | 134 (3) | 52 (3) | |
Charlson Comorbidity Index, n (%) | <0.001 | ||
0 | 1,635 (36) | 532 (29) | |
12 | 1,590 (35) | 675 (37) | |
39 | 1,366 (30) | 602 (33) | |
Self‐reported comorbidities | |||
Anemia/sickle cell disease | 1,201 (26) | 408 (23) | 0.003 |
Asthma/COPD | 1,251 (28) | 432 (24) | 0.006 |
Cancer* | 300 (7) | 371 (20) | <0.001 |
Depression | 1,035 (23) | 411 (23) | 0.887 |
Diabetes | 1,381 (30) | 584 (32) | 0.087 |
Gastrointestinal | 1,140 (25) | 485 (27) | 0.104 |
Cardiac | 1,336 (29) | 520 (29) | 0.770 |
Hypertension | 2,566 (56) | 1,042 (58) | 0.222 |
HIV/AIDS | 151 (3) | 40 (2) | 0.022 |
Kidney disease | 828 (18) | 459 (25) | <0.001 |
Liver disease | 313 (7) | 417 (23) | <0.001 |
Stroke | 543 (12) | 201 (11) | 0.417 |
Education level | 0.066 | ||
High school | 2,248 (49) | 832 (46) | |
Junior college/college | 1,878 (41) | 781 (43) | |
Postgraduate | 388 (8) | 173 (10) | |
Don't know | 77 (2) | 23 (1) | |
Academic year, n (%) | <0.001 | ||
July 2007 June 2008 | 938 (20) | 90 (5) | |
July 2008 June 2009 | 702 (15) | 148 (8) | |
July 2009 June 2010 | 576(13) | 85 (5) | |
July 2010 June 2011 | 602 (13) | 138 (8) | |
July 2011 June 2012 | 769 (17) | 574 (32) | |
July 2012 June 2013 | 1,004 (22) | 774 (43) | |
Length of stay, d, mean SD | 4.8 7.3 | 5.5 6.4 | <0.01 |
Prior hospitalization (within 1 year), yes, n (%) | 2,379 (52) | 1,039 (58) | <0.01 |
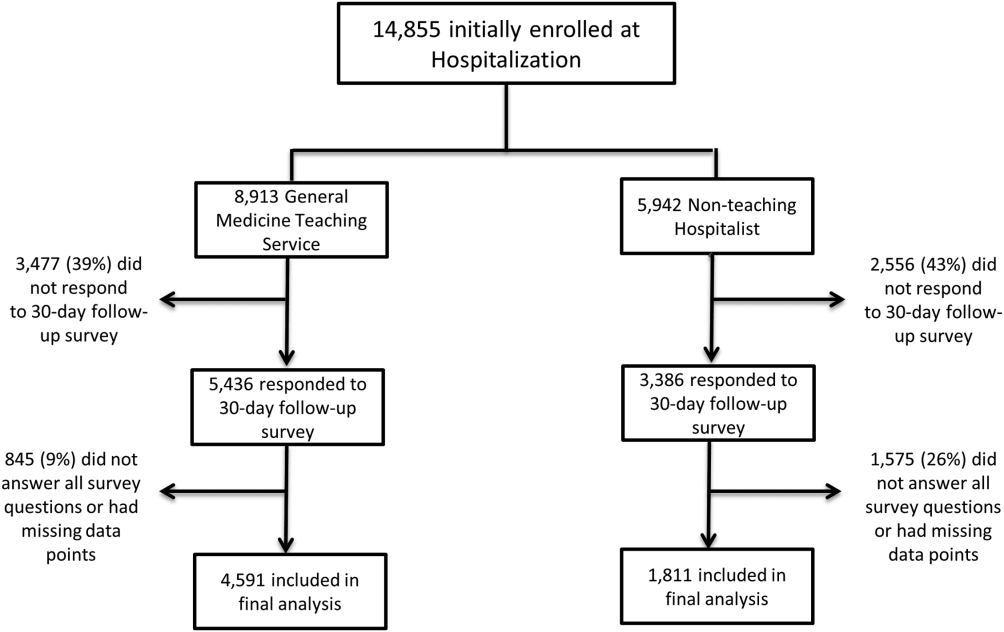
Unadjusted results revealed that patients on the hospitalist service were more confident in their abilities to identify their physician(s) (50% vs 45%, P < 0.001), perceived greater ability in understanding the role of their physician(s) (54% vs 50%, P < 0.001), and reported greater satisfaction with coordination and teamwork (68% vs 64%, P = 0.006) and with overall care (73% vs 67%, P < 0.001) (Figure 2).
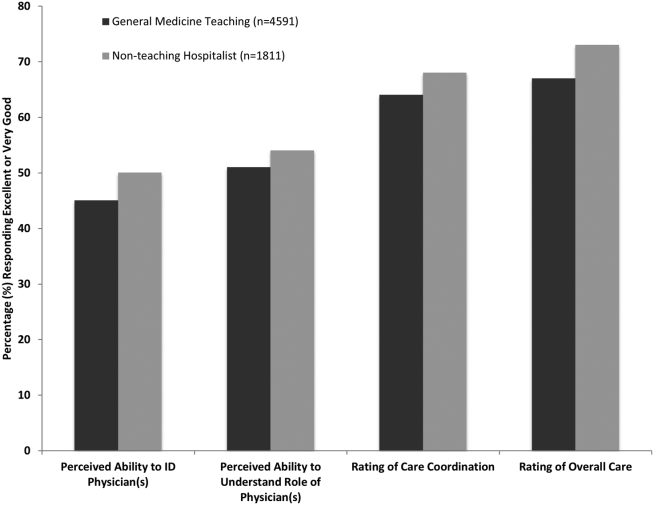
From the mixed‐effects regression models it was discovered that admission to the hospitalist service was associated with a higher odds ratio (OR) of reporting overall care as excellent or very good (OR: 1.33; 95% confidence interval [CI]: 1.15‐1.47). There was no difference between services in patients' ability to identify their physician(s) (OR: 0.89; 95% CI: 0.61‐1.11), in patients reporting a better understanding of the role of their physician(s) (OR: 1.09; 95% CI: 0.94‐1.23), or in their rating of overall coordination and teamwork (OR: 0.71; 95% CI: 0.42‐1.89).
A subgroup analysis was performed on the 25% of hospitalist attendings in the general medicine teaching service comparing this cohort to the hospitalist services, and it was found that patients perceived better overall care on the hospitalist service (OR: 1.17; 95% CI: 1.01‐ 1.31) than on the general medicine service (Table 2). All other domains in the subgroup analysis were not statistically significant. Finally, an ordinal logistic regression was performed for each of these outcomes, but it did not show any major differences compared to the logistic regression of dichotomous outcomes.
Domains in Patient Experience* | Odds Ratio (95% CI) | P Value |
---|---|---|
| ||
How would you rate your ability to identify the physicians and trainees on your general medicine team during the hospitalization? | ||
Model 1 | 0.89 (0.611.11) | 0.32 |
Model 2 | 0.98 (0.671.22) | 0.86 |
How would you rate your understanding of the roles of the physicians and trainees on your general medicine team? | ||
Model 1 | 1.09 (0.941.23) | 0.25 |
Model 2 | 1.19 (0.981.36) | 0.08 |
How would you rate the overall coordination and teamwork among the doctors and nurses who care for you during your hospital stay? | ||
Model 1 | 0.71 (0.421.89) | 0.18 |
Model 2 | 0.82 (0.651.20) | 0.23 |
Overall, how would you rate the care you received at the hospital? | ||
Model 1 | 1.33 (1.151.47) | 0.001 |
Model 2 | 1.17 (1.011.31) | 0.04 |
DISCUSSION
This study is the first to directly compare measures of patient experience on hospitalist and general medicine teaching services in a large, multiyear comparison across multiple domains. In adjusted analysis, we found that patients on nonteaching hospitalist services rated their overall care better than those on general medicine teaching services, whereas no differences in patients' ability to identify their physician(s), understand their role in their care, or rating of coordination of care were found. Although the magnitude of the differences in rating of overall care may appear small, it remains noteworthy because of the recent focus on patient experience at the reimbursement level, where small differences in performance can lead to large changes in payment. Because of the observational design of this study, it is important to consider mechanisms that could account for our findings.
The first are the structural differences between the 2 services. Our subgroup analysis comparing patients rating of overall care on a general medicine service with a hospitalist attending to a pure hospitalist cohort found a significant difference between the groups, indicating that the structural differences between the 2 groups may be a significant contributor to patient satisfaction ratings. Under the care of a hospitalist service, a patient would only interact with a single physician on a daily basis, possibly leading to a more meaningful relationship and improved communication between patient and provider. Alternatively, while on a general medicine teaching service, patients would likely interact with multiple physicians, as a result making their confidence in their ability to identify and perception at understanding physicians' roles more challenging.[18] This dilemma is further compounded by duty hour restrictions, which have subsequently led to increased fragmentation in housestaff scheduling. The patient experience on the general medicine teaching service may be further complicated by recent data that show residents spend a minority of time in direct patient care,[19, 20] which could additionally contribute to patients' inability to understand who their physicians are and to the decreased satisfaction with their care. This combination of structural complexity, duty hour reform, and reduced direct patient interaction would likely decrease the chance a patient will interact with the same resident on a consistent basis,[5, 21] thus making the ability to truly understand who their caretakers are, and the role they play, more difficult.
Another contributing factor could be the use of NPAs on our hospitalist service. Given that these providers often see the patient on a more continual basis, hospitalized patients' exposure to a single, continuous caretaker may be a factor in our findings.[22] Furthermore, with studies showing that hospitalists also spend a small fraction of their day in direct patient care,[23, 24, 25] the use of NPAs may allow our hospitalists to spend greater amounts of time with their patients, thus improving patients' rating of their overall care and influencing their perceived ability to understand their physician's role.
Although there was no difference between general medicine teaching and hospitalist services with respect to patient understanding of their roles, our data suggest that both groups would benefit from interventions to target this area. Focused attempts at improving patient's ability to identify and explain the roles of their inpatient physician(s) have been performed. For example, previous studies have attempted to improve a patient's ability to identify their physician through physician facecards[8, 9] or the use of other simple interventions (ie, bedside whiteboards).[4, 26] Results from such interventions are mixed, as they have demonstrated the capacity to improve patients' ability to identify who their physician is, whereas few have shown any appreciable improvement in patient satisfaction.[26]
Although our findings suggest that structural differences in team composition may be a possible explanation, it is also important to consider how the quality of care a patient receives affects their experience. For instance, hospitalists have been shown to produce moderate improvements in patient‐centered outcomes such as 30‐day readmission[27] and hospital length of stay[14, 28, 29, 30, 31] when compared to other care providers, which in turn could be reflected in the patient's perception of their overall care. In a large national study of acute care hospitals using the Hospital Consumer Assessment of Healthcare Providers and Systems survey, Chen and colleagues found that for most measures of patient satisfaction, hospitals with greater use of hospitalist care were associated with better patient‐centered care.[13] These outcomes were in part driven by patient‐centered domains such as discharge planning, pain control, and medication management. It is possible that patients are sensitive to the improved outcomes that are associated with hospitalist services, and reflect this in their measures of patient satisfaction.
Last, because this is an observational study and not a randomized trial, it is possible that the clinical differences in the patients cared for by these services could have led to our findings. Although the clinical significance of the differences in patient demographics were small, patients seen on the hospitalist service were more likely to be older white males, with a slightly longer LOS, greater comorbidities, and more hospitalizations in the previous year than those seen on the general medicine teaching service. Additionally, our hospitalist service frequently cares for highly specific subpopulations (ie, liver and renal transplant patients, and oncology patients), which could have influenced our results. For example, transplant patients who may be very grateful for their second chance, are preferentially admitted to the hospitalist service, which could have biased our results in favor of hospitalists.[32] Unfortunately, we were unable to control for all such factors.
Although we hope that multivariable analysis can adjust for many of these differences, we are not able to account for possible unmeasured confounders such as time of day of admission, health literacy, personality differences, physician turnover, or nursing and other ancillary care that could contribute to these findings. In addition to its observational study design, our study has several other limitations. First, our study was performed at a single institution, thus limiting its generalizability. Second, as a retrospective study based on observational data, no definitive conclusions regarding causality can be made. Third, although our response rate was low, it is comparable to other studies that have examined underserved populations.[33, 34] Fourth, because our survey was performed 30 days after hospitalization, this may impart imprecision on our outcomes measures. Finally, we were not able to mitigate selection bias through imputation for missing data .
All together, given the small absolute differences between the groups in patients' ratings of their overall care compared to large differences in possible confounders, these findings call for further exploration into the significance and possible mechanisms of these outcomes. Our study raises the potential possibility that the structural component of a care team may play a role in overall patient satisfaction. If this is the case, future studies of team structure could help inform how best to optimize this component for the patient experience. On the other hand, if process differences are to explain our findings, it is important to distill the types of processes hospitalists are using to improve the patient experience and potentially export this to resident services.
Finally, if similar results were found in other institutions, these findings could have implications on how hospitals respond to new payment models that are linked to patient‐experience measures. For example, the Hospital Value‐Based Purchasing Program currently links the Centers for Medicare and Medicaid Services payments to a set of quality measures that consist of (1) clinical processes of care (70%) and (2) the patient experience (30%).[1] Given this linkage, any small changes in the domain of patient satisfaction could have large payment implications on a national level.
CONCLUSION
In summary, in this large‐scale multiyear study, patients cared for by a nonteaching hospitalist service reported greater satisfaction with their overall care than patients cared for by a general medicine teaching service. This difference could be mediated by the structural differences between these 2 services. As hospitals seek to optimize patient experiences in an era where reimbursement models are now being linked to patient‐experience measures, future work should focus on further understanding the mechanisms for these findings.
Disclosures
Financial support for this work was provided by the Robert Wood Johnson Investigator Program (RWJF Grant ID 63910 PI Meltzer), a Midcareer Career Development Award from the National Institute of Aging (1 K24 AG031326‐01, PI Meltzer), and a Clinical and Translational Science Award (NIH/NCATS 2UL1TR000430‐08, PI Solway, Meltzer Core Leader). The authors report no conflicts of interest.
The hospitalized patient experience has become an area of increased focus for hospitals given the recent coupling of patient satisfaction to reimbursement rates for Medicare patients.[1] Although patient experiences are multifactorial, 1 component is the relationship that hospitalized patients develop with their inpatient physicians. In recognition of the importance of this relationship, several organizations including the Society of Hospital Medicine, Society of General Internal Medicine, American College of Physicians, the American College of Emergency Physicians, and the Accreditation Council for Graduate Medical Education have recommended that patients know and understand who is guiding their care at all times during their hospitalization.[2, 3] Unfortunately, previous studies have shown that hospitalized patients often lack the ability to identify[4, 5] and understand their course of care.[6, 7] This may be due to numerous clinical factors including lack of a prior relationship, rapid pace of clinical care, and the frequent transitions of care found in both hospitalists and general medicine teaching services.[5, 8, 9] Regardless of the cause, one could hypothesize that patients who are unable to identify or understand the role of their physician may be less informed about their hospitalization, which may lead to further confusion, dissatisfaction, and ultimately a poor experience.
Given the proliferation of nonteaching hospitalist services in teaching hospitals, it is important to understand if patient experiences differ between general medicine teaching and hospitalist services. Several reasons could explain why patient experiences may vary on these services. For example, patients on a hospitalist service will likely interact with a single physician caretaker, which may give a feeling of more personalized care. In contrast, patients on general medicine teaching services are cared for by larger teams of residents under the supervision of an attending physician. Residents are also subjected to duty‐hour restrictions, clinic responsibilities, and other educational requirements that may impede the continuity of care for hospitalized patients.[10, 11, 12] Although 1 study has shown that hospitalist‐intensive hospitals perform better on patient satisfaction measures,[13] no study to date has compared patient‐reported experiences on general medicine teaching and nonteaching hospitalist services. This study aimed to evaluate the hospitalized patient experience on both teaching and nonteaching hospitalist services by assessing several patient‐reported measures of their experience, namely their confidence in their ability to identify their physician(s), understand their roles, and their rating of both the coordination and overall care.
METHODS
Study Design
We performed a retrospective cohort analysis at the University of Chicago Medical Center between July 2007 and June 2013. Data were acquired as part of the Hospitalist Project, an ongoing study that is used to evaluate the impact of hospitalists, and now serves as infrastructure to continue research related to hospital care at University of Chicago.[14] Patients were cared for by either the general medicine teaching service or the nonteaching hospitalist service. General medicine teaching services were composed of an attending physician who rotates for 2 weeks at a time, a second‐ or third‐year medicine resident, 1 to 2 medicine interns, and 1 to 2 medical students.[15] The attending physician assigned to the patient's hospitalization was the attending listed on the first day of hospitalization, regardless of the length of hospitalization. Nonteaching hospitalist services consisted of a single hospitalist who worked 7‐day shifts, and were assisted by a nurse practitioner/physician's assistant (NPA). The majority of attendings on the hospitalist service were less than 5 years out of residency. Both services admitted 7 days a week, with patients initially admitted to the general medicine teaching service until resident caps were met, after which all subsequent admissions were admitted to the hospitalist service. In addition, the hospitalist service is also responsible for specific patient subpopulations, such as lung and renal transplants, and oncologic patients who have previously established care with our institution.
Data Collection
During a 30‐day posthospitalization follow‐up questionnaire, patients were surveyed regarding their confidence in their ability to identify and understand the roles of their physician(s) and their perceptions of the overall coordination of care and their overall care, using a 5‐point Likert scale (1 = poor understanding to 5 = excellent understanding). Questions related to satisfaction with care and coordination were derived from the Picker‐Commonwealth Survey, a previously validated survey meant to evaluate patient‐centered care.[16] Patients were also asked to report their race, level of education, comorbid diseases, and whether they had any prior hospitalizations within 1 year. Chart review was performed to obtain patient age, gender, and hospital length of stay (LOS), and calculated Charlson Comorbidity Index (CCI).[17] Patients with missing data or responses to survey questions were excluded from final analysis. The University of Chicago Institutional Review Board approved the study protocol, and all patients provided written consented prior to participation.
Data Analysis
After initial analysis noted that outcomes were skewed, the decision was made to dichotomize the data and use logistic rather than linear regression models. Patient responses to the follow‐up phone questionnaire were dichotomized to reflect the top 2 categories (excellent and very good). Pearson 2 analysis was used to assess for any differences in demographic characteristics, disease severity, and measures of patient experience between the 2 services. To assess if service type was associated with differences in our 4 measures of patient experience, we created a 3‐level mixed‐effects logistic regression using a logit function while controlling for age, gender, race, CCI, LOS, previous hospitalizations within 1 year, level of education, and academic year. These models studied the longitudinal association between teaching service and the 4 outcome measures, while also controlling for the cluster effect of time nested within individual patients who were clustered within physicians. The model included random intercepts at both the patient and physician level and also included a random effect of service (teaching vs nonteaching) at the patient level. A Hausman test was used to determine if these random‐effects models improved fit over a fixed‐effects model, and the intraclass correlations were compared using likelihood ratio tests to determine the appropriateness of a 3‐level versus 2‐level model. Data management and 2 analyses were performed using Stata version 13.0 (StataCorp, College Station, TX), and mixed‐effects regression models were done in SuperMix (Scientific Software International, Skokie, IL).
RESULTS
In total, 14,855 patients were enrolled during their hospitalization with 57% and 61% completing the 30‐day follow‐up survey on the hospitalist and general medicine teaching service, respectively. In total, 4131 (69%) and 4322 (48%) of the hospitalist and general medicine services, respectively, either did not answer all survey questions, or were missing basic demographic data, and thus were excluded. Data from 4591 patients on the general medicine teaching (52% of those enrolled at hospitalization) and 1811 on the hospitalist service (31% of those enrolled at hospitalization) were used for final analysis (Figure 1). Respondents were predominantly female (61% and 56%), African American (75% and 63%), with a mean age of 56.2 (19.4) and 57.1 (16.1) years, for the general medicine teaching and hospitalist services, respectively. A majority of patients (71% and 66%) had a CCI of 0 to 3 on both services. There were differences in self‐reported comorbidities between the 2 groups, with hospitalist services having a higher prevalence of cancer (20% vs 7%), renal disease (25% vs 18%), and liver disease (23% vs 7%). Patients on the hospitalist service had a longer mean LOS (5.5 vs 4.8 days), a greater percentage of a hospitalization within 1 year (58% vs 52%), and a larger proportion who were admitted in 2011 to 2013 compared to 2007 to 2010 (75% vs 39%), when compared to the general medicine teaching services. Median LOS and interquartile ranges were similar between both groups. Although most baseline demographics were statistically different between the 2 groups (Table 1), these differences were likely clinically insignificant. Compared to those who responded to the follow‐up survey, nonresponders were more likely to be African American (73% and 64%, P < 0.001) and female (60% and 56%, P < 0.01). The nonresponders were more likely to be hospitalized in the past 1 year (62% and 53%, P < 0.001) and have a lower CCI (CCI 03 [75% and 80%, P < 0.001]) compared to responders. Demographics between responders and nonresponders were also statistically different from one another.
Variable | General Medicine Teaching | Nonteaching Hospitalist | P Value |
---|---|---|---|
| |||
Total (n) | 4,591 | 1,811 | <0.001 |
Attending classification, hospitalist, n (%) | 1,147 (25) | 1,811 (100) | |
Response rate, % | 61 | 57 | <0.01 |
Age, y, mean SD | 56.2 19.4 | 57.1 16.1 | <0.01 |
Gender, n (%) | <0.01 | ||
Male | 1,796 (39) | 805 (44) | |
Female | 2,795 (61) | 1,004 (56) | |
Race, n (%) | <0.01 | ||
African American | 3,440 (75) | 1,092 (63) | |
White | 900 (20) | 571 (32) | |
Asian/Pacific | 38 (1) | 17 (1) | |
Other | 20 (1) | 10 (1) | |
Unknown | 134 (3) | 52 (3) | |
Charlson Comorbidity Index, n (%) | <0.001 | ||
0 | 1,635 (36) | 532 (29) | |
12 | 1,590 (35) | 675 (37) | |
39 | 1,366 (30) | 602 (33) | |
Self‐reported comorbidities | |||
Anemia/sickle cell disease | 1,201 (26) | 408 (23) | 0.003 |
Asthma/COPD | 1,251 (28) | 432 (24) | 0.006 |
Cancer* | 300 (7) | 371 (20) | <0.001 |
Depression | 1,035 (23) | 411 (23) | 0.887 |
Diabetes | 1,381 (30) | 584 (32) | 0.087 |
Gastrointestinal | 1,140 (25) | 485 (27) | 0.104 |
Cardiac | 1,336 (29) | 520 (29) | 0.770 |
Hypertension | 2,566 (56) | 1,042 (58) | 0.222 |
HIV/AIDS | 151 (3) | 40 (2) | 0.022 |
Kidney disease | 828 (18) | 459 (25) | <0.001 |
Liver disease | 313 (7) | 417 (23) | <0.001 |
Stroke | 543 (12) | 201 (11) | 0.417 |
Education level | 0.066 | ||
High school | 2,248 (49) | 832 (46) | |
Junior college/college | 1,878 (41) | 781 (43) | |
Postgraduate | 388 (8) | 173 (10) | |
Don't know | 77 (2) | 23 (1) | |
Academic year, n (%) | <0.001 | ||
July 2007 June 2008 | 938 (20) | 90 (5) | |
July 2008 June 2009 | 702 (15) | 148 (8) | |
July 2009 June 2010 | 576(13) | 85 (5) | |
July 2010 June 2011 | 602 (13) | 138 (8) | |
July 2011 June 2012 | 769 (17) | 574 (32) | |
July 2012 June 2013 | 1,004 (22) | 774 (43) | |
Length of stay, d, mean SD | 4.8 7.3 | 5.5 6.4 | <0.01 |
Prior hospitalization (within 1 year), yes, n (%) | 2,379 (52) | 1,039 (58) | <0.01 |
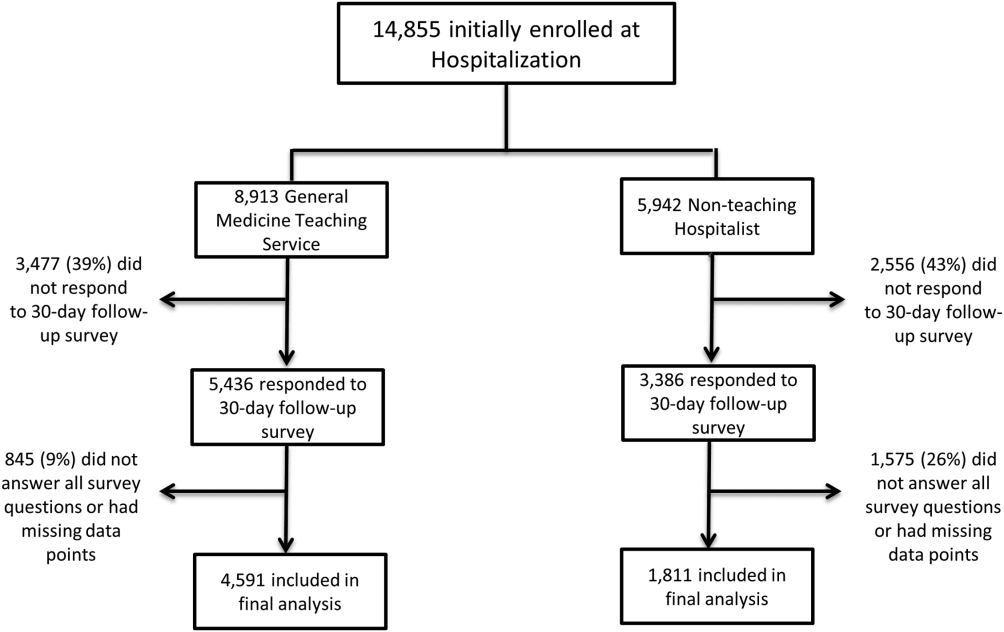
Unadjusted results revealed that patients on the hospitalist service were more confident in their abilities to identify their physician(s) (50% vs 45%, P < 0.001), perceived greater ability in understanding the role of their physician(s) (54% vs 50%, P < 0.001), and reported greater satisfaction with coordination and teamwork (68% vs 64%, P = 0.006) and with overall care (73% vs 67%, P < 0.001) (Figure 2).
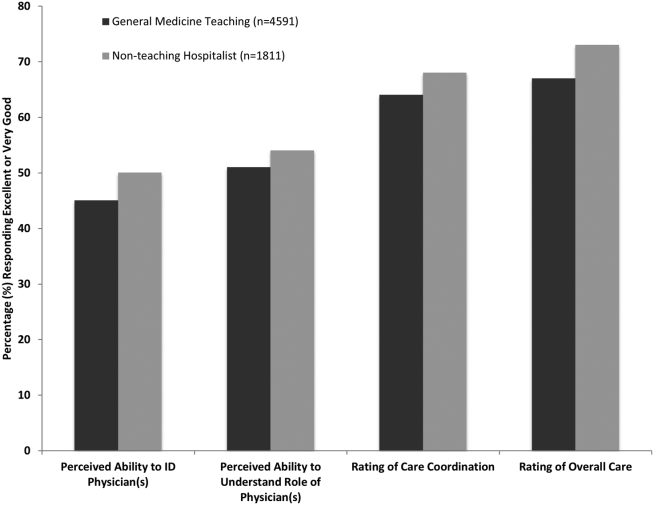
From the mixed‐effects regression models it was discovered that admission to the hospitalist service was associated with a higher odds ratio (OR) of reporting overall care as excellent or very good (OR: 1.33; 95% confidence interval [CI]: 1.15‐1.47). There was no difference between services in patients' ability to identify their physician(s) (OR: 0.89; 95% CI: 0.61‐1.11), in patients reporting a better understanding of the role of their physician(s) (OR: 1.09; 95% CI: 0.94‐1.23), or in their rating of overall coordination and teamwork (OR: 0.71; 95% CI: 0.42‐1.89).
A subgroup analysis was performed on the 25% of hospitalist attendings in the general medicine teaching service comparing this cohort to the hospitalist services, and it was found that patients perceived better overall care on the hospitalist service (OR: 1.17; 95% CI: 1.01‐ 1.31) than on the general medicine service (Table 2). All other domains in the subgroup analysis were not statistically significant. Finally, an ordinal logistic regression was performed for each of these outcomes, but it did not show any major differences compared to the logistic regression of dichotomous outcomes.
Domains in Patient Experience* | Odds Ratio (95% CI) | P Value |
---|---|---|
| ||
How would you rate your ability to identify the physicians and trainees on your general medicine team during the hospitalization? | ||
Model 1 | 0.89 (0.611.11) | 0.32 |
Model 2 | 0.98 (0.671.22) | 0.86 |
How would you rate your understanding of the roles of the physicians and trainees on your general medicine team? | ||
Model 1 | 1.09 (0.941.23) | 0.25 |
Model 2 | 1.19 (0.981.36) | 0.08 |
How would you rate the overall coordination and teamwork among the doctors and nurses who care for you during your hospital stay? | ||
Model 1 | 0.71 (0.421.89) | 0.18 |
Model 2 | 0.82 (0.651.20) | 0.23 |
Overall, how would you rate the care you received at the hospital? | ||
Model 1 | 1.33 (1.151.47) | 0.001 |
Model 2 | 1.17 (1.011.31) | 0.04 |
DISCUSSION
This study is the first to directly compare measures of patient experience on hospitalist and general medicine teaching services in a large, multiyear comparison across multiple domains. In adjusted analysis, we found that patients on nonteaching hospitalist services rated their overall care better than those on general medicine teaching services, whereas no differences in patients' ability to identify their physician(s), understand their role in their care, or rating of coordination of care were found. Although the magnitude of the differences in rating of overall care may appear small, it remains noteworthy because of the recent focus on patient experience at the reimbursement level, where small differences in performance can lead to large changes in payment. Because of the observational design of this study, it is important to consider mechanisms that could account for our findings.
The first are the structural differences between the 2 services. Our subgroup analysis comparing patients rating of overall care on a general medicine service with a hospitalist attending to a pure hospitalist cohort found a significant difference between the groups, indicating that the structural differences between the 2 groups may be a significant contributor to patient satisfaction ratings. Under the care of a hospitalist service, a patient would only interact with a single physician on a daily basis, possibly leading to a more meaningful relationship and improved communication between patient and provider. Alternatively, while on a general medicine teaching service, patients would likely interact with multiple physicians, as a result making their confidence in their ability to identify and perception at understanding physicians' roles more challenging.[18] This dilemma is further compounded by duty hour restrictions, which have subsequently led to increased fragmentation in housestaff scheduling. The patient experience on the general medicine teaching service may be further complicated by recent data that show residents spend a minority of time in direct patient care,[19, 20] which could additionally contribute to patients' inability to understand who their physicians are and to the decreased satisfaction with their care. This combination of structural complexity, duty hour reform, and reduced direct patient interaction would likely decrease the chance a patient will interact with the same resident on a consistent basis,[5, 21] thus making the ability to truly understand who their caretakers are, and the role they play, more difficult.
Another contributing factor could be the use of NPAs on our hospitalist service. Given that these providers often see the patient on a more continual basis, hospitalized patients' exposure to a single, continuous caretaker may be a factor in our findings.[22] Furthermore, with studies showing that hospitalists also spend a small fraction of their day in direct patient care,[23, 24, 25] the use of NPAs may allow our hospitalists to spend greater amounts of time with their patients, thus improving patients' rating of their overall care and influencing their perceived ability to understand their physician's role.
Although there was no difference between general medicine teaching and hospitalist services with respect to patient understanding of their roles, our data suggest that both groups would benefit from interventions to target this area. Focused attempts at improving patient's ability to identify and explain the roles of their inpatient physician(s) have been performed. For example, previous studies have attempted to improve a patient's ability to identify their physician through physician facecards[8, 9] or the use of other simple interventions (ie, bedside whiteboards).[4, 26] Results from such interventions are mixed, as they have demonstrated the capacity to improve patients' ability to identify who their physician is, whereas few have shown any appreciable improvement in patient satisfaction.[26]
Although our findings suggest that structural differences in team composition may be a possible explanation, it is also important to consider how the quality of care a patient receives affects their experience. For instance, hospitalists have been shown to produce moderate improvements in patient‐centered outcomes such as 30‐day readmission[27] and hospital length of stay[14, 28, 29, 30, 31] when compared to other care providers, which in turn could be reflected in the patient's perception of their overall care. In a large national study of acute care hospitals using the Hospital Consumer Assessment of Healthcare Providers and Systems survey, Chen and colleagues found that for most measures of patient satisfaction, hospitals with greater use of hospitalist care were associated with better patient‐centered care.[13] These outcomes were in part driven by patient‐centered domains such as discharge planning, pain control, and medication management. It is possible that patients are sensitive to the improved outcomes that are associated with hospitalist services, and reflect this in their measures of patient satisfaction.
Last, because this is an observational study and not a randomized trial, it is possible that the clinical differences in the patients cared for by these services could have led to our findings. Although the clinical significance of the differences in patient demographics were small, patients seen on the hospitalist service were more likely to be older white males, with a slightly longer LOS, greater comorbidities, and more hospitalizations in the previous year than those seen on the general medicine teaching service. Additionally, our hospitalist service frequently cares for highly specific subpopulations (ie, liver and renal transplant patients, and oncology patients), which could have influenced our results. For example, transplant patients who may be very grateful for their second chance, are preferentially admitted to the hospitalist service, which could have biased our results in favor of hospitalists.[32] Unfortunately, we were unable to control for all such factors.
Although we hope that multivariable analysis can adjust for many of these differences, we are not able to account for possible unmeasured confounders such as time of day of admission, health literacy, personality differences, physician turnover, or nursing and other ancillary care that could contribute to these findings. In addition to its observational study design, our study has several other limitations. First, our study was performed at a single institution, thus limiting its generalizability. Second, as a retrospective study based on observational data, no definitive conclusions regarding causality can be made. Third, although our response rate was low, it is comparable to other studies that have examined underserved populations.[33, 34] Fourth, because our survey was performed 30 days after hospitalization, this may impart imprecision on our outcomes measures. Finally, we were not able to mitigate selection bias through imputation for missing data .
All together, given the small absolute differences between the groups in patients' ratings of their overall care compared to large differences in possible confounders, these findings call for further exploration into the significance and possible mechanisms of these outcomes. Our study raises the potential possibility that the structural component of a care team may play a role in overall patient satisfaction. If this is the case, future studies of team structure could help inform how best to optimize this component for the patient experience. On the other hand, if process differences are to explain our findings, it is important to distill the types of processes hospitalists are using to improve the patient experience and potentially export this to resident services.
Finally, if similar results were found in other institutions, these findings could have implications on how hospitals respond to new payment models that are linked to patient‐experience measures. For example, the Hospital Value‐Based Purchasing Program currently links the Centers for Medicare and Medicaid Services payments to a set of quality measures that consist of (1) clinical processes of care (70%) and (2) the patient experience (30%).[1] Given this linkage, any small changes in the domain of patient satisfaction could have large payment implications on a national level.
CONCLUSION
In summary, in this large‐scale multiyear study, patients cared for by a nonteaching hospitalist service reported greater satisfaction with their overall care than patients cared for by a general medicine teaching service. This difference could be mediated by the structural differences between these 2 services. As hospitals seek to optimize patient experiences in an era where reimbursement models are now being linked to patient‐experience measures, future work should focus on further understanding the mechanisms for these findings.
Disclosures
Financial support for this work was provided by the Robert Wood Johnson Investigator Program (RWJF Grant ID 63910 PI Meltzer), a Midcareer Career Development Award from the National Institute of Aging (1 K24 AG031326‐01, PI Meltzer), and a Clinical and Translational Science Award (NIH/NCATS 2UL1TR000430‐08, PI Solway, Meltzer Core Leader). The authors report no conflicts of interest.
- Hospital Consumer Assessment of Healthcare Providers and Systems. HCAHPS fact sheet. CAHPS hospital survey August 2013. Available at: http://www.hcahpsonline.org/files/August_2013_HCAHPS_Fact_Sheet3.pdf. Accessed February 2, 2015.
- Transitions of Care Consensus policy statement: American College of Physicians, Society of General Internal Medicine, Society of Hospital Medicine, American Geriatrics Society, American College Of Emergency Physicians, and Society for Academic Emergency Medicine. J Hosp Med. 2009;4(6):364–370. , , , et al.
- Accreditation Council for Graduate Medical Education. Common program requirements. Available at: http://www.acgme.org/acgmeweb/Portals/0/PFAssets/ProgramRequirements/CPRs2013.pdf. Accessed January 15, 2015.
- Increasing a patient's ability to identify his or her attending physician using a patient room display. Arch Intern Med. 2010;170(12):1084–1085. , , .
- Ability of hospitalized patients to identify their in‐hospital physicians. Arch Intern Med. 2009;169(2):199–201. , , , , , .
- Hospitalized patients' understanding of their plan of care. Mayo Clin Proc. 2010;85(1):47–52. , , , et al.
- Patient‐physician communication at hospital discharge and patients' understanding of the postdischarge treatment plan. Arch Intern Med. 1997;157(9):1026–1030. , , , et al.
- Improving inpatients' identification of their doctors: use of FACE cards. Jt Comm J Qual Patient Saf. 2009;35(12):613–619. , , , et al.
- The impact of facecards on patients' knowledge, satisfaction, trust, and agreement with hospital physicians: a pilot study. J Hosp Med. 2014;9(3):137–141. , , , , , .
- Restructuring an inpatient resident service to improve outcomes for residents, students, and patients. Acad Med. 2011;86(12):1500–1507. , , .
- Residency training in the modern era: the pipe dream of less time to learn more, care better, and be more professional. Arch Intern Med. 2005;165(22):2561–2562. , , .
- Managing discontinuity in academic medical centers: strategies for a safe and effective resident sign‐out. J Hosp Med. 2006;1(4):257–266. , , , , .
- Hospitalist staffing and patient satisfaction in the national Medicare population. J Hosp Med. 2013;8(3):126–131. , , , .
- Effects of physician experience on costs and outcomes on an academic general medicine service: results of a trial of hospitalists. Ann Intern Med. 2002;137(11):866–874. , , , et al.
- The Effects of on‐duty napping on intern sleep time and fatigue. Ann Intern Med. 2006;144(11):792–798. , , , , , .
- Patients evaluate their hospital care: a national survey. Health Aff (Millwood). 1991;10(4):254–267. , , , et al.
- A new method of classifying prognostic comorbidity in longitudinal studies: development and validation. J Chronic Dis. 1987;40(5):373–383. , , , .
- Agency for Healthcare Research and Quality. Welcome to HCUPnet. Available at: http://hcupnet.ahrq.gov/HCUPnet.jsp?Id=F70FC59C286BADCB371(4):293–295.
- In the wake of the 2003 and 2011 duty hours regulations, how do internal medicine interns spend their time? J Gen Intern Med. 2013;28(8):1042–1047. , , , et al.
- The composition of intern work while on call. J Gen Intern Med. 2012;27(11):1432–1437. , , , , , .
- Effect of the 2011 vs 2003 duty hour regulation‐compliant models on sleep duration, trainee education, and continuity of patient care among internal medicine house staff: a randomized trial. JAMA Intern Med. 2013;173(8):649–655. , , , et al.
- The impact of hospitalist discontinuity on hospital cost, readmissions, and patient satisfaction. J Gen Intern Med. 2014;29(7):1004–1008. , , , et al.
- Hospitalist time usage and cyclicality: opportunities to improve efficiency. J Hosp Med. 2010;5(6):329–334. , , , , .
- Where did the day go?—a time‐motion study of hospitalists. J Hosp Med. 2010;5(6):323–328. , , , et al.
- How hospitalists spend their time: insights on efficiency and safety. J Hosp Med. 2006;1(2):88–93. , , .
- Patient satisfaction associated with correct identification of physician's photographs. Mayo Clin Proc. 2001;76(6):604–608. , , .
- Comparing patient outcomes of academician‐preceptors, hospitalist‐preceptors, and hospitalists on internal medicine services in an academic medical center. J Gen Intern Med. 2014;29(12):1672–1678. , , , .
- Comparison of processes and outcomes of pneumonia care between hospitalists and community‐based primary care physicians. Mayo Clin Proc. 2002;77(10):1053–1058. , , , .
- Outcomes of care by hospitalists, general internists, and family physicians. N Engl J Med. 2007;357(25):2589–2600. , , , , , .
- A systematic review of outcomes and quality measures in adult patients cared for by hospitalists vs nonhospitalists. Mayo Clin Proc. 2009;84(3):248–254. .
- Do hospitalist physicians improve the quality of inpatient care delivery? A systematic review of process, efficiency and outcome measures. BMC Med. 2011;9(1):58. , .
- Patients' experiences of everyday life after lung transplantation. J Clin Nurs. 2009;18(24):3472–3479. , .
- Optimal design features for surveying low‐income populations. J Health Care Poor Underserved. 2005;16(4):677–690. , , , et al.
- Hospital Consumer Assessment of Healthcare Providers and Systems. HCAHPS fact sheet. CAHPS hospital survey August 2013. Available at: http://www.hcahpsonline.org/files/August_2013_HCAHPS_Fact_Sheet3.pdf. Accessed February 2, 2015.
- Transitions of Care Consensus policy statement: American College of Physicians, Society of General Internal Medicine, Society of Hospital Medicine, American Geriatrics Society, American College Of Emergency Physicians, and Society for Academic Emergency Medicine. J Hosp Med. 2009;4(6):364–370. , , , et al.
- Accreditation Council for Graduate Medical Education. Common program requirements. Available at: http://www.acgme.org/acgmeweb/Portals/0/PFAssets/ProgramRequirements/CPRs2013.pdf. Accessed January 15, 2015.
- Increasing a patient's ability to identify his or her attending physician using a patient room display. Arch Intern Med. 2010;170(12):1084–1085. , , .
- Ability of hospitalized patients to identify their in‐hospital physicians. Arch Intern Med. 2009;169(2):199–201. , , , , , .
- Hospitalized patients' understanding of their plan of care. Mayo Clin Proc. 2010;85(1):47–52. , , , et al.
- Patient‐physician communication at hospital discharge and patients' understanding of the postdischarge treatment plan. Arch Intern Med. 1997;157(9):1026–1030. , , , et al.
- Improving inpatients' identification of their doctors: use of FACE cards. Jt Comm J Qual Patient Saf. 2009;35(12):613–619. , , , et al.
- The impact of facecards on patients' knowledge, satisfaction, trust, and agreement with hospital physicians: a pilot study. J Hosp Med. 2014;9(3):137–141. , , , , , .
- Restructuring an inpatient resident service to improve outcomes for residents, students, and patients. Acad Med. 2011;86(12):1500–1507. , , .
- Residency training in the modern era: the pipe dream of less time to learn more, care better, and be more professional. Arch Intern Med. 2005;165(22):2561–2562. , , .
- Managing discontinuity in academic medical centers: strategies for a safe and effective resident sign‐out. J Hosp Med. 2006;1(4):257–266. , , , , .
- Hospitalist staffing and patient satisfaction in the national Medicare population. J Hosp Med. 2013;8(3):126–131. , , , .
- Effects of physician experience on costs and outcomes on an academic general medicine service: results of a trial of hospitalists. Ann Intern Med. 2002;137(11):866–874. , , , et al.
- The Effects of on‐duty napping on intern sleep time and fatigue. Ann Intern Med. 2006;144(11):792–798. , , , , , .
- Patients evaluate their hospital care: a national survey. Health Aff (Millwood). 1991;10(4):254–267. , , , et al.
- A new method of classifying prognostic comorbidity in longitudinal studies: development and validation. J Chronic Dis. 1987;40(5):373–383. , , , .
- Agency for Healthcare Research and Quality. Welcome to HCUPnet. Available at: http://hcupnet.ahrq.gov/HCUPnet.jsp?Id=F70FC59C286BADCB371(4):293–295.
- In the wake of the 2003 and 2011 duty hours regulations, how do internal medicine interns spend their time? J Gen Intern Med. 2013;28(8):1042–1047. , , , et al.
- The composition of intern work while on call. J Gen Intern Med. 2012;27(11):1432–1437. , , , , , .
- Effect of the 2011 vs 2003 duty hour regulation‐compliant models on sleep duration, trainee education, and continuity of patient care among internal medicine house staff: a randomized trial. JAMA Intern Med. 2013;173(8):649–655. , , , et al.
- The impact of hospitalist discontinuity on hospital cost, readmissions, and patient satisfaction. J Gen Intern Med. 2014;29(7):1004–1008. , , , et al.
- Hospitalist time usage and cyclicality: opportunities to improve efficiency. J Hosp Med. 2010;5(6):329–334. , , , , .
- Where did the day go?—a time‐motion study of hospitalists. J Hosp Med. 2010;5(6):323–328. , , , et al.
- How hospitalists spend their time: insights on efficiency and safety. J Hosp Med. 2006;1(2):88–93. , , .
- Patient satisfaction associated with correct identification of physician's photographs. Mayo Clin Proc. 2001;76(6):604–608. , , .
- Comparing patient outcomes of academician‐preceptors, hospitalist‐preceptors, and hospitalists on internal medicine services in an academic medical center. J Gen Intern Med. 2014;29(12):1672–1678. , , , .
- Comparison of processes and outcomes of pneumonia care between hospitalists and community‐based primary care physicians. Mayo Clin Proc. 2002;77(10):1053–1058. , , , .
- Outcomes of care by hospitalists, general internists, and family physicians. N Engl J Med. 2007;357(25):2589–2600. , , , , , .
- A systematic review of outcomes and quality measures in adult patients cared for by hospitalists vs nonhospitalists. Mayo Clin Proc. 2009;84(3):248–254. .
- Do hospitalist physicians improve the quality of inpatient care delivery? A systematic review of process, efficiency and outcome measures. BMC Med. 2011;9(1):58. , .
- Patients' experiences of everyday life after lung transplantation. J Clin Nurs. 2009;18(24):3472–3479. , .
- Optimal design features for surveying low‐income populations. J Health Care Poor Underserved. 2005;16(4):677–690. , , , et al.
© 2015 Society of Hospital Medicine
CKD Awareness in Hospitalized Patients
Chronic kidney disease (CKD) affects over 13% of the US population and is associated with increased morbidity, mortality, and healthcare costs.[1] However, only 10% of individuals with CKD are aware of their diagnoses.[2] Even in those with stage 5 CKD, only 60% of individuals are aware of their CKD.[3] To our knowledge, no work has examined CKD awareness in a hospitalized patient population.
Patient awareness of their CKD diagnosis is important because progression of kidney disease can be slowed by patient self‐management of diabetes and hypertension.[4] Patient awareness of CKD may also increase acceptance of preend‐stage renal disease (ESRD) patient education and nephrology referral, which have been shown to delay CKD progression and improve clinical status at dialysis initiation.[5] However, only 60% of patients with advanced CKD have visited a nephrologist in the past year or have seen a nephrologist prior to dialysis initiation.[1]
The hospital is an important site for patient education and linkage to outpatient care for patients with CKD.[6] The hospital serves high‐risk patients who may not be well connected to outpatient care or who have lesswell‐controlled disease.[6, 7] Thus, hospitalization represents an opportunity to identify existing CKD and to use a multidisciplinary approach to preventative care, patient education, and patient‐provider planning for renal replacement therapy needs. In our cross‐sectional study in an urban, minority‐serving hospital, we sought to determine what patient factors were associated with hospitalized patients correctly self‐identifying as having CKD.
METHODS
Subjects and Data
We used data from the University of Chicago Hospitalist Project, a study of hospitalized patient outcomes.[8] Within 48 hours of hospitalization, all general medicine patients or their proxies are approached to enroll. During one‐on‐one inpatient interviews, a trained research assistant obtains demographic, health status, and healthcare utilization information. Participants consent for study staff to review their medical records. More than 80% of general medicine patients enroll.
We obtained data on 1234 general medicine patients discharged between January 1, 2012 and March 31, 2013 with an International Classification of Diseases, Ninth Revision (ICD‐9) code for chronic kidney disease (ICD‐9 codes 585.0585.5, 585.9) in their first 20 admission diagnoses. These codes are highly specific for CKD but have lower sensitivity.[9] We excluded all patients with a history of transplant (996.81, V42.0, n=90) or ESRD (585.6, n=416). We excluded repeat admissions during the study period (n=138). Our final sample included 590 unique patients with ICD‐9 diagnosis of CKD without ESRD.
Demographic, Clinical, and Health Service Utilization Characteristics
Our outcome was CKD awareness, the patient's correct self‐report of kidney disease. Patients selected their chronic medical conditions from a list read to them and were specifically asked if they had kidney problems. Demographic characteristics including age, gender, race/ethnicity, marital status, and education were also obtained. Healthcare utilization variables included how often the patient saw their primary medical care provider in the past year and whether patients had a prior hospitalization in the last year.
Health status variables such as mental status, diabetes, hypertension, and CKD stage were also assessed. Mental status was quantified using the telephone version of the Mini‐Mental State Examination (MMSE), scored from 1 to 22.10 We defined diabetes as ICD‐9 codes 250.0250.00, and hypertension as ICD‐9 codes 401.0, 401.9, 403, 405.09, 405.19, 405.91, 405.99, or by patient self‐report. CKD stage was based on the estimated glomerular filtration rate (eGFR) from the medical record using Kidney Disease Outcomes Quality Initiative guidelines.[11] We used the mode of the eGFR to calculate the appropriate CKD stage for those with more than 1 eGFR value from the hospitalization (576/590, 98%). The eGFR was calculated by the modified Modification of Diet in Renal Disease equation: (GFR (mL/min/1.73 m2)=175 (Screatinine)1.154 (Age)0.203 (0.742 if female) x (1.212 if African American)) recommended by the National Kidney Disease Education Program.[12]
Analysis
We used logistic regression to analyze the influence of the demographic, clinical, and healthcare utilization covariates on the likelihood of a patient reporting kidney problems. For the multivariate analysis, we sequentially added variables in a step‐wise fashion. We adjusted for (1) demographic factors: gender, race, ethnicity, marital status, and education; (2) eGFR‐calculated CKD stage and comorbidities: diabetes, hypertension, and mental status; and (3) healthcare utilization in the last 12 months: any hospitalizations and the number of visits to a health provider.
RESULTS
Patient Characteristics and Bivariable Association With Patient CKD Self‐Report
Table 1 shows demographic, clinical, and health service use characteristics for 590 patients with ICD‐9 coded CKD. In the bivariable model in Table 1, age, race, marital status and comorbidities, were associated with patient self‐report of CKD. Patients older than 80 years with physician‐identified CKD through ICD‐9 coding had 57% lower odds of reporting CKD than their younger counterparts. Patients of other races (nonwhite, non‐African American), married patients, and those with CKD stages 4 and 5 were much more likely to correctly self‐report CKD. Patients with higher MMSE score, diabetes, or hypertension had greater odds of CKD self‐report (all P<0.05).
Total, N=590 | Bivariable Odds Ratio (95% CI) | Multivariable With Stepwise Addition (95% CI) | |||
---|---|---|---|---|---|
Model 1, Demographic Factors | Model 2, Plus CKD Stage and Other Comorbidities | Model 3, Plus Health Service Use | |||
| |||||
Female | 312 (52.98%) | 1.21 (0.85‐1.70) | 1.64 (1.09‐2.47)* | 1.28 (0.79‐2.06) | 1.36 (0.75‐2.48) |
Age, y | |||||
Below 54 | 138 (23.4%) | REF | REF | REF | REF |
5466 | 150 (24.4%) | 0.96 (0.59‐1.54) | 0.95 (0.56‐1.59) | 1.01 (0.56‐1.81) | 0.83 (0.41‐1.68) |
6779 | 161 (27.3%) | 0.77 (0.48‐1.23) | 0.67 (0.39‐1.15) | 0.66 (0.35‐1.23) | 0.72(0.34‐1.52) |
Above 80 | 141 (23.9%) | 0.43 (0.26‐0.74) | 0.32 (0.17‐0.60) | 0.42 (0.20‐0.91)* | 0.37 (0.15‐0.93)* |
Race | |||||
African American | 449 (82.1%) | REF | REF | REF | REF |
White | 75 (13.7%) | 1.47 (0.89‐2.42) | 1.53 (0.86‐2.71) | 1.56 (0.78‐3.12) | 1.08 (0.47‐2.49) |
Other | 23 (4.2%) | 3.62 (1.53‐8.54) | 5.29 (1.78‐15.73) | 6.19 (1.72‐22.29) | 11.63 (1.80‐75.21)* |
Ethnicity (Hispanic is reference group) | 21 (4.0%) | 0.97 (0.38‐2.44) | 0.43 (0.13‐1.45) | 0.71 (0.16‐3.09) | 0.46 (0.06‐3.57) |
Married | 171 (32.6%) | 1.49 (1.03‐2.18)* | 1.32 (0.85‐2.04) | 1.20 (0.73‐1.98) | 1.77 (0.94‐3.33) |
Education | |||||
Less than high school | 128 (25.2%) | REF | REF | REF | REF |
High school grad/some college | 296 (58.2%) | 1.05 (0.68‐1.63) | 0.93 (0.58‐1.49) | 0.95 (0.54‐1.67) | 0.85 (0.42‐1.72) |
College grad or higher | 85 (16.7%) | 1.13 (0.64‐2.01) | 0.96 (0.51‐1.78) | 1.07 (0.51‐2.23) | 0.69 (0.27‐1.77) |
CKD stage (eGFR calculated, mode) | |||||
12 | 115 (20.0%) | 0.55 (0.32‐0.95)* | 0.52 (0.27‐1.01) | 0.28 (0.11‐0.69) | |
3 | 300 (52.1%) | REF | REF | REF | |
4 | 112 (19.4%) | 2.43 (1.55‐3.81) | 2.69 (1.52‐4.76) | 3.07 (1.56‐6.07) | |
5 | 49 (8.5%) | 4.50 (2.39‐8.48) | 3.94 (1.81‐8.56) | 5.16 (1.85‐14.41) | |
Diabetes (ICD‐9 coded or self‐report)‖ | 292 (49.5%) | 1.52 (1.07‐2.15)* | 1.54 (0.97‐2.45) | 1.26 (0.71‐2.26) | |
Hypertension (ICD‐9 coded or self‐report) | 436 (73.9%) | 3.36 (2.09‐5.41) | 1.53 (0.80‐2.90) | 1.26 (0.57‐2.81) | |
Mini‐Mental State Exam score# | 19.7 (2.5) | 1.13 (1.03‐1.23) | 1.09 (0.98‐1.22) | 1.22 (1.06‐1.42) | |
Hospitalized in last 12 months | 253 (46.9%) | 1.50 (1.05‐2.13)* | 1.12 (0.65‐1.95) | ||
No. of visits to health provider | |||||
Once/year or less | 80 (18.4%) | REF | REF | ||
23 times/year | 58 (13.3%) | 0.80 (0.40‐1.60) | 0.44 (0.17‐1.16) | ||
4+ times/year | 298 (68.4%) | 0.60 (0.36‐0.99)* | 0.38 (0.19‐0.74) |
Multivariable Associations With Patient CKD Self‐Report
Age, race, and CKD stage remained consistently associated with CKD self‐report, although the magnitude of the effects (odds ratios [ORs]) varied across models (Table 1). Across all the models, patients older than 80 years were still significantly less likely than younger patients to self‐report CKD (ORs ranging from 0.32 to 0.42, all P<0.05). Patients classified as being of other race were found to have a 5.29 to 11.63 greater odds of CKD self‐report than African American patients (all P<0.05).
Patients with CKD stage 4 and 5 were more likely to self‐report than patients with CKD stage 3 (ORs ranging from 2.693.07 for stage 4 and 3.945.16 for stage 5, P<0.05). In the final model, every unit increase in MMSE score increased the odds of CKD self‐report by 22%. In addition, patients who saw their health provider 4 or more times per year were 62% less likely to self‐report CKD than patients who saw their provider 1 or fewer times per year (OR: 0.38, P<0.05).
A large proportion of patients (68.6%) were CKD unspecified by ICD‐9 codes, and only 27% of the unspecified group reported having CKD (Table 2). Examining the eGFR CKD stage of the CKD unspecified group showed that 22.8% were eGFR‐determined CKD stage 1 to 2, 57.1% were CKD stage 3, 14.8% were CKD stage 4, and 5.3% were CKD stage 5. Patients had 2 to 3 times greater odds of correct CKD self‐report if physicians had correctly identified their CKD stage (bivariable OR: 2.42, 95% confidence interval [CI]: 1.57‐3.72, multivariable OR: 3.22, 95% CI: 0.99‐10.46) (analysis not shown).
CKD Stage* | Physician (ICD‐9) Coded | eGFR Calculated | eGFR Coded With ICD‐9 Correct |
---|---|---|---|
| |||
Unspecified | 110 (27.2%) | ||
12 | 5 (31.2%) | 20 (17.4%) | 0 |
3 | 25 (27.2%) | 83 (27.7%) | 17 (29.8%) |
4 | 40 (63.5%) | 54 (48.2%) | 25 (69.4%) |
5 | 11 (78.6%) | 31 (63.3%) | 10 (83.3%) |
DISCUSSION
Although prior work has examined CKD awareness in the general population and in high‐risk cohorts,[2, 3, 13] this is the first study examining CKD awareness in an urban, underserved hospitalized population. We found that overall patients' CKD awareness was low (32%), but increased as high as 63% for CKD stage 5, even after controlling for patient demographic, clinical characteristics, and healthcare use. Our overall rate of CKD awareness was higher than prior studies overall and at lower CKD stages.[2, 3, 13] Our work is consistent with prior literature that shows increasing CKD awareness with advancing CKD stage.[2, 3, 13]
Older patients (>80 years) had lower awareness of CKD. Older patients are more likely to have a near normal creatinine, despite a markedly reduced eGFR, so their CKD may go unnoticed.[14] Even with appropriate recognition, providers may also feel like their CKD is unlikely to progress to ESRD, given its stability and/or their competing risk of death.[15] Finally, older hospitalized patients may also be less likely to report a CKD diagnosis due to difficulty in recall due to denial, dementia, or delirium.
One limitation is that our case‐finding for CKD was physician ICD‐9 coding, which is highly specific but not sensitive.[9] The majority of patients with physician‐identified CKD were CKD unspecified, perhaps due to poor coding, physician underdocumentation, or physician under‐recognition of CKD stage. Although only 27% of the CKD unspecified group correctly self‐identified as having CKD, over 75% were found to be CKD stage 3 or higher, which should trigger additional monitoring or care based on guidelines.[10] In addition, despite statistical significance, we may not be able to make meaningful inferences about our small other group (nonwhite, non‐African American). Our sample was from 1 hospitalan urban, academic, tertiary care center with a large proportion of African American patientswhich may limit generalizability. The multivariable model will need to be tested in other populations for reproducibility.
Our study significantly contributes to the literature by examining patient awareness of CKD in a high‐risk, urban, hospitalized minority population. Other study strengths include use of basic demographic information, as well as survey and laboratory data for a richer examination of the associations between patient factors and CKD awareness.
CONCLUSION
Hospitalized patients with CKD have a low CKD awareness. Patient awareness of their CKD is increased with physician documentation of CKD severity. Patient awareness of their CKD must be coupled with provider awareness and CKD documentation to link patients to multidisciplinary CKD education and care to slow CKD progression and reduce associated cardiovascular and metabolic complications. Further work is needed across hospitals to determineand improveCKD awareness among both patients and providers.
Disclosures
Dr. Saunders was supported by Pilot and Feasibility Funding from the Chicago Center for Diabetes Translation Research (NIDDK P30 DK092949). Dr. Chin was supported by NIDDK K24 DK071933. Dr. Meltzer was supported by NIA T35 AG029795. Dr. Saunders had full access to all of the study data and takes responsibility for the integrity of the data and accuracy of the data analysis. An abstract of this article was presented at the Society of Hospital Medicine Annual Meeting in Las Vegas, Nevada in March 2014 and at the Society of General Internal Medicine Annual Meeting in San Diego, California in April 2014. The authors report no conflicts of interest.
- United States Renal Data System. Annual Data Report: Atlas of Chronic Kidney Disease and End‐Stage Renal Disease in the United States. Bethesda, MD: National Institutes of Health; 2012.
- Chronic kidney disease awareness among individuals with clinical markers of kidney dysfunction. Clin J Am Soc Neph. 2011;6(8):1838–1844. , , , et al.
- Comparison of CKD awareness in a screening population using the Modification of Diet in Renal Disease (MDRD) Study and CKD Epidemiology Collaboration (CKD‐EPI) equations. Am J Kidney Dis. 2011;57(3 suppl 2):S17–S23. , , , et al.
- Preserving renal function in adults with hypertension and diabetes: a consensus approach. Am J Kidney Dis. 2000;36(3):646–661. , , , et al.
- Multidisciplinary predialysis education decreases the incidence of dialysis and reduces mortality—a controlled cohort study based on the NKFDOQI guidelines. Nephrol Dial Transplant. 2009;24(11):3426–3433. , , , et al.
- Pre‐dialysis hospital use and late referrals in incident dialysis patients in England: a retrospective cohort study. Nephrol Dial Transplant. 2015;30(1):124–129. , , .
- Do hospitals that provide heart failure patient education prior to discharge also promote continuity of care? A report from OPTIMIZE‐HF. J Card Fail. 2006;12(6 suppl):S111. , , , et al.
- Effects of physician experience on costs and outcomes on an academic general medicine service: results of a trial of hospitalists. Ann Intern Med. 2002;137(11):866–874. , , , et al.
- Failure of ICD‐9‐CM codes to identify patients with comorbid chronic kidney disease in diabetes. Health Serv Res. 2006;41(2):564–580. , , , et al.
- Validation of a telephone version of the mini‐mental state examination. J Am Geriatr Soc. 1992;40(7):697–702. , , , .
- National Kidney Foundation. Kidney disease outcomes quality initiative guidelines 2002. Available at: http://www2.kidney.org/professionals/KDOQI/guidelines_ckd/toc.htm. Accessed October 9, 2014.
- A more accurate method to estimate glomerular filtration rate from serum creatinine: a new prediction equation. Ann Intern Med. 1999;130(6):461–470. , , , , , .
- Prevalence and awareness of CKD among African Americans: the Jackson Heart Study. Am J Kidney Dis. 2009;53(2):238–247. , , , et al.
- Magnitude of underascertainment of impaired kidney function in older adults with normal serum creatinine. J Am Geriatr Soc. 2007;55(6):816–823. , , , , , .
- Prediction, Progression, and Outcomes of Chronic Kidney Disease in Older Adults. J Am Soc Neph. 2009;20(6):1199–1209. , , , et al.
Chronic kidney disease (CKD) affects over 13% of the US population and is associated with increased morbidity, mortality, and healthcare costs.[1] However, only 10% of individuals with CKD are aware of their diagnoses.[2] Even in those with stage 5 CKD, only 60% of individuals are aware of their CKD.[3] To our knowledge, no work has examined CKD awareness in a hospitalized patient population.
Patient awareness of their CKD diagnosis is important because progression of kidney disease can be slowed by patient self‐management of diabetes and hypertension.[4] Patient awareness of CKD may also increase acceptance of preend‐stage renal disease (ESRD) patient education and nephrology referral, which have been shown to delay CKD progression and improve clinical status at dialysis initiation.[5] However, only 60% of patients with advanced CKD have visited a nephrologist in the past year or have seen a nephrologist prior to dialysis initiation.[1]
The hospital is an important site for patient education and linkage to outpatient care for patients with CKD.[6] The hospital serves high‐risk patients who may not be well connected to outpatient care or who have lesswell‐controlled disease.[6, 7] Thus, hospitalization represents an opportunity to identify existing CKD and to use a multidisciplinary approach to preventative care, patient education, and patient‐provider planning for renal replacement therapy needs. In our cross‐sectional study in an urban, minority‐serving hospital, we sought to determine what patient factors were associated with hospitalized patients correctly self‐identifying as having CKD.
METHODS
Subjects and Data
We used data from the University of Chicago Hospitalist Project, a study of hospitalized patient outcomes.[8] Within 48 hours of hospitalization, all general medicine patients or their proxies are approached to enroll. During one‐on‐one inpatient interviews, a trained research assistant obtains demographic, health status, and healthcare utilization information. Participants consent for study staff to review their medical records. More than 80% of general medicine patients enroll.
We obtained data on 1234 general medicine patients discharged between January 1, 2012 and March 31, 2013 with an International Classification of Diseases, Ninth Revision (ICD‐9) code for chronic kidney disease (ICD‐9 codes 585.0585.5, 585.9) in their first 20 admission diagnoses. These codes are highly specific for CKD but have lower sensitivity.[9] We excluded all patients with a history of transplant (996.81, V42.0, n=90) or ESRD (585.6, n=416). We excluded repeat admissions during the study period (n=138). Our final sample included 590 unique patients with ICD‐9 diagnosis of CKD without ESRD.
Demographic, Clinical, and Health Service Utilization Characteristics
Our outcome was CKD awareness, the patient's correct self‐report of kidney disease. Patients selected their chronic medical conditions from a list read to them and were specifically asked if they had kidney problems. Demographic characteristics including age, gender, race/ethnicity, marital status, and education were also obtained. Healthcare utilization variables included how often the patient saw their primary medical care provider in the past year and whether patients had a prior hospitalization in the last year.
Health status variables such as mental status, diabetes, hypertension, and CKD stage were also assessed. Mental status was quantified using the telephone version of the Mini‐Mental State Examination (MMSE), scored from 1 to 22.10 We defined diabetes as ICD‐9 codes 250.0250.00, and hypertension as ICD‐9 codes 401.0, 401.9, 403, 405.09, 405.19, 405.91, 405.99, or by patient self‐report. CKD stage was based on the estimated glomerular filtration rate (eGFR) from the medical record using Kidney Disease Outcomes Quality Initiative guidelines.[11] We used the mode of the eGFR to calculate the appropriate CKD stage for those with more than 1 eGFR value from the hospitalization (576/590, 98%). The eGFR was calculated by the modified Modification of Diet in Renal Disease equation: (GFR (mL/min/1.73 m2)=175 (Screatinine)1.154 (Age)0.203 (0.742 if female) x (1.212 if African American)) recommended by the National Kidney Disease Education Program.[12]
Analysis
We used logistic regression to analyze the influence of the demographic, clinical, and healthcare utilization covariates on the likelihood of a patient reporting kidney problems. For the multivariate analysis, we sequentially added variables in a step‐wise fashion. We adjusted for (1) demographic factors: gender, race, ethnicity, marital status, and education; (2) eGFR‐calculated CKD stage and comorbidities: diabetes, hypertension, and mental status; and (3) healthcare utilization in the last 12 months: any hospitalizations and the number of visits to a health provider.
RESULTS
Patient Characteristics and Bivariable Association With Patient CKD Self‐Report
Table 1 shows demographic, clinical, and health service use characteristics for 590 patients with ICD‐9 coded CKD. In the bivariable model in Table 1, age, race, marital status and comorbidities, were associated with patient self‐report of CKD. Patients older than 80 years with physician‐identified CKD through ICD‐9 coding had 57% lower odds of reporting CKD than their younger counterparts. Patients of other races (nonwhite, non‐African American), married patients, and those with CKD stages 4 and 5 were much more likely to correctly self‐report CKD. Patients with higher MMSE score, diabetes, or hypertension had greater odds of CKD self‐report (all P<0.05).
Total, N=590 | Bivariable Odds Ratio (95% CI) | Multivariable With Stepwise Addition (95% CI) | |||
---|---|---|---|---|---|
Model 1, Demographic Factors | Model 2, Plus CKD Stage and Other Comorbidities | Model 3, Plus Health Service Use | |||
| |||||
Female | 312 (52.98%) | 1.21 (0.85‐1.70) | 1.64 (1.09‐2.47)* | 1.28 (0.79‐2.06) | 1.36 (0.75‐2.48) |
Age, y | |||||
Below 54 | 138 (23.4%) | REF | REF | REF | REF |
5466 | 150 (24.4%) | 0.96 (0.59‐1.54) | 0.95 (0.56‐1.59) | 1.01 (0.56‐1.81) | 0.83 (0.41‐1.68) |
6779 | 161 (27.3%) | 0.77 (0.48‐1.23) | 0.67 (0.39‐1.15) | 0.66 (0.35‐1.23) | 0.72(0.34‐1.52) |
Above 80 | 141 (23.9%) | 0.43 (0.26‐0.74) | 0.32 (0.17‐0.60) | 0.42 (0.20‐0.91)* | 0.37 (0.15‐0.93)* |
Race | |||||
African American | 449 (82.1%) | REF | REF | REF | REF |
White | 75 (13.7%) | 1.47 (0.89‐2.42) | 1.53 (0.86‐2.71) | 1.56 (0.78‐3.12) | 1.08 (0.47‐2.49) |
Other | 23 (4.2%) | 3.62 (1.53‐8.54) | 5.29 (1.78‐15.73) | 6.19 (1.72‐22.29) | 11.63 (1.80‐75.21)* |
Ethnicity (Hispanic is reference group) | 21 (4.0%) | 0.97 (0.38‐2.44) | 0.43 (0.13‐1.45) | 0.71 (0.16‐3.09) | 0.46 (0.06‐3.57) |
Married | 171 (32.6%) | 1.49 (1.03‐2.18)* | 1.32 (0.85‐2.04) | 1.20 (0.73‐1.98) | 1.77 (0.94‐3.33) |
Education | |||||
Less than high school | 128 (25.2%) | REF | REF | REF | REF |
High school grad/some college | 296 (58.2%) | 1.05 (0.68‐1.63) | 0.93 (0.58‐1.49) | 0.95 (0.54‐1.67) | 0.85 (0.42‐1.72) |
College grad or higher | 85 (16.7%) | 1.13 (0.64‐2.01) | 0.96 (0.51‐1.78) | 1.07 (0.51‐2.23) | 0.69 (0.27‐1.77) |
CKD stage (eGFR calculated, mode) | |||||
12 | 115 (20.0%) | 0.55 (0.32‐0.95)* | 0.52 (0.27‐1.01) | 0.28 (0.11‐0.69) | |
3 | 300 (52.1%) | REF | REF | REF | |
4 | 112 (19.4%) | 2.43 (1.55‐3.81) | 2.69 (1.52‐4.76) | 3.07 (1.56‐6.07) | |
5 | 49 (8.5%) | 4.50 (2.39‐8.48) | 3.94 (1.81‐8.56) | 5.16 (1.85‐14.41) | |
Diabetes (ICD‐9 coded or self‐report)‖ | 292 (49.5%) | 1.52 (1.07‐2.15)* | 1.54 (0.97‐2.45) | 1.26 (0.71‐2.26) | |
Hypertension (ICD‐9 coded or self‐report) | 436 (73.9%) | 3.36 (2.09‐5.41) | 1.53 (0.80‐2.90) | 1.26 (0.57‐2.81) | |
Mini‐Mental State Exam score# | 19.7 (2.5) | 1.13 (1.03‐1.23) | 1.09 (0.98‐1.22) | 1.22 (1.06‐1.42) | |
Hospitalized in last 12 months | 253 (46.9%) | 1.50 (1.05‐2.13)* | 1.12 (0.65‐1.95) | ||
No. of visits to health provider | |||||
Once/year or less | 80 (18.4%) | REF | REF | ||
23 times/year | 58 (13.3%) | 0.80 (0.40‐1.60) | 0.44 (0.17‐1.16) | ||
4+ times/year | 298 (68.4%) | 0.60 (0.36‐0.99)* | 0.38 (0.19‐0.74) |
Multivariable Associations With Patient CKD Self‐Report
Age, race, and CKD stage remained consistently associated with CKD self‐report, although the magnitude of the effects (odds ratios [ORs]) varied across models (Table 1). Across all the models, patients older than 80 years were still significantly less likely than younger patients to self‐report CKD (ORs ranging from 0.32 to 0.42, all P<0.05). Patients classified as being of other race were found to have a 5.29 to 11.63 greater odds of CKD self‐report than African American patients (all P<0.05).
Patients with CKD stage 4 and 5 were more likely to self‐report than patients with CKD stage 3 (ORs ranging from 2.693.07 for stage 4 and 3.945.16 for stage 5, P<0.05). In the final model, every unit increase in MMSE score increased the odds of CKD self‐report by 22%. In addition, patients who saw their health provider 4 or more times per year were 62% less likely to self‐report CKD than patients who saw their provider 1 or fewer times per year (OR: 0.38, P<0.05).
A large proportion of patients (68.6%) were CKD unspecified by ICD‐9 codes, and only 27% of the unspecified group reported having CKD (Table 2). Examining the eGFR CKD stage of the CKD unspecified group showed that 22.8% were eGFR‐determined CKD stage 1 to 2, 57.1% were CKD stage 3, 14.8% were CKD stage 4, and 5.3% were CKD stage 5. Patients had 2 to 3 times greater odds of correct CKD self‐report if physicians had correctly identified their CKD stage (bivariable OR: 2.42, 95% confidence interval [CI]: 1.57‐3.72, multivariable OR: 3.22, 95% CI: 0.99‐10.46) (analysis not shown).
CKD Stage* | Physician (ICD‐9) Coded | eGFR Calculated | eGFR Coded With ICD‐9 Correct |
---|---|---|---|
| |||
Unspecified | 110 (27.2%) | ||
12 | 5 (31.2%) | 20 (17.4%) | 0 |
3 | 25 (27.2%) | 83 (27.7%) | 17 (29.8%) |
4 | 40 (63.5%) | 54 (48.2%) | 25 (69.4%) |
5 | 11 (78.6%) | 31 (63.3%) | 10 (83.3%) |
DISCUSSION
Although prior work has examined CKD awareness in the general population and in high‐risk cohorts,[2, 3, 13] this is the first study examining CKD awareness in an urban, underserved hospitalized population. We found that overall patients' CKD awareness was low (32%), but increased as high as 63% for CKD stage 5, even after controlling for patient demographic, clinical characteristics, and healthcare use. Our overall rate of CKD awareness was higher than prior studies overall and at lower CKD stages.[2, 3, 13] Our work is consistent with prior literature that shows increasing CKD awareness with advancing CKD stage.[2, 3, 13]
Older patients (>80 years) had lower awareness of CKD. Older patients are more likely to have a near normal creatinine, despite a markedly reduced eGFR, so their CKD may go unnoticed.[14] Even with appropriate recognition, providers may also feel like their CKD is unlikely to progress to ESRD, given its stability and/or their competing risk of death.[15] Finally, older hospitalized patients may also be less likely to report a CKD diagnosis due to difficulty in recall due to denial, dementia, or delirium.
One limitation is that our case‐finding for CKD was physician ICD‐9 coding, which is highly specific but not sensitive.[9] The majority of patients with physician‐identified CKD were CKD unspecified, perhaps due to poor coding, physician underdocumentation, or physician under‐recognition of CKD stage. Although only 27% of the CKD unspecified group correctly self‐identified as having CKD, over 75% were found to be CKD stage 3 or higher, which should trigger additional monitoring or care based on guidelines.[10] In addition, despite statistical significance, we may not be able to make meaningful inferences about our small other group (nonwhite, non‐African American). Our sample was from 1 hospitalan urban, academic, tertiary care center with a large proportion of African American patientswhich may limit generalizability. The multivariable model will need to be tested in other populations for reproducibility.
Our study significantly contributes to the literature by examining patient awareness of CKD in a high‐risk, urban, hospitalized minority population. Other study strengths include use of basic demographic information, as well as survey and laboratory data for a richer examination of the associations between patient factors and CKD awareness.
CONCLUSION
Hospitalized patients with CKD have a low CKD awareness. Patient awareness of their CKD is increased with physician documentation of CKD severity. Patient awareness of their CKD must be coupled with provider awareness and CKD documentation to link patients to multidisciplinary CKD education and care to slow CKD progression and reduce associated cardiovascular and metabolic complications. Further work is needed across hospitals to determineand improveCKD awareness among both patients and providers.
Disclosures
Dr. Saunders was supported by Pilot and Feasibility Funding from the Chicago Center for Diabetes Translation Research (NIDDK P30 DK092949). Dr. Chin was supported by NIDDK K24 DK071933. Dr. Meltzer was supported by NIA T35 AG029795. Dr. Saunders had full access to all of the study data and takes responsibility for the integrity of the data and accuracy of the data analysis. An abstract of this article was presented at the Society of Hospital Medicine Annual Meeting in Las Vegas, Nevada in March 2014 and at the Society of General Internal Medicine Annual Meeting in San Diego, California in April 2014. The authors report no conflicts of interest.
Chronic kidney disease (CKD) affects over 13% of the US population and is associated with increased morbidity, mortality, and healthcare costs.[1] However, only 10% of individuals with CKD are aware of their diagnoses.[2] Even in those with stage 5 CKD, only 60% of individuals are aware of their CKD.[3] To our knowledge, no work has examined CKD awareness in a hospitalized patient population.
Patient awareness of their CKD diagnosis is important because progression of kidney disease can be slowed by patient self‐management of diabetes and hypertension.[4] Patient awareness of CKD may also increase acceptance of preend‐stage renal disease (ESRD) patient education and nephrology referral, which have been shown to delay CKD progression and improve clinical status at dialysis initiation.[5] However, only 60% of patients with advanced CKD have visited a nephrologist in the past year or have seen a nephrologist prior to dialysis initiation.[1]
The hospital is an important site for patient education and linkage to outpatient care for patients with CKD.[6] The hospital serves high‐risk patients who may not be well connected to outpatient care or who have lesswell‐controlled disease.[6, 7] Thus, hospitalization represents an opportunity to identify existing CKD and to use a multidisciplinary approach to preventative care, patient education, and patient‐provider planning for renal replacement therapy needs. In our cross‐sectional study in an urban, minority‐serving hospital, we sought to determine what patient factors were associated with hospitalized patients correctly self‐identifying as having CKD.
METHODS
Subjects and Data
We used data from the University of Chicago Hospitalist Project, a study of hospitalized patient outcomes.[8] Within 48 hours of hospitalization, all general medicine patients or their proxies are approached to enroll. During one‐on‐one inpatient interviews, a trained research assistant obtains demographic, health status, and healthcare utilization information. Participants consent for study staff to review their medical records. More than 80% of general medicine patients enroll.
We obtained data on 1234 general medicine patients discharged between January 1, 2012 and March 31, 2013 with an International Classification of Diseases, Ninth Revision (ICD‐9) code for chronic kidney disease (ICD‐9 codes 585.0585.5, 585.9) in their first 20 admission diagnoses. These codes are highly specific for CKD but have lower sensitivity.[9] We excluded all patients with a history of transplant (996.81, V42.0, n=90) or ESRD (585.6, n=416). We excluded repeat admissions during the study period (n=138). Our final sample included 590 unique patients with ICD‐9 diagnosis of CKD without ESRD.
Demographic, Clinical, and Health Service Utilization Characteristics
Our outcome was CKD awareness, the patient's correct self‐report of kidney disease. Patients selected their chronic medical conditions from a list read to them and were specifically asked if they had kidney problems. Demographic characteristics including age, gender, race/ethnicity, marital status, and education were also obtained. Healthcare utilization variables included how often the patient saw their primary medical care provider in the past year and whether patients had a prior hospitalization in the last year.
Health status variables such as mental status, diabetes, hypertension, and CKD stage were also assessed. Mental status was quantified using the telephone version of the Mini‐Mental State Examination (MMSE), scored from 1 to 22.10 We defined diabetes as ICD‐9 codes 250.0250.00, and hypertension as ICD‐9 codes 401.0, 401.9, 403, 405.09, 405.19, 405.91, 405.99, or by patient self‐report. CKD stage was based on the estimated glomerular filtration rate (eGFR) from the medical record using Kidney Disease Outcomes Quality Initiative guidelines.[11] We used the mode of the eGFR to calculate the appropriate CKD stage for those with more than 1 eGFR value from the hospitalization (576/590, 98%). The eGFR was calculated by the modified Modification of Diet in Renal Disease equation: (GFR (mL/min/1.73 m2)=175 (Screatinine)1.154 (Age)0.203 (0.742 if female) x (1.212 if African American)) recommended by the National Kidney Disease Education Program.[12]
Analysis
We used logistic regression to analyze the influence of the demographic, clinical, and healthcare utilization covariates on the likelihood of a patient reporting kidney problems. For the multivariate analysis, we sequentially added variables in a step‐wise fashion. We adjusted for (1) demographic factors: gender, race, ethnicity, marital status, and education; (2) eGFR‐calculated CKD stage and comorbidities: diabetes, hypertension, and mental status; and (3) healthcare utilization in the last 12 months: any hospitalizations and the number of visits to a health provider.
RESULTS
Patient Characteristics and Bivariable Association With Patient CKD Self‐Report
Table 1 shows demographic, clinical, and health service use characteristics for 590 patients with ICD‐9 coded CKD. In the bivariable model in Table 1, age, race, marital status and comorbidities, were associated with patient self‐report of CKD. Patients older than 80 years with physician‐identified CKD through ICD‐9 coding had 57% lower odds of reporting CKD than their younger counterparts. Patients of other races (nonwhite, non‐African American), married patients, and those with CKD stages 4 and 5 were much more likely to correctly self‐report CKD. Patients with higher MMSE score, diabetes, or hypertension had greater odds of CKD self‐report (all P<0.05).
Total, N=590 | Bivariable Odds Ratio (95% CI) | Multivariable With Stepwise Addition (95% CI) | |||
---|---|---|---|---|---|
Model 1, Demographic Factors | Model 2, Plus CKD Stage and Other Comorbidities | Model 3, Plus Health Service Use | |||
| |||||
Female | 312 (52.98%) | 1.21 (0.85‐1.70) | 1.64 (1.09‐2.47)* | 1.28 (0.79‐2.06) | 1.36 (0.75‐2.48) |
Age, y | |||||
Below 54 | 138 (23.4%) | REF | REF | REF | REF |
5466 | 150 (24.4%) | 0.96 (0.59‐1.54) | 0.95 (0.56‐1.59) | 1.01 (0.56‐1.81) | 0.83 (0.41‐1.68) |
6779 | 161 (27.3%) | 0.77 (0.48‐1.23) | 0.67 (0.39‐1.15) | 0.66 (0.35‐1.23) | 0.72(0.34‐1.52) |
Above 80 | 141 (23.9%) | 0.43 (0.26‐0.74) | 0.32 (0.17‐0.60) | 0.42 (0.20‐0.91)* | 0.37 (0.15‐0.93)* |
Race | |||||
African American | 449 (82.1%) | REF | REF | REF | REF |
White | 75 (13.7%) | 1.47 (0.89‐2.42) | 1.53 (0.86‐2.71) | 1.56 (0.78‐3.12) | 1.08 (0.47‐2.49) |
Other | 23 (4.2%) | 3.62 (1.53‐8.54) | 5.29 (1.78‐15.73) | 6.19 (1.72‐22.29) | 11.63 (1.80‐75.21)* |
Ethnicity (Hispanic is reference group) | 21 (4.0%) | 0.97 (0.38‐2.44) | 0.43 (0.13‐1.45) | 0.71 (0.16‐3.09) | 0.46 (0.06‐3.57) |
Married | 171 (32.6%) | 1.49 (1.03‐2.18)* | 1.32 (0.85‐2.04) | 1.20 (0.73‐1.98) | 1.77 (0.94‐3.33) |
Education | |||||
Less than high school | 128 (25.2%) | REF | REF | REF | REF |
High school grad/some college | 296 (58.2%) | 1.05 (0.68‐1.63) | 0.93 (0.58‐1.49) | 0.95 (0.54‐1.67) | 0.85 (0.42‐1.72) |
College grad or higher | 85 (16.7%) | 1.13 (0.64‐2.01) | 0.96 (0.51‐1.78) | 1.07 (0.51‐2.23) | 0.69 (0.27‐1.77) |
CKD stage (eGFR calculated, mode) | |||||
12 | 115 (20.0%) | 0.55 (0.32‐0.95)* | 0.52 (0.27‐1.01) | 0.28 (0.11‐0.69) | |
3 | 300 (52.1%) | REF | REF | REF | |
4 | 112 (19.4%) | 2.43 (1.55‐3.81) | 2.69 (1.52‐4.76) | 3.07 (1.56‐6.07) | |
5 | 49 (8.5%) | 4.50 (2.39‐8.48) | 3.94 (1.81‐8.56) | 5.16 (1.85‐14.41) | |
Diabetes (ICD‐9 coded or self‐report)‖ | 292 (49.5%) | 1.52 (1.07‐2.15)* | 1.54 (0.97‐2.45) | 1.26 (0.71‐2.26) | |
Hypertension (ICD‐9 coded or self‐report) | 436 (73.9%) | 3.36 (2.09‐5.41) | 1.53 (0.80‐2.90) | 1.26 (0.57‐2.81) | |
Mini‐Mental State Exam score# | 19.7 (2.5) | 1.13 (1.03‐1.23) | 1.09 (0.98‐1.22) | 1.22 (1.06‐1.42) | |
Hospitalized in last 12 months | 253 (46.9%) | 1.50 (1.05‐2.13)* | 1.12 (0.65‐1.95) | ||
No. of visits to health provider | |||||
Once/year or less | 80 (18.4%) | REF | REF | ||
23 times/year | 58 (13.3%) | 0.80 (0.40‐1.60) | 0.44 (0.17‐1.16) | ||
4+ times/year | 298 (68.4%) | 0.60 (0.36‐0.99)* | 0.38 (0.19‐0.74) |
Multivariable Associations With Patient CKD Self‐Report
Age, race, and CKD stage remained consistently associated with CKD self‐report, although the magnitude of the effects (odds ratios [ORs]) varied across models (Table 1). Across all the models, patients older than 80 years were still significantly less likely than younger patients to self‐report CKD (ORs ranging from 0.32 to 0.42, all P<0.05). Patients classified as being of other race were found to have a 5.29 to 11.63 greater odds of CKD self‐report than African American patients (all P<0.05).
Patients with CKD stage 4 and 5 were more likely to self‐report than patients with CKD stage 3 (ORs ranging from 2.693.07 for stage 4 and 3.945.16 for stage 5, P<0.05). In the final model, every unit increase in MMSE score increased the odds of CKD self‐report by 22%. In addition, patients who saw their health provider 4 or more times per year were 62% less likely to self‐report CKD than patients who saw their provider 1 or fewer times per year (OR: 0.38, P<0.05).
A large proportion of patients (68.6%) were CKD unspecified by ICD‐9 codes, and only 27% of the unspecified group reported having CKD (Table 2). Examining the eGFR CKD stage of the CKD unspecified group showed that 22.8% were eGFR‐determined CKD stage 1 to 2, 57.1% were CKD stage 3, 14.8% were CKD stage 4, and 5.3% were CKD stage 5. Patients had 2 to 3 times greater odds of correct CKD self‐report if physicians had correctly identified their CKD stage (bivariable OR: 2.42, 95% confidence interval [CI]: 1.57‐3.72, multivariable OR: 3.22, 95% CI: 0.99‐10.46) (analysis not shown).
CKD Stage* | Physician (ICD‐9) Coded | eGFR Calculated | eGFR Coded With ICD‐9 Correct |
---|---|---|---|
| |||
Unspecified | 110 (27.2%) | ||
12 | 5 (31.2%) | 20 (17.4%) | 0 |
3 | 25 (27.2%) | 83 (27.7%) | 17 (29.8%) |
4 | 40 (63.5%) | 54 (48.2%) | 25 (69.4%) |
5 | 11 (78.6%) | 31 (63.3%) | 10 (83.3%) |
DISCUSSION
Although prior work has examined CKD awareness in the general population and in high‐risk cohorts,[2, 3, 13] this is the first study examining CKD awareness in an urban, underserved hospitalized population. We found that overall patients' CKD awareness was low (32%), but increased as high as 63% for CKD stage 5, even after controlling for patient demographic, clinical characteristics, and healthcare use. Our overall rate of CKD awareness was higher than prior studies overall and at lower CKD stages.[2, 3, 13] Our work is consistent with prior literature that shows increasing CKD awareness with advancing CKD stage.[2, 3, 13]
Older patients (>80 years) had lower awareness of CKD. Older patients are more likely to have a near normal creatinine, despite a markedly reduced eGFR, so their CKD may go unnoticed.[14] Even with appropriate recognition, providers may also feel like their CKD is unlikely to progress to ESRD, given its stability and/or their competing risk of death.[15] Finally, older hospitalized patients may also be less likely to report a CKD diagnosis due to difficulty in recall due to denial, dementia, or delirium.
One limitation is that our case‐finding for CKD was physician ICD‐9 coding, which is highly specific but not sensitive.[9] The majority of patients with physician‐identified CKD were CKD unspecified, perhaps due to poor coding, physician underdocumentation, or physician under‐recognition of CKD stage. Although only 27% of the CKD unspecified group correctly self‐identified as having CKD, over 75% were found to be CKD stage 3 or higher, which should trigger additional monitoring or care based on guidelines.[10] In addition, despite statistical significance, we may not be able to make meaningful inferences about our small other group (nonwhite, non‐African American). Our sample was from 1 hospitalan urban, academic, tertiary care center with a large proportion of African American patientswhich may limit generalizability. The multivariable model will need to be tested in other populations for reproducibility.
Our study significantly contributes to the literature by examining patient awareness of CKD in a high‐risk, urban, hospitalized minority population. Other study strengths include use of basic demographic information, as well as survey and laboratory data for a richer examination of the associations between patient factors and CKD awareness.
CONCLUSION
Hospitalized patients with CKD have a low CKD awareness. Patient awareness of their CKD is increased with physician documentation of CKD severity. Patient awareness of their CKD must be coupled with provider awareness and CKD documentation to link patients to multidisciplinary CKD education and care to slow CKD progression and reduce associated cardiovascular and metabolic complications. Further work is needed across hospitals to determineand improveCKD awareness among both patients and providers.
Disclosures
Dr. Saunders was supported by Pilot and Feasibility Funding from the Chicago Center for Diabetes Translation Research (NIDDK P30 DK092949). Dr. Chin was supported by NIDDK K24 DK071933. Dr. Meltzer was supported by NIA T35 AG029795. Dr. Saunders had full access to all of the study data and takes responsibility for the integrity of the data and accuracy of the data analysis. An abstract of this article was presented at the Society of Hospital Medicine Annual Meeting in Las Vegas, Nevada in March 2014 and at the Society of General Internal Medicine Annual Meeting in San Diego, California in April 2014. The authors report no conflicts of interest.
- United States Renal Data System. Annual Data Report: Atlas of Chronic Kidney Disease and End‐Stage Renal Disease in the United States. Bethesda, MD: National Institutes of Health; 2012.
- Chronic kidney disease awareness among individuals with clinical markers of kidney dysfunction. Clin J Am Soc Neph. 2011;6(8):1838–1844. , , , et al.
- Comparison of CKD awareness in a screening population using the Modification of Diet in Renal Disease (MDRD) Study and CKD Epidemiology Collaboration (CKD‐EPI) equations. Am J Kidney Dis. 2011;57(3 suppl 2):S17–S23. , , , et al.
- Preserving renal function in adults with hypertension and diabetes: a consensus approach. Am J Kidney Dis. 2000;36(3):646–661. , , , et al.
- Multidisciplinary predialysis education decreases the incidence of dialysis and reduces mortality—a controlled cohort study based on the NKFDOQI guidelines. Nephrol Dial Transplant. 2009;24(11):3426–3433. , , , et al.
- Pre‐dialysis hospital use and late referrals in incident dialysis patients in England: a retrospective cohort study. Nephrol Dial Transplant. 2015;30(1):124–129. , , .
- Do hospitals that provide heart failure patient education prior to discharge also promote continuity of care? A report from OPTIMIZE‐HF. J Card Fail. 2006;12(6 suppl):S111. , , , et al.
- Effects of physician experience on costs and outcomes on an academic general medicine service: results of a trial of hospitalists. Ann Intern Med. 2002;137(11):866–874. , , , et al.
- Failure of ICD‐9‐CM codes to identify patients with comorbid chronic kidney disease in diabetes. Health Serv Res. 2006;41(2):564–580. , , , et al.
- Validation of a telephone version of the mini‐mental state examination. J Am Geriatr Soc. 1992;40(7):697–702. , , , .
- National Kidney Foundation. Kidney disease outcomes quality initiative guidelines 2002. Available at: http://www2.kidney.org/professionals/KDOQI/guidelines_ckd/toc.htm. Accessed October 9, 2014.
- A more accurate method to estimate glomerular filtration rate from serum creatinine: a new prediction equation. Ann Intern Med. 1999;130(6):461–470. , , , , , .
- Prevalence and awareness of CKD among African Americans: the Jackson Heart Study. Am J Kidney Dis. 2009;53(2):238–247. , , , et al.
- Magnitude of underascertainment of impaired kidney function in older adults with normal serum creatinine. J Am Geriatr Soc. 2007;55(6):816–823. , , , , , .
- Prediction, Progression, and Outcomes of Chronic Kidney Disease in Older Adults. J Am Soc Neph. 2009;20(6):1199–1209. , , , et al.
- United States Renal Data System. Annual Data Report: Atlas of Chronic Kidney Disease and End‐Stage Renal Disease in the United States. Bethesda, MD: National Institutes of Health; 2012.
- Chronic kidney disease awareness among individuals with clinical markers of kidney dysfunction. Clin J Am Soc Neph. 2011;6(8):1838–1844. , , , et al.
- Comparison of CKD awareness in a screening population using the Modification of Diet in Renal Disease (MDRD) Study and CKD Epidemiology Collaboration (CKD‐EPI) equations. Am J Kidney Dis. 2011;57(3 suppl 2):S17–S23. , , , et al.
- Preserving renal function in adults with hypertension and diabetes: a consensus approach. Am J Kidney Dis. 2000;36(3):646–661. , , , et al.
- Multidisciplinary predialysis education decreases the incidence of dialysis and reduces mortality—a controlled cohort study based on the NKFDOQI guidelines. Nephrol Dial Transplant. 2009;24(11):3426–3433. , , , et al.
- Pre‐dialysis hospital use and late referrals in incident dialysis patients in England: a retrospective cohort study. Nephrol Dial Transplant. 2015;30(1):124–129. , , .
- Do hospitals that provide heart failure patient education prior to discharge also promote continuity of care? A report from OPTIMIZE‐HF. J Card Fail. 2006;12(6 suppl):S111. , , , et al.
- Effects of physician experience on costs and outcomes on an academic general medicine service: results of a trial of hospitalists. Ann Intern Med. 2002;137(11):866–874. , , , et al.
- Failure of ICD‐9‐CM codes to identify patients with comorbid chronic kidney disease in diabetes. Health Serv Res. 2006;41(2):564–580. , , , et al.
- Validation of a telephone version of the mini‐mental state examination. J Am Geriatr Soc. 1992;40(7):697–702. , , , .
- National Kidney Foundation. Kidney disease outcomes quality initiative guidelines 2002. Available at: http://www2.kidney.org/professionals/KDOQI/guidelines_ckd/toc.htm. Accessed October 9, 2014.
- A more accurate method to estimate glomerular filtration rate from serum creatinine: a new prediction equation. Ann Intern Med. 1999;130(6):461–470. , , , , , .
- Prevalence and awareness of CKD among African Americans: the Jackson Heart Study. Am J Kidney Dis. 2009;53(2):238–247. , , , et al.
- Magnitude of underascertainment of impaired kidney function in older adults with normal serum creatinine. J Am Geriatr Soc. 2007;55(6):816–823. , , , , , .
- Prediction, Progression, and Outcomes of Chronic Kidney Disease in Older Adults. J Am Soc Neph. 2009;20(6):1199–1209. , , , et al.
Research Agenda for Older Patient Care
Older adults with high levels of medical complexity occupy an increasing fraction of beds in acute‐care hospitals in the United States.[1, 2] By 2007, patients age 65 years and older accounted for nearly half of adult inpatient days of care.[1] These patients are commonly cared for by hospitalists who number more than 40,000.[3] Although hospitalists are most often trained in internal medicine, they have typically received limited formal geriatrics training. Increasingly, access to experts in geriatric medicine is limited.[4] Further, hospitalists and others who practice in acute care are limited by the lack of research to address the needs of the older adult population, specifically in the diagnosis and management of conditions encountered during acute illness.
To better support hospitalists in providing acute inpatient geriatric care, the Society of Hospital Medicine (SHM) partnered with the Association of Specialty Professors to develop a research agenda to bridge this gap. Using methodology from the James Lind Alliance (JLA) and the Patient Centered Outcomes Research Institute (PCORI), the SHM joined with older adult advocacy groups, professional societies of providers, and funders to create a geriatric‐focused acute‐care research agenda, highlighting 10 key research questions.[5, 6, 7] The goal of this approach was to produce and promote high integrity, evidence‐based information that comes from research guided by patients, caregivers, and the broader healthcare community.[8] In this article, we describe the methodology and results of this agenda‐setting process, referred to as the Acute Care of Older Patients (ACOP) Priority Setting Partnership.
METHODS
Overview
This project focused on topic generation, the first step in the PCORI framework for identification and prioritization of research areas.[5] We employed a specific and defined methodology to elicit and prioritize potential research topics incorporating input from representatives of older patients, family caregivers, and healthcare providers.[6]
To elicit this input, we chose a collaborative and consultative approach to stakeholder engagement, drawing heavily from the published work of the JLA, an initiative promoting patient‐clinician partnerships in health research developed in the United Kingdom.[6] We previously described the approach elsewhere.[7]
The ACOP process for determining the research agenda consisted of 4 steps: (1) convene, (2) consult, (3) collate, and (4) prioritize.[6] Through these steps, detailed below, we were able to obtain input from a broad group of stakeholders and engage the stakeholders in a process of reducing and refining our research questions.
Convene
The steering committee (the article's authors) convened a stakeholder partnership group that included stakeholders representing patients and caregivers, advocacy organizations for the elderly, organizations that address diseases and conditions common among hospitalized older patients, provider professional societies (eg, hospitalists, subspecialists, and nurses and social workers), payers, and funders. Patient, caregiver, and advocacy organizations were identified based on their engagement in aging and health policy advocacy by SHM staff and 1 author who had completed a Health and Aging Policy Fellowship (H.L.W.).
The steering committee issued e‐mail invitations to stakeholder organizations, making initial inquiries through professional staff and relevant committee chairs. Second inquiries were made via e‐mail to each organization's volunteer leadership. We developed a webinar that outlined the overall research agenda setting process and distributed the webinar to all stakeholders. The stakeholder organizations were asked to commit to (1) surveying their memberships and (2) participating actively in prioritization by e‐mail and at a 1‐day meeting in Washington DC.
Consult
Each stakeholder organization conducted a survey of its membership via an Internet‐based survey in the summer of 2013 (see Supporting Information, Appendix A, in the online version of this article). Stakeholder organizations were asked to provide up to 75 survey responses each. Though a standard survey was used, the steering committee was not prescriptive in the methodology of survey distribution to accommodate the structure and communication methods of the individual stakeholder organizations. Survey respondents were asked to identify up to 5 unanswered questions relevant to the acute care of older persons and also provide demographic information.
Collate
In the collating process, we clarified and categorized the unanswered questions submitted in the individual surveys. Each question was initially reviewed by a member of the steering committee, using explicit criteria (see Supporting Information, Appendix B, in the online version of this article). Questions that did not meet all 4 criteria were removed. For questions that met all criteria, we clarified language, combined similar questions, and categorized each question. Categories were created in a grounded process, in which individual reviewers assigned categories based on the content of the questions. Each question could be assigned to up to 2 categories. Each question was then reviewed by a second member of the steering committee using the same 4 criteria. As part of this review, similar questions were consolidated, and when possible, questions were rewritten in a standard format.[6]
Finally, the steering committee reviewed previously published research agendas looking for additional relevant unanswered questions, specifically the New Frontiers Research Agenda created by the American Geriatrics Society in conjunction with participating subspecialty societies,[9] the Cochrane Library, and other systematic reviews identified in the literature via PubMed search.[10, 11, 12, 13, 14, 15]
Prioritize
The resulting list of unanswered questions was prioritized in 2 phases. First, the list was e‐mailed to all stakeholder organizations. The organizations were asked to vote on their top 10 priorities from this list using an online ballot, assigning 10 points to their highest priority down to 1 point for their lowest priority. In so doing, they were asked to consider explicit criteria (see Supporting Information, Appendix B, in the online version of this article). Each organization had only 1 ballot and could arrive at their top 10 list in any manner they wished. The balloting from this phase was used to develop a list of unanswered questions for the second round of in‐person prioritization. Each priority's scores were totaled across all voting organizations. The 29 priorities with the highest point totals were brought to the final prioritization round because of a natural cut point at priority number 29, rather than number 30.
For the final prioritization round, the steering committee facilitated an in‐person meeting in Washington, DC in October 2013 using nominal group technique (NGT) methodologies to arrive at consensus.[16] During this process stakeholders were asked to consider additional criteria (see Supporting Information, Appendix B, in the online version of this article).
RESULTS
Table 1 lists the organizations who engaged in 1 or more parts of the topic generation process. Eighteen stakeholder organizations agreed to participate in the convening process. Ten organizations did not respond to our solicitation and 1 declined to participate.
Organization (N=18) | Consultation % of Survey Responses (N=580) | Prioritization Round 1 | Prioritization Round 2 |
---|---|---|---|
Alzheimer's Association | 7.0% | Yes | Yes |
American Academy of Neurology | 3.4% | Yes | Yes |
American Association of Retired Persons | 0.8% | No | No |
American College of Cardiology | 11.4% | Yes | Yes |
American College of Emergency Physicians | 1.3% | No | No |
American College of Surgeons | 1.0% | Yes | Yes |
American Geriatrics Society | 7.6% | Yes | Yes |
American Hospital Association | 1.7% | Yes | No |
Centers for Medicare & Medicaid Services | 0.8% | Yes | Yes |
Gerontological Society of America | 18.9% | Yes | Yes |
National Alliance for Caregiving | 1.0% | Yes | Yes |
National Association of Social Workers | 5.9% | Yes | Yes |
National Coalition for Healthcare | 0.6% | No | No |
National Institute on Aging | 2.1% | Yes | Yes |
National Partnership for Women and Families | 0.0% | Yes | Yes |
Nursing Improving Care for Healthsystem Elders | 28.6% | Yes | No |
Society of Critical Care Medicine | 12.0% | Yes | Yes |
Society of Hospital Medicine | 4.6% | Yes | Yes |
Seventeen stakeholder organizations obtained survey responses from a total of 580 individuals (range, 3150 per organization), who were asked to identify important unanswered questions in the acute care of older persons. Survey respondents were typically female (77%), white (85%), aged 45 to 65 years (65%), and identified themselves as health professionals (90%). Twenty‐six percent of respondents also identified as patients or family caregivers. Their surveys included 1299 individual questions.
Figure 1 summarizes our collation and prioritization process and reports the numbers of questions resulting at each stage. Nine hundred nineteen questions were removed during the first review conducted by steering committee members, and 31 question categories were identified. An additional 305 questions were removed in the second review, with 75 questions remaining. As the final step of the collating process, literature review identified 39 relevant questions not already suggested or moved forward through our consultation and collation process. These questions were added to the list of unanswered questions.
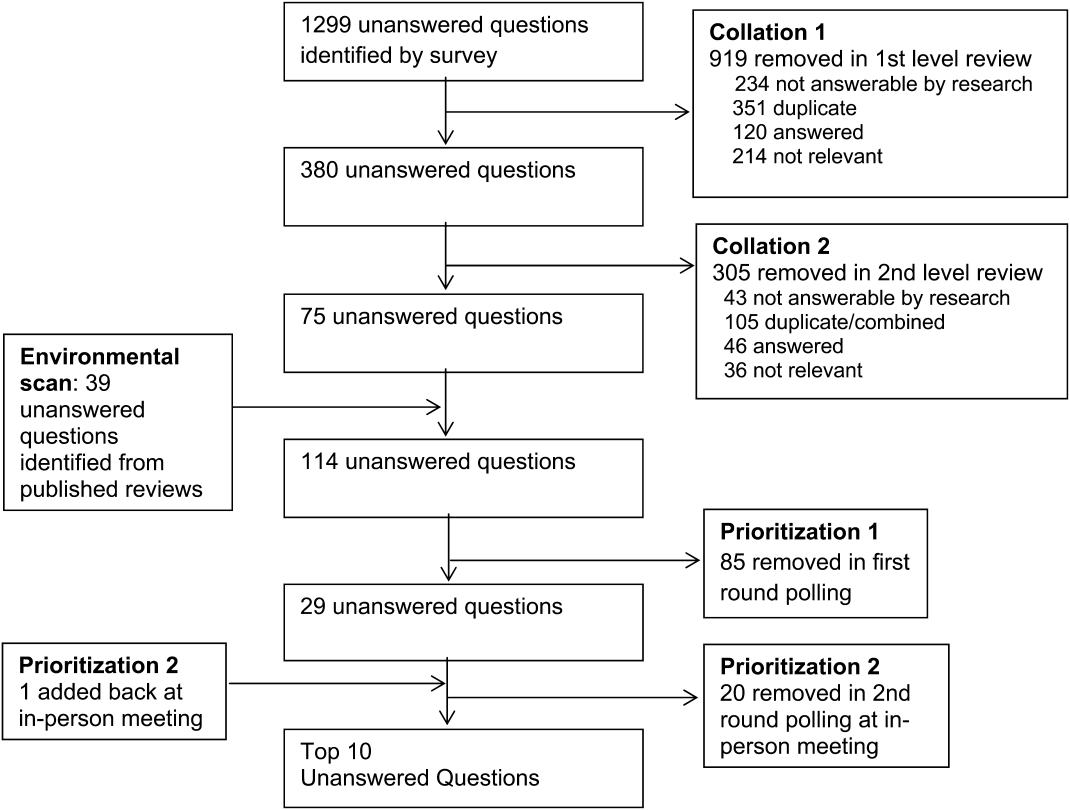
In the first round of prioritization, this list of 114 questions was emailed to each stakeholder organization (Table 1). After the stakeholder voting process was completed, 29 unanswered questions remained (see Supporting Information, Appendix C, in the online version of this article). These questions were refined and prioritized in the in‐person meeting to create the final list of 10 questions. The stakeholders present in the meeting represented 13 organizations (Table 1). Using the NGT with several rounds of small group breakouts and large group deliberation, 9 of the top 10 questions were selected from the list of 29. One additional highly relevant question that had been removed earlier in the collation process regarding workforce was added back by the stakeholder group.
This prioritized research agenda appears in Table 2 and below, organized alphabetically by topic.
- Advanced care planning: What approaches for determining and communicating goals of care across and within healthcare settings are most effective in promoting goal‐concordant care for hospitalized older patients?
- Care transitions: What is the comparative effectiveness of transitional care models on patient‐centered outcomes for hospitalized older adults?
- Delirium: What practices are most effective for consistent recognition, prevention, and treatment of delirium subtypes among hospitalized older adults?
- Dementia: Does universal assessment of hospitalized older adults for cognitive impairment (eg, at presentation and/or discharge) lead to more appropriate application of geriatric care principles and improve patient‐centered outcomes?
- Depression: Does identifying depressive symptoms during a hospital stay and initiating a therapeutic plan prior to discharge improve patient‐centered and/or disease‐specific outcomes?
- Medications: What systems interventions improve medication management for older adults (ie, appropriateness of medication choices and dosing, compliance, cost) in the hospital and postacute care?
- Models of care: For which populations of hospitalized older adults does systematic implementation of geriatric care principles/processes improve patient‐centered outcomes?
- Physical function: What is the comparative effectiveness of interventions that promote in‐hospital mobility, improve and preserve physical function, and reduce falls among older hospitalized patients?
- Surgery: What perioperative strategies can be used to optimize care processes and improve outcomes in older surgical patients?
- Training: What is the most effective approach to training hospital‐based providers in geriatric and palliative care competencies?
Topic | Scope of Problem | What Is known | Unanswered Question | Proposed Dimensions |
---|---|---|---|---|
| ||||
Advanced‐care planning | Older persons who lack decision‐making capacity often do not have surrogates or clear goals of care documented.[19] Advanced‐care directives are associated with an increase in patient autonomy and empowerment, and although 15% to 25% of adults completed the documentation in 2004,[20] a recent study found completion rates have increased to 72%.[21] | Nursing home residents with advanced directives are less likely to be hospitalized.[22, 23] Advanced directive tools, such as POLST, work to translate patient preferences to medical order.[24] standardized patient transfer tools may help to improve transitions between nursing homes and hospitals.[25] However, advanced care planning fails to integrate into courses of care if providers are unwilling or unskilled in using advanced care documentation.[26] | What approaches for determining and communicating goals of care across and within healthcare settings are most effective in promoting goal‐concordant care for hospitalized older patients? | Potential interventions: |
Decision aids | ||||
Standard interdisciplinary advanced care planning approach | ||||
Patient advocates | ||||
Potential outcomes might include: | ||||
Completion of advanced directives and healthcare power of attorney | ||||
Patient‐centered outcomesa | ||||
Care transitions | Hospital readmission from home and skilled nursing facilities occurs within 30 days in up to a quarter of patients.[27, 28] The discharge of complex older hospitalized patients is fraught with challenges. The quality of the hospital discharge process can influence outcomes for vulnerable older patients.[29, 30, 31, 32] Studies measuring the quality of hospital discharge frequently find deficits in documentation of assessment of geriatric syndromes,[33] poor patient/caregiver understanding,[34, 35] and poor communication and follow‐up with postacute providers.[35, 36, 37, 38] | As many as 10 separate domains may influence the success of a discharge.[39] There is limited evidence, regarding quality‐of‐care transitions for hospitalized older patients. The Coordinated‐Transitional Care Program found that follow‐up with telecommunication decreased readmission rates and improved transitional care for a high‐risk condition veteran population.[40] There is modest evidence for single interventions,[41] whereas the most effective hospital‐to‐community care interventions address multiple processes in nongeriatric populations.[39, 42, 43] | What is the comparative effectiveness of the transitional care models on patient‐centered outcomes for hospitalized older adults? | Possible models: |
Established vs novel care‐transition models | ||||
Disease‐specific vs general approaches | ||||
Accountable care models | ||||
Caregiver and family engagement | ||||
Community engagement | ||||
Populations of interest: | ||||
Patients with dementia | ||||
Patients with multimorbidity | ||||
Patients with geriatric syndromes | ||||
Patients with psychiatric disease | ||||
Racially and ethnically diverse patients | ||||
Outcomes: | ||||
Readmission | ||||
Other adverse events | ||||
Cost and healthcare utilization | ||||
Patient‐centered outcomesa | ||||
Delirium | Among older inpatients, the prevalence of delirium varies with severity of illness. Among general medical patients, in‐hospital prevalence ranges from 10% to 25 %.[44, 45] In the ICU, prevalence estimates are higher, ranging from 25% to as high as 80%.[46, 47] Delirium independently predicts increased length of stay,[48, 49] long‐term cognitive impairment,[50, 51] functional decline,[51] institutionalization,[52] and short‐ and long‐term mortality.[52, 53, 54] | Multicomponent strategies have been shown to be effective in preventing delirium. A systematic review of 19 such interventions identified the most commonly included such as[55]: early mobilization, nutrition supplements, medication review, pain management, sleep enhancement, vision/hearing protocols, and specialized geriatric care. Studies have included general medical patients, postoperative patients, and patients in the ICU. The majority of these studies found reductions in either delirium incidence (including postoperative), delirium prevalence, or delirium duration. Although medications have not been effective in treating delirium in general medical patients,[48] the choice and dose of sedative agents has been shown to impact delirium in the ICU.[56, 57, 58] | What practices are most effective for consistent recognition, prevention, and treatment of delirium subtypes (hypoactive, hyperactive, and mixed) among hospitalized older adults? | Outcomes to examine: |
Delirium incidence (including postoperative) | ||||
Delirium duration | ||||
Delirium‐/coma‐free days | ||||
Delirium prevalence at discharge | ||||
Subsyndromal delirium | ||||
Potential prevention and treatment modalities: | ||||
Family education or psychosocial interventions | ||||
Pharmacologic interventions | ||||
Environmental modifications | ||||
Possible areas of focus: | ||||
Special populations | ||||
Patients with varying stages of dementia | ||||
Patients with multimorbidity | ||||
Patients with geriatric syndromes | ||||
Observation patients | ||||
Diverse settings | ||||
Emergency department | ||||
Perioperative | ||||
Skilled nursing/rehab/long‐term acute‐care facilities | ||||
Dementia | 13% to 63% of older persons in the hospital have dementia.[59] Dementia is often unrecognized among hospitalized patients.[60] The presence of dementia is associated with a more rapid functional decline during admission and delayed hospital discharge.[59] Patients with dementia require more nursing hours, and are more likely to have complications[61] or die in care homes rather than in their preferred site.[59] | Several tools have been validated to screen for dementia in the hospital setting.[62] Studies have assessed approaches to diagnosing delirium in hospitalized patients with dementia.[63] Cognitive and functional stimulation interventions may have a positive impact on reducing behavioral issues.[64, 65] | Does universal assessment of hospitalized older adults for cognitive impairment (eg, at presentation and/or discharge) lead to more appropriate application of geriatric care principles and improve patient centered outcomes? | Potential interventions: |
Dementia or delirium care | ||||
Patient/family communication and engagement strategies | ||||
Maintenance/recovery of independent functional status | ||||
Potential outcomes: | ||||
Patient‐centered outcomesa | ||||
Length of stay, cost, and healthcare utilization (including palliative care) | ||||
Immediate invasive vs early conservative treatments pursued | ||||
Depression | Depression is a common geriatric syndrome among acutely ill older patients, occurring in up to 45% of patients.[66, 67] Rates of depression are similar among patients discharged following a critical illness, with somatic, rather than cognitive‐affective complaints being the most prevalent.[68] Depression among inpatients or immediately following hospitalization independently predicts worse functional outcomes,[69] cognitive decline,[70] hospital readmission,[71, 72] and long‐term mortality.[69, 73] Finally, geriatric patients are known to respond differently to medical treatment.[74, 75] | Although highly prevalent, depression is poorly recognized and managed in the inpatient setting. Depression is recognized in only 50% of patients, with previously undiagnosed or untreated depression being at highest risk for being missed.[76] The role of treatment of depression in the inpatient setting is poorly understood, particularly for those with newly recognized depression or depressive symptoms. Some novel collaborative care and telephone outreach programs have led to increases in depression treatment in patients with specific medical and surgical conditions, resulting in early promising mental health and comorbid outcomes.[77, 78] The efficacy of such programs for older patients is unknown. | Does identifying depressive symptoms during a hospital stay and initiating a therapeutic plan prior to discharge improve patient‐centered and/or disease‐specific outcomes? | Possible areas of focus: |
Comprehensive geriatric and psychosocial assessment; | ||||
Inpatient vs outpatient initiation of pharmacological therapy | ||||
Integration of confusion assessment method into therapeutic approaches | ||||
Linkages with outpatient mental health resources | ||||
Medications | Medication exposure, particularly potentially inappropriate medications, is common in hospitalized elders.[79] Medication errorsof dosage, type, and discrepancy between what a patient takes at home and what is known to his/her prescribing physicianare common and adversely affects patient safety.[80] Geriatric populations are disproportionately affected, especially those taking more than 5 prescription medications per day.[81] | Numerous strategies including electronic alerts, screening protocols, and potentially inappropriate medication lists (Beers list, STOPP) exist, though the optimal strategies to limit the use of potentially inappropriate medications is not yet known.[82, 83, 84] | What systems interventions improve medication management for older adults (ie, appropriateness of medication choices and dosing, compliance, cost) in hospital and post‐acute care? | Possible areas of focus: |
Use of healthcare information technology | ||||
Communication across sites of care | ||||
Reducing medication‐related adverse events | ||||
Engagement of family caregivers | ||||
Patient‐centered strategies to simplify regimens | ||||
Models of care | Hospitalization marks a time of high risk for older patients. Up to half die during hospitalization or within the year following the hospitalization. There is high risk of nosocomial events, and more than a third experience a decline in health resulting in longer hospitalizations and/or placement in extended‐care facilities.[73, 85, 86] | Comprehensive inpatient care for older adults (acute care for elders units, geriatric evaluation and management units, geriatric consultation services) were studied in 2 meta‐analyses, 5 RCTs, and 1 quasiexperimental study and summarized in a systematic review.[87] The studies reported improved quality of care (1 of 1 article), quality of life (3 of 4), functional autonomy (5 of 6), survival (3 of 6), and equal or lower healthcare utilization (7 of 8). | For which populations of hospitalized older adults does systematic implementation of geriatric care principles/processes improve patient‐centered outcomes? | Potential populations: |
Patients of the emergency department, critical care, perioperative, and targeted medical/surgical units | ||||
Examples of care principles: | ||||
Geriatric assessment, early mobility, medication management, delirium prevention, advanced‐care planning, risk‐factor modification, caregiver engagement | ||||
Potential outcomes: | ||||
Patient‐centered outcomesa | ||||
Cost | ||||
Physical function | Half of older patients will lose functional capacity during hospitalization.[88] Loss of physical function, particularly of lower extremities, is a risk factor for nursing home placement.[89, 90] Older hospitalized patients spend the majority (up to 80%) of their time lying in bed, even when they are capable of walking independently.[91] | Loss of independences with ADL capabilities is associated with longer hospital stays, higher readmission rates, and higher mortality risk.[92] Excessive time in bed during a hospital stay is also associated with falls.[93] Often, hospital nursing protocols and physician orders increase in‐hospital immobility in patients.[91, 94] However, nursing‐driven mobility protocols can improve functional outcomes of older hospitalized patients.[95, 96] | What is the comparative effectiveness of interventions that promote in‐hospital mobility, improve and preserve physical function, and reduce falls among older hospitalized patients? | Potential interventions: |
Intensive physical therapy | ||||
Incidental functional training | ||||
Restraint reduction | ||||
Medication management | ||||
Potential outcomes: | ||||
Discharge location | ||||
Delirium, pressure ulcers, and falls | ||||
Surgery | An increasing number of persons over age 65 years are undergoing surgical procedures.[97] These persons are at increased risk for developing delirium/cogitative dysfunction,[98] loss of functional status,[99] and exacerbations of chronic illness.[97] Additionally, pain management may be harder to address in this population.[100] Current outcomes may not reflect the clinical needs of elder surgical patients.[101] | Tailored drug selection and nursing protocols may prevent delirium.[98] Postoperative cognitive dysfunction may require weeks for resolution. Identifying frail patients preoperatively may lead to more appropriate risk stratification and improved surgical outcomes.[99] Pain management strategies focused on mitigating cognitive impact and other effects may also be beneficial.[100] Development of risk‐adjustment tools specific to older populations, as well as measures of frailty and patient‐centered care, have been proposed.[101] | What perioperative strategies can be used to optimize care processes and improve outcomes in older surgical patients? | Potential strategies: |
Preoperative risk assessment and optimization for frail or multimorbid older patients | ||||
Perioperative management protocols for frail or multimorbid older patients | ||||
Potential outcomes: | ||||
Postoperative patient centered outcomesa | ||||
Perioperative cost, healthcare utilization | ||||
Training | Adults over age 65 years comprise 13.2 % of the US population, but account for >30% of hospital discharges and 50% of hospital days.[86, 102, 103] By 2030, there will only be 1 geriatrician for every 3798 Americans >75 years.[4] Between 1997 and 2006, the odds that a hospitalist would treat a hospitalized Medicare patient rose 29% per year.[3] | Train the trainer programs for physicians include the CHAMP, the AGESP, and the PAGE. Education for nurses include the NICHE. Outcomes include improved self‐confidence, attitudes, teaching skills, and geriatric care environment.[104, 105, 106] | What is the most effective approach to training hospital‐based providers in geriatric and palliative care competencies? | Potential interventions: |
Mentored implementation | ||||
Train the trainer | ||||
Technical support |
Table 2 also contains a capsule summary of the scope of the problem addressed by each research priority, a capsule summary of related work in the content area (what is known) not intended as a systematic review, and proposed dimensions or subquestions suggested by the stakeholders at the final prioritization meeting
DISCUSSION
Older hospitalized patients account for an increasing number and proportion of hospitalized patients,[1, 2] and hospitalists increasingly are responsible for inpatient care for this population.[3] The knowledge required for hospitalists to deliver optimal care and improve outcomes has not kept pace with the rapid growth of either hospitalists or hospitalized elders. Through a rigorous prioritization process, we identified 10 areas that deserve the highest priority in directing future research efforts to improve care for the older hospitalized patient. Assessment, prevention, and treatment of geriatric syndromes in the hospital account for almost half of the priority areas. Additional research is needed to improve advanced care planning, develop new care models, and develop training models for future hospitalists competent in geriatric and palliative care competencies.
A decade ago, the American Geriatric Society and the John A. Hartford Foundation embarked upon a research agenda aimed at improving the care of hospitalized elders cared for by specialists (ie, New Frontiers in Geriatrics Research: An Agenda for Surgical and Related Medical Specialties).[9] This effort differed in many important ways from the current priortization process. First, the New Frontiers agenda focused upon specific diseases, whereas the ACOP agenda addresses geriatric syndromes that cut across multiple diseases. Second, the New Frontiers agenda was made by researchers and based upon published literature, whereas the ACOP agenda involved the input of multiple stakeholders. Finally, the New Frontiers prioritized a research agenda across a number of surgical specialties, emergency medicine, and geriatric rehabilitation. Hospital medicine, however, was still early in its development and was not considered a unique specialty. Since that time, hospital medicine has matured into a unique specialty, with increased numbers of hospitalists,[3] increased research in hospital medicine,[17] and a separate recertification pathway for internal medicine licensure.[18] To date, there has not been a similar effort performed to direct geriatric research efforts for hospital medicine.
For researchers working in the field of hospital medicine, this list of topics has several implications. First, as hospitalists are commonly generalists, hospitalist researchers may be particularly well‐suited to study syndromes that cut across specialties. However, this does raise concerns about funding sources, as most National Institutes of Health institutes are disease‐focused. Funders that are not disease‐focused such as PCORI, National Institute on Aging, National Institute of Nursing Research, and Agency for Healthcare Research and Quality, and private foundations (Hartford, Robert Wood Johnson, and Commonwealth) may be more fruitful sources of funding for this work, but funding may be challenging. Nonetheless, the increased focus on patient‐centered work may increase funders' interest in such work. Second, the topics on this list would suggest that interventions will not be pharmacologic, but will focus on nonpharmacologic, behavioral, and social interventions. Similarly, outcomes of interest must expand beyond utilization metrics such as length of stay and mortality, to include functional status and symptom management, and goal‐concordant care. Therefore, research in geriatric acute care will necessarily be multidisciplinary.
Although these 10 high‐priority areas have been selected, this prioritized list is inherently limited by our methodology. First, our survey question was not focused on a disease state, and this wording may have resulted in the list favoring geriatric syndromes rather than common disease processes. Additionally, the resulting questions encompass large research areas and not specific questions about discrete interventions. Our results may also have been skewed by the types of engaged respondents who participated in the consultation, collating, and prioritization phases. In particular, we had a large response from geriatric medicine nurses, whereas some stakeholder groups provided no survey responses. Thus, these respondents were not representative of all possible stakeholders, nor were the survey respondents necessarily representative of each of their organizations. Nonetheless, the participants self‐identified as representative of diverse viewpoints that included patients, caregivers, and advocacy groups, with the majority of stakeholder organizations remaining engaged through the completion of the process. Thus, the general nature of this agenda helps us focus upon larger areas of importance, leaving researchers the flexibility to choose to narrow the focus on a specific research question that may include potential interventions and unique outcomes. Finally, our methodology may have inadvertently limited the number of patient and family caregiver voices in the process given our approach to large advocacy groups, our desire to be inclusive of healthcare professional organizations, and our survey methodology. Other methodologies may have reached more patients and caregivers, yet many healthcare professionals have served as family caregivers to frail elders requiring hospitalization and may have been in an ideal position to answer the survey.
In conclusion, several forces are shaping the future of acute inpatient care. These include the changing demographics of the hospitalized patient population, a rapid increase in the proportion of multimorbid hospitalized older adults, an inpatient workforce (hospitalists, generalists, and subspecialists) with potentially limited geriatrics training, and gaps in evidence‐based guidance to inform diagnostic and therapeutic decision making for acutely ill older patients. Training programs in hospital medicine should be aware of and could benefit from the resulting list of unanswered questions. Our findings also have implications for training to enrich education in geriatrics. Moreover, there is growing recognition that patients and other stakeholders deserve a greater voice in determining the direction of research. In addition to efforts to improve patient‐centeredness of research, these areas have been uniquely identified by stakeholders as important, and therefore are in line with newer priorities of PCORI. This project followed a road map resulting in a patient‐centered research agenda at the intersection of hospital medicine and geriatric medicine.[7] In creating this agenda, we relied heavily on the framework proposed by PCORI. We propose to pursue a dissemination and evaluation strategy for this research agenda as well as additional prioritization steps. We believe the adoption of this methodology will create a knowledge base that is rigorously derived and most relevant to the care of hospitalized older adults and their families. Its application will ultimately result in improved outcomes for hospitalized older adults.
Acknowledgements
The authors acknowledge Claudia Stahl, Society of Hospital Medicine; Cynthia Drake, University of Colorado; and the ACOP stakeholder organizations.
Disclosures: This work was supported by the Association of Specialty Professors/American Society of Internal Medicine and the John A. Hartford Foundation. Dr. Vasilevskis was supported by the National Institute on Aging of the National Institutes of Health under award number K23AG040157 and the Veterans Affairs Clinical Research Center of Excellence, and the Geriatric Research, Education and Clinical Center (GRECC). Dr. Vasilevskis' institution receives grant funding for an aspect of submitted work. Dr. Meltzer is a PCORI Methodology Committee member. The content is solely the responsibility of the authors and does not necessarily represent the official views of the National Institutes of Health or the Department of Veterans' Affairs. The authors report no conflicts of interest.
- National Hospital Discharge Survey: 2007 summary. Natl Health Stat Report. 2010(29):1–20, 24. , , , , .
- Centers for Medicare 2012. Available at: http://www.cms.gov/Research‐Statistics‐Data‐and‐Systems/Statistics‐Trends‐and‐Reports/Chronic‐Conditions/Downloads/2012Chartbook.pdf. Accessed December 12, 2014.
- Growth in the care of older patients by hospitalists in the United States. N Engl J Med. 2009;360(11):1102–1112. , , , .
- A revelation of numbers: will America's eldercare workforce be ready to care for an aging America? Generations. 2010;34(4):11–19. , .
- Patient‐Centered Outcomes Research Institute Methodology Committee. The PCORI methodology report. Available at: http://www.pcori.org/assets/2013/11/PCORI‐Methodology‐Report.pdf. Published November 2013. Accessed December 19, 2013.
- The James Lind Alliance. JLA method. Available at: http://www.lindalliance.org/JLA_Method.asp. Accessed December 19, 2013.
- Road map to a patient‐centered research agenda at the intersection of hospital medicine and geriatric medicine. J Gen Intern Med. 2014;29(6):926–931. , , , , .
- Patient‐Centered Outcomes Research Institute. About us. Available at: http://www.pcori.org/about‐us. Accessed February 23, 2015.
- New Frontiers of Geriatrics Research: An Agenda for Surgical and Related Medical Specialties. New York, NY: American Geriatrics Society; 2004. , , .
- Linking the NIH strategic plan to the research agenda for social workers in health and aging. J Gerontol Soc Work. 2010;53(1):77–93. , , , .
- Assessing the capacity to make everyday decisions: a guide for clinicians and an agenda for future research. Am J Geriatr Psychiatry. 2007;15(2):101–111. , .
- Practitioners' views on elder mistreatment research priorities: recommendations from a Research‐to‐Practice Consensus conference. J Elder Abuse Negl. 2011;23(2):115–126. , , , et al.
- The intersection between geriatrics and palliative care: a call for a new research agenda. J Am Geriatr Soc. 2005;53(9):1593–1598. , .
- The cancer aging interface: a research agenda. J Clin Oncol. 2007;25(14):1945–1948. .
- Clinical care of persons with dementia in the emergency department: a review of the literature and agenda for research. J Am Geriatr Soc. 2012;60(9):1742–1748. , , , .
- A group process model for problem identification and program planning. J Appl Behav Sci. 1971;7(4):466–492. , .
- Research and publication trends in hospital medicine. J Hosp Med. 2014;9(3):148–154. , , , , .
- ABFM and ABIM to jointly participate in recognition of focused practice (rfp) in hospital medicine pilot approved by abms. Ann Fam Med. 2010;8(1):87. .
- Medical decision‐making for older adults without family. J Am Geriatr Soc. 2012;60(11):2144–2150. , , , .
- Promoting advance directives among elderly primary care patients. J Gen Intern Med. 2004;19(9):944–951. , , , , , .
- Advance directive completion by elderly Americans: a decade of change. J Am Geriatr Soc. 2014;62(4):706–710. , , .
- Care transitions by older adults from nursing homes to hospitals: implications for long‐term care practice, geriatrics education, and research. J Am Med Dir Assoc. 2010;11(4):231–238. , .
- Decisions to hospitalize nursing home residents dying with advanced dementia. J Am Geriatr Soc. 2005;53(8):1396–1401. , , , .
- A comparison of methods to communicate treatment preferences in nursing facilities: traditional practices versus the physician orders for life‐sustaining treatment program. J Am Geriatr Soc. 2010;58(7):1241–1248. , , , , , .
- Interventions to improve transitional care between nursing homes and hospitals: a systematic review. J Am Geriatr Soc. 2010;58(4):777–782. , , , , .
- Opening end‐of‐life discussions: how to introduce Voicing My CHOiCES, an advance care planning guide for adolescents and young adults [published online ahead of print March 13, 2014]. Palliat Support Care. doi: 10.1017/S1478951514000054. , , .
- The revolving door of rehospitalization from skilled nursing facilities. Health Aff (Millwood). 2010;29(1):57–64. , , , .
- Rehospitalizations among patients in the Medicare fee‐for‐service program. N Engl J Med. 2009;360(14):1418–1428. , , .
- Predictors of rehospitalization among elderly patients admitted to a rehabilitation hospital: the role of polypharmacy, functional status, and length of stay. J Am Med Dir Assoc. 2013;14(10):761–767. , , , et al.
- Mobility after hospital discharge as a marker for 30‐day readmission. J Gerontol A Biol Sci Med Sci. 2013;68(7):805–810. , , , et al.
- The association between the quality of inpatient care and early readmission: a meta‐analysis of the evidence. Med Care. 1997;35(10):1044–1059. , , , , .
- Association of impaired functional status at hospital discharge and subsequent rehospitalization. J Hosp Med. May 2014;9(5):277–282. , , , , , .
- A prospective cohort study of geriatric syndromes among older medical patients admitted to acute care hospitals. J Am Geriatr Soc. 2011;59(11):2001–2008. , , , , , .
- Hospital discharge instructions: comprehension and compliance among older adults. J Gen Intern Med. 2014;29(11):1491–1498. , , , et al.
- Problems after discharge and understanding of communication with their primary care physicians among hospitalized seniors: a mixed methods study. J Hosp Med. 2010;5(7):385–391. , , , et al.
- Communication and information deficits in patients discharged to rehabilitation facilities: an evaluation of five acute care hospitals. J Hosp Med. 2009;4(8):E28–E33. , , , et al.
- The consequences of poor communication during transitions from hospital to skilled nursing facility: a qualitative study. J Am Geriatr Soc. 2013;61(7):1095–1102. , , , , , .
- Deficits in communication and information transfer between hospital‐based and primary care physicians: implications for patient safety and continuity of care. JAMA. 2007;297(8):831–841. , , , , , .
- Moving beyond readmission penalties: creating an ideal process to improve transitional care. J Hosp Med. 2013;8(2):102–109. , , , .
- Low‐cost transitional care with nurse managers making mostly phone contact with patients cut rehospitalization at a VA hospital. Health Aff (Millwood). 2012;31(12):2659–2668. , , , et al.
- Transitional care interventions to prevent readmissions for persons with heart failure: a systematic review and meta‐analysis. Ann Intern Med. 2014;160(11):774–784. , , , et al.
- Interventions to reduce 30‐day rehospitalization: a systematic review. Ann Intern Med. 2011;155(8):520–528. , , , , .
- Hospital‐initiated transitional care interventions as a patient safety strategy: a systematic review. Ann Intern Med. 2013;158(5 pt 2):433–440. , , , , , .
- Epidemiology and risk factors for delirium across hospital settings. Best Pract Res Clin Anaesthesiol. 2012;26(3):277–287. , , , .
- Does delirium contribute to poor hospital outcomes? A three‐site epidemiologic study. J Gen Intern Med. 1998;13(4):234–242. , , , , .
- Incidence, risk factors and consequences of ICU delirium. Intensive Care Med. 2007;33(1):66–73. , , , .
- Delirium in mechanically ventilated patients: validity and reliability of the confusion assessment method for the intensive care unit (CAM‐ICU). JAMA. 2001;286(21):2703–2710. , , , et al.
- Impact and recognition of cognitive impairment among hospitalized elders. J Hosp Med. 2010;5(2):69–75. , , , et al.
- The impact of delirium in the intensive care unit on hospital length of stay. Intensive Care Med. 2001;27(12):1892–1900. , , , et al.
- Long‐term cognitive impairment after critical illness. N Engl J Med. 2014;370(2):185–186. , , .
- Delirium in the ICU and subsequent long‐term disability among survivors of mechanical ventilation. Crit Care Med. 2014;42(2):369–377. , , , et al.
- Delirium in elderly patients and the risk of postdischarge mortality, institutionalization, and dementia: a meta‐analysis. JAMA. 2010;304(4):443–451. , , , , , .
- Delirium as a predictor of mortality in mechanically ventilated patients in the intensive care unit. JAMA. 2004;291(14):1753–1762. , , , et al.
- Days of delirium are associated with 1‐year mortality in an older intensive care unit population. Am J Respir Crit Care Med. 2009;180(11):1092–1097. , , , , , .
- In‐facility delirium prevention programs as a patient safety strategy: a systematic review. Ann Intern Med. 2013;158(5 pt 2):375–380. , .
- Dexmedetomidine vs midazolam for sedation of critically ill patients: a randomized trial. JAMA. 2009;301(5):489–499. , , , et al.
- Effect of sedation with dexmedetomidine vs lorazepam on acute brain dysfunction in mechanically ventilated patients: the MENDS randomized controlled trial. JAMA. 2007;298(22):2644–2653. , , , et al.
- Protocolized intensive care unit management of analgesia, sedation, and delirium improves analgesia and subsyndromal delirium rates. Anesth Analg. 2010;111(2):451–463. , , , , , .
- A systematic review of the prevalence, associations and outcomes of dementia in older general hospital inpatients. Int Psychogeriatr. 2011;23(3):344–355. , .
- Cognitive impairment is undetected in medical inpatients: a study of mortality and recognition amongst healthcare professionals. BMC Geriatr. 2012;12:47. , , , .
- How can we keep patients with dementia safe in our acute hospitals? A review of challenges and solutions. J R Soc Med. 2013;106(9):355–361. , , .
- Screening for dementia in general hospital inpatients: a systematic review and meta‐analysis of available instruments. Age Ageing. 2013;42(6):689–695. , , .
- Tools to detect delirium superimposed on dementia: a systematic review. J Am Geriatr Soc. 2012;60(11):2005–2013. , , , et al.
- Functional analysis‐based interventions for challenging behaviour in dementia. Cochrane Database Syst Rev. 2012;2:CD006929. , , , , , .
- Cognitive stimulation to improve cognitive functioning in people with dementia. Cochrane Database Syst Rev. 2012;2:CD005562. , , , .
- The prevalence and correlates of major and minor depression in older medical inpatients. J Am Geriatr Soc. 2005;53(8):1344–1353. , , , et al.
- Major depressive disorder in hospitalized medically ill patients: an examination of young and elderly male veterans. J Am Geriatr Soc. 1991;39(9):881–890. , , , , , .
- Depression, post‐traumatic stress disorder, and functional disability in survivors of critical illness in the BRAIN‐ICU study: a longitudinal cohort study. Lancet Respir Med. 2014;2(5):369–379. , , , et al.
- Depressive symptoms after hospitalization in older adults: function and mortality outcomes. J Am Geriatr Soc. 2012;60(12):2254–2262. , , , et al.
- 12‐month cognitive outcomes of major and minor depression in older medical patients. Am J Geriatr Psychiatry. 2008;16(9):742–751. , , , , .
- Depression is a risk factor for rehospitalization in medical inpatients. Prim Care Companion J Clin Psychiatry. 2007;9(4):256–262. , , , et al.
- Dose‐response relationship between depressive symptoms and hospital readmission. J Hosp Med. 2014;9(6):358–364. , , , , , .
- Depressive symptoms and 3‐year mortality in older hospitalized medical patients. Ann Intern Med. 1999;130(7):563–569. , , , , , .
- Support for the vascular depression hypothesis in late‐life depression: results of a 2‐site, prospective, antidepressant treatment trial. Arch Gen Psychiatry. 2010;67(3):277–285. , , , et al.
- Executive dysfunction and the course of geriatric depression. Biol Psychiatry. 2005;58(3):204–210. , , , , , .
- Recognition of depression in older medical inpatients. J Gen Intern Med. 2007;22(5):559–564. , , , , .
- A collaborative care depression management program for cardiac inpatients: depression characteristics and in‐hospital outcomes. Psychosomatics. 2011;52(1):26–33. , , , , , .
- Impact of a depression care management program for hospitalized cardiac patients. Circ Cardiovasc Qual Outcomes. 2011;4(2):198–205. , , , , , .
- Potentially inappropriate medication use in hospitalized elders. J Hosp Med. 2008;3(2):91–102. , , , et al.
- Prevalence, incidence and nature of prescribing errors in hospital inpatients: a systematic review. Drug Saf. 2009;32(5):379–389. , , , , , .
- Minimizing adverse drug events in older patients. Am Fam Physician. 2007;76(12):1837–1844. , .
- STOPP (Screening Tool of Older Person's Prescriptions) and START (Screening Tool to Alert doctors to Right Treatment). Consensus validation. Int J Clin Pharmacol Ther. 2008;46(2):72–83. , , , , .
- American Geriatrics Society 2012 Beers Criteria Update Expert Panel. American Geriatrics Society updated Beers Criteria for potentially inappropriate medication use in older adults. J Am Geriatr Soc. 2012;60(4):616–631.
- Preventing potentially inappropriate medication use in hospitalized older patients with a computerized provider order entry warning system. Arch Intern Med. 2010;170(15):1331–1336. , , , .
- Hazards of Hospitalization of the Elderly. Ann Intern Med. 1993;118(3):219–223. .
- Improving health care for older persons. Ann Intern Med. 2003;139(5 part 2):421–424. .
- Successful models of comprehensive care for older adults with chronic conditions: evidence for the Institute of Medicine's "retooling for an aging America" report. J Am Geriatr Soc. 2009;57(12):2328–2337. , , , , , .
- Recovery of activities of daily living in older adults after hospitalization for acute medical illness. J Am Geriatr Soc. 2008;56(12):2171–2179. , , , et al.
- Changes in functional status and the risks of subsequent nursing home placement and death. J Gerontol. 1993;48(3):S94–S101. , , , .
- Risk factors for nursing home placement in a population‐based dementia cohort. J Am Geriatr Soc. 2000;48(5):519–525. , , .
- The underrecognized epidemic of low mobility during hospitalization of older adults. J Am Geriatr Soc. 2009;57(9):1660–1665. , , , .
- A systematic review of predictors and screening instruments to identify older hospitalized patients at risk for functional decline. J Clin Nurs. 2007;16(1):46–57. , , , , .
- Immobility and falls. Clin Geriatr Med. 1998;14(4):699–726. .
- Prevalence and outcomes of low mobility in hospitalized older patients. J Am Geriatr Soc. 2004;52(8):1263–1270. , , .
- Impact of a nurse‐driven mobility protocol on functional decline in hospitalized older adults. J Nurs Care Qual. 2009;24(4):325–331. , , .
- Impact of early mobilization protocol on the medical‐surgical inpatient population: an integrated review of literature. Clin Nurse Spec. 2012;26(2):87–94. , .
- The aging population and its impact on the surgery workforce. Ann Surg. 2003;238(2):170–177. , , , .
- Perioperative care of the elderly patient: an update. Cleve Clin J Med. 2009;76(suppl 4):S16–S21. .
- Frailty in the older surgical patient: a review. Age Ageing. 2012;41(2):142–147. , , .
- The assessment and management of peri‐operative pain in older adults. Anaesthesia. 2014;69(suppl 1):54–60. .
- National Research Strategies: what outcomes are important in peri‐operative elderly care? Anaesthesia. 2014;69(suppl 1):61–69. , .
- 2002 National Hospital Discharge Survey. Adv Data. 2004;342:1–30. , .
- Hospitalization in the United States, 2002. Rockville, MD: Agency for Healthcare Research and Quality; 2005. , .
- The Curriculum for the Hospitalized Aging Medical Patient program: a collaborative faculty development program for hospitalists, general internists, and geriatricians. J Hosp Med. 2008;3(5):384–393. , , , et al.
- Advancement of geriatrics education. J Hosp Med. 2011;6(6):370. , .
- Advancing geriatrics education: an efficient faculty development program for academic hospitalists increases geriatric teaching. J Hosp Med. 2010;5(9):541–546. , , , , , .
Older adults with high levels of medical complexity occupy an increasing fraction of beds in acute‐care hospitals in the United States.[1, 2] By 2007, patients age 65 years and older accounted for nearly half of adult inpatient days of care.[1] These patients are commonly cared for by hospitalists who number more than 40,000.[3] Although hospitalists are most often trained in internal medicine, they have typically received limited formal geriatrics training. Increasingly, access to experts in geriatric medicine is limited.[4] Further, hospitalists and others who practice in acute care are limited by the lack of research to address the needs of the older adult population, specifically in the diagnosis and management of conditions encountered during acute illness.
To better support hospitalists in providing acute inpatient geriatric care, the Society of Hospital Medicine (SHM) partnered with the Association of Specialty Professors to develop a research agenda to bridge this gap. Using methodology from the James Lind Alliance (JLA) and the Patient Centered Outcomes Research Institute (PCORI), the SHM joined with older adult advocacy groups, professional societies of providers, and funders to create a geriatric‐focused acute‐care research agenda, highlighting 10 key research questions.[5, 6, 7] The goal of this approach was to produce and promote high integrity, evidence‐based information that comes from research guided by patients, caregivers, and the broader healthcare community.[8] In this article, we describe the methodology and results of this agenda‐setting process, referred to as the Acute Care of Older Patients (ACOP) Priority Setting Partnership.
METHODS
Overview
This project focused on topic generation, the first step in the PCORI framework for identification and prioritization of research areas.[5] We employed a specific and defined methodology to elicit and prioritize potential research topics incorporating input from representatives of older patients, family caregivers, and healthcare providers.[6]
To elicit this input, we chose a collaborative and consultative approach to stakeholder engagement, drawing heavily from the published work of the JLA, an initiative promoting patient‐clinician partnerships in health research developed in the United Kingdom.[6] We previously described the approach elsewhere.[7]
The ACOP process for determining the research agenda consisted of 4 steps: (1) convene, (2) consult, (3) collate, and (4) prioritize.[6] Through these steps, detailed below, we were able to obtain input from a broad group of stakeholders and engage the stakeholders in a process of reducing and refining our research questions.
Convene
The steering committee (the article's authors) convened a stakeholder partnership group that included stakeholders representing patients and caregivers, advocacy organizations for the elderly, organizations that address diseases and conditions common among hospitalized older patients, provider professional societies (eg, hospitalists, subspecialists, and nurses and social workers), payers, and funders. Patient, caregiver, and advocacy organizations were identified based on their engagement in aging and health policy advocacy by SHM staff and 1 author who had completed a Health and Aging Policy Fellowship (H.L.W.).
The steering committee issued e‐mail invitations to stakeholder organizations, making initial inquiries through professional staff and relevant committee chairs. Second inquiries were made via e‐mail to each organization's volunteer leadership. We developed a webinar that outlined the overall research agenda setting process and distributed the webinar to all stakeholders. The stakeholder organizations were asked to commit to (1) surveying their memberships and (2) participating actively in prioritization by e‐mail and at a 1‐day meeting in Washington DC.
Consult
Each stakeholder organization conducted a survey of its membership via an Internet‐based survey in the summer of 2013 (see Supporting Information, Appendix A, in the online version of this article). Stakeholder organizations were asked to provide up to 75 survey responses each. Though a standard survey was used, the steering committee was not prescriptive in the methodology of survey distribution to accommodate the structure and communication methods of the individual stakeholder organizations. Survey respondents were asked to identify up to 5 unanswered questions relevant to the acute care of older persons and also provide demographic information.
Collate
In the collating process, we clarified and categorized the unanswered questions submitted in the individual surveys. Each question was initially reviewed by a member of the steering committee, using explicit criteria (see Supporting Information, Appendix B, in the online version of this article). Questions that did not meet all 4 criteria were removed. For questions that met all criteria, we clarified language, combined similar questions, and categorized each question. Categories were created in a grounded process, in which individual reviewers assigned categories based on the content of the questions. Each question could be assigned to up to 2 categories. Each question was then reviewed by a second member of the steering committee using the same 4 criteria. As part of this review, similar questions were consolidated, and when possible, questions were rewritten in a standard format.[6]
Finally, the steering committee reviewed previously published research agendas looking for additional relevant unanswered questions, specifically the New Frontiers Research Agenda created by the American Geriatrics Society in conjunction with participating subspecialty societies,[9] the Cochrane Library, and other systematic reviews identified in the literature via PubMed search.[10, 11, 12, 13, 14, 15]
Prioritize
The resulting list of unanswered questions was prioritized in 2 phases. First, the list was e‐mailed to all stakeholder organizations. The organizations were asked to vote on their top 10 priorities from this list using an online ballot, assigning 10 points to their highest priority down to 1 point for their lowest priority. In so doing, they were asked to consider explicit criteria (see Supporting Information, Appendix B, in the online version of this article). Each organization had only 1 ballot and could arrive at their top 10 list in any manner they wished. The balloting from this phase was used to develop a list of unanswered questions for the second round of in‐person prioritization. Each priority's scores were totaled across all voting organizations. The 29 priorities with the highest point totals were brought to the final prioritization round because of a natural cut point at priority number 29, rather than number 30.
For the final prioritization round, the steering committee facilitated an in‐person meeting in Washington, DC in October 2013 using nominal group technique (NGT) methodologies to arrive at consensus.[16] During this process stakeholders were asked to consider additional criteria (see Supporting Information, Appendix B, in the online version of this article).
RESULTS
Table 1 lists the organizations who engaged in 1 or more parts of the topic generation process. Eighteen stakeholder organizations agreed to participate in the convening process. Ten organizations did not respond to our solicitation and 1 declined to participate.
Organization (N=18) | Consultation % of Survey Responses (N=580) | Prioritization Round 1 | Prioritization Round 2 |
---|---|---|---|
Alzheimer's Association | 7.0% | Yes | Yes |
American Academy of Neurology | 3.4% | Yes | Yes |
American Association of Retired Persons | 0.8% | No | No |
American College of Cardiology | 11.4% | Yes | Yes |
American College of Emergency Physicians | 1.3% | No | No |
American College of Surgeons | 1.0% | Yes | Yes |
American Geriatrics Society | 7.6% | Yes | Yes |
American Hospital Association | 1.7% | Yes | No |
Centers for Medicare & Medicaid Services | 0.8% | Yes | Yes |
Gerontological Society of America | 18.9% | Yes | Yes |
National Alliance for Caregiving | 1.0% | Yes | Yes |
National Association of Social Workers | 5.9% | Yes | Yes |
National Coalition for Healthcare | 0.6% | No | No |
National Institute on Aging | 2.1% | Yes | Yes |
National Partnership for Women and Families | 0.0% | Yes | Yes |
Nursing Improving Care for Healthsystem Elders | 28.6% | Yes | No |
Society of Critical Care Medicine | 12.0% | Yes | Yes |
Society of Hospital Medicine | 4.6% | Yes | Yes |
Seventeen stakeholder organizations obtained survey responses from a total of 580 individuals (range, 3150 per organization), who were asked to identify important unanswered questions in the acute care of older persons. Survey respondents were typically female (77%), white (85%), aged 45 to 65 years (65%), and identified themselves as health professionals (90%). Twenty‐six percent of respondents also identified as patients or family caregivers. Their surveys included 1299 individual questions.
Figure 1 summarizes our collation and prioritization process and reports the numbers of questions resulting at each stage. Nine hundred nineteen questions were removed during the first review conducted by steering committee members, and 31 question categories were identified. An additional 305 questions were removed in the second review, with 75 questions remaining. As the final step of the collating process, literature review identified 39 relevant questions not already suggested or moved forward through our consultation and collation process. These questions were added to the list of unanswered questions.
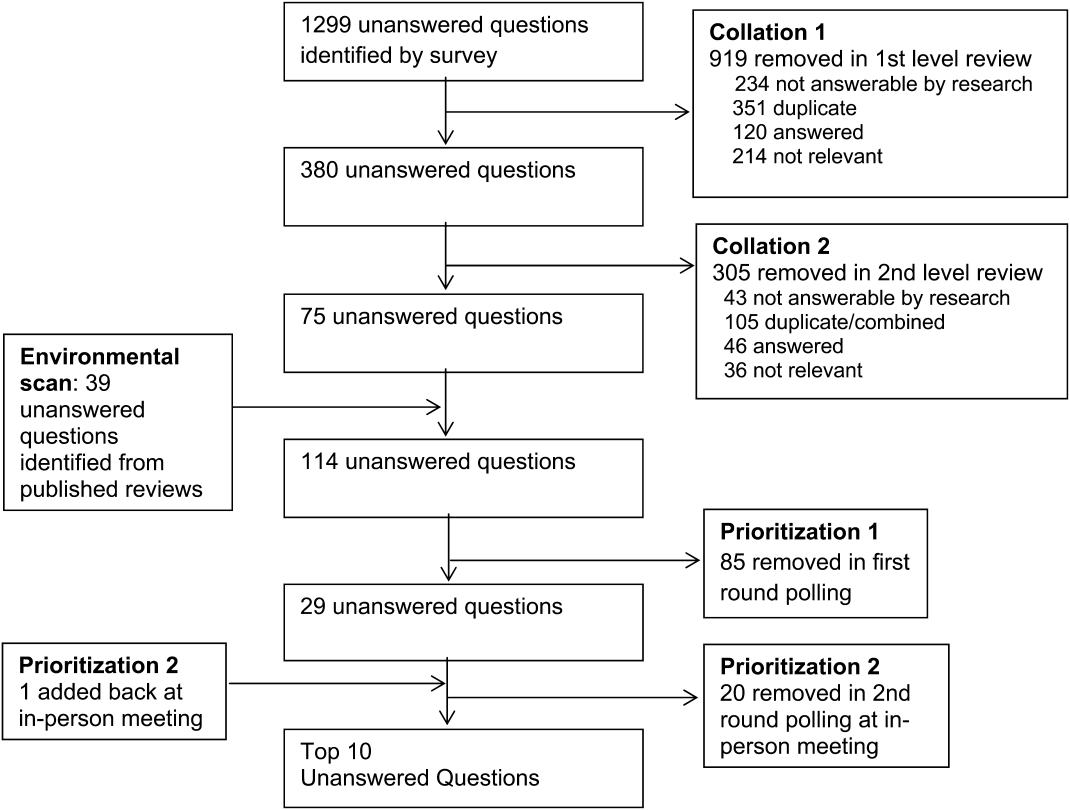
In the first round of prioritization, this list of 114 questions was emailed to each stakeholder organization (Table 1). After the stakeholder voting process was completed, 29 unanswered questions remained (see Supporting Information, Appendix C, in the online version of this article). These questions were refined and prioritized in the in‐person meeting to create the final list of 10 questions. The stakeholders present in the meeting represented 13 organizations (Table 1). Using the NGT with several rounds of small group breakouts and large group deliberation, 9 of the top 10 questions were selected from the list of 29. One additional highly relevant question that had been removed earlier in the collation process regarding workforce was added back by the stakeholder group.
This prioritized research agenda appears in Table 2 and below, organized alphabetically by topic.
- Advanced care planning: What approaches for determining and communicating goals of care across and within healthcare settings are most effective in promoting goal‐concordant care for hospitalized older patients?
- Care transitions: What is the comparative effectiveness of transitional care models on patient‐centered outcomes for hospitalized older adults?
- Delirium: What practices are most effective for consistent recognition, prevention, and treatment of delirium subtypes among hospitalized older adults?
- Dementia: Does universal assessment of hospitalized older adults for cognitive impairment (eg, at presentation and/or discharge) lead to more appropriate application of geriatric care principles and improve patient‐centered outcomes?
- Depression: Does identifying depressive symptoms during a hospital stay and initiating a therapeutic plan prior to discharge improve patient‐centered and/or disease‐specific outcomes?
- Medications: What systems interventions improve medication management for older adults (ie, appropriateness of medication choices and dosing, compliance, cost) in the hospital and postacute care?
- Models of care: For which populations of hospitalized older adults does systematic implementation of geriatric care principles/processes improve patient‐centered outcomes?
- Physical function: What is the comparative effectiveness of interventions that promote in‐hospital mobility, improve and preserve physical function, and reduce falls among older hospitalized patients?
- Surgery: What perioperative strategies can be used to optimize care processes and improve outcomes in older surgical patients?
- Training: What is the most effective approach to training hospital‐based providers in geriatric and palliative care competencies?
Topic | Scope of Problem | What Is known | Unanswered Question | Proposed Dimensions |
---|---|---|---|---|
| ||||
Advanced‐care planning | Older persons who lack decision‐making capacity often do not have surrogates or clear goals of care documented.[19] Advanced‐care directives are associated with an increase in patient autonomy and empowerment, and although 15% to 25% of adults completed the documentation in 2004,[20] a recent study found completion rates have increased to 72%.[21] | Nursing home residents with advanced directives are less likely to be hospitalized.[22, 23] Advanced directive tools, such as POLST, work to translate patient preferences to medical order.[24] standardized patient transfer tools may help to improve transitions between nursing homes and hospitals.[25] However, advanced care planning fails to integrate into courses of care if providers are unwilling or unskilled in using advanced care documentation.[26] | What approaches for determining and communicating goals of care across and within healthcare settings are most effective in promoting goal‐concordant care for hospitalized older patients? | Potential interventions: |
Decision aids | ||||
Standard interdisciplinary advanced care planning approach | ||||
Patient advocates | ||||
Potential outcomes might include: | ||||
Completion of advanced directives and healthcare power of attorney | ||||
Patient‐centered outcomesa | ||||
Care transitions | Hospital readmission from home and skilled nursing facilities occurs within 30 days in up to a quarter of patients.[27, 28] The discharge of complex older hospitalized patients is fraught with challenges. The quality of the hospital discharge process can influence outcomes for vulnerable older patients.[29, 30, 31, 32] Studies measuring the quality of hospital discharge frequently find deficits in documentation of assessment of geriatric syndromes,[33] poor patient/caregiver understanding,[34, 35] and poor communication and follow‐up with postacute providers.[35, 36, 37, 38] | As many as 10 separate domains may influence the success of a discharge.[39] There is limited evidence, regarding quality‐of‐care transitions for hospitalized older patients. The Coordinated‐Transitional Care Program found that follow‐up with telecommunication decreased readmission rates and improved transitional care for a high‐risk condition veteran population.[40] There is modest evidence for single interventions,[41] whereas the most effective hospital‐to‐community care interventions address multiple processes in nongeriatric populations.[39, 42, 43] | What is the comparative effectiveness of the transitional care models on patient‐centered outcomes for hospitalized older adults? | Possible models: |
Established vs novel care‐transition models | ||||
Disease‐specific vs general approaches | ||||
Accountable care models | ||||
Caregiver and family engagement | ||||
Community engagement | ||||
Populations of interest: | ||||
Patients with dementia | ||||
Patients with multimorbidity | ||||
Patients with geriatric syndromes | ||||
Patients with psychiatric disease | ||||
Racially and ethnically diverse patients | ||||
Outcomes: | ||||
Readmission | ||||
Other adverse events | ||||
Cost and healthcare utilization | ||||
Patient‐centered outcomesa | ||||
Delirium | Among older inpatients, the prevalence of delirium varies with severity of illness. Among general medical patients, in‐hospital prevalence ranges from 10% to 25 %.[44, 45] In the ICU, prevalence estimates are higher, ranging from 25% to as high as 80%.[46, 47] Delirium independently predicts increased length of stay,[48, 49] long‐term cognitive impairment,[50, 51] functional decline,[51] institutionalization,[52] and short‐ and long‐term mortality.[52, 53, 54] | Multicomponent strategies have been shown to be effective in preventing delirium. A systematic review of 19 such interventions identified the most commonly included such as[55]: early mobilization, nutrition supplements, medication review, pain management, sleep enhancement, vision/hearing protocols, and specialized geriatric care. Studies have included general medical patients, postoperative patients, and patients in the ICU. The majority of these studies found reductions in either delirium incidence (including postoperative), delirium prevalence, or delirium duration. Although medications have not been effective in treating delirium in general medical patients,[48] the choice and dose of sedative agents has been shown to impact delirium in the ICU.[56, 57, 58] | What practices are most effective for consistent recognition, prevention, and treatment of delirium subtypes (hypoactive, hyperactive, and mixed) among hospitalized older adults? | Outcomes to examine: |
Delirium incidence (including postoperative) | ||||
Delirium duration | ||||
Delirium‐/coma‐free days | ||||
Delirium prevalence at discharge | ||||
Subsyndromal delirium | ||||
Potential prevention and treatment modalities: | ||||
Family education or psychosocial interventions | ||||
Pharmacologic interventions | ||||
Environmental modifications | ||||
Possible areas of focus: | ||||
Special populations | ||||
Patients with varying stages of dementia | ||||
Patients with multimorbidity | ||||
Patients with geriatric syndromes | ||||
Observation patients | ||||
Diverse settings | ||||
Emergency department | ||||
Perioperative | ||||
Skilled nursing/rehab/long‐term acute‐care facilities | ||||
Dementia | 13% to 63% of older persons in the hospital have dementia.[59] Dementia is often unrecognized among hospitalized patients.[60] The presence of dementia is associated with a more rapid functional decline during admission and delayed hospital discharge.[59] Patients with dementia require more nursing hours, and are more likely to have complications[61] or die in care homes rather than in their preferred site.[59] | Several tools have been validated to screen for dementia in the hospital setting.[62] Studies have assessed approaches to diagnosing delirium in hospitalized patients with dementia.[63] Cognitive and functional stimulation interventions may have a positive impact on reducing behavioral issues.[64, 65] | Does universal assessment of hospitalized older adults for cognitive impairment (eg, at presentation and/or discharge) lead to more appropriate application of geriatric care principles and improve patient centered outcomes? | Potential interventions: |
Dementia or delirium care | ||||
Patient/family communication and engagement strategies | ||||
Maintenance/recovery of independent functional status | ||||
Potential outcomes: | ||||
Patient‐centered outcomesa | ||||
Length of stay, cost, and healthcare utilization (including palliative care) | ||||
Immediate invasive vs early conservative treatments pursued | ||||
Depression | Depression is a common geriatric syndrome among acutely ill older patients, occurring in up to 45% of patients.[66, 67] Rates of depression are similar among patients discharged following a critical illness, with somatic, rather than cognitive‐affective complaints being the most prevalent.[68] Depression among inpatients or immediately following hospitalization independently predicts worse functional outcomes,[69] cognitive decline,[70] hospital readmission,[71, 72] and long‐term mortality.[69, 73] Finally, geriatric patients are known to respond differently to medical treatment.[74, 75] | Although highly prevalent, depression is poorly recognized and managed in the inpatient setting. Depression is recognized in only 50% of patients, with previously undiagnosed or untreated depression being at highest risk for being missed.[76] The role of treatment of depression in the inpatient setting is poorly understood, particularly for those with newly recognized depression or depressive symptoms. Some novel collaborative care and telephone outreach programs have led to increases in depression treatment in patients with specific medical and surgical conditions, resulting in early promising mental health and comorbid outcomes.[77, 78] The efficacy of such programs for older patients is unknown. | Does identifying depressive symptoms during a hospital stay and initiating a therapeutic plan prior to discharge improve patient‐centered and/or disease‐specific outcomes? | Possible areas of focus: |
Comprehensive geriatric and psychosocial assessment; | ||||
Inpatient vs outpatient initiation of pharmacological therapy | ||||
Integration of confusion assessment method into therapeutic approaches | ||||
Linkages with outpatient mental health resources | ||||
Medications | Medication exposure, particularly potentially inappropriate medications, is common in hospitalized elders.[79] Medication errorsof dosage, type, and discrepancy between what a patient takes at home and what is known to his/her prescribing physicianare common and adversely affects patient safety.[80] Geriatric populations are disproportionately affected, especially those taking more than 5 prescription medications per day.[81] | Numerous strategies including electronic alerts, screening protocols, and potentially inappropriate medication lists (Beers list, STOPP) exist, though the optimal strategies to limit the use of potentially inappropriate medications is not yet known.[82, 83, 84] | What systems interventions improve medication management for older adults (ie, appropriateness of medication choices and dosing, compliance, cost) in hospital and post‐acute care? | Possible areas of focus: |
Use of healthcare information technology | ||||
Communication across sites of care | ||||
Reducing medication‐related adverse events | ||||
Engagement of family caregivers | ||||
Patient‐centered strategies to simplify regimens | ||||
Models of care | Hospitalization marks a time of high risk for older patients. Up to half die during hospitalization or within the year following the hospitalization. There is high risk of nosocomial events, and more than a third experience a decline in health resulting in longer hospitalizations and/or placement in extended‐care facilities.[73, 85, 86] | Comprehensive inpatient care for older adults (acute care for elders units, geriatric evaluation and management units, geriatric consultation services) were studied in 2 meta‐analyses, 5 RCTs, and 1 quasiexperimental study and summarized in a systematic review.[87] The studies reported improved quality of care (1 of 1 article), quality of life (3 of 4), functional autonomy (5 of 6), survival (3 of 6), and equal or lower healthcare utilization (7 of 8). | For which populations of hospitalized older adults does systematic implementation of geriatric care principles/processes improve patient‐centered outcomes? | Potential populations: |
Patients of the emergency department, critical care, perioperative, and targeted medical/surgical units | ||||
Examples of care principles: | ||||
Geriatric assessment, early mobility, medication management, delirium prevention, advanced‐care planning, risk‐factor modification, caregiver engagement | ||||
Potential outcomes: | ||||
Patient‐centered outcomesa | ||||
Cost | ||||
Physical function | Half of older patients will lose functional capacity during hospitalization.[88] Loss of physical function, particularly of lower extremities, is a risk factor for nursing home placement.[89, 90] Older hospitalized patients spend the majority (up to 80%) of their time lying in bed, even when they are capable of walking independently.[91] | Loss of independences with ADL capabilities is associated with longer hospital stays, higher readmission rates, and higher mortality risk.[92] Excessive time in bed during a hospital stay is also associated with falls.[93] Often, hospital nursing protocols and physician orders increase in‐hospital immobility in patients.[91, 94] However, nursing‐driven mobility protocols can improve functional outcomes of older hospitalized patients.[95, 96] | What is the comparative effectiveness of interventions that promote in‐hospital mobility, improve and preserve physical function, and reduce falls among older hospitalized patients? | Potential interventions: |
Intensive physical therapy | ||||
Incidental functional training | ||||
Restraint reduction | ||||
Medication management | ||||
Potential outcomes: | ||||
Discharge location | ||||
Delirium, pressure ulcers, and falls | ||||
Surgery | An increasing number of persons over age 65 years are undergoing surgical procedures.[97] These persons are at increased risk for developing delirium/cogitative dysfunction,[98] loss of functional status,[99] and exacerbations of chronic illness.[97] Additionally, pain management may be harder to address in this population.[100] Current outcomes may not reflect the clinical needs of elder surgical patients.[101] | Tailored drug selection and nursing protocols may prevent delirium.[98] Postoperative cognitive dysfunction may require weeks for resolution. Identifying frail patients preoperatively may lead to more appropriate risk stratification and improved surgical outcomes.[99] Pain management strategies focused on mitigating cognitive impact and other effects may also be beneficial.[100] Development of risk‐adjustment tools specific to older populations, as well as measures of frailty and patient‐centered care, have been proposed.[101] | What perioperative strategies can be used to optimize care processes and improve outcomes in older surgical patients? | Potential strategies: |
Preoperative risk assessment and optimization for frail or multimorbid older patients | ||||
Perioperative management protocols for frail or multimorbid older patients | ||||
Potential outcomes: | ||||
Postoperative patient centered outcomesa | ||||
Perioperative cost, healthcare utilization | ||||
Training | Adults over age 65 years comprise 13.2 % of the US population, but account for >30% of hospital discharges and 50% of hospital days.[86, 102, 103] By 2030, there will only be 1 geriatrician for every 3798 Americans >75 years.[4] Between 1997 and 2006, the odds that a hospitalist would treat a hospitalized Medicare patient rose 29% per year.[3] | Train the trainer programs for physicians include the CHAMP, the AGESP, and the PAGE. Education for nurses include the NICHE. Outcomes include improved self‐confidence, attitudes, teaching skills, and geriatric care environment.[104, 105, 106] | What is the most effective approach to training hospital‐based providers in geriatric and palliative care competencies? | Potential interventions: |
Mentored implementation | ||||
Train the trainer | ||||
Technical support |
Table 2 also contains a capsule summary of the scope of the problem addressed by each research priority, a capsule summary of related work in the content area (what is known) not intended as a systematic review, and proposed dimensions or subquestions suggested by the stakeholders at the final prioritization meeting
DISCUSSION
Older hospitalized patients account for an increasing number and proportion of hospitalized patients,[1, 2] and hospitalists increasingly are responsible for inpatient care for this population.[3] The knowledge required for hospitalists to deliver optimal care and improve outcomes has not kept pace with the rapid growth of either hospitalists or hospitalized elders. Through a rigorous prioritization process, we identified 10 areas that deserve the highest priority in directing future research efforts to improve care for the older hospitalized patient. Assessment, prevention, and treatment of geriatric syndromes in the hospital account for almost half of the priority areas. Additional research is needed to improve advanced care planning, develop new care models, and develop training models for future hospitalists competent in geriatric and palliative care competencies.
A decade ago, the American Geriatric Society and the John A. Hartford Foundation embarked upon a research agenda aimed at improving the care of hospitalized elders cared for by specialists (ie, New Frontiers in Geriatrics Research: An Agenda for Surgical and Related Medical Specialties).[9] This effort differed in many important ways from the current priortization process. First, the New Frontiers agenda focused upon specific diseases, whereas the ACOP agenda addresses geriatric syndromes that cut across multiple diseases. Second, the New Frontiers agenda was made by researchers and based upon published literature, whereas the ACOP agenda involved the input of multiple stakeholders. Finally, the New Frontiers prioritized a research agenda across a number of surgical specialties, emergency medicine, and geriatric rehabilitation. Hospital medicine, however, was still early in its development and was not considered a unique specialty. Since that time, hospital medicine has matured into a unique specialty, with increased numbers of hospitalists,[3] increased research in hospital medicine,[17] and a separate recertification pathway for internal medicine licensure.[18] To date, there has not been a similar effort performed to direct geriatric research efforts for hospital medicine.
For researchers working in the field of hospital medicine, this list of topics has several implications. First, as hospitalists are commonly generalists, hospitalist researchers may be particularly well‐suited to study syndromes that cut across specialties. However, this does raise concerns about funding sources, as most National Institutes of Health institutes are disease‐focused. Funders that are not disease‐focused such as PCORI, National Institute on Aging, National Institute of Nursing Research, and Agency for Healthcare Research and Quality, and private foundations (Hartford, Robert Wood Johnson, and Commonwealth) may be more fruitful sources of funding for this work, but funding may be challenging. Nonetheless, the increased focus on patient‐centered work may increase funders' interest in such work. Second, the topics on this list would suggest that interventions will not be pharmacologic, but will focus on nonpharmacologic, behavioral, and social interventions. Similarly, outcomes of interest must expand beyond utilization metrics such as length of stay and mortality, to include functional status and symptom management, and goal‐concordant care. Therefore, research in geriatric acute care will necessarily be multidisciplinary.
Although these 10 high‐priority areas have been selected, this prioritized list is inherently limited by our methodology. First, our survey question was not focused on a disease state, and this wording may have resulted in the list favoring geriatric syndromes rather than common disease processes. Additionally, the resulting questions encompass large research areas and not specific questions about discrete interventions. Our results may also have been skewed by the types of engaged respondents who participated in the consultation, collating, and prioritization phases. In particular, we had a large response from geriatric medicine nurses, whereas some stakeholder groups provided no survey responses. Thus, these respondents were not representative of all possible stakeholders, nor were the survey respondents necessarily representative of each of their organizations. Nonetheless, the participants self‐identified as representative of diverse viewpoints that included patients, caregivers, and advocacy groups, with the majority of stakeholder organizations remaining engaged through the completion of the process. Thus, the general nature of this agenda helps us focus upon larger areas of importance, leaving researchers the flexibility to choose to narrow the focus on a specific research question that may include potential interventions and unique outcomes. Finally, our methodology may have inadvertently limited the number of patient and family caregiver voices in the process given our approach to large advocacy groups, our desire to be inclusive of healthcare professional organizations, and our survey methodology. Other methodologies may have reached more patients and caregivers, yet many healthcare professionals have served as family caregivers to frail elders requiring hospitalization and may have been in an ideal position to answer the survey.
In conclusion, several forces are shaping the future of acute inpatient care. These include the changing demographics of the hospitalized patient population, a rapid increase in the proportion of multimorbid hospitalized older adults, an inpatient workforce (hospitalists, generalists, and subspecialists) with potentially limited geriatrics training, and gaps in evidence‐based guidance to inform diagnostic and therapeutic decision making for acutely ill older patients. Training programs in hospital medicine should be aware of and could benefit from the resulting list of unanswered questions. Our findings also have implications for training to enrich education in geriatrics. Moreover, there is growing recognition that patients and other stakeholders deserve a greater voice in determining the direction of research. In addition to efforts to improve patient‐centeredness of research, these areas have been uniquely identified by stakeholders as important, and therefore are in line with newer priorities of PCORI. This project followed a road map resulting in a patient‐centered research agenda at the intersection of hospital medicine and geriatric medicine.[7] In creating this agenda, we relied heavily on the framework proposed by PCORI. We propose to pursue a dissemination and evaluation strategy for this research agenda as well as additional prioritization steps. We believe the adoption of this methodology will create a knowledge base that is rigorously derived and most relevant to the care of hospitalized older adults and their families. Its application will ultimately result in improved outcomes for hospitalized older adults.
Acknowledgements
The authors acknowledge Claudia Stahl, Society of Hospital Medicine; Cynthia Drake, University of Colorado; and the ACOP stakeholder organizations.
Disclosures: This work was supported by the Association of Specialty Professors/American Society of Internal Medicine and the John A. Hartford Foundation. Dr. Vasilevskis was supported by the National Institute on Aging of the National Institutes of Health under award number K23AG040157 and the Veterans Affairs Clinical Research Center of Excellence, and the Geriatric Research, Education and Clinical Center (GRECC). Dr. Vasilevskis' institution receives grant funding for an aspect of submitted work. Dr. Meltzer is a PCORI Methodology Committee member. The content is solely the responsibility of the authors and does not necessarily represent the official views of the National Institutes of Health or the Department of Veterans' Affairs. The authors report no conflicts of interest.
Older adults with high levels of medical complexity occupy an increasing fraction of beds in acute‐care hospitals in the United States.[1, 2] By 2007, patients age 65 years and older accounted for nearly half of adult inpatient days of care.[1] These patients are commonly cared for by hospitalists who number more than 40,000.[3] Although hospitalists are most often trained in internal medicine, they have typically received limited formal geriatrics training. Increasingly, access to experts in geriatric medicine is limited.[4] Further, hospitalists and others who practice in acute care are limited by the lack of research to address the needs of the older adult population, specifically in the diagnosis and management of conditions encountered during acute illness.
To better support hospitalists in providing acute inpatient geriatric care, the Society of Hospital Medicine (SHM) partnered with the Association of Specialty Professors to develop a research agenda to bridge this gap. Using methodology from the James Lind Alliance (JLA) and the Patient Centered Outcomes Research Institute (PCORI), the SHM joined with older adult advocacy groups, professional societies of providers, and funders to create a geriatric‐focused acute‐care research agenda, highlighting 10 key research questions.[5, 6, 7] The goal of this approach was to produce and promote high integrity, evidence‐based information that comes from research guided by patients, caregivers, and the broader healthcare community.[8] In this article, we describe the methodology and results of this agenda‐setting process, referred to as the Acute Care of Older Patients (ACOP) Priority Setting Partnership.
METHODS
Overview
This project focused on topic generation, the first step in the PCORI framework for identification and prioritization of research areas.[5] We employed a specific and defined methodology to elicit and prioritize potential research topics incorporating input from representatives of older patients, family caregivers, and healthcare providers.[6]
To elicit this input, we chose a collaborative and consultative approach to stakeholder engagement, drawing heavily from the published work of the JLA, an initiative promoting patient‐clinician partnerships in health research developed in the United Kingdom.[6] We previously described the approach elsewhere.[7]
The ACOP process for determining the research agenda consisted of 4 steps: (1) convene, (2) consult, (3) collate, and (4) prioritize.[6] Through these steps, detailed below, we were able to obtain input from a broad group of stakeholders and engage the stakeholders in a process of reducing and refining our research questions.
Convene
The steering committee (the article's authors) convened a stakeholder partnership group that included stakeholders representing patients and caregivers, advocacy organizations for the elderly, organizations that address diseases and conditions common among hospitalized older patients, provider professional societies (eg, hospitalists, subspecialists, and nurses and social workers), payers, and funders. Patient, caregiver, and advocacy organizations were identified based on their engagement in aging and health policy advocacy by SHM staff and 1 author who had completed a Health and Aging Policy Fellowship (H.L.W.).
The steering committee issued e‐mail invitations to stakeholder organizations, making initial inquiries through professional staff and relevant committee chairs. Second inquiries were made via e‐mail to each organization's volunteer leadership. We developed a webinar that outlined the overall research agenda setting process and distributed the webinar to all stakeholders. The stakeholder organizations were asked to commit to (1) surveying their memberships and (2) participating actively in prioritization by e‐mail and at a 1‐day meeting in Washington DC.
Consult
Each stakeholder organization conducted a survey of its membership via an Internet‐based survey in the summer of 2013 (see Supporting Information, Appendix A, in the online version of this article). Stakeholder organizations were asked to provide up to 75 survey responses each. Though a standard survey was used, the steering committee was not prescriptive in the methodology of survey distribution to accommodate the structure and communication methods of the individual stakeholder organizations. Survey respondents were asked to identify up to 5 unanswered questions relevant to the acute care of older persons and also provide demographic information.
Collate
In the collating process, we clarified and categorized the unanswered questions submitted in the individual surveys. Each question was initially reviewed by a member of the steering committee, using explicit criteria (see Supporting Information, Appendix B, in the online version of this article). Questions that did not meet all 4 criteria were removed. For questions that met all criteria, we clarified language, combined similar questions, and categorized each question. Categories were created in a grounded process, in which individual reviewers assigned categories based on the content of the questions. Each question could be assigned to up to 2 categories. Each question was then reviewed by a second member of the steering committee using the same 4 criteria. As part of this review, similar questions were consolidated, and when possible, questions were rewritten in a standard format.[6]
Finally, the steering committee reviewed previously published research agendas looking for additional relevant unanswered questions, specifically the New Frontiers Research Agenda created by the American Geriatrics Society in conjunction with participating subspecialty societies,[9] the Cochrane Library, and other systematic reviews identified in the literature via PubMed search.[10, 11, 12, 13, 14, 15]
Prioritize
The resulting list of unanswered questions was prioritized in 2 phases. First, the list was e‐mailed to all stakeholder organizations. The organizations were asked to vote on their top 10 priorities from this list using an online ballot, assigning 10 points to their highest priority down to 1 point for their lowest priority. In so doing, they were asked to consider explicit criteria (see Supporting Information, Appendix B, in the online version of this article). Each organization had only 1 ballot and could arrive at their top 10 list in any manner they wished. The balloting from this phase was used to develop a list of unanswered questions for the second round of in‐person prioritization. Each priority's scores were totaled across all voting organizations. The 29 priorities with the highest point totals were brought to the final prioritization round because of a natural cut point at priority number 29, rather than number 30.
For the final prioritization round, the steering committee facilitated an in‐person meeting in Washington, DC in October 2013 using nominal group technique (NGT) methodologies to arrive at consensus.[16] During this process stakeholders were asked to consider additional criteria (see Supporting Information, Appendix B, in the online version of this article).
RESULTS
Table 1 lists the organizations who engaged in 1 or more parts of the topic generation process. Eighteen stakeholder organizations agreed to participate in the convening process. Ten organizations did not respond to our solicitation and 1 declined to participate.
Organization (N=18) | Consultation % of Survey Responses (N=580) | Prioritization Round 1 | Prioritization Round 2 |
---|---|---|---|
Alzheimer's Association | 7.0% | Yes | Yes |
American Academy of Neurology | 3.4% | Yes | Yes |
American Association of Retired Persons | 0.8% | No | No |
American College of Cardiology | 11.4% | Yes | Yes |
American College of Emergency Physicians | 1.3% | No | No |
American College of Surgeons | 1.0% | Yes | Yes |
American Geriatrics Society | 7.6% | Yes | Yes |
American Hospital Association | 1.7% | Yes | No |
Centers for Medicare & Medicaid Services | 0.8% | Yes | Yes |
Gerontological Society of America | 18.9% | Yes | Yes |
National Alliance for Caregiving | 1.0% | Yes | Yes |
National Association of Social Workers | 5.9% | Yes | Yes |
National Coalition for Healthcare | 0.6% | No | No |
National Institute on Aging | 2.1% | Yes | Yes |
National Partnership for Women and Families | 0.0% | Yes | Yes |
Nursing Improving Care for Healthsystem Elders | 28.6% | Yes | No |
Society of Critical Care Medicine | 12.0% | Yes | Yes |
Society of Hospital Medicine | 4.6% | Yes | Yes |
Seventeen stakeholder organizations obtained survey responses from a total of 580 individuals (range, 3150 per organization), who were asked to identify important unanswered questions in the acute care of older persons. Survey respondents were typically female (77%), white (85%), aged 45 to 65 years (65%), and identified themselves as health professionals (90%). Twenty‐six percent of respondents also identified as patients or family caregivers. Their surveys included 1299 individual questions.
Figure 1 summarizes our collation and prioritization process and reports the numbers of questions resulting at each stage. Nine hundred nineteen questions were removed during the first review conducted by steering committee members, and 31 question categories were identified. An additional 305 questions were removed in the second review, with 75 questions remaining. As the final step of the collating process, literature review identified 39 relevant questions not already suggested or moved forward through our consultation and collation process. These questions were added to the list of unanswered questions.
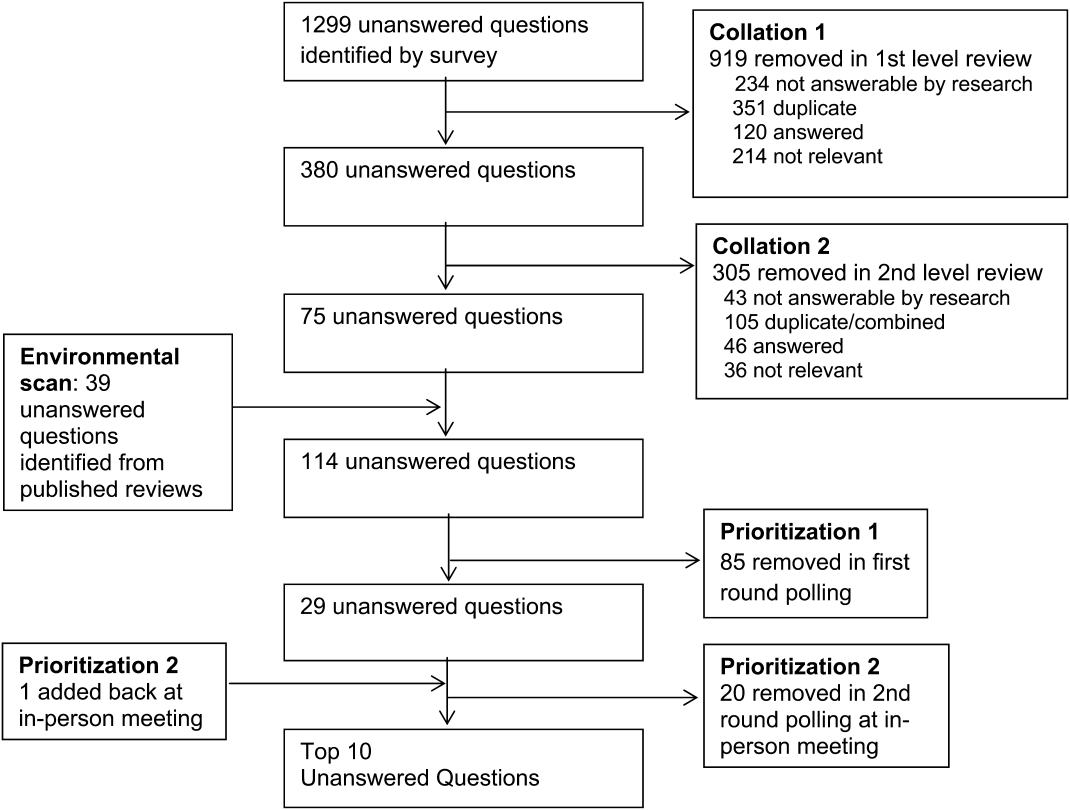
In the first round of prioritization, this list of 114 questions was emailed to each stakeholder organization (Table 1). After the stakeholder voting process was completed, 29 unanswered questions remained (see Supporting Information, Appendix C, in the online version of this article). These questions were refined and prioritized in the in‐person meeting to create the final list of 10 questions. The stakeholders present in the meeting represented 13 organizations (Table 1). Using the NGT with several rounds of small group breakouts and large group deliberation, 9 of the top 10 questions were selected from the list of 29. One additional highly relevant question that had been removed earlier in the collation process regarding workforce was added back by the stakeholder group.
This prioritized research agenda appears in Table 2 and below, organized alphabetically by topic.
- Advanced care planning: What approaches for determining and communicating goals of care across and within healthcare settings are most effective in promoting goal‐concordant care for hospitalized older patients?
- Care transitions: What is the comparative effectiveness of transitional care models on patient‐centered outcomes for hospitalized older adults?
- Delirium: What practices are most effective for consistent recognition, prevention, and treatment of delirium subtypes among hospitalized older adults?
- Dementia: Does universal assessment of hospitalized older adults for cognitive impairment (eg, at presentation and/or discharge) lead to more appropriate application of geriatric care principles and improve patient‐centered outcomes?
- Depression: Does identifying depressive symptoms during a hospital stay and initiating a therapeutic plan prior to discharge improve patient‐centered and/or disease‐specific outcomes?
- Medications: What systems interventions improve medication management for older adults (ie, appropriateness of medication choices and dosing, compliance, cost) in the hospital and postacute care?
- Models of care: For which populations of hospitalized older adults does systematic implementation of geriatric care principles/processes improve patient‐centered outcomes?
- Physical function: What is the comparative effectiveness of interventions that promote in‐hospital mobility, improve and preserve physical function, and reduce falls among older hospitalized patients?
- Surgery: What perioperative strategies can be used to optimize care processes and improve outcomes in older surgical patients?
- Training: What is the most effective approach to training hospital‐based providers in geriatric and palliative care competencies?
Topic | Scope of Problem | What Is known | Unanswered Question | Proposed Dimensions |
---|---|---|---|---|
| ||||
Advanced‐care planning | Older persons who lack decision‐making capacity often do not have surrogates or clear goals of care documented.[19] Advanced‐care directives are associated with an increase in patient autonomy and empowerment, and although 15% to 25% of adults completed the documentation in 2004,[20] a recent study found completion rates have increased to 72%.[21] | Nursing home residents with advanced directives are less likely to be hospitalized.[22, 23] Advanced directive tools, such as POLST, work to translate patient preferences to medical order.[24] standardized patient transfer tools may help to improve transitions between nursing homes and hospitals.[25] However, advanced care planning fails to integrate into courses of care if providers are unwilling or unskilled in using advanced care documentation.[26] | What approaches for determining and communicating goals of care across and within healthcare settings are most effective in promoting goal‐concordant care for hospitalized older patients? | Potential interventions: |
Decision aids | ||||
Standard interdisciplinary advanced care planning approach | ||||
Patient advocates | ||||
Potential outcomes might include: | ||||
Completion of advanced directives and healthcare power of attorney | ||||
Patient‐centered outcomesa | ||||
Care transitions | Hospital readmission from home and skilled nursing facilities occurs within 30 days in up to a quarter of patients.[27, 28] The discharge of complex older hospitalized patients is fraught with challenges. The quality of the hospital discharge process can influence outcomes for vulnerable older patients.[29, 30, 31, 32] Studies measuring the quality of hospital discharge frequently find deficits in documentation of assessment of geriatric syndromes,[33] poor patient/caregiver understanding,[34, 35] and poor communication and follow‐up with postacute providers.[35, 36, 37, 38] | As many as 10 separate domains may influence the success of a discharge.[39] There is limited evidence, regarding quality‐of‐care transitions for hospitalized older patients. The Coordinated‐Transitional Care Program found that follow‐up with telecommunication decreased readmission rates and improved transitional care for a high‐risk condition veteran population.[40] There is modest evidence for single interventions,[41] whereas the most effective hospital‐to‐community care interventions address multiple processes in nongeriatric populations.[39, 42, 43] | What is the comparative effectiveness of the transitional care models on patient‐centered outcomes for hospitalized older adults? | Possible models: |
Established vs novel care‐transition models | ||||
Disease‐specific vs general approaches | ||||
Accountable care models | ||||
Caregiver and family engagement | ||||
Community engagement | ||||
Populations of interest: | ||||
Patients with dementia | ||||
Patients with multimorbidity | ||||
Patients with geriatric syndromes | ||||
Patients with psychiatric disease | ||||
Racially and ethnically diverse patients | ||||
Outcomes: | ||||
Readmission | ||||
Other adverse events | ||||
Cost and healthcare utilization | ||||
Patient‐centered outcomesa | ||||
Delirium | Among older inpatients, the prevalence of delirium varies with severity of illness. Among general medical patients, in‐hospital prevalence ranges from 10% to 25 %.[44, 45] In the ICU, prevalence estimates are higher, ranging from 25% to as high as 80%.[46, 47] Delirium independently predicts increased length of stay,[48, 49] long‐term cognitive impairment,[50, 51] functional decline,[51] institutionalization,[52] and short‐ and long‐term mortality.[52, 53, 54] | Multicomponent strategies have been shown to be effective in preventing delirium. A systematic review of 19 such interventions identified the most commonly included such as[55]: early mobilization, nutrition supplements, medication review, pain management, sleep enhancement, vision/hearing protocols, and specialized geriatric care. Studies have included general medical patients, postoperative patients, and patients in the ICU. The majority of these studies found reductions in either delirium incidence (including postoperative), delirium prevalence, or delirium duration. Although medications have not been effective in treating delirium in general medical patients,[48] the choice and dose of sedative agents has been shown to impact delirium in the ICU.[56, 57, 58] | What practices are most effective for consistent recognition, prevention, and treatment of delirium subtypes (hypoactive, hyperactive, and mixed) among hospitalized older adults? | Outcomes to examine: |
Delirium incidence (including postoperative) | ||||
Delirium duration | ||||
Delirium‐/coma‐free days | ||||
Delirium prevalence at discharge | ||||
Subsyndromal delirium | ||||
Potential prevention and treatment modalities: | ||||
Family education or psychosocial interventions | ||||
Pharmacologic interventions | ||||
Environmental modifications | ||||
Possible areas of focus: | ||||
Special populations | ||||
Patients with varying stages of dementia | ||||
Patients with multimorbidity | ||||
Patients with geriatric syndromes | ||||
Observation patients | ||||
Diverse settings | ||||
Emergency department | ||||
Perioperative | ||||
Skilled nursing/rehab/long‐term acute‐care facilities | ||||
Dementia | 13% to 63% of older persons in the hospital have dementia.[59] Dementia is often unrecognized among hospitalized patients.[60] The presence of dementia is associated with a more rapid functional decline during admission and delayed hospital discharge.[59] Patients with dementia require more nursing hours, and are more likely to have complications[61] or die in care homes rather than in their preferred site.[59] | Several tools have been validated to screen for dementia in the hospital setting.[62] Studies have assessed approaches to diagnosing delirium in hospitalized patients with dementia.[63] Cognitive and functional stimulation interventions may have a positive impact on reducing behavioral issues.[64, 65] | Does universal assessment of hospitalized older adults for cognitive impairment (eg, at presentation and/or discharge) lead to more appropriate application of geriatric care principles and improve patient centered outcomes? | Potential interventions: |
Dementia or delirium care | ||||
Patient/family communication and engagement strategies | ||||
Maintenance/recovery of independent functional status | ||||
Potential outcomes: | ||||
Patient‐centered outcomesa | ||||
Length of stay, cost, and healthcare utilization (including palliative care) | ||||
Immediate invasive vs early conservative treatments pursued | ||||
Depression | Depression is a common geriatric syndrome among acutely ill older patients, occurring in up to 45% of patients.[66, 67] Rates of depression are similar among patients discharged following a critical illness, with somatic, rather than cognitive‐affective complaints being the most prevalent.[68] Depression among inpatients or immediately following hospitalization independently predicts worse functional outcomes,[69] cognitive decline,[70] hospital readmission,[71, 72] and long‐term mortality.[69, 73] Finally, geriatric patients are known to respond differently to medical treatment.[74, 75] | Although highly prevalent, depression is poorly recognized and managed in the inpatient setting. Depression is recognized in only 50% of patients, with previously undiagnosed or untreated depression being at highest risk for being missed.[76] The role of treatment of depression in the inpatient setting is poorly understood, particularly for those with newly recognized depression or depressive symptoms. Some novel collaborative care and telephone outreach programs have led to increases in depression treatment in patients with specific medical and surgical conditions, resulting in early promising mental health and comorbid outcomes.[77, 78] The efficacy of such programs for older patients is unknown. | Does identifying depressive symptoms during a hospital stay and initiating a therapeutic plan prior to discharge improve patient‐centered and/or disease‐specific outcomes? | Possible areas of focus: |
Comprehensive geriatric and psychosocial assessment; | ||||
Inpatient vs outpatient initiation of pharmacological therapy | ||||
Integration of confusion assessment method into therapeutic approaches | ||||
Linkages with outpatient mental health resources | ||||
Medications | Medication exposure, particularly potentially inappropriate medications, is common in hospitalized elders.[79] Medication errorsof dosage, type, and discrepancy between what a patient takes at home and what is known to his/her prescribing physicianare common and adversely affects patient safety.[80] Geriatric populations are disproportionately affected, especially those taking more than 5 prescription medications per day.[81] | Numerous strategies including electronic alerts, screening protocols, and potentially inappropriate medication lists (Beers list, STOPP) exist, though the optimal strategies to limit the use of potentially inappropriate medications is not yet known.[82, 83, 84] | What systems interventions improve medication management for older adults (ie, appropriateness of medication choices and dosing, compliance, cost) in hospital and post‐acute care? | Possible areas of focus: |
Use of healthcare information technology | ||||
Communication across sites of care | ||||
Reducing medication‐related adverse events | ||||
Engagement of family caregivers | ||||
Patient‐centered strategies to simplify regimens | ||||
Models of care | Hospitalization marks a time of high risk for older patients. Up to half die during hospitalization or within the year following the hospitalization. There is high risk of nosocomial events, and more than a third experience a decline in health resulting in longer hospitalizations and/or placement in extended‐care facilities.[73, 85, 86] | Comprehensive inpatient care for older adults (acute care for elders units, geriatric evaluation and management units, geriatric consultation services) were studied in 2 meta‐analyses, 5 RCTs, and 1 quasiexperimental study and summarized in a systematic review.[87] The studies reported improved quality of care (1 of 1 article), quality of life (3 of 4), functional autonomy (5 of 6), survival (3 of 6), and equal or lower healthcare utilization (7 of 8). | For which populations of hospitalized older adults does systematic implementation of geriatric care principles/processes improve patient‐centered outcomes? | Potential populations: |
Patients of the emergency department, critical care, perioperative, and targeted medical/surgical units | ||||
Examples of care principles: | ||||
Geriatric assessment, early mobility, medication management, delirium prevention, advanced‐care planning, risk‐factor modification, caregiver engagement | ||||
Potential outcomes: | ||||
Patient‐centered outcomesa | ||||
Cost | ||||
Physical function | Half of older patients will lose functional capacity during hospitalization.[88] Loss of physical function, particularly of lower extremities, is a risk factor for nursing home placement.[89, 90] Older hospitalized patients spend the majority (up to 80%) of their time lying in bed, even when they are capable of walking independently.[91] | Loss of independences with ADL capabilities is associated with longer hospital stays, higher readmission rates, and higher mortality risk.[92] Excessive time in bed during a hospital stay is also associated with falls.[93] Often, hospital nursing protocols and physician orders increase in‐hospital immobility in patients.[91, 94] However, nursing‐driven mobility protocols can improve functional outcomes of older hospitalized patients.[95, 96] | What is the comparative effectiveness of interventions that promote in‐hospital mobility, improve and preserve physical function, and reduce falls among older hospitalized patients? | Potential interventions: |
Intensive physical therapy | ||||
Incidental functional training | ||||
Restraint reduction | ||||
Medication management | ||||
Potential outcomes: | ||||
Discharge location | ||||
Delirium, pressure ulcers, and falls | ||||
Surgery | An increasing number of persons over age 65 years are undergoing surgical procedures.[97] These persons are at increased risk for developing delirium/cogitative dysfunction,[98] loss of functional status,[99] and exacerbations of chronic illness.[97] Additionally, pain management may be harder to address in this population.[100] Current outcomes may not reflect the clinical needs of elder surgical patients.[101] | Tailored drug selection and nursing protocols may prevent delirium.[98] Postoperative cognitive dysfunction may require weeks for resolution. Identifying frail patients preoperatively may lead to more appropriate risk stratification and improved surgical outcomes.[99] Pain management strategies focused on mitigating cognitive impact and other effects may also be beneficial.[100] Development of risk‐adjustment tools specific to older populations, as well as measures of frailty and patient‐centered care, have been proposed.[101] | What perioperative strategies can be used to optimize care processes and improve outcomes in older surgical patients? | Potential strategies: |
Preoperative risk assessment and optimization for frail or multimorbid older patients | ||||
Perioperative management protocols for frail or multimorbid older patients | ||||
Potential outcomes: | ||||
Postoperative patient centered outcomesa | ||||
Perioperative cost, healthcare utilization | ||||
Training | Adults over age 65 years comprise 13.2 % of the US population, but account for >30% of hospital discharges and 50% of hospital days.[86, 102, 103] By 2030, there will only be 1 geriatrician for every 3798 Americans >75 years.[4] Between 1997 and 2006, the odds that a hospitalist would treat a hospitalized Medicare patient rose 29% per year.[3] | Train the trainer programs for physicians include the CHAMP, the AGESP, and the PAGE. Education for nurses include the NICHE. Outcomes include improved self‐confidence, attitudes, teaching skills, and geriatric care environment.[104, 105, 106] | What is the most effective approach to training hospital‐based providers in geriatric and palliative care competencies? | Potential interventions: |
Mentored implementation | ||||
Train the trainer | ||||
Technical support |
Table 2 also contains a capsule summary of the scope of the problem addressed by each research priority, a capsule summary of related work in the content area (what is known) not intended as a systematic review, and proposed dimensions or subquestions suggested by the stakeholders at the final prioritization meeting
DISCUSSION
Older hospitalized patients account for an increasing number and proportion of hospitalized patients,[1, 2] and hospitalists increasingly are responsible for inpatient care for this population.[3] The knowledge required for hospitalists to deliver optimal care and improve outcomes has not kept pace with the rapid growth of either hospitalists or hospitalized elders. Through a rigorous prioritization process, we identified 10 areas that deserve the highest priority in directing future research efforts to improve care for the older hospitalized patient. Assessment, prevention, and treatment of geriatric syndromes in the hospital account for almost half of the priority areas. Additional research is needed to improve advanced care planning, develop new care models, and develop training models for future hospitalists competent in geriatric and palliative care competencies.
A decade ago, the American Geriatric Society and the John A. Hartford Foundation embarked upon a research agenda aimed at improving the care of hospitalized elders cared for by specialists (ie, New Frontiers in Geriatrics Research: An Agenda for Surgical and Related Medical Specialties).[9] This effort differed in many important ways from the current priortization process. First, the New Frontiers agenda focused upon specific diseases, whereas the ACOP agenda addresses geriatric syndromes that cut across multiple diseases. Second, the New Frontiers agenda was made by researchers and based upon published literature, whereas the ACOP agenda involved the input of multiple stakeholders. Finally, the New Frontiers prioritized a research agenda across a number of surgical specialties, emergency medicine, and geriatric rehabilitation. Hospital medicine, however, was still early in its development and was not considered a unique specialty. Since that time, hospital medicine has matured into a unique specialty, with increased numbers of hospitalists,[3] increased research in hospital medicine,[17] and a separate recertification pathway for internal medicine licensure.[18] To date, there has not been a similar effort performed to direct geriatric research efforts for hospital medicine.
For researchers working in the field of hospital medicine, this list of topics has several implications. First, as hospitalists are commonly generalists, hospitalist researchers may be particularly well‐suited to study syndromes that cut across specialties. However, this does raise concerns about funding sources, as most National Institutes of Health institutes are disease‐focused. Funders that are not disease‐focused such as PCORI, National Institute on Aging, National Institute of Nursing Research, and Agency for Healthcare Research and Quality, and private foundations (Hartford, Robert Wood Johnson, and Commonwealth) may be more fruitful sources of funding for this work, but funding may be challenging. Nonetheless, the increased focus on patient‐centered work may increase funders' interest in such work. Second, the topics on this list would suggest that interventions will not be pharmacologic, but will focus on nonpharmacologic, behavioral, and social interventions. Similarly, outcomes of interest must expand beyond utilization metrics such as length of stay and mortality, to include functional status and symptom management, and goal‐concordant care. Therefore, research in geriatric acute care will necessarily be multidisciplinary.
Although these 10 high‐priority areas have been selected, this prioritized list is inherently limited by our methodology. First, our survey question was not focused on a disease state, and this wording may have resulted in the list favoring geriatric syndromes rather than common disease processes. Additionally, the resulting questions encompass large research areas and not specific questions about discrete interventions. Our results may also have been skewed by the types of engaged respondents who participated in the consultation, collating, and prioritization phases. In particular, we had a large response from geriatric medicine nurses, whereas some stakeholder groups provided no survey responses. Thus, these respondents were not representative of all possible stakeholders, nor were the survey respondents necessarily representative of each of their organizations. Nonetheless, the participants self‐identified as representative of diverse viewpoints that included patients, caregivers, and advocacy groups, with the majority of stakeholder organizations remaining engaged through the completion of the process. Thus, the general nature of this agenda helps us focus upon larger areas of importance, leaving researchers the flexibility to choose to narrow the focus on a specific research question that may include potential interventions and unique outcomes. Finally, our methodology may have inadvertently limited the number of patient and family caregiver voices in the process given our approach to large advocacy groups, our desire to be inclusive of healthcare professional organizations, and our survey methodology. Other methodologies may have reached more patients and caregivers, yet many healthcare professionals have served as family caregivers to frail elders requiring hospitalization and may have been in an ideal position to answer the survey.
In conclusion, several forces are shaping the future of acute inpatient care. These include the changing demographics of the hospitalized patient population, a rapid increase in the proportion of multimorbid hospitalized older adults, an inpatient workforce (hospitalists, generalists, and subspecialists) with potentially limited geriatrics training, and gaps in evidence‐based guidance to inform diagnostic and therapeutic decision making for acutely ill older patients. Training programs in hospital medicine should be aware of and could benefit from the resulting list of unanswered questions. Our findings also have implications for training to enrich education in geriatrics. Moreover, there is growing recognition that patients and other stakeholders deserve a greater voice in determining the direction of research. In addition to efforts to improve patient‐centeredness of research, these areas have been uniquely identified by stakeholders as important, and therefore are in line with newer priorities of PCORI. This project followed a road map resulting in a patient‐centered research agenda at the intersection of hospital medicine and geriatric medicine.[7] In creating this agenda, we relied heavily on the framework proposed by PCORI. We propose to pursue a dissemination and evaluation strategy for this research agenda as well as additional prioritization steps. We believe the adoption of this methodology will create a knowledge base that is rigorously derived and most relevant to the care of hospitalized older adults and their families. Its application will ultimately result in improved outcomes for hospitalized older adults.
Acknowledgements
The authors acknowledge Claudia Stahl, Society of Hospital Medicine; Cynthia Drake, University of Colorado; and the ACOP stakeholder organizations.
Disclosures: This work was supported by the Association of Specialty Professors/American Society of Internal Medicine and the John A. Hartford Foundation. Dr. Vasilevskis was supported by the National Institute on Aging of the National Institutes of Health under award number K23AG040157 and the Veterans Affairs Clinical Research Center of Excellence, and the Geriatric Research, Education and Clinical Center (GRECC). Dr. Vasilevskis' institution receives grant funding for an aspect of submitted work. Dr. Meltzer is a PCORI Methodology Committee member. The content is solely the responsibility of the authors and does not necessarily represent the official views of the National Institutes of Health or the Department of Veterans' Affairs. The authors report no conflicts of interest.
- National Hospital Discharge Survey: 2007 summary. Natl Health Stat Report. 2010(29):1–20, 24. , , , , .
- Centers for Medicare 2012. Available at: http://www.cms.gov/Research‐Statistics‐Data‐and‐Systems/Statistics‐Trends‐and‐Reports/Chronic‐Conditions/Downloads/2012Chartbook.pdf. Accessed December 12, 2014.
- Growth in the care of older patients by hospitalists in the United States. N Engl J Med. 2009;360(11):1102–1112. , , , .
- A revelation of numbers: will America's eldercare workforce be ready to care for an aging America? Generations. 2010;34(4):11–19. , .
- Patient‐Centered Outcomes Research Institute Methodology Committee. The PCORI methodology report. Available at: http://www.pcori.org/assets/2013/11/PCORI‐Methodology‐Report.pdf. Published November 2013. Accessed December 19, 2013.
- The James Lind Alliance. JLA method. Available at: http://www.lindalliance.org/JLA_Method.asp. Accessed December 19, 2013.
- Road map to a patient‐centered research agenda at the intersection of hospital medicine and geriatric medicine. J Gen Intern Med. 2014;29(6):926–931. , , , , .
- Patient‐Centered Outcomes Research Institute. About us. Available at: http://www.pcori.org/about‐us. Accessed February 23, 2015.
- New Frontiers of Geriatrics Research: An Agenda for Surgical and Related Medical Specialties. New York, NY: American Geriatrics Society; 2004. , , .
- Linking the NIH strategic plan to the research agenda for social workers in health and aging. J Gerontol Soc Work. 2010;53(1):77–93. , , , .
- Assessing the capacity to make everyday decisions: a guide for clinicians and an agenda for future research. Am J Geriatr Psychiatry. 2007;15(2):101–111. , .
- Practitioners' views on elder mistreatment research priorities: recommendations from a Research‐to‐Practice Consensus conference. J Elder Abuse Negl. 2011;23(2):115–126. , , , et al.
- The intersection between geriatrics and palliative care: a call for a new research agenda. J Am Geriatr Soc. 2005;53(9):1593–1598. , .
- The cancer aging interface: a research agenda. J Clin Oncol. 2007;25(14):1945–1948. .
- Clinical care of persons with dementia in the emergency department: a review of the literature and agenda for research. J Am Geriatr Soc. 2012;60(9):1742–1748. , , , .
- A group process model for problem identification and program planning. J Appl Behav Sci. 1971;7(4):466–492. , .
- Research and publication trends in hospital medicine. J Hosp Med. 2014;9(3):148–154. , , , , .
- ABFM and ABIM to jointly participate in recognition of focused practice (rfp) in hospital medicine pilot approved by abms. Ann Fam Med. 2010;8(1):87. .
- Medical decision‐making for older adults without family. J Am Geriatr Soc. 2012;60(11):2144–2150. , , , .
- Promoting advance directives among elderly primary care patients. J Gen Intern Med. 2004;19(9):944–951. , , , , , .
- Advance directive completion by elderly Americans: a decade of change. J Am Geriatr Soc. 2014;62(4):706–710. , , .
- Care transitions by older adults from nursing homes to hospitals: implications for long‐term care practice, geriatrics education, and research. J Am Med Dir Assoc. 2010;11(4):231–238. , .
- Decisions to hospitalize nursing home residents dying with advanced dementia. J Am Geriatr Soc. 2005;53(8):1396–1401. , , , .
- A comparison of methods to communicate treatment preferences in nursing facilities: traditional practices versus the physician orders for life‐sustaining treatment program. J Am Geriatr Soc. 2010;58(7):1241–1248. , , , , , .
- Interventions to improve transitional care between nursing homes and hospitals: a systematic review. J Am Geriatr Soc. 2010;58(4):777–782. , , , , .
- Opening end‐of‐life discussions: how to introduce Voicing My CHOiCES, an advance care planning guide for adolescents and young adults [published online ahead of print March 13, 2014]. Palliat Support Care. doi: 10.1017/S1478951514000054. , , .
- The revolving door of rehospitalization from skilled nursing facilities. Health Aff (Millwood). 2010;29(1):57–64. , , , .
- Rehospitalizations among patients in the Medicare fee‐for‐service program. N Engl J Med. 2009;360(14):1418–1428. , , .
- Predictors of rehospitalization among elderly patients admitted to a rehabilitation hospital: the role of polypharmacy, functional status, and length of stay. J Am Med Dir Assoc. 2013;14(10):761–767. , , , et al.
- Mobility after hospital discharge as a marker for 30‐day readmission. J Gerontol A Biol Sci Med Sci. 2013;68(7):805–810. , , , et al.
- The association between the quality of inpatient care and early readmission: a meta‐analysis of the evidence. Med Care. 1997;35(10):1044–1059. , , , , .
- Association of impaired functional status at hospital discharge and subsequent rehospitalization. J Hosp Med. May 2014;9(5):277–282. , , , , , .
- A prospective cohort study of geriatric syndromes among older medical patients admitted to acute care hospitals. J Am Geriatr Soc. 2011;59(11):2001–2008. , , , , , .
- Hospital discharge instructions: comprehension and compliance among older adults. J Gen Intern Med. 2014;29(11):1491–1498. , , , et al.
- Problems after discharge and understanding of communication with their primary care physicians among hospitalized seniors: a mixed methods study. J Hosp Med. 2010;5(7):385–391. , , , et al.
- Communication and information deficits in patients discharged to rehabilitation facilities: an evaluation of five acute care hospitals. J Hosp Med. 2009;4(8):E28–E33. , , , et al.
- The consequences of poor communication during transitions from hospital to skilled nursing facility: a qualitative study. J Am Geriatr Soc. 2013;61(7):1095–1102. , , , , , .
- Deficits in communication and information transfer between hospital‐based and primary care physicians: implications for patient safety and continuity of care. JAMA. 2007;297(8):831–841. , , , , , .
- Moving beyond readmission penalties: creating an ideal process to improve transitional care. J Hosp Med. 2013;8(2):102–109. , , , .
- Low‐cost transitional care with nurse managers making mostly phone contact with patients cut rehospitalization at a VA hospital. Health Aff (Millwood). 2012;31(12):2659–2668. , , , et al.
- Transitional care interventions to prevent readmissions for persons with heart failure: a systematic review and meta‐analysis. Ann Intern Med. 2014;160(11):774–784. , , , et al.
- Interventions to reduce 30‐day rehospitalization: a systematic review. Ann Intern Med. 2011;155(8):520–528. , , , , .
- Hospital‐initiated transitional care interventions as a patient safety strategy: a systematic review. Ann Intern Med. 2013;158(5 pt 2):433–440. , , , , , .
- Epidemiology and risk factors for delirium across hospital settings. Best Pract Res Clin Anaesthesiol. 2012;26(3):277–287. , , , .
- Does delirium contribute to poor hospital outcomes? A three‐site epidemiologic study. J Gen Intern Med. 1998;13(4):234–242. , , , , .
- Incidence, risk factors and consequences of ICU delirium. Intensive Care Med. 2007;33(1):66–73. , , , .
- Delirium in mechanically ventilated patients: validity and reliability of the confusion assessment method for the intensive care unit (CAM‐ICU). JAMA. 2001;286(21):2703–2710. , , , et al.
- Impact and recognition of cognitive impairment among hospitalized elders. J Hosp Med. 2010;5(2):69–75. , , , et al.
- The impact of delirium in the intensive care unit on hospital length of stay. Intensive Care Med. 2001;27(12):1892–1900. , , , et al.
- Long‐term cognitive impairment after critical illness. N Engl J Med. 2014;370(2):185–186. , , .
- Delirium in the ICU and subsequent long‐term disability among survivors of mechanical ventilation. Crit Care Med. 2014;42(2):369–377. , , , et al.
- Delirium in elderly patients and the risk of postdischarge mortality, institutionalization, and dementia: a meta‐analysis. JAMA. 2010;304(4):443–451. , , , , , .
- Delirium as a predictor of mortality in mechanically ventilated patients in the intensive care unit. JAMA. 2004;291(14):1753–1762. , , , et al.
- Days of delirium are associated with 1‐year mortality in an older intensive care unit population. Am J Respir Crit Care Med. 2009;180(11):1092–1097. , , , , , .
- In‐facility delirium prevention programs as a patient safety strategy: a systematic review. Ann Intern Med. 2013;158(5 pt 2):375–380. , .
- Dexmedetomidine vs midazolam for sedation of critically ill patients: a randomized trial. JAMA. 2009;301(5):489–499. , , , et al.
- Effect of sedation with dexmedetomidine vs lorazepam on acute brain dysfunction in mechanically ventilated patients: the MENDS randomized controlled trial. JAMA. 2007;298(22):2644–2653. , , , et al.
- Protocolized intensive care unit management of analgesia, sedation, and delirium improves analgesia and subsyndromal delirium rates. Anesth Analg. 2010;111(2):451–463. , , , , , .
- A systematic review of the prevalence, associations and outcomes of dementia in older general hospital inpatients. Int Psychogeriatr. 2011;23(3):344–355. , .
- Cognitive impairment is undetected in medical inpatients: a study of mortality and recognition amongst healthcare professionals. BMC Geriatr. 2012;12:47. , , , .
- How can we keep patients with dementia safe in our acute hospitals? A review of challenges and solutions. J R Soc Med. 2013;106(9):355–361. , , .
- Screening for dementia in general hospital inpatients: a systematic review and meta‐analysis of available instruments. Age Ageing. 2013;42(6):689–695. , , .
- Tools to detect delirium superimposed on dementia: a systematic review. J Am Geriatr Soc. 2012;60(11):2005–2013. , , , et al.
- Functional analysis‐based interventions for challenging behaviour in dementia. Cochrane Database Syst Rev. 2012;2:CD006929. , , , , , .
- Cognitive stimulation to improve cognitive functioning in people with dementia. Cochrane Database Syst Rev. 2012;2:CD005562. , , , .
- The prevalence and correlates of major and minor depression in older medical inpatients. J Am Geriatr Soc. 2005;53(8):1344–1353. , , , et al.
- Major depressive disorder in hospitalized medically ill patients: an examination of young and elderly male veterans. J Am Geriatr Soc. 1991;39(9):881–890. , , , , , .
- Depression, post‐traumatic stress disorder, and functional disability in survivors of critical illness in the BRAIN‐ICU study: a longitudinal cohort study. Lancet Respir Med. 2014;2(5):369–379. , , , et al.
- Depressive symptoms after hospitalization in older adults: function and mortality outcomes. J Am Geriatr Soc. 2012;60(12):2254–2262. , , , et al.
- 12‐month cognitive outcomes of major and minor depression in older medical patients. Am J Geriatr Psychiatry. 2008;16(9):742–751. , , , , .
- Depression is a risk factor for rehospitalization in medical inpatients. Prim Care Companion J Clin Psychiatry. 2007;9(4):256–262. , , , et al.
- Dose‐response relationship between depressive symptoms and hospital readmission. J Hosp Med. 2014;9(6):358–364. , , , , , .
- Depressive symptoms and 3‐year mortality in older hospitalized medical patients. Ann Intern Med. 1999;130(7):563–569. , , , , , .
- Support for the vascular depression hypothesis in late‐life depression: results of a 2‐site, prospective, antidepressant treatment trial. Arch Gen Psychiatry. 2010;67(3):277–285. , , , et al.
- Executive dysfunction and the course of geriatric depression. Biol Psychiatry. 2005;58(3):204–210. , , , , , .
- Recognition of depression in older medical inpatients. J Gen Intern Med. 2007;22(5):559–564. , , , , .
- A collaborative care depression management program for cardiac inpatients: depression characteristics and in‐hospital outcomes. Psychosomatics. 2011;52(1):26–33. , , , , , .
- Impact of a depression care management program for hospitalized cardiac patients. Circ Cardiovasc Qual Outcomes. 2011;4(2):198–205. , , , , , .
- Potentially inappropriate medication use in hospitalized elders. J Hosp Med. 2008;3(2):91–102. , , , et al.
- Prevalence, incidence and nature of prescribing errors in hospital inpatients: a systematic review. Drug Saf. 2009;32(5):379–389. , , , , , .
- Minimizing adverse drug events in older patients. Am Fam Physician. 2007;76(12):1837–1844. , .
- STOPP (Screening Tool of Older Person's Prescriptions) and START (Screening Tool to Alert doctors to Right Treatment). Consensus validation. Int J Clin Pharmacol Ther. 2008;46(2):72–83. , , , , .
- American Geriatrics Society 2012 Beers Criteria Update Expert Panel. American Geriatrics Society updated Beers Criteria for potentially inappropriate medication use in older adults. J Am Geriatr Soc. 2012;60(4):616–631.
- Preventing potentially inappropriate medication use in hospitalized older patients with a computerized provider order entry warning system. Arch Intern Med. 2010;170(15):1331–1336. , , , .
- Hazards of Hospitalization of the Elderly. Ann Intern Med. 1993;118(3):219–223. .
- Improving health care for older persons. Ann Intern Med. 2003;139(5 part 2):421–424. .
- Successful models of comprehensive care for older adults with chronic conditions: evidence for the Institute of Medicine's "retooling for an aging America" report. J Am Geriatr Soc. 2009;57(12):2328–2337. , , , , , .
- Recovery of activities of daily living in older adults after hospitalization for acute medical illness. J Am Geriatr Soc. 2008;56(12):2171–2179. , , , et al.
- Changes in functional status and the risks of subsequent nursing home placement and death. J Gerontol. 1993;48(3):S94–S101. , , , .
- Risk factors for nursing home placement in a population‐based dementia cohort. J Am Geriatr Soc. 2000;48(5):519–525. , , .
- The underrecognized epidemic of low mobility during hospitalization of older adults. J Am Geriatr Soc. 2009;57(9):1660–1665. , , , .
- A systematic review of predictors and screening instruments to identify older hospitalized patients at risk for functional decline. J Clin Nurs. 2007;16(1):46–57. , , , , .
- Immobility and falls. Clin Geriatr Med. 1998;14(4):699–726. .
- Prevalence and outcomes of low mobility in hospitalized older patients. J Am Geriatr Soc. 2004;52(8):1263–1270. , , .
- Impact of a nurse‐driven mobility protocol on functional decline in hospitalized older adults. J Nurs Care Qual. 2009;24(4):325–331. , , .
- Impact of early mobilization protocol on the medical‐surgical inpatient population: an integrated review of literature. Clin Nurse Spec. 2012;26(2):87–94. , .
- The aging population and its impact on the surgery workforce. Ann Surg. 2003;238(2):170–177. , , , .
- Perioperative care of the elderly patient: an update. Cleve Clin J Med. 2009;76(suppl 4):S16–S21. .
- Frailty in the older surgical patient: a review. Age Ageing. 2012;41(2):142–147. , , .
- The assessment and management of peri‐operative pain in older adults. Anaesthesia. 2014;69(suppl 1):54–60. .
- National Research Strategies: what outcomes are important in peri‐operative elderly care? Anaesthesia. 2014;69(suppl 1):61–69. , .
- 2002 National Hospital Discharge Survey. Adv Data. 2004;342:1–30. , .
- Hospitalization in the United States, 2002. Rockville, MD: Agency for Healthcare Research and Quality; 2005. , .
- The Curriculum for the Hospitalized Aging Medical Patient program: a collaborative faculty development program for hospitalists, general internists, and geriatricians. J Hosp Med. 2008;3(5):384–393. , , , et al.
- Advancement of geriatrics education. J Hosp Med. 2011;6(6):370. , .
- Advancing geriatrics education: an efficient faculty development program for academic hospitalists increases geriatric teaching. J Hosp Med. 2010;5(9):541–546. , , , , , .
- National Hospital Discharge Survey: 2007 summary. Natl Health Stat Report. 2010(29):1–20, 24. , , , , .
- Centers for Medicare 2012. Available at: http://www.cms.gov/Research‐Statistics‐Data‐and‐Systems/Statistics‐Trends‐and‐Reports/Chronic‐Conditions/Downloads/2012Chartbook.pdf. Accessed December 12, 2014.
- Growth in the care of older patients by hospitalists in the United States. N Engl J Med. 2009;360(11):1102–1112. , , , .
- A revelation of numbers: will America's eldercare workforce be ready to care for an aging America? Generations. 2010;34(4):11–19. , .
- Patient‐Centered Outcomes Research Institute Methodology Committee. The PCORI methodology report. Available at: http://www.pcori.org/assets/2013/11/PCORI‐Methodology‐Report.pdf. Published November 2013. Accessed December 19, 2013.
- The James Lind Alliance. JLA method. Available at: http://www.lindalliance.org/JLA_Method.asp. Accessed December 19, 2013.
- Road map to a patient‐centered research agenda at the intersection of hospital medicine and geriatric medicine. J Gen Intern Med. 2014;29(6):926–931. , , , , .
- Patient‐Centered Outcomes Research Institute. About us. Available at: http://www.pcori.org/about‐us. Accessed February 23, 2015.
- New Frontiers of Geriatrics Research: An Agenda for Surgical and Related Medical Specialties. New York, NY: American Geriatrics Society; 2004. , , .
- Linking the NIH strategic plan to the research agenda for social workers in health and aging. J Gerontol Soc Work. 2010;53(1):77–93. , , , .
- Assessing the capacity to make everyday decisions: a guide for clinicians and an agenda for future research. Am J Geriatr Psychiatry. 2007;15(2):101–111. , .
- Practitioners' views on elder mistreatment research priorities: recommendations from a Research‐to‐Practice Consensus conference. J Elder Abuse Negl. 2011;23(2):115–126. , , , et al.
- The intersection between geriatrics and palliative care: a call for a new research agenda. J Am Geriatr Soc. 2005;53(9):1593–1598. , .
- The cancer aging interface: a research agenda. J Clin Oncol. 2007;25(14):1945–1948. .
- Clinical care of persons with dementia in the emergency department: a review of the literature and agenda for research. J Am Geriatr Soc. 2012;60(9):1742–1748. , , , .
- A group process model for problem identification and program planning. J Appl Behav Sci. 1971;7(4):466–492. , .
- Research and publication trends in hospital medicine. J Hosp Med. 2014;9(3):148–154. , , , , .
- ABFM and ABIM to jointly participate in recognition of focused practice (rfp) in hospital medicine pilot approved by abms. Ann Fam Med. 2010;8(1):87. .
- Medical decision‐making for older adults without family. J Am Geriatr Soc. 2012;60(11):2144–2150. , , , .
- Promoting advance directives among elderly primary care patients. J Gen Intern Med. 2004;19(9):944–951. , , , , , .
- Advance directive completion by elderly Americans: a decade of change. J Am Geriatr Soc. 2014;62(4):706–710. , , .
- Care transitions by older adults from nursing homes to hospitals: implications for long‐term care practice, geriatrics education, and research. J Am Med Dir Assoc. 2010;11(4):231–238. , .
- Decisions to hospitalize nursing home residents dying with advanced dementia. J Am Geriatr Soc. 2005;53(8):1396–1401. , , , .
- A comparison of methods to communicate treatment preferences in nursing facilities: traditional practices versus the physician orders for life‐sustaining treatment program. J Am Geriatr Soc. 2010;58(7):1241–1248. , , , , , .
- Interventions to improve transitional care between nursing homes and hospitals: a systematic review. J Am Geriatr Soc. 2010;58(4):777–782. , , , , .
- Opening end‐of‐life discussions: how to introduce Voicing My CHOiCES, an advance care planning guide for adolescents and young adults [published online ahead of print March 13, 2014]. Palliat Support Care. doi: 10.1017/S1478951514000054. , , .
- The revolving door of rehospitalization from skilled nursing facilities. Health Aff (Millwood). 2010;29(1):57–64. , , , .
- Rehospitalizations among patients in the Medicare fee‐for‐service program. N Engl J Med. 2009;360(14):1418–1428. , , .
- Predictors of rehospitalization among elderly patients admitted to a rehabilitation hospital: the role of polypharmacy, functional status, and length of stay. J Am Med Dir Assoc. 2013;14(10):761–767. , , , et al.
- Mobility after hospital discharge as a marker for 30‐day readmission. J Gerontol A Biol Sci Med Sci. 2013;68(7):805–810. , , , et al.
- The association between the quality of inpatient care and early readmission: a meta‐analysis of the evidence. Med Care. 1997;35(10):1044–1059. , , , , .
- Association of impaired functional status at hospital discharge and subsequent rehospitalization. J Hosp Med. May 2014;9(5):277–282. , , , , , .
- A prospective cohort study of geriatric syndromes among older medical patients admitted to acute care hospitals. J Am Geriatr Soc. 2011;59(11):2001–2008. , , , , , .
- Hospital discharge instructions: comprehension and compliance among older adults. J Gen Intern Med. 2014;29(11):1491–1498. , , , et al.
- Problems after discharge and understanding of communication with their primary care physicians among hospitalized seniors: a mixed methods study. J Hosp Med. 2010;5(7):385–391. , , , et al.
- Communication and information deficits in patients discharged to rehabilitation facilities: an evaluation of five acute care hospitals. J Hosp Med. 2009;4(8):E28–E33. , , , et al.
- The consequences of poor communication during transitions from hospital to skilled nursing facility: a qualitative study. J Am Geriatr Soc. 2013;61(7):1095–1102. , , , , , .
- Deficits in communication and information transfer between hospital‐based and primary care physicians: implications for patient safety and continuity of care. JAMA. 2007;297(8):831–841. , , , , , .
- Moving beyond readmission penalties: creating an ideal process to improve transitional care. J Hosp Med. 2013;8(2):102–109. , , , .
- Low‐cost transitional care with nurse managers making mostly phone contact with patients cut rehospitalization at a VA hospital. Health Aff (Millwood). 2012;31(12):2659–2668. , , , et al.
- Transitional care interventions to prevent readmissions for persons with heart failure: a systematic review and meta‐analysis. Ann Intern Med. 2014;160(11):774–784. , , , et al.
- Interventions to reduce 30‐day rehospitalization: a systematic review. Ann Intern Med. 2011;155(8):520–528. , , , , .
- Hospital‐initiated transitional care interventions as a patient safety strategy: a systematic review. Ann Intern Med. 2013;158(5 pt 2):433–440. , , , , , .
- Epidemiology and risk factors for delirium across hospital settings. Best Pract Res Clin Anaesthesiol. 2012;26(3):277–287. , , , .
- Does delirium contribute to poor hospital outcomes? A three‐site epidemiologic study. J Gen Intern Med. 1998;13(4):234–242. , , , , .
- Incidence, risk factors and consequences of ICU delirium. Intensive Care Med. 2007;33(1):66–73. , , , .
- Delirium in mechanically ventilated patients: validity and reliability of the confusion assessment method for the intensive care unit (CAM‐ICU). JAMA. 2001;286(21):2703–2710. , , , et al.
- Impact and recognition of cognitive impairment among hospitalized elders. J Hosp Med. 2010;5(2):69–75. , , , et al.
- The impact of delirium in the intensive care unit on hospital length of stay. Intensive Care Med. 2001;27(12):1892–1900. , , , et al.
- Long‐term cognitive impairment after critical illness. N Engl J Med. 2014;370(2):185–186. , , .
- Delirium in the ICU and subsequent long‐term disability among survivors of mechanical ventilation. Crit Care Med. 2014;42(2):369–377. , , , et al.
- Delirium in elderly patients and the risk of postdischarge mortality, institutionalization, and dementia: a meta‐analysis. JAMA. 2010;304(4):443–451. , , , , , .
- Delirium as a predictor of mortality in mechanically ventilated patients in the intensive care unit. JAMA. 2004;291(14):1753–1762. , , , et al.
- Days of delirium are associated with 1‐year mortality in an older intensive care unit population. Am J Respir Crit Care Med. 2009;180(11):1092–1097. , , , , , .
- In‐facility delirium prevention programs as a patient safety strategy: a systematic review. Ann Intern Med. 2013;158(5 pt 2):375–380. , .
- Dexmedetomidine vs midazolam for sedation of critically ill patients: a randomized trial. JAMA. 2009;301(5):489–499. , , , et al.
- Effect of sedation with dexmedetomidine vs lorazepam on acute brain dysfunction in mechanically ventilated patients: the MENDS randomized controlled trial. JAMA. 2007;298(22):2644–2653. , , , et al.
- Protocolized intensive care unit management of analgesia, sedation, and delirium improves analgesia and subsyndromal delirium rates. Anesth Analg. 2010;111(2):451–463. , , , , , .
- A systematic review of the prevalence, associations and outcomes of dementia in older general hospital inpatients. Int Psychogeriatr. 2011;23(3):344–355. , .
- Cognitive impairment is undetected in medical inpatients: a study of mortality and recognition amongst healthcare professionals. BMC Geriatr. 2012;12:47. , , , .
- How can we keep patients with dementia safe in our acute hospitals? A review of challenges and solutions. J R Soc Med. 2013;106(9):355–361. , , .
- Screening for dementia in general hospital inpatients: a systematic review and meta‐analysis of available instruments. Age Ageing. 2013;42(6):689–695. , , .
- Tools to detect delirium superimposed on dementia: a systematic review. J Am Geriatr Soc. 2012;60(11):2005–2013. , , , et al.
- Functional analysis‐based interventions for challenging behaviour in dementia. Cochrane Database Syst Rev. 2012;2:CD006929. , , , , , .
- Cognitive stimulation to improve cognitive functioning in people with dementia. Cochrane Database Syst Rev. 2012;2:CD005562. , , , .
- The prevalence and correlates of major and minor depression in older medical inpatients. J Am Geriatr Soc. 2005;53(8):1344–1353. , , , et al.
- Major depressive disorder in hospitalized medically ill patients: an examination of young and elderly male veterans. J Am Geriatr Soc. 1991;39(9):881–890. , , , , , .
- Depression, post‐traumatic stress disorder, and functional disability in survivors of critical illness in the BRAIN‐ICU study: a longitudinal cohort study. Lancet Respir Med. 2014;2(5):369–379. , , , et al.
- Depressive symptoms after hospitalization in older adults: function and mortality outcomes. J Am Geriatr Soc. 2012;60(12):2254–2262. , , , et al.
- 12‐month cognitive outcomes of major and minor depression in older medical patients. Am J Geriatr Psychiatry. 2008;16(9):742–751. , , , , .
- Depression is a risk factor for rehospitalization in medical inpatients. Prim Care Companion J Clin Psychiatry. 2007;9(4):256–262. , , , et al.
- Dose‐response relationship between depressive symptoms and hospital readmission. J Hosp Med. 2014;9(6):358–364. , , , , , .
- Depressive symptoms and 3‐year mortality in older hospitalized medical patients. Ann Intern Med. 1999;130(7):563–569. , , , , , .
- Support for the vascular depression hypothesis in late‐life depression: results of a 2‐site, prospective, antidepressant treatment trial. Arch Gen Psychiatry. 2010;67(3):277–285. , , , et al.
- Executive dysfunction and the course of geriatric depression. Biol Psychiatry. 2005;58(3):204–210. , , , , , .
- Recognition of depression in older medical inpatients. J Gen Intern Med. 2007;22(5):559–564. , , , , .
- A collaborative care depression management program for cardiac inpatients: depression characteristics and in‐hospital outcomes. Psychosomatics. 2011;52(1):26–33. , , , , , .
- Impact of a depression care management program for hospitalized cardiac patients. Circ Cardiovasc Qual Outcomes. 2011;4(2):198–205. , , , , , .
- Potentially inappropriate medication use in hospitalized elders. J Hosp Med. 2008;3(2):91–102. , , , et al.
- Prevalence, incidence and nature of prescribing errors in hospital inpatients: a systematic review. Drug Saf. 2009;32(5):379–389. , , , , , .
- Minimizing adverse drug events in older patients. Am Fam Physician. 2007;76(12):1837–1844. , .
- STOPP (Screening Tool of Older Person's Prescriptions) and START (Screening Tool to Alert doctors to Right Treatment). Consensus validation. Int J Clin Pharmacol Ther. 2008;46(2):72–83. , , , , .
- American Geriatrics Society 2012 Beers Criteria Update Expert Panel. American Geriatrics Society updated Beers Criteria for potentially inappropriate medication use in older adults. J Am Geriatr Soc. 2012;60(4):616–631.
- Preventing potentially inappropriate medication use in hospitalized older patients with a computerized provider order entry warning system. Arch Intern Med. 2010;170(15):1331–1336. , , , .
- Hazards of Hospitalization of the Elderly. Ann Intern Med. 1993;118(3):219–223. .
- Improving health care for older persons. Ann Intern Med. 2003;139(5 part 2):421–424. .
- Successful models of comprehensive care for older adults with chronic conditions: evidence for the Institute of Medicine's "retooling for an aging America" report. J Am Geriatr Soc. 2009;57(12):2328–2337. , , , , , .
- Recovery of activities of daily living in older adults after hospitalization for acute medical illness. J Am Geriatr Soc. 2008;56(12):2171–2179. , , , et al.
- Changes in functional status and the risks of subsequent nursing home placement and death. J Gerontol. 1993;48(3):S94–S101. , , , .
- Risk factors for nursing home placement in a population‐based dementia cohort. J Am Geriatr Soc. 2000;48(5):519–525. , , .
- The underrecognized epidemic of low mobility during hospitalization of older adults. J Am Geriatr Soc. 2009;57(9):1660–1665. , , , .
- A systematic review of predictors and screening instruments to identify older hospitalized patients at risk for functional decline. J Clin Nurs. 2007;16(1):46–57. , , , , .
- Immobility and falls. Clin Geriatr Med. 1998;14(4):699–726. .
- Prevalence and outcomes of low mobility in hospitalized older patients. J Am Geriatr Soc. 2004;52(8):1263–1270. , , .
- Impact of a nurse‐driven mobility protocol on functional decline in hospitalized older adults. J Nurs Care Qual. 2009;24(4):325–331. , , .
- Impact of early mobilization protocol on the medical‐surgical inpatient population: an integrated review of literature. Clin Nurse Spec. 2012;26(2):87–94. , .
- The aging population and its impact on the surgery workforce. Ann Surg. 2003;238(2):170–177. , , , .
- Perioperative care of the elderly patient: an update. Cleve Clin J Med. 2009;76(suppl 4):S16–S21. .
- Frailty in the older surgical patient: a review. Age Ageing. 2012;41(2):142–147. , , .
- The assessment and management of peri‐operative pain in older adults. Anaesthesia. 2014;69(suppl 1):54–60. .
- National Research Strategies: what outcomes are important in peri‐operative elderly care? Anaesthesia. 2014;69(suppl 1):61–69. , .
- 2002 National Hospital Discharge Survey. Adv Data. 2004;342:1–30. , .
- Hospitalization in the United States, 2002. Rockville, MD: Agency for Healthcare Research and Quality; 2005. , .
- The Curriculum for the Hospitalized Aging Medical Patient program: a collaborative faculty development program for hospitalists, general internists, and geriatricians. J Hosp Med. 2008;3(5):384–393. , , , et al.
- Advancement of geriatrics education. J Hosp Med. 2011;6(6):370. , .
- Advancing geriatrics education: an efficient faculty development program for academic hospitalists increases geriatric teaching. J Hosp Med. 2010;5(9):541–546. , , , , , .
Memory and Sleep in Hospital Patients
Hospitalization is often utilized as a teachable moment, as patients are provided with education about treatment and disease management, particularly at discharge.[1, 2, 3] However, memory impairment among hospitalized patients may undermine the utility of the teachable moment. In one study of community‐dwelling seniors admitted to the hospital, one‐third had previously unrecognized poor memory at discharge.[4]
Sleep loss may be an underappreciated contributor to short‐term memory deficits in inpatients, particularly in seniors, who have baseline higher rates of sleep disruptions and sleep disorders.[5] Patients often receive 2 hours less sleep than at home and experience poor quality sleep due to disruptions.[6, 7] Robust studies of healthy subjects in laboratory settings demonstrate that sleep loss leads to decreased attention and worse recall, and that more sleep is associated with better memory performance.[8, 9]
Very few studies have examined memory in hospitalized patients. Although word‐list tasks are often used to assess memory because they are quick and easy to administer, these tasks may not accurately reflect memory for a set of instructions provided at patient discharge. Finally, no studies have examined the association between inpatient sleep loss and memory. Thus, our primary aim in this study was to examine memory performance in older, hospitalized patients using a word listbased memory task and a more complex medical vignette task. Our second aim was to investigate the relationship between in‐hospital sleep and memory.
METHODS
Study Design
We conducted a prospective cohort study with subjects enrolled in an ongoing sleep study at the University of Chicago Medical Center.[10] Eligible subjects were on the general medicine or hematology/oncology service, at least 50 years old, community dwelling, ambulatory, and without detectable cognitive impairment on the Mini Mental State Exam[11] or Short Portable Mental Status Questionnaire.[12, 13] Patients were excluded if they had a documented sleep disorder (ie, obstructive sleep apnea), were transferred from an intensive care unit or were in droplet or airborne isolation, had a bedrest order, or had already spent over 72 hours in the hospital prior to enrollment. These criteria were used to select a population appropriate for wristwatch actigraphy and with low likelihood of baseline memory impairment. The University of Chicago Institutional Review Board approved this study, and participants provided written consent.
Data Collection
Memory Testing
Memory was evaluated using the University of Southern California Repeatable Episodic Memory Test (USC‐REMT), a validated verbal memory test in which subjects listen to a list of 15 words and then complete free‐recall and recognition of the list.[14, 15] Free‐recall tests subjects' ability to procure information without cues. In contrast, recognition requires subjects to pick out the words they just heard from distractors, an easier task. The USC‐REMT contains multiple functionally equivalent different word lists, and may be administered more than once to the same subject without learning effects.[15] Immediate and delayed memory were tested by asking the subject to complete the tasks immediately after listening to the word list and 24‐hours after listening to the list, respectively.
Immediate Recall and Recognition
Recall and recognition following a night of sleep in the hospital was the primary outcome for this study. After 1 night of actigraphy recorded sleep, subjects listened as a 15‐item word list (word list A) was read aloud. For the free‐recall task, subjects were asked to repeat back all the words they could remember immediately after hearing the list. For the recognition task, subjects were read a new list of 15 words, including a mix of words from the previous list and new distractor words. They answered yes if they thought the word had previously been read to them and no if they thought the word was new.
Delayed Recall and Delayed Recognition
At the conclusion of study enrollment on day 1 prior to the night of actigraphy, subjects were shown a laminated paper with a printed word list (word list B) from the USC‐REMT. They were given 2 minutes to study the sheet and were informed they would be asked to remember the words the following day. One day later, after the night of actigraphy recorded sleep, subjects completed the free recall and yes/no recognition task based on what they remembered from word list B. This established delayed recall and recognition scores.
Medical Vignette
Because it is unclear how word recall and recognition tasks approximate remembering discharge instructions, we developed a 5‐sentence vignette about an outpatient medical encounter, based on the logical memory component of the Wechsler Memory Scale IV, a commonly used, validated test of memory assessment.[16, 17] After the USC‐REMT was administered following a night of sleep in the hospital, patients listened to a story and were immediately asked to repeat back in free form as much information as possible from the story. Responses were recorded by trained research assistants. The story is comprised of short sentences with simple ideas and vocabulary (see Supporting Information, Appendix 1, in the online version of this article).
Sleep: Wrist Actigraphy and Karolinska Sleep Log
Patient sleep was measured by actigraphy following the protocol described previously by our group.[7] Patients wore a wrist actigraphy monitor (Actiwatch 2; Philips Respironics, Inc., Murrysville, PA) to collect data on sleep duration and quality. The monitor detects wrist movement by measuring acceleration.[18] Actigraphy has been validated against polysomnography, demonstrating a correlation in sleep duration of 0.82 in insomniacs and 0.97 in healthy subjects.[19] Sleep duration and sleep efficiency overnight were calculated from the actigraphy data using Actiware 5 software.[20] Sleep duration was defined by the software based on low levels of recorded movement. Sleep efficiency was calculated as the percentage of time asleep out of the subjects' self‐reported time in bed, which was obtained using the Karolinska Sleep Log.[21]
The Karolinska Sleep Log questionnaire also asks patients to rate their sleep quality, restlessness during sleep, ease of falling asleep and the ability to sleep through the night on a 5‐point scale. The Karolinska Sleep Quality Index (KSQI) is calculated by averaging the latter 4 items.[22] A score of 3 or less classifies the subject in an insomniac range.[7, 21]
Demographic Information
Demographic information, including age, race, and gender were obtained by chart audit.
Data Analysis
Data were entered into REDCap, a secure online tool for managing survey data.[23]
Memory Scoring
For immediate and delayed recall scores, subjects received 1 point for every word they remembered correctly, with a maximum score of 15 words. We defined poor memory on the immediate recall test as a score of 3 or lower, based on a score utilized by Lindquist et al.[4] in a similar task. This score was less than half of the mean score of 6.63 obtained by Parker et al. for a sample of healthy 60 to 79 year olds in a sensitivity study of the USC‐REMT.[14] For immediate and delayed recognition, subjects received 1 point for correctly identifying whether a word had been on the word list they heard or whether it was a distractor, with a maximum score of 15.
A key was created to standardize scoring of the medical vignette by assigning 1 point to specific correctly remembered items from the story (see Supporting Information, Appendix 2A, in the online version of this article). These points were added to obtain a total score for correctly remembered vignette items. It was also noted when a vignette item was remembered incorrectly, for example, when the patient remembered left foot instead of right foot. Each incorrectly remembered item received 1 point, and these were summed to create the total score for incorrectly remembered vignette items (see Supporting Information, Appendix 2A, in the online version of this article for the scoring guide). Forgotten items were assigned 0 points. Two independent raters scored each subject's responses, and their scores were averaged for each item. Inter‐rater reliability was calculated as percentage of agreement across responses.
Statistical Analysis
Descriptive statistics were performed on the memory task data. Tests for skew and curtosis were performed for recall and recognition task data. The mean and standard deviation (SD) were calculated for normally distributed data, and the median and interquartile range (IQR) were obtained for data that showed significant skew. Mean and SD were also calculated for sleep duration and sleep efficiency measured by actigraphy.
Two‐tailed t tests were used to examine the association between memory and gender and African American race. Cuzick's nonparametric test of trend was used to test the association between age quartile and recall and recognition scores.[24] Mean and standard deviation for the correct total score and incorrect total score for the medical vignette were calculated. Pearson's correlation coefficient was used to examine the association between USC‐REMT memory measures and medical vignette score.
Pearson's correlation coefficient was calculated to test the associations between sleep duration and memory scores (immediate and delayed recall, immediate and delayed recognition, medical vignette task). This test was repeated to examine the relationship between sleep efficiency and the above memory scores. Linear regression models were used to characterize the relationship between inpatient sleep duration and efficiency and memory task performance. Two‐tailed t tests were used to compare sleep metrics (duration and efficiency) between high‐ and low‐memory groups, with low memory defined as immediate recall of 3 words.
All statistical tests were conducted using Stata 12.0 software (StataCorp, College Station, TX). Statistical significance was defined as P<0.05.
RESULTS
From April 11, 2013 to May 3, 2014, 322 patients were eligible for our study. Of these, 99 patients were enrolled in the study. We were able to collect sleep actigraphy data and immediate memory scores from 59 on day 2 of the study (Figure 1).
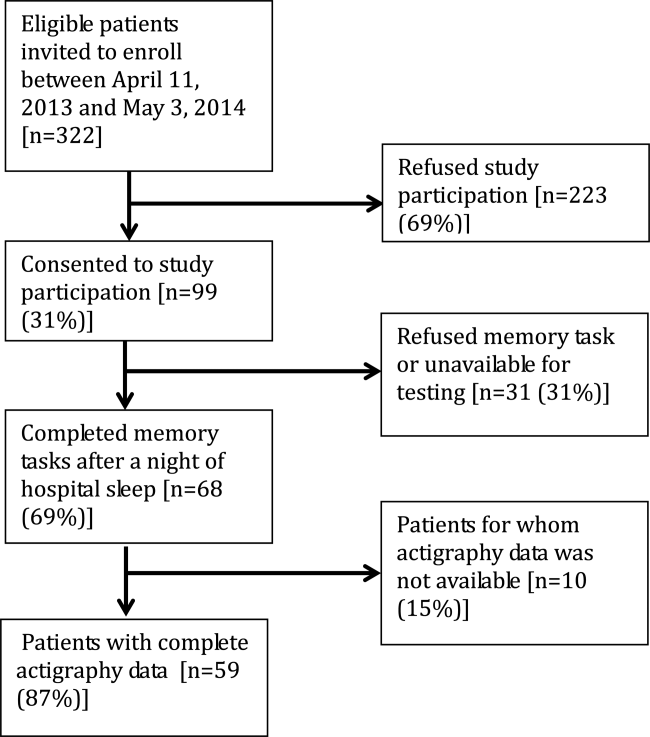
The study population had a mean age of 61.6 years (SD=9.3 years). Demographic information is presented in Table 1. Average nightly sleep in the hospital was 5.44 hours (326.4 minutes, SD=134.5 minutes), whereas mean sleep efficiency was 70.9 (SD=17.1), which is below the normal threshold of 85%.[25, 26] Forty‐four percent had a KSQI score of 3, representing in‐hospital sleep quality in the insomniac range.
Value | |
---|---|
| |
Patient characteristics | |
Age, y, mean (SD) | 61.6 (9.3) |
Female, n (%) | 36 (61.0%) |
BMI, n (%) | |
Underweight (<18.5) | 3 (5.1%) |
Normal weight (18.524.9) | 16 (27.1%) |
Overweight (25.029.9) | 14 (23.7%) |
Obese (30.0) | 26 (44.1%) |
African American, n (%) | 43 (72.9%) |
Non‐Hispanic, n (%) | 57 (96.6%) |
Education, n (%) | |
Did not finish high school | 13 (23.2%) |
High school graduate | 13 (23.2%) |
Some college or junior college | 16 (28.6%) |
College graduate or postgraduate degree | 13 (23.2%) |
Discharge diagnosis (ICD‐9‐CM classification), n (%) | |
Circulatory system disease | 5 (8.5%) |
Digestive system disease | 9 (15.3%) |
Genitourinary system disease | 4 (6.8%) |
Musculoskeletal system disease | 3 (5.1%) |
Respiratory system disease | 5 (8.5%) |
Sensory organ disease | 1 (1.7%) |
Skin and subcutaneous tissue disease | 3 (5.1%) |
Endocrine, nutritional, and metabolic disease | 7 (11.9%) |
Infection and parasitic disease | 6 (10.2%) |
Injury and poisoning | 4 (6.8%) |
Mental disorders | 2 (3.4%) |
Neoplasm | 5 (8.5%) |
Symptoms, signs, and ill‐defined conditions | 5 (8.5%) |
Comorbidities by self‐report, n=57, n (%) | |
Cancer | 6 (10.5%) |
Depression | 15 (26.3%) |
Diabetes | 15 (26.3%) |
Heart trouble | 16 (28.1%) |
HIV/AIDS | 2 (3.5%) |
Kidney disease | 10 (17.5%) |
Liver disease | 9 (15.8%) |
Stroke | 4 (7.0%) |
Subject on the hematology and oncology service, n (%) | 6 (10.2%) |
Sleep characteristics | |
Nights in hospital prior to enrollment, n (%) | |
0 nights | 12 (20.3%) |
1 night | 24 (40.7%) |
2 nights | 17 (28.8%) |
3 nights | 6 (10.1%) |
Received pharmacologic sleep aids, n (%) | 10 (17.0%) |
Karolinska Sleep Quality Index scores, score 3, n (%) | 26 (44.1%) |
Sleep duration, min, mean (SD) | 326.4 (134.5) |
Sleep efficiency, %, mean (SD) | 70.9 (17.1) |
Memory test scores are presented in Figure 2. Nearly half (49%) of patients had poor memory, defined by a score of 3 words (Figure 2). Immediate recall scores varied significantly with age quartile, with older subjects recalling fewer words (Q1 [age 50.453.6 years] mean=4.9 words; Q2 [age 54.059.2 years] mean=4.1 words; Q3 [age 59.466.9 years] mean=3.7 words; Q4 [age 68.285.0 years] mean=2.5 words; P=0.001). Immediate recognition scores did not vary significantly by age quartile (Q1 [age 50.453.6 years] mean=10.3 words; Q2 [age 54.059.2 years] mean =10.3 words; Q3 [age 59.466.9 years)] mean=11.8 words; Q4 [age 68.285.0 years] mean=10.4 words; P=0.992). Fifty‐two subjects completed the delayed memory tasks. The median delayed recall score was low, at 1 word (IQR=02), with 44% of subjects remembering 0 items. Delayed memory scores were not associated with age quartile. There was no association between any memory scores and gender or African American race.
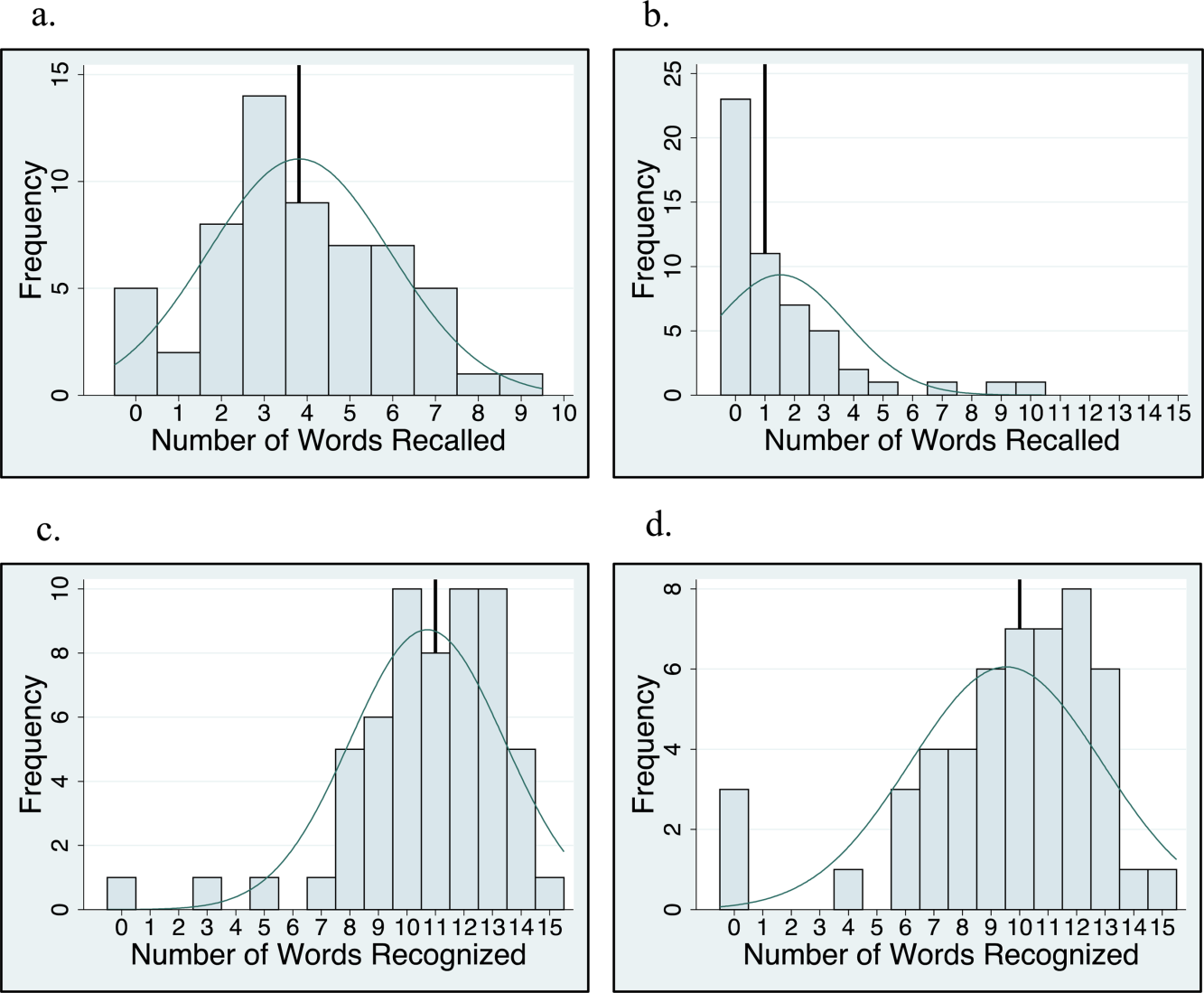
For 35 subjects in this study, we piloted the use of the medical vignette memory task. Two raters scored subject responses. Of the 525 total items, there was 98.1% agreement between the 2 raters, and only 7 out of 35 subjects' total scores differed between the 2 raters (see Supporting Information, Appendix 2B, in the online version of this article for detailed results). Median number of items remembered correctly was 4 out of 15 (IQR=26). Median number of incorrectly remembered items was 0.5 (IQR=01). Up to 57% (20 subjects) incorrectly remembered at least 1 item. The medical vignette memory score was significantly correlated with immediate recall score (r=0.49, P<0.01), but not immediate recognition score (r=0.24, P=0.16), delayed recall (r=0.13, P=0.47), or delayed recognition (r=0.01, P=0.96). There was a negative relationship between the number of items correctly recalled by a subject and the number of incorrectly recalled items on the medical vignette memory task that did not reach statistical significance (r=0.32, P=0.06).
There was no association between sleep duration, sleep efficiency, and KSQI with memory scores (immediate and delayed recall, immediate and delayed recognition, medical vignette task) (Table 2.) The relationship between objective sleep measures and immediate memory are plotted in Figure 3. Finally, there was no significant difference in sleep duration or efficiency between groups with high memory (immediate recall of >3 words) and low memory (immediate recall of 3 words).
Independent Variables | ||||
---|---|---|---|---|
Sleep Duration, h | Sleep Efficiency, % | Karolinska Sleep Quality Index | ||
Immediate recall (n=59) | Pearson's r | 0.044 | 0.2 | 0.18 |
coefficient | 0.042 | 0.025 | 0.27 | |
P value | 0.74 | 0.12 | 0.16 | |
Immediate recognition (n=59) | Pearson's r | 0.066 | 0.037 | 0.13 |
coefficient | 0.080 | 0.0058 | 0.25 | |
P value | 0.62 | 0.78 | 0.31 | |
Delayed recall (n=52) | Pearson's r | 0.028 | 0.0020 | 0.0081 |
coefficient | 0.027 | 0.00025 | 0.012 | |
P value | 0.85 | 0.99 | 0.96 | |
Delayed recognition (n=52) | Pearson's r | 0.21 | 0.12 | 0.15 |
coefficient | 0.31 | 0.024 | 0.35 | |
P value | 0.13 | 0.39 | 0.29 |
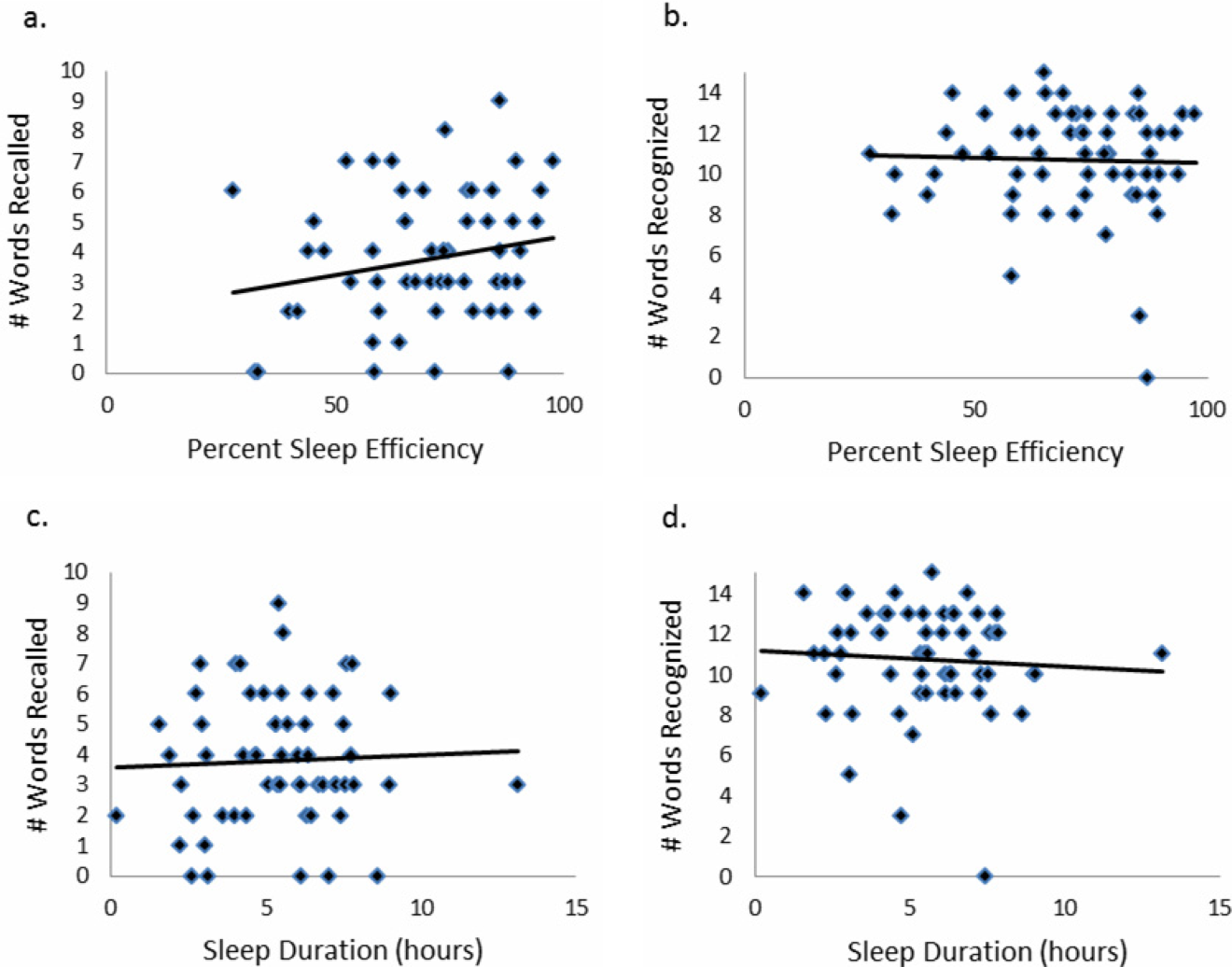
CONCLUSIONS/DISCUSSION
This study demonstrated that roughly half of hospitalized older adults without diagnosed memory or cognitive impairment had poor memory using an immediate word recall task. Although performance on an immediate word recall task may not be considered a good approximation for remembering discharge instructions, immediate recall did correlate with performance on a more complex medical vignette memory task. Though our subjects had low sleep efficiency and duration while in the hospital, memory performance was not significantly associated with inpatient sleep.
Perhaps the most concerning finding in this study was the substantial number of subjects who had poor memory. In addition to scoring approximately 1 SD lower than the community sample of healthy older adults tested in the sensitivity study of USC‐REMT,[14] our subjects also scored lower on immediate recall when compared to another hospitalized patient study.[4] In the study by Lindquist et al. that utilized a similar 15‐item word recall task in hospitalized patients, 29% of subjects were found to have poor memory (recall score of 3 words), compared to 49% in our study. In our 24‐hour delayed recall task we found that 44% of our patients could not recall a single word, with 65% remembering 1 word or fewer. In their study, Lindquist et al. similarly found that greater than 50% of subjects qualified as poor memory by recalling 1 or fewer words after merely an 8‐minute delay. Given these findings, hospitalization may not be the optimal teachable moment that it is often suggested to be. Use of transition coaches, memory aids like written instructions and reminders, and involvement of caregivers are likely critical to ensuring inpatients retain instructions and knowledge. More focus also needs to be given to older patients, who often have the worst memory. Technology tools, such as the Vocera Good To Go app, could allow medical professionals to make audio recordings of discharge instructions that patients may access at any time on a mobile device.
This study also has implications for how to measure memory in inpatients. For example, a vignette‐based memory test may be appropriate for assessing inpatient memory for discharge instructions. Our task was easy to administer and correlated with immediate recall scores. Furthermore, the story‐based task helps us to establish a sense of how much information from a paragraph is truly remembered. Our data show that only 4 items of 15 were remembered, and the majority of subjects actually misremembered 1 item. This latter measure sheds light on the rate of inaccuracy of patient recall. It is worth noting also that word recognition showed a ceiling effect in our sample, suggesting the task was too easy. In contrast, delayed recall was too difficult, as scores showed a floor effect, with over half of our sample unable to recall a single word after a 24‐hour delay.
This is the first study to assess the relationship between sleep loss and memory in hospitalized patients. We found that memory scores were not significantly associated with sleep duration, sleep efficiency, or with the self‐reported KSQI. Memory during hospitalization may be affected by factors other than sleep, like cognition, obscuring the relationship between sleep and memory. It is also possible that we were unable to see a significant association between sleep and memory because of universally low sleep duration and efficiency scores in the hospital.
Our study has several limitations. Most importantly, this study includes a small number of subjects who were hospitalized on a general medicine service at a single institution, limiting generalizability. Also importantly, our data capture only 1 night of sleep, and this may limit our study's ability to detect an association between hospital sleep and memory. More longitudinal data measuring sleep and memory across a longer period of time may reveal the distinct contribution of in‐hospital sleep. We also excluded patients with known cognitive impairment from enrollment, limiting our patient population to those with only high cognitive reserve. We hypothesize that patients with dementia experience both increased sleep disturbance and greater decline in memory during hospitalization. In addition, we are unable to test causal associations in this observational study. Furthermore, we applied a standardized memory test, the USC‐REMT, in a hospital setting, where noise and other disruptions at the time of test administration cannot be completely controlled. This makes it difficult to compare our results with those of community‐dwelling members taking the test under optimal conditions. Finally, because we created our own medical vignette task, future testing to validate this method against other memory testing is warranted.
In conclusion, our results show that memory in older hospitalized inpatients is often impaired, despite patients' appearing cognitively intact. These deficits in memory are revealed by a word recall task and also by a medical vignette task that more closely approximates memory for complex discharge instructions.
Disclosure
This work was funded by the National Institute on Aging Short‐Term Aging‐Related Research Program (5T35AG029795),the National Institute on Aging Career Development Award (K23AG033763), and the National Heart Lung and Blood Institute (R25 HL116372).
- Importance of in‐hospital initiation of evidence‐based medical therapies for heart failure: taking advantage of the teachable moment. Congest Heart Fail. 2005;11(3):153–154. .
- Smoking cessation in hospitalized patients: results of a randomized trial. Arch Intern Med. 1997;157(4):409–415. , , , , .
- Smoking cessation interventions for hospitalized smokers: a systematic review. Arch Intern Med. 2008;168(18):1950–1960. , , .
- Improvements in cognition following hospital discharge of community dwelling seniors. J Gen Intern Med. 2011;26(7):765–770. , , , , .
- Sleep and aging: 1. sleep disorders commonly found in older people. Can Med Assoc J. 2007;176(9):1299–1304. , , , .
- Noise and sleep among adult medical inpatients: far from a quiet night. Arch Intern Med. 2012;172(1):68–70. .
- Perceived control and sleep in hospitalized older adults: a sound hypothesis? J Hosp Med. 2013;8(4):184–190. , , , , , .
- A meta‐analysis of the impact of short‐term sleep deprivation on cognitive variables. Psychol Bull. 2010;136(3):375–389. , .
- Sleep deprivation: Impact on cognitive performance. Neuropsychiatr Dis Treat. 2007;3(5):553–567. , .
- Effects of physician experience on costs and outcomes on an academic general medicine service: results of a trial of hospitalists. Ann Intern Med. 2002;137(11):866–874. , , , et al.
- “Mini‐mental state”: a practical method for grading the cognitive state of patients for the clinician. J Psychiatr Res. 1975;12(3):189–198. , , .
- A short portable mental status questionnaire for the assessment of organic brain deficit in elderly patients. J Am Geriatr Soc. 1975;10:433–441. .
- Reliability and validity of the Short Portable Mental Status Questionnaire administered by telephone. J Geriatr Psychiatry Neurol. 1994;7(1):33–38. , , , .
- Aging, recall and recognition: a study on the sensitivity of the University of Southern California Repeatable Episodic Memory Test (USC‐REMT). J Clin Exp Neuropsychol. 2004;26(3):428–440. , , , .
- University of southern california repeatable episodic memory test. J Clin Exp Neuropsychol. 1995;17(6):926–936. , , , , .
- Development of alternate paragraphs for the logical memory subtest of the Wechsler Memory Scale‐Revised. Clin Neuropsychol. 1997;11(4):370–374. , , .
- A Compendium of Neuropsychological Tests: Administration, Norms, and Commentary. 3rd ed. New York, NY: Oxford University Press; 2009. , , .
- Review of physical activity measurement using accelerometers in older adults: considerations for research design and conduct. Prev Med. 2009;48(2):108–114. .
- The actigraph data analysis software: I. A novel approach to scoring and interpreting sleep‐wake activity. Percept Mot Skills. 1997;85(1):207–216. , , , , , .
- Evaluation of immobility time for sleep latency in actigraphy. Sleep Med. 2009;10(6):621–625. , , , et al.
- The subjective meaning of sleep quality: a comparison of individuals with and without insomnia. Sleep. 2008;31(3):383–393. , , , , .
- Objective components of individual differences in subjective sleep quality. J Sleep Res. 1997;6(4):217–220. , .
- Research electronic data capture (REDCap)—a metadata‐driven methodology and workflow process for providing translational research informatics support. J Biomed Inform. 2009;42(2):377–381. , , , , , .
- A Wilcoxon‐type test for trend. Stat Med. 1985;4(1):87–90. .
- Derivation of research diagnostic criteria for insomnia: report of an American Academy of Sleep Medicine Work Group. Sleep. 2004;27(8):1567–1596. , , , et al.
- Quantitative criteria for insomnia. Behav Res Ther. 2003;41(4):427–445. , , , , .
Hospitalization is often utilized as a teachable moment, as patients are provided with education about treatment and disease management, particularly at discharge.[1, 2, 3] However, memory impairment among hospitalized patients may undermine the utility of the teachable moment. In one study of community‐dwelling seniors admitted to the hospital, one‐third had previously unrecognized poor memory at discharge.[4]
Sleep loss may be an underappreciated contributor to short‐term memory deficits in inpatients, particularly in seniors, who have baseline higher rates of sleep disruptions and sleep disorders.[5] Patients often receive 2 hours less sleep than at home and experience poor quality sleep due to disruptions.[6, 7] Robust studies of healthy subjects in laboratory settings demonstrate that sleep loss leads to decreased attention and worse recall, and that more sleep is associated with better memory performance.[8, 9]
Very few studies have examined memory in hospitalized patients. Although word‐list tasks are often used to assess memory because they are quick and easy to administer, these tasks may not accurately reflect memory for a set of instructions provided at patient discharge. Finally, no studies have examined the association between inpatient sleep loss and memory. Thus, our primary aim in this study was to examine memory performance in older, hospitalized patients using a word listbased memory task and a more complex medical vignette task. Our second aim was to investigate the relationship between in‐hospital sleep and memory.
METHODS
Study Design
We conducted a prospective cohort study with subjects enrolled in an ongoing sleep study at the University of Chicago Medical Center.[10] Eligible subjects were on the general medicine or hematology/oncology service, at least 50 years old, community dwelling, ambulatory, and without detectable cognitive impairment on the Mini Mental State Exam[11] or Short Portable Mental Status Questionnaire.[12, 13] Patients were excluded if they had a documented sleep disorder (ie, obstructive sleep apnea), were transferred from an intensive care unit or were in droplet or airborne isolation, had a bedrest order, or had already spent over 72 hours in the hospital prior to enrollment. These criteria were used to select a population appropriate for wristwatch actigraphy and with low likelihood of baseline memory impairment. The University of Chicago Institutional Review Board approved this study, and participants provided written consent.
Data Collection
Memory Testing
Memory was evaluated using the University of Southern California Repeatable Episodic Memory Test (USC‐REMT), a validated verbal memory test in which subjects listen to a list of 15 words and then complete free‐recall and recognition of the list.[14, 15] Free‐recall tests subjects' ability to procure information without cues. In contrast, recognition requires subjects to pick out the words they just heard from distractors, an easier task. The USC‐REMT contains multiple functionally equivalent different word lists, and may be administered more than once to the same subject without learning effects.[15] Immediate and delayed memory were tested by asking the subject to complete the tasks immediately after listening to the word list and 24‐hours after listening to the list, respectively.
Immediate Recall and Recognition
Recall and recognition following a night of sleep in the hospital was the primary outcome for this study. After 1 night of actigraphy recorded sleep, subjects listened as a 15‐item word list (word list A) was read aloud. For the free‐recall task, subjects were asked to repeat back all the words they could remember immediately after hearing the list. For the recognition task, subjects were read a new list of 15 words, including a mix of words from the previous list and new distractor words. They answered yes if they thought the word had previously been read to them and no if they thought the word was new.
Delayed Recall and Delayed Recognition
At the conclusion of study enrollment on day 1 prior to the night of actigraphy, subjects were shown a laminated paper with a printed word list (word list B) from the USC‐REMT. They were given 2 minutes to study the sheet and were informed they would be asked to remember the words the following day. One day later, after the night of actigraphy recorded sleep, subjects completed the free recall and yes/no recognition task based on what they remembered from word list B. This established delayed recall and recognition scores.
Medical Vignette
Because it is unclear how word recall and recognition tasks approximate remembering discharge instructions, we developed a 5‐sentence vignette about an outpatient medical encounter, based on the logical memory component of the Wechsler Memory Scale IV, a commonly used, validated test of memory assessment.[16, 17] After the USC‐REMT was administered following a night of sleep in the hospital, patients listened to a story and were immediately asked to repeat back in free form as much information as possible from the story. Responses were recorded by trained research assistants. The story is comprised of short sentences with simple ideas and vocabulary (see Supporting Information, Appendix 1, in the online version of this article).
Sleep: Wrist Actigraphy and Karolinska Sleep Log
Patient sleep was measured by actigraphy following the protocol described previously by our group.[7] Patients wore a wrist actigraphy monitor (Actiwatch 2; Philips Respironics, Inc., Murrysville, PA) to collect data on sleep duration and quality. The monitor detects wrist movement by measuring acceleration.[18] Actigraphy has been validated against polysomnography, demonstrating a correlation in sleep duration of 0.82 in insomniacs and 0.97 in healthy subjects.[19] Sleep duration and sleep efficiency overnight were calculated from the actigraphy data using Actiware 5 software.[20] Sleep duration was defined by the software based on low levels of recorded movement. Sleep efficiency was calculated as the percentage of time asleep out of the subjects' self‐reported time in bed, which was obtained using the Karolinska Sleep Log.[21]
The Karolinska Sleep Log questionnaire also asks patients to rate their sleep quality, restlessness during sleep, ease of falling asleep and the ability to sleep through the night on a 5‐point scale. The Karolinska Sleep Quality Index (KSQI) is calculated by averaging the latter 4 items.[22] A score of 3 or less classifies the subject in an insomniac range.[7, 21]
Demographic Information
Demographic information, including age, race, and gender were obtained by chart audit.
Data Analysis
Data were entered into REDCap, a secure online tool for managing survey data.[23]
Memory Scoring
For immediate and delayed recall scores, subjects received 1 point for every word they remembered correctly, with a maximum score of 15 words. We defined poor memory on the immediate recall test as a score of 3 or lower, based on a score utilized by Lindquist et al.[4] in a similar task. This score was less than half of the mean score of 6.63 obtained by Parker et al. for a sample of healthy 60 to 79 year olds in a sensitivity study of the USC‐REMT.[14] For immediate and delayed recognition, subjects received 1 point for correctly identifying whether a word had been on the word list they heard or whether it was a distractor, with a maximum score of 15.
A key was created to standardize scoring of the medical vignette by assigning 1 point to specific correctly remembered items from the story (see Supporting Information, Appendix 2A, in the online version of this article). These points were added to obtain a total score for correctly remembered vignette items. It was also noted when a vignette item was remembered incorrectly, for example, when the patient remembered left foot instead of right foot. Each incorrectly remembered item received 1 point, and these were summed to create the total score for incorrectly remembered vignette items (see Supporting Information, Appendix 2A, in the online version of this article for the scoring guide). Forgotten items were assigned 0 points. Two independent raters scored each subject's responses, and their scores were averaged for each item. Inter‐rater reliability was calculated as percentage of agreement across responses.
Statistical Analysis
Descriptive statistics were performed on the memory task data. Tests for skew and curtosis were performed for recall and recognition task data. The mean and standard deviation (SD) were calculated for normally distributed data, and the median and interquartile range (IQR) were obtained for data that showed significant skew. Mean and SD were also calculated for sleep duration and sleep efficiency measured by actigraphy.
Two‐tailed t tests were used to examine the association between memory and gender and African American race. Cuzick's nonparametric test of trend was used to test the association between age quartile and recall and recognition scores.[24] Mean and standard deviation for the correct total score and incorrect total score for the medical vignette were calculated. Pearson's correlation coefficient was used to examine the association between USC‐REMT memory measures and medical vignette score.
Pearson's correlation coefficient was calculated to test the associations between sleep duration and memory scores (immediate and delayed recall, immediate and delayed recognition, medical vignette task). This test was repeated to examine the relationship between sleep efficiency and the above memory scores. Linear regression models were used to characterize the relationship between inpatient sleep duration and efficiency and memory task performance. Two‐tailed t tests were used to compare sleep metrics (duration and efficiency) between high‐ and low‐memory groups, with low memory defined as immediate recall of 3 words.
All statistical tests were conducted using Stata 12.0 software (StataCorp, College Station, TX). Statistical significance was defined as P<0.05.
RESULTS
From April 11, 2013 to May 3, 2014, 322 patients were eligible for our study. Of these, 99 patients were enrolled in the study. We were able to collect sleep actigraphy data and immediate memory scores from 59 on day 2 of the study (Figure 1).
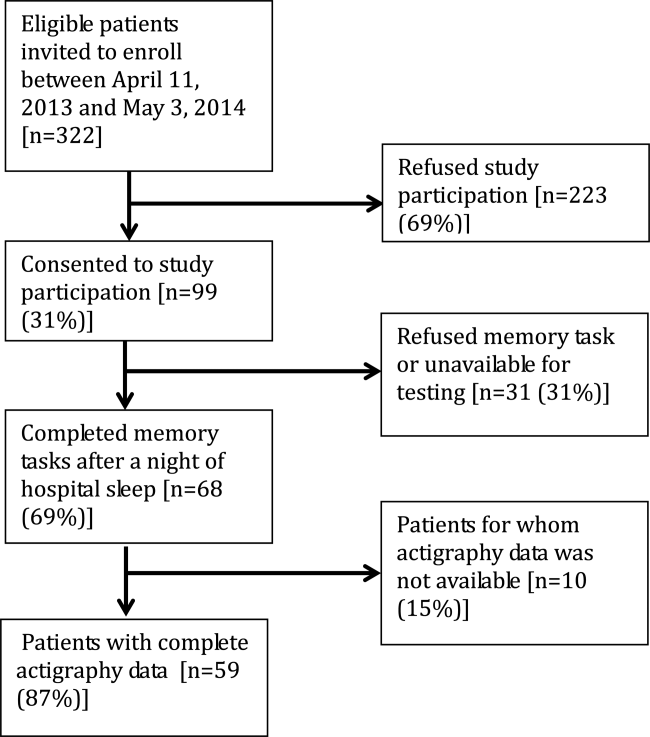
The study population had a mean age of 61.6 years (SD=9.3 years). Demographic information is presented in Table 1. Average nightly sleep in the hospital was 5.44 hours (326.4 minutes, SD=134.5 minutes), whereas mean sleep efficiency was 70.9 (SD=17.1), which is below the normal threshold of 85%.[25, 26] Forty‐four percent had a KSQI score of 3, representing in‐hospital sleep quality in the insomniac range.
Value | |
---|---|
| |
Patient characteristics | |
Age, y, mean (SD) | 61.6 (9.3) |
Female, n (%) | 36 (61.0%) |
BMI, n (%) | |
Underweight (<18.5) | 3 (5.1%) |
Normal weight (18.524.9) | 16 (27.1%) |
Overweight (25.029.9) | 14 (23.7%) |
Obese (30.0) | 26 (44.1%) |
African American, n (%) | 43 (72.9%) |
Non‐Hispanic, n (%) | 57 (96.6%) |
Education, n (%) | |
Did not finish high school | 13 (23.2%) |
High school graduate | 13 (23.2%) |
Some college or junior college | 16 (28.6%) |
College graduate or postgraduate degree | 13 (23.2%) |
Discharge diagnosis (ICD‐9‐CM classification), n (%) | |
Circulatory system disease | 5 (8.5%) |
Digestive system disease | 9 (15.3%) |
Genitourinary system disease | 4 (6.8%) |
Musculoskeletal system disease | 3 (5.1%) |
Respiratory system disease | 5 (8.5%) |
Sensory organ disease | 1 (1.7%) |
Skin and subcutaneous tissue disease | 3 (5.1%) |
Endocrine, nutritional, and metabolic disease | 7 (11.9%) |
Infection and parasitic disease | 6 (10.2%) |
Injury and poisoning | 4 (6.8%) |
Mental disorders | 2 (3.4%) |
Neoplasm | 5 (8.5%) |
Symptoms, signs, and ill‐defined conditions | 5 (8.5%) |
Comorbidities by self‐report, n=57, n (%) | |
Cancer | 6 (10.5%) |
Depression | 15 (26.3%) |
Diabetes | 15 (26.3%) |
Heart trouble | 16 (28.1%) |
HIV/AIDS | 2 (3.5%) |
Kidney disease | 10 (17.5%) |
Liver disease | 9 (15.8%) |
Stroke | 4 (7.0%) |
Subject on the hematology and oncology service, n (%) | 6 (10.2%) |
Sleep characteristics | |
Nights in hospital prior to enrollment, n (%) | |
0 nights | 12 (20.3%) |
1 night | 24 (40.7%) |
2 nights | 17 (28.8%) |
3 nights | 6 (10.1%) |
Received pharmacologic sleep aids, n (%) | 10 (17.0%) |
Karolinska Sleep Quality Index scores, score 3, n (%) | 26 (44.1%) |
Sleep duration, min, mean (SD) | 326.4 (134.5) |
Sleep efficiency, %, mean (SD) | 70.9 (17.1) |
Memory test scores are presented in Figure 2. Nearly half (49%) of patients had poor memory, defined by a score of 3 words (Figure 2). Immediate recall scores varied significantly with age quartile, with older subjects recalling fewer words (Q1 [age 50.453.6 years] mean=4.9 words; Q2 [age 54.059.2 years] mean=4.1 words; Q3 [age 59.466.9 years] mean=3.7 words; Q4 [age 68.285.0 years] mean=2.5 words; P=0.001). Immediate recognition scores did not vary significantly by age quartile (Q1 [age 50.453.6 years] mean=10.3 words; Q2 [age 54.059.2 years] mean =10.3 words; Q3 [age 59.466.9 years)] mean=11.8 words; Q4 [age 68.285.0 years] mean=10.4 words; P=0.992). Fifty‐two subjects completed the delayed memory tasks. The median delayed recall score was low, at 1 word (IQR=02), with 44% of subjects remembering 0 items. Delayed memory scores were not associated with age quartile. There was no association between any memory scores and gender or African American race.
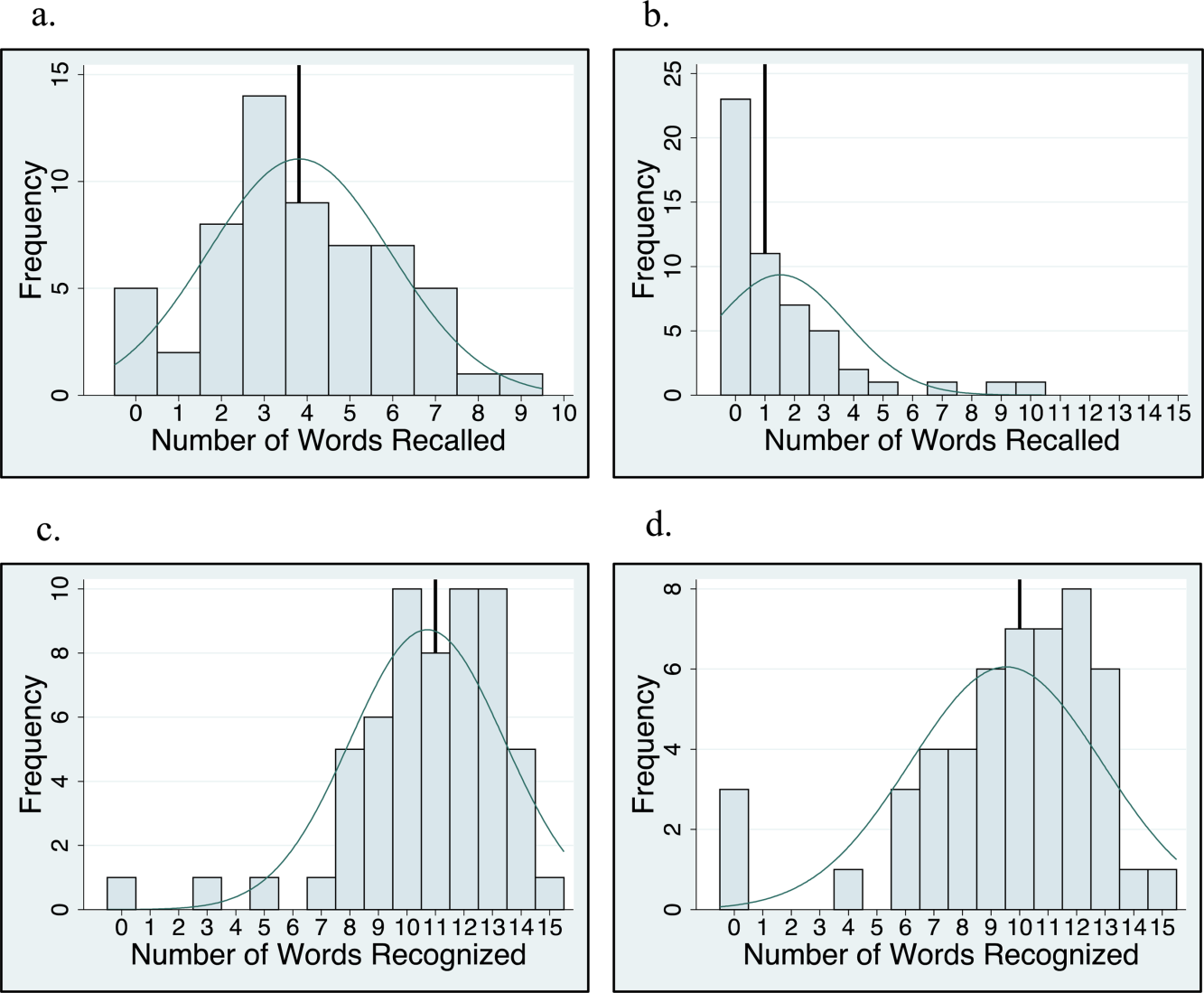
For 35 subjects in this study, we piloted the use of the medical vignette memory task. Two raters scored subject responses. Of the 525 total items, there was 98.1% agreement between the 2 raters, and only 7 out of 35 subjects' total scores differed between the 2 raters (see Supporting Information, Appendix 2B, in the online version of this article for detailed results). Median number of items remembered correctly was 4 out of 15 (IQR=26). Median number of incorrectly remembered items was 0.5 (IQR=01). Up to 57% (20 subjects) incorrectly remembered at least 1 item. The medical vignette memory score was significantly correlated with immediate recall score (r=0.49, P<0.01), but not immediate recognition score (r=0.24, P=0.16), delayed recall (r=0.13, P=0.47), or delayed recognition (r=0.01, P=0.96). There was a negative relationship between the number of items correctly recalled by a subject and the number of incorrectly recalled items on the medical vignette memory task that did not reach statistical significance (r=0.32, P=0.06).
There was no association between sleep duration, sleep efficiency, and KSQI with memory scores (immediate and delayed recall, immediate and delayed recognition, medical vignette task) (Table 2.) The relationship between objective sleep measures and immediate memory are plotted in Figure 3. Finally, there was no significant difference in sleep duration or efficiency between groups with high memory (immediate recall of >3 words) and low memory (immediate recall of 3 words).
Independent Variables | ||||
---|---|---|---|---|
Sleep Duration, h | Sleep Efficiency, % | Karolinska Sleep Quality Index | ||
Immediate recall (n=59) | Pearson's r | 0.044 | 0.2 | 0.18 |
coefficient | 0.042 | 0.025 | 0.27 | |
P value | 0.74 | 0.12 | 0.16 | |
Immediate recognition (n=59) | Pearson's r | 0.066 | 0.037 | 0.13 |
coefficient | 0.080 | 0.0058 | 0.25 | |
P value | 0.62 | 0.78 | 0.31 | |
Delayed recall (n=52) | Pearson's r | 0.028 | 0.0020 | 0.0081 |
coefficient | 0.027 | 0.00025 | 0.012 | |
P value | 0.85 | 0.99 | 0.96 | |
Delayed recognition (n=52) | Pearson's r | 0.21 | 0.12 | 0.15 |
coefficient | 0.31 | 0.024 | 0.35 | |
P value | 0.13 | 0.39 | 0.29 |
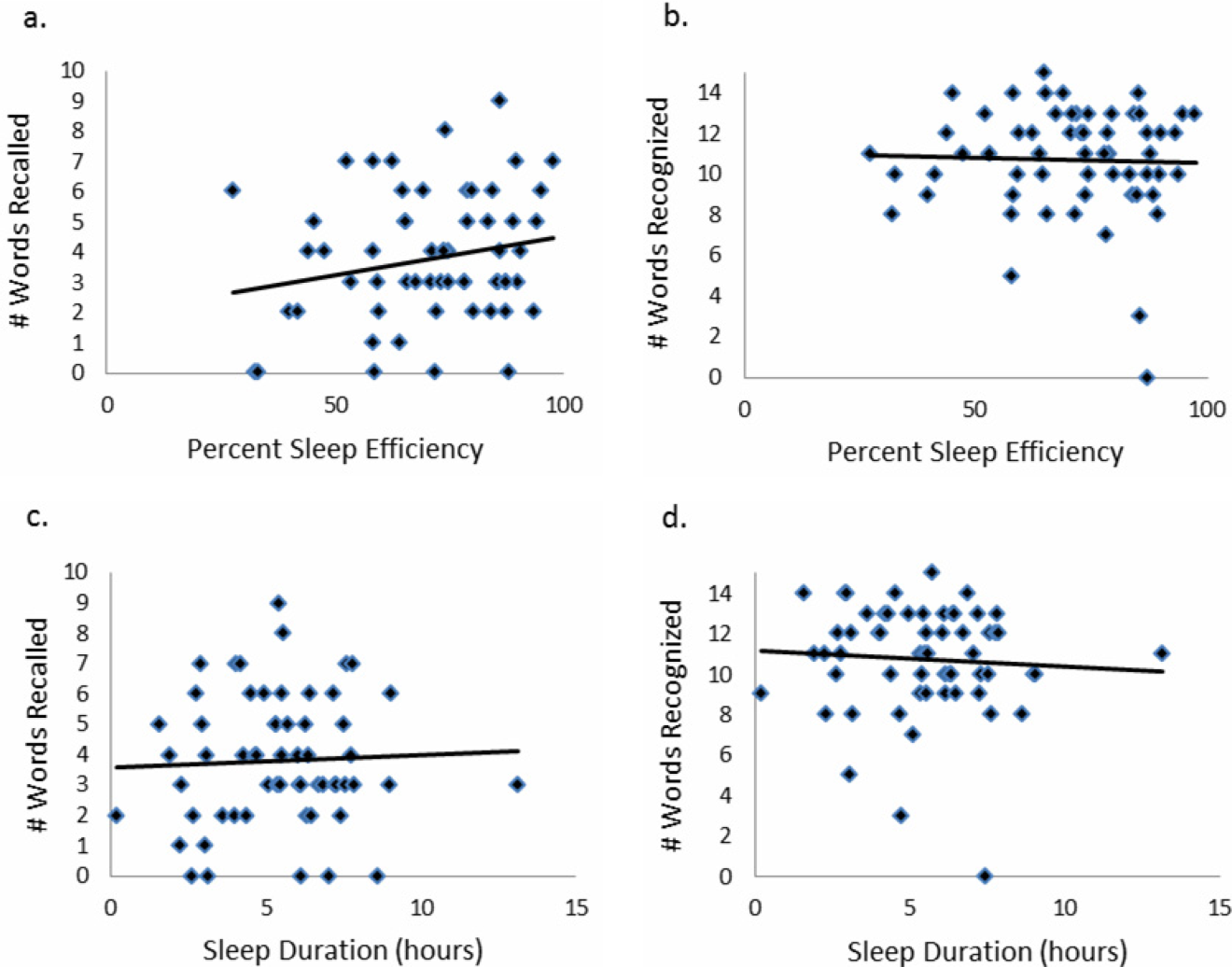
CONCLUSIONS/DISCUSSION
This study demonstrated that roughly half of hospitalized older adults without diagnosed memory or cognitive impairment had poor memory using an immediate word recall task. Although performance on an immediate word recall task may not be considered a good approximation for remembering discharge instructions, immediate recall did correlate with performance on a more complex medical vignette memory task. Though our subjects had low sleep efficiency and duration while in the hospital, memory performance was not significantly associated with inpatient sleep.
Perhaps the most concerning finding in this study was the substantial number of subjects who had poor memory. In addition to scoring approximately 1 SD lower than the community sample of healthy older adults tested in the sensitivity study of USC‐REMT,[14] our subjects also scored lower on immediate recall when compared to another hospitalized patient study.[4] In the study by Lindquist et al. that utilized a similar 15‐item word recall task in hospitalized patients, 29% of subjects were found to have poor memory (recall score of 3 words), compared to 49% in our study. In our 24‐hour delayed recall task we found that 44% of our patients could not recall a single word, with 65% remembering 1 word or fewer. In their study, Lindquist et al. similarly found that greater than 50% of subjects qualified as poor memory by recalling 1 or fewer words after merely an 8‐minute delay. Given these findings, hospitalization may not be the optimal teachable moment that it is often suggested to be. Use of transition coaches, memory aids like written instructions and reminders, and involvement of caregivers are likely critical to ensuring inpatients retain instructions and knowledge. More focus also needs to be given to older patients, who often have the worst memory. Technology tools, such as the Vocera Good To Go app, could allow medical professionals to make audio recordings of discharge instructions that patients may access at any time on a mobile device.
This study also has implications for how to measure memory in inpatients. For example, a vignette‐based memory test may be appropriate for assessing inpatient memory for discharge instructions. Our task was easy to administer and correlated with immediate recall scores. Furthermore, the story‐based task helps us to establish a sense of how much information from a paragraph is truly remembered. Our data show that only 4 items of 15 were remembered, and the majority of subjects actually misremembered 1 item. This latter measure sheds light on the rate of inaccuracy of patient recall. It is worth noting also that word recognition showed a ceiling effect in our sample, suggesting the task was too easy. In contrast, delayed recall was too difficult, as scores showed a floor effect, with over half of our sample unable to recall a single word after a 24‐hour delay.
This is the first study to assess the relationship between sleep loss and memory in hospitalized patients. We found that memory scores were not significantly associated with sleep duration, sleep efficiency, or with the self‐reported KSQI. Memory during hospitalization may be affected by factors other than sleep, like cognition, obscuring the relationship between sleep and memory. It is also possible that we were unable to see a significant association between sleep and memory because of universally low sleep duration and efficiency scores in the hospital.
Our study has several limitations. Most importantly, this study includes a small number of subjects who were hospitalized on a general medicine service at a single institution, limiting generalizability. Also importantly, our data capture only 1 night of sleep, and this may limit our study's ability to detect an association between hospital sleep and memory. More longitudinal data measuring sleep and memory across a longer period of time may reveal the distinct contribution of in‐hospital sleep. We also excluded patients with known cognitive impairment from enrollment, limiting our patient population to those with only high cognitive reserve. We hypothesize that patients with dementia experience both increased sleep disturbance and greater decline in memory during hospitalization. In addition, we are unable to test causal associations in this observational study. Furthermore, we applied a standardized memory test, the USC‐REMT, in a hospital setting, where noise and other disruptions at the time of test administration cannot be completely controlled. This makes it difficult to compare our results with those of community‐dwelling members taking the test under optimal conditions. Finally, because we created our own medical vignette task, future testing to validate this method against other memory testing is warranted.
In conclusion, our results show that memory in older hospitalized inpatients is often impaired, despite patients' appearing cognitively intact. These deficits in memory are revealed by a word recall task and also by a medical vignette task that more closely approximates memory for complex discharge instructions.
Disclosure
This work was funded by the National Institute on Aging Short‐Term Aging‐Related Research Program (5T35AG029795),the National Institute on Aging Career Development Award (K23AG033763), and the National Heart Lung and Blood Institute (R25 HL116372).
Hospitalization is often utilized as a teachable moment, as patients are provided with education about treatment and disease management, particularly at discharge.[1, 2, 3] However, memory impairment among hospitalized patients may undermine the utility of the teachable moment. In one study of community‐dwelling seniors admitted to the hospital, one‐third had previously unrecognized poor memory at discharge.[4]
Sleep loss may be an underappreciated contributor to short‐term memory deficits in inpatients, particularly in seniors, who have baseline higher rates of sleep disruptions and sleep disorders.[5] Patients often receive 2 hours less sleep than at home and experience poor quality sleep due to disruptions.[6, 7] Robust studies of healthy subjects in laboratory settings demonstrate that sleep loss leads to decreased attention and worse recall, and that more sleep is associated with better memory performance.[8, 9]
Very few studies have examined memory in hospitalized patients. Although word‐list tasks are often used to assess memory because they are quick and easy to administer, these tasks may not accurately reflect memory for a set of instructions provided at patient discharge. Finally, no studies have examined the association between inpatient sleep loss and memory. Thus, our primary aim in this study was to examine memory performance in older, hospitalized patients using a word listbased memory task and a more complex medical vignette task. Our second aim was to investigate the relationship between in‐hospital sleep and memory.
METHODS
Study Design
We conducted a prospective cohort study with subjects enrolled in an ongoing sleep study at the University of Chicago Medical Center.[10] Eligible subjects were on the general medicine or hematology/oncology service, at least 50 years old, community dwelling, ambulatory, and without detectable cognitive impairment on the Mini Mental State Exam[11] or Short Portable Mental Status Questionnaire.[12, 13] Patients were excluded if they had a documented sleep disorder (ie, obstructive sleep apnea), were transferred from an intensive care unit or were in droplet or airborne isolation, had a bedrest order, or had already spent over 72 hours in the hospital prior to enrollment. These criteria were used to select a population appropriate for wristwatch actigraphy and with low likelihood of baseline memory impairment. The University of Chicago Institutional Review Board approved this study, and participants provided written consent.
Data Collection
Memory Testing
Memory was evaluated using the University of Southern California Repeatable Episodic Memory Test (USC‐REMT), a validated verbal memory test in which subjects listen to a list of 15 words and then complete free‐recall and recognition of the list.[14, 15] Free‐recall tests subjects' ability to procure information without cues. In contrast, recognition requires subjects to pick out the words they just heard from distractors, an easier task. The USC‐REMT contains multiple functionally equivalent different word lists, and may be administered more than once to the same subject without learning effects.[15] Immediate and delayed memory were tested by asking the subject to complete the tasks immediately after listening to the word list and 24‐hours after listening to the list, respectively.
Immediate Recall and Recognition
Recall and recognition following a night of sleep in the hospital was the primary outcome for this study. After 1 night of actigraphy recorded sleep, subjects listened as a 15‐item word list (word list A) was read aloud. For the free‐recall task, subjects were asked to repeat back all the words they could remember immediately after hearing the list. For the recognition task, subjects were read a new list of 15 words, including a mix of words from the previous list and new distractor words. They answered yes if they thought the word had previously been read to them and no if they thought the word was new.
Delayed Recall and Delayed Recognition
At the conclusion of study enrollment on day 1 prior to the night of actigraphy, subjects were shown a laminated paper with a printed word list (word list B) from the USC‐REMT. They were given 2 minutes to study the sheet and were informed they would be asked to remember the words the following day. One day later, after the night of actigraphy recorded sleep, subjects completed the free recall and yes/no recognition task based on what they remembered from word list B. This established delayed recall and recognition scores.
Medical Vignette
Because it is unclear how word recall and recognition tasks approximate remembering discharge instructions, we developed a 5‐sentence vignette about an outpatient medical encounter, based on the logical memory component of the Wechsler Memory Scale IV, a commonly used, validated test of memory assessment.[16, 17] After the USC‐REMT was administered following a night of sleep in the hospital, patients listened to a story and were immediately asked to repeat back in free form as much information as possible from the story. Responses were recorded by trained research assistants. The story is comprised of short sentences with simple ideas and vocabulary (see Supporting Information, Appendix 1, in the online version of this article).
Sleep: Wrist Actigraphy and Karolinska Sleep Log
Patient sleep was measured by actigraphy following the protocol described previously by our group.[7] Patients wore a wrist actigraphy monitor (Actiwatch 2; Philips Respironics, Inc., Murrysville, PA) to collect data on sleep duration and quality. The monitor detects wrist movement by measuring acceleration.[18] Actigraphy has been validated against polysomnography, demonstrating a correlation in sleep duration of 0.82 in insomniacs and 0.97 in healthy subjects.[19] Sleep duration and sleep efficiency overnight were calculated from the actigraphy data using Actiware 5 software.[20] Sleep duration was defined by the software based on low levels of recorded movement. Sleep efficiency was calculated as the percentage of time asleep out of the subjects' self‐reported time in bed, which was obtained using the Karolinska Sleep Log.[21]
The Karolinska Sleep Log questionnaire also asks patients to rate their sleep quality, restlessness during sleep, ease of falling asleep and the ability to sleep through the night on a 5‐point scale. The Karolinska Sleep Quality Index (KSQI) is calculated by averaging the latter 4 items.[22] A score of 3 or less classifies the subject in an insomniac range.[7, 21]
Demographic Information
Demographic information, including age, race, and gender were obtained by chart audit.
Data Analysis
Data were entered into REDCap, a secure online tool for managing survey data.[23]
Memory Scoring
For immediate and delayed recall scores, subjects received 1 point for every word they remembered correctly, with a maximum score of 15 words. We defined poor memory on the immediate recall test as a score of 3 or lower, based on a score utilized by Lindquist et al.[4] in a similar task. This score was less than half of the mean score of 6.63 obtained by Parker et al. for a sample of healthy 60 to 79 year olds in a sensitivity study of the USC‐REMT.[14] For immediate and delayed recognition, subjects received 1 point for correctly identifying whether a word had been on the word list they heard or whether it was a distractor, with a maximum score of 15.
A key was created to standardize scoring of the medical vignette by assigning 1 point to specific correctly remembered items from the story (see Supporting Information, Appendix 2A, in the online version of this article). These points were added to obtain a total score for correctly remembered vignette items. It was also noted when a vignette item was remembered incorrectly, for example, when the patient remembered left foot instead of right foot. Each incorrectly remembered item received 1 point, and these were summed to create the total score for incorrectly remembered vignette items (see Supporting Information, Appendix 2A, in the online version of this article for the scoring guide). Forgotten items were assigned 0 points. Two independent raters scored each subject's responses, and their scores were averaged for each item. Inter‐rater reliability was calculated as percentage of agreement across responses.
Statistical Analysis
Descriptive statistics were performed on the memory task data. Tests for skew and curtosis were performed for recall and recognition task data. The mean and standard deviation (SD) were calculated for normally distributed data, and the median and interquartile range (IQR) were obtained for data that showed significant skew. Mean and SD were also calculated for sleep duration and sleep efficiency measured by actigraphy.
Two‐tailed t tests were used to examine the association between memory and gender and African American race. Cuzick's nonparametric test of trend was used to test the association between age quartile and recall and recognition scores.[24] Mean and standard deviation for the correct total score and incorrect total score for the medical vignette were calculated. Pearson's correlation coefficient was used to examine the association between USC‐REMT memory measures and medical vignette score.
Pearson's correlation coefficient was calculated to test the associations between sleep duration and memory scores (immediate and delayed recall, immediate and delayed recognition, medical vignette task). This test was repeated to examine the relationship between sleep efficiency and the above memory scores. Linear regression models were used to characterize the relationship between inpatient sleep duration and efficiency and memory task performance. Two‐tailed t tests were used to compare sleep metrics (duration and efficiency) between high‐ and low‐memory groups, with low memory defined as immediate recall of 3 words.
All statistical tests were conducted using Stata 12.0 software (StataCorp, College Station, TX). Statistical significance was defined as P<0.05.
RESULTS
From April 11, 2013 to May 3, 2014, 322 patients were eligible for our study. Of these, 99 patients were enrolled in the study. We were able to collect sleep actigraphy data and immediate memory scores from 59 on day 2 of the study (Figure 1).
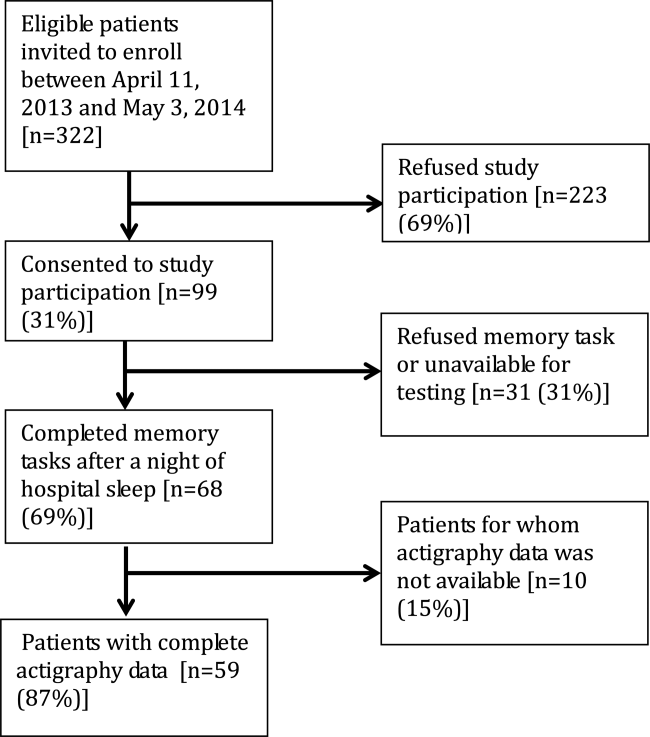
The study population had a mean age of 61.6 years (SD=9.3 years). Demographic information is presented in Table 1. Average nightly sleep in the hospital was 5.44 hours (326.4 minutes, SD=134.5 minutes), whereas mean sleep efficiency was 70.9 (SD=17.1), which is below the normal threshold of 85%.[25, 26] Forty‐four percent had a KSQI score of 3, representing in‐hospital sleep quality in the insomniac range.
Value | |
---|---|
| |
Patient characteristics | |
Age, y, mean (SD) | 61.6 (9.3) |
Female, n (%) | 36 (61.0%) |
BMI, n (%) | |
Underweight (<18.5) | 3 (5.1%) |
Normal weight (18.524.9) | 16 (27.1%) |
Overweight (25.029.9) | 14 (23.7%) |
Obese (30.0) | 26 (44.1%) |
African American, n (%) | 43 (72.9%) |
Non‐Hispanic, n (%) | 57 (96.6%) |
Education, n (%) | |
Did not finish high school | 13 (23.2%) |
High school graduate | 13 (23.2%) |
Some college or junior college | 16 (28.6%) |
College graduate or postgraduate degree | 13 (23.2%) |
Discharge diagnosis (ICD‐9‐CM classification), n (%) | |
Circulatory system disease | 5 (8.5%) |
Digestive system disease | 9 (15.3%) |
Genitourinary system disease | 4 (6.8%) |
Musculoskeletal system disease | 3 (5.1%) |
Respiratory system disease | 5 (8.5%) |
Sensory organ disease | 1 (1.7%) |
Skin and subcutaneous tissue disease | 3 (5.1%) |
Endocrine, nutritional, and metabolic disease | 7 (11.9%) |
Infection and parasitic disease | 6 (10.2%) |
Injury and poisoning | 4 (6.8%) |
Mental disorders | 2 (3.4%) |
Neoplasm | 5 (8.5%) |
Symptoms, signs, and ill‐defined conditions | 5 (8.5%) |
Comorbidities by self‐report, n=57, n (%) | |
Cancer | 6 (10.5%) |
Depression | 15 (26.3%) |
Diabetes | 15 (26.3%) |
Heart trouble | 16 (28.1%) |
HIV/AIDS | 2 (3.5%) |
Kidney disease | 10 (17.5%) |
Liver disease | 9 (15.8%) |
Stroke | 4 (7.0%) |
Subject on the hematology and oncology service, n (%) | 6 (10.2%) |
Sleep characteristics | |
Nights in hospital prior to enrollment, n (%) | |
0 nights | 12 (20.3%) |
1 night | 24 (40.7%) |
2 nights | 17 (28.8%) |
3 nights | 6 (10.1%) |
Received pharmacologic sleep aids, n (%) | 10 (17.0%) |
Karolinska Sleep Quality Index scores, score 3, n (%) | 26 (44.1%) |
Sleep duration, min, mean (SD) | 326.4 (134.5) |
Sleep efficiency, %, mean (SD) | 70.9 (17.1) |
Memory test scores are presented in Figure 2. Nearly half (49%) of patients had poor memory, defined by a score of 3 words (Figure 2). Immediate recall scores varied significantly with age quartile, with older subjects recalling fewer words (Q1 [age 50.453.6 years] mean=4.9 words; Q2 [age 54.059.2 years] mean=4.1 words; Q3 [age 59.466.9 years] mean=3.7 words; Q4 [age 68.285.0 years] mean=2.5 words; P=0.001). Immediate recognition scores did not vary significantly by age quartile (Q1 [age 50.453.6 years] mean=10.3 words; Q2 [age 54.059.2 years] mean =10.3 words; Q3 [age 59.466.9 years)] mean=11.8 words; Q4 [age 68.285.0 years] mean=10.4 words; P=0.992). Fifty‐two subjects completed the delayed memory tasks. The median delayed recall score was low, at 1 word (IQR=02), with 44% of subjects remembering 0 items. Delayed memory scores were not associated with age quartile. There was no association between any memory scores and gender or African American race.
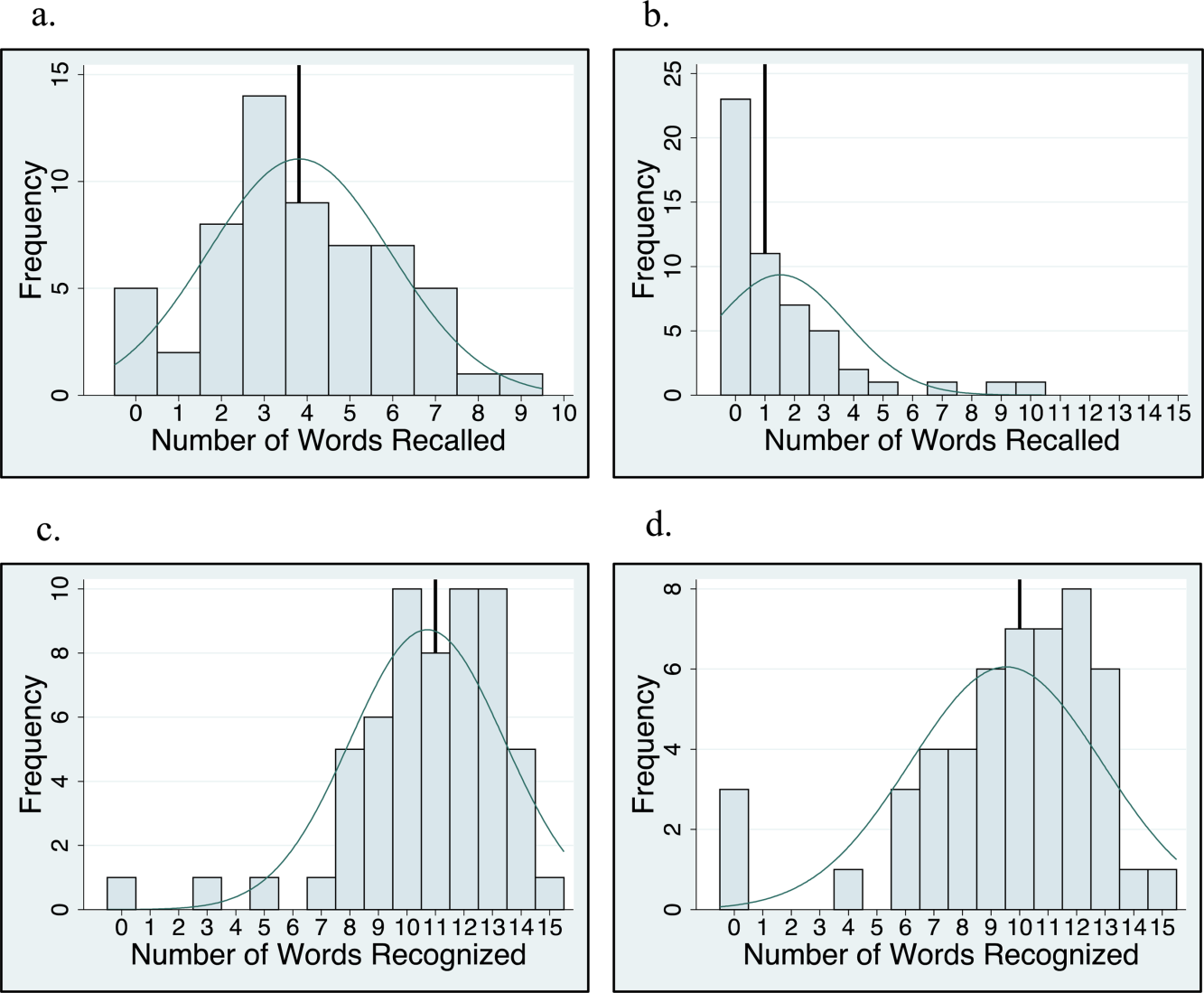
For 35 subjects in this study, we piloted the use of the medical vignette memory task. Two raters scored subject responses. Of the 525 total items, there was 98.1% agreement between the 2 raters, and only 7 out of 35 subjects' total scores differed between the 2 raters (see Supporting Information, Appendix 2B, in the online version of this article for detailed results). Median number of items remembered correctly was 4 out of 15 (IQR=26). Median number of incorrectly remembered items was 0.5 (IQR=01). Up to 57% (20 subjects) incorrectly remembered at least 1 item. The medical vignette memory score was significantly correlated with immediate recall score (r=0.49, P<0.01), but not immediate recognition score (r=0.24, P=0.16), delayed recall (r=0.13, P=0.47), or delayed recognition (r=0.01, P=0.96). There was a negative relationship between the number of items correctly recalled by a subject and the number of incorrectly recalled items on the medical vignette memory task that did not reach statistical significance (r=0.32, P=0.06).
There was no association between sleep duration, sleep efficiency, and KSQI with memory scores (immediate and delayed recall, immediate and delayed recognition, medical vignette task) (Table 2.) The relationship between objective sleep measures and immediate memory are plotted in Figure 3. Finally, there was no significant difference in sleep duration or efficiency between groups with high memory (immediate recall of >3 words) and low memory (immediate recall of 3 words).
Independent Variables | ||||
---|---|---|---|---|
Sleep Duration, h | Sleep Efficiency, % | Karolinska Sleep Quality Index | ||
Immediate recall (n=59) | Pearson's r | 0.044 | 0.2 | 0.18 |
coefficient | 0.042 | 0.025 | 0.27 | |
P value | 0.74 | 0.12 | 0.16 | |
Immediate recognition (n=59) | Pearson's r | 0.066 | 0.037 | 0.13 |
coefficient | 0.080 | 0.0058 | 0.25 | |
P value | 0.62 | 0.78 | 0.31 | |
Delayed recall (n=52) | Pearson's r | 0.028 | 0.0020 | 0.0081 |
coefficient | 0.027 | 0.00025 | 0.012 | |
P value | 0.85 | 0.99 | 0.96 | |
Delayed recognition (n=52) | Pearson's r | 0.21 | 0.12 | 0.15 |
coefficient | 0.31 | 0.024 | 0.35 | |
P value | 0.13 | 0.39 | 0.29 |
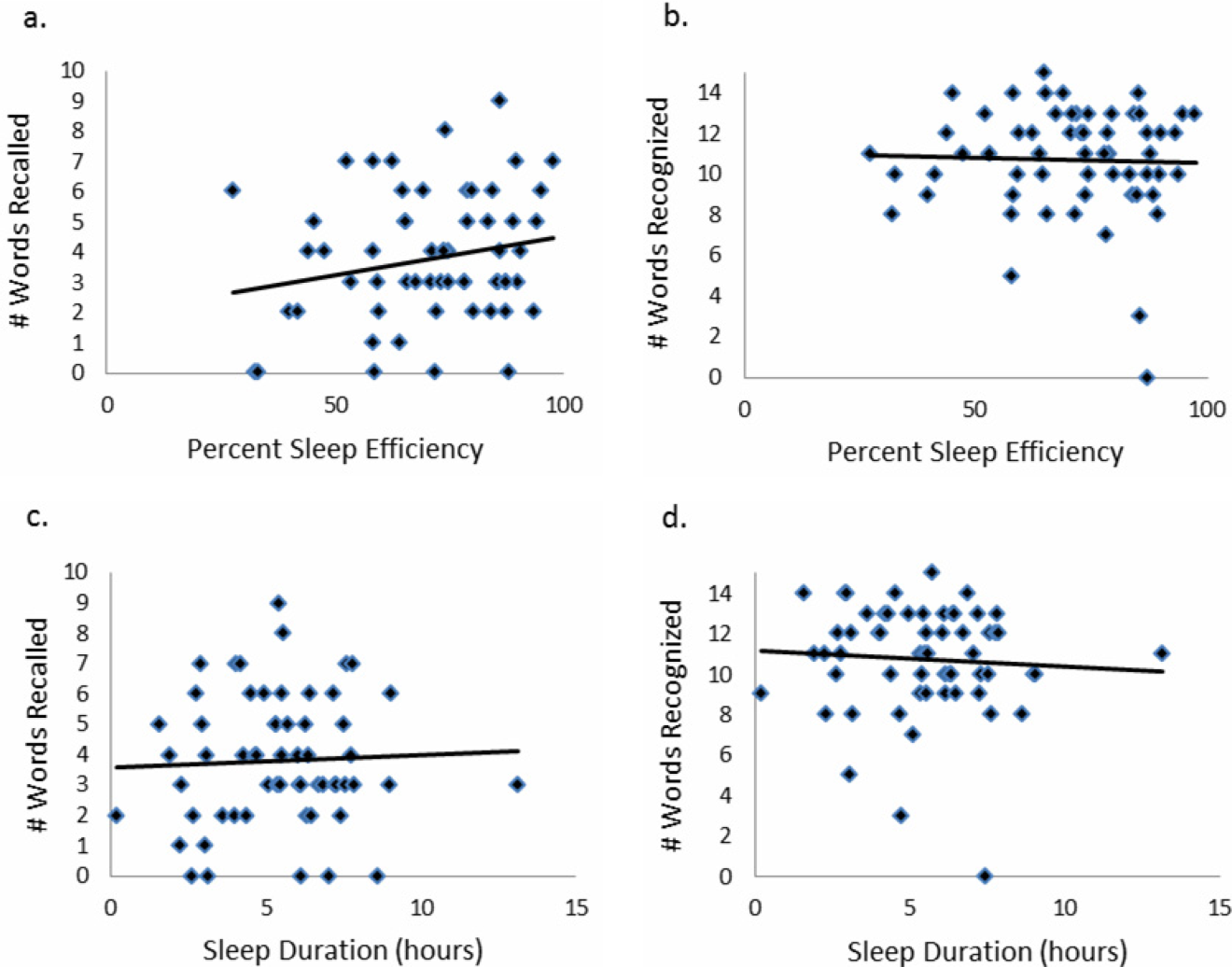
CONCLUSIONS/DISCUSSION
This study demonstrated that roughly half of hospitalized older adults without diagnosed memory or cognitive impairment had poor memory using an immediate word recall task. Although performance on an immediate word recall task may not be considered a good approximation for remembering discharge instructions, immediate recall did correlate with performance on a more complex medical vignette memory task. Though our subjects had low sleep efficiency and duration while in the hospital, memory performance was not significantly associated with inpatient sleep.
Perhaps the most concerning finding in this study was the substantial number of subjects who had poor memory. In addition to scoring approximately 1 SD lower than the community sample of healthy older adults tested in the sensitivity study of USC‐REMT,[14] our subjects also scored lower on immediate recall when compared to another hospitalized patient study.[4] In the study by Lindquist et al. that utilized a similar 15‐item word recall task in hospitalized patients, 29% of subjects were found to have poor memory (recall score of 3 words), compared to 49% in our study. In our 24‐hour delayed recall task we found that 44% of our patients could not recall a single word, with 65% remembering 1 word or fewer. In their study, Lindquist et al. similarly found that greater than 50% of subjects qualified as poor memory by recalling 1 or fewer words after merely an 8‐minute delay. Given these findings, hospitalization may not be the optimal teachable moment that it is often suggested to be. Use of transition coaches, memory aids like written instructions and reminders, and involvement of caregivers are likely critical to ensuring inpatients retain instructions and knowledge. More focus also needs to be given to older patients, who often have the worst memory. Technology tools, such as the Vocera Good To Go app, could allow medical professionals to make audio recordings of discharge instructions that patients may access at any time on a mobile device.
This study also has implications for how to measure memory in inpatients. For example, a vignette‐based memory test may be appropriate for assessing inpatient memory for discharge instructions. Our task was easy to administer and correlated with immediate recall scores. Furthermore, the story‐based task helps us to establish a sense of how much information from a paragraph is truly remembered. Our data show that only 4 items of 15 were remembered, and the majority of subjects actually misremembered 1 item. This latter measure sheds light on the rate of inaccuracy of patient recall. It is worth noting also that word recognition showed a ceiling effect in our sample, suggesting the task was too easy. In contrast, delayed recall was too difficult, as scores showed a floor effect, with over half of our sample unable to recall a single word after a 24‐hour delay.
This is the first study to assess the relationship between sleep loss and memory in hospitalized patients. We found that memory scores were not significantly associated with sleep duration, sleep efficiency, or with the self‐reported KSQI. Memory during hospitalization may be affected by factors other than sleep, like cognition, obscuring the relationship between sleep and memory. It is also possible that we were unable to see a significant association between sleep and memory because of universally low sleep duration and efficiency scores in the hospital.
Our study has several limitations. Most importantly, this study includes a small number of subjects who were hospitalized on a general medicine service at a single institution, limiting generalizability. Also importantly, our data capture only 1 night of sleep, and this may limit our study's ability to detect an association between hospital sleep and memory. More longitudinal data measuring sleep and memory across a longer period of time may reveal the distinct contribution of in‐hospital sleep. We also excluded patients with known cognitive impairment from enrollment, limiting our patient population to those with only high cognitive reserve. We hypothesize that patients with dementia experience both increased sleep disturbance and greater decline in memory during hospitalization. In addition, we are unable to test causal associations in this observational study. Furthermore, we applied a standardized memory test, the USC‐REMT, in a hospital setting, where noise and other disruptions at the time of test administration cannot be completely controlled. This makes it difficult to compare our results with those of community‐dwelling members taking the test under optimal conditions. Finally, because we created our own medical vignette task, future testing to validate this method against other memory testing is warranted.
In conclusion, our results show that memory in older hospitalized inpatients is often impaired, despite patients' appearing cognitively intact. These deficits in memory are revealed by a word recall task and also by a medical vignette task that more closely approximates memory for complex discharge instructions.
Disclosure
This work was funded by the National Institute on Aging Short‐Term Aging‐Related Research Program (5T35AG029795),the National Institute on Aging Career Development Award (K23AG033763), and the National Heart Lung and Blood Institute (R25 HL116372).
- Importance of in‐hospital initiation of evidence‐based medical therapies for heart failure: taking advantage of the teachable moment. Congest Heart Fail. 2005;11(3):153–154. .
- Smoking cessation in hospitalized patients: results of a randomized trial. Arch Intern Med. 1997;157(4):409–415. , , , , .
- Smoking cessation interventions for hospitalized smokers: a systematic review. Arch Intern Med. 2008;168(18):1950–1960. , , .
- Improvements in cognition following hospital discharge of community dwelling seniors. J Gen Intern Med. 2011;26(7):765–770. , , , , .
- Sleep and aging: 1. sleep disorders commonly found in older people. Can Med Assoc J. 2007;176(9):1299–1304. , , , .
- Noise and sleep among adult medical inpatients: far from a quiet night. Arch Intern Med. 2012;172(1):68–70. .
- Perceived control and sleep in hospitalized older adults: a sound hypothesis? J Hosp Med. 2013;8(4):184–190. , , , , , .
- A meta‐analysis of the impact of short‐term sleep deprivation on cognitive variables. Psychol Bull. 2010;136(3):375–389. , .
- Sleep deprivation: Impact on cognitive performance. Neuropsychiatr Dis Treat. 2007;3(5):553–567. , .
- Effects of physician experience on costs and outcomes on an academic general medicine service: results of a trial of hospitalists. Ann Intern Med. 2002;137(11):866–874. , , , et al.
- “Mini‐mental state”: a practical method for grading the cognitive state of patients for the clinician. J Psychiatr Res. 1975;12(3):189–198. , , .
- A short portable mental status questionnaire for the assessment of organic brain deficit in elderly patients. J Am Geriatr Soc. 1975;10:433–441. .
- Reliability and validity of the Short Portable Mental Status Questionnaire administered by telephone. J Geriatr Psychiatry Neurol. 1994;7(1):33–38. , , , .
- Aging, recall and recognition: a study on the sensitivity of the University of Southern California Repeatable Episodic Memory Test (USC‐REMT). J Clin Exp Neuropsychol. 2004;26(3):428–440. , , , .
- University of southern california repeatable episodic memory test. J Clin Exp Neuropsychol. 1995;17(6):926–936. , , , , .
- Development of alternate paragraphs for the logical memory subtest of the Wechsler Memory Scale‐Revised. Clin Neuropsychol. 1997;11(4):370–374. , , .
- A Compendium of Neuropsychological Tests: Administration, Norms, and Commentary. 3rd ed. New York, NY: Oxford University Press; 2009. , , .
- Review of physical activity measurement using accelerometers in older adults: considerations for research design and conduct. Prev Med. 2009;48(2):108–114. .
- The actigraph data analysis software: I. A novel approach to scoring and interpreting sleep‐wake activity. Percept Mot Skills. 1997;85(1):207–216. , , , , , .
- Evaluation of immobility time for sleep latency in actigraphy. Sleep Med. 2009;10(6):621–625. , , , et al.
- The subjective meaning of sleep quality: a comparison of individuals with and without insomnia. Sleep. 2008;31(3):383–393. , , , , .
- Objective components of individual differences in subjective sleep quality. J Sleep Res. 1997;6(4):217–220. , .
- Research electronic data capture (REDCap)—a metadata‐driven methodology and workflow process for providing translational research informatics support. J Biomed Inform. 2009;42(2):377–381. , , , , , .
- A Wilcoxon‐type test for trend. Stat Med. 1985;4(1):87–90. .
- Derivation of research diagnostic criteria for insomnia: report of an American Academy of Sleep Medicine Work Group. Sleep. 2004;27(8):1567–1596. , , , et al.
- Quantitative criteria for insomnia. Behav Res Ther. 2003;41(4):427–445. , , , , .
- Importance of in‐hospital initiation of evidence‐based medical therapies for heart failure: taking advantage of the teachable moment. Congest Heart Fail. 2005;11(3):153–154. .
- Smoking cessation in hospitalized patients: results of a randomized trial. Arch Intern Med. 1997;157(4):409–415. , , , , .
- Smoking cessation interventions for hospitalized smokers: a systematic review. Arch Intern Med. 2008;168(18):1950–1960. , , .
- Improvements in cognition following hospital discharge of community dwelling seniors. J Gen Intern Med. 2011;26(7):765–770. , , , , .
- Sleep and aging: 1. sleep disorders commonly found in older people. Can Med Assoc J. 2007;176(9):1299–1304. , , , .
- Noise and sleep among adult medical inpatients: far from a quiet night. Arch Intern Med. 2012;172(1):68–70. .
- Perceived control and sleep in hospitalized older adults: a sound hypothesis? J Hosp Med. 2013;8(4):184–190. , , , , , .
- A meta‐analysis of the impact of short‐term sleep deprivation on cognitive variables. Psychol Bull. 2010;136(3):375–389. , .
- Sleep deprivation: Impact on cognitive performance. Neuropsychiatr Dis Treat. 2007;3(5):553–567. , .
- Effects of physician experience on costs and outcomes on an academic general medicine service: results of a trial of hospitalists. Ann Intern Med. 2002;137(11):866–874. , , , et al.
- “Mini‐mental state”: a practical method for grading the cognitive state of patients for the clinician. J Psychiatr Res. 1975;12(3):189–198. , , .
- A short portable mental status questionnaire for the assessment of organic brain deficit in elderly patients. J Am Geriatr Soc. 1975;10:433–441. .
- Reliability and validity of the Short Portable Mental Status Questionnaire administered by telephone. J Geriatr Psychiatry Neurol. 1994;7(1):33–38. , , , .
- Aging, recall and recognition: a study on the sensitivity of the University of Southern California Repeatable Episodic Memory Test (USC‐REMT). J Clin Exp Neuropsychol. 2004;26(3):428–440. , , , .
- University of southern california repeatable episodic memory test. J Clin Exp Neuropsychol. 1995;17(6):926–936. , , , , .
- Development of alternate paragraphs for the logical memory subtest of the Wechsler Memory Scale‐Revised. Clin Neuropsychol. 1997;11(4):370–374. , , .
- A Compendium of Neuropsychological Tests: Administration, Norms, and Commentary. 3rd ed. New York, NY: Oxford University Press; 2009. , , .
- Review of physical activity measurement using accelerometers in older adults: considerations for research design and conduct. Prev Med. 2009;48(2):108–114. .
- The actigraph data analysis software: I. A novel approach to scoring and interpreting sleep‐wake activity. Percept Mot Skills. 1997;85(1):207–216. , , , , , .
- Evaluation of immobility time for sleep latency in actigraphy. Sleep Med. 2009;10(6):621–625. , , , et al.
- The subjective meaning of sleep quality: a comparison of individuals with and without insomnia. Sleep. 2008;31(3):383–393. , , , , .
- Objective components of individual differences in subjective sleep quality. J Sleep Res. 1997;6(4):217–220. , .
- Research electronic data capture (REDCap)—a metadata‐driven methodology and workflow process for providing translational research informatics support. J Biomed Inform. 2009;42(2):377–381. , , , , , .
- A Wilcoxon‐type test for trend. Stat Med. 1985;4(1):87–90. .
- Derivation of research diagnostic criteria for insomnia: report of an American Academy of Sleep Medicine Work Group. Sleep. 2004;27(8):1567–1596. , , , et al.
- Quantitative criteria for insomnia. Behav Res Ther. 2003;41(4):427–445. , , , , .
© 2015 Society of Hospital Medicine
Inpatients With Poor Vision
Vision impairment is an under‐recognized risk factor for adverse events among hospitalized patients.[1, 2, 3] Inpatients with poor vision are at increased risk for falls and delirium[1, 3] and have more difficulty taking medications.[4, 5] They may also be at risk for being unable to read critical health information, including consent forms and discharge instructions, or decreased quality of life such as simply ordering food from menus. However, vision is neither routinely tested nor documented for inpatients. Low‐cost ($8 and up) nonprescription reading glasses, known as readers may be a simple, high‐value intervention to improve inpatients' vision. We aimed to study initial feasibility and efficacy of screening and correcting inpatients' vision.
METHODS
From June 2012 through January 2014, research assistants (RAs) identified eligible (adults [18 years], English speaking) participants daily from electronic medical records as part of an ongoing study of general medicine inpatients measuring quality‐of‐care at the University of Chicago Medicine.[6] RAs tested visual acuity using Snellen pocket charts (participants wore corrective lenses if available). For eligible participants, readers were tested with sequential fitting (+2/+2.25/+2.75/+3.25) until vision was corrected (sufficient vision: at least 20/50 acuity in at least 1 eye).[7] Eligible participants included those with insufficient vision who were not already wearing corrective lenses and had no documented blindness or medically severe vision loss, for whom nonprescription readers would be unlikely to correct vision deficiencies such as cataracts or glaucoma. The study was approved by the University of Chicago Institutional Review Board (IRB #9967).
Of note, although readers are typically used in populations over 40 years of age, readers were fitted for all participants to assess their utility for any hospitalized adult patient. Upon completing the vision screening and readers interventions, participants received instruction on how to access vision care and how to obtain readers (if they corrected vision) after hospital discharge.
Descriptive statistics and tests of comparison, including t tests and [2] tests, were used when appropriate. All analyses were performed using Stata version 12 (StataCorp, College Station, TX).
RESULTS
Over 800 participants' vision was screened (n=853); the majority were female (56%, 480/853), African American (76%, 650/853), with a mean age of 53.4 years (standard deviation 18.7), consistent with our study site's demographics. Over one‐third (36%, 304/853) of participants had insufficient vision. Older (65 years) participants (56%, 136/244) were more likely to have insufficient vision than younger participants (28%, 168/608; P<0.001).
Participants with insufficient vision were wearing their own corrective lenses during the testing (150/304, 49%), did not use corrective lenses (53/304, 17%), or were without available corrective lenses (99/304, 33%) (Figure 1A).
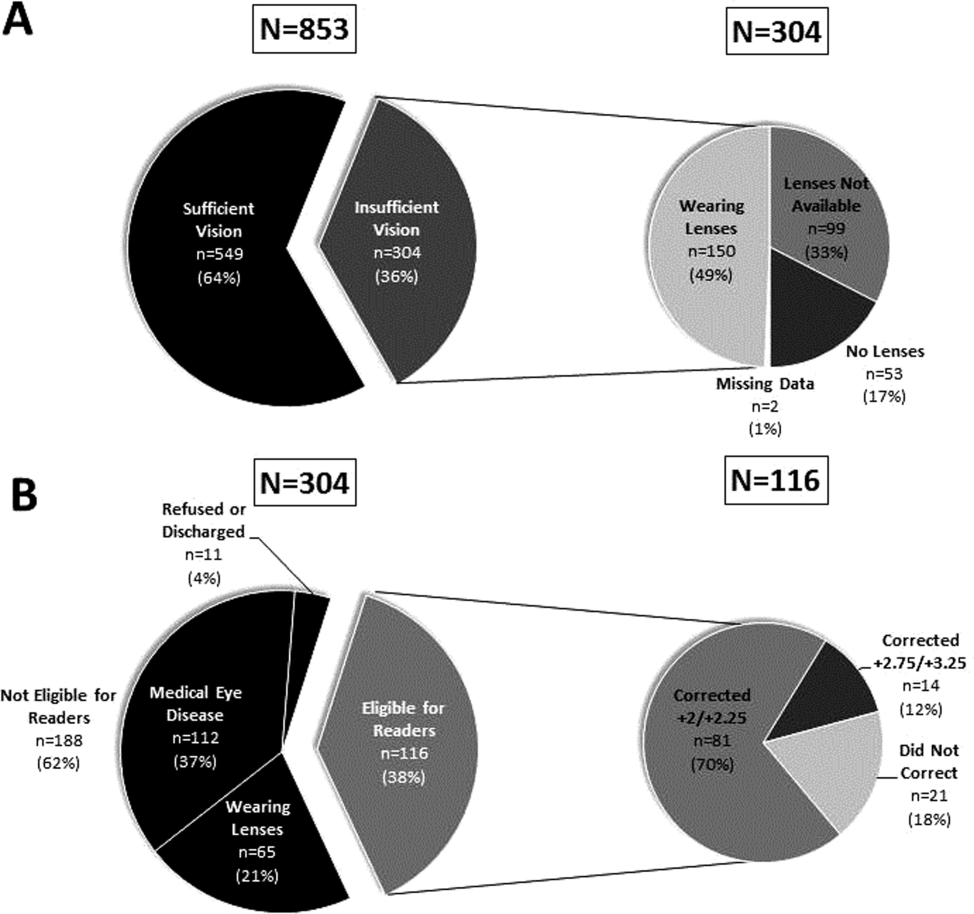
One‐hundred sixteen of 304 participants approached for the readers intervention were eligible (112 reported medical eye disease, 65 were wearing lenses, and 11 refused or were discharged before intervention implementation).
Nonprescription readers corrected the majority of eligible participants' vision (82%, 95/116). Most participants' (81/116, 70%) vision was corrected using the 2 lowest calibration readers (+2/+2.25); another 14 participants' (12%) vision was corrected with higher‐strength lenses (+2.75/+3.25) (Figure 1B)
DISCUSSION
We found that over one‐third of the inpatients we examined have poor vision. Furthermore, among an easily identified subgroup of inpatients with poor vision, low‐cost readers successfully corrected most participants' vision. Although preventive health is not commonly considered an inpatient issue, hospitalists and other clinicians working in the inpatient setting can play an important role in identifying opportunities to provide high‐value care related to patients' vision.
Several important ethical, safety, and cost considerations related to these findings exist. Hospitalized patients commonly sign written informed consent; therefore, due diligence to ensure patients' ability to read and understand the forms is imperative. Further, inpatient delirium is common, particularly among older patients.[8] Existing or new onset delirium occurs in up to 24% to 35% of elderly inpatients.[8] Vision is an important risk factor for multifactorial inpatient delirium, and early vision correction has been shown to improve delirium rates, as part of a multicomponent intervention.[9] Hospital‐related patient costs per delirium episode have been estimated at $16,303 to $64,421.[10] The cost of a multicomponent intervention was $6341 per case of delirium prevented,[9] whereas only 1 potentially critical component, the cost of readers ($8+), would pale in comparison.[1] Vision screening takes approximately 2.25 minutes plus 2 to 6 minutes for the readers' assessment, with little training and high fidelity. Therefore, this easily implemented, potentially cost saving, intervention targeting inpatients with poor vision may improve patient safety and quality of life in the hospital and even after discharge.
Limitations of the study include considerations of generalizability, as participants were from a single, urban, academic medical center. Additionally, long‐term benefits of the readers intervention were not assessed in this study. Finally, RAs provided the assessments; therefore, further work is required to determine costs of efficient large‐scale clinical implementation through nurse‐led programs.
Despite these study limitations, the surprisingly high prevalence of poor vision among inpatients is a call to action for hospitalists. Future work should investigate the impact and cost of vision correction on hospital outcomes such as patient satisfaction, reduced rehospitalizations, and decreased delirium.[11]
Acknowledgements
The authors thank several individuals for their assistance with this project. Andrea Flores, MA, Senior Programmer, helped with programming and data support. Kristin Constantine, BA, Project Manager, helped with developing and implementing the database for this project. Edward Kim, BA, Project Manager, helped with management of the database and data collection. The authors also thank Ainoa Coltri and the Hospitalist Project research assistants for assistance with data collection, Frank Zadravecz, MPH, for assistance with the creation of figures, and Nicole Twu, MS, for assistance with the project. The authors thank other students who helped to collect data for this project, including Allison Louis, Victoria Moreira, and Esther Schoenfeld.
Disclosures: Dr. Press is supported by a career development award from the National Heart Lung and Blood Institute (NIH K23HL118151). A pilot award from The Center on the Demography and Economics of Aging (CoA, National Institute of Aging P30 AG012857) supported this project. Dr. Matthiesen and Ms. Ranadive received support from the Summer Research Program funded by the National Institutes on Aging Short‐Term Aging‐Related Research Program (T35AG029795). Dr. Matthiesen also received funding from the Calvin Fentress Fellowship Program. Dr. Hariprasad reports being a consultant or participating on a speaker's bureau for Alcon, Allergan, Regeneron, Genentech, Optos, OD‐OS, Bayer, Clearside Biomedical, and Ocular Therapeutix. Dr. Meltzer received funding from the National Institutes on Aging Short‐Term Aging‐Related Research Program (T35AG029795), and from the Agency for Healthcare Quality and Research through the Hospital Medicine and Economics Center for Education and Research in Therapeutics (U18 HS016967‐01), and from the National Institute of Aging through a Midcareer Career Development Award (K24 AG031326‐01), from the National Cancer Institute (KM1 CA156717), and from the National Center for Advancing Translational Science (2UL1TR000430‐06). Dr. Arora received funding from the National Institutes on Aging Short‐Term Aging‐Related Research Program (T35AG029795) and National Institutes on Aging (K23AG033763).
- Risk factors and risk assessment tools for falls in hospital in‐patients: a systematic review. Age Ageing. 2004;33(2):122–130. , , , .
- More than meets the eye: relationship between low health literacy and poor vision in hospitalized patients. J Health Commun. 2013;18(suppl 1):197–204. , , , , .
- Risk factors for delirium at discharge: development and validation of a predictive model. Arch Intern Med. 2007;167(13):1406–1413. , , , , , .
- Misuse of respiratory inhalers in hospitalized patients with asthma or COPD. J Gen Intern Med. 2011;26(6):635–642. , , , et al.
- Can elderly people take their medicine? Patient Educ Couns. 2005;59(2):186–191. , , .
- Effects of physician experience on costs and outcomes on an academic general medicine service: results of a trial of hospitalists. Ann Intern Med. 2002;137(11):866–874. , , , et al.
- Prospective evaluation of visual acuity assessment: a comparison of Snellen versus ETDRS charts in clinical practice (An AOS Thesis). Trans Am Ophthalmol Soc. 2009;107:311–324. .
- Delirium. The occurrence and persistence of symptoms among elderly hospitalized patients. Arch Intern Med. 1992;152(2):334–340. , , , et al.
- A multicomponent intervention to prevent delirium in hospitalized older patients. N Engl J Med. 1999;340(9):669–676. , , , et al.
- One‐year health care costs associated with delirium in the elderly population. Arch Intern Med. 2008;168(1):27–32. , , , , .
- A low‐vision rehabilitation program for patients with mild cognitive deficits. JAMA Ophthalmol. 2013;131(7):912–919. , , , et al.
Vision impairment is an under‐recognized risk factor for adverse events among hospitalized patients.[1, 2, 3] Inpatients with poor vision are at increased risk for falls and delirium[1, 3] and have more difficulty taking medications.[4, 5] They may also be at risk for being unable to read critical health information, including consent forms and discharge instructions, or decreased quality of life such as simply ordering food from menus. However, vision is neither routinely tested nor documented for inpatients. Low‐cost ($8 and up) nonprescription reading glasses, known as readers may be a simple, high‐value intervention to improve inpatients' vision. We aimed to study initial feasibility and efficacy of screening and correcting inpatients' vision.
METHODS
From June 2012 through January 2014, research assistants (RAs) identified eligible (adults [18 years], English speaking) participants daily from electronic medical records as part of an ongoing study of general medicine inpatients measuring quality‐of‐care at the University of Chicago Medicine.[6] RAs tested visual acuity using Snellen pocket charts (participants wore corrective lenses if available). For eligible participants, readers were tested with sequential fitting (+2/+2.25/+2.75/+3.25) until vision was corrected (sufficient vision: at least 20/50 acuity in at least 1 eye).[7] Eligible participants included those with insufficient vision who were not already wearing corrective lenses and had no documented blindness or medically severe vision loss, for whom nonprescription readers would be unlikely to correct vision deficiencies such as cataracts or glaucoma. The study was approved by the University of Chicago Institutional Review Board (IRB #9967).
Of note, although readers are typically used in populations over 40 years of age, readers were fitted for all participants to assess their utility for any hospitalized adult patient. Upon completing the vision screening and readers interventions, participants received instruction on how to access vision care and how to obtain readers (if they corrected vision) after hospital discharge.
Descriptive statistics and tests of comparison, including t tests and [2] tests, were used when appropriate. All analyses were performed using Stata version 12 (StataCorp, College Station, TX).
RESULTS
Over 800 participants' vision was screened (n=853); the majority were female (56%, 480/853), African American (76%, 650/853), with a mean age of 53.4 years (standard deviation 18.7), consistent with our study site's demographics. Over one‐third (36%, 304/853) of participants had insufficient vision. Older (65 years) participants (56%, 136/244) were more likely to have insufficient vision than younger participants (28%, 168/608; P<0.001).
Participants with insufficient vision were wearing their own corrective lenses during the testing (150/304, 49%), did not use corrective lenses (53/304, 17%), or were without available corrective lenses (99/304, 33%) (Figure 1A).
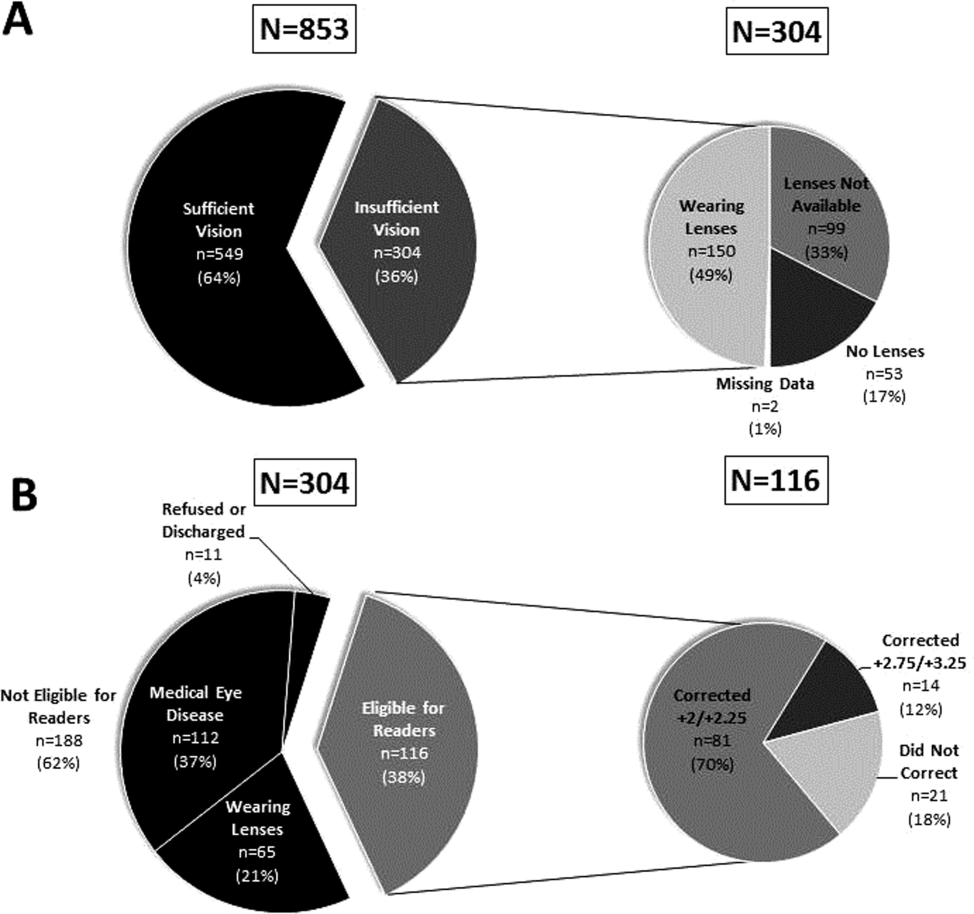
One‐hundred sixteen of 304 participants approached for the readers intervention were eligible (112 reported medical eye disease, 65 were wearing lenses, and 11 refused or were discharged before intervention implementation).
Nonprescription readers corrected the majority of eligible participants' vision (82%, 95/116). Most participants' (81/116, 70%) vision was corrected using the 2 lowest calibration readers (+2/+2.25); another 14 participants' (12%) vision was corrected with higher‐strength lenses (+2.75/+3.25) (Figure 1B)
DISCUSSION
We found that over one‐third of the inpatients we examined have poor vision. Furthermore, among an easily identified subgroup of inpatients with poor vision, low‐cost readers successfully corrected most participants' vision. Although preventive health is not commonly considered an inpatient issue, hospitalists and other clinicians working in the inpatient setting can play an important role in identifying opportunities to provide high‐value care related to patients' vision.
Several important ethical, safety, and cost considerations related to these findings exist. Hospitalized patients commonly sign written informed consent; therefore, due diligence to ensure patients' ability to read and understand the forms is imperative. Further, inpatient delirium is common, particularly among older patients.[8] Existing or new onset delirium occurs in up to 24% to 35% of elderly inpatients.[8] Vision is an important risk factor for multifactorial inpatient delirium, and early vision correction has been shown to improve delirium rates, as part of a multicomponent intervention.[9] Hospital‐related patient costs per delirium episode have been estimated at $16,303 to $64,421.[10] The cost of a multicomponent intervention was $6341 per case of delirium prevented,[9] whereas only 1 potentially critical component, the cost of readers ($8+), would pale in comparison.[1] Vision screening takes approximately 2.25 minutes plus 2 to 6 minutes for the readers' assessment, with little training and high fidelity. Therefore, this easily implemented, potentially cost saving, intervention targeting inpatients with poor vision may improve patient safety and quality of life in the hospital and even after discharge.
Limitations of the study include considerations of generalizability, as participants were from a single, urban, academic medical center. Additionally, long‐term benefits of the readers intervention were not assessed in this study. Finally, RAs provided the assessments; therefore, further work is required to determine costs of efficient large‐scale clinical implementation through nurse‐led programs.
Despite these study limitations, the surprisingly high prevalence of poor vision among inpatients is a call to action for hospitalists. Future work should investigate the impact and cost of vision correction on hospital outcomes such as patient satisfaction, reduced rehospitalizations, and decreased delirium.[11]
Acknowledgements
The authors thank several individuals for their assistance with this project. Andrea Flores, MA, Senior Programmer, helped with programming and data support. Kristin Constantine, BA, Project Manager, helped with developing and implementing the database for this project. Edward Kim, BA, Project Manager, helped with management of the database and data collection. The authors also thank Ainoa Coltri and the Hospitalist Project research assistants for assistance with data collection, Frank Zadravecz, MPH, for assistance with the creation of figures, and Nicole Twu, MS, for assistance with the project. The authors thank other students who helped to collect data for this project, including Allison Louis, Victoria Moreira, and Esther Schoenfeld.
Disclosures: Dr. Press is supported by a career development award from the National Heart Lung and Blood Institute (NIH K23HL118151). A pilot award from The Center on the Demography and Economics of Aging (CoA, National Institute of Aging P30 AG012857) supported this project. Dr. Matthiesen and Ms. Ranadive received support from the Summer Research Program funded by the National Institutes on Aging Short‐Term Aging‐Related Research Program (T35AG029795). Dr. Matthiesen also received funding from the Calvin Fentress Fellowship Program. Dr. Hariprasad reports being a consultant or participating on a speaker's bureau for Alcon, Allergan, Regeneron, Genentech, Optos, OD‐OS, Bayer, Clearside Biomedical, and Ocular Therapeutix. Dr. Meltzer received funding from the National Institutes on Aging Short‐Term Aging‐Related Research Program (T35AG029795), and from the Agency for Healthcare Quality and Research through the Hospital Medicine and Economics Center for Education and Research in Therapeutics (U18 HS016967‐01), and from the National Institute of Aging through a Midcareer Career Development Award (K24 AG031326‐01), from the National Cancer Institute (KM1 CA156717), and from the National Center for Advancing Translational Science (2UL1TR000430‐06). Dr. Arora received funding from the National Institutes on Aging Short‐Term Aging‐Related Research Program (T35AG029795) and National Institutes on Aging (K23AG033763).
Vision impairment is an under‐recognized risk factor for adverse events among hospitalized patients.[1, 2, 3] Inpatients with poor vision are at increased risk for falls and delirium[1, 3] and have more difficulty taking medications.[4, 5] They may also be at risk for being unable to read critical health information, including consent forms and discharge instructions, or decreased quality of life such as simply ordering food from menus. However, vision is neither routinely tested nor documented for inpatients. Low‐cost ($8 and up) nonprescription reading glasses, known as readers may be a simple, high‐value intervention to improve inpatients' vision. We aimed to study initial feasibility and efficacy of screening and correcting inpatients' vision.
METHODS
From June 2012 through January 2014, research assistants (RAs) identified eligible (adults [18 years], English speaking) participants daily from electronic medical records as part of an ongoing study of general medicine inpatients measuring quality‐of‐care at the University of Chicago Medicine.[6] RAs tested visual acuity using Snellen pocket charts (participants wore corrective lenses if available). For eligible participants, readers were tested with sequential fitting (+2/+2.25/+2.75/+3.25) until vision was corrected (sufficient vision: at least 20/50 acuity in at least 1 eye).[7] Eligible participants included those with insufficient vision who were not already wearing corrective lenses and had no documented blindness or medically severe vision loss, for whom nonprescription readers would be unlikely to correct vision deficiencies such as cataracts or glaucoma. The study was approved by the University of Chicago Institutional Review Board (IRB #9967).
Of note, although readers are typically used in populations over 40 years of age, readers were fitted for all participants to assess their utility for any hospitalized adult patient. Upon completing the vision screening and readers interventions, participants received instruction on how to access vision care and how to obtain readers (if they corrected vision) after hospital discharge.
Descriptive statistics and tests of comparison, including t tests and [2] tests, were used when appropriate. All analyses were performed using Stata version 12 (StataCorp, College Station, TX).
RESULTS
Over 800 participants' vision was screened (n=853); the majority were female (56%, 480/853), African American (76%, 650/853), with a mean age of 53.4 years (standard deviation 18.7), consistent with our study site's demographics. Over one‐third (36%, 304/853) of participants had insufficient vision. Older (65 years) participants (56%, 136/244) were more likely to have insufficient vision than younger participants (28%, 168/608; P<0.001).
Participants with insufficient vision were wearing their own corrective lenses during the testing (150/304, 49%), did not use corrective lenses (53/304, 17%), or were without available corrective lenses (99/304, 33%) (Figure 1A).
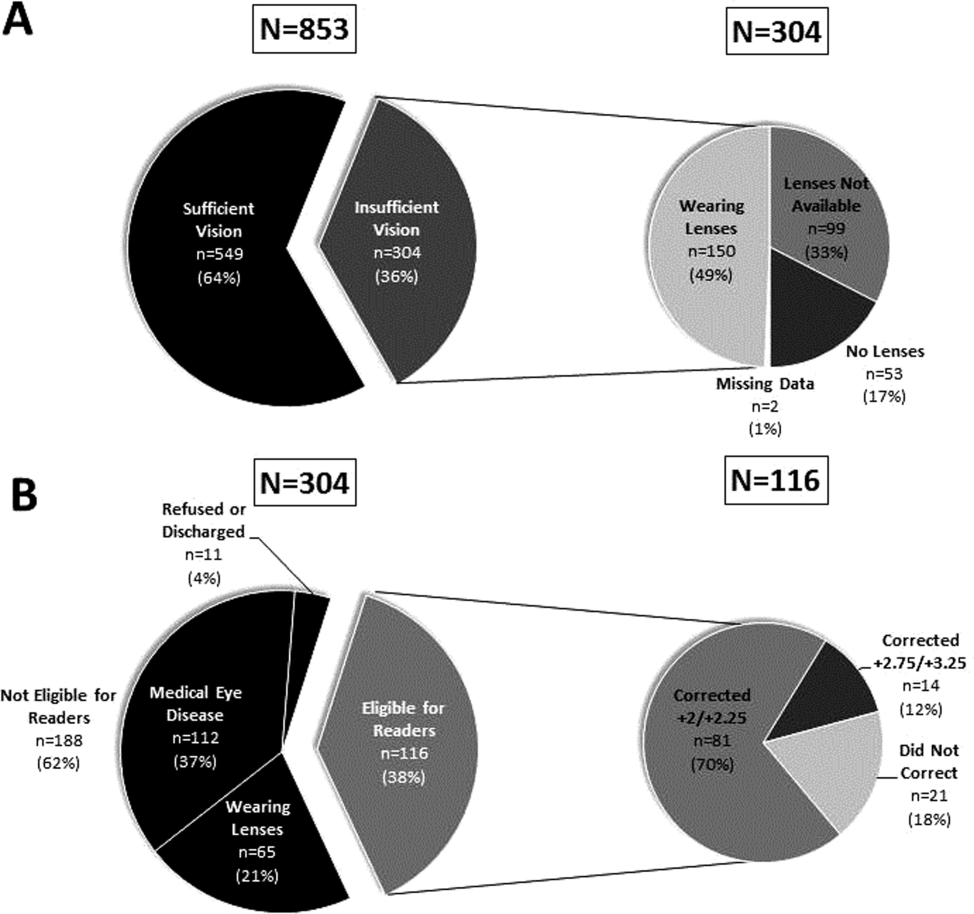
One‐hundred sixteen of 304 participants approached for the readers intervention were eligible (112 reported medical eye disease, 65 were wearing lenses, and 11 refused or were discharged before intervention implementation).
Nonprescription readers corrected the majority of eligible participants' vision (82%, 95/116). Most participants' (81/116, 70%) vision was corrected using the 2 lowest calibration readers (+2/+2.25); another 14 participants' (12%) vision was corrected with higher‐strength lenses (+2.75/+3.25) (Figure 1B)
DISCUSSION
We found that over one‐third of the inpatients we examined have poor vision. Furthermore, among an easily identified subgroup of inpatients with poor vision, low‐cost readers successfully corrected most participants' vision. Although preventive health is not commonly considered an inpatient issue, hospitalists and other clinicians working in the inpatient setting can play an important role in identifying opportunities to provide high‐value care related to patients' vision.
Several important ethical, safety, and cost considerations related to these findings exist. Hospitalized patients commonly sign written informed consent; therefore, due diligence to ensure patients' ability to read and understand the forms is imperative. Further, inpatient delirium is common, particularly among older patients.[8] Existing or new onset delirium occurs in up to 24% to 35% of elderly inpatients.[8] Vision is an important risk factor for multifactorial inpatient delirium, and early vision correction has been shown to improve delirium rates, as part of a multicomponent intervention.[9] Hospital‐related patient costs per delirium episode have been estimated at $16,303 to $64,421.[10] The cost of a multicomponent intervention was $6341 per case of delirium prevented,[9] whereas only 1 potentially critical component, the cost of readers ($8+), would pale in comparison.[1] Vision screening takes approximately 2.25 minutes plus 2 to 6 minutes for the readers' assessment, with little training and high fidelity. Therefore, this easily implemented, potentially cost saving, intervention targeting inpatients with poor vision may improve patient safety and quality of life in the hospital and even after discharge.
Limitations of the study include considerations of generalizability, as participants were from a single, urban, academic medical center. Additionally, long‐term benefits of the readers intervention were not assessed in this study. Finally, RAs provided the assessments; therefore, further work is required to determine costs of efficient large‐scale clinical implementation through nurse‐led programs.
Despite these study limitations, the surprisingly high prevalence of poor vision among inpatients is a call to action for hospitalists. Future work should investigate the impact and cost of vision correction on hospital outcomes such as patient satisfaction, reduced rehospitalizations, and decreased delirium.[11]
Acknowledgements
The authors thank several individuals for their assistance with this project. Andrea Flores, MA, Senior Programmer, helped with programming and data support. Kristin Constantine, BA, Project Manager, helped with developing and implementing the database for this project. Edward Kim, BA, Project Manager, helped with management of the database and data collection. The authors also thank Ainoa Coltri and the Hospitalist Project research assistants for assistance with data collection, Frank Zadravecz, MPH, for assistance with the creation of figures, and Nicole Twu, MS, for assistance with the project. The authors thank other students who helped to collect data for this project, including Allison Louis, Victoria Moreira, and Esther Schoenfeld.
Disclosures: Dr. Press is supported by a career development award from the National Heart Lung and Blood Institute (NIH K23HL118151). A pilot award from The Center on the Demography and Economics of Aging (CoA, National Institute of Aging P30 AG012857) supported this project. Dr. Matthiesen and Ms. Ranadive received support from the Summer Research Program funded by the National Institutes on Aging Short‐Term Aging‐Related Research Program (T35AG029795). Dr. Matthiesen also received funding from the Calvin Fentress Fellowship Program. Dr. Hariprasad reports being a consultant or participating on a speaker's bureau for Alcon, Allergan, Regeneron, Genentech, Optos, OD‐OS, Bayer, Clearside Biomedical, and Ocular Therapeutix. Dr. Meltzer received funding from the National Institutes on Aging Short‐Term Aging‐Related Research Program (T35AG029795), and from the Agency for Healthcare Quality and Research through the Hospital Medicine and Economics Center for Education and Research in Therapeutics (U18 HS016967‐01), and from the National Institute of Aging through a Midcareer Career Development Award (K24 AG031326‐01), from the National Cancer Institute (KM1 CA156717), and from the National Center for Advancing Translational Science (2UL1TR000430‐06). Dr. Arora received funding from the National Institutes on Aging Short‐Term Aging‐Related Research Program (T35AG029795) and National Institutes on Aging (K23AG033763).
- Risk factors and risk assessment tools for falls in hospital in‐patients: a systematic review. Age Ageing. 2004;33(2):122–130. , , , .
- More than meets the eye: relationship between low health literacy and poor vision in hospitalized patients. J Health Commun. 2013;18(suppl 1):197–204. , , , , .
- Risk factors for delirium at discharge: development and validation of a predictive model. Arch Intern Med. 2007;167(13):1406–1413. , , , , , .
- Misuse of respiratory inhalers in hospitalized patients with asthma or COPD. J Gen Intern Med. 2011;26(6):635–642. , , , et al.
- Can elderly people take their medicine? Patient Educ Couns. 2005;59(2):186–191. , , .
- Effects of physician experience on costs and outcomes on an academic general medicine service: results of a trial of hospitalists. Ann Intern Med. 2002;137(11):866–874. , , , et al.
- Prospective evaluation of visual acuity assessment: a comparison of Snellen versus ETDRS charts in clinical practice (An AOS Thesis). Trans Am Ophthalmol Soc. 2009;107:311–324. .
- Delirium. The occurrence and persistence of symptoms among elderly hospitalized patients. Arch Intern Med. 1992;152(2):334–340. , , , et al.
- A multicomponent intervention to prevent delirium in hospitalized older patients. N Engl J Med. 1999;340(9):669–676. , , , et al.
- One‐year health care costs associated with delirium in the elderly population. Arch Intern Med. 2008;168(1):27–32. , , , , .
- A low‐vision rehabilitation program for patients with mild cognitive deficits. JAMA Ophthalmol. 2013;131(7):912–919. , , , et al.
- Risk factors and risk assessment tools for falls in hospital in‐patients: a systematic review. Age Ageing. 2004;33(2):122–130. , , , .
- More than meets the eye: relationship between low health literacy and poor vision in hospitalized patients. J Health Commun. 2013;18(suppl 1):197–204. , , , , .
- Risk factors for delirium at discharge: development and validation of a predictive model. Arch Intern Med. 2007;167(13):1406–1413. , , , , , .
- Misuse of respiratory inhalers in hospitalized patients with asthma or COPD. J Gen Intern Med. 2011;26(6):635–642. , , , et al.
- Can elderly people take their medicine? Patient Educ Couns. 2005;59(2):186–191. , , .
- Effects of physician experience on costs and outcomes on an academic general medicine service: results of a trial of hospitalists. Ann Intern Med. 2002;137(11):866–874. , , , et al.
- Prospective evaluation of visual acuity assessment: a comparison of Snellen versus ETDRS charts in clinical practice (An AOS Thesis). Trans Am Ophthalmol Soc. 2009;107:311–324. .
- Delirium. The occurrence and persistence of symptoms among elderly hospitalized patients. Arch Intern Med. 1992;152(2):334–340. , , , et al.
- A multicomponent intervention to prevent delirium in hospitalized older patients. N Engl J Med. 1999;340(9):669–676. , , , et al.
- One‐year health care costs associated with delirium in the elderly population. Arch Intern Med. 2008;168(1):27–32. , , , , .
- A low‐vision rehabilitation program for patients with mild cognitive deficits. JAMA Ophthalmol. 2013;131(7):912–919. , , , et al.
Sleep in Hospitalized Adults
Lack of sleep is a common problem in hospitalized patients and is associated with poorer health outcomes, especially in older patients.[1, 2, 3] Prior studies highlight a multitude of factors that can result in sleep loss in the hospital[3, 4, 5, 6] with 1 of the most common causes of sleep disruption in the hospital being noise.[7, 8, 9]
In addition to external factors, such as hospital noise, there may be inherent characteristics that predispose certain patients to greater sleep loss when hospitalized. One such measure is the construct of perceived control or the psychological measure of how much individuals expect themselves to be capable of bringing about desired outcomes.[10] Among older patients, low perceived control is associated with increased rates of physician visits, hospitalizations, and death.[11, 12] In contrast, patients who feel more in control of their environment may experience positive health benefits.[13]
Yet, when patients are placed in a hospital setting, they experience a significant reduction in control over their environment along with an increase in dependency on medical staff and therapies.[14, 15] For example, hospitalized patients are restricted in their personal decisions, such as what clothes they can wear and what they can eat and are not in charge of their own schedules, including their sleep time.
Although prior studies suggest that perceived control over sleep is related to actual sleep among community‐dwelling adults,[16, 17] no study has examined this relationship in hospitalized adults. Therefore, the aim of our study was to examine the possible association between perceived control, noise levels, and sleep in hospitalized middle‐aged and older patients.
METHODS
Study Design
We conducted a prospective cohort study of subjects recruited from a large ongoing study of admitted patients at the University of Chicago inpatient general medicine service.[18] Because we were interested in middle‐aged and older adults who are most sensitive to sleep disruptions, patients who were age 50 years and over, ambulatory, and living in the community were eligible for the study.[19] Exclusion criteria were cognitive impairment (telephone version of the Mini‐Mental State Exam <17 out of 22), preexisting sleeping disorders identified via patient charts, such as obstructive sleep apnea and narcolepsy, transfer from the intensive care unit (ICU), and admission to the hospital more than 72 hours prior to enrollment.[20] These inclusion and exclusion criteria were selected to identify a patient population with minimal sleep disturbances at baseline. Patients under isolation were excluded because they are not visited as frequently by the healthcare team.[21, 22] Most general medicine rooms were double occupancy but efforts were made to make patient rooms single when possible or required (ie, isolation for infection control). The study was approved by the University of Chicago Institutional Review Board.
Subjective Data Collection
Baseline levels of perceived control over sleep, or the amount of control patients believe they have over their sleep, were assessed using 2 different scales. The first tool was the 8‐item Sleep Locus of Control (SLOC) scale,[17] which ranges from 8 to 48, with higher values corresponding to a greater internal locus of control over sleep. An internal sleep locus of control indicates beliefs that patients feel that they are primarily responsible for their own sleep as opposed to an external locus of control which indicates beliefs that good sleep is due to luck or chance. For example, patients were asked how strongly they agree or disagree with statements, such as, If I take care of myself, I can avoid insomnia and People who never get insomnia are just plain lucky (see Supporting Information, Appendix 2, in the online version of this article). The second tool was the 9‐item Sleep Self‐Efficacy (SSE) scale,[23] which ranges from 9 to 45, with higher values corresponding to greater confidence patients have in their ability to sleep. One of the items asks, How confident are you that you can lie in bed feeling physically relaxed (see Supporting Information, Appendix 1, in the online version of this article)? Both instruments have been validated in an outpatient setting.[23] These surveys were given immediately on enrollment in the study to measure baseline perceived control.
Baseline sleep habits were also collected on enrollment using the Epworth Sleepiness Scale,[24, 25] a standard validated survey that assesses excess daytime sleepiness in various common situations. For each day in the hospital, patients were asked to report in‐hospital sleep quality using the Karolinska Sleep Log.[26] The Karolinska Sleep Quality Index (KSQI) is calculated from 4 items on the Karolinska Sleep Log (sleep quality, sleep restlessness, slept throughout the night, ease of falling asleep). The questions are on a 5‐point scale and the 4 items are averaged for a final score out of 5 with a higher number indicating better subjective sleep quality. The item How much was your sleep disturbed by noise? on the Karolinska Sleep Log was used to assess the degree to which noise was a disruptor of sleep. This question was also on a 5‐point scale with higher scores indicating greater disruptiveness of noise. Patients were also asked how disruptive noise from roommates was on a nightly basis using this same scale.
Objective Data Collection
Wrist activity monitors (Actiwatch 2; Respironics, Inc., Murrysville, PA)[27, 28, 29, 30] were used to measure patient sleep. Actiware 5 software (Respironics, Inc.)[31] was used to estimate quantitative measures of sleep time and efficiency. Sleep time is defined as the total duration of time spent sleeping at night and sleep efficiency is defined as the fraction of time, reported as a percentage, spent sleeping by actigraphy out of the total time patients reported they were sleeping.
Sound levels in patient rooms were recorded using Larson Davis 720 Sound Level Monitors (Larson Davis, Inc., Provo, UT). These monitors store functional average sound pressure levels in A‐weighted decibels called the Leq over 1‐hour intervals. The Leq is the average sound level over the given time interval. Minimum (Lmin) and maximum (Lmax) sound levels are also stored. The LD SLM Utility Program (Larson Davis, Inc.) was used to extract the sound level measurements recorded by the monitors.
Demographic information (age, gender, race, ethnicity, highest level of education, length of stay in the hospital, and comorbidities) was obtained from hospital charts via an ongoing study of admitted patients at the University of Chicago Medical Center inpatient general medicine service.[18] Chart audits were performed to determine whether patients received pharmacologic sleep aids in the hospital.
Data Analysis
Descriptive statistics were used to summarize mean sleep duration and sleep efficiency in the hospital as well as SLOC and SSE. Because the SSE scores were not normally distributed, the scores were dichotomized at the median to create a variable denoting high and low SSE. Additionally, because the distribution of responses to the noise disruption question was skewed to the right, reports of noise disruptions were grouped into not disruptive (score=1) and disruptive (score>1).
Two‐sample t tests with equal variances were used to assess the relationship between perceived control measures (high/low SLOC, SSE) and objective sleep measures (sleep time, sleep efficiency). Multivariate linear regression was used to test the association between high SSE (independent variable) and sleep time (dependent variable), clustering for multiple nights of data within the subject. Multivariate logistic regression, also adjusting for subject, was used to test the association between high SSE and noise disruptiveness and the association between high SSE and Karolinska scores. Leq, Lmax, and Lmin were all tested using stepwise forward regression. Because our prior work[9] demonstrated that noise levels separated into tertiles were significantly associated with sleep time, our analysis also used noise levels separated into tertiles. Stepwise forward regression was used to add basic patient demographics (gender, race, age) to the models. Statistical significance was defined as P<0.05, and all statistical analysis was done using Stata 11.0 (StataCorp, College Station, TX).
RESULTS
From April 2010 to May 2012, 1134 patients were screened by study personnel for this study via an ongoing study of hospitalized patients on the inpatient general medicine ward. Of the 361 (31.8%) eligible patients, 206 (57.1%) consented to participate. Of the subjects enrolled in the study, 118 were able to complete at least 1 night of actigraphy, sound monitoring, and subjective assessment for a total of 185 patient nights (Figure 1).
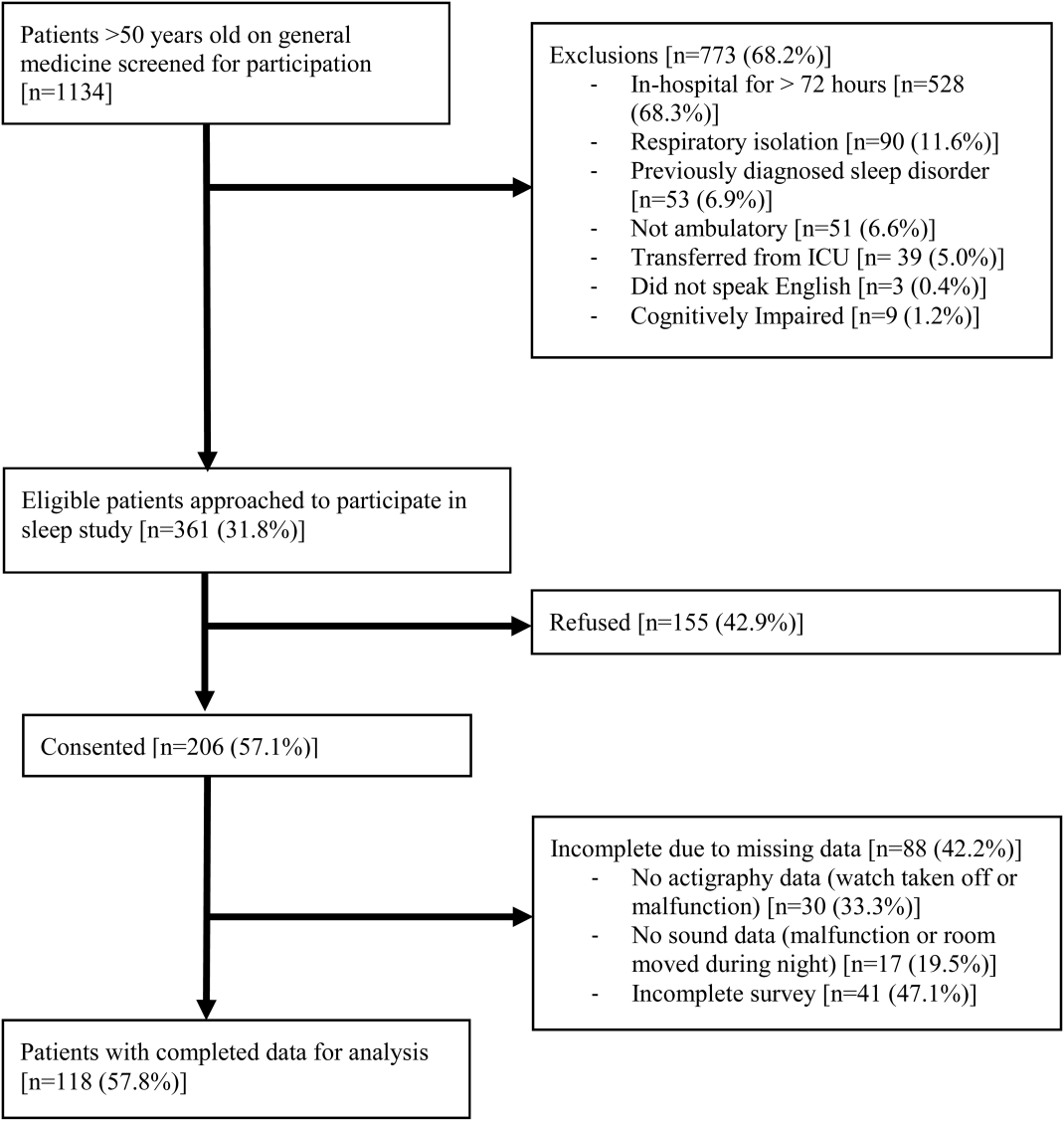
The majority of patients were female (57%), African American (67%), and non‐Hispanic (97%). The mean age was 65 years (standard deviation [SD], 11.6 years), and the median length of stay was 4 days (interquartile range [IQR], 36). The majority of patients also had hypertension (67%), with chronic obstructive pulmonary disease [COPD] (31%) and congestive heart failure (31%) being the next most common comorbidities. About two‐thirds of subjects (64%) were characterized as average or above average sleepers with Epworth Sleepiness Scale scores 9[20] (Table 1). Only 5% of patients received pharmacological sleep aids.
Value, n (%)a | |
---|---|
| |
Patient characteristics | |
Age, mean (SD), y | 63 (12) |
Length of stay, median (IQR), db | 4 (36) |
Female | 67 (57) |
African American | 79 (67) |
Hispanic | 3 (3) |
High school graduate | 92 (78) |
Comorbidities | |
Hypertension | 79 (66) |
Chronic obstructive pulmonary disease | 37 (31) |
Congestive heart failure | 37 (31) |
Diabetes | 36 (30) |
End stage renal disease | 23 (19) |
Baseline sleep characteristics | |
Sleep duration, mean (SD), minc | 333 (128) |
Epworth Sleepiness Scale, score 9d | 73 (64) |
The mean baseline SLOC score was 30.4 (SD, 6.7), with a median of 31 (IQR, 2735). The mean baseline SSE score was 32.1 (SD, 9.4), with a median of 34 (IQR, 2441). Fifty‐four patients were categorized as having high sleep self‐efficacy (high SSE), which we defined as scoring above the median of 34.
Average in‐hospital sleep was 5.5 hours (333 minutes; SD, 128 minutes) which was significantly shorter than the self‐reported sleep duration of 6.5 hours prior to admission (387 minutes, SD, 125 minutes; P=0.0001). The mean sleep efficiency was 73% (SD, 19%) with 55% of actigraphy nights below the normal range of 80% efficiency for adults.[19] Median KSQI was 3.5 (IQR, 2.254.75), with 41% of the patients with a KSQI 3, putting them in the insomniac range.[32] The median score on the noise disruptiveness question was 1 (IQR, 14) with 42% of reports coded as disruptive defined as a score >1 on the 5‐point scale. The median score on the roommate disruptiveness question was 1 (IQR, 11) with 77% of responses coded as not disruptive defined as a score of 1 on the 5‐point scale.
A 2‐sample t test with equal variances showed that those patients reporting high SSE were more likely to sleep longer in the hospital than those reporting low SSE (364 minutes 95% confidence interval [CI]: 340, 388 vs 309 minutes 95% CI: 283, 336; P=0.003) (Figure 2). Patients with high SSE were also more likely to have a normal sleep efficiency (above 80%) compared to those with low SSE (54% 95% CI: 43, 65 vs 38% 95% CI: 28,47; P=0.028). Last, there was a trend toward patients reporting higher SSE to also report less noise disruption compared to those patients with low SSE ([42%] 95% CI: 31, 53 vs [56%] 95% CI: 46, 65; P=0.063) (Figure 3).
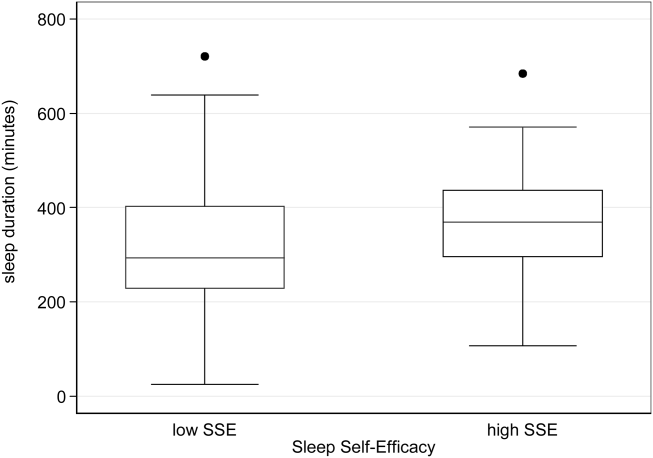
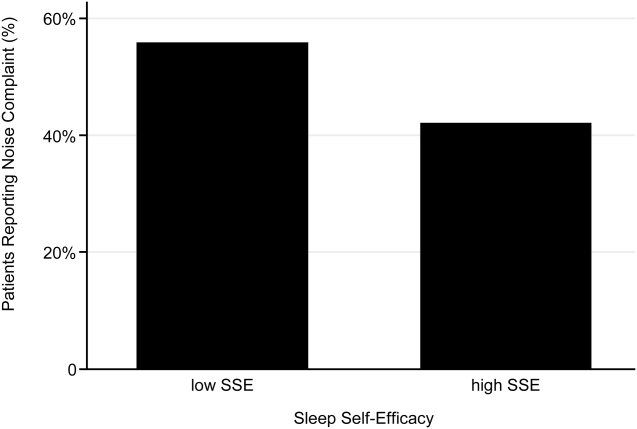
Linear regression clustered by subject showed that high SSE was associated with longer sleep duration (55 minutes 95% CI: 14, 97; P=0.010). Furthermore, high SSE was significantly associated with longer sleep duration after controlling for both objective noise level and patient demographics in the model using stepwise forward regression (50 minutes 95% CI: 11, 90; P=0.014) (Table 2).
Sleep Duration (min) | Model 1 Beta [95% CI]a | Model 2 Beta [95% CI]a |
---|---|---|
| ||
High SSE | 55 [14, 97]b | 50 [11, 90]b |
Lmin tert 3 | 14 [59, 29] | |
Lmin tert 2 | 21 [65, 23] | |
Female | 49 [10, 89]b | |
African American | 16 [59, 27] | |
Age | 1 [0.9, 3] | |
Karolinska Sleep Quality | Model 1 OR [95% CI]c | Model 2 OR [95% CI]c |
High SSE | 2.04 [1.12, 3.71]b | 2.01 [1.06, 3.79]b |
Lmin tert 3 | 0.90 [0.37, 2.2] | |
Lmin tert 2 | 0.86 [0.38, 1.94] | |
Female | 1.78 [0.90, 3.52] | |
African American | 1.19 [0.60, 2.38] | |
Age | 1.02 [0.99, 1.05] | |
Noise Complaints | Model 1 OR [95% CI]d | Model 2 OR [95% CI]d |
High SSE | 0.57 [0.30, 1.12] | 0.49 [0.25, 0.96]b |
Lmin tert 3 | 0.85 [0.39, 1.84] | |
Lmin tert 2 | 0.91 [0.43, 1.93] | |
Female | 1.40 [0.71, 2.78] | |
African American | 0.35 [0.17, 0.70] | |
Age | 1.00 [0.96, 1.03] | |
Age2e | 1.00 [1.00, 1.00] |
Logistic regression clustered by subject demonstrated that patients with high SSE had 2 times higher odds of having a KSQI score above 3 (95% CI: 1.12, 3.71; P=0.020). This association was still significant after controlling for noise and patient demographics (OR: 2.01; 95% CI: 1.06, 3.79; P=0.032). After controlling for noise levels and patient demographics, there was a statistically significant association between high SSE and lower odds of noise complaints (OR: 0.49; 95% CI: 0.25, 0.96; P=0.039) (Table 2). Although demographic characteristics were not associated with high SSE, those patients with high SSE had lower odds of being in the loudest tertile rooms (OR: 0.34; 95% CI: 0.15, 0.74; P=0.007).
In multivariate linear regression analyses, there were no significant relationships between SLOC scores and KSQI, reported noise disruptiveness, and markers of sleep (sleep duration or sleep efficiency).
DISCUSSION
This study is the first to examine the relationship between perceived control, noise levels, and objective measurements of sleep in a hospital setting. One measure of perceived control, namely SSE, was associated with objective sleep duration, subjective and objective sleep quality, noise levels in patient rooms, and perhaps also patient complaints of noise. These associations remained significant after controlling for objective noise levels and patient demographics, suggesting that SSE is independently related to sleep.
In contrast to SSE, SLOC was not found to be significantly associated with either subjective or objective measures of sleep quality. The lack of association may be due to the fact that the SLOC questionnaire does not translate as well to the inpatient setting as the SSE questionnaire. The SLOC questionnaire focuses on general beliefs about sleep whereas the SSE questionnaire focuses on personal beliefs about one's own ability sleep in the immediate future, which may make it more relevant in the inpatient setting (see Supporting Information, Appendix 1 and 2, in the online version of this article).
Given our findings, it is important to identify why patients with high SSE have better sleep and fewer noise complaints. One possibility is that sleep self‐efficacy is an inherited trait unique to each person that is also predictive of a patient's sleep patterns. However, is it also possible that those patients with high SSE feel more empowered to take control of their environment, allowing them to advocate for better sleep? This hypothesis is further strengthened by the finding that those patients with high SSE on study entry were less likely to be in the noisiest rooms. This raises the possibility that at least 1 of the mechanisms by which high SSE may be protective against sleep loss is through patients taking an active role in noise reduction, such as closing the door or advocating for their sleep with staff. However, we did not directly observe or ask patients whether doors of patient rooms were open or closed or whether the patients took other measures to advocate for their own sleep. Thus, further work is necessary to understand the mechanisms by which sleep self‐efficacy may influence sleep.
One potential avenue for future research is to explore possible interventions for boosting sleep self‐efficacy in the hospital. Although most interventions have focused on environmental noise and staff‐based education, empowering patients through boosting SSE may be a helpful adjunct to improving hospital sleep.[33, 34] Currently, the SSE scale is not commonly used in the inpatient setting. Motivational interviewing and patient coaching could be explored as potential tools for boosting SSE. Furthermore, even if SSE is not easily changed, measuring SSE in patients newly admitted to the hospital may be useful in identifying patients most susceptible to sleep disruptions. Efforts to identify patients with low SSE should go hand‐in‐hand with measures to reduce noise. Addressing both patient‐level and environmental factors simultaneously may be the best strategy for improving sleep in an inpatient hospital setting.
In contrast to our prior study, it is worth noting that we did not find any significant relationships between overall noise levels and sleep.[9] In this dataset, nighttime noise is still a predictor of sleep loss in the hospital. However, when we restrict our sample to those who answered the SSE questionnaire and had nighttime noise recorded, we lose a significant number of observations. Because of our interest in testing the relationship between SSE and sleep, we chose to control for overall noise (which enabled us to retain more observations). We also did not find any interactions between SSE and noise in our regression models. Further work is warranted with larger sample sizes to better understand the role of SSE in the context of sleep and noise levels. In addition, females also received more sleep than males in our study.
There are several limitations to this study. This study was carried out at a single service at a single institution, limiting the ability to generalize the findings to other hospital settings. This study had a relatively high rate of patients who were unable to complete at least 1 night of data collection (42%), often due to watch removal for imaging or procedures, which may also affect the representativeness of our sample. Moreover, we can only examine associations and not causal relationships. The SSE scale has never been used in hospitalized patients, making comparisons between scores from hospitalized patients and population controls difficult. In addition, the SSE scale also has not been dichotomized in previous studies into high and low SSE. However, a sensitivity analysis with raw SSE scores did not change the results of our study. It can be difficult to perform actigraphy measurements in the hospital because many patients spend most of their time in bed. Because we chose a relatively healthy cohort of patients without significant limitations in mobility, actigraphy could still be used to differentiate time spent awake from time spent sleeping. Because we did not perform polysomnography, we cannot explore the role of sleep architecture which is an important component of sleep quality. Although the use of pharmacologic sleep aids is a potential confounding factor, the rate of use was very low in our cohort and unlikely to significantly affect our results. Continued study of this patient population is warranted to further develop the findings.
In conclusion, patients with high SSE sleep better in the hospital, tend to be in quieter rooms, and may report fewer noise complaints. Our findings suggest that a greater confidence in the ability to sleep may be beneficial in hospitalized adults. In addition to noise control, hospitals should also consider targeting patients with low SSE when designing novel interventions to improve in‐hospital sleep.
Disclosures
This work was supported by funding from the National Institute on Aging through a Short‐Term Aging‐Related Research Program (1 T35 AG029795), National Institute on Aging career development award (K23AG033763), a midcareer career development award (1K24AG031326), a program project (P01AG‐11412), an Agency for Healthcare Research and Quality Centers for Education and Research on Therapeutics grant (1U18HS016967), and a National Institute on Aging Clinical Translational Sciences award (UL1 RR024999). Dr. Arora had full access to all of the data in the study and takes responsibility for the integrity of the data and the accuracy of the statistical analysis. The funding agencies had no role in the design of the study; the collection, analysis, and interpretation of the data; or the decision to approve publication of the finished manuscript. The authors report no conflicts of interest.
- The metabolic consequences of sleep deprivation. Sleep Med Rev. 2007;11(3):163–178. , , , .
- Poor self‐reported sleep quality predicts mortality within one year of inpatient post‐acute rehabilitation among older adults. Sleep. 2011;34(12):1715–1721. , , , , , .
- The sleep of older people in hospital and nursing homes. J Clin Nurs. 1999;8:360–368. , , , et al.
- Sleep in hospitalized medical patients, part 1: factors affecting sleep. J Hosp Med. 2008; 3:473–482. , , et al.
- Nocturnal care interactions with patients in critical care units. Am J Crit Care. 2004;13:102–112; quiz 114–115. , , , et al.
- Patient perception of sleep quality and etiology of sleep disruption in the intensive care unit. Am J Respir Crit Care Med. 1999;159:1155–1162. , , .
- Sleep in acute care settings: an integrative review. J Nurs Scholarsh. 2000;32(1):31–38. .
- Sleep disruption due to hospital noises: a prospective evaluation. Ann Int Med. 2012;157(3): 170–179. , , , et al.
- Noise and sleep among adult medical inpatients: far from a quiet night. Arch Intern Med. 2012;172:68–70. , , , et al.
- Generalized expectancies for internal versus external control of reinforcement. Psychol Monogr. 1966;80:1–28. .
- Psychosocial risk factors and mortality: a prospective study with special focus on social support, social participation, and locus of control in Norway. J Epidemiol Community Health. 1998;52:476–481. , .
- The interactive effect of perceived control and functional status on health and mortality among young‐old and old‐old adults. J Gerontol B Psychol Sci Soc Sci. 1997;52:P118–P126. , .
- Role‐specific feelings of control and mortality. Psychol Aging. 2000;15:617–626. , .
- Patient empowerment in intensive care—an interview study. Intensive Crit Care Nurs. 2006;22:370–377. , , .
- Exploring the relationship between personal control and the hospital environment. J Clin Nurs. 2008;17:1601–1609. , , .
- Effects of volitional lifestyle on sleep‐life habits in the aged. Psychiatry Clin Neurosci. 1998;52:183–184. , , , et al.
- Sleep locus of control: report on a new scale. Behav Sleep Med. 2004;2:79–93. , , , et al.
- Effects of physician experience on costs and outcomes on an academic general medicine service: results of a trial of hospitalists. Ann Intern Med. 2002;137:866–874. , , , et al.
- The effects of age, sex, ethnicity, and sleep‐disordered breathing on sleep architecture. Arch Intern Med. 2004;164:406–418. , , , et al.
- Validation of a telephone version of the mini‐mental state examination. J Am Geriatr Soc. 1992;40:697–702. , , , et al.
- Contact isolation in surgical patients: a barrier to care? Surgery. 2003;134:180–188. , , , et al.
- Adverse effects of contact isolation. Lancet. 1999;354:1177–1178. , .
- Behavioral Treatment for Persistent Insomnia. Elmsford, NY: Pergamon Press; 1987. .
- A new method for measuring daytime sleepiness: the Epworth sleepiness scale. Sleep. 1991;14:540–545. .
- Reliability and factor analysis of the Epworth Sleepiness Scale. Sleep. 1992;15:376–381. .
- Objective components of individual differences in subjective sleep quality. J Sleep Res. 1997;6:217–220. , .
- The role of actigraphy in the study of sleep and circadian rhythms. Sleep. 2003;26:342–392. , , , et al.
- Practice parameters for the use of actigraphy in the assessment of sleep and sleep disorders: an update for 2007. Sleep. 2007;30:519–529. , , , et al.
- The role of actigraphy in the evaluation of sleep disorders. Sleep. 1995;18:288–302. , , , et al.
- Clinical review: sleep measurement in critical care patients: research and clinical implications. Crit Care. 2007;11:226. , , , et al.
- Evaluation of immobility time for sleep latency in actigraphy. Sleep Med. 2009;10:621–625. , , , et al.
- The subjective meaning of sleep quality: a comparison of individuals with and without insomnia. Sleep. 2008;31:383–393. , , , et al.
- Sleep in hospitalized medical patients, part 2: behavioral and pharmacological management of sleep disturbances. J Hosp Med. 2009;4:50–59. , , , et al.
- A nonpharmacologic sleep protocol for hospitalized older patients. J Am Geriatr Soc. 1998;46(6):700–705. , , , .
Lack of sleep is a common problem in hospitalized patients and is associated with poorer health outcomes, especially in older patients.[1, 2, 3] Prior studies highlight a multitude of factors that can result in sleep loss in the hospital[3, 4, 5, 6] with 1 of the most common causes of sleep disruption in the hospital being noise.[7, 8, 9]
In addition to external factors, such as hospital noise, there may be inherent characteristics that predispose certain patients to greater sleep loss when hospitalized. One such measure is the construct of perceived control or the psychological measure of how much individuals expect themselves to be capable of bringing about desired outcomes.[10] Among older patients, low perceived control is associated with increased rates of physician visits, hospitalizations, and death.[11, 12] In contrast, patients who feel more in control of their environment may experience positive health benefits.[13]
Yet, when patients are placed in a hospital setting, they experience a significant reduction in control over their environment along with an increase in dependency on medical staff and therapies.[14, 15] For example, hospitalized patients are restricted in their personal decisions, such as what clothes they can wear and what they can eat and are not in charge of their own schedules, including their sleep time.
Although prior studies suggest that perceived control over sleep is related to actual sleep among community‐dwelling adults,[16, 17] no study has examined this relationship in hospitalized adults. Therefore, the aim of our study was to examine the possible association between perceived control, noise levels, and sleep in hospitalized middle‐aged and older patients.
METHODS
Study Design
We conducted a prospective cohort study of subjects recruited from a large ongoing study of admitted patients at the University of Chicago inpatient general medicine service.[18] Because we were interested in middle‐aged and older adults who are most sensitive to sleep disruptions, patients who were age 50 years and over, ambulatory, and living in the community were eligible for the study.[19] Exclusion criteria were cognitive impairment (telephone version of the Mini‐Mental State Exam <17 out of 22), preexisting sleeping disorders identified via patient charts, such as obstructive sleep apnea and narcolepsy, transfer from the intensive care unit (ICU), and admission to the hospital more than 72 hours prior to enrollment.[20] These inclusion and exclusion criteria were selected to identify a patient population with minimal sleep disturbances at baseline. Patients under isolation were excluded because they are not visited as frequently by the healthcare team.[21, 22] Most general medicine rooms were double occupancy but efforts were made to make patient rooms single when possible or required (ie, isolation for infection control). The study was approved by the University of Chicago Institutional Review Board.
Subjective Data Collection
Baseline levels of perceived control over sleep, or the amount of control patients believe they have over their sleep, were assessed using 2 different scales. The first tool was the 8‐item Sleep Locus of Control (SLOC) scale,[17] which ranges from 8 to 48, with higher values corresponding to a greater internal locus of control over sleep. An internal sleep locus of control indicates beliefs that patients feel that they are primarily responsible for their own sleep as opposed to an external locus of control which indicates beliefs that good sleep is due to luck or chance. For example, patients were asked how strongly they agree or disagree with statements, such as, If I take care of myself, I can avoid insomnia and People who never get insomnia are just plain lucky (see Supporting Information, Appendix 2, in the online version of this article). The second tool was the 9‐item Sleep Self‐Efficacy (SSE) scale,[23] which ranges from 9 to 45, with higher values corresponding to greater confidence patients have in their ability to sleep. One of the items asks, How confident are you that you can lie in bed feeling physically relaxed (see Supporting Information, Appendix 1, in the online version of this article)? Both instruments have been validated in an outpatient setting.[23] These surveys were given immediately on enrollment in the study to measure baseline perceived control.
Baseline sleep habits were also collected on enrollment using the Epworth Sleepiness Scale,[24, 25] a standard validated survey that assesses excess daytime sleepiness in various common situations. For each day in the hospital, patients were asked to report in‐hospital sleep quality using the Karolinska Sleep Log.[26] The Karolinska Sleep Quality Index (KSQI) is calculated from 4 items on the Karolinska Sleep Log (sleep quality, sleep restlessness, slept throughout the night, ease of falling asleep). The questions are on a 5‐point scale and the 4 items are averaged for a final score out of 5 with a higher number indicating better subjective sleep quality. The item How much was your sleep disturbed by noise? on the Karolinska Sleep Log was used to assess the degree to which noise was a disruptor of sleep. This question was also on a 5‐point scale with higher scores indicating greater disruptiveness of noise. Patients were also asked how disruptive noise from roommates was on a nightly basis using this same scale.
Objective Data Collection
Wrist activity monitors (Actiwatch 2; Respironics, Inc., Murrysville, PA)[27, 28, 29, 30] were used to measure patient sleep. Actiware 5 software (Respironics, Inc.)[31] was used to estimate quantitative measures of sleep time and efficiency. Sleep time is defined as the total duration of time spent sleeping at night and sleep efficiency is defined as the fraction of time, reported as a percentage, spent sleeping by actigraphy out of the total time patients reported they were sleeping.
Sound levels in patient rooms were recorded using Larson Davis 720 Sound Level Monitors (Larson Davis, Inc., Provo, UT). These monitors store functional average sound pressure levels in A‐weighted decibels called the Leq over 1‐hour intervals. The Leq is the average sound level over the given time interval. Minimum (Lmin) and maximum (Lmax) sound levels are also stored. The LD SLM Utility Program (Larson Davis, Inc.) was used to extract the sound level measurements recorded by the monitors.
Demographic information (age, gender, race, ethnicity, highest level of education, length of stay in the hospital, and comorbidities) was obtained from hospital charts via an ongoing study of admitted patients at the University of Chicago Medical Center inpatient general medicine service.[18] Chart audits were performed to determine whether patients received pharmacologic sleep aids in the hospital.
Data Analysis
Descriptive statistics were used to summarize mean sleep duration and sleep efficiency in the hospital as well as SLOC and SSE. Because the SSE scores were not normally distributed, the scores were dichotomized at the median to create a variable denoting high and low SSE. Additionally, because the distribution of responses to the noise disruption question was skewed to the right, reports of noise disruptions were grouped into not disruptive (score=1) and disruptive (score>1).
Two‐sample t tests with equal variances were used to assess the relationship between perceived control measures (high/low SLOC, SSE) and objective sleep measures (sleep time, sleep efficiency). Multivariate linear regression was used to test the association between high SSE (independent variable) and sleep time (dependent variable), clustering for multiple nights of data within the subject. Multivariate logistic regression, also adjusting for subject, was used to test the association between high SSE and noise disruptiveness and the association between high SSE and Karolinska scores. Leq, Lmax, and Lmin were all tested using stepwise forward regression. Because our prior work[9] demonstrated that noise levels separated into tertiles were significantly associated with sleep time, our analysis also used noise levels separated into tertiles. Stepwise forward regression was used to add basic patient demographics (gender, race, age) to the models. Statistical significance was defined as P<0.05, and all statistical analysis was done using Stata 11.0 (StataCorp, College Station, TX).
RESULTS
From April 2010 to May 2012, 1134 patients were screened by study personnel for this study via an ongoing study of hospitalized patients on the inpatient general medicine ward. Of the 361 (31.8%) eligible patients, 206 (57.1%) consented to participate. Of the subjects enrolled in the study, 118 were able to complete at least 1 night of actigraphy, sound monitoring, and subjective assessment for a total of 185 patient nights (Figure 1).
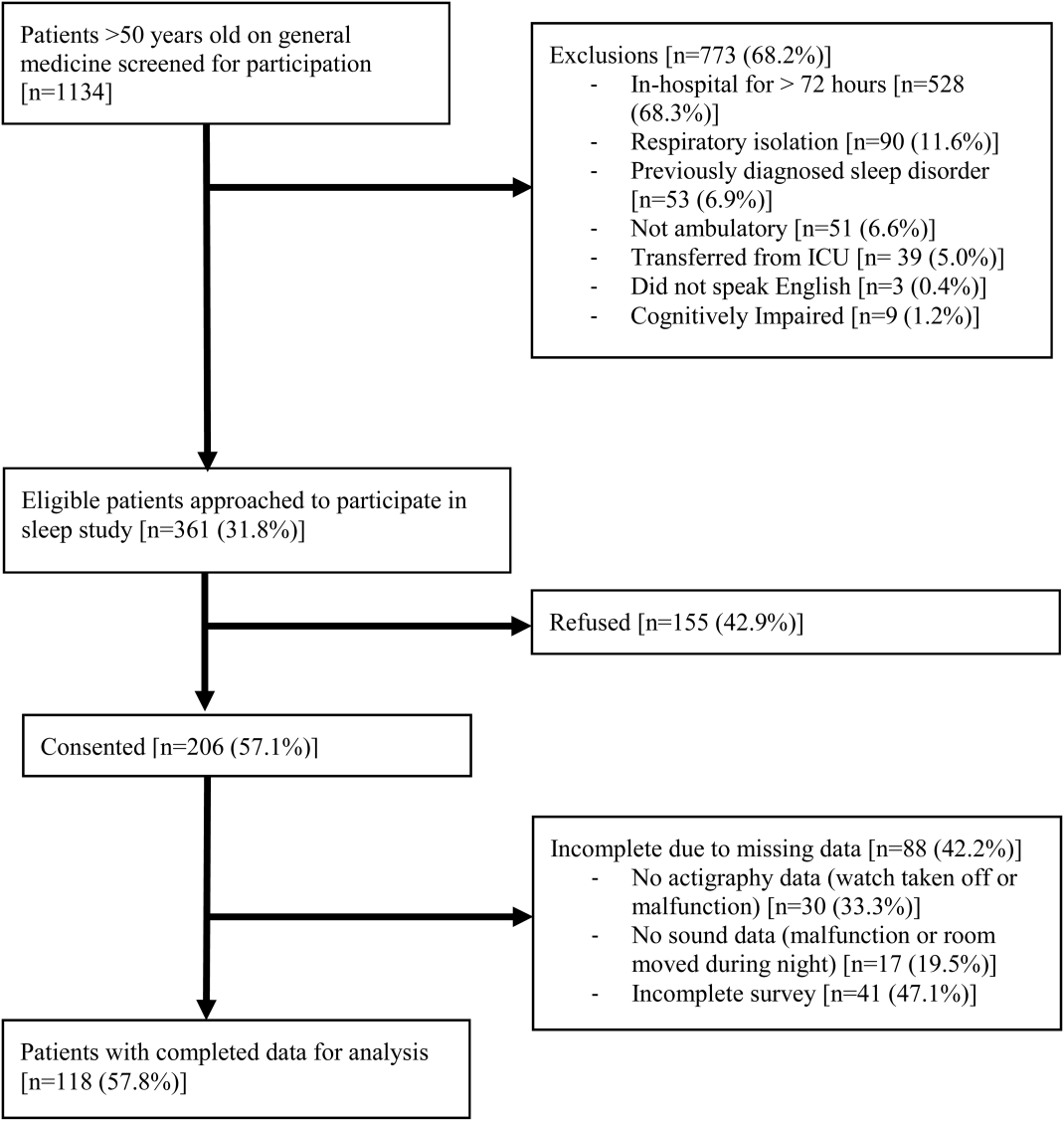
The majority of patients were female (57%), African American (67%), and non‐Hispanic (97%). The mean age was 65 years (standard deviation [SD], 11.6 years), and the median length of stay was 4 days (interquartile range [IQR], 36). The majority of patients also had hypertension (67%), with chronic obstructive pulmonary disease [COPD] (31%) and congestive heart failure (31%) being the next most common comorbidities. About two‐thirds of subjects (64%) were characterized as average or above average sleepers with Epworth Sleepiness Scale scores 9[20] (Table 1). Only 5% of patients received pharmacological sleep aids.
Value, n (%)a | |
---|---|
| |
Patient characteristics | |
Age, mean (SD), y | 63 (12) |
Length of stay, median (IQR), db | 4 (36) |
Female | 67 (57) |
African American | 79 (67) |
Hispanic | 3 (3) |
High school graduate | 92 (78) |
Comorbidities | |
Hypertension | 79 (66) |
Chronic obstructive pulmonary disease | 37 (31) |
Congestive heart failure | 37 (31) |
Diabetes | 36 (30) |
End stage renal disease | 23 (19) |
Baseline sleep characteristics | |
Sleep duration, mean (SD), minc | 333 (128) |
Epworth Sleepiness Scale, score 9d | 73 (64) |
The mean baseline SLOC score was 30.4 (SD, 6.7), with a median of 31 (IQR, 2735). The mean baseline SSE score was 32.1 (SD, 9.4), with a median of 34 (IQR, 2441). Fifty‐four patients were categorized as having high sleep self‐efficacy (high SSE), which we defined as scoring above the median of 34.
Average in‐hospital sleep was 5.5 hours (333 minutes; SD, 128 minutes) which was significantly shorter than the self‐reported sleep duration of 6.5 hours prior to admission (387 minutes, SD, 125 minutes; P=0.0001). The mean sleep efficiency was 73% (SD, 19%) with 55% of actigraphy nights below the normal range of 80% efficiency for adults.[19] Median KSQI was 3.5 (IQR, 2.254.75), with 41% of the patients with a KSQI 3, putting them in the insomniac range.[32] The median score on the noise disruptiveness question was 1 (IQR, 14) with 42% of reports coded as disruptive defined as a score >1 on the 5‐point scale. The median score on the roommate disruptiveness question was 1 (IQR, 11) with 77% of responses coded as not disruptive defined as a score of 1 on the 5‐point scale.
A 2‐sample t test with equal variances showed that those patients reporting high SSE were more likely to sleep longer in the hospital than those reporting low SSE (364 minutes 95% confidence interval [CI]: 340, 388 vs 309 minutes 95% CI: 283, 336; P=0.003) (Figure 2). Patients with high SSE were also more likely to have a normal sleep efficiency (above 80%) compared to those with low SSE (54% 95% CI: 43, 65 vs 38% 95% CI: 28,47; P=0.028). Last, there was a trend toward patients reporting higher SSE to also report less noise disruption compared to those patients with low SSE ([42%] 95% CI: 31, 53 vs [56%] 95% CI: 46, 65; P=0.063) (Figure 3).
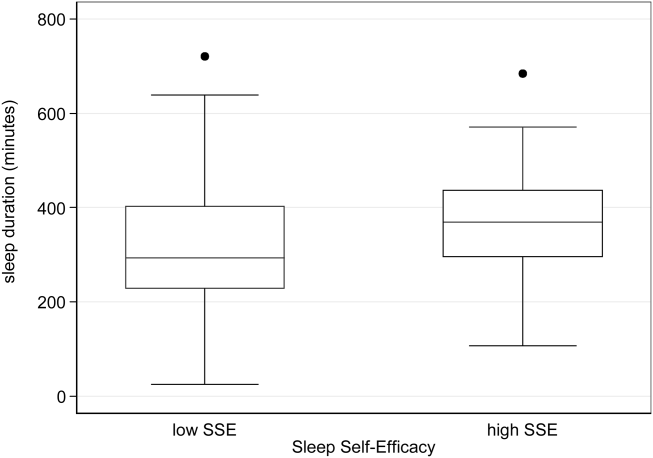
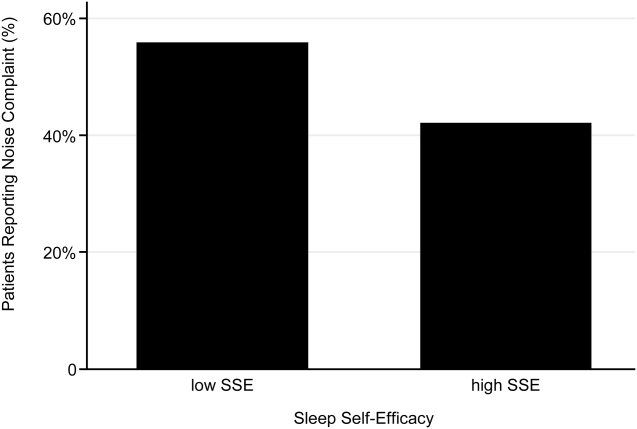
Linear regression clustered by subject showed that high SSE was associated with longer sleep duration (55 minutes 95% CI: 14, 97; P=0.010). Furthermore, high SSE was significantly associated with longer sleep duration after controlling for both objective noise level and patient demographics in the model using stepwise forward regression (50 minutes 95% CI: 11, 90; P=0.014) (Table 2).
Sleep Duration (min) | Model 1 Beta [95% CI]a | Model 2 Beta [95% CI]a |
---|---|---|
| ||
High SSE | 55 [14, 97]b | 50 [11, 90]b |
Lmin tert 3 | 14 [59, 29] | |
Lmin tert 2 | 21 [65, 23] | |
Female | 49 [10, 89]b | |
African American | 16 [59, 27] | |
Age | 1 [0.9, 3] | |
Karolinska Sleep Quality | Model 1 OR [95% CI]c | Model 2 OR [95% CI]c |
High SSE | 2.04 [1.12, 3.71]b | 2.01 [1.06, 3.79]b |
Lmin tert 3 | 0.90 [0.37, 2.2] | |
Lmin tert 2 | 0.86 [0.38, 1.94] | |
Female | 1.78 [0.90, 3.52] | |
African American | 1.19 [0.60, 2.38] | |
Age | 1.02 [0.99, 1.05] | |
Noise Complaints | Model 1 OR [95% CI]d | Model 2 OR [95% CI]d |
High SSE | 0.57 [0.30, 1.12] | 0.49 [0.25, 0.96]b |
Lmin tert 3 | 0.85 [0.39, 1.84] | |
Lmin tert 2 | 0.91 [0.43, 1.93] | |
Female | 1.40 [0.71, 2.78] | |
African American | 0.35 [0.17, 0.70] | |
Age | 1.00 [0.96, 1.03] | |
Age2e | 1.00 [1.00, 1.00] |
Logistic regression clustered by subject demonstrated that patients with high SSE had 2 times higher odds of having a KSQI score above 3 (95% CI: 1.12, 3.71; P=0.020). This association was still significant after controlling for noise and patient demographics (OR: 2.01; 95% CI: 1.06, 3.79; P=0.032). After controlling for noise levels and patient demographics, there was a statistically significant association between high SSE and lower odds of noise complaints (OR: 0.49; 95% CI: 0.25, 0.96; P=0.039) (Table 2). Although demographic characteristics were not associated with high SSE, those patients with high SSE had lower odds of being in the loudest tertile rooms (OR: 0.34; 95% CI: 0.15, 0.74; P=0.007).
In multivariate linear regression analyses, there were no significant relationships between SLOC scores and KSQI, reported noise disruptiveness, and markers of sleep (sleep duration or sleep efficiency).
DISCUSSION
This study is the first to examine the relationship between perceived control, noise levels, and objective measurements of sleep in a hospital setting. One measure of perceived control, namely SSE, was associated with objective sleep duration, subjective and objective sleep quality, noise levels in patient rooms, and perhaps also patient complaints of noise. These associations remained significant after controlling for objective noise levels and patient demographics, suggesting that SSE is independently related to sleep.
In contrast to SSE, SLOC was not found to be significantly associated with either subjective or objective measures of sleep quality. The lack of association may be due to the fact that the SLOC questionnaire does not translate as well to the inpatient setting as the SSE questionnaire. The SLOC questionnaire focuses on general beliefs about sleep whereas the SSE questionnaire focuses on personal beliefs about one's own ability sleep in the immediate future, which may make it more relevant in the inpatient setting (see Supporting Information, Appendix 1 and 2, in the online version of this article).
Given our findings, it is important to identify why patients with high SSE have better sleep and fewer noise complaints. One possibility is that sleep self‐efficacy is an inherited trait unique to each person that is also predictive of a patient's sleep patterns. However, is it also possible that those patients with high SSE feel more empowered to take control of their environment, allowing them to advocate for better sleep? This hypothesis is further strengthened by the finding that those patients with high SSE on study entry were less likely to be in the noisiest rooms. This raises the possibility that at least 1 of the mechanisms by which high SSE may be protective against sleep loss is through patients taking an active role in noise reduction, such as closing the door or advocating for their sleep with staff. However, we did not directly observe or ask patients whether doors of patient rooms were open or closed or whether the patients took other measures to advocate for their own sleep. Thus, further work is necessary to understand the mechanisms by which sleep self‐efficacy may influence sleep.
One potential avenue for future research is to explore possible interventions for boosting sleep self‐efficacy in the hospital. Although most interventions have focused on environmental noise and staff‐based education, empowering patients through boosting SSE may be a helpful adjunct to improving hospital sleep.[33, 34] Currently, the SSE scale is not commonly used in the inpatient setting. Motivational interviewing and patient coaching could be explored as potential tools for boosting SSE. Furthermore, even if SSE is not easily changed, measuring SSE in patients newly admitted to the hospital may be useful in identifying patients most susceptible to sleep disruptions. Efforts to identify patients with low SSE should go hand‐in‐hand with measures to reduce noise. Addressing both patient‐level and environmental factors simultaneously may be the best strategy for improving sleep in an inpatient hospital setting.
In contrast to our prior study, it is worth noting that we did not find any significant relationships between overall noise levels and sleep.[9] In this dataset, nighttime noise is still a predictor of sleep loss in the hospital. However, when we restrict our sample to those who answered the SSE questionnaire and had nighttime noise recorded, we lose a significant number of observations. Because of our interest in testing the relationship between SSE and sleep, we chose to control for overall noise (which enabled us to retain more observations). We also did not find any interactions between SSE and noise in our regression models. Further work is warranted with larger sample sizes to better understand the role of SSE in the context of sleep and noise levels. In addition, females also received more sleep than males in our study.
There are several limitations to this study. This study was carried out at a single service at a single institution, limiting the ability to generalize the findings to other hospital settings. This study had a relatively high rate of patients who were unable to complete at least 1 night of data collection (42%), often due to watch removal for imaging or procedures, which may also affect the representativeness of our sample. Moreover, we can only examine associations and not causal relationships. The SSE scale has never been used in hospitalized patients, making comparisons between scores from hospitalized patients and population controls difficult. In addition, the SSE scale also has not been dichotomized in previous studies into high and low SSE. However, a sensitivity analysis with raw SSE scores did not change the results of our study. It can be difficult to perform actigraphy measurements in the hospital because many patients spend most of their time in bed. Because we chose a relatively healthy cohort of patients without significant limitations in mobility, actigraphy could still be used to differentiate time spent awake from time spent sleeping. Because we did not perform polysomnography, we cannot explore the role of sleep architecture which is an important component of sleep quality. Although the use of pharmacologic sleep aids is a potential confounding factor, the rate of use was very low in our cohort and unlikely to significantly affect our results. Continued study of this patient population is warranted to further develop the findings.
In conclusion, patients with high SSE sleep better in the hospital, tend to be in quieter rooms, and may report fewer noise complaints. Our findings suggest that a greater confidence in the ability to sleep may be beneficial in hospitalized adults. In addition to noise control, hospitals should also consider targeting patients with low SSE when designing novel interventions to improve in‐hospital sleep.
Disclosures
This work was supported by funding from the National Institute on Aging through a Short‐Term Aging‐Related Research Program (1 T35 AG029795), National Institute on Aging career development award (K23AG033763), a midcareer career development award (1K24AG031326), a program project (P01AG‐11412), an Agency for Healthcare Research and Quality Centers for Education and Research on Therapeutics grant (1U18HS016967), and a National Institute on Aging Clinical Translational Sciences award (UL1 RR024999). Dr. Arora had full access to all of the data in the study and takes responsibility for the integrity of the data and the accuracy of the statistical analysis. The funding agencies had no role in the design of the study; the collection, analysis, and interpretation of the data; or the decision to approve publication of the finished manuscript. The authors report no conflicts of interest.
Lack of sleep is a common problem in hospitalized patients and is associated with poorer health outcomes, especially in older patients.[1, 2, 3] Prior studies highlight a multitude of factors that can result in sleep loss in the hospital[3, 4, 5, 6] with 1 of the most common causes of sleep disruption in the hospital being noise.[7, 8, 9]
In addition to external factors, such as hospital noise, there may be inherent characteristics that predispose certain patients to greater sleep loss when hospitalized. One such measure is the construct of perceived control or the psychological measure of how much individuals expect themselves to be capable of bringing about desired outcomes.[10] Among older patients, low perceived control is associated with increased rates of physician visits, hospitalizations, and death.[11, 12] In contrast, patients who feel more in control of their environment may experience positive health benefits.[13]
Yet, when patients are placed in a hospital setting, they experience a significant reduction in control over their environment along with an increase in dependency on medical staff and therapies.[14, 15] For example, hospitalized patients are restricted in their personal decisions, such as what clothes they can wear and what they can eat and are not in charge of their own schedules, including their sleep time.
Although prior studies suggest that perceived control over sleep is related to actual sleep among community‐dwelling adults,[16, 17] no study has examined this relationship in hospitalized adults. Therefore, the aim of our study was to examine the possible association between perceived control, noise levels, and sleep in hospitalized middle‐aged and older patients.
METHODS
Study Design
We conducted a prospective cohort study of subjects recruited from a large ongoing study of admitted patients at the University of Chicago inpatient general medicine service.[18] Because we were interested in middle‐aged and older adults who are most sensitive to sleep disruptions, patients who were age 50 years and over, ambulatory, and living in the community were eligible for the study.[19] Exclusion criteria were cognitive impairment (telephone version of the Mini‐Mental State Exam <17 out of 22), preexisting sleeping disorders identified via patient charts, such as obstructive sleep apnea and narcolepsy, transfer from the intensive care unit (ICU), and admission to the hospital more than 72 hours prior to enrollment.[20] These inclusion and exclusion criteria were selected to identify a patient population with minimal sleep disturbances at baseline. Patients under isolation were excluded because they are not visited as frequently by the healthcare team.[21, 22] Most general medicine rooms were double occupancy but efforts were made to make patient rooms single when possible or required (ie, isolation for infection control). The study was approved by the University of Chicago Institutional Review Board.
Subjective Data Collection
Baseline levels of perceived control over sleep, or the amount of control patients believe they have over their sleep, were assessed using 2 different scales. The first tool was the 8‐item Sleep Locus of Control (SLOC) scale,[17] which ranges from 8 to 48, with higher values corresponding to a greater internal locus of control over sleep. An internal sleep locus of control indicates beliefs that patients feel that they are primarily responsible for their own sleep as opposed to an external locus of control which indicates beliefs that good sleep is due to luck or chance. For example, patients were asked how strongly they agree or disagree with statements, such as, If I take care of myself, I can avoid insomnia and People who never get insomnia are just plain lucky (see Supporting Information, Appendix 2, in the online version of this article). The second tool was the 9‐item Sleep Self‐Efficacy (SSE) scale,[23] which ranges from 9 to 45, with higher values corresponding to greater confidence patients have in their ability to sleep. One of the items asks, How confident are you that you can lie in bed feeling physically relaxed (see Supporting Information, Appendix 1, in the online version of this article)? Both instruments have been validated in an outpatient setting.[23] These surveys were given immediately on enrollment in the study to measure baseline perceived control.
Baseline sleep habits were also collected on enrollment using the Epworth Sleepiness Scale,[24, 25] a standard validated survey that assesses excess daytime sleepiness in various common situations. For each day in the hospital, patients were asked to report in‐hospital sleep quality using the Karolinska Sleep Log.[26] The Karolinska Sleep Quality Index (KSQI) is calculated from 4 items on the Karolinska Sleep Log (sleep quality, sleep restlessness, slept throughout the night, ease of falling asleep). The questions are on a 5‐point scale and the 4 items are averaged for a final score out of 5 with a higher number indicating better subjective sleep quality. The item How much was your sleep disturbed by noise? on the Karolinska Sleep Log was used to assess the degree to which noise was a disruptor of sleep. This question was also on a 5‐point scale with higher scores indicating greater disruptiveness of noise. Patients were also asked how disruptive noise from roommates was on a nightly basis using this same scale.
Objective Data Collection
Wrist activity monitors (Actiwatch 2; Respironics, Inc., Murrysville, PA)[27, 28, 29, 30] were used to measure patient sleep. Actiware 5 software (Respironics, Inc.)[31] was used to estimate quantitative measures of sleep time and efficiency. Sleep time is defined as the total duration of time spent sleeping at night and sleep efficiency is defined as the fraction of time, reported as a percentage, spent sleeping by actigraphy out of the total time patients reported they were sleeping.
Sound levels in patient rooms were recorded using Larson Davis 720 Sound Level Monitors (Larson Davis, Inc., Provo, UT). These monitors store functional average sound pressure levels in A‐weighted decibels called the Leq over 1‐hour intervals. The Leq is the average sound level over the given time interval. Minimum (Lmin) and maximum (Lmax) sound levels are also stored. The LD SLM Utility Program (Larson Davis, Inc.) was used to extract the sound level measurements recorded by the monitors.
Demographic information (age, gender, race, ethnicity, highest level of education, length of stay in the hospital, and comorbidities) was obtained from hospital charts via an ongoing study of admitted patients at the University of Chicago Medical Center inpatient general medicine service.[18] Chart audits were performed to determine whether patients received pharmacologic sleep aids in the hospital.
Data Analysis
Descriptive statistics were used to summarize mean sleep duration and sleep efficiency in the hospital as well as SLOC and SSE. Because the SSE scores were not normally distributed, the scores were dichotomized at the median to create a variable denoting high and low SSE. Additionally, because the distribution of responses to the noise disruption question was skewed to the right, reports of noise disruptions were grouped into not disruptive (score=1) and disruptive (score>1).
Two‐sample t tests with equal variances were used to assess the relationship between perceived control measures (high/low SLOC, SSE) and objective sleep measures (sleep time, sleep efficiency). Multivariate linear regression was used to test the association between high SSE (independent variable) and sleep time (dependent variable), clustering for multiple nights of data within the subject. Multivariate logistic regression, also adjusting for subject, was used to test the association between high SSE and noise disruptiveness and the association between high SSE and Karolinska scores. Leq, Lmax, and Lmin were all tested using stepwise forward regression. Because our prior work[9] demonstrated that noise levels separated into tertiles were significantly associated with sleep time, our analysis also used noise levels separated into tertiles. Stepwise forward regression was used to add basic patient demographics (gender, race, age) to the models. Statistical significance was defined as P<0.05, and all statistical analysis was done using Stata 11.0 (StataCorp, College Station, TX).
RESULTS
From April 2010 to May 2012, 1134 patients were screened by study personnel for this study via an ongoing study of hospitalized patients on the inpatient general medicine ward. Of the 361 (31.8%) eligible patients, 206 (57.1%) consented to participate. Of the subjects enrolled in the study, 118 were able to complete at least 1 night of actigraphy, sound monitoring, and subjective assessment for a total of 185 patient nights (Figure 1).
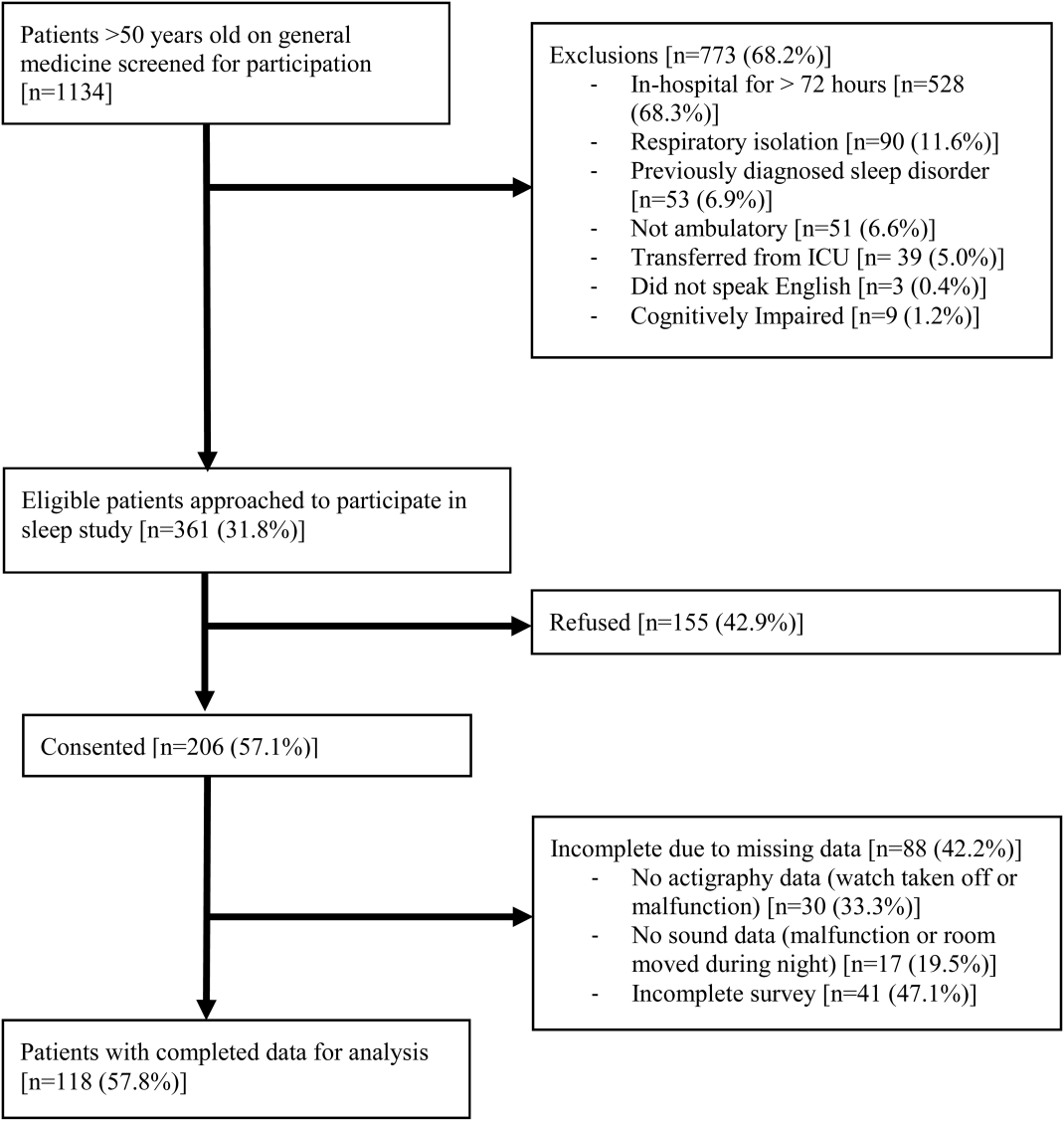
The majority of patients were female (57%), African American (67%), and non‐Hispanic (97%). The mean age was 65 years (standard deviation [SD], 11.6 years), and the median length of stay was 4 days (interquartile range [IQR], 36). The majority of patients also had hypertension (67%), with chronic obstructive pulmonary disease [COPD] (31%) and congestive heart failure (31%) being the next most common comorbidities. About two‐thirds of subjects (64%) were characterized as average or above average sleepers with Epworth Sleepiness Scale scores 9[20] (Table 1). Only 5% of patients received pharmacological sleep aids.
Value, n (%)a | |
---|---|
| |
Patient characteristics | |
Age, mean (SD), y | 63 (12) |
Length of stay, median (IQR), db | 4 (36) |
Female | 67 (57) |
African American | 79 (67) |
Hispanic | 3 (3) |
High school graduate | 92 (78) |
Comorbidities | |
Hypertension | 79 (66) |
Chronic obstructive pulmonary disease | 37 (31) |
Congestive heart failure | 37 (31) |
Diabetes | 36 (30) |
End stage renal disease | 23 (19) |
Baseline sleep characteristics | |
Sleep duration, mean (SD), minc | 333 (128) |
Epworth Sleepiness Scale, score 9d | 73 (64) |
The mean baseline SLOC score was 30.4 (SD, 6.7), with a median of 31 (IQR, 2735). The mean baseline SSE score was 32.1 (SD, 9.4), with a median of 34 (IQR, 2441). Fifty‐four patients were categorized as having high sleep self‐efficacy (high SSE), which we defined as scoring above the median of 34.
Average in‐hospital sleep was 5.5 hours (333 minutes; SD, 128 minutes) which was significantly shorter than the self‐reported sleep duration of 6.5 hours prior to admission (387 minutes, SD, 125 minutes; P=0.0001). The mean sleep efficiency was 73% (SD, 19%) with 55% of actigraphy nights below the normal range of 80% efficiency for adults.[19] Median KSQI was 3.5 (IQR, 2.254.75), with 41% of the patients with a KSQI 3, putting them in the insomniac range.[32] The median score on the noise disruptiveness question was 1 (IQR, 14) with 42% of reports coded as disruptive defined as a score >1 on the 5‐point scale. The median score on the roommate disruptiveness question was 1 (IQR, 11) with 77% of responses coded as not disruptive defined as a score of 1 on the 5‐point scale.
A 2‐sample t test with equal variances showed that those patients reporting high SSE were more likely to sleep longer in the hospital than those reporting low SSE (364 minutes 95% confidence interval [CI]: 340, 388 vs 309 minutes 95% CI: 283, 336; P=0.003) (Figure 2). Patients with high SSE were also more likely to have a normal sleep efficiency (above 80%) compared to those with low SSE (54% 95% CI: 43, 65 vs 38% 95% CI: 28,47; P=0.028). Last, there was a trend toward patients reporting higher SSE to also report less noise disruption compared to those patients with low SSE ([42%] 95% CI: 31, 53 vs [56%] 95% CI: 46, 65; P=0.063) (Figure 3).
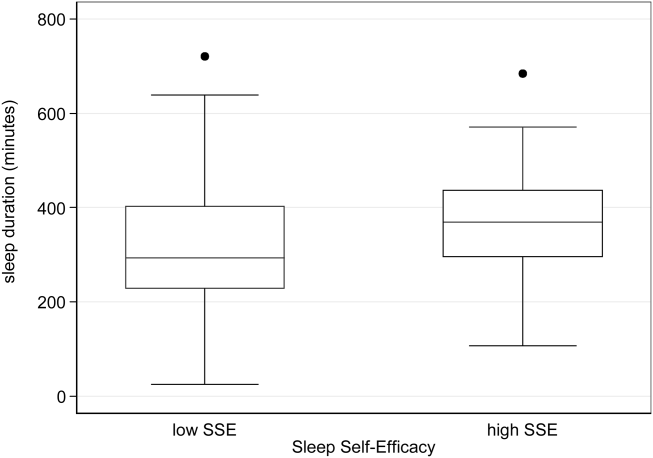
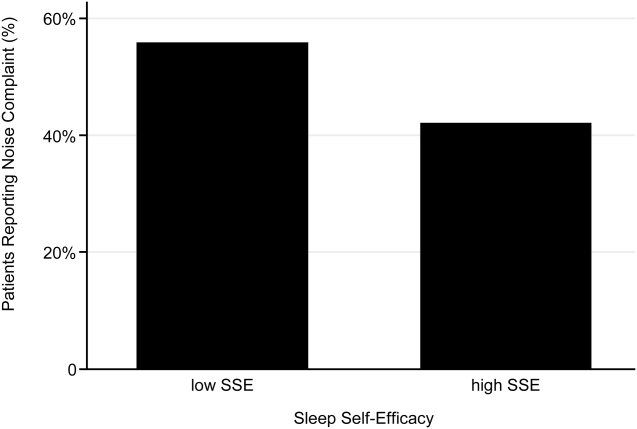
Linear regression clustered by subject showed that high SSE was associated with longer sleep duration (55 minutes 95% CI: 14, 97; P=0.010). Furthermore, high SSE was significantly associated with longer sleep duration after controlling for both objective noise level and patient demographics in the model using stepwise forward regression (50 minutes 95% CI: 11, 90; P=0.014) (Table 2).
Sleep Duration (min) | Model 1 Beta [95% CI]a | Model 2 Beta [95% CI]a |
---|---|---|
| ||
High SSE | 55 [14, 97]b | 50 [11, 90]b |
Lmin tert 3 | 14 [59, 29] | |
Lmin tert 2 | 21 [65, 23] | |
Female | 49 [10, 89]b | |
African American | 16 [59, 27] | |
Age | 1 [0.9, 3] | |
Karolinska Sleep Quality | Model 1 OR [95% CI]c | Model 2 OR [95% CI]c |
High SSE | 2.04 [1.12, 3.71]b | 2.01 [1.06, 3.79]b |
Lmin tert 3 | 0.90 [0.37, 2.2] | |
Lmin tert 2 | 0.86 [0.38, 1.94] | |
Female | 1.78 [0.90, 3.52] | |
African American | 1.19 [0.60, 2.38] | |
Age | 1.02 [0.99, 1.05] | |
Noise Complaints | Model 1 OR [95% CI]d | Model 2 OR [95% CI]d |
High SSE | 0.57 [0.30, 1.12] | 0.49 [0.25, 0.96]b |
Lmin tert 3 | 0.85 [0.39, 1.84] | |
Lmin tert 2 | 0.91 [0.43, 1.93] | |
Female | 1.40 [0.71, 2.78] | |
African American | 0.35 [0.17, 0.70] | |
Age | 1.00 [0.96, 1.03] | |
Age2e | 1.00 [1.00, 1.00] |
Logistic regression clustered by subject demonstrated that patients with high SSE had 2 times higher odds of having a KSQI score above 3 (95% CI: 1.12, 3.71; P=0.020). This association was still significant after controlling for noise and patient demographics (OR: 2.01; 95% CI: 1.06, 3.79; P=0.032). After controlling for noise levels and patient demographics, there was a statistically significant association between high SSE and lower odds of noise complaints (OR: 0.49; 95% CI: 0.25, 0.96; P=0.039) (Table 2). Although demographic characteristics were not associated with high SSE, those patients with high SSE had lower odds of being in the loudest tertile rooms (OR: 0.34; 95% CI: 0.15, 0.74; P=0.007).
In multivariate linear regression analyses, there were no significant relationships between SLOC scores and KSQI, reported noise disruptiveness, and markers of sleep (sleep duration or sleep efficiency).
DISCUSSION
This study is the first to examine the relationship between perceived control, noise levels, and objective measurements of sleep in a hospital setting. One measure of perceived control, namely SSE, was associated with objective sleep duration, subjective and objective sleep quality, noise levels in patient rooms, and perhaps also patient complaints of noise. These associations remained significant after controlling for objective noise levels and patient demographics, suggesting that SSE is independently related to sleep.
In contrast to SSE, SLOC was not found to be significantly associated with either subjective or objective measures of sleep quality. The lack of association may be due to the fact that the SLOC questionnaire does not translate as well to the inpatient setting as the SSE questionnaire. The SLOC questionnaire focuses on general beliefs about sleep whereas the SSE questionnaire focuses on personal beliefs about one's own ability sleep in the immediate future, which may make it more relevant in the inpatient setting (see Supporting Information, Appendix 1 and 2, in the online version of this article).
Given our findings, it is important to identify why patients with high SSE have better sleep and fewer noise complaints. One possibility is that sleep self‐efficacy is an inherited trait unique to each person that is also predictive of a patient's sleep patterns. However, is it also possible that those patients with high SSE feel more empowered to take control of their environment, allowing them to advocate for better sleep? This hypothesis is further strengthened by the finding that those patients with high SSE on study entry were less likely to be in the noisiest rooms. This raises the possibility that at least 1 of the mechanisms by which high SSE may be protective against sleep loss is through patients taking an active role in noise reduction, such as closing the door or advocating for their sleep with staff. However, we did not directly observe or ask patients whether doors of patient rooms were open or closed or whether the patients took other measures to advocate for their own sleep. Thus, further work is necessary to understand the mechanisms by which sleep self‐efficacy may influence sleep.
One potential avenue for future research is to explore possible interventions for boosting sleep self‐efficacy in the hospital. Although most interventions have focused on environmental noise and staff‐based education, empowering patients through boosting SSE may be a helpful adjunct to improving hospital sleep.[33, 34] Currently, the SSE scale is not commonly used in the inpatient setting. Motivational interviewing and patient coaching could be explored as potential tools for boosting SSE. Furthermore, even if SSE is not easily changed, measuring SSE in patients newly admitted to the hospital may be useful in identifying patients most susceptible to sleep disruptions. Efforts to identify patients with low SSE should go hand‐in‐hand with measures to reduce noise. Addressing both patient‐level and environmental factors simultaneously may be the best strategy for improving sleep in an inpatient hospital setting.
In contrast to our prior study, it is worth noting that we did not find any significant relationships between overall noise levels and sleep.[9] In this dataset, nighttime noise is still a predictor of sleep loss in the hospital. However, when we restrict our sample to those who answered the SSE questionnaire and had nighttime noise recorded, we lose a significant number of observations. Because of our interest in testing the relationship between SSE and sleep, we chose to control for overall noise (which enabled us to retain more observations). We also did not find any interactions between SSE and noise in our regression models. Further work is warranted with larger sample sizes to better understand the role of SSE in the context of sleep and noise levels. In addition, females also received more sleep than males in our study.
There are several limitations to this study. This study was carried out at a single service at a single institution, limiting the ability to generalize the findings to other hospital settings. This study had a relatively high rate of patients who were unable to complete at least 1 night of data collection (42%), often due to watch removal for imaging or procedures, which may also affect the representativeness of our sample. Moreover, we can only examine associations and not causal relationships. The SSE scale has never been used in hospitalized patients, making comparisons between scores from hospitalized patients and population controls difficult. In addition, the SSE scale also has not been dichotomized in previous studies into high and low SSE. However, a sensitivity analysis with raw SSE scores did not change the results of our study. It can be difficult to perform actigraphy measurements in the hospital because many patients spend most of their time in bed. Because we chose a relatively healthy cohort of patients without significant limitations in mobility, actigraphy could still be used to differentiate time spent awake from time spent sleeping. Because we did not perform polysomnography, we cannot explore the role of sleep architecture which is an important component of sleep quality. Although the use of pharmacologic sleep aids is a potential confounding factor, the rate of use was very low in our cohort and unlikely to significantly affect our results. Continued study of this patient population is warranted to further develop the findings.
In conclusion, patients with high SSE sleep better in the hospital, tend to be in quieter rooms, and may report fewer noise complaints. Our findings suggest that a greater confidence in the ability to sleep may be beneficial in hospitalized adults. In addition to noise control, hospitals should also consider targeting patients with low SSE when designing novel interventions to improve in‐hospital sleep.
Disclosures
This work was supported by funding from the National Institute on Aging through a Short‐Term Aging‐Related Research Program (1 T35 AG029795), National Institute on Aging career development award (K23AG033763), a midcareer career development award (1K24AG031326), a program project (P01AG‐11412), an Agency for Healthcare Research and Quality Centers for Education and Research on Therapeutics grant (1U18HS016967), and a National Institute on Aging Clinical Translational Sciences award (UL1 RR024999). Dr. Arora had full access to all of the data in the study and takes responsibility for the integrity of the data and the accuracy of the statistical analysis. The funding agencies had no role in the design of the study; the collection, analysis, and interpretation of the data; or the decision to approve publication of the finished manuscript. The authors report no conflicts of interest.
- The metabolic consequences of sleep deprivation. Sleep Med Rev. 2007;11(3):163–178. , , , .
- Poor self‐reported sleep quality predicts mortality within one year of inpatient post‐acute rehabilitation among older adults. Sleep. 2011;34(12):1715–1721. , , , , , .
- The sleep of older people in hospital and nursing homes. J Clin Nurs. 1999;8:360–368. , , , et al.
- Sleep in hospitalized medical patients, part 1: factors affecting sleep. J Hosp Med. 2008; 3:473–482. , , et al.
- Nocturnal care interactions with patients in critical care units. Am J Crit Care. 2004;13:102–112; quiz 114–115. , , , et al.
- Patient perception of sleep quality and etiology of sleep disruption in the intensive care unit. Am J Respir Crit Care Med. 1999;159:1155–1162. , , .
- Sleep in acute care settings: an integrative review. J Nurs Scholarsh. 2000;32(1):31–38. .
- Sleep disruption due to hospital noises: a prospective evaluation. Ann Int Med. 2012;157(3): 170–179. , , , et al.
- Noise and sleep among adult medical inpatients: far from a quiet night. Arch Intern Med. 2012;172:68–70. , , , et al.
- Generalized expectancies for internal versus external control of reinforcement. Psychol Monogr. 1966;80:1–28. .
- Psychosocial risk factors and mortality: a prospective study with special focus on social support, social participation, and locus of control in Norway. J Epidemiol Community Health. 1998;52:476–481. , .
- The interactive effect of perceived control and functional status on health and mortality among young‐old and old‐old adults. J Gerontol B Psychol Sci Soc Sci. 1997;52:P118–P126. , .
- Role‐specific feelings of control and mortality. Psychol Aging. 2000;15:617–626. , .
- Patient empowerment in intensive care—an interview study. Intensive Crit Care Nurs. 2006;22:370–377. , , .
- Exploring the relationship between personal control and the hospital environment. J Clin Nurs. 2008;17:1601–1609. , , .
- Effects of volitional lifestyle on sleep‐life habits in the aged. Psychiatry Clin Neurosci. 1998;52:183–184. , , , et al.
- Sleep locus of control: report on a new scale. Behav Sleep Med. 2004;2:79–93. , , , et al.
- Effects of physician experience on costs and outcomes on an academic general medicine service: results of a trial of hospitalists. Ann Intern Med. 2002;137:866–874. , , , et al.
- The effects of age, sex, ethnicity, and sleep‐disordered breathing on sleep architecture. Arch Intern Med. 2004;164:406–418. , , , et al.
- Validation of a telephone version of the mini‐mental state examination. J Am Geriatr Soc. 1992;40:697–702. , , , et al.
- Contact isolation in surgical patients: a barrier to care? Surgery. 2003;134:180–188. , , , et al.
- Adverse effects of contact isolation. Lancet. 1999;354:1177–1178. , .
- Behavioral Treatment for Persistent Insomnia. Elmsford, NY: Pergamon Press; 1987. .
- A new method for measuring daytime sleepiness: the Epworth sleepiness scale. Sleep. 1991;14:540–545. .
- Reliability and factor analysis of the Epworth Sleepiness Scale. Sleep. 1992;15:376–381. .
- Objective components of individual differences in subjective sleep quality. J Sleep Res. 1997;6:217–220. , .
- The role of actigraphy in the study of sleep and circadian rhythms. Sleep. 2003;26:342–392. , , , et al.
- Practice parameters for the use of actigraphy in the assessment of sleep and sleep disorders: an update for 2007. Sleep. 2007;30:519–529. , , , et al.
- The role of actigraphy in the evaluation of sleep disorders. Sleep. 1995;18:288–302. , , , et al.
- Clinical review: sleep measurement in critical care patients: research and clinical implications. Crit Care. 2007;11:226. , , , et al.
- Evaluation of immobility time for sleep latency in actigraphy. Sleep Med. 2009;10:621–625. , , , et al.
- The subjective meaning of sleep quality: a comparison of individuals with and without insomnia. Sleep. 2008;31:383–393. , , , et al.
- Sleep in hospitalized medical patients, part 2: behavioral and pharmacological management of sleep disturbances. J Hosp Med. 2009;4:50–59. , , , et al.
- A nonpharmacologic sleep protocol for hospitalized older patients. J Am Geriatr Soc. 1998;46(6):700–705. , , , .
- The metabolic consequences of sleep deprivation. Sleep Med Rev. 2007;11(3):163–178. , , , .
- Poor self‐reported sleep quality predicts mortality within one year of inpatient post‐acute rehabilitation among older adults. Sleep. 2011;34(12):1715–1721. , , , , , .
- The sleep of older people in hospital and nursing homes. J Clin Nurs. 1999;8:360–368. , , , et al.
- Sleep in hospitalized medical patients, part 1: factors affecting sleep. J Hosp Med. 2008; 3:473–482. , , et al.
- Nocturnal care interactions with patients in critical care units. Am J Crit Care. 2004;13:102–112; quiz 114–115. , , , et al.
- Patient perception of sleep quality and etiology of sleep disruption in the intensive care unit. Am J Respir Crit Care Med. 1999;159:1155–1162. , , .
- Sleep in acute care settings: an integrative review. J Nurs Scholarsh. 2000;32(1):31–38. .
- Sleep disruption due to hospital noises: a prospective evaluation. Ann Int Med. 2012;157(3): 170–179. , , , et al.
- Noise and sleep among adult medical inpatients: far from a quiet night. Arch Intern Med. 2012;172:68–70. , , , et al.
- Generalized expectancies for internal versus external control of reinforcement. Psychol Monogr. 1966;80:1–28. .
- Psychosocial risk factors and mortality: a prospective study with special focus on social support, social participation, and locus of control in Norway. J Epidemiol Community Health. 1998;52:476–481. , .
- The interactive effect of perceived control and functional status on health and mortality among young‐old and old‐old adults. J Gerontol B Psychol Sci Soc Sci. 1997;52:P118–P126. , .
- Role‐specific feelings of control and mortality. Psychol Aging. 2000;15:617–626. , .
- Patient empowerment in intensive care—an interview study. Intensive Crit Care Nurs. 2006;22:370–377. , , .
- Exploring the relationship between personal control and the hospital environment. J Clin Nurs. 2008;17:1601–1609. , , .
- Effects of volitional lifestyle on sleep‐life habits in the aged. Psychiatry Clin Neurosci. 1998;52:183–184. , , , et al.
- Sleep locus of control: report on a new scale. Behav Sleep Med. 2004;2:79–93. , , , et al.
- Effects of physician experience on costs and outcomes on an academic general medicine service: results of a trial of hospitalists. Ann Intern Med. 2002;137:866–874. , , , et al.
- The effects of age, sex, ethnicity, and sleep‐disordered breathing on sleep architecture. Arch Intern Med. 2004;164:406–418. , , , et al.
- Validation of a telephone version of the mini‐mental state examination. J Am Geriatr Soc. 1992;40:697–702. , , , et al.
- Contact isolation in surgical patients: a barrier to care? Surgery. 2003;134:180–188. , , , et al.
- Adverse effects of contact isolation. Lancet. 1999;354:1177–1178. , .
- Behavioral Treatment for Persistent Insomnia. Elmsford, NY: Pergamon Press; 1987. .
- A new method for measuring daytime sleepiness: the Epworth sleepiness scale. Sleep. 1991;14:540–545. .
- Reliability and factor analysis of the Epworth Sleepiness Scale. Sleep. 1992;15:376–381. .
- Objective components of individual differences in subjective sleep quality. J Sleep Res. 1997;6:217–220. , .
- The role of actigraphy in the study of sleep and circadian rhythms. Sleep. 2003;26:342–392. , , , et al.
- Practice parameters for the use of actigraphy in the assessment of sleep and sleep disorders: an update for 2007. Sleep. 2007;30:519–529. , , , et al.
- The role of actigraphy in the evaluation of sleep disorders. Sleep. 1995;18:288–302. , , , et al.
- Clinical review: sleep measurement in critical care patients: research and clinical implications. Crit Care. 2007;11:226. , , , et al.
- Evaluation of immobility time for sleep latency in actigraphy. Sleep Med. 2009;10:621–625. , , , et al.
- The subjective meaning of sleep quality: a comparison of individuals with and without insomnia. Sleep. 2008;31:383–393. , , , et al.
- Sleep in hospitalized medical patients, part 2: behavioral and pharmacological management of sleep disturbances. J Hosp Med. 2009;4:50–59. , , , et al.
- A nonpharmacologic sleep protocol for hospitalized older patients. J Am Geriatr Soc. 1998;46(6):700–705. , , , .
Copyright © 2013 Society of Hospital Medicine
Attendings' Perception of Housestaff
Clinical supervision in graduate medical education (GME) emphasizes patient safety while promoting development of clinical expertise by allowing trainees progressive independence.[1, 2, 3] The importance of the balance between supervision and autonomy has been recognized by accreditation organizations, namely the Institute of Medicine and the Accreditation Council for Graduate Medical Education (ACGME).[4, 5] However, little is known of best practices in supervision, and the model of progressive independence in clinical training lacks empirical support.[3] Limited evidence suggests that enhanced clinical supervision may have positive effects on patient and education‐related outcomes.[6, 7, 8, 9, 10, 11, 12, 13, 14, 15] However, a more nuanced understanding of potential effects of enhanced supervision on resident autonomy and decision making is still required, particularly as preliminary work on increased on‐site hospitalist supervision has yielded mixed results.[16, 17, 18, 19]
Understanding how trainees are entrusted with autonomy will be integral to the ACGME's Next Accreditation System.[20] Entrustable Professional Activities are benchmarks by which resident readiness to progress through training will be judged.[21] The extent to which trainees are entrusted with autonomy is largely determined by the subjective assessment of immediate supervisors, as autonomy is rarely measured or quantified.[3, 22, 23] This judgment of autonomy, most frequently performed by ward attendings, may be subject to significant variation and influenced by factors other than the resident's competence and clinical abilities.
To that end, it is worth considering what factors may affect attending perception of housestaff autonomy and decision making. Recent changes in the GME environment and policy implementation have altered the landscape of the attending workforce considerably. The growth of the hospitalist movement in teaching hospitals, in part due to duty hours, has led to more residents being supervised by hospitalists, who may perceive trainee autonomy differently than other attendings do.[24] This study aims to examine whether factors such as attending demographics and short‐term and long‐term secular trends influence attending perception of housestaff autonomy and participation in decision making.
METHODS
Study Design
From 2001 to 2008, attending physicians at a single academic institution were surveyed at the end of inpatient general medicine teaching rotations.[25] The University of Chicago general medicine service consists of ward teams of an attending physician (internists, hospitalists, or subspecialists), 1 senior resident, and 1 or 2 interns. Attendings serve for 2‐ or 4‐week rotations. Attendings were consented for participation and received a 40‐item, paper‐based survey at the rotation's end. The institutional review board approved this study.
Data Collection
From the 40 survey items, 2 statements were selected for analysis: The intern(s) were truly involved in decision making about their patients and My resident felt that s/he had sufficient autonomy this month. These items have been used in previous work studying attending‐resident dynamics.[19, 26] Attendings also reported demographic and professional information as well as self‐identified hospitalist status, ascertained by the question Do you consider yourself to be a hospitalist? Survey month and year were also recorded. We conducted a secondary data analysis of an inclusive sample of responses to the questions of interest.
Statistical Analysis
Descriptive statistics were used to summarize survey responses and demographics. Survey questions consisted of Likert‐type items. Because the distribution of responses was skewed toward strong agreement for both questions, we collapsed scores into 2 categories (Strongly Agree and Do Not Strongly Agree).[19] Perception of sufficient trainee autonomy was defined as a response of Strongly Agree. The Pearson 2 test was used to compare proportions, and t tests were used to compare mean years since completion of residency and weeks on service between different groups.
Multivariate logistic regression with stepwise forward regression was used to model the relationship between attending sex, institutional hospitalist designation, years of experience, implementation of duty‐hours restrictions, and academic season, and perception of trainee autonomy and decision making. Academic seasons were defined as summer (JulySeptember), fall (OctoberDecember), winter (JanuaryMarch) and spring (AprilJune).[26] Years of experience were divided into tertiles of years since residency: 04 years, 511 years, and >11 years. To account for the possibility that the effect of hospitalist specialty varied by experience, interaction terms were constructed. The interaction term hospitalist*early‐career was used as the reference group.
RESULTS
Seven hundred thirty‐eight surveys were distributed to attendings on inpatient general medicine teaching services from 2001 to 2008; 70% (n=514) were included in the analysis. Table 1 provides demographic characteristics of the respondents. Roughly half (47%) were female, and 23% were hospitalists. Experience ranged from 0 to 35 years, with a median of 7 years. Weeks on service per year ranged from 1 to 27, with a median of 6 weeks. Hospitalists represented a less‐experienced group of attendings, as their mean experience was 4.5 years (standard deviation [SD] 4.5) compared with 11.2 years (SD 7.7) for nonhospitalists (P<0.001). Hospitalists attended more frequently, with a mean 14.2 weeks on service (SD 6.5) compared with 5.8 weeks (SD 3.4) for nonhospitalists (P<0.001). Nineteen percent (n=98) of surveys were completed prior to the first ACGME duty‐hours restriction in 2003. Responses were distributed fairly equally across the academic year, with 29% completed in summer, 26% in fall, 24% in winter, and 21% in spring.
Characteristics | Value |
---|---|
| |
Female, n (%) | 275 (47) |
Hospitalist, n (%) | 125 (23) |
Years since completion of residency | |
Mean, median, SD | 9.3, 7, 7.6 |
IQR | 314 |
04, n (%) | 167 (36) |
511, n (%) | 146 (32) |
>11, n (%) | 149 (32) |
Weeks on service per yearb | |
Mean, median, SD | 8.1, 6, 5.8 |
IQR | 412 |
Forty‐four percent (n=212) of attendings perceived adequate intern involvement in decision making, and 50% (n=238) perceived sufficient resident autonomy. The correlation coefficient between these 2 measures was 0.66.
Attending Factors Associated With Perception of Trainee Autonomy
In univariate analysis, hospitalists perceived sufficient trainee autonomy less frequently than nonhospitalists; 33% perceived adequate intern involvement in decision making compared with 48% of nonhospitalists (21=6.7, P=0.01), and 42% perceived sufficient resident autonomy compared with 54% of nonhospitalists (21=3.9, P=0.048) (Table 2).
Attending Characteristics, n (%) | Agree With Intern Involvement in Decision Making | Agree With Sufficient Resident Autonomy |
---|---|---|
| ||
Designation | ||
Hospitalist | 29 (33) | 37 (42) |
Nonhospitalist | 163 (48) | 180 (54) |
Years since completion of residency | ||
04 | 37 (27) | 49 (36) |
511 | 77 (53) | 88 (61) |
>11 | 77 (53) | 81 (56) |
Sex | ||
F | 98 (46) | 100 (47) |
M | 113 (43) | 138 (53) |
Secular factors, n (%) | ||
Pre‐2003 duty‐hours restrictions | 56 (57) | 62 (65) |
Post‐2003 duty‐hours restrictions | 156 (41) | 176 (46) |
Season of survey | ||
Summer (JulySeptember) | 61 (45) | 69 (51) |
Fall (OctoberDecember) | 53 (42) | 59 (48) |
Winter (JanuaryMarch) | 42 (37) | 52 (46) |
Spring (AprilJune) | 56 (54) | 58 (57) |
Perception of trainee autonomy increased with experience (Table 2). About 30% of early‐career attendings (04 years experience) perceived sufficient autonomy and involvement in decision making compared with >50% agreement in the later‐career tertiles (intern decision making: 22=25.1, P<0.001; resident autonomy: 22=18.9, P<0.001). Attendings perceiving more intern decision making involvement had a mean 11 years of experience (SD 7.1), whereas those perceiving less had a mean of 8.8 years (SD 7.8; P=0.003). Mean years of experience were similar for perception of resident autonomy (10.6 years [SD 7.2] vs 8.9 years [SD 7.8], P=0.021).
Sex was not associated with differences in perception of intern decision making (21=0.39, P=0.53) or resident autonomy (21=1.4, P=0.236) (Table 2).
Secular Factors Associated With Perception of Trainee Autonomy
The implementation of duty‐hour restrictions in 2003 was associated with decreased attending perception of autonomy. Only 41% of attendings perceived adequate intern involvement in decision making following the restrictions, compared with 57% before the restrictions were instituted (21=8.2, P=0.004). Similarly, 46% of attendings agreed with sufficient resident autonomy post‐duty hours, compared with 65% prior (21=10.1, P=0.001) (Table 2).
Academic season was also associated with differences in perception of autonomy (Table 2). In spring, 54% of attendings perceived adequate intern involvement in decision making, compared with 42% in the other seasons combined (21=5.34, P=0.021). Perception of resident autonomy was also higher in spring, though this was not statistically significant (57% in spring vs 48% in the other seasons; 21=2.37, P=0.123).
Multivariate Analyses
Variation in attending perception of housestaff autonomy by attending characteristics persisted in multivariate analysis. Table 3 shows ORs for perception of adequate intern involvement in decision making and sufficient resident autonomy. Sex was not a significant predictor of agreement with either statement. The odds that an attending would perceive adequate intern involvement in decision making were higher for later‐career attendings compared with early‐career attendings (ie, 04 years); attendings who completed residency 511 years ago were 2.16 more likely to perceive adequate involvement (OR: 2.16, 95% CI: 1.17‐3.97, P=0.013), and those >11 years from residency were 2.05 more likely (OR: 2.05, 95% CI: 1.16‐3.63, P=0.014). Later‐career attendings also had nonsignificant higher odds of perceiving sufficient resident autonomy compared with early‐career attendings (511 years, OR: 1.73, 95% CI: 0.963.14, P=0.07; >11 years, OR: 1.50, 95% CI: 0.862.62, P=0.154).
Interns Involved With Decision Making | Resident Had Sufficient Autonomy | |||
---|---|---|---|---|
| ||||
Covariate | OR (95% CI) | P Value | OR (95% CI) | P Value |
Attending characteristics | ||||
04 years of experience | ||||
511 years of experience | 2.16 (1.17‐3.97) | 0.013 | 1.73 (0.96‐3.14) | 0.07 |
>11 years of experience | 2.05 (1.16‐3.63) | 0.014 | 1.50 (0.86‐2.62) | 0.154 |
Hospitalist | 0.19 (0.06‐0.58) | 0.004 | 0.27 (0.11‐0.66) | 0.004 |
Hospitalist 04 years of experiencea | ||||
Hospitalist 511 years of experiencea | 7.36 (1.86‐29.1) | 0.004 | 5.85 (1.75‐19.6) | 0.004 |
Hospitalist >11 years of experiencea | 21.2 (1.73‐260) | 0.017 | 14.4 (1.31‐159) | 0.029 |
Female sex | 1.41 (0.92‐2.17) | 0.115 | 0.92 (0.60‐1.40) | 0.69 |
Secular factors | ||||
Post‐2003 duty hours | 0.51 (0.29‐0.87) | 0.014 | 0.49 (0.28‐0.86) | 0.012 |
Spring academic season | 1.94 (1.18‐3.19) | 0.009 | 1.59 (0.97‐2.60) | 0.064 |
Hospitalists were associated with 81% lower odds of perceiving adequate intern involvement in decision making (OR: 0.19, 95% CI: 0.060.58, P=0.004) and 73% lower odds of perceiving sufficient resident autonomy compared with nonhospitalists (OR: 0.27, 95% CI: 0.110.66, P=0.004). However, there was a significant interaction between hospitalists and experience; compared with early‐career hospitalists, experienced hospitalists had higher odds of perceiving both adequate intern involvement in decision making (511 years, OR: 7.36, 95% CI: 1.8629.1, P=0.004; >11 years, OR: 21.2, 95% CI: 1.73260, P=0.017) and sufficient resident autonomy (511 years, OR: 5.85, 95% CI: 1.7519.6, P=0.004; >11 years, OR: 14.4, 95% CI: 1.3159, P=0.029) (Table 3).
Secular trends also remained associated with differences in perception of housestaff autonomy (Table 3). Attendings had 49% lower odds of perceiving adequate intern involvement in decision making in the years following duty‐hour limits compared with the years prior (OR: 0.51, 95% CI: 0.29‐0.87, P=0.014). Similarly, odds of perceiving sufficient resident autonomy were 51% lower post‐duty hours (OR: 0.49, 95% CI: 0.280.86, P=0.012). Spring season was associated with 94% higher odds of perceiving adequate intern involvement in decision making compared with other seasons (OR: 1.94, 95% 1.183.19, P=0.009). There were also nonsignificant higher odds of perception of sufficient resident autonomy in spring (OR: 1.59, 95% CI: 0.972.60, P=0.064). To address the possibility of associations due to secular trends resulting from repeated measures of attendings, models using attending fixed effects were also used. Clustering by attending, the associations between duty hours and perceiving sufficient resident autonomy and intern decision making both remained significant, but the association of spring season did not.
DISCUSSION
This study highlights that attendings' perception of housestaff autonomy varies by attending characteristics and secular trends. Specifically, early‐career attendings and hospitalists were less likely to perceive sufficient housestaff autonomy and involvement in decision making. However, there was a significant hospitalist‐experience interaction, such that more‐experienced hospitalists were associated with higher odds of perceiving sufficient autonomy than would be expected from the effect of experience alone. With respect to secular trends, attendings perceived more trainee autonomy in the last quarter of the academic year, and less autonomy after implementation of resident duty‐hour restrictions in 2003.
As Entrustable Professional Activities unveil a new emphasis on the notion of entrustment, it will be critical to ensure that attending assessment of resident performance is uniform and a valid judge of when to entrust autonomy.[27, 28] If, as suggested by these findings, perception of autonomy varies based on attending characteristics, all faculty may benefit from strategies to standardize assessment and evaluation skills to ensure trainees are appropriately progressing through various milestones to achieve competence. Our results suggest that faculty development may be particularly important for early‐career attendings and especially hospitalists.
Early‐career attendings may perceive less housestaff autonomy due to a reluctance to relinquish control over patient‐care duties and decision making when the attending is only a few years from residency. Hospitalists are relatively junior in most institutions and may be similar to early‐career attendings in that regard. It is noteworthy, however, that experienced hospitalists are associated with even greater perception of autonomy than would be predicted by years of experience alone. Hospitalists may gain experience at a rate faster than nonhospitalists, which could affect how they perceive autonomy and decision making in trainees and may make them more comfortable entrusting autonomy to housestaff. Early‐career hospitalists likely represent a heterogeneous group of physicians, in both 1‐year clinical hospitalists as well as academic‐career hospitalists, who may have different approaches to managing housestaff teams. Residents are less likely to fear hospitalists limiting their autonomy after exposure to working with hospitalists as teaching attendings, and our findings may suggest a corollary in that hospitalists may be more likely to perceive sufficient autonomy with more exposure to working with housestaff.[19]
Attendings perceived less housestaff autonomy following the 2003 duty‐hour limits. This may be due to attendings assuming more responsibilities that were traditionally performed by residents.[26, 29] This shifting of responsibility may lead to perception of less‐active housestaff decision making and less‐evident autonomy. These findings suggest autonomy may become even more restricted after implementation of the 2011 duty‐hour restrictions, which included 16‐hour shifts for interns.[5] Further studies are warranted in examining the effect of these new limits. Entrustment of autonomy and allowance for decision making is an essential part of any learning environment that allows residents to develop clinical reasoning skills, and it will be critical to adopt new strategies to encourage professional growth of housestaff in this new era.[30]
Attendings also perceived autonomy differently by academic season. Spring represents the season by which housestaff are most experienced and by which attendings may be most familiar with individual team members. Additionally, there may be a stronger emphasis on supervision and adherence to traditional hierarchy earlier in the academic year as interns and junior residents are learning their new roles.[30] These findings may have implications for system changes to support development of more functional educational dyads between attendings and trainees, especially early in the academic year.[31]
There are several limitations to our findings. This is a single‐institution study restricted to the general‐medicine service; thus generalizability is limited. Our outcome measures, the survey items of interest, question perception of housestaff autonomy but do not query the appropriateness of that autonomy, an important construct in entrustment. Additionally, self‐reported answers could be subject to recall bias. Although data were collected over 8 years, the most recent trends of residency training are not reflected. Although there was a significant interaction involving experienced hospitalists, wide confidence intervals and large standard errors likely reflect the relatively few individuals in this category. Though there was a large number of overall respondents, our interaction terms included few advanced‐career hospitalists, likely secondary to hospital medicine's relative youth as a specialty.
As this study focuses only on perception of autonomy, future work must investigate autonomy from a practical standpoint. It is conceivable that if factors such as attending characteristics and secular trends influence perception, they may also be associated with variation in how attendings entrust autonomy and provide supervision. To what extent perception and practice are linked remains to be studied, but it will be important to determine if variation due to these factors may also be associated with inconsistent and uneven supervisory practices that would adversely affect resident education and patient safety.
Finally, future work must include the viewpoint of the recipients of autonomy: the residents and interns. A significant limitation of the current study is the lack of the resident perspective, as our survey was only administered to attendings. Autonomy is clearly a 2‐way relationship, and attending perception must be corroborated by the resident's experience. It is possible attendings may perceive that their housestaff have sufficient autonomy, but residents may view this autonomy as inappropriate or unavoidable due an absentee attending who does not adequately supervise.[32] Future work must examine how resident and attending perceptions of autonomy correlate, and whether discordance or concordance in these perceptions influence satisfaction with attending‐resident relationships, education, and patient care.
In conclusion, significant variation existed among attending physicians with respect to perception of housestaff autonomy, an important aspect of entrustment and clinical supervision. This variation was present for hospitalists, among different levels of attending experience, and a significant interaction was found between these 2 factors. Additionally, secular trends were associated with differences in perception of autonomy. As entrustment of residents with progressive levels of autonomy becomes more integrated within the requirements for advancement in residency, a greater understanding of factors affecting entrustment will be critical in helping faculty develop skills to appropriately assess trainee professional growth and development.
Acknowledgments
The authors thank all members of the Multicenter Hospitalist Project for their assistance with this project.
Disclosures: The authors acknowledge funding from the AHRQ/CERT 5 U18 HS016967‐01. The funder had no role in the design of the study; the collection, analysis, and interpretation of the data; or the decision to approve publication of the finished manuscript. Prior presentations of the data include the 2012 Department of Medicine Research Day at the University of Chicago, the 2012 Society of Hospital Medicine Annual Meeting in San Diego, California, and the 2012 Midwest Society of General Medicine Meeting in Chicago, Illinois. All coauthors have seen and agree with the contents of the manuscript. The submission was not under review by any other publication. The authors report no conflicts of interest.
- Effective supervision in clinical practice settings: a literature review. Med Educ. 2000;34(10):827–840. , .
- Deliberate practice and acquisition of expert performance: a general overview. Acad Emerg Med. 2008;15(11):988–994. .
- Progressive independence in clinical training: a tradition worth defending? Acad Med. 2005;80(10 suppl):S106–S111. , , , et al.
- Committee on Optimizing Graduate Medical Trainee (Resident) Hours and Work Schedules to Improve Patient Safety, Institute of Medicine. Ulmer C, Wolman D, Johns M, eds. Resident Duty Hours: Enhancing Sleep, Supervision, and Safety. Washington, DC: National Academies Press; 2008.
- ACGME Duty Hour Task Force. The new recommendations on duty hours from the ACGME Task Force. N Engl J Med. 2010;363(2):e3. , , ;
- Positive impact of pediatric critical care fellows on mortality: is it merely a function of resident supervision? Crit Care Med. 1997;25(10):1622–1623. .
- The effect of supervision of residents on quality of care in five university‐affiliated emergency departments. Acad Med. 1998;73(7):776–782. , , , et al.
- Increased faculty presence on inpatient teaching services. Mayo Clin Proc. 2004;79(3):332–336. , , , et al.
- How medical residents perceive the quality of supervision provided by attending doctors in the clinical setting. Med Educ. 2005;39(7):696–703. , , , et al.
- Resident supervision in the operating room: does this impact on outcome? J Trauma. 1993;35(4):556–560. , , .
- Effects of supervision by attending anesthesiologists on complications of emergency tracheal intubation. Anesthesiology. 2008;109(6):973–937. , , , et al.
- Around‐the‐clock attending radiology coverage is essential to avoid mistakes in the care of trauma patients. Am Surg. 2001;67(12):1175–1177. , , , et al.
- Supervision in the outpatient clinic: effects on teaching and patient care. J Gen Int Med. 1993;8(7):378–380. , .
- How medical students and residents describe the roles and characteristics of their influential clinical teachers. Acad Med. 2000;75(8):843–845. , .
- A systematic review: the effect of clinical supervision on patient and residency education outcomes. Acad Med. 2012;87(4):428–442. , , , et al.
- for the SGIM Housestaff Oversight Subcommittee. Survey of overnight academic hospitalist supervision of trainees. J Hosp Med. 2012;7(7):521–523. , , , et al;
- Effects of increased overnight supervision on resident education, decision‐making, and autonomy. J Hosp Med. 2012;7(8):606–610. , , , et al.
- The effect of overnight in‐house attending coverage on perceptions of care and education on a general medical service. J Grad Med Educ. 2010;2(1):53–56. , , , et al.
- Resident satisfaction on an academic hospitalist service: time to teach. Am J Med. 2002;112(7):597–601. , , , et al.
- The next GME accreditation system—rationale and benefits. N Engl J Med. 2012;366(11):1051–1056. , , , et al.
- Competency‐based postgraduate training: can we bridge the gap between theory and clinical practice? Acad Med. 2007;82(6):542–547. , .
- Trust, competence, and the supervisor's role in postgraduate training. BMJ. 2006;333(7571):748–751. .
- Measuring progressive independence with the resident supervision index: empirical approach. J Grad Med Educ. 2010;2(1):17–30. , , , et al.
- The emerging role of “hospitalists” in the American health care system. N Engl J Med. 1996;335(7):514–517. , .
- Effect of ACGME duty hours on attending physician teaching and satisfaction. Arch Intern Med. 2008;168(11):1226–1227. , .
- Association of workload of on‐call interns with on‐call sleep duration, shift duration, and participation in educational activities. JAMA. 2008;300(10):1146–1153. , , , et al.
- Entrustability of professional activities and competency‐based training. Med Educ. 2005;39:1176–1177. .
- When do supervising physicians decide to entrust residents with unsupervised tasks? Acad Med. 2010;85(9):1399–1400. , , , et al.
- Effect of residency duty‐hour limits. Arch Intern Med. 2007;167(14):1487–1492. , , et al.
- Strategies for improving teaching practices: a comprehensive approach to faculty development. Acad Med. 1998;73:387–396. , .
- A framework for effective training for supervisors. Med Teach. 2002;24:385–389. , , .
- On‐call supervision and resident autonomy: from micromanager to absentee attending. Am J Med. 2009;122(8):784–788. , , , et al.
Clinical supervision in graduate medical education (GME) emphasizes patient safety while promoting development of clinical expertise by allowing trainees progressive independence.[1, 2, 3] The importance of the balance between supervision and autonomy has been recognized by accreditation organizations, namely the Institute of Medicine and the Accreditation Council for Graduate Medical Education (ACGME).[4, 5] However, little is known of best practices in supervision, and the model of progressive independence in clinical training lacks empirical support.[3] Limited evidence suggests that enhanced clinical supervision may have positive effects on patient and education‐related outcomes.[6, 7, 8, 9, 10, 11, 12, 13, 14, 15] However, a more nuanced understanding of potential effects of enhanced supervision on resident autonomy and decision making is still required, particularly as preliminary work on increased on‐site hospitalist supervision has yielded mixed results.[16, 17, 18, 19]
Understanding how trainees are entrusted with autonomy will be integral to the ACGME's Next Accreditation System.[20] Entrustable Professional Activities are benchmarks by which resident readiness to progress through training will be judged.[21] The extent to which trainees are entrusted with autonomy is largely determined by the subjective assessment of immediate supervisors, as autonomy is rarely measured or quantified.[3, 22, 23] This judgment of autonomy, most frequently performed by ward attendings, may be subject to significant variation and influenced by factors other than the resident's competence and clinical abilities.
To that end, it is worth considering what factors may affect attending perception of housestaff autonomy and decision making. Recent changes in the GME environment and policy implementation have altered the landscape of the attending workforce considerably. The growth of the hospitalist movement in teaching hospitals, in part due to duty hours, has led to more residents being supervised by hospitalists, who may perceive trainee autonomy differently than other attendings do.[24] This study aims to examine whether factors such as attending demographics and short‐term and long‐term secular trends influence attending perception of housestaff autonomy and participation in decision making.
METHODS
Study Design
From 2001 to 2008, attending physicians at a single academic institution were surveyed at the end of inpatient general medicine teaching rotations.[25] The University of Chicago general medicine service consists of ward teams of an attending physician (internists, hospitalists, or subspecialists), 1 senior resident, and 1 or 2 interns. Attendings serve for 2‐ or 4‐week rotations. Attendings were consented for participation and received a 40‐item, paper‐based survey at the rotation's end. The institutional review board approved this study.
Data Collection
From the 40 survey items, 2 statements were selected for analysis: The intern(s) were truly involved in decision making about their patients and My resident felt that s/he had sufficient autonomy this month. These items have been used in previous work studying attending‐resident dynamics.[19, 26] Attendings also reported demographic and professional information as well as self‐identified hospitalist status, ascertained by the question Do you consider yourself to be a hospitalist? Survey month and year were also recorded. We conducted a secondary data analysis of an inclusive sample of responses to the questions of interest.
Statistical Analysis
Descriptive statistics were used to summarize survey responses and demographics. Survey questions consisted of Likert‐type items. Because the distribution of responses was skewed toward strong agreement for both questions, we collapsed scores into 2 categories (Strongly Agree and Do Not Strongly Agree).[19] Perception of sufficient trainee autonomy was defined as a response of Strongly Agree. The Pearson 2 test was used to compare proportions, and t tests were used to compare mean years since completion of residency and weeks on service between different groups.
Multivariate logistic regression with stepwise forward regression was used to model the relationship between attending sex, institutional hospitalist designation, years of experience, implementation of duty‐hours restrictions, and academic season, and perception of trainee autonomy and decision making. Academic seasons were defined as summer (JulySeptember), fall (OctoberDecember), winter (JanuaryMarch) and spring (AprilJune).[26] Years of experience were divided into tertiles of years since residency: 04 years, 511 years, and >11 years. To account for the possibility that the effect of hospitalist specialty varied by experience, interaction terms were constructed. The interaction term hospitalist*early‐career was used as the reference group.
RESULTS
Seven hundred thirty‐eight surveys were distributed to attendings on inpatient general medicine teaching services from 2001 to 2008; 70% (n=514) were included in the analysis. Table 1 provides demographic characteristics of the respondents. Roughly half (47%) were female, and 23% were hospitalists. Experience ranged from 0 to 35 years, with a median of 7 years. Weeks on service per year ranged from 1 to 27, with a median of 6 weeks. Hospitalists represented a less‐experienced group of attendings, as their mean experience was 4.5 years (standard deviation [SD] 4.5) compared with 11.2 years (SD 7.7) for nonhospitalists (P<0.001). Hospitalists attended more frequently, with a mean 14.2 weeks on service (SD 6.5) compared with 5.8 weeks (SD 3.4) for nonhospitalists (P<0.001). Nineteen percent (n=98) of surveys were completed prior to the first ACGME duty‐hours restriction in 2003. Responses were distributed fairly equally across the academic year, with 29% completed in summer, 26% in fall, 24% in winter, and 21% in spring.
Characteristics | Value |
---|---|
| |
Female, n (%) | 275 (47) |
Hospitalist, n (%) | 125 (23) |
Years since completion of residency | |
Mean, median, SD | 9.3, 7, 7.6 |
IQR | 314 |
04, n (%) | 167 (36) |
511, n (%) | 146 (32) |
>11, n (%) | 149 (32) |
Weeks on service per yearb | |
Mean, median, SD | 8.1, 6, 5.8 |
IQR | 412 |
Forty‐four percent (n=212) of attendings perceived adequate intern involvement in decision making, and 50% (n=238) perceived sufficient resident autonomy. The correlation coefficient between these 2 measures was 0.66.
Attending Factors Associated With Perception of Trainee Autonomy
In univariate analysis, hospitalists perceived sufficient trainee autonomy less frequently than nonhospitalists; 33% perceived adequate intern involvement in decision making compared with 48% of nonhospitalists (21=6.7, P=0.01), and 42% perceived sufficient resident autonomy compared with 54% of nonhospitalists (21=3.9, P=0.048) (Table 2).
Attending Characteristics, n (%) | Agree With Intern Involvement in Decision Making | Agree With Sufficient Resident Autonomy |
---|---|---|
| ||
Designation | ||
Hospitalist | 29 (33) | 37 (42) |
Nonhospitalist | 163 (48) | 180 (54) |
Years since completion of residency | ||
04 | 37 (27) | 49 (36) |
511 | 77 (53) | 88 (61) |
>11 | 77 (53) | 81 (56) |
Sex | ||
F | 98 (46) | 100 (47) |
M | 113 (43) | 138 (53) |
Secular factors, n (%) | ||
Pre‐2003 duty‐hours restrictions | 56 (57) | 62 (65) |
Post‐2003 duty‐hours restrictions | 156 (41) | 176 (46) |
Season of survey | ||
Summer (JulySeptember) | 61 (45) | 69 (51) |
Fall (OctoberDecember) | 53 (42) | 59 (48) |
Winter (JanuaryMarch) | 42 (37) | 52 (46) |
Spring (AprilJune) | 56 (54) | 58 (57) |
Perception of trainee autonomy increased with experience (Table 2). About 30% of early‐career attendings (04 years experience) perceived sufficient autonomy and involvement in decision making compared with >50% agreement in the later‐career tertiles (intern decision making: 22=25.1, P<0.001; resident autonomy: 22=18.9, P<0.001). Attendings perceiving more intern decision making involvement had a mean 11 years of experience (SD 7.1), whereas those perceiving less had a mean of 8.8 years (SD 7.8; P=0.003). Mean years of experience were similar for perception of resident autonomy (10.6 years [SD 7.2] vs 8.9 years [SD 7.8], P=0.021).
Sex was not associated with differences in perception of intern decision making (21=0.39, P=0.53) or resident autonomy (21=1.4, P=0.236) (Table 2).
Secular Factors Associated With Perception of Trainee Autonomy
The implementation of duty‐hour restrictions in 2003 was associated with decreased attending perception of autonomy. Only 41% of attendings perceived adequate intern involvement in decision making following the restrictions, compared with 57% before the restrictions were instituted (21=8.2, P=0.004). Similarly, 46% of attendings agreed with sufficient resident autonomy post‐duty hours, compared with 65% prior (21=10.1, P=0.001) (Table 2).
Academic season was also associated with differences in perception of autonomy (Table 2). In spring, 54% of attendings perceived adequate intern involvement in decision making, compared with 42% in the other seasons combined (21=5.34, P=0.021). Perception of resident autonomy was also higher in spring, though this was not statistically significant (57% in spring vs 48% in the other seasons; 21=2.37, P=0.123).
Multivariate Analyses
Variation in attending perception of housestaff autonomy by attending characteristics persisted in multivariate analysis. Table 3 shows ORs for perception of adequate intern involvement in decision making and sufficient resident autonomy. Sex was not a significant predictor of agreement with either statement. The odds that an attending would perceive adequate intern involvement in decision making were higher for later‐career attendings compared with early‐career attendings (ie, 04 years); attendings who completed residency 511 years ago were 2.16 more likely to perceive adequate involvement (OR: 2.16, 95% CI: 1.17‐3.97, P=0.013), and those >11 years from residency were 2.05 more likely (OR: 2.05, 95% CI: 1.16‐3.63, P=0.014). Later‐career attendings also had nonsignificant higher odds of perceiving sufficient resident autonomy compared with early‐career attendings (511 years, OR: 1.73, 95% CI: 0.963.14, P=0.07; >11 years, OR: 1.50, 95% CI: 0.862.62, P=0.154).
Interns Involved With Decision Making | Resident Had Sufficient Autonomy | |||
---|---|---|---|---|
| ||||
Covariate | OR (95% CI) | P Value | OR (95% CI) | P Value |
Attending characteristics | ||||
04 years of experience | ||||
511 years of experience | 2.16 (1.17‐3.97) | 0.013 | 1.73 (0.96‐3.14) | 0.07 |
>11 years of experience | 2.05 (1.16‐3.63) | 0.014 | 1.50 (0.86‐2.62) | 0.154 |
Hospitalist | 0.19 (0.06‐0.58) | 0.004 | 0.27 (0.11‐0.66) | 0.004 |
Hospitalist 04 years of experiencea | ||||
Hospitalist 511 years of experiencea | 7.36 (1.86‐29.1) | 0.004 | 5.85 (1.75‐19.6) | 0.004 |
Hospitalist >11 years of experiencea | 21.2 (1.73‐260) | 0.017 | 14.4 (1.31‐159) | 0.029 |
Female sex | 1.41 (0.92‐2.17) | 0.115 | 0.92 (0.60‐1.40) | 0.69 |
Secular factors | ||||
Post‐2003 duty hours | 0.51 (0.29‐0.87) | 0.014 | 0.49 (0.28‐0.86) | 0.012 |
Spring academic season | 1.94 (1.18‐3.19) | 0.009 | 1.59 (0.97‐2.60) | 0.064 |
Hospitalists were associated with 81% lower odds of perceiving adequate intern involvement in decision making (OR: 0.19, 95% CI: 0.060.58, P=0.004) and 73% lower odds of perceiving sufficient resident autonomy compared with nonhospitalists (OR: 0.27, 95% CI: 0.110.66, P=0.004). However, there was a significant interaction between hospitalists and experience; compared with early‐career hospitalists, experienced hospitalists had higher odds of perceiving both adequate intern involvement in decision making (511 years, OR: 7.36, 95% CI: 1.8629.1, P=0.004; >11 years, OR: 21.2, 95% CI: 1.73260, P=0.017) and sufficient resident autonomy (511 years, OR: 5.85, 95% CI: 1.7519.6, P=0.004; >11 years, OR: 14.4, 95% CI: 1.3159, P=0.029) (Table 3).
Secular trends also remained associated with differences in perception of housestaff autonomy (Table 3). Attendings had 49% lower odds of perceiving adequate intern involvement in decision making in the years following duty‐hour limits compared with the years prior (OR: 0.51, 95% CI: 0.29‐0.87, P=0.014). Similarly, odds of perceiving sufficient resident autonomy were 51% lower post‐duty hours (OR: 0.49, 95% CI: 0.280.86, P=0.012). Spring season was associated with 94% higher odds of perceiving adequate intern involvement in decision making compared with other seasons (OR: 1.94, 95% 1.183.19, P=0.009). There were also nonsignificant higher odds of perception of sufficient resident autonomy in spring (OR: 1.59, 95% CI: 0.972.60, P=0.064). To address the possibility of associations due to secular trends resulting from repeated measures of attendings, models using attending fixed effects were also used. Clustering by attending, the associations between duty hours and perceiving sufficient resident autonomy and intern decision making both remained significant, but the association of spring season did not.
DISCUSSION
This study highlights that attendings' perception of housestaff autonomy varies by attending characteristics and secular trends. Specifically, early‐career attendings and hospitalists were less likely to perceive sufficient housestaff autonomy and involvement in decision making. However, there was a significant hospitalist‐experience interaction, such that more‐experienced hospitalists were associated with higher odds of perceiving sufficient autonomy than would be expected from the effect of experience alone. With respect to secular trends, attendings perceived more trainee autonomy in the last quarter of the academic year, and less autonomy after implementation of resident duty‐hour restrictions in 2003.
As Entrustable Professional Activities unveil a new emphasis on the notion of entrustment, it will be critical to ensure that attending assessment of resident performance is uniform and a valid judge of when to entrust autonomy.[27, 28] If, as suggested by these findings, perception of autonomy varies based on attending characteristics, all faculty may benefit from strategies to standardize assessment and evaluation skills to ensure trainees are appropriately progressing through various milestones to achieve competence. Our results suggest that faculty development may be particularly important for early‐career attendings and especially hospitalists.
Early‐career attendings may perceive less housestaff autonomy due to a reluctance to relinquish control over patient‐care duties and decision making when the attending is only a few years from residency. Hospitalists are relatively junior in most institutions and may be similar to early‐career attendings in that regard. It is noteworthy, however, that experienced hospitalists are associated with even greater perception of autonomy than would be predicted by years of experience alone. Hospitalists may gain experience at a rate faster than nonhospitalists, which could affect how they perceive autonomy and decision making in trainees and may make them more comfortable entrusting autonomy to housestaff. Early‐career hospitalists likely represent a heterogeneous group of physicians, in both 1‐year clinical hospitalists as well as academic‐career hospitalists, who may have different approaches to managing housestaff teams. Residents are less likely to fear hospitalists limiting their autonomy after exposure to working with hospitalists as teaching attendings, and our findings may suggest a corollary in that hospitalists may be more likely to perceive sufficient autonomy with more exposure to working with housestaff.[19]
Attendings perceived less housestaff autonomy following the 2003 duty‐hour limits. This may be due to attendings assuming more responsibilities that were traditionally performed by residents.[26, 29] This shifting of responsibility may lead to perception of less‐active housestaff decision making and less‐evident autonomy. These findings suggest autonomy may become even more restricted after implementation of the 2011 duty‐hour restrictions, which included 16‐hour shifts for interns.[5] Further studies are warranted in examining the effect of these new limits. Entrustment of autonomy and allowance for decision making is an essential part of any learning environment that allows residents to develop clinical reasoning skills, and it will be critical to adopt new strategies to encourage professional growth of housestaff in this new era.[30]
Attendings also perceived autonomy differently by academic season. Spring represents the season by which housestaff are most experienced and by which attendings may be most familiar with individual team members. Additionally, there may be a stronger emphasis on supervision and adherence to traditional hierarchy earlier in the academic year as interns and junior residents are learning their new roles.[30] These findings may have implications for system changes to support development of more functional educational dyads between attendings and trainees, especially early in the academic year.[31]
There are several limitations to our findings. This is a single‐institution study restricted to the general‐medicine service; thus generalizability is limited. Our outcome measures, the survey items of interest, question perception of housestaff autonomy but do not query the appropriateness of that autonomy, an important construct in entrustment. Additionally, self‐reported answers could be subject to recall bias. Although data were collected over 8 years, the most recent trends of residency training are not reflected. Although there was a significant interaction involving experienced hospitalists, wide confidence intervals and large standard errors likely reflect the relatively few individuals in this category. Though there was a large number of overall respondents, our interaction terms included few advanced‐career hospitalists, likely secondary to hospital medicine's relative youth as a specialty.
As this study focuses only on perception of autonomy, future work must investigate autonomy from a practical standpoint. It is conceivable that if factors such as attending characteristics and secular trends influence perception, they may also be associated with variation in how attendings entrust autonomy and provide supervision. To what extent perception and practice are linked remains to be studied, but it will be important to determine if variation due to these factors may also be associated with inconsistent and uneven supervisory practices that would adversely affect resident education and patient safety.
Finally, future work must include the viewpoint of the recipients of autonomy: the residents and interns. A significant limitation of the current study is the lack of the resident perspective, as our survey was only administered to attendings. Autonomy is clearly a 2‐way relationship, and attending perception must be corroborated by the resident's experience. It is possible attendings may perceive that their housestaff have sufficient autonomy, but residents may view this autonomy as inappropriate or unavoidable due an absentee attending who does not adequately supervise.[32] Future work must examine how resident and attending perceptions of autonomy correlate, and whether discordance or concordance in these perceptions influence satisfaction with attending‐resident relationships, education, and patient care.
In conclusion, significant variation existed among attending physicians with respect to perception of housestaff autonomy, an important aspect of entrustment and clinical supervision. This variation was present for hospitalists, among different levels of attending experience, and a significant interaction was found between these 2 factors. Additionally, secular trends were associated with differences in perception of autonomy. As entrustment of residents with progressive levels of autonomy becomes more integrated within the requirements for advancement in residency, a greater understanding of factors affecting entrustment will be critical in helping faculty develop skills to appropriately assess trainee professional growth and development.
Acknowledgments
The authors thank all members of the Multicenter Hospitalist Project for their assistance with this project.
Disclosures: The authors acknowledge funding from the AHRQ/CERT 5 U18 HS016967‐01. The funder had no role in the design of the study; the collection, analysis, and interpretation of the data; or the decision to approve publication of the finished manuscript. Prior presentations of the data include the 2012 Department of Medicine Research Day at the University of Chicago, the 2012 Society of Hospital Medicine Annual Meeting in San Diego, California, and the 2012 Midwest Society of General Medicine Meeting in Chicago, Illinois. All coauthors have seen and agree with the contents of the manuscript. The submission was not under review by any other publication. The authors report no conflicts of interest.
Clinical supervision in graduate medical education (GME) emphasizes patient safety while promoting development of clinical expertise by allowing trainees progressive independence.[1, 2, 3] The importance of the balance between supervision and autonomy has been recognized by accreditation organizations, namely the Institute of Medicine and the Accreditation Council for Graduate Medical Education (ACGME).[4, 5] However, little is known of best practices in supervision, and the model of progressive independence in clinical training lacks empirical support.[3] Limited evidence suggests that enhanced clinical supervision may have positive effects on patient and education‐related outcomes.[6, 7, 8, 9, 10, 11, 12, 13, 14, 15] However, a more nuanced understanding of potential effects of enhanced supervision on resident autonomy and decision making is still required, particularly as preliminary work on increased on‐site hospitalist supervision has yielded mixed results.[16, 17, 18, 19]
Understanding how trainees are entrusted with autonomy will be integral to the ACGME's Next Accreditation System.[20] Entrustable Professional Activities are benchmarks by which resident readiness to progress through training will be judged.[21] The extent to which trainees are entrusted with autonomy is largely determined by the subjective assessment of immediate supervisors, as autonomy is rarely measured or quantified.[3, 22, 23] This judgment of autonomy, most frequently performed by ward attendings, may be subject to significant variation and influenced by factors other than the resident's competence and clinical abilities.
To that end, it is worth considering what factors may affect attending perception of housestaff autonomy and decision making. Recent changes in the GME environment and policy implementation have altered the landscape of the attending workforce considerably. The growth of the hospitalist movement in teaching hospitals, in part due to duty hours, has led to more residents being supervised by hospitalists, who may perceive trainee autonomy differently than other attendings do.[24] This study aims to examine whether factors such as attending demographics and short‐term and long‐term secular trends influence attending perception of housestaff autonomy and participation in decision making.
METHODS
Study Design
From 2001 to 2008, attending physicians at a single academic institution were surveyed at the end of inpatient general medicine teaching rotations.[25] The University of Chicago general medicine service consists of ward teams of an attending physician (internists, hospitalists, or subspecialists), 1 senior resident, and 1 or 2 interns. Attendings serve for 2‐ or 4‐week rotations. Attendings were consented for participation and received a 40‐item, paper‐based survey at the rotation's end. The institutional review board approved this study.
Data Collection
From the 40 survey items, 2 statements were selected for analysis: The intern(s) were truly involved in decision making about their patients and My resident felt that s/he had sufficient autonomy this month. These items have been used in previous work studying attending‐resident dynamics.[19, 26] Attendings also reported demographic and professional information as well as self‐identified hospitalist status, ascertained by the question Do you consider yourself to be a hospitalist? Survey month and year were also recorded. We conducted a secondary data analysis of an inclusive sample of responses to the questions of interest.
Statistical Analysis
Descriptive statistics were used to summarize survey responses and demographics. Survey questions consisted of Likert‐type items. Because the distribution of responses was skewed toward strong agreement for both questions, we collapsed scores into 2 categories (Strongly Agree and Do Not Strongly Agree).[19] Perception of sufficient trainee autonomy was defined as a response of Strongly Agree. The Pearson 2 test was used to compare proportions, and t tests were used to compare mean years since completion of residency and weeks on service between different groups.
Multivariate logistic regression with stepwise forward regression was used to model the relationship between attending sex, institutional hospitalist designation, years of experience, implementation of duty‐hours restrictions, and academic season, and perception of trainee autonomy and decision making. Academic seasons were defined as summer (JulySeptember), fall (OctoberDecember), winter (JanuaryMarch) and spring (AprilJune).[26] Years of experience were divided into tertiles of years since residency: 04 years, 511 years, and >11 years. To account for the possibility that the effect of hospitalist specialty varied by experience, interaction terms were constructed. The interaction term hospitalist*early‐career was used as the reference group.
RESULTS
Seven hundred thirty‐eight surveys were distributed to attendings on inpatient general medicine teaching services from 2001 to 2008; 70% (n=514) were included in the analysis. Table 1 provides demographic characteristics of the respondents. Roughly half (47%) were female, and 23% were hospitalists. Experience ranged from 0 to 35 years, with a median of 7 years. Weeks on service per year ranged from 1 to 27, with a median of 6 weeks. Hospitalists represented a less‐experienced group of attendings, as their mean experience was 4.5 years (standard deviation [SD] 4.5) compared with 11.2 years (SD 7.7) for nonhospitalists (P<0.001). Hospitalists attended more frequently, with a mean 14.2 weeks on service (SD 6.5) compared with 5.8 weeks (SD 3.4) for nonhospitalists (P<0.001). Nineteen percent (n=98) of surveys were completed prior to the first ACGME duty‐hours restriction in 2003. Responses were distributed fairly equally across the academic year, with 29% completed in summer, 26% in fall, 24% in winter, and 21% in spring.
Characteristics | Value |
---|---|
| |
Female, n (%) | 275 (47) |
Hospitalist, n (%) | 125 (23) |
Years since completion of residency | |
Mean, median, SD | 9.3, 7, 7.6 |
IQR | 314 |
04, n (%) | 167 (36) |
511, n (%) | 146 (32) |
>11, n (%) | 149 (32) |
Weeks on service per yearb | |
Mean, median, SD | 8.1, 6, 5.8 |
IQR | 412 |
Forty‐four percent (n=212) of attendings perceived adequate intern involvement in decision making, and 50% (n=238) perceived sufficient resident autonomy. The correlation coefficient between these 2 measures was 0.66.
Attending Factors Associated With Perception of Trainee Autonomy
In univariate analysis, hospitalists perceived sufficient trainee autonomy less frequently than nonhospitalists; 33% perceived adequate intern involvement in decision making compared with 48% of nonhospitalists (21=6.7, P=0.01), and 42% perceived sufficient resident autonomy compared with 54% of nonhospitalists (21=3.9, P=0.048) (Table 2).
Attending Characteristics, n (%) | Agree With Intern Involvement in Decision Making | Agree With Sufficient Resident Autonomy |
---|---|---|
| ||
Designation | ||
Hospitalist | 29 (33) | 37 (42) |
Nonhospitalist | 163 (48) | 180 (54) |
Years since completion of residency | ||
04 | 37 (27) | 49 (36) |
511 | 77 (53) | 88 (61) |
>11 | 77 (53) | 81 (56) |
Sex | ||
F | 98 (46) | 100 (47) |
M | 113 (43) | 138 (53) |
Secular factors, n (%) | ||
Pre‐2003 duty‐hours restrictions | 56 (57) | 62 (65) |
Post‐2003 duty‐hours restrictions | 156 (41) | 176 (46) |
Season of survey | ||
Summer (JulySeptember) | 61 (45) | 69 (51) |
Fall (OctoberDecember) | 53 (42) | 59 (48) |
Winter (JanuaryMarch) | 42 (37) | 52 (46) |
Spring (AprilJune) | 56 (54) | 58 (57) |
Perception of trainee autonomy increased with experience (Table 2). About 30% of early‐career attendings (04 years experience) perceived sufficient autonomy and involvement in decision making compared with >50% agreement in the later‐career tertiles (intern decision making: 22=25.1, P<0.001; resident autonomy: 22=18.9, P<0.001). Attendings perceiving more intern decision making involvement had a mean 11 years of experience (SD 7.1), whereas those perceiving less had a mean of 8.8 years (SD 7.8; P=0.003). Mean years of experience were similar for perception of resident autonomy (10.6 years [SD 7.2] vs 8.9 years [SD 7.8], P=0.021).
Sex was not associated with differences in perception of intern decision making (21=0.39, P=0.53) or resident autonomy (21=1.4, P=0.236) (Table 2).
Secular Factors Associated With Perception of Trainee Autonomy
The implementation of duty‐hour restrictions in 2003 was associated with decreased attending perception of autonomy. Only 41% of attendings perceived adequate intern involvement in decision making following the restrictions, compared with 57% before the restrictions were instituted (21=8.2, P=0.004). Similarly, 46% of attendings agreed with sufficient resident autonomy post‐duty hours, compared with 65% prior (21=10.1, P=0.001) (Table 2).
Academic season was also associated with differences in perception of autonomy (Table 2). In spring, 54% of attendings perceived adequate intern involvement in decision making, compared with 42% in the other seasons combined (21=5.34, P=0.021). Perception of resident autonomy was also higher in spring, though this was not statistically significant (57% in spring vs 48% in the other seasons; 21=2.37, P=0.123).
Multivariate Analyses
Variation in attending perception of housestaff autonomy by attending characteristics persisted in multivariate analysis. Table 3 shows ORs for perception of adequate intern involvement in decision making and sufficient resident autonomy. Sex was not a significant predictor of agreement with either statement. The odds that an attending would perceive adequate intern involvement in decision making were higher for later‐career attendings compared with early‐career attendings (ie, 04 years); attendings who completed residency 511 years ago were 2.16 more likely to perceive adequate involvement (OR: 2.16, 95% CI: 1.17‐3.97, P=0.013), and those >11 years from residency were 2.05 more likely (OR: 2.05, 95% CI: 1.16‐3.63, P=0.014). Later‐career attendings also had nonsignificant higher odds of perceiving sufficient resident autonomy compared with early‐career attendings (511 years, OR: 1.73, 95% CI: 0.963.14, P=0.07; >11 years, OR: 1.50, 95% CI: 0.862.62, P=0.154).
Interns Involved With Decision Making | Resident Had Sufficient Autonomy | |||
---|---|---|---|---|
| ||||
Covariate | OR (95% CI) | P Value | OR (95% CI) | P Value |
Attending characteristics | ||||
04 years of experience | ||||
511 years of experience | 2.16 (1.17‐3.97) | 0.013 | 1.73 (0.96‐3.14) | 0.07 |
>11 years of experience | 2.05 (1.16‐3.63) | 0.014 | 1.50 (0.86‐2.62) | 0.154 |
Hospitalist | 0.19 (0.06‐0.58) | 0.004 | 0.27 (0.11‐0.66) | 0.004 |
Hospitalist 04 years of experiencea | ||||
Hospitalist 511 years of experiencea | 7.36 (1.86‐29.1) | 0.004 | 5.85 (1.75‐19.6) | 0.004 |
Hospitalist >11 years of experiencea | 21.2 (1.73‐260) | 0.017 | 14.4 (1.31‐159) | 0.029 |
Female sex | 1.41 (0.92‐2.17) | 0.115 | 0.92 (0.60‐1.40) | 0.69 |
Secular factors | ||||
Post‐2003 duty hours | 0.51 (0.29‐0.87) | 0.014 | 0.49 (0.28‐0.86) | 0.012 |
Spring academic season | 1.94 (1.18‐3.19) | 0.009 | 1.59 (0.97‐2.60) | 0.064 |
Hospitalists were associated with 81% lower odds of perceiving adequate intern involvement in decision making (OR: 0.19, 95% CI: 0.060.58, P=0.004) and 73% lower odds of perceiving sufficient resident autonomy compared with nonhospitalists (OR: 0.27, 95% CI: 0.110.66, P=0.004). However, there was a significant interaction between hospitalists and experience; compared with early‐career hospitalists, experienced hospitalists had higher odds of perceiving both adequate intern involvement in decision making (511 years, OR: 7.36, 95% CI: 1.8629.1, P=0.004; >11 years, OR: 21.2, 95% CI: 1.73260, P=0.017) and sufficient resident autonomy (511 years, OR: 5.85, 95% CI: 1.7519.6, P=0.004; >11 years, OR: 14.4, 95% CI: 1.3159, P=0.029) (Table 3).
Secular trends also remained associated with differences in perception of housestaff autonomy (Table 3). Attendings had 49% lower odds of perceiving adequate intern involvement in decision making in the years following duty‐hour limits compared with the years prior (OR: 0.51, 95% CI: 0.29‐0.87, P=0.014). Similarly, odds of perceiving sufficient resident autonomy were 51% lower post‐duty hours (OR: 0.49, 95% CI: 0.280.86, P=0.012). Spring season was associated with 94% higher odds of perceiving adequate intern involvement in decision making compared with other seasons (OR: 1.94, 95% 1.183.19, P=0.009). There were also nonsignificant higher odds of perception of sufficient resident autonomy in spring (OR: 1.59, 95% CI: 0.972.60, P=0.064). To address the possibility of associations due to secular trends resulting from repeated measures of attendings, models using attending fixed effects were also used. Clustering by attending, the associations between duty hours and perceiving sufficient resident autonomy and intern decision making both remained significant, but the association of spring season did not.
DISCUSSION
This study highlights that attendings' perception of housestaff autonomy varies by attending characteristics and secular trends. Specifically, early‐career attendings and hospitalists were less likely to perceive sufficient housestaff autonomy and involvement in decision making. However, there was a significant hospitalist‐experience interaction, such that more‐experienced hospitalists were associated with higher odds of perceiving sufficient autonomy than would be expected from the effect of experience alone. With respect to secular trends, attendings perceived more trainee autonomy in the last quarter of the academic year, and less autonomy after implementation of resident duty‐hour restrictions in 2003.
As Entrustable Professional Activities unveil a new emphasis on the notion of entrustment, it will be critical to ensure that attending assessment of resident performance is uniform and a valid judge of when to entrust autonomy.[27, 28] If, as suggested by these findings, perception of autonomy varies based on attending characteristics, all faculty may benefit from strategies to standardize assessment and evaluation skills to ensure trainees are appropriately progressing through various milestones to achieve competence. Our results suggest that faculty development may be particularly important for early‐career attendings and especially hospitalists.
Early‐career attendings may perceive less housestaff autonomy due to a reluctance to relinquish control over patient‐care duties and decision making when the attending is only a few years from residency. Hospitalists are relatively junior in most institutions and may be similar to early‐career attendings in that regard. It is noteworthy, however, that experienced hospitalists are associated with even greater perception of autonomy than would be predicted by years of experience alone. Hospitalists may gain experience at a rate faster than nonhospitalists, which could affect how they perceive autonomy and decision making in trainees and may make them more comfortable entrusting autonomy to housestaff. Early‐career hospitalists likely represent a heterogeneous group of physicians, in both 1‐year clinical hospitalists as well as academic‐career hospitalists, who may have different approaches to managing housestaff teams. Residents are less likely to fear hospitalists limiting their autonomy after exposure to working with hospitalists as teaching attendings, and our findings may suggest a corollary in that hospitalists may be more likely to perceive sufficient autonomy with more exposure to working with housestaff.[19]
Attendings perceived less housestaff autonomy following the 2003 duty‐hour limits. This may be due to attendings assuming more responsibilities that were traditionally performed by residents.[26, 29] This shifting of responsibility may lead to perception of less‐active housestaff decision making and less‐evident autonomy. These findings suggest autonomy may become even more restricted after implementation of the 2011 duty‐hour restrictions, which included 16‐hour shifts for interns.[5] Further studies are warranted in examining the effect of these new limits. Entrustment of autonomy and allowance for decision making is an essential part of any learning environment that allows residents to develop clinical reasoning skills, and it will be critical to adopt new strategies to encourage professional growth of housestaff in this new era.[30]
Attendings also perceived autonomy differently by academic season. Spring represents the season by which housestaff are most experienced and by which attendings may be most familiar with individual team members. Additionally, there may be a stronger emphasis on supervision and adherence to traditional hierarchy earlier in the academic year as interns and junior residents are learning their new roles.[30] These findings may have implications for system changes to support development of more functional educational dyads between attendings and trainees, especially early in the academic year.[31]
There are several limitations to our findings. This is a single‐institution study restricted to the general‐medicine service; thus generalizability is limited. Our outcome measures, the survey items of interest, question perception of housestaff autonomy but do not query the appropriateness of that autonomy, an important construct in entrustment. Additionally, self‐reported answers could be subject to recall bias. Although data were collected over 8 years, the most recent trends of residency training are not reflected. Although there was a significant interaction involving experienced hospitalists, wide confidence intervals and large standard errors likely reflect the relatively few individuals in this category. Though there was a large number of overall respondents, our interaction terms included few advanced‐career hospitalists, likely secondary to hospital medicine's relative youth as a specialty.
As this study focuses only on perception of autonomy, future work must investigate autonomy from a practical standpoint. It is conceivable that if factors such as attending characteristics and secular trends influence perception, they may also be associated with variation in how attendings entrust autonomy and provide supervision. To what extent perception and practice are linked remains to be studied, but it will be important to determine if variation due to these factors may also be associated with inconsistent and uneven supervisory practices that would adversely affect resident education and patient safety.
Finally, future work must include the viewpoint of the recipients of autonomy: the residents and interns. A significant limitation of the current study is the lack of the resident perspective, as our survey was only administered to attendings. Autonomy is clearly a 2‐way relationship, and attending perception must be corroborated by the resident's experience. It is possible attendings may perceive that their housestaff have sufficient autonomy, but residents may view this autonomy as inappropriate or unavoidable due an absentee attending who does not adequately supervise.[32] Future work must examine how resident and attending perceptions of autonomy correlate, and whether discordance or concordance in these perceptions influence satisfaction with attending‐resident relationships, education, and patient care.
In conclusion, significant variation existed among attending physicians with respect to perception of housestaff autonomy, an important aspect of entrustment and clinical supervision. This variation was present for hospitalists, among different levels of attending experience, and a significant interaction was found between these 2 factors. Additionally, secular trends were associated with differences in perception of autonomy. As entrustment of residents with progressive levels of autonomy becomes more integrated within the requirements for advancement in residency, a greater understanding of factors affecting entrustment will be critical in helping faculty develop skills to appropriately assess trainee professional growth and development.
Acknowledgments
The authors thank all members of the Multicenter Hospitalist Project for their assistance with this project.
Disclosures: The authors acknowledge funding from the AHRQ/CERT 5 U18 HS016967‐01. The funder had no role in the design of the study; the collection, analysis, and interpretation of the data; or the decision to approve publication of the finished manuscript. Prior presentations of the data include the 2012 Department of Medicine Research Day at the University of Chicago, the 2012 Society of Hospital Medicine Annual Meeting in San Diego, California, and the 2012 Midwest Society of General Medicine Meeting in Chicago, Illinois. All coauthors have seen and agree with the contents of the manuscript. The submission was not under review by any other publication. The authors report no conflicts of interest.
- Effective supervision in clinical practice settings: a literature review. Med Educ. 2000;34(10):827–840. , .
- Deliberate practice and acquisition of expert performance: a general overview. Acad Emerg Med. 2008;15(11):988–994. .
- Progressive independence in clinical training: a tradition worth defending? Acad Med. 2005;80(10 suppl):S106–S111. , , , et al.
- Committee on Optimizing Graduate Medical Trainee (Resident) Hours and Work Schedules to Improve Patient Safety, Institute of Medicine. Ulmer C, Wolman D, Johns M, eds. Resident Duty Hours: Enhancing Sleep, Supervision, and Safety. Washington, DC: National Academies Press; 2008.
- ACGME Duty Hour Task Force. The new recommendations on duty hours from the ACGME Task Force. N Engl J Med. 2010;363(2):e3. , , ;
- Positive impact of pediatric critical care fellows on mortality: is it merely a function of resident supervision? Crit Care Med. 1997;25(10):1622–1623. .
- The effect of supervision of residents on quality of care in five university‐affiliated emergency departments. Acad Med. 1998;73(7):776–782. , , , et al.
- Increased faculty presence on inpatient teaching services. Mayo Clin Proc. 2004;79(3):332–336. , , , et al.
- How medical residents perceive the quality of supervision provided by attending doctors in the clinical setting. Med Educ. 2005;39(7):696–703. , , , et al.
- Resident supervision in the operating room: does this impact on outcome? J Trauma. 1993;35(4):556–560. , , .
- Effects of supervision by attending anesthesiologists on complications of emergency tracheal intubation. Anesthesiology. 2008;109(6):973–937. , , , et al.
- Around‐the‐clock attending radiology coverage is essential to avoid mistakes in the care of trauma patients. Am Surg. 2001;67(12):1175–1177. , , , et al.
- Supervision in the outpatient clinic: effects on teaching and patient care. J Gen Int Med. 1993;8(7):378–380. , .
- How medical students and residents describe the roles and characteristics of their influential clinical teachers. Acad Med. 2000;75(8):843–845. , .
- A systematic review: the effect of clinical supervision on patient and residency education outcomes. Acad Med. 2012;87(4):428–442. , , , et al.
- for the SGIM Housestaff Oversight Subcommittee. Survey of overnight academic hospitalist supervision of trainees. J Hosp Med. 2012;7(7):521–523. , , , et al;
- Effects of increased overnight supervision on resident education, decision‐making, and autonomy. J Hosp Med. 2012;7(8):606–610. , , , et al.
- The effect of overnight in‐house attending coverage on perceptions of care and education on a general medical service. J Grad Med Educ. 2010;2(1):53–56. , , , et al.
- Resident satisfaction on an academic hospitalist service: time to teach. Am J Med. 2002;112(7):597–601. , , , et al.
- The next GME accreditation system—rationale and benefits. N Engl J Med. 2012;366(11):1051–1056. , , , et al.
- Competency‐based postgraduate training: can we bridge the gap between theory and clinical practice? Acad Med. 2007;82(6):542–547. , .
- Trust, competence, and the supervisor's role in postgraduate training. BMJ. 2006;333(7571):748–751. .
- Measuring progressive independence with the resident supervision index: empirical approach. J Grad Med Educ. 2010;2(1):17–30. , , , et al.
- The emerging role of “hospitalists” in the American health care system. N Engl J Med. 1996;335(7):514–517. , .
- Effect of ACGME duty hours on attending physician teaching and satisfaction. Arch Intern Med. 2008;168(11):1226–1227. , .
- Association of workload of on‐call interns with on‐call sleep duration, shift duration, and participation in educational activities. JAMA. 2008;300(10):1146–1153. , , , et al.
- Entrustability of professional activities and competency‐based training. Med Educ. 2005;39:1176–1177. .
- When do supervising physicians decide to entrust residents with unsupervised tasks? Acad Med. 2010;85(9):1399–1400. , , , et al.
- Effect of residency duty‐hour limits. Arch Intern Med. 2007;167(14):1487–1492. , , et al.
- Strategies for improving teaching practices: a comprehensive approach to faculty development. Acad Med. 1998;73:387–396. , .
- A framework for effective training for supervisors. Med Teach. 2002;24:385–389. , , .
- On‐call supervision and resident autonomy: from micromanager to absentee attending. Am J Med. 2009;122(8):784–788. , , , et al.
- Effective supervision in clinical practice settings: a literature review. Med Educ. 2000;34(10):827–840. , .
- Deliberate practice and acquisition of expert performance: a general overview. Acad Emerg Med. 2008;15(11):988–994. .
- Progressive independence in clinical training: a tradition worth defending? Acad Med. 2005;80(10 suppl):S106–S111. , , , et al.
- Committee on Optimizing Graduate Medical Trainee (Resident) Hours and Work Schedules to Improve Patient Safety, Institute of Medicine. Ulmer C, Wolman D, Johns M, eds. Resident Duty Hours: Enhancing Sleep, Supervision, and Safety. Washington, DC: National Academies Press; 2008.
- ACGME Duty Hour Task Force. The new recommendations on duty hours from the ACGME Task Force. N Engl J Med. 2010;363(2):e3. , , ;
- Positive impact of pediatric critical care fellows on mortality: is it merely a function of resident supervision? Crit Care Med. 1997;25(10):1622–1623. .
- The effect of supervision of residents on quality of care in five university‐affiliated emergency departments. Acad Med. 1998;73(7):776–782. , , , et al.
- Increased faculty presence on inpatient teaching services. Mayo Clin Proc. 2004;79(3):332–336. , , , et al.
- How medical residents perceive the quality of supervision provided by attending doctors in the clinical setting. Med Educ. 2005;39(7):696–703. , , , et al.
- Resident supervision in the operating room: does this impact on outcome? J Trauma. 1993;35(4):556–560. , , .
- Effects of supervision by attending anesthesiologists on complications of emergency tracheal intubation. Anesthesiology. 2008;109(6):973–937. , , , et al.
- Around‐the‐clock attending radiology coverage is essential to avoid mistakes in the care of trauma patients. Am Surg. 2001;67(12):1175–1177. , , , et al.
- Supervision in the outpatient clinic: effects on teaching and patient care. J Gen Int Med. 1993;8(7):378–380. , .
- How medical students and residents describe the roles and characteristics of their influential clinical teachers. Acad Med. 2000;75(8):843–845. , .
- A systematic review: the effect of clinical supervision on patient and residency education outcomes. Acad Med. 2012;87(4):428–442. , , , et al.
- for the SGIM Housestaff Oversight Subcommittee. Survey of overnight academic hospitalist supervision of trainees. J Hosp Med. 2012;7(7):521–523. , , , et al;
- Effects of increased overnight supervision on resident education, decision‐making, and autonomy. J Hosp Med. 2012;7(8):606–610. , , , et al.
- The effect of overnight in‐house attending coverage on perceptions of care and education on a general medical service. J Grad Med Educ. 2010;2(1):53–56. , , , et al.
- Resident satisfaction on an academic hospitalist service: time to teach. Am J Med. 2002;112(7):597–601. , , , et al.
- The next GME accreditation system—rationale and benefits. N Engl J Med. 2012;366(11):1051–1056. , , , et al.
- Competency‐based postgraduate training: can we bridge the gap between theory and clinical practice? Acad Med. 2007;82(6):542–547. , .
- Trust, competence, and the supervisor's role in postgraduate training. BMJ. 2006;333(7571):748–751. .
- Measuring progressive independence with the resident supervision index: empirical approach. J Grad Med Educ. 2010;2(1):17–30. , , , et al.
- The emerging role of “hospitalists” in the American health care system. N Engl J Med. 1996;335(7):514–517. , .
- Effect of ACGME duty hours on attending physician teaching and satisfaction. Arch Intern Med. 2008;168(11):1226–1227. , .
- Association of workload of on‐call interns with on‐call sleep duration, shift duration, and participation in educational activities. JAMA. 2008;300(10):1146–1153. , , , et al.
- Entrustability of professional activities and competency‐based training. Med Educ. 2005;39:1176–1177. .
- When do supervising physicians decide to entrust residents with unsupervised tasks? Acad Med. 2010;85(9):1399–1400. , , , et al.
- Effect of residency duty‐hour limits. Arch Intern Med. 2007;167(14):1487–1492. , , et al.
- Strategies for improving teaching practices: a comprehensive approach to faculty development. Acad Med. 1998;73:387–396. , .
- A framework for effective training for supervisors. Med Teach. 2002;24:385–389. , , .
- On‐call supervision and resident autonomy: from micromanager to absentee attending. Am J Med. 2009;122(8):784–788. , , , et al.
Copyright © 2013 Society of Hospital Medicine
Provider Expectations and Experiences
Comanagement is common in hospital medicine practice. And yet, there is no consensus about how comanagement is different from traditional consultative practice. At its core, hospitalist comanagement is a practice arrangement wherein hospitalists and other specialists manage complex patients collaboratively. Beyond this, Huddleston et al. distinguish comanagement from traditional consultations in the comanaging hospitalists' prerogative to provide direct medical care in addition to consultative advice.1 Siegal focuses on the shared responsibility and authority among partnering providers in the comanagement model.2 Whinney and Michota see comanagement as patient care referral at the onset of a care episode, in contrast to consultations that are activated to address emergent problems.3 In a recent study that found the growing adoption of medical comanagement in Medicare beneficiaries (as much as 40% of surgical hospitalizations in 2006), comanagement was defined as an intensive form of consultation involving a claim for evaluation and management services on greater than 70% of inpatient days.4
In addition to the intensity, frequency, timing, responsibility, and authority of care, comanagement may be described by participating physicians' roles. With recent attention on multidisciplinary teams and an increasing focus on collaborative care, many of the hierarchical relations among healthcare providers are breaking down.5 Several studies of multidisciplinary teams suggest that more egalitarian, rather than hierarchical, problem‐solving and decision‐making among team members are beneficial to patients.67 However, neither the intended nor natural team structure under comanagement is known. We sought to shed some light on provider interactions by characterizing the expectations and experiences of providers of a comanaged service. The findings yielded an opportunity to generate an evolving, but conceptually supported definition of comanagement.
SETTING
We conducted a survey study of providers participating in a comanaged inpatient hepatology service at the University of Chicago Medical Center, a 572‐bed urban teaching hospital. The service was created in 2006, partly to address staffing problems related to housestaff work hour restrictions and partly to improve the care of candidates and recipients of liver transplantation. Nonsurgical floor patients with liver diseases were managed on the service by two collaborating teams of providers. The hepatology team consisted of an attending physician and a fellow, while the hospitalist team consisted of a hospitalist and one or two nonphysician providers (physician assistant or nurse practitioner). The practice model is characterized as comanagement because of the highly interdependent nature of the team's daily tasks and the norms of intensive communication, through formal joint daily rounds and informal direct exchanges of instructions and updates. Hepatologists were mainly responsible for coordinating admissions, managing issues related to liver dysfunction, communicating with transplant surgeons if necessary, and arranging postdischarge care. Hospitalists were responsible for admitting patients, managing routine (eg, ordering daily labs) and urgent issues (eg, responding to critical lab values) during hospitalizations, coordinating with ancillary and consultative staff, and discharging patients. Occasional meetings between the hepatology and hospital medicine groups were used to clarify assignment of responsibilities. Floor nurses received in‐servicing at the commencement of the service. Additional details about the service are described elsewhere.8
DATA COLLECTION AND ANALYSIS
For the purpose of our analysis, we defined interactions between any member of the hospitalist and hepatologist teams as pertinent to comanagement. The hospitalist nonphysician provider (NPP) and hepatologistfellow relationships are governed by the more traditional hierarchical dynamics based on supervision and authority according to laws and regulations. At the beginning of the study period, each participant completed nine items of a Baseline Survey that addressed respondents' expectations and preferences for the management of an ideally comanaged service. Responses were solicited using a 4‐point Likert‐type scale and were dichotomized such that agree and somewhat agree were grouped, while disagree and somewhat disagree were grouped for data analysis. Items were generated to address the salient issues of comanagement after reviewing the pertinent literature.
Subsequently, participants were asked to complete Repeated Surveys immediately before each change in membership of the comanaged team between April and October 2008. The surveys were hand delivered by one of the authors (K.H.) on the last day of each team's rotation and were often completed immediately. The seven items of the Repeated Survey reprised items from the Baseline Survey that were rephrased to allow respondents to report their direct experiences on specific teams. Because all providers rotated on the service more than once during the study period, the average value for each Likert‐type response across multiple surveys completed by a single provider was calculated before being dichotomized at the midpoint (<2.5, agree; 2.5, disagree). We reported proportions of respondents in agreement with survey item statements.
Comparison statistics across providers were generated using the chi‐square test. Differences in proportions between related items of the Baseline and Repeated Surveys were compared using the two‐sample test of proportions. All analyses were conducted using a statistics application (STATA 10.0, College Station, TX) with alpha equal to, or less than, 0.05 considered significant. The Institutional Review Board of the University of Chicago approved this project.
RESULTS
All 43 providers completed the Baseline Survey. During the study period, 32 of these participants rotated on the service and completed 177 of the 233 Repeated Surveys (79%) administered. The responses describe team interactions on the 47 unique combinations of providers comprising the comanaged teams. Details of the response rates are shown in Table 1.
Baseline Survey, Completed/ Administered (%) | Repeated Surveys, Completed/ Administered (%) | Respondents Completing Repeated Surveys, n | Repeated Surveys Completed per Respondent, Median (IQR) | |
---|---|---|---|---|
| ||||
Hospitalists | 18/18 (100) | 36/43 (84) | 15 | 2 (2, 3) |
NPPs | 5/5 (100) | 92/97 (95) | 5 | 20 (18, 20) |
Hepatologists | 6/6 (100) | 26/42 (62) | 6 | 7 (3.75, 8) |
Fellows | 12/12 (100) | 23/42 (55) | 6 | 7 (5.5, 8.5) |
Total | 43/43 (100) | 177/223 (79) | 32 | 4.5 (2, 8.25) |
As shown in Table 2A, items 13, more members of the hospitalist team preferred to be informed about every management decision compared to members of the hepatologist team. Conversely, more of members of the hepatologist team than the hospitalist team preferred their comanaging partners to participate in every decision. A statistically similar proportion of respondents in each of the professional roles indicated desire for greater influence in directing management decisions (Table 2B, item 1).
A. Baseline Survey | Hospitalists, % (n = 18) | NPPs, % (n = 5) | Hepatologists, % (n = 6) | GI Fellows, % (n = 12) | P‐value |
---|---|---|---|---|---|
| |||||
1. I prefer to be informed about every decision. | 83 | 100 | 17 | 42 | <0.01 |
2. I prefer to participate in every decision. | 67 | 100 | 33 | 50 | 0.11 |
3. I prefer that my comanager participate in every decision. | 22 | 20 | 50 | 75 | 0.02 |
4. I prefer to have the final say in every decision. | 50 | 80 | 50 | 33 | 0.38 |
5. There should be one physician leader to direct the overall management of the patients' hospital course. | 89* | 100 | 67 | 83 | 0.43 |
6. Physician consensus should always be sought in every clinical decision. | 22 | 40 | 50 | 67 | 0.11 |
7. I have a clear understanding of my role on the comanagement service. | 61 | 80 | 83 | 75 | 0.66 |
8. I have as much a sense of ownership of patients on the comanaged service as on a non‐comanaged service. | 61 | 60 | 83 | 50 | 0.60 |
9. Comanagement tends to improve patient care. | 94 | 100* | 83 | 100* | 0.47 |
B. Repeated Surveys | Hospitalists, % (n = 15) | NPPs, % (n = 5) | Hepatologists, % (n = 6) | GI Fellows, % (n = 6) | P‐value |
1. I would have liked greater influence in directing the overall management. | 40 | 60 | 0 | 17 | 0.12 |
2. I was responsible for work in clinical areas I was not comfortable managing. | 0 | 0 | 0 | 0 | NA |
3. There was one physician leader to direct the overall management of the patients' hospital course. | 60* | 80 | 67 | 83 | 0.70 |
4. Physician consensus was always sought in every clinical decision. | 40 | 40 | 50 | 67 | 0.72 |
5. I (have/had) a clear understanding of my role on the comanagement service. | 73 | 80 | 100 | 83 | 0.57 |
6. I had as much a sense of ownership of patients on the comanaged service as on a non‐comanaged service. | 53 | 80 | 100 | 67 | 0.20 |
7. Patients on my service received better care than they would have without comanagement. | 93 | 40* | 67 | 50* | 0.06 |
For the majority of surveyed areas, there was concordance between expectations and experiences of providers on comanagement. Most providers, regardless of professional role, agreed that there should be a single physician leader to direct the overall management (Table 2A, item 5). The majority perceived that a single physician directed the overall management of the patients' hospital course, although fewer hospitalists did so compared with baseline expectations (Table 2B, item 3). Many respondents felt at baseline that physician consensus should govern every management decision, and a similar proportion actually experienced consensus‐seeking on service.
We found that the proportion of providers reporting an understanding of their role increased slightly, though not significantly, from before (Table 2A, item 7) to after rotating on the comanaged service (Table 2B, item 5). Although not statistically significant, there was a trend towards hospitalists and gastrointestinal (GI) fellows reporting a lack of patient ownership, both before and after serving on the comanaged service. Finally, nearly all respondents reported that comanagement should improve care quality, although only the attending hospitalist and hepatologist felt that their experience on the comanaged service actually improved patient care (Table 2B, item 7).
DISCUSSION
In this survey of providers participating on a comanaged medical service, most reported understanding their role in the collaborative arrangement and had an initial perception that comanagement should improve patient care quality. We found that hospitalists preferred and were expected to participate in care globally, while hepatologists themselves preferred and were expected not to focus on every management decision. The prevalence of desire for ultimate authority across the professional roles suggests tensions that exist in this care model around how decisions are made. The majority of providers preferred and experienced a single physician leader under comanagement, but many also experienced consensus‐seeking for every management decision.
From these findings, we conclude that decision‐making processes are not uniform under comanagement and that some role ambiguity is present, but there appears to be a pattern of natural roles. This pattern can be defined by focus (general for hospitalists vs specialty‐specific for hepatologists), rather than by responsibilities for managing particular medical problems. The preference among both generalists and specialists for the broader involvement of hospitalist comanagers suggests an implicit recognition of the need for integrated management to overcome the silo‐effect within the comanagement structure.9 Although details about how such integration was achieved are not available in our data, we found that comanagement may be distinct from traditional consultative practice in that the consultants (hospitalists in this case) manage not only general medical problems, such as diabetes or hypertension, but hospitalizations more generally. From a mission‐based standpoint, comanagement may be seen as a collaborative management of complex patients by two or more clinical experts with distinct knowledge, skills, or focus enacted for the purpose of improving care quality.
The focus of comanagement on improving quality is in line with the founding charge of the hospital medicine specialty to raise hospital care quality.10 In fact, the distinction between comanagement and consultation may be meaningful only if comanagers can work with specialists to implement evidence‐based practice, process improvement, and address quality and cost concerns. But as seen in NPPs and fellows' skepticism of improved quality under comanagement, there is still clearly work to be done to validate this model through measurable improvement in patients' experiences and outcomes. Proving the advantages of comanagement as a platform for practice improvement remains future work.11
Collaborative arrangements create natural tensions related to team function.5 This is seen in the similar proportion of hospitalists and hepatologists indicating desire for final decision‐making authority. Although comanagement evokes assumptions about egalitarian provider interactions involving shared decision‐making and responsibility, it seems to function empirically under hierarchical as well as consensus‐seeking forms of decision‐making. Providers at the top of hierarchical teams typically experience their work as interdependent and collaborative, and report more positive interactions with other care providers.12 Based on the fact that no hepatologists wanted more influence over decision‐making, we assume that hepatologists were the physician leaders for most of the studied comanaged teams. Under situations characterized by high levels of complexity and interdependence, a team governed by a single leader may often be more effective than one governed by shared authority.8 However, even under hierarchical models, a more participatory than supervisory leadership can help avoid alienating partners through a pattern of we decide, you carry it out that is often associated with ineffective leadership styles.1314 In fact, this alienating effect on providers in subordinate roles (ie, NPPs and fellows) may have contributed to the negative perception of the team's function on improving patient care.
This study is limited in the following ways. We did not have 100% participation in the Repeated Surveys. Attitudes and experiences of participants in a single comanagement practice are not representative of all comanaging providers. However, the goal of this studyto collect unique survey data from providers themselves to inform an evolving definition of comanagementis modest enough in scope to not require a generalizable sample. Because this study unearthed differences in expectations and experiences within a single site, they may serve as a lower bound for the extent of differences across and within multiple sites. In addition, comanagement enacted for complex medical patients is not as common as the comanagement of surgical patients. Moreover, comanagement models in academic hospitals may have structural features and priorities not found in community settings. Whether or not these disparate models share enough in common to be categorized under a single rubric is a valid question.
Although the teamwork structure and provider roles within comanagement vary, the practice arrangement's preoccupation with quality can be seen as its defining feature. Limited evidence, to date,1, 1519 and the rapid proliferation of the model, suggest that quality and efficiency advantages can be obtained from an effective implementation of comanagement. As in any team‐based care model, a common understanding of roles and expectations are essential to enhancing teamwork. Our interpretation of the mission of comanagement may further enhance teamwork through an explicit articulation of shared goals.
- Medical and surgical comanagement after elective hip and knee arthroplasty: A randomized, controlled trial.Ann Intern Med.2004;141(1):28–38. , , , et al.
- Just because you can, doesn't mean that you should: A call for the rational application of hospitalist comanagement.J Hosp Med.2008;3(5):398–402. .
- Surgical comanagement: A natural evolution of hospitalist practice.J Hosp Med.2008;3(5):394–397. , .
- Comanagement of hospitalized surgical patients by medicine physicians in the United States.Arch Intern Med.2010;170(4):363–368. , , , , .
- Structure and meaning in multidisciplinary teamwork.Sociol Health Illn.1998;20(6):848–873. .
- Human factors and cardiac surgery: A multicenter study.J Thorac Cardiov Surg.2000;119(4):661–670. , , , , .
- The effects of a collaborative model of primary care on the mortality and hospital use of community‐dwelling older adults.J Gerontol A‐Biol.2001;56(2):M106–M112. , , .
- Effects of provider characteristics on care coordination under comanagement.J Hosp Med.2010;5:508–513. , , , , , .
- Crossing the Quality Chasm: A New Health System for the Twenty‐First Century.Washington, DC:Institute of Medicine;2001. , , .
- The emerging role of “hospitalists” in the American health care system.N Engl J Med.1996;335(7):514–517. , .
- Internal medicine comanagement of surgical patients: Can we afford to do this?Arch Intern Med.2010;170(22):1965–1966. .
- Operating room teamwork among physicians and nurses: Teamwork in the eye of the beholder.J Am Coll Surg.2006;202(5):746–752. , , , et al.
- “We decide, you carry it out”: A social network analysis of multidisciplinary longterm care teams.Soc Sci Med.1997;45(9):1411–1421. .
- Patterns of aggressive behavior in experimentally created social climates.J Soc Psychol.1939;10:271–301. , , .
- Comanagement of surgical patients between neurosurgeons and hospitalists.Arch Intern Med.2010;170(22):2004–2010. , , , et al.
- Outcomes for older patients with hip fractures: The impact of orthopedic and geriatric medicine cocare.J Orthop Trauma.2006;20(3):172–180. , , , , , .
- Effects of a hospitalist model on elderly patients with hip fracture.Arch Intern Med.2005;165(7):796–801. , , , et al.
- Hip fractures in geriatric patients. Results of an interdisciplinary hospital care program.Clin Orthop Relat Res.1992(274):213–225. , , , .
- Impact of a comanaged Geriatric Fracture Center on short‐term hip fracture outcomes.Arch Intern Med.2009;169(18):1712–1717. , , , .
Comanagement is common in hospital medicine practice. And yet, there is no consensus about how comanagement is different from traditional consultative practice. At its core, hospitalist comanagement is a practice arrangement wherein hospitalists and other specialists manage complex patients collaboratively. Beyond this, Huddleston et al. distinguish comanagement from traditional consultations in the comanaging hospitalists' prerogative to provide direct medical care in addition to consultative advice.1 Siegal focuses on the shared responsibility and authority among partnering providers in the comanagement model.2 Whinney and Michota see comanagement as patient care referral at the onset of a care episode, in contrast to consultations that are activated to address emergent problems.3 In a recent study that found the growing adoption of medical comanagement in Medicare beneficiaries (as much as 40% of surgical hospitalizations in 2006), comanagement was defined as an intensive form of consultation involving a claim for evaluation and management services on greater than 70% of inpatient days.4
In addition to the intensity, frequency, timing, responsibility, and authority of care, comanagement may be described by participating physicians' roles. With recent attention on multidisciplinary teams and an increasing focus on collaborative care, many of the hierarchical relations among healthcare providers are breaking down.5 Several studies of multidisciplinary teams suggest that more egalitarian, rather than hierarchical, problem‐solving and decision‐making among team members are beneficial to patients.67 However, neither the intended nor natural team structure under comanagement is known. We sought to shed some light on provider interactions by characterizing the expectations and experiences of providers of a comanaged service. The findings yielded an opportunity to generate an evolving, but conceptually supported definition of comanagement.
SETTING
We conducted a survey study of providers participating in a comanaged inpatient hepatology service at the University of Chicago Medical Center, a 572‐bed urban teaching hospital. The service was created in 2006, partly to address staffing problems related to housestaff work hour restrictions and partly to improve the care of candidates and recipients of liver transplantation. Nonsurgical floor patients with liver diseases were managed on the service by two collaborating teams of providers. The hepatology team consisted of an attending physician and a fellow, while the hospitalist team consisted of a hospitalist and one or two nonphysician providers (physician assistant or nurse practitioner). The practice model is characterized as comanagement because of the highly interdependent nature of the team's daily tasks and the norms of intensive communication, through formal joint daily rounds and informal direct exchanges of instructions and updates. Hepatologists were mainly responsible for coordinating admissions, managing issues related to liver dysfunction, communicating with transplant surgeons if necessary, and arranging postdischarge care. Hospitalists were responsible for admitting patients, managing routine (eg, ordering daily labs) and urgent issues (eg, responding to critical lab values) during hospitalizations, coordinating with ancillary and consultative staff, and discharging patients. Occasional meetings between the hepatology and hospital medicine groups were used to clarify assignment of responsibilities. Floor nurses received in‐servicing at the commencement of the service. Additional details about the service are described elsewhere.8
DATA COLLECTION AND ANALYSIS
For the purpose of our analysis, we defined interactions between any member of the hospitalist and hepatologist teams as pertinent to comanagement. The hospitalist nonphysician provider (NPP) and hepatologistfellow relationships are governed by the more traditional hierarchical dynamics based on supervision and authority according to laws and regulations. At the beginning of the study period, each participant completed nine items of a Baseline Survey that addressed respondents' expectations and preferences for the management of an ideally comanaged service. Responses were solicited using a 4‐point Likert‐type scale and were dichotomized such that agree and somewhat agree were grouped, while disagree and somewhat disagree were grouped for data analysis. Items were generated to address the salient issues of comanagement after reviewing the pertinent literature.
Subsequently, participants were asked to complete Repeated Surveys immediately before each change in membership of the comanaged team between April and October 2008. The surveys were hand delivered by one of the authors (K.H.) on the last day of each team's rotation and were often completed immediately. The seven items of the Repeated Survey reprised items from the Baseline Survey that were rephrased to allow respondents to report their direct experiences on specific teams. Because all providers rotated on the service more than once during the study period, the average value for each Likert‐type response across multiple surveys completed by a single provider was calculated before being dichotomized at the midpoint (<2.5, agree; 2.5, disagree). We reported proportions of respondents in agreement with survey item statements.
Comparison statistics across providers were generated using the chi‐square test. Differences in proportions between related items of the Baseline and Repeated Surveys were compared using the two‐sample test of proportions. All analyses were conducted using a statistics application (STATA 10.0, College Station, TX) with alpha equal to, or less than, 0.05 considered significant. The Institutional Review Board of the University of Chicago approved this project.
RESULTS
All 43 providers completed the Baseline Survey. During the study period, 32 of these participants rotated on the service and completed 177 of the 233 Repeated Surveys (79%) administered. The responses describe team interactions on the 47 unique combinations of providers comprising the comanaged teams. Details of the response rates are shown in Table 1.
Baseline Survey, Completed/ Administered (%) | Repeated Surveys, Completed/ Administered (%) | Respondents Completing Repeated Surveys, n | Repeated Surveys Completed per Respondent, Median (IQR) | |
---|---|---|---|---|
| ||||
Hospitalists | 18/18 (100) | 36/43 (84) | 15 | 2 (2, 3) |
NPPs | 5/5 (100) | 92/97 (95) | 5 | 20 (18, 20) |
Hepatologists | 6/6 (100) | 26/42 (62) | 6 | 7 (3.75, 8) |
Fellows | 12/12 (100) | 23/42 (55) | 6 | 7 (5.5, 8.5) |
Total | 43/43 (100) | 177/223 (79) | 32 | 4.5 (2, 8.25) |
As shown in Table 2A, items 13, more members of the hospitalist team preferred to be informed about every management decision compared to members of the hepatologist team. Conversely, more of members of the hepatologist team than the hospitalist team preferred their comanaging partners to participate in every decision. A statistically similar proportion of respondents in each of the professional roles indicated desire for greater influence in directing management decisions (Table 2B, item 1).
A. Baseline Survey | Hospitalists, % (n = 18) | NPPs, % (n = 5) | Hepatologists, % (n = 6) | GI Fellows, % (n = 12) | P‐value |
---|---|---|---|---|---|
| |||||
1. I prefer to be informed about every decision. | 83 | 100 | 17 | 42 | <0.01 |
2. I prefer to participate in every decision. | 67 | 100 | 33 | 50 | 0.11 |
3. I prefer that my comanager participate in every decision. | 22 | 20 | 50 | 75 | 0.02 |
4. I prefer to have the final say in every decision. | 50 | 80 | 50 | 33 | 0.38 |
5. There should be one physician leader to direct the overall management of the patients' hospital course. | 89* | 100 | 67 | 83 | 0.43 |
6. Physician consensus should always be sought in every clinical decision. | 22 | 40 | 50 | 67 | 0.11 |
7. I have a clear understanding of my role on the comanagement service. | 61 | 80 | 83 | 75 | 0.66 |
8. I have as much a sense of ownership of patients on the comanaged service as on a non‐comanaged service. | 61 | 60 | 83 | 50 | 0.60 |
9. Comanagement tends to improve patient care. | 94 | 100* | 83 | 100* | 0.47 |
B. Repeated Surveys | Hospitalists, % (n = 15) | NPPs, % (n = 5) | Hepatologists, % (n = 6) | GI Fellows, % (n = 6) | P‐value |
1. I would have liked greater influence in directing the overall management. | 40 | 60 | 0 | 17 | 0.12 |
2. I was responsible for work in clinical areas I was not comfortable managing. | 0 | 0 | 0 | 0 | NA |
3. There was one physician leader to direct the overall management of the patients' hospital course. | 60* | 80 | 67 | 83 | 0.70 |
4. Physician consensus was always sought in every clinical decision. | 40 | 40 | 50 | 67 | 0.72 |
5. I (have/had) a clear understanding of my role on the comanagement service. | 73 | 80 | 100 | 83 | 0.57 |
6. I had as much a sense of ownership of patients on the comanaged service as on a non‐comanaged service. | 53 | 80 | 100 | 67 | 0.20 |
7. Patients on my service received better care than they would have without comanagement. | 93 | 40* | 67 | 50* | 0.06 |
For the majority of surveyed areas, there was concordance between expectations and experiences of providers on comanagement. Most providers, regardless of professional role, agreed that there should be a single physician leader to direct the overall management (Table 2A, item 5). The majority perceived that a single physician directed the overall management of the patients' hospital course, although fewer hospitalists did so compared with baseline expectations (Table 2B, item 3). Many respondents felt at baseline that physician consensus should govern every management decision, and a similar proportion actually experienced consensus‐seeking on service.
We found that the proportion of providers reporting an understanding of their role increased slightly, though not significantly, from before (Table 2A, item 7) to after rotating on the comanaged service (Table 2B, item 5). Although not statistically significant, there was a trend towards hospitalists and gastrointestinal (GI) fellows reporting a lack of patient ownership, both before and after serving on the comanaged service. Finally, nearly all respondents reported that comanagement should improve care quality, although only the attending hospitalist and hepatologist felt that their experience on the comanaged service actually improved patient care (Table 2B, item 7).
DISCUSSION
In this survey of providers participating on a comanaged medical service, most reported understanding their role in the collaborative arrangement and had an initial perception that comanagement should improve patient care quality. We found that hospitalists preferred and were expected to participate in care globally, while hepatologists themselves preferred and were expected not to focus on every management decision. The prevalence of desire for ultimate authority across the professional roles suggests tensions that exist in this care model around how decisions are made. The majority of providers preferred and experienced a single physician leader under comanagement, but many also experienced consensus‐seeking for every management decision.
From these findings, we conclude that decision‐making processes are not uniform under comanagement and that some role ambiguity is present, but there appears to be a pattern of natural roles. This pattern can be defined by focus (general for hospitalists vs specialty‐specific for hepatologists), rather than by responsibilities for managing particular medical problems. The preference among both generalists and specialists for the broader involvement of hospitalist comanagers suggests an implicit recognition of the need for integrated management to overcome the silo‐effect within the comanagement structure.9 Although details about how such integration was achieved are not available in our data, we found that comanagement may be distinct from traditional consultative practice in that the consultants (hospitalists in this case) manage not only general medical problems, such as diabetes or hypertension, but hospitalizations more generally. From a mission‐based standpoint, comanagement may be seen as a collaborative management of complex patients by two or more clinical experts with distinct knowledge, skills, or focus enacted for the purpose of improving care quality.
The focus of comanagement on improving quality is in line with the founding charge of the hospital medicine specialty to raise hospital care quality.10 In fact, the distinction between comanagement and consultation may be meaningful only if comanagers can work with specialists to implement evidence‐based practice, process improvement, and address quality and cost concerns. But as seen in NPPs and fellows' skepticism of improved quality under comanagement, there is still clearly work to be done to validate this model through measurable improvement in patients' experiences and outcomes. Proving the advantages of comanagement as a platform for practice improvement remains future work.11
Collaborative arrangements create natural tensions related to team function.5 This is seen in the similar proportion of hospitalists and hepatologists indicating desire for final decision‐making authority. Although comanagement evokes assumptions about egalitarian provider interactions involving shared decision‐making and responsibility, it seems to function empirically under hierarchical as well as consensus‐seeking forms of decision‐making. Providers at the top of hierarchical teams typically experience their work as interdependent and collaborative, and report more positive interactions with other care providers.12 Based on the fact that no hepatologists wanted more influence over decision‐making, we assume that hepatologists were the physician leaders for most of the studied comanaged teams. Under situations characterized by high levels of complexity and interdependence, a team governed by a single leader may often be more effective than one governed by shared authority.8 However, even under hierarchical models, a more participatory than supervisory leadership can help avoid alienating partners through a pattern of we decide, you carry it out that is often associated with ineffective leadership styles.1314 In fact, this alienating effect on providers in subordinate roles (ie, NPPs and fellows) may have contributed to the negative perception of the team's function on improving patient care.
This study is limited in the following ways. We did not have 100% participation in the Repeated Surveys. Attitudes and experiences of participants in a single comanagement practice are not representative of all comanaging providers. However, the goal of this studyto collect unique survey data from providers themselves to inform an evolving definition of comanagementis modest enough in scope to not require a generalizable sample. Because this study unearthed differences in expectations and experiences within a single site, they may serve as a lower bound for the extent of differences across and within multiple sites. In addition, comanagement enacted for complex medical patients is not as common as the comanagement of surgical patients. Moreover, comanagement models in academic hospitals may have structural features and priorities not found in community settings. Whether or not these disparate models share enough in common to be categorized under a single rubric is a valid question.
Although the teamwork structure and provider roles within comanagement vary, the practice arrangement's preoccupation with quality can be seen as its defining feature. Limited evidence, to date,1, 1519 and the rapid proliferation of the model, suggest that quality and efficiency advantages can be obtained from an effective implementation of comanagement. As in any team‐based care model, a common understanding of roles and expectations are essential to enhancing teamwork. Our interpretation of the mission of comanagement may further enhance teamwork through an explicit articulation of shared goals.
Comanagement is common in hospital medicine practice. And yet, there is no consensus about how comanagement is different from traditional consultative practice. At its core, hospitalist comanagement is a practice arrangement wherein hospitalists and other specialists manage complex patients collaboratively. Beyond this, Huddleston et al. distinguish comanagement from traditional consultations in the comanaging hospitalists' prerogative to provide direct medical care in addition to consultative advice.1 Siegal focuses on the shared responsibility and authority among partnering providers in the comanagement model.2 Whinney and Michota see comanagement as patient care referral at the onset of a care episode, in contrast to consultations that are activated to address emergent problems.3 In a recent study that found the growing adoption of medical comanagement in Medicare beneficiaries (as much as 40% of surgical hospitalizations in 2006), comanagement was defined as an intensive form of consultation involving a claim for evaluation and management services on greater than 70% of inpatient days.4
In addition to the intensity, frequency, timing, responsibility, and authority of care, comanagement may be described by participating physicians' roles. With recent attention on multidisciplinary teams and an increasing focus on collaborative care, many of the hierarchical relations among healthcare providers are breaking down.5 Several studies of multidisciplinary teams suggest that more egalitarian, rather than hierarchical, problem‐solving and decision‐making among team members are beneficial to patients.67 However, neither the intended nor natural team structure under comanagement is known. We sought to shed some light on provider interactions by characterizing the expectations and experiences of providers of a comanaged service. The findings yielded an opportunity to generate an evolving, but conceptually supported definition of comanagement.
SETTING
We conducted a survey study of providers participating in a comanaged inpatient hepatology service at the University of Chicago Medical Center, a 572‐bed urban teaching hospital. The service was created in 2006, partly to address staffing problems related to housestaff work hour restrictions and partly to improve the care of candidates and recipients of liver transplantation. Nonsurgical floor patients with liver diseases were managed on the service by two collaborating teams of providers. The hepatology team consisted of an attending physician and a fellow, while the hospitalist team consisted of a hospitalist and one or two nonphysician providers (physician assistant or nurse practitioner). The practice model is characterized as comanagement because of the highly interdependent nature of the team's daily tasks and the norms of intensive communication, through formal joint daily rounds and informal direct exchanges of instructions and updates. Hepatologists were mainly responsible for coordinating admissions, managing issues related to liver dysfunction, communicating with transplant surgeons if necessary, and arranging postdischarge care. Hospitalists were responsible for admitting patients, managing routine (eg, ordering daily labs) and urgent issues (eg, responding to critical lab values) during hospitalizations, coordinating with ancillary and consultative staff, and discharging patients. Occasional meetings between the hepatology and hospital medicine groups were used to clarify assignment of responsibilities. Floor nurses received in‐servicing at the commencement of the service. Additional details about the service are described elsewhere.8
DATA COLLECTION AND ANALYSIS
For the purpose of our analysis, we defined interactions between any member of the hospitalist and hepatologist teams as pertinent to comanagement. The hospitalist nonphysician provider (NPP) and hepatologistfellow relationships are governed by the more traditional hierarchical dynamics based on supervision and authority according to laws and regulations. At the beginning of the study period, each participant completed nine items of a Baseline Survey that addressed respondents' expectations and preferences for the management of an ideally comanaged service. Responses were solicited using a 4‐point Likert‐type scale and were dichotomized such that agree and somewhat agree were grouped, while disagree and somewhat disagree were grouped for data analysis. Items were generated to address the salient issues of comanagement after reviewing the pertinent literature.
Subsequently, participants were asked to complete Repeated Surveys immediately before each change in membership of the comanaged team between April and October 2008. The surveys were hand delivered by one of the authors (K.H.) on the last day of each team's rotation and were often completed immediately. The seven items of the Repeated Survey reprised items from the Baseline Survey that were rephrased to allow respondents to report their direct experiences on specific teams. Because all providers rotated on the service more than once during the study period, the average value for each Likert‐type response across multiple surveys completed by a single provider was calculated before being dichotomized at the midpoint (<2.5, agree; 2.5, disagree). We reported proportions of respondents in agreement with survey item statements.
Comparison statistics across providers were generated using the chi‐square test. Differences in proportions between related items of the Baseline and Repeated Surveys were compared using the two‐sample test of proportions. All analyses were conducted using a statistics application (STATA 10.0, College Station, TX) with alpha equal to, or less than, 0.05 considered significant. The Institutional Review Board of the University of Chicago approved this project.
RESULTS
All 43 providers completed the Baseline Survey. During the study period, 32 of these participants rotated on the service and completed 177 of the 233 Repeated Surveys (79%) administered. The responses describe team interactions on the 47 unique combinations of providers comprising the comanaged teams. Details of the response rates are shown in Table 1.
Baseline Survey, Completed/ Administered (%) | Repeated Surveys, Completed/ Administered (%) | Respondents Completing Repeated Surveys, n | Repeated Surveys Completed per Respondent, Median (IQR) | |
---|---|---|---|---|
| ||||
Hospitalists | 18/18 (100) | 36/43 (84) | 15 | 2 (2, 3) |
NPPs | 5/5 (100) | 92/97 (95) | 5 | 20 (18, 20) |
Hepatologists | 6/6 (100) | 26/42 (62) | 6 | 7 (3.75, 8) |
Fellows | 12/12 (100) | 23/42 (55) | 6 | 7 (5.5, 8.5) |
Total | 43/43 (100) | 177/223 (79) | 32 | 4.5 (2, 8.25) |
As shown in Table 2A, items 13, more members of the hospitalist team preferred to be informed about every management decision compared to members of the hepatologist team. Conversely, more of members of the hepatologist team than the hospitalist team preferred their comanaging partners to participate in every decision. A statistically similar proportion of respondents in each of the professional roles indicated desire for greater influence in directing management decisions (Table 2B, item 1).
A. Baseline Survey | Hospitalists, % (n = 18) | NPPs, % (n = 5) | Hepatologists, % (n = 6) | GI Fellows, % (n = 12) | P‐value |
---|---|---|---|---|---|
| |||||
1. I prefer to be informed about every decision. | 83 | 100 | 17 | 42 | <0.01 |
2. I prefer to participate in every decision. | 67 | 100 | 33 | 50 | 0.11 |
3. I prefer that my comanager participate in every decision. | 22 | 20 | 50 | 75 | 0.02 |
4. I prefer to have the final say in every decision. | 50 | 80 | 50 | 33 | 0.38 |
5. There should be one physician leader to direct the overall management of the patients' hospital course. | 89* | 100 | 67 | 83 | 0.43 |
6. Physician consensus should always be sought in every clinical decision. | 22 | 40 | 50 | 67 | 0.11 |
7. I have a clear understanding of my role on the comanagement service. | 61 | 80 | 83 | 75 | 0.66 |
8. I have as much a sense of ownership of patients on the comanaged service as on a non‐comanaged service. | 61 | 60 | 83 | 50 | 0.60 |
9. Comanagement tends to improve patient care. | 94 | 100* | 83 | 100* | 0.47 |
B. Repeated Surveys | Hospitalists, % (n = 15) | NPPs, % (n = 5) | Hepatologists, % (n = 6) | GI Fellows, % (n = 6) | P‐value |
1. I would have liked greater influence in directing the overall management. | 40 | 60 | 0 | 17 | 0.12 |
2. I was responsible for work in clinical areas I was not comfortable managing. | 0 | 0 | 0 | 0 | NA |
3. There was one physician leader to direct the overall management of the patients' hospital course. | 60* | 80 | 67 | 83 | 0.70 |
4. Physician consensus was always sought in every clinical decision. | 40 | 40 | 50 | 67 | 0.72 |
5. I (have/had) a clear understanding of my role on the comanagement service. | 73 | 80 | 100 | 83 | 0.57 |
6. I had as much a sense of ownership of patients on the comanaged service as on a non‐comanaged service. | 53 | 80 | 100 | 67 | 0.20 |
7. Patients on my service received better care than they would have without comanagement. | 93 | 40* | 67 | 50* | 0.06 |
For the majority of surveyed areas, there was concordance between expectations and experiences of providers on comanagement. Most providers, regardless of professional role, agreed that there should be a single physician leader to direct the overall management (Table 2A, item 5). The majority perceived that a single physician directed the overall management of the patients' hospital course, although fewer hospitalists did so compared with baseline expectations (Table 2B, item 3). Many respondents felt at baseline that physician consensus should govern every management decision, and a similar proportion actually experienced consensus‐seeking on service.
We found that the proportion of providers reporting an understanding of their role increased slightly, though not significantly, from before (Table 2A, item 7) to after rotating on the comanaged service (Table 2B, item 5). Although not statistically significant, there was a trend towards hospitalists and gastrointestinal (GI) fellows reporting a lack of patient ownership, both before and after serving on the comanaged service. Finally, nearly all respondents reported that comanagement should improve care quality, although only the attending hospitalist and hepatologist felt that their experience on the comanaged service actually improved patient care (Table 2B, item 7).
DISCUSSION
In this survey of providers participating on a comanaged medical service, most reported understanding their role in the collaborative arrangement and had an initial perception that comanagement should improve patient care quality. We found that hospitalists preferred and were expected to participate in care globally, while hepatologists themselves preferred and were expected not to focus on every management decision. The prevalence of desire for ultimate authority across the professional roles suggests tensions that exist in this care model around how decisions are made. The majority of providers preferred and experienced a single physician leader under comanagement, but many also experienced consensus‐seeking for every management decision.
From these findings, we conclude that decision‐making processes are not uniform under comanagement and that some role ambiguity is present, but there appears to be a pattern of natural roles. This pattern can be defined by focus (general for hospitalists vs specialty‐specific for hepatologists), rather than by responsibilities for managing particular medical problems. The preference among both generalists and specialists for the broader involvement of hospitalist comanagers suggests an implicit recognition of the need for integrated management to overcome the silo‐effect within the comanagement structure.9 Although details about how such integration was achieved are not available in our data, we found that comanagement may be distinct from traditional consultative practice in that the consultants (hospitalists in this case) manage not only general medical problems, such as diabetes or hypertension, but hospitalizations more generally. From a mission‐based standpoint, comanagement may be seen as a collaborative management of complex patients by two or more clinical experts with distinct knowledge, skills, or focus enacted for the purpose of improving care quality.
The focus of comanagement on improving quality is in line with the founding charge of the hospital medicine specialty to raise hospital care quality.10 In fact, the distinction between comanagement and consultation may be meaningful only if comanagers can work with specialists to implement evidence‐based practice, process improvement, and address quality and cost concerns. But as seen in NPPs and fellows' skepticism of improved quality under comanagement, there is still clearly work to be done to validate this model through measurable improvement in patients' experiences and outcomes. Proving the advantages of comanagement as a platform for practice improvement remains future work.11
Collaborative arrangements create natural tensions related to team function.5 This is seen in the similar proportion of hospitalists and hepatologists indicating desire for final decision‐making authority. Although comanagement evokes assumptions about egalitarian provider interactions involving shared decision‐making and responsibility, it seems to function empirically under hierarchical as well as consensus‐seeking forms of decision‐making. Providers at the top of hierarchical teams typically experience their work as interdependent and collaborative, and report more positive interactions with other care providers.12 Based on the fact that no hepatologists wanted more influence over decision‐making, we assume that hepatologists were the physician leaders for most of the studied comanaged teams. Under situations characterized by high levels of complexity and interdependence, a team governed by a single leader may often be more effective than one governed by shared authority.8 However, even under hierarchical models, a more participatory than supervisory leadership can help avoid alienating partners through a pattern of we decide, you carry it out that is often associated with ineffective leadership styles.1314 In fact, this alienating effect on providers in subordinate roles (ie, NPPs and fellows) may have contributed to the negative perception of the team's function on improving patient care.
This study is limited in the following ways. We did not have 100% participation in the Repeated Surveys. Attitudes and experiences of participants in a single comanagement practice are not representative of all comanaging providers. However, the goal of this studyto collect unique survey data from providers themselves to inform an evolving definition of comanagementis modest enough in scope to not require a generalizable sample. Because this study unearthed differences in expectations and experiences within a single site, they may serve as a lower bound for the extent of differences across and within multiple sites. In addition, comanagement enacted for complex medical patients is not as common as the comanagement of surgical patients. Moreover, comanagement models in academic hospitals may have structural features and priorities not found in community settings. Whether or not these disparate models share enough in common to be categorized under a single rubric is a valid question.
Although the teamwork structure and provider roles within comanagement vary, the practice arrangement's preoccupation with quality can be seen as its defining feature. Limited evidence, to date,1, 1519 and the rapid proliferation of the model, suggest that quality and efficiency advantages can be obtained from an effective implementation of comanagement. As in any team‐based care model, a common understanding of roles and expectations are essential to enhancing teamwork. Our interpretation of the mission of comanagement may further enhance teamwork through an explicit articulation of shared goals.
- Medical and surgical comanagement after elective hip and knee arthroplasty: A randomized, controlled trial.Ann Intern Med.2004;141(1):28–38. , , , et al.
- Just because you can, doesn't mean that you should: A call for the rational application of hospitalist comanagement.J Hosp Med.2008;3(5):398–402. .
- Surgical comanagement: A natural evolution of hospitalist practice.J Hosp Med.2008;3(5):394–397. , .
- Comanagement of hospitalized surgical patients by medicine physicians in the United States.Arch Intern Med.2010;170(4):363–368. , , , , .
- Structure and meaning in multidisciplinary teamwork.Sociol Health Illn.1998;20(6):848–873. .
- Human factors and cardiac surgery: A multicenter study.J Thorac Cardiov Surg.2000;119(4):661–670. , , , , .
- The effects of a collaborative model of primary care on the mortality and hospital use of community‐dwelling older adults.J Gerontol A‐Biol.2001;56(2):M106–M112. , , .
- Effects of provider characteristics on care coordination under comanagement.J Hosp Med.2010;5:508–513. , , , , , .
- Crossing the Quality Chasm: A New Health System for the Twenty‐First Century.Washington, DC:Institute of Medicine;2001. , , .
- The emerging role of “hospitalists” in the American health care system.N Engl J Med.1996;335(7):514–517. , .
- Internal medicine comanagement of surgical patients: Can we afford to do this?Arch Intern Med.2010;170(22):1965–1966. .
- Operating room teamwork among physicians and nurses: Teamwork in the eye of the beholder.J Am Coll Surg.2006;202(5):746–752. , , , et al.
- “We decide, you carry it out”: A social network analysis of multidisciplinary longterm care teams.Soc Sci Med.1997;45(9):1411–1421. .
- Patterns of aggressive behavior in experimentally created social climates.J Soc Psychol.1939;10:271–301. , , .
- Comanagement of surgical patients between neurosurgeons and hospitalists.Arch Intern Med.2010;170(22):2004–2010. , , , et al.
- Outcomes for older patients with hip fractures: The impact of orthopedic and geriatric medicine cocare.J Orthop Trauma.2006;20(3):172–180. , , , , , .
- Effects of a hospitalist model on elderly patients with hip fracture.Arch Intern Med.2005;165(7):796–801. , , , et al.
- Hip fractures in geriatric patients. Results of an interdisciplinary hospital care program.Clin Orthop Relat Res.1992(274):213–225. , , , .
- Impact of a comanaged Geriatric Fracture Center on short‐term hip fracture outcomes.Arch Intern Med.2009;169(18):1712–1717. , , , .
- Medical and surgical comanagement after elective hip and knee arthroplasty: A randomized, controlled trial.Ann Intern Med.2004;141(1):28–38. , , , et al.
- Just because you can, doesn't mean that you should: A call for the rational application of hospitalist comanagement.J Hosp Med.2008;3(5):398–402. .
- Surgical comanagement: A natural evolution of hospitalist practice.J Hosp Med.2008;3(5):394–397. , .
- Comanagement of hospitalized surgical patients by medicine physicians in the United States.Arch Intern Med.2010;170(4):363–368. , , , , .
- Structure and meaning in multidisciplinary teamwork.Sociol Health Illn.1998;20(6):848–873. .
- Human factors and cardiac surgery: A multicenter study.J Thorac Cardiov Surg.2000;119(4):661–670. , , , , .
- The effects of a collaborative model of primary care on the mortality and hospital use of community‐dwelling older adults.J Gerontol A‐Biol.2001;56(2):M106–M112. , , .
- Effects of provider characteristics on care coordination under comanagement.J Hosp Med.2010;5:508–513. , , , , , .
- Crossing the Quality Chasm: A New Health System for the Twenty‐First Century.Washington, DC:Institute of Medicine;2001. , , .
- The emerging role of “hospitalists” in the American health care system.N Engl J Med.1996;335(7):514–517. , .
- Internal medicine comanagement of surgical patients: Can we afford to do this?Arch Intern Med.2010;170(22):1965–1966. .
- Operating room teamwork among physicians and nurses: Teamwork in the eye of the beholder.J Am Coll Surg.2006;202(5):746–752. , , , et al.
- “We decide, you carry it out”: A social network analysis of multidisciplinary longterm care teams.Soc Sci Med.1997;45(9):1411–1421. .
- Patterns of aggressive behavior in experimentally created social climates.J Soc Psychol.1939;10:271–301. , , .
- Comanagement of surgical patients between neurosurgeons and hospitalists.Arch Intern Med.2010;170(22):2004–2010. , , , et al.
- Outcomes for older patients with hip fractures: The impact of orthopedic and geriatric medicine cocare.J Orthop Trauma.2006;20(3):172–180. , , , , , .
- Effects of a hospitalist model on elderly patients with hip fracture.Arch Intern Med.2005;165(7):796–801. , , , et al.
- Hip fractures in geriatric patients. Results of an interdisciplinary hospital care program.Clin Orthop Relat Res.1992(274):213–225. , , , .
- Impact of a comanaged Geriatric Fracture Center on short‐term hip fracture outcomes.Arch Intern Med.2009;169(18):1712–1717. , , , .
Copyright © 2011 Society of Hospital Medicine